Progress Report on Cloudy Radiance Data Assimilation in
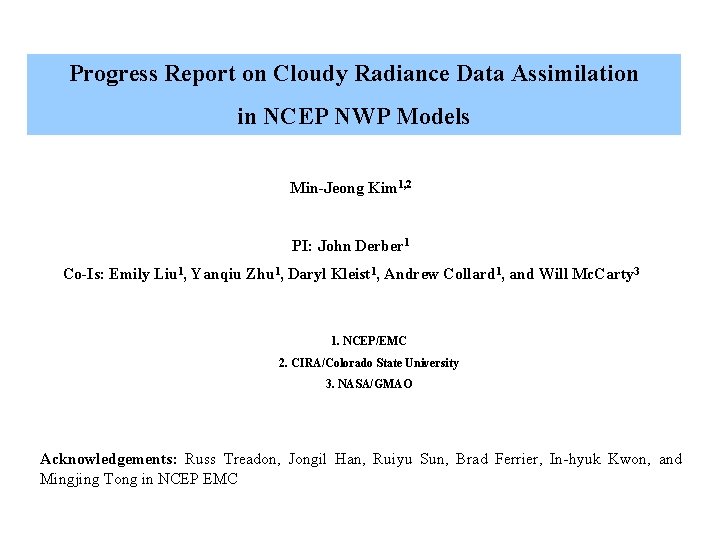
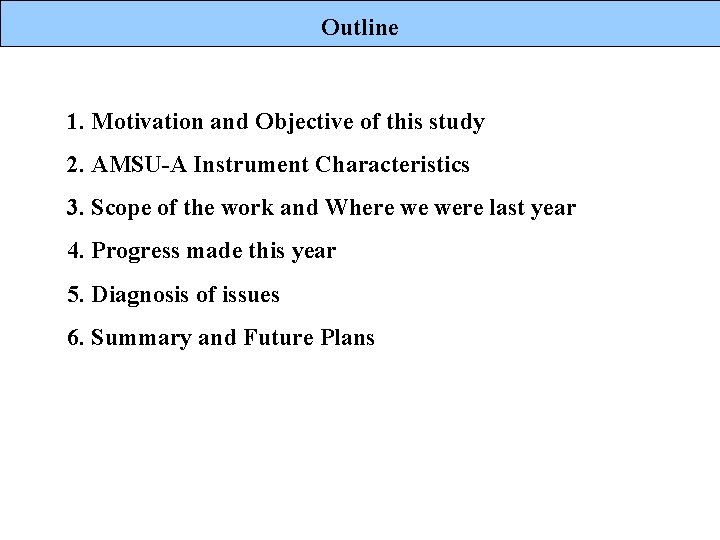
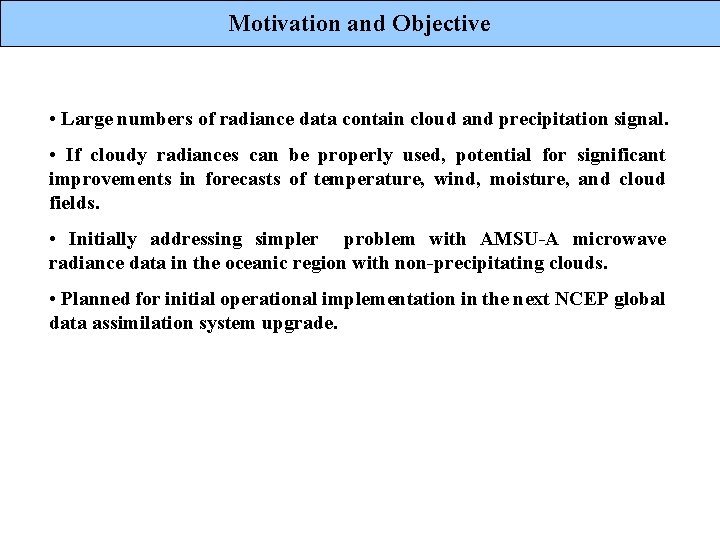
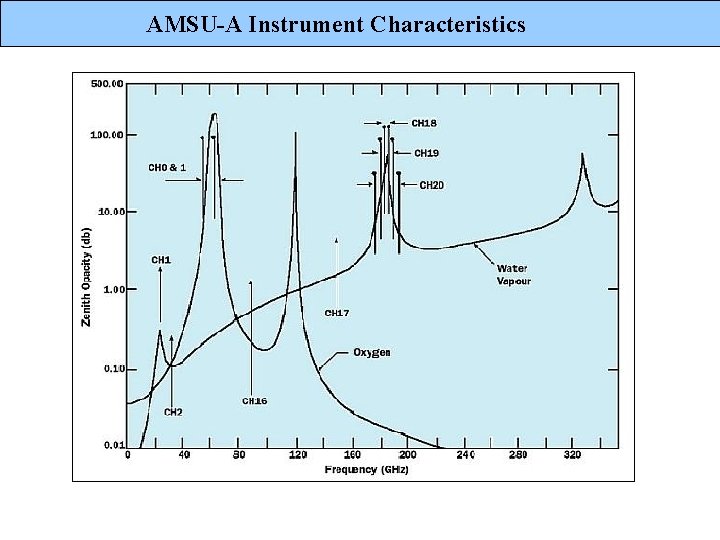
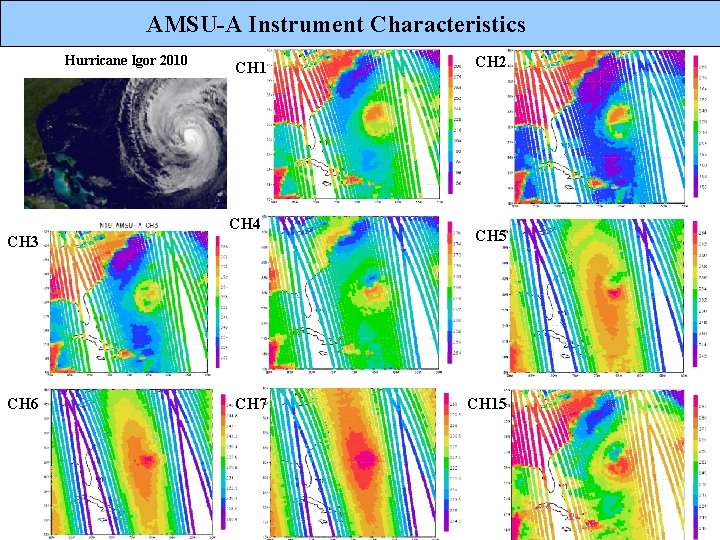
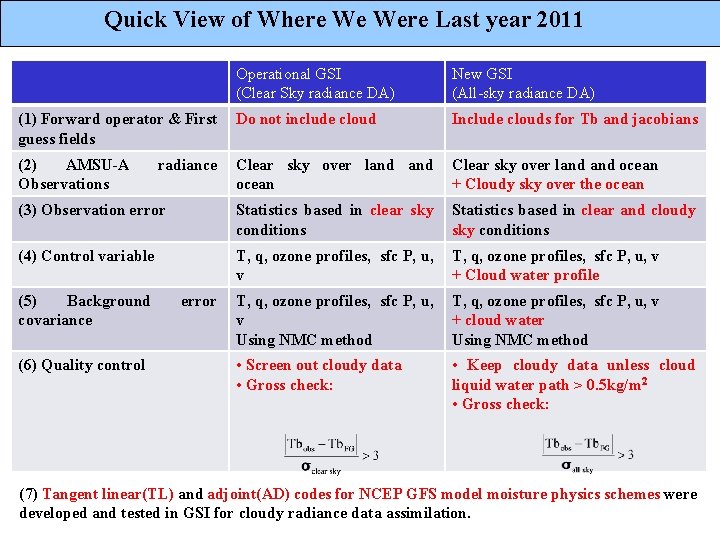
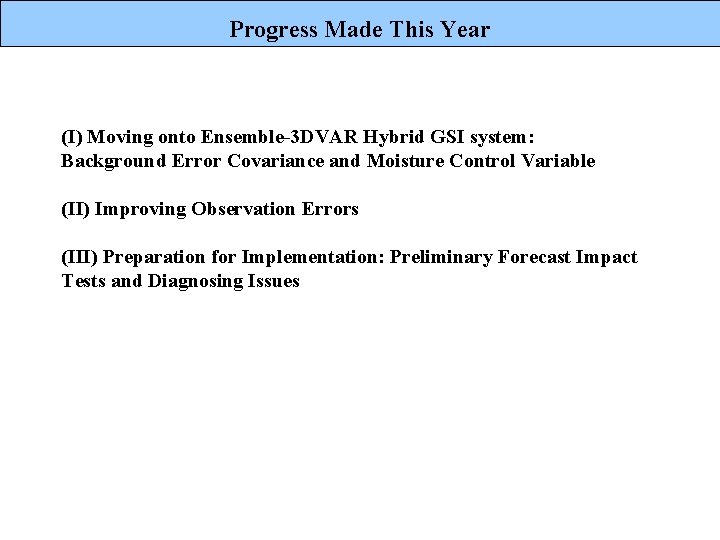
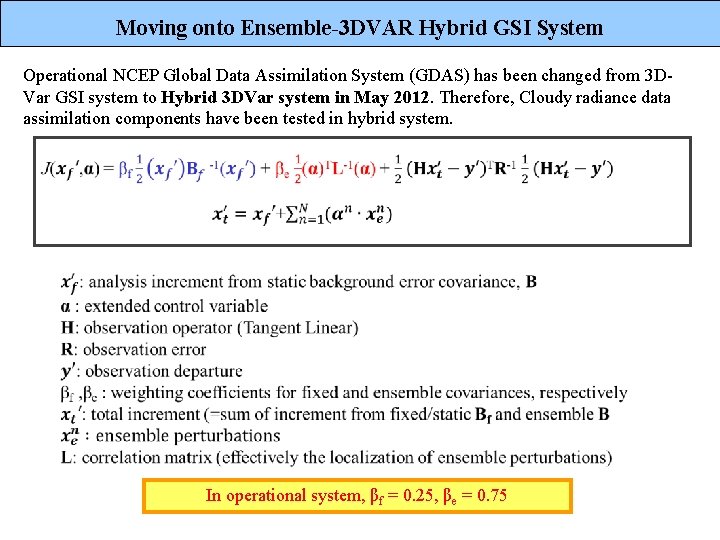
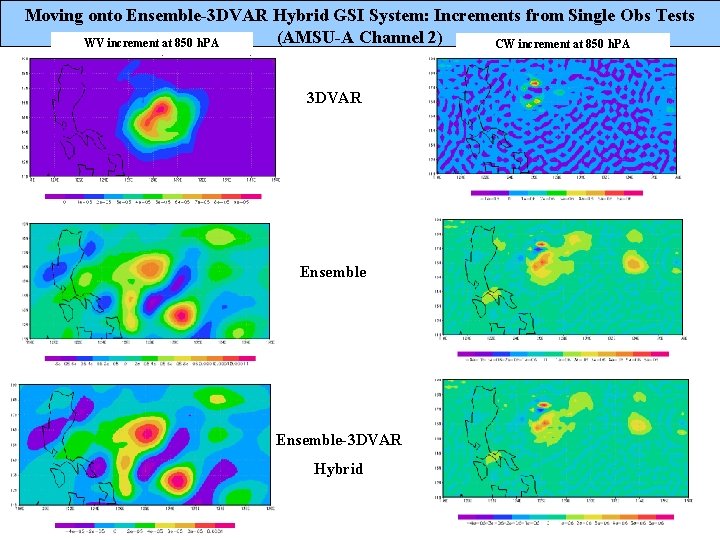
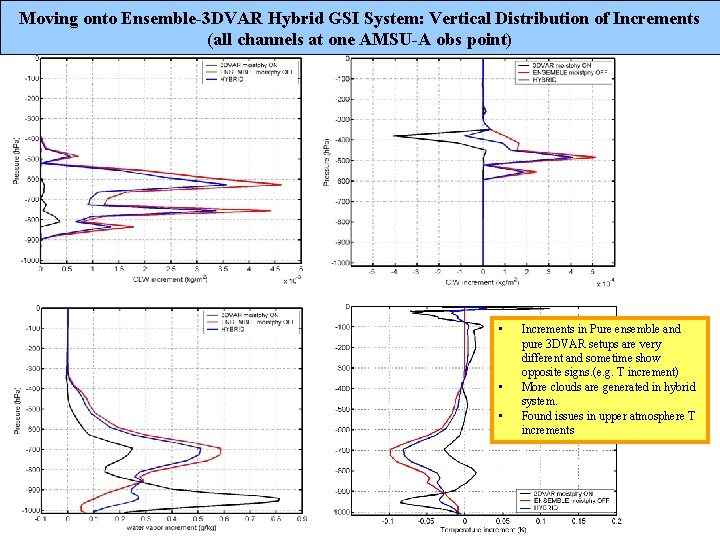
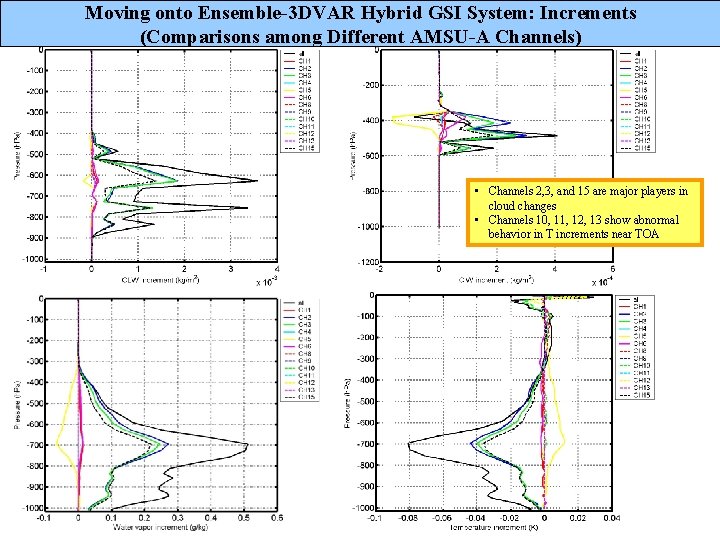
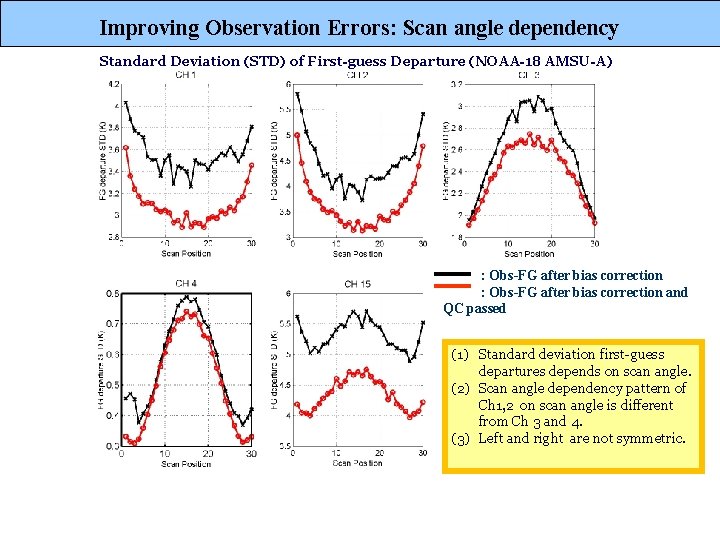
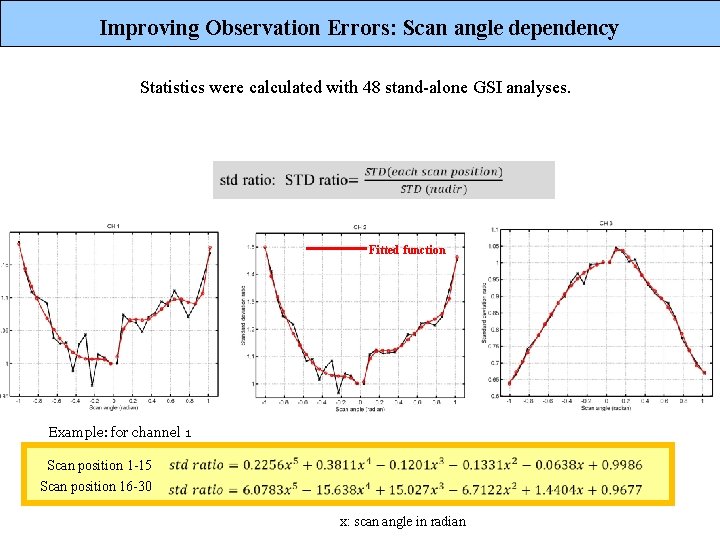
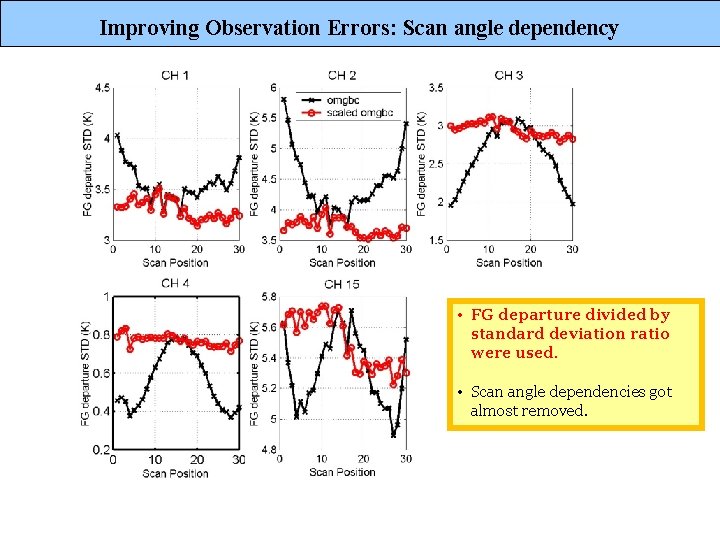
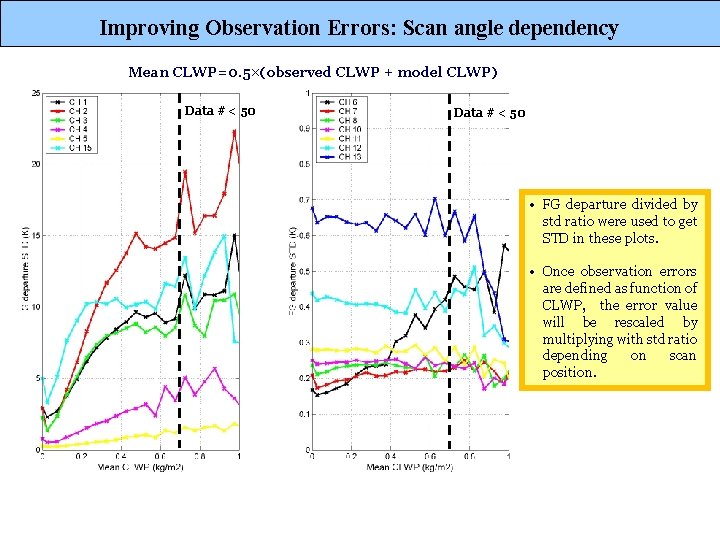
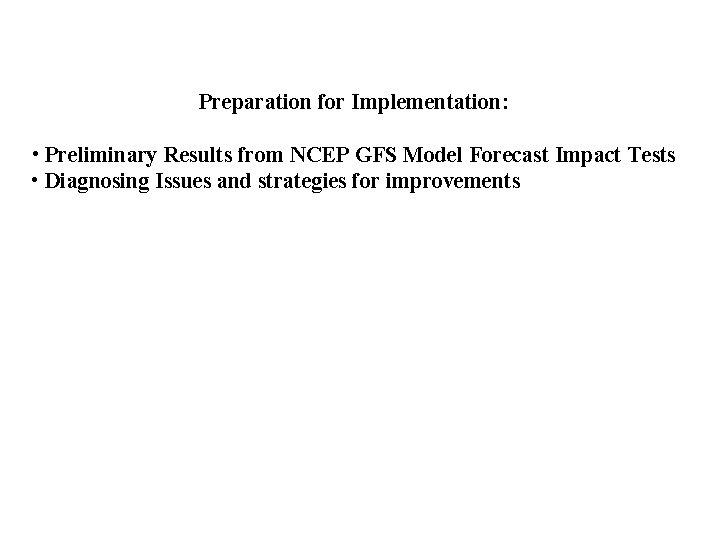
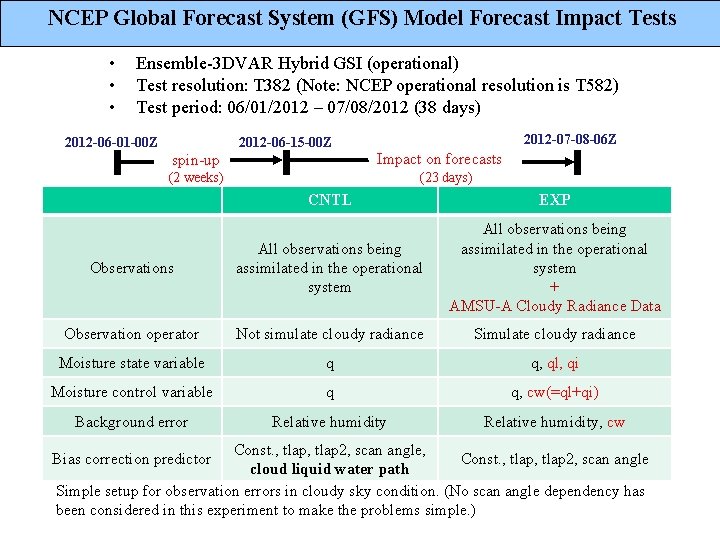
