Edgepreserving model regularization for parameters estimation Alejandro A
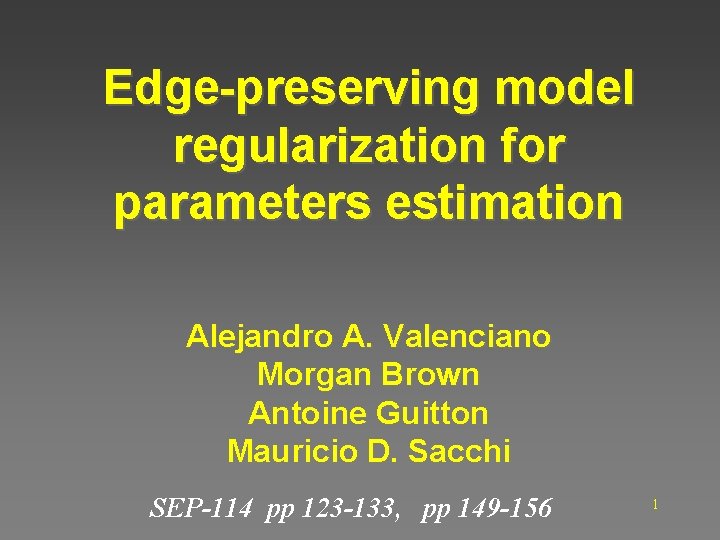
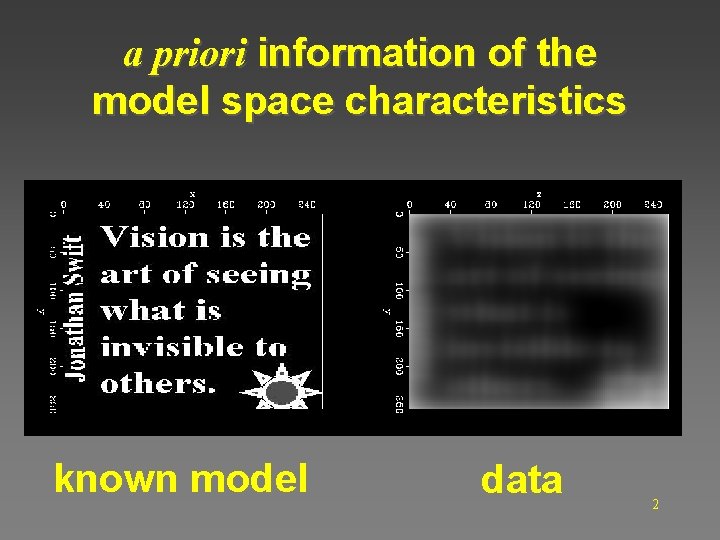
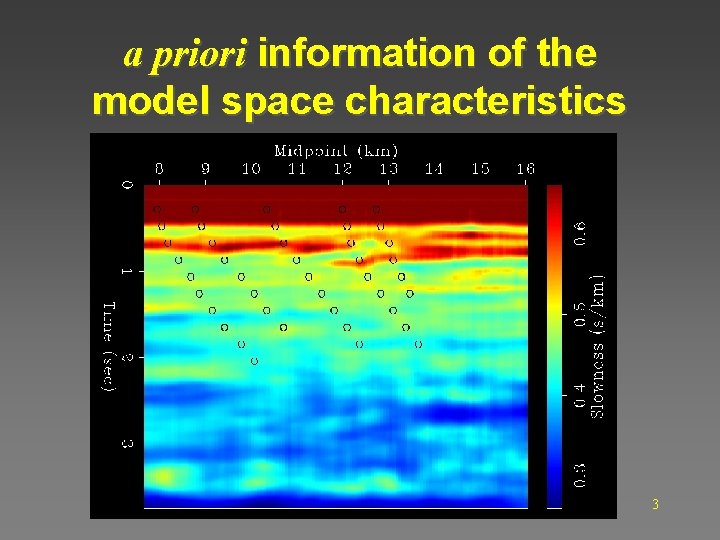
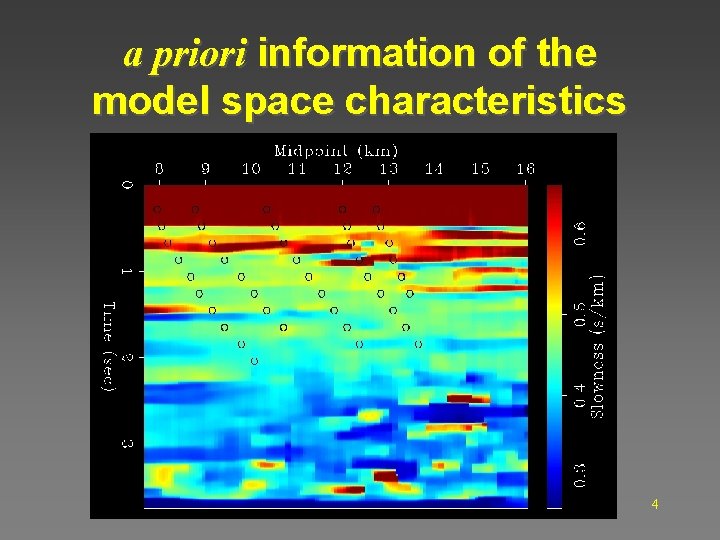
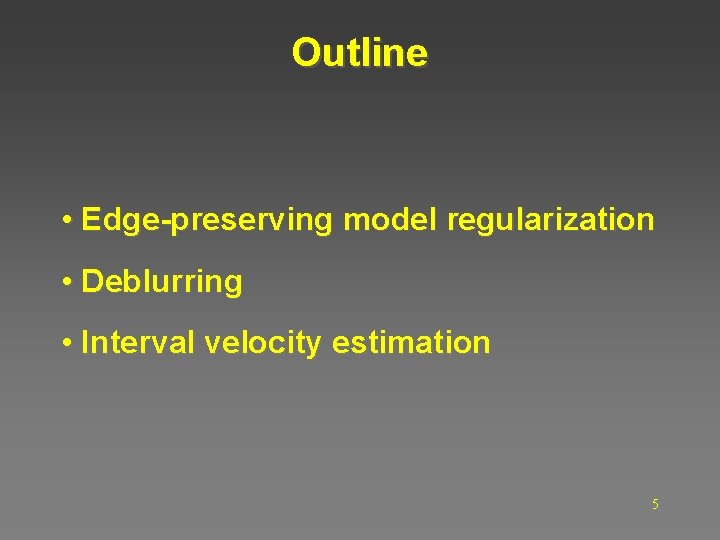
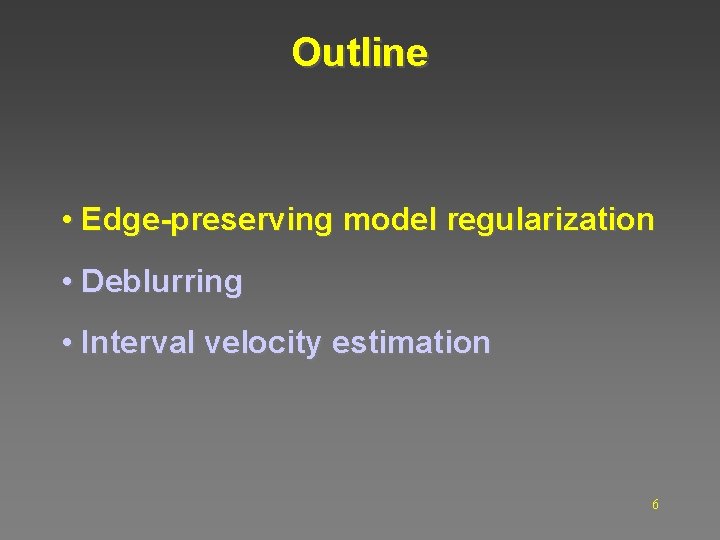
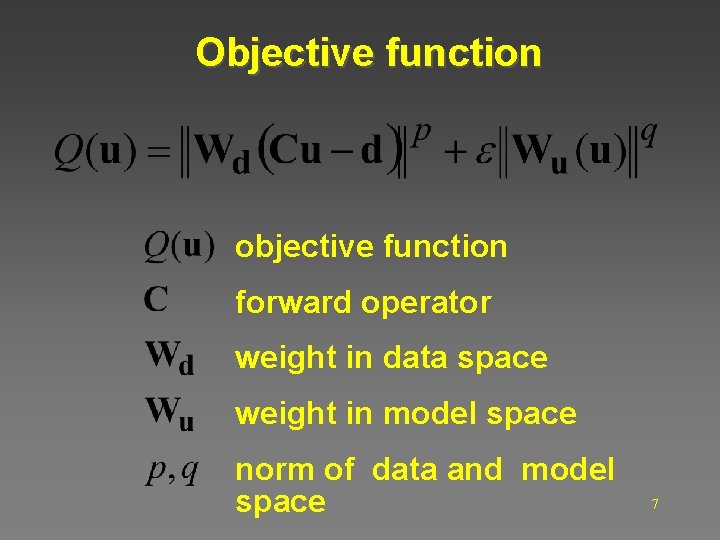
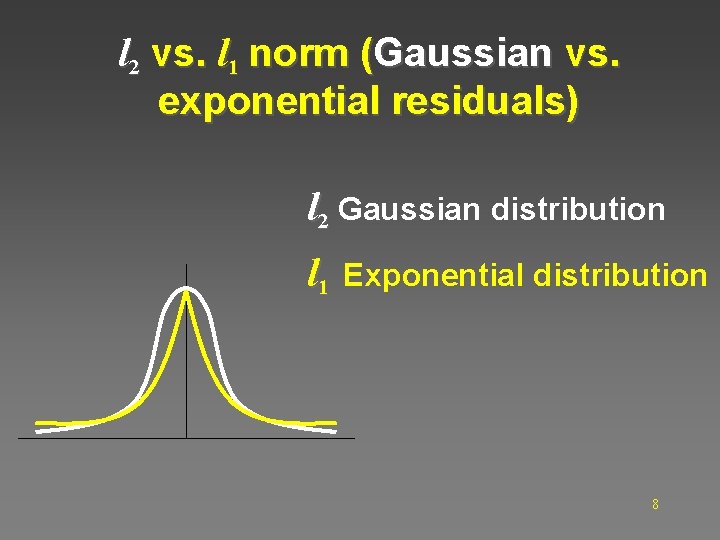
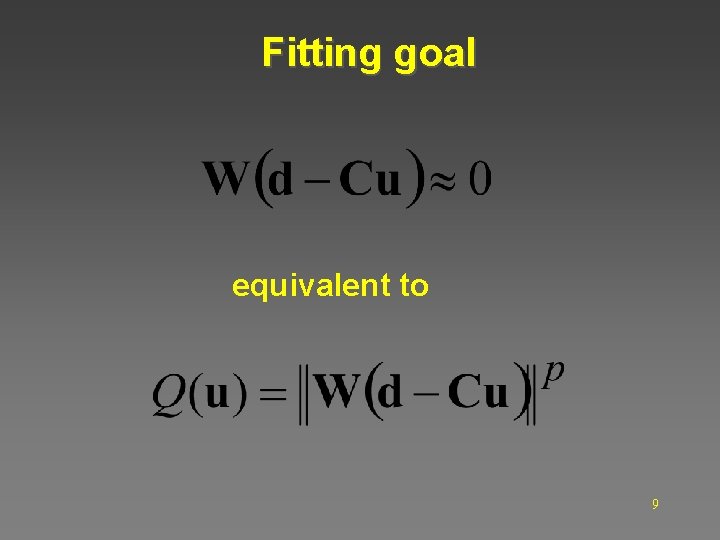
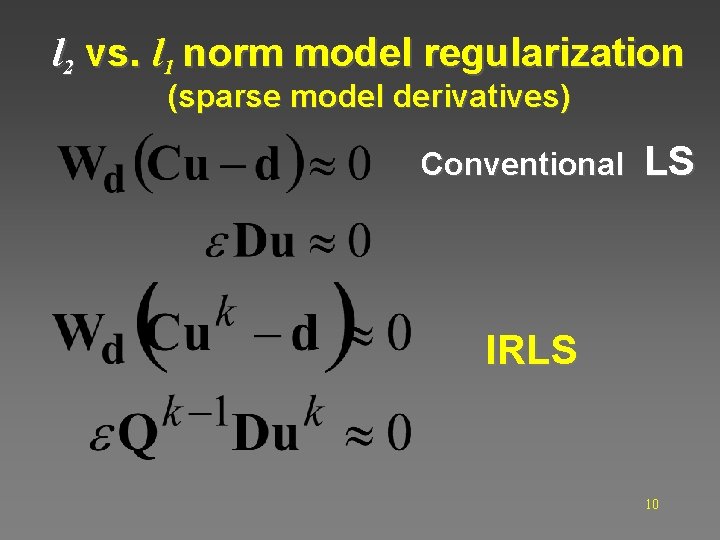
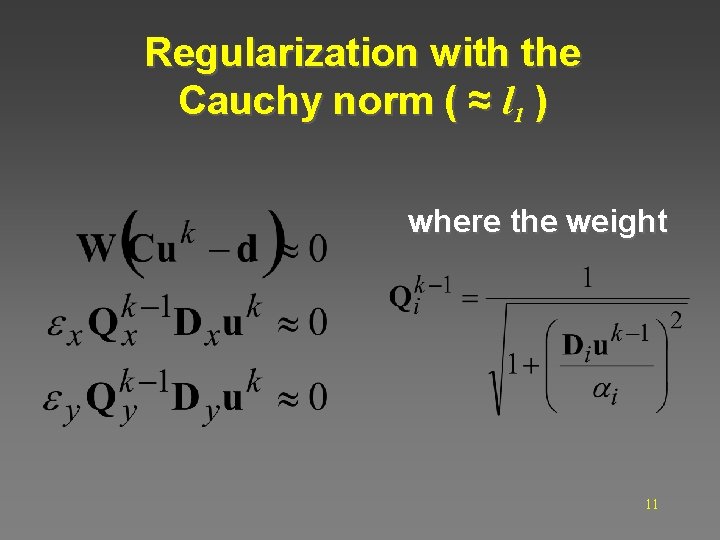
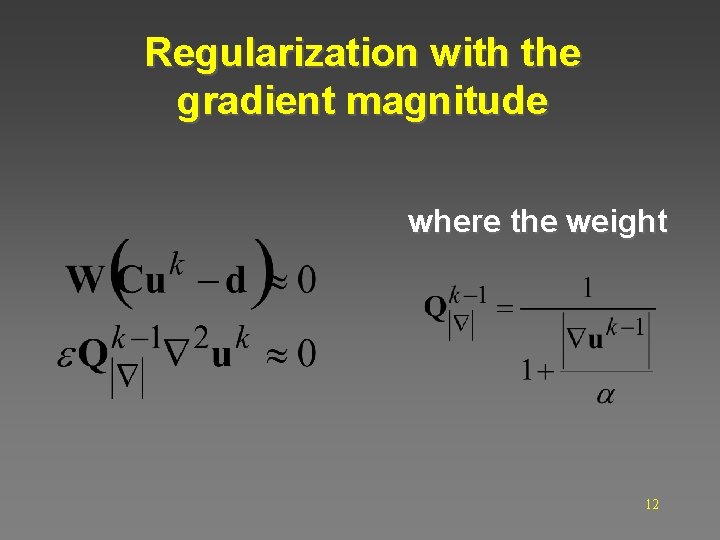
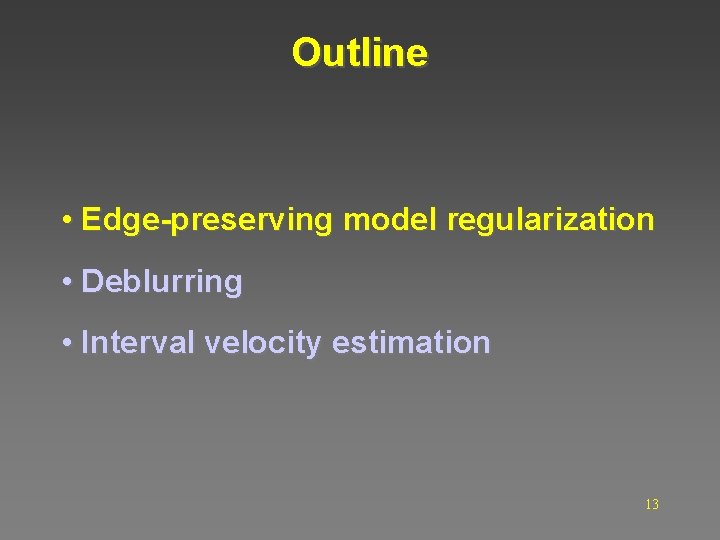
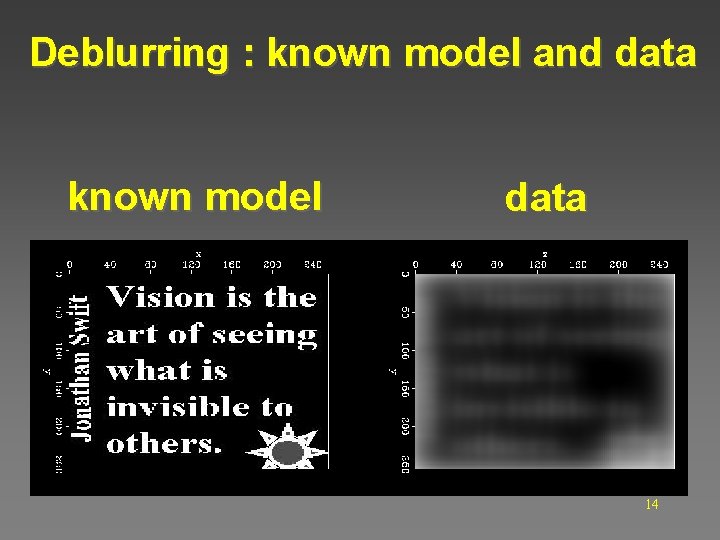
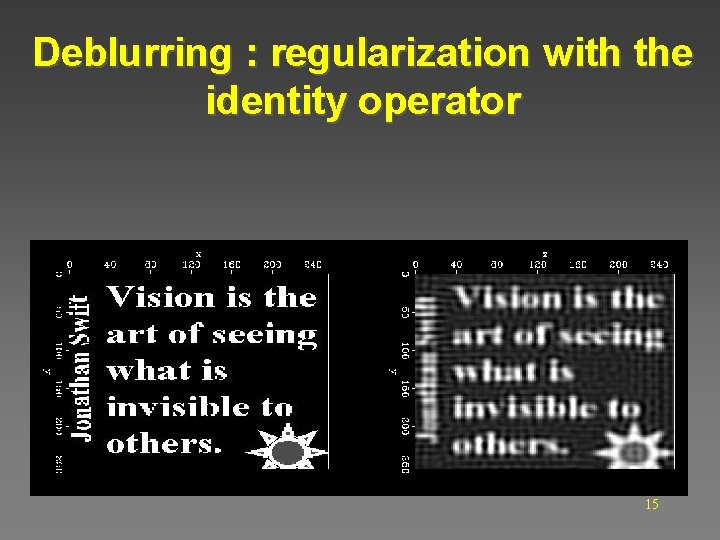
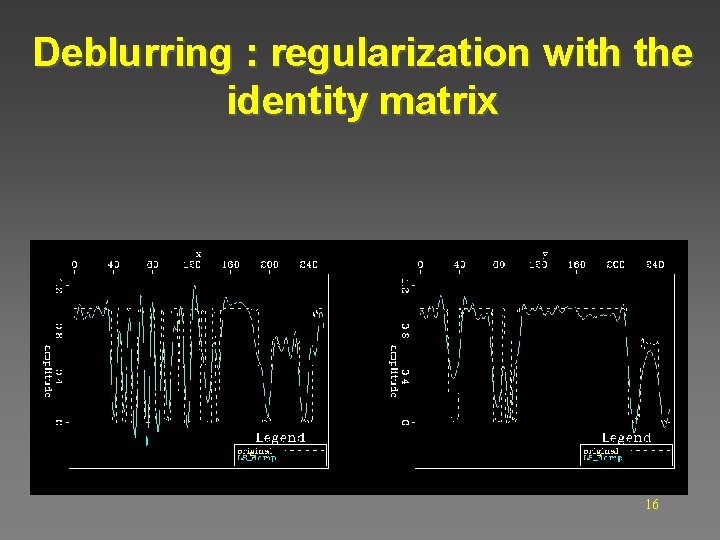
