Factorial design II III Factorial ANOVA Week 7
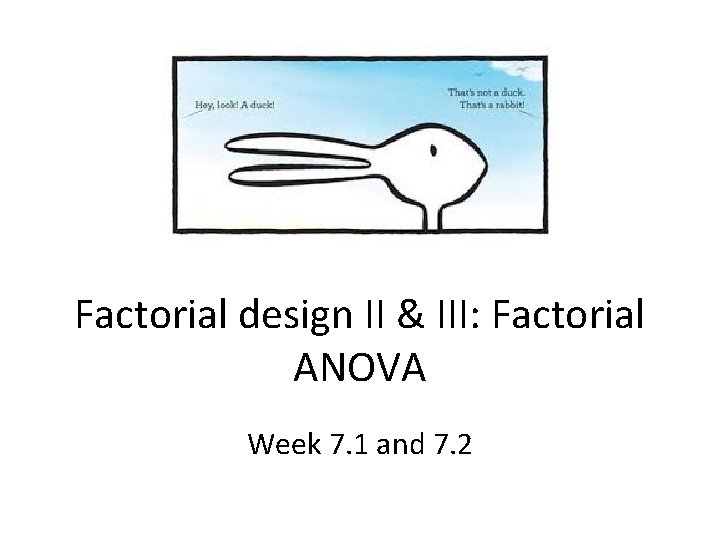
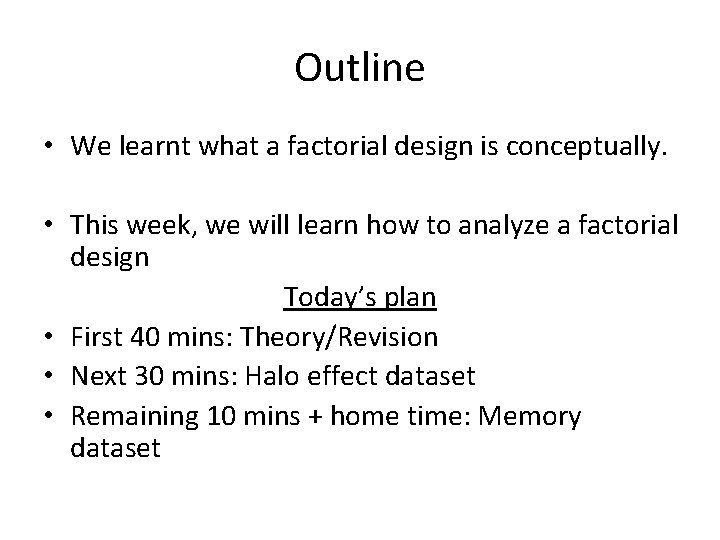
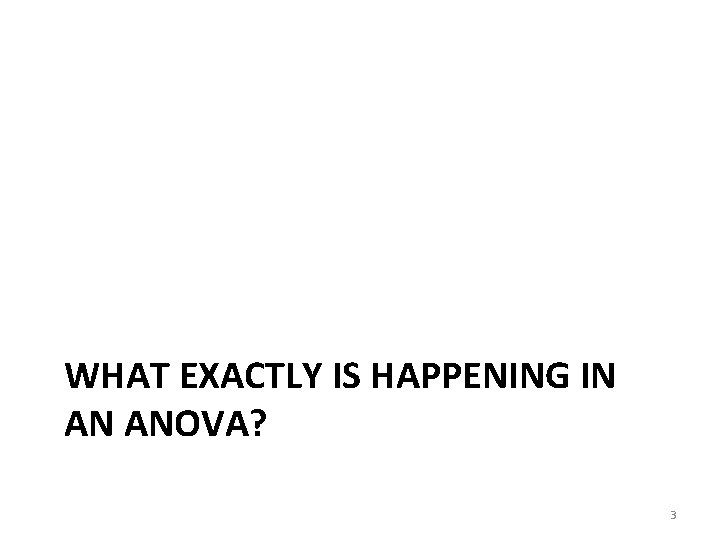
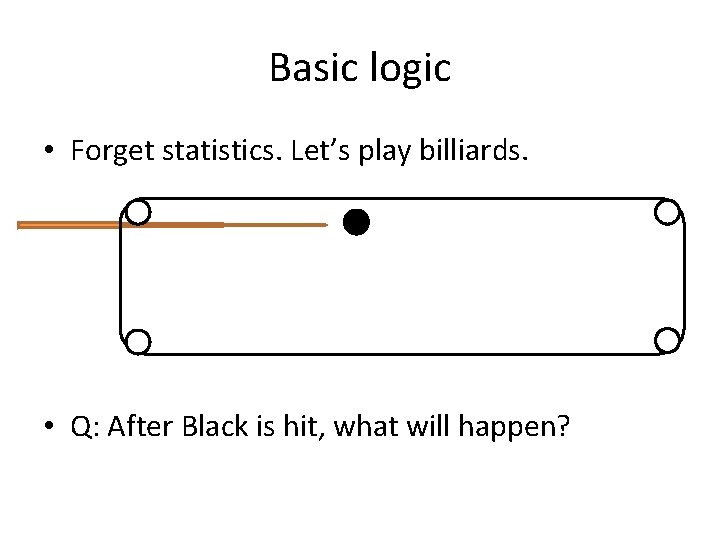
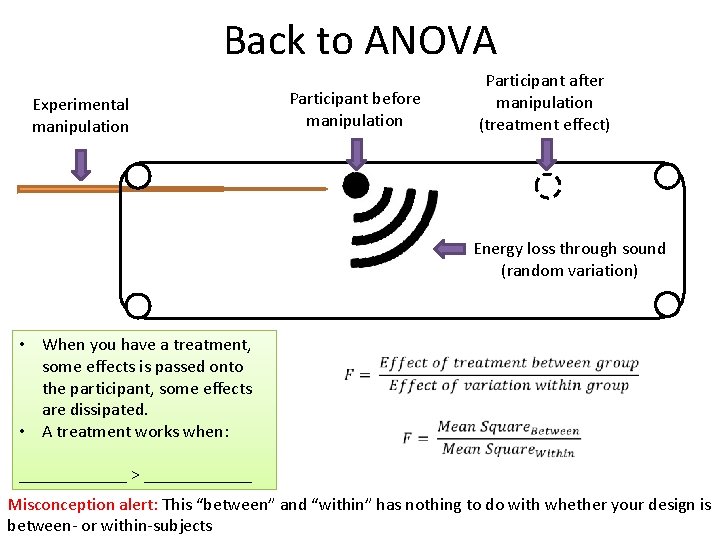
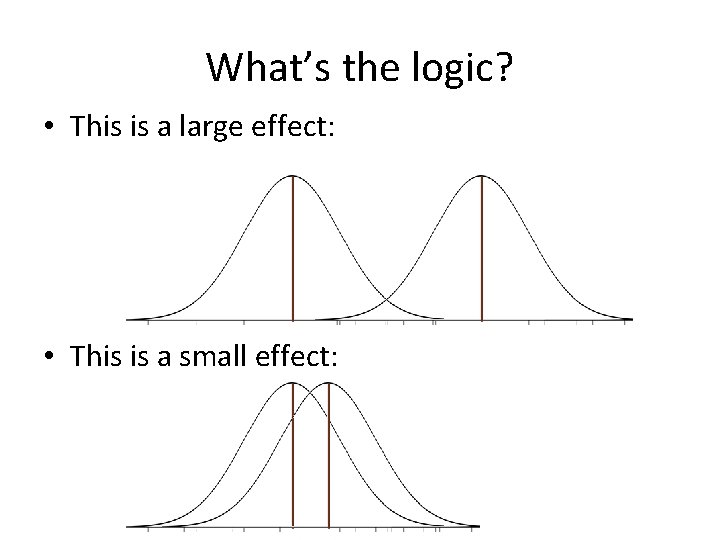
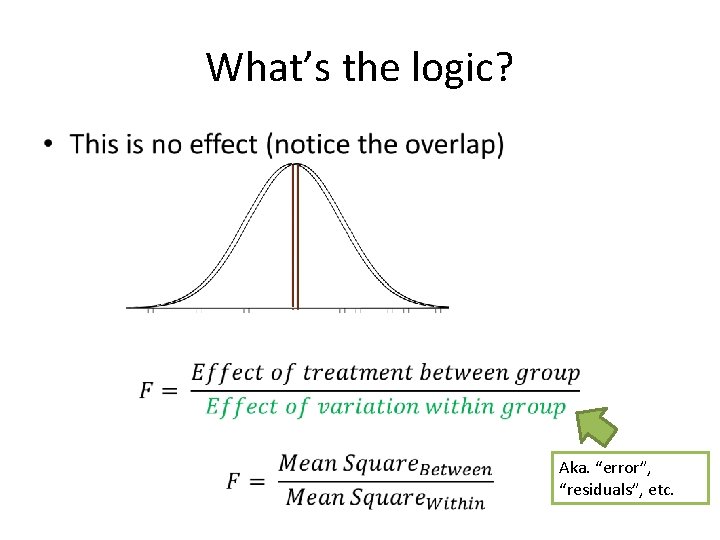
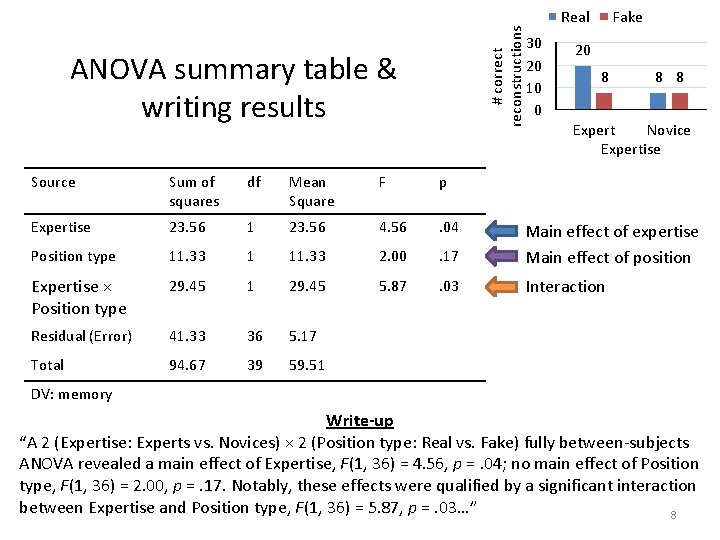
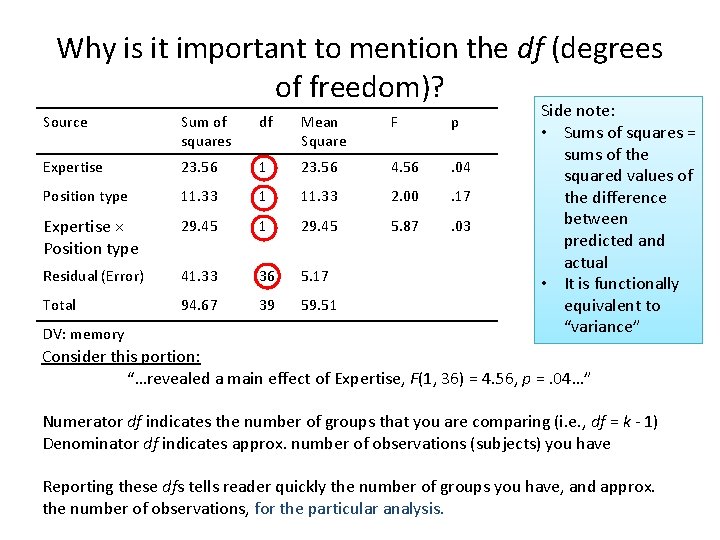
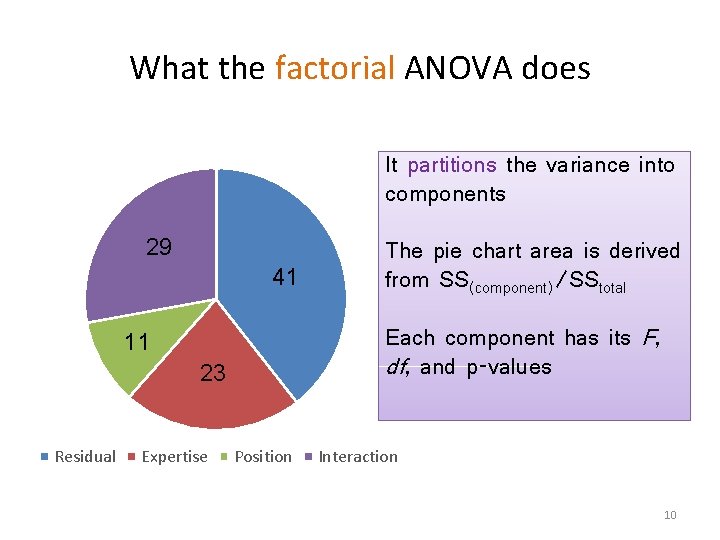
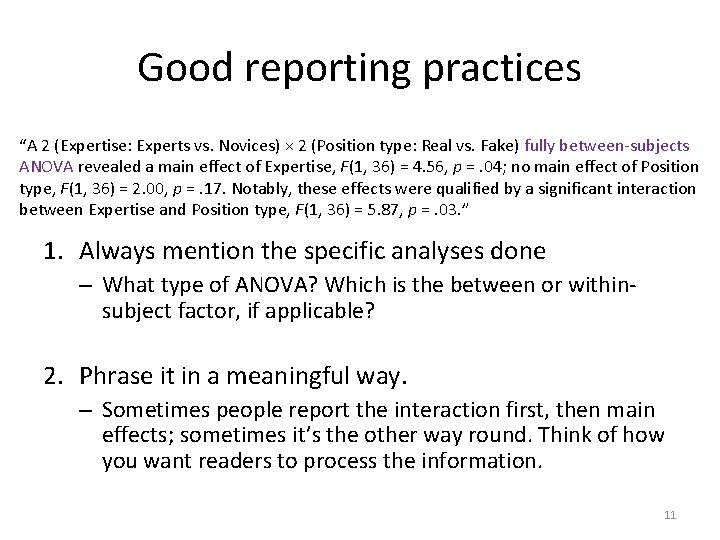