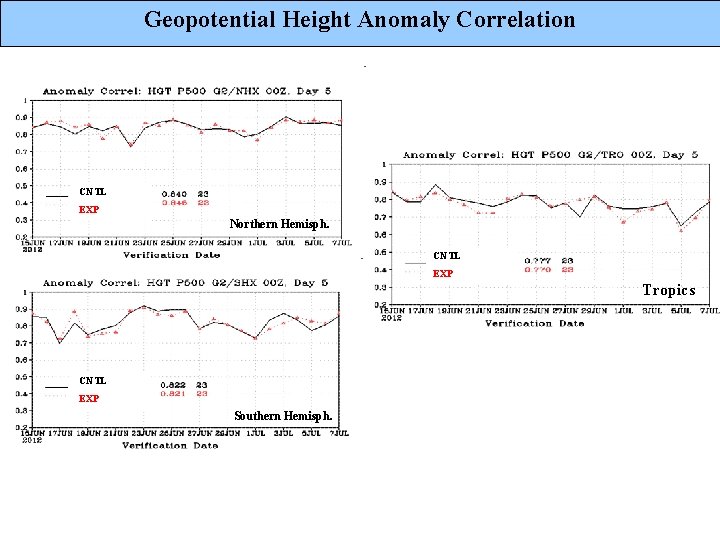
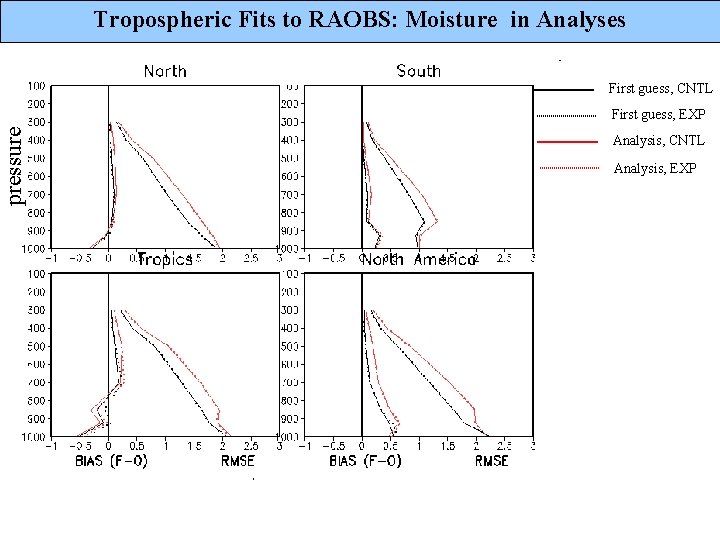
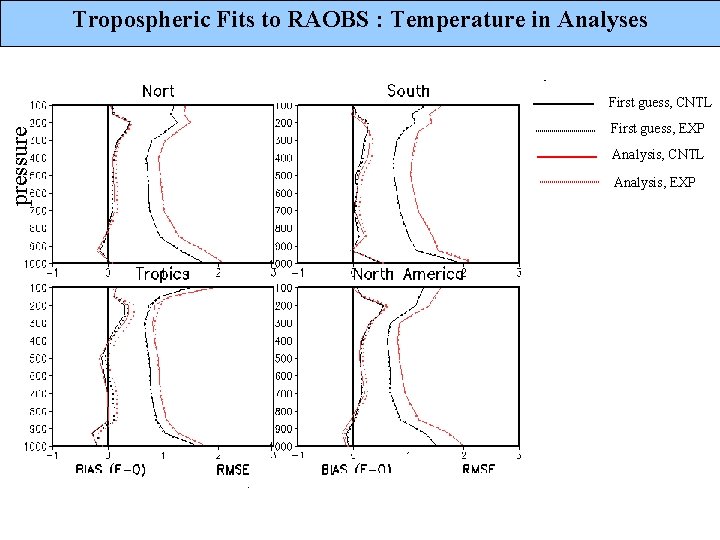
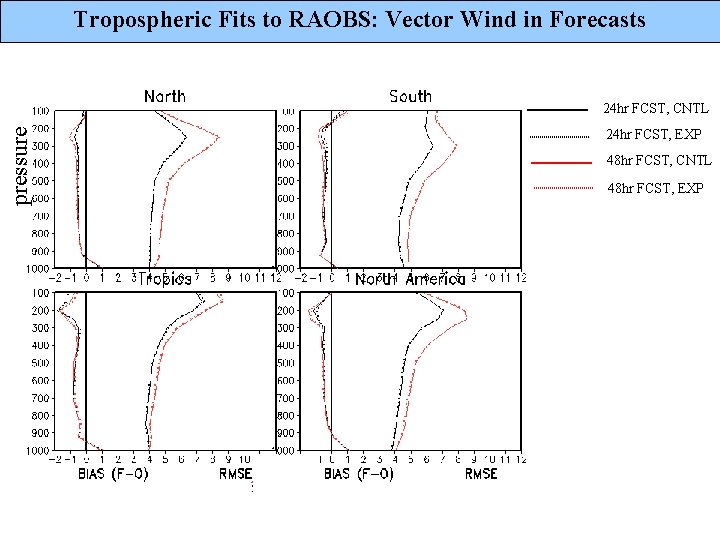
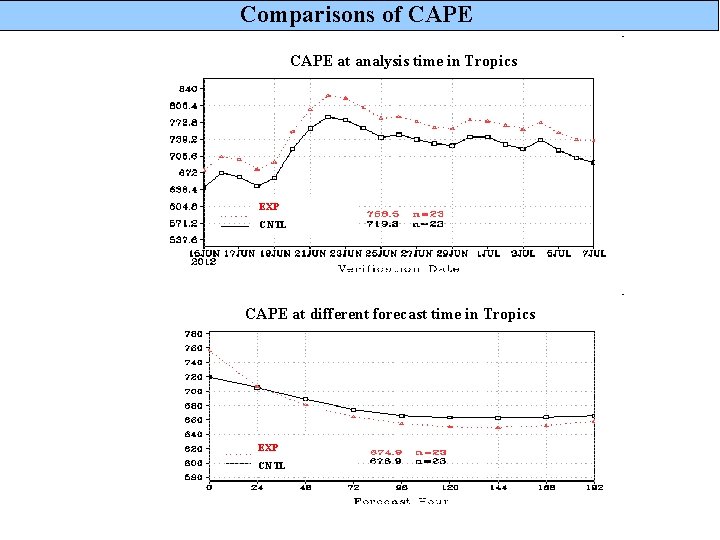
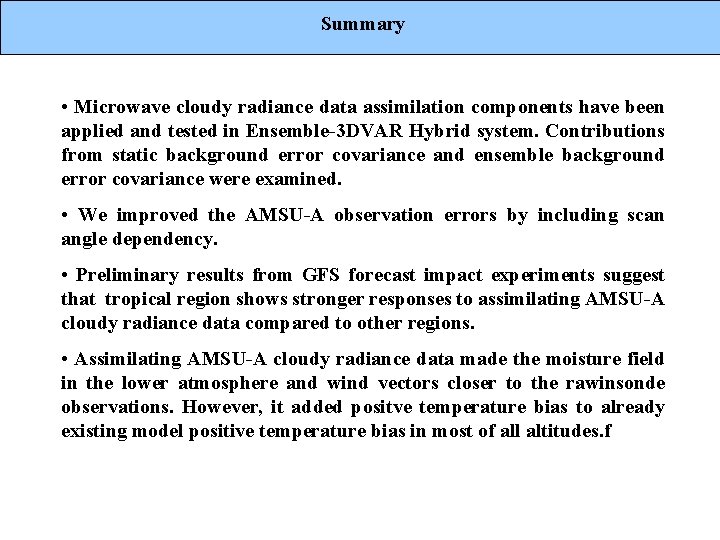
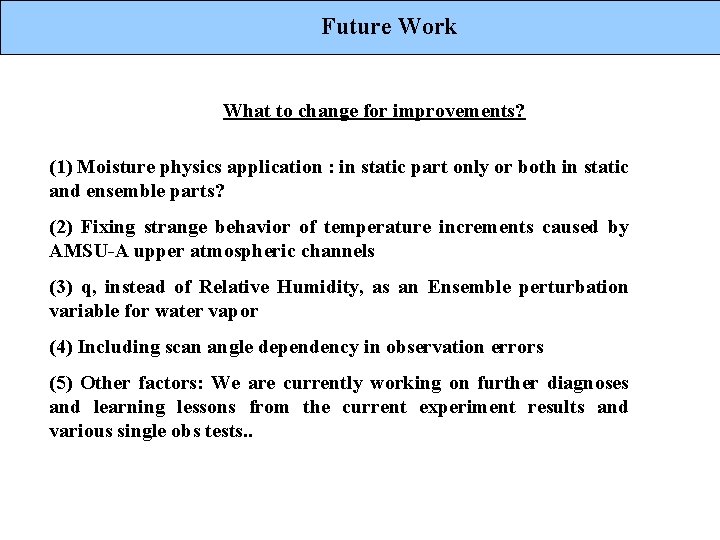
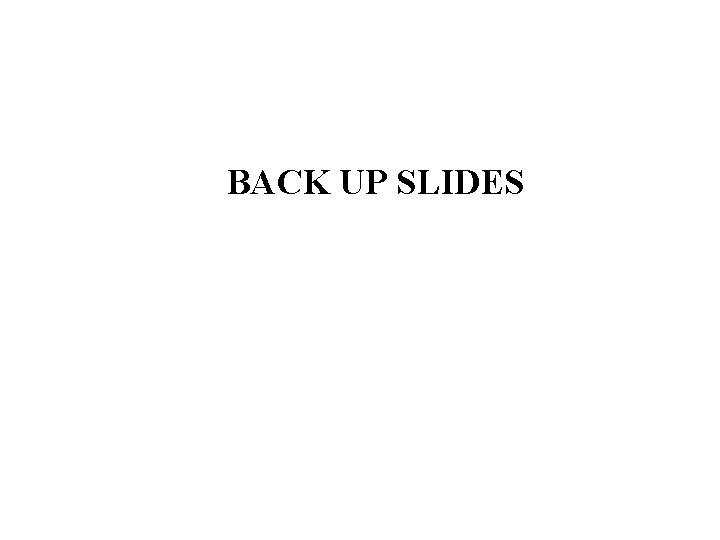
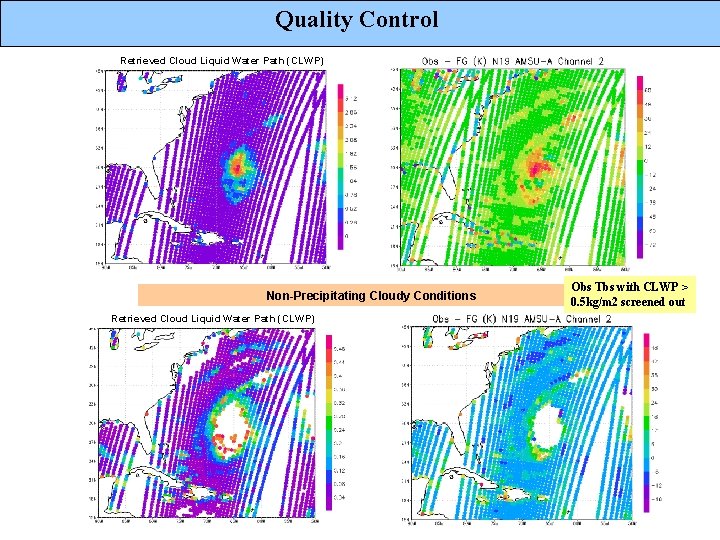
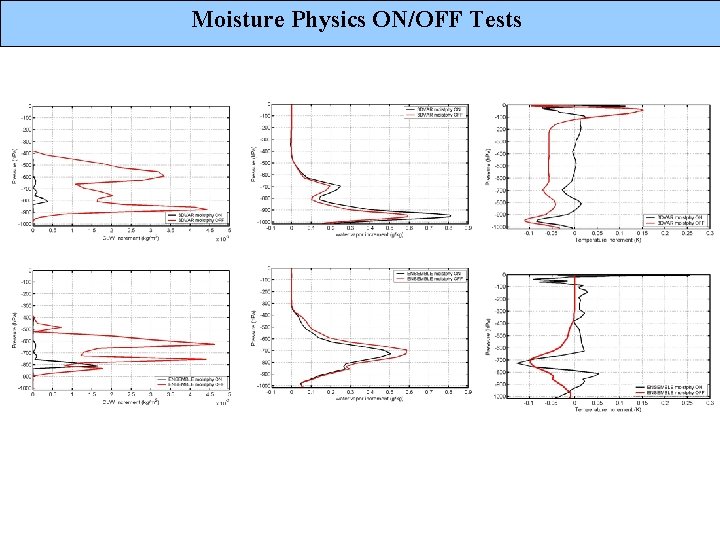
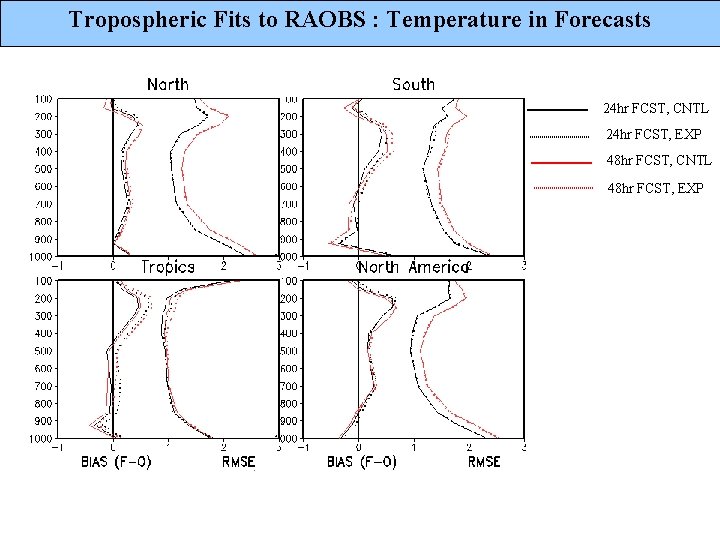
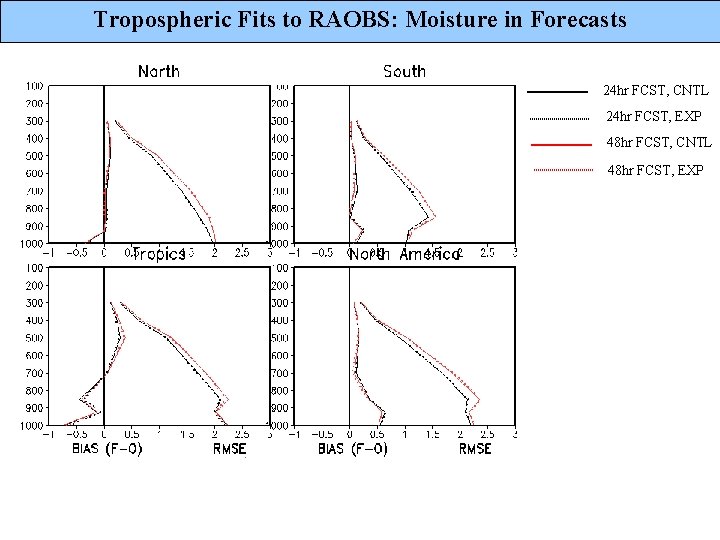
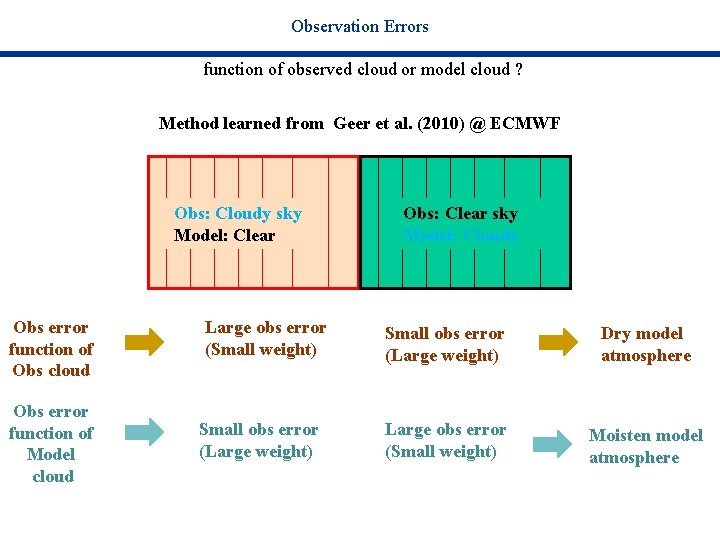
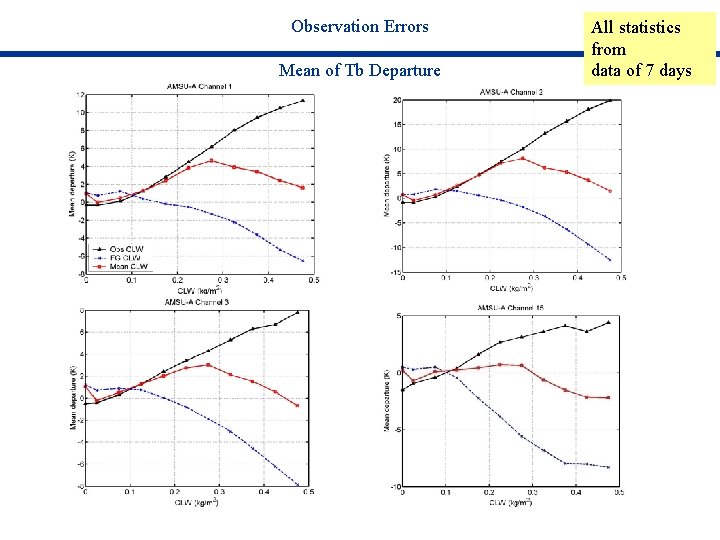
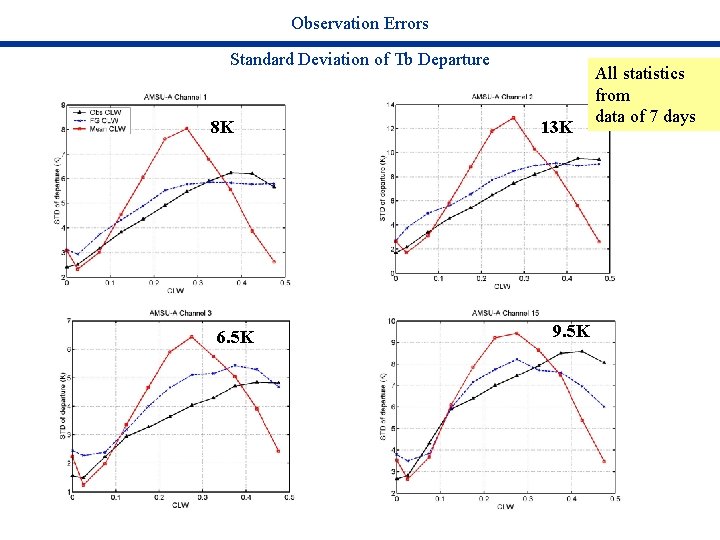
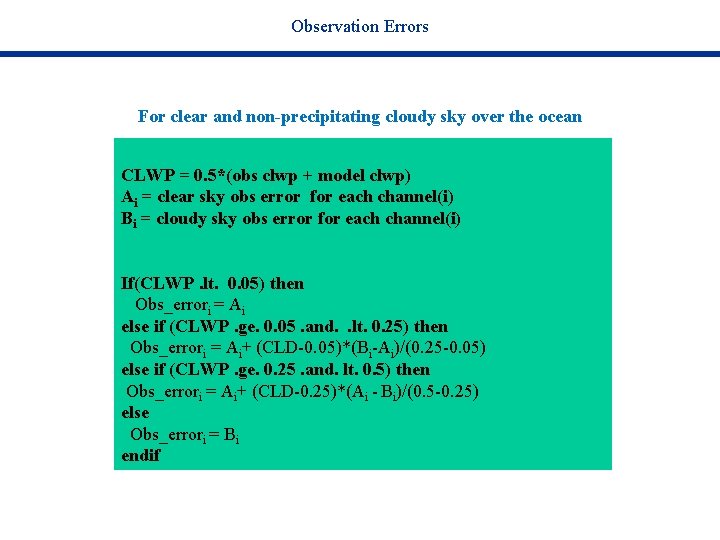
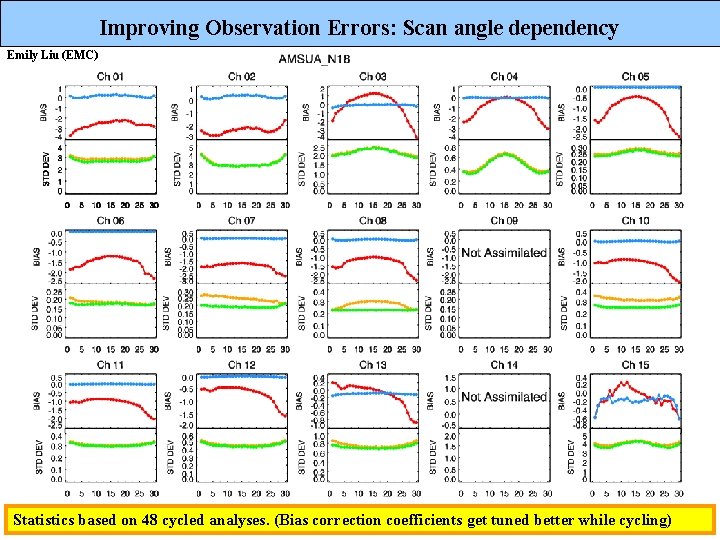
