Generative Models of MEEG Group inversion and MEGEEGf
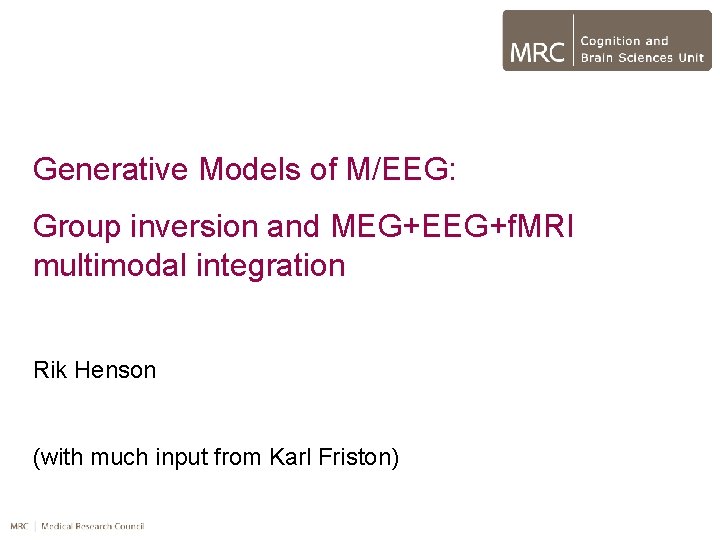
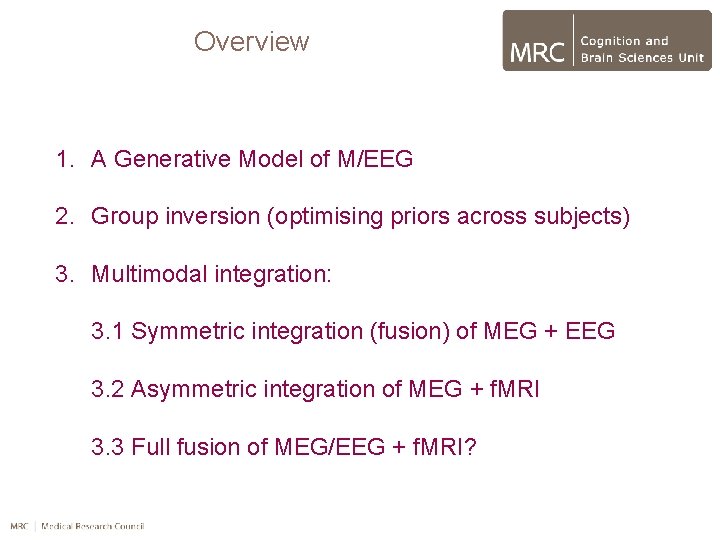
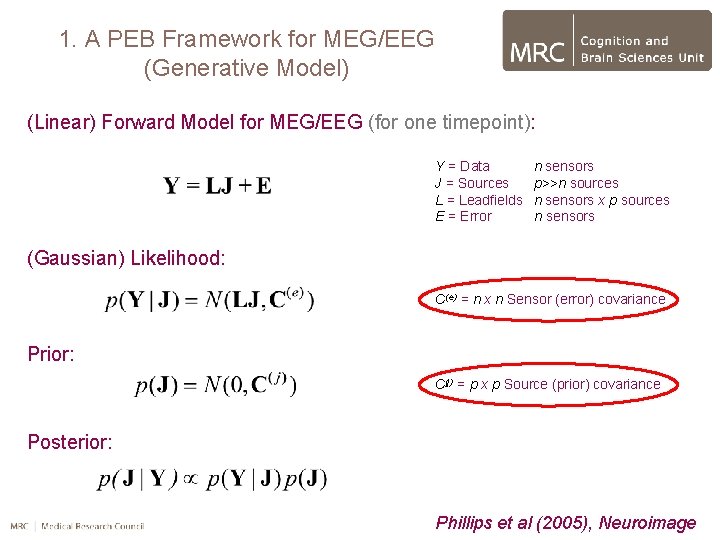
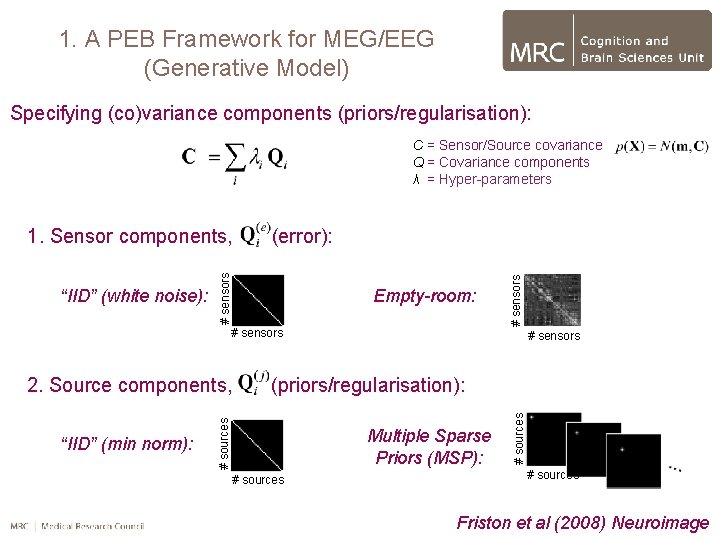
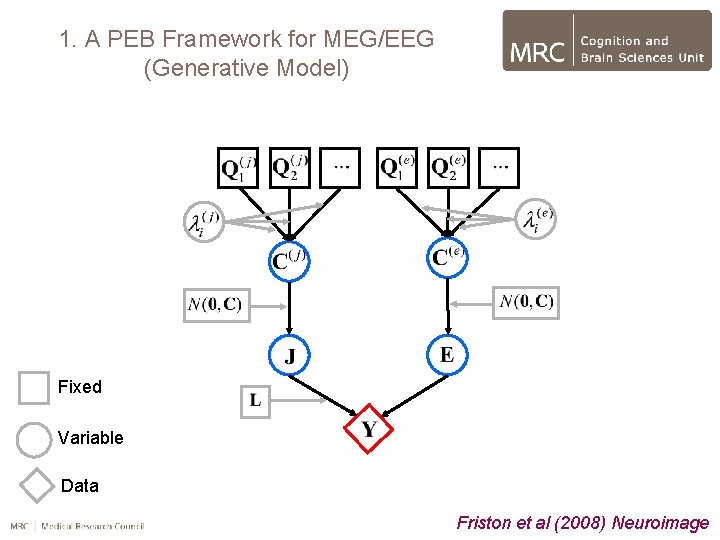
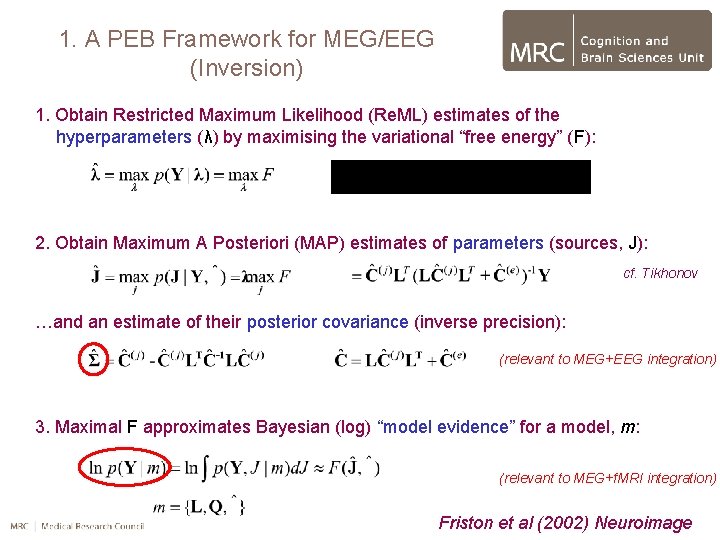
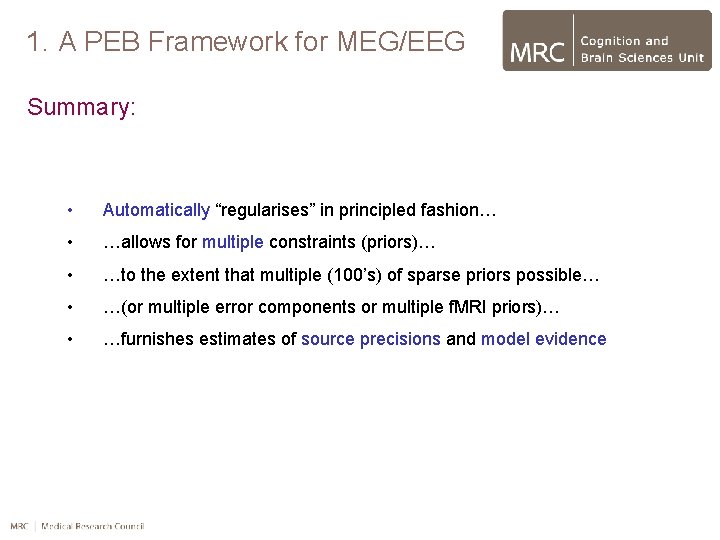
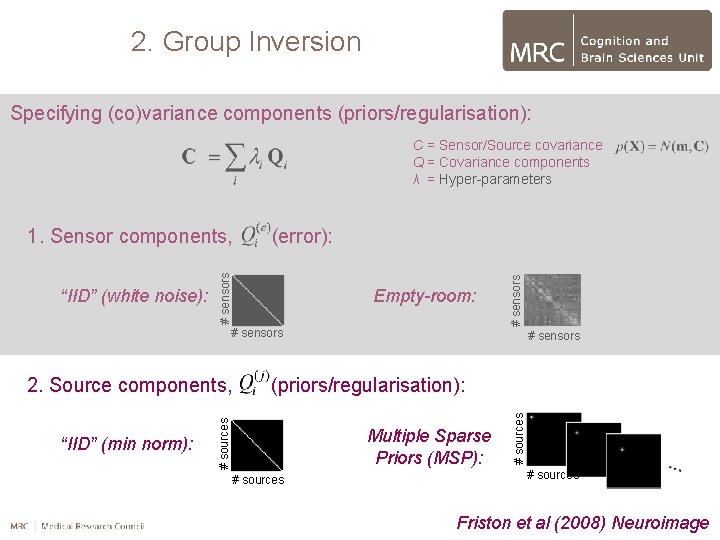
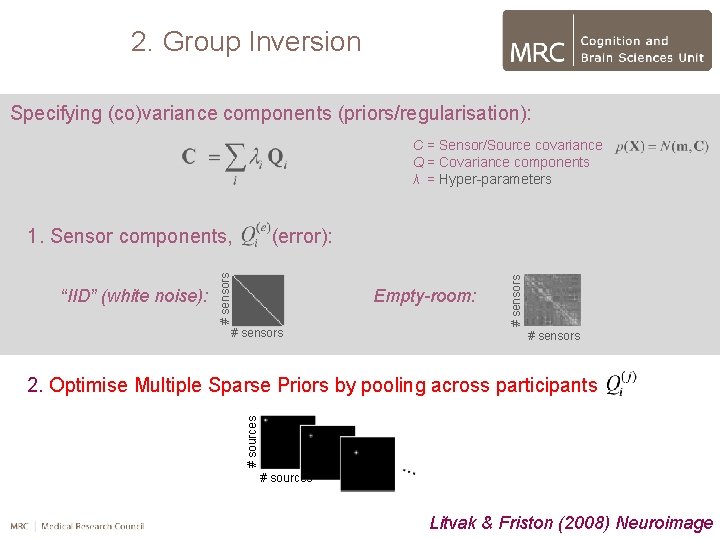
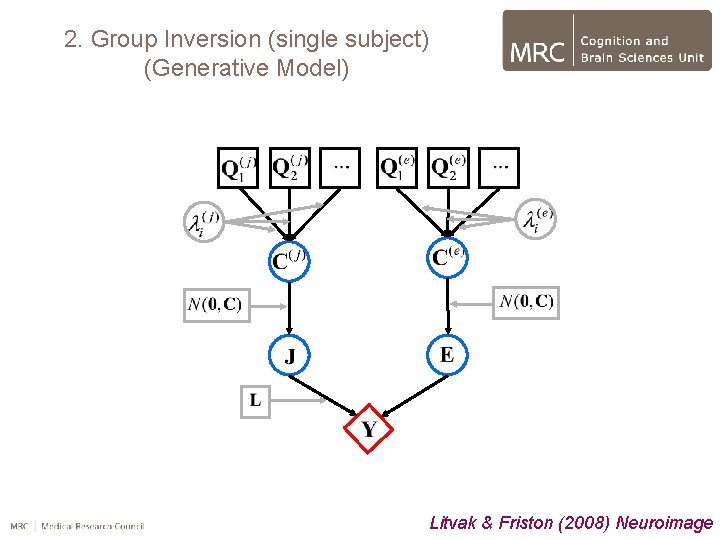
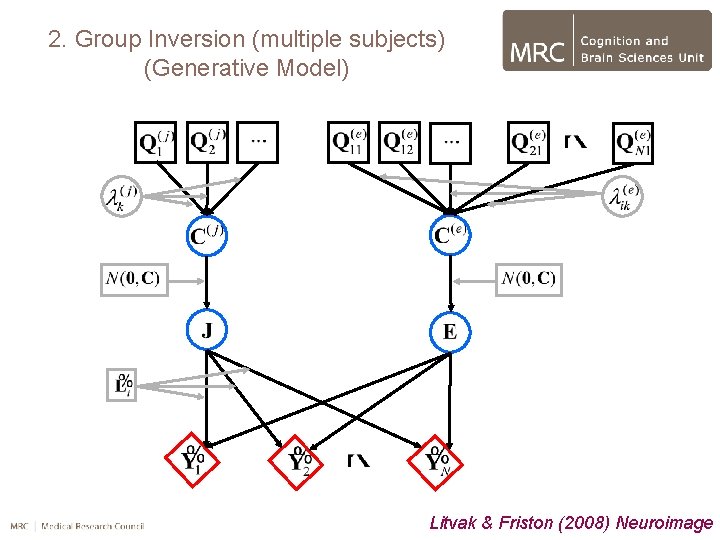
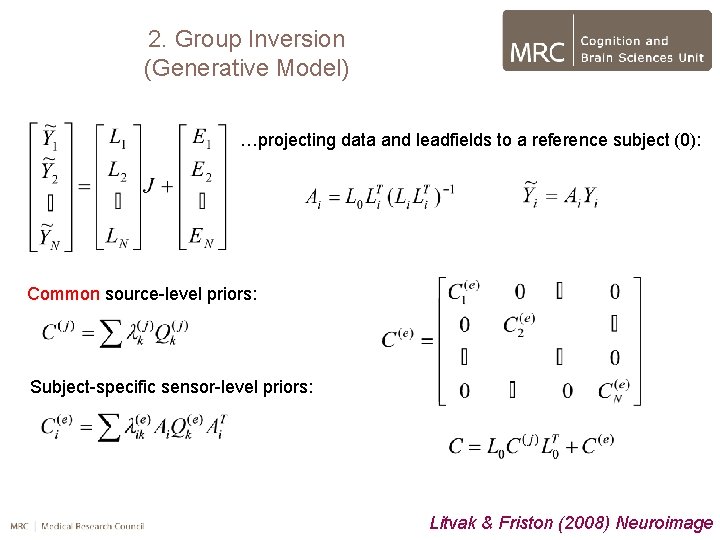
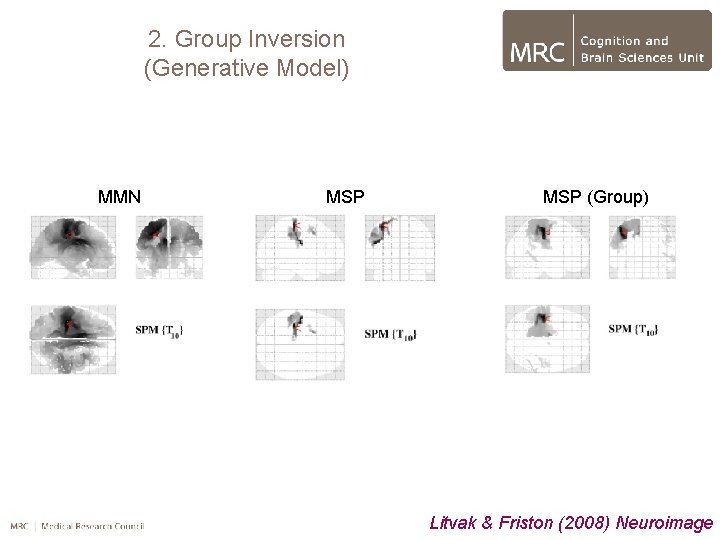
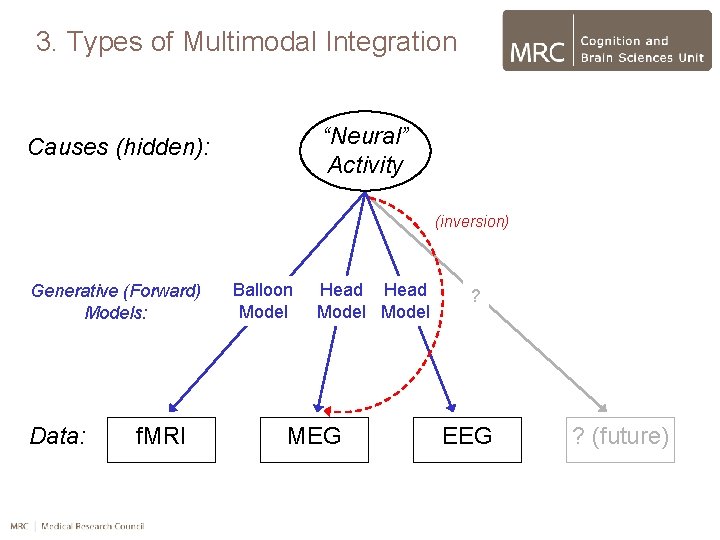
