Statistics and Quantitative Analysis U 4320 Segment 8
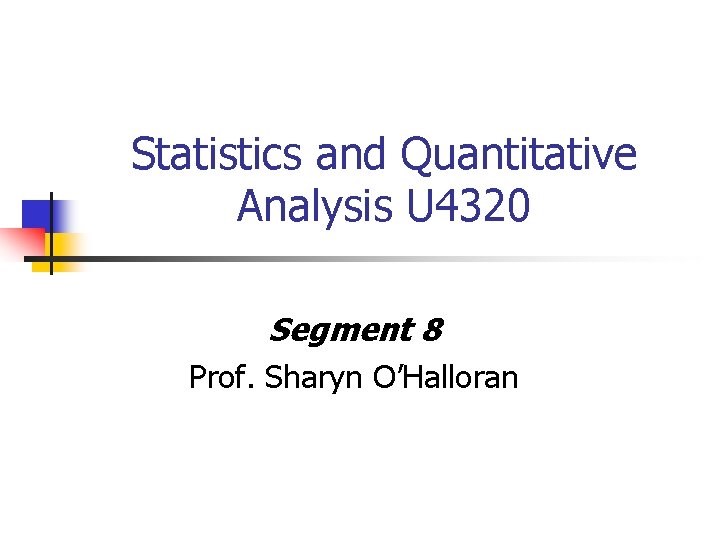
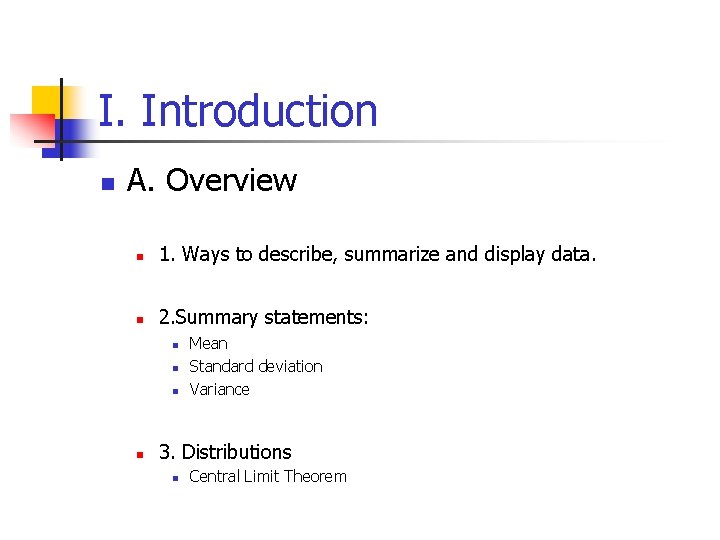
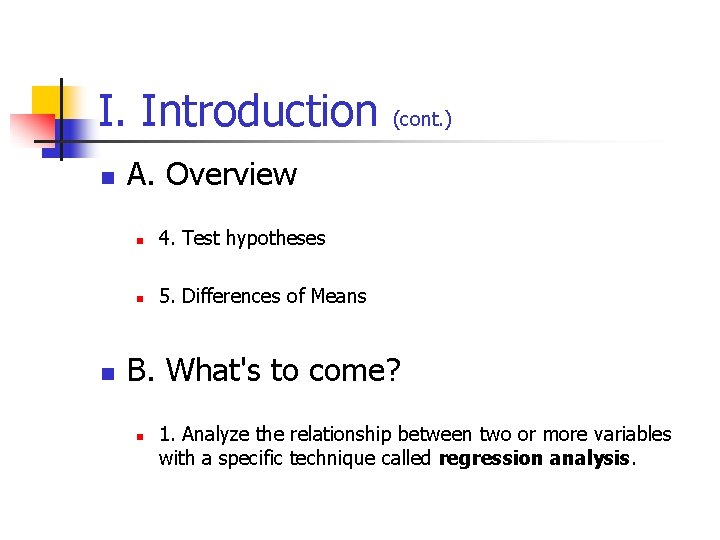
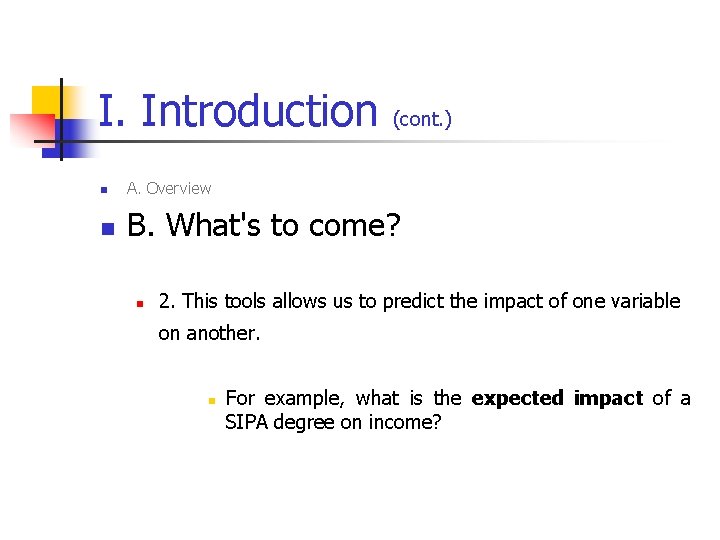
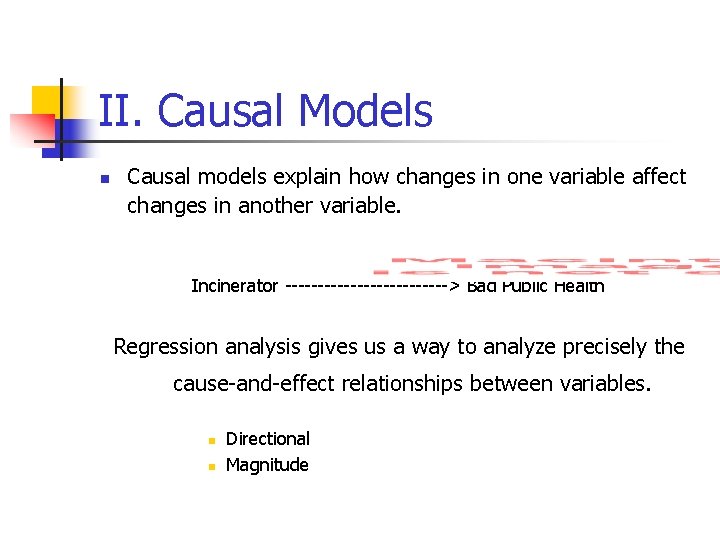
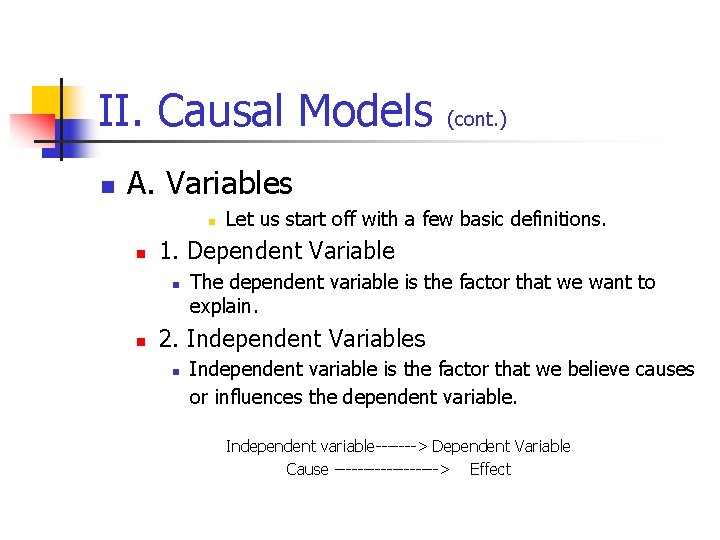
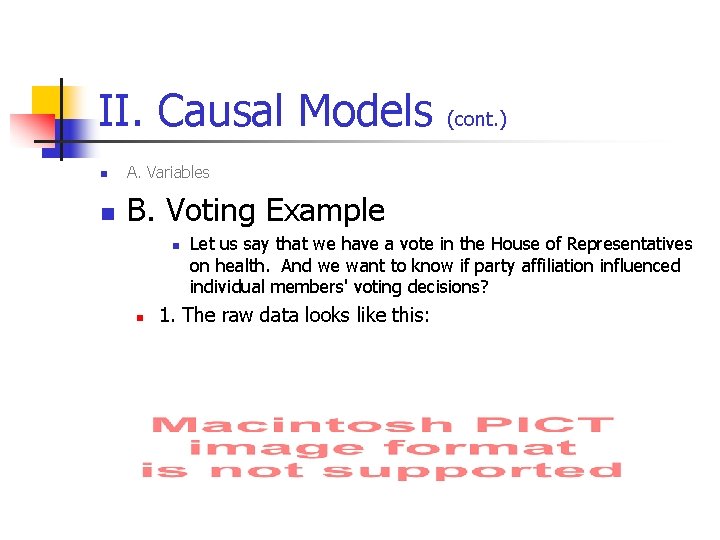
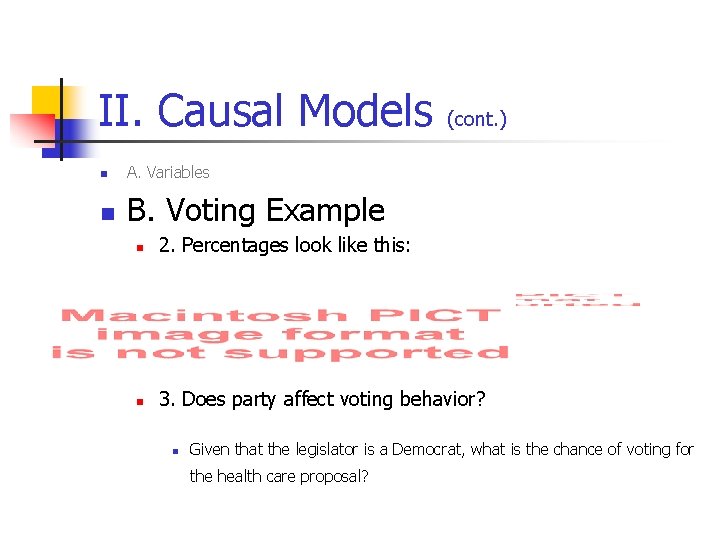
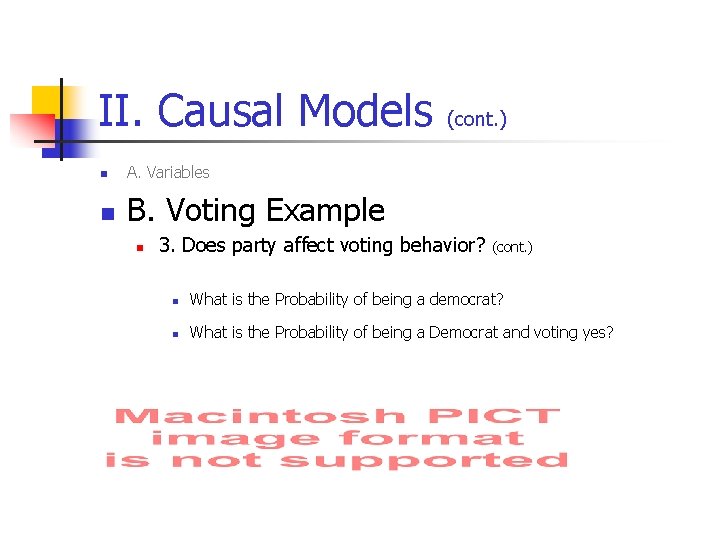
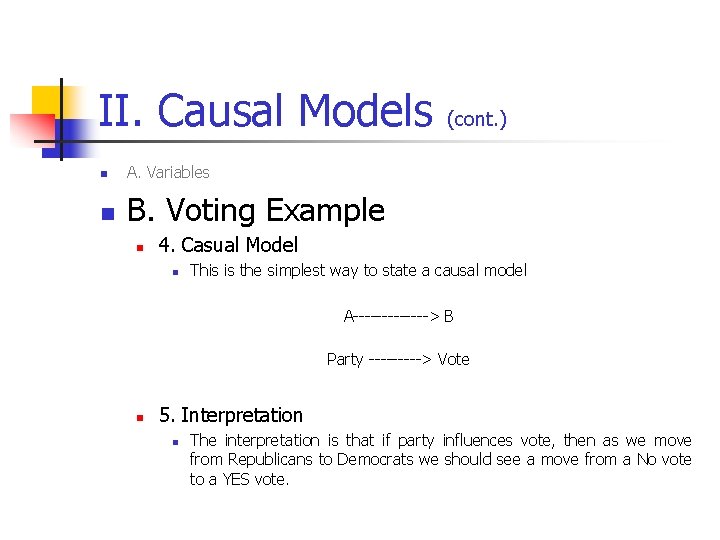
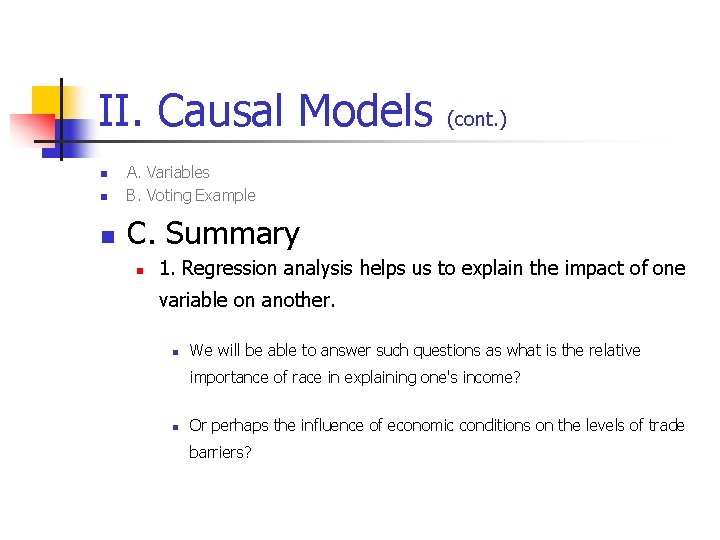
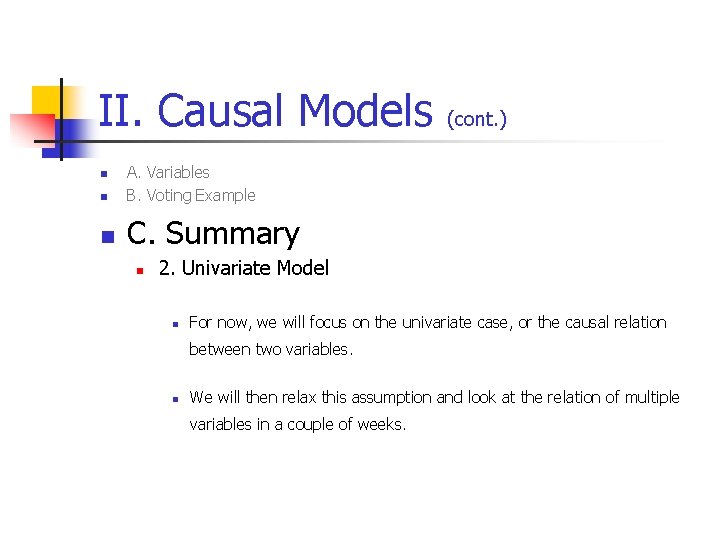
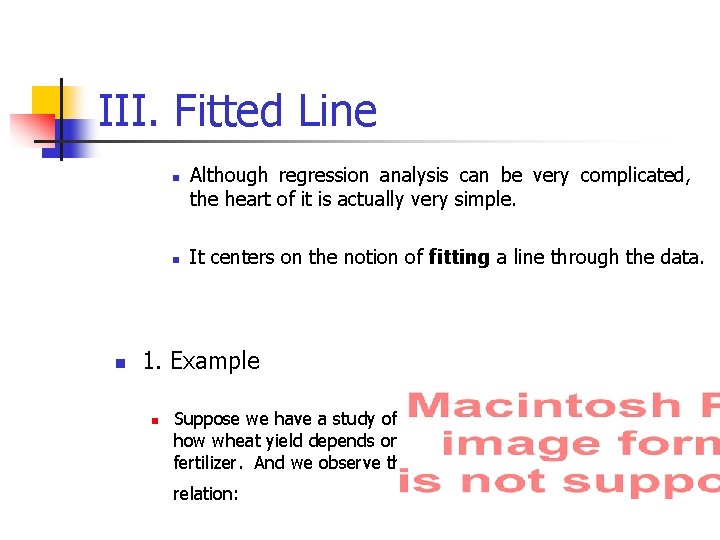
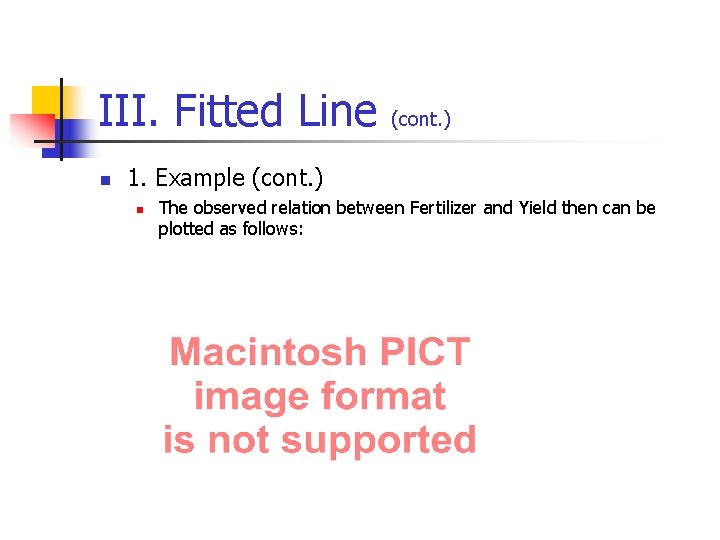
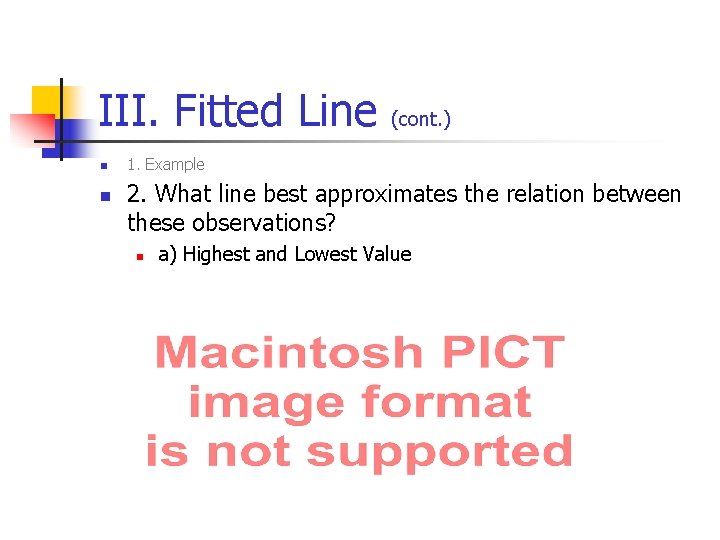
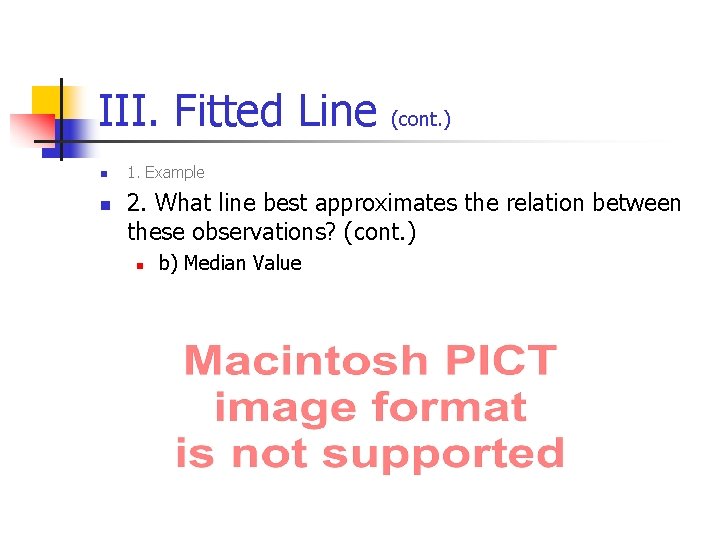
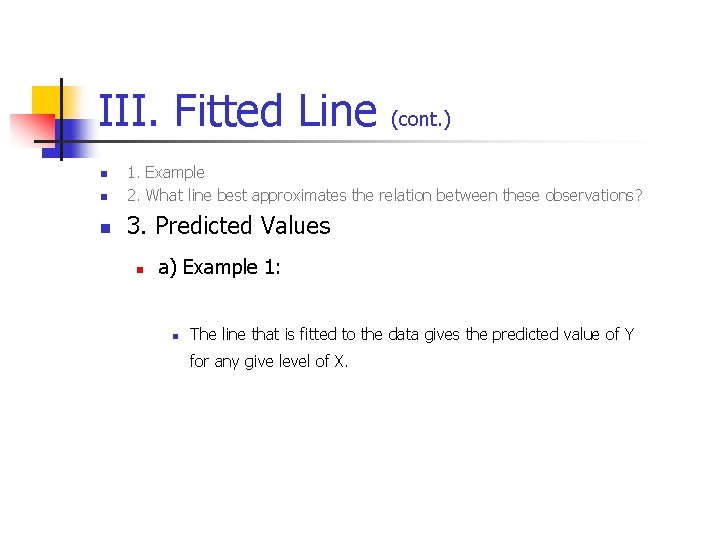
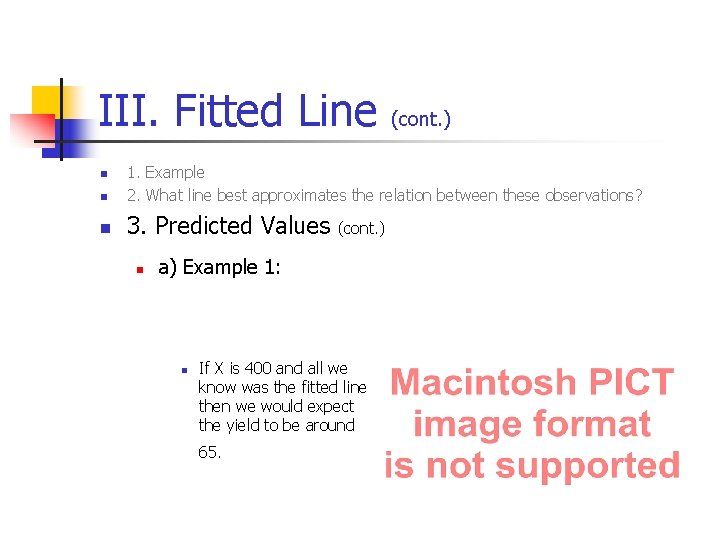
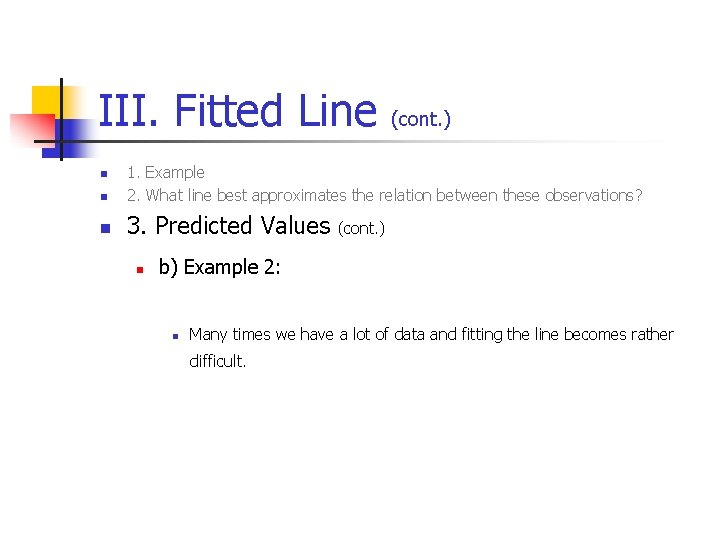