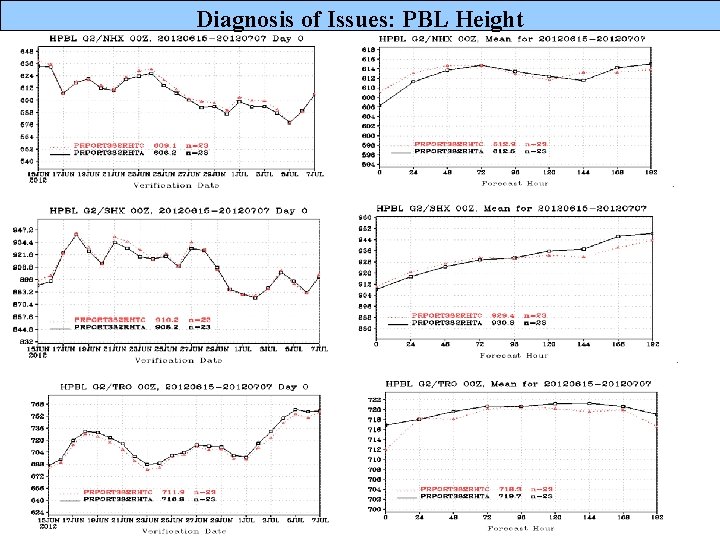
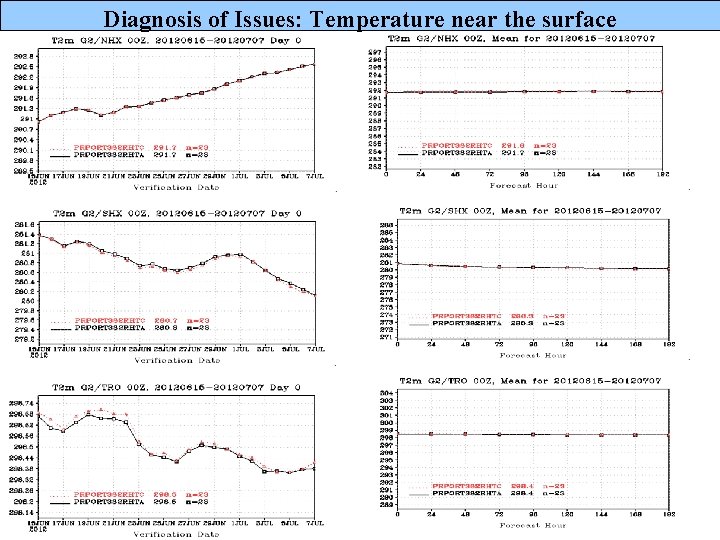
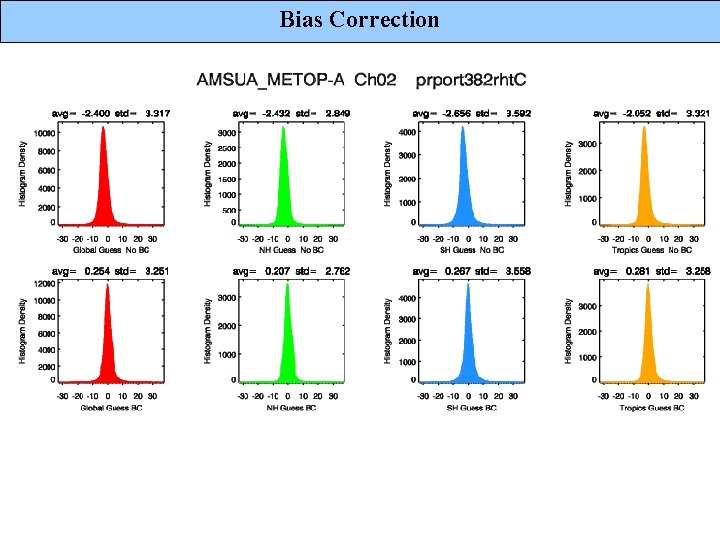
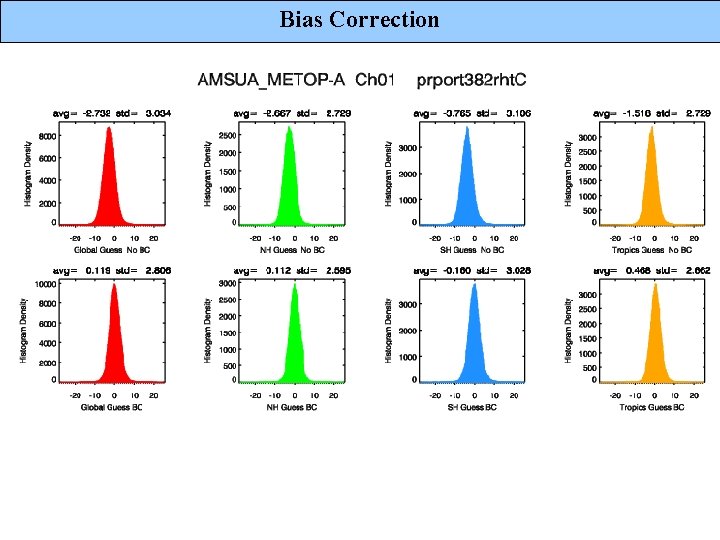
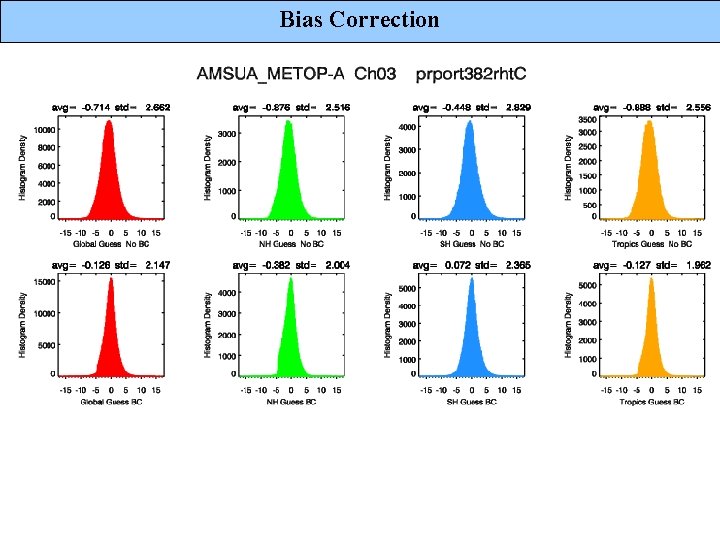
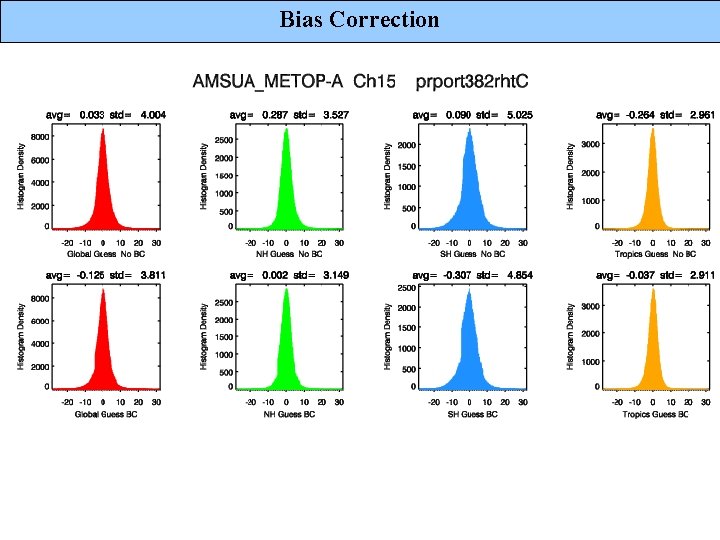
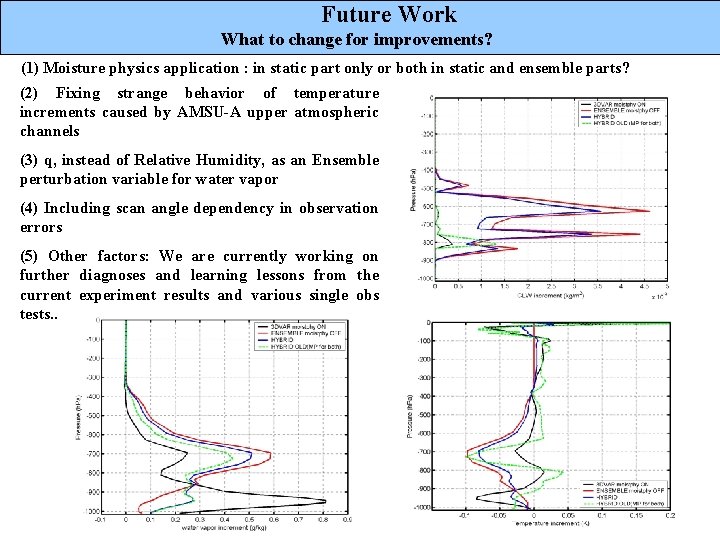
- Slides: 41
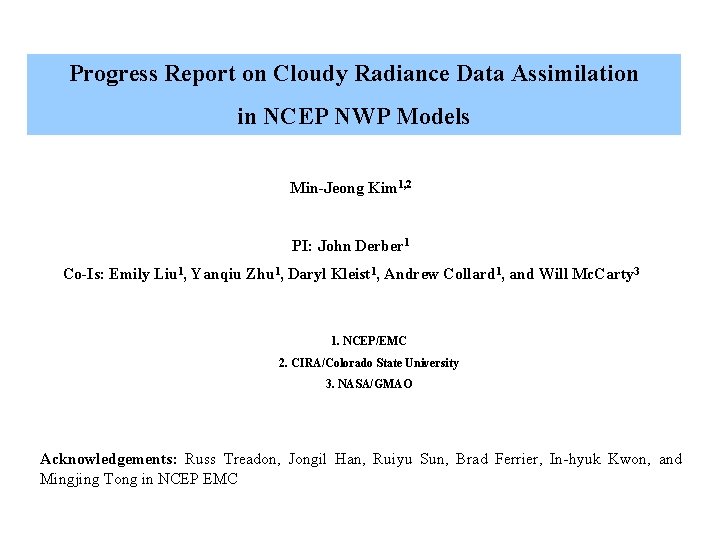
Progress Report on Cloudy Radiance Data Assimilation in NCEP NWP Models Min-Jeong Kim 1, 2 PI: John Derber 1 Co-Is: Emily Liu 1, Yanqiu Zhu 1, Daryl Kleist 1, Andrew Collard 1, and Will Mc. Carty 3 1. NCEP/EMC 2. CIRA/Colorado State University 3. NASA/GMAO Acknowledgements: Russ Treadon, Jongil Han, Ruiyu Sun, Brad Ferrier, In-hyuk Kwon, and Mingjing Tong in NCEP EMC
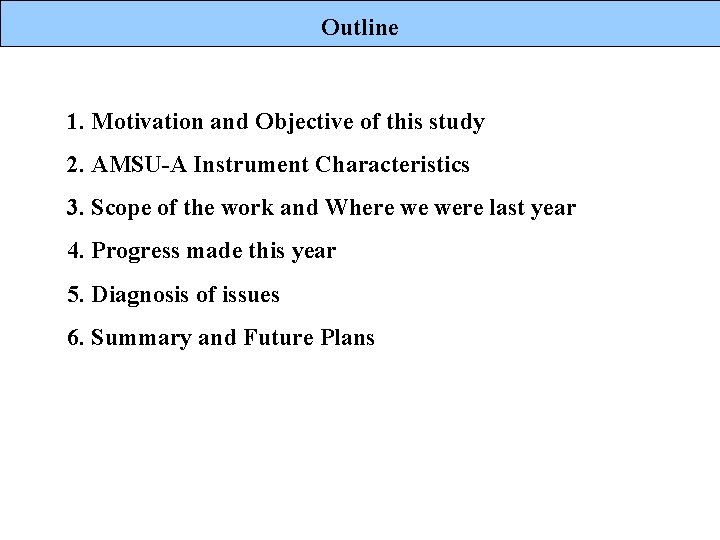