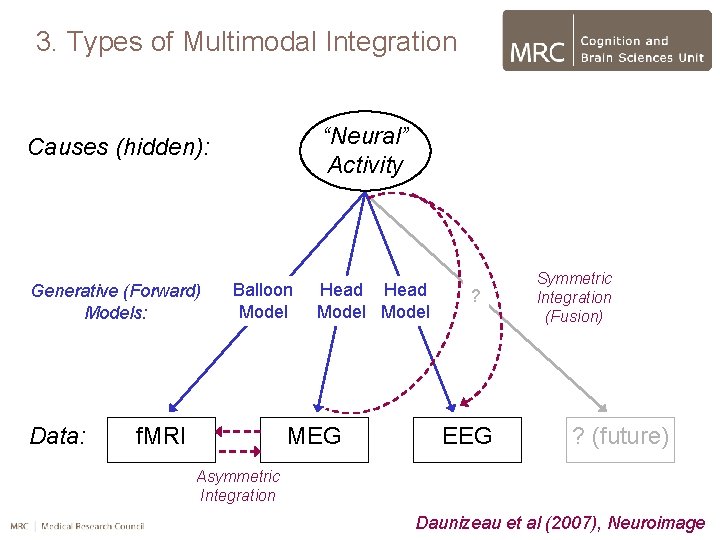
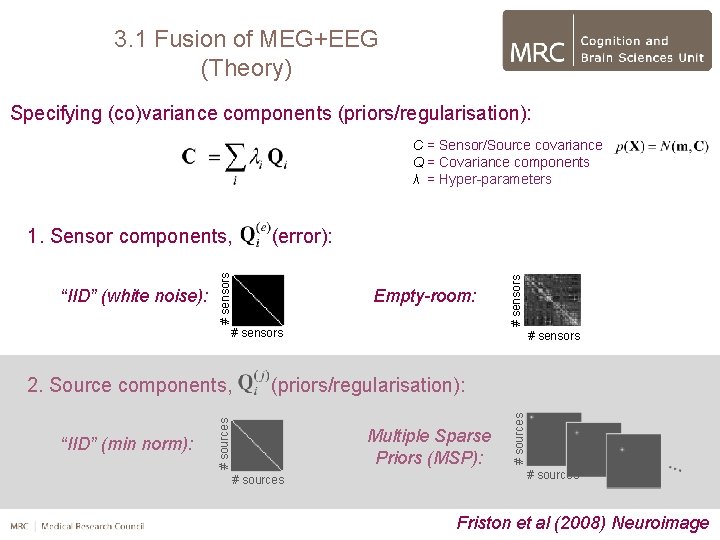
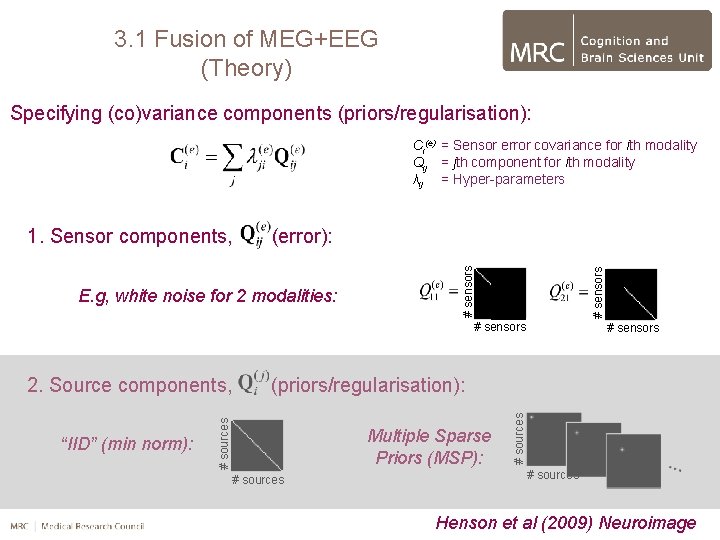
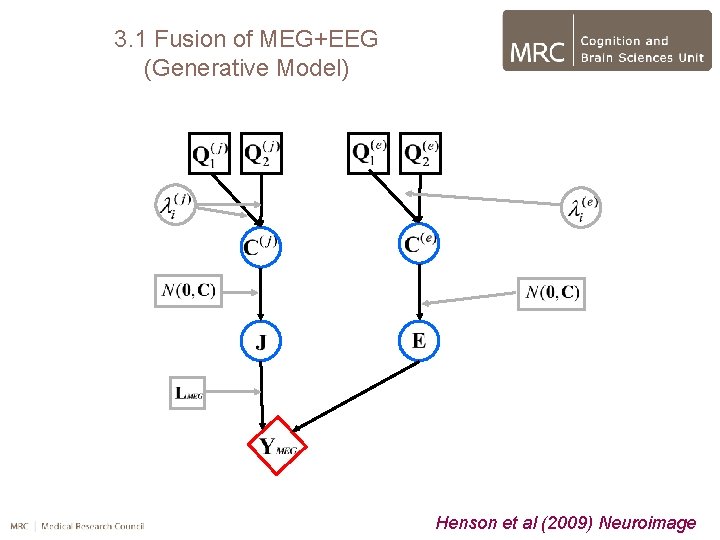
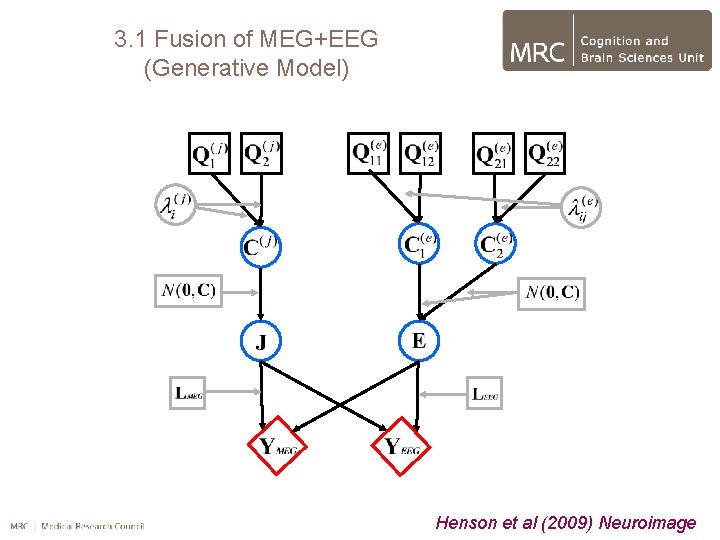
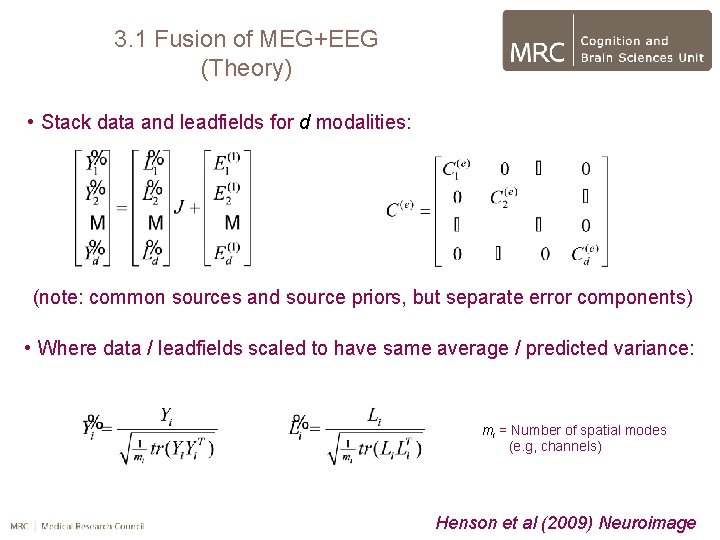
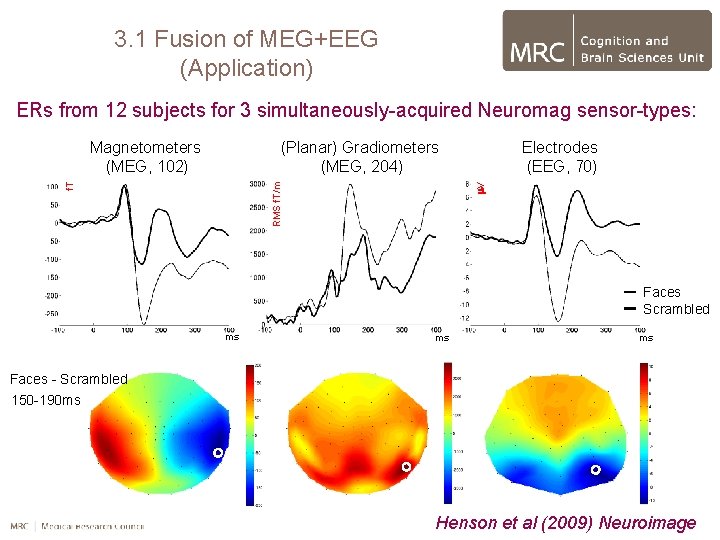
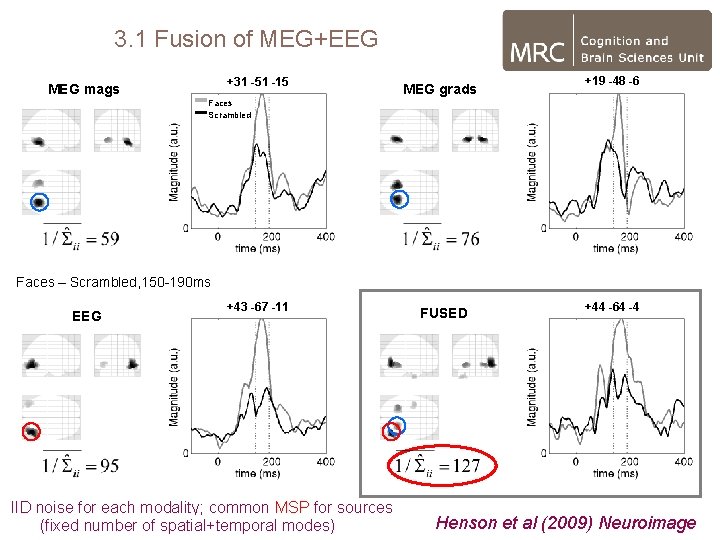
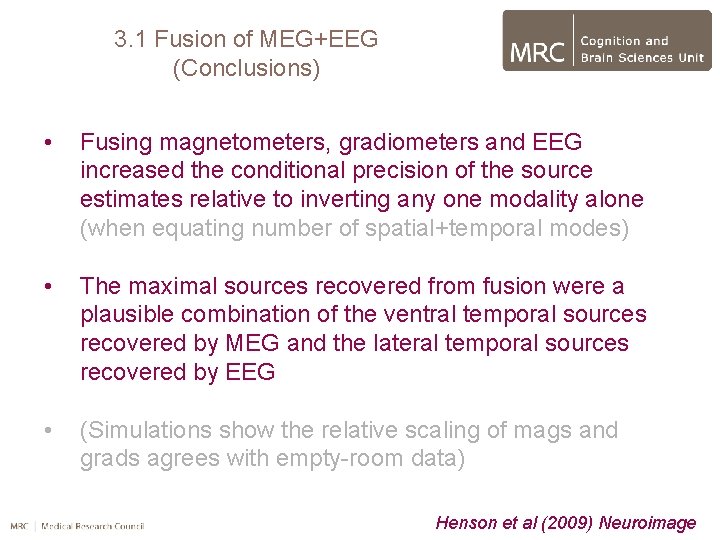
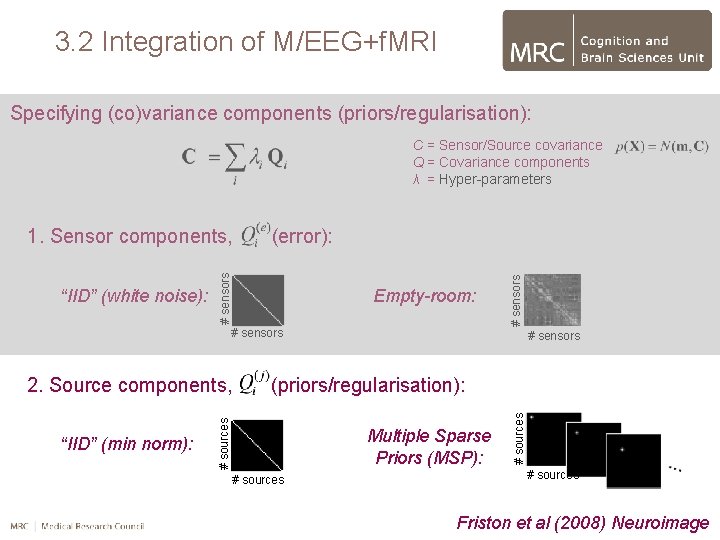
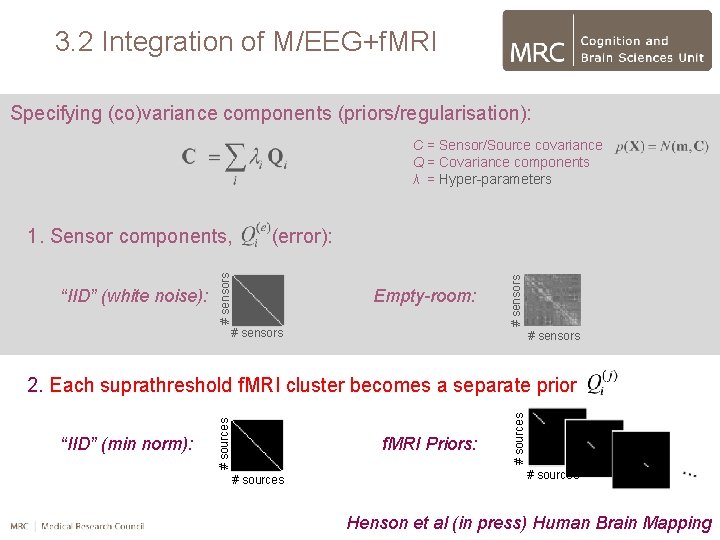
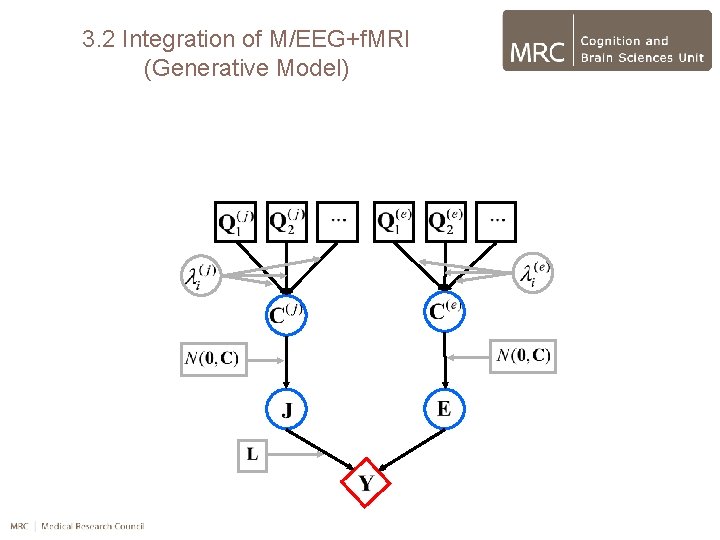
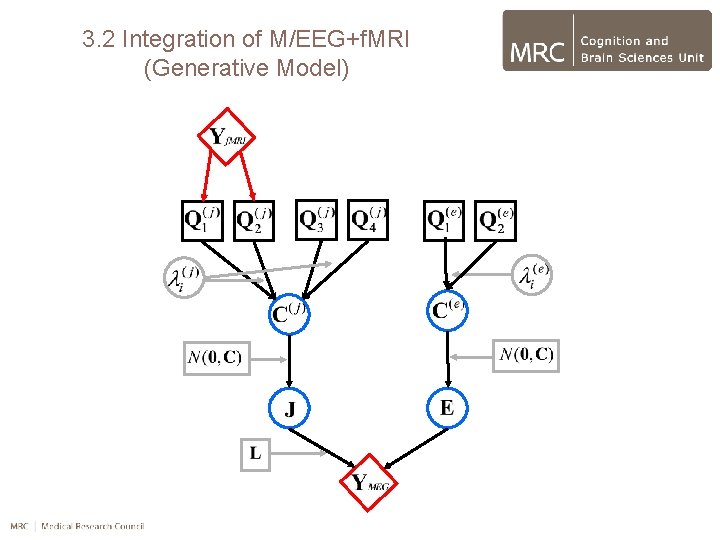
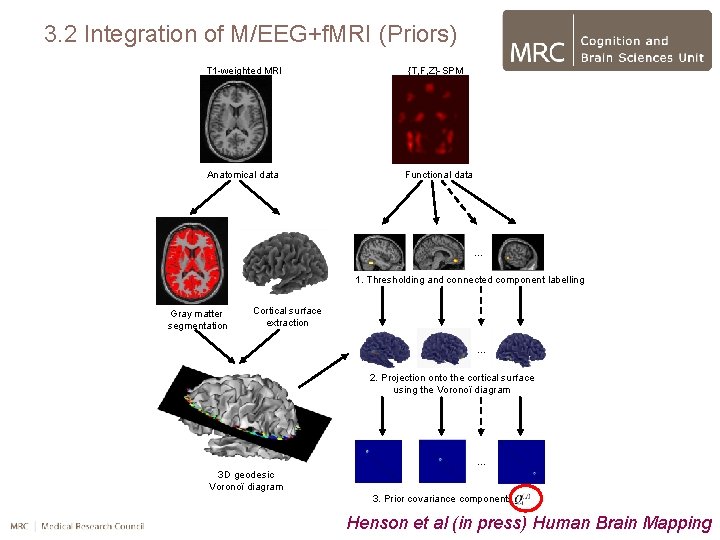
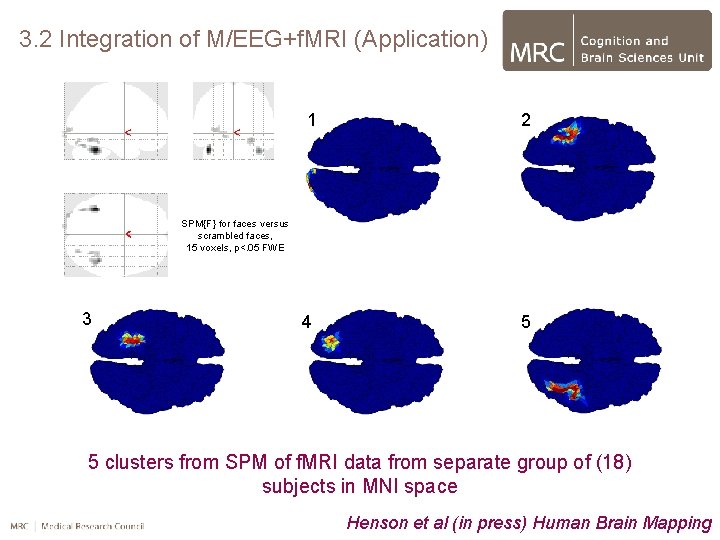
