Assimilation of AIRS Radiance Data within the Rapid
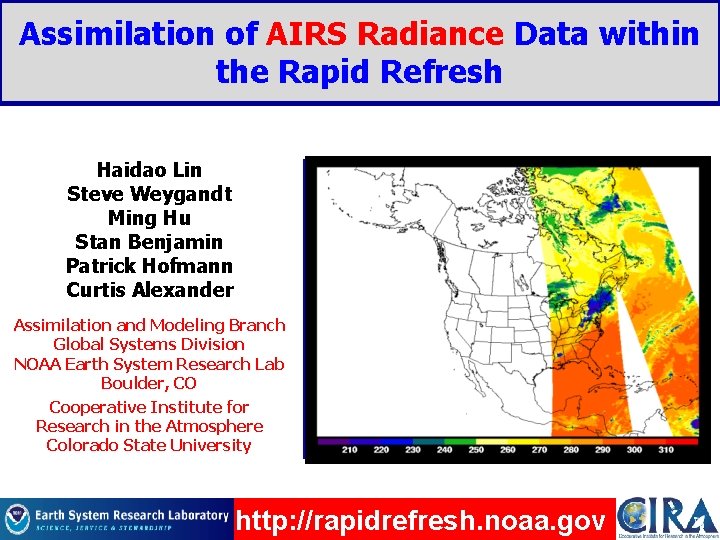
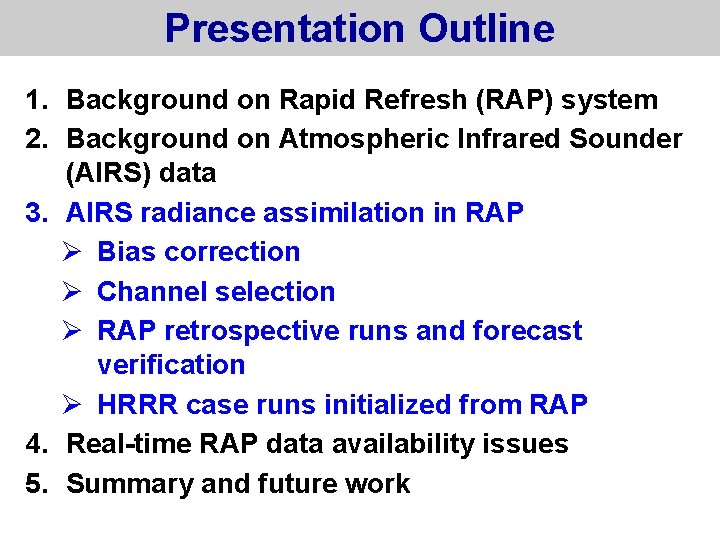
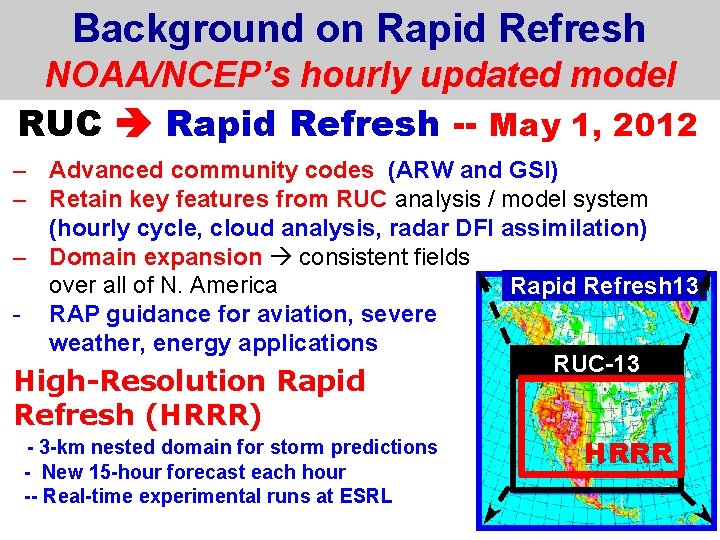
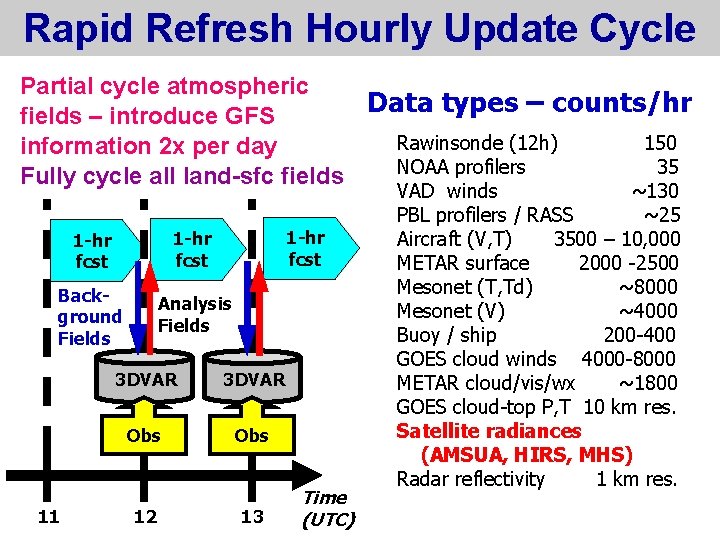
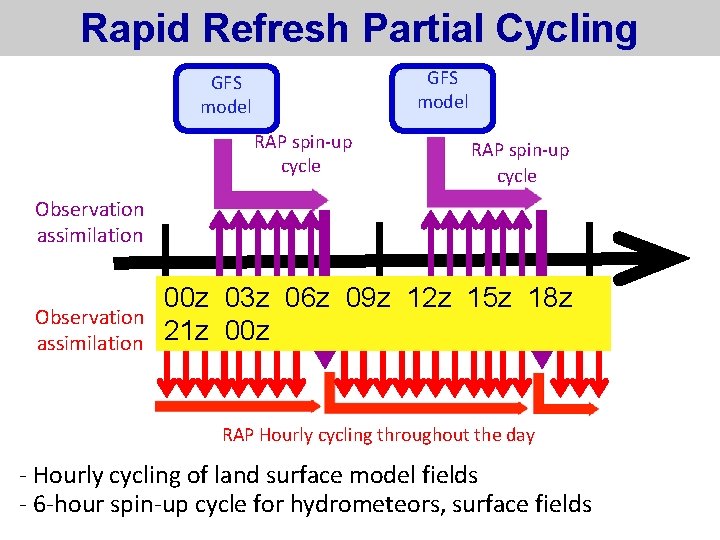
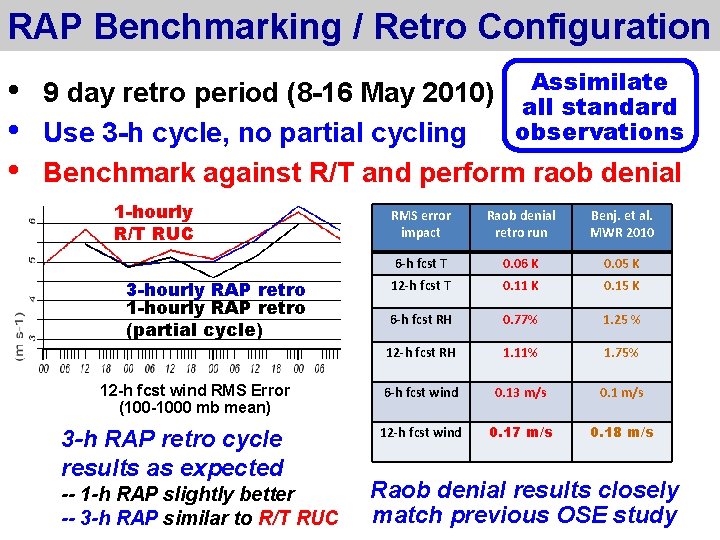
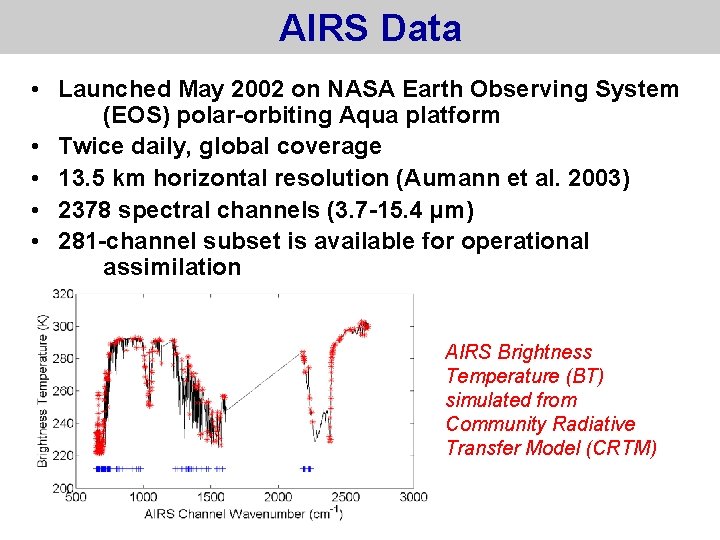
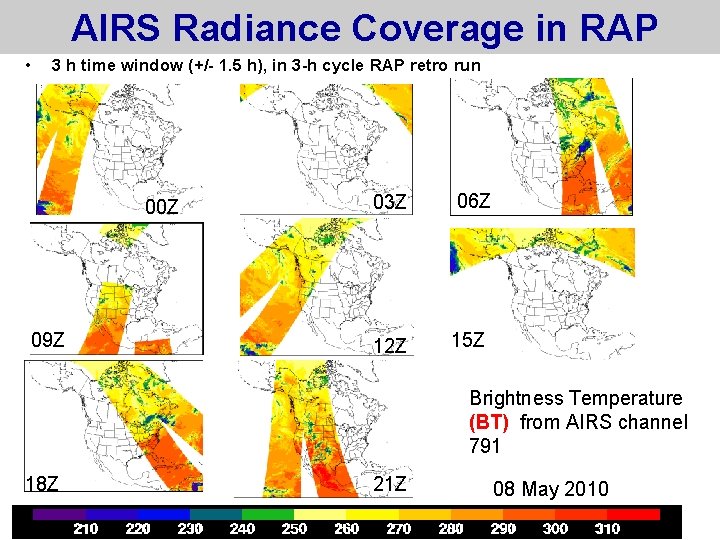
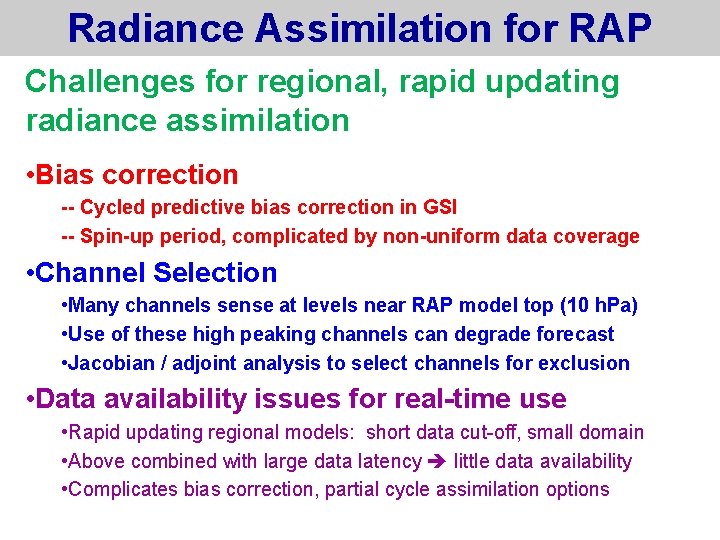
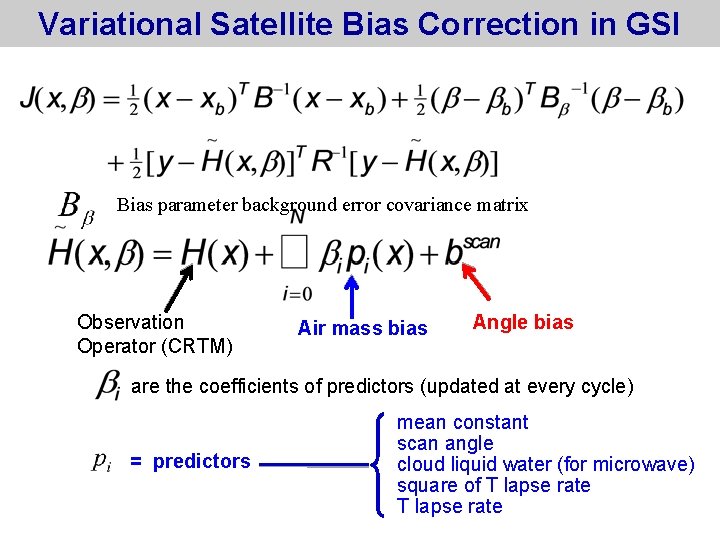
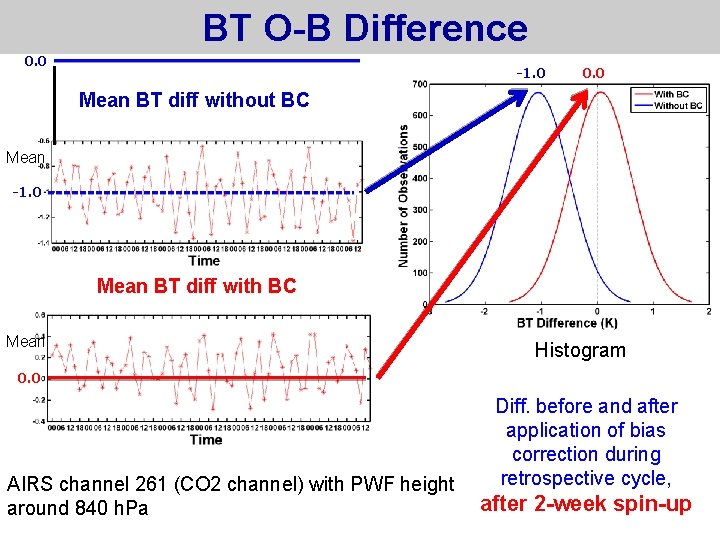
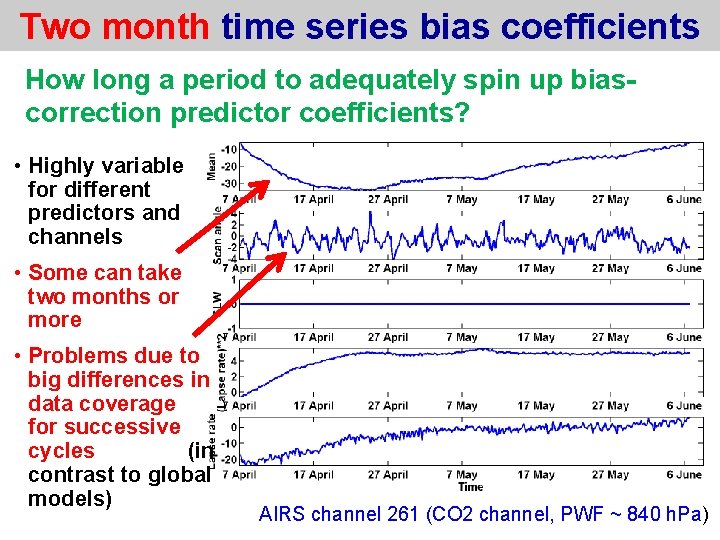
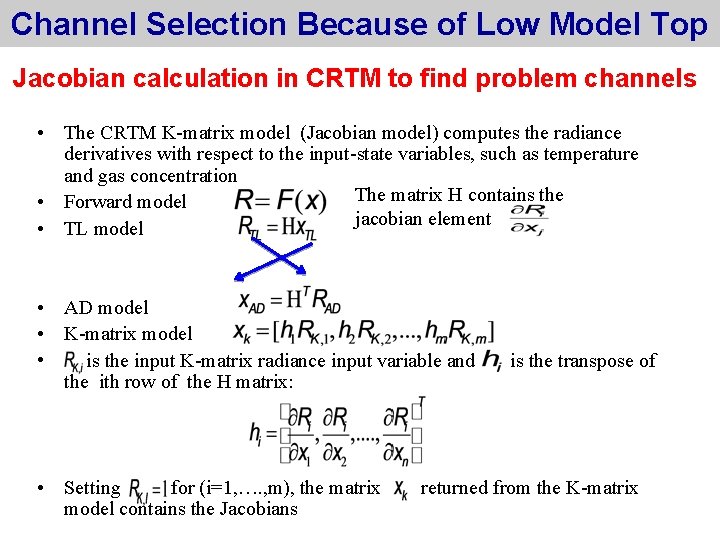
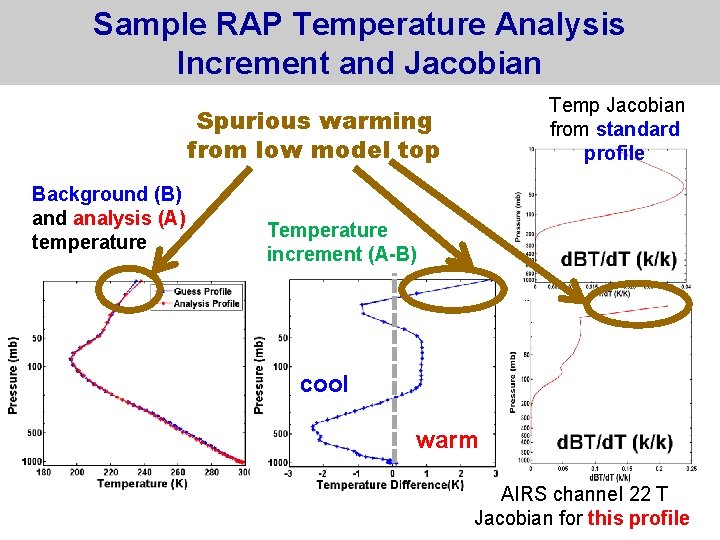
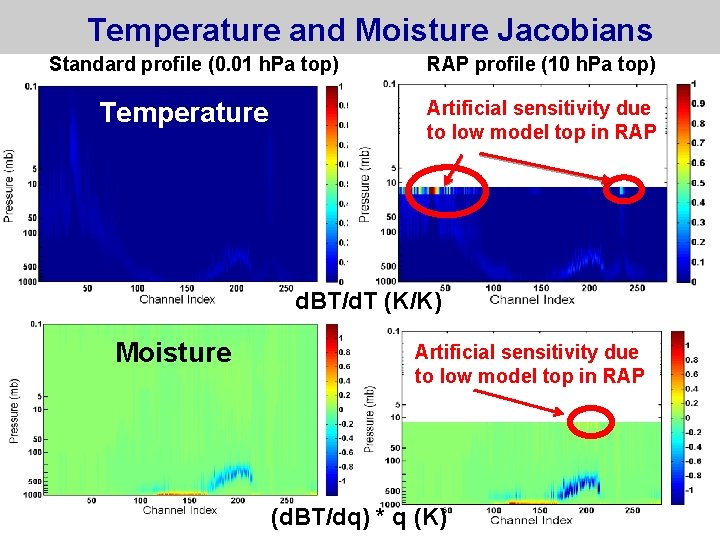
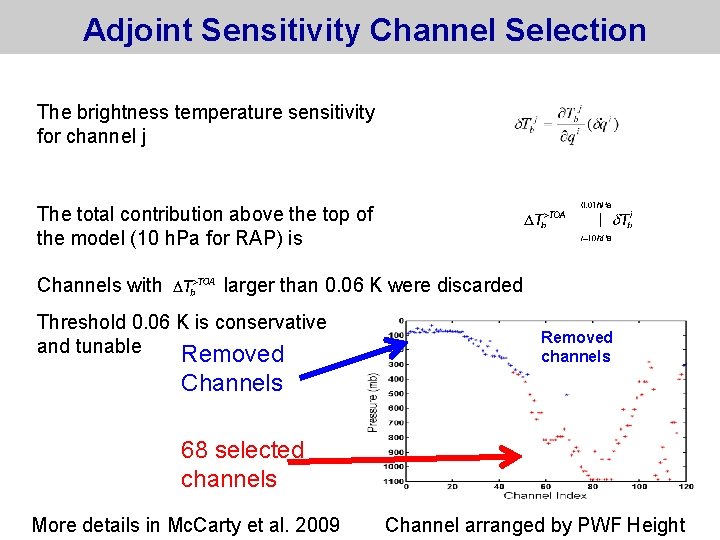
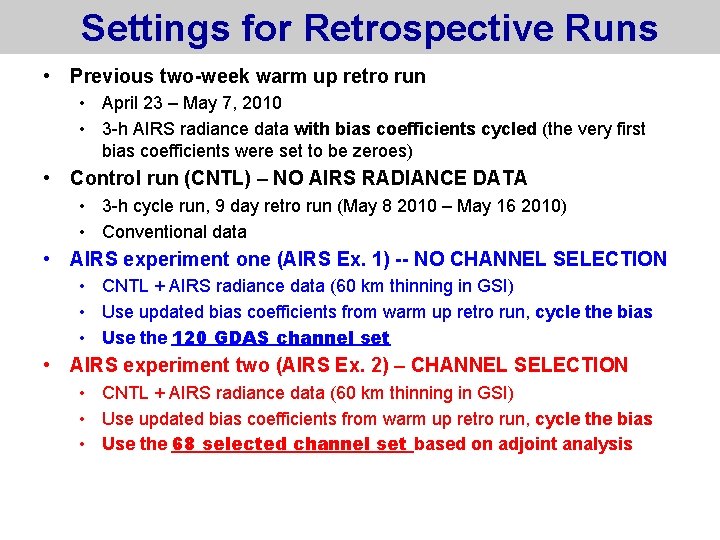
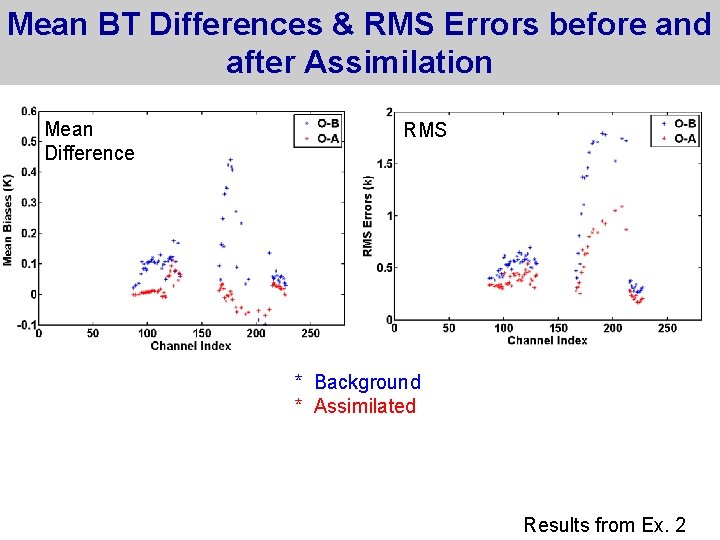
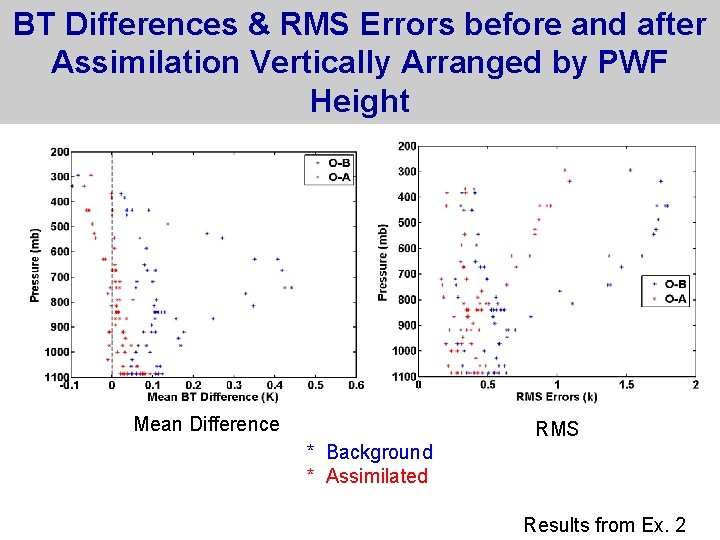
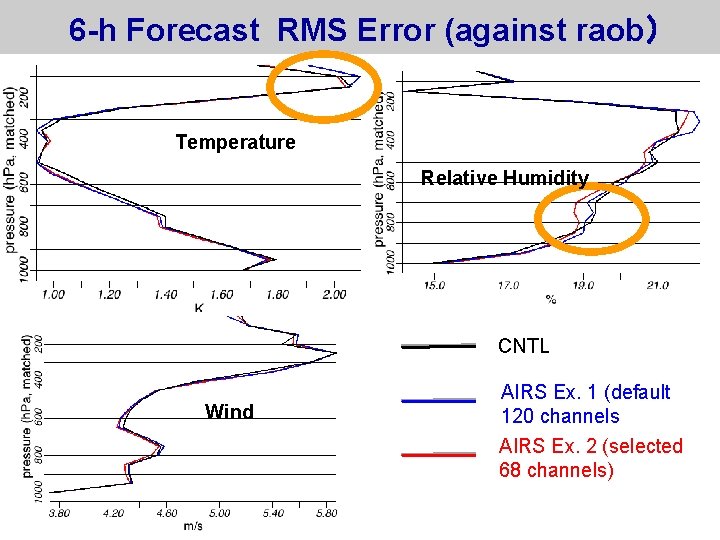
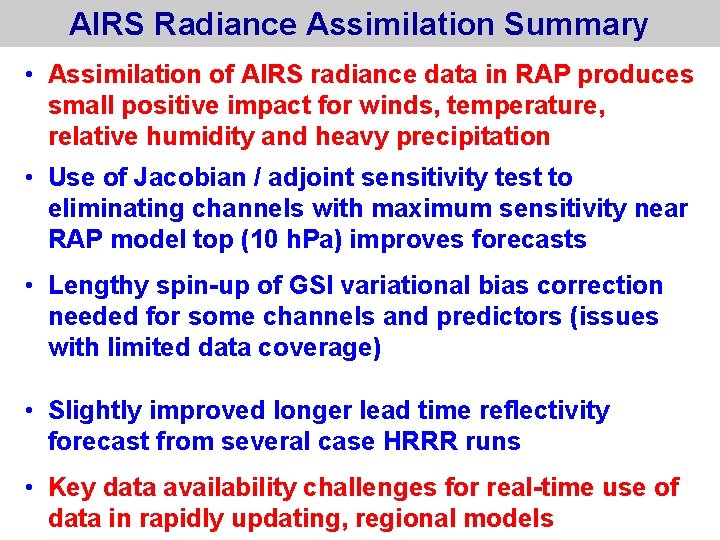
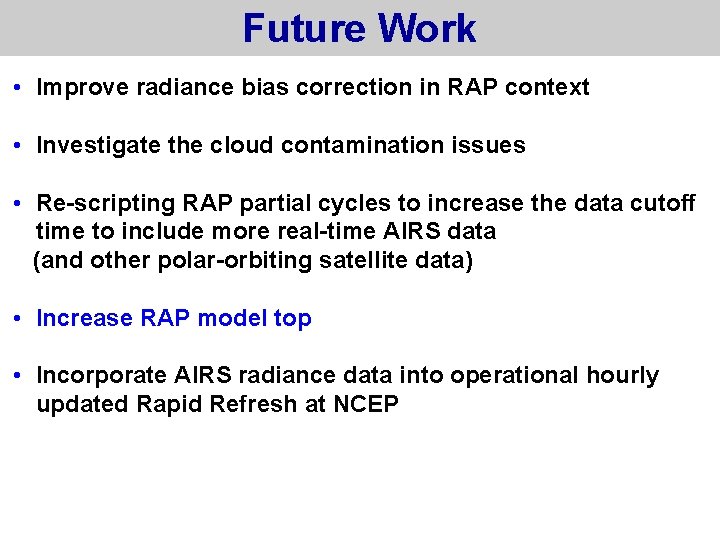
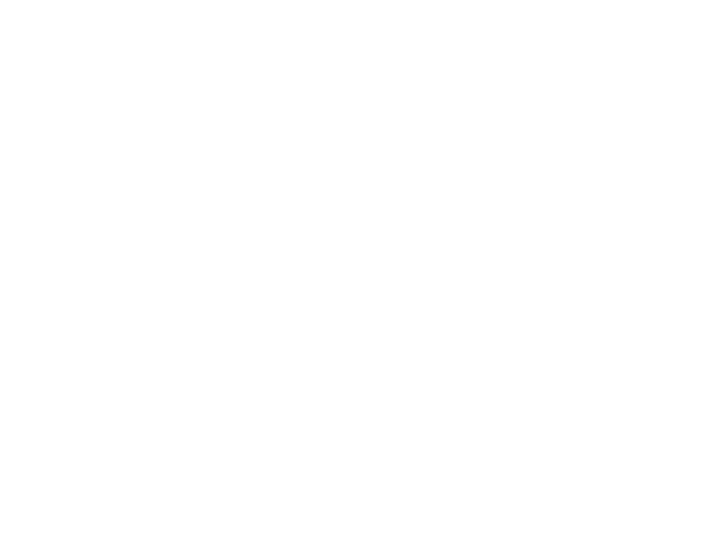