Outline 1. Motivation and Objective of this study 2. AMSU-A Instrument Characteristics 3. Scope of the work and Where we were last year 4. Progress made this year 5. Diagnosis of issues 6. Summary and Future Plans
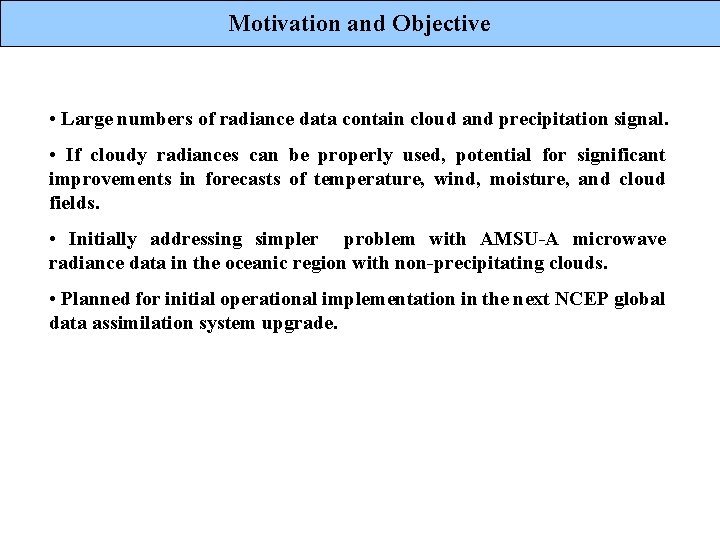
Motivation and Objective • Large numbers of radiance data contain cloud and precipitation signal. • If cloudy radiances can be properly used, potential for significant improvements in forecasts of temperature, wind, moisture, and cloud fields. • Initially addressing simpler problem with AMSU-A microwave radiance data in the oceanic region with non-precipitating clouds. • Planned for initial operational implementation in the next NCEP global data assimilation system upgrade.