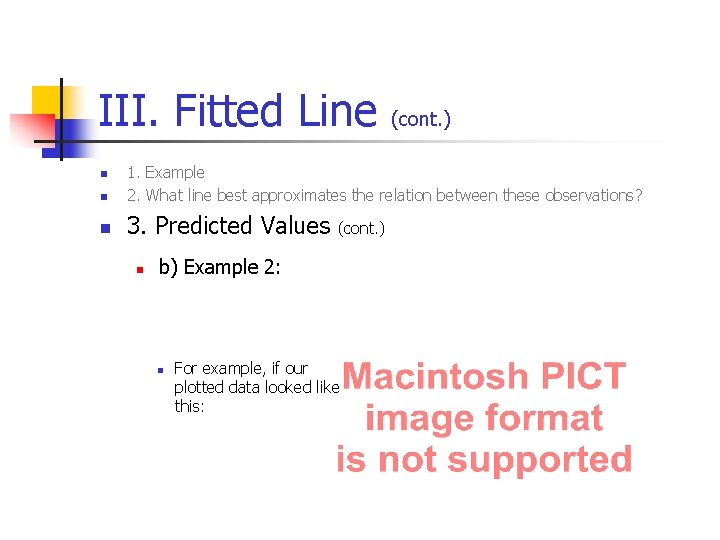
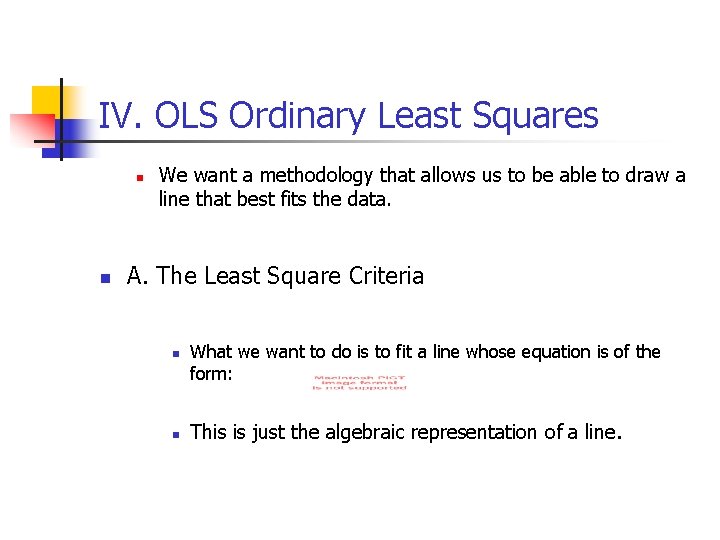
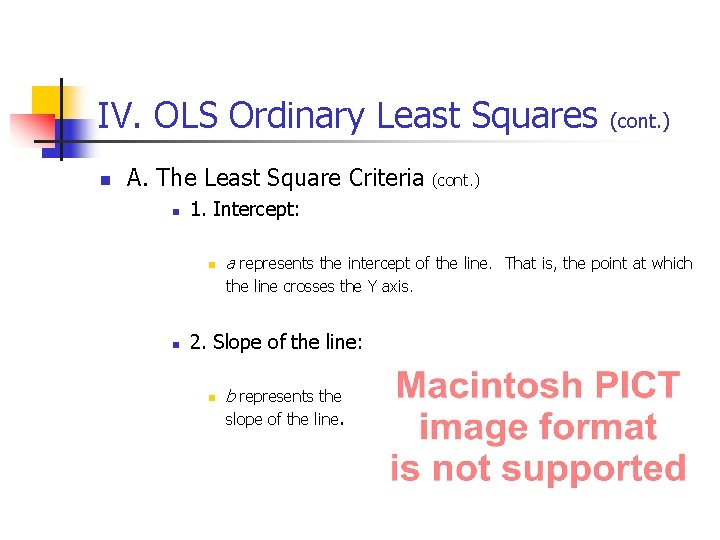
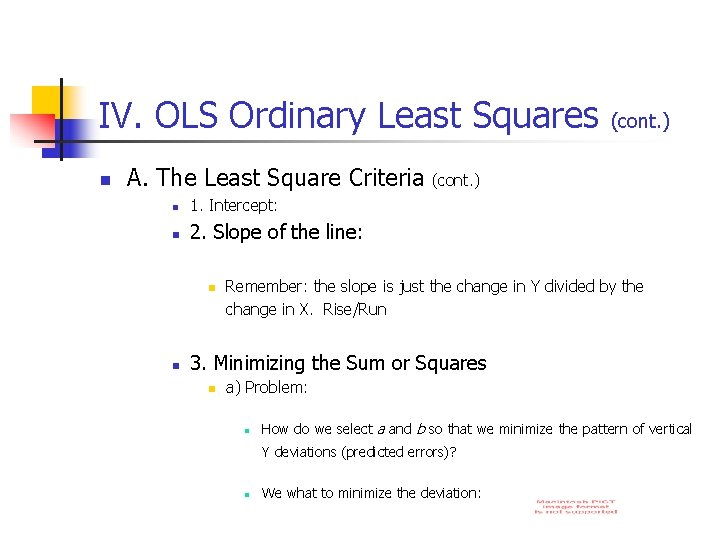
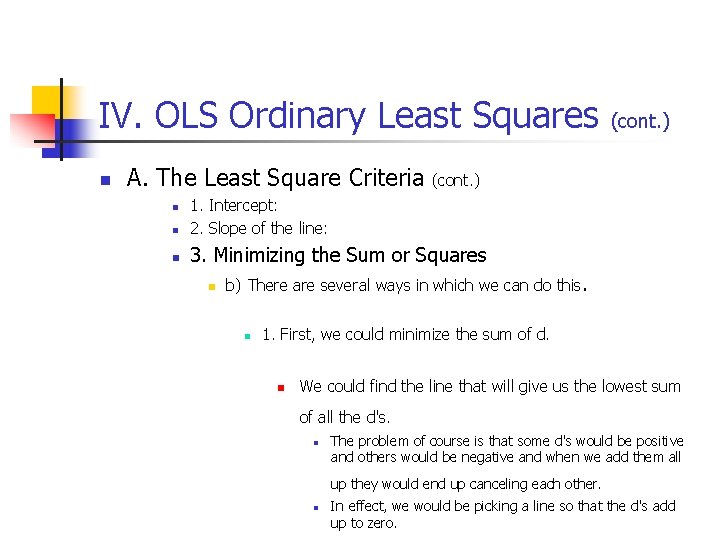
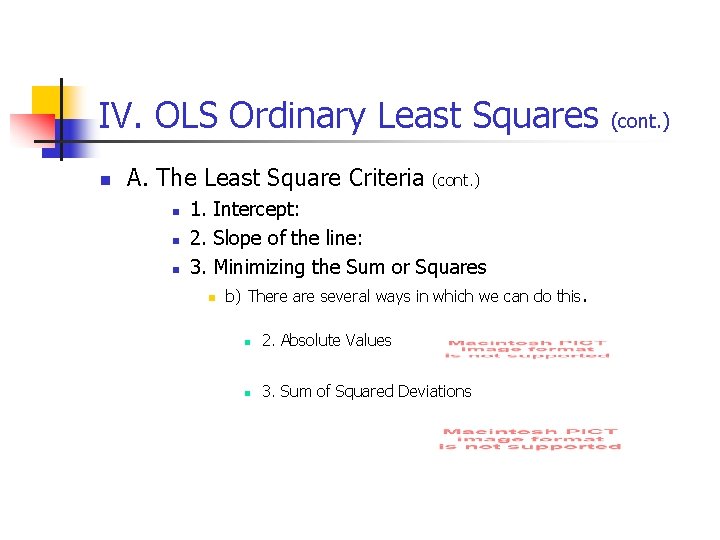
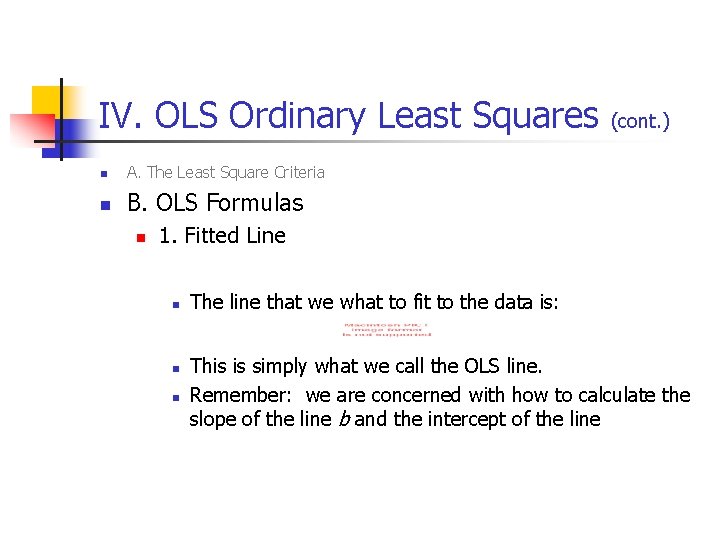
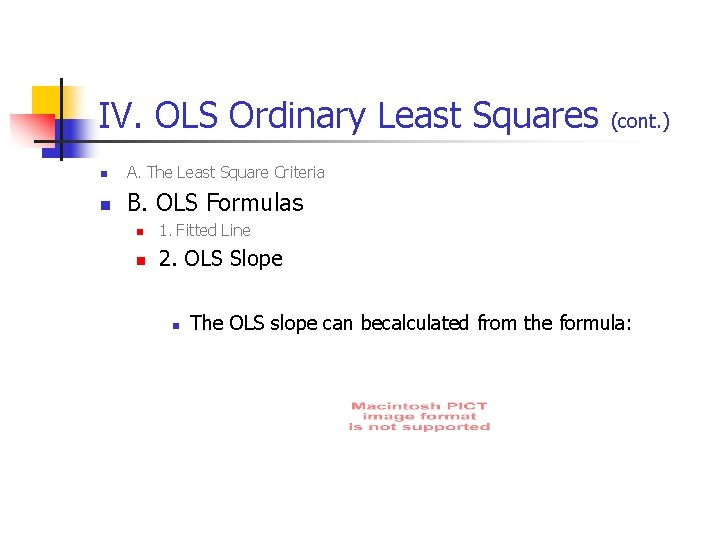
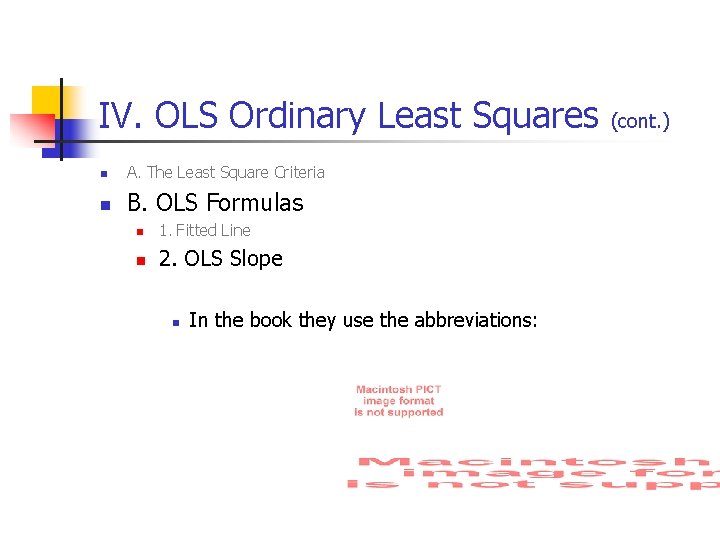
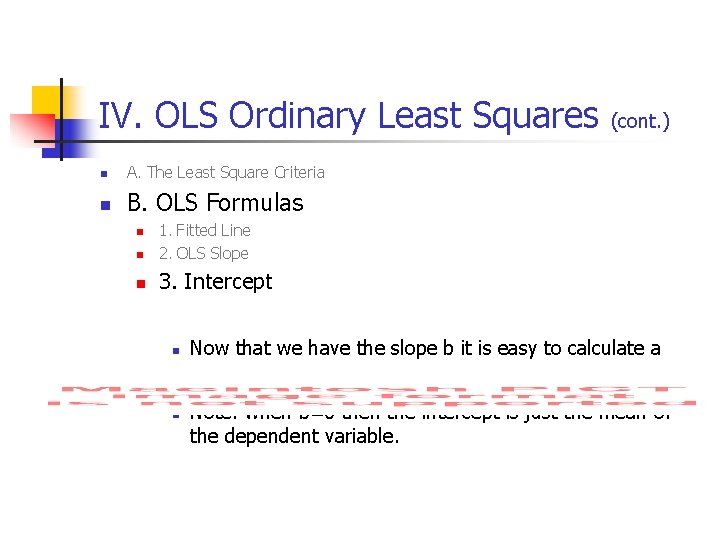
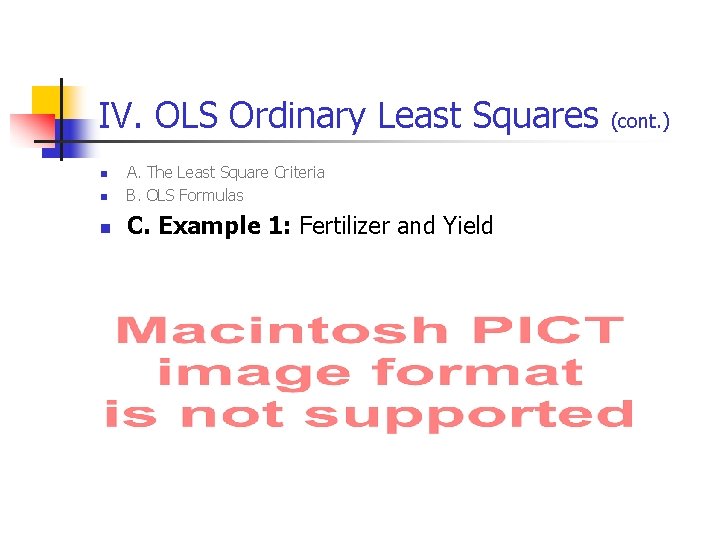
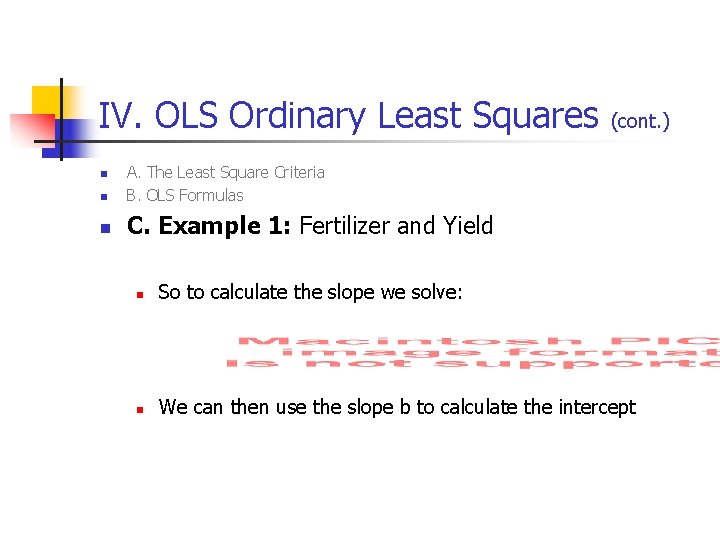
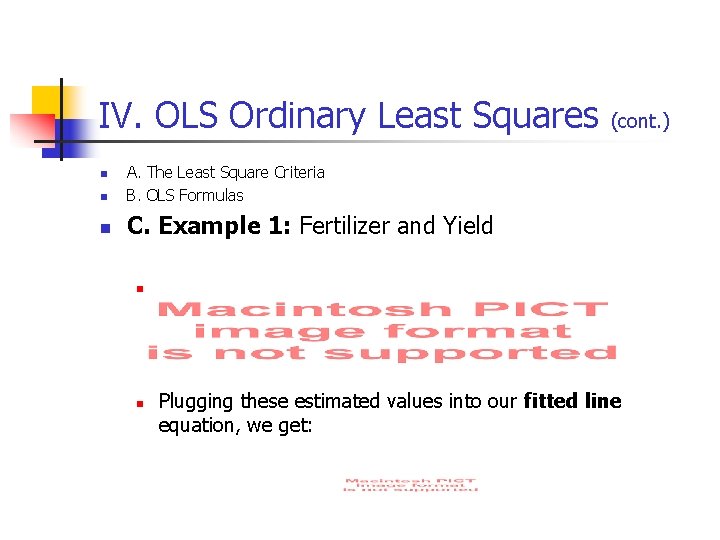
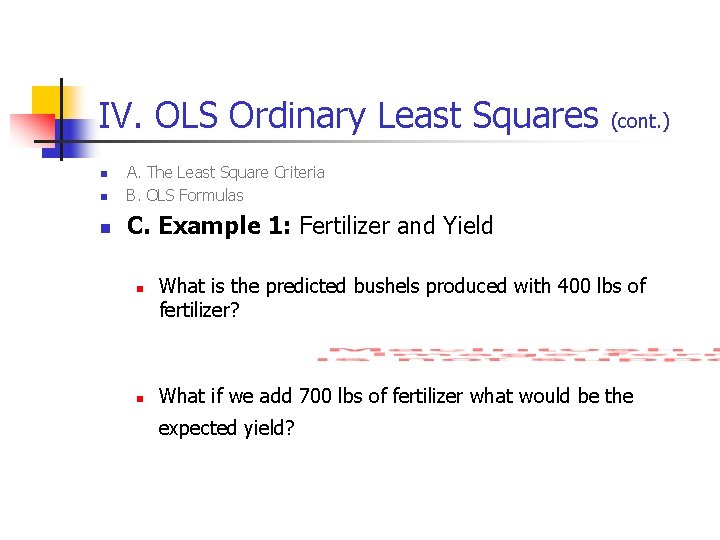
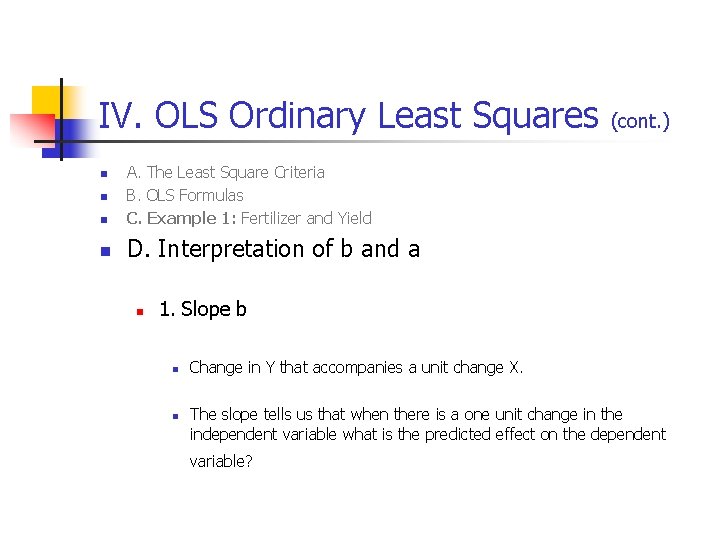
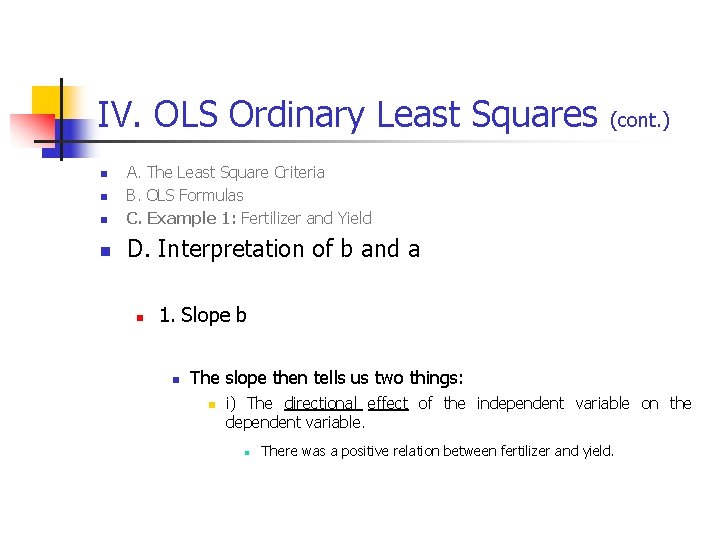
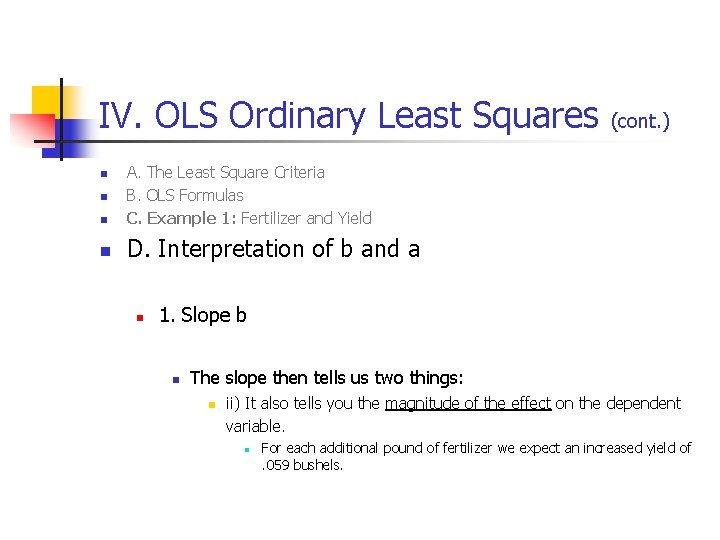
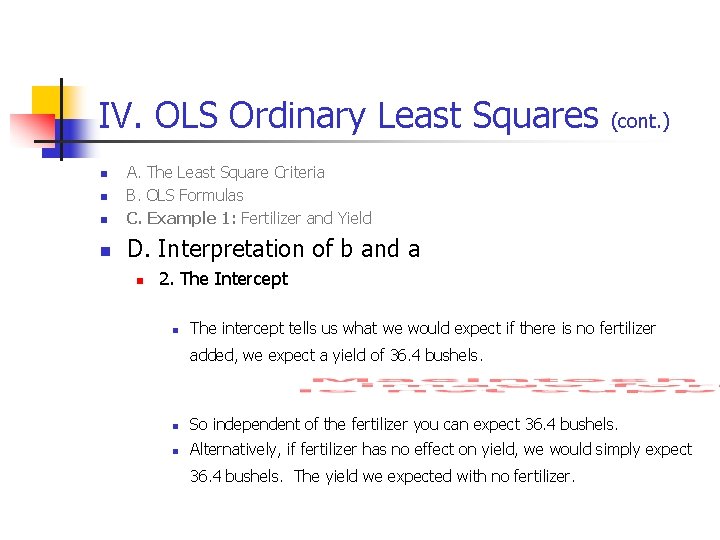
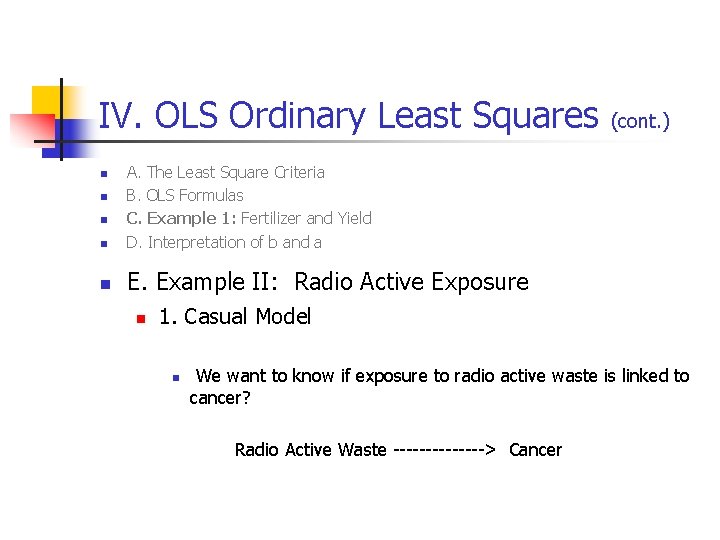