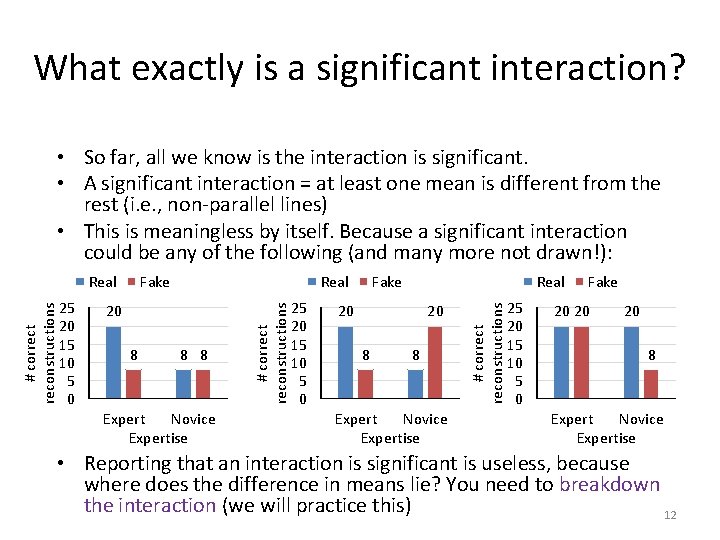
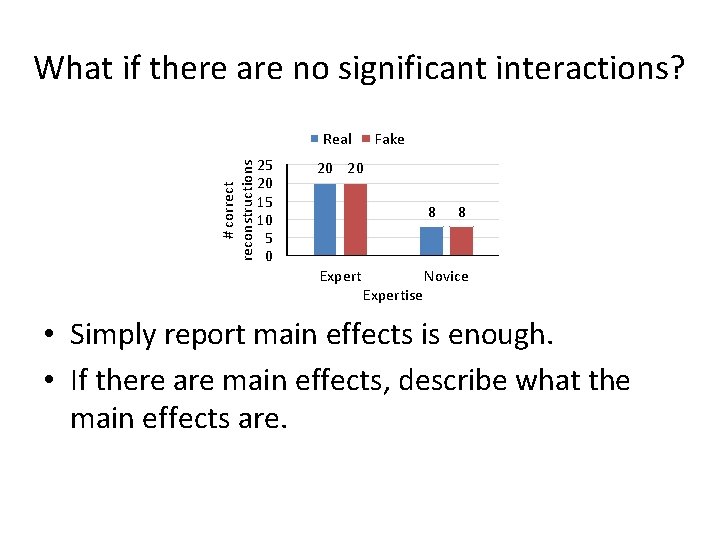
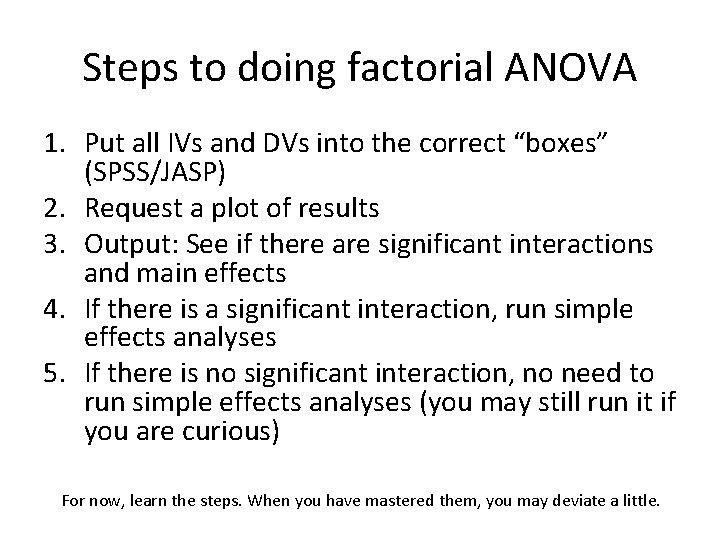
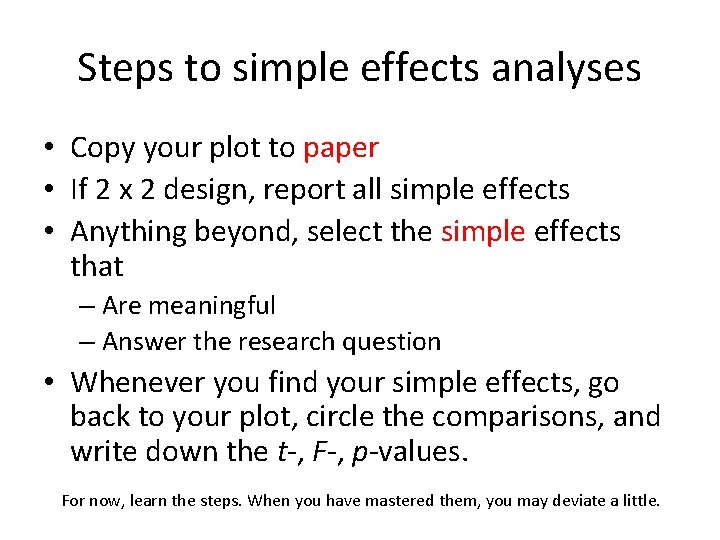
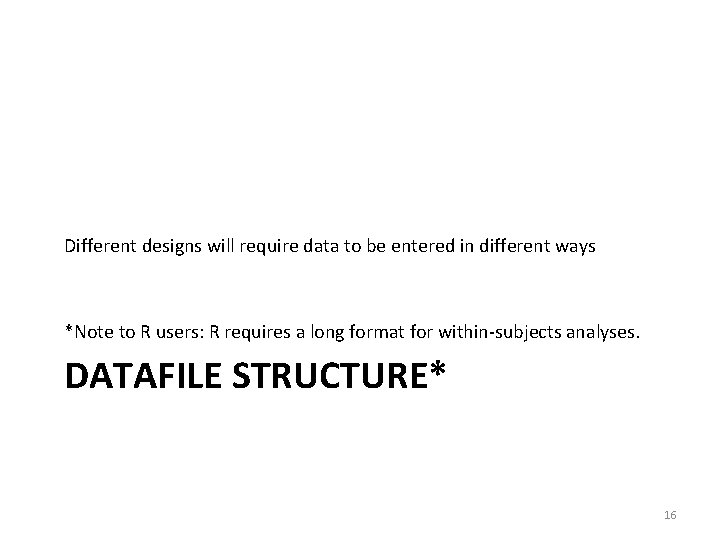
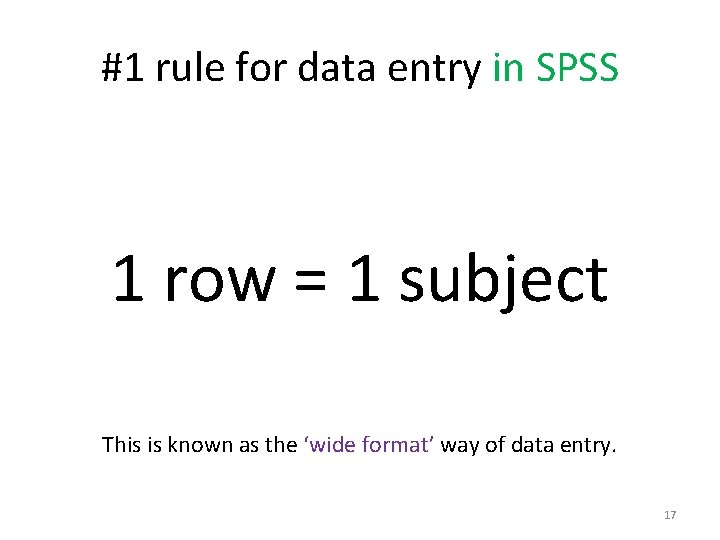
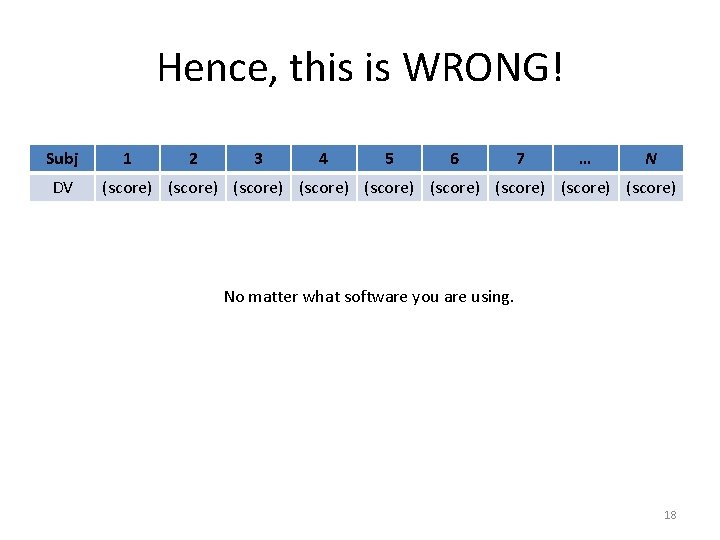
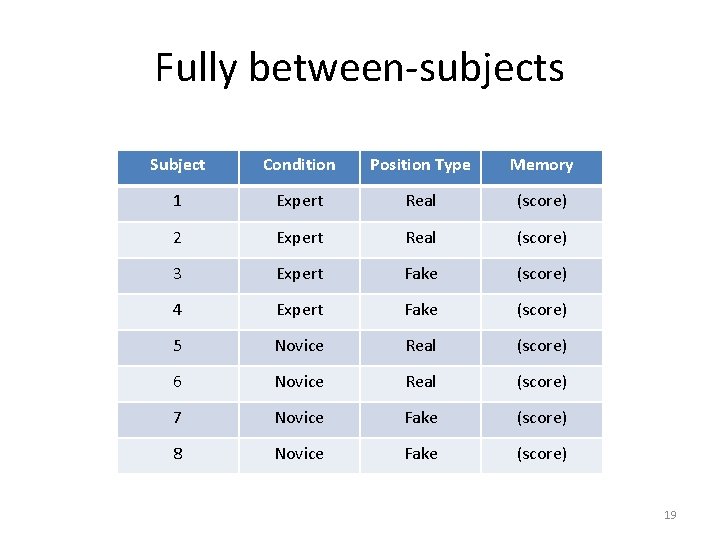
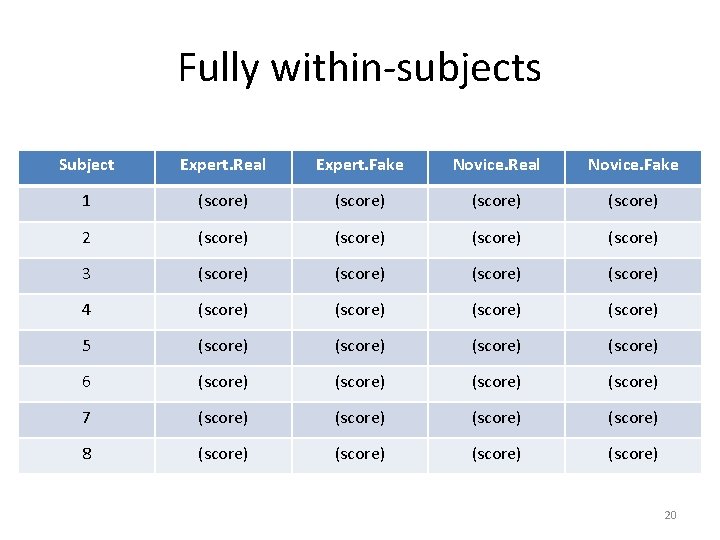
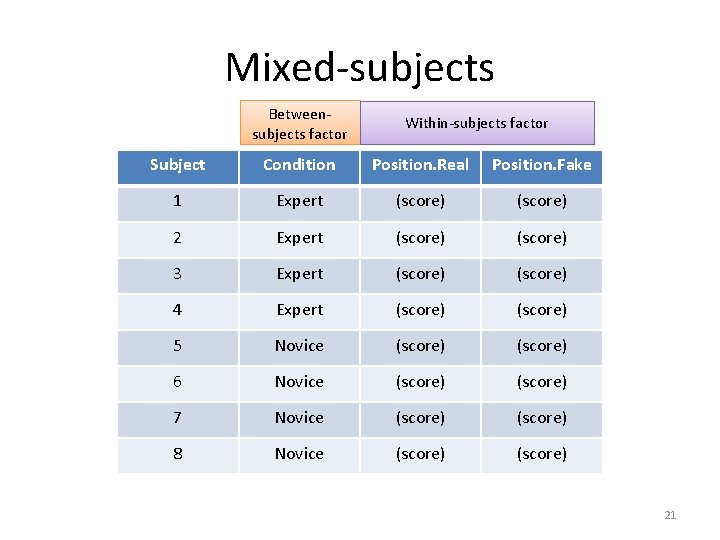
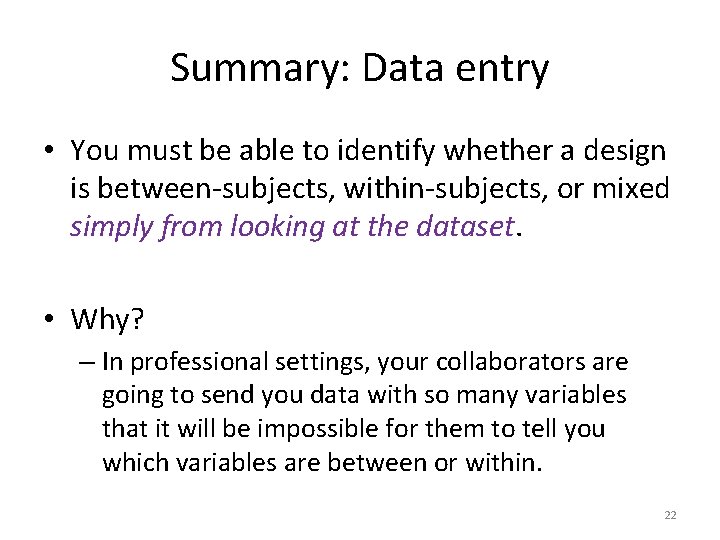
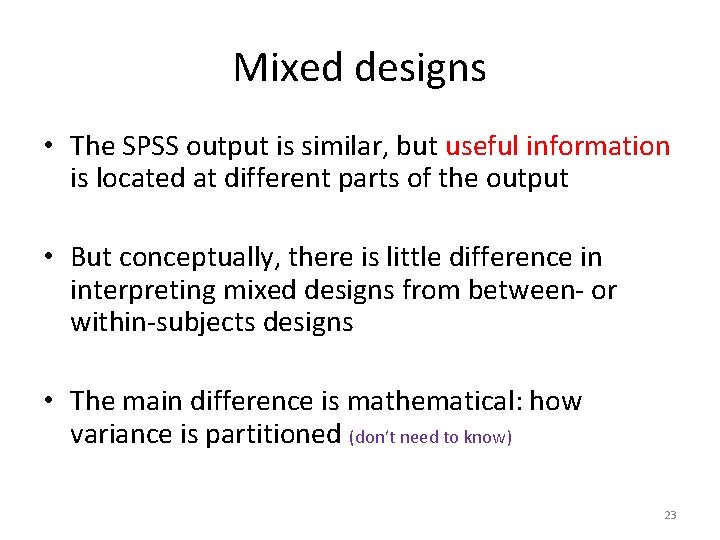
