Chapter 9 Regression Wisdom math 2200 Sifting residuals
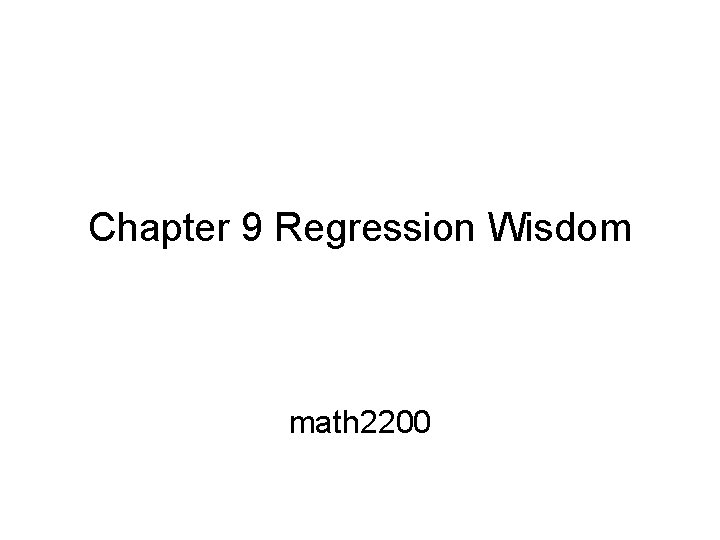
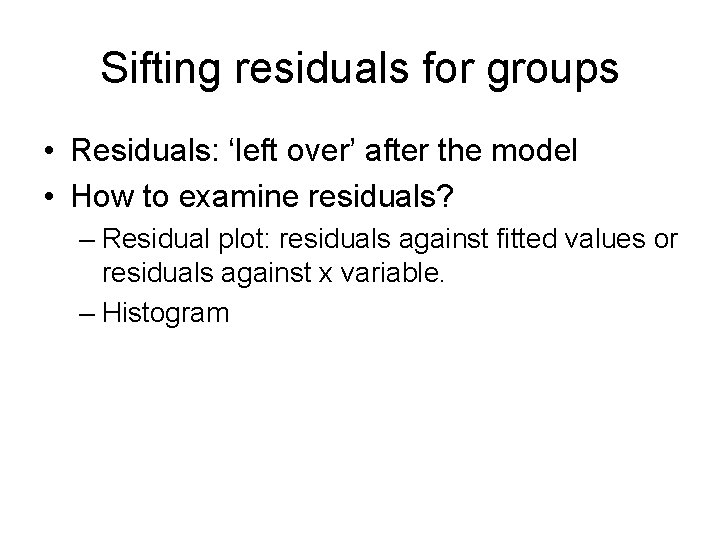
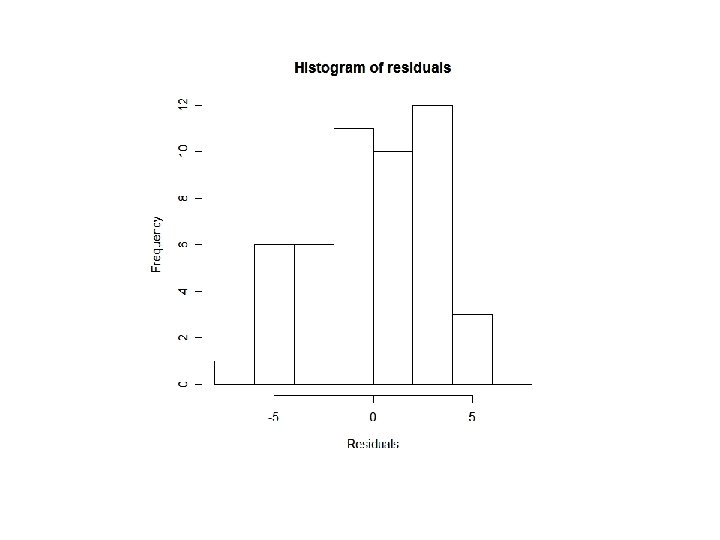
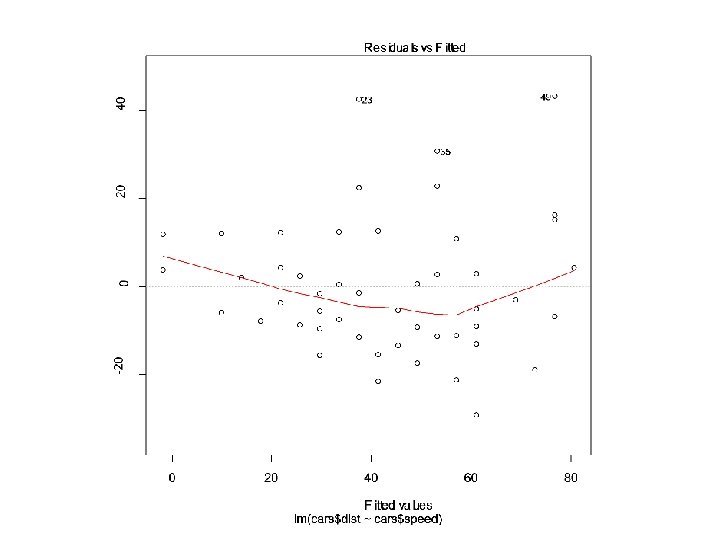
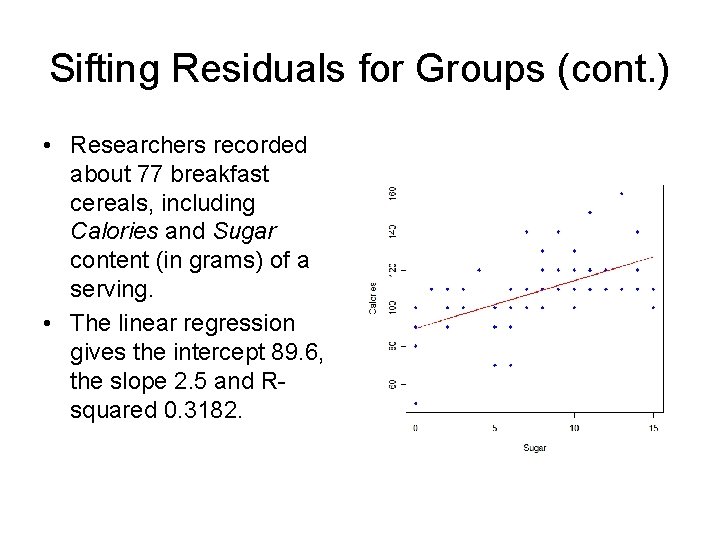
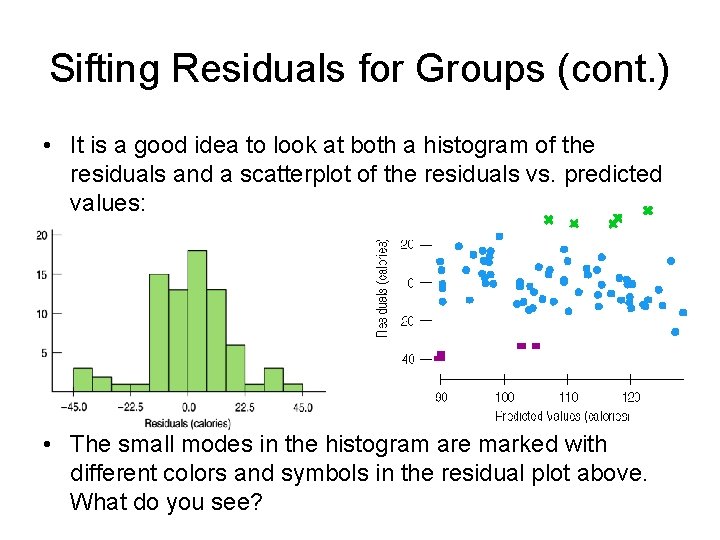
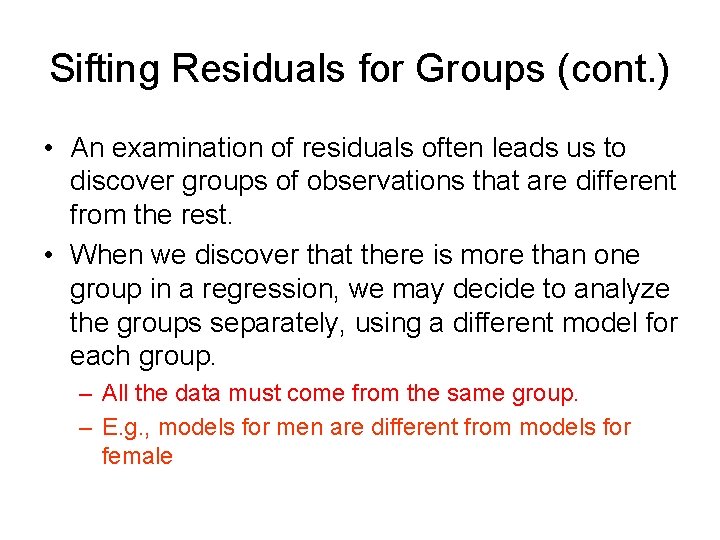
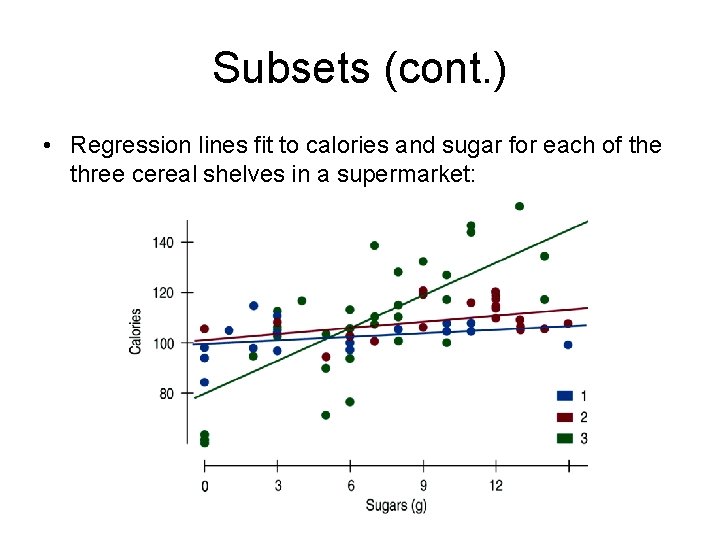
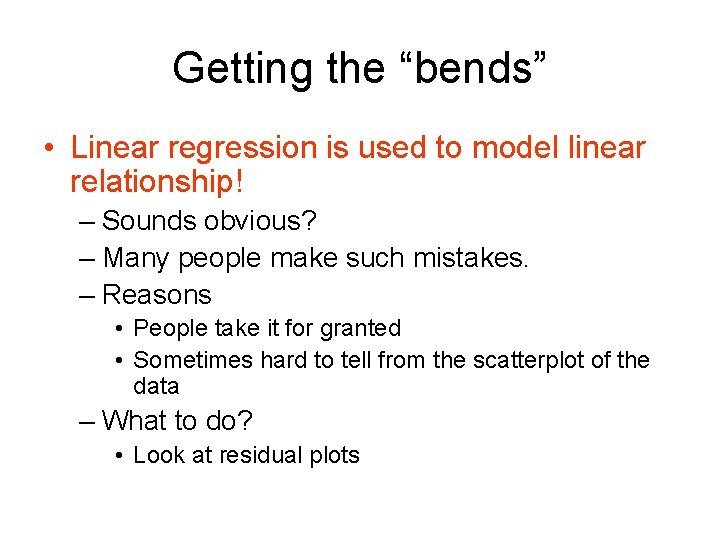
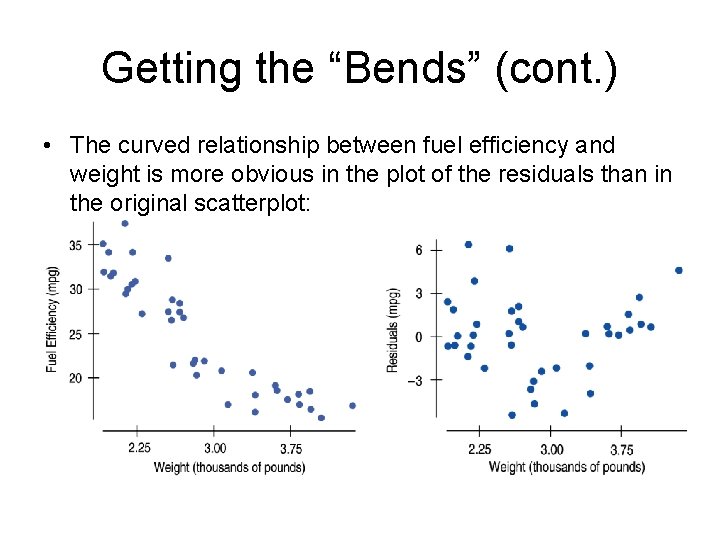
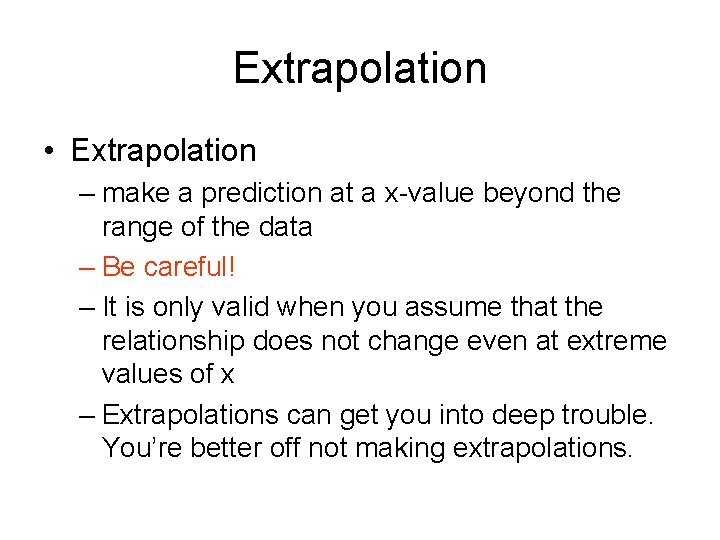
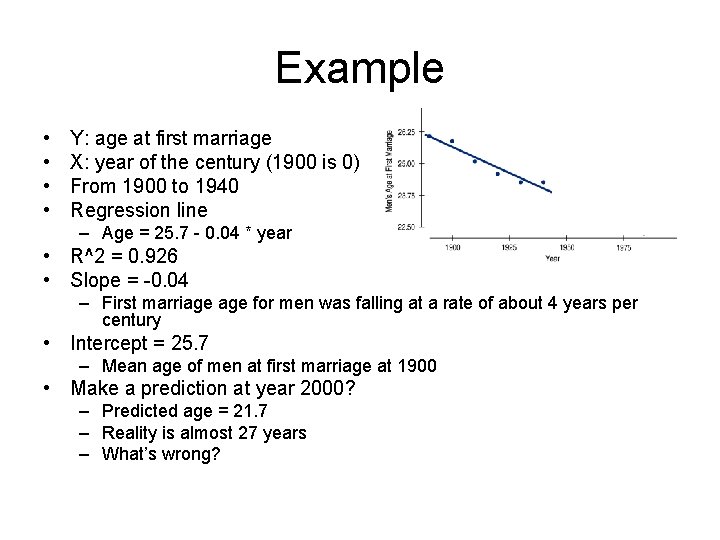
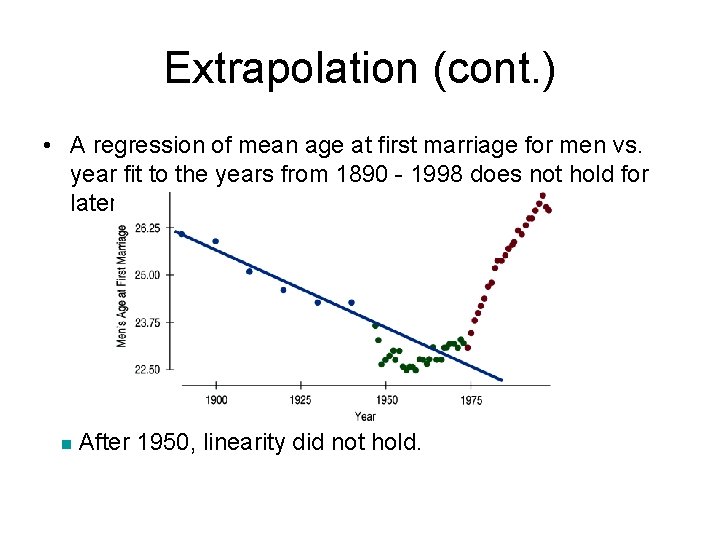