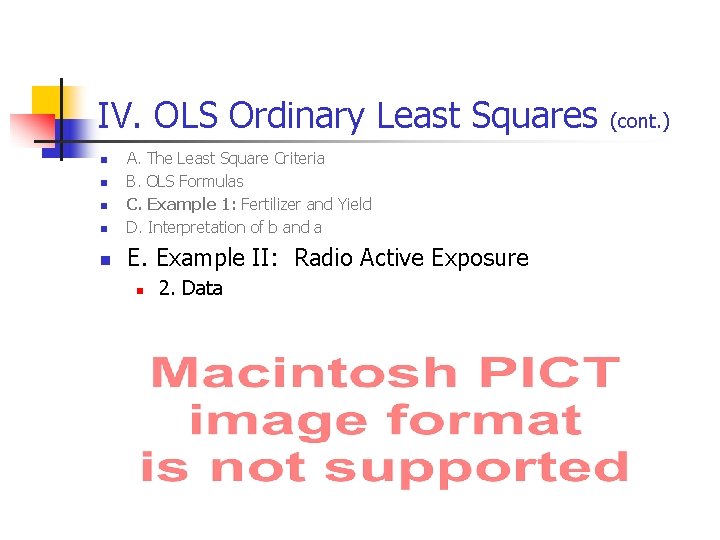
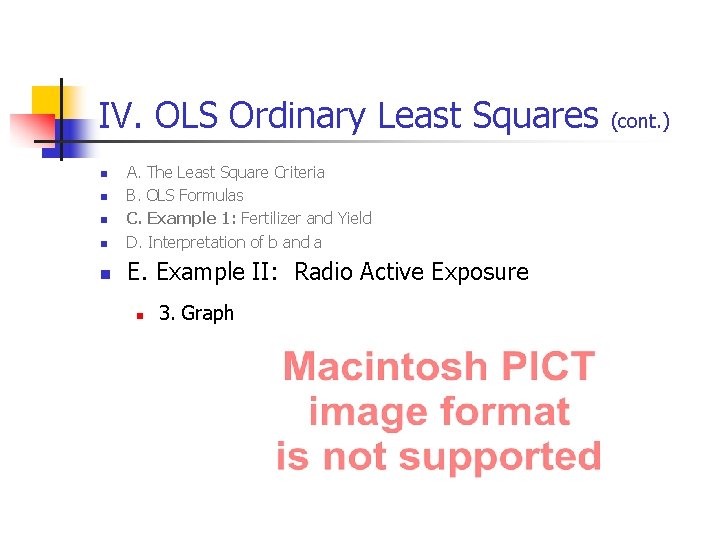
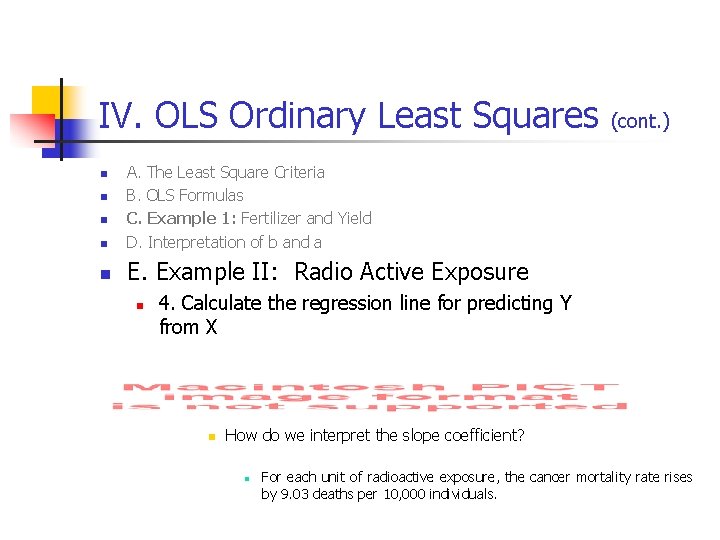
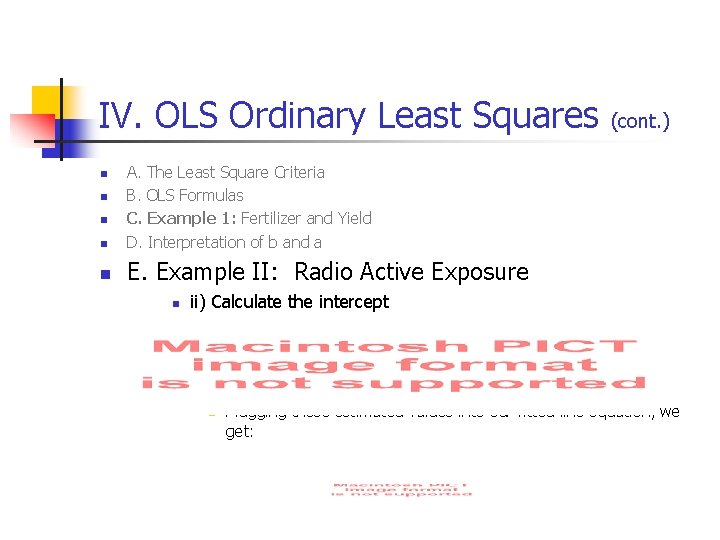
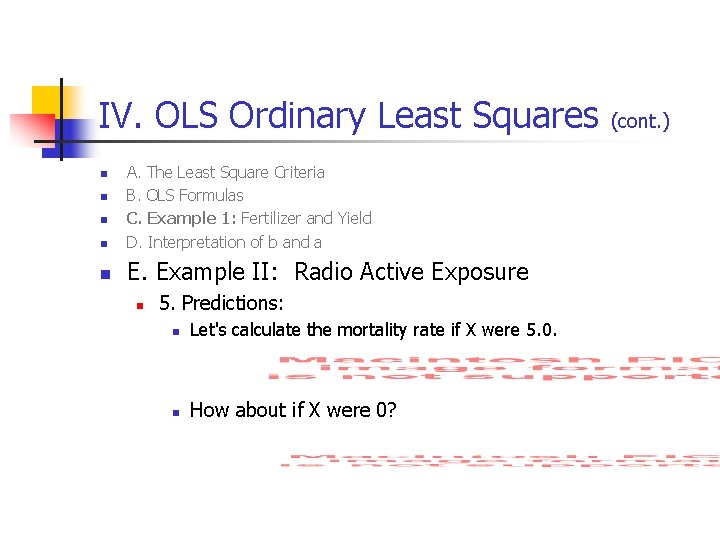
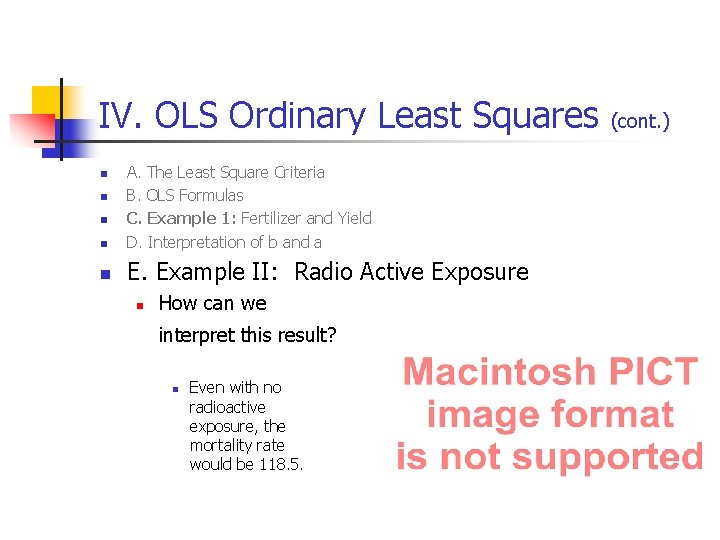
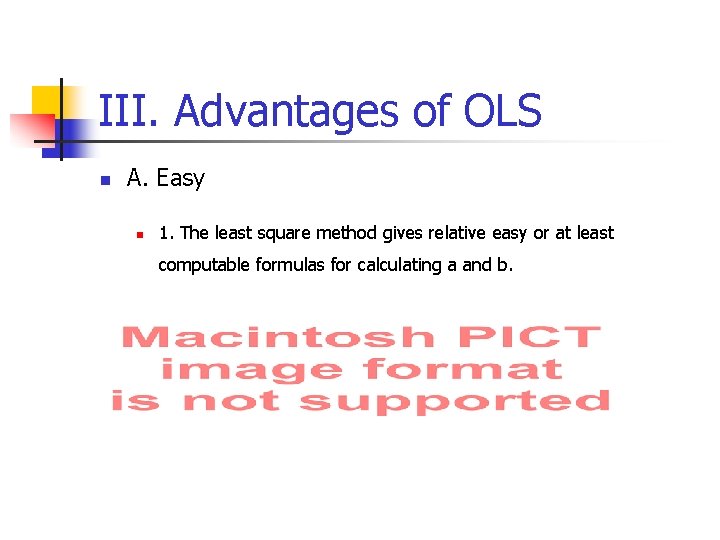
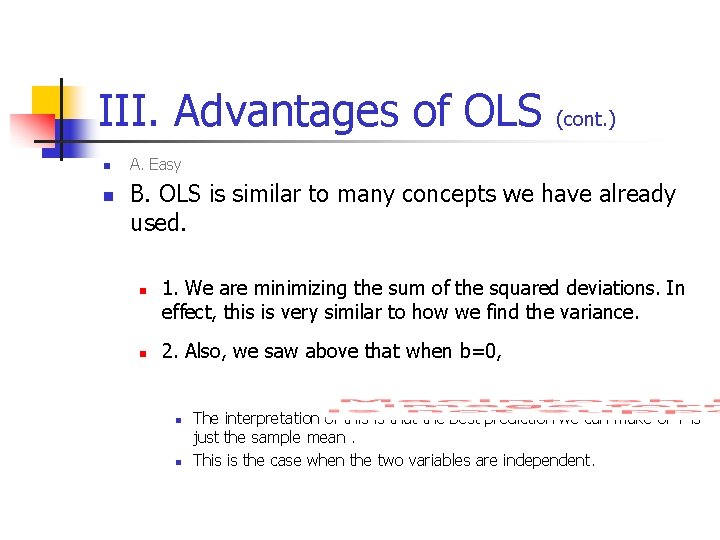
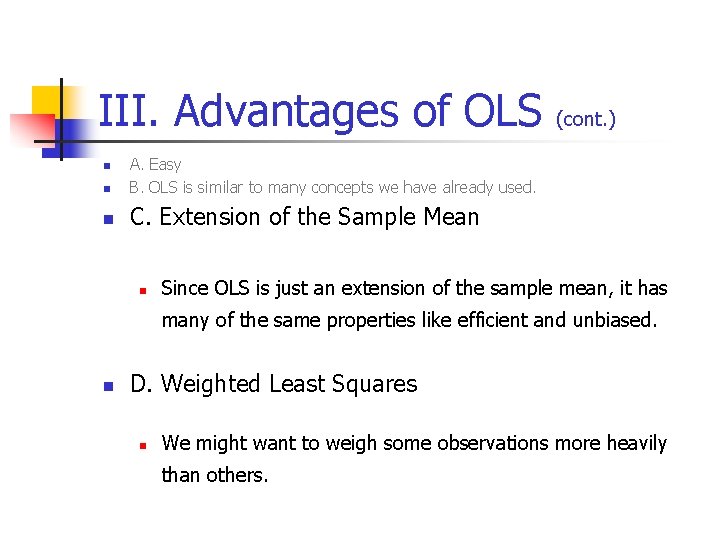
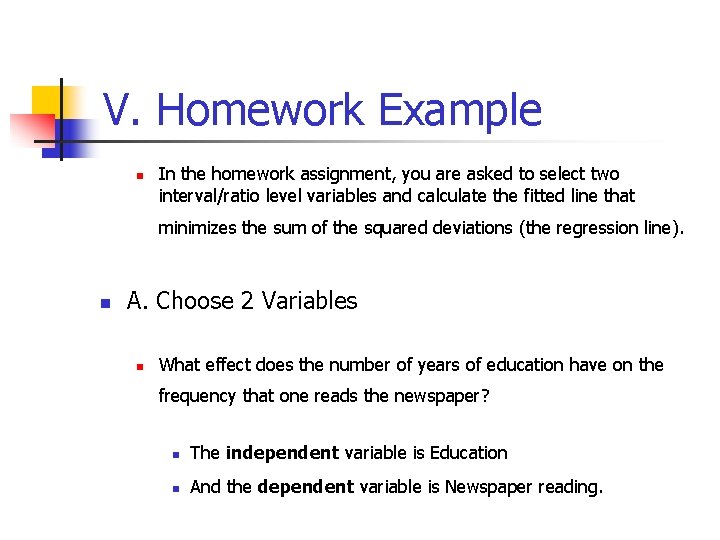
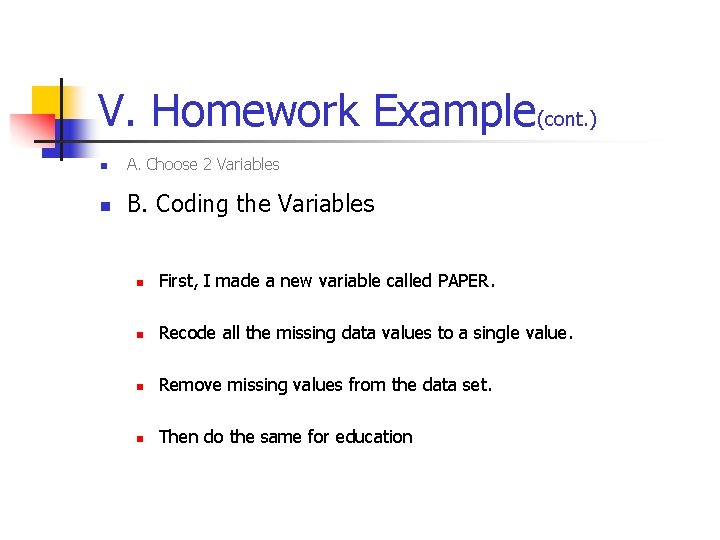
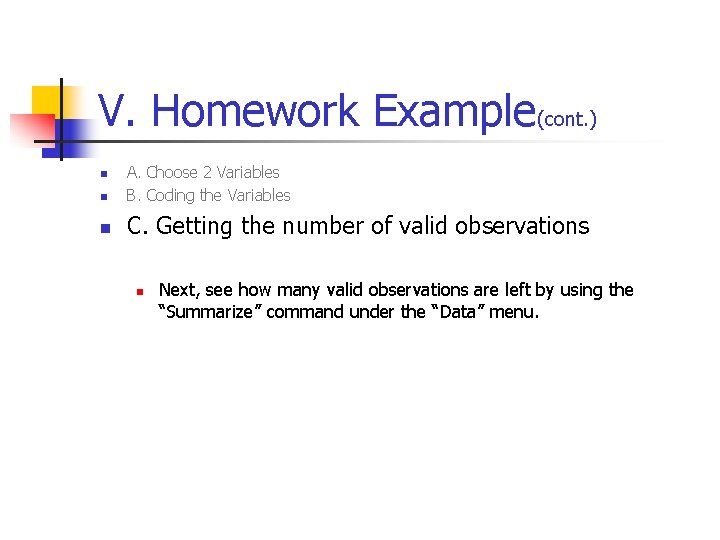
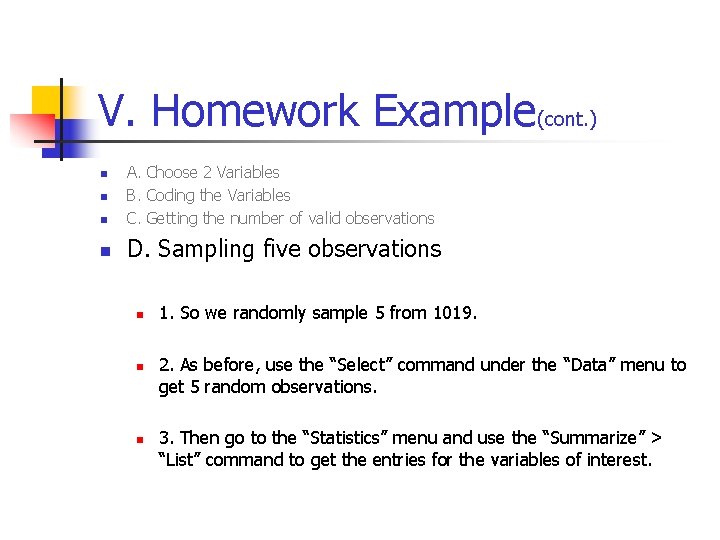
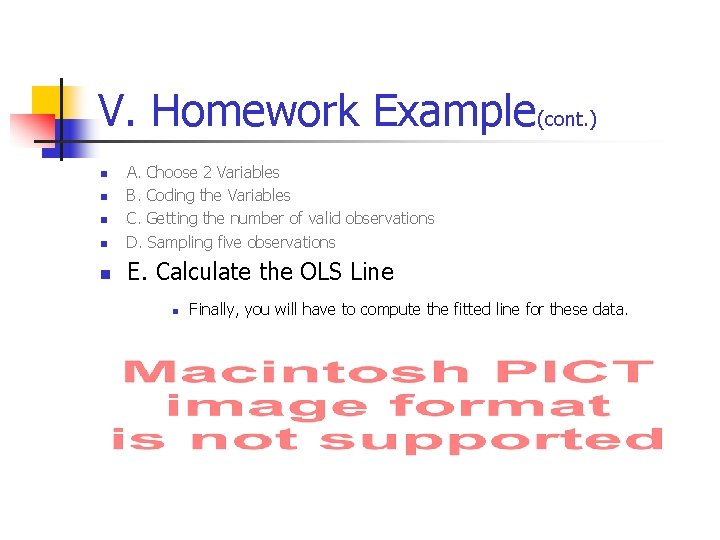
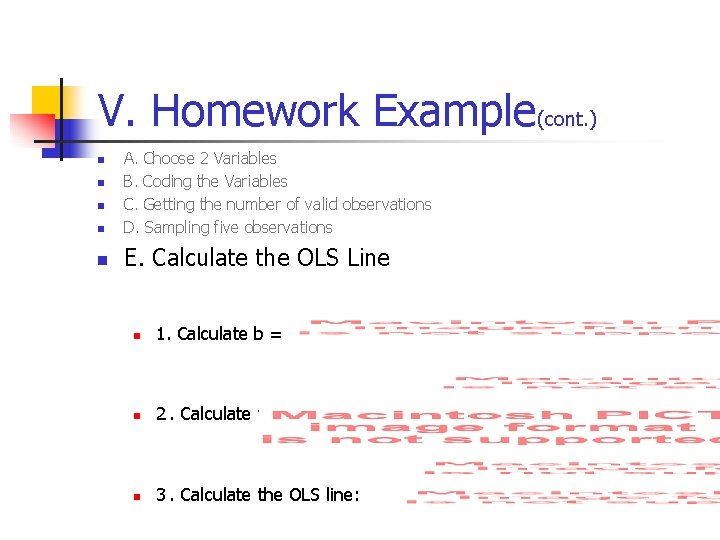
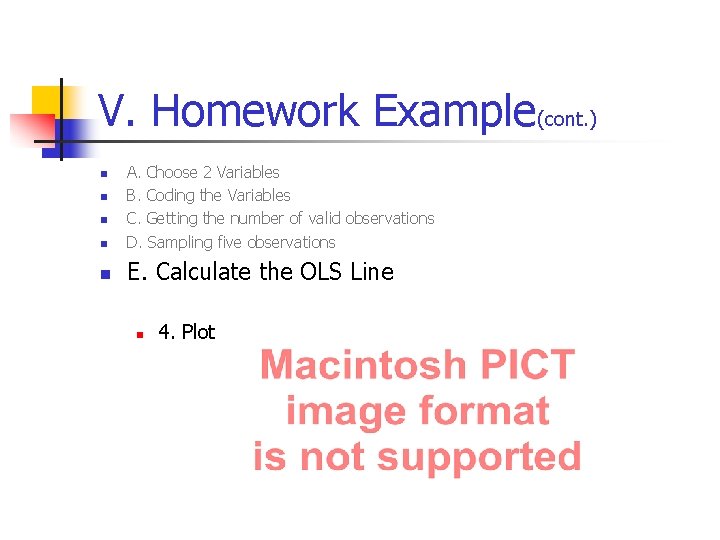
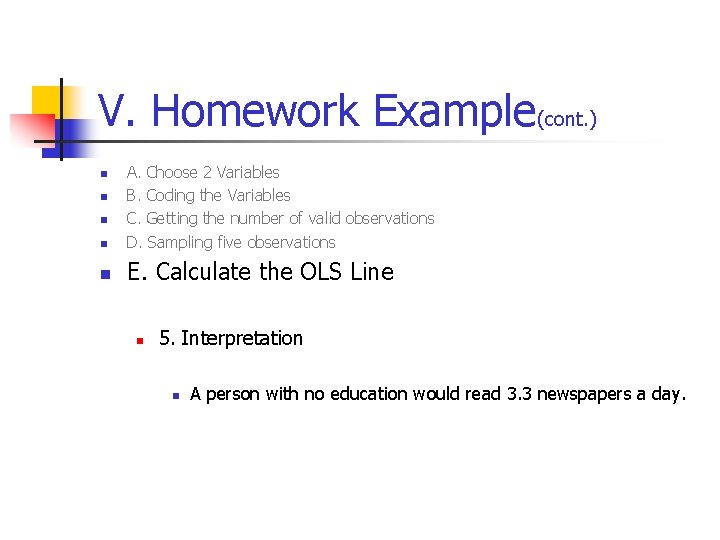
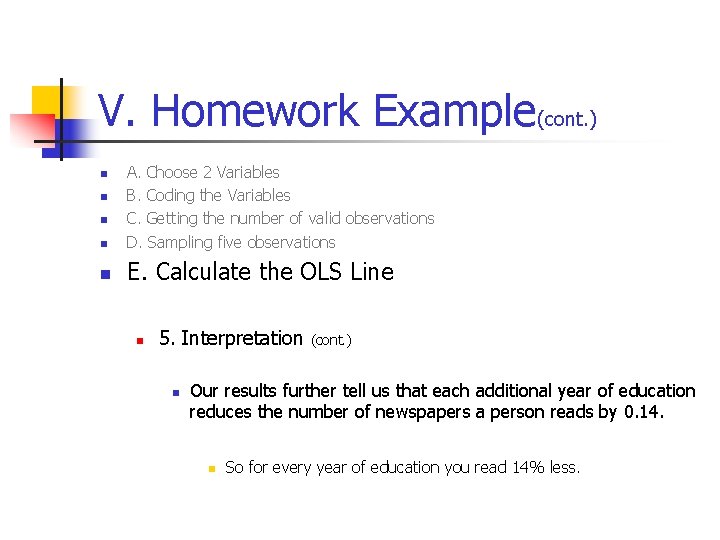
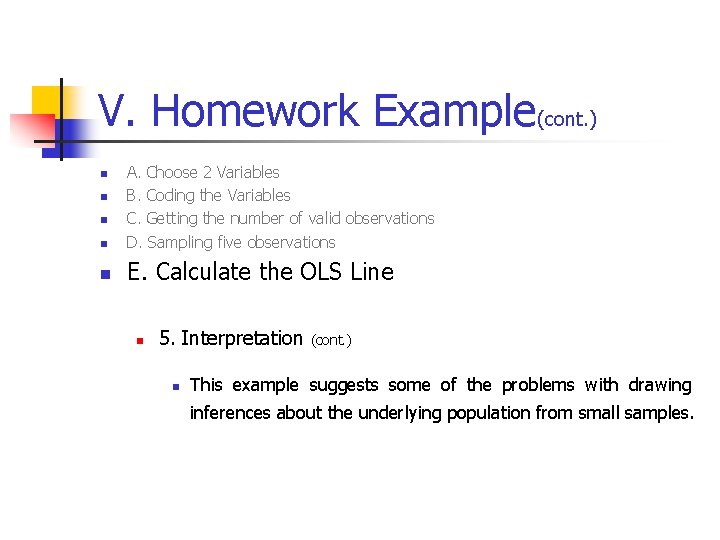
- Slides: 57
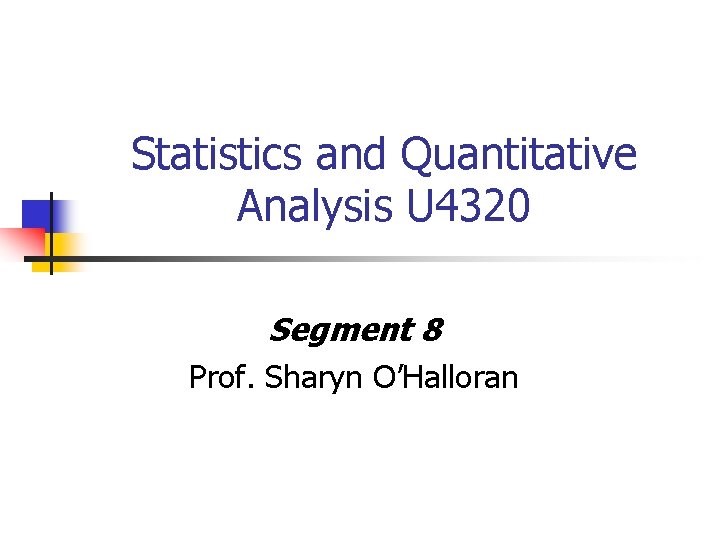