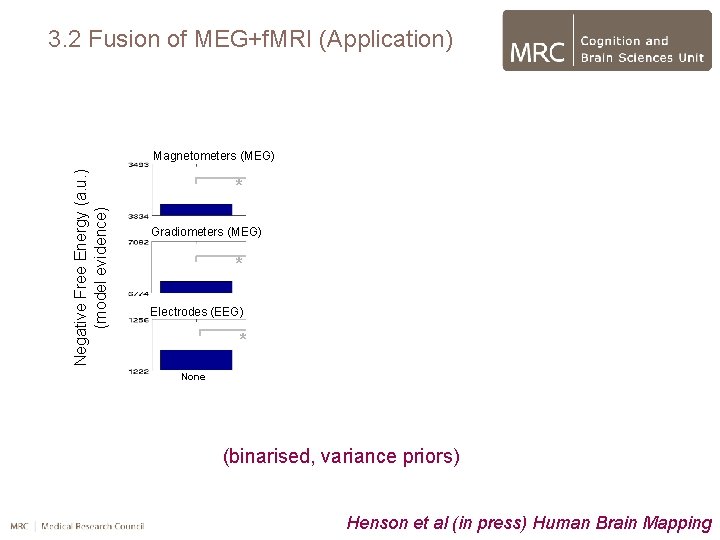
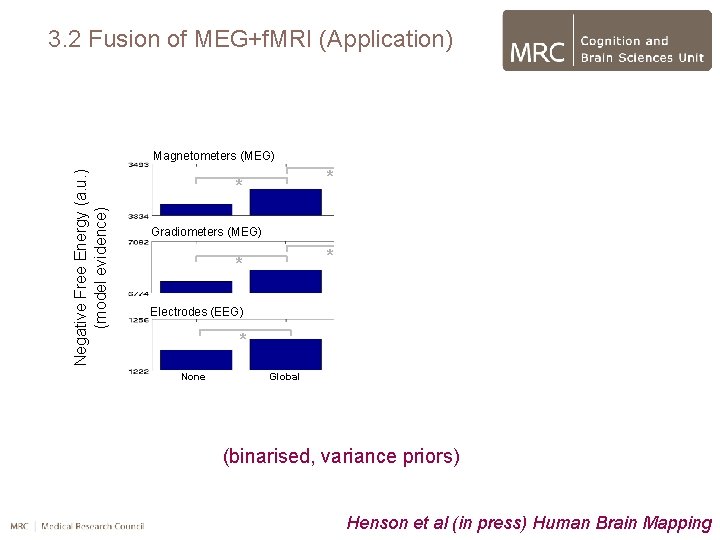
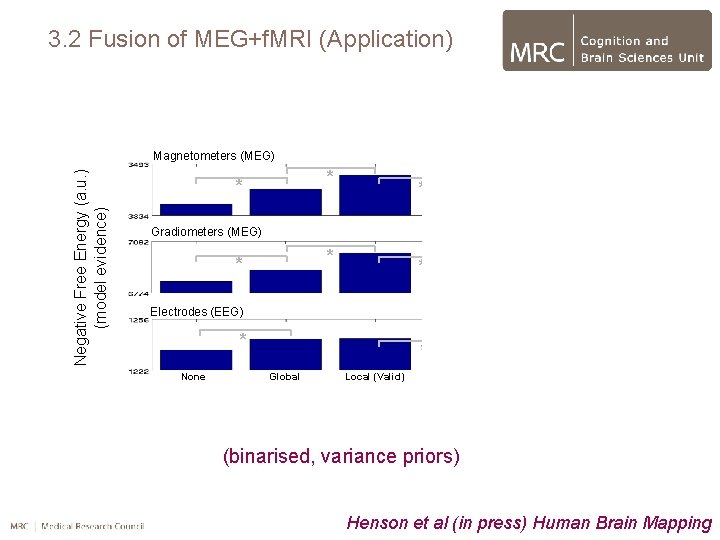
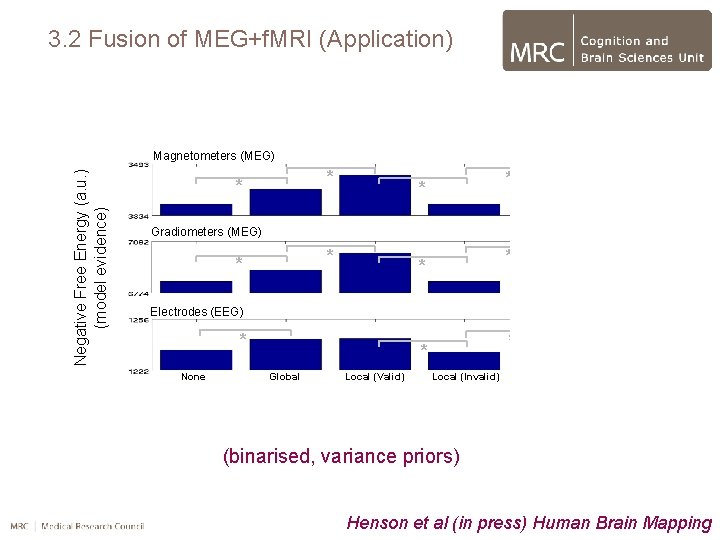
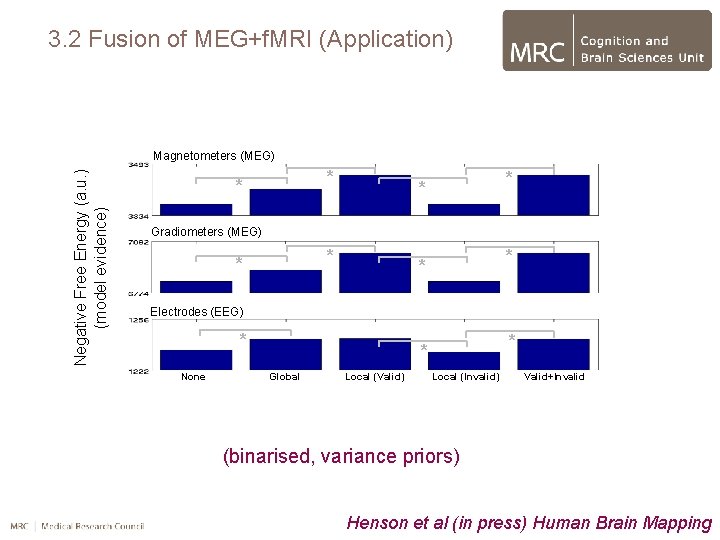
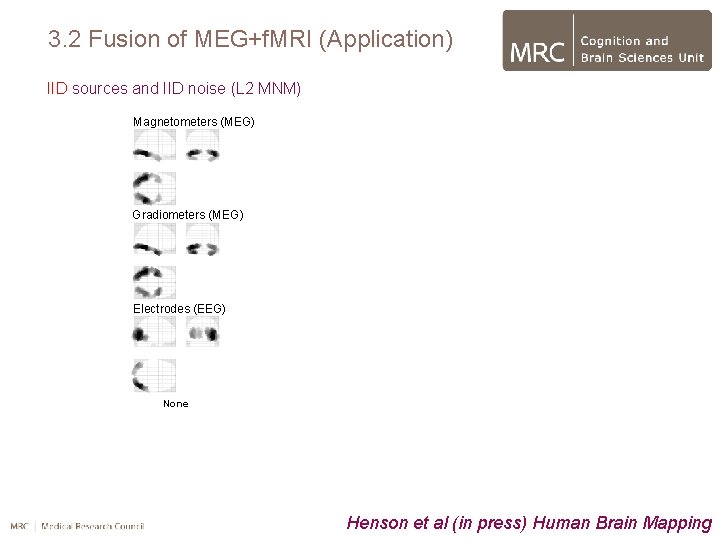
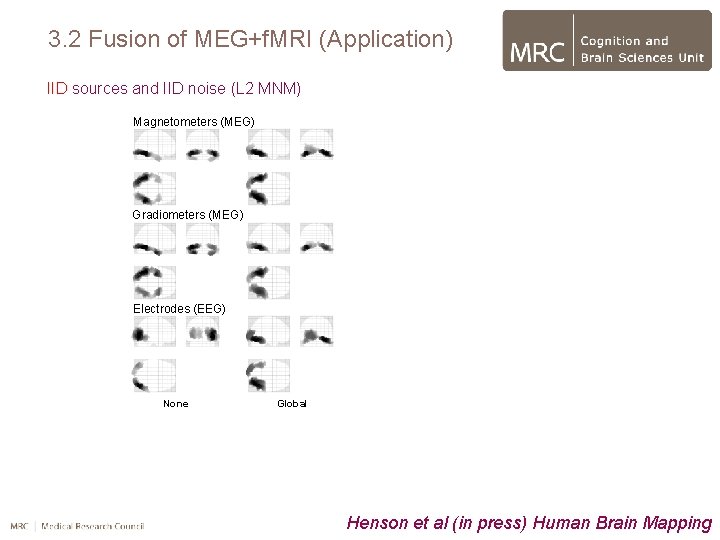
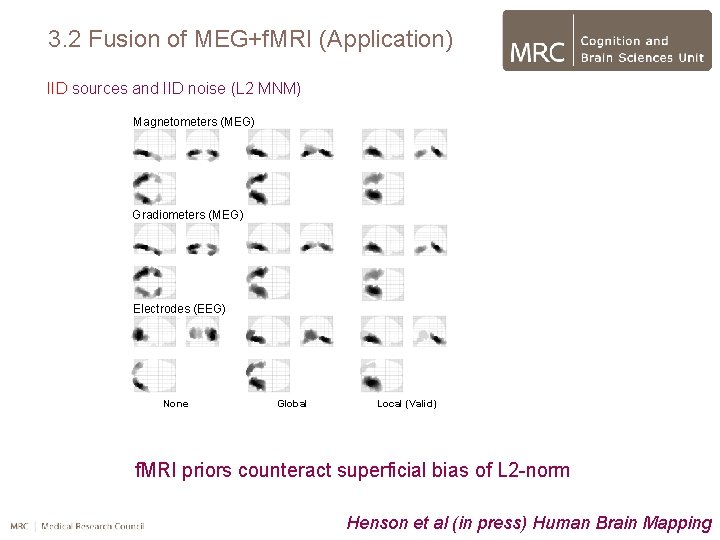
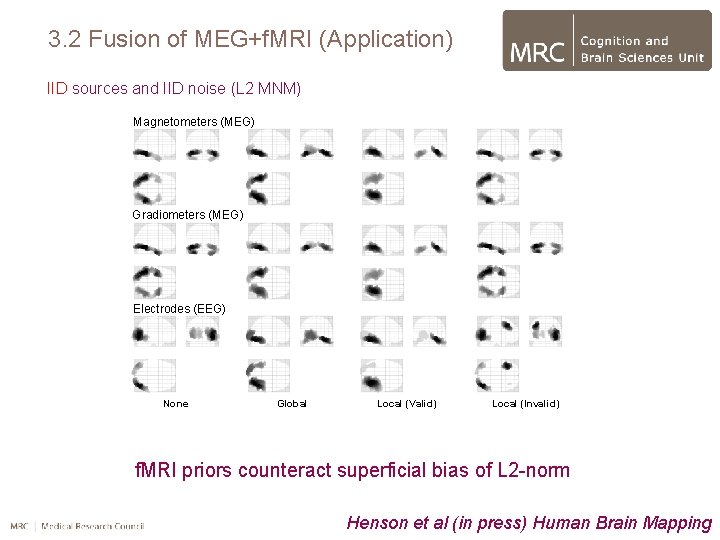
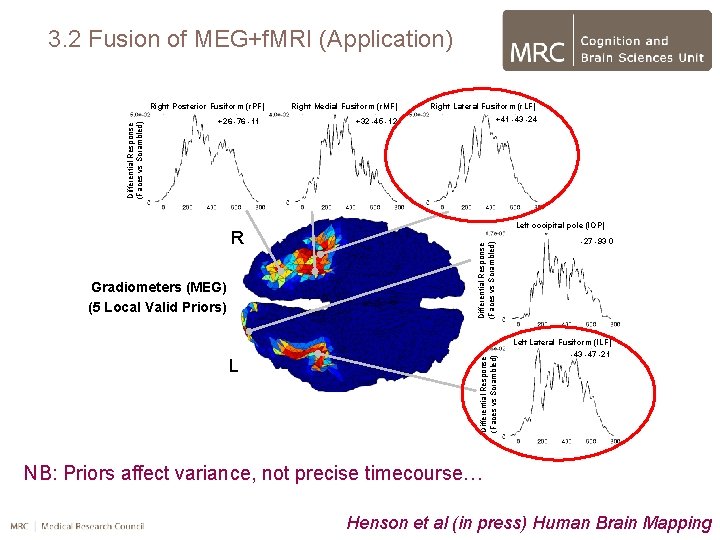
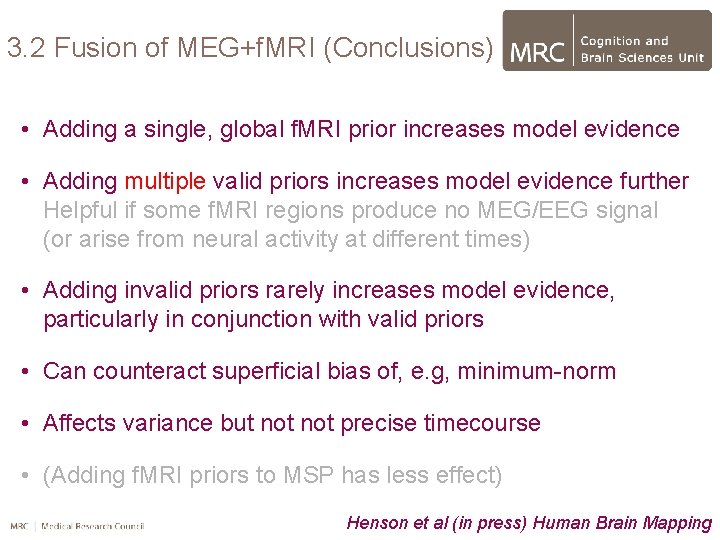
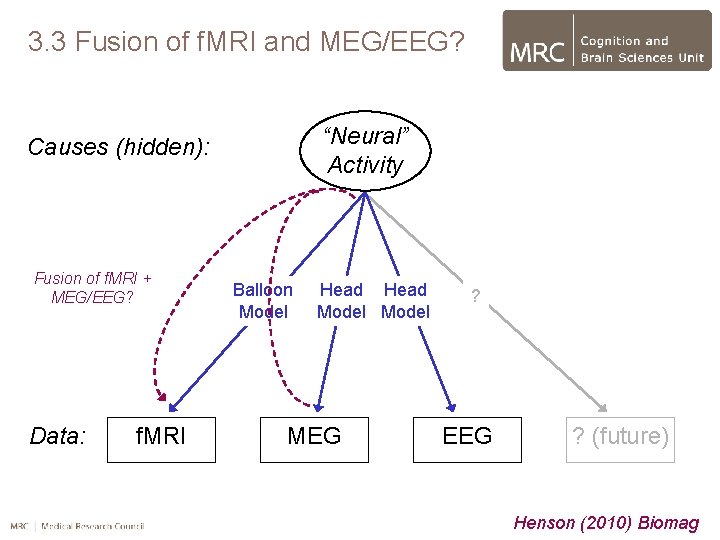
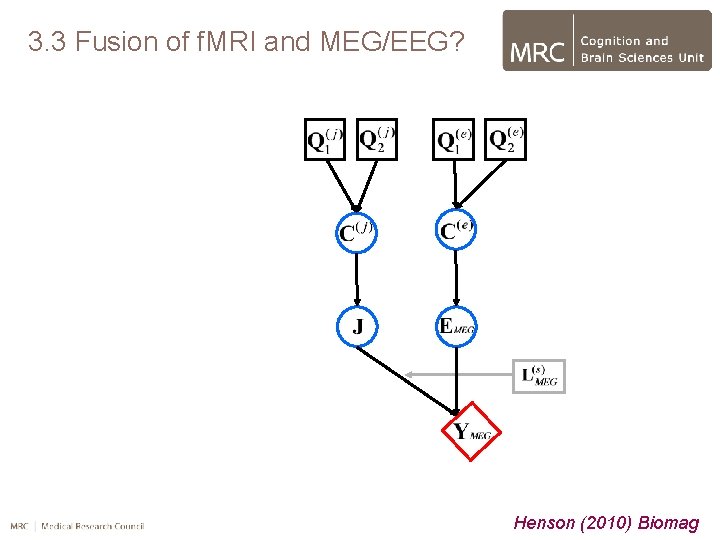
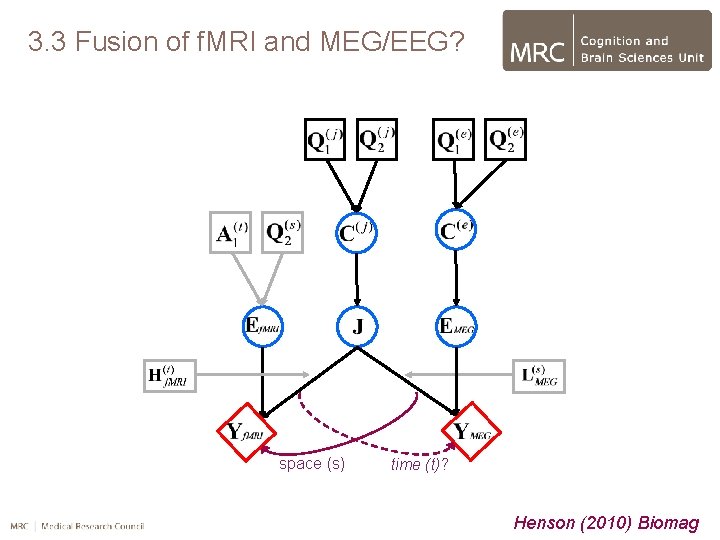
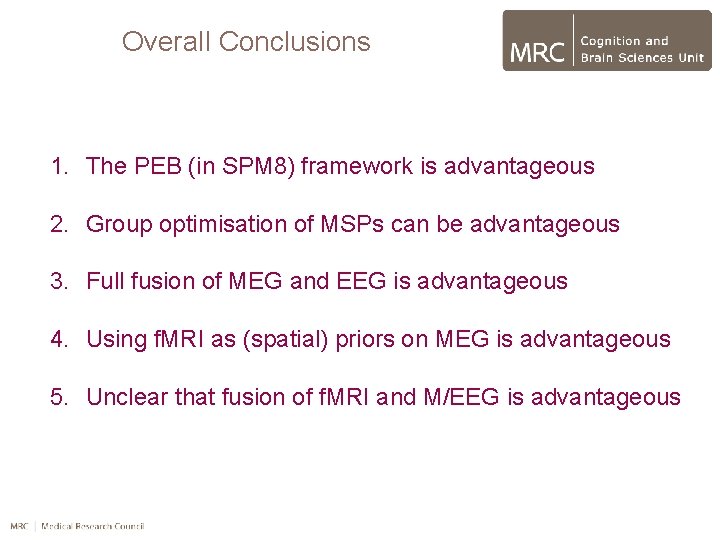