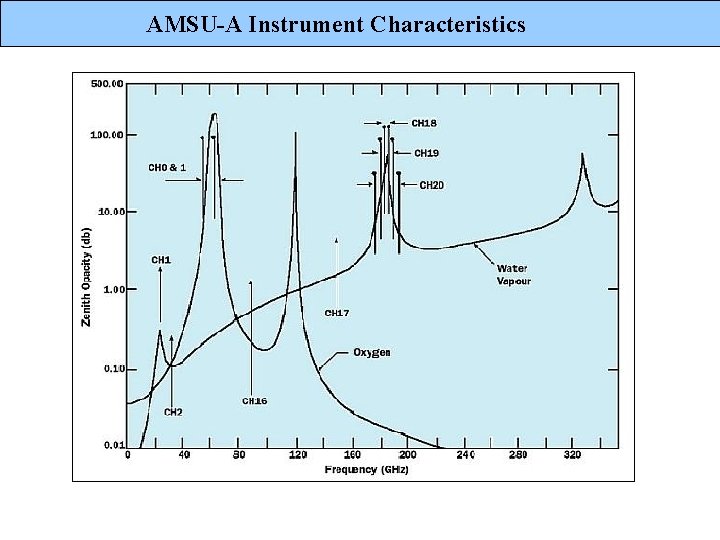
AMSU-A Instrument Characteristics
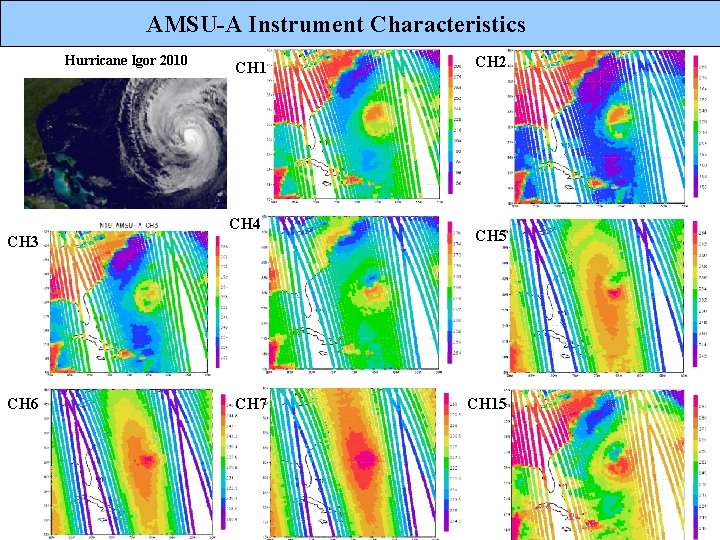
AMSU-A Instrument Characteristics Hurricane Igor 2010 CH 1 CH 4 CH 3 CH 6 CH 7 CH 2 CH 5 CH 15
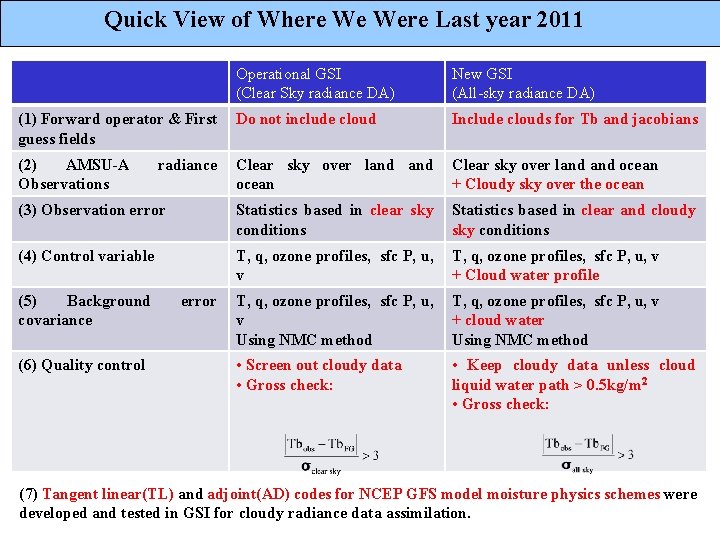
Quick View of Where We Were Last year 2011 Operational GSI (Clear Sky radiance DA) New GSI (All-sky radiance DA) (1) Forward operator & First guess fields Do not include cloud Include clouds for Tb and jacobians (2) AMSU-A Observations Clear sky over land and ocean + Cloudy sky over the ocean (3) Observation error Statistics based in clear sky conditions Statistics based in clear and cloudy sky conditions (4) Control variable T, q, ozone profiles, sfc P, u, v Not cloud water T, q, including ozone profiles, sfc P, u, T, q, ozone profiles, sfc P, u, v + Cloud water profile (5) Background covariance (6) Quality control radiance error v Using NMC method • Screen out cloudy data • Gross check: T, q, ozone profiles, sfc P, u, v + cloud water Using NMC method • Keep cloudy data unless cloud liquid water path > 0. 5 kg/m 2 • Gross check: (7) Tangent linear(TL) and adjoint(AD) codes for NCEP GFS model moisture physics schemes were developed and tested in GSI for cloudy radiance data assimilation.
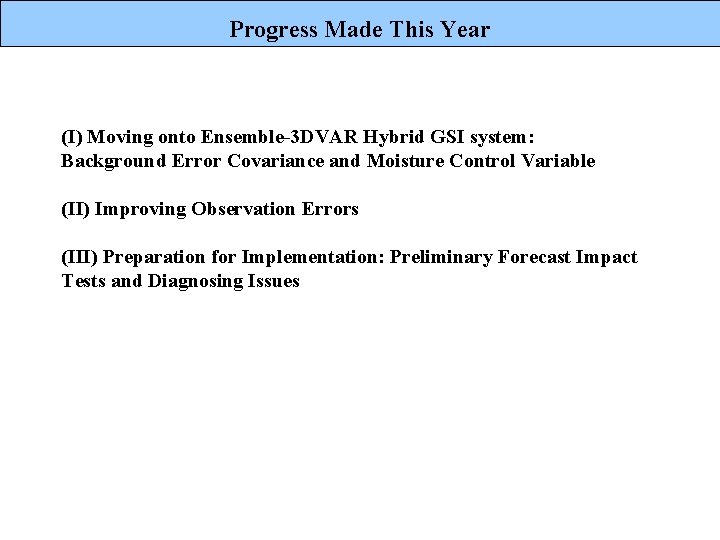
Progress Made This Year (I) Moving onto Ensemble-3 DVAR Hybrid GSI system: Background Error Covariance and Moisture Control Variable (II) Improving Observation Errors (III) Preparation for Implementation: Preliminary Forecast Impact Tests and Diagnosing Issues
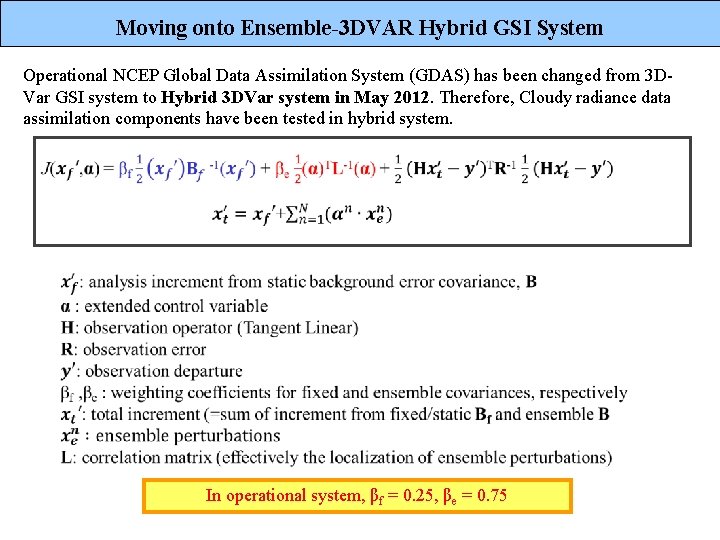
Moving onto Ensemble-3 DVAR Hybrid GSI System Operational NCEP Global Data Assimilation System (GDAS) has been changed from 3 DVar GSI system to Hybrid 3 DVar system in May 2012. Therefore, Cloudy radiance data assimilation components have been tested in hybrid system. In operational system, βf = 0. 25, βe = 0. 75
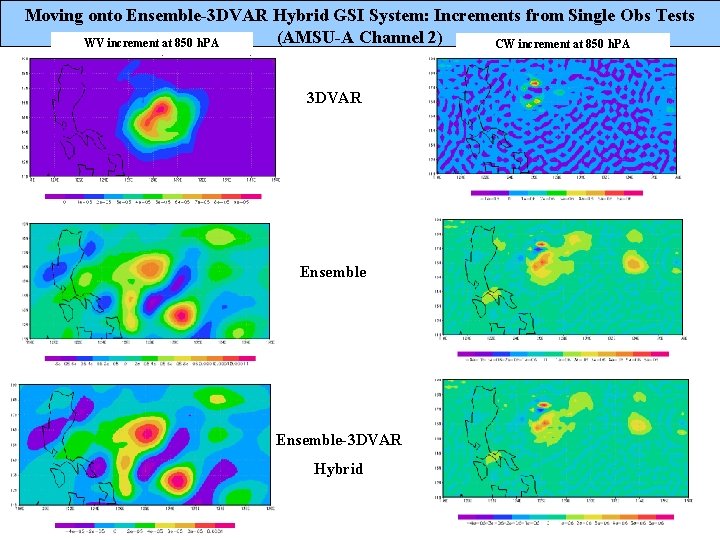
Moving onto Ensemble-3 DVAR Hybrid GSI System: Increments from Single Obs Tests (AMSU-A Channel 2) WV increment at 850 h. PA CW increment at 850 h. PA 3 DVAR Ensemble-3 DVAR Hybrid
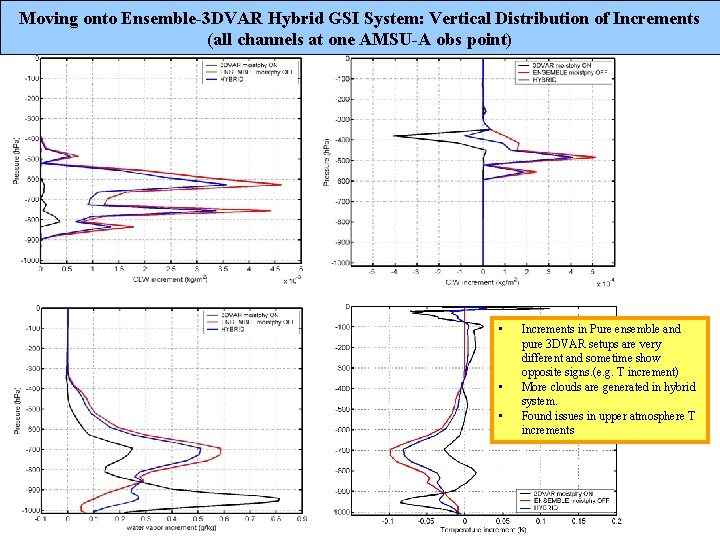
Moving onto Ensemble-3 DVAR Hybrid GSI System: Vertical Distribution of Increments (all channels at one AMSU-A obs point) • • • Increments in Pure ensemble and pure 3 DVAR setups are very different and sometime show opposite signs. (e. g. T increment) More clouds are generated in hybrid system. Found issues in upper atmosphere T increments
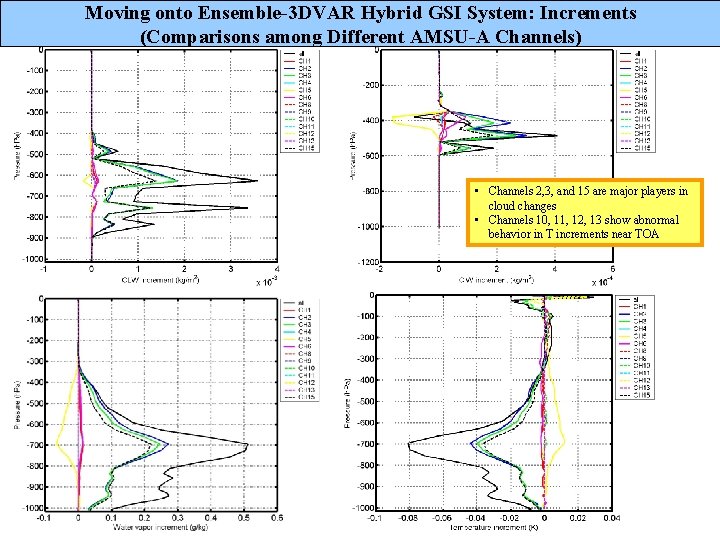
Moving onto Ensemble-3 DVAR Hybrid GSI System: Increments (Comparisons among Different AMSU-A Channels) • Channels 2, 3, and 15 are major players in cloud changes • Channels 10, 11, 12, 13 show abnormal behavior in T increments near TOA
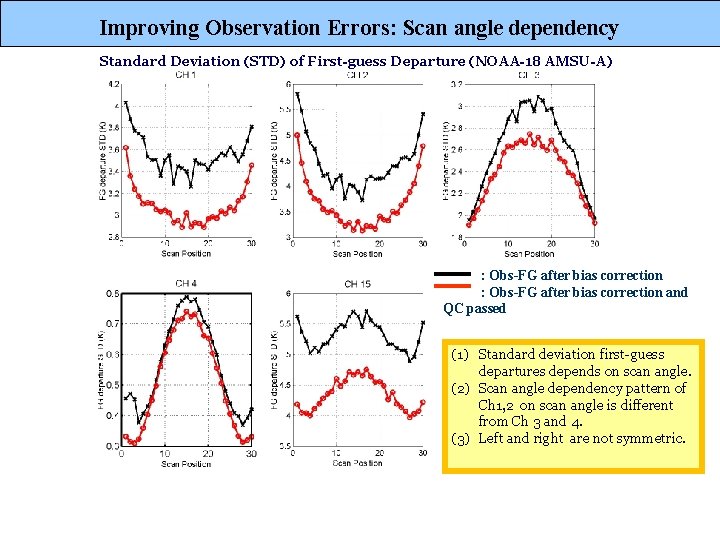
Improving Observation Errors: Scan angle dependency Standard Deviation (STD) of First-guess Departure (NOAA-18 AMSU-A) : Obs-FG after bias correction and QC passed (1) Standard deviation first-guess departures depends on scan angle. (2) Scan angle dependency pattern of Ch 1, 2 on scan angle is different from Ch 3 and 4. (3) Left and right are not symmetric.