Statistics and Quantitative Analysis U 4320 Segment 8 Prof. Sharyn O’Halloran
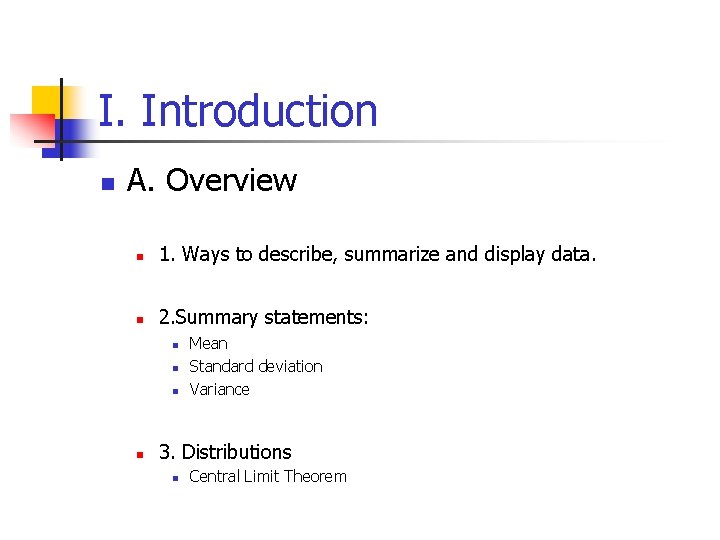
I. Introduction n A. Overview n 1. Ways to describe, summarize and display data. n 2. Summary statements: n n Mean Standard deviation Variance 3. Distributions n Central Limit Theorem
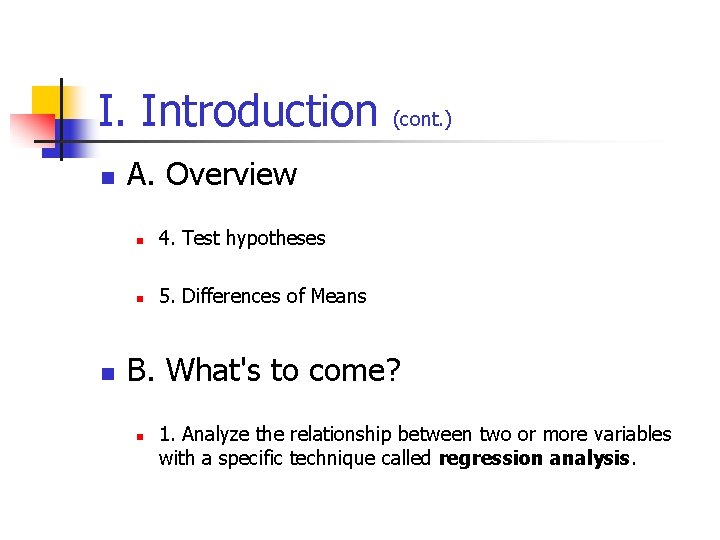
I. Introduction n n (cont. ) A. Overview n 4. Test hypotheses n 5. Differences of Means B. What's to come? n 1. Analyze the relationship between two or more variables with a specific technique called regression analysis.
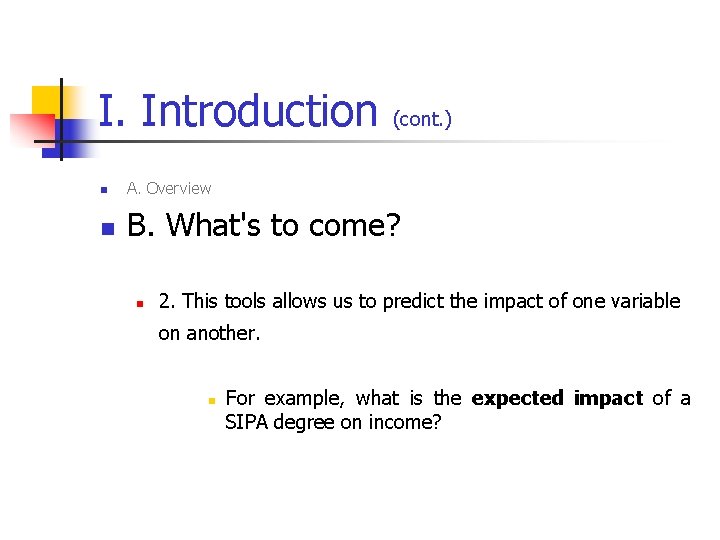
I. Introduction (cont. ) n A. Overview n B. What's to come? n 2. This tools allows us to predict the impact of one variable on another. n For example, what is the expected impact of a SIPA degree on income?