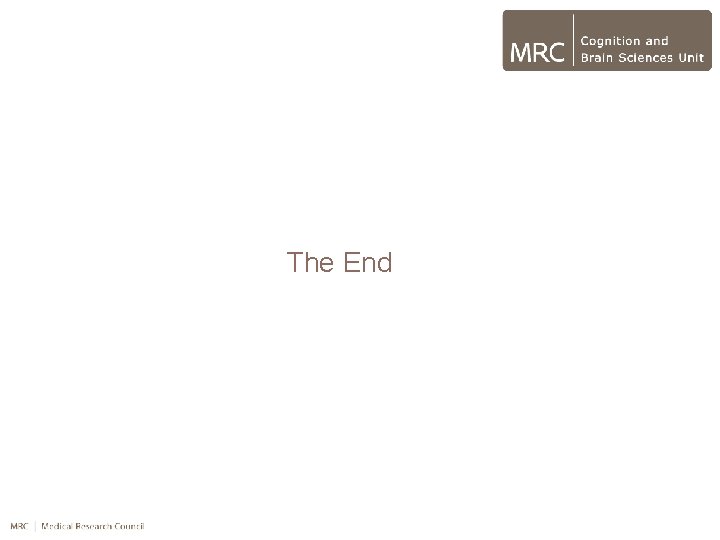
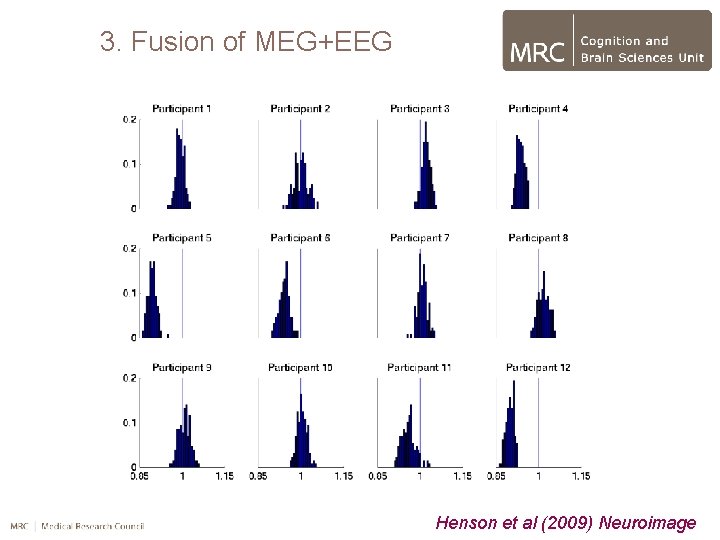
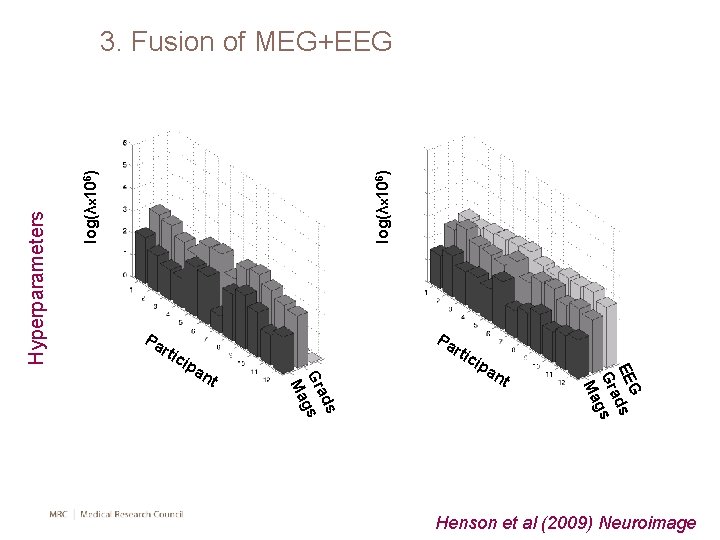
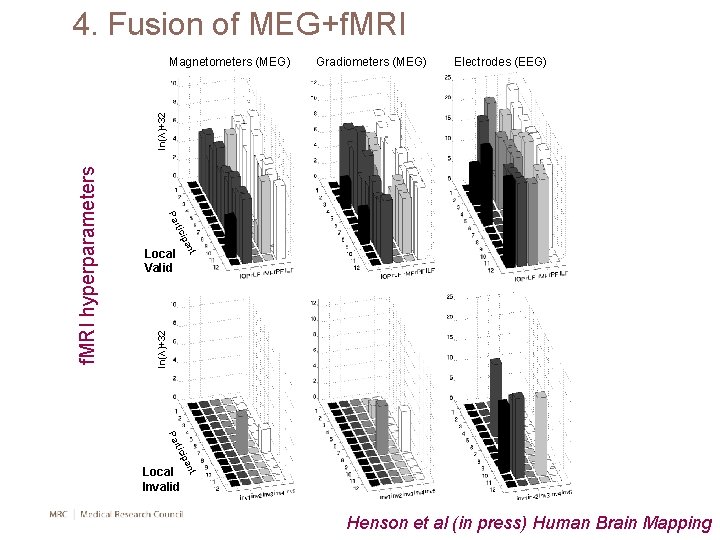
- Slides: 48
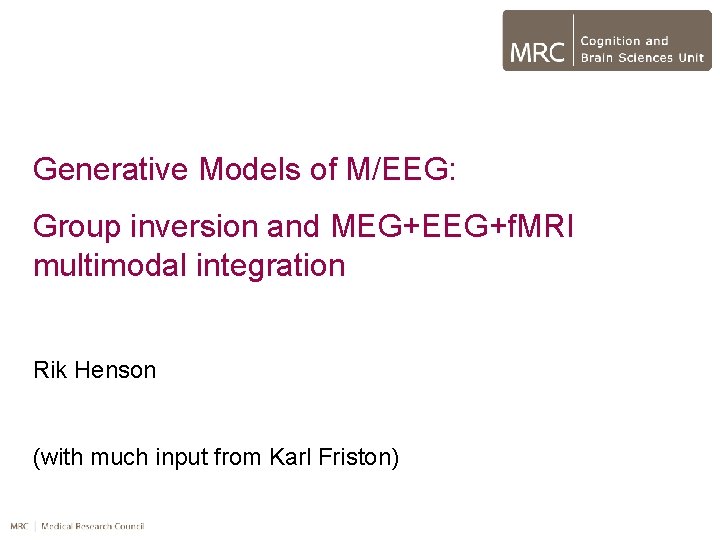
Generative Models of M/EEG: Group inversion and MEG+EEG+f. MRI multimodal integration Rik Henson (with much input from Karl Friston)
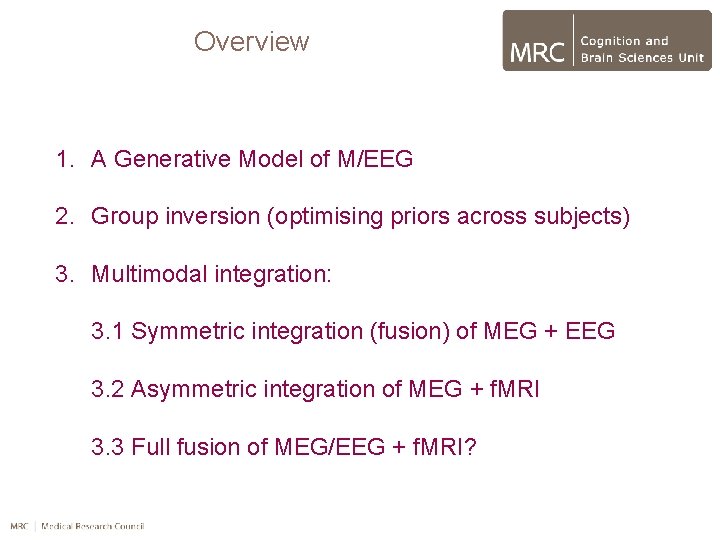
Overview 1. A Generative Model of M/EEG 2. Group inversion (optimising priors across subjects) 3. Multimodal integration: 3. 1 Symmetric integration (fusion) of MEG + EEG 3. 2 Asymmetric integration of MEG + f. MRI 3. 3 Full fusion of MEG/EEG + f. MRI?