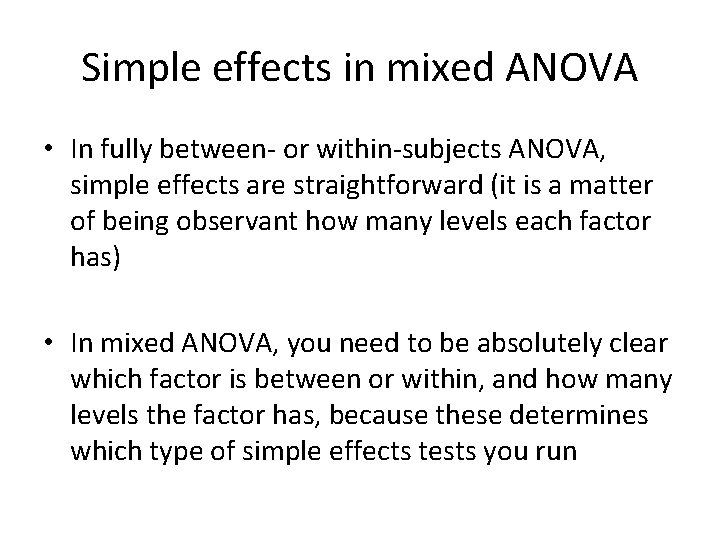
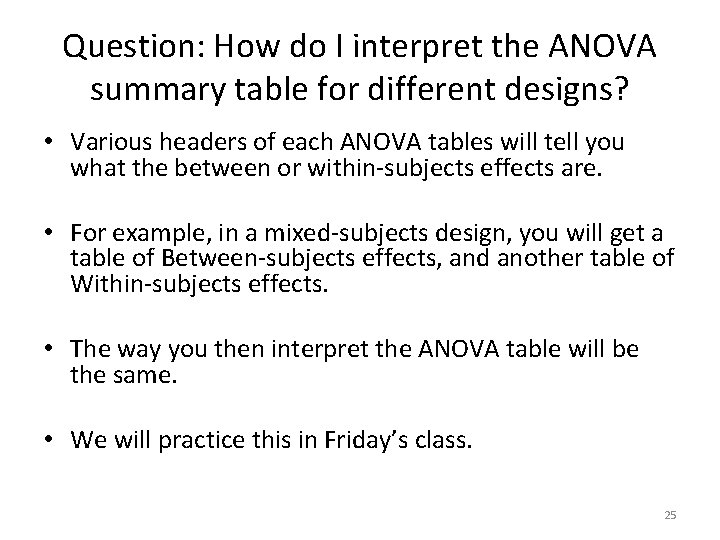
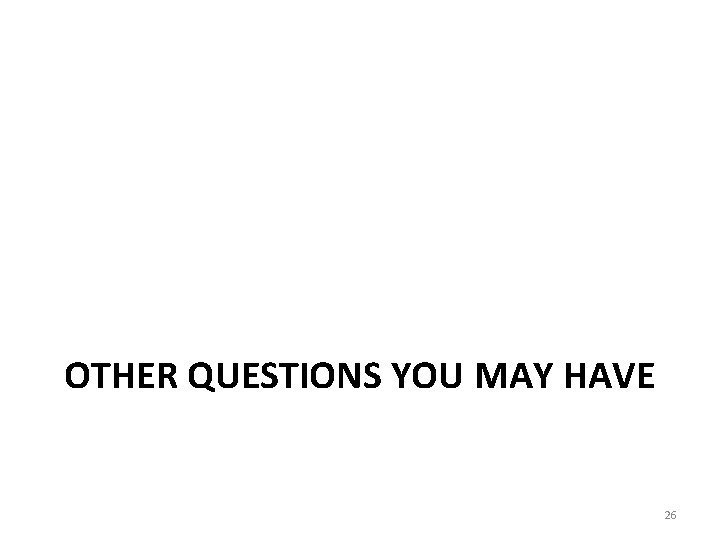
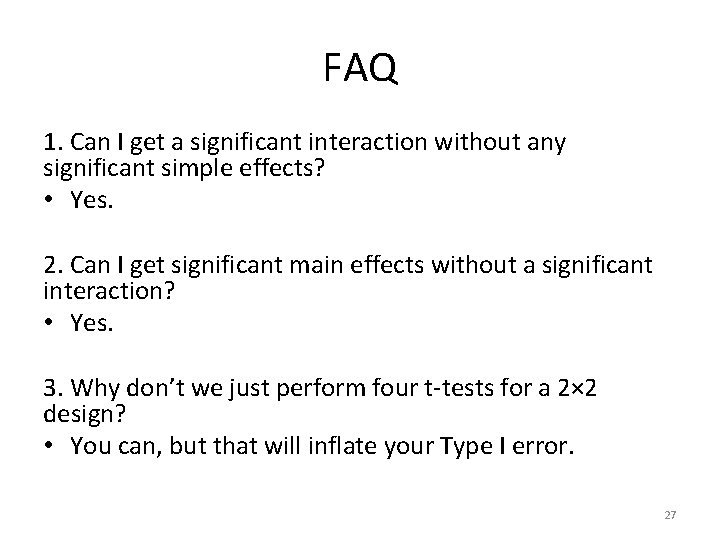
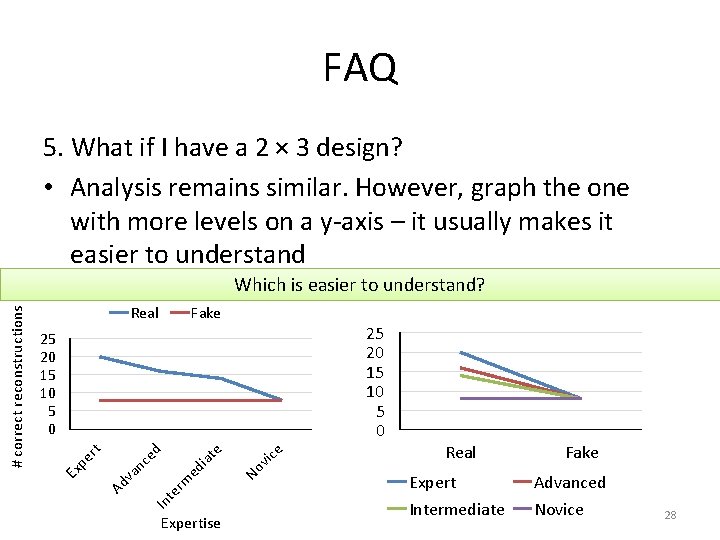
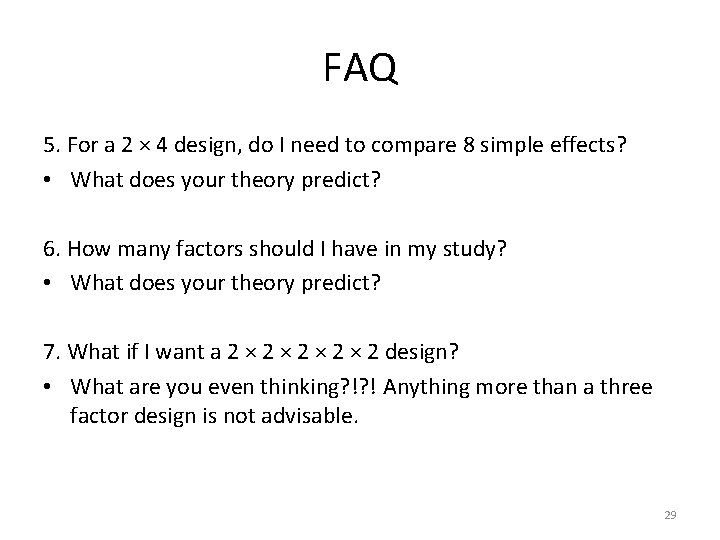
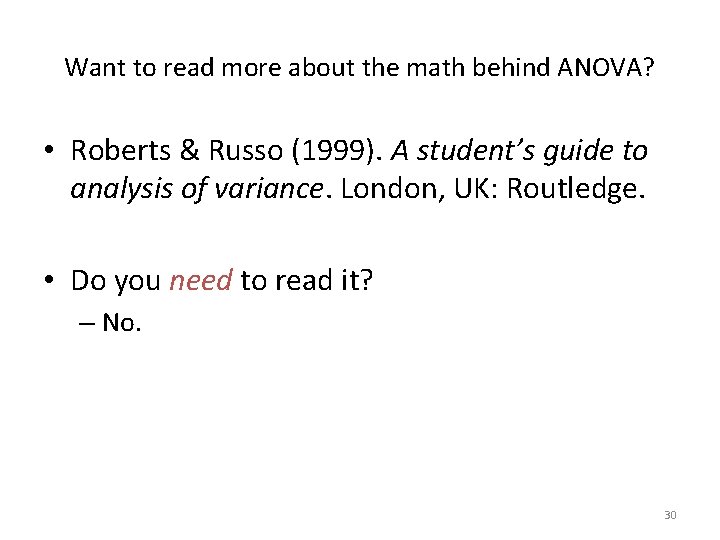
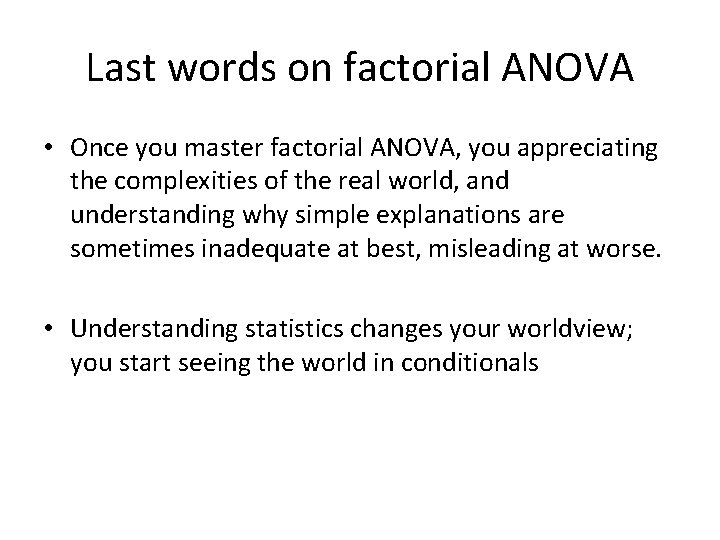
- Slides: 31
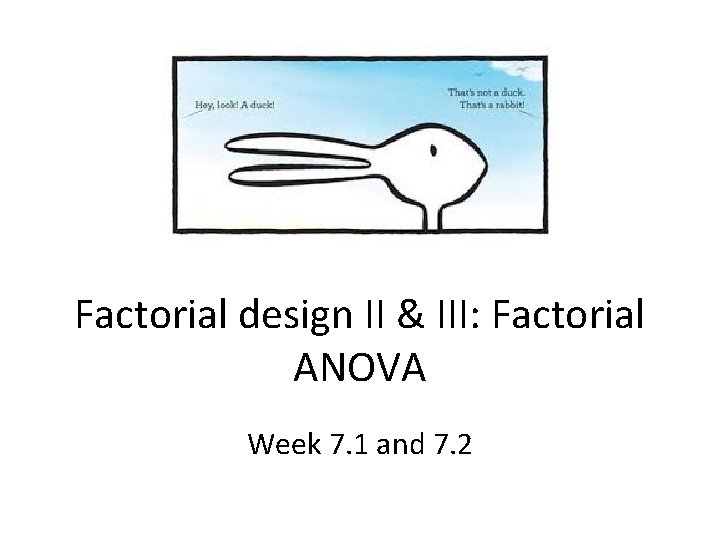
Factorial design II & III: Factorial ANOVA Week 7. 1 and 7. 2
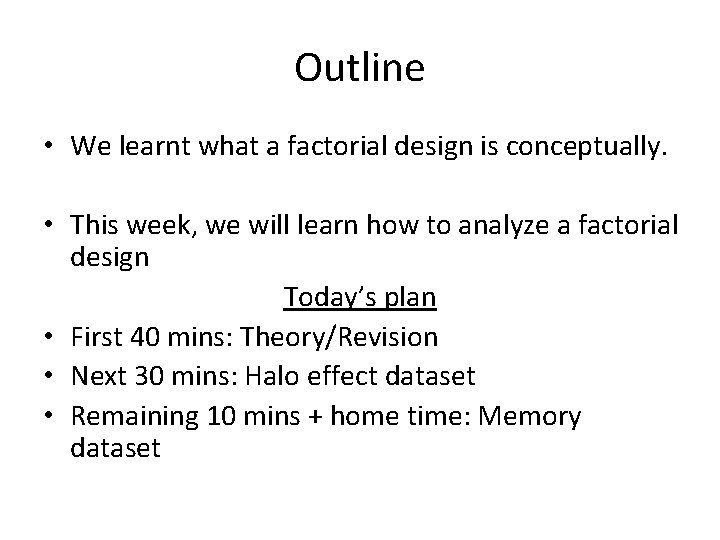