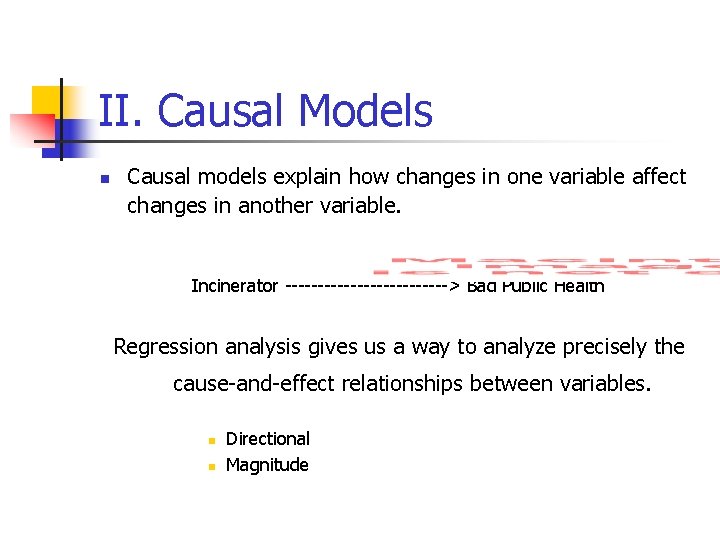
II. Causal Models n Causal models explain how changes in one variable affect changes in another variable. Incinerator -------------> Bad Public Health Regression analysis gives us a way to analyze precisely the cause-and-effect relationships between variables. n n Directional Magnitude
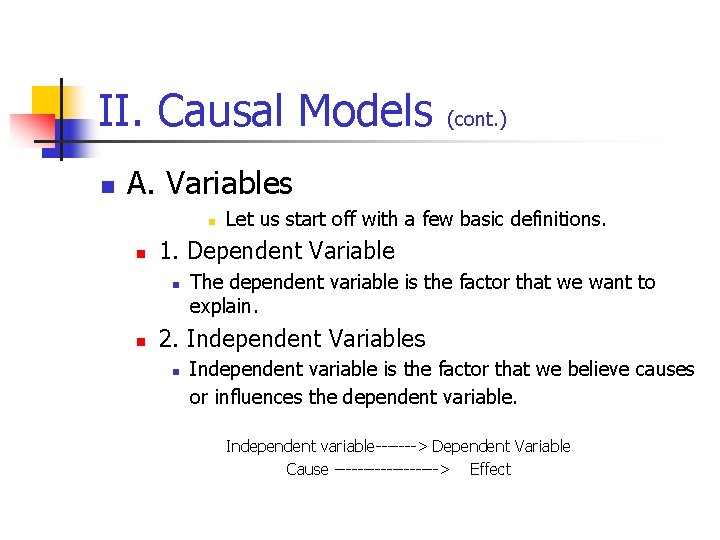
II. Causal Models n (cont. ) A. Variables n n 1. Dependent Variable n n Let us start off with a few basic definitions. The dependent variable is the factor that we want to explain. 2. Independent Variables n Independent variable is the factor that we believe causes or influences the dependent variable. Independent variable-------> Dependent Variable Cause ---------> Effect
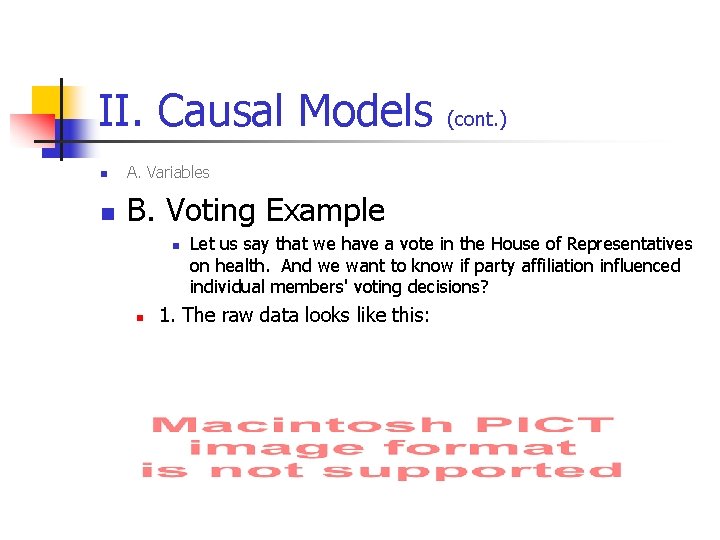
II. Causal Models n A. Variables n B. Voting Example n n (cont. ) Let us say that we have a vote in the House of Representatives on health. And we want to know if party affiliation influenced individual members' voting decisions? 1. The raw data looks like this:
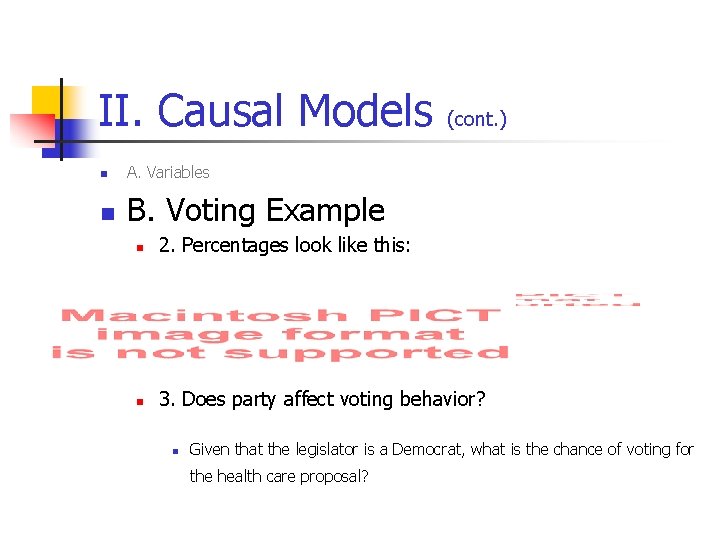
II. Causal Models n A. Variables n B. Voting Example (cont. ) n 2. Percentages look like this: n 3. Does party affect voting behavior? n Given that the legislator is a Democrat, what is the chance of voting for the health care proposal?
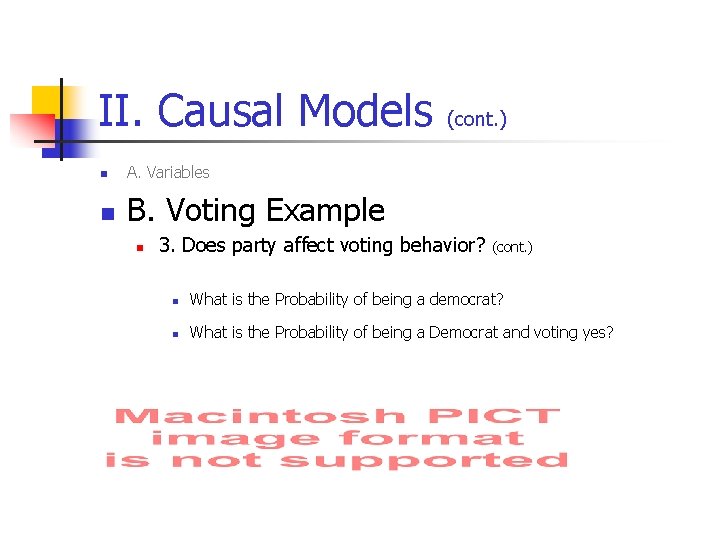
II. Causal Models n A. Variables n B. Voting Example n (cont. ) 3. Does party affect voting behavior? (cont. ) n What is the Probability of being a democrat? n What is the Probability of being a Democrat and voting yes?
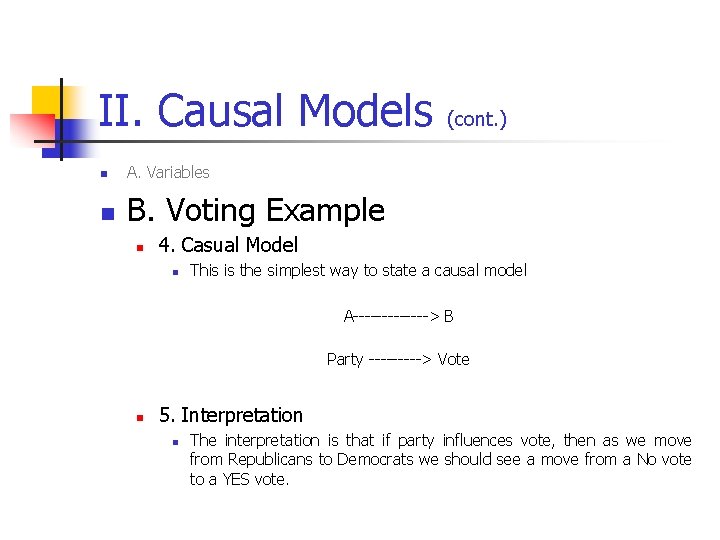
II. Causal Models n A. Variables n B. Voting Example n (cont. ) 4. Casual Model n This is the simplest way to state a causal model A-------> B Party -----> Vote n 5. Interpretation n The interpretation is that if party influences vote, then as we move from Republicans to Democrats we should see a move from a No vote to a YES vote.
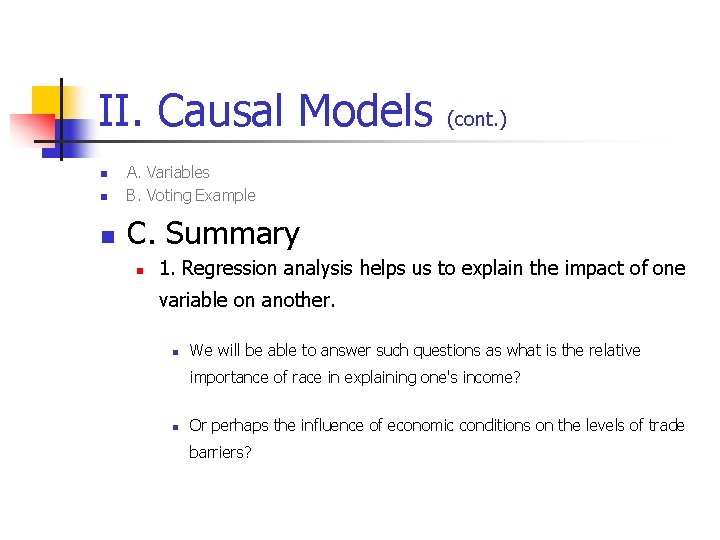
II. Causal Models n A. Variables B. Voting Example n C. Summary n n (cont. ) 1. Regression analysis helps us to explain the impact of one variable on another. n We will be able to answer such questions as what is the relative importance of race in explaining one's income? n Or perhaps the influence of economic conditions on the levels of trade barriers?
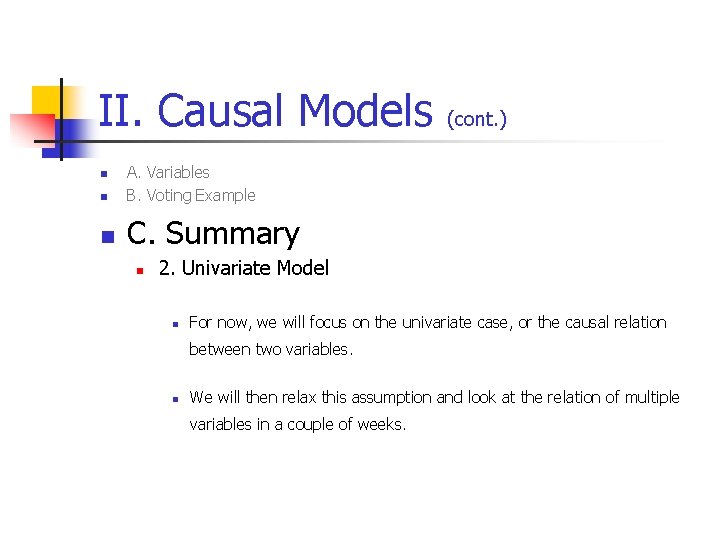
II. Causal Models n A. Variables B. Voting Example n C. Summary n n (cont. ) 2. Univariate Model n For now, we will focus on the univariate case, or the causal relation between two variables. n We will then relax this assumption and look at the relation of multiple variables in a couple of weeks.
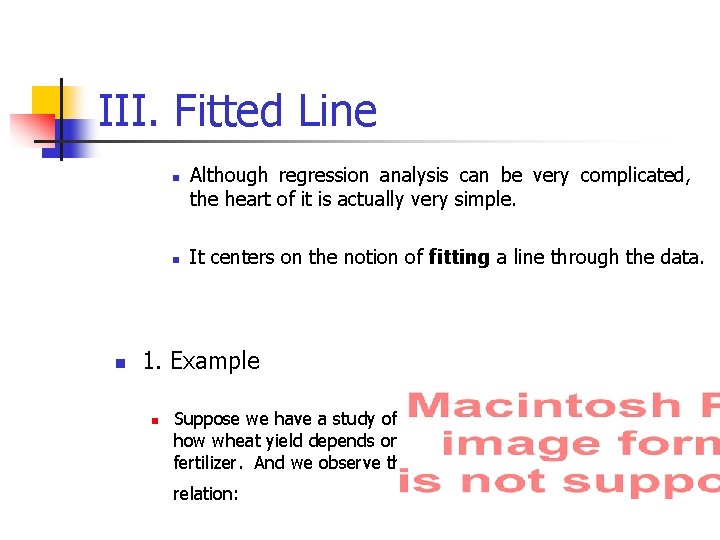
III. Fitted Line n n n Although regression analysis can be very complicated, the heart of it is actually very simple. It centers on the notion of fitting a line through the data. 1. Example n Suppose we have a study of how wheat yield depends on fertilizer. And we observe this relation:
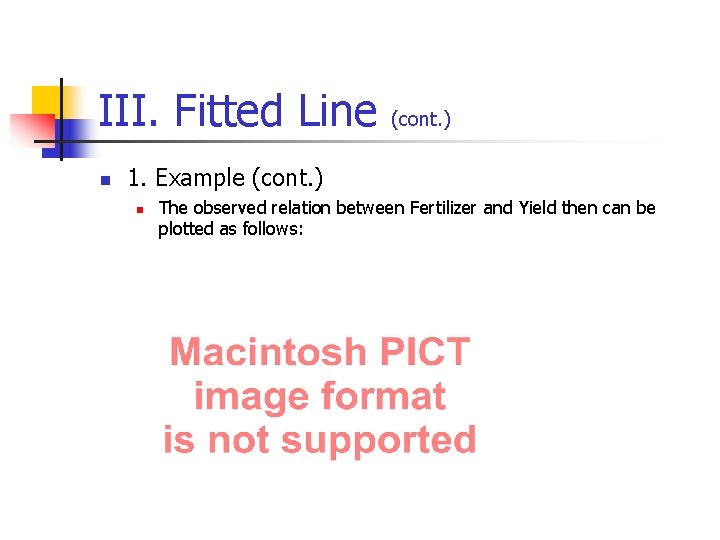
III. Fitted Line n (cont. ) 1. Example (cont. ) n The observed relation between Fertilizer and Yield then can be plotted as follows:
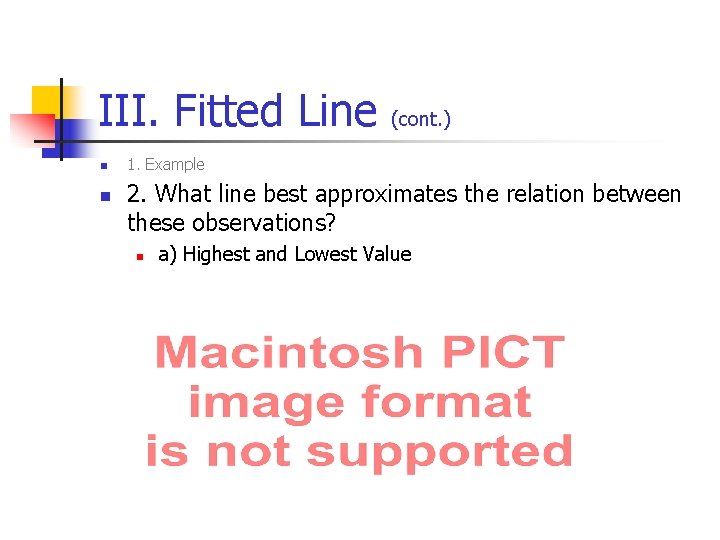
III. Fitted Line n n (cont. ) 1. Example 2. What line best approximates the relation between these observations? n a) Highest and Lowest Value
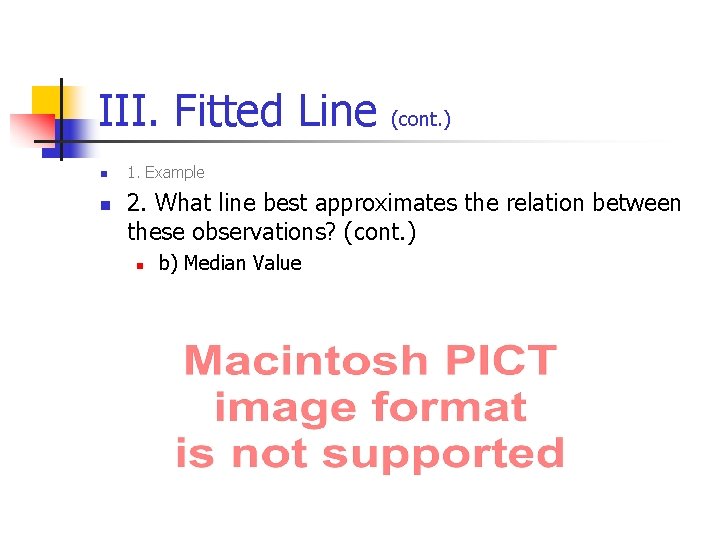
III. Fitted Line n n (cont. ) 1. Example 2. What line best approximates the relation between these observations? (cont. ) n b) Median Value
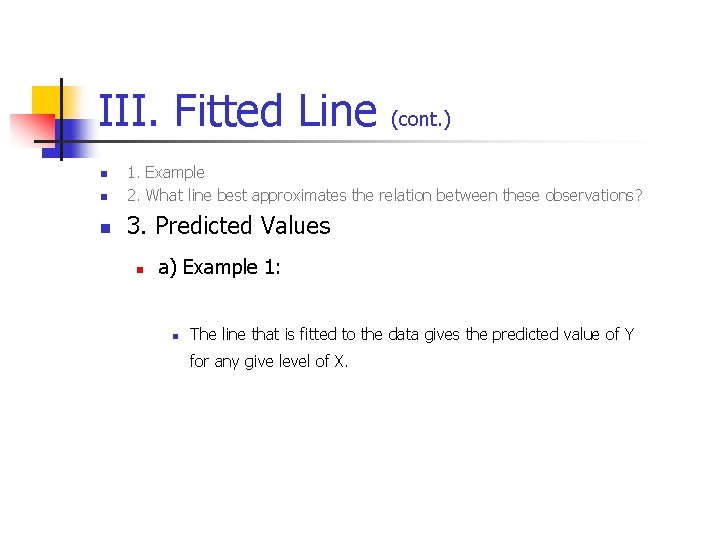
III. Fitted Line (cont. ) n 1. Example 2. What line best approximates the relation between these observations? n 3. Predicted Values n n a) Example 1: n The line that is fitted to the data gives the predicted value of Y for any give level of X.
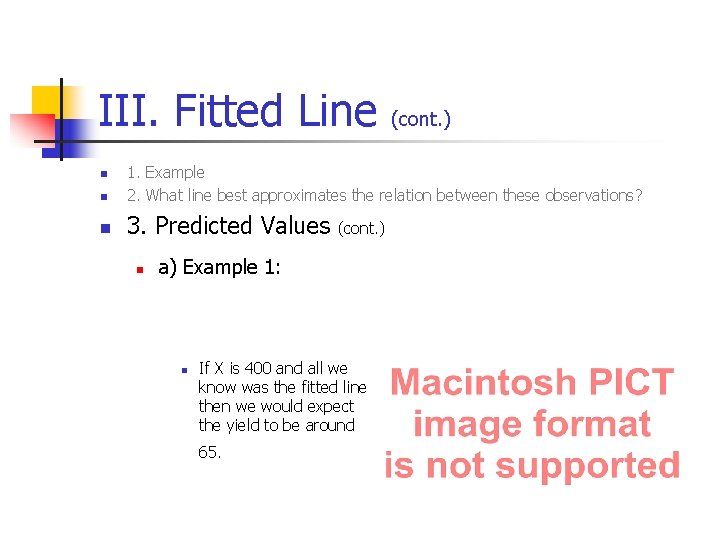
III. Fitted Line (cont. ) n 1. Example 2. What line best approximates the relation between these observations? n 3. Predicted Values n n (cont. ) a) Example 1: n If X is 400 and all we know was the fitted line then we would expect the yield to be around 65.
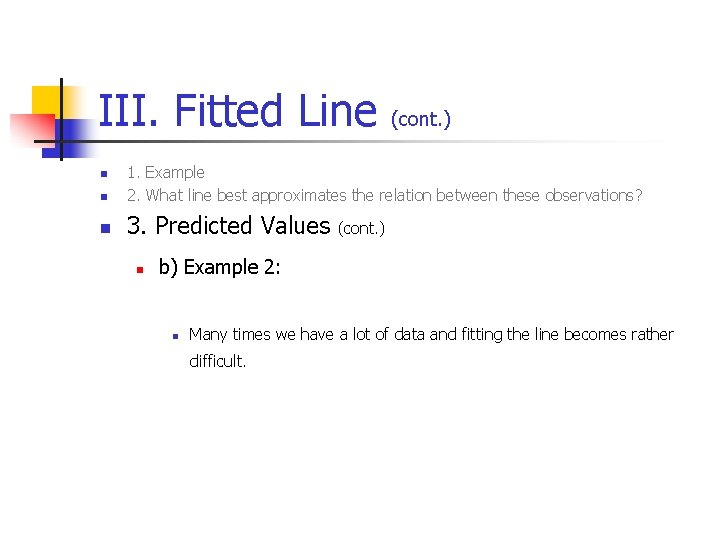
III. Fitted Line (cont. ) n 1. Example 2. What line best approximates the relation between these observations? n 3. Predicted Values n n (cont. ) b) Example 2: n Many times we have a lot of data and fitting the line becomes rather difficult.