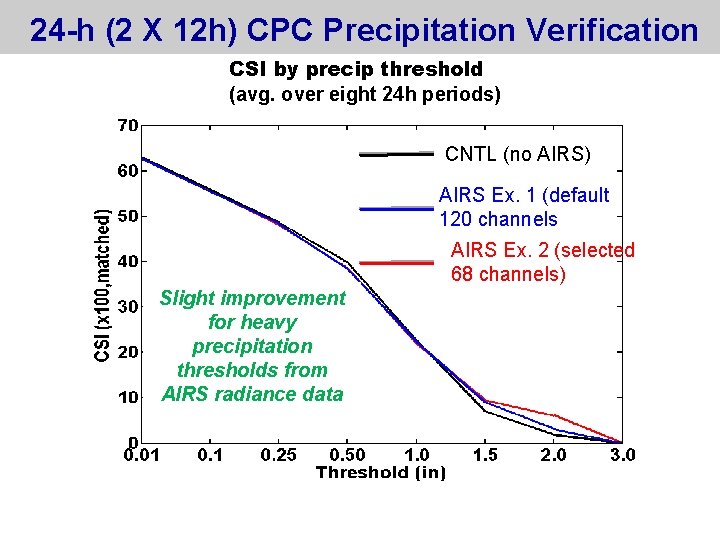
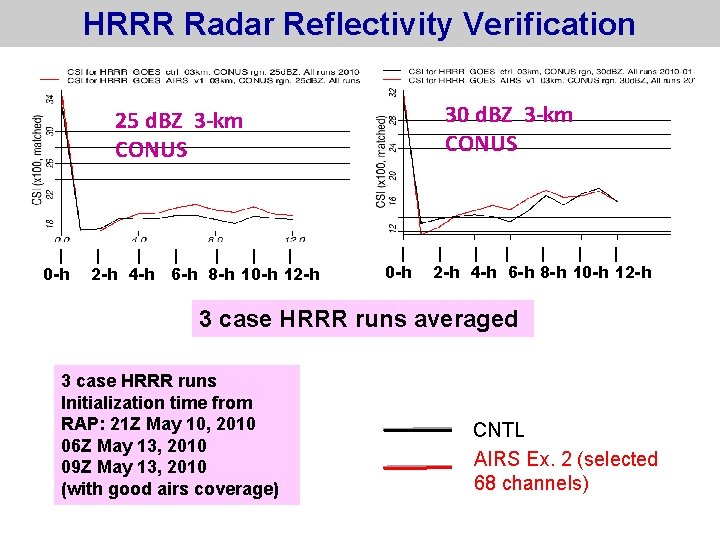
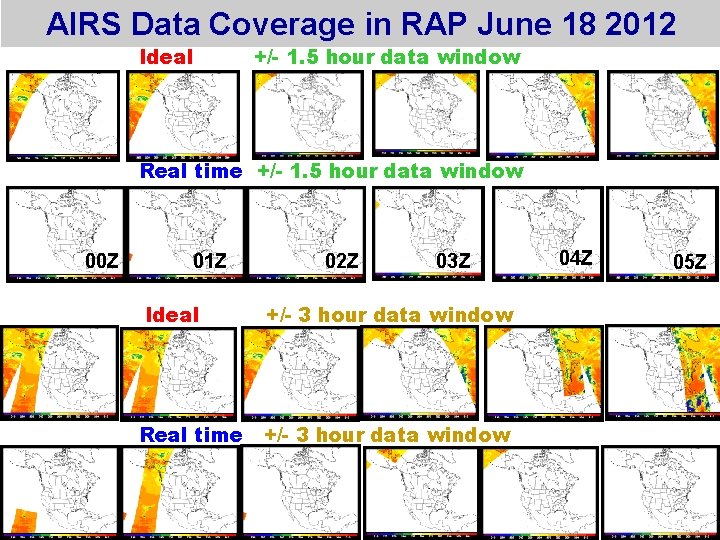
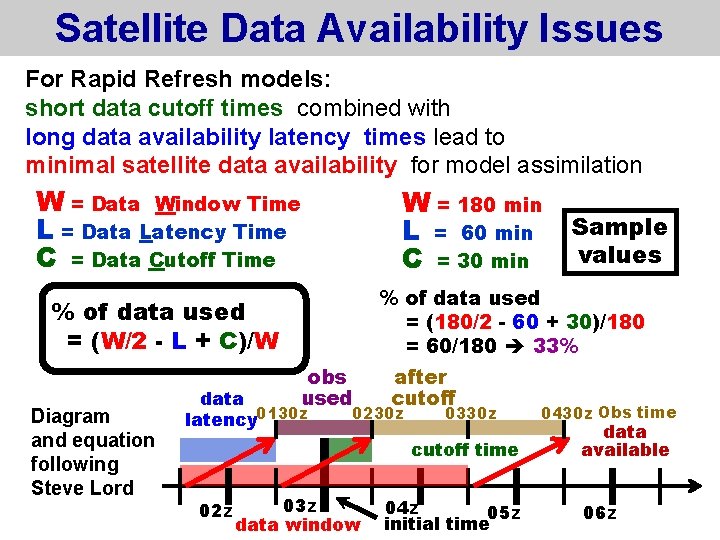
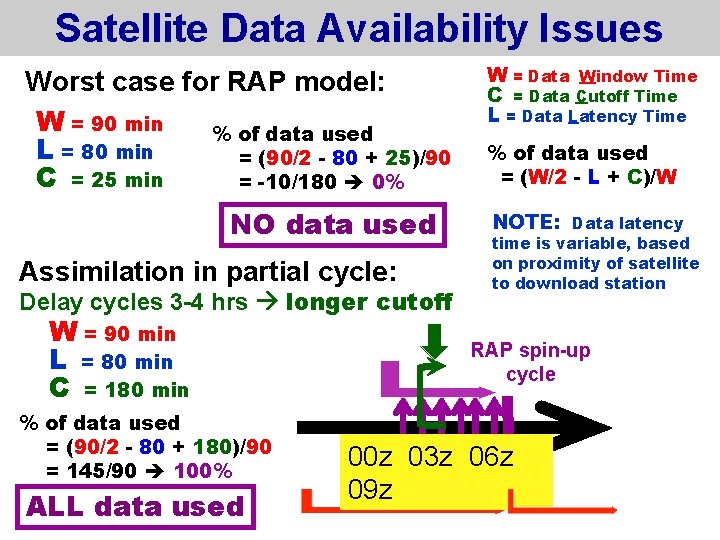
- Slides: 28
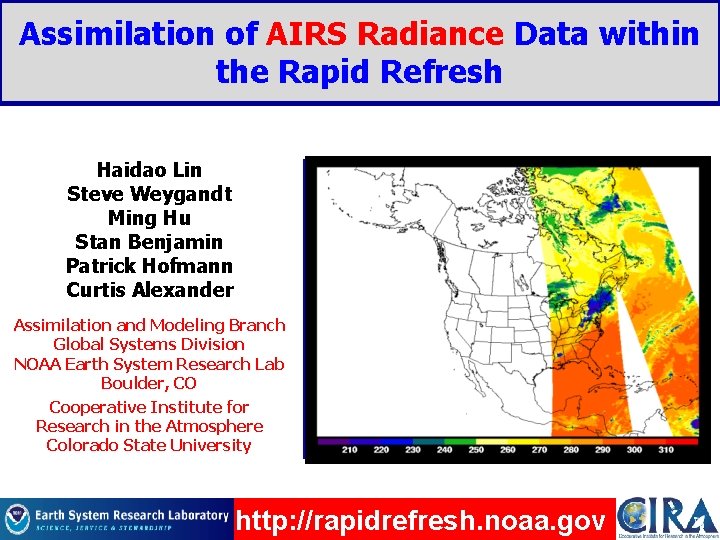
Assimilation of AIRS Radiance Data within the Rapid Refresh Haidao Lin Steve Weygandt Ming Hu Stan Benjamin Patrick Hofmann Curtis Alexander Rapid Refresh domain Assimilation and Modeling Branch Global Systems Division NOAA Earth System Research Lab Boulder, CO Cooperative Institute for Research in the Atmosphere Colorado State University http: //rapidrefresh. noaa. gov
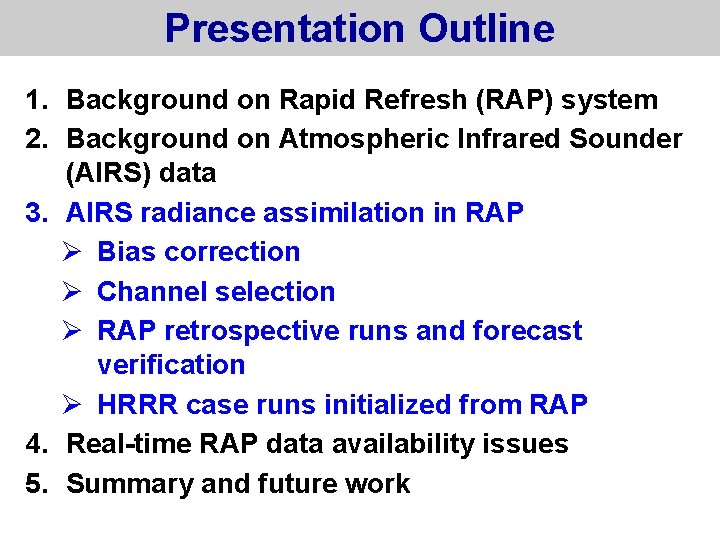
Presentation Outline 1. Background on Rapid Refresh (RAP) system 2. Background on Atmospheric Infrared Sounder (AIRS) data 3. AIRS radiance assimilation in RAP Ø Bias correction Ø Channel selection Ø RAP retrospective runs and forecast verification Ø HRRR case runs initialized from RAP 4. Real-time RAP data availability issues 5. Summary and future work