Outline • We learnt what a factorial design is conceptually. • This week, we will learn how to analyze a factorial design Today’s plan • First 40 mins: Theory/Revision • Next 30 mins: Halo effect dataset • Remaining 10 mins + home time: Memory dataset
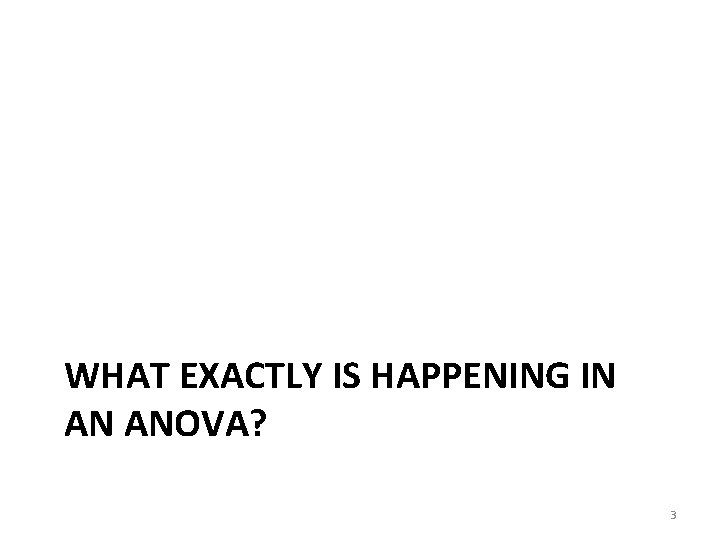
WHAT EXACTLY IS HAPPENING IN AN ANOVA? 3
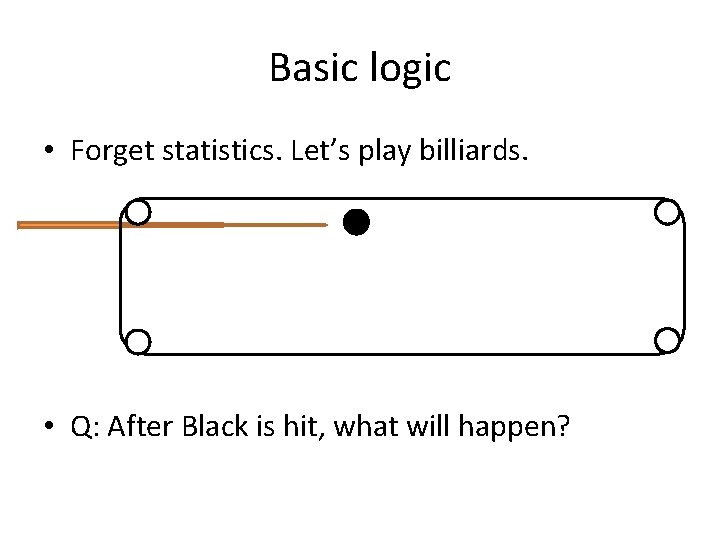
Basic logic • Forget statistics. Let’s play billiards. • Q: After Black is hit, what will happen?