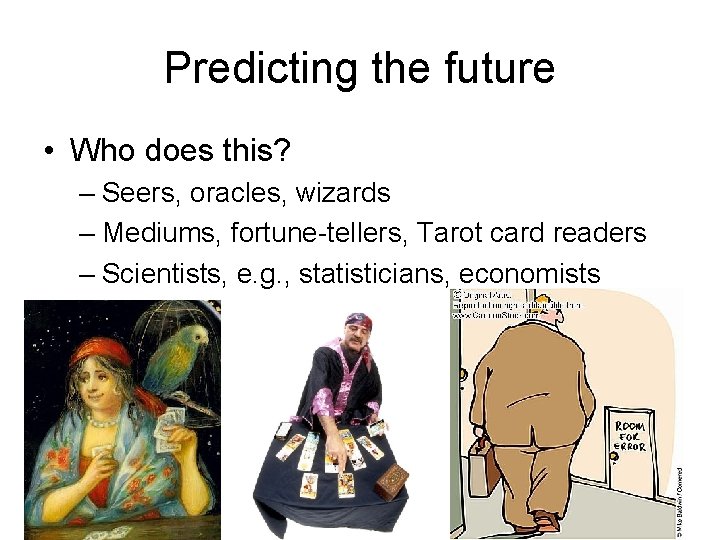
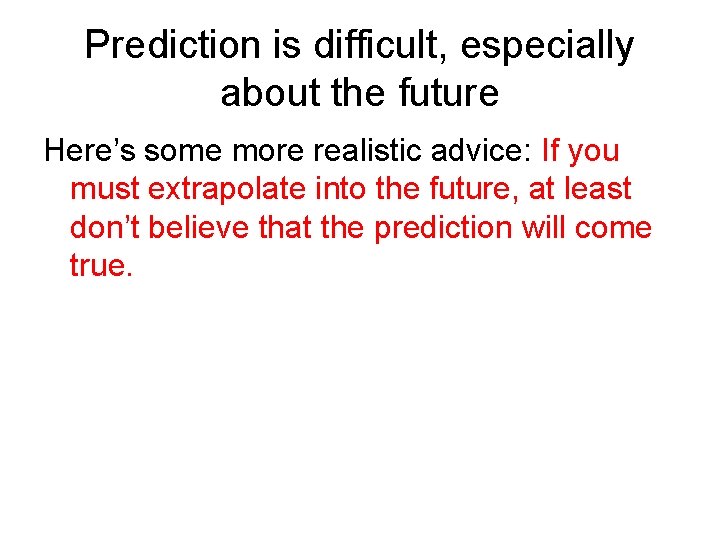
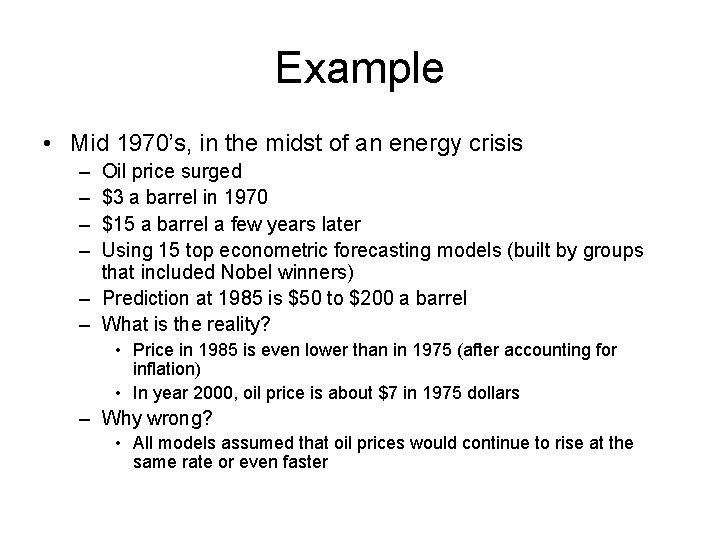
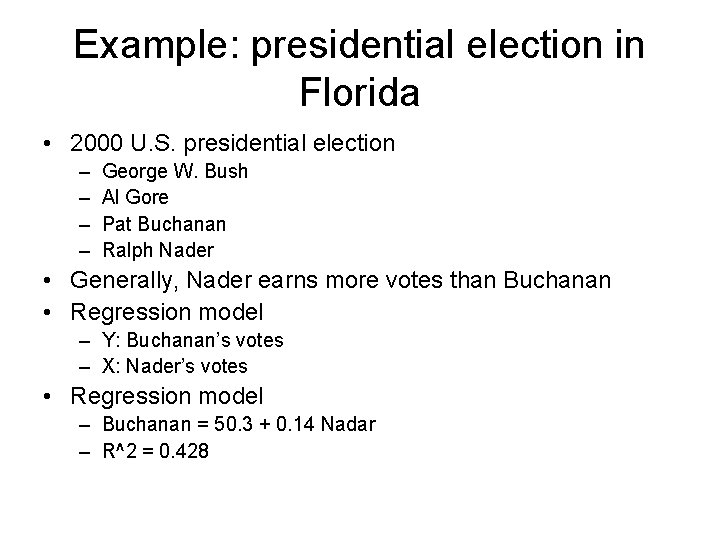
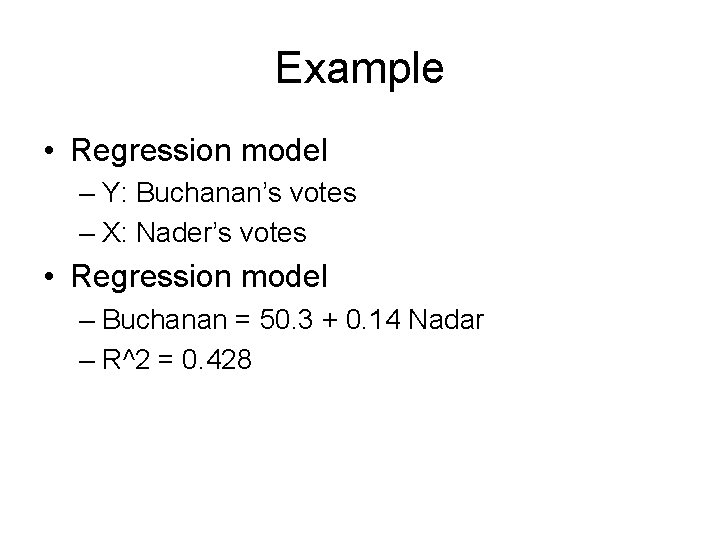
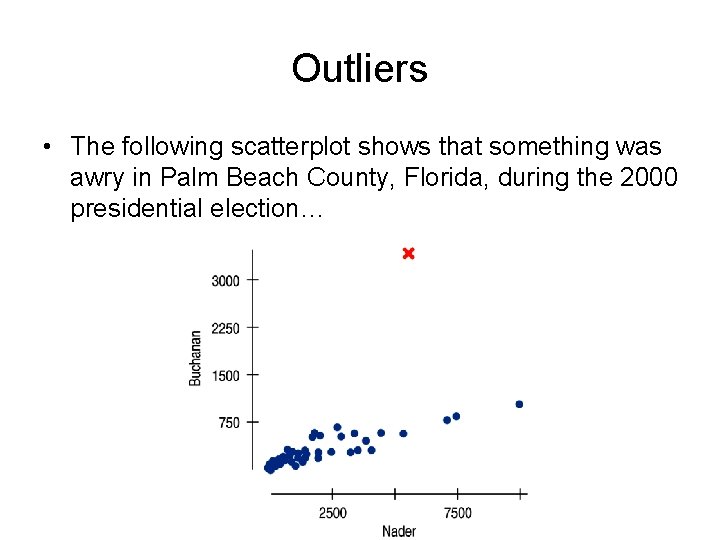
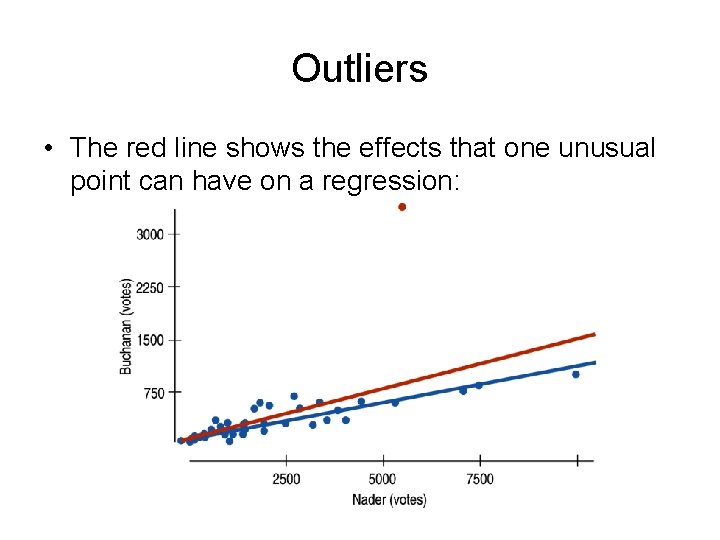
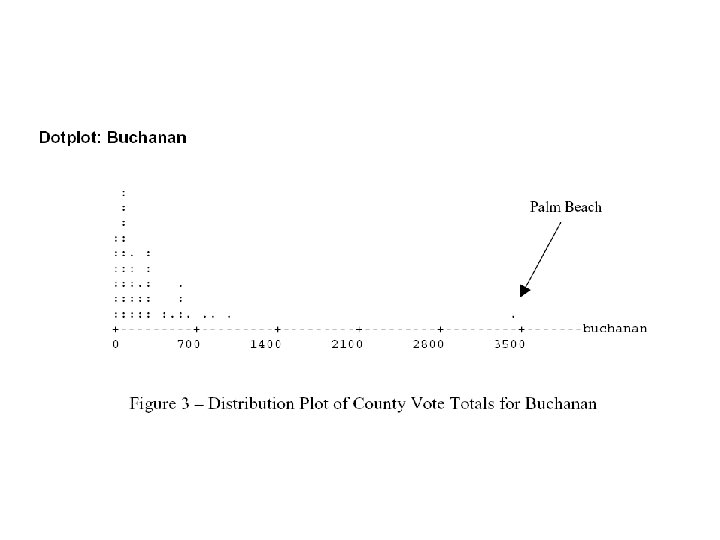
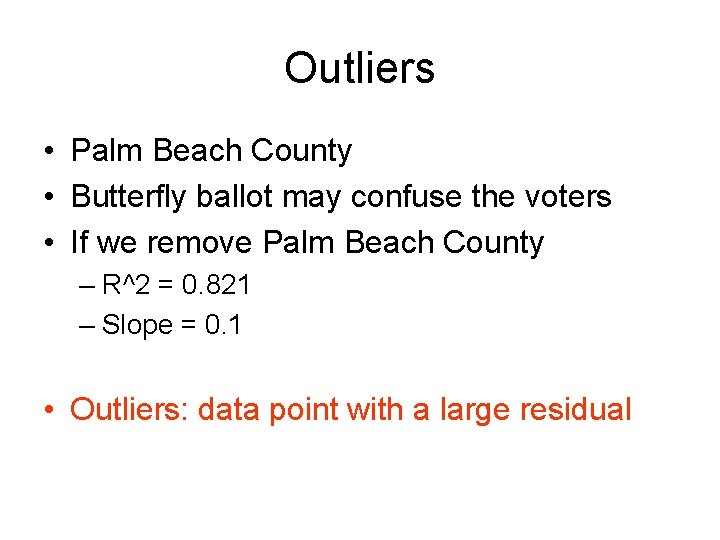
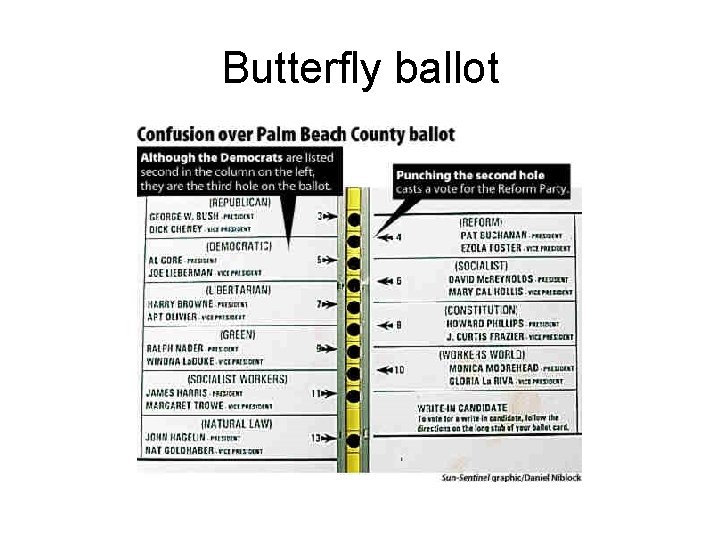
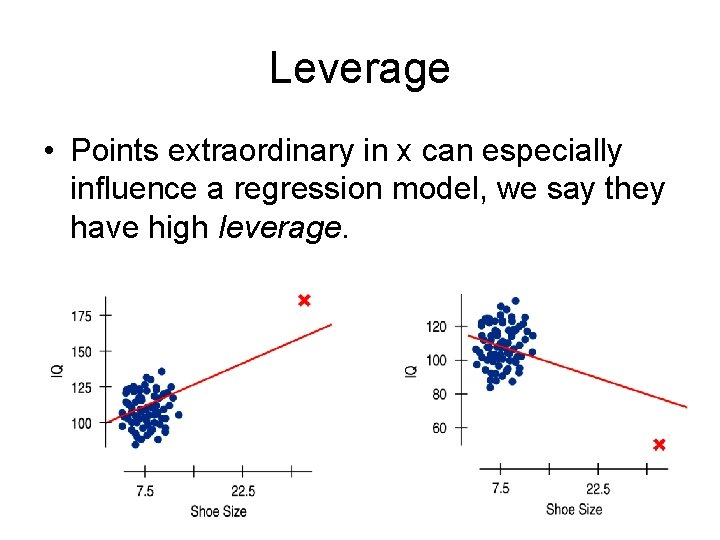
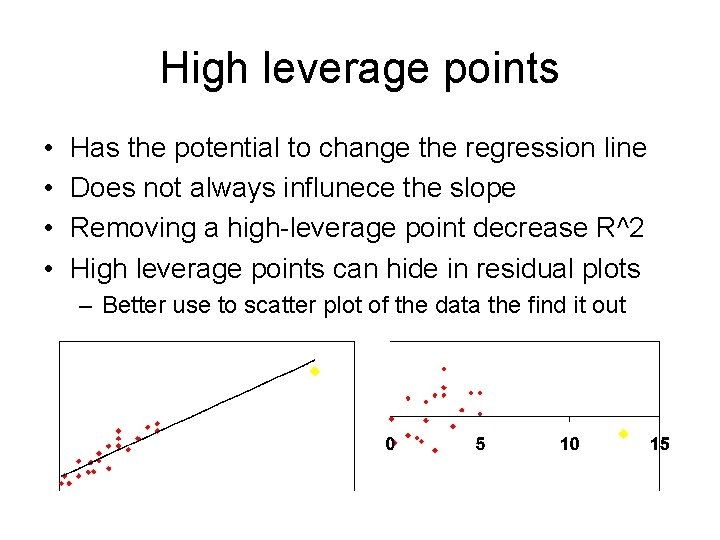
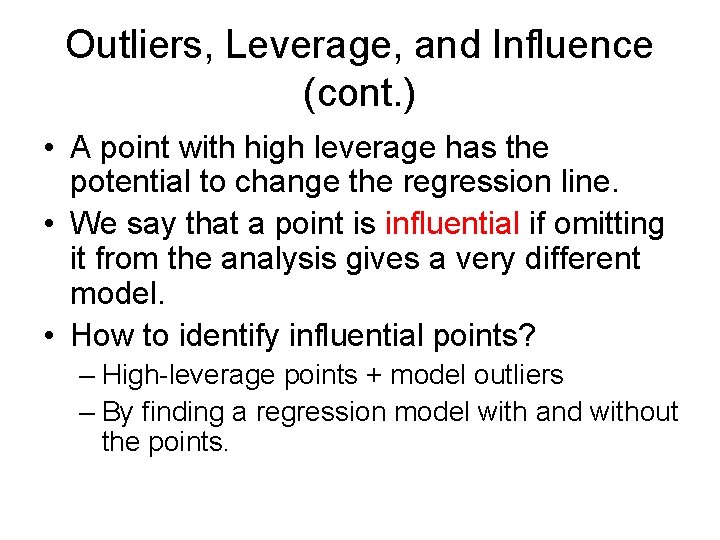
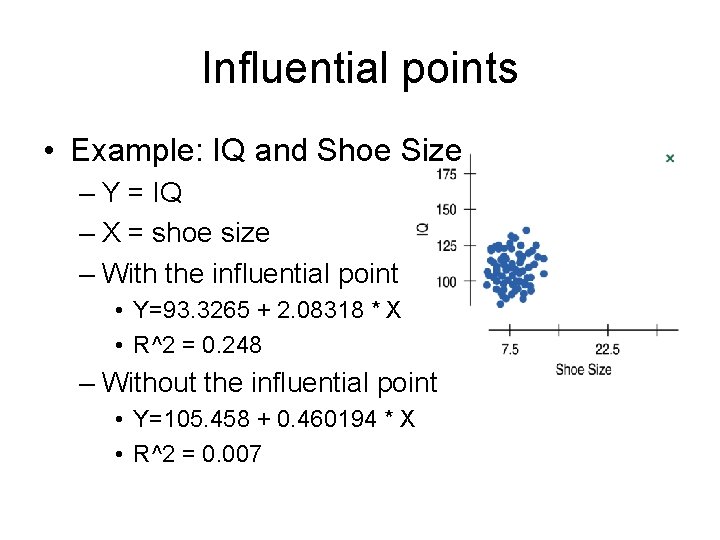
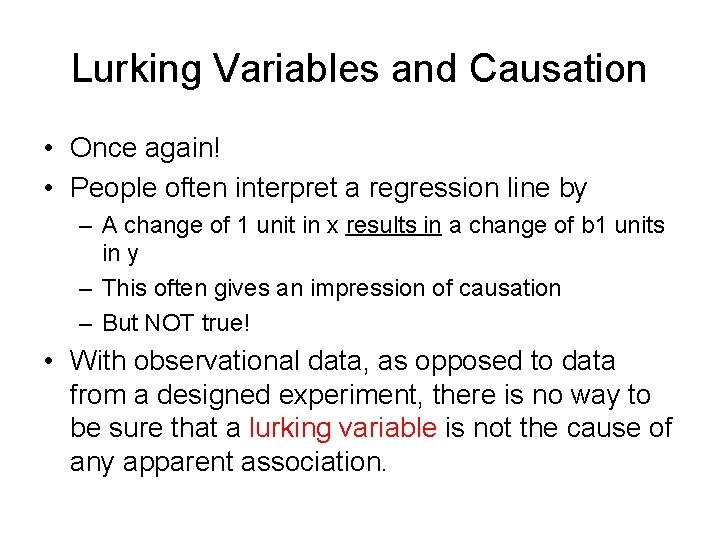