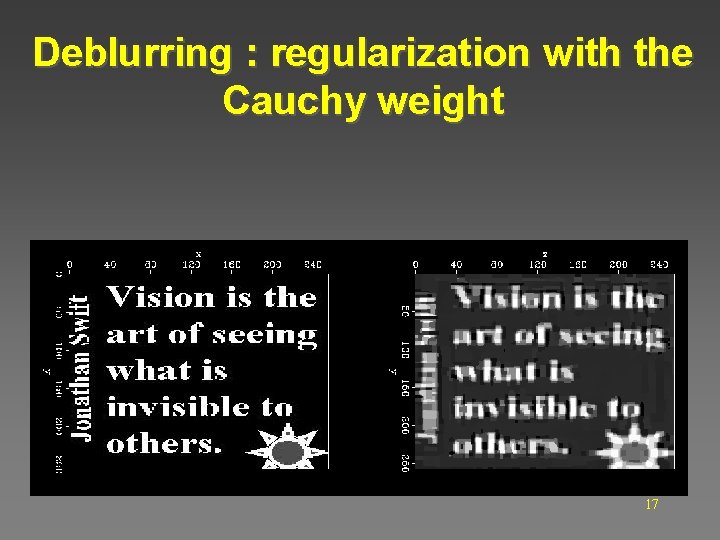
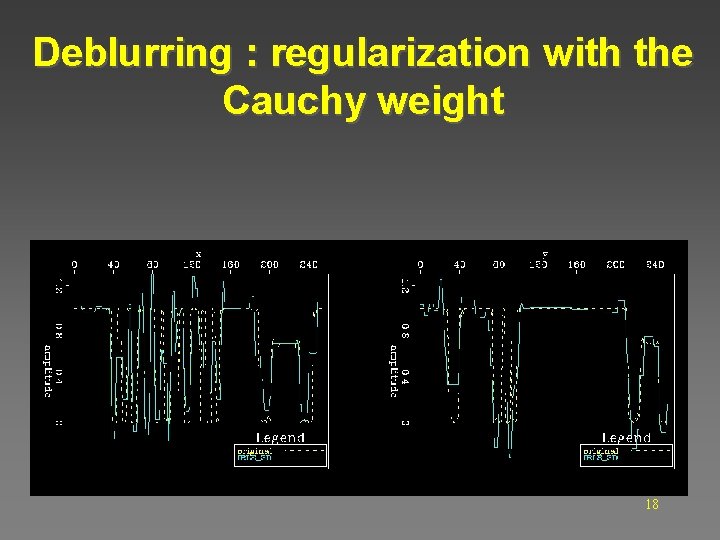
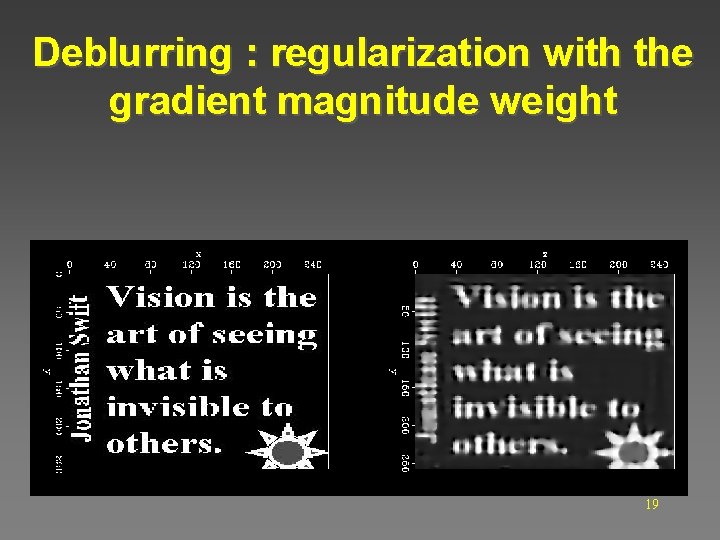
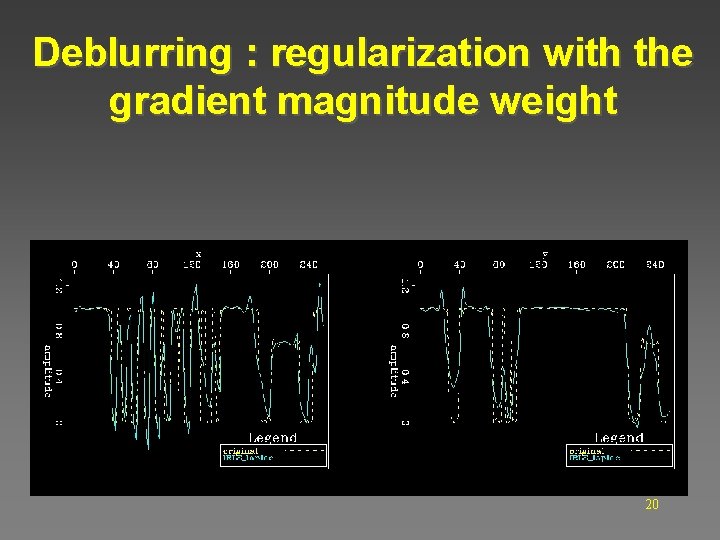
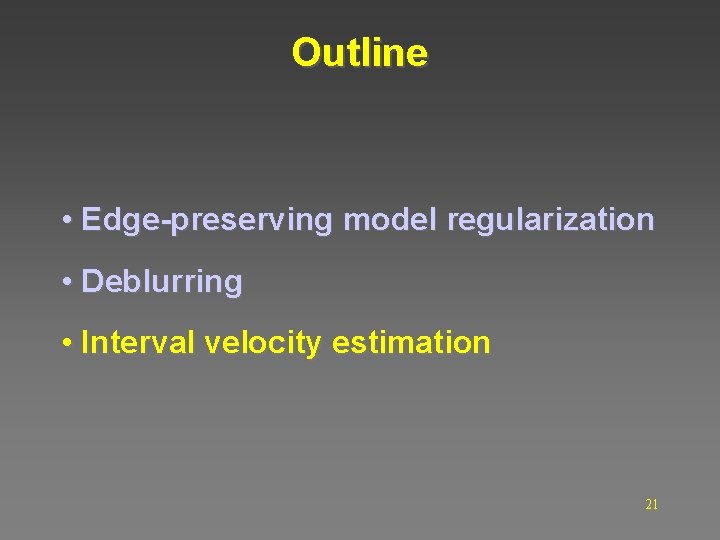
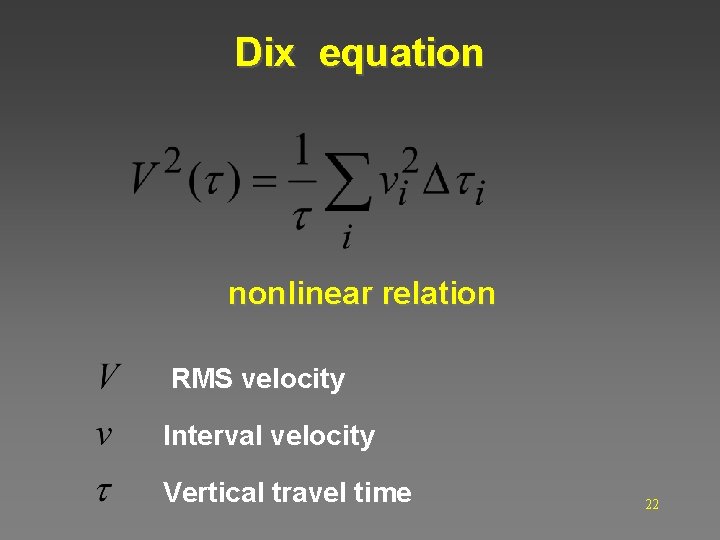
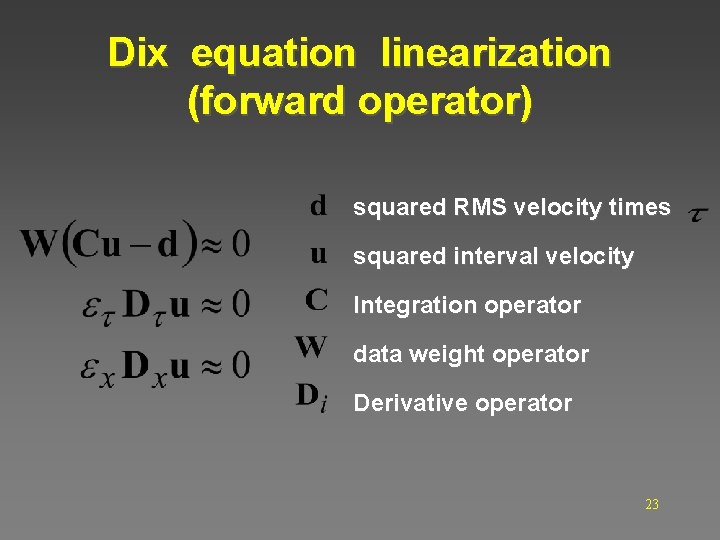
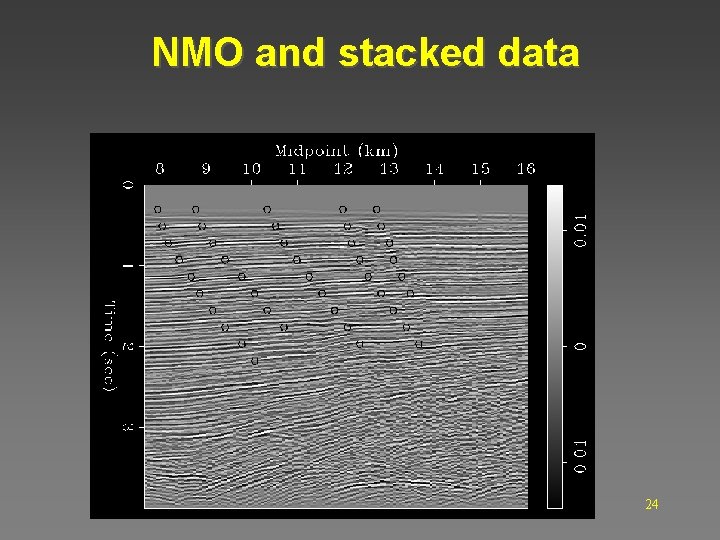
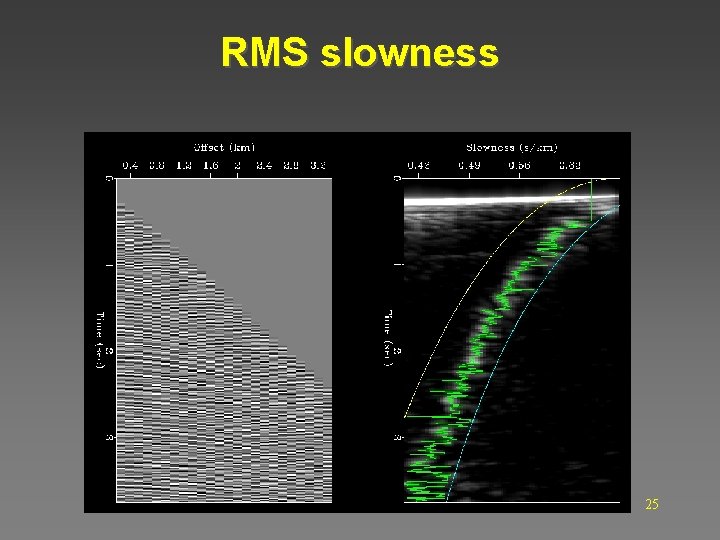
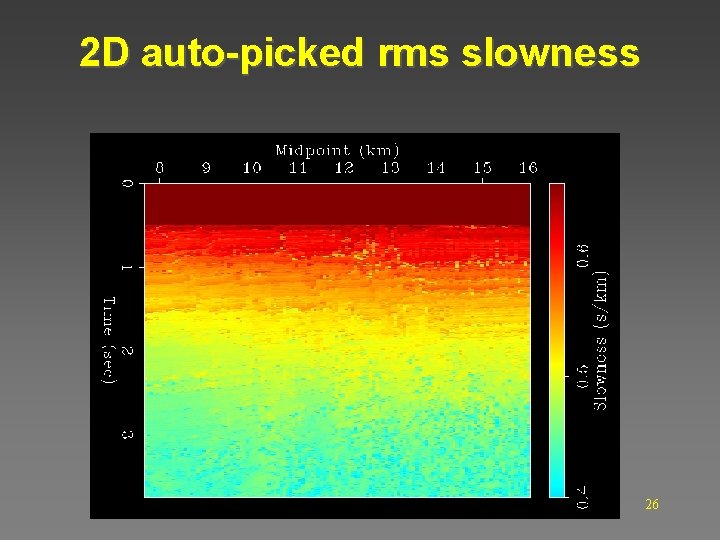
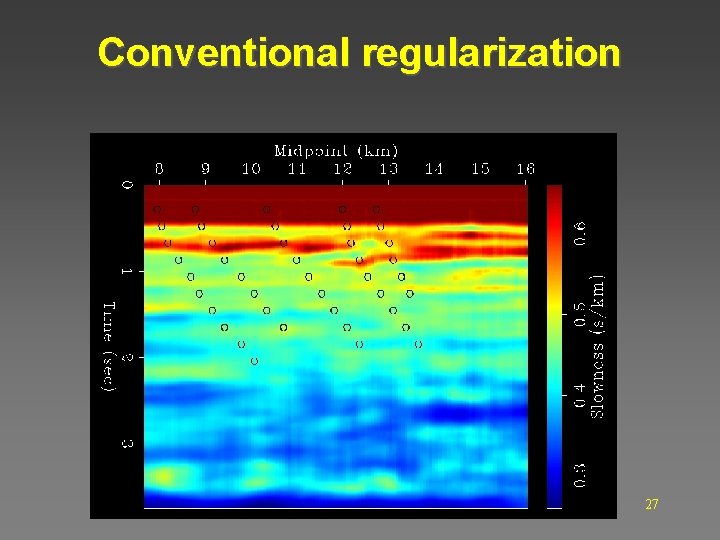
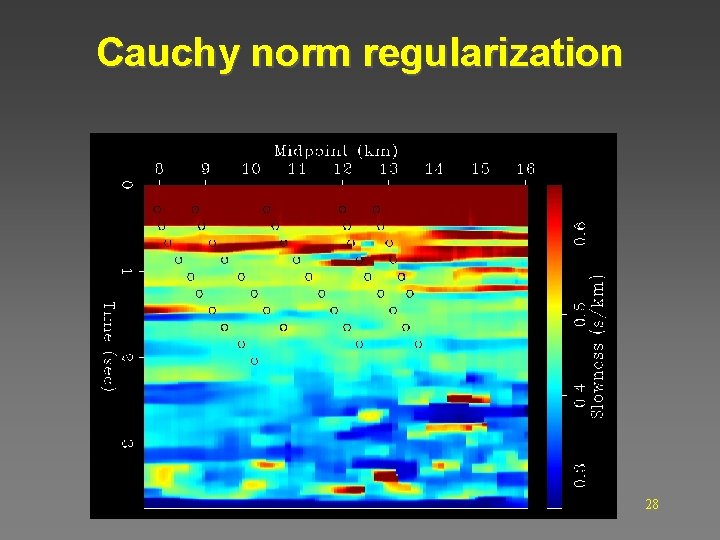
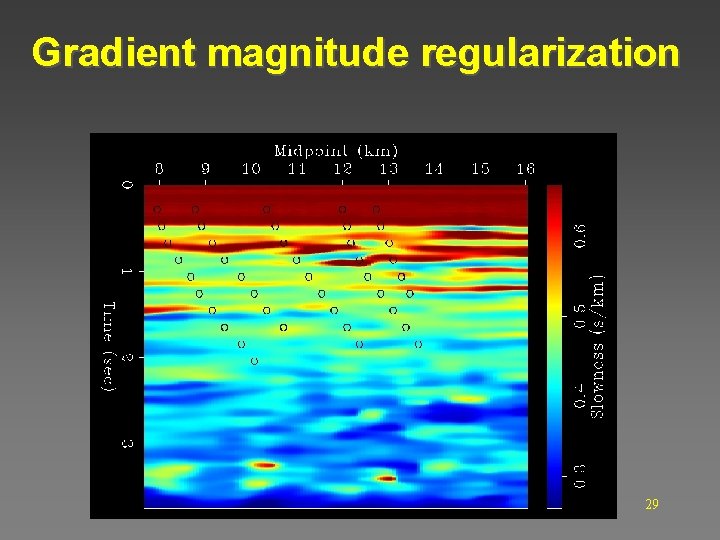
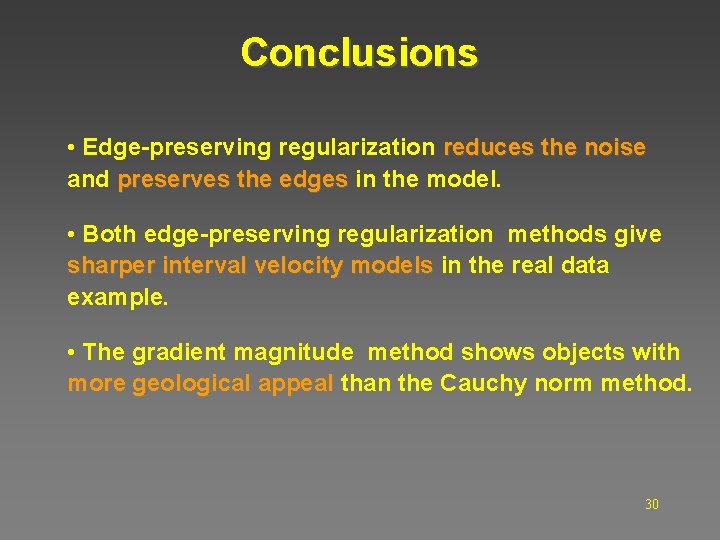
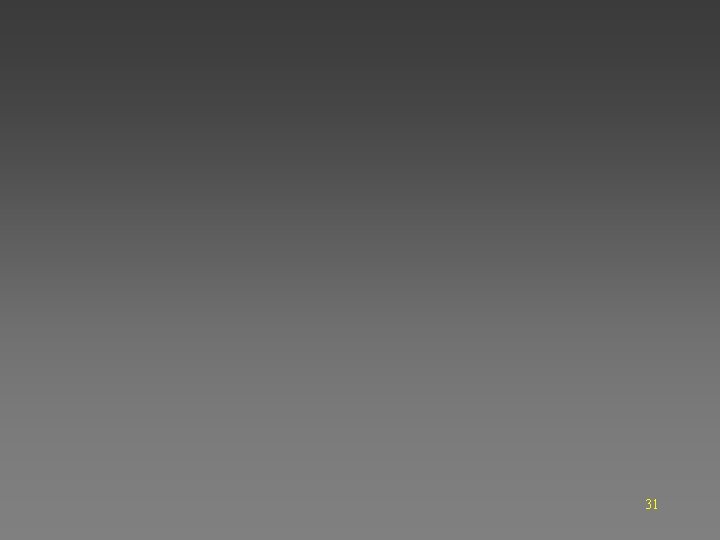
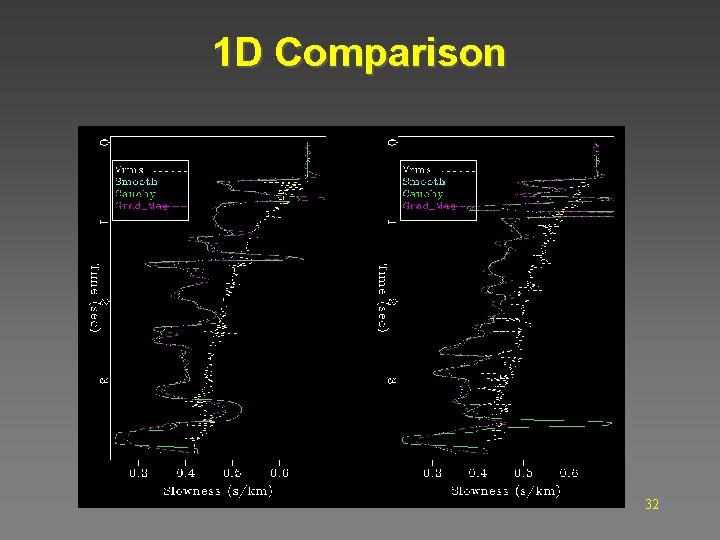
- Slides: 32
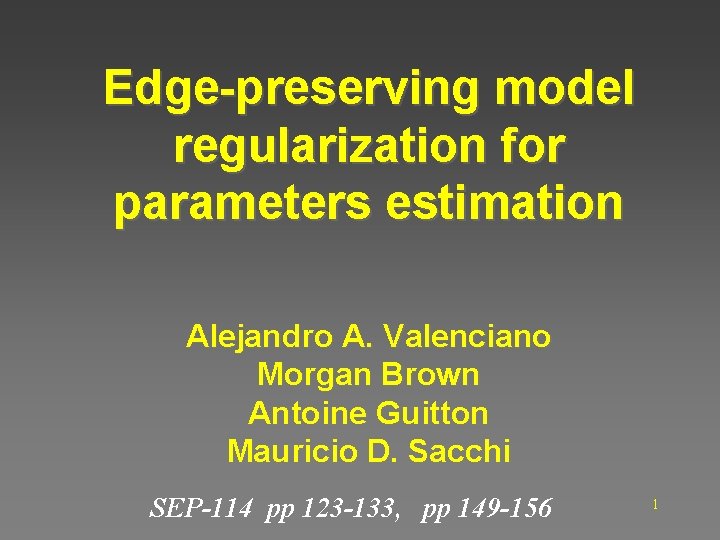