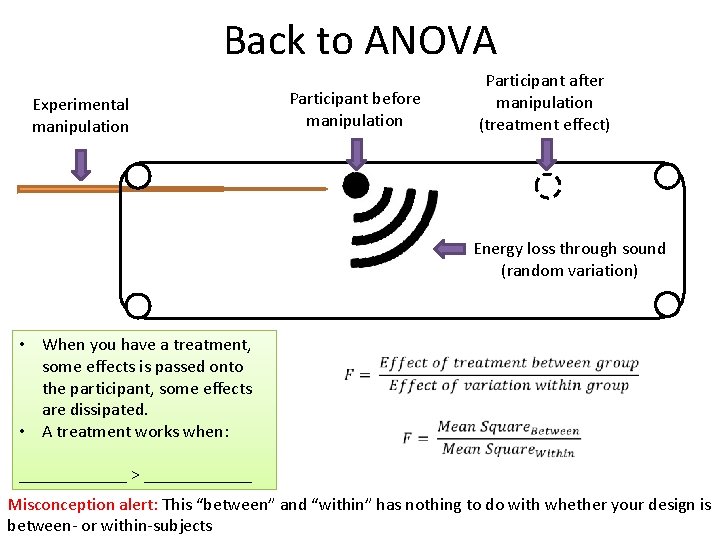
Back to ANOVA Participant before manipulation Experimental manipulation Participant after manipulation (treatment effect) Energy loss through sound (random variation) • When you have a treatment, some effects is passed onto the participant, some effects are dissipated. • A treatment works when: • ______ > ______ Misconception alert: This “between” and “within” has nothing to do with whether your design is between- or within-subjects
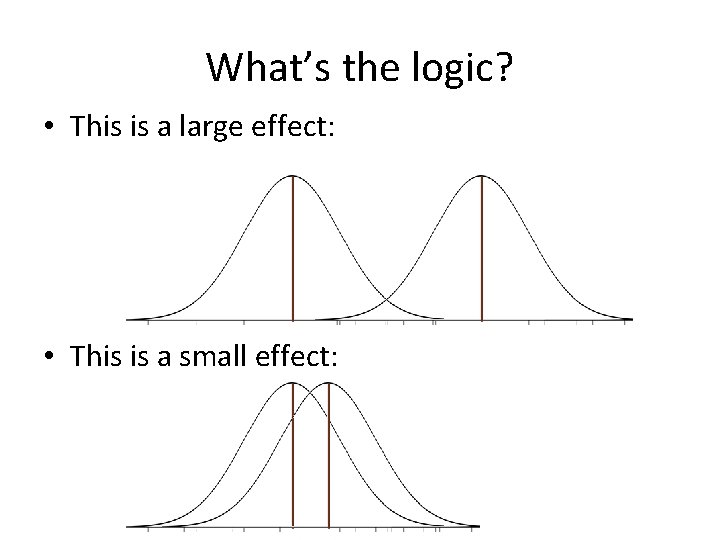
What’s the logic? • This is a large effect: • This is a small effect:
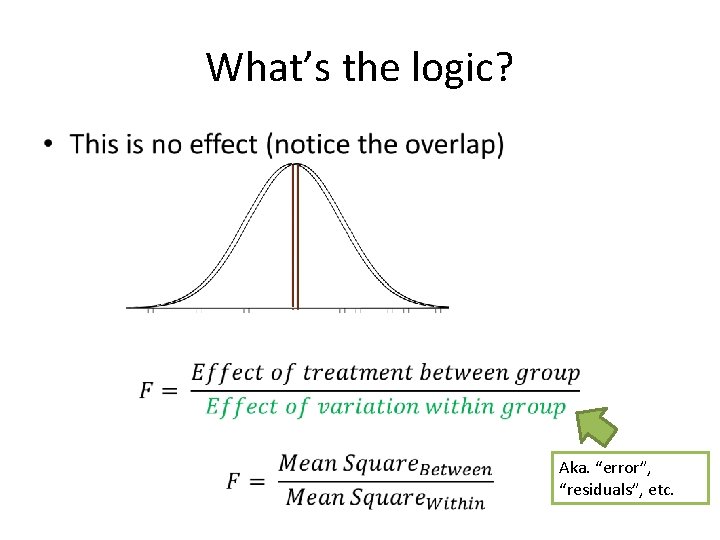
What’s the logic? • Aka. “error”, “residuals”, etc.
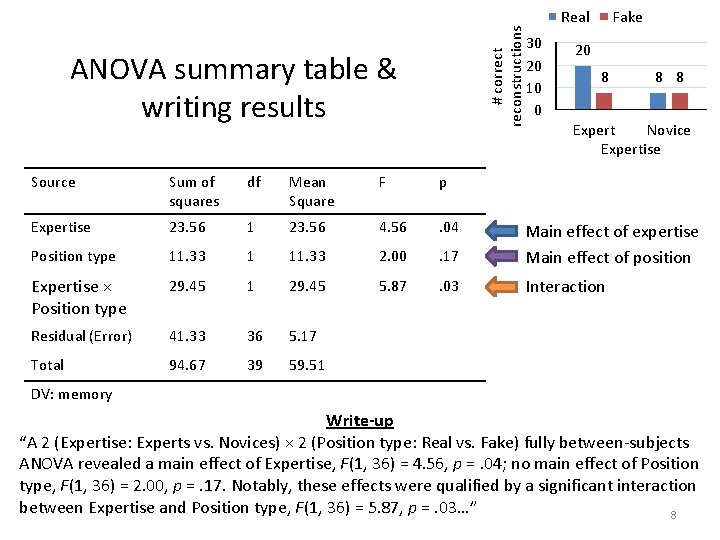
# correct reconstructions ANOVA summary table & writing results Real 30 20 10 0 Fake 20 8 8 8 Expert Novice Expertise Source Sum of squares df Mean Square F p Expertise 23. 56 1 23. 56 4. 56 . 04 Position type 11. 33 1 11. 33 2. 00 . 17 Main effect of expertise Main effect of position Expertise × Position type 29. 45 1 29. 45 5. 87 . 03 Interaction Residual (Error) 41. 33 36 5. 17 Total 94. 67 39 59. 51 DV: memory Write-up “A 2 (Expertise: Experts vs. Novices) × 2 (Position type: Real vs. Fake) fully between-subjects ANOVA revealed a main effect of Expertise, F(1, 36) = 4. 56, p =. 04; no main effect of Position type, F(1, 36) = 2. 00, p =. 17. Notably, these effects were qualified by a significant interaction between Expertise and Position type, F(1, 36) = 5. 87, p =. 03…” 8
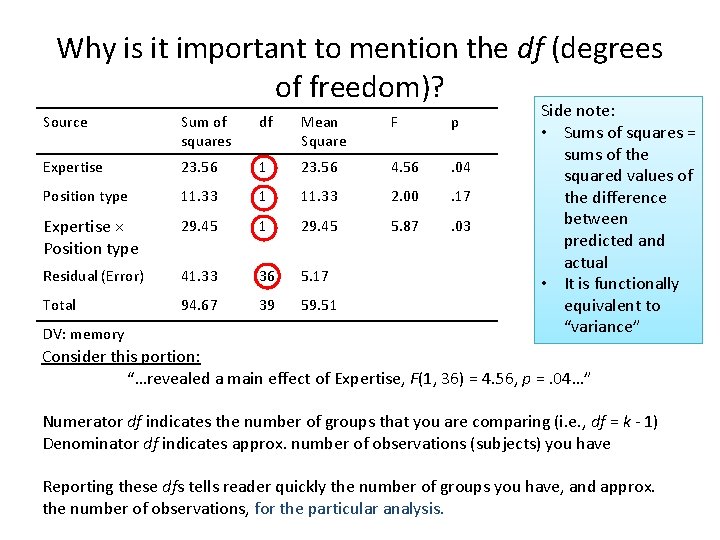
Why is it important to mention the df (degrees of freedom)? Source Sum of squares df Mean Square F p Expertise 23. 56 1 23. 56 4. 56 . 04 Position type 11. 33 1 11. 33 2. 00 . 17 Expertise × Position type 29. 45 1 29. 45 5. 87 . 03 Residual (Error) 41. 33 36 5. 17 Total 94. 67 39 59. 51 DV: memory Side note: • Sums of squares = sums of the squared values of the difference between predicted and actual • It is functionally equivalent to “variance” Consider this portion: “…revealed a main effect of Expertise, F(1, 36) = 4. 56, p =. 04…” Numerator df indicates the number of groups that you are comparing (i. e. , df = k - 1) Denominator df indicates approx. number of observations (subjects) you have Reporting these dfs tells reader quickly the number of groups you have, and approx. the number of observations, for the particular analysis.