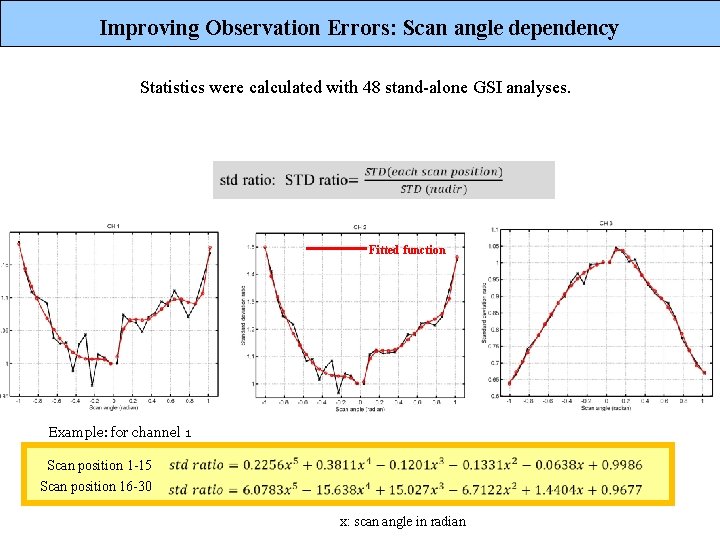
Improving Observation Errors: Scan angle dependency Statistics were calculated with 48 stand-alone GSI analyses. Fitted function Example: for channel 1 Scan position 1 -15 Scan position 16 -30 x: scan angle in radian
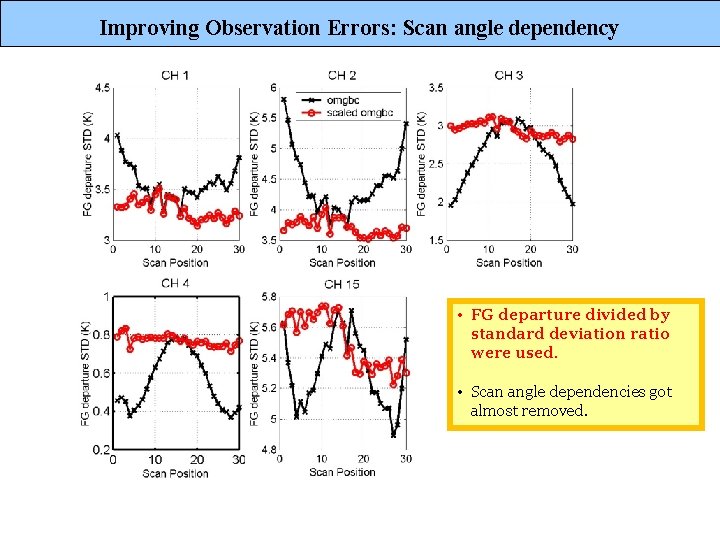
Improving Observation Errors: Scan angle dependency • FG departure divided by standard deviation ratio were used. • Scan angle dependencies got almost removed.
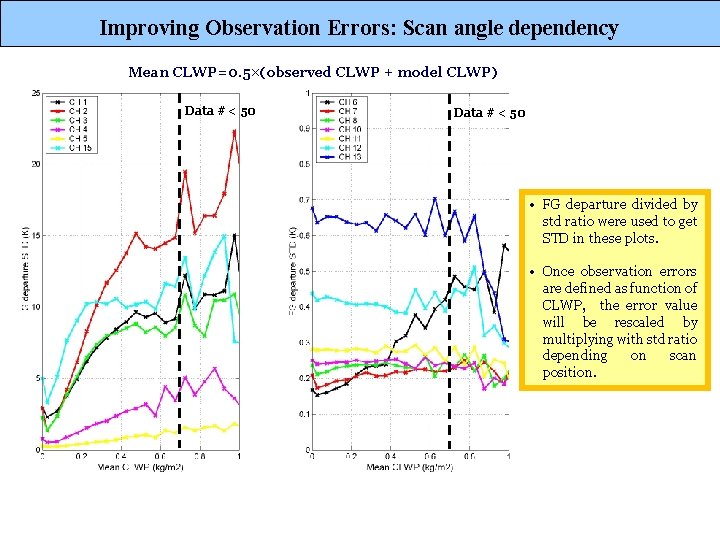
Improving Observation Errors: Scan angle dependency Mean CLWP=0. 5×(observed CLWP + model CLWP) Data # < 50 • FG departure divided by std ratio were used to get STD in these plots. • Once observation errors are defined as function of CLWP, the error value will be rescaled by multiplying with std ratio depending on scan position.
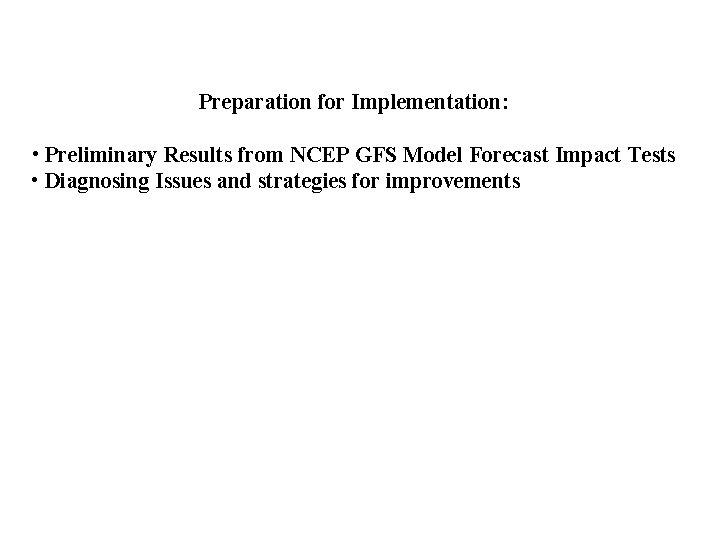
Preparation for Implementation: • Preliminary Results from NCEP GFS Model Forecast Impact Tests • Diagnosing Issues and strategies for improvements
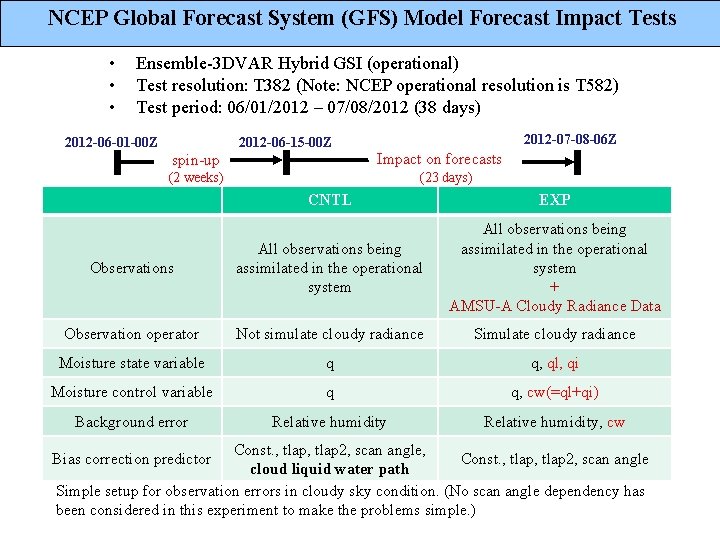
NCEP Global Forecast System (GFS) Model Forecast Impact Tests • • • Ensemble-3 DVAR Hybrid GSI (operational) Test resolution: T 382 (Note: NCEP operational resolution is T 582) Test period: 06/01/2012 – 07/08/2012 (38 days) 2012 -06 -01 -00 Z 2012 -07 -08 -06 Z 2012 -06 -15 -00 Z Impact on forecasts spin-up (23 days) (2 weeks) CNTL EXP Observations All observations being assimilated in the operational system + AMSU-A Cloudy Radiance Data Observation operator Not simulate cloudy radiance Simulate cloudy radiance Moisture state variable q q, ql, qi Moisture control variable q q, cw(=ql+qi) Background error Relative humidity, cw Const. , tlap 2, scan angle, Const. , tlap 2, scan angle cloud liquid water path Simple setup for observation errors in cloudy sky condition. (No scan angle dependency has been considered in this experiment to make the problems simple. ) Bias correction predictor
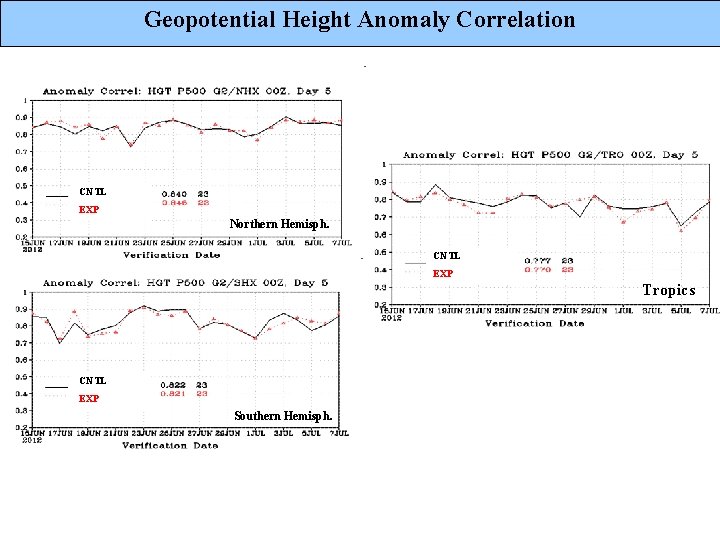
Geopotential Height Anomaly Correlation CNTL EXP Northern Hemisph. CNTL EXP Tropics CNTL EXP Southern Hemisph.
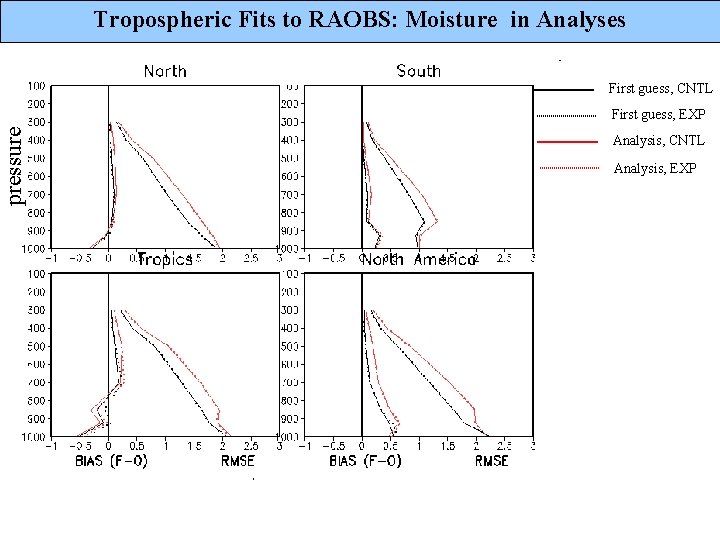
pressure Tropospheric Fits to RAOBS: Moisture in Analyses First guess, CNTL First guess, EXP Analysis, CNTL Analysis, EXP
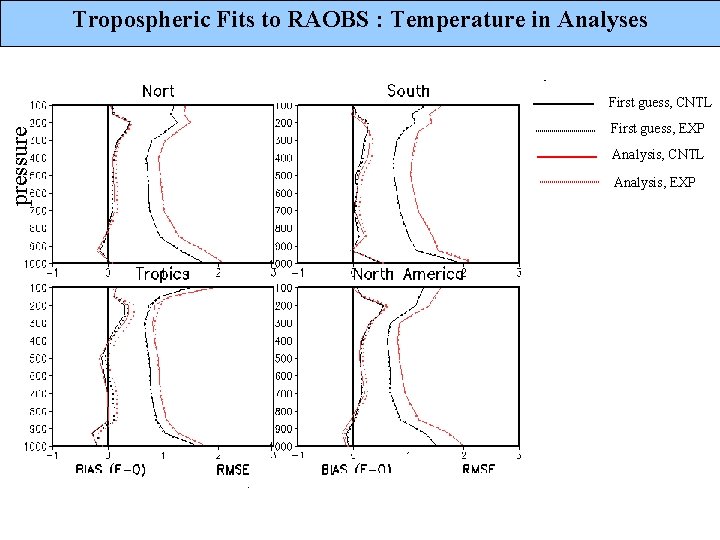
Tropospheric Fits to RAOBS : Temperature in Analyses pressure First guess, CNTL First guess, EXP Analysis, CNTL Analysis, EXP
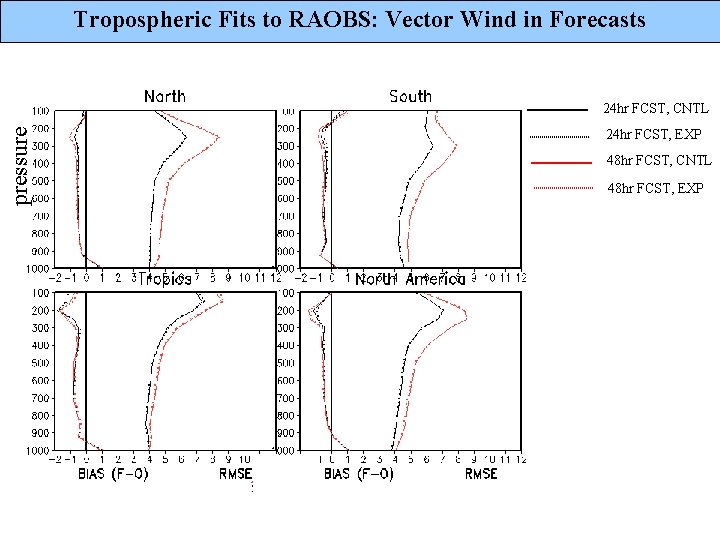
Tropospheric Fits to RAOBS: Vector Wind in Forecasts pressure 24 hr FCST, CNTL 24 hr FCST, EXP 48 hr FCST, CNTL 48 hr FCST, EXP
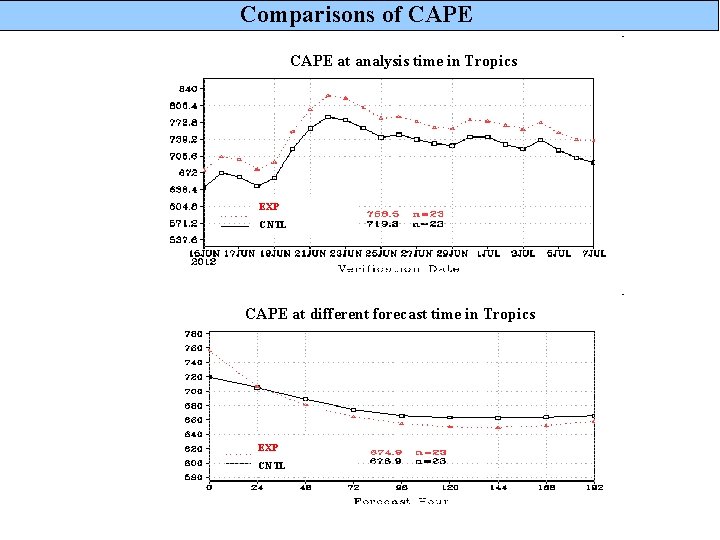
Comparisons of CAPE at analysis time in Tropics EXP CNTL CAPE at different forecast time in Tropics EXP CNTL
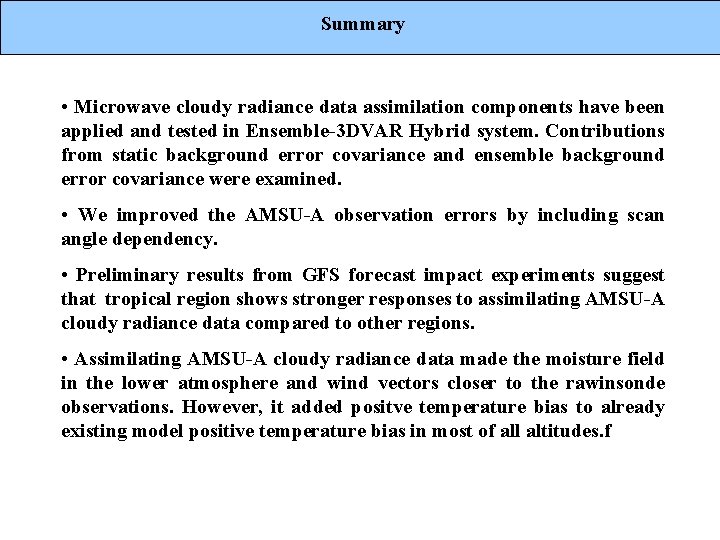
Summary • Microwave cloudy radiance data assimilation components have been applied and tested in Ensemble-3 DVAR Hybrid system. Contributions from static background error covariance and ensemble background error covariance were examined. • We improved the AMSU-A observation errors by including scan angle dependency. • Preliminary results from GFS forecast impact experiments suggest that tropical region shows stronger responses to assimilating AMSU-A cloudy radiance data compared to other regions. • Assimilating AMSU-A cloudy radiance data made the moisture field in the lower atmosphere and wind vectors closer to the rawinsonde observations. However, it added positve temperature bias to already existing model positive temperature bias in most of all altitudes. f
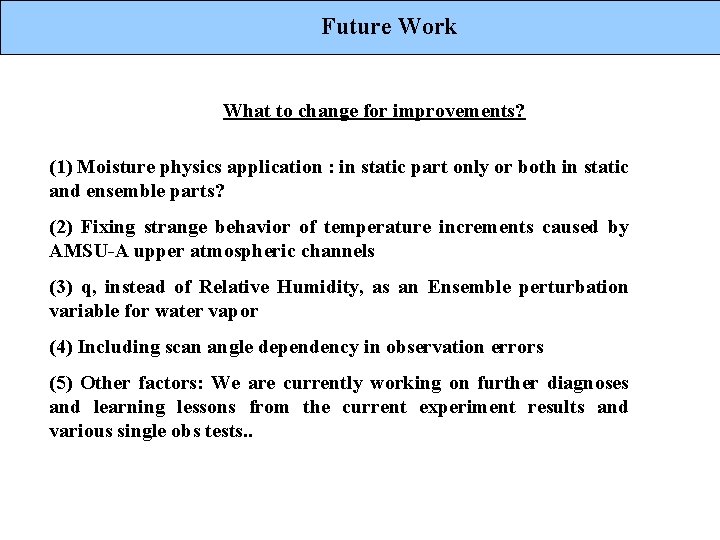
Future Work What to change for improvements? (1) Moisture physics application : in static part only or both in static and ensemble parts? (2) Fixing strange behavior of temperature increments caused by AMSU-A upper atmospheric channels (3) q, instead of Relative Humidity, as an Ensemble perturbation variable for water vapor (4) Including scan angle dependency in observation errors (5) Other factors: We are currently working on further diagnoses and learning lessons from the current experiment results and various single obs tests. .