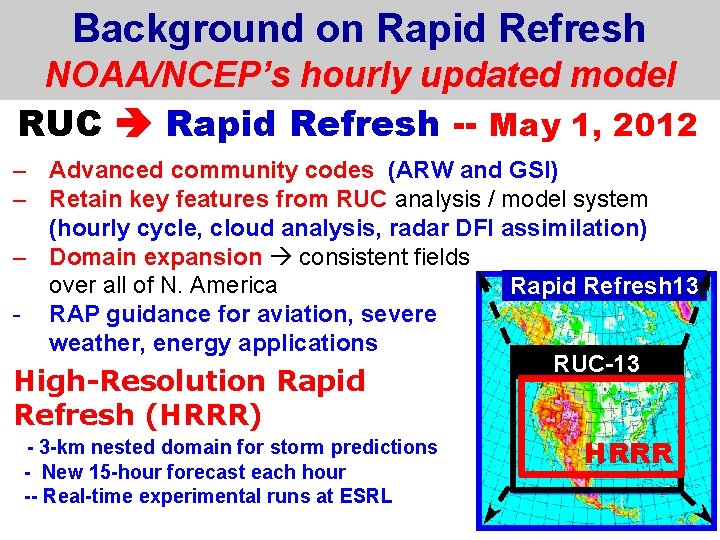
Background on Rapid Refresh NOAA/NCEP’s hourly updated model RUC Rapid Refresh -- May 1, 2012 – Advanced community codes (ARW and GSI) – Retain key features from RUC analysis / model system (hourly cycle, cloud analysis, radar DFI assimilation) – Domain expansion consistent fields over all of N. America Rapid Refresh 13 - RAP guidance for aviation, severe weather, energy applications RUC-13 High-Resolution Rapid Refresh (HRRR) - 3 -km nested domain for storm predictions - New 15 -hour forecast each hour -- Real-time experimental runs at ESRL HRRR
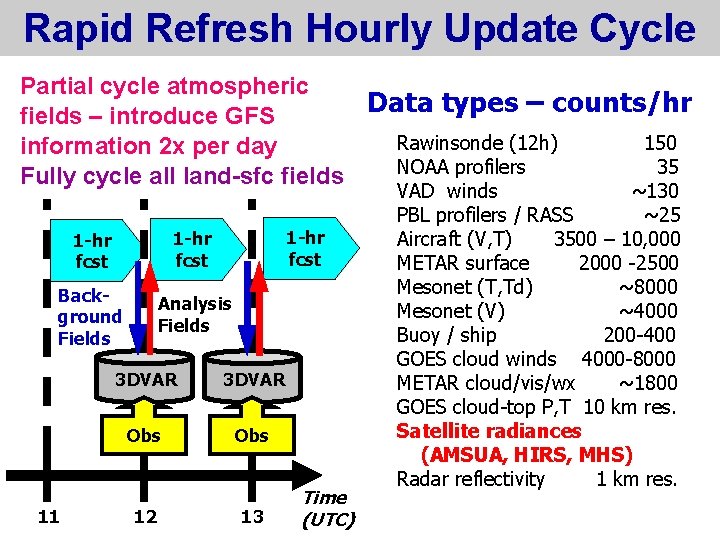
Rapid Refresh Hourly Update Cycle Partial cycle atmospheric Data types – counts/hr fields – introduce GFS Rawinsonde (12 h) 150 information 2 x per day NOAA profilers 35 Fully cycle all land-sfc fields 1 -hr fcst Background Fields Analysis Fields 11 3 DVAR Obs 12 13 Time (UTC) VAD winds ~130 PBL profilers / RASS ~25 Aircraft (V, T) 3500 – 10, 000 METAR surface 2000 -2500 Mesonet (T, Td) ~8000 Mesonet (V) ~4000 Buoy / ship 200 -400 GOES cloud winds 4000 -8000 METAR cloud/vis/wx ~1800 GOES cloud-top P, T 10 km res. Satellite radiances (AMSUA, HIRS, MHS) Radar reflectivity 1 km res.
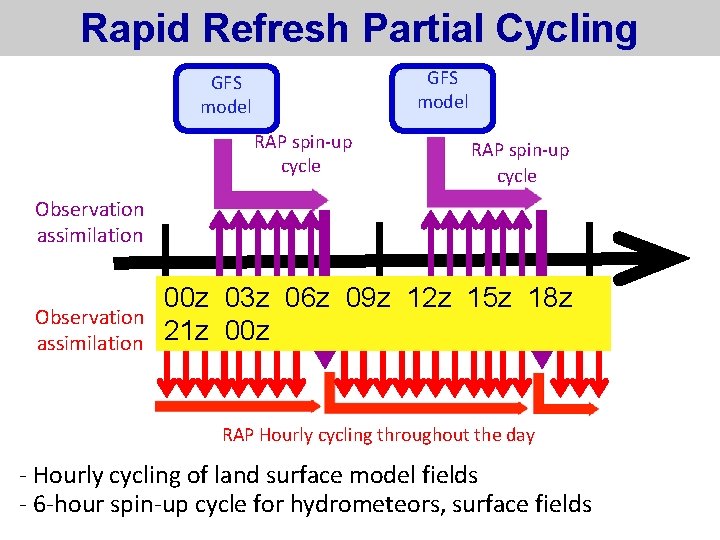
Rapid Refresh Partial Cycling GFS model RAP spin-up cycle Observation assimilation 00 z 03 z 06 z 09 z 12 z 15 z 18 z 21 z 00 z RAP Hourly cycling throughout the day - Hourly cycling of land surface model fields - 6 -hour spin-up cycle for hydrometeors, surface fields
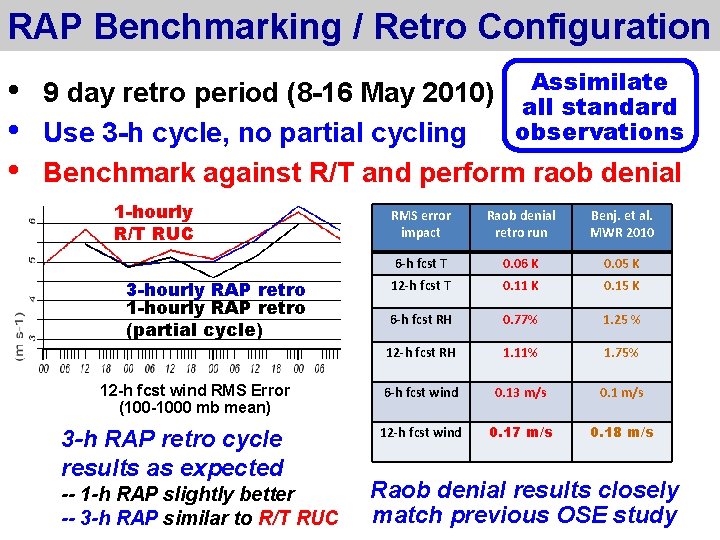
RAP Benchmarking / Retro Configuration • • • Assimilate 9 day retro period (8 -16 May 2010) all standard observations Use 3 -h cycle, no partial cycling Benchmark against R/T and perform raob denial 1 -hourly R/T RUC 3 -hourly RAP retro 1 -hourly RAP retro (partial cycle) 12 -h fcst wind RMS Error (100 -1000 mb mean) 3 -h RAP retro cycle results as expected -- 1 -h RAP slightly better -- 3 -h RAP similar to R/T RUC RMS error impact Raob denial retro run Benj. et al. MWR 2010 6 -h fcst T 0. 