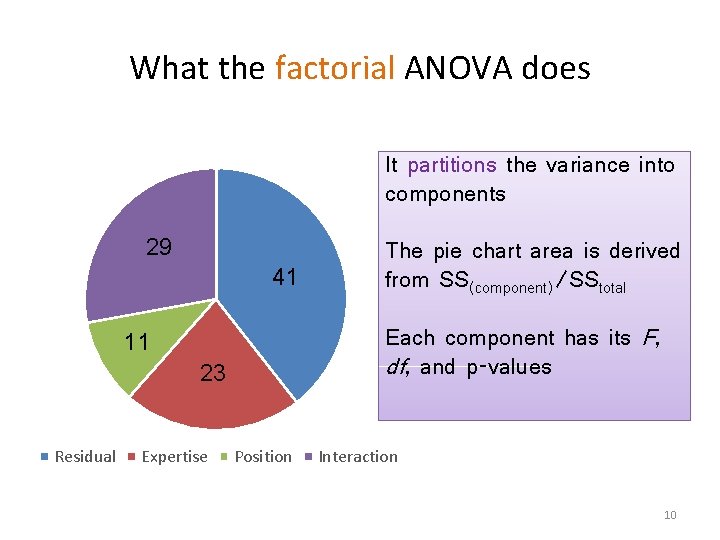
What the factorial ANOVA does It partitions the variance into components 29 41 Each component has its F, df, and p-values 11 23 Residual Expertise The pie chart area is derived from SS(component)/SStotal Position Interaction 10
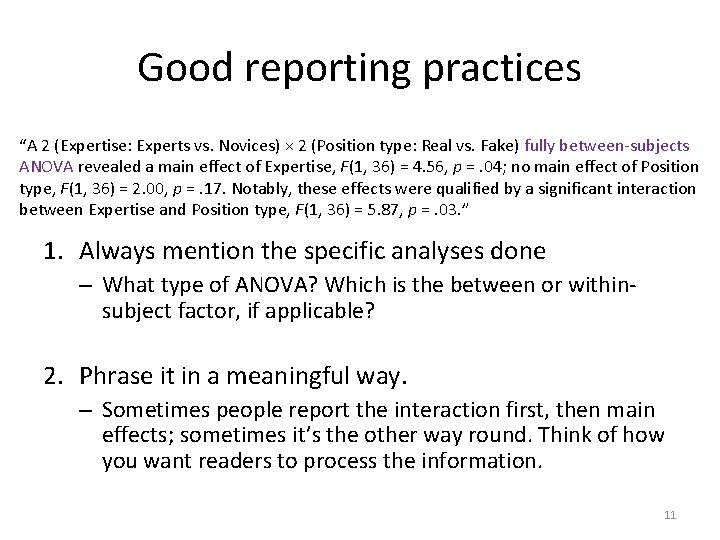
Good reporting practices “A 2 (Expertise: Experts vs. Novices) × 2 (Position type: Real vs. Fake) fully between-subjects ANOVA revealed a main effect of Expertise, F(1, 36) = 4. 56, p =. 04; no main effect of Position type, F(1, 36) = 2. 00, p =. 17. Notably, these effects were qualified by a significant interaction between Expertise and Position type, F(1, 36) = 5. 87, p =. 03. ” 1. Always mention the specific analyses done – What type of ANOVA? Which is the between or withinsubject factor, if applicable? 2. Phrase it in a meaningful way. – Sometimes people report the interaction first, then main effects; sometimes it’s the other way round. Think of how you want readers to process the information. 11
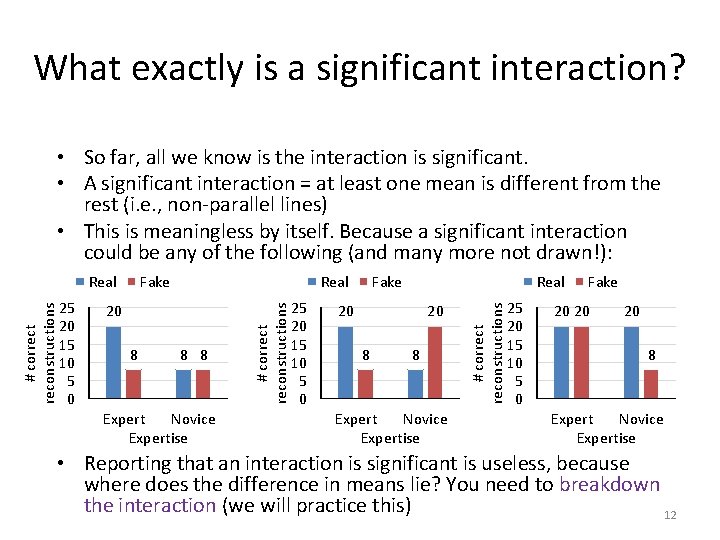
What exactly is a significant interaction? • So far, all we know is the interaction is significant. • A significant interaction = at least one mean is different from the rest (i. e. , non-parallel lines) • This is meaningless by itself. Because a significant interaction could be any of the following (and many more not drawn!): Real 20 8 8 8 Expert Novice Expertise 25 20 15 10 5 0 Fake Real 20 20 8 8 Expert Novice Expertise # correct reconstructions 25 20 15 10 5 0 Fake # correct reconstructions Real 25 20 15 10 5 0 Fake 20 20 20 8 Expert Novice Expertise • Reporting that an interaction is significant is useless, because where does the difference in means lie? You need to breakdown the interaction (we will practice this) 12
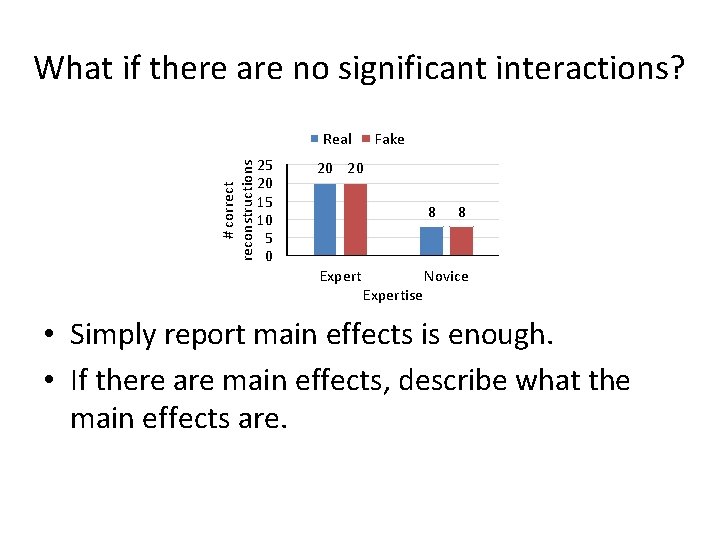
What if there are no significant interactions? # correct reconstructions Real 25 20 15 10 5 0 Fake 20 20 8 Expertise 8 Novice • Simply report main effects is enough. • If there are main effects, describe what the main effects are.
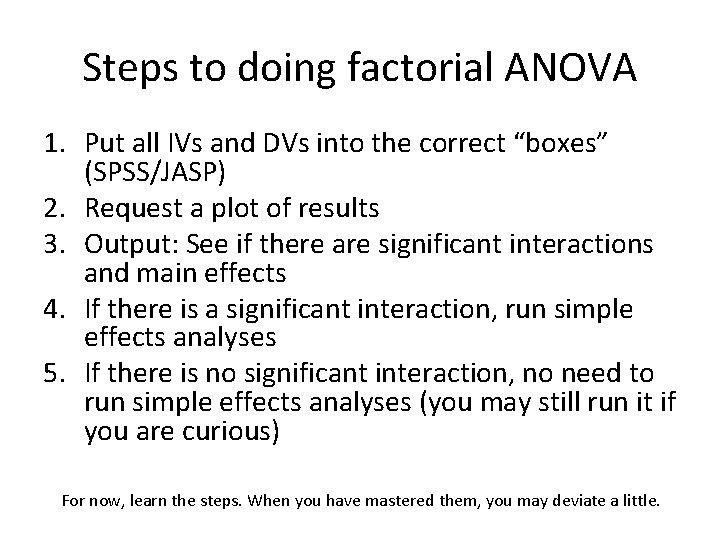
Steps to doing factorial ANOVA 1. Put all IVs and DVs into the correct “boxes” (SPSS/JASP) 2. Request a plot of results 3. Output: See if there are significant interactions and main effects 4. If there is a significant interaction, run simple effects analyses 5. If there is no significant interaction, no need to run simple effects analyses (you may still run it if you are curious) For now, learn the steps. When you have mastered them, you may deviate a little.
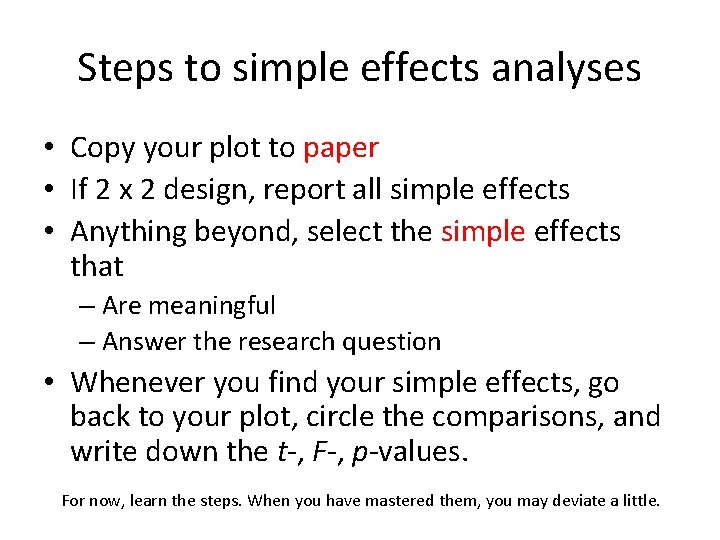
Steps to simple effects analyses • Copy your plot to paper • If 2 x 2 design, report all simple effects • Anything beyond, select the simple effects that – Are meaningful – Answer the research question • Whenever you find your simple effects, go back to your plot, circle the comparisons, and write down the t-, F-, p-values. For now, learn the steps. When you have mastered them, you may deviate a little.