Edge-preserving model regularization for parameters estimation Alejandro A. Valenciano Morgan Brown Antoine Guitton Mauricio D. Sacchi SEP-114 pp 123 -133, pp 149 -156 1
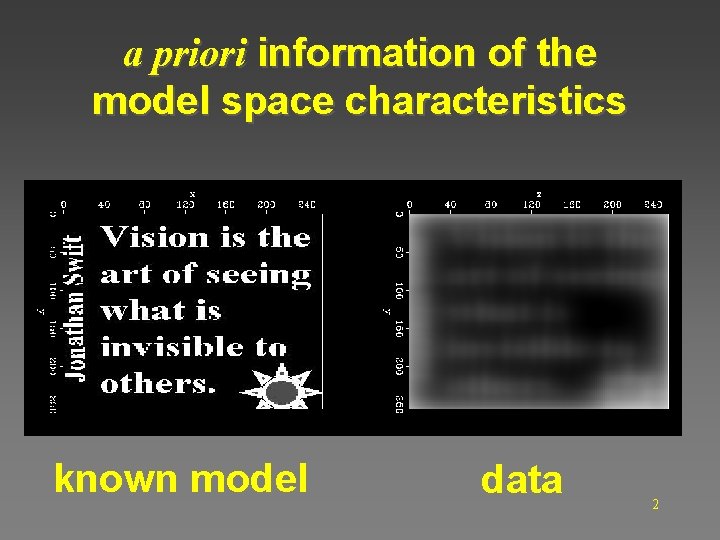
a priori information of the model space characteristics known model data 2
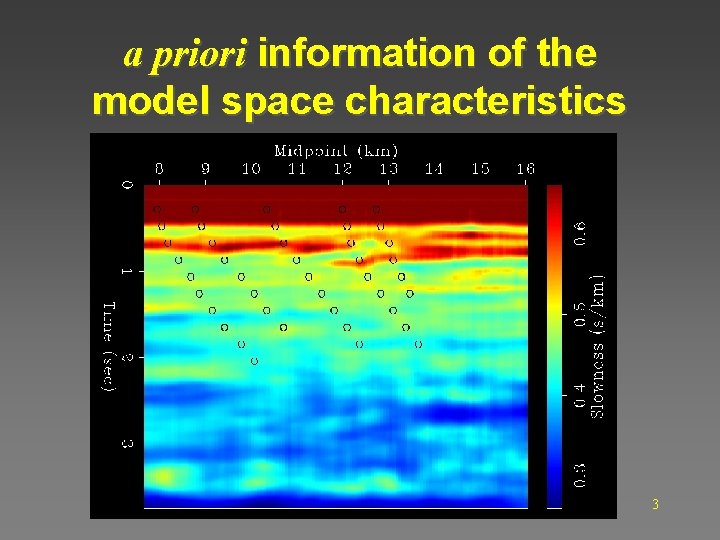
a priori information of the model space characteristics 3
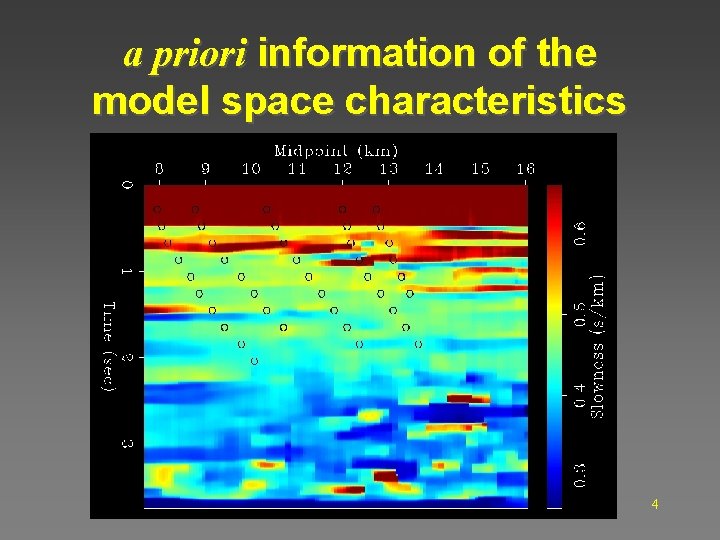
a priori information of the model space characteristics 4
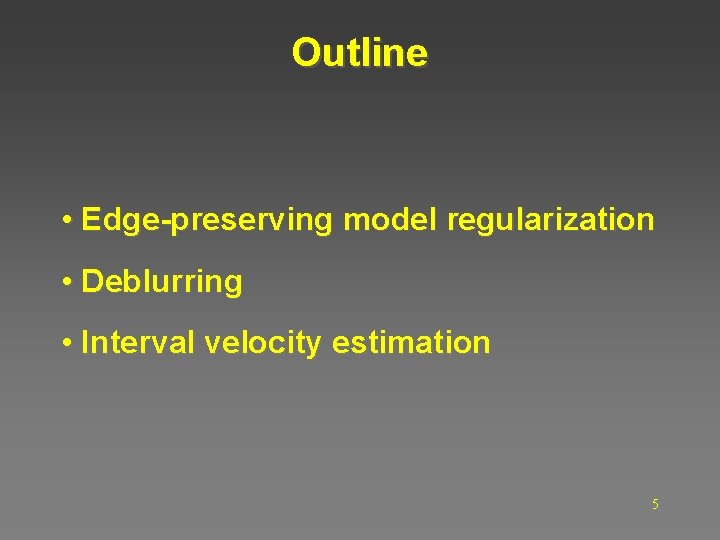
Outline • Edge-preserving model regularization • Deblurring • Interval velocity estimation 5
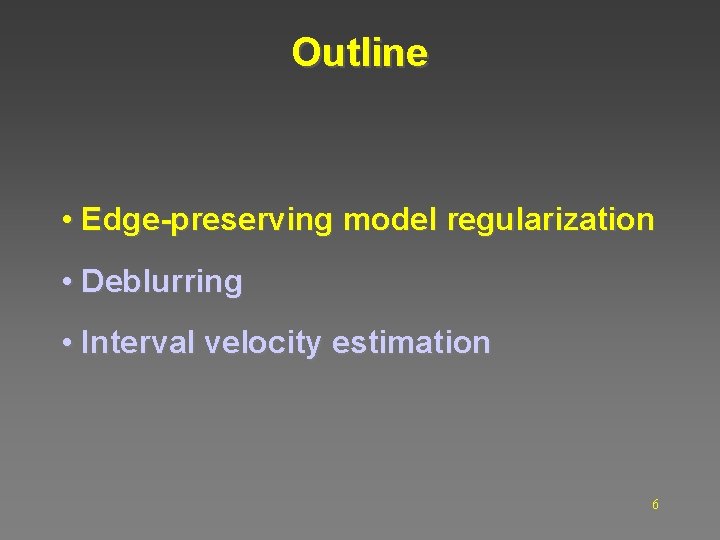
Outline • Edge-preserving model regularization • Deblurring • Interval velocity estimation 6
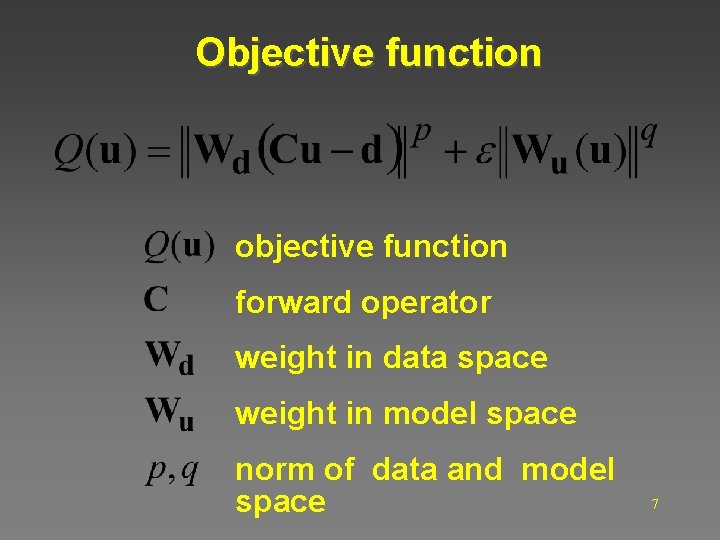
