GEOGG 121 Methods Monte Carlo methods revision Dr
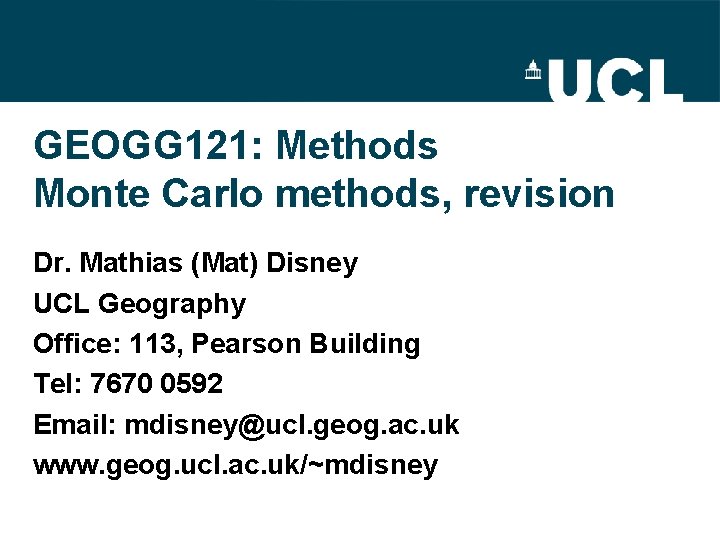
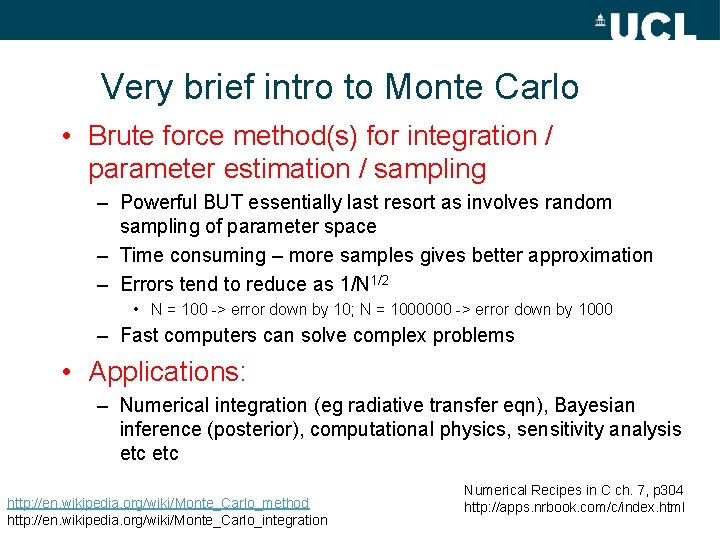
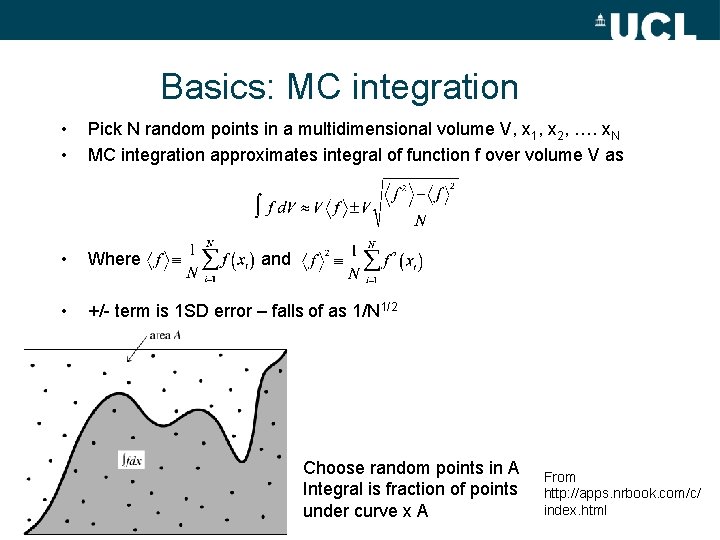
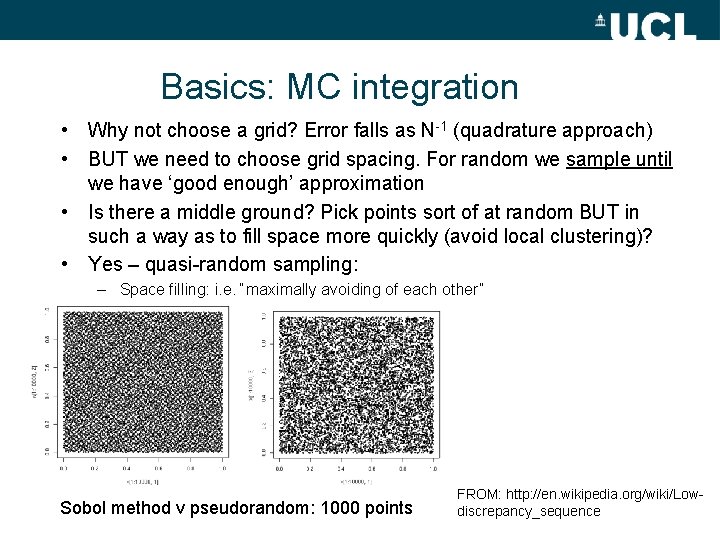
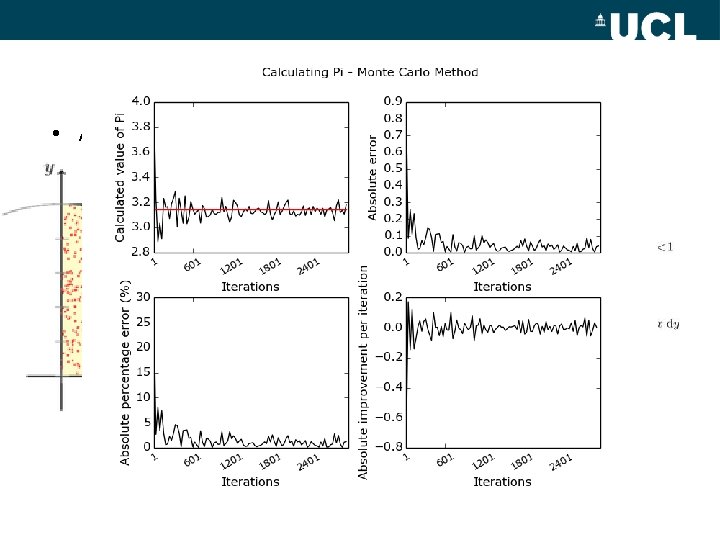
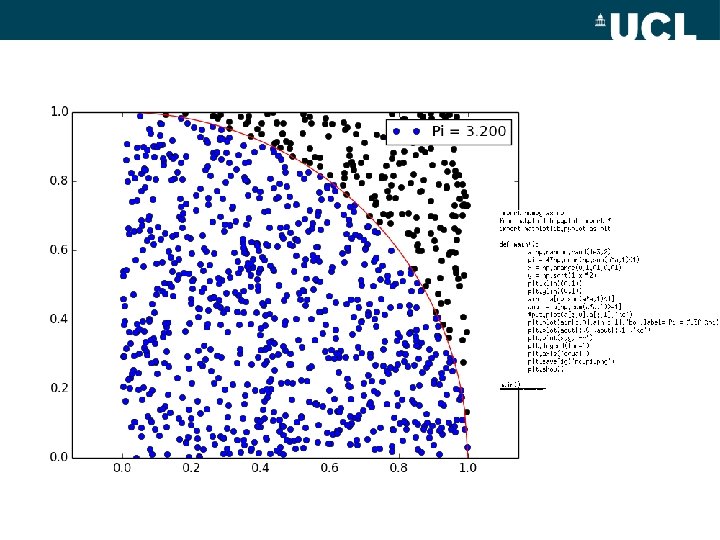
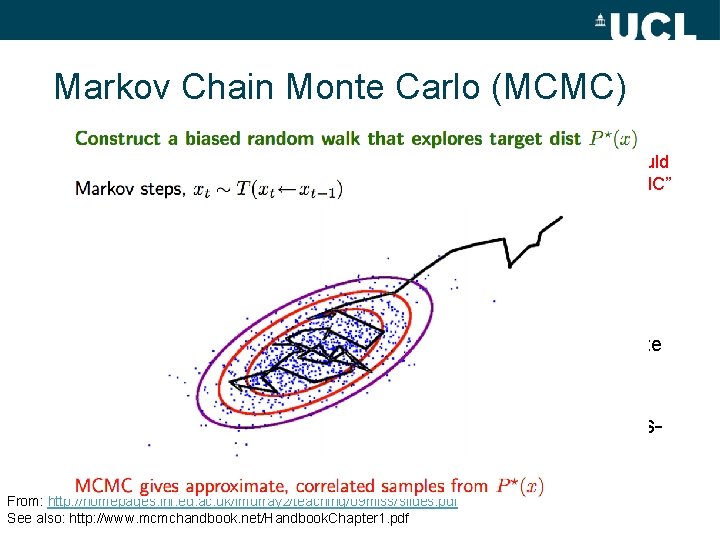
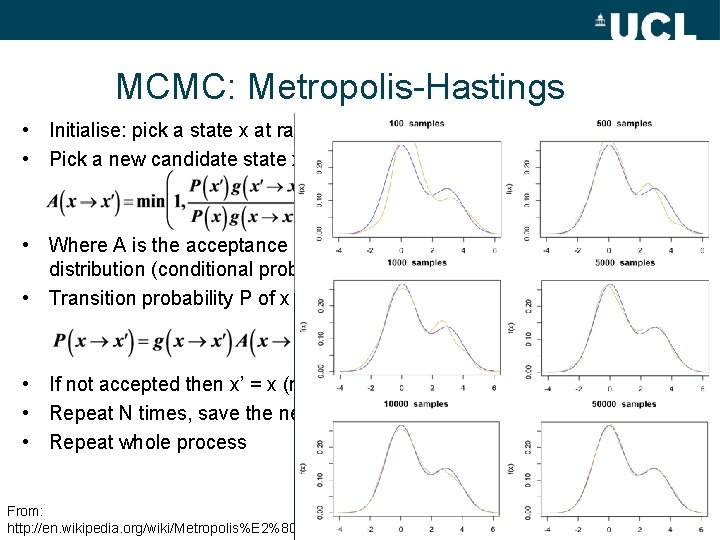
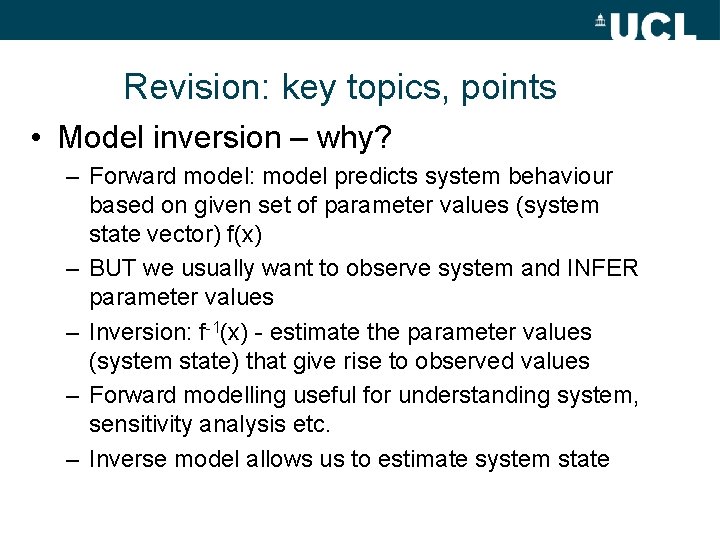
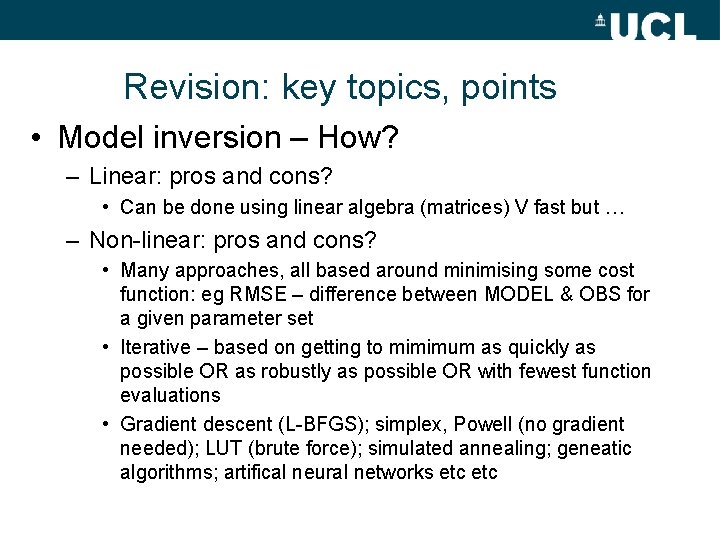
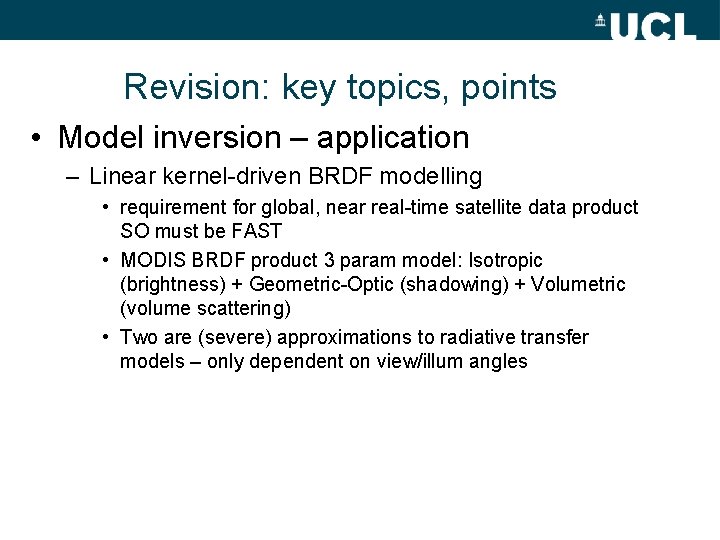
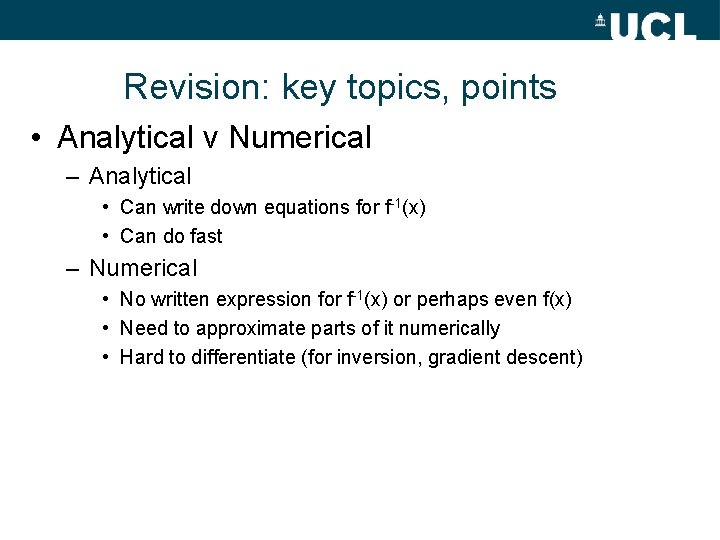
- Slides: 12
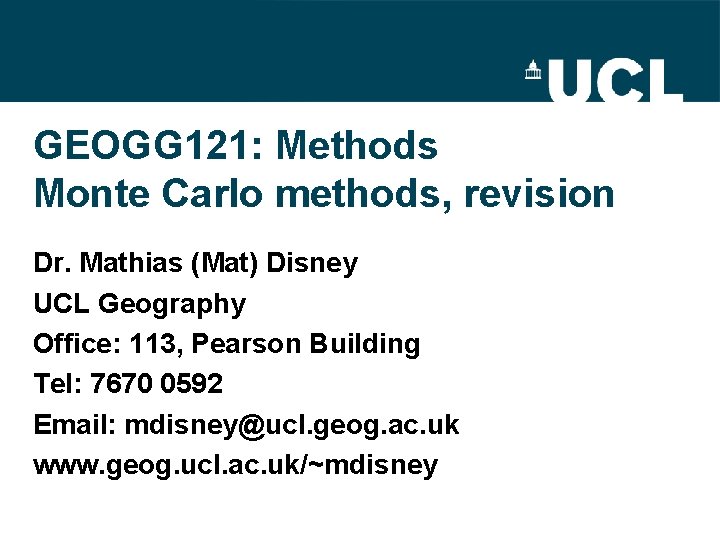
GEOGG 121: Methods Monte Carlo methods, revision Dr. Mathias (Mat) Disney UCL Geography Office: 113, Pearson Building Tel: 7670 0592 Email: mdisney@ucl. geog. ac. uk www. geog. ucl. ac. uk/~mdisney