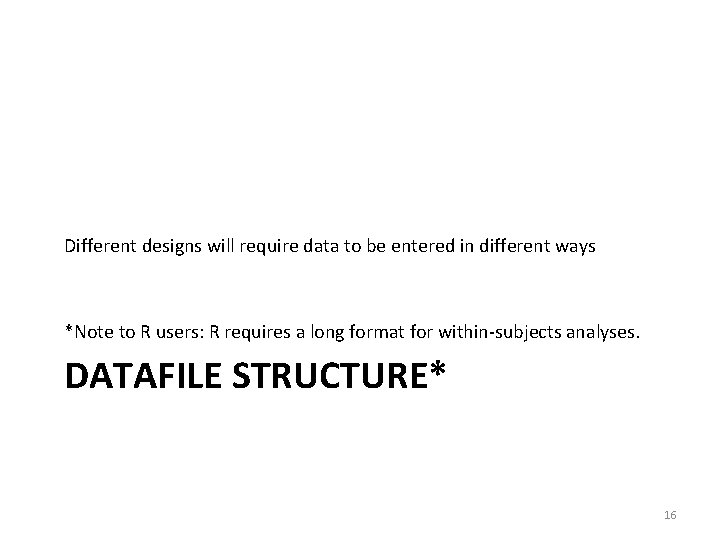
Different designs will require data to be entered in different ways *Note to R users: R requires a long format for within-subjects analyses. DATAFILE STRUCTURE* 16
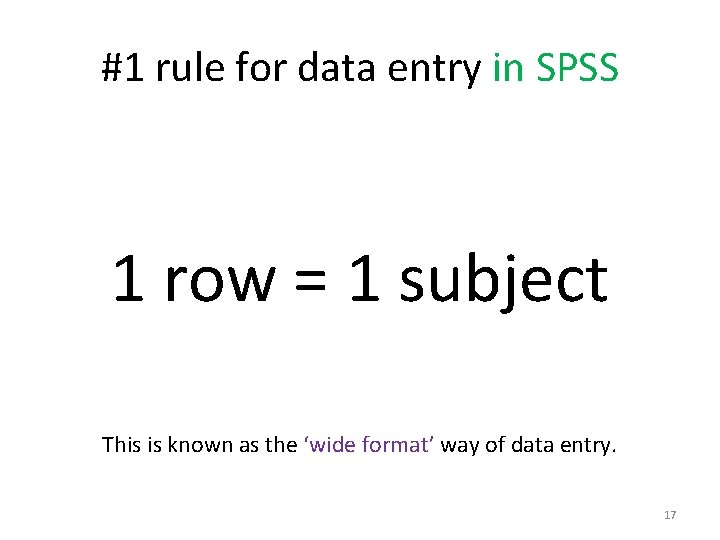
#1 rule for data entry in SPSS 1 row = 1 subject This is known as the ‘wide format’ way of data entry. 17
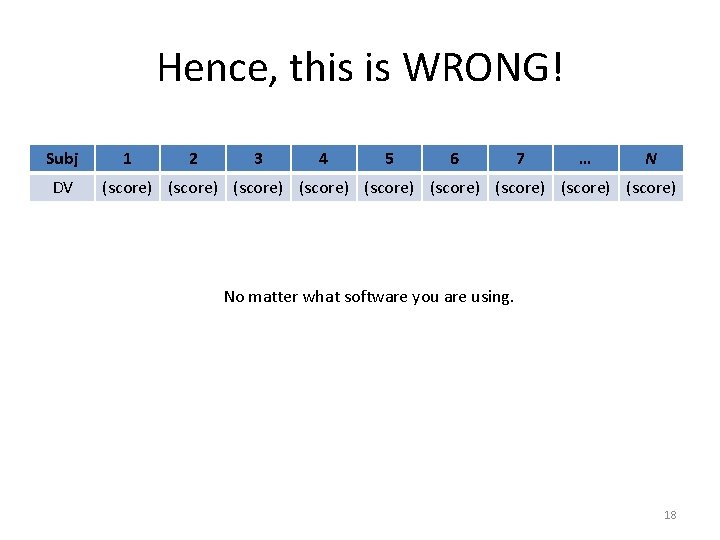
Hence, this is WRONG! Subj DV 1 2 3 4 5 6 7 … N (score) (score) (score) No matter what software you are using. 18
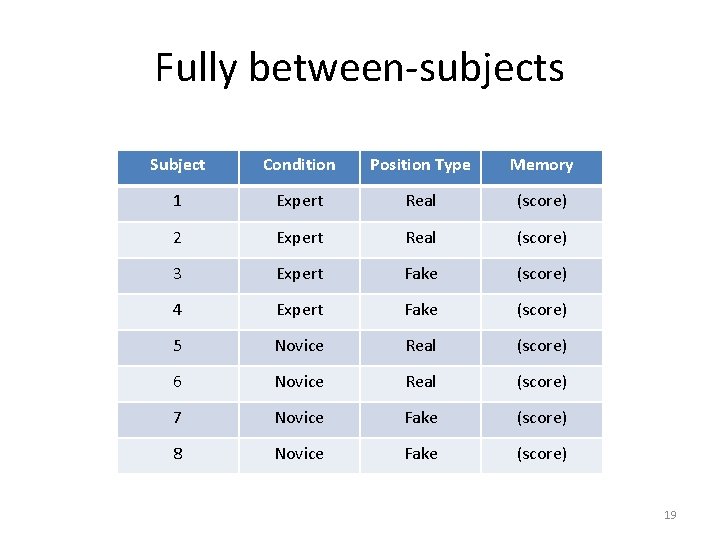
Fully between-subjects Subject Condition Position Type Memory 1 Expert Real (score) 2 Expert Real (score) 3 Expert Fake (score) 4 Expert Fake (score) 5 Novice Real (score) 6 Novice Real (score) 7 Novice Fake (score) 8 Novice Fake (score) 19
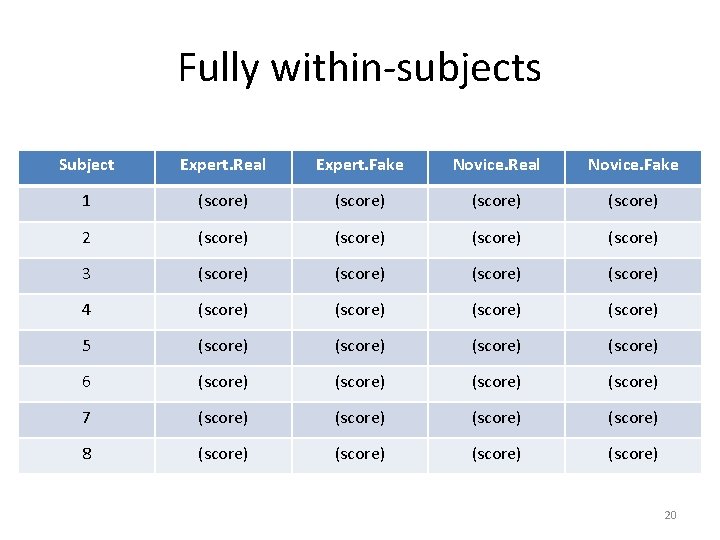
Fully within-subjects Subject Expert. Real Expert. Fake Novice. Real Novice. Fake 1 (score) 2 (score) 3 (score) 4 (score) 5 (score) 6 (score) 7 (score) 8 (score) 20
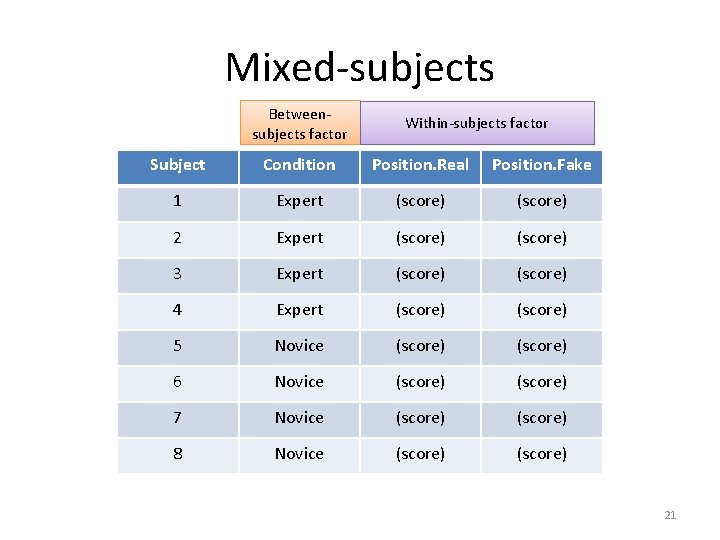
Mixed-subjects Betweensubjects factor Within-subjects factor Subject Condition Position. Real Position. Fake 1 Expert (score) 2 Expert (score) 3 Expert (score) 4 Expert (score) 5 Novice (score) 6 Novice (score) 7 Novice (score) 8 Novice (score) 21
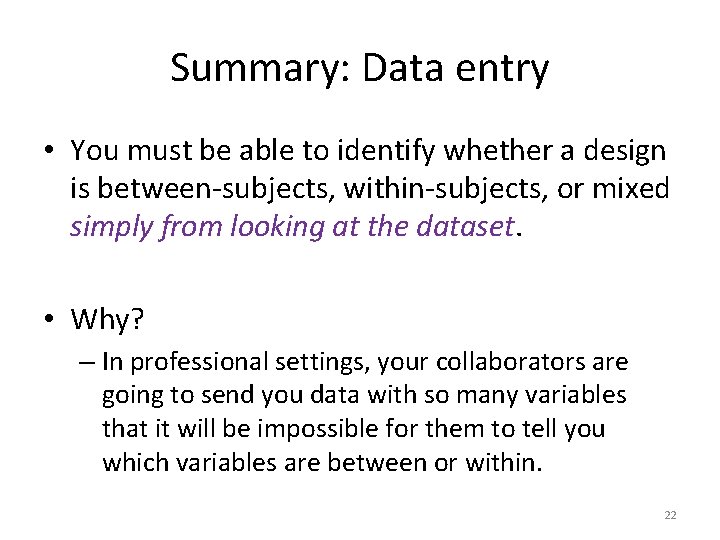
Summary: Data entry • You must be able to identify whether a design is between-subjects, within-subjects, or mixed simply from looking at the dataset. • Why? – In professional settings, your collaborators are going to send you data with so many variables that it will be impossible for them to tell you which variables are between or within. 22
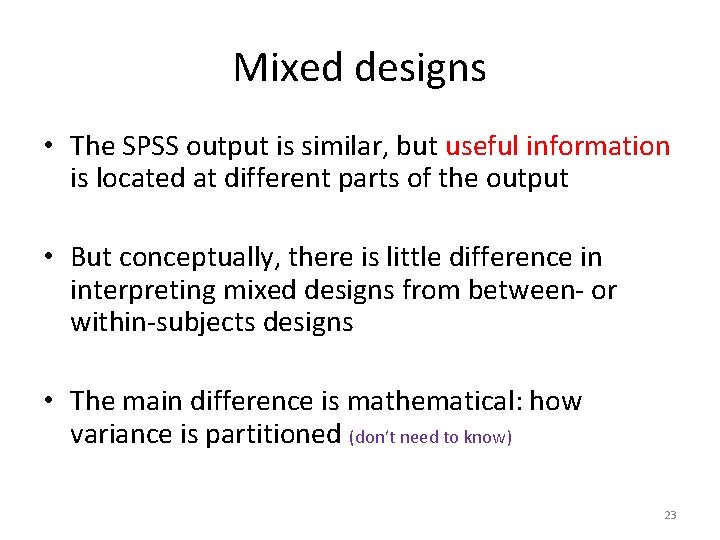
Mixed designs • The SPSS output is similar, but useful information is located at different parts of the output • But conceptually, there is little difference in interpreting mixed designs from between- or within-subjects designs • The main difference is mathematical: how variance is partitioned (don’t need to know) 23
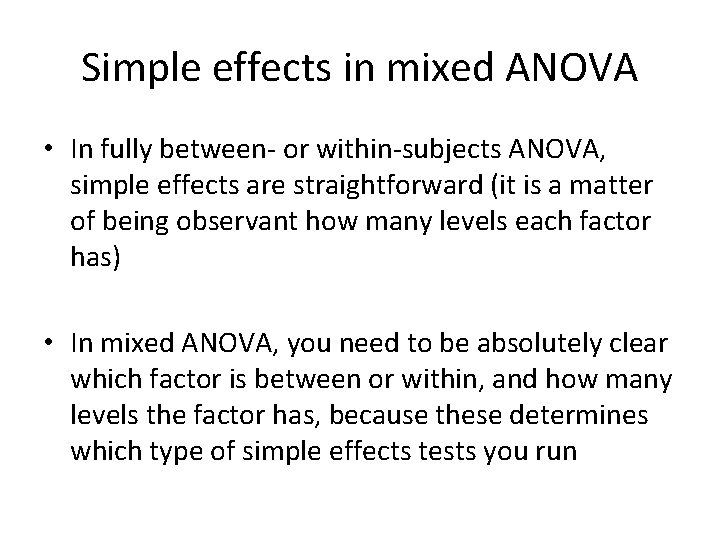
Simple effects in mixed ANOVA • In fully between- or within-subjects ANOVA, simple effects are straightforward (it is a matter of being observant how many levels each factor has) • In mixed ANOVA, you need to be absolutely clear which factor is between or within, and how many levels the factor has, because these determines which type of simple effects tests you run
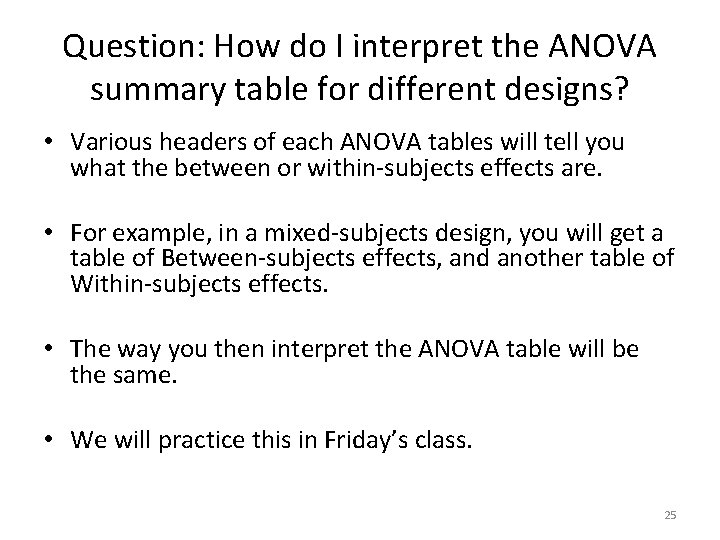
Question: How do I interpret the ANOVA summary table for different designs? • Various headers of each ANOVA tables will tell you what the between or within-subjects effects are. • For example, in a mixed-subjects design, you will get a table of Between-subjects effects, and another table of Within-subjects effects. • The way you then interpret the ANOVA table will be the same. • We will practice this in Friday’s class. 25