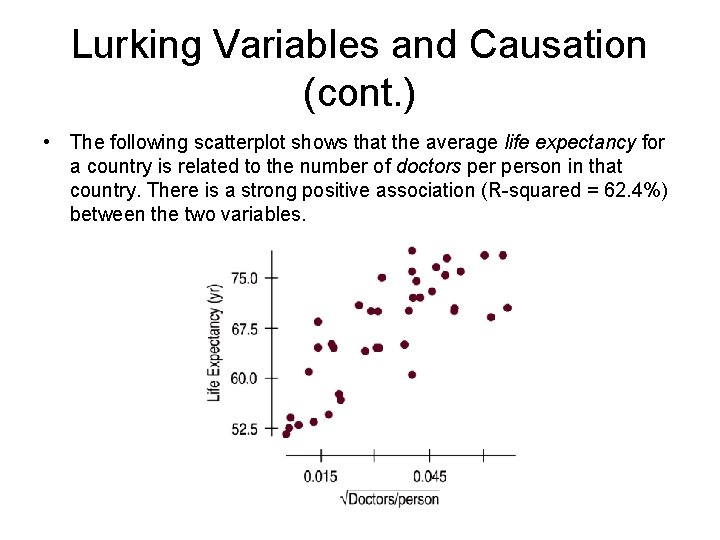
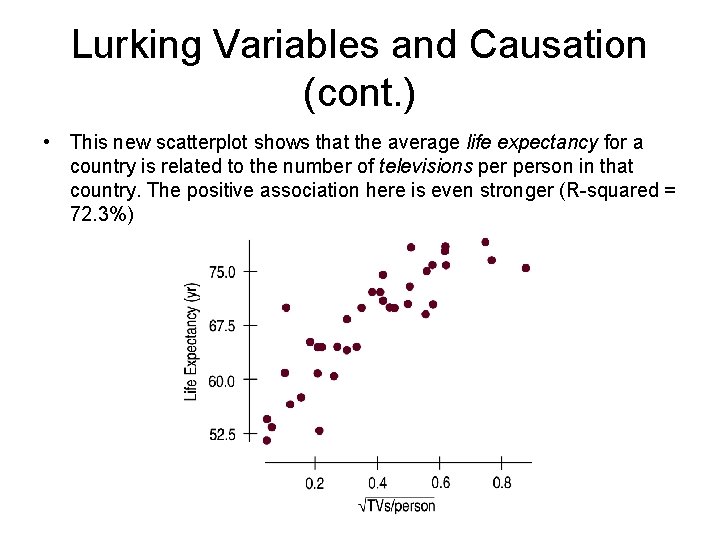
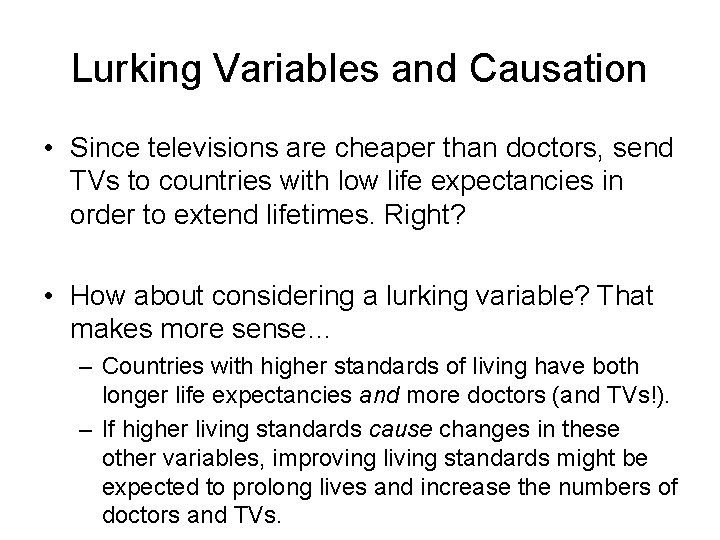
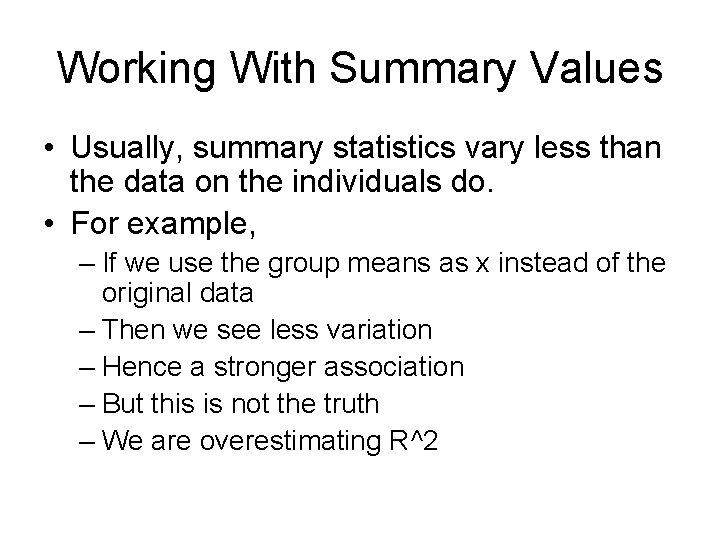
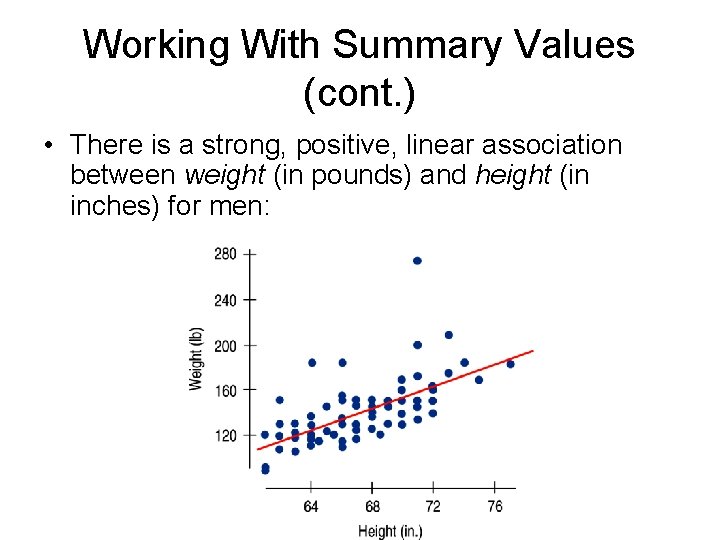
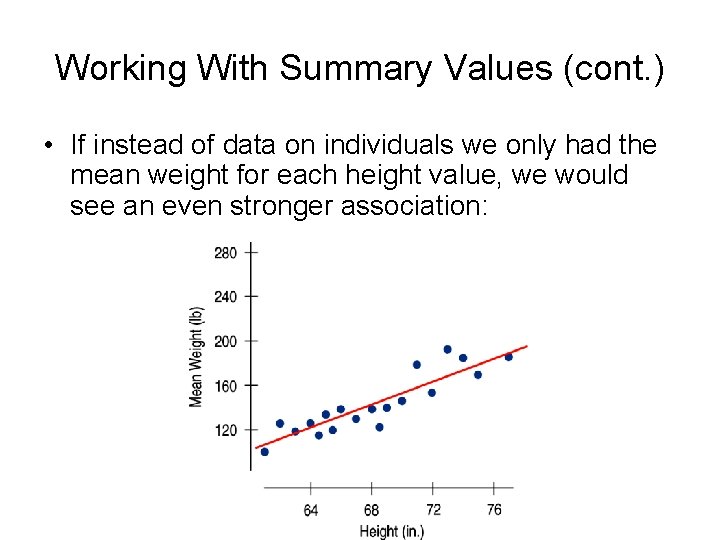
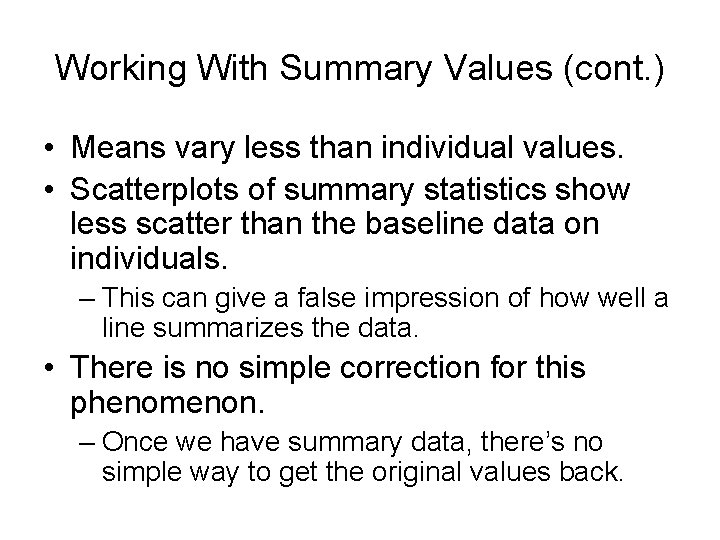
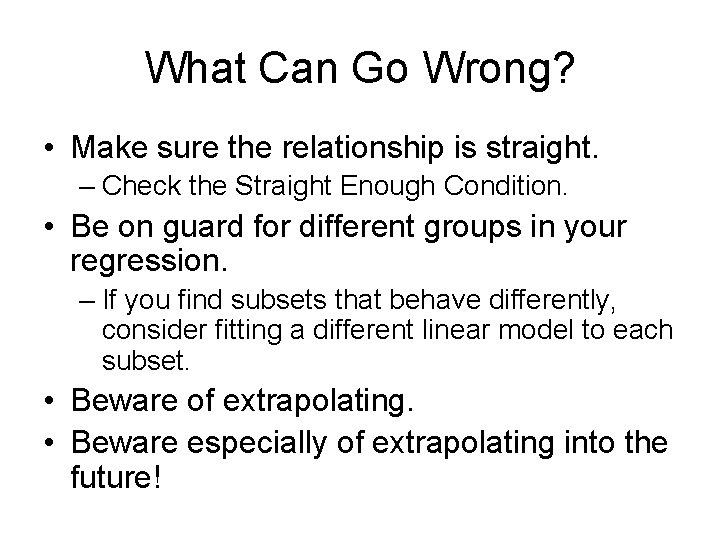
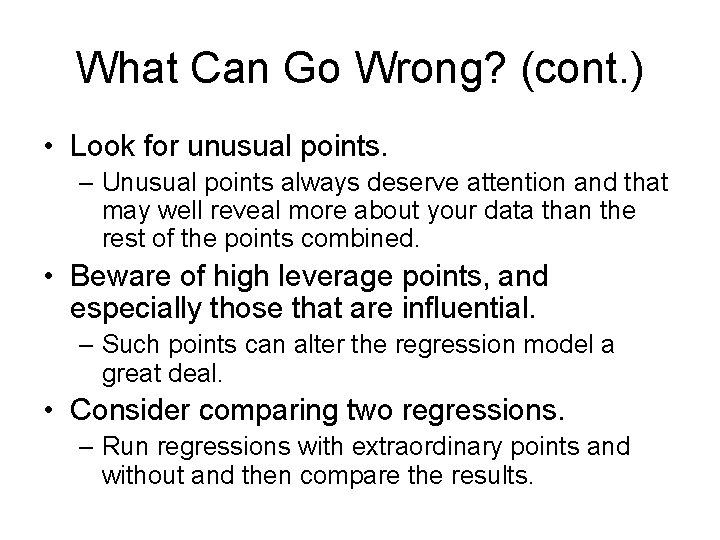
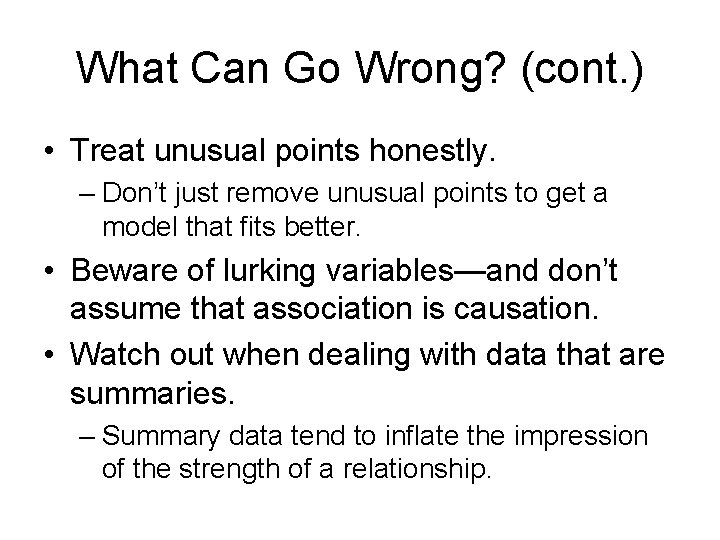
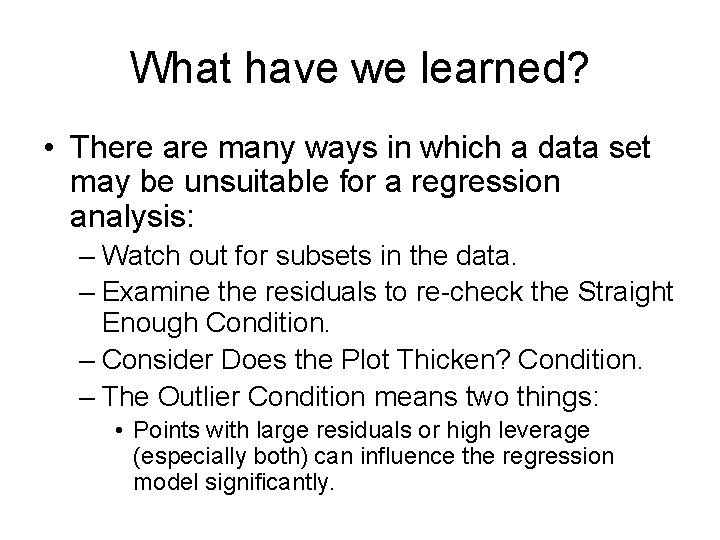
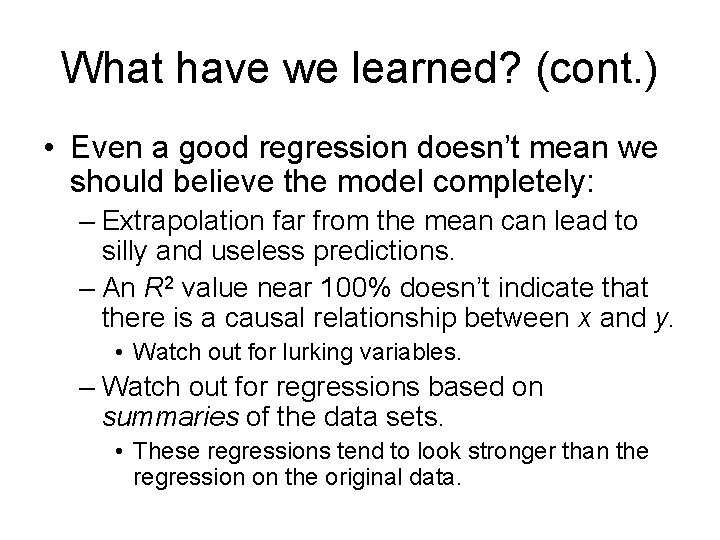
- Slides: 40
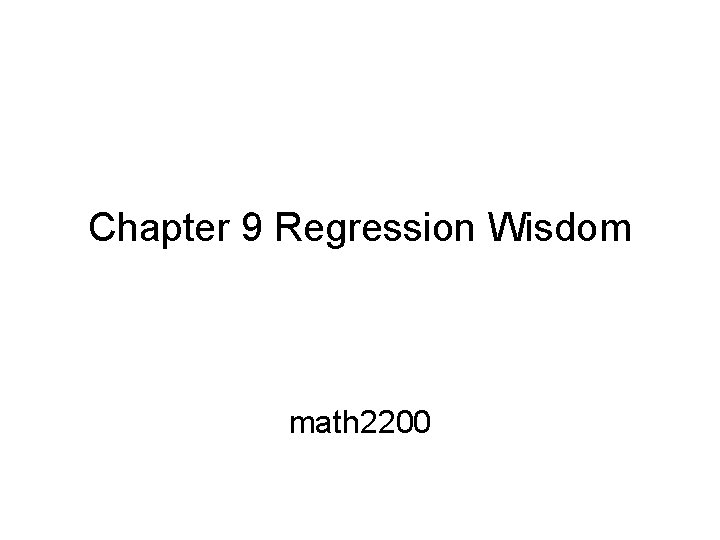
Chapter 9 Regression Wisdom math 2200