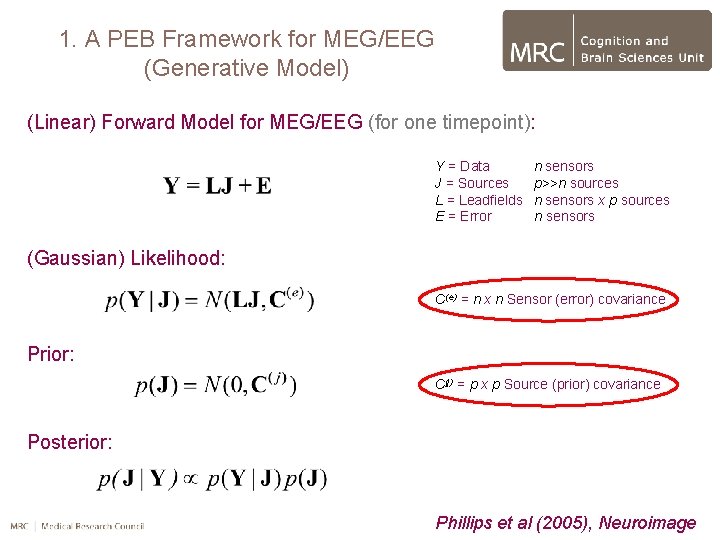
1. A PEB Framework for MEG/EEG (Generative Model) (Linear) Forward Model for MEG/EEG (for one timepoint): Y = Data J = Sources L = Leadfields E = Error n sensors p>>n sources n sensors x p sources n sensors (Gaussian) Likelihood: C(e) = n x n Sensor (error) covariance Prior: C(j) = p x p Source (prior) covariance Posterior: Phillips et al (2005), Neuroimage
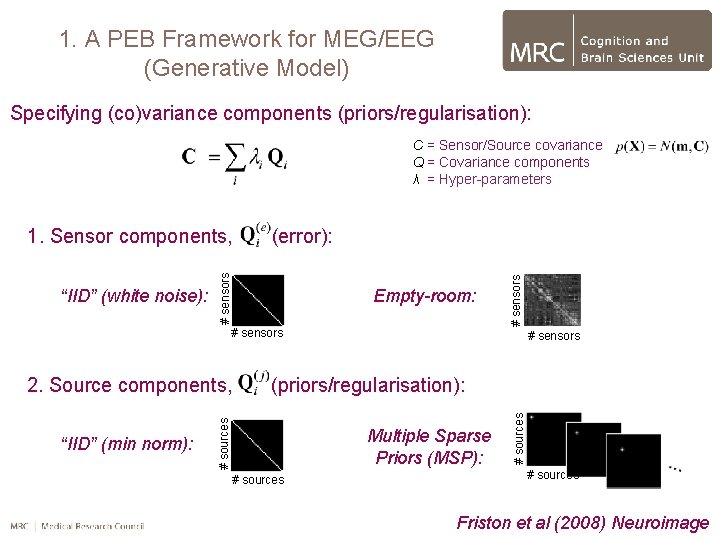
1. A PEB Framework for MEG/EEG (Generative Model) Specifying (co)variance components (priors/regularisation): C = Sensor/Source covariance Q = Covariance components λ = Hyper-parameters # sensors “IID” (min norm): # sensors (priors/regularisation): # sources 2. Source components, # sensors Empty-room: Multiple Sparse Priors (MSP): # sources “IID” (white noise): (error): # sensors 1. Sensor components, # sources Friston et al (2008) Neuroimage
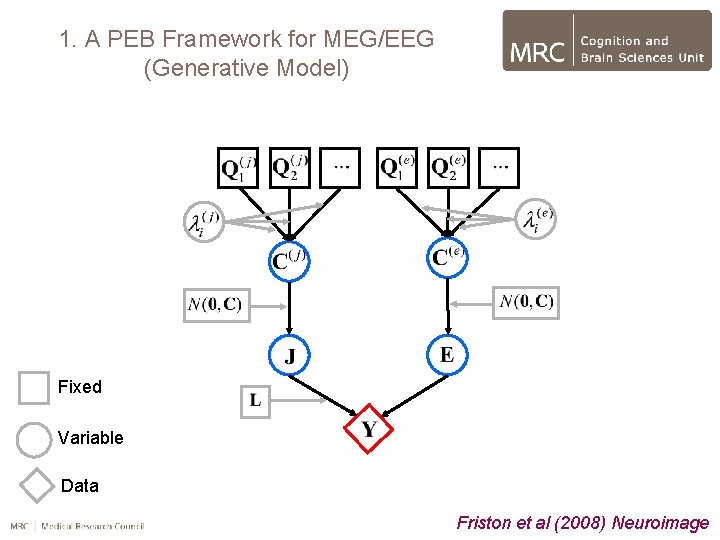
1. A PEB Framework for MEG/EEG (Generative Model) Fixed Variable Data Friston et al (2008) Neuroimage
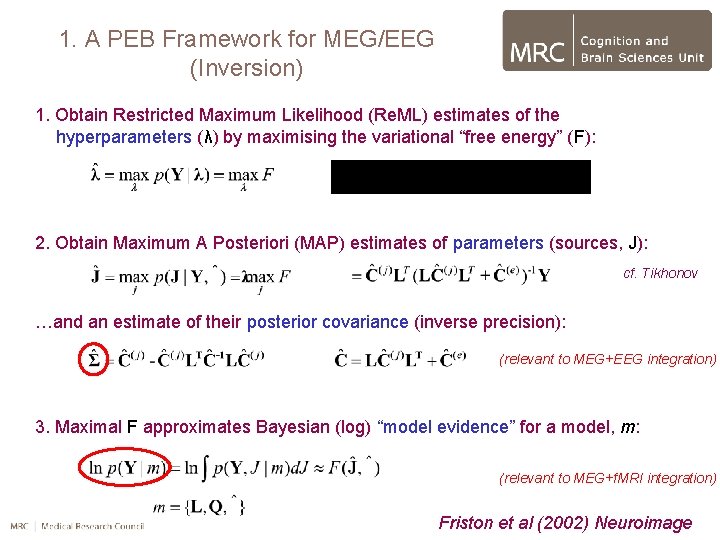
1. A PEB Framework for MEG/EEG (Inversion) 1. Obtain Restricted Maximum Likelihood (Re. ML) estimates of the hyperparameters (λ) by maximising the variational “free energy” (F): 2. Obtain Maximum A Posteriori (MAP) estimates of parameters (sources, J): cf. Tikhonov …and an estimate of their posterior covariance (inverse precision): (relevant to MEG+EEG integration) 3. Maximal F approximates Bayesian (log) “model evidence” for a model, m: (relevant to MEG+f. MRI integration) Friston et al (2002) Neuroimage
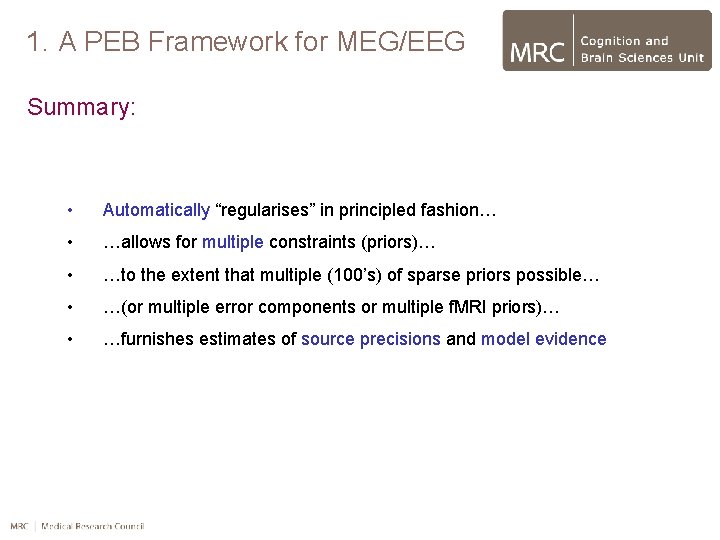
1. A PEB Framework for MEG/EEG Summary: • Automatically “regularises” in principled fashion… • …allows for multiple constraints (priors)… • …to the extent that multiple (100’s) of sparse priors possible… • …(or multiple error components or multiple f. MRI priors)… • …furnishes estimates of source precisions and model evidence
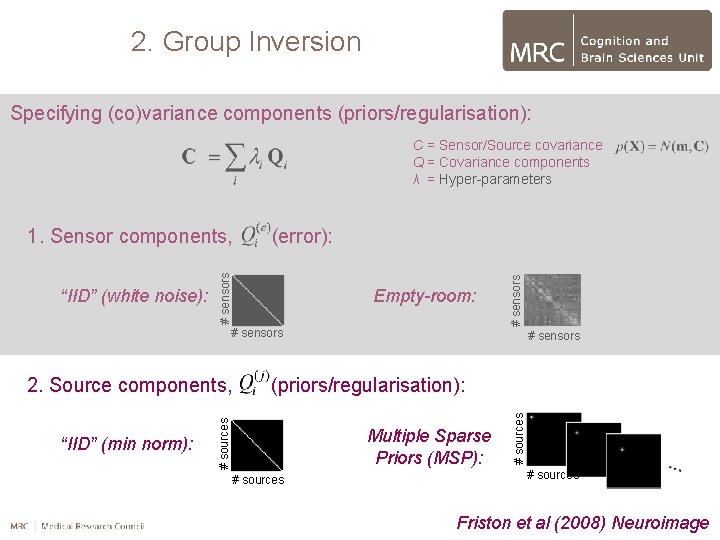
2. Group Inversion Specifying (co)variance components (priors/regularisation): C = Sensor/Source covariance Q = Covariance components λ = Hyper-parameters # sensors “IID” (min norm): # sensors (priors/regularisation): # sources 2. Source components, # sensors Empty-room: Multiple Sparse Priors (MSP): # sources “IID” (white noise): (error): # sensors 1. Sensor components, # sources Friston et al (2008) Neuroimage
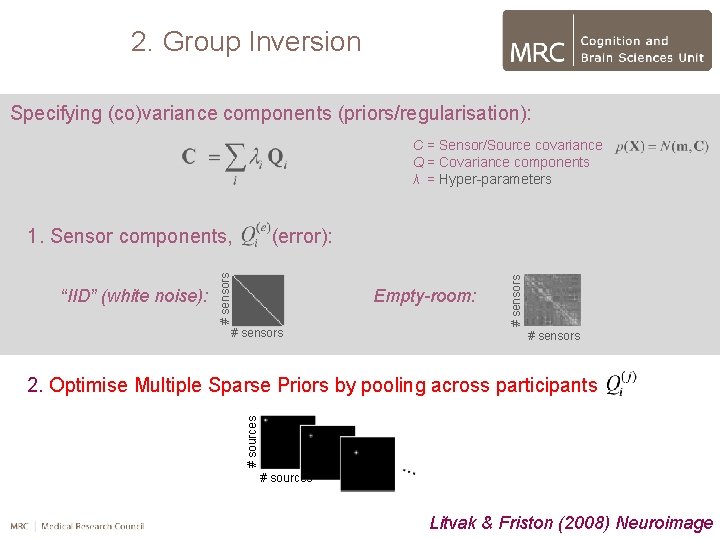
2. Group Inversion Specifying (co)variance components (priors/regularisation): C = Sensor/Source covariance Q = Covariance components λ = Hyper-parameters Empty-room: # sensors “IID” (white noise): (error): # sensors 1. Sensor components, # sensors # sources 2. Optimise Multiple Sparse Priors by pooling across participants # sources Litvak & Friston (2008) Neuroimage
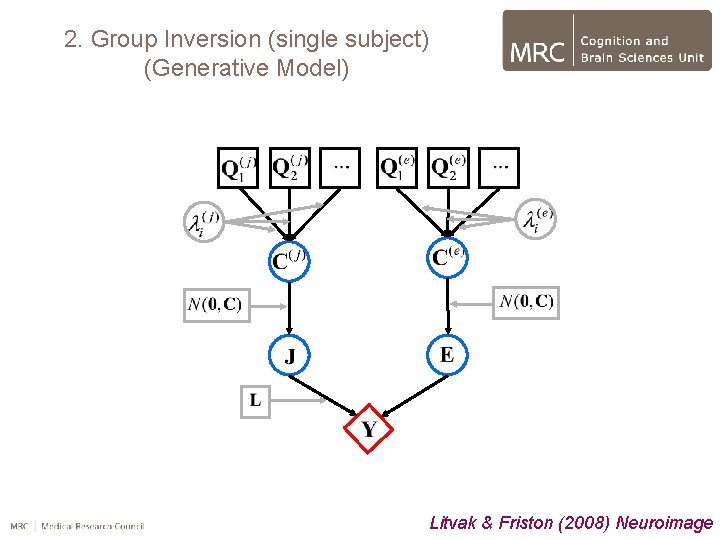
2. Group Inversion (single subject) (Generative Model) Litvak & Friston (2008) Neuroimage
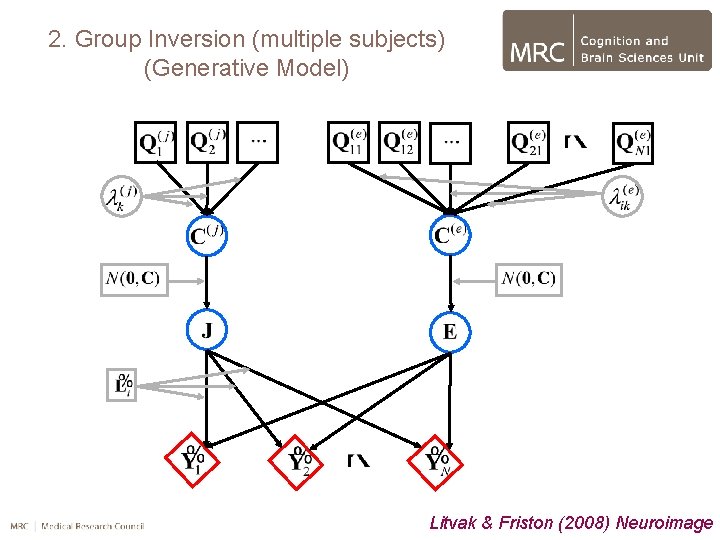
2. Group Inversion (multiple subjects) (Generative Model) Litvak & Friston (2008) Neuroimage
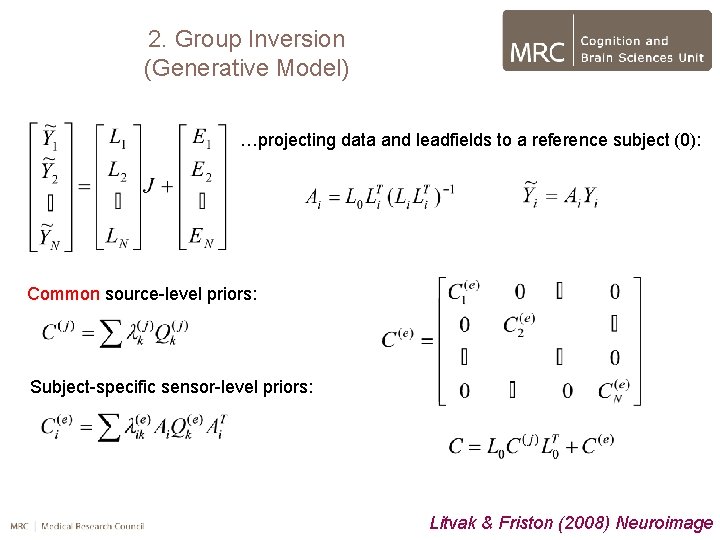
2. Group Inversion (Generative Model) …projecting data and leadfields to a reference subject (0): Common source-level priors: Subject-specific sensor-level priors: Litvak & Friston (2008) Neuroimage
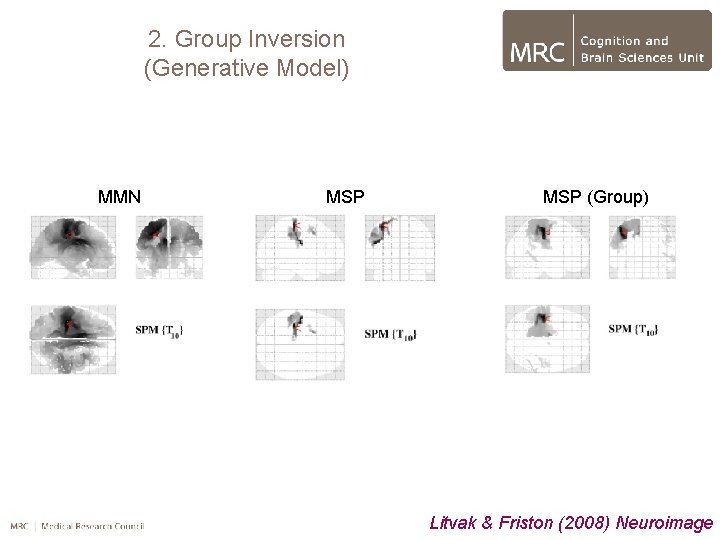
2. Group Inversion (Generative Model) MMN MSP (Group) Litvak & Friston (2008) Neuroimage
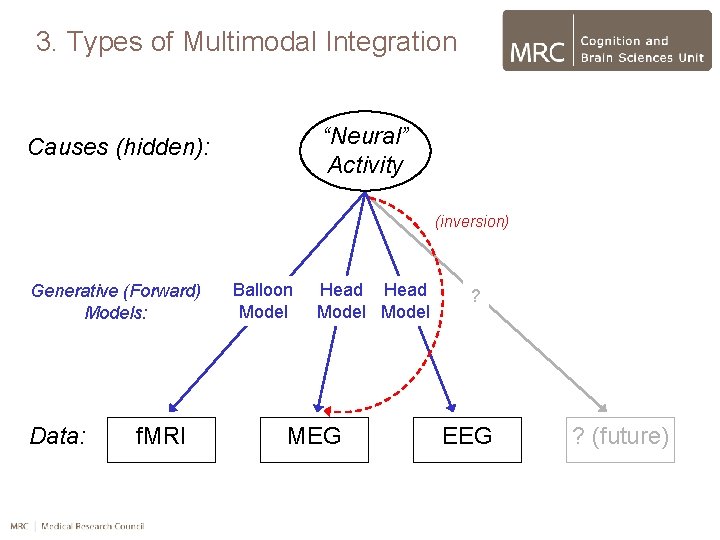
3. Types of Multimodal Integration “Neural” Activity Causes (hidden): (inversion) Generative (Forward) Models: Data: f. MRI Balloon Model Head Model MEG ? EEG ? (future)
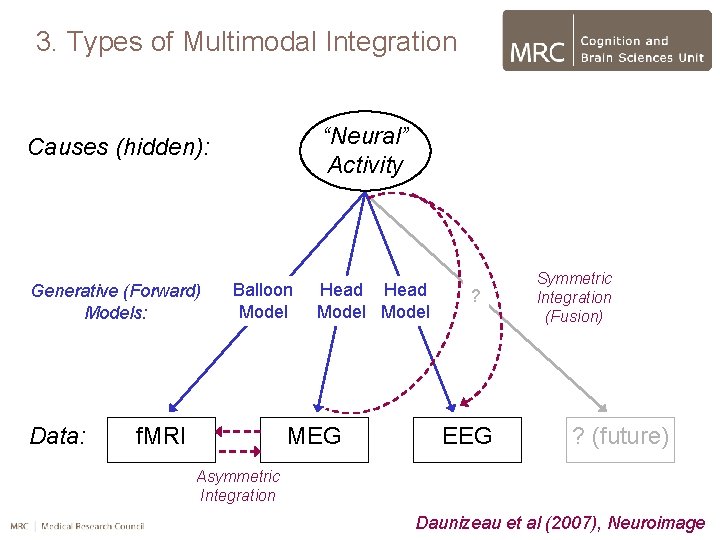