Objective function objective function forward operator weight in data space weight in model space norm of data and model space 7
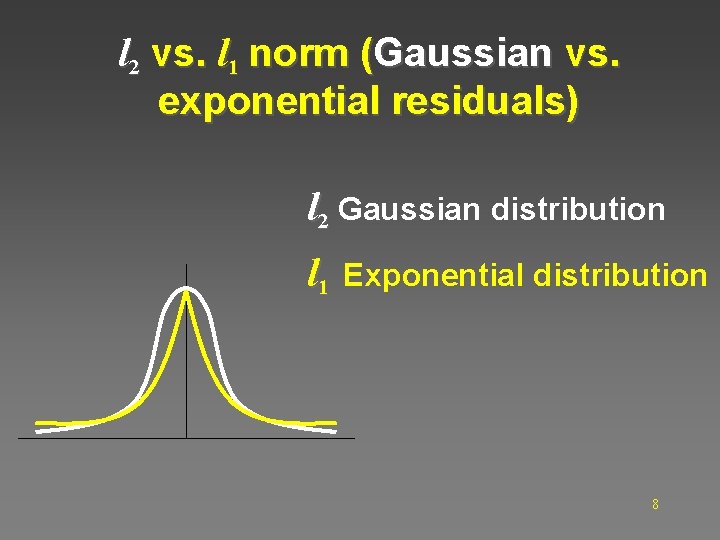
l 2 vs. l 1 norm (Gaussian vs. exponential residuals) l 2 Gaussian distribution l 1 Exponential distribution 8
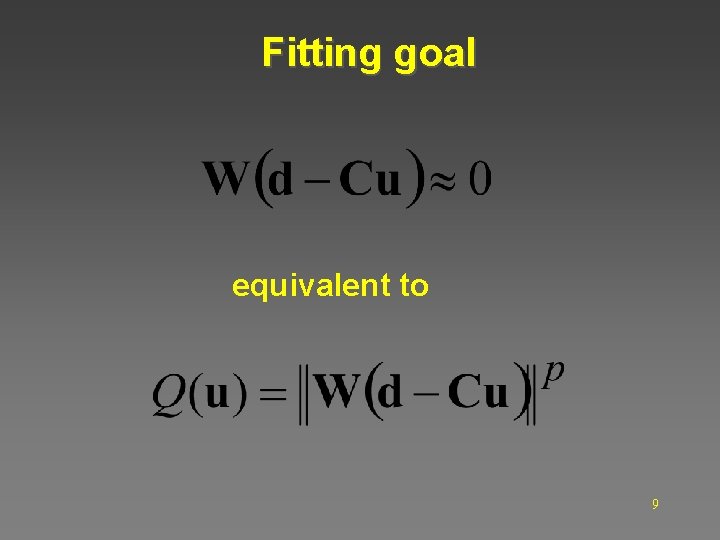
Fitting goal equivalent to 9
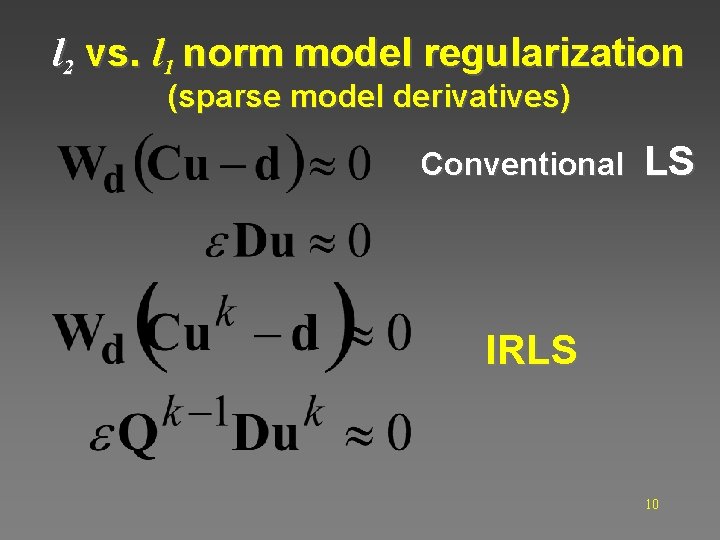
l 2 vs. l 1 norm model regularization (sparse model derivatives) Conventional LS IRLS 10
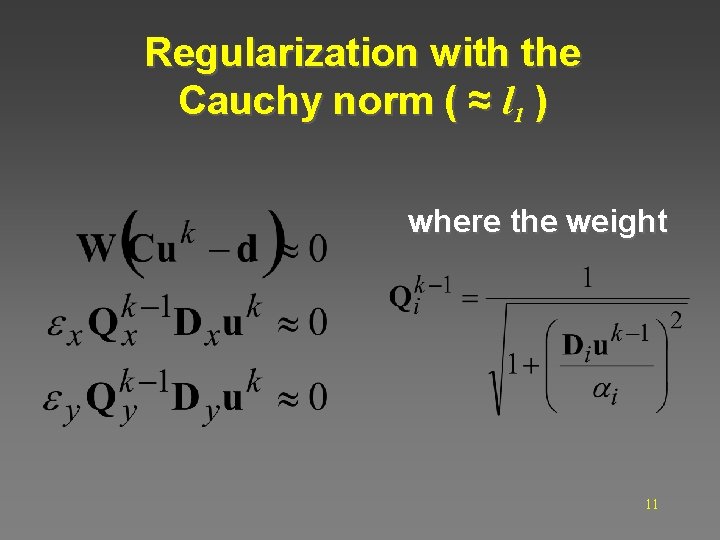
Regularization with the Cauchy norm ( ≈ l 1 ) where the weight 11
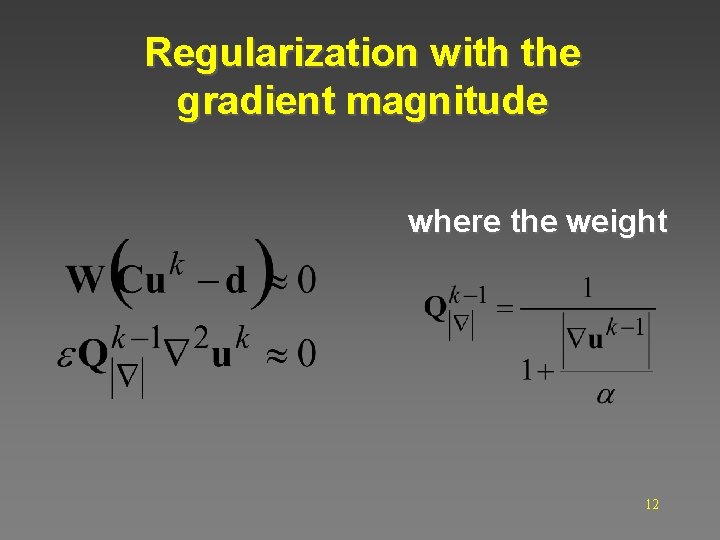
Regularization with the gradient magnitude where the weight 12
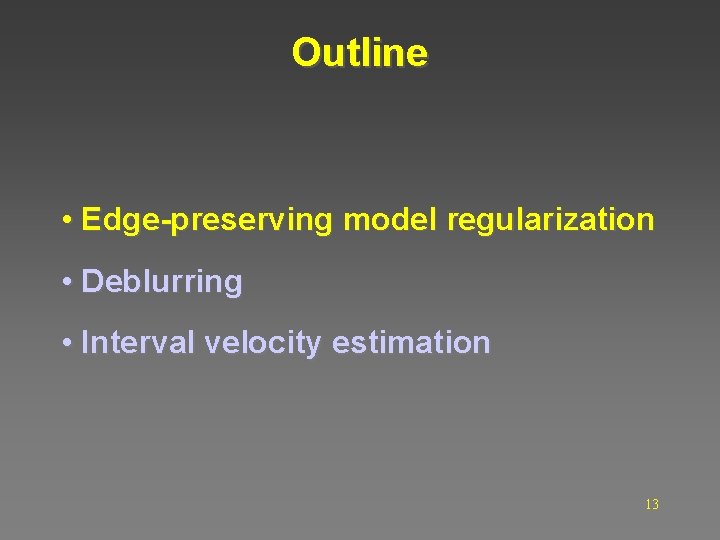
