Research for Social Workers On theme identification and
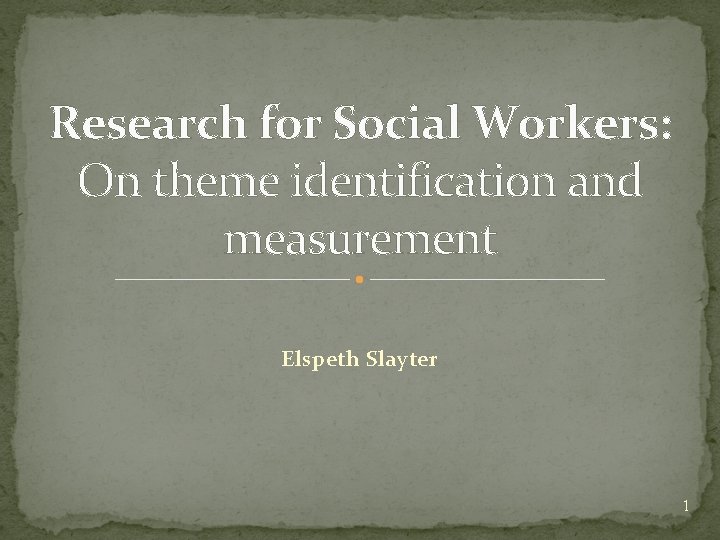
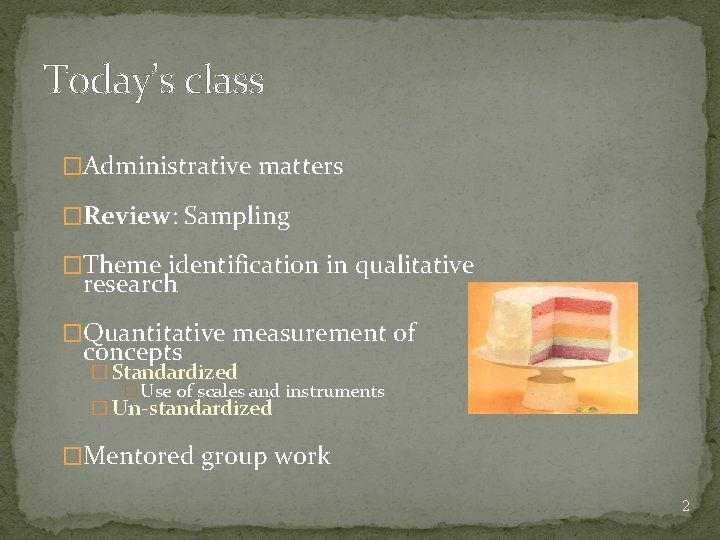
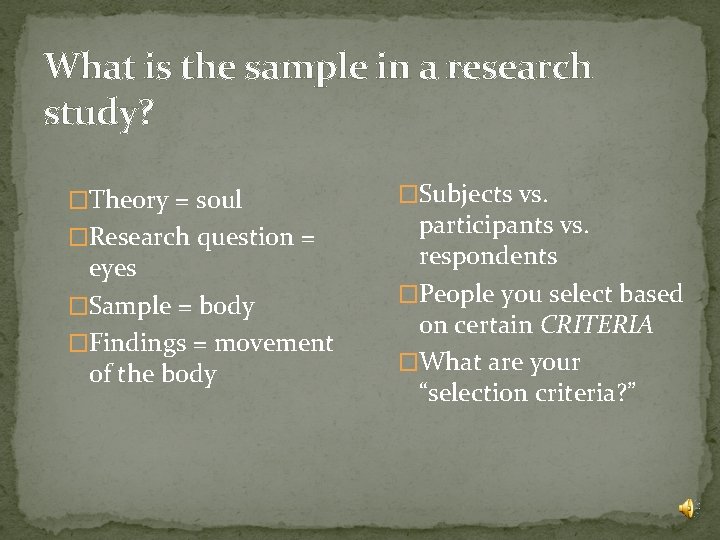
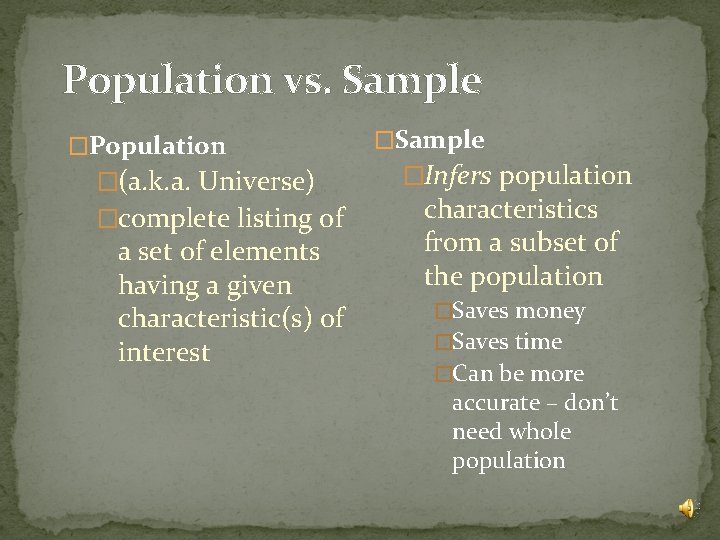
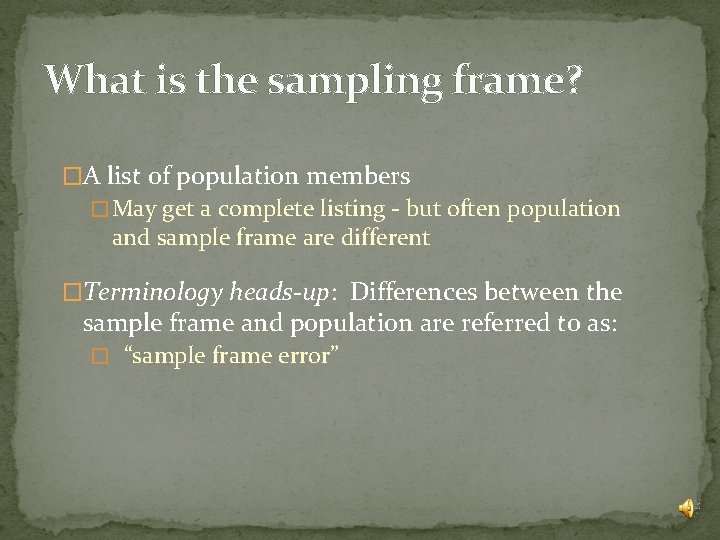
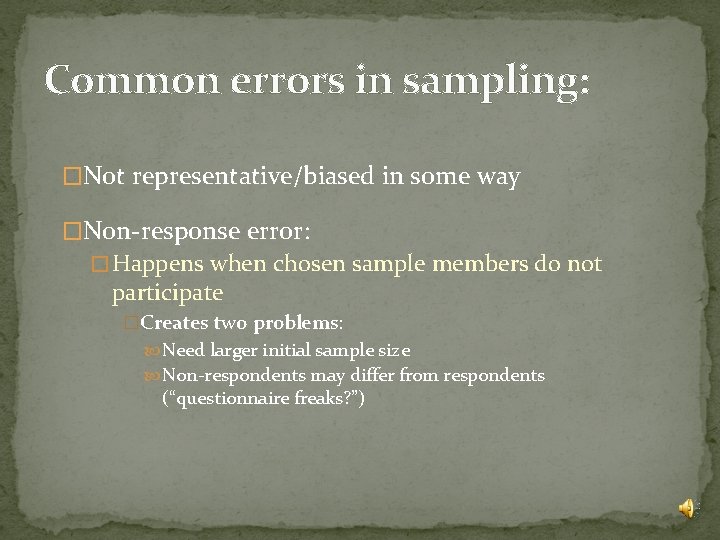
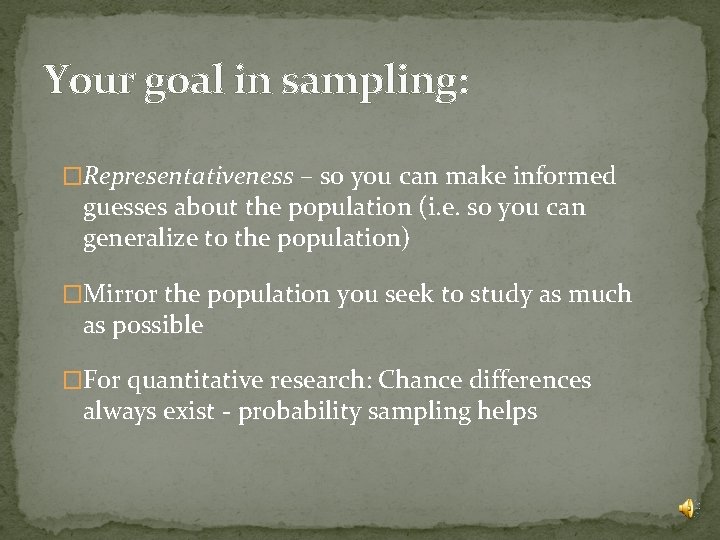
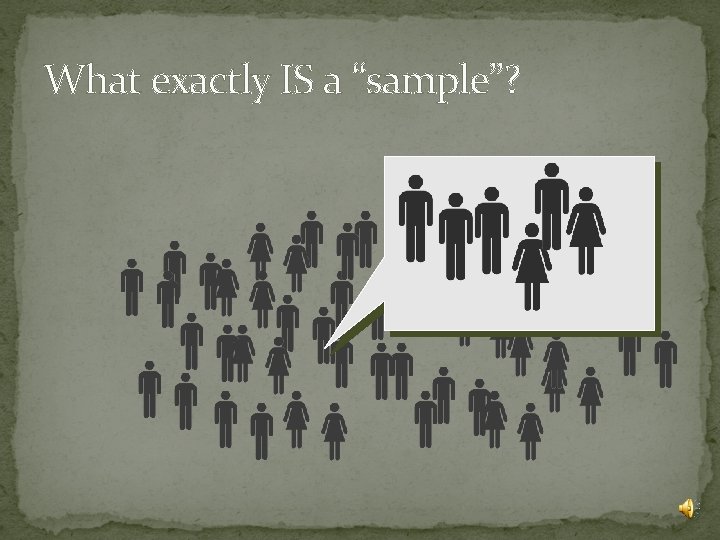
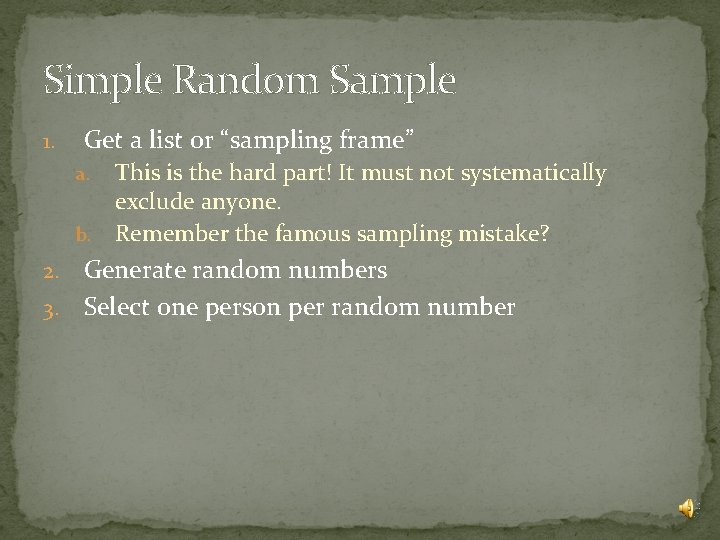
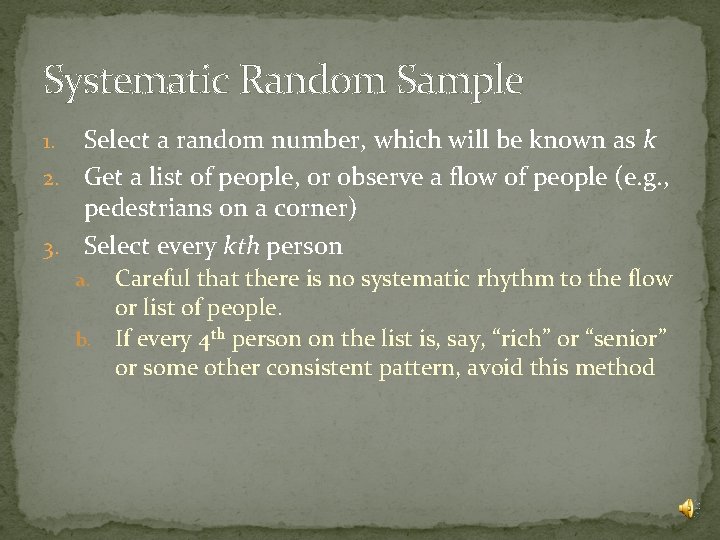
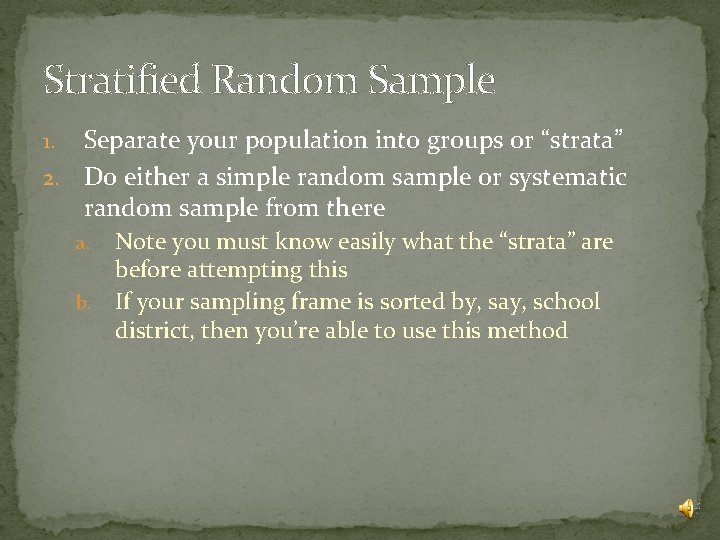
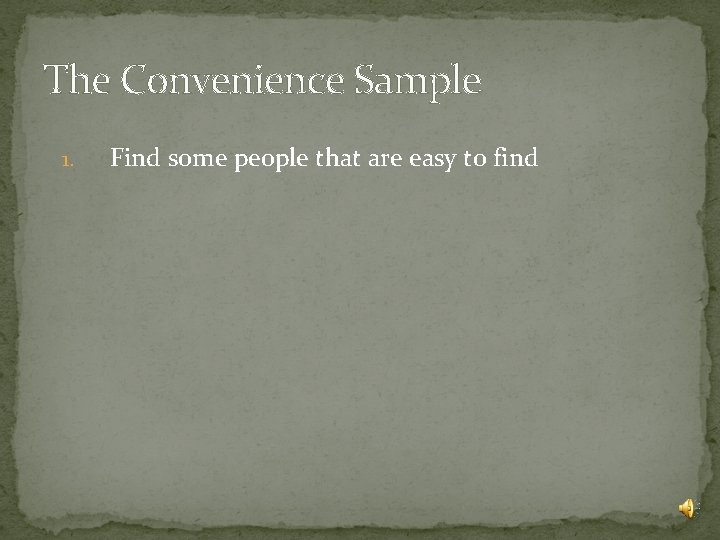
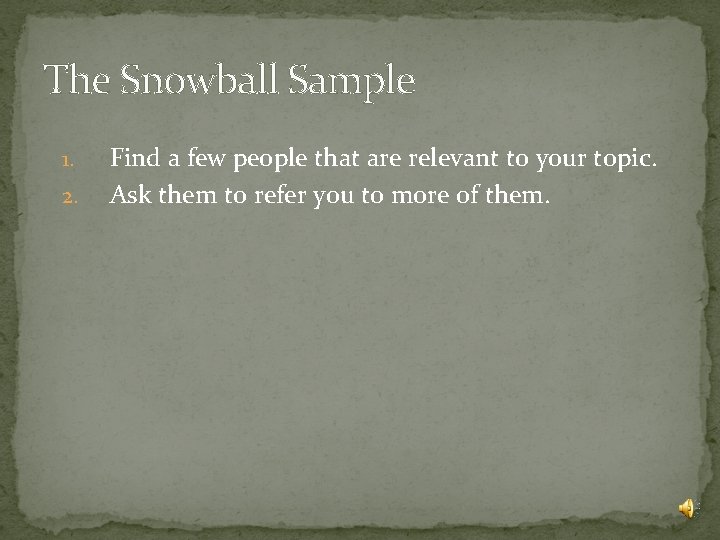
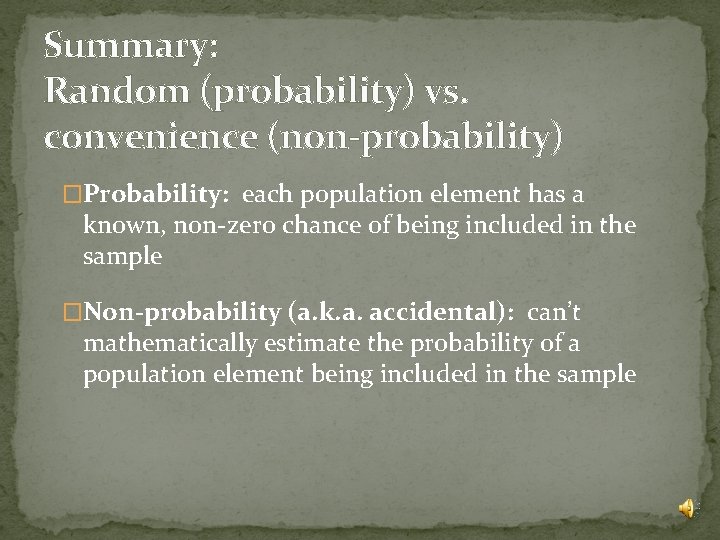
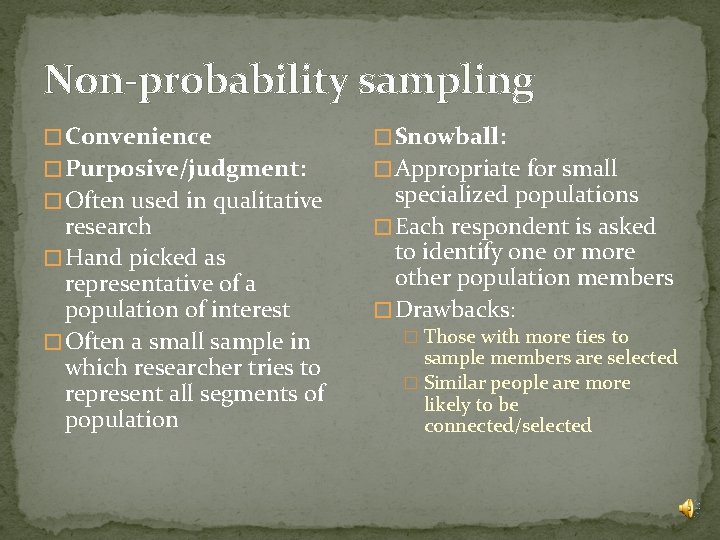
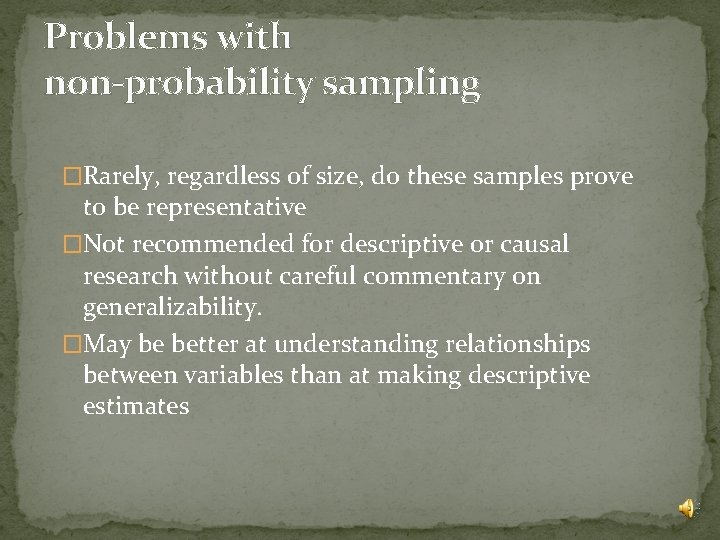
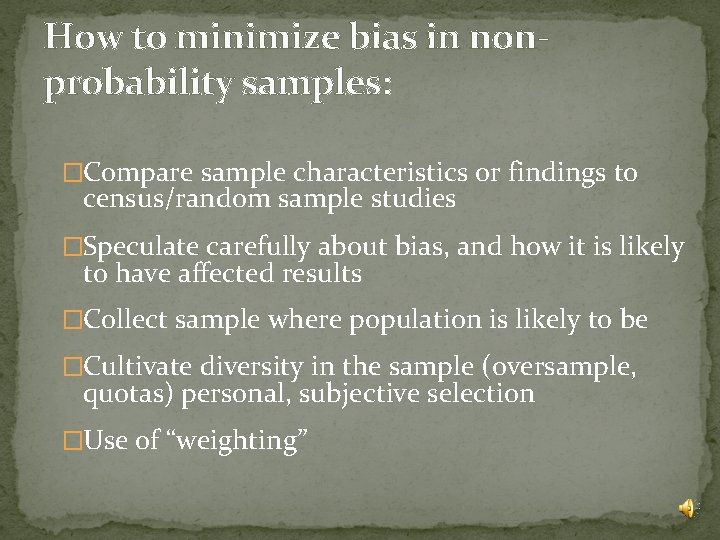
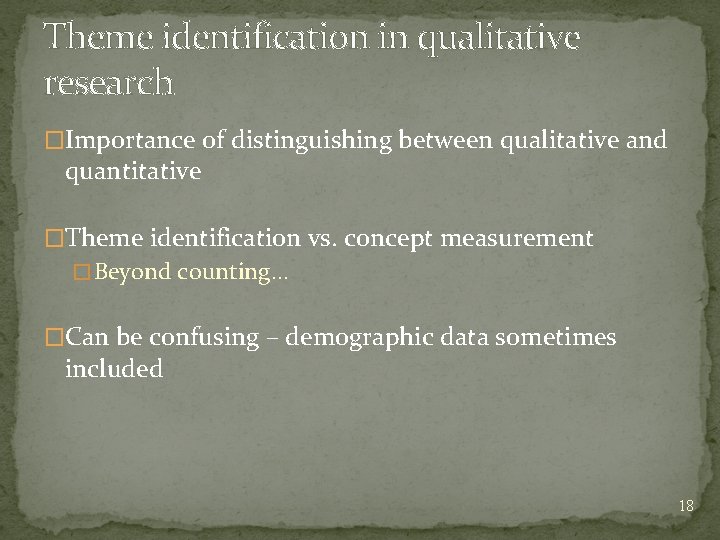
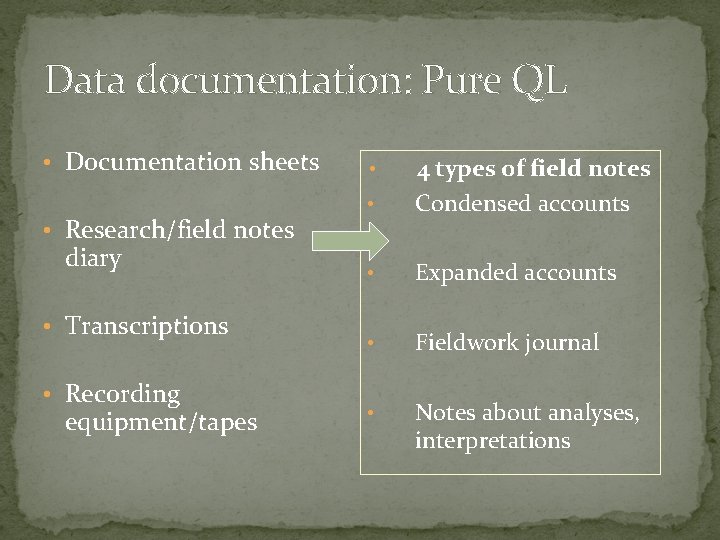
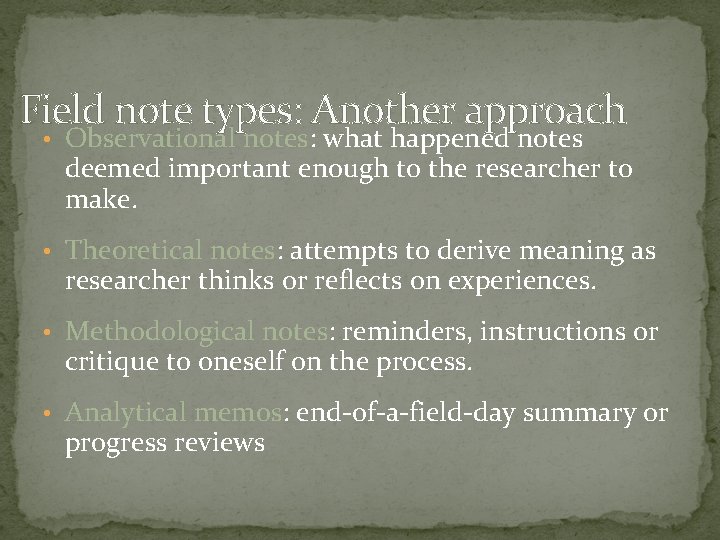
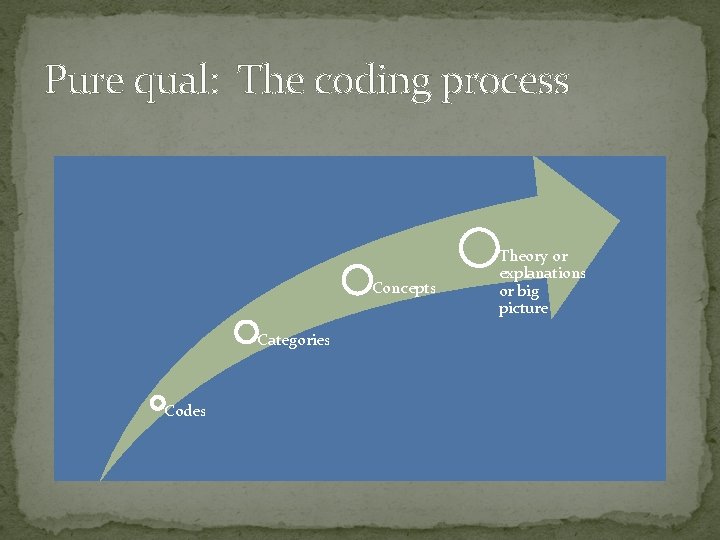
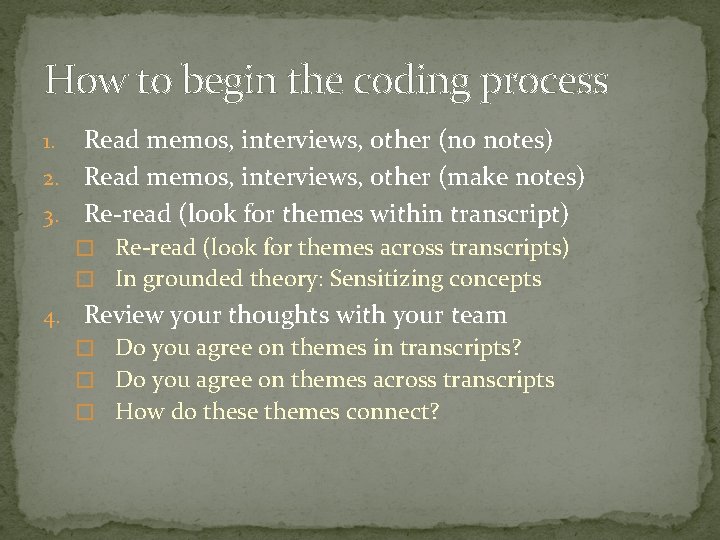
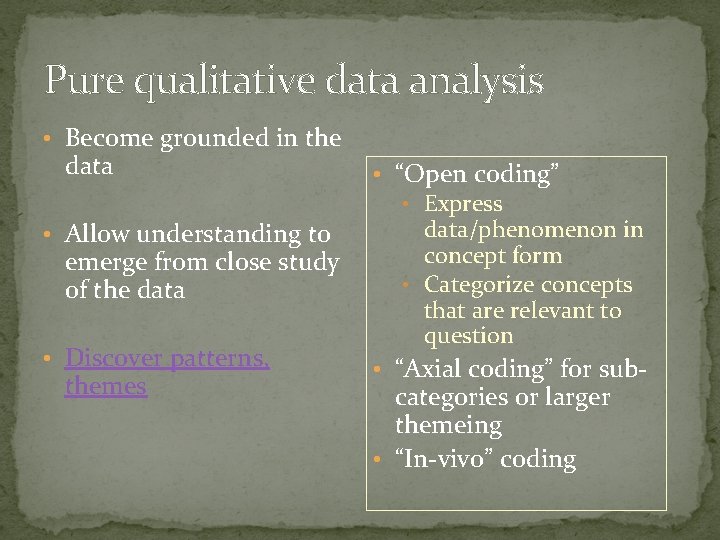
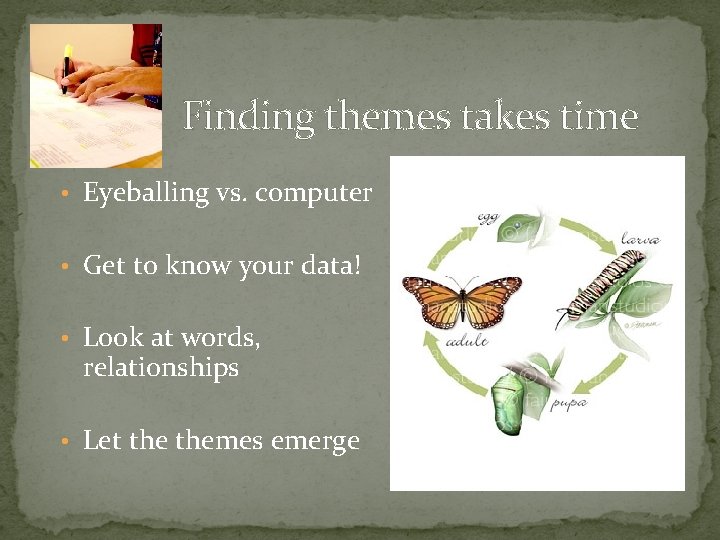