3. Types of Multimodal Integration “Neural” Activity Causes (hidden): Generative (Forward) Models: Data: Balloon Model f. MRI Head Model MEG ? EEG Symmetric Integration (Fusion) ? (future) Asymmetric Integration Daunizeau et al (2007), Neuroimage
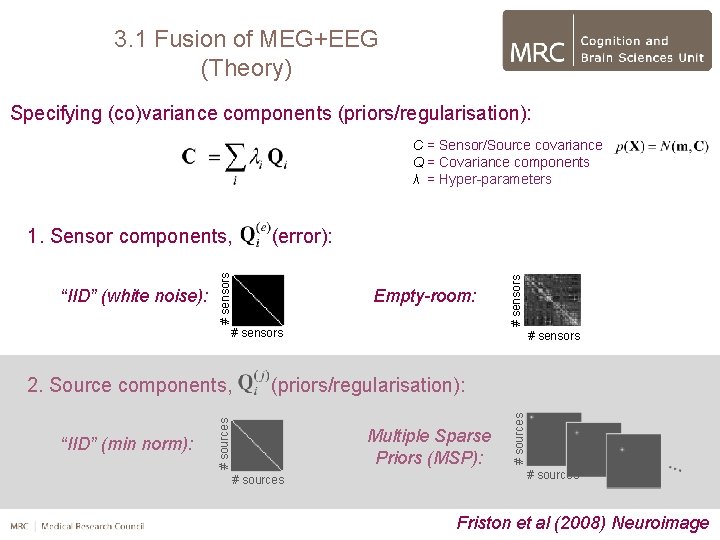
3. 1 Fusion of MEG+EEG (Theory) Specifying (co)variance components (priors/regularisation): C = Sensor/Source covariance Q = Covariance components λ = Hyper-parameters # sensors “IID” (min norm): # sensors (priors/regularisation): # sources 2. Source components, # sensors Empty-room: Multiple Sparse Priors (MSP): # sources “IID” (white noise): (error): # sensors 1. Sensor components, # sources Friston et al (2008) Neuroimage
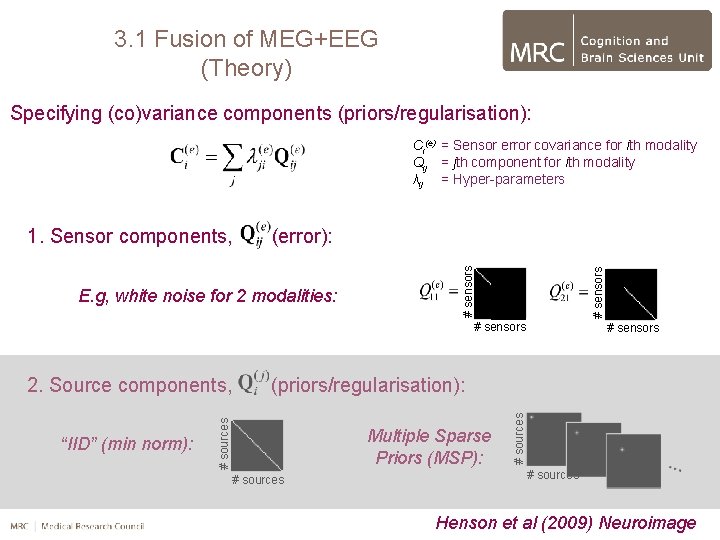
3. 1 Fusion of MEG+EEG (Theory) Specifying (co)variance components (priors/regularisation): Ci(e) = Sensor error covariance for ith modality Qij = jth component for ith modality λij = Hyper-parameters (error): # sensors E. g, white noise for 2 modalities: # sensors 1. Sensor components, # sensors Multiple Sparse Priors (MSP): # sources “IID” (min norm): (priors/regularisation): # sources 2. Source components, # sensors # sources Henson et al (2009) Neuroimage
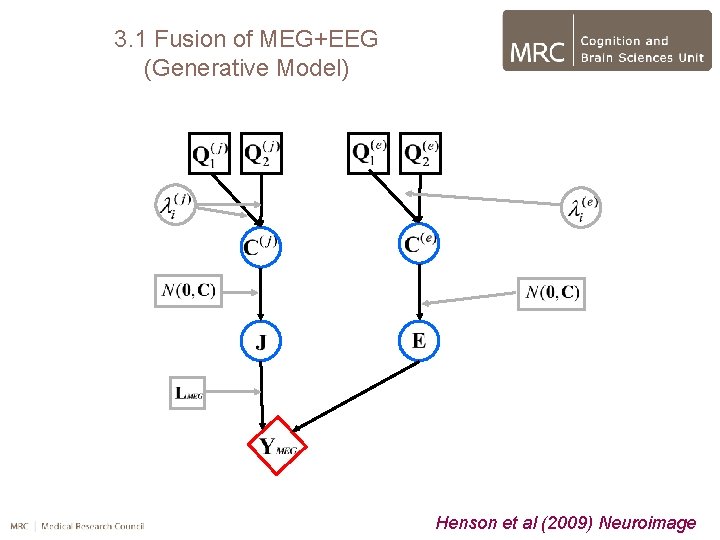
3. 1 Fusion of MEG+EEG (Generative Model) Henson et al (2009) Neuroimage
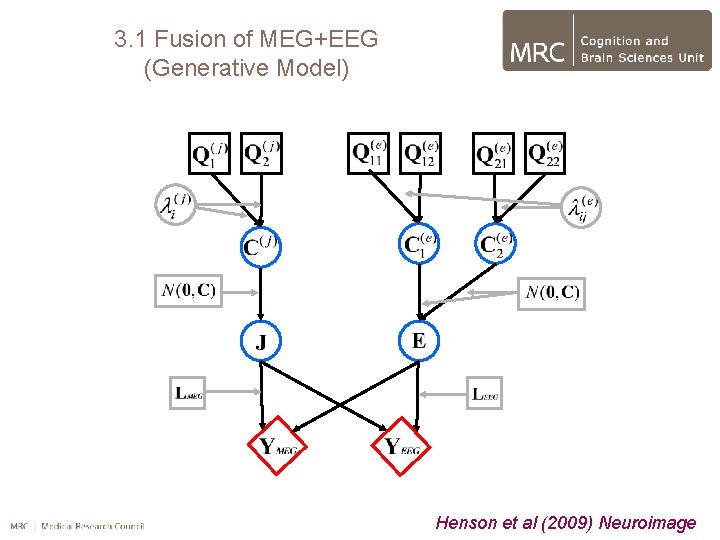
3. 1 Fusion of MEG+EEG (Generative Model) Henson et al (2009) Neuroimage
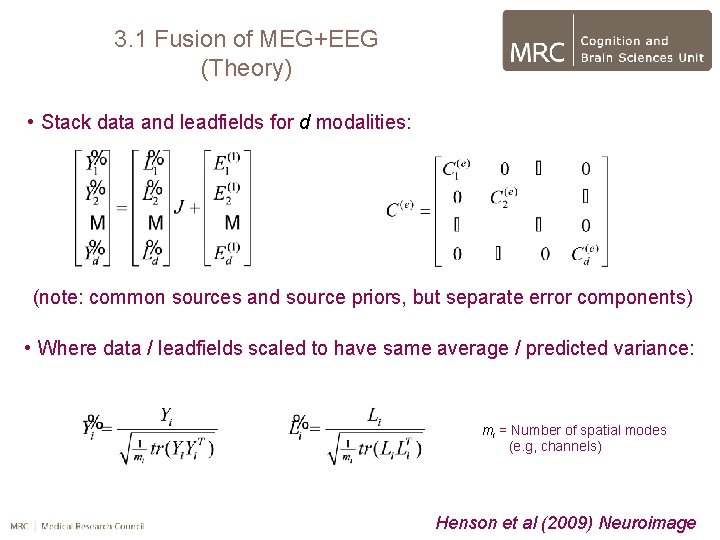
3. 1 Fusion of MEG+EEG (Theory) • Stack data and leadfields for d modalities: (note: common sources and source priors, but separate error components) • Where data / leadfields scaled to have same average / predicted variance: mi = Number of spatial modes (e. g, channels) Henson et al (2009) Neuroimage
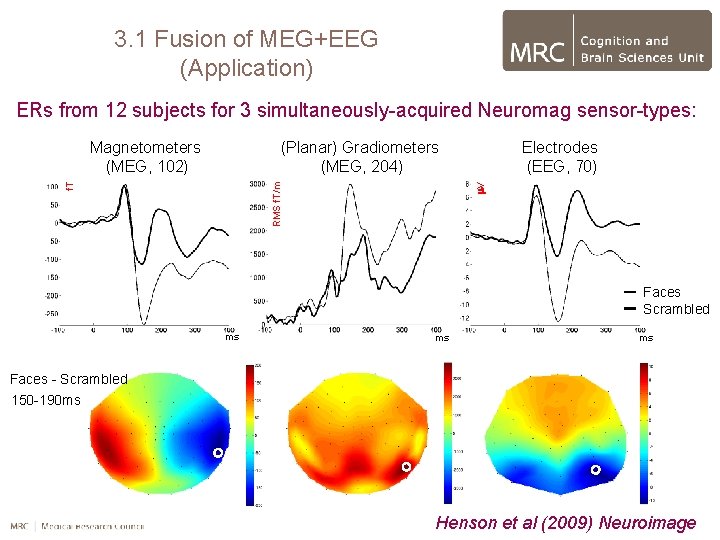
3. 1 Fusion of MEG+EEG (Application) ERs from 12 subjects for 3 simultaneously-acquired Neuromag sensor-types: (Planar) Gradiometers (MEG, 204) Electrodes (EEG, 70) m. V f. T RMS f. T/m Magnetometers (MEG, 102) Faces Scrambled ms ms ms Faces - Scrambled 150 -190 ms Henson et al (2009) Neuroimage
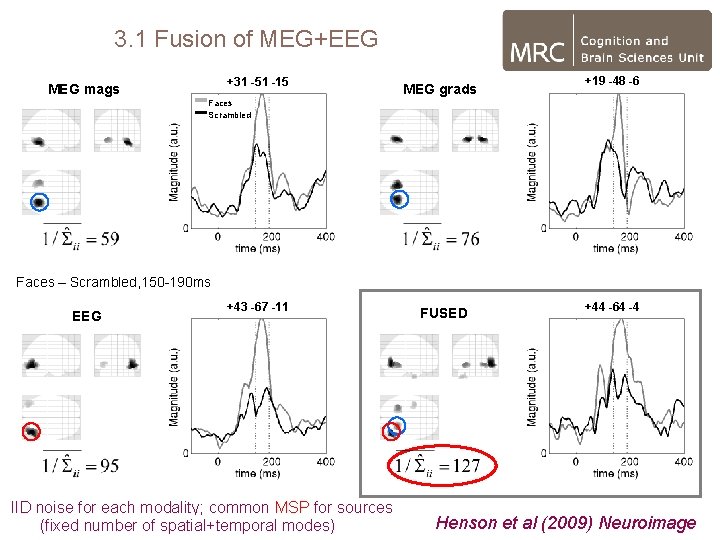
3. 1 Fusion of MEG+EEG +31 -51 -15 MEG mags MEG grads +19 -48 -6 Faces Scrambled Faces – Scrambled, 150 -190 ms EEG +43 -67 -11 IID noise for each modality; common MSP for sources (fixed number of spatial+temporal modes) FUSED +44 -64 -4 Henson et al (2009) Neuroimage
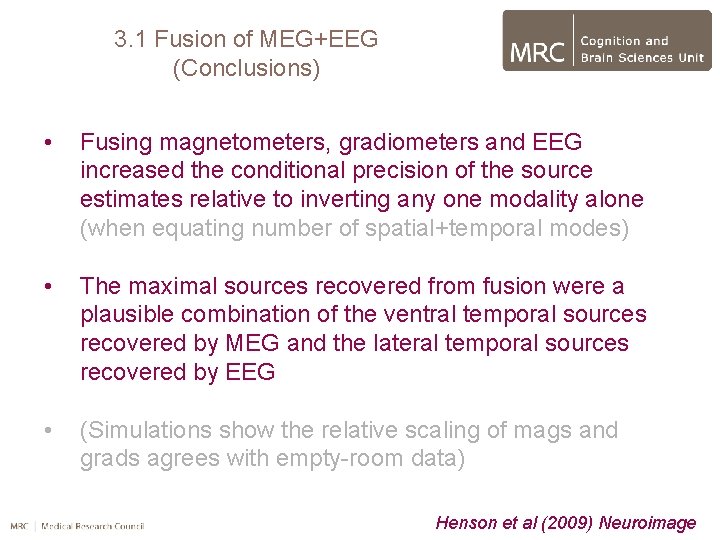
3. 1 Fusion of MEG+EEG (Conclusions) • Fusing magnetometers, gradiometers and EEG increased the conditional precision of the source estimates relative to inverting any one modality alone (when equating number of spatial+temporal modes) • The maximal sources recovered from fusion were a plausible combination of the ventral temporal sources recovered by MEG and the lateral temporal sources recovered by EEG • (Simulations show the relative scaling of mags and grads agrees with empty-room data) Henson et al (2009) Neuroimage
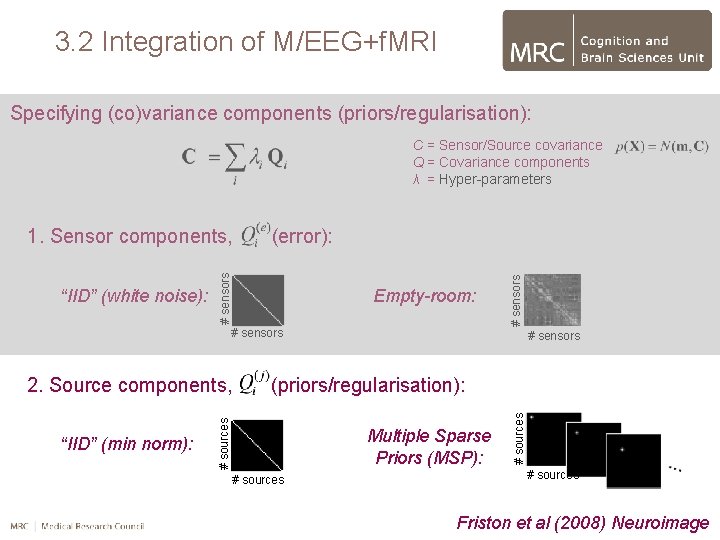
3. 2 Integration of M/EEG+f. MRI Specifying (co)variance components (priors/regularisation): C = Sensor/Source covariance Q = Covariance components λ = Hyper-parameters # sensors “IID” (min norm): # sensors (priors/regularisation): # sources 2. Source components, # sensors Empty-room: Multiple Sparse Priors (MSP): # sources “IID” (white noise): (error): # sensors 1. Sensor components, # sources Friston et al (2008) Neuroimage
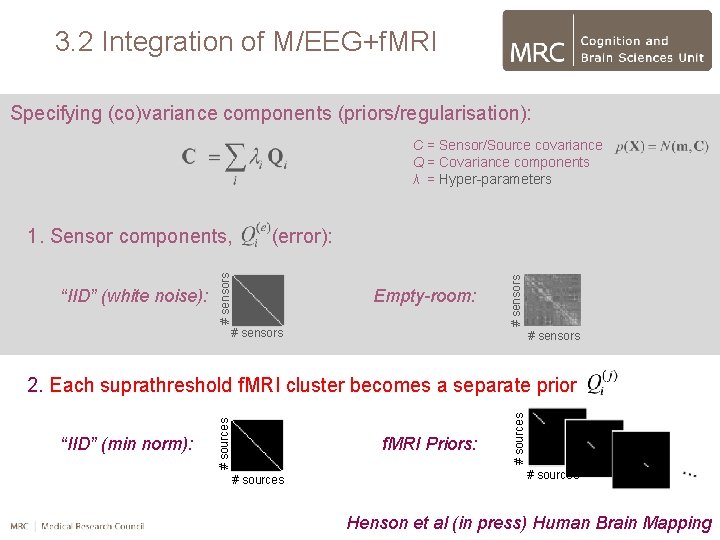
3. 2 Integration of M/EEG+f. MRI Specifying (co)variance components (priors/regularisation): C = Sensor/Source covariance Q = Covariance components λ = Hyper-parameters Empty-room: # sensors “IID” (white noise): (error): # sensors 1. Sensor components, # sensors f. MRI Priors: # sources “IID” (min norm): # sources 2. Each suprathreshold f. MRI cluster becomes a separate prior # sources Henson et al (in press) Human Brain Mapping