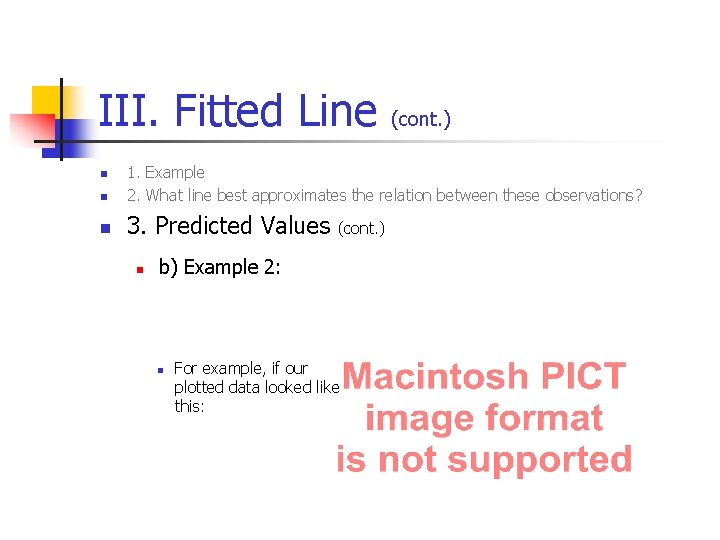
III. Fitted Line (cont. ) n 1. Example 2. What line best approximates the relation between these observations? n 3. Predicted Values n n (cont. ) b) Example 2: n For example, if our plotted data looked like this:
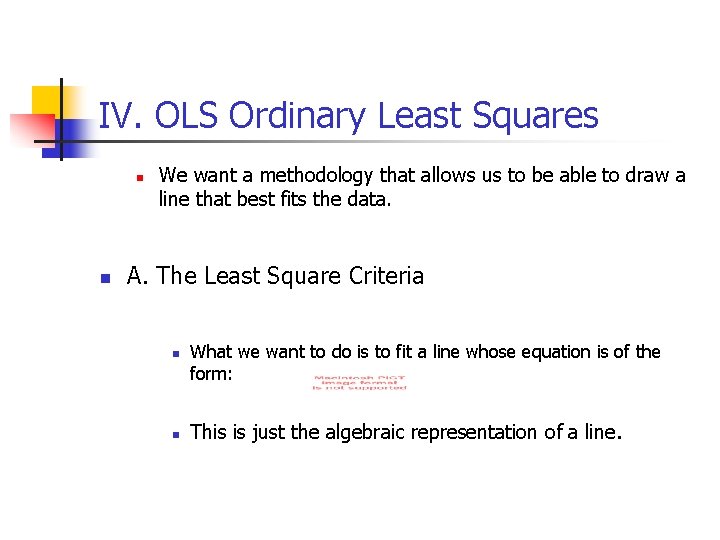
IV. OLS Ordinary Least Squares n n We want a methodology that allows us to be able to draw a line that best fits the data. A. The Least Square Criteria n n What we want to do is to fit a line whose equation is of the form: This is just the algebraic representation of a line.
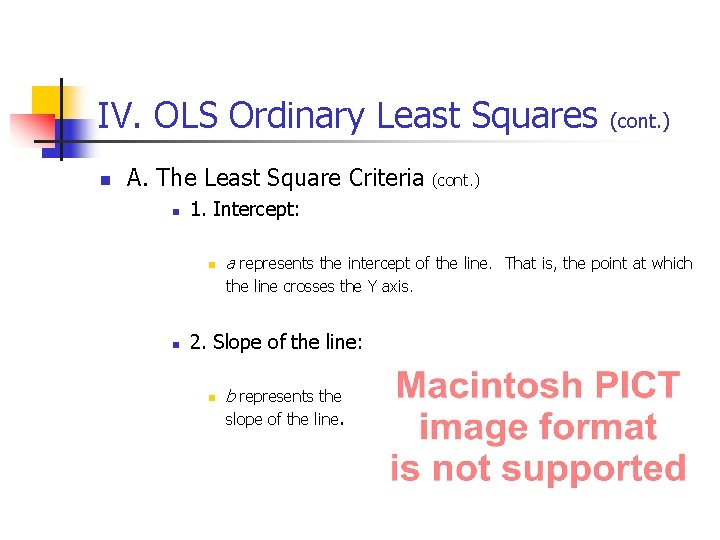
IV. OLS Ordinary Least Squares n A. The Least Square Criteria n (cont. ) 1. Intercept: n a represents the intercept of the line. That is, the point at which the line crosses the Y axis. n (cont. ) 2. Slope of the line: n b represents the slope of the line.
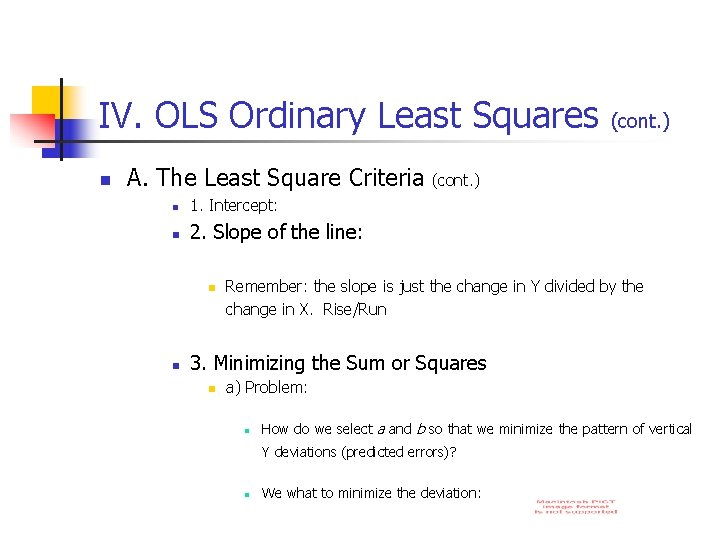
IV. OLS Ordinary Least Squares n A. The Least Square Criteria n 1. Intercept: n 2. Slope of the line: n n (cont. ) Remember: the slope is just the change in Y divided by the change in X. Rise/Run 3. Minimizing the Sum or Squares n a) Problem: n How do we select a and b so that we minimize the pattern of vertical Y deviations (predicted errors)? n We what to minimize the deviation:
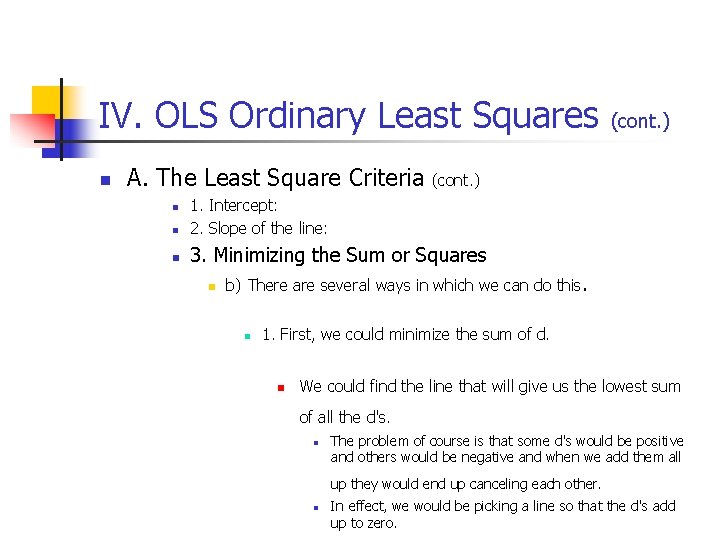
IV. OLS Ordinary Least Squares n A. The Least Square Criteria (cont. ) n 1. Intercept: 2. Slope of the line: n 3. Minimizing the Sum or Squares n n (cont. ) b) There are several ways in which we can do this. n 1. First, we could minimize the sum of d. n We could find the line that will give us the lowest sum of all the d's. n The problem of course is that some d's would be positive and others would be negative and when we add them all up they would end up canceling each other. n In effect, we would be picking a line so that the d's add up to zero.
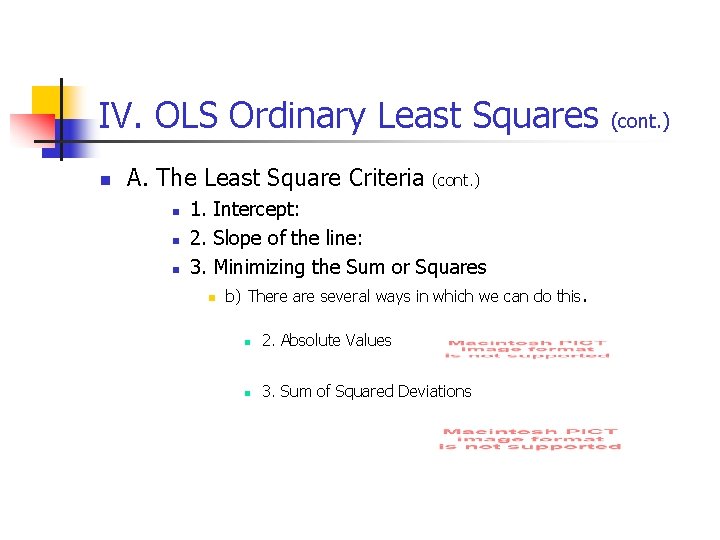
IV. OLS Ordinary Least Squares n A. The Least Square Criteria n n n (cont. ) 1. Intercept: 2. Slope of the line: 3. Minimizing the Sum or Squares n b) There are several ways in which we can do this. n 2. Absolute Values n 3. Sum of Squared Deviations (cont. )
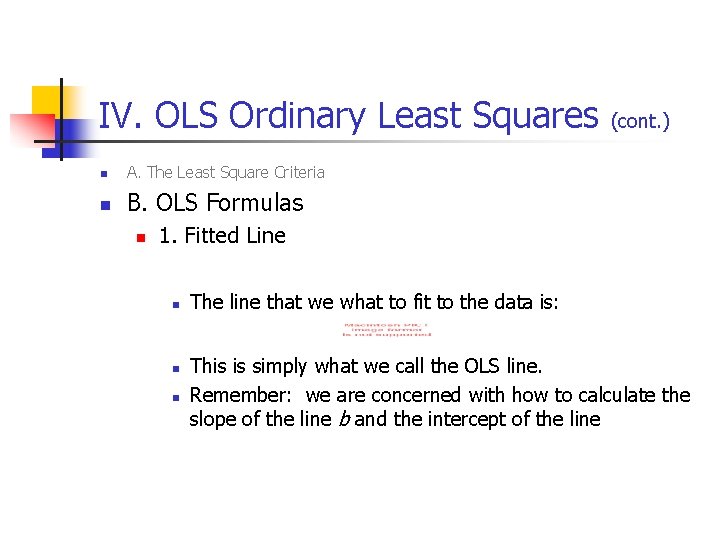
IV. OLS Ordinary Least Squares n A. The Least Square Criteria n B. OLS Formulas n (cont. ) 1. Fitted Line n n n The line that we what to fit to the data is: This is simply what we call the OLS line. Remember: we are concerned with how to calculate the slope of the line b and the intercept of the line
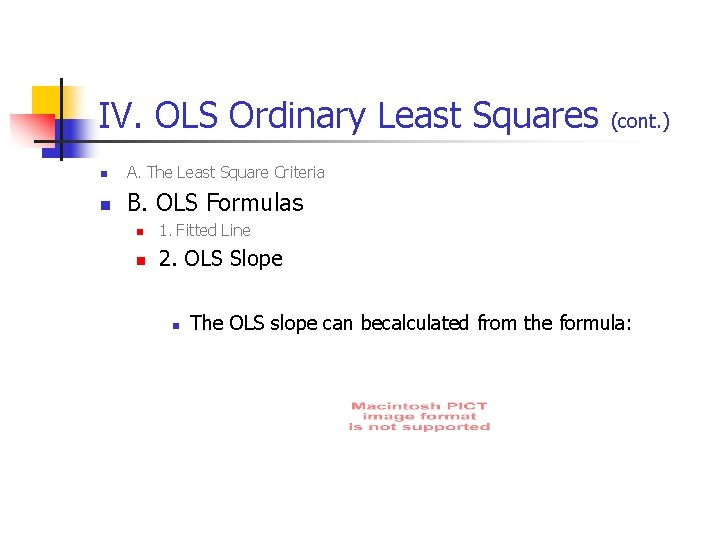
IV. OLS Ordinary Least Squares n A. The Least Square Criteria n B. OLS Formulas n 1. Fitted Line n 2. OLS Slope n (cont. ) The OLS slope can becalculated from the formula:
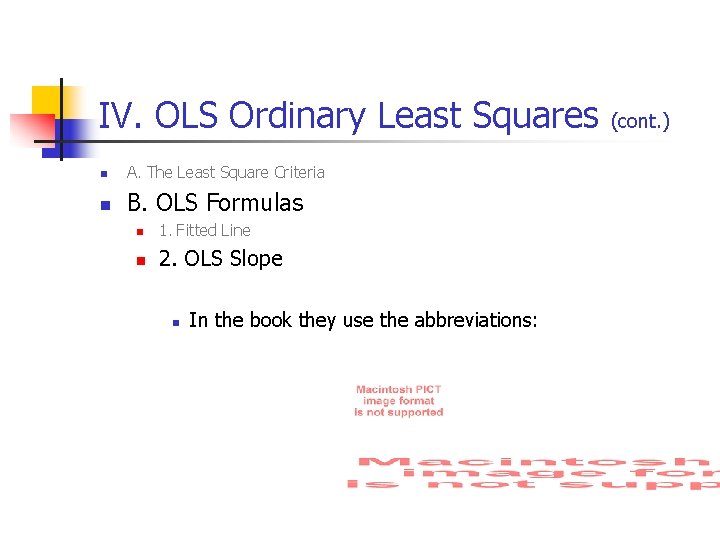
IV. OLS Ordinary Least Squares n A. The Least Square Criteria n B. OLS Formulas n 1. Fitted Line n 2. OLS Slope n In the book they use the abbreviations: (cont. )
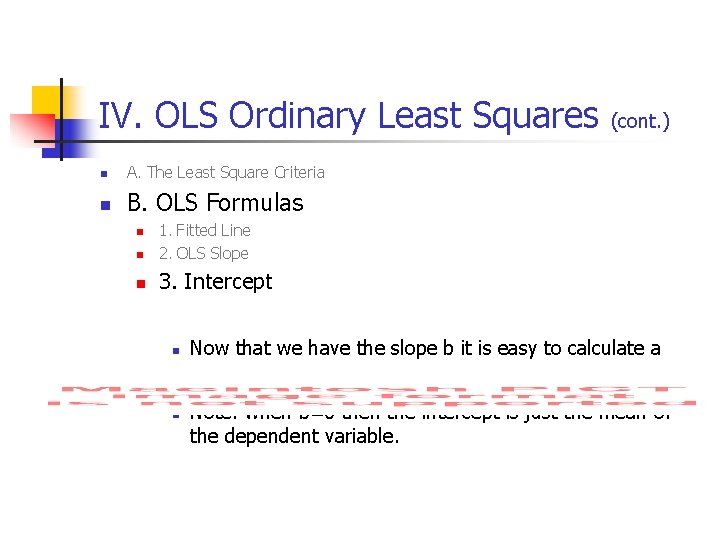
IV. OLS Ordinary Least Squares n A. The Least Square Criteria n B. OLS Formulas n 1. Fitted Line 2. OLS Slope n 3. Intercept n n n (cont. ) Now that we have the slope b it is easy to calculate a Note: when b=0 then the intercept is just the mean of the dependent variable.
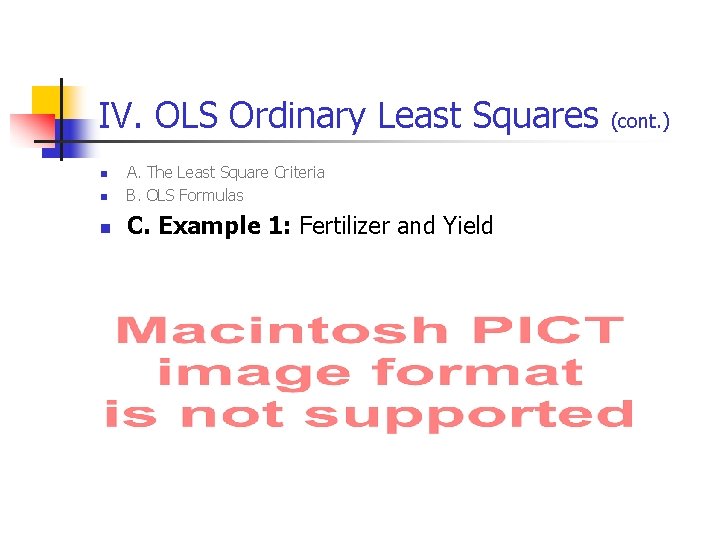
IV. OLS Ordinary Least Squares n A. The Least Square Criteria B. OLS Formulas n C. Example 1: Fertilizer and Yield n (cont. )
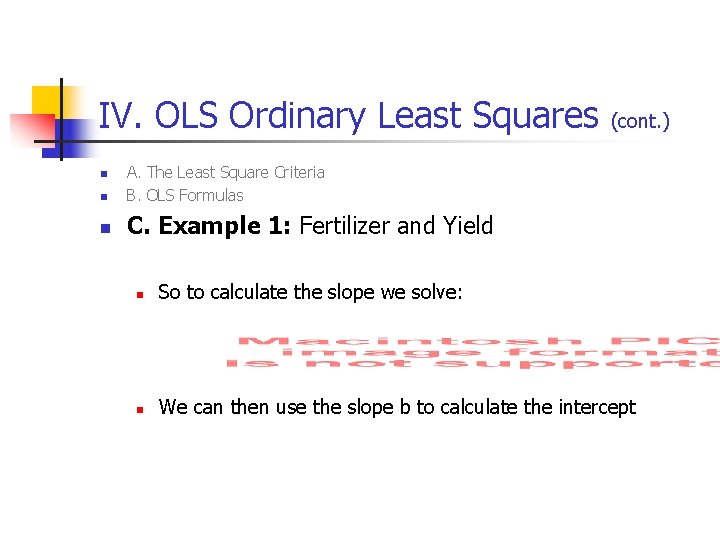
IV. OLS Ordinary Least Squares n A. The Least Square Criteria B. OLS Formulas n C. Example 1: Fertilizer and Yield n (cont. ) n So to calculate the slope we solve: n We can then use the slope b to calculate the intercept
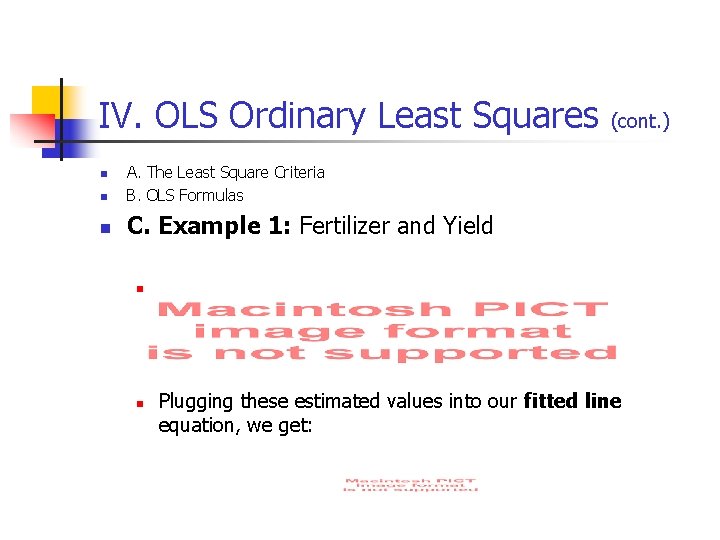
IV. OLS Ordinary Least Squares n A. The Least Square Criteria B. OLS Formulas n C. Example 1: Fertilizer and Yield n n n (cont. ) Remember: Plugging these estimated values into our fitted line equation, we get:
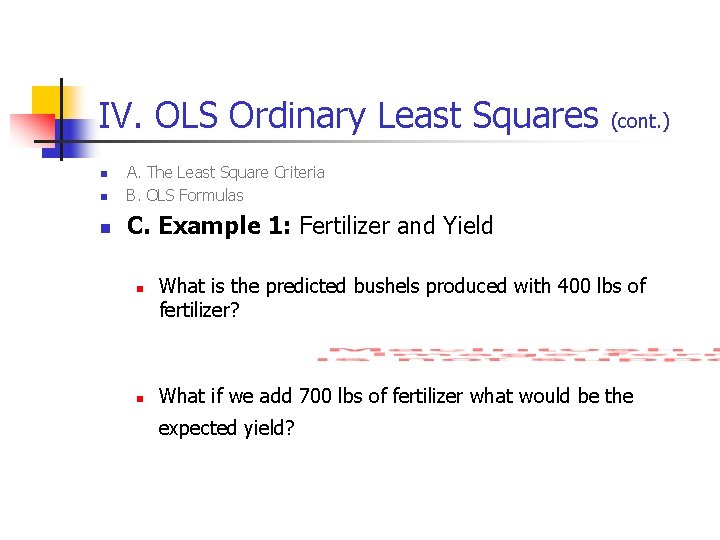
IV. OLS Ordinary Least Squares n A. The Least Square Criteria B. OLS Formulas n C. Example 1: Fertilizer and Yield n n n (cont. ) What is the predicted bushels produced with 400 lbs of fertilizer? What if we add 700 lbs of fertilizer what would be the expected yield?