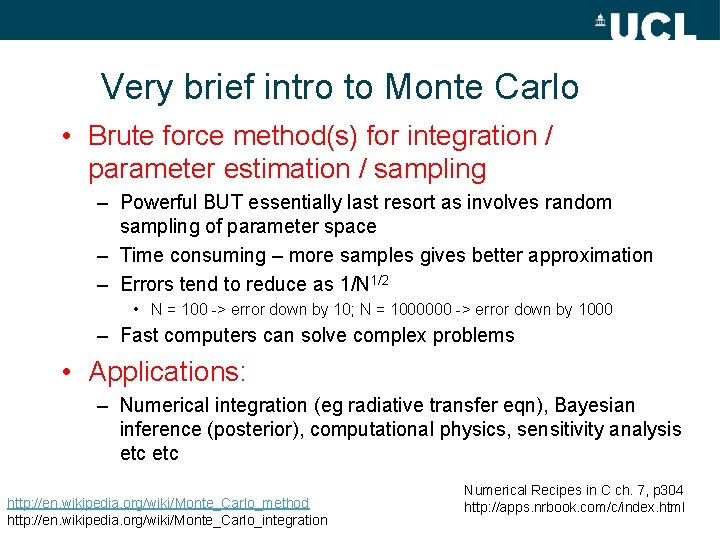
Very brief intro to Monte Carlo • Brute force method(s) for integration / parameter estimation / sampling – Powerful BUT essentially last resort as involves random sampling of parameter space – Time consuming – more samples gives better approximation – Errors tend to reduce as 1/N 1/2 • N = 100 -> error down by 10; N = 1000000 -> error down by 1000 – Fast computers can solve complex problems • Applications: – Numerical integration (eg radiative transfer eqn), Bayesian inference (posterior), computational physics, sensitivity analysis etc http: //en. wikipedia. org/wiki/Monte_Carlo_method http: //en. wikipedia. org/wiki/Monte_Carlo_integration Numerical Recipes in C ch. 7, p 304 http: //apps. nrbook. com/c/index. html
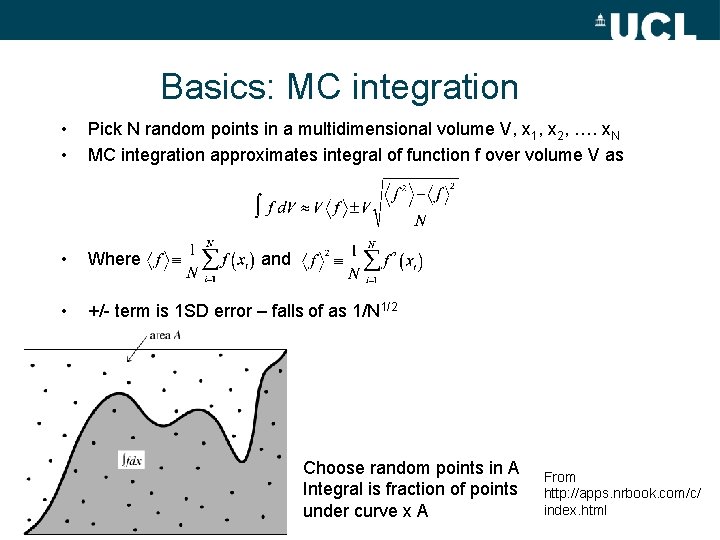