06 K 0. 05 K 12 -h fcst T 0. 11 K 0. 15 K 6 -h fcst RH 0. 77% 1. 25 % 12 -h fcst RH 1. 11% 1. 75% 6 -h fcst wind 0. 13 m/s 0. 1 m/s 12 -h fcst wind 0. 17 m/s 0. 18 m/s Raob denial results closely match previous OSE study
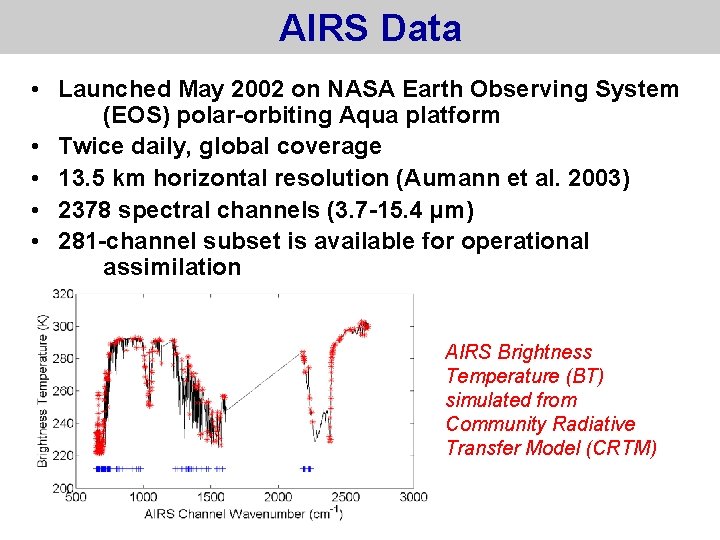
AIRS Data • Launched May 2002 on NASA Earth Observing System (EOS) polar-orbiting Aqua platform • Twice daily, global coverage • 13. 5 km horizontal resolution (Aumann et al. 2003) • 2378 spectral channels (3. 7 -15. 4 µm) • 281 -channel subset is available for operational assimilation AIRS Brightness Temperature (BT) simulated from Community Radiative Transfer Model (CRTM)
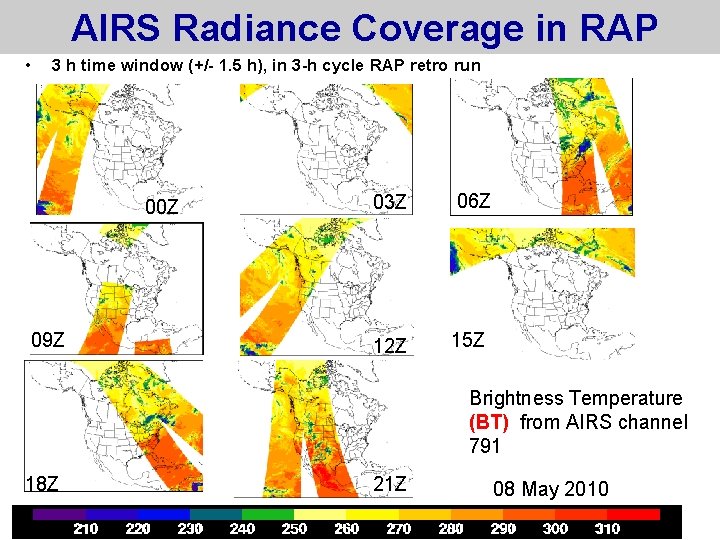
AIRS Radiance Coverage in RAP • 3 h time window (+/- 1. 5 h), in 3 -h cycle RAP retro run 00 Z 09 Z 03 Z 06 Z 12 Z 15 Z Brightness Temperature (BT) from AIRS channel 791 18 Z 21 Z 08 May 2010
