Fuzzy Measures and Integrals 1 2 3 4
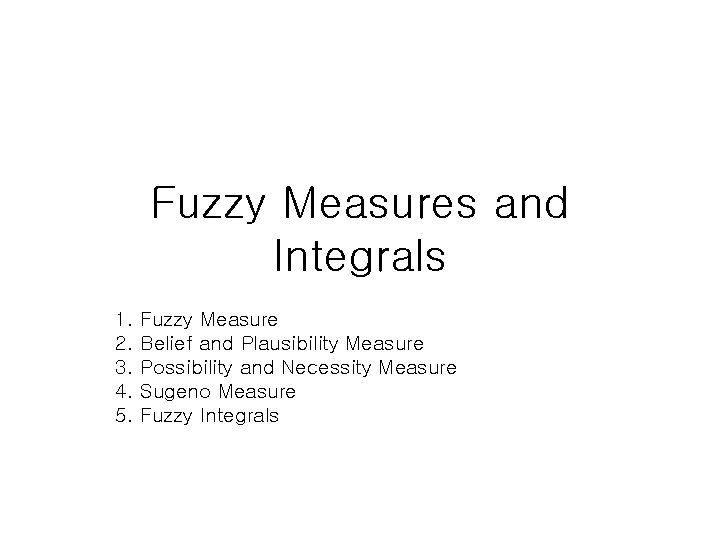
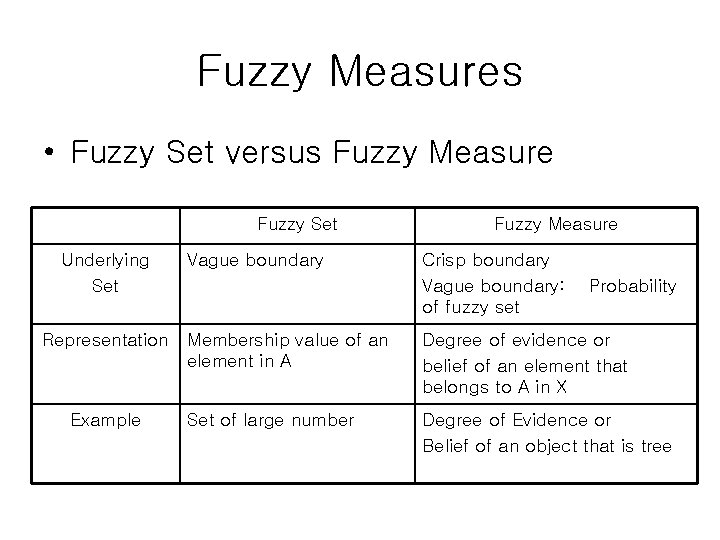
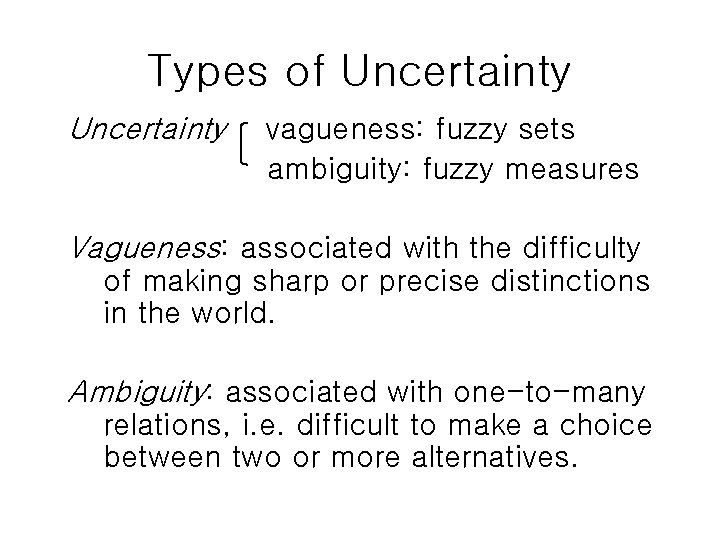
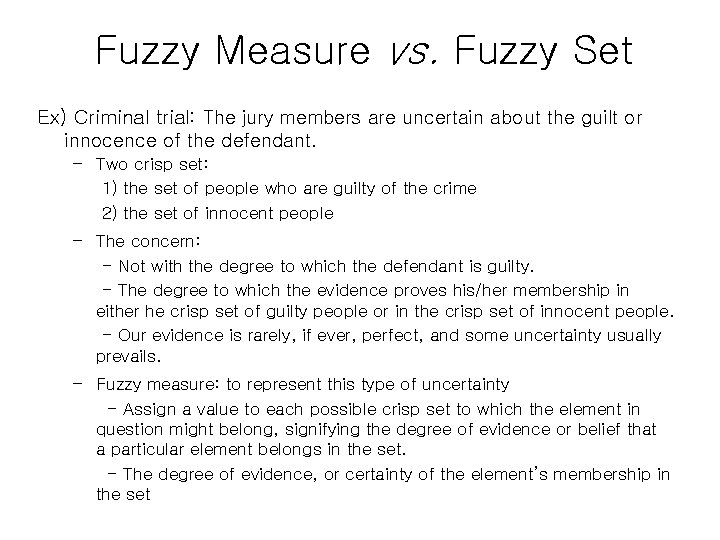
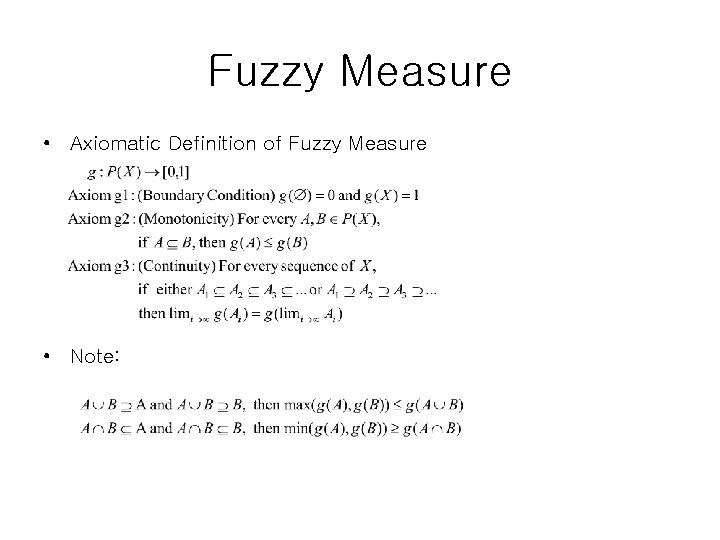
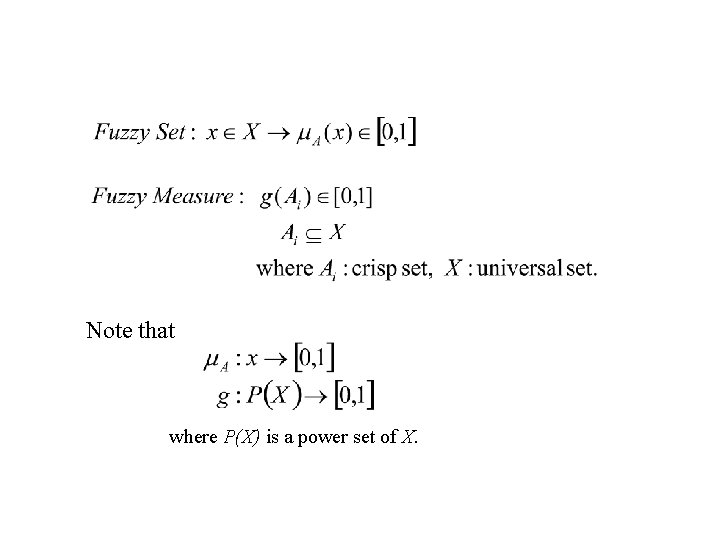
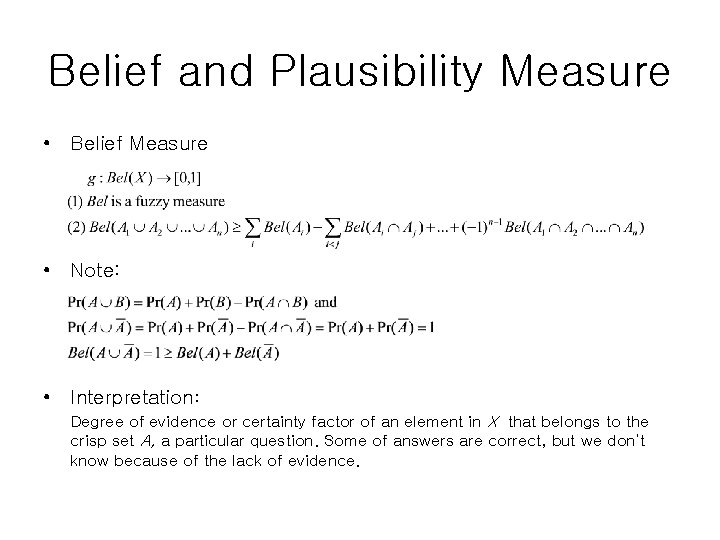
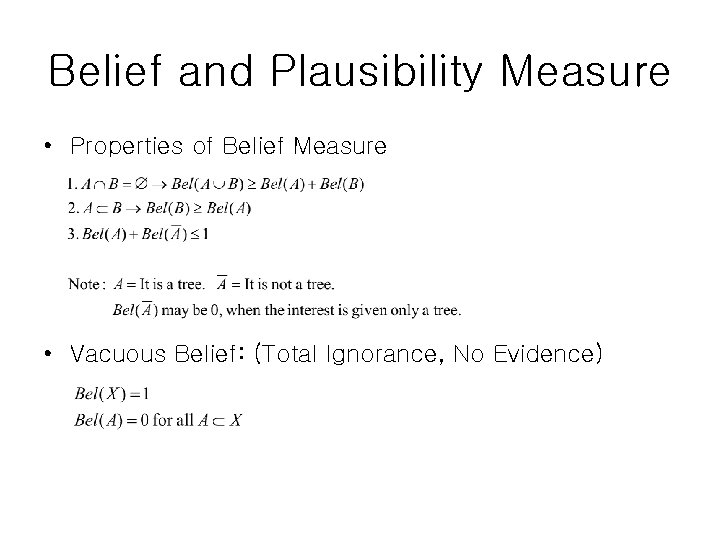
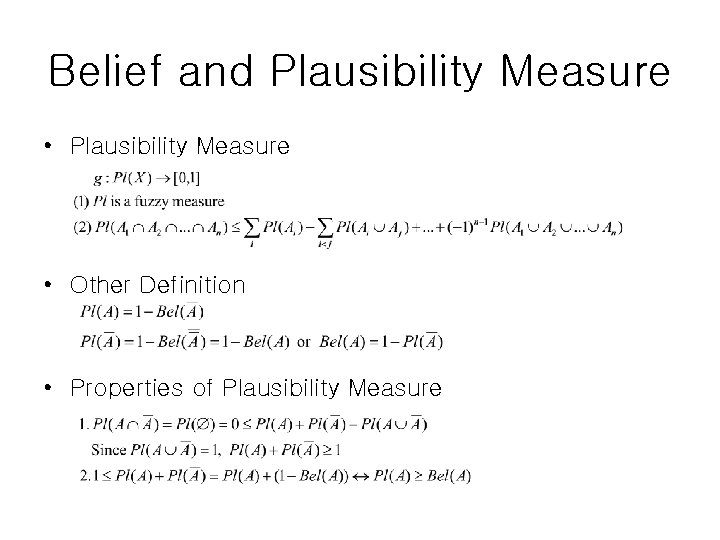
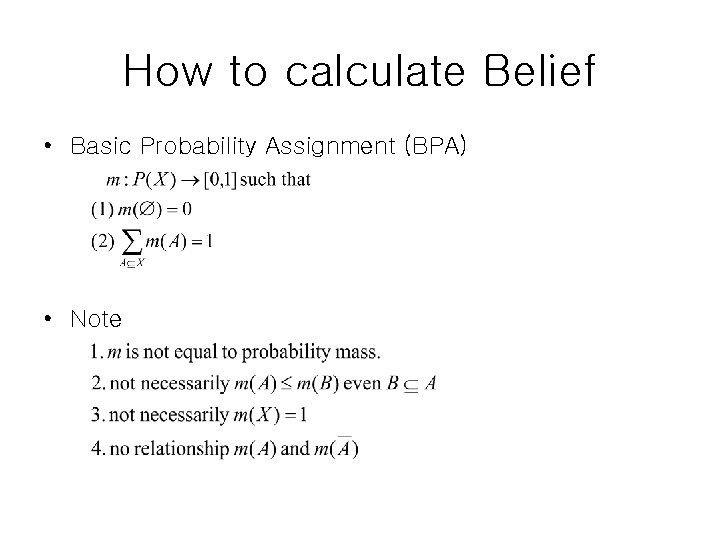
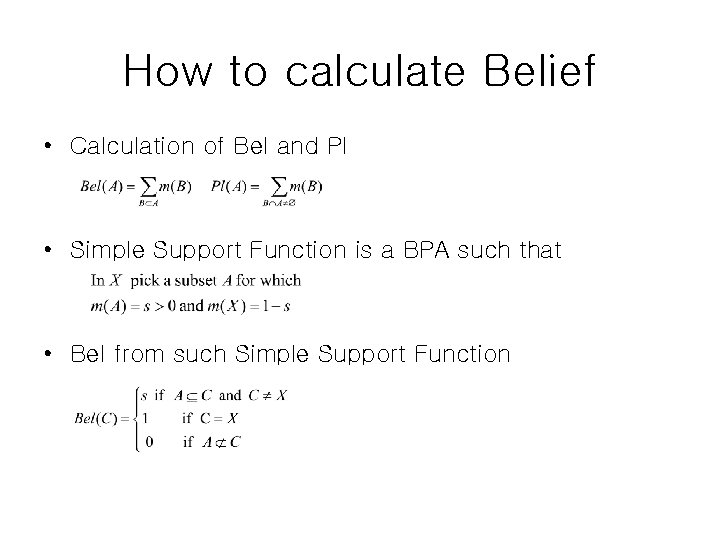
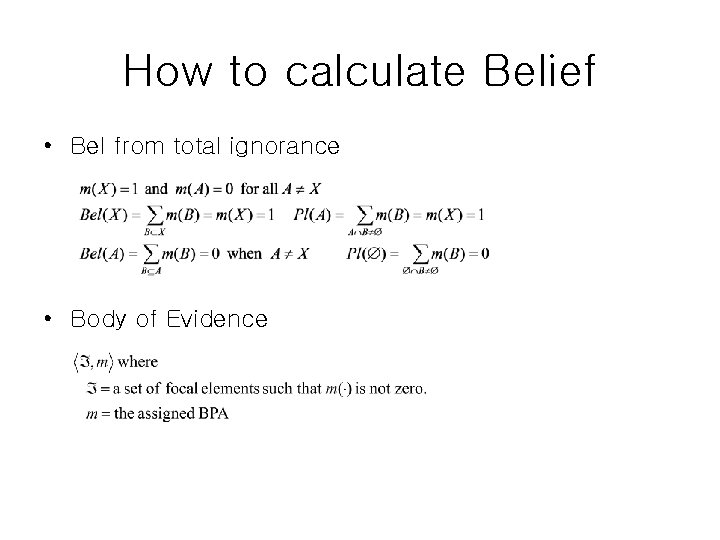
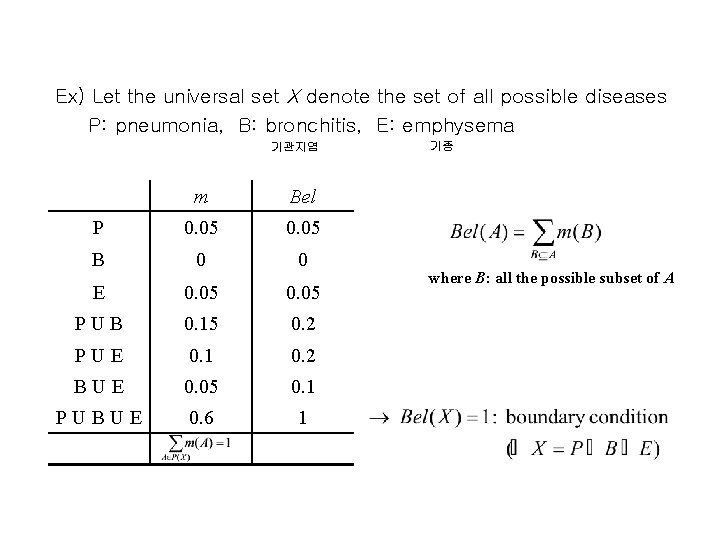