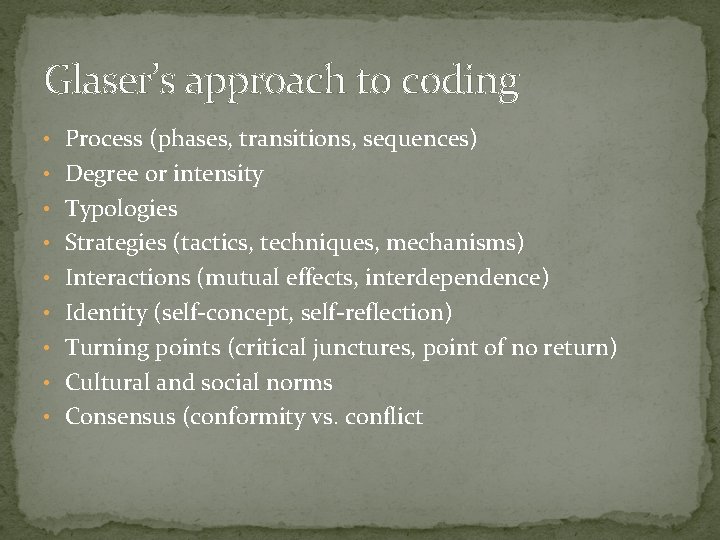
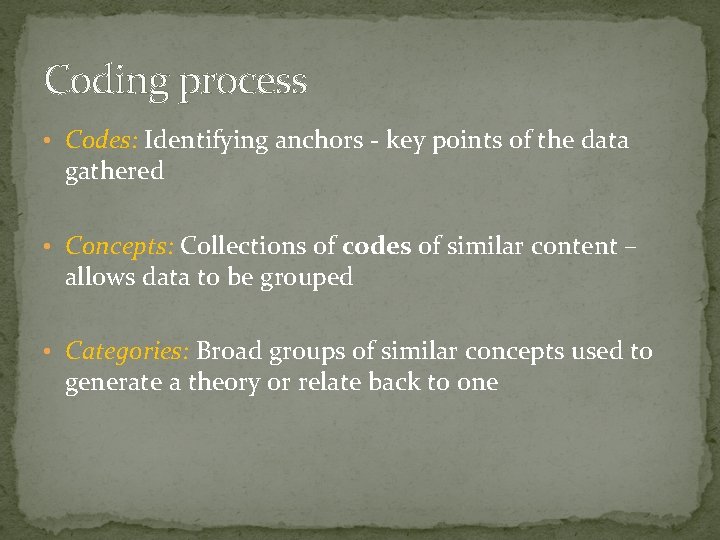
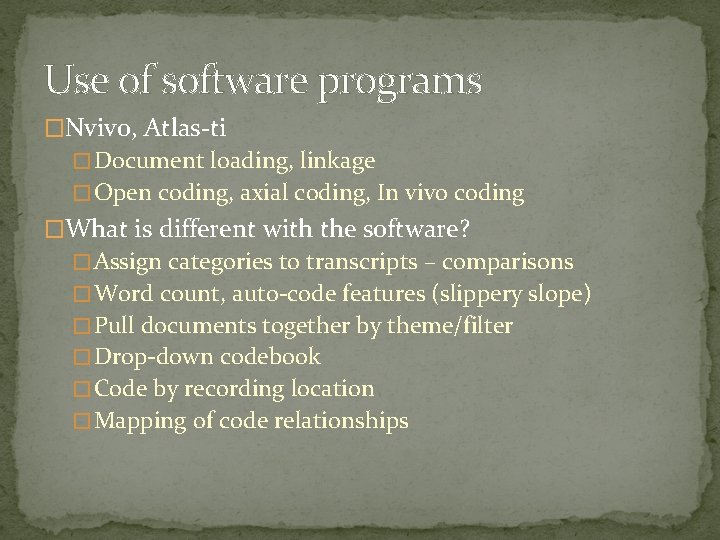
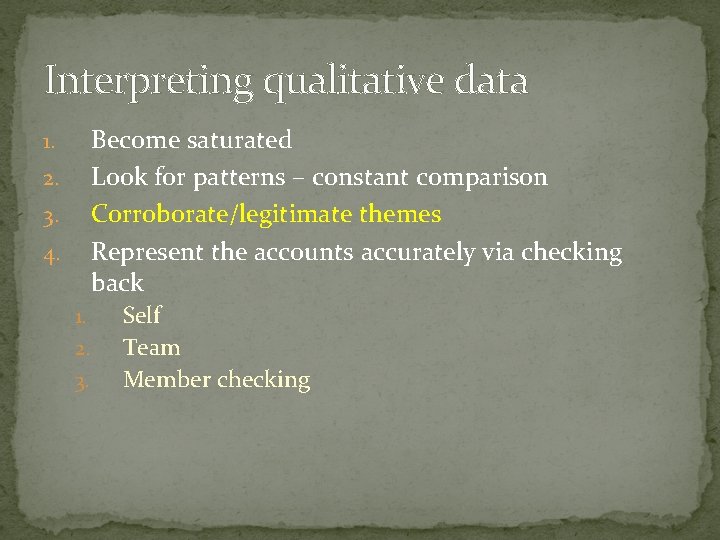
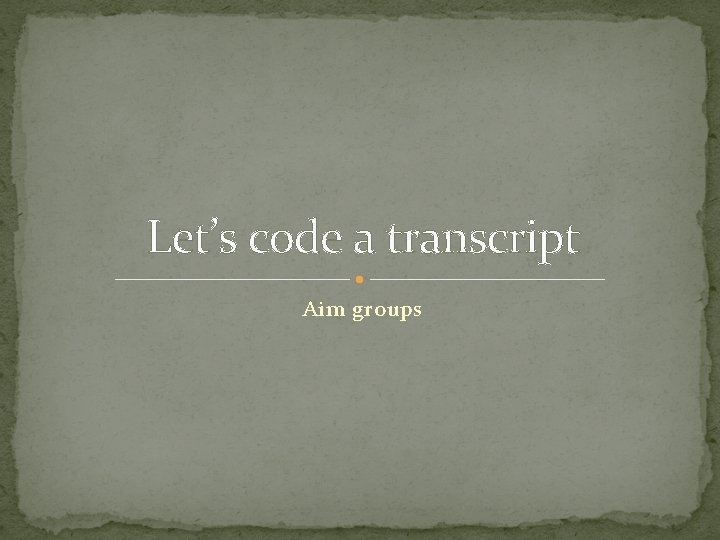
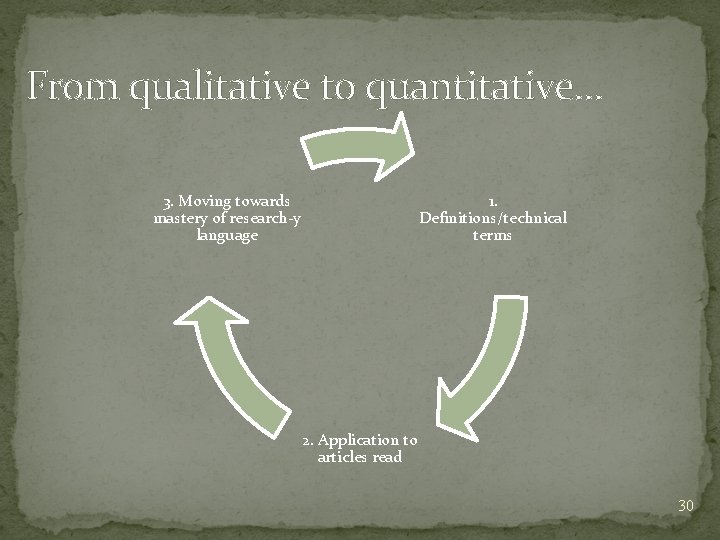
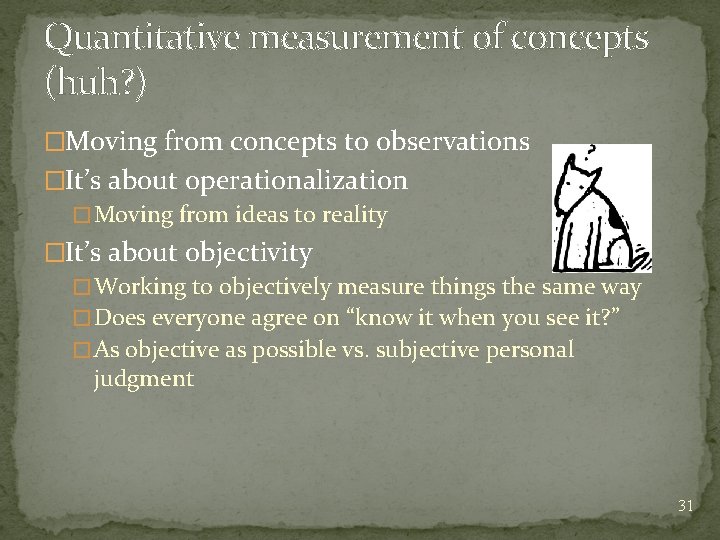
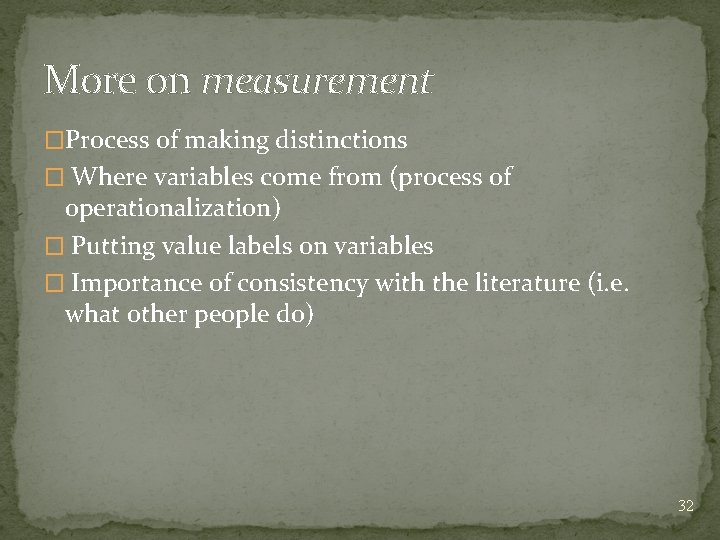
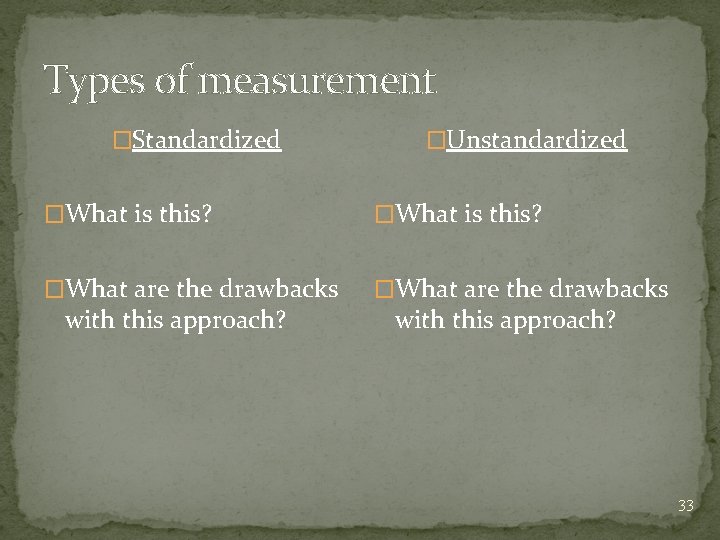
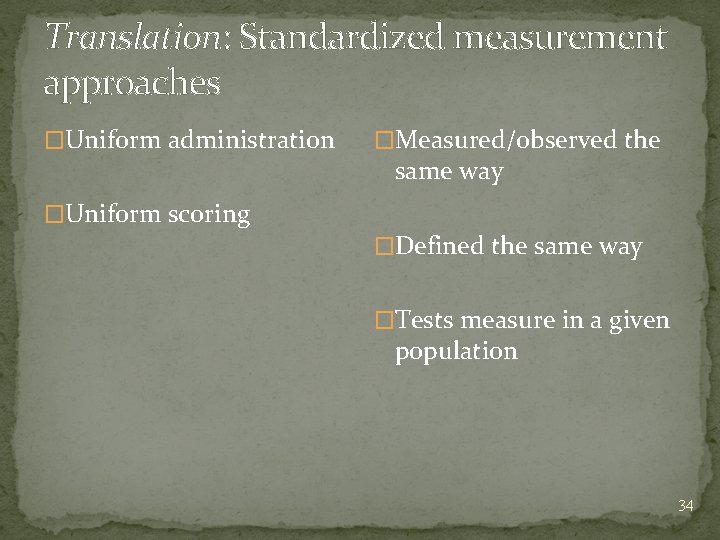
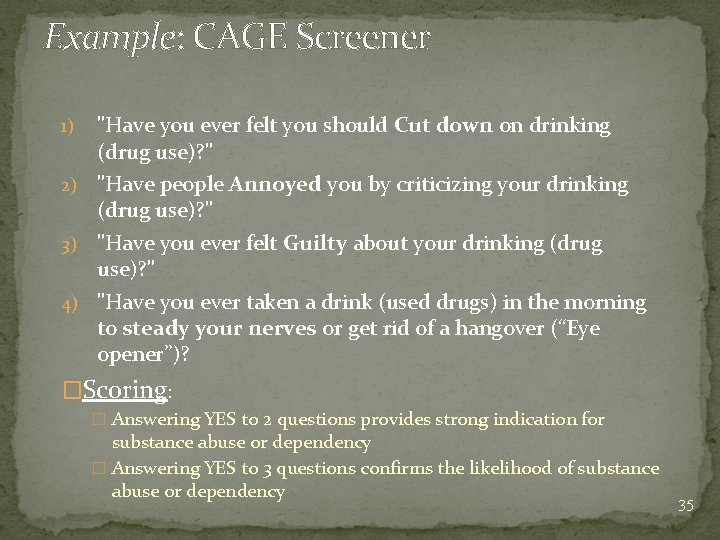
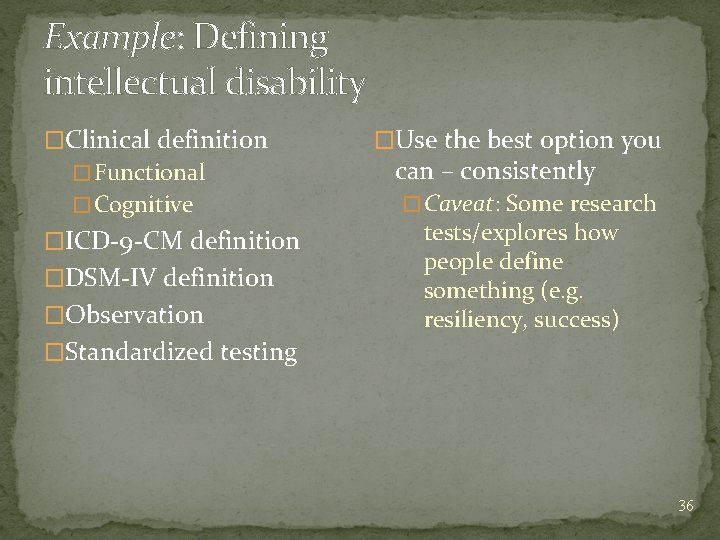
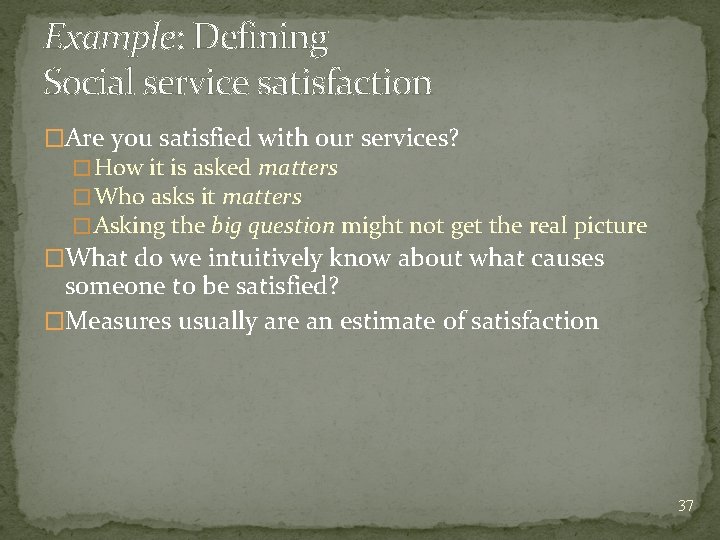
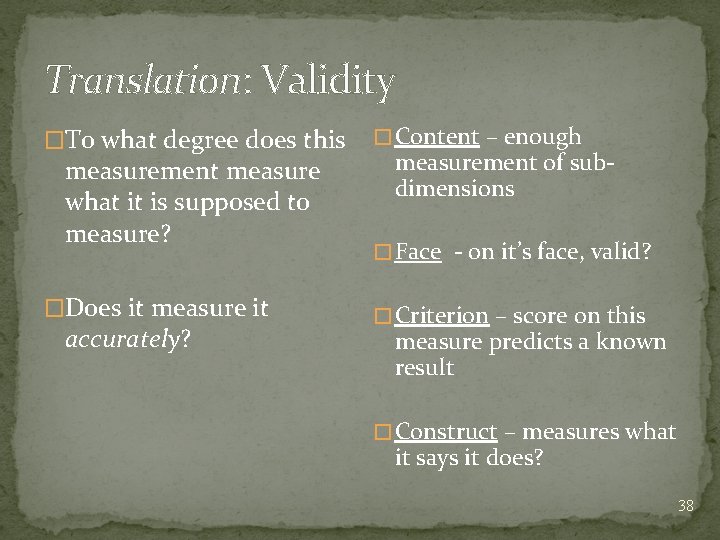
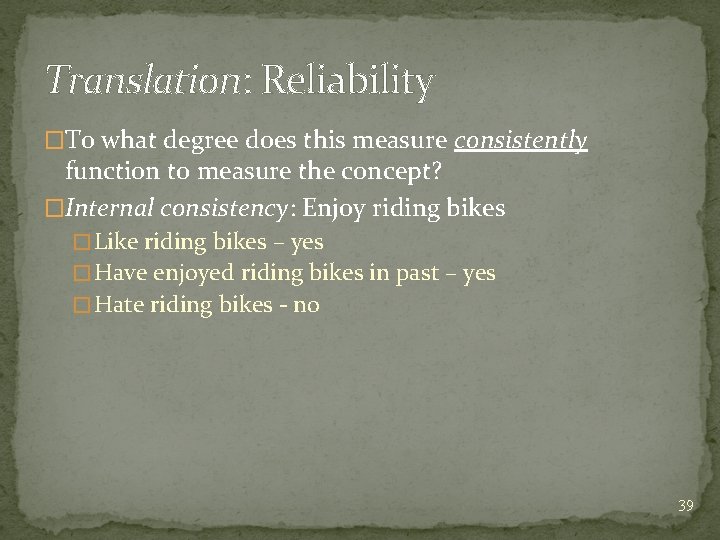
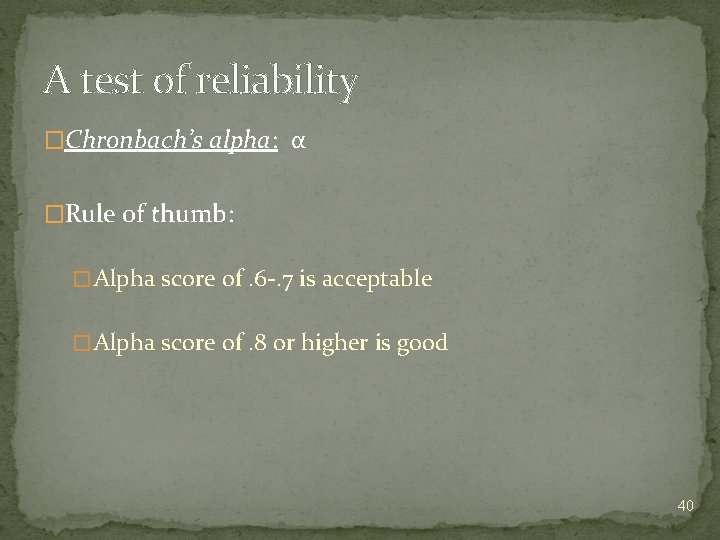
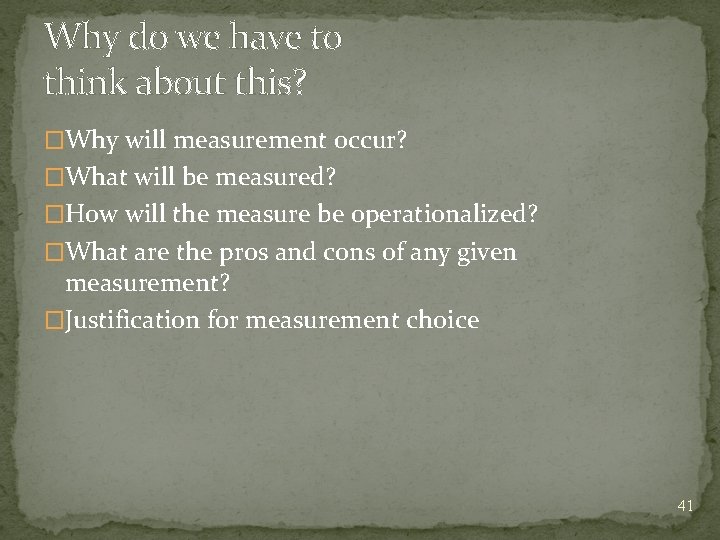
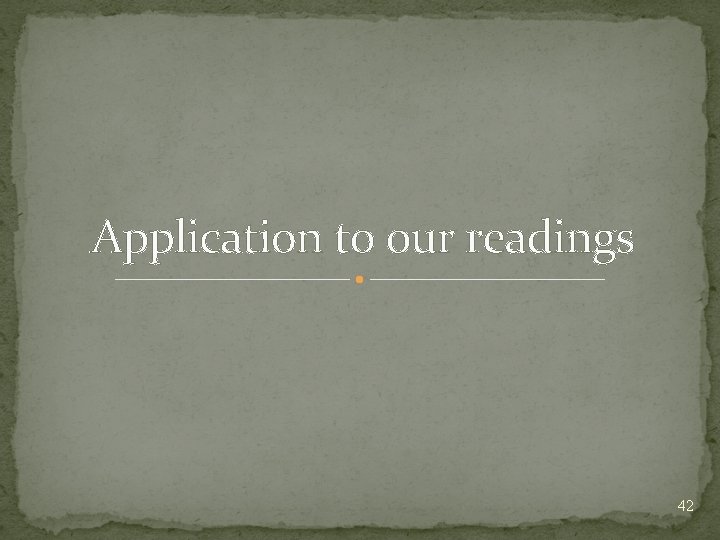
- Slides: 42
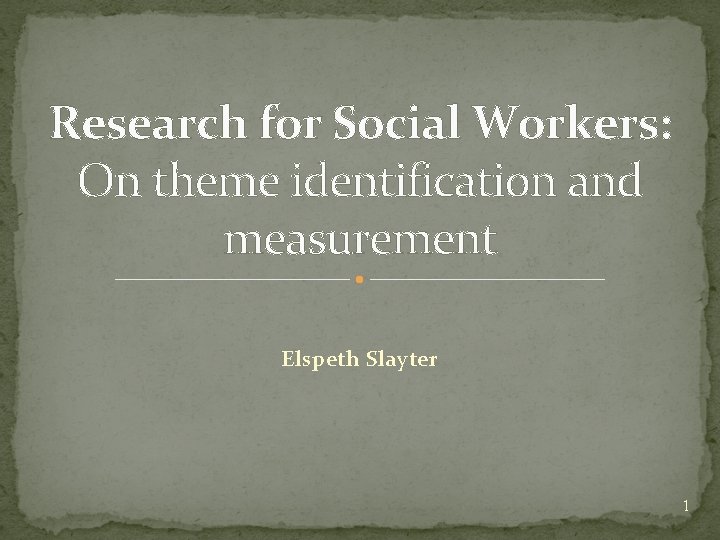
Research for Social Workers: On theme identification and measurement Elspeth Slayter 1
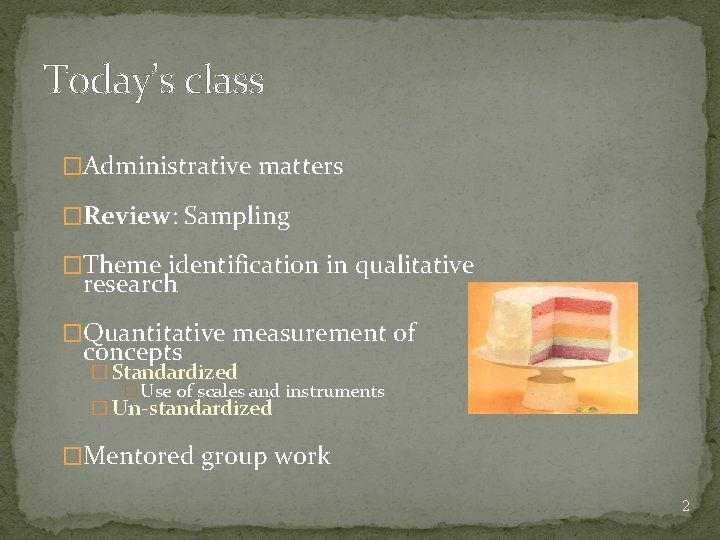