Basics: MC integration • • Pick N random points in a multidimensional volume V, x 1, x 2, …. x. N MC integration approximates integral of function f over volume V as • Where • +/- term is 1 SD error – falls of as 1/N 1/2 and Choose random points in A Integral is fraction of points under curve x A From http: //apps. nrbook. com/c/ index. html
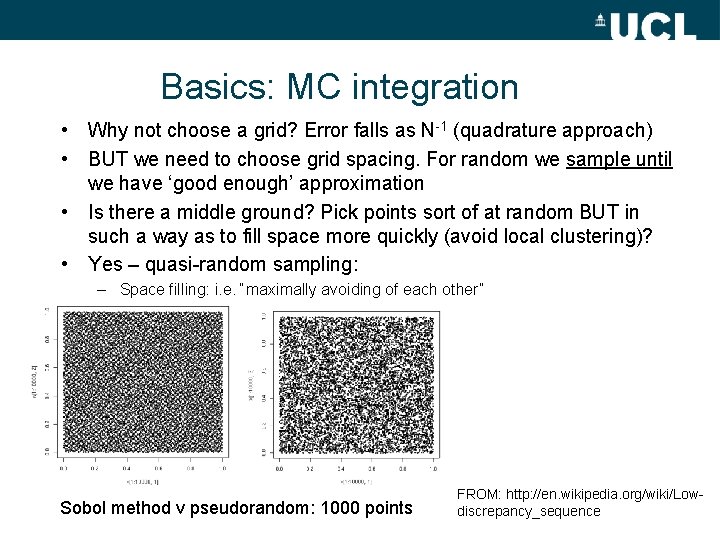
Basics: MC integration • Why not choose a grid? Error falls as N-1 (quadrature approach) • BUT we need to choose grid spacing. For random we sample until we have ‘good enough’ approximation • Is there a middle ground? Pick points sort of at random BUT in such a way as to fill space more quickly (avoid local clustering)? • Yes – quasi-random sampling: – Space filling: i. e. “maximally avoiding of each other” Sobol method v pseudorandom: 1000 points FROM: http: //en. wikipedia. org/wiki/Lowdiscrepancy_sequence