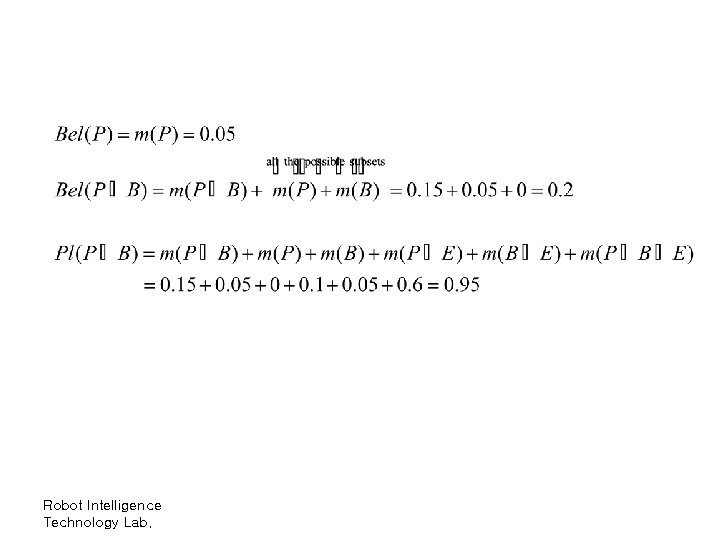
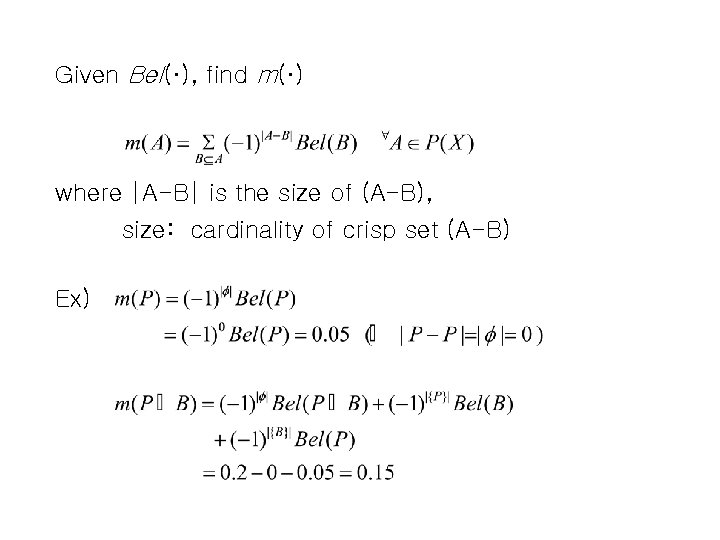
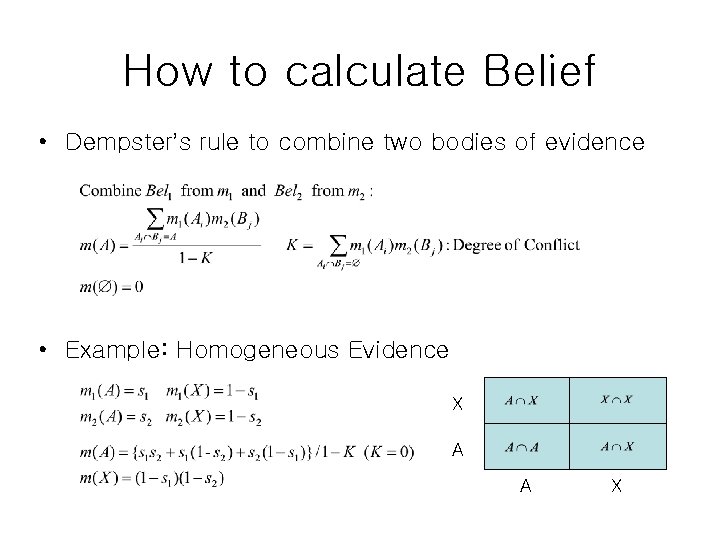
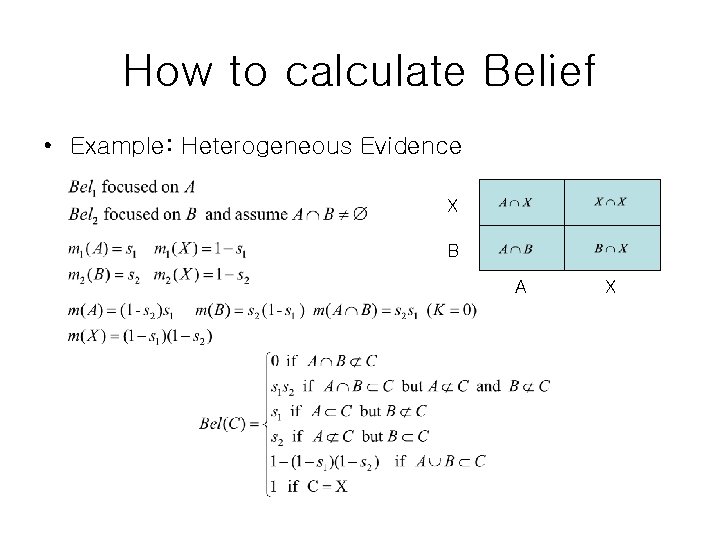
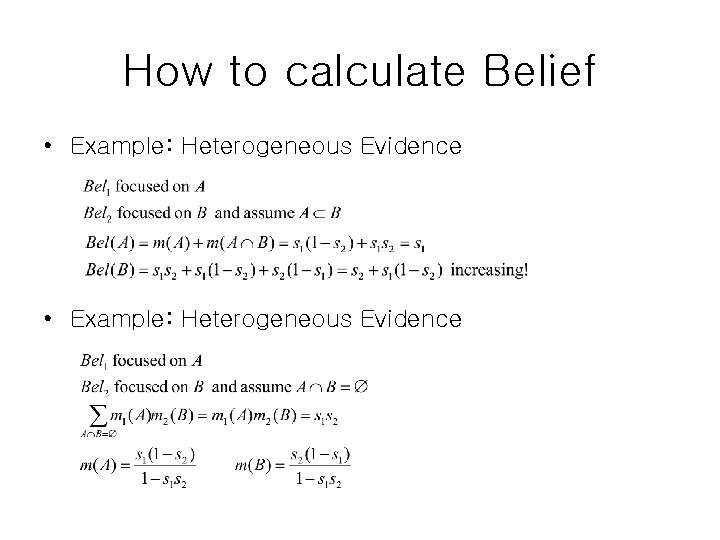
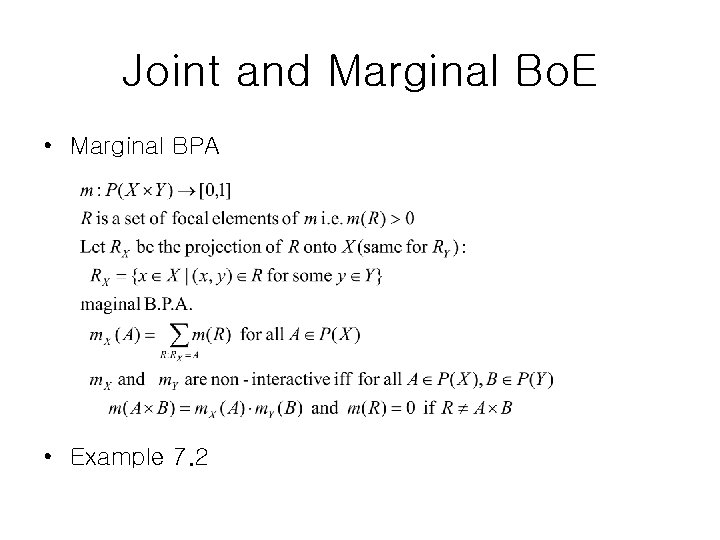
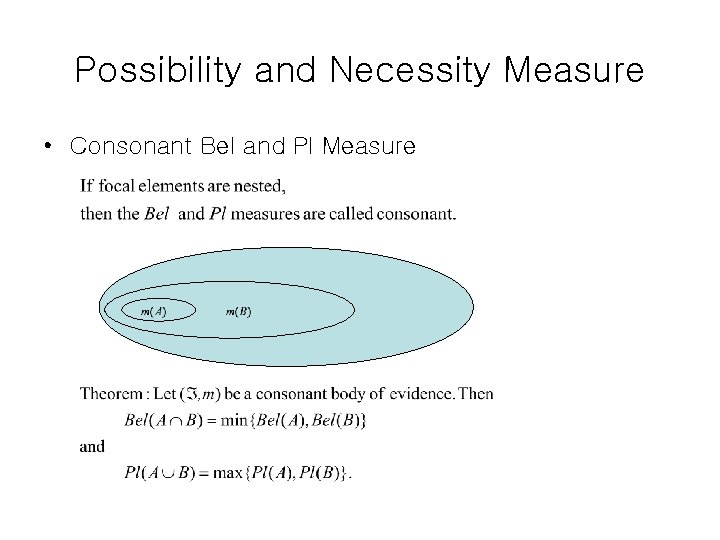
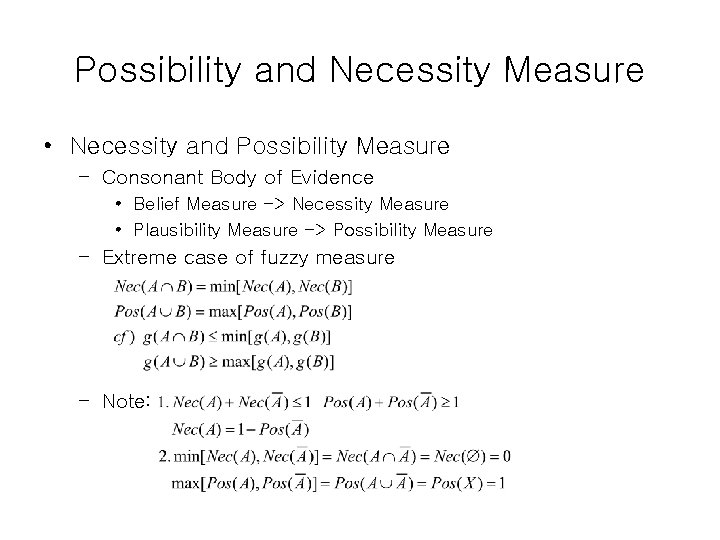
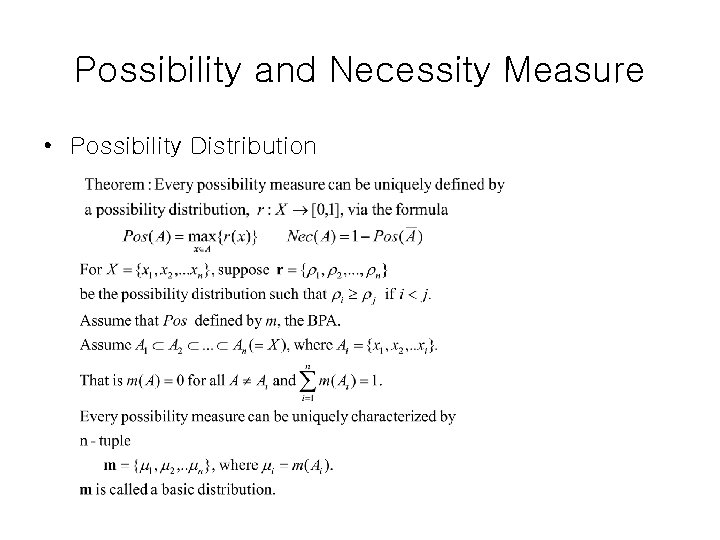
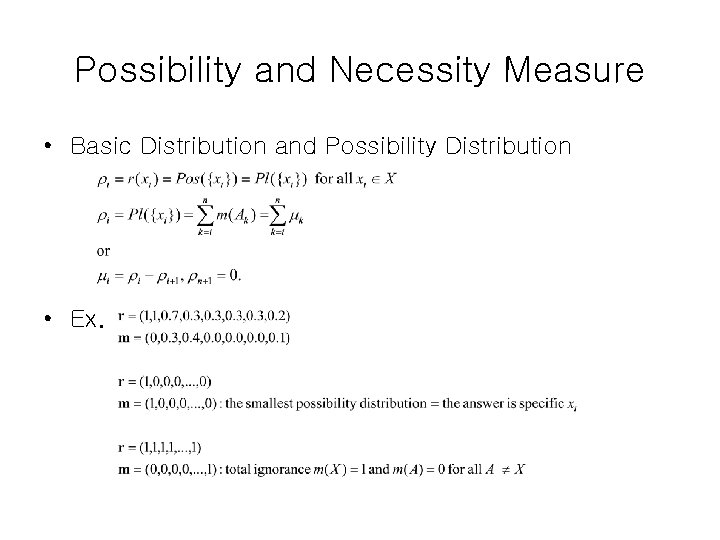
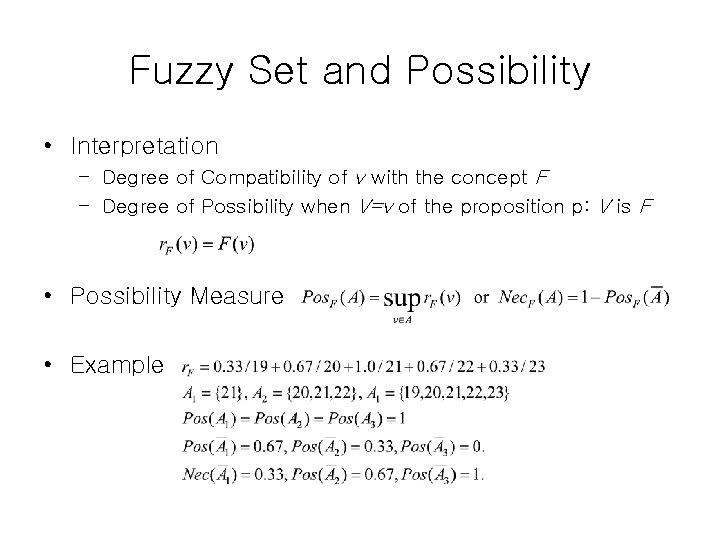
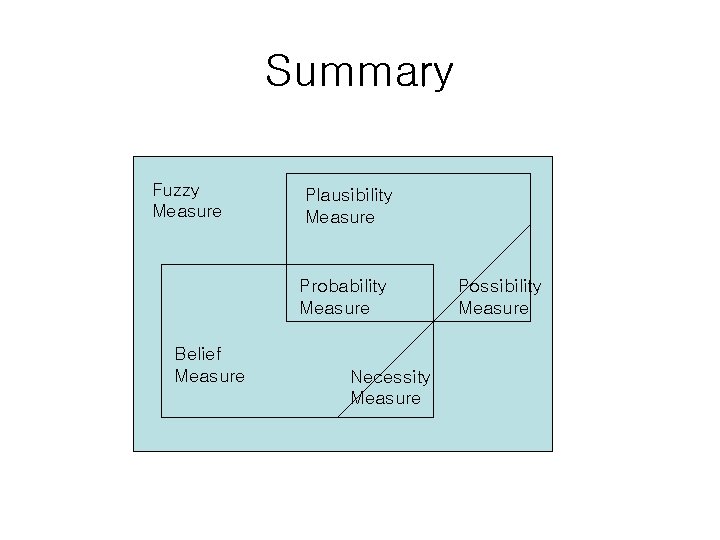
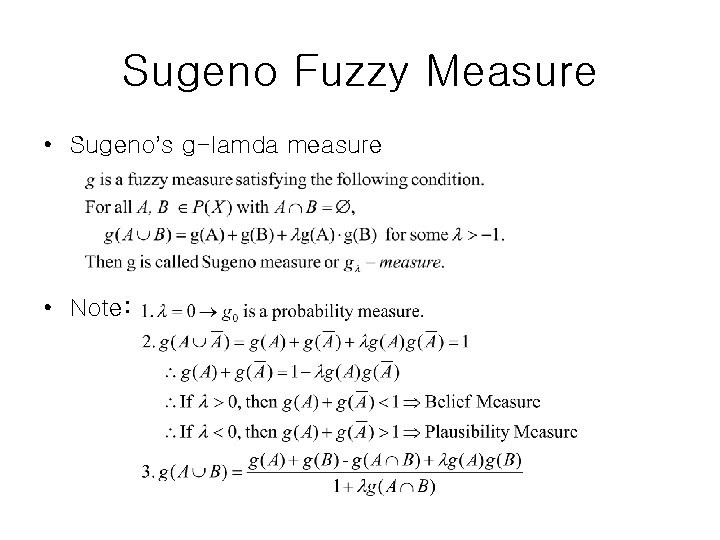
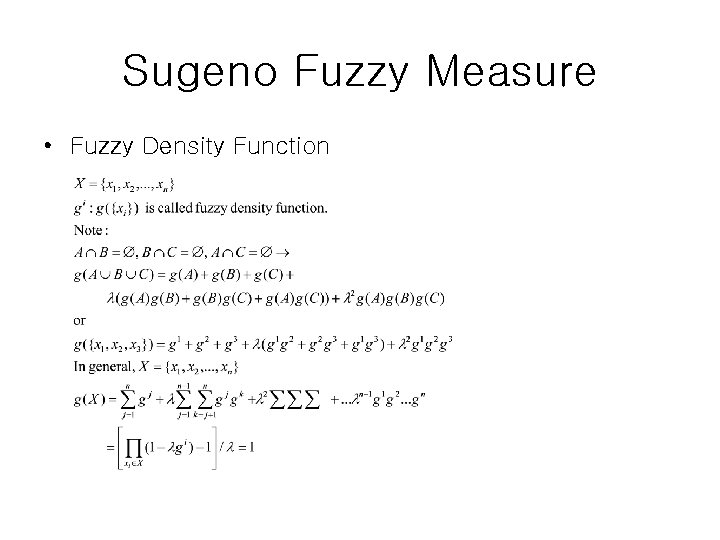
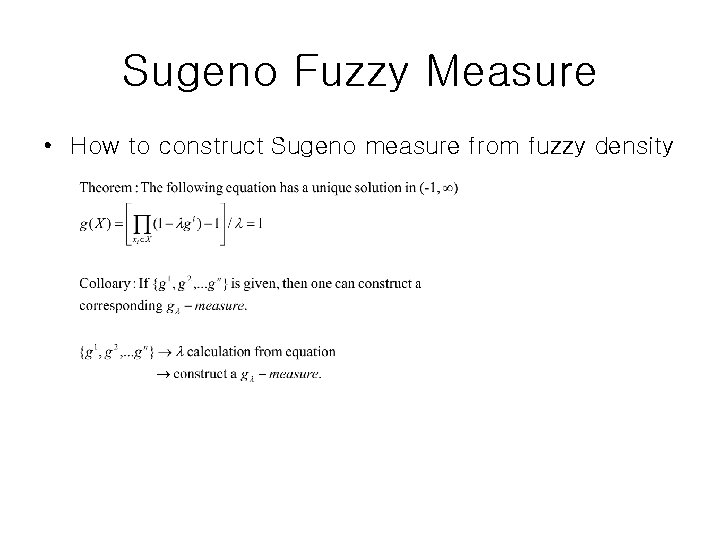