Today’s class �Administrative matters �Review: Sampling �Theme identification in qualitative research �Quantitative measurement of concepts � Standardized � Use of scales and instruments � Un-standardized �Mentored group work 2
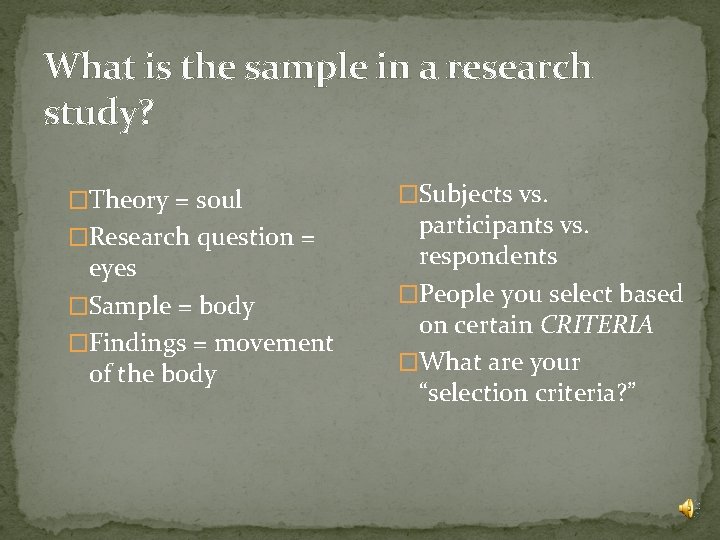
What is the sample in a research study? �Theory = soul �Research question = eyes �Sample = body �Findings = movement of the body �Subjects vs. participants vs. respondents �People you select based on certain CRITERIA �What are your “selection criteria? ”
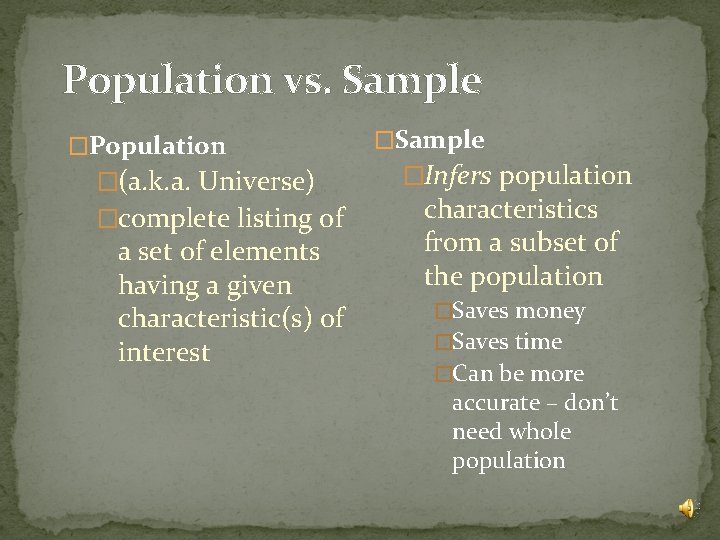
Population vs. Sample �Population �(a. k. a. Universe) �complete listing of a set of elements having a given characteristic(s) of interest �Sample �Infers population characteristics from a subset of the population �Saves money �Saves time �Can be more accurate – don’t need whole population
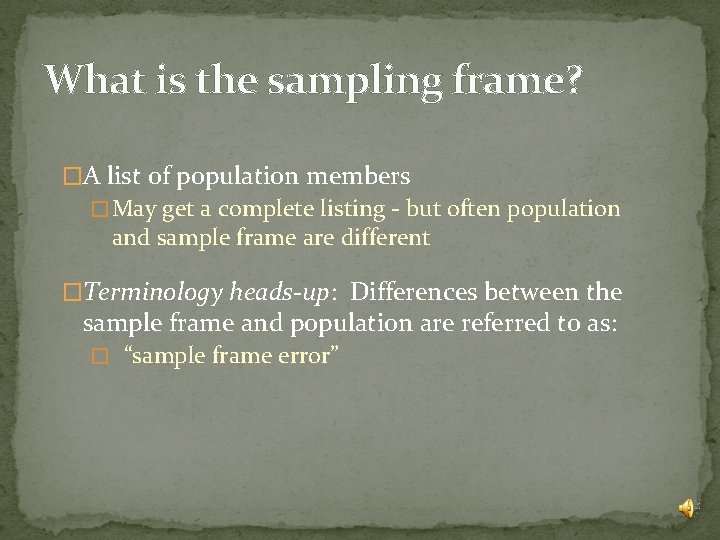
What is the sampling frame? �A list of population members � May get a complete listing - but often population and sample frame are different �Terminology heads-up: Differences between the sample frame and population are referred to as: � “sample frame error”