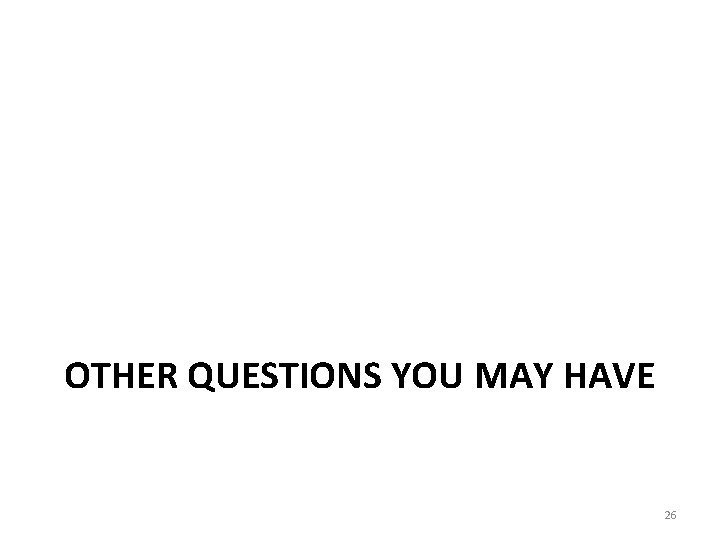
OTHER QUESTIONS YOU MAY HAVE 26
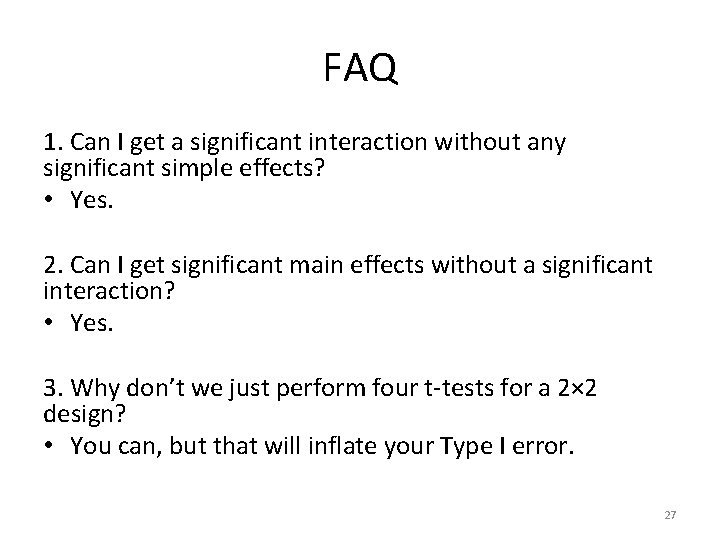
FAQ 1. Can I get a significant interaction without any significant simple effects? • Yes. 2. Can I get significant main effects without a significant interaction? • Yes. 3. Why don’t we just perform four t-tests for a 2× 2 design? • You can, but that will inflate your Type I error. 27
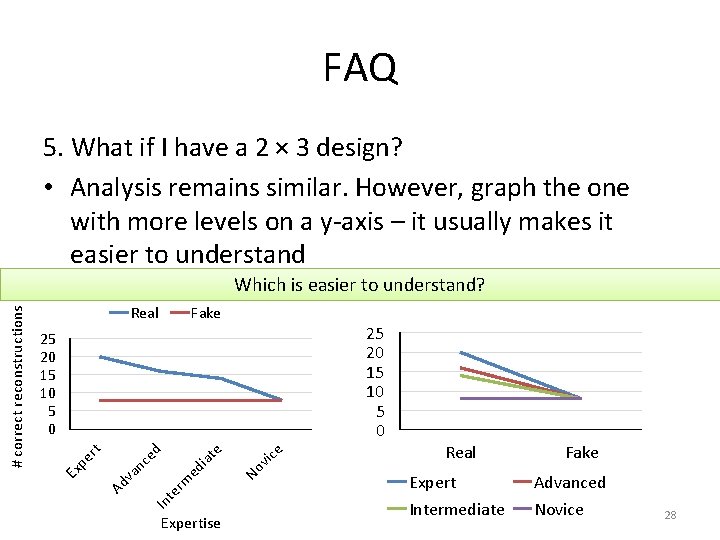
FAQ 5. What if I have a 2 × 3 design? • Analysis remains similar. However, graph the one with more levels on a y-axis – it usually makes it easier to understand Real Fake 25 20 15 10 5 0 ed rm te In Expertise ice No v ia te ed nc Ad va pe rt 25 20 15 10 5 0 Ex # correct reconstructions Which is easier to understand? Real Fake Expert Advanced Intermediate Novice 28
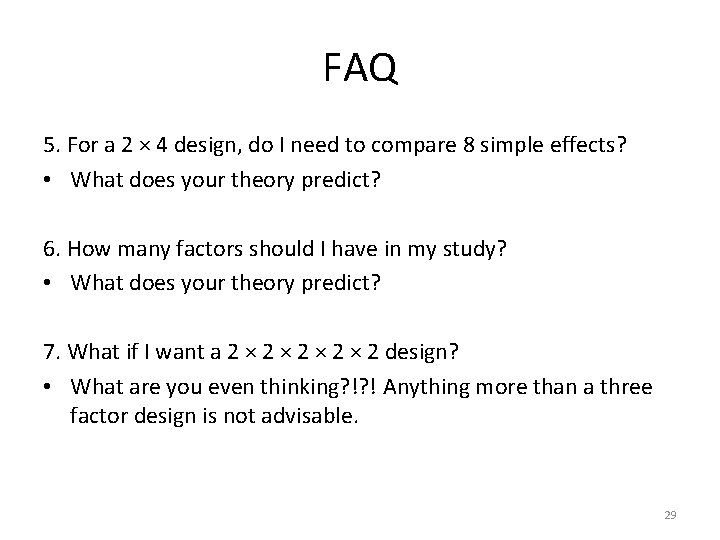
FAQ 5. For a 2 × 4 design, do I need to compare 8 simple effects? • What does your theory predict? 6. How many factors should I have in my study? • What does your theory predict? 7. What if I want a 2 × 2 × 2 design? • What are you even thinking? !? ! Anything more than a three factor design is not advisable. 29
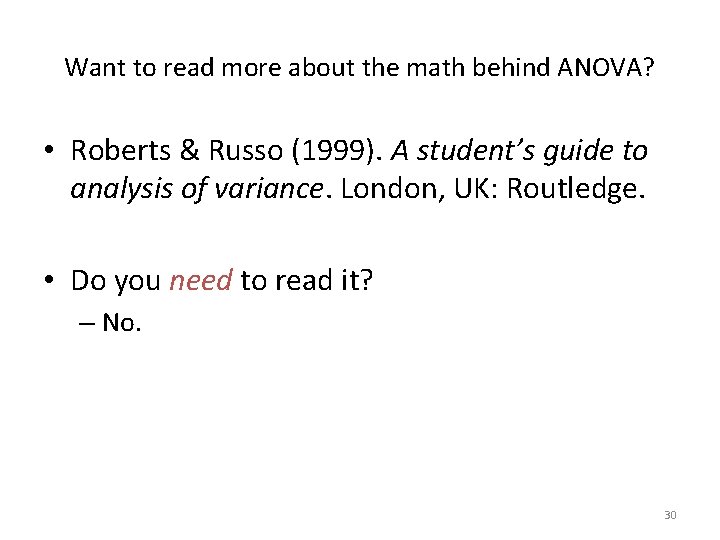
Want to read more about the math behind ANOVA? • Roberts & Russo (1999). A student’s guide to analysis of variance. London, UK: Routledge. • Do you need to read it? – No. 30
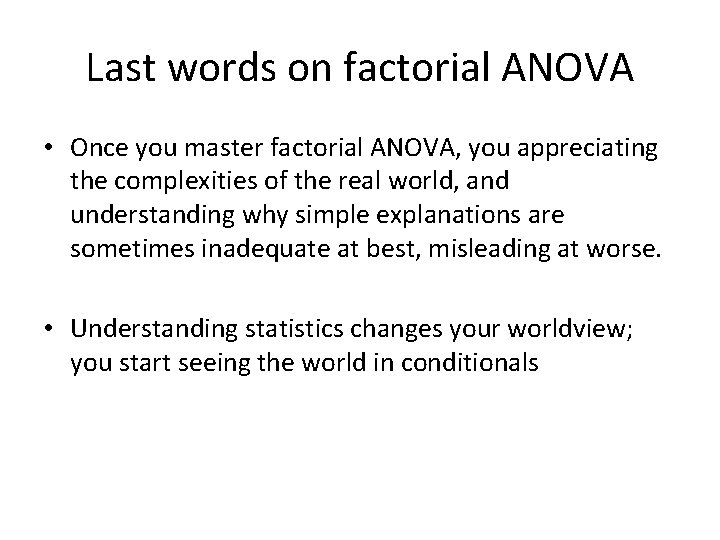
Last words on factorial ANOVA • Once you master factorial ANOVA, you appreciating the complexities of the real world, and understanding why simple explanations are sometimes inadequate at best, misleading at worse. • Understanding statistics changes your worldview; you start seeing the world in conditionals
Factorial anova
Factorial anova
Beda one way anova dan two way anova
One way anova vs two way anova
Uji two way anova
Hamlet act iii scene iii
Week by week plans for documenting children's development
Expericorr
2x2 factorial design
What is between and within subjects design
Factorial design
Between subjects factorial design
Within participants design
Design of experiments six sigma
Rancangan faktorial
8 factorial
Three way factorial design
Simple effect in factorial design
Factorial randomized block design
Factorial randomized block design
Jmp factorial design
Advantages of factorial design
Randomized block design example
Design treatment
One half factorial
Factorial research design in psychology
Manova with repeated measures
Anova matrix
Contoh rancangan petak terbagi
Factorial button on calculator
Formula for permutation and combination
Descomposición factorial