Outline • Edge-preserving model regularization • Deblurring • Interval velocity estimation 13
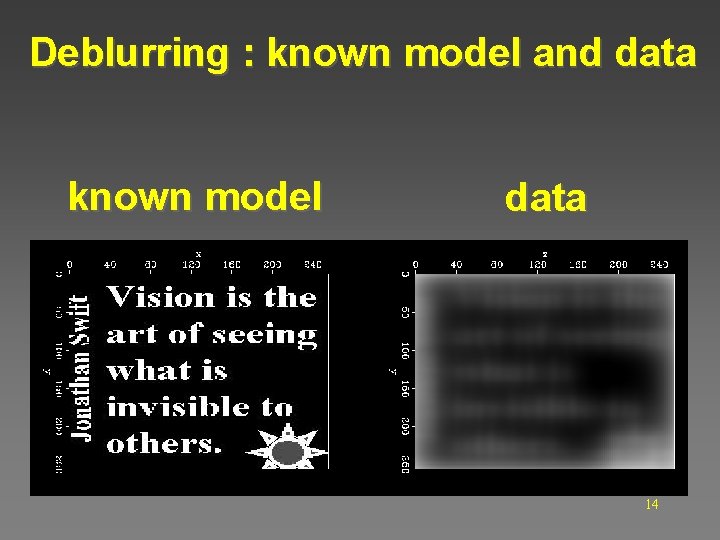
Deblurring : known model and data known model data 14
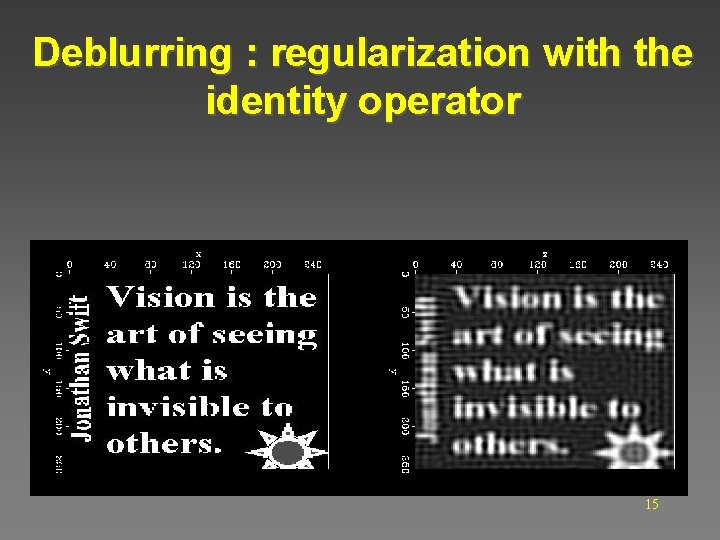
Deblurring : regularization with the identity operator 15
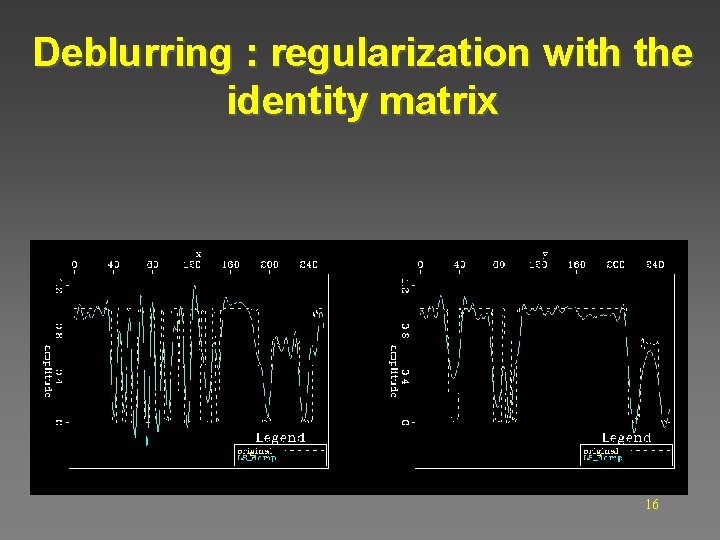
Deblurring : regularization with the identity matrix 16
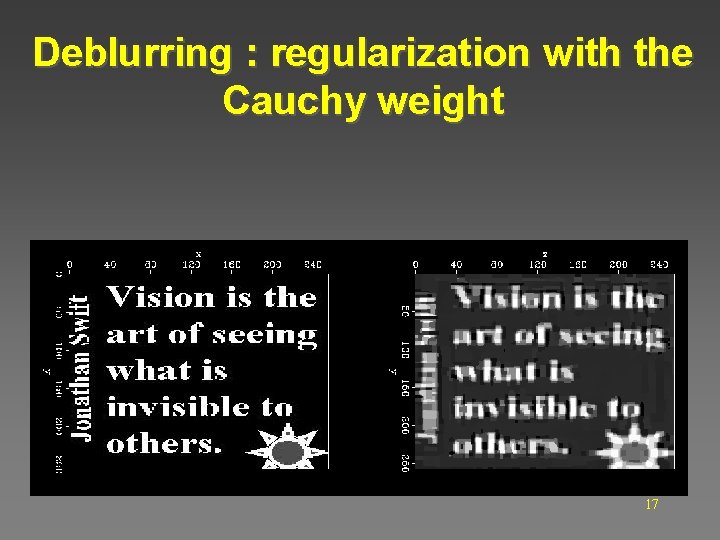
Deblurring : regularization with the Cauchy weight 17
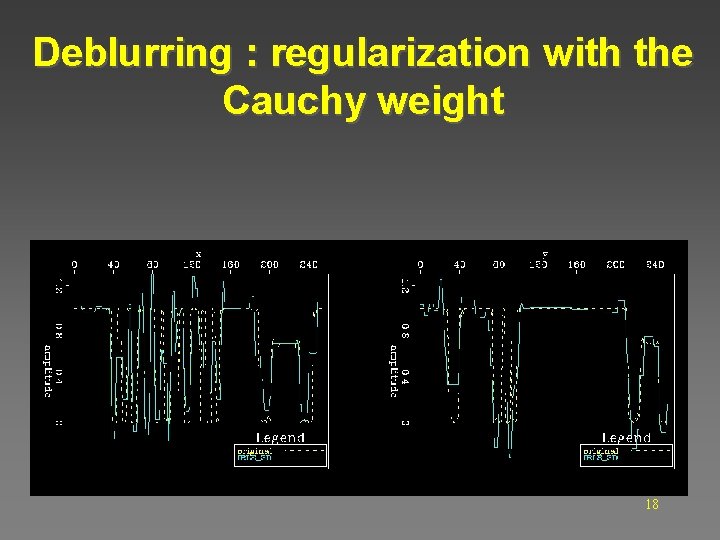
Deblurring : regularization with the Cauchy weight 18
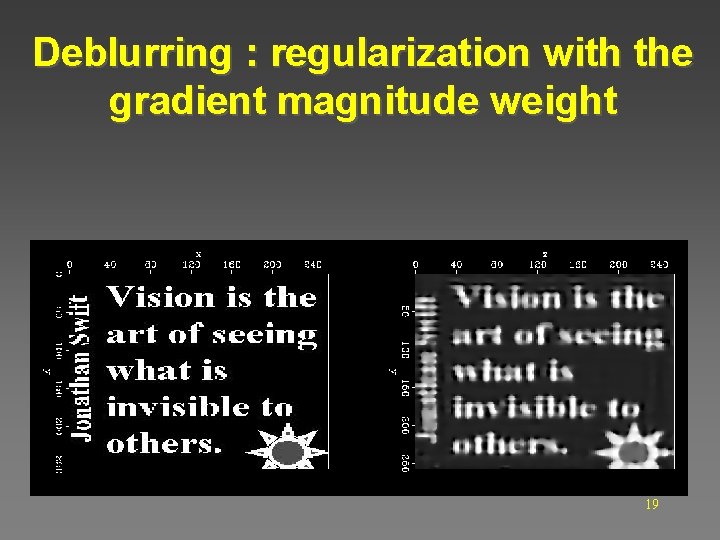
Deblurring : regularization with the gradient magnitude weight 19
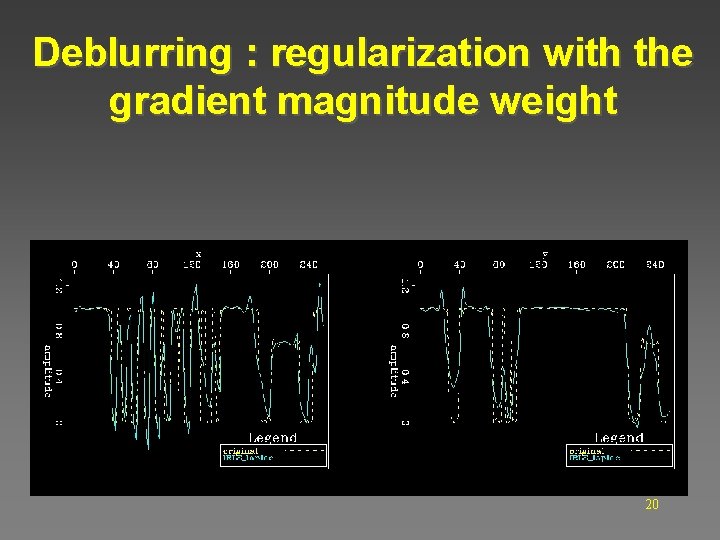