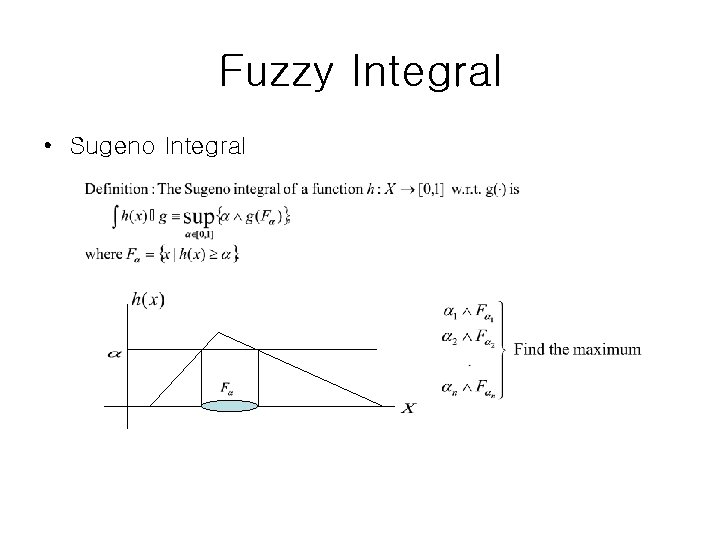
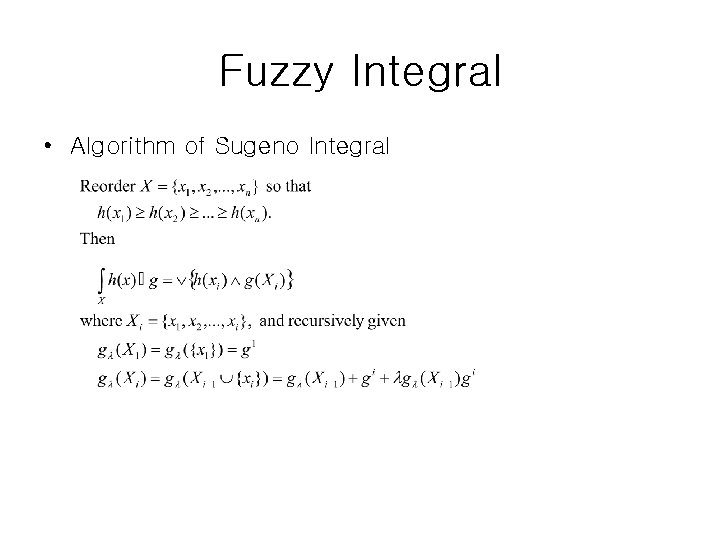
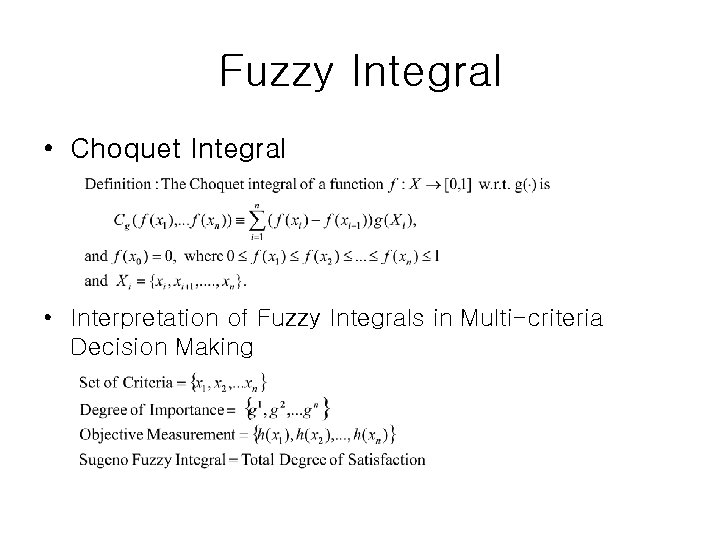
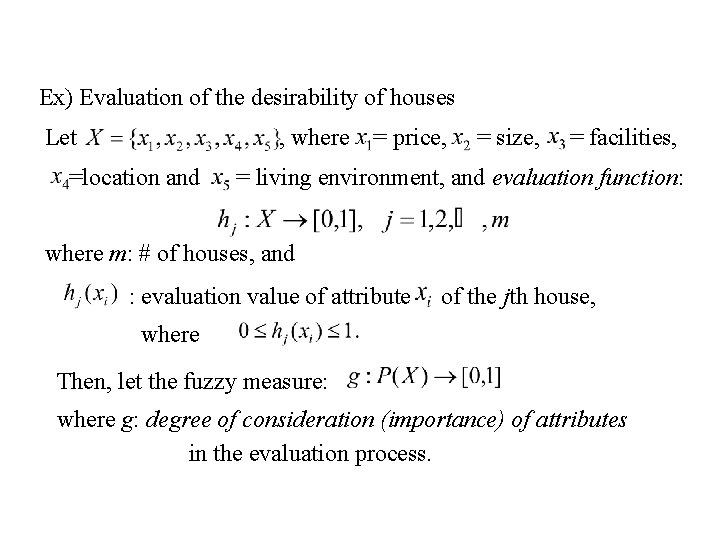
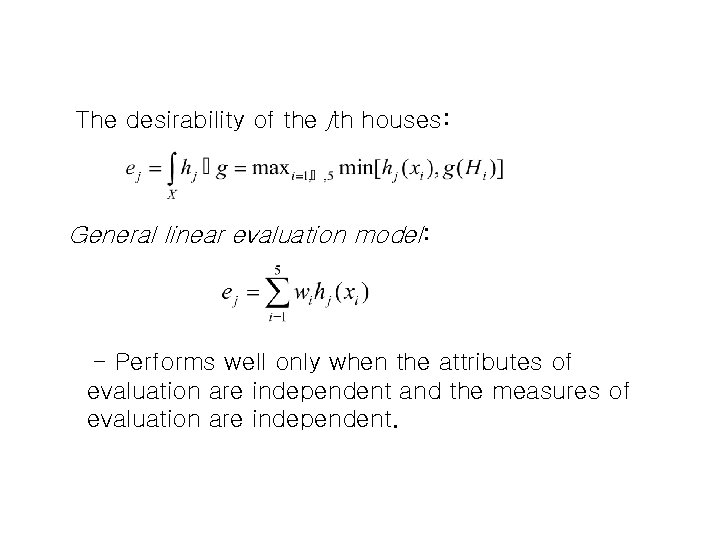
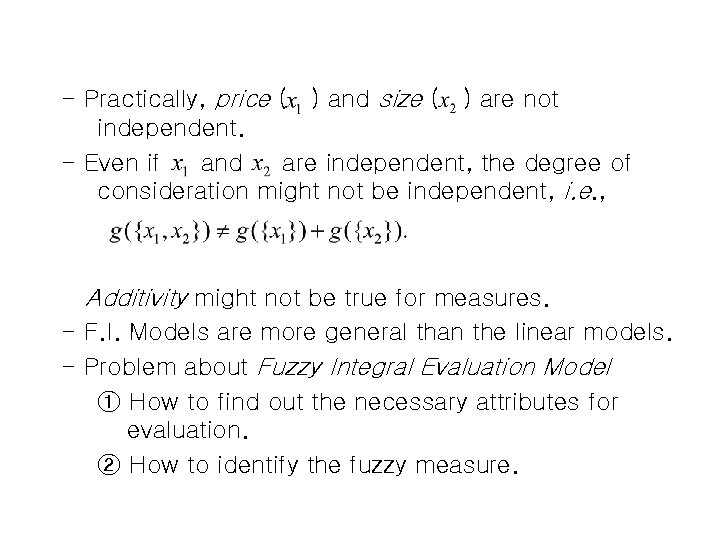
- Slides: 34
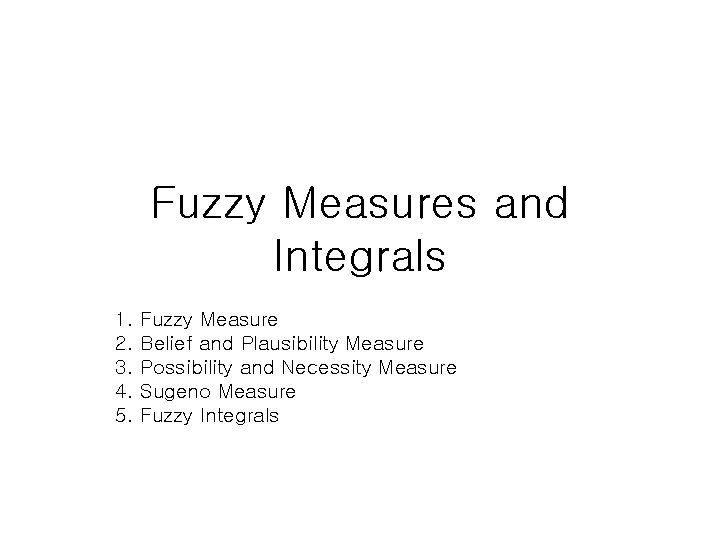
Fuzzy Measures and Integrals 1. 2. 3. 4. 5. Fuzzy Measure Belief and Plausibility Measure Possibility and Necessity Measure Sugeno Measure Fuzzy Integrals
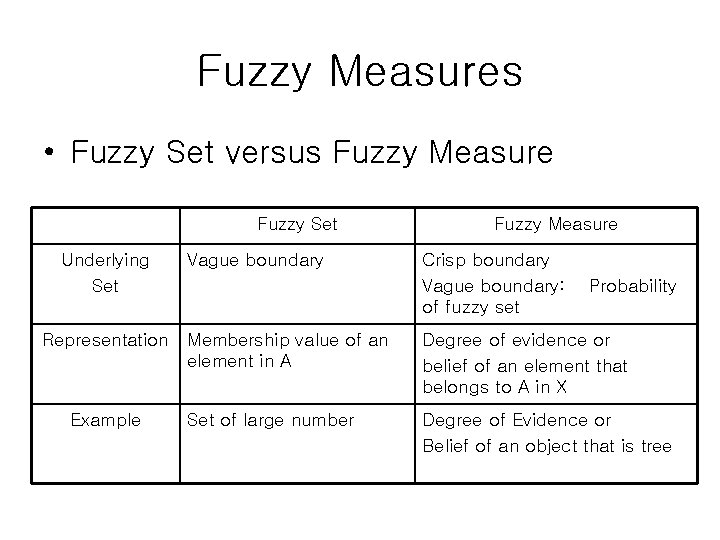
Fuzzy Measures • Fuzzy Set versus Fuzzy Measure Fuzzy Set Underlying Set Representation