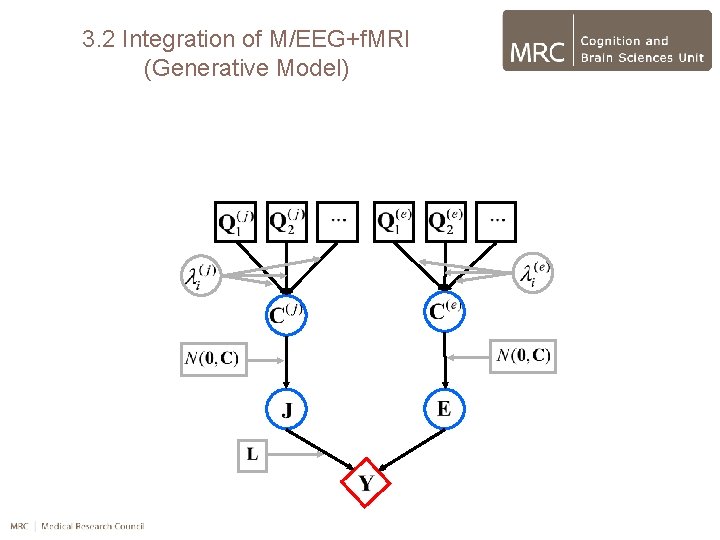
3. 2 Integration of M/EEG+f. MRI (Generative Model)
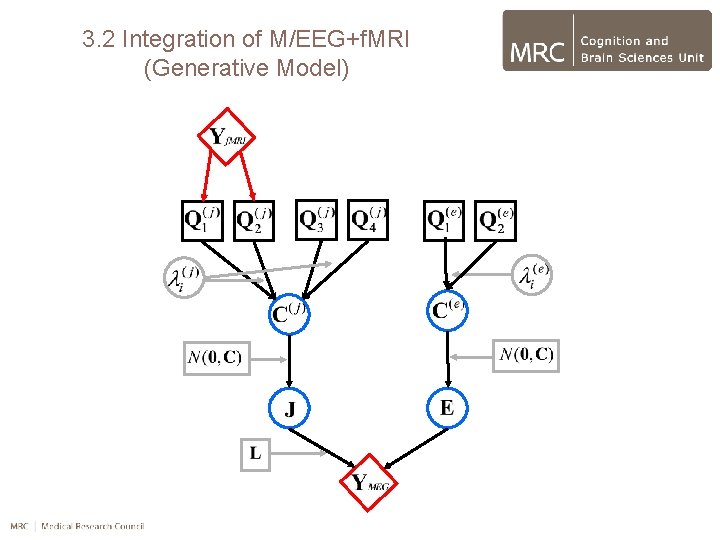
3. 2 Integration of M/EEG+f. MRI (Generative Model)
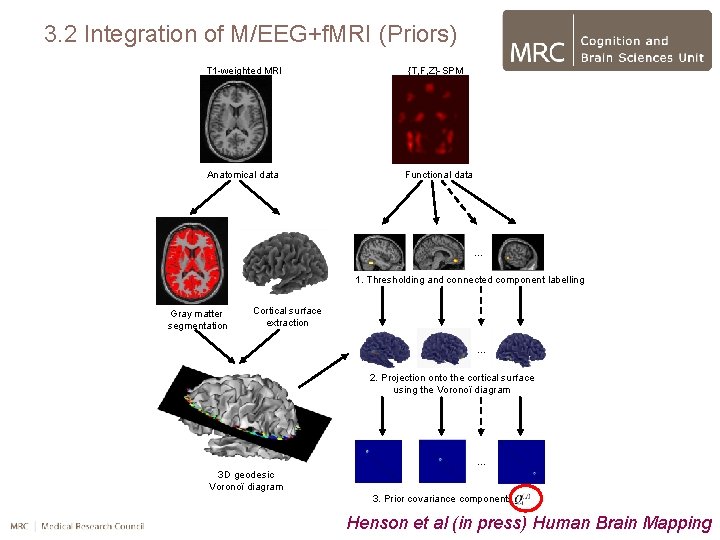
3. 2 Integration of M/EEG+f. MRI (Priors) T 1 -weighted MRI {T, F, Z}-SPM Anatomical data Functional data … 1. Thresholding and connected component labelling Gray matter segmentation Cortical surface extraction … 2. Projection onto the cortical surface using the Voronoï diagram … 3 D geodesic Voronoï diagram 3. Prior covariance components Henson et al (in press) Human Brain Mapping
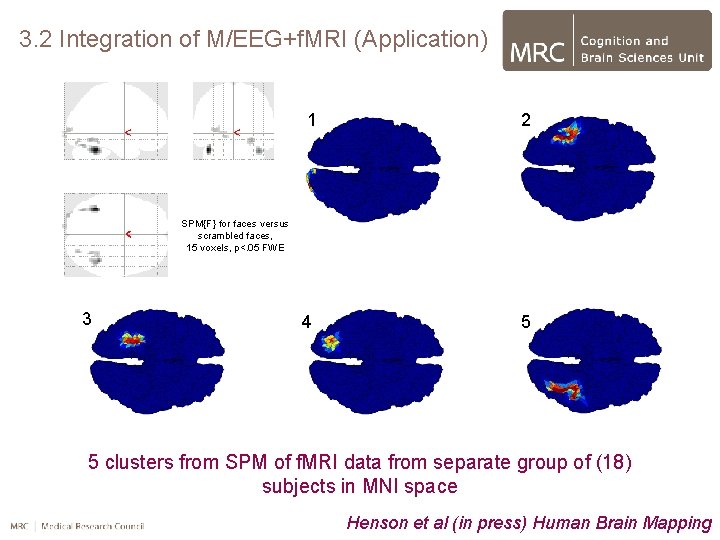
3. 2 Integration of M/EEG+f. MRI (Application) 1 2 4 5 SPM{F} for faces versus scrambled faces, 15 voxels, p<. 05 FWE 3 5 clusters from SPM of f. MRI data from separate group of (18) subjects in MNI space Henson et al (in press) Human Brain Mapping
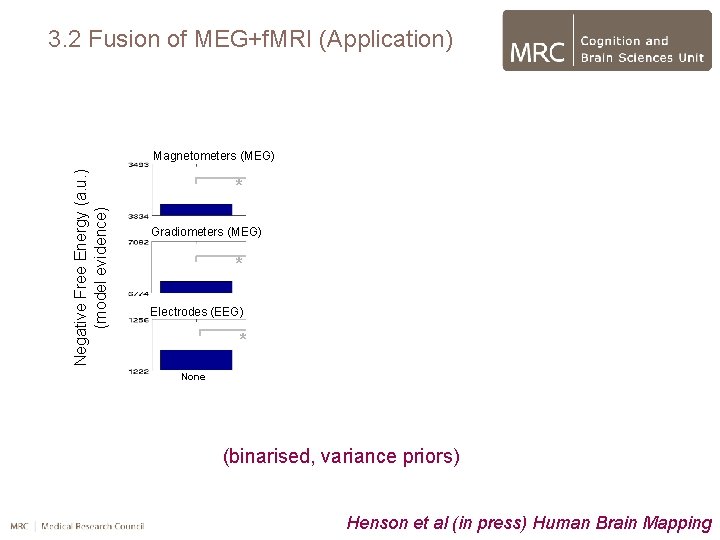
3. 2 Fusion of MEG+f. MRI (Application) Negative Free Energy (a. u. ) (model evidence) Magnetometers (MEG) * * Gradiometers (MEG) * * Electrodes (EEG) * None * * Global Local (Valid) Local (Invalid) Valid+Invalid (binarised, variance priors) Henson et al (in press) Human Brain Mapping
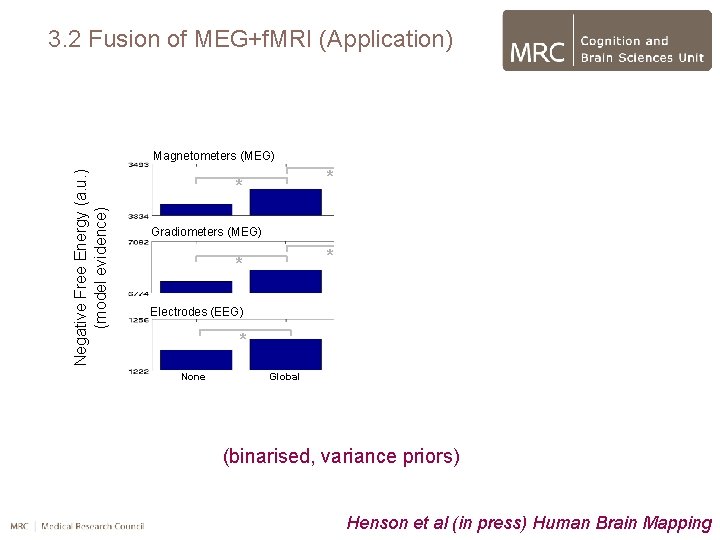
3. 2 Fusion of MEG+f. MRI (Application) Negative Free Energy (a. u. ) (model evidence) Magnetometers (MEG) * * Gradiometers (MEG) * * Electrodes (EEG) * None * * Global Local (Valid) Local (Invalid) Valid+Invalid (binarised, variance priors) Henson et al (in press) Human Brain Mapping
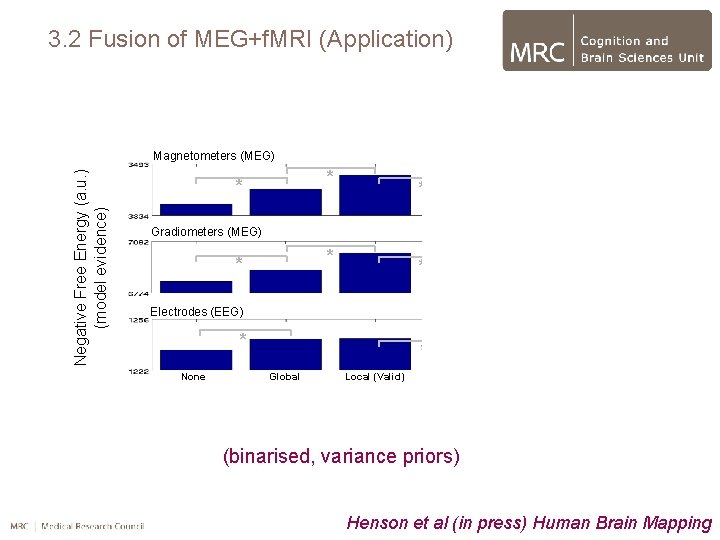
3. 2 Fusion of MEG+f. MRI (Application) Negative Free Energy (a. u. ) (model evidence) Magnetometers (MEG) * * Gradiometers (MEG) * * Electrodes (EEG) * None * * Global Local (Valid) Local (Invalid) Valid+Invalid (binarised, variance priors) Henson et al (in press) Human Brain Mapping
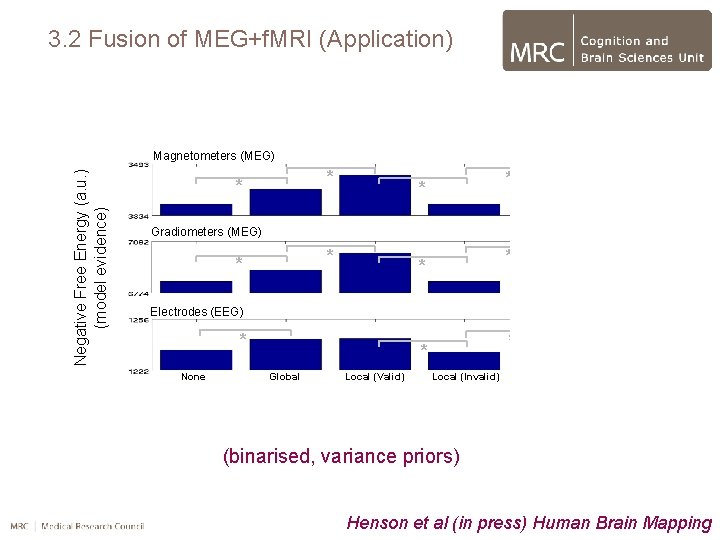
3. 2 Fusion of MEG+f. MRI (Application) Negative Free Energy (a. u. ) (model evidence) Magnetometers (MEG) * * Gradiometers (MEG) * * Electrodes (EEG) * None * * Global Local (Valid) Local (Invalid) Valid+Invalid (binarised, variance priors) Henson et al (in press) Human Brain Mapping
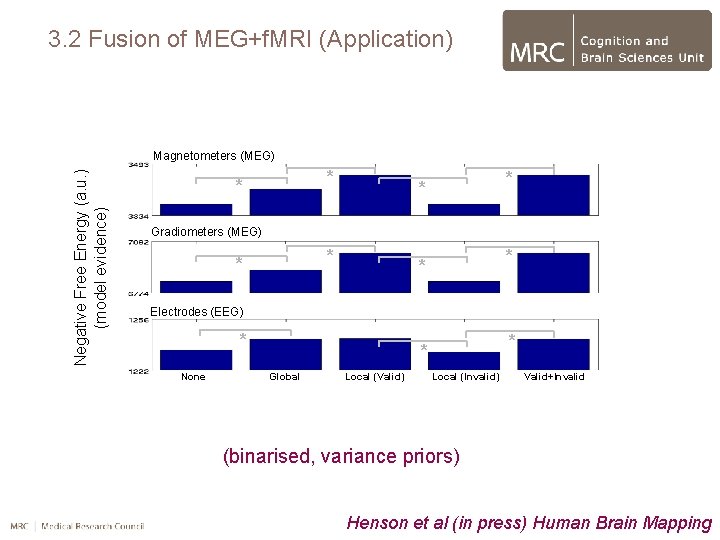
3. 2 Fusion of MEG+f. MRI (Application) Negative Free Energy (a. u. ) (model evidence) Magnetometers (MEG) * * Gradiometers (MEG) * * Electrodes (EEG) * None * * Global Local (Valid) Local (Invalid) Valid+Invalid (binarised, variance priors) Henson et al (in press) Human Brain Mapping
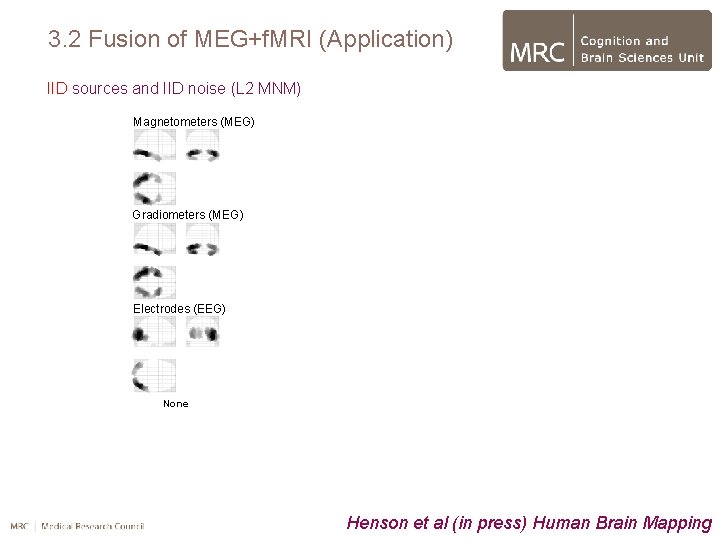
3. 2 Fusion of MEG+f. MRI (Application) IID sources and IID noise (L 2 MNM) Magnetometers (MEG) Gradiometers (MEG) Electrodes (EEG) None Global Local (Valid) Local (Invalid) Henson et al (in press) Human Brain Mapping
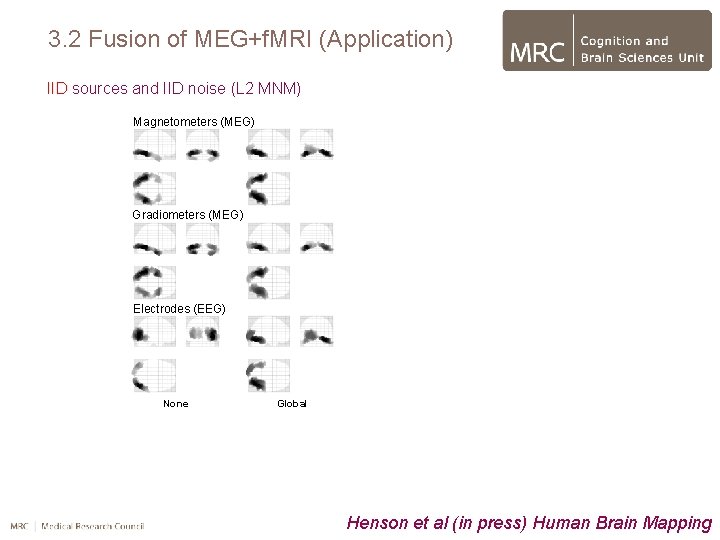
3. 2 Fusion of MEG+f. MRI (Application) IID sources and IID noise (L 2 MNM) Magnetometers (MEG) Gradiometers (MEG) Electrodes (EEG) None Global Local (Valid) Local (Invalid) Henson et al (in press) Human Brain Mapping
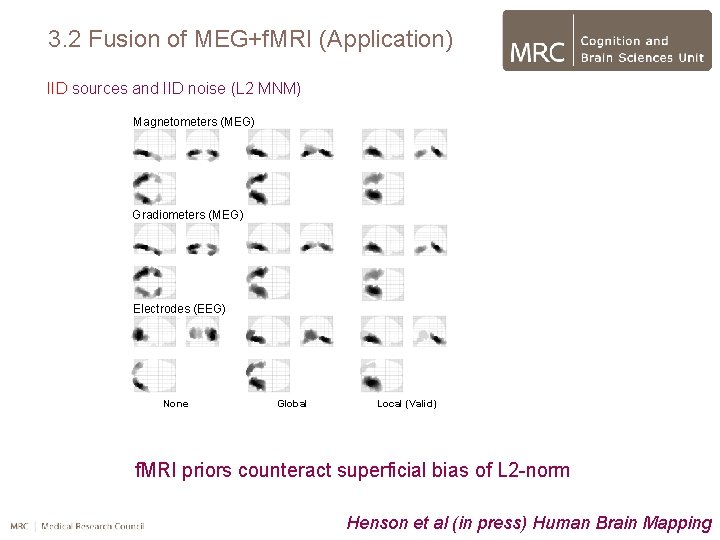
3. 2 Fusion of MEG+f. MRI (Application) IID sources and IID noise (L 2 MNM) Magnetometers (MEG) Gradiometers (MEG) Electrodes (EEG) None Global Local (Valid) Local (Invalid) f. MRI priors counteract superficial bias of L 2 -norm Henson et al (in press) Human Brain Mapping
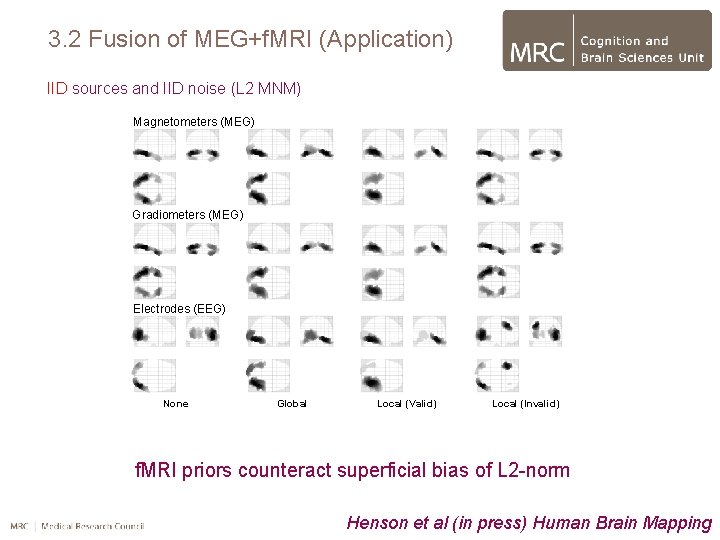
3. 2 Fusion of MEG+f. MRI (Application) IID sources and IID noise (L 2 MNM) Magnetometers (MEG) Gradiometers (MEG) Electrodes (EEG) None Global Local (Valid) Local (Invalid) f. MRI priors counteract superficial bias of L 2 -norm Henson et al (in press) Human Brain Mapping
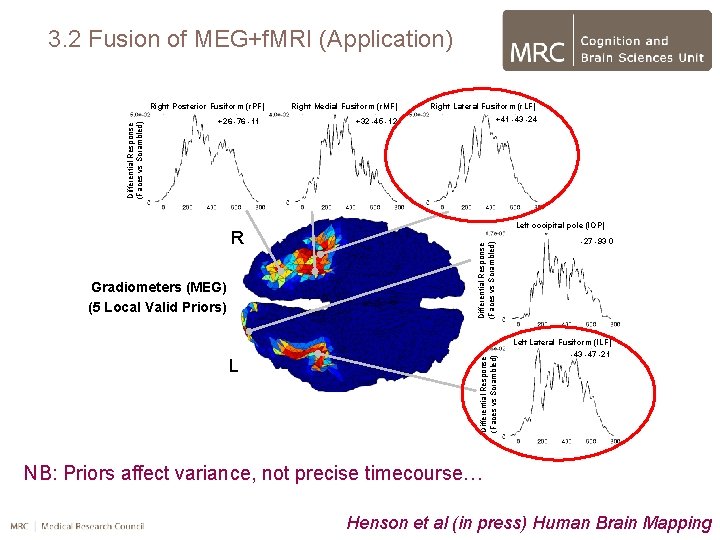