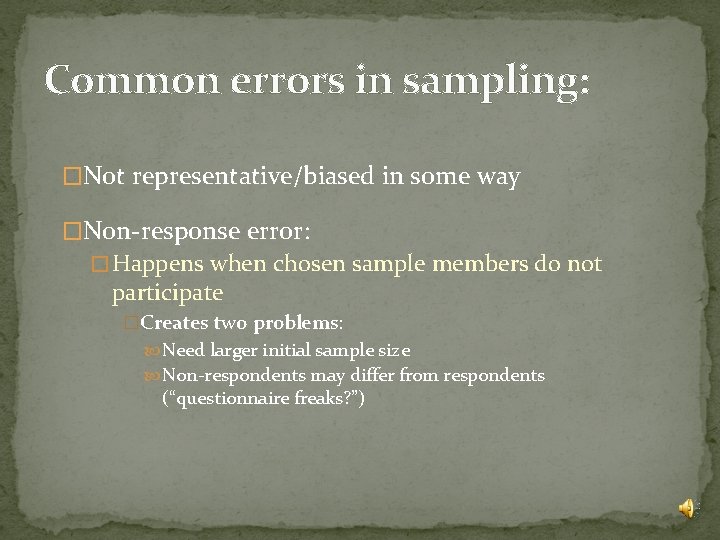
Common errors in sampling: �Not representative/biased in some way �Non-response error: � Happens when chosen sample members do not participate �Creates two problems: Need larger initial sample size Non-respondents may differ from respondents (“questionnaire freaks? ”)
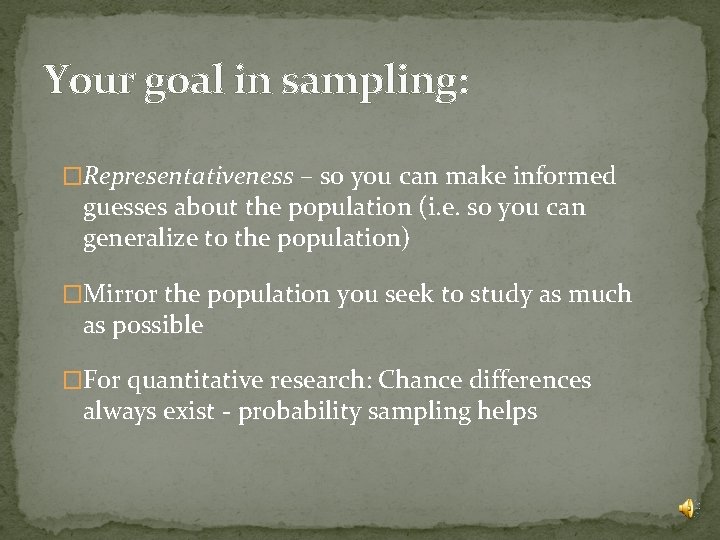
Your goal in sampling: �Representativeness – so you can make informed guesses about the population (i. e. so you can generalize to the population) �Mirror the population you seek to study as much as possible �For quantitative research: Chance differences always exist - probability sampling helps
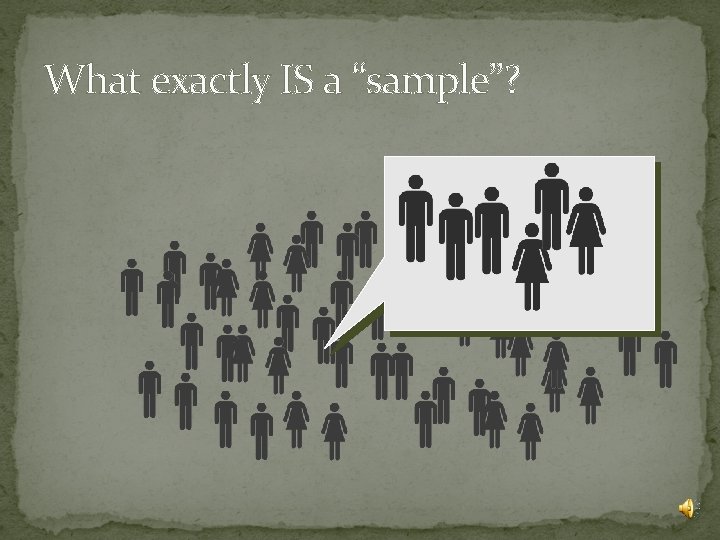
What exactly IS a “sample”?
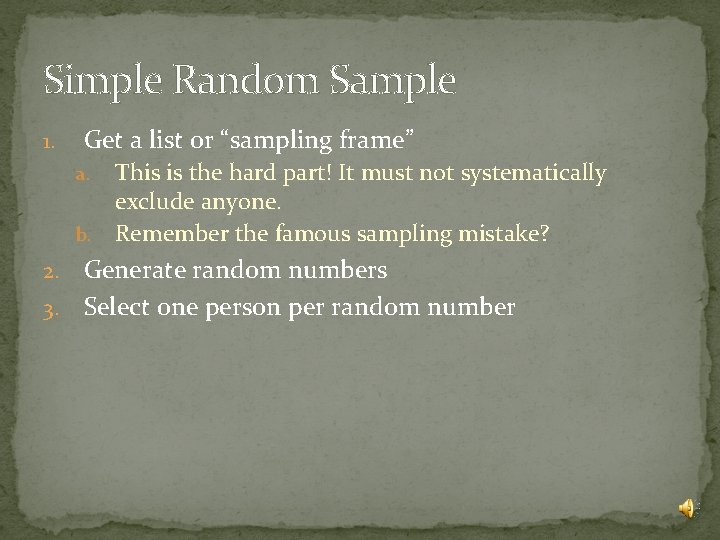
Simple Random Sample 1. Get a list or “sampling frame” This is the hard part! It must not systematically exclude anyone. b. Remember the famous sampling mistake? a. Generate random numbers 3. Select one person per random number 2.
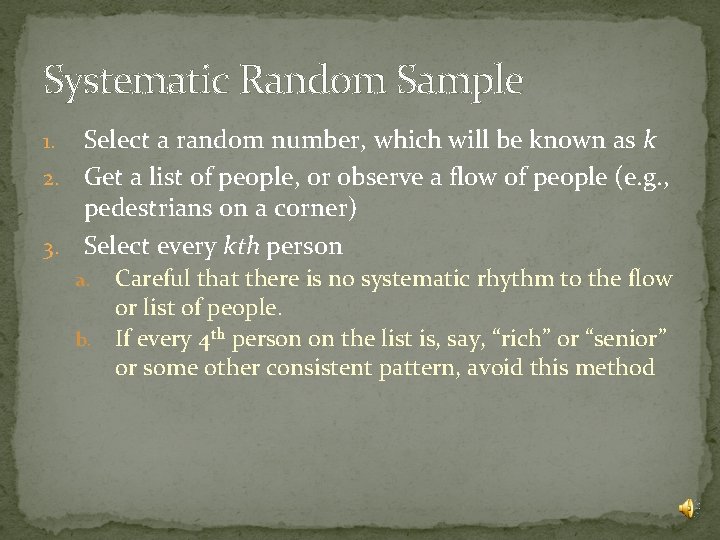