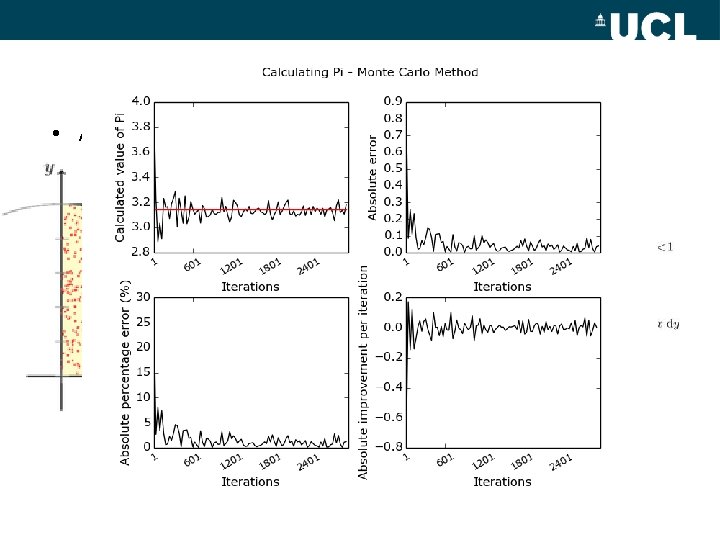
MC approximation of Pi? • A simple example of MC methods in practice
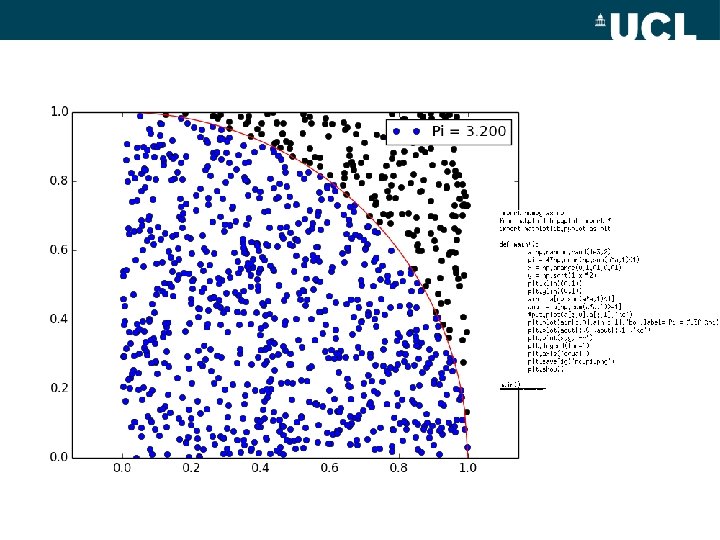
MC approximation of Pi? • A simple example of MC methods in practice • In Python? – – import numpy as np a = np. random. rand(10, 2) np. sum(a*a, 1)<1 array([ True, False, True, True], dtype=bool) – 4*np. mean(np. sum(a*a, 1)<1) 2. 399999999
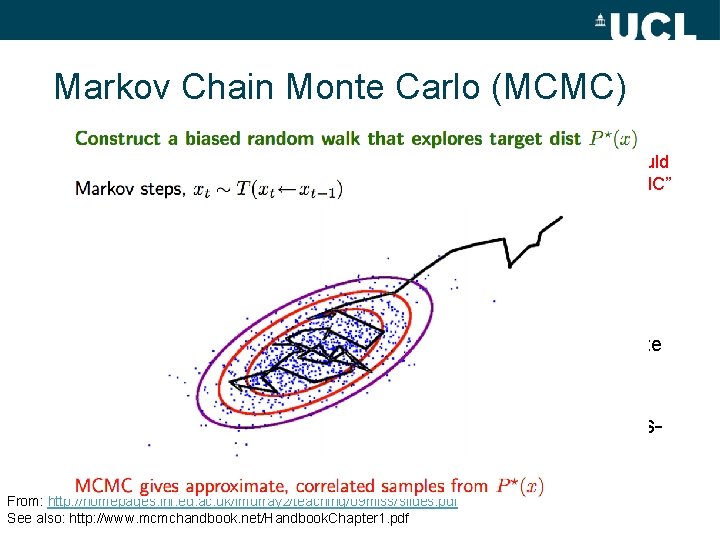
Markov Chain Monte Carlo (MCMC) • Integration / parameter estimation / sampling – From 80 s: “It was rapidly realised that most Bayesian inference could be done by MCMC, whereas very little could be done without MCMC” (Geyer, 2010) • Formally – MCMC methods sample from probability distribution (eg a posterior) based