Deblurring : regularization with the gradient magnitude weight 20
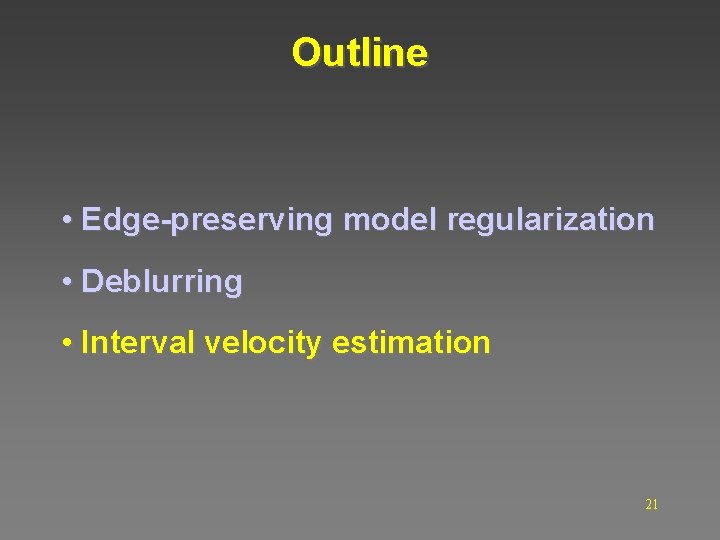
Outline • Edge-preserving model regularization • Deblurring • Interval velocity estimation 21
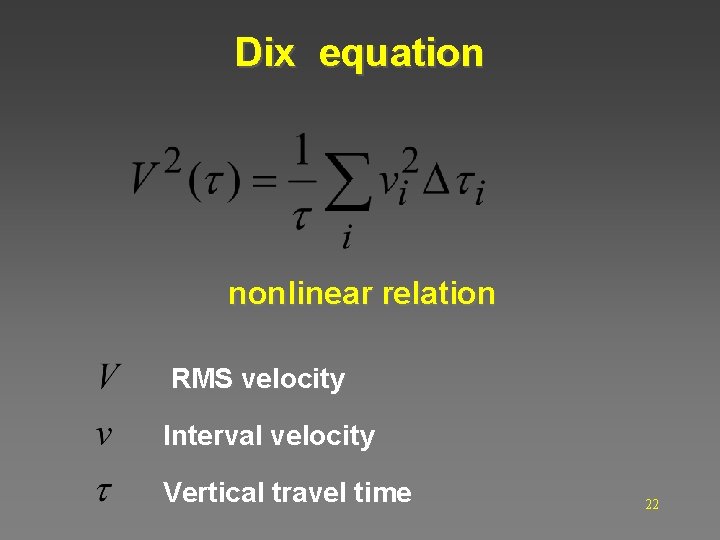
Dix equation nonlinear relation RMS velocity Interval velocity Vertical travel time 22
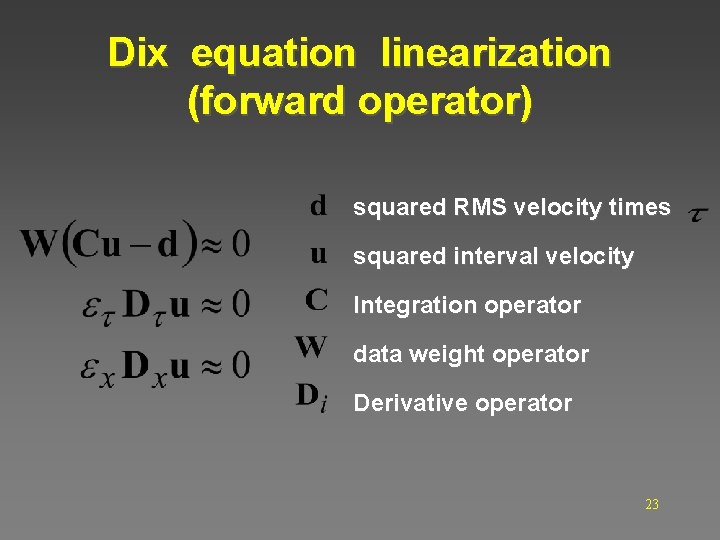
Dix equation linearization (forward operator) squared RMS velocity times squared interval velocity Integration operator data weight operator Derivative operator 23
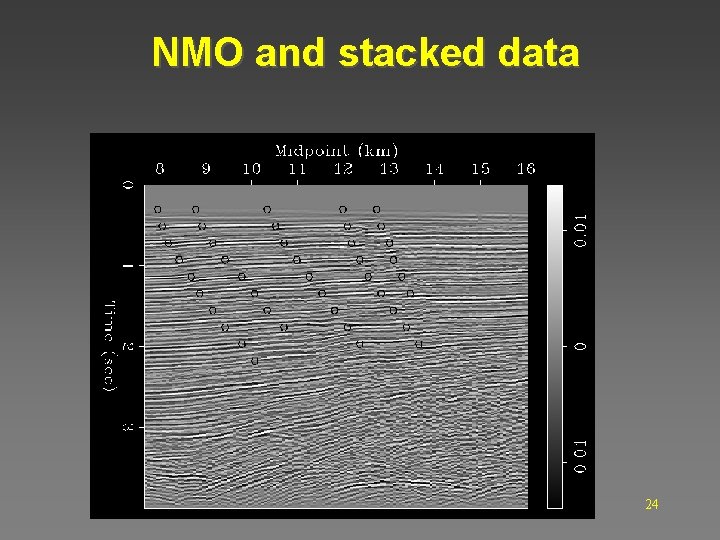
NMO and stacked data 24
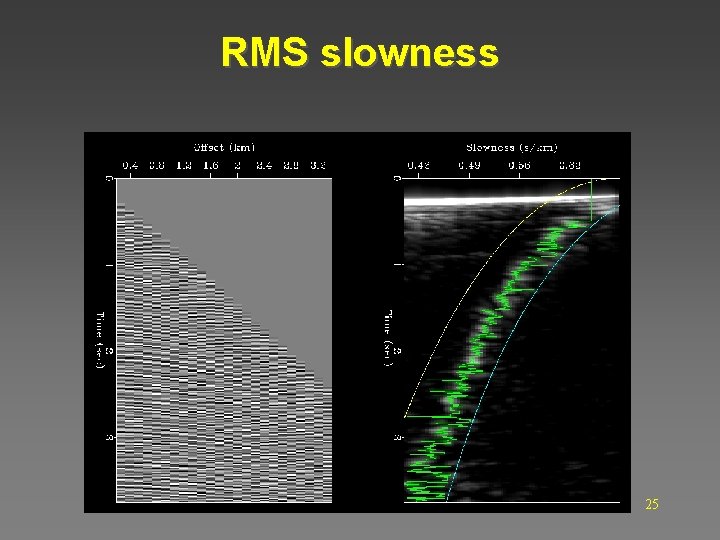
RMS slowness 25
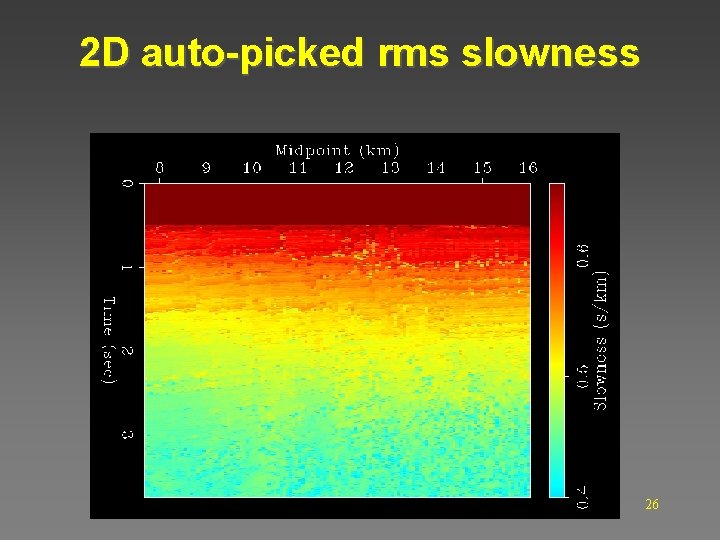
2 D auto-picked rms slowness 26
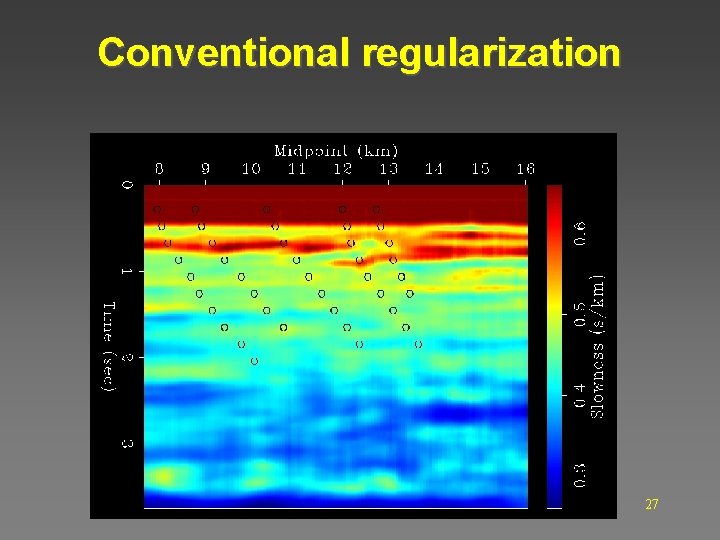