Systematic Random Sample Select a random number, which will be known as k 2. Get a list of people, or observe a flow of people (e. g. , pedestrians on a corner) 3. Select every kth person 1. Careful that there is no systematic rhythm to the flow or list of people. b. If every 4 th person on the list is, say, “rich” or “senior” or some other consistent pattern, avoid this method a.
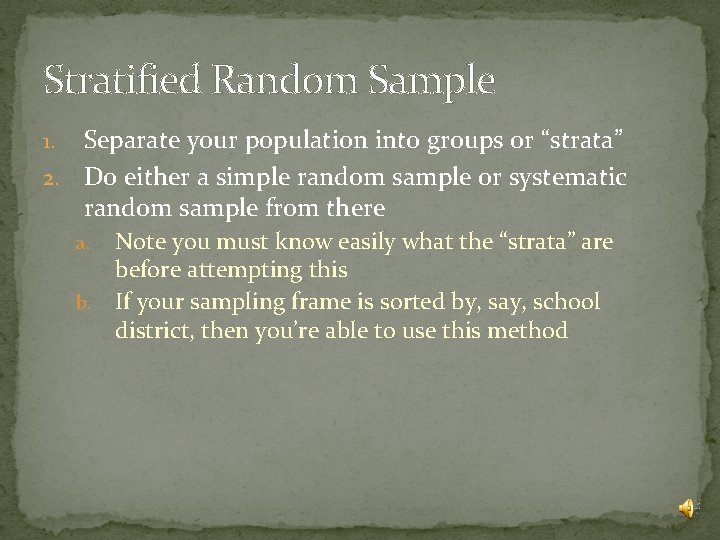
Stratified Random Sample Separate your population into groups or “strata” 2. Do either a simple random sample or systematic random sample from there 1. Note you must know easily what the “strata” are before attempting this b. If your sampling frame is sorted by, say, school district, then you’re able to use this method a.
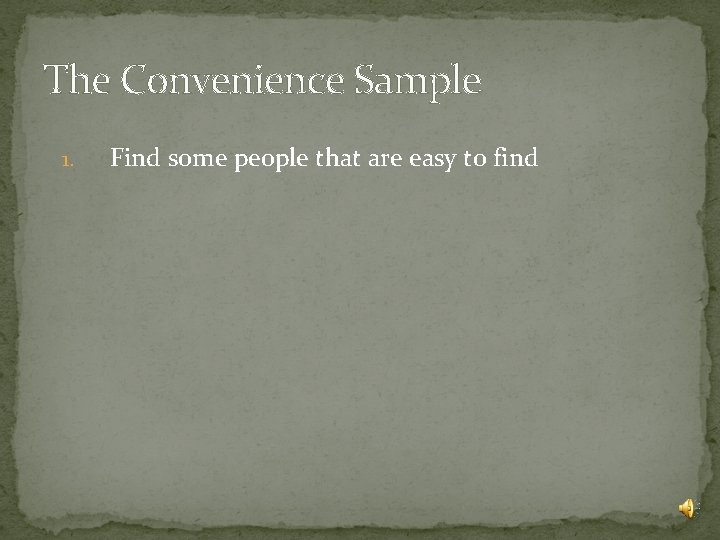
The Convenience Sample 1. Find some people that are easy to find
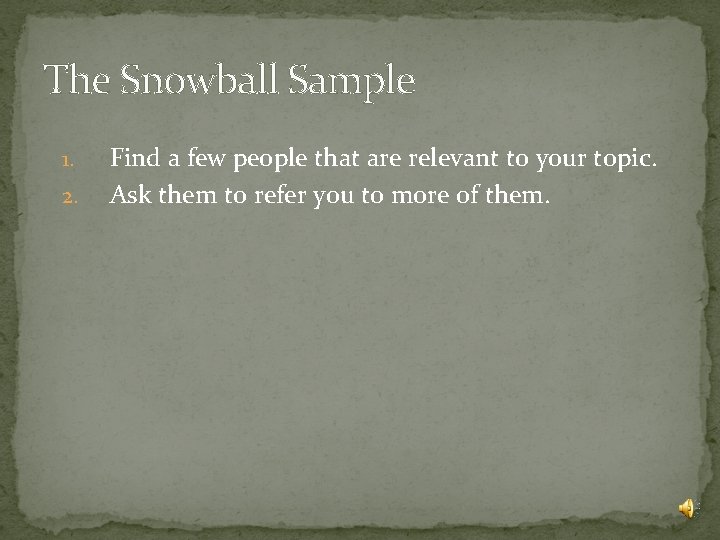
The Snowball Sample 1. 2. Find a few people that are relevant to your topic. Ask them to refer you to more of them.