Example Vague boundary Fuzzy Measure Crisp boundary Vague boundary: of fuzzy set Probability Membership value of an element in A Degree of evidence or belief of an element that belongs to A in X Set of large number Degree of Evidence or Belief of an object that is tree
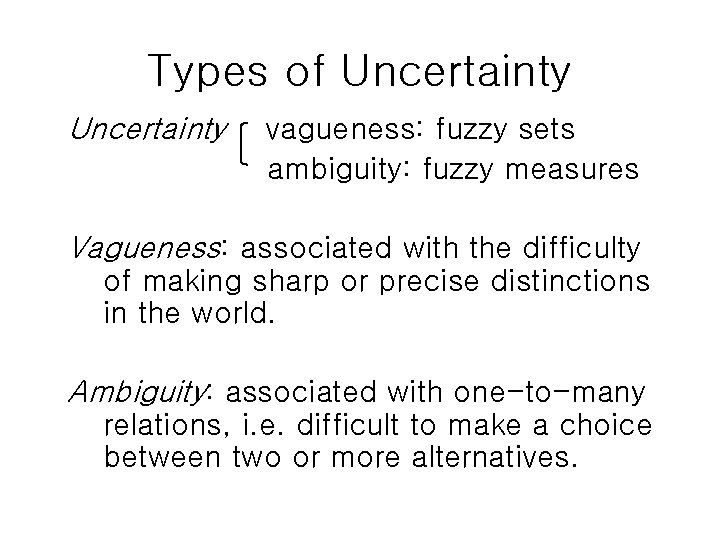
Types of Uncertainty vagueness: fuzzy sets ambiguity: fuzzy measures Vagueness: associated with the difficulty of making sharp or precise distinctions in the world. Ambiguity: associated with one-to-many relations, i. e. difficult to make a choice between two or more alternatives.