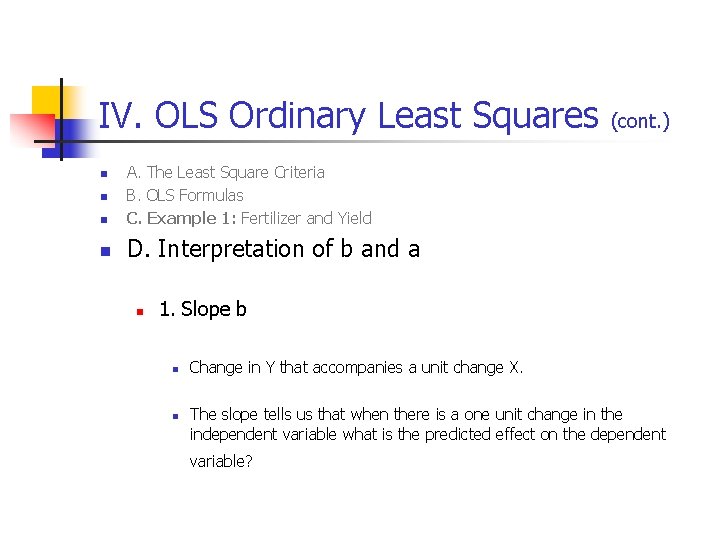
IV. OLS Ordinary Least Squares n A. The Least Square Criteria B. OLS Formulas C. Example 1: Fertilizer and Yield n D. Interpretation of b and a n n n (cont. ) 1. Slope b n n Change in Y that accompanies a unit change X. The slope tells us that when there is a one unit change in the independent variable what is the predicted effect on the dependent variable?
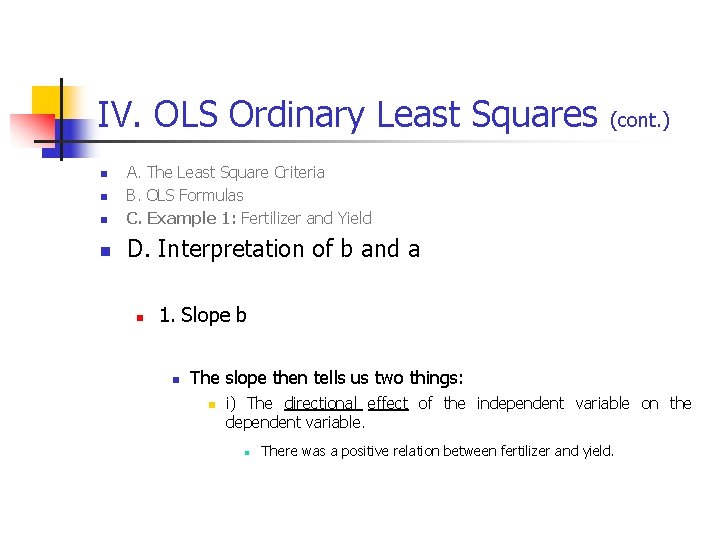
IV. OLS Ordinary Least Squares n A. The Least Square Criteria B. OLS Formulas C. Example 1: Fertilizer and Yield n D. Interpretation of b and a n n n (cont. ) 1. Slope b n The slope then tells us two things: n i) The directional effect of the independent variable on the dependent variable. n There was a positive relation between fertilizer and yield.
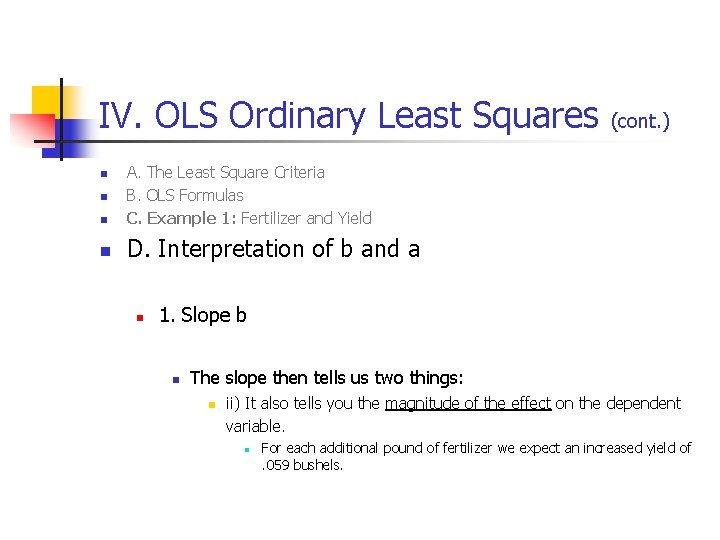
IV. OLS Ordinary Least Squares n A. The Least Square Criteria B. OLS Formulas C. Example 1: Fertilizer and Yield n D. Interpretation of b and a n n n (cont. ) 1. Slope b n The slope then tells us two things: n ii) It also tells you the magnitude of the effect on the dependent variable. n For each additional pound of fertilizer we expect an increased yield of. 059 bushels.
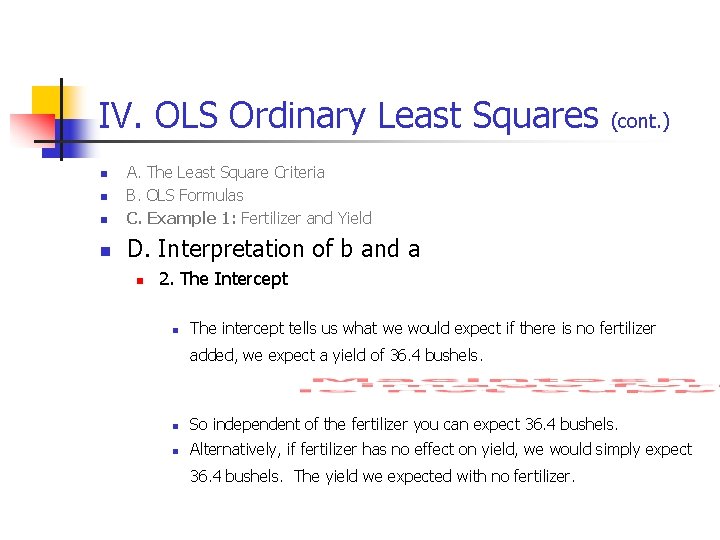
IV. OLS Ordinary Least Squares n A. The Least Square Criteria B. OLS Formulas C. Example 1: Fertilizer and Yield n D. Interpretation of b and a n n n (cont. ) 2. The Intercept n The intercept tells us what we would expect if there is no fertilizer added, we expect a yield of 36. 4 bushels. n So independent of the fertilizer you can expect 36. 4 bushels. n Alternatively, if fertilizer has no effect on yield, we would simply expect 36. 4 bushels. The yield we expected with no fertilizer.
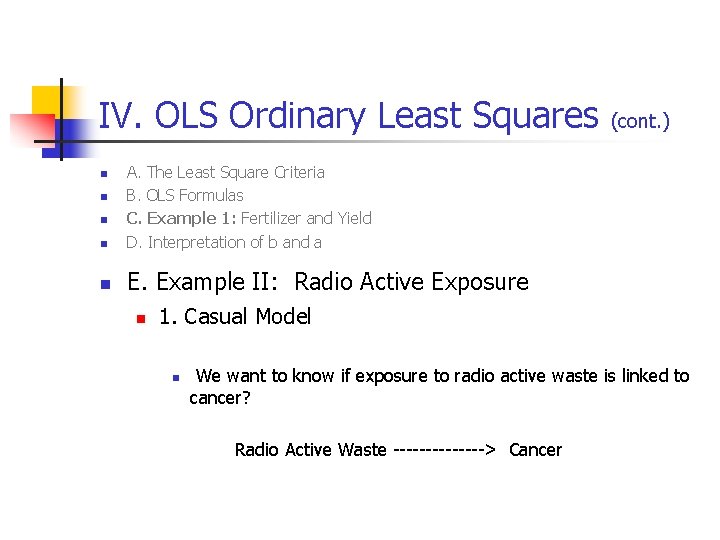
IV. OLS Ordinary Least Squares n A. The Least Square Criteria B. OLS Formulas C. Example 1: Fertilizer and Yield D. Interpretation of b and a n E. Example II: Radio Active Exposure n n (cont. ) 1. Casual Model n We want to know if exposure to radio active waste is linked to cancer? Radio Active Waste -------> Cancer
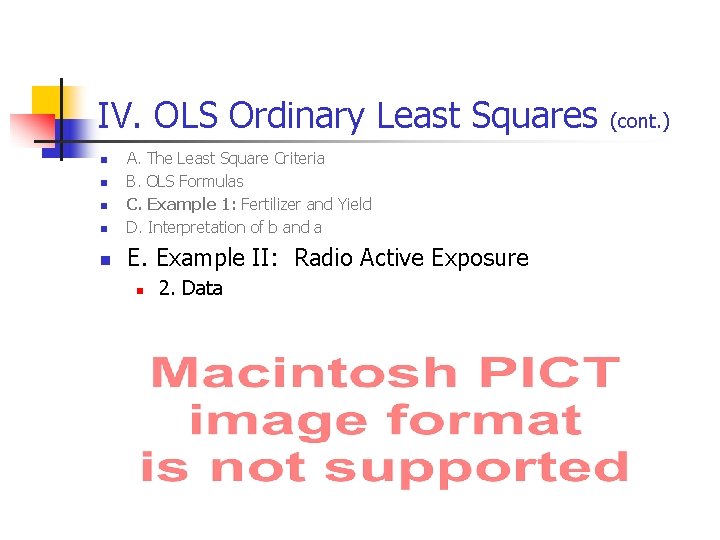
IV. OLS Ordinary Least Squares n A. The Least Square Criteria B. OLS Formulas C. Example 1: Fertilizer and Yield D. Interpretation of b and a n E. Example II: Radio Active Exposure n n 2. Data (cont. )
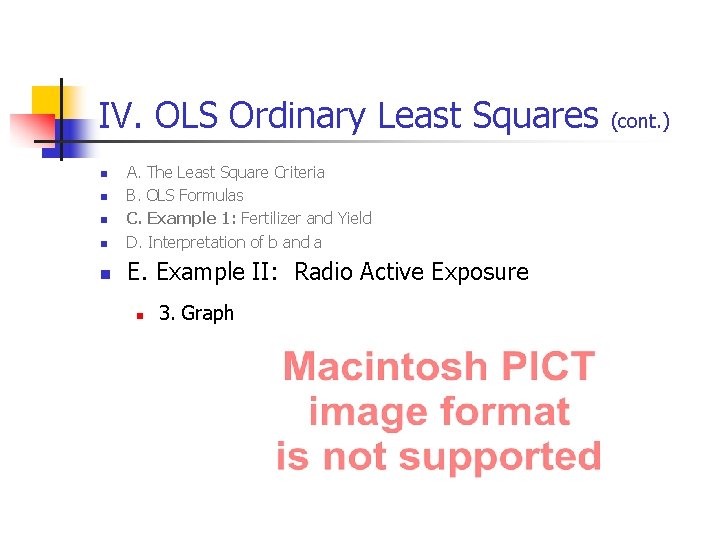
IV. OLS Ordinary Least Squares n A. The Least Square Criteria B. OLS Formulas C. Example 1: Fertilizer and Yield D. Interpretation of b and a n E. Example II: Radio Active Exposure n n 3. Graph (cont. )
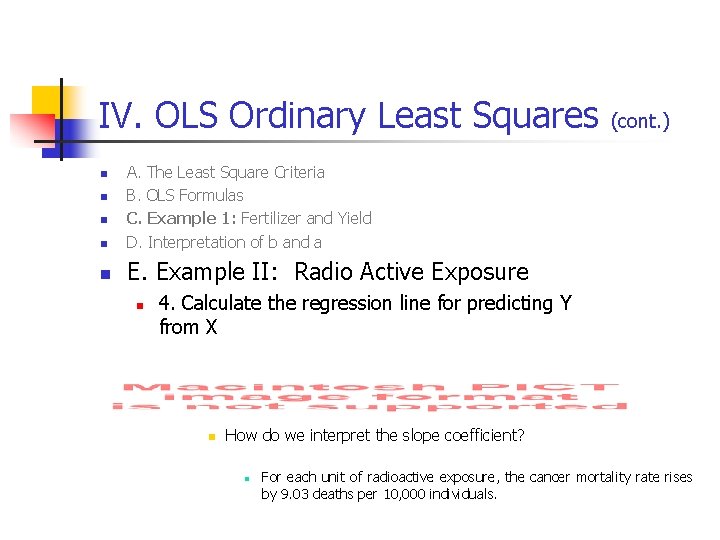
IV. OLS Ordinary Least Squares n A. The Least Square Criteria B. OLS Formulas C. Example 1: Fertilizer and Yield D. Interpretation of b and a n E. Example II: Radio Active Exposure n n (cont. ) 4. Calculate the regression line for predicting Y from X n i) Slope n How do we interpret the slope coefficient? n For each unit of radioactive exposure, the cancer mortality rate rises by 9. 03 deaths per 10, 000 individuals.
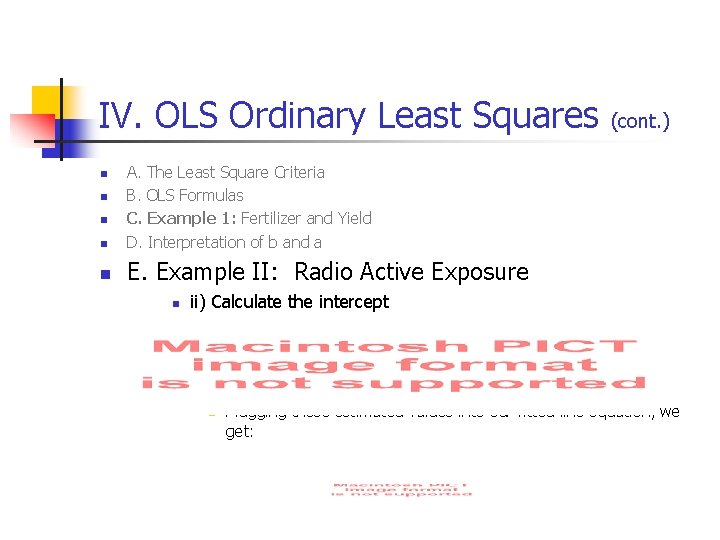
IV. OLS Ordinary Least Squares n A. The Least Square Criteria B. OLS Formulas C. Example 1: Fertilizer and Yield D. Interpretation of b and a n E. Example II: Radio Active Exposure n n (cont. ) ii) Calculate the intercept n Plugging these estimated values into our fitted line equation, we get:
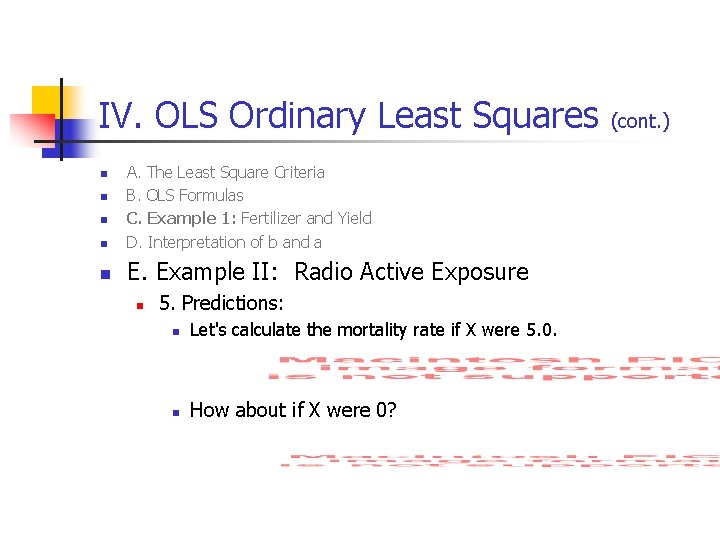
IV. OLS Ordinary Least Squares n A. The Least Square Criteria B. OLS Formulas C. Example 1: Fertilizer and Yield D. Interpretation of b and a n E. Example II: Radio Active Exposure n n 5. Predictions: n Let's calculate the mortality rate if X were 5. 0. n How about if X were 0? (cont. )
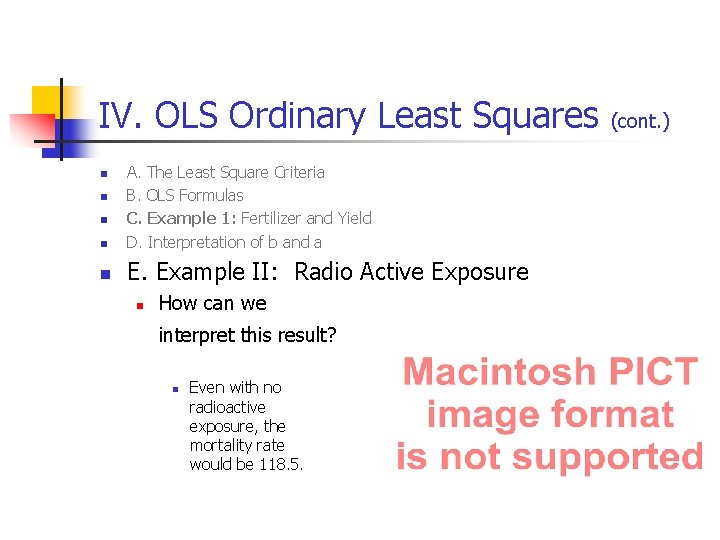
IV. OLS Ordinary Least Squares n A. The Least Square Criteria B. OLS Formulas C. Example 1: Fertilizer and Yield D. Interpretation of b and a n E. Example II: Radio Active Exposure n n How can we interpret this result? n Even with no radioactive exposure, the mortality rate would be 118. 5. (cont. )
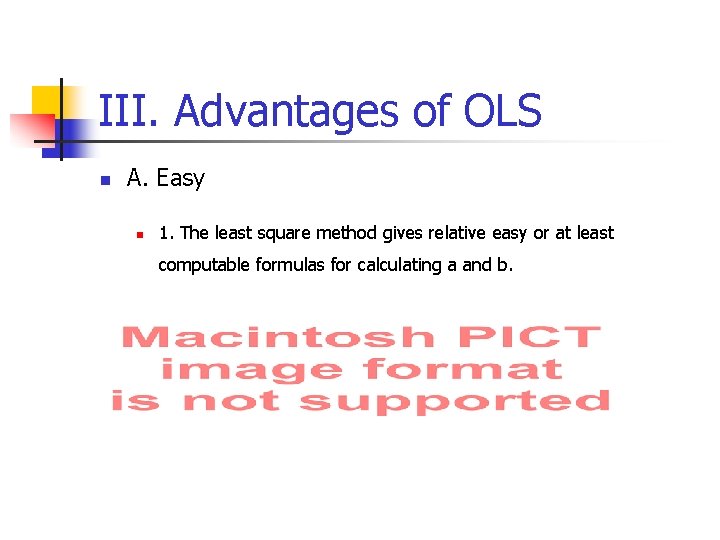
III. Advantages of OLS n A. Easy n 1. The least square method gives relative easy or at least computable formulas for calculating a and b.