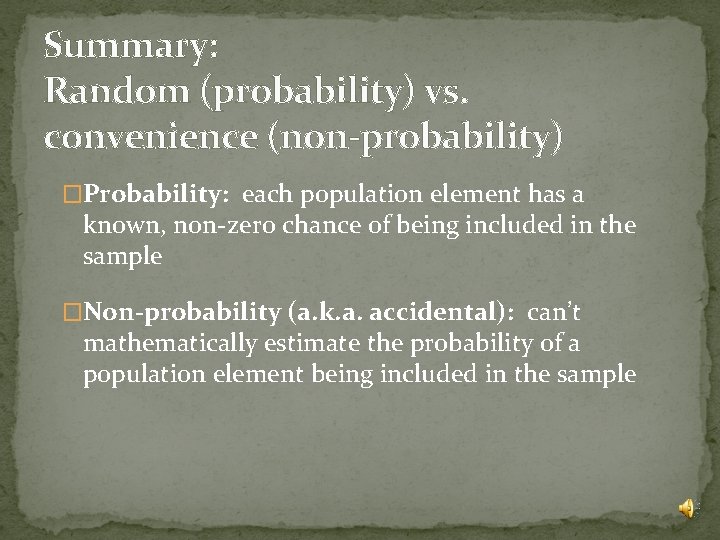
Summary: Random (probability) vs. convenience (non-probability) �Probability: each population element has a known, non-zero chance of being included in the sample �Non-probability (a. k. a. accidental): can’t mathematically estimate the probability of a population element being included in the sample
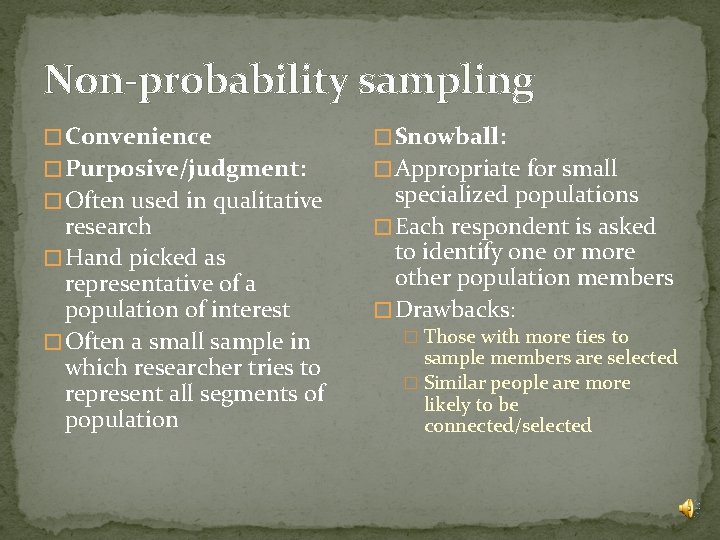
Non-probability sampling � Convenience � Snowball: � Purposive/judgment: � Appropriate for small � Often used in qualitative research � Hand picked as representative of a population of interest � Often a small sample in which researcher tries to represent all segments of population specialized populations � Each respondent is asked to identify one or more other population members � Drawbacks: � Those with more ties to sample members are selected � Similar people are more likely to be connected/selected
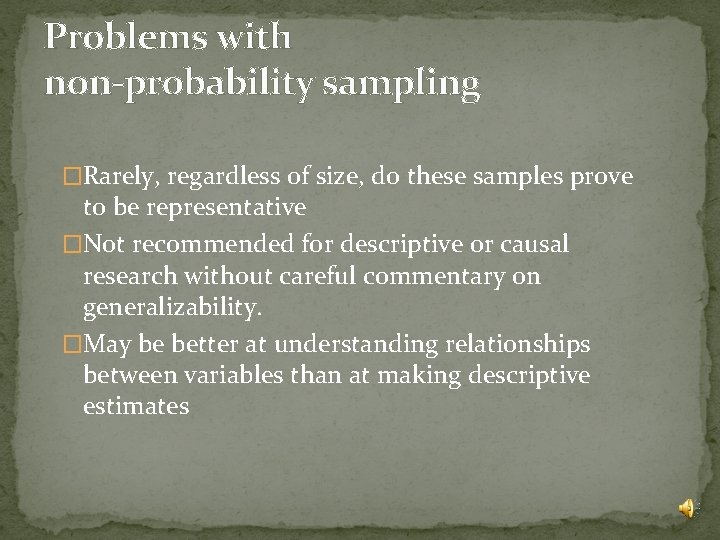
Problems with non-probability sampling �Rarely, regardless of size, do these samples prove to be representative �Not recommended for descriptive or causal research without careful commentary on generalizability. �May be better at understanding relationships between variables than at making descriptive estimates
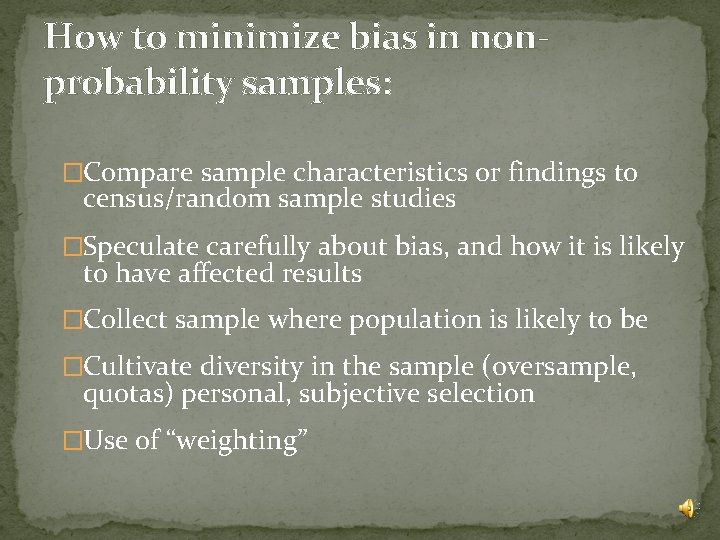
How to minimize bias in nonprobability samples: �Compare sample characteristics or findings to census/random sample studies �Speculate carefully about bias, and how it is likely to have affected results �Collect sample where population is likely to be �Cultivate diversity in the sample (oversample, quotas) personal, subjective selection �Use of “weighting”
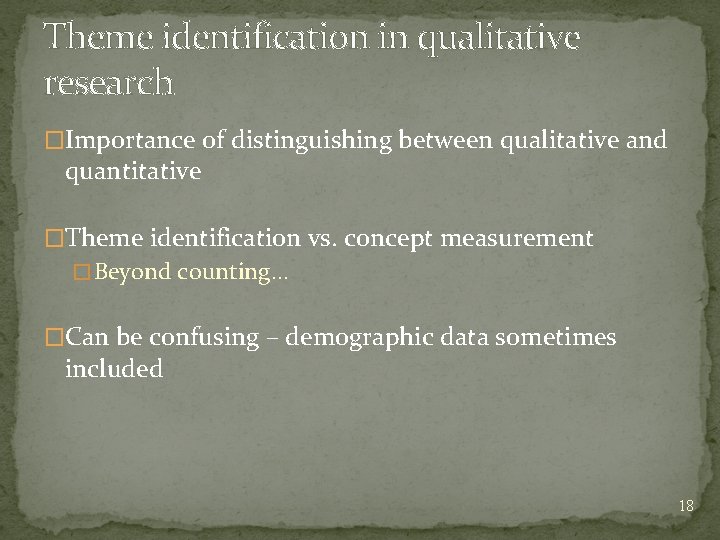
Theme identification in qualitative research �Importance of distinguishing between qualitative and quantitative �Theme identification vs. concept measurement � Beyond counting… �Can be confusing – demographic data sometimes included 18
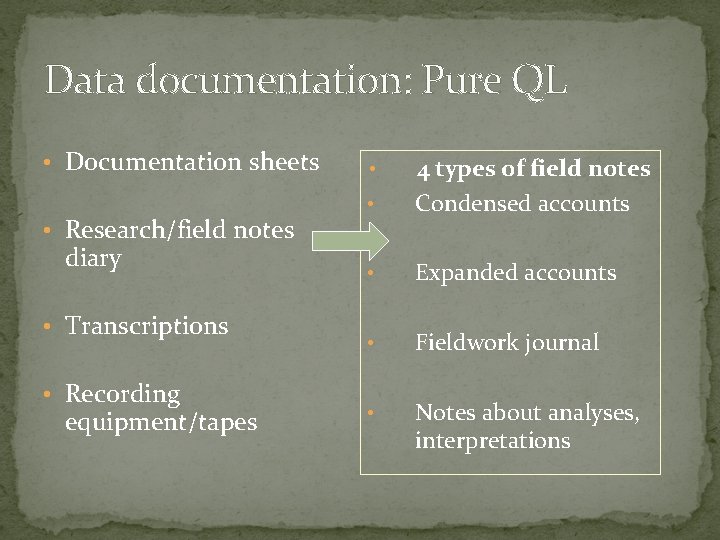
Data documentation: Pure QL • Documentation sheets • Research/field notes diary • Transcriptions • Recording equipment/tapes • 4 types of field notes Condensed accounts • Expanded accounts • Fieldwork journal • Notes about analyses, interpretations •
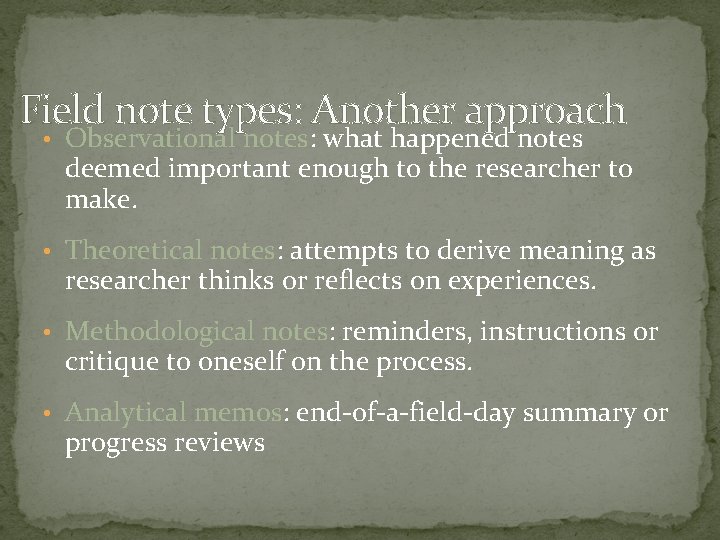
Field note types: Another approach • Observational notes: what happened notes deemed important enough to the researcher to make. • Theoretical notes: attempts to derive meaning as researcher thinks or reflects on experiences. • Methodological notes: reminders, instructions or critique to oneself on the process. • Analytical memos: end-of-a-field-day summary or progress reviews
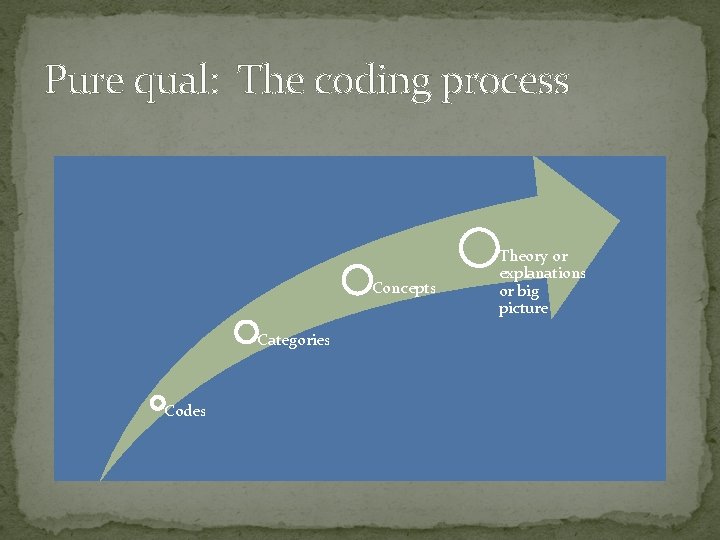
Pure qual: The coding process Concepts Categories Codes Theory or explanations or big picture
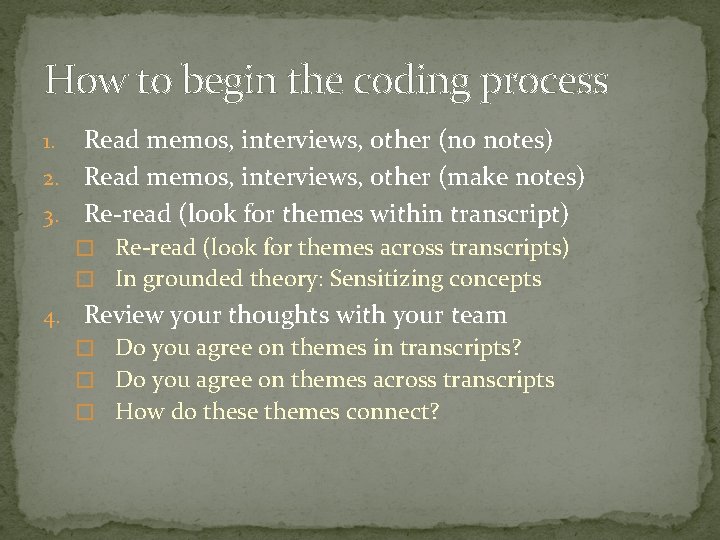
How to begin the coding process Read memos, interviews, other (no notes) 2. Read memos, interviews, other (make notes) 3. Re-read (look for themes within transcript) 1. � Re-read (look for themes across transcripts) � In grounded theory: Sensitizing concepts 4. Review your thoughts with your team � Do you agree on themes in transcripts? � Do you agree on themes across transcripts � How do these themes connect?