Conventional regularization 27
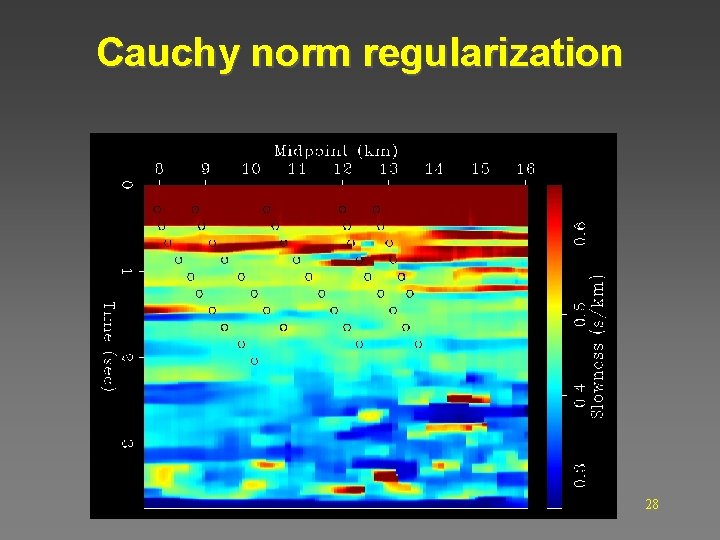
Cauchy norm regularization 28
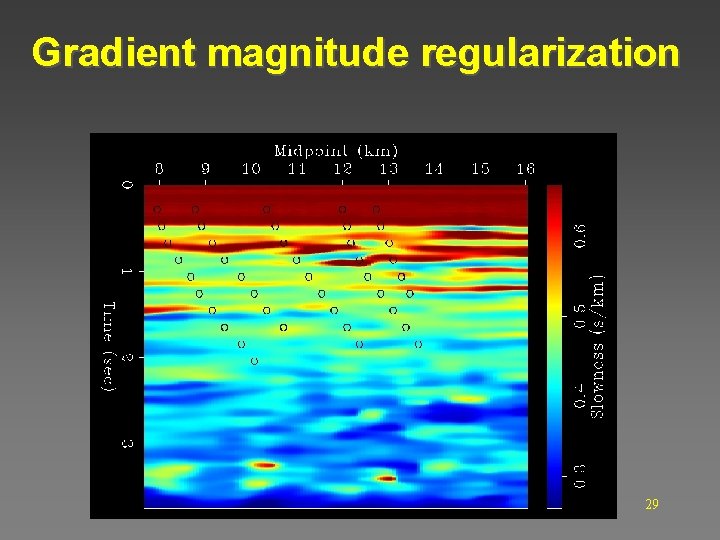
Gradient magnitude regularization 29
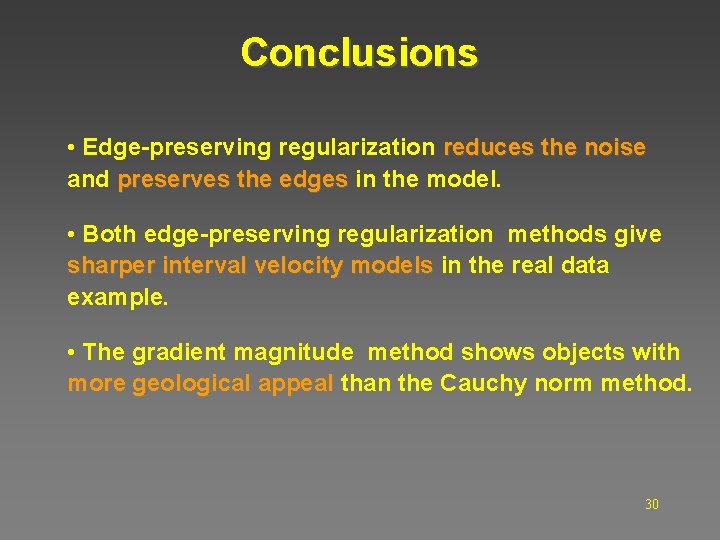
Conclusions • Edge-preserving regularization reduces the noise and preserves the edges in the model. • Both edge-preserving regularization methods give sharper interval velocity models in the real data example. • The gradient magnitude method shows objects with more geological appeal than the Cauchy norm method. 30
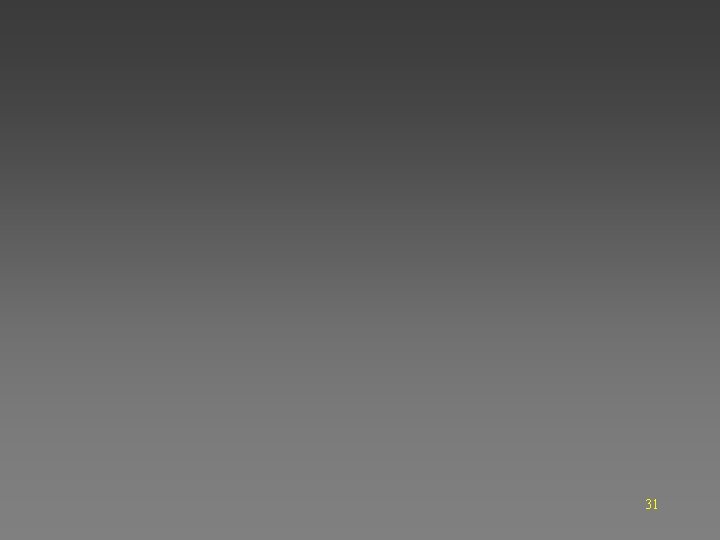
31
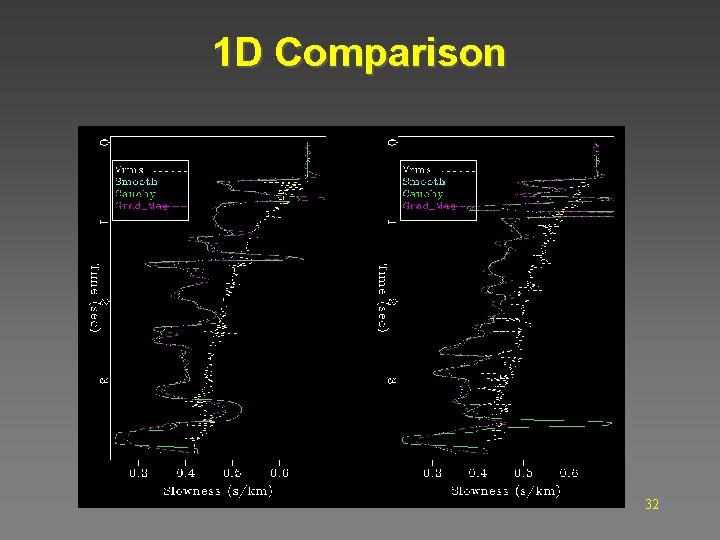
1 D Comparison 32
Cutout regularization
Logistic regression andrew ng
Graph laplacian regularization
Nn regularization
Cocomo model cost estimation
Cocomo cost estimation model
Detailed cocomo model formula
Iso 22301 utbildning
Typiska novell drag
Tack för att ni lyssnade bild
Vad står k.r.å.k.a.n för
Shingelfrisyren
En lathund för arbete med kontinuitetshantering
Särskild löneskatt för pensionskostnader
Tidbok
Sura för anatom
Förklara densitet för barn
Datorkunskap för nybörjare
Boverket ka
Tes debattartikel
Delegerande ledarskap
Nyckelkompetenser för livslångt lärande
Påbyggnader för flakfordon
Tryck formel
Publik sektor
Jag har gått inunder stjärnor text
Presentera för publik crossboss
Teckenspråk minoritetsspråk argument
Vem räknas som jude
Klassificeringsstruktur för kommunala verksamheter
Fimbrietratt
Claes martinsson
Cks