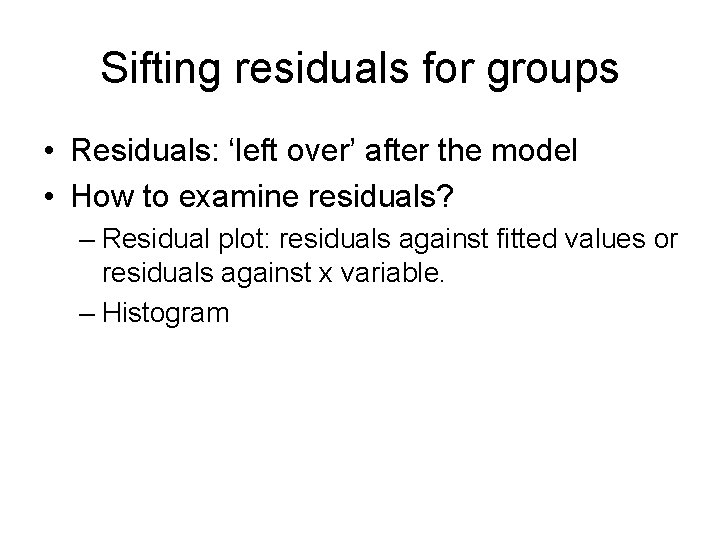
Sifting residuals for groups • Residuals: ‘left over’ after the model • How to examine residuals? – Residual plot: residuals against fitted values or residuals against x variable. – Histogram
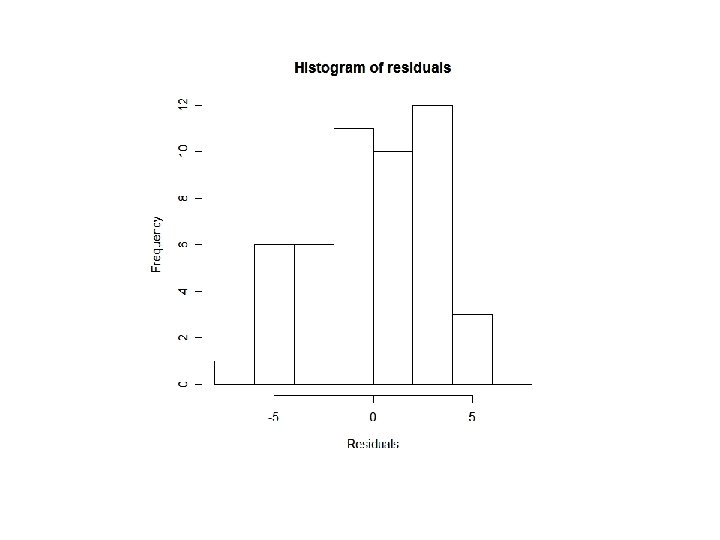
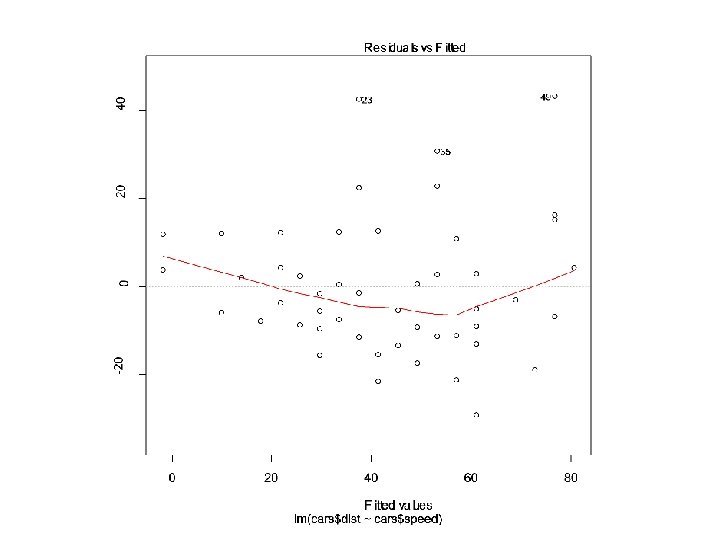
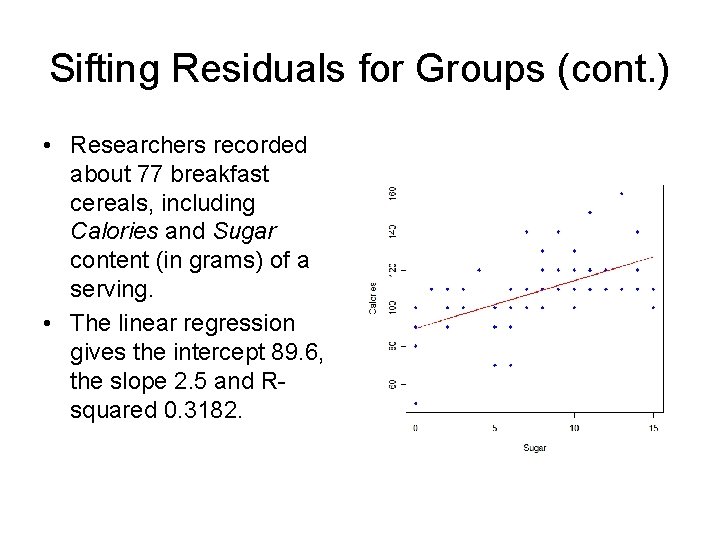
Sifting Residuals for Groups (cont. ) • Researchers recorded about 77 breakfast cereals, including Calories and Sugar content (in grams) of a serving. • The linear regression gives the intercept 89. 6, the slope 2. 5 and Rsquared 0. 3182.