on constructing a Markov Chain with the desired distribution as its equilibrium (tends to) distribution – Markov Chain: system of random transitions where next state dpeends on only on current, not preceding chain (ie no “memory” of how we got here) • Many implementations of MCMC including Metropolis. Hastings, Gibbs Sampler etc. From: http: //homepages. inf. ed. ac. uk/imurray 2/teaching/09 mlss/slides. pdf See also: http: //www. mcmchandbook. net/Handbook. Chapter 1. pdf
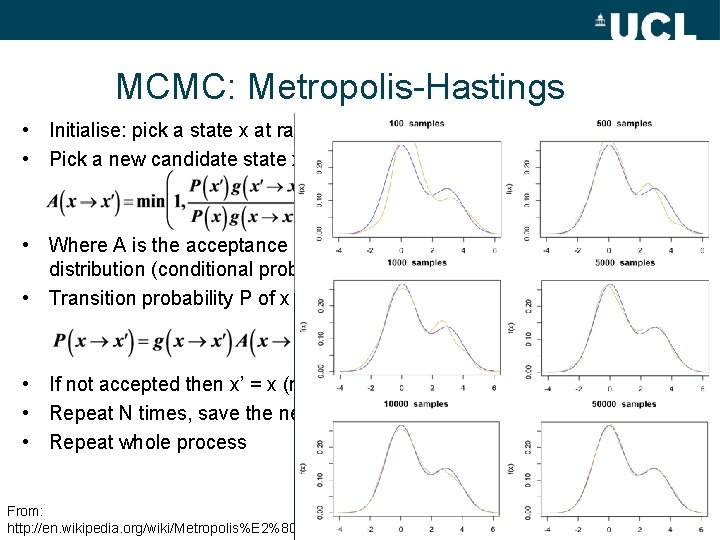
MCMC: Metropolis-Hastings • Initialise: pick a state x at random • Pick a new candidate state x’ at random. Accept based on criteria • Where A is the acceptance distribution, is the proposal distribution (conditional prob of proposing state x’, given x) • Transition probability P of x -> x’ • If not accepted then x’ = x (no change) OR state transits to x’ • Repeat N times, save the new state x’ • Repeat whole process From: http: //en. wikipedia. org/wiki/Metropolis%E 2%80
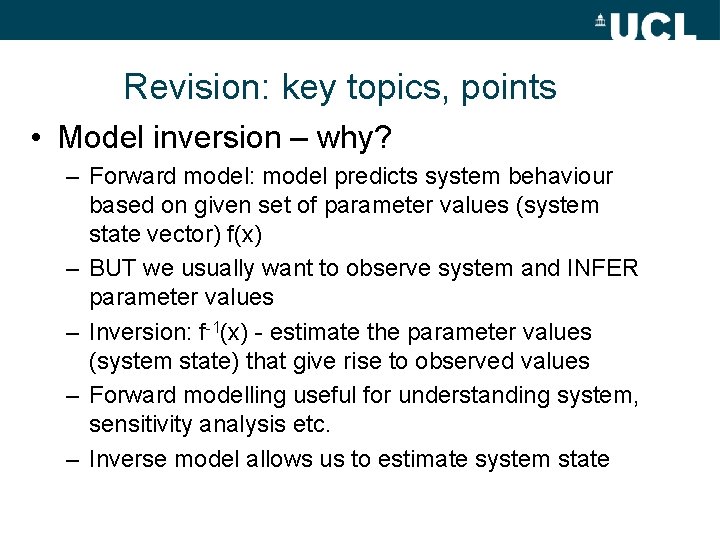
Revision: key topics, points • Model inversion – why? – Forward model: model predicts system behaviour based on given set of parameter values (system state vector) f(x) – BUT we usually want to observe system and INFER parameter values – Inversion: f-1(x) - estimate the parameter values (system state) that give rise to observed values – Forward modelling useful for understanding system, sensitivity analysis etc. – Inverse model allows us to estimate system state
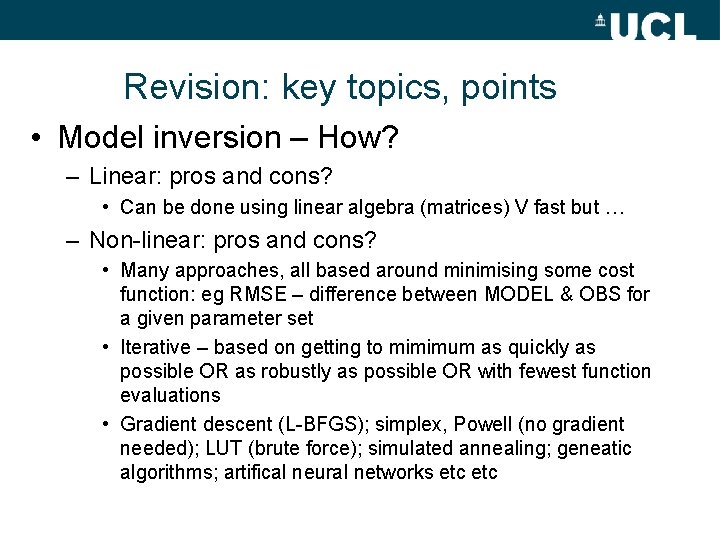