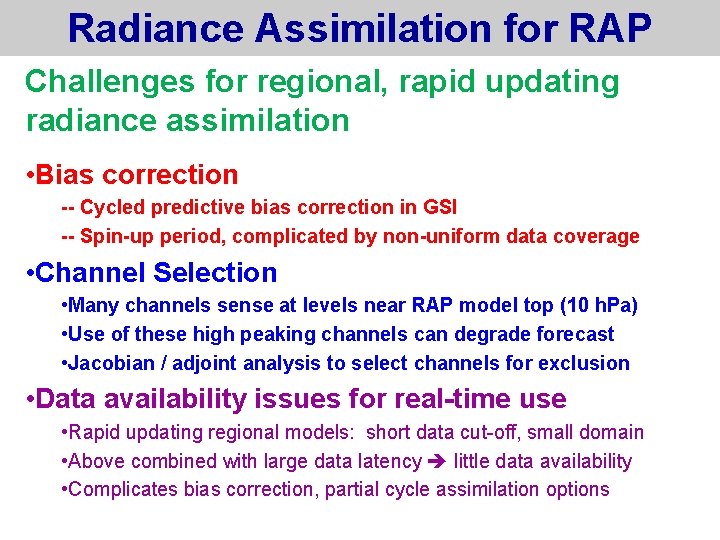
Radiance Assimilation for RAP Challenges for regional, rapid updating radiance assimilation • Bias correction -- Cycled predictive bias correction in GSI -- Spin-up period, complicated by non-uniform data coverage • Channel Selection • Many channels sense at levels near RAP model top (10 h. Pa) • Use of these high peaking channels can degrade forecast • Jacobian / adjoint analysis to select channels for exclusion • Data availability issues for real-time use • Rapid updating regional models: short data cut-off, small domain • Above combined with large data latency little data availability • Complicates bias correction, partial cycle assimilation options
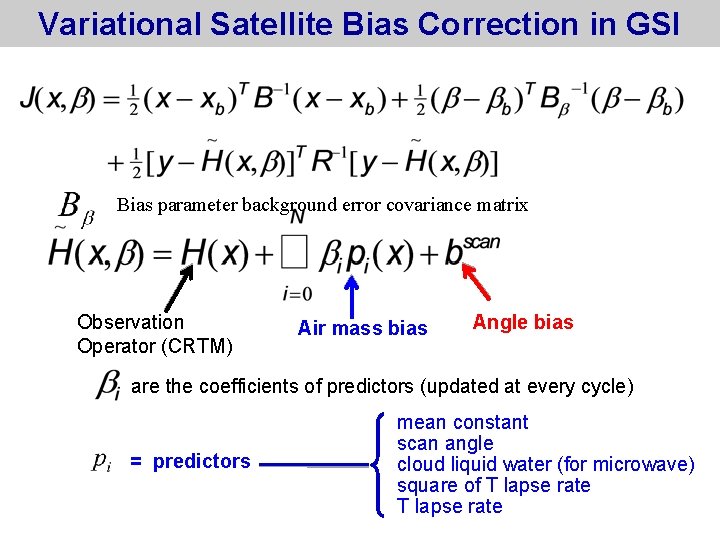
Variational Satellite Bias Correction in GSI Bias parameter background error covariance matrix Observation Operator (CRTM) Air mass bias Angle bias are the coefficients of predictors (updated at every cycle) = predictors mean constant scan angle cloud liquid water (for microwave) square of T lapse rate