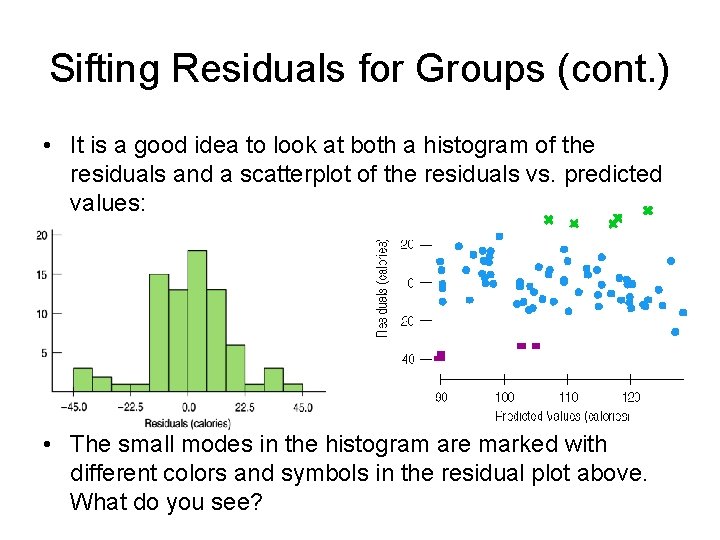
Sifting Residuals for Groups (cont. ) • It is a good idea to look at both a histogram of the residuals and a scatterplot of the residuals vs. predicted values: • The small modes in the histogram are marked with different colors and symbols in the residual plot above. What do you see?
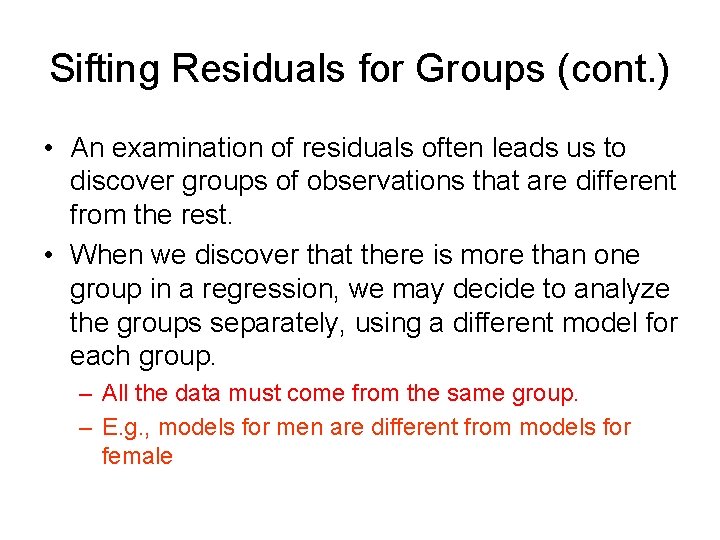
Sifting Residuals for Groups (cont. ) • An examination of residuals often leads us to discover groups of observations that are different from the rest. • When we discover that there is more than one group in a regression, we may decide to analyze the groups separately, using a different model for each group. – All the data must come from the same group. – E. g. , models for men are different from models for female
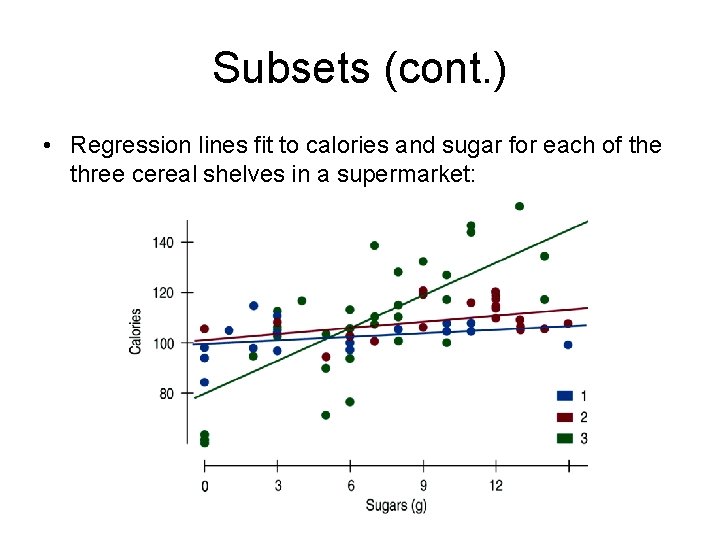
Subsets (cont. ) • Regression lines fit to calories and sugar for each of the three cereal shelves in a supermarket:
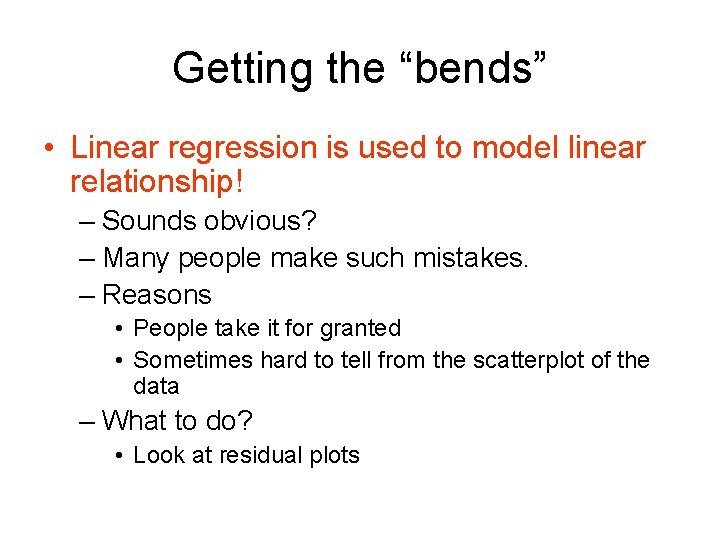
Getting the “bends” • Linear regression is used to model linear relationship! – Sounds obvious? – Many people make such mistakes. – Reasons • People take it for granted • Sometimes hard to tell from the scatterplot of the data – What to do? • Look at residual plots
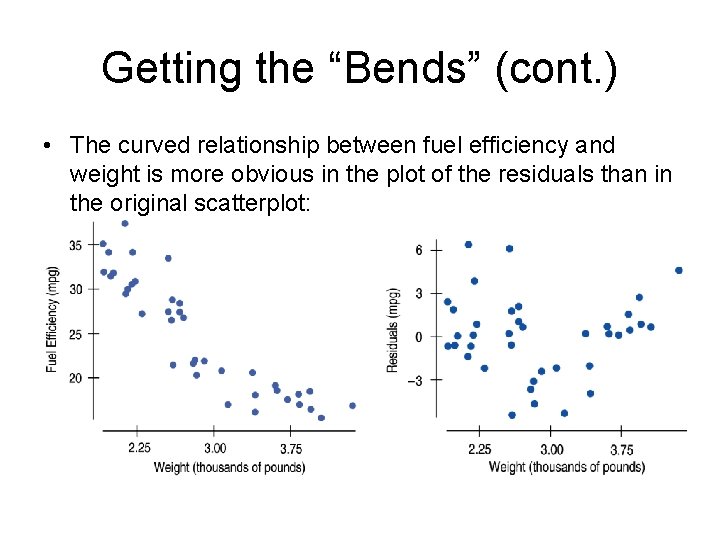
Getting the “Bends” (cont. ) • The curved relationship between fuel efficiency and weight is more obvious in the plot of the residuals than in the original scatterplot:
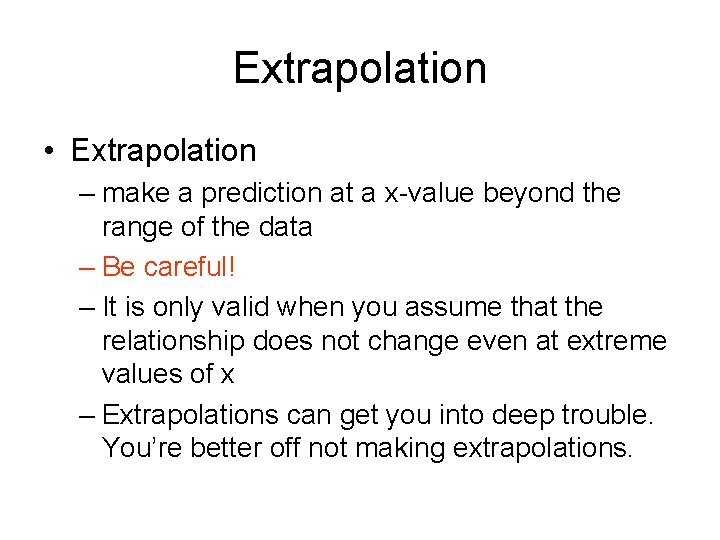
Extrapolation • Extrapolation – make a prediction at a x-value beyond the range of the data – Be careful! – It is only valid when you assume that the relationship does not change even at extreme values of x – Extrapolations can get you into deep trouble. You’re better off not making extrapolations.
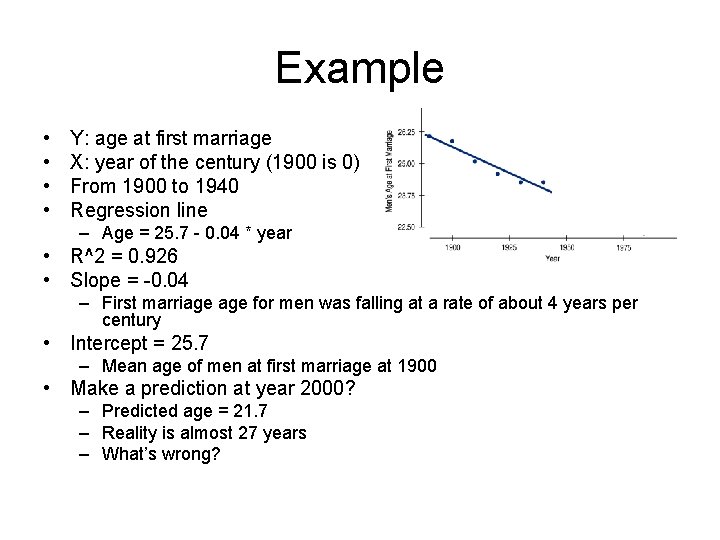
Example • • Y: age at first marriage X: year of the century (1900 is 0) From 1900 to 1940 Regression line – Age = 25. 7 - 0. 04 * year • R^2 = 0. 926 • Slope = -0. 04 – First marriage for men was falling at a rate of about 4 years per century • Intercept = 25. 7 – Mean age of men at first marriage at 1900 • Make a prediction at year 2000? – Predicted age = 21. 7 – Reality is almost 27 years – What’s wrong?
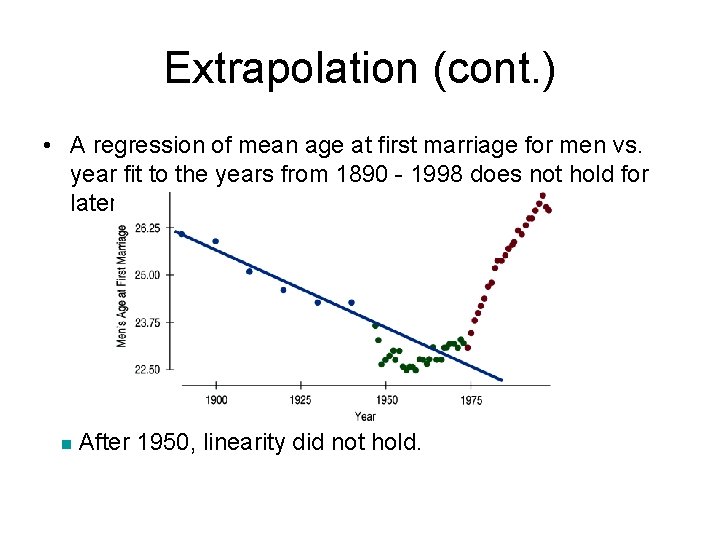
Extrapolation (cont. ) • A regression of mean age at first marriage for men vs. year fit to the years from 1890 - 1998 does not hold for later years: n After 1950, linearity did not hold.