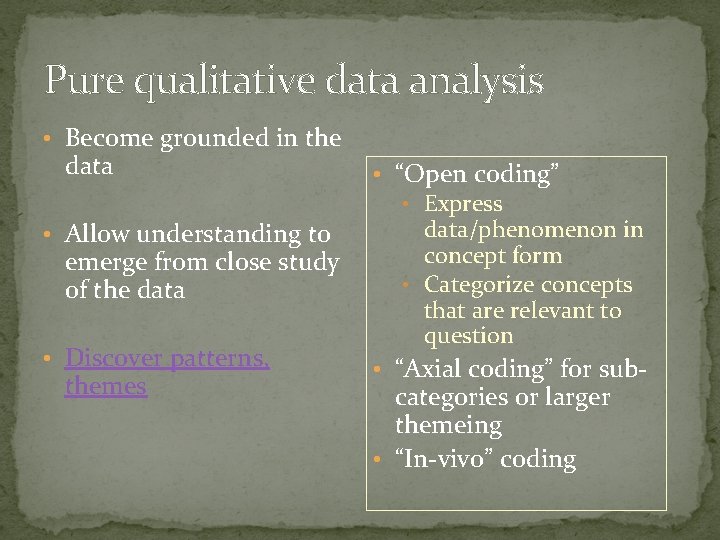
Pure qualitative data analysis • Become grounded in the data • Allow understanding to emerge from close study of the data • Discover patterns, themes • “Open coding” • Express data/phenomenon in concept form • Categorize concepts that are relevant to question • “Axial coding” for sub- categories or larger themeing • “In-vivo” coding
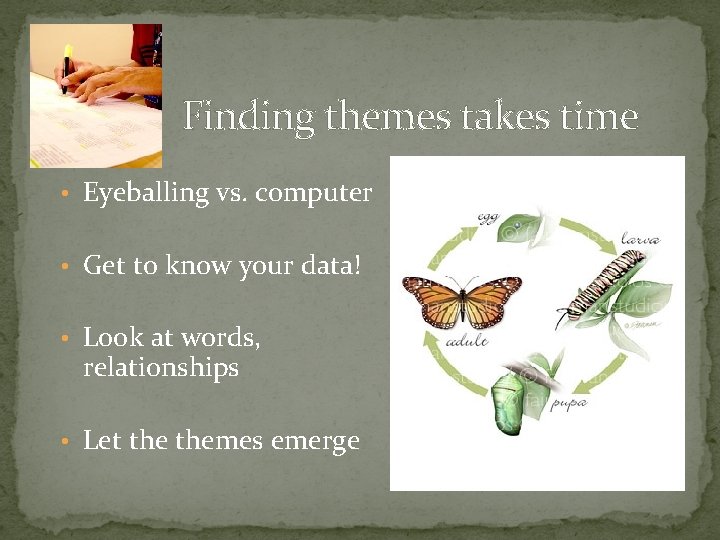
Finding themes takes time • Eyeballing vs. computer • Get to know your data! • Look at words, relationships • Let themes emerge
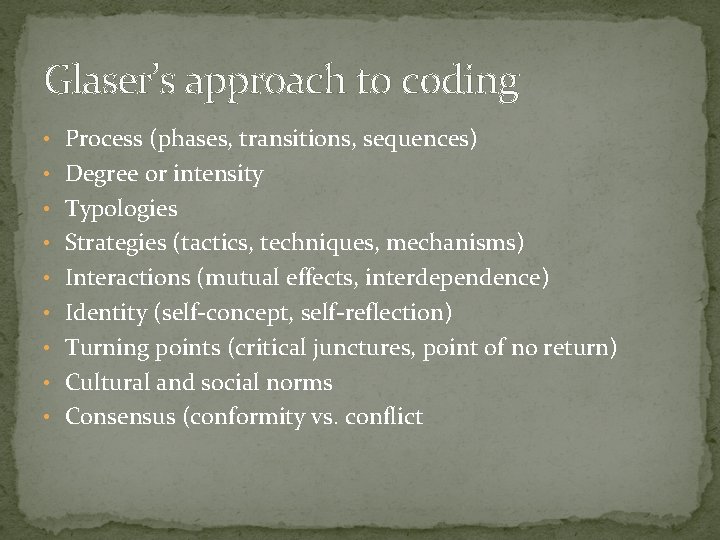
Glaser’s approach to coding • Process (phases, transitions, sequences) • Degree or intensity • Typologies • Strategies (tactics, techniques, mechanisms) • Interactions (mutual effects, interdependence) • Identity (self-concept, self-reflection) • Turning points (critical junctures, point of no return) • Cultural and social norms • Consensus (conformity vs. conflict
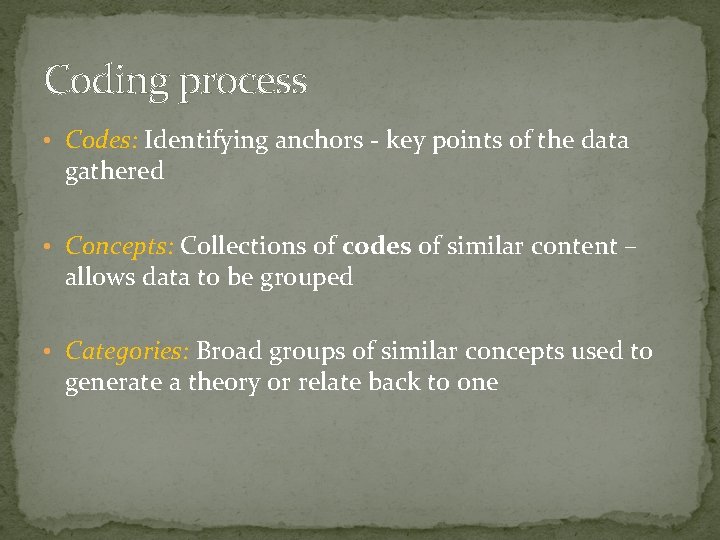
Coding process • Codes: Identifying anchors - key points of the data gathered • Concepts: Collections of codes of similar content – allows data to be grouped • Categories: Broad groups of similar concepts used to generate a theory or relate back to one
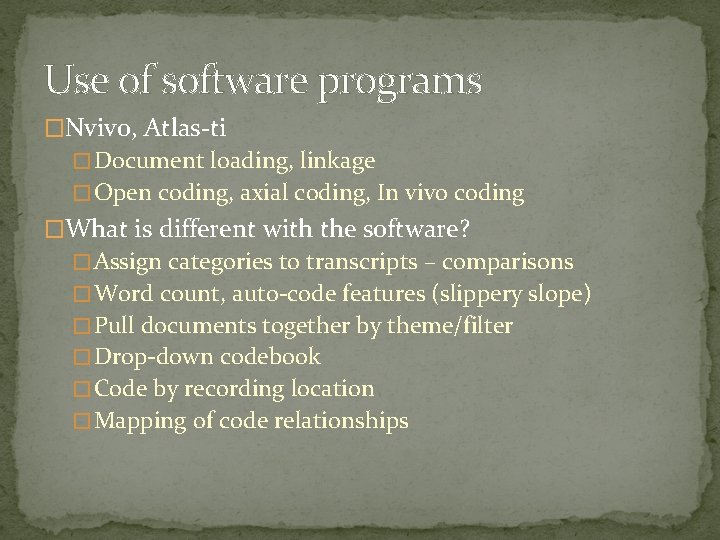
Use of software programs �Nvivo, Atlas-ti � Document loading, linkage � Open coding, axial coding, In vivo coding �What is different with the software? � Assign categories to transcripts – comparisons � Word count, auto-code features (slippery slope) � Pull documents together by theme/filter � Drop-down codebook � Code by recording location � Mapping of code relationships
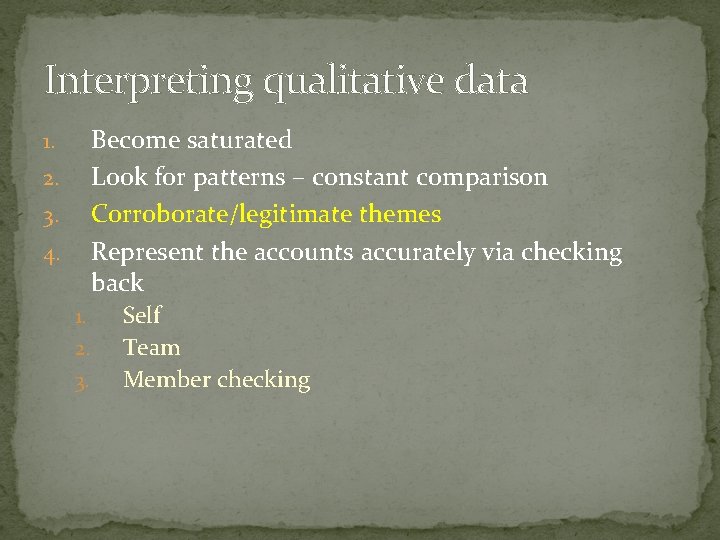
Interpreting qualitative data Become saturated Look for patterns – constant comparison Corroborate/legitimate themes Represent the accounts accurately via checking back 1. 2. 3. 4. 1. 2. 3. Self Team Member checking
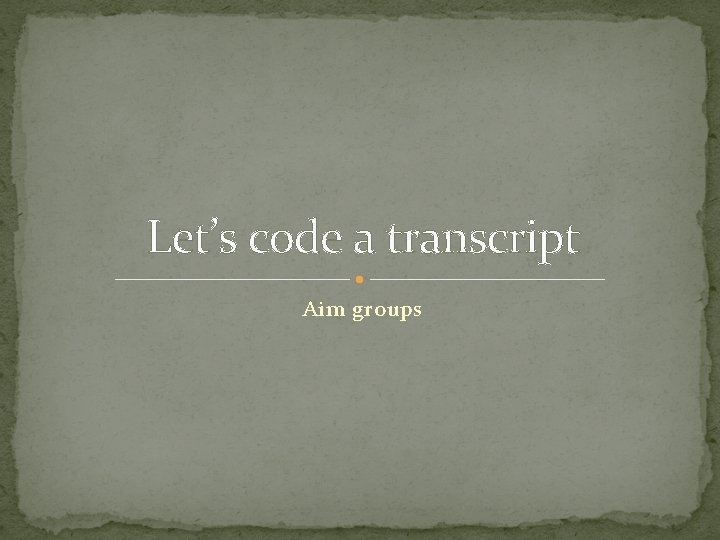
Let’s code a transcript Aim groups
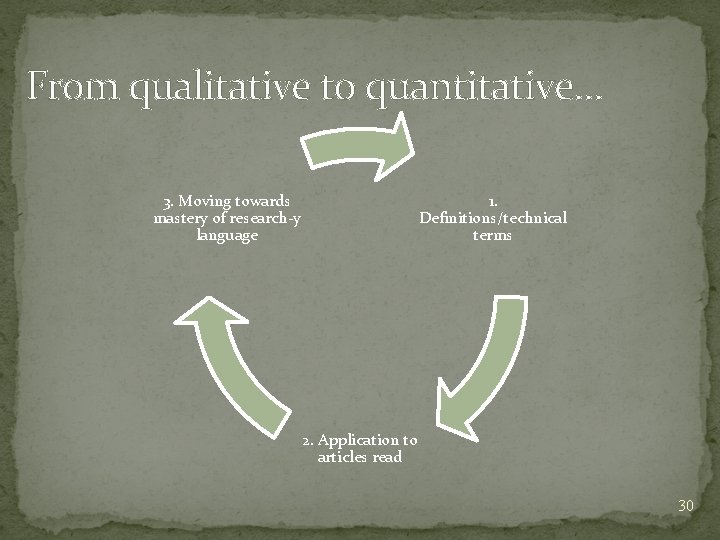
From qualitative to quantitative… 3. Moving towards mastery of research-y language 1. Definitions/technical terms 2. Application to articles read 30
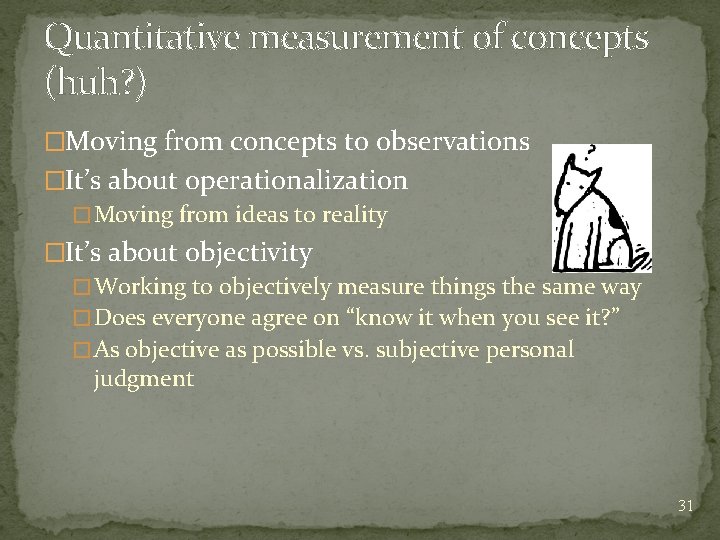
Quantitative measurement of concepts (huh? ) �Moving from concepts to observations �It’s about operationalization � Moving from ideas to reality �It’s about objectivity � Working to objectively measure things the same way � Does everyone agree on “know it when you see it? ” � As objective as possible vs. subjective personal judgment 31
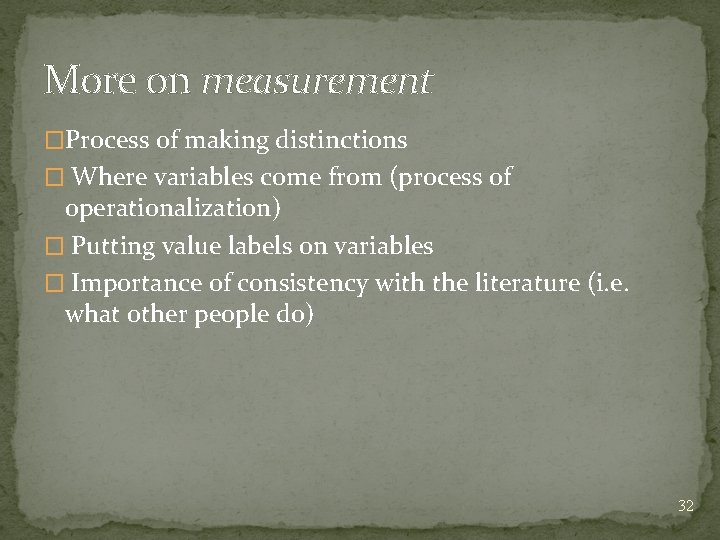
More on measurement �Process of making distinctions � Where variables come from (process of operationalization) � Putting value labels on variables � Importance of consistency with the literature (i. e. what other people do) 32
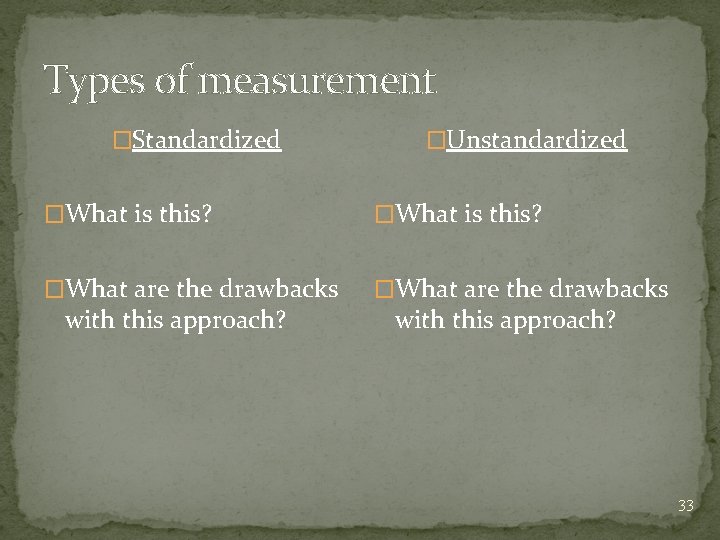
Types of measurement �Standardized �Unstandardized �What is this? �What are the drawbacks with this approach? 33
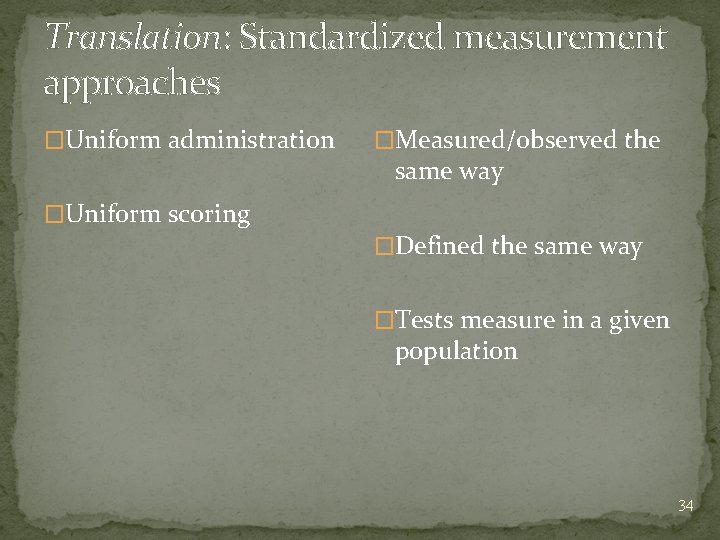
Translation: Standardized measurement approaches �Uniform administration �Uniform scoring �Measured/observed the same way �Defined the same way �Tests measure in a given population 34
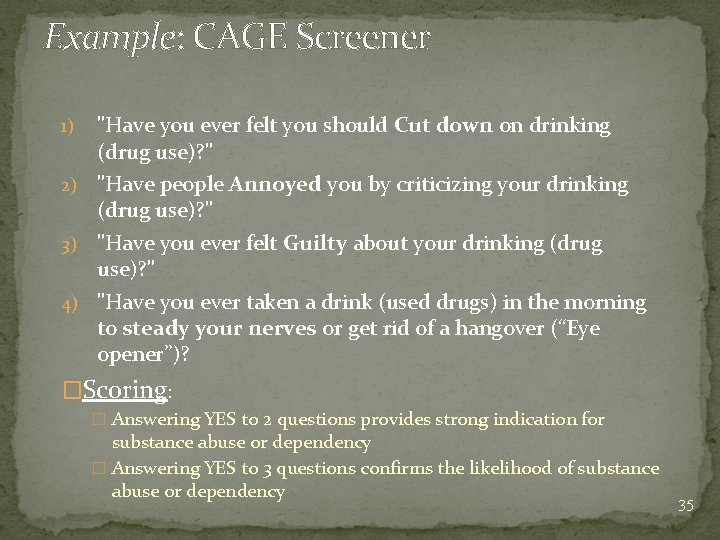