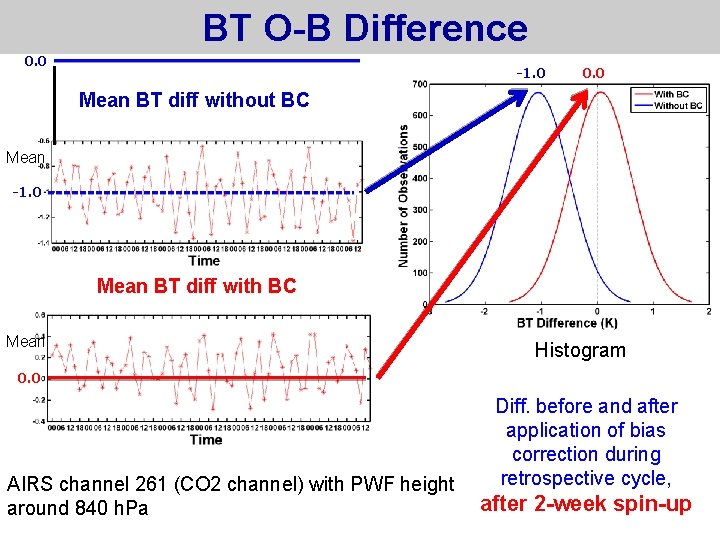
BT O-B Difference 0. 0 -1. 0 0. 0 Mean BT diff without BC Mean -1. 0 Mean BT diff with BC Mean Histogram 0. 0 AIRS channel 261 (CO 2 channel) with PWF height around 840 h. Pa Diff. before and after application of bias correction during retrospective cycle, after 2 -week spin-up
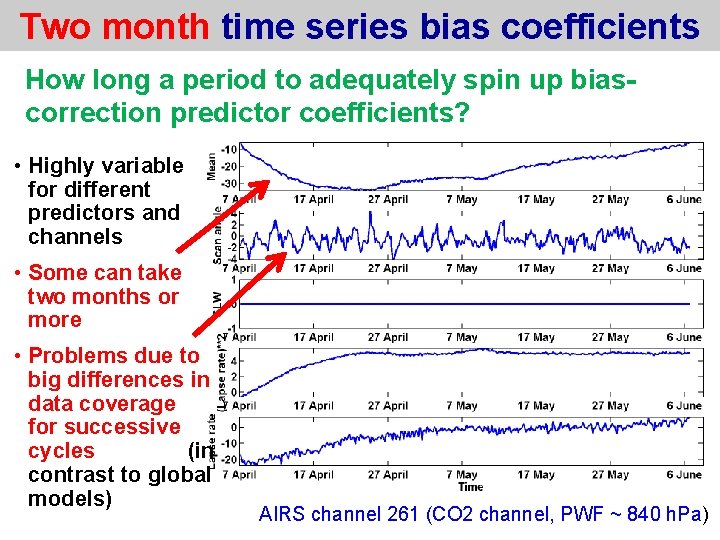
Two month time series bias coefficients How long a period to adequately spin up biascorrection predictor coefficients? • Highly variable for different predictors and channels • Some can take two months or more • Problems due to big differences in data coverage for successive cycles (in contrast to global models) AIRS channel 261 (CO 2 channel, PWF ~ 840 h. Pa)
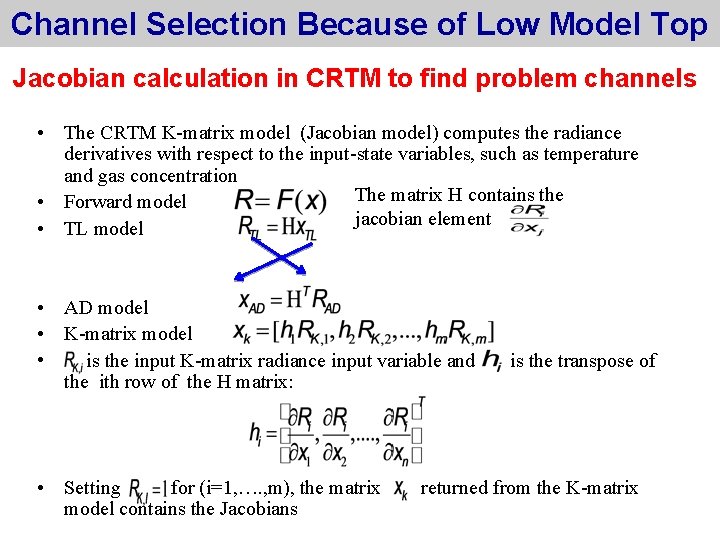
Channel Selection Because of Low Model Top Jacobian calculation in CRTM to find problem channels • The CRTM K-matrix model (Jacobian model) computes the