3. 2 Fusion of MEG+f. MRI (Application) R Gradiometers (MEG) (5 Local Valid Priors) L Right Medial Fusiform (r. MF) Right Lateral Fusiform (r. LF) +32 -45 -12 +41 -43 -24 Left occipital pole (l. OP) Differential Response (Faces vs Scrambled) +26 -76 -11 Differential Response (Faces vs Scrambled) Right Posterior Fusiform (r. PF) -27 -93 0 Left Lateral Fusiform (l. LF) -43 -47 -21 NB: Priors affect variance, not precise timecourse… Henson et al (in press) Human Brain Mapping
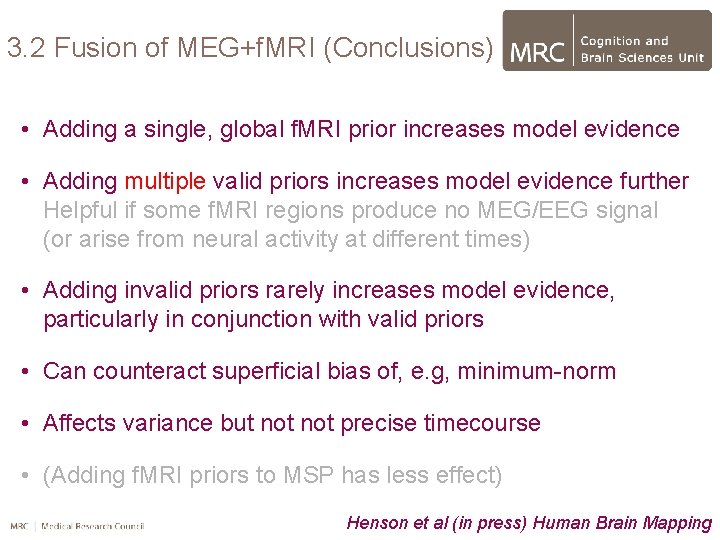
3. 2 Fusion of MEG+f. MRI (Conclusions) • Adding a single, global f. MRI prior increases model evidence • Adding multiple valid priors increases model evidence further Helpful if some f. MRI regions produce no MEG/EEG signal (or arise from neural activity at different times) • Adding invalid priors rarely increases model evidence, particularly in conjunction with valid priors • Can counteract superficial bias of, e. g, minimum-norm • Affects variance but not precise timecourse • (Adding f. MRI priors to MSP has less effect) Henson et al (in press) Human Brain Mapping
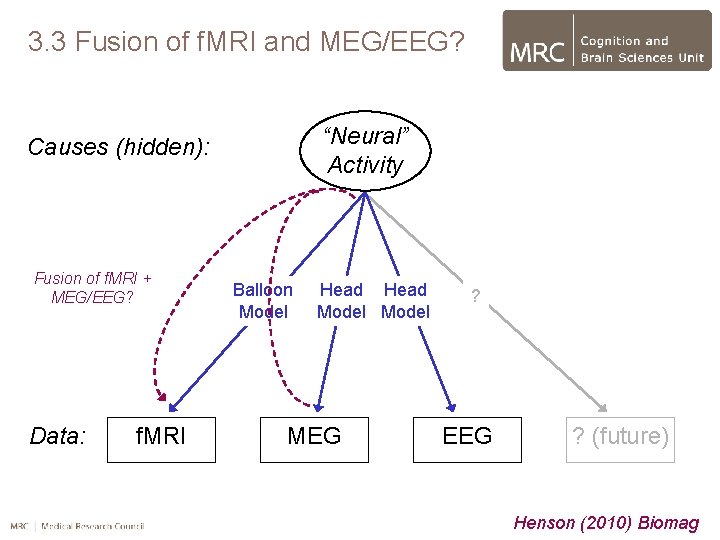
3. 3 Fusion of f. MRI and MEG/EEG? “Neural” Activity Causes (hidden): Fusion of f. MRI + MEG/EEG? Data: f. MRI Balloon Model Head Model MEG ? EEG ? (future) Henson (2010) Biomag
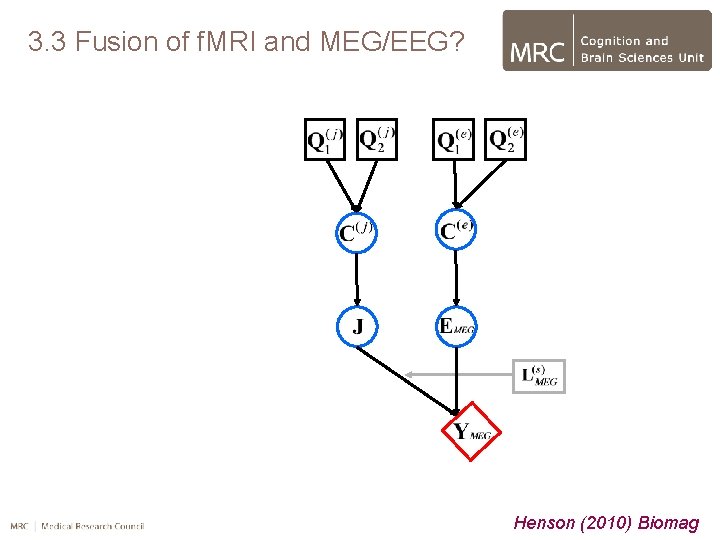
3. 3 Fusion of f. MRI and MEG/EEG? Henson (2010) Biomag
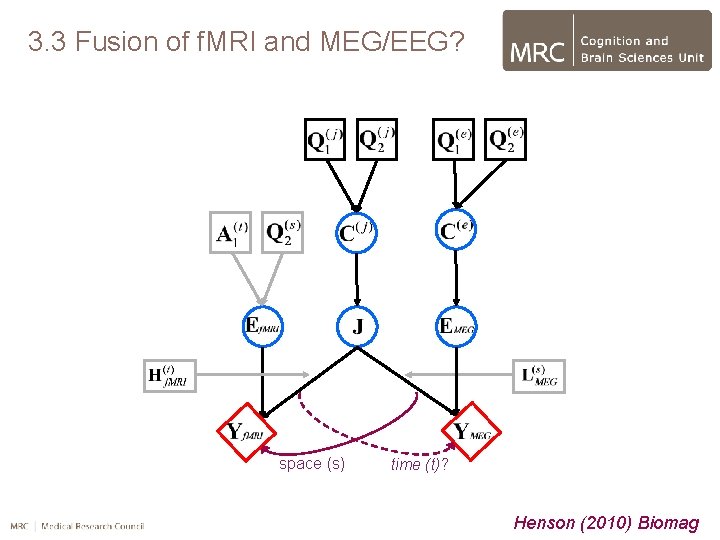
3. 3 Fusion of f. MRI and MEG/EEG? space (s) time (t)? Henson (2010) Biomag
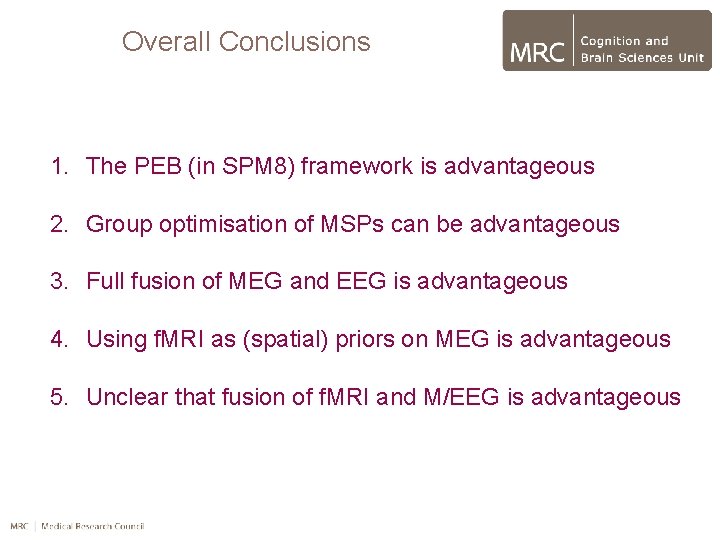
Overall Conclusions 1. The PEB (in SPM 8) framework is advantageous 2. Group optimisation of MSPs can be advantageous 3. Full fusion of MEG and EEG is advantageous 4. Using f. MRI as (spatial) priors on MEG is advantageous 5. Unclear that fusion of f. MRI and M/EEG is advantageous
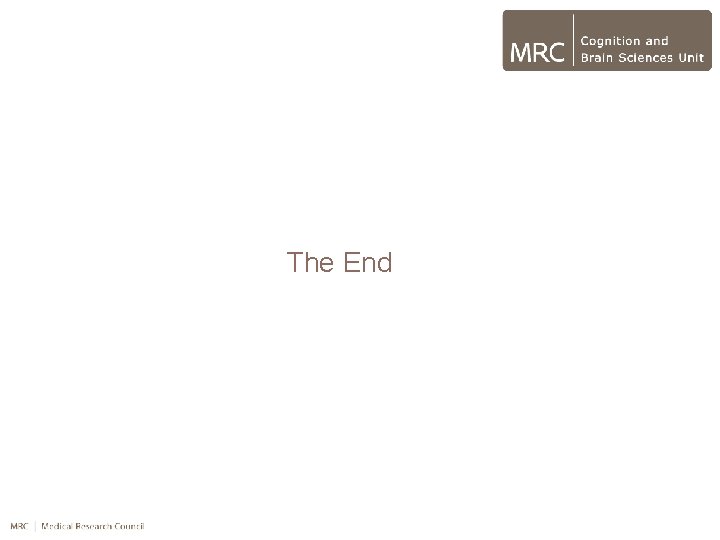
The End
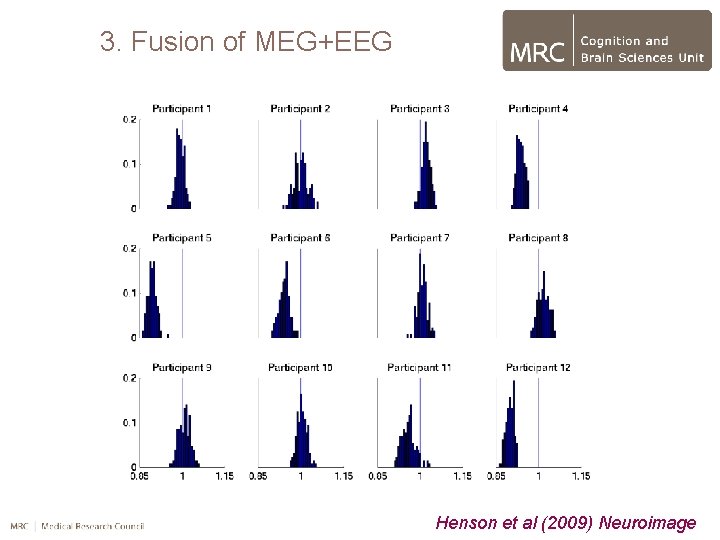
3. Fusion of MEG+EEG Henson et al (2009) Neuroimage
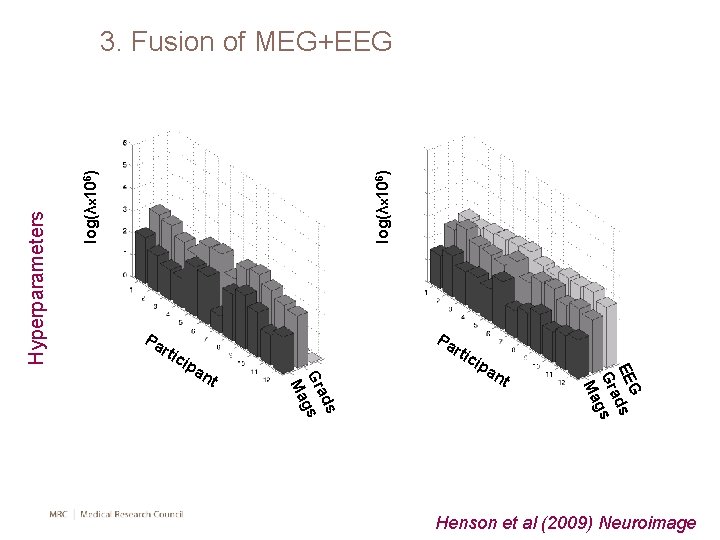
log(λx 106) Hyperparameters 3. Fusion of MEG+EEG Pa rti t cip s ad Gr gs Ma an an t G EE ds a Gr gs Ma cip Pa rti Henson et al (2009) Neuroimage
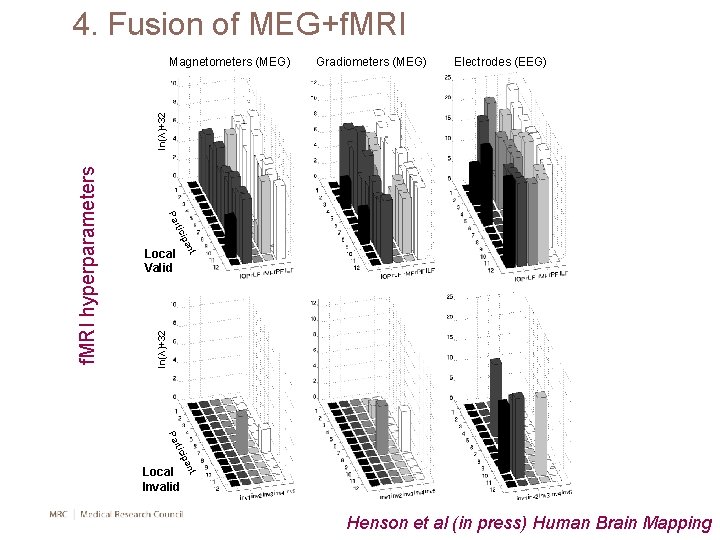
4. Fusion of MEG+f. MRI Gradiometers (MEG) Electrodes (EEG) ln(λ)+32 nt Local Valid ipa rtic Pa f. MRI hyperparameters ln(λ)+32 Magnetometers (MEG) nt ipa rtic Pa Local Invalid Henson et al (in press) Human Brain Mapping
Meeg definition
Do deep generative models know what they don’t know?
Taxonomy of generative models
Generative vs discriminative models
Theis reference
On unifying deep generative models
Modal and semi modal verbs
Generative linguistics and cognitive psychology
What is generative learning aba
Structuralism grammar
Phrase structure rules
Ontologisk realisme
Generative recursion
Deep and surface structure in linguistics
Bentley generative components
Hudson ladder of safety culture
Generative lymphoid organs
Generative thinking boards
Quantum generative adversarial learning
Generative adversarial network
Spectral normalization
Conditional generator
Generative meditation
Advantages of capp
Generative design grasshopper
Generative grammar examples
Nlp generative model
Generative adversarial networks
Generative vs discriminative
Lda generative model
Generative thinking definition
Remedial model of social work
Anova within group and between group
Types of social group
Amino group and carboxyl group
Amino group and carboxyl group
Imt group 2 specialties
Joining together group theory and group skills
Genetic drift
Allegory rhetorical device
Emphatic structure
Negative adverbials examples
Fronting and inversion
Eversion and inversion
Inversion and eversion
Fronting and inversion
Group polarization psychology definition
Y = a(b)^x
Group 2 nitrates thermal stability