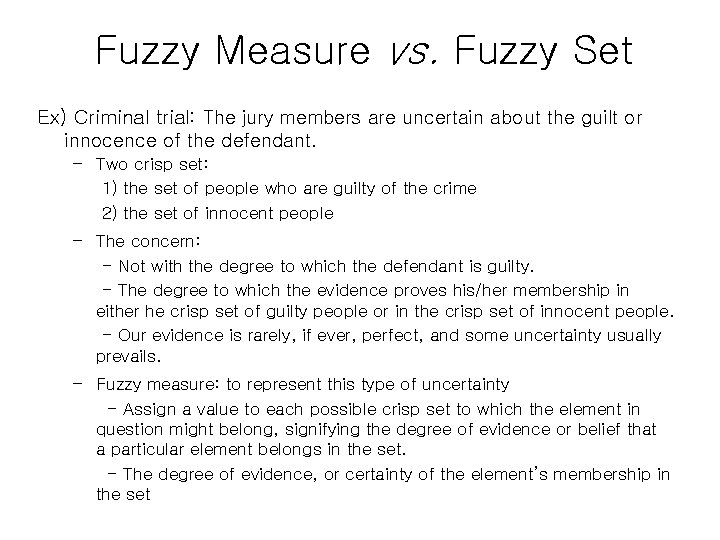
Fuzzy Measure vs. Fuzzy Set Ex) Criminal trial: The jury members are uncertain about the guilt or innocence of the defendant. – Two crisp set: 1) the set of people who are guilty of the crime 2) the set of innocent people – The concern: - Not with the degree to which the defendant is guilty. - The degree to which the evidence proves his/her membership in either he crisp set of guilty people or in the crisp set of innocent people. - Our evidence is rarely, if ever, perfect, and some uncertainty usually prevails. – Fuzzy measure: to represent this type of uncertainty - Assign a value to each possible crisp set to which the element in question might belong, signifying the degree of evidence or belief that a particular element belongs in the set. - The degree of evidence, or certainty of the element’s membership in the set
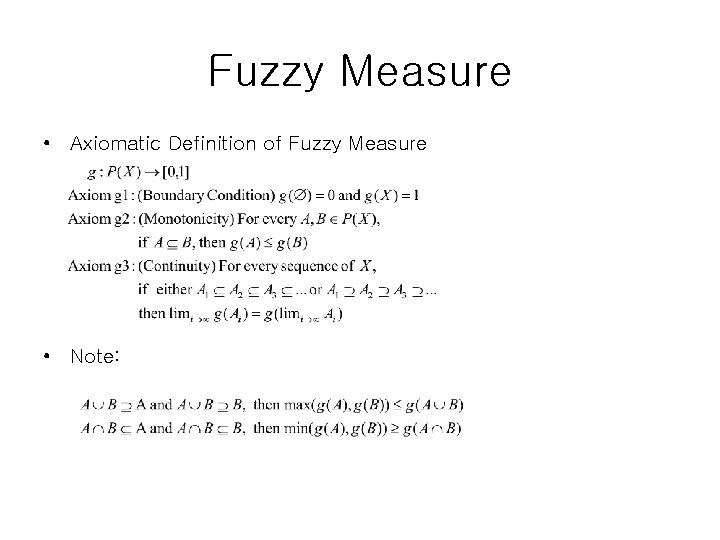
Fuzzy Measure • Axiomatic Definition of Fuzzy Measure • Note:
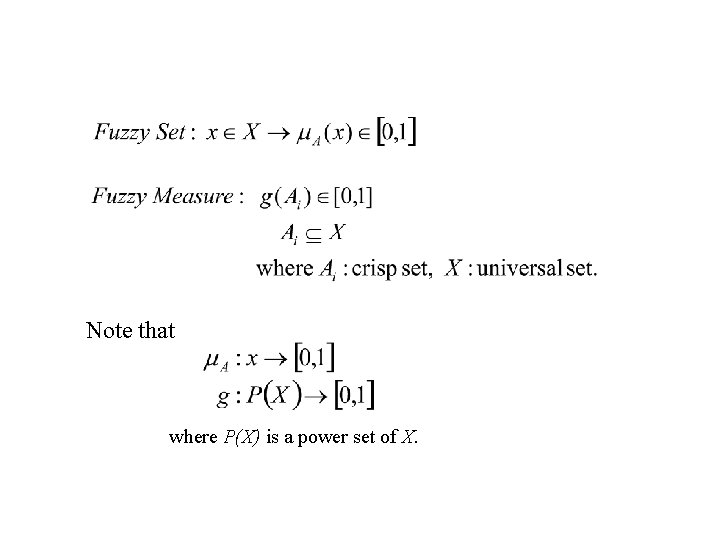
Note that where P(X) is a power set of X.
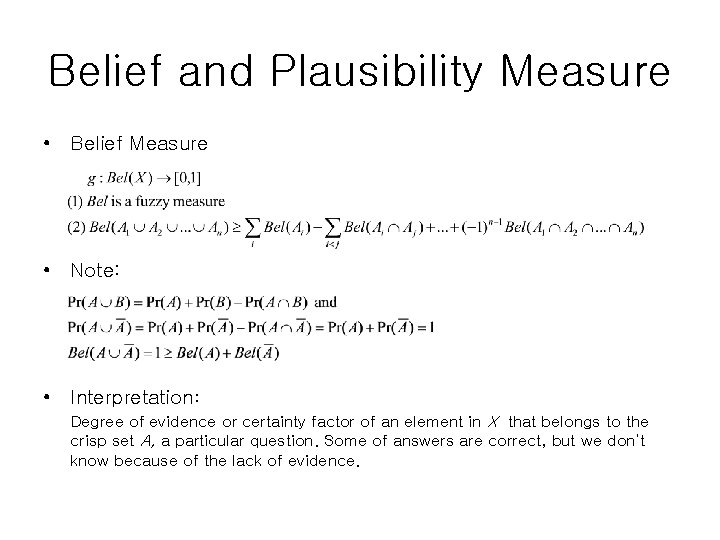
Belief and Plausibility Measure • Belief Measure • Note: • Interpretation: Degree of evidence or certainty factor of an element in X that belongs to the crisp set A, a particular question. Some of answers are correct, but we don’t know because of the lack of evidence.
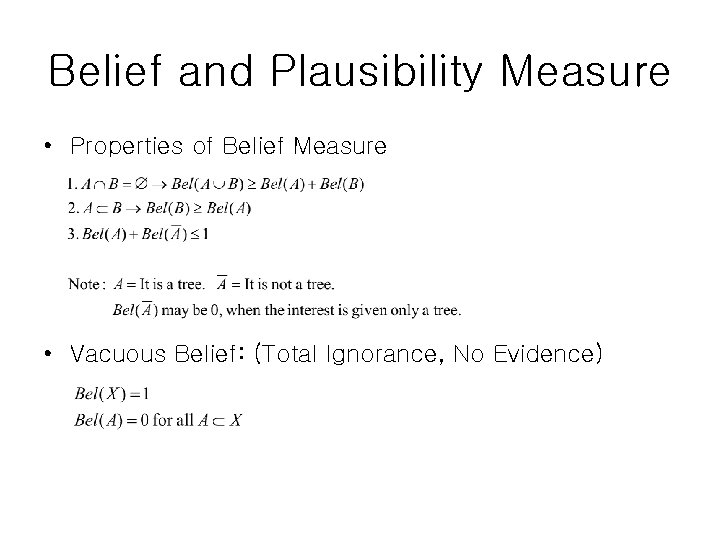
Belief and Plausibility Measure • Properties of Belief Measure • Vacuous Belief: (Total Ignorance, No Evidence)
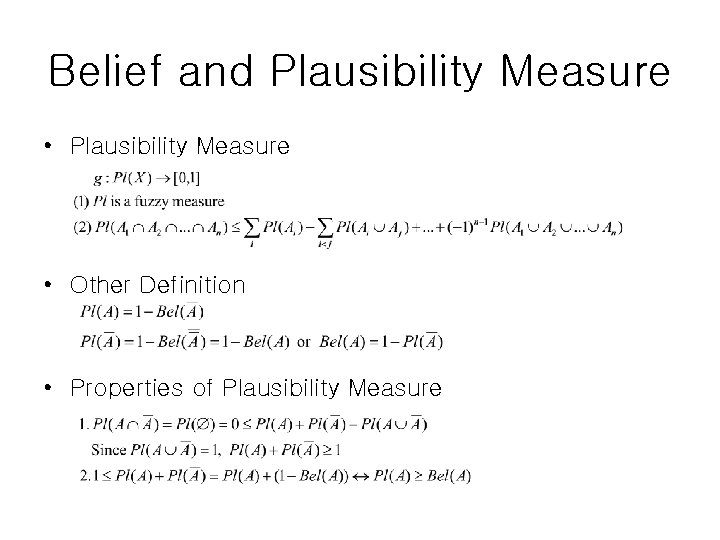
Belief and Plausibility Measure • Plausibility Measure • Other Definition • Properties of Plausibility Measure