Example: CAGE Screener "Have you ever felt you should Cut down on drinking (drug use)? " 2) "Have people Annoyed you by criticizing your drinking (drug use)? " 3) "Have you ever felt Guilty about your drinking (drug use)? " 4) "Have you ever taken a drink (used drugs) in the morning to steady your nerves or get rid of a hangover (“Eye opener”)? 1) �Scoring: � Answering YES to 2 questions provides strong indication for substance abuse or dependency � Answering YES to 3 questions confirms the likelihood of substance abuse or dependency 35
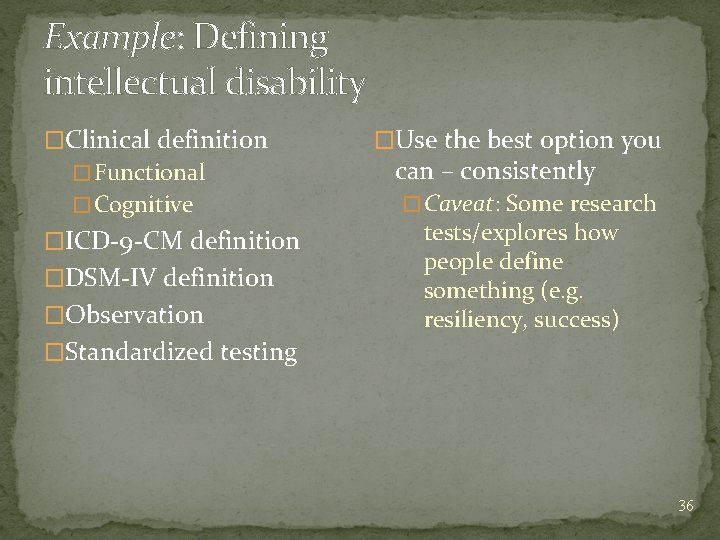
Example: Defining intellectual disability �Clinical definition � Functional � Cognitive �ICD-9 -CM definition �DSM-IV definition �Observation �Use the best option you can – consistently � Caveat: Some research tests/explores how people define something (e. g. resiliency, success) �Standardized testing 36
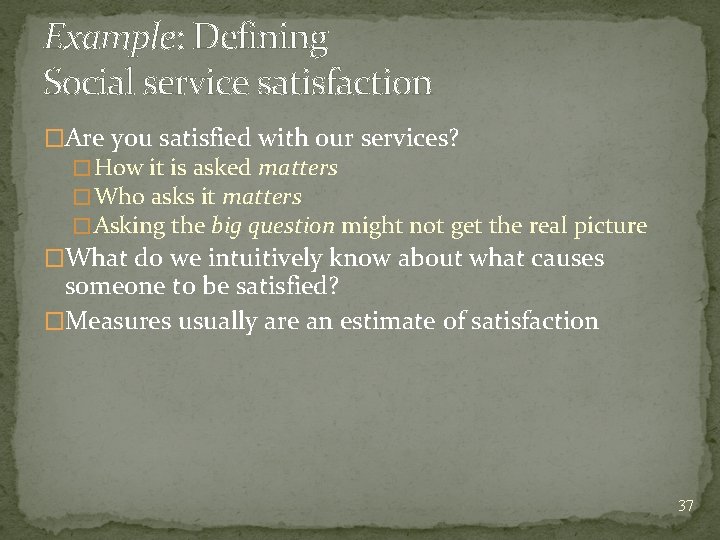
Example: Defining Social service satisfaction �Are you satisfied with our services? � How it is asked matters � Who asks it matters � Asking the big question might not get the real picture �What do we intuitively know about what causes someone to be satisfied? �Measures usually are an estimate of satisfaction 37
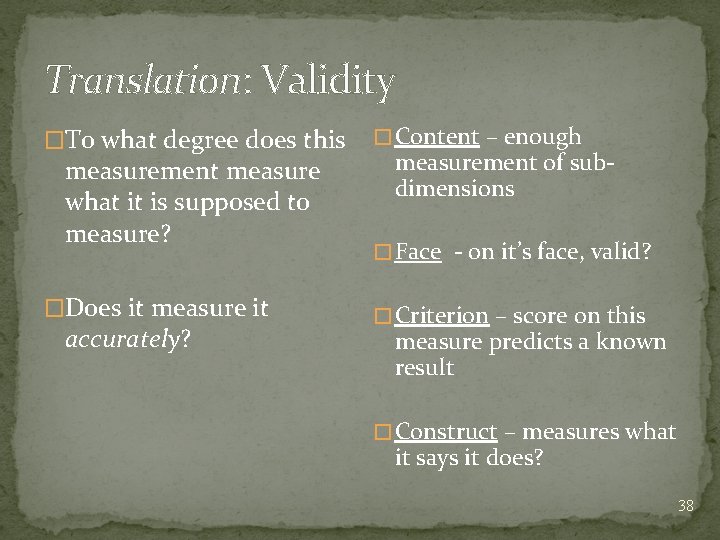
Translation: Validity �To what degree does this � Content – enough measurement measure what it is supposed to measure? �Does it measure it accurately? measurement of subdimensions � Face - on it’s face, valid? � Criterion – score on this measure predicts a known result � Construct – measures what it says it does? 38
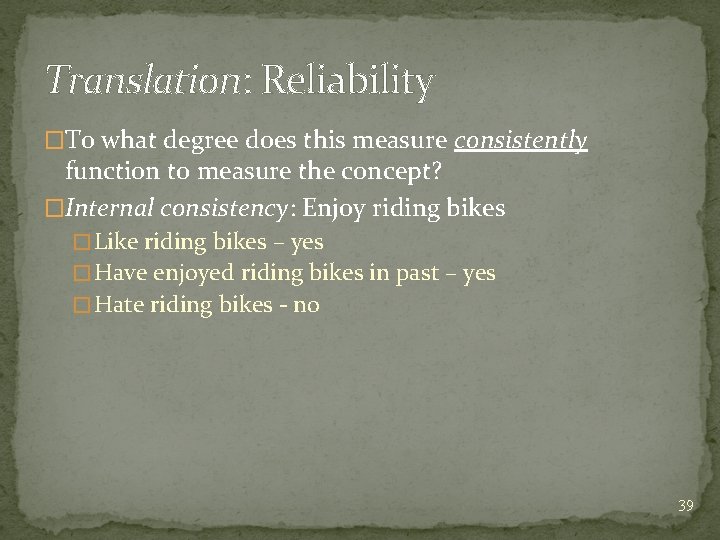
Translation: Reliability �To what degree does this measure consistently function to measure the concept? �Internal consistency: Enjoy riding bikes � Like riding bikes – yes � Have enjoyed riding bikes in past – yes � Hate riding bikes - no 39
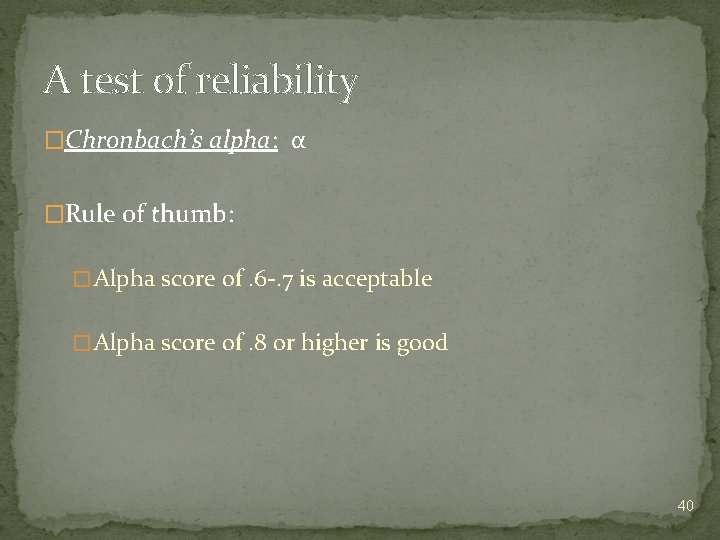
A test of reliability �Chronbach’s alpha: α �Rule of thumb: � Alpha score of. 6 -. 7 is acceptable � Alpha score of. 8 or higher is good 40
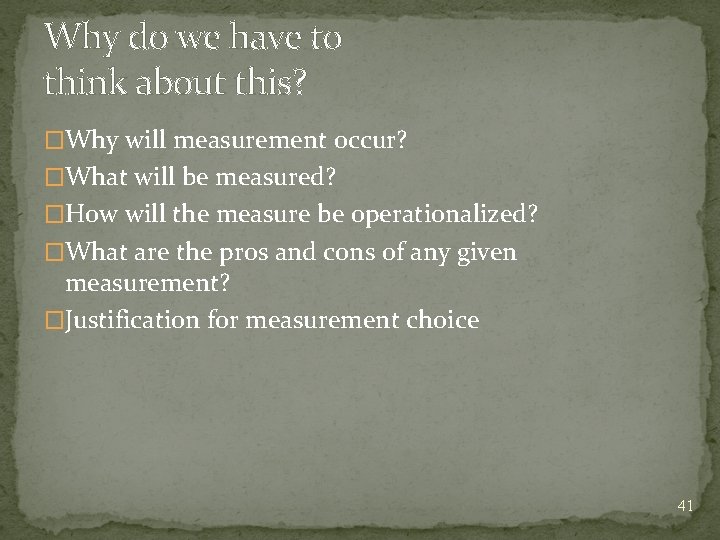
Why do we have to think about this? �Why will measurement occur? �What will be measured? �How will the measure be operationalized? �What are the pros and cons of any given measurement? �Justification for measurement choice 41
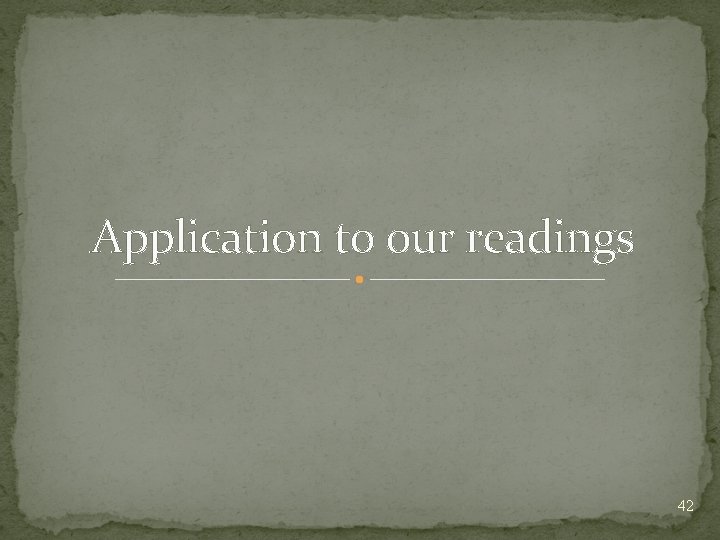
Application to our readings 42
Positive identification example
Gim social work
Social work assessment questions
Communication and interpersonal skills in social work
National association of forensic social workers
National association of social workers texas
Objective in problem formulation means
Theme identification adalah
Social thinking and social influence
Social thinking social influence social relations
Theme and genre
What is the difference between theme and universal theme?
Formuö
Typiska novell drag
Nationell inriktning för artificiell intelligens
Vad står k.r.å.k.a.n för
Shingelfrisyren
En lathund för arbete med kontinuitetshantering
Personalliggare bygg undantag
Personlig tidbok
Sura för anatom
Densitet vatten
Datorkunskap för nybörjare
Boverket ka
Att skriva debattartikel
Magnetsjukhus
Nyckelkompetenser för livslångt lärande
Påbyggnader för flakfordon
Vätsketryck formel
Publik sektor
Bo bergman jag fryser om dina händer
Presentera för publik crossboss
Jiddisch
Plats för toran ark
Treserva lathund
Mjälthilus
Bästa kameran för astrofoto
Cks
Byggprocessen steg för steg
Mat för idrottare
Verktyg för automatisering av utbetalningar
Rutin för avvikelsehantering
Smärtskolan kunskap för livet