Revision: key topics, points • Model inversion – How? – Linear: pros and cons? • Can be done using linear algebra (matrices) V fast but … – Non-linear: pros and cons? • Many approaches, all based around minimising some cost function: eg RMSE – difference between MODEL & OBS for a given parameter set • Iterative – based on getting to mimimum as quickly as possible OR as robustly as possible OR with fewest function evaluations • Gradient descent (L-BFGS); simplex, Powell (no gradient needed); LUT (brute force); simulated annealing; geneatic algorithms; artifical neural networks etc
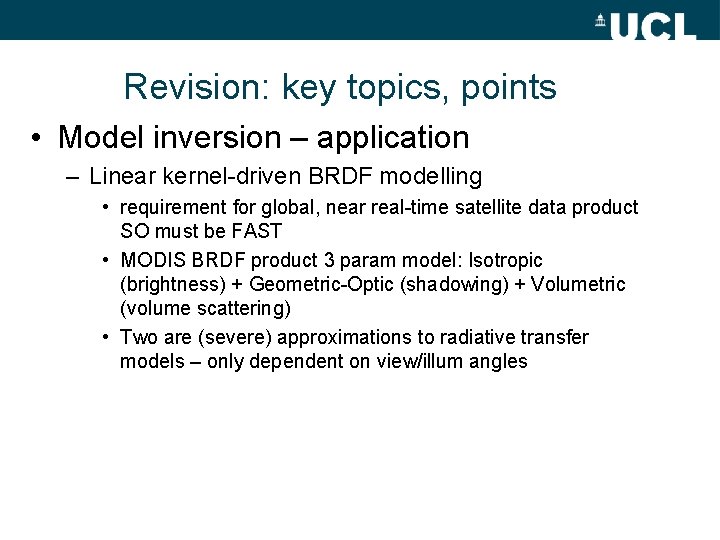
Revision: key topics, points • Model inversion – application – Linear kernel-driven BRDF modelling • requirement for global, near real-time satellite data product SO must be FAST • MODIS BRDF product 3 param model: Isotropic (brightness) + Geometric-Optic (shadowing) + Volumetric (volume scattering) • Two are (severe) approximations to radiative transfer models – only dependent on view/illum angles
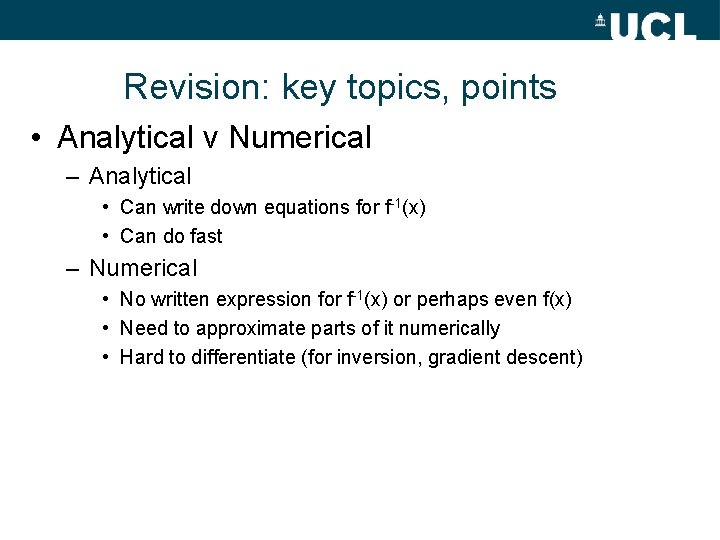
Revision: key topics, points • Analytical v Numerical – Analytical • Can write down equations for f-1(x) • Can do fast – Numerical • No written expression for f-1(x) or perhaps even f(x) • Need to approximate parts of it numerically • Hard to differentiate (for inversion, gradient descent)
Monte carlo radiation transport
Simulacion monte carlo en excel
Monte carlo
Monte carlo path tracing
Monte carlo simulation particle physics
Count of monte carlo
Contoh simulasi monte carlo
The monte carlo
Continuous time monte carlo
Villa monte carlo
Kinetic monte carlo python
Viterbi algorithm
Monte carlo simulation dice roll matlab