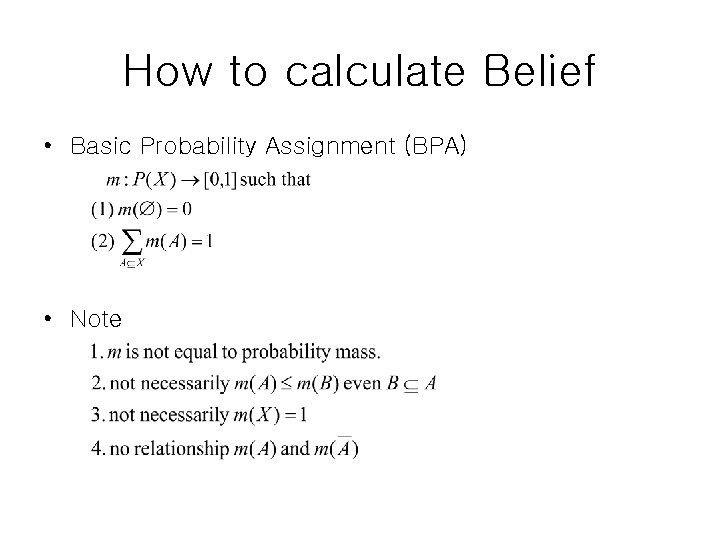
How to calculate Belief • Basic Probability Assignment (BPA) • Note
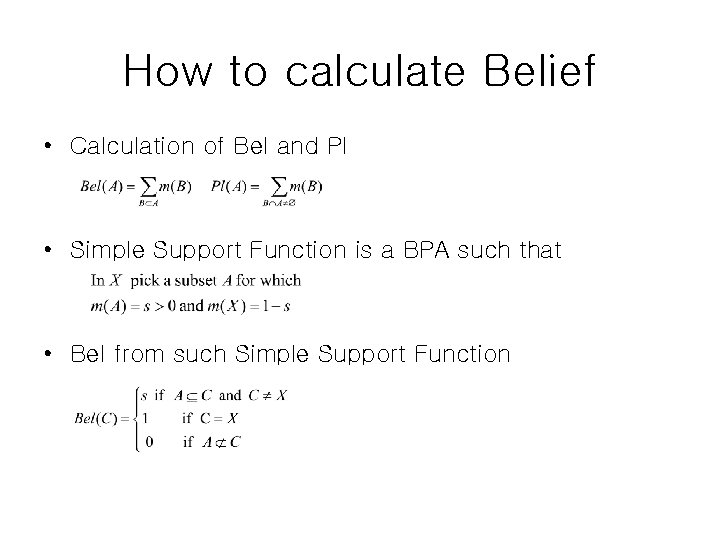
How to calculate Belief • Calculation of Bel and Pl • Simple Support Function is a BPA such that • Bel from such Simple Support Function
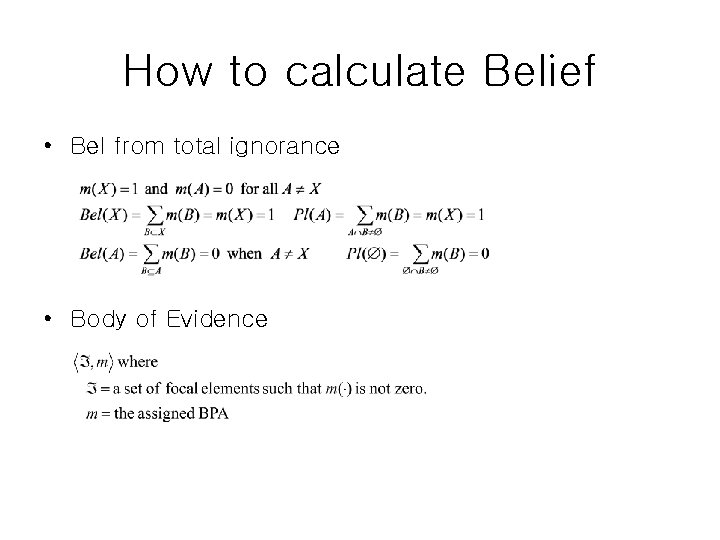
How to calculate Belief • Bel from total ignorance • Body of Evidence
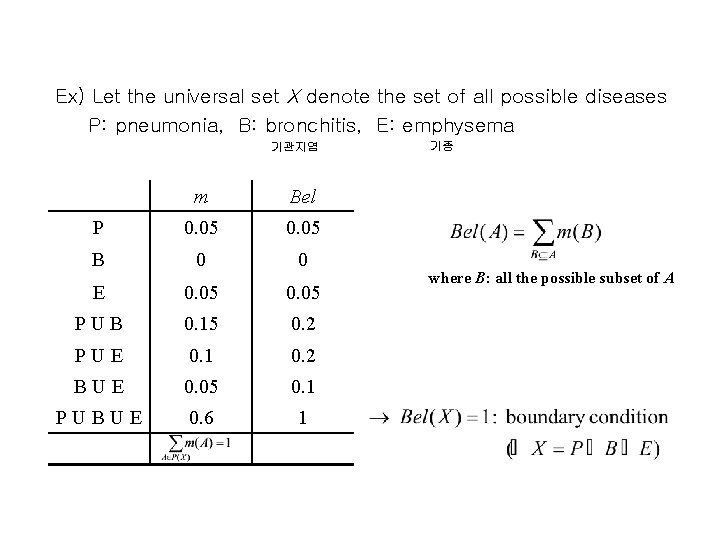
Ex) Let the universal set X denote the set of all possible diseases P: pneumonia, B: bronchitis, E: emphysema 기관지염 m Bel P 0. 05 B 0 0 E 0. 05 PUB 0. 15 0. 2 PUE 0. 1 0. 2 BUE 0. 05 0. 1 PUBUE 0. 6 1 기종 where B: all the possible subset of A
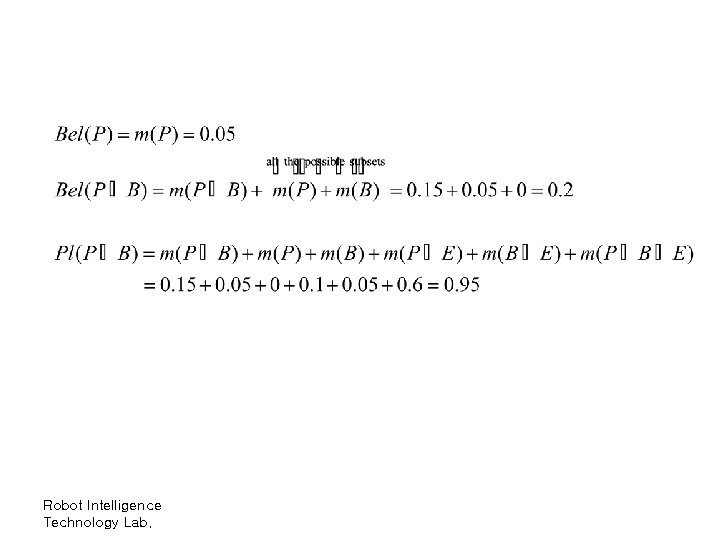
Robot Intelligence Technology Lab.
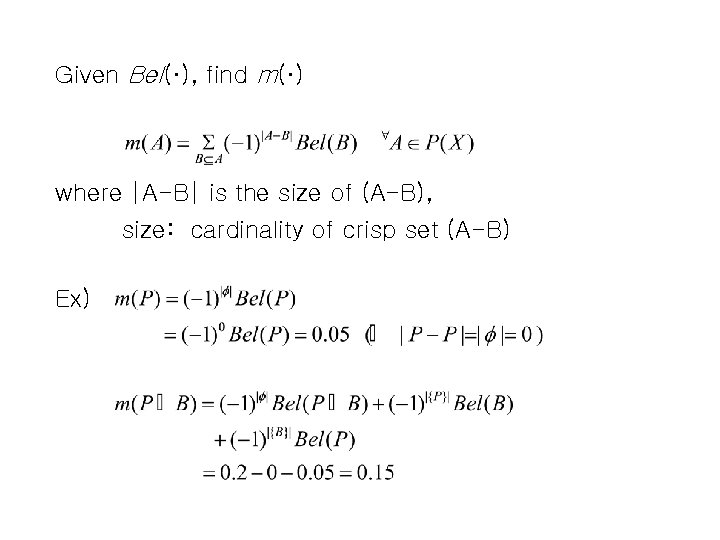
Given Bel(·), find m(·) where |A-B| is the size of (A-B), size: cardinality of crisp set (A-B) Ex)
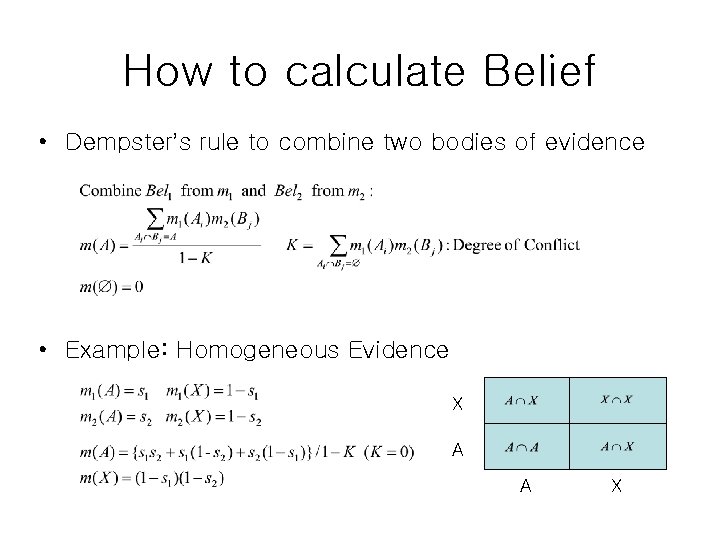
How to calculate Belief • Dempster’s rule to combine two bodies of evidence • Example: Homogeneous Evidence X A A X
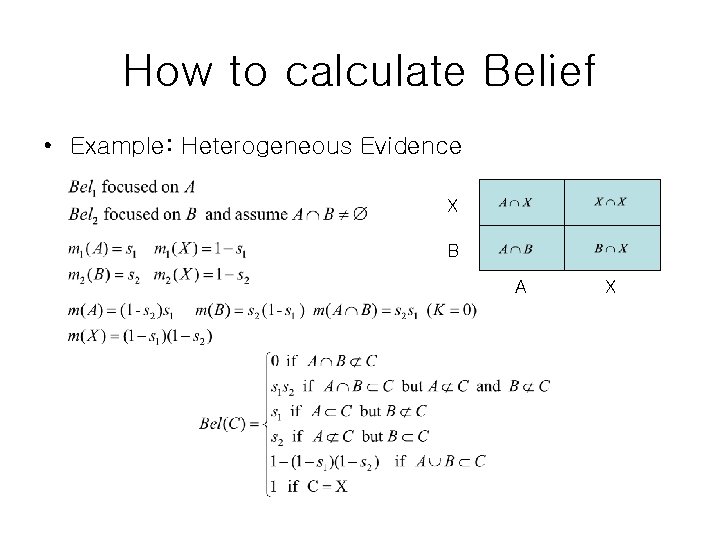
How to calculate Belief • Example: Heterogeneous Evidence X B A X
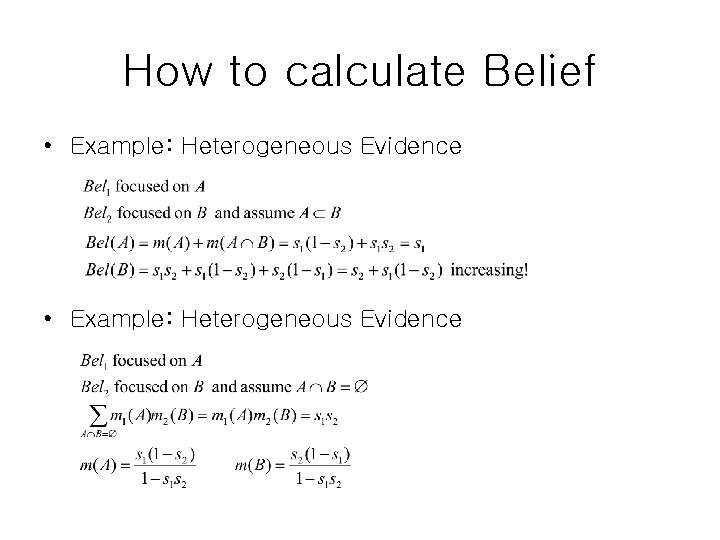
How to calculate Belief • Example: Heterogeneous Evidence
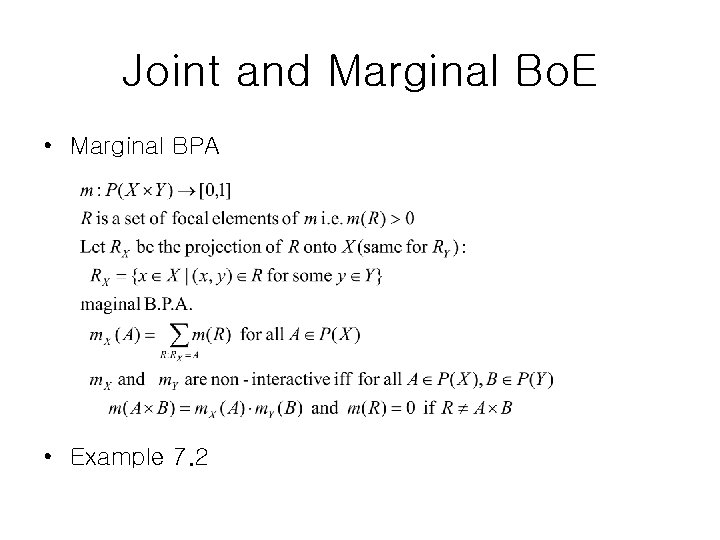
Joint and Marginal Bo. E • Marginal BPA • Example 7. 2
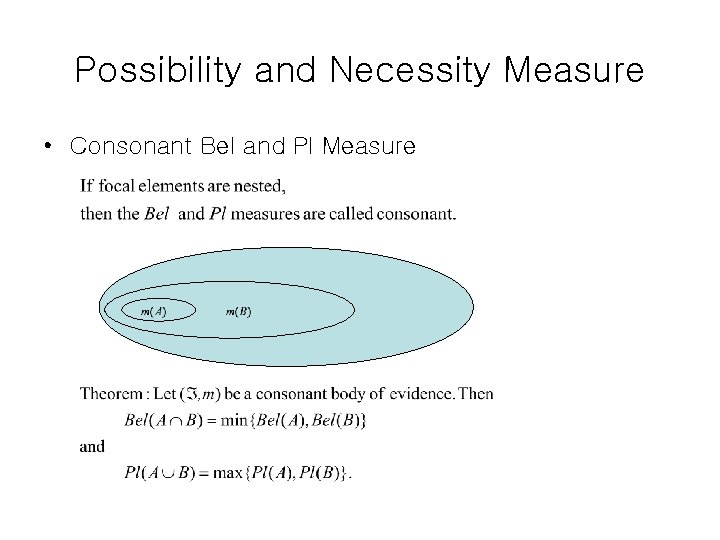
Possibility and Necessity Measure • Consonant Bel and Pl Measure
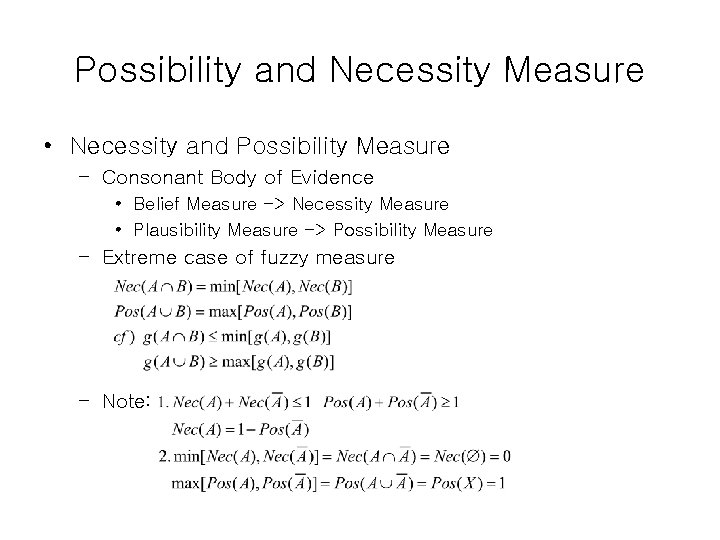
Possibility and Necessity Measure • Necessity and Possibility Measure – Consonant Body of Evidence • Belief Measure -> Necessity Measure • Plausibility Measure -> Possibility Measure – Extreme case of fuzzy measure – Note:
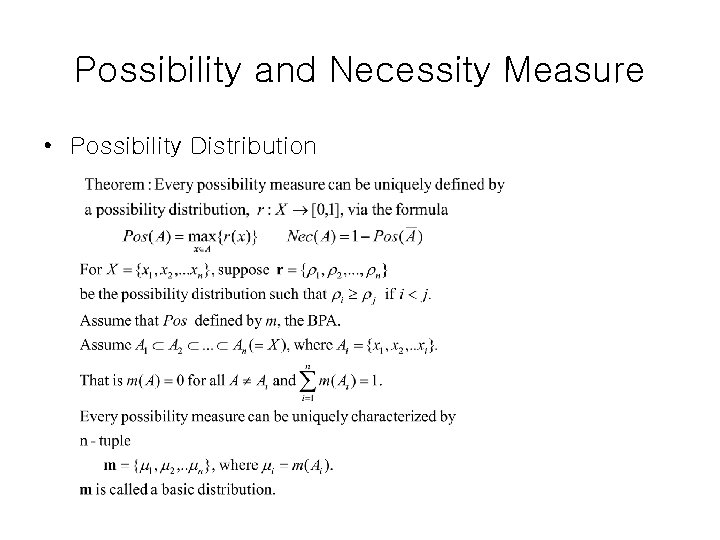
Possibility and Necessity Measure • Possibility Distribution
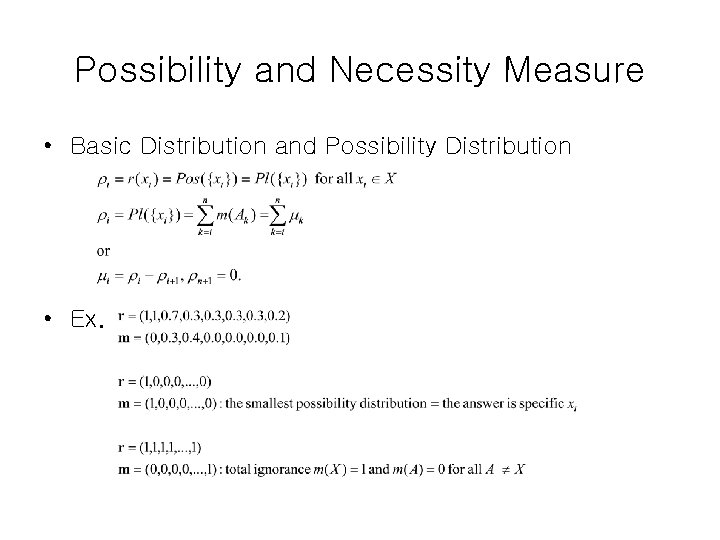
Possibility and Necessity Measure • Basic Distribution and Possibility Distribution • Ex.
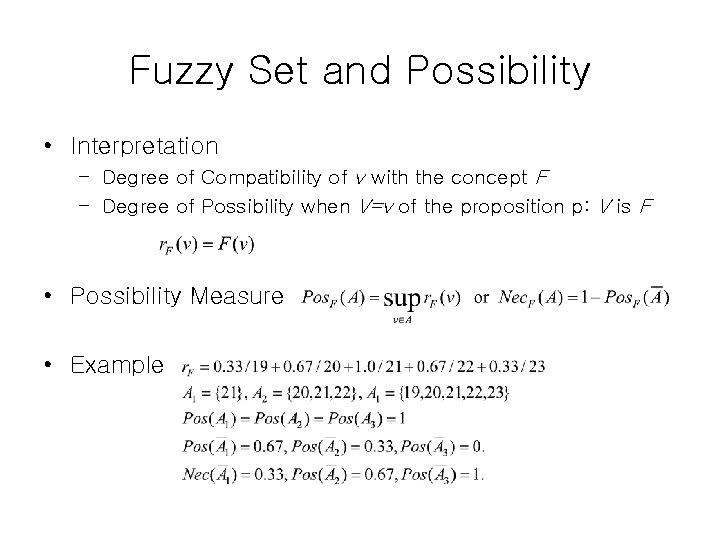
Fuzzy Set and Possibility • Interpretation – Degree of Compatibility of v with the concept F – Degree of Possibility when V=v of the proposition p: V is F • Possibility Measure • Example
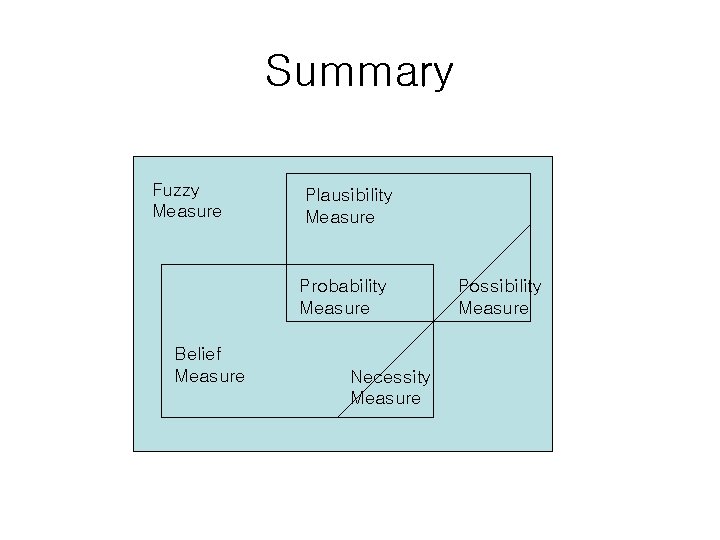
Summary Fuzzy Measure Plausibility Measure Probability Measure Belief Measure Necessity Measure Possibility Measure
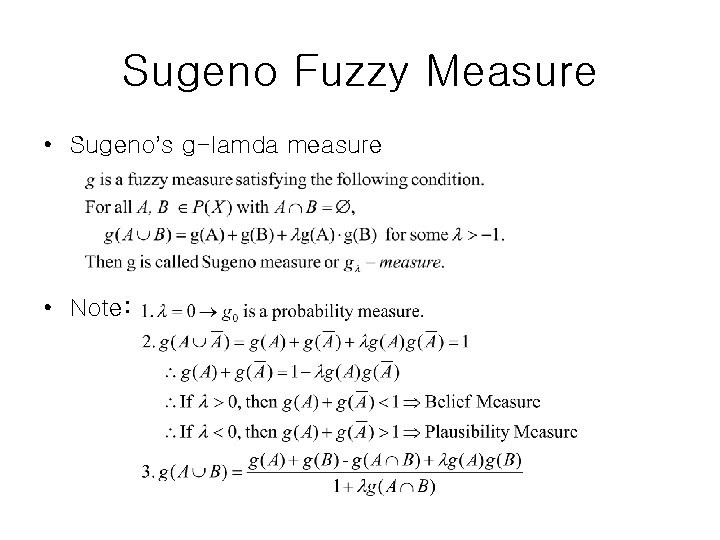
Sugeno Fuzzy Measure • Sugeno’s g-lamda measure • Note:
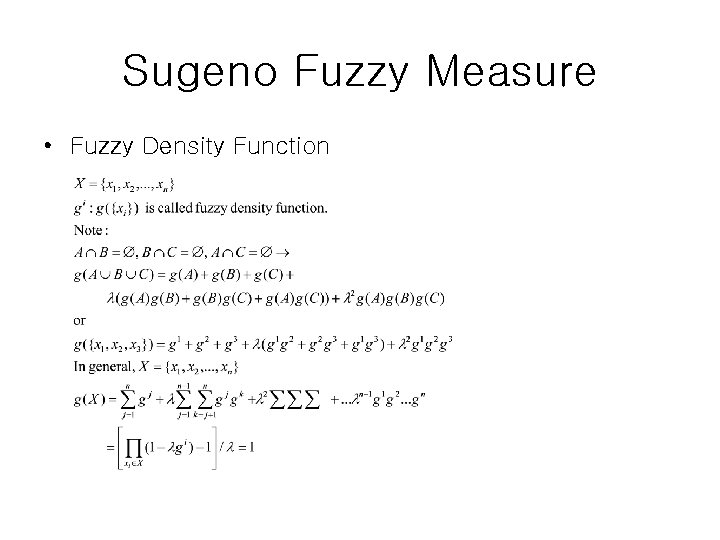
Sugeno Fuzzy Measure • Fuzzy Density Function
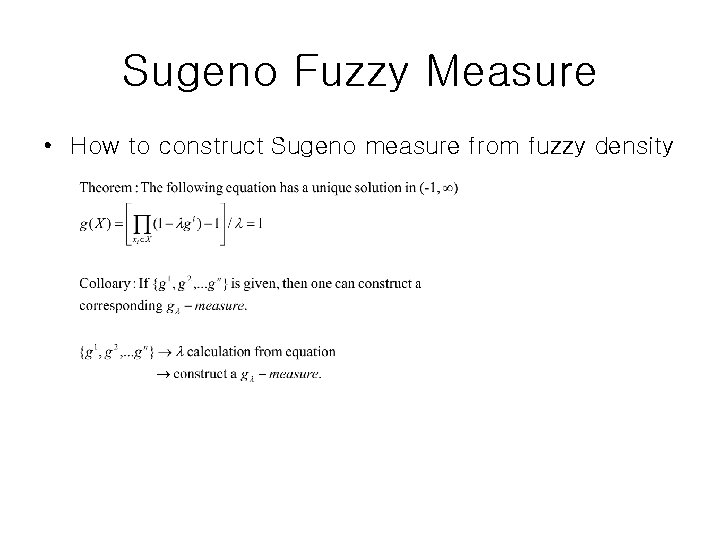
Sugeno Fuzzy Measure • How to construct Sugeno measure from fuzzy density
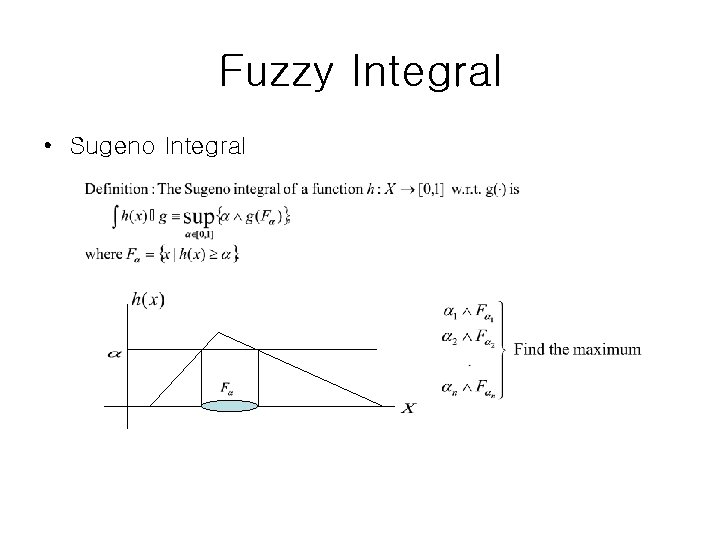
Fuzzy Integral • Sugeno Integral
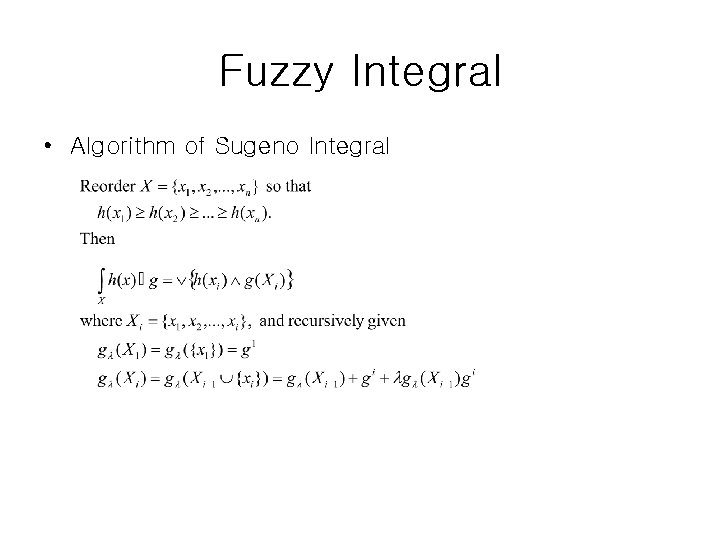
Fuzzy Integral • Algorithm of Sugeno Integral
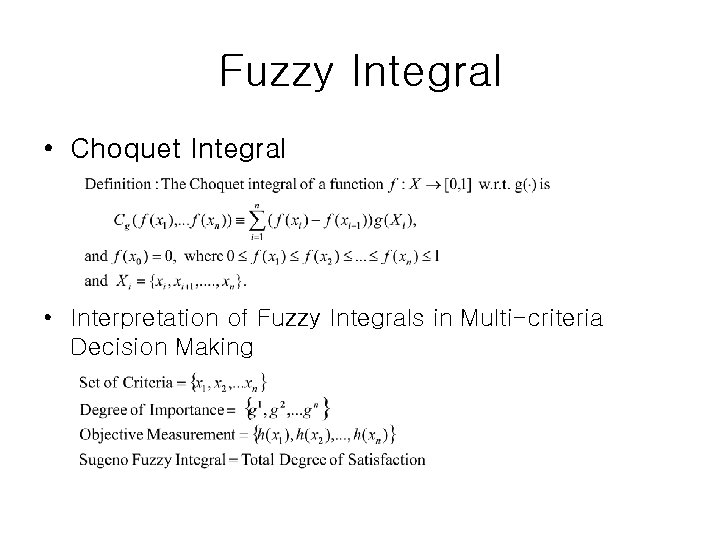
Fuzzy Integral • Choquet Integral • Interpretation of Fuzzy Integrals in Multi-criteria Decision Making
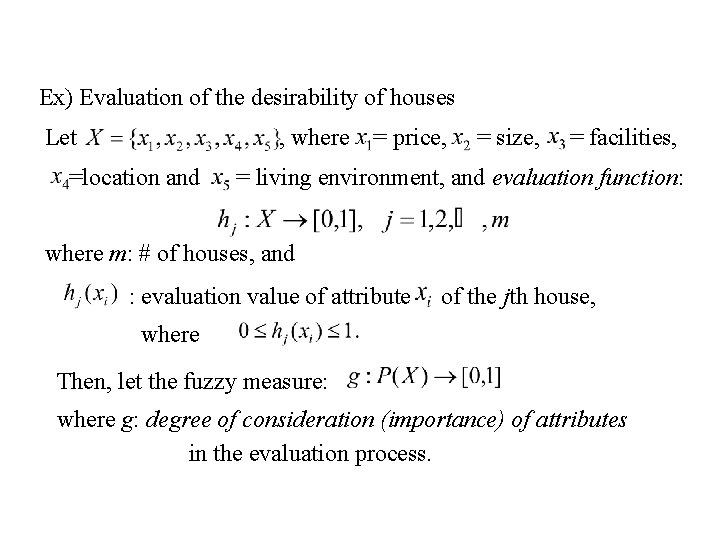
Ex) Evaluation of the desirability of houses Let , where =location and = price, = size, = facilities, = living environment, and evaluation function: where m: # of houses, and : evaluation value of attribute of the jth house, where Then, let the fuzzy measure: where g: degree of consideration (importance) of attributes in the evaluation process.