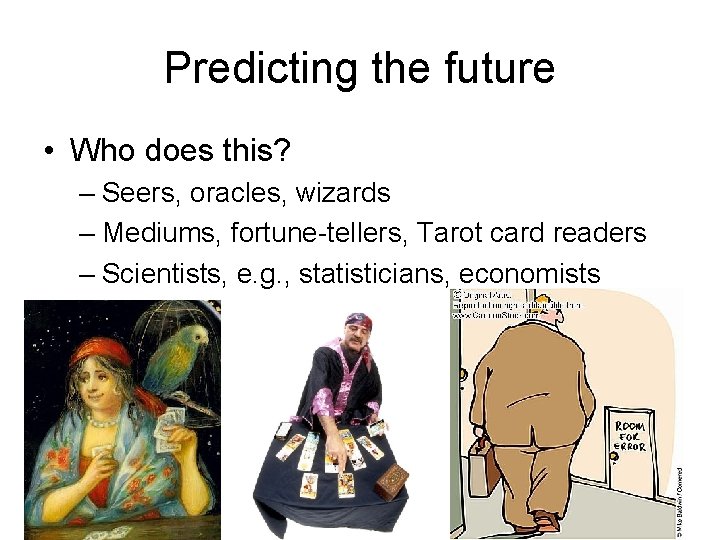
Predicting the future • Who does this? – Seers, oracles, wizards – Mediums, fortune-tellers, Tarot card readers – Scientists, e. g. , statisticians, economists
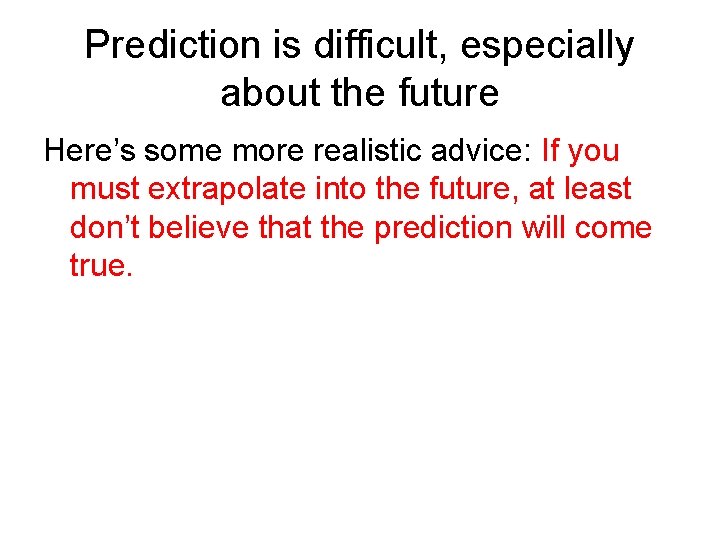
Prediction is difficult, especially about the future Here’s some more realistic advice: If you must extrapolate into the future, at least don’t believe that the prediction will come true.
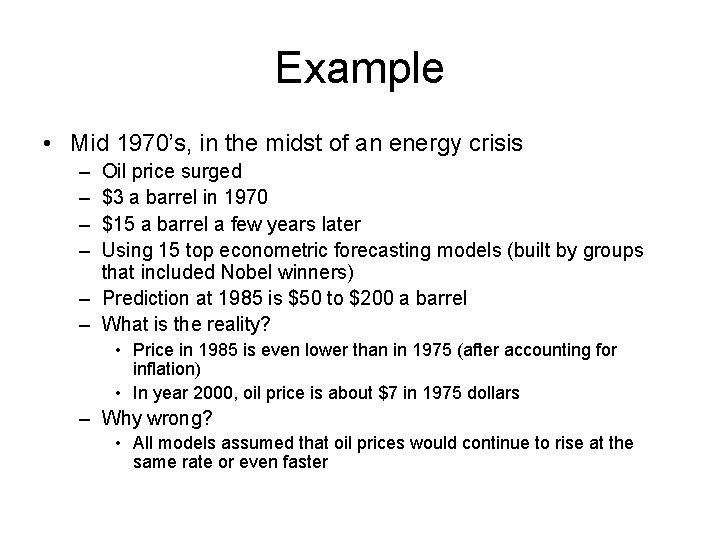
Example • Mid 1970’s, in the midst of an energy crisis – – Oil price surged $3 a barrel in 1970 $15 a barrel a few years later Using 15 top econometric forecasting models (built by groups that included Nobel winners) – Prediction at 1985 is $50 to $200 a barrel – What is the reality? • Price in 1985 is even lower than in 1975 (after accounting for inflation) • In year 2000, oil price is about $7 in 1975 dollars – Why wrong? • All models assumed that oil prices would continue to rise at the same rate or even faster
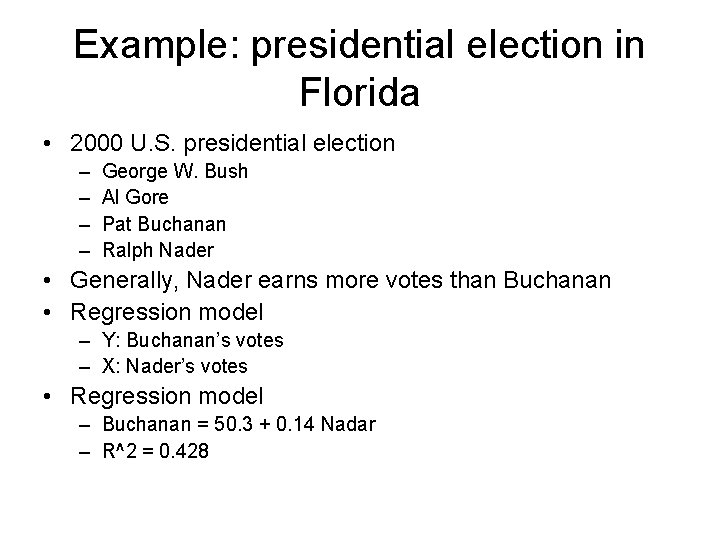
Example: presidential election in Florida • 2000 U. S. presidential election – – George W. Bush Al Gore Pat Buchanan Ralph Nader • Generally, Nader earns more votes than Buchanan • Regression model – Y: Buchanan’s votes – X: Nader’s votes • Regression model – Buchanan = 50. 3 + 0. 14 Nadar – R^2 = 0. 428
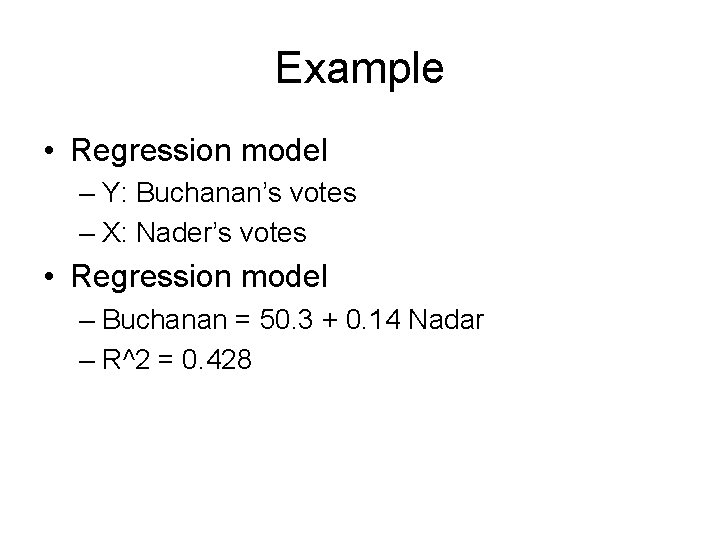
Example • Regression model – Y: Buchanan’s votes – X: Nader’s votes • Regression model – Buchanan = 50. 3 + 0. 14 Nadar – R^2 = 0. 428
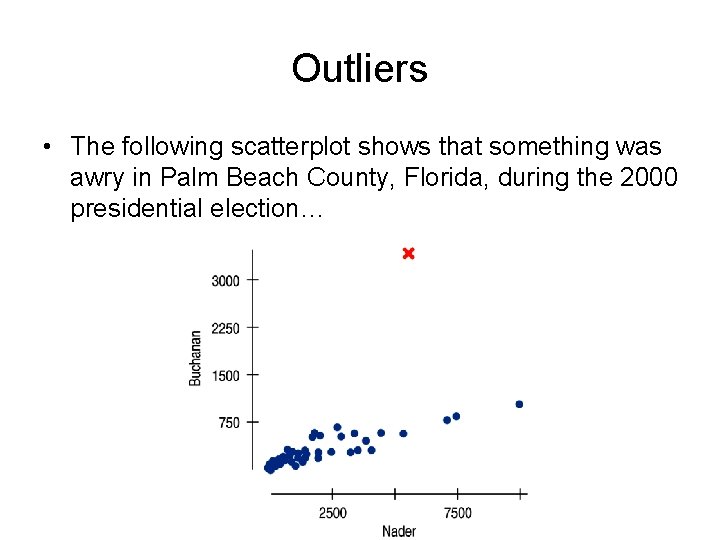
Outliers • The following scatterplot shows that something was awry in Palm Beach County, Florida, during the 2000 presidential election…
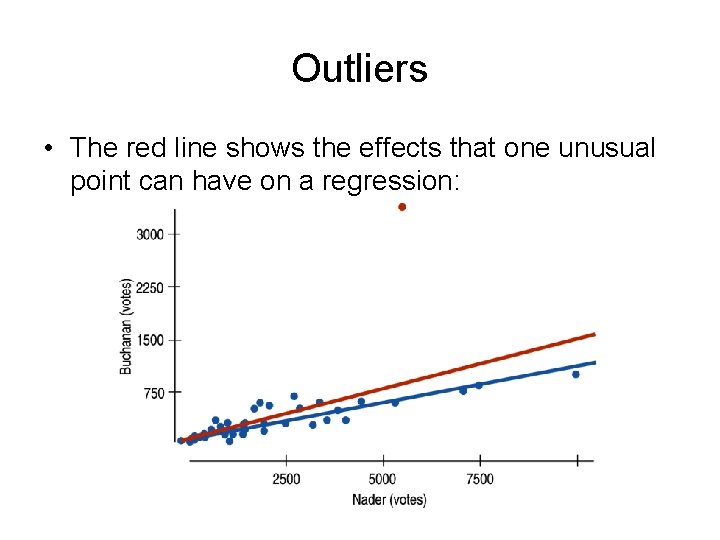
Outliers • The red line shows the effects that one unusual point can have on a regression:
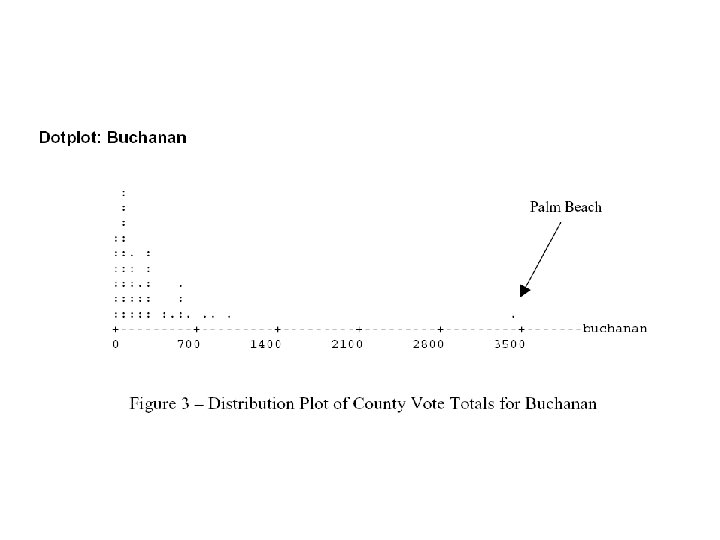
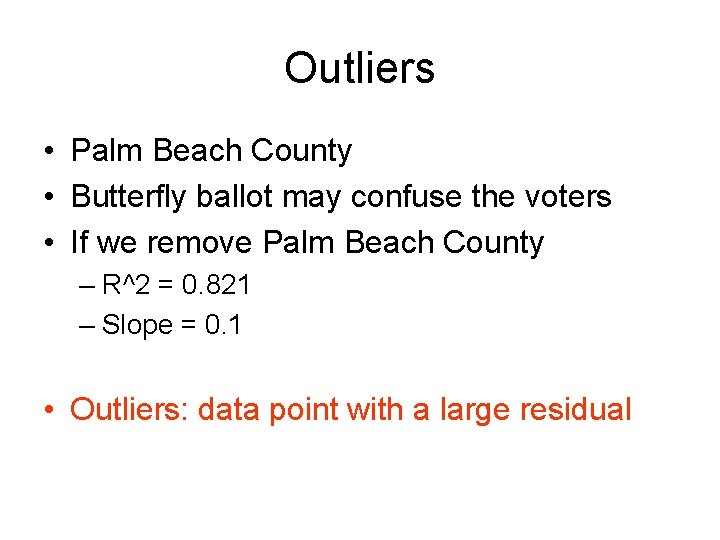
Outliers • Palm Beach County • Butterfly ballot may confuse the voters • If we remove Palm Beach County – R^2 = 0. 821 – Slope = 0. 1 • Outliers: data point with a large residual
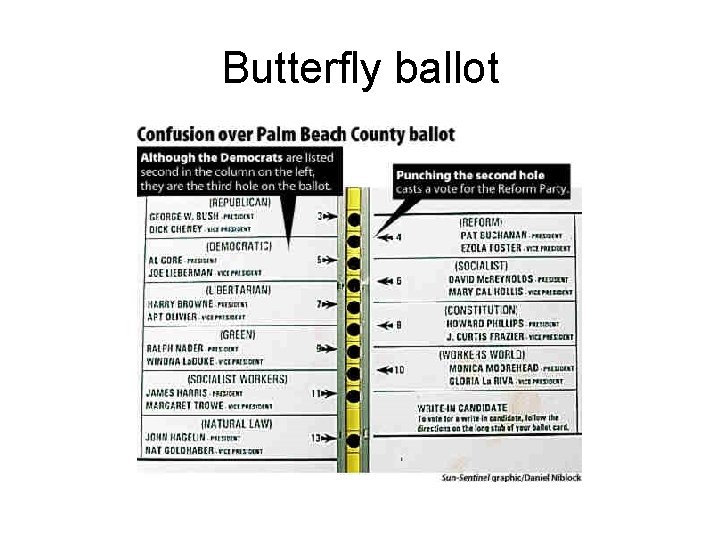
Butterfly ballot
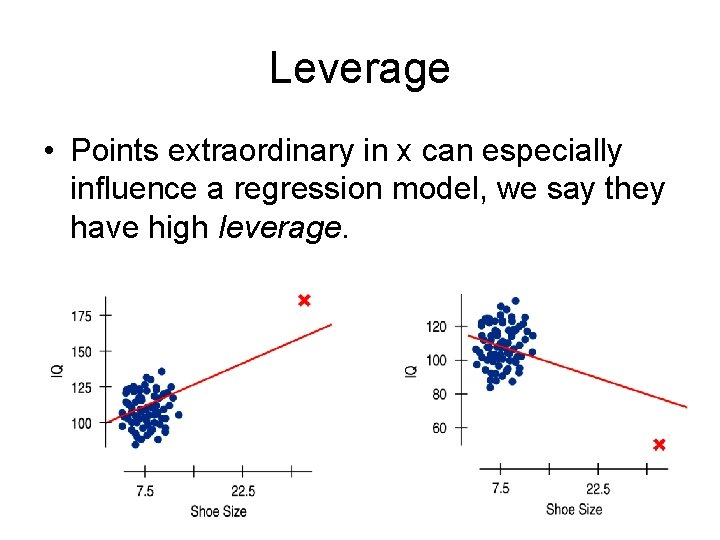
Leverage • Points extraordinary in x can especially influence a regression model, we say they have high leverage.