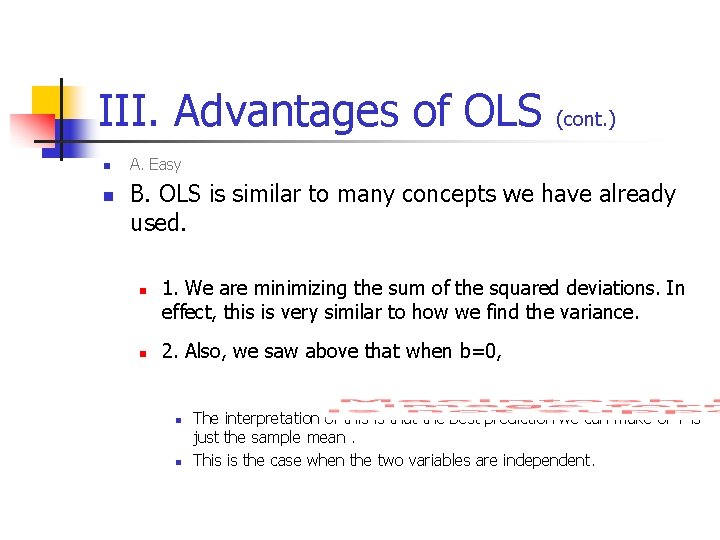
III. Advantages of OLS n n (cont. ) A. Easy B. OLS is similar to many concepts we have already used. n n 1. We are minimizing the sum of the squared deviations. In effect, this is very similar to how we find the variance. 2. Also, we saw above that when b=0, n n The interpretation of this is that the best prediction we can make of Y is just the sample mean. This is the case when the two variables are independent.
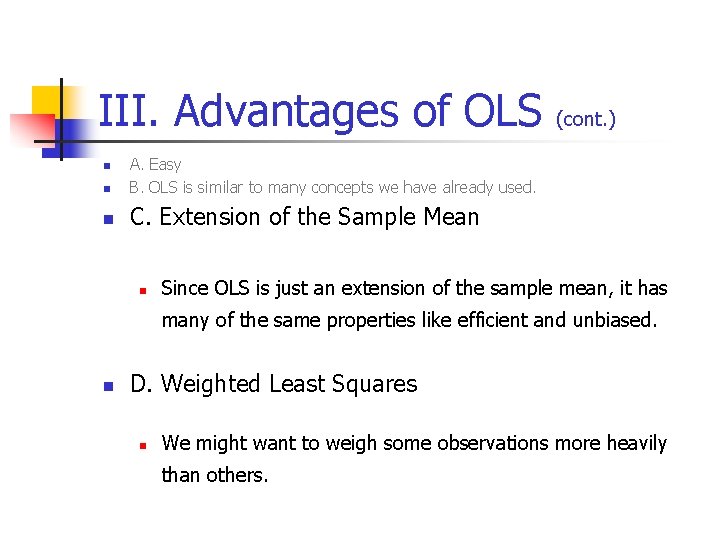
III. Advantages of OLS n A. Easy B. OLS is similar to many concepts we have already used. n C. Extension of the Sample Mean n n (cont. ) Since OLS is just an extension of the sample mean, it has many of the same properties like efficient and unbiased. n D. Weighted Least Squares n We might want to weigh some observations more heavily than others.
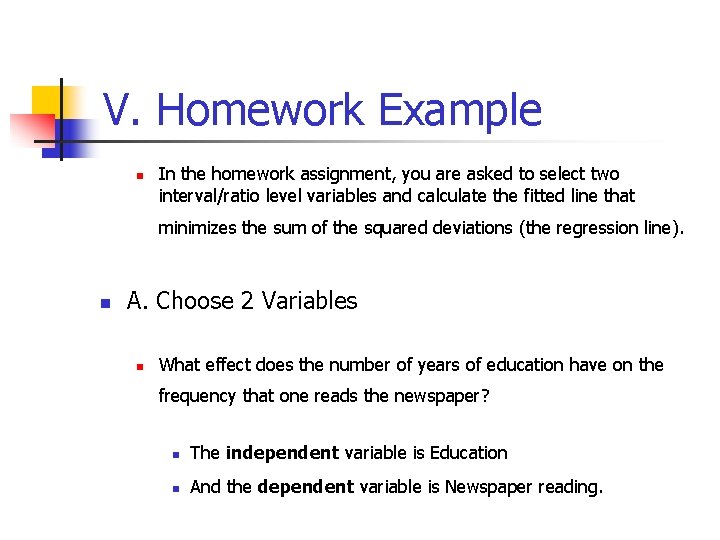
V. Homework Example n In the homework assignment, you are asked to select two interval/ratio level variables and calculate the fitted line that minimizes the sum of the squared deviations (the regression line). n A. Choose 2 Variables n What effect does the number of years of education have on the frequency that one reads the newspaper? n The independent variable is Education n And the dependent variable is Newspaper reading.
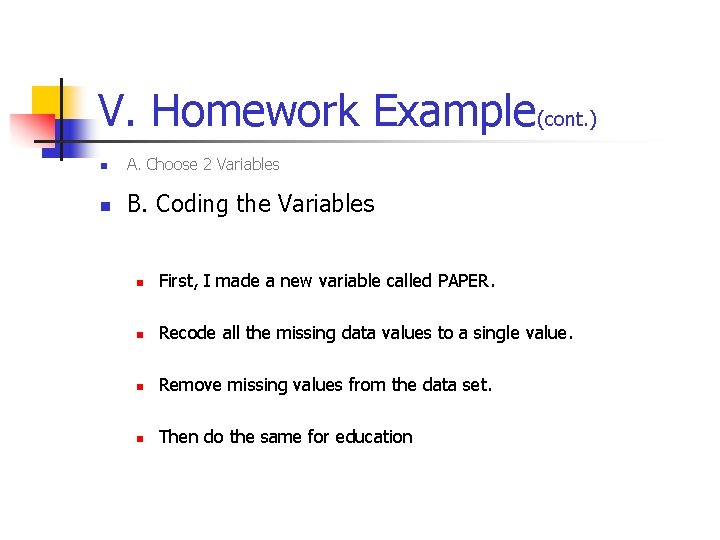
V. Homework Example(cont. ) n A. Choose 2 Variables n B. Coding the Variables n First, I made a new variable called PAPER. n Recode all the missing data values to a single value. n Remove missing values from the data set. n Then do the same for education
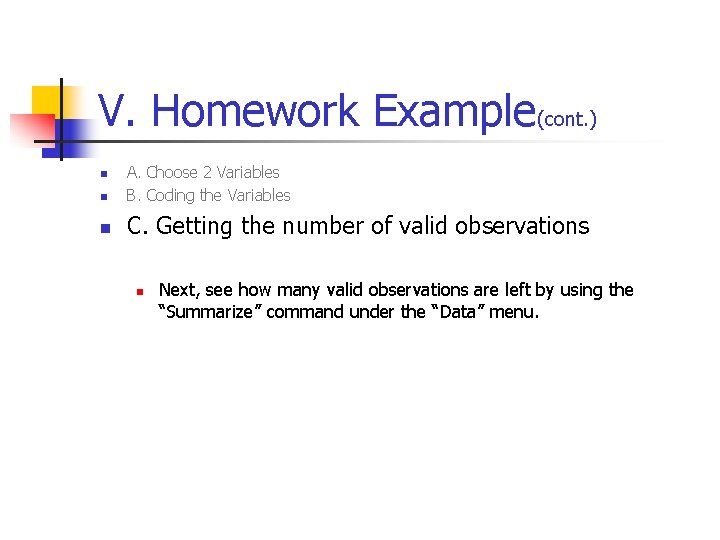
V. Homework Example(cont. ) n A. Choose 2 Variables B. Coding the Variables n C. Getting the number of valid observations n n Next, see how many valid observations are left by using the “Summarize” command under the “Data” menu.
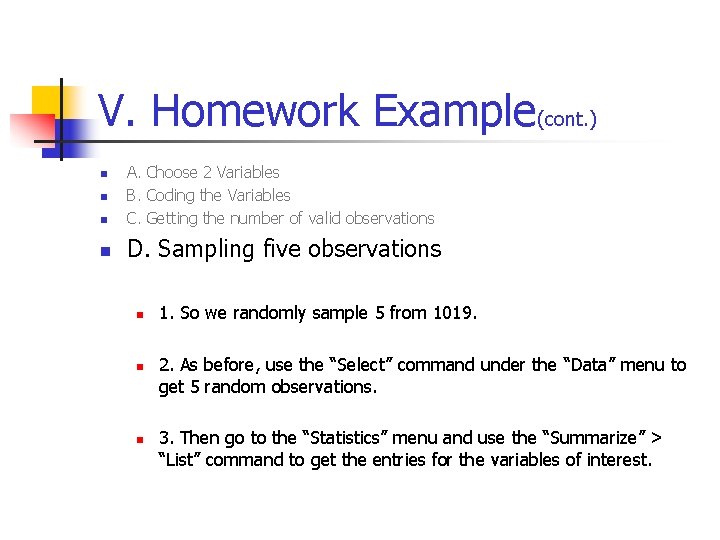
V. Homework Example(cont. ) n A. Choose 2 Variables B. Coding the Variables C. Getting the number of valid observations n D. Sampling five observations n n n 1. So we randomly sample 5 from 1019. 2. As before, use the “Select” command under the “Data” menu to get 5 random observations. 3. Then go to the “Statistics” menu and use the “Summarize” > “List” command to get the entries for the variables of interest.
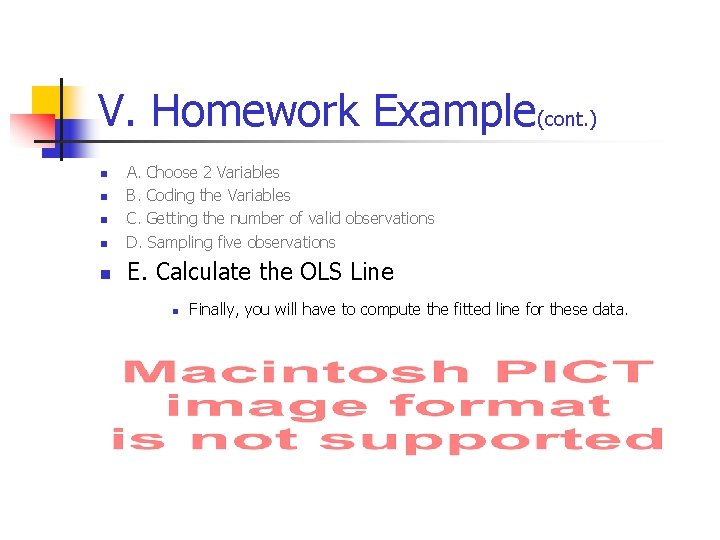
V. Homework Example(cont. ) n A. Choose 2 Variables B. Coding the Variables C. Getting the number of valid observations D. Sampling five observations n E. Calculate the OLS Line n n Finally, you will have to compute the fitted line for these data.
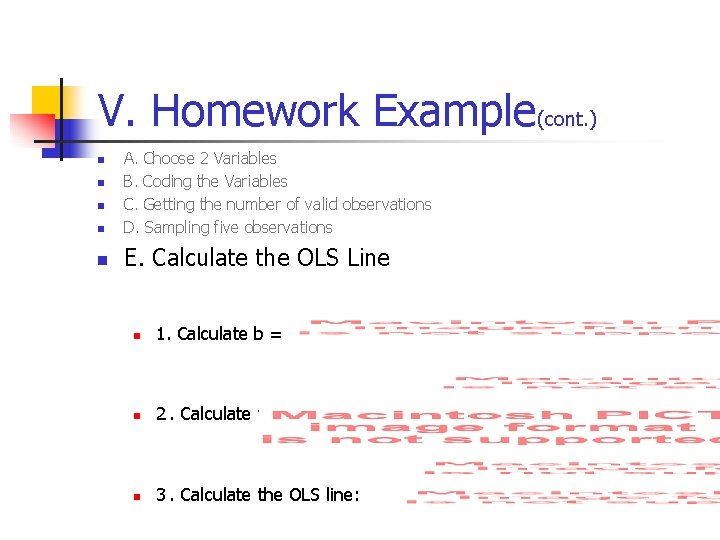
V. Homework Example(cont. ) n A. Choose 2 Variables B. Coding the Variables C. Getting the number of valid observations D. Sampling five observations n E. Calculate the OLS Line n n 1. Calculate b = n 2. Calculate the intercept: n 3. Calculate the OLS line:
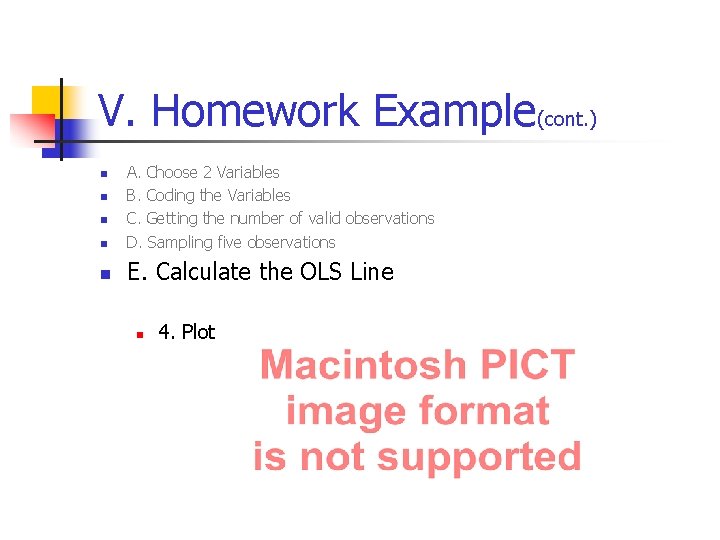
V. Homework Example(cont. ) n A. Choose 2 Variables B. Coding the Variables C. Getting the number of valid observations D. Sampling five observations n E. Calculate the OLS Line n n 4. Plot
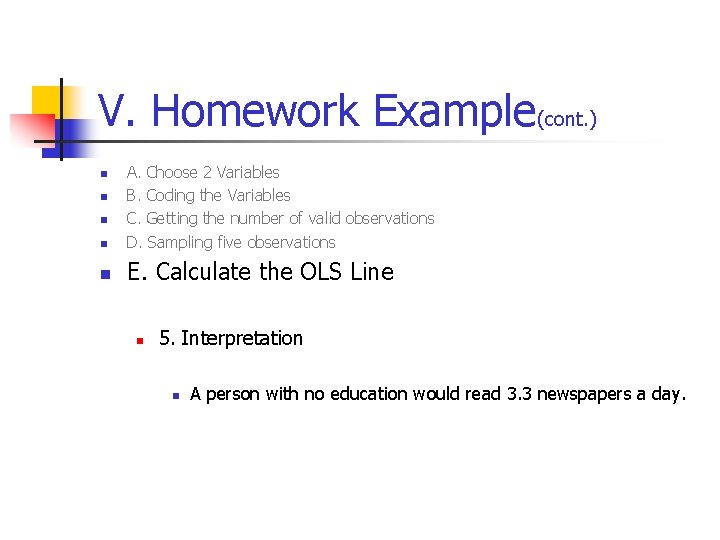
V. Homework Example(cont. ) n A. Choose 2 Variables B. Coding the Variables C. Getting the number of valid observations D. Sampling five observations n E. Calculate the OLS Line n n 5. Interpretation n A person with no education would read 3. 3 newspapers a day.
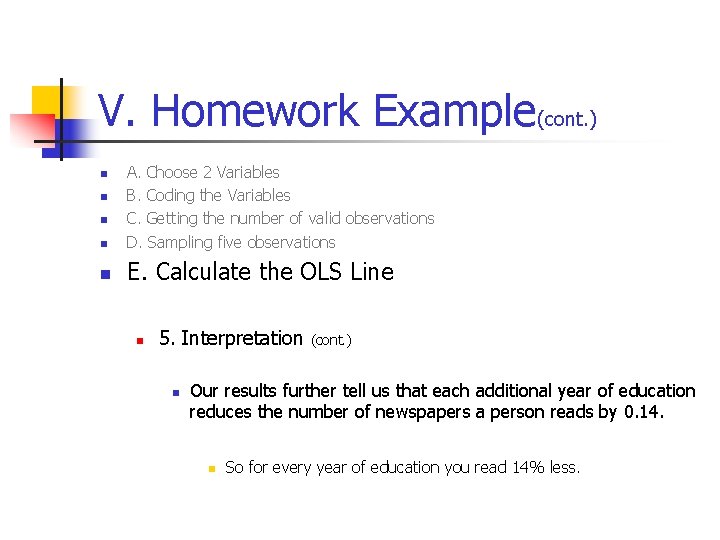
V. Homework Example(cont. ) n A. Choose 2 Variables B. Coding the Variables C. Getting the number of valid observations D. Sampling five observations n E. Calculate the OLS Line n n 5. Interpretation n (cont. ) Our results further tell us that each additional year of education reduces the number of newspapers a person reads by 0. 14. n So for every year of education you read 14% less.
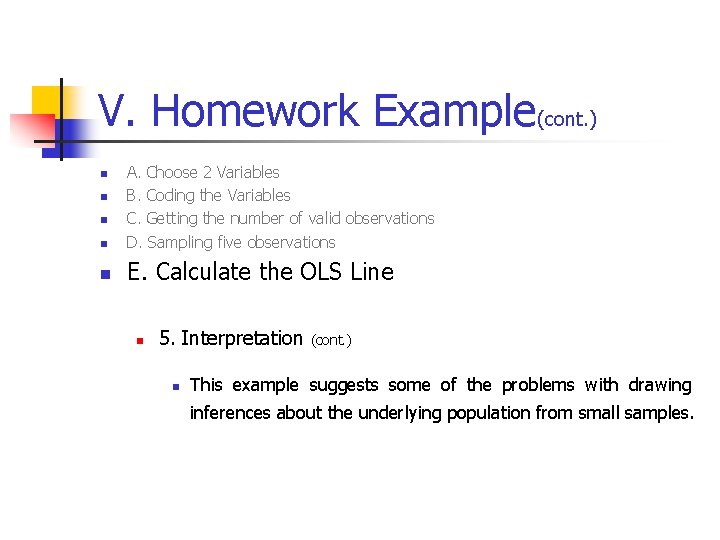
V. Homework Example(cont. ) n A. Choose 2 Variables B. Coding the Variables C. Getting the number of valid observations D. Sampling five observations n E. Calculate the OLS Line n n 5. Interpretation n (cont. ) This example suggests some of the problems with drawing inferences about the underlying population from small samples.
7680 4320
Cs 4320
Arsitektur komunikasi satelit
Identifying market segments and targets
Quantitative classification in statistics
Introduction to statistics what is statistics
Quantitative research examples
Quantitative vs qualitative data collection
Which of the following is an example of quantitative
Quantitative analysis definition
Protein estimation by lowry method
Quantitative immunohistochemistry image analysis
Quantitative analysis definition
Qualitative vs quantitative data analysis
Ap gov scotus comparison frq example
Define quantitative analysis
Define quantitative analysis
Quantitative process analysis
T test in quantitative research
Contoh typical performance
Semi quantitative analysis definition
Multiple regression research design
Cissp quantitative risk analysis
Quantitative analysis for management chapter 3 answers
Greece v galloway frq
Quantitative item analysis definition
Quantitative demand analysis
Quantitative analysis cal poly
Quantitative analysis
Quantitative analysis of organic compounds ppt
How to develop a quantitative analysis model
Objectives of time
Stoichiometry
Qualitative vs quantitative
Difference between qualitative and quantitative data
Quantitative vs qualitative observations
Assessment is qualitative or quantitative
Quantitative variables examples
Qualitative variables and quantitative variables
Qualitative tests for lipids lab report
What are qualitative observations?
Similarities between qualitative and quantitative research
Similarities between qualitative and quantitative research
Econometrics and quantitative economics
Qualitative properties of matter
Interpreting quantitative data
Chapter 3 exploring quantitative data answers
Quantitative and verbal reasoning
Qualitative and quantitative difference
Qualitative and quantitative difference
What is the sample size in qualitative research?
Inheritance of quantitative traits
Examples of qualitative research
Disadvantage of qualitative research
Qualitative and quantitative
Qtem masters network
Examples of mixed methods research
Interpretation of data