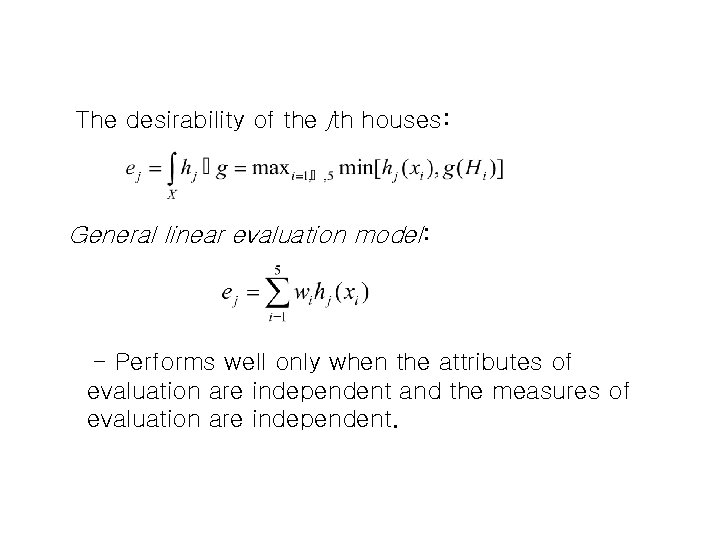
The desirability of the jth houses: General linear evaluation model: - Performs well only when the attributes of evaluation are independent and the measures of evaluation are independent.
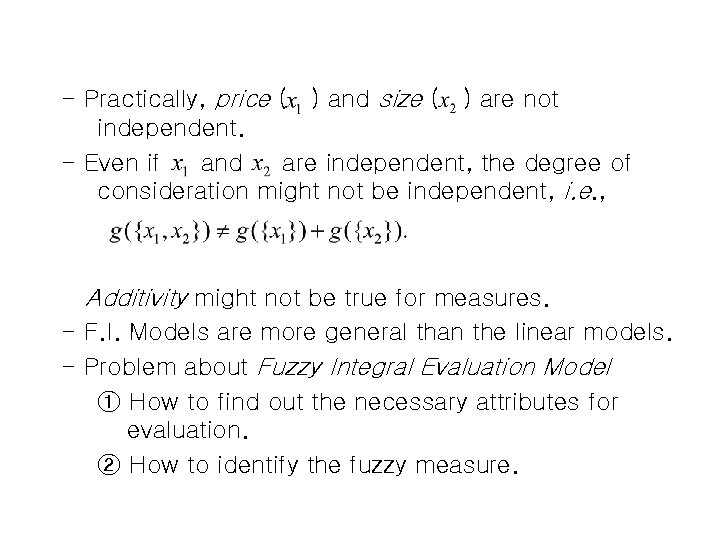
- Practically, price ( ) and size ( ) are not independent. - Even if and are independent, the degree of consideration might not be independent, i. e. , Additivity might not be true for measures. - F. I. Models are more general than the linear models. - Problem about Fuzzy Integral Evaluation Model ① How to find out the necessary attributes for evaluation. ② How to identify the fuzzy measure.
Image sets
Belief and plausibility measures in fuzzy
Repeated-measures design
Radiation integrals and auxiliary potential functions
Fourier series and integrals
Integrals involving powers of secant and tangent
Substitution rule
Calculus theorems
Riemann sum sigma notation calculator
Symbol for convolution
Sec 7
Indefinite integral and definite integral
Properties of indefinite integrals
Convolution sum and convolution integral
Integral trig identities
Rule for integration
Integral garis dan integral permukaan
Slidetodoc. com
Improper integrals
Rate of change examples
Maurits haverkort
Application of residue theorem to evaluate real integrals
Calculus chapter 5 integrals
General power rule
Application of residue theorem to evaluate real integrals
Konsep integral
Chain rule integration
Additive interval property
Double integral polar coordinates
Change of variables multiple integrals
Is integral the area under a curve
Fubini's theorem
Product rule in integration
Hard trig integrals
Double integrals over rectangles