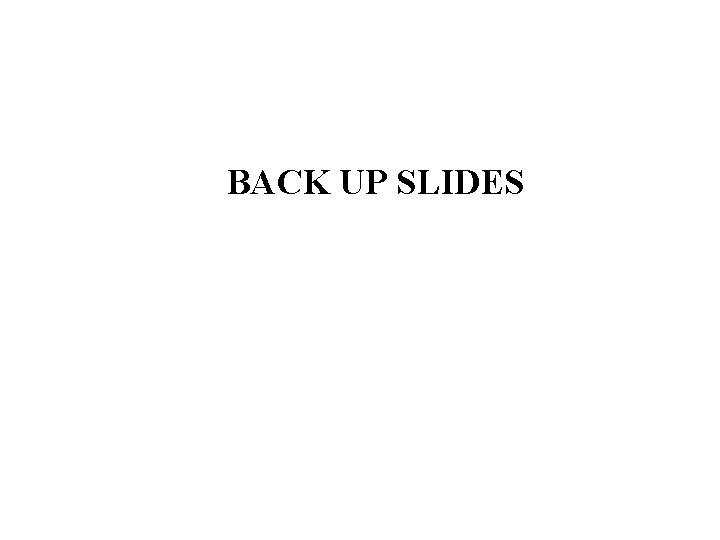
BACK UP SLIDES
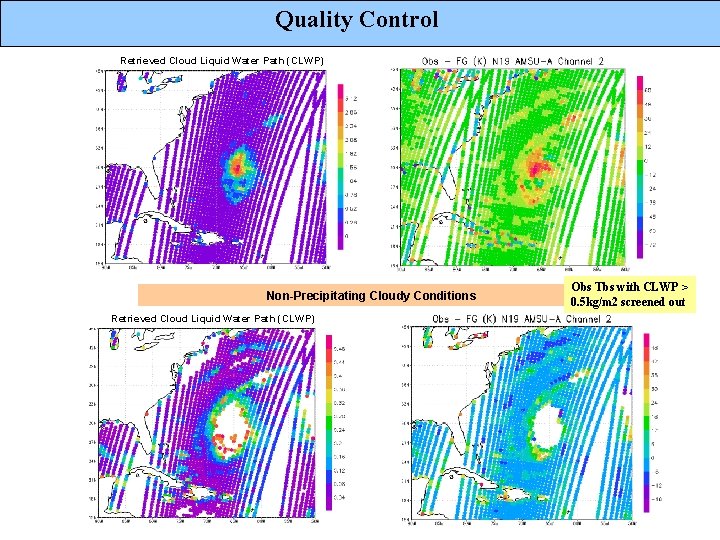
Quality Control Retrieved Cloud Liquid Water Path (CLWP) Non-Precipitating Cloudy Conditions Retrieved Cloud Liquid Water Path (CLWP) Obs Tbs with CLWP > 0. 5 kg/m 2 screened out
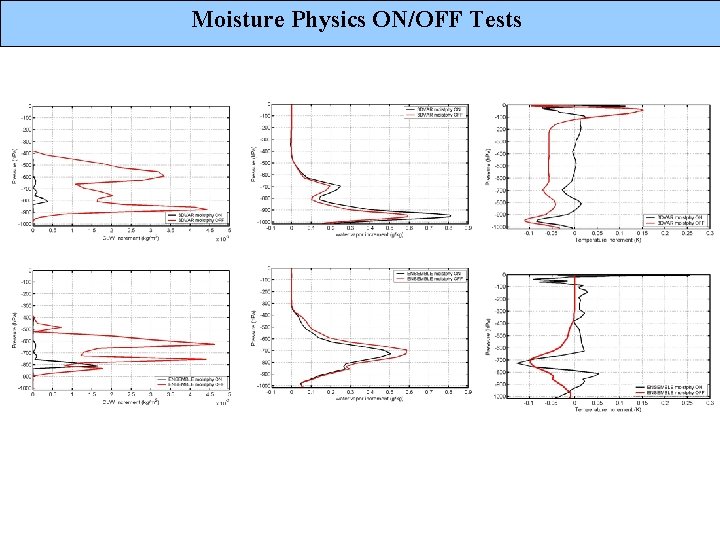
Moisture Physics ON/OFF Tests
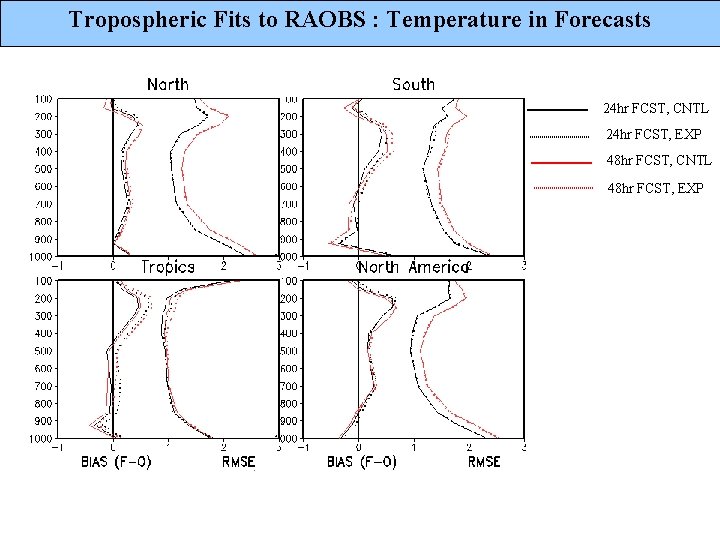
Tropospheric Fits to RAOBS : Temperature in Forecasts 24 hr FCST, CNTL 24 hr FCST, EXP 48 hr FCST, CNTL 48 hr FCST, EXP
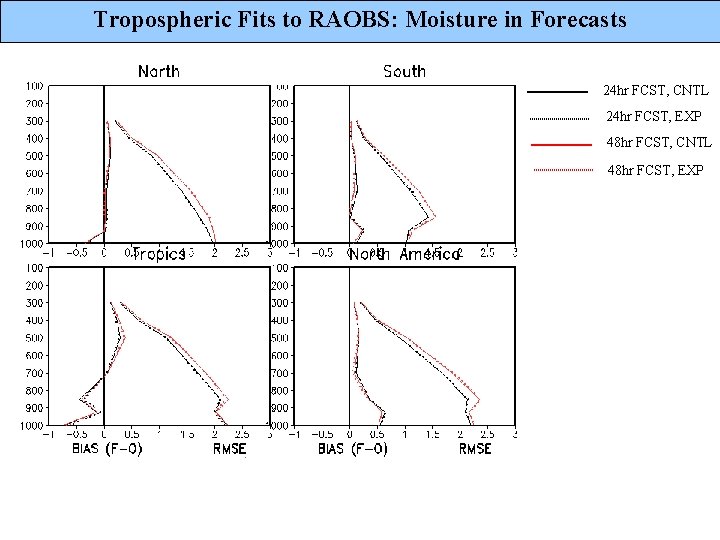
Tropospheric Fits to RAOBS: Moisture in Forecasts 24 hr FCST, CNTL 24 hr FCST, EXP 48 hr FCST, CNTL 48 hr FCST, EXP
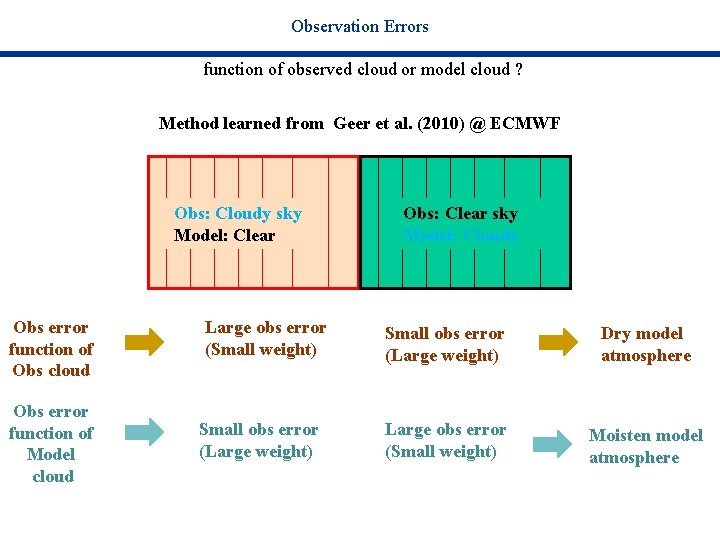
Observation Errors function of observed cloud or model cloud ? Method learned from Geer et al. (2010) @ ECMWF Obs: Cloudy sky Model: Clear Obs error function of Obs cloud Obs error function of Model cloud Large obs error (Small weight) Small obs error (Large weight) Obs: Clear sky Model: Cloudy Small obs error (Large weight) Dry model atmosphere Large obs error (Small weight) Moisten model atmosphere
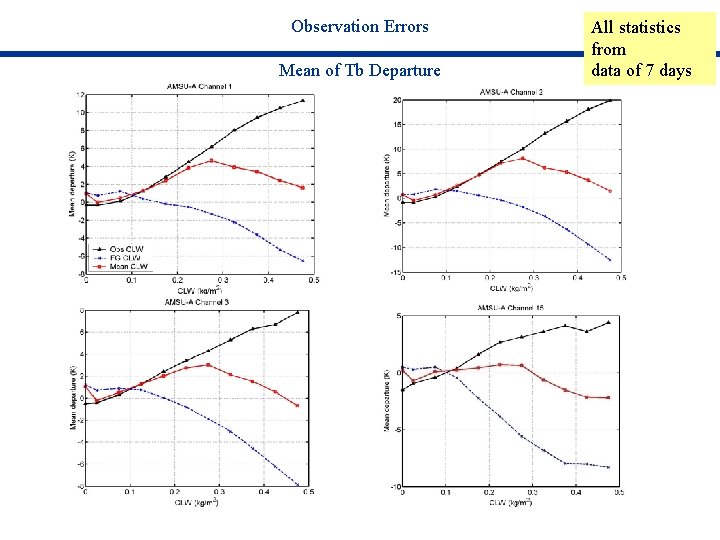
Observation Errors Mean of Tb Departure All statistics from data of 7 days
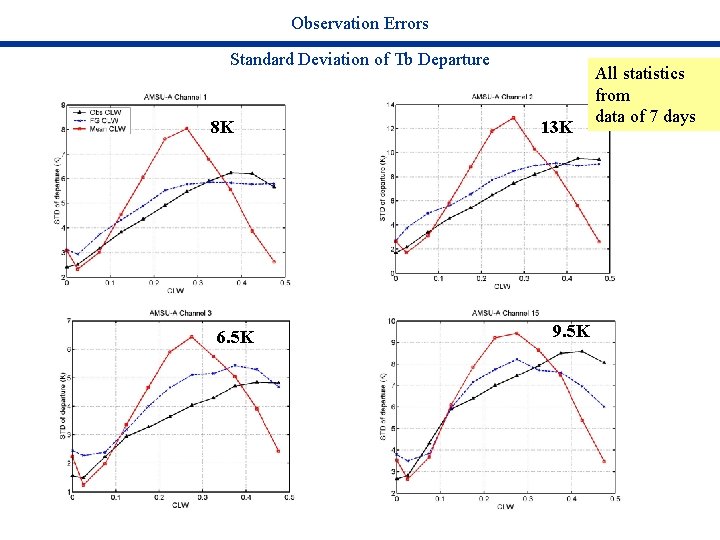
Observation Errors Standard Deviation of Tb Departure 8 K 6. 5 K 13 K 9. 5 K All statistics from data of 7 days
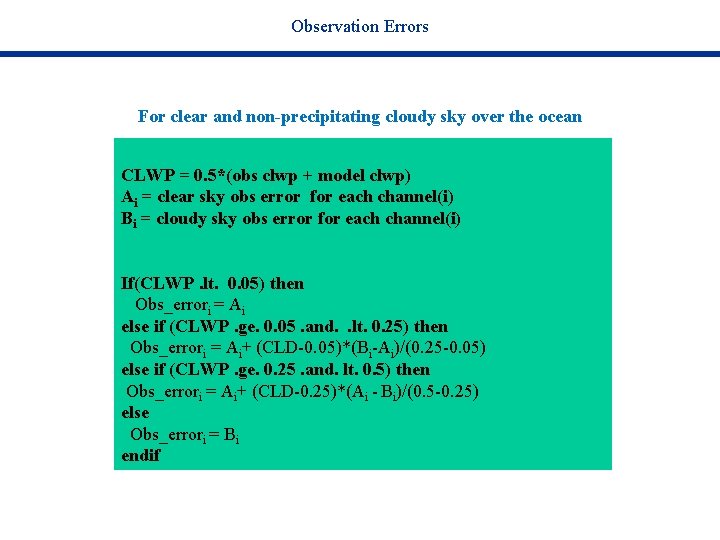
Observation Errors For clear and non-precipitating cloudy sky over the ocean CLWP = 0. 5*(obs clwp + model clwp) Ai = clear sky obs error for each channel(i) Bi = cloudy sky obs error for each channel(i) If(CLWP. lt. 0. 05) then Obs_errori = Ai else if (CLWP. ge. 0. 05. and. . lt. 0. 25) then Obs_errori = Ai+ (CLD-0. 05)*(Bi-Ai)/(0. 25 -0. 05) else if (CLWP. ge. 0. 25. and. lt. 0. 5) then Obs_errori = Ai+ (CLD-0. 25)*(Ai - Bi)/(0. 5 -0. 25) else Obs_errori = Bi endif
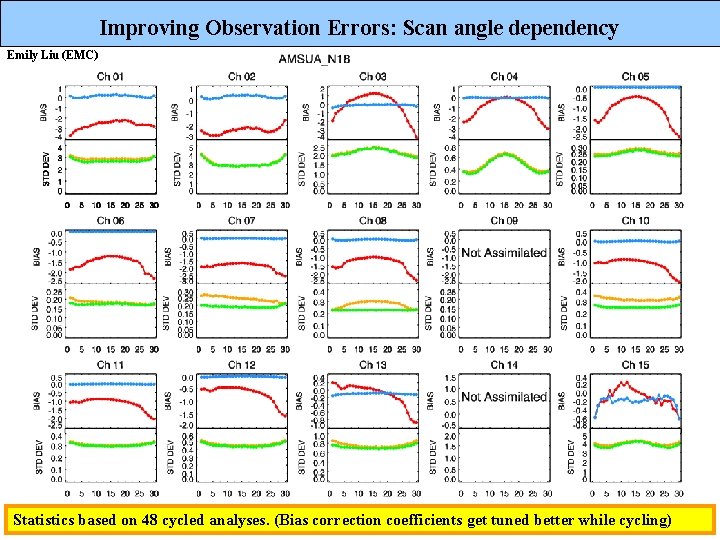
Improving Observation Errors: Scan angle dependency Emily Liu (EMC) Statistics based on 48 cycled analyses. (Bias correction coefficients get tuned better while cycling)