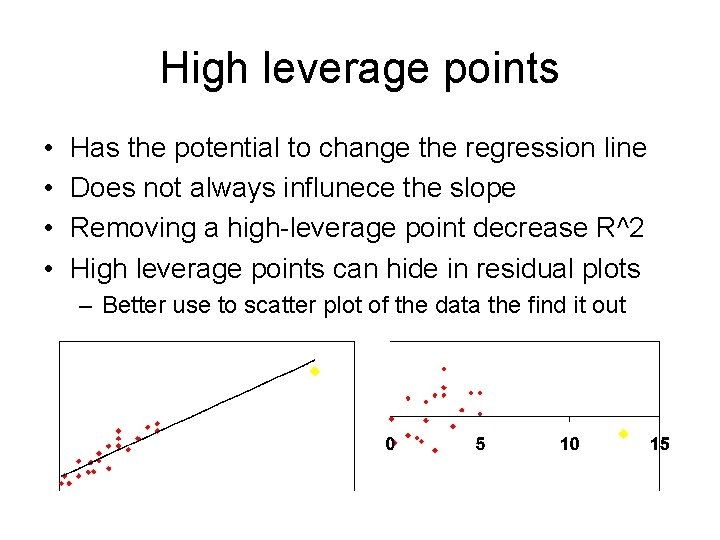
High leverage points • • Has the potential to change the regression line Does not always influnece the slope Removing a high-leverage point decrease R^2 High leverage points can hide in residual plots – Better use to scatter plot of the data the find it out
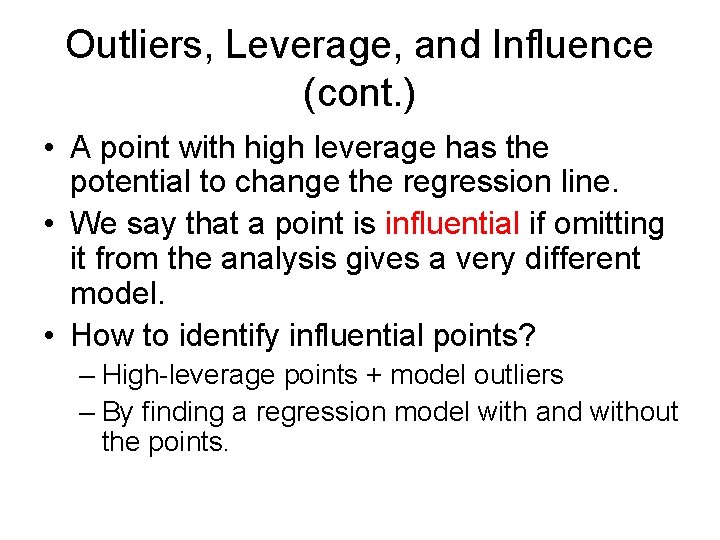
Outliers, Leverage, and Influence (cont. ) • A point with high leverage has the potential to change the regression line. • We say that a point is influential if omitting it from the analysis gives a very different model. • How to identify influential points? – High-leverage points + model outliers – By finding a regression model with and without the points.
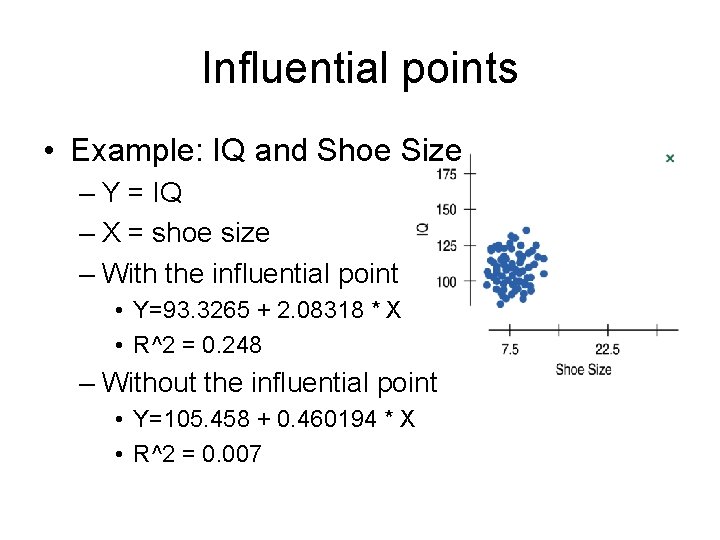
Influential points • Example: IQ and Shoe Size – Y = IQ – X = shoe size – With the influential point • Y=93. 3265 + 2. 08318 * X • R^2 = 0. 248 – Without the influential point • Y=105. 458 + 0. 460194 * X • R^2 = 0. 007
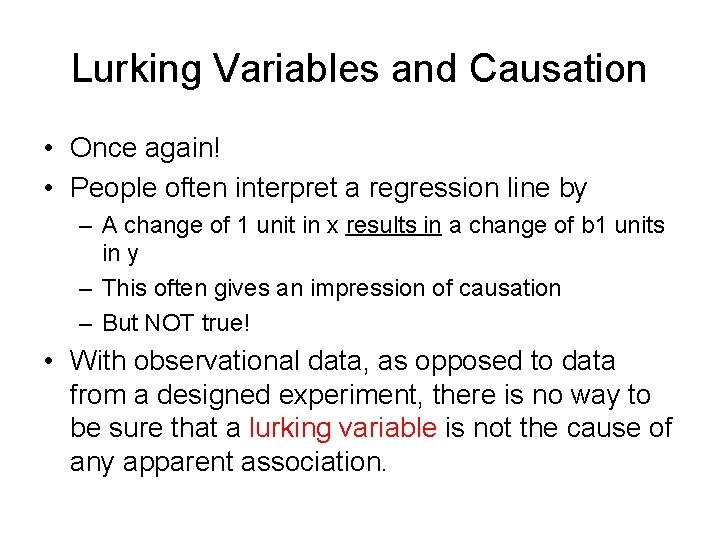
Lurking Variables and Causation • Once again! • People often interpret a regression line by – A change of 1 unit in x results in a change of b 1 units in y – This often gives an impression of causation – But NOT true! • With observational data, as opposed to data from a designed experiment, there is no way to be sure that a lurking variable is not the cause of any apparent association.
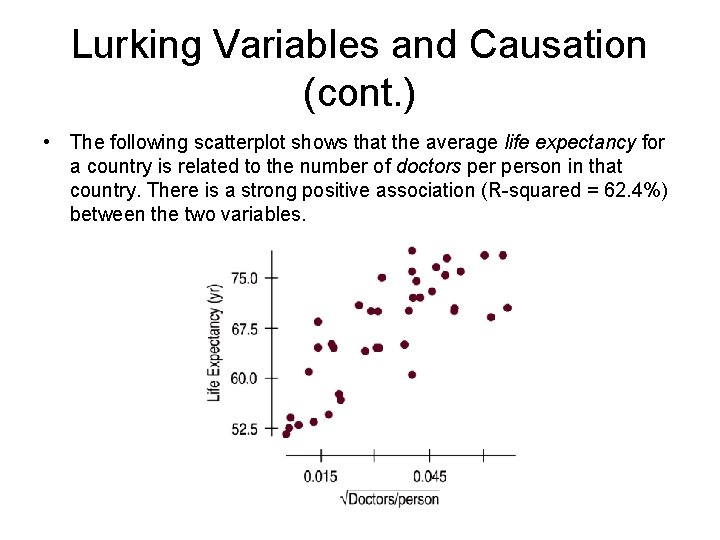
Lurking Variables and Causation (cont. ) • The following scatterplot shows that the average life expectancy for a country is related to the number of doctors person in that country. There is a strong positive association (R-squared = 62. 4%) between the two variables.
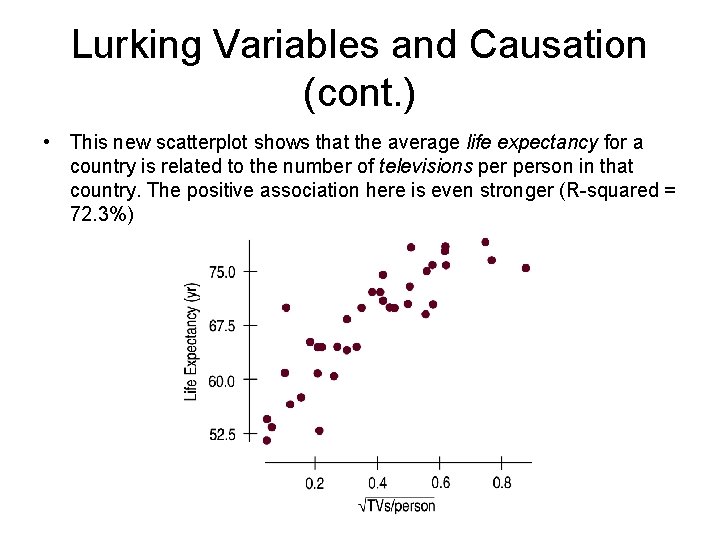
Lurking Variables and Causation (cont. ) • This new scatterplot shows that the average life expectancy for a country is related to the number of televisions person in that country. The positive association here is even stronger (R-squared = 72. 3%)
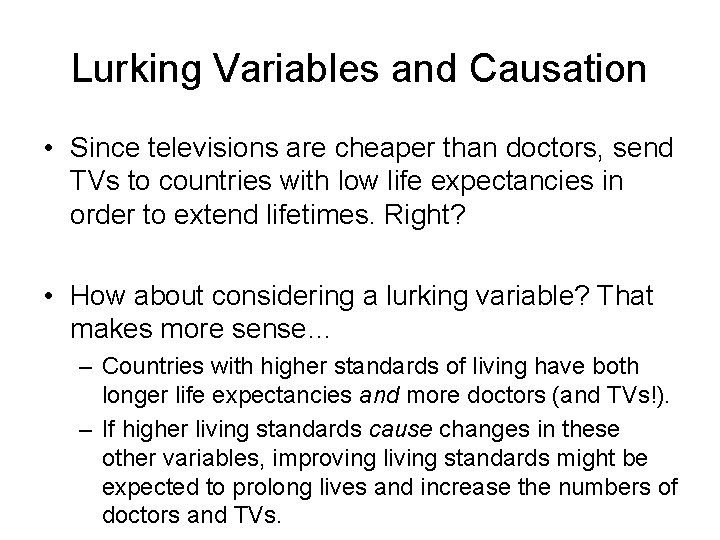
Lurking Variables and Causation • Since televisions are cheaper than doctors, send TVs to countries with low life expectancies in order to extend lifetimes. Right? • How about considering a lurking variable? That makes more sense… – Countries with higher standards of living have both longer life expectancies and more doctors (and TVs!). – If higher living standards cause changes in these other variables, improving living standards might be expected to prolong lives and increase the numbers of doctors and TVs.