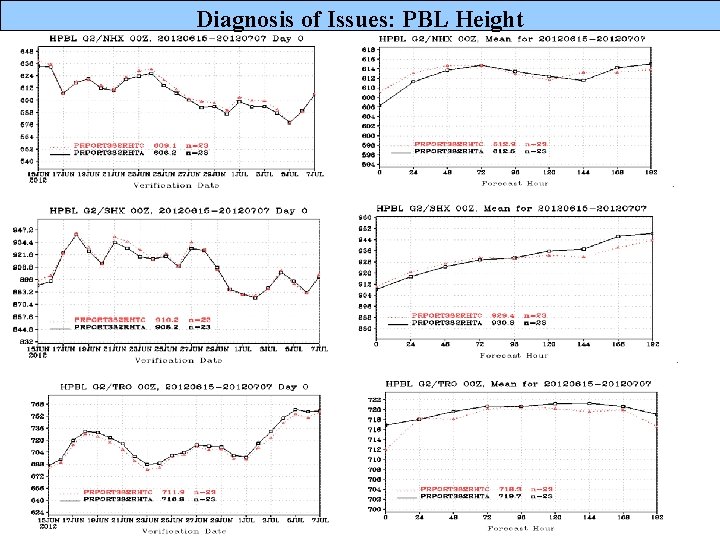
Diagnosis of Issues: PBL Height
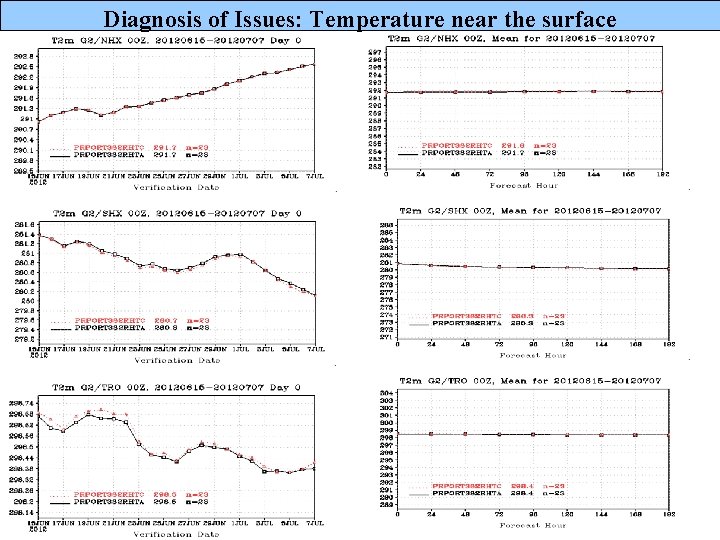
Diagnosis of Issues: Temperature near the surface
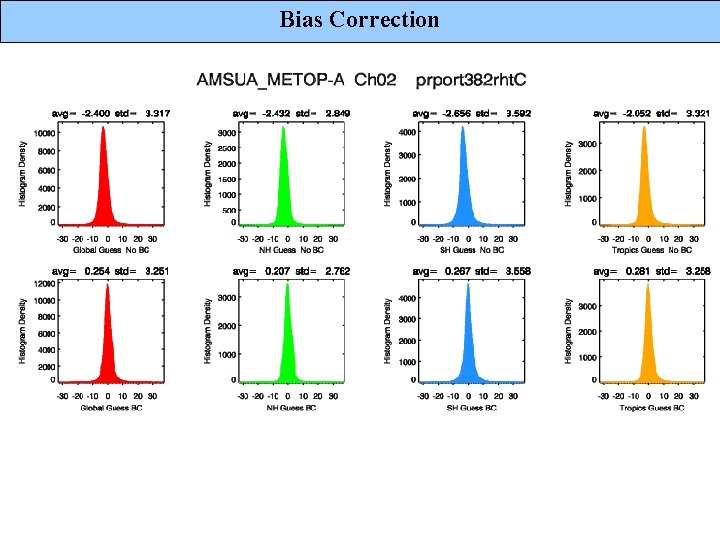
Bias Correction
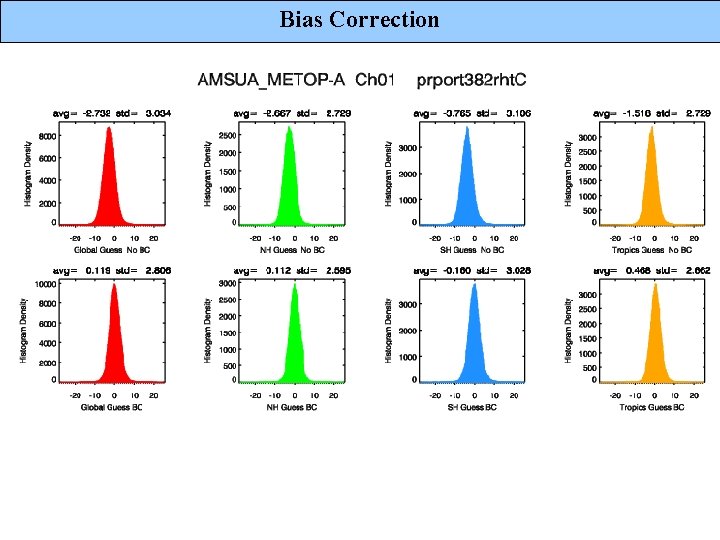
Bias Correction
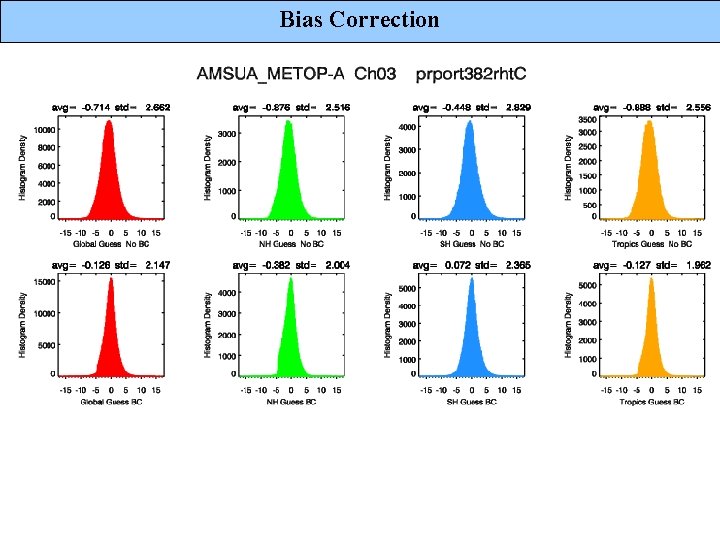
Bias Correction
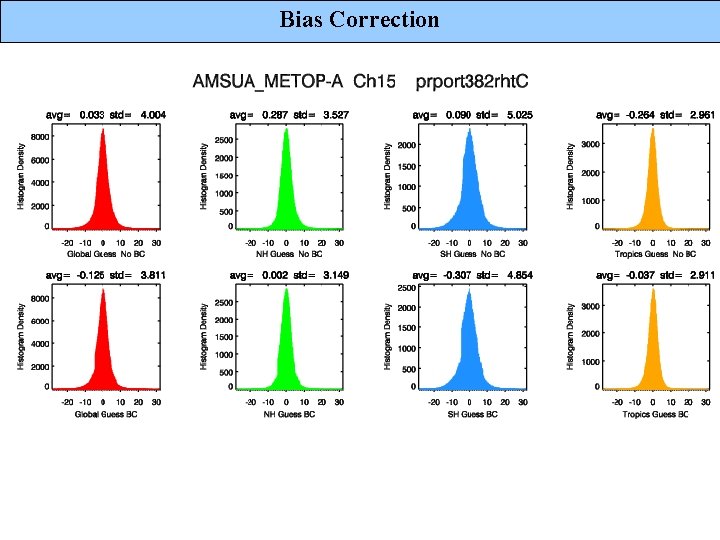
Bias Correction
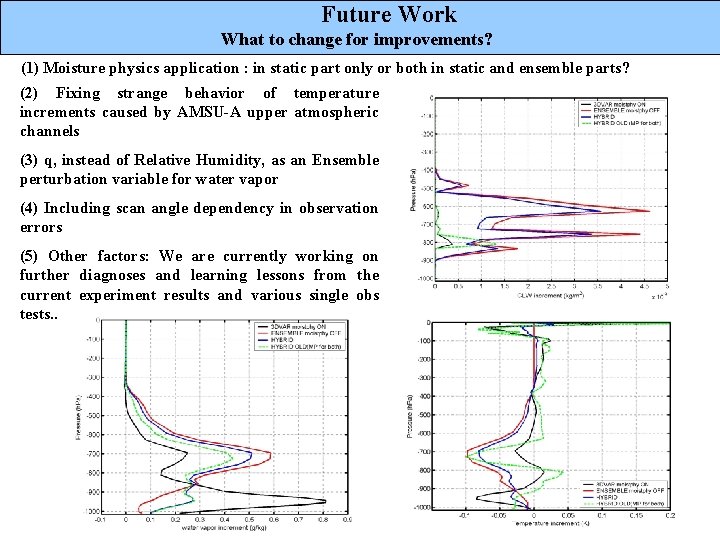
Future Work What to change for improvements? (1) Moisture physics application : in static part only or both in static and ensemble parts? (2) Fixing strange behavior of temperature increments caused by AMSU-A upper atmospheric channels (3) q, instead of Relative Humidity, as an Ensemble perturbation variable for water vapor (4) Including scan angle dependency in observation errors (5) Other factors: We are currently working on further diagnoses and learning lessons from the current experiment results and various single obs tests. .
Difference between status report and progress report
Physical progress and financial progress
Jedi data assimilation
Data assimilation
Data assimilation tutorial
Data assimilation
Data assimilation
Data assimilation
Data assimilation
Data assimilation
Radiance equation
Total radiance
Reflectance vs radiance
Total radiance
Radiance to irradiance conversion
Spherical harmonics lighting
Radiance map
Precomputed radiance transfer
How to report project progress
Ecd progress report
Engineering final year project presentation sample
Yale dissertation progress report
Memorandum to supervisor
Status in progress
Essentials of technical communication
Progress report for internship
Growing success grade chart
Tceq p2 annual progress report
Seo progress report
Progress report example
Crf software
Noun of windy
Image of alliteration
Windy cloudy rainy sunny
Bayes net toolbox for matlab
Grey cloudy discharge
Grey cloudy discharge
A cloudy liquid that contains insoluble particles
Suspension heterogeneous mixture
Urobilinuria causes
Trabeculodysgenesis
Characteristics of normal urine