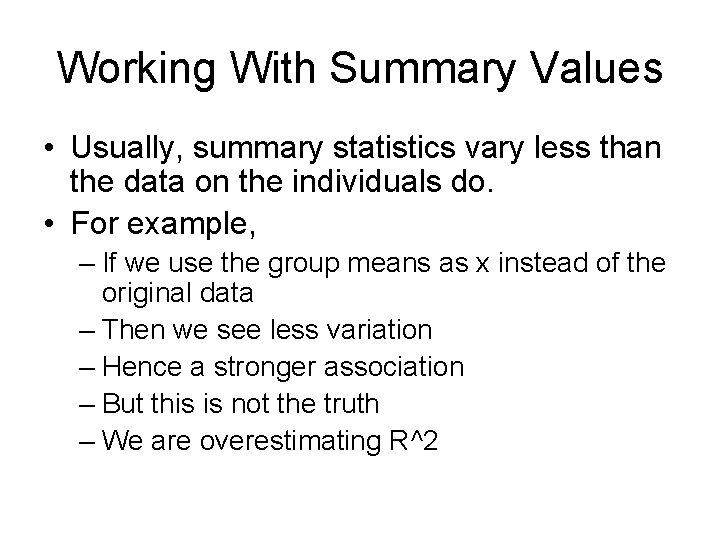
Working With Summary Values • Usually, summary statistics vary less than the data on the individuals do. • For example, – If we use the group means as x instead of the original data – Then we see less variation – Hence a stronger association – But this is not the truth – We are overestimating R^2
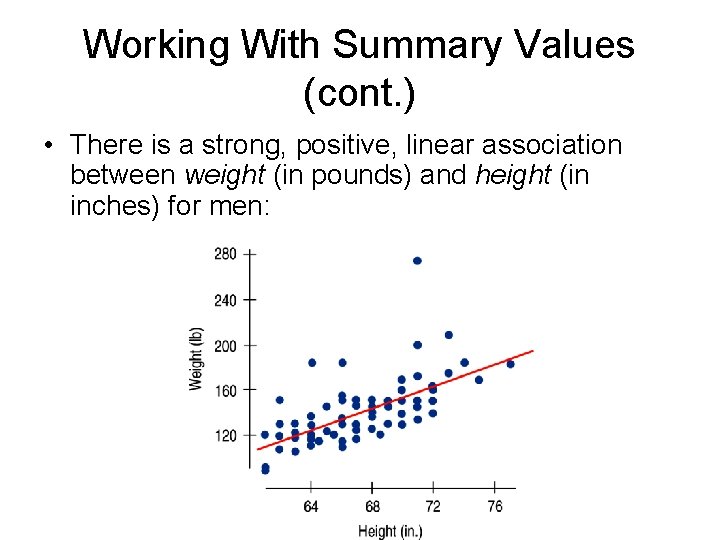
Working With Summary Values (cont. ) • There is a strong, positive, linear association between weight (in pounds) and height (in inches) for men:
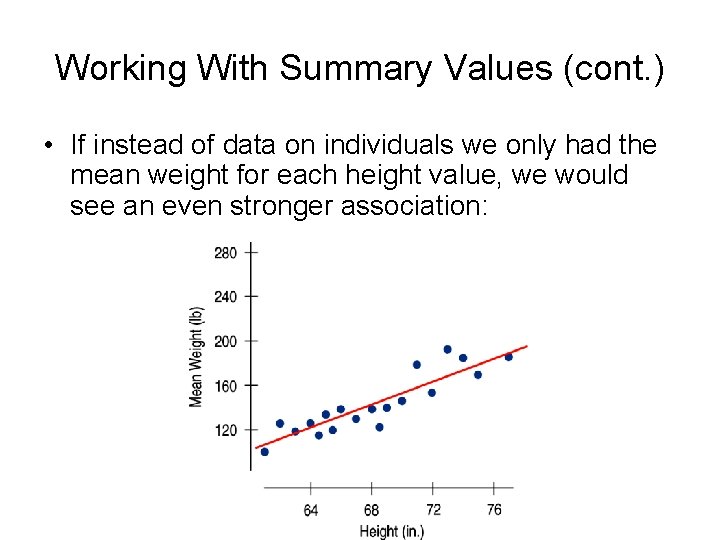
Working With Summary Values (cont. ) • If instead of data on individuals we only had the mean weight for each height value, we would see an even stronger association:
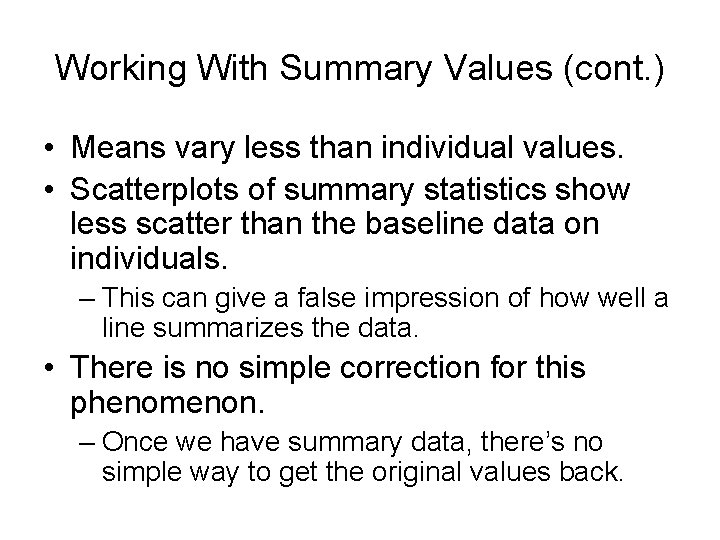
Working With Summary Values (cont. ) • Means vary less than individual values. • Scatterplots of summary statistics show less scatter than the baseline data on individuals. – This can give a false impression of how well a line summarizes the data. • There is no simple correction for this phenomenon. – Once we have summary data, there’s no simple way to get the original values back.
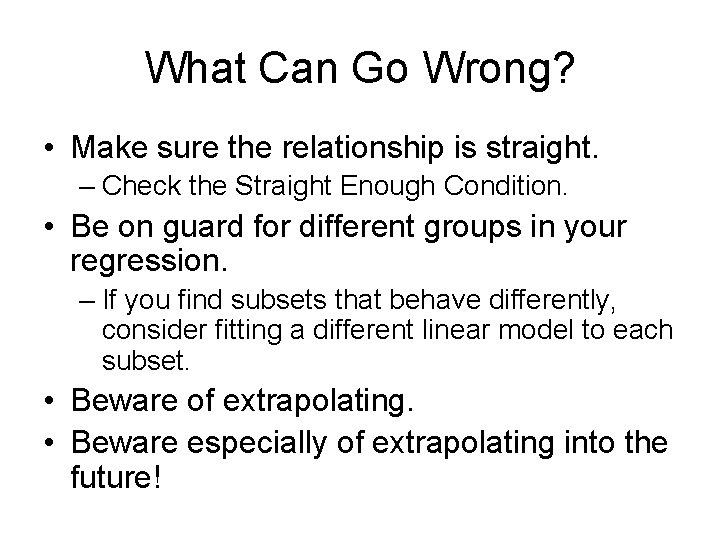
What Can Go Wrong? • Make sure the relationship is straight. – Check the Straight Enough Condition. • Be on guard for different groups in your regression. – If you find subsets that behave differently, consider fitting a different linear model to each subset. • Beware of extrapolating. • Beware especially of extrapolating into the future!
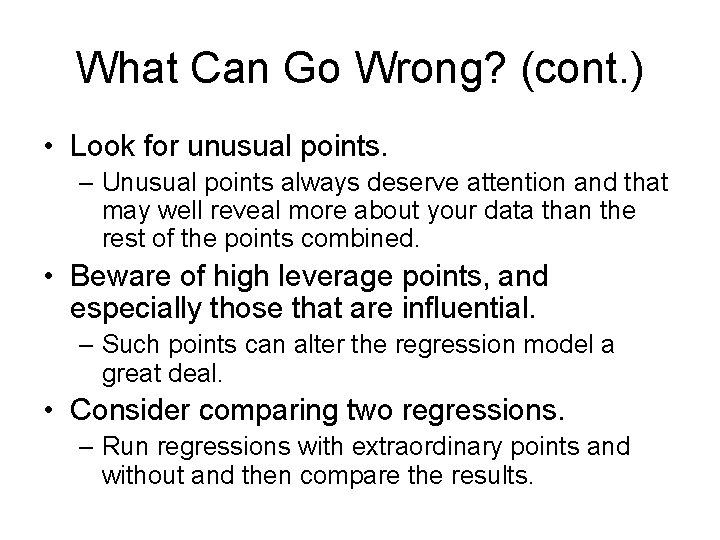
What Can Go Wrong? (cont. ) • Look for unusual points. – Unusual points always deserve attention and that may well reveal more about your data than the rest of the points combined. • Beware of high leverage points, and especially those that are influential. – Such points can alter the regression model a great deal. • Consider comparing two regressions. – Run regressions with extraordinary points and without and then compare the results.
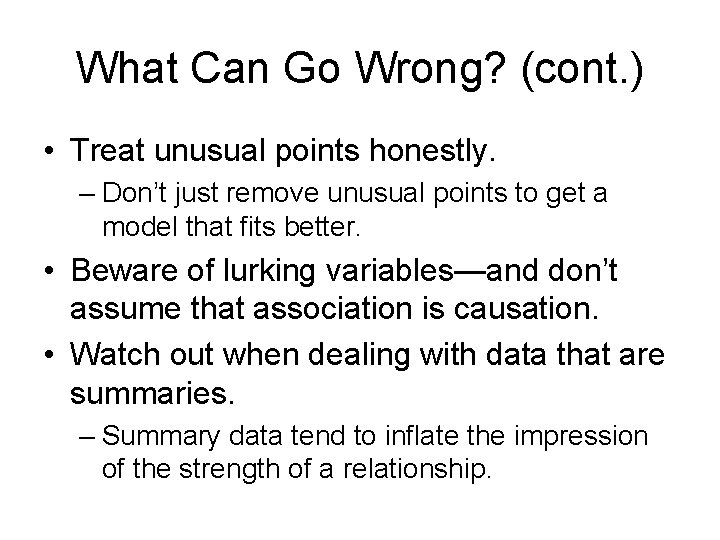
What Can Go Wrong? (cont. ) • Treat unusual points honestly. – Don’t just remove unusual points to get a model that fits better. • Beware of lurking variables—and don’t assume that association is causation. • Watch out when dealing with data that are summaries. – Summary data tend to inflate the impression of the strength of a relationship.
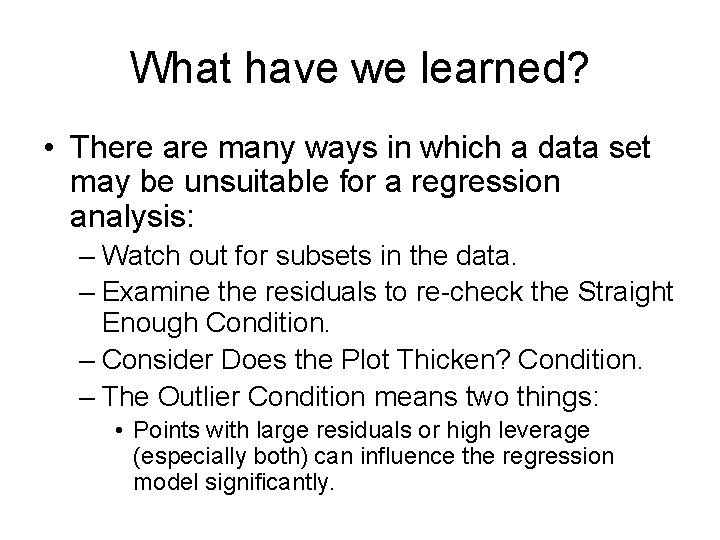
What have we learned? • There are many ways in which a data set may be unsuitable for a regression analysis: – Watch out for subsets in the data. – Examine the residuals to re-check the Straight Enough Condition. – Consider Does the Plot Thicken? Condition. – The Outlier Condition means two things: • Points with large residuals or high leverage (especially both) can influence the regression model significantly.
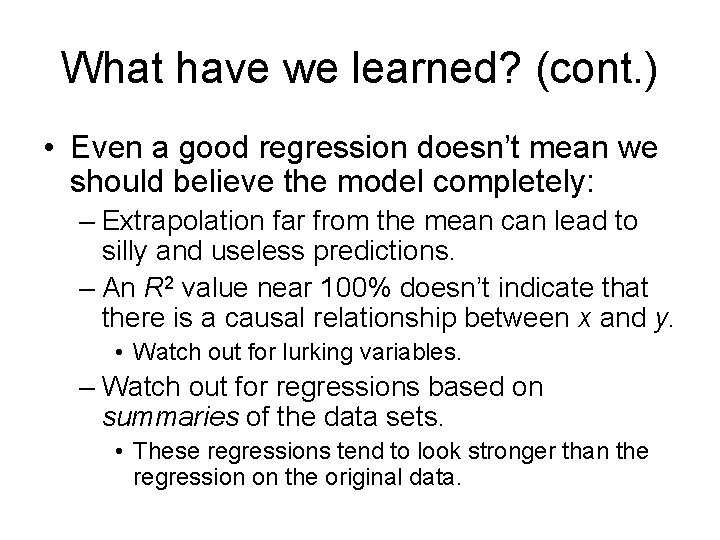
What have we learned? (cont. ) • Even a good regression doesn’t mean we should believe the model completely: – Extrapolation far from the mean can lead to silly and useless predictions. – An R 2 value near 100% doesn’t indicate that there is a causal relationship between x and y. • Watch out for lurking variables. – Watch out for regressions based on summaries of the data sets. • These regressions tend to look stronger than the regression on the original data.
Chapter 9 regression wisdom
James 3 15 16
Godly wisdom vs worldly wisdom
Heavenly wisdom
How to make a residual plot on ti 84
Section 10 topic 5 residuals and residual plots
Participations and residuals
Schoenfeld residuals spss
Qnorm stata
Lesson 15 interpreting residuals from a line
Residual alkalinity
Mekki farmacia
Decreto 2200/05
Mgh formula
Iso2200
R-2070 reinf
Mesta 2200
Mycenaean chronology
Linear regression vs multiple regression
Multiple regression vs linear regression
Survival analysis vs logistic regression
Logistic regression vs linear regression
Evaporation mixture example
Sieving mixtures examples
Bird feet adaptations
Define size separation in pharmaceutics
Hit the button
Chapter 26 inferences for regression
Chapter 26 inferences for regression
Worthless regression chapter 16
Chapter 27: inferences for regression
Chapter 7 linear regression
Sample regression function
Useless regression chapter 16
Chapter 8 linear regression
Bps 4389
Chapter 8 linear regression
Wisdom is proved right by her children
Wisdom 8:7
Proverbs 3:1-35
Hasty generalization logical fallacy