radiance derivatives with respect to the input-state variables, such as temperature and gas concentration The matrix H contains the • Forward model jacobian element • TL model • AD model • K-matrix model • is the input K-matrix radiance input variable and the ith row of the H matrix: • Setting for (i=1, …. , m), the matrix model contains the Jacobians is the transpose of returned from the K-matrix
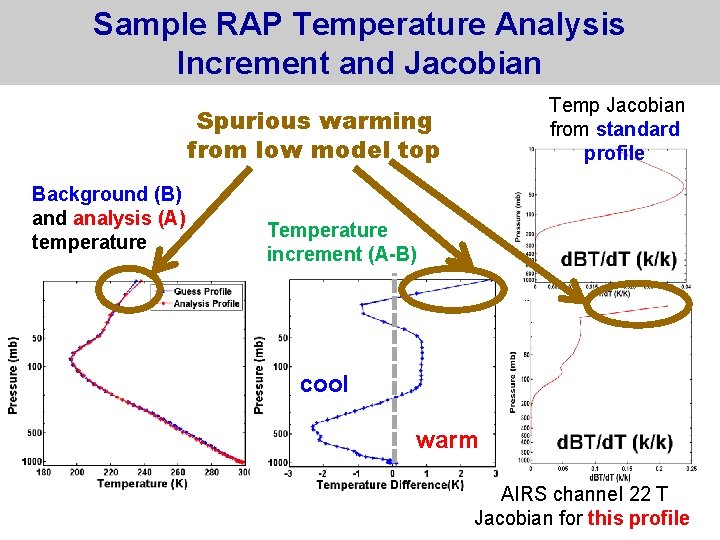
Sample RAP Temperature Analysis Increment and Jacobian Temp Jacobian from standard profile Spurious warming from low model top Background (B) and analysis (A) temperature Temperature increment (A-B) cool warm AIRS channel 22 T Jacobian for this profile
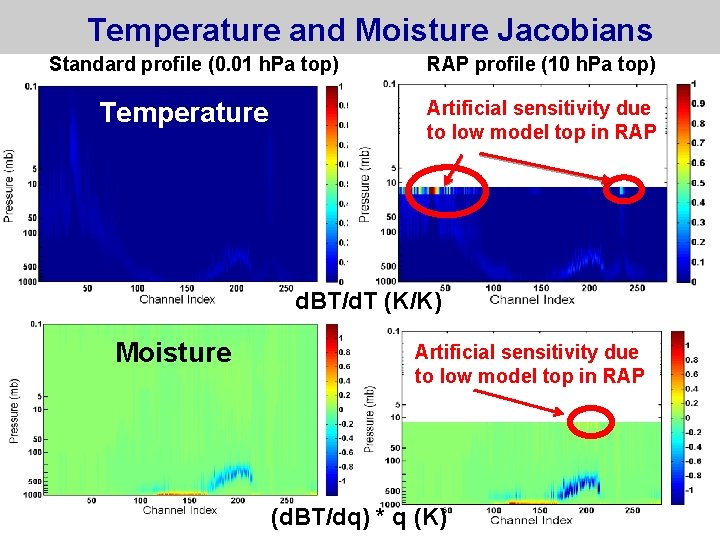
Temperature and Moisture Jacobians Standard profile (0. 01 h. Pa top) Temperature RAP profile (10 h. Pa top) Artificial sensitivity due to low model top in RAP d. BT/d. T (K/K) Moisture Artificial sensitivity due to low model top in RAP (d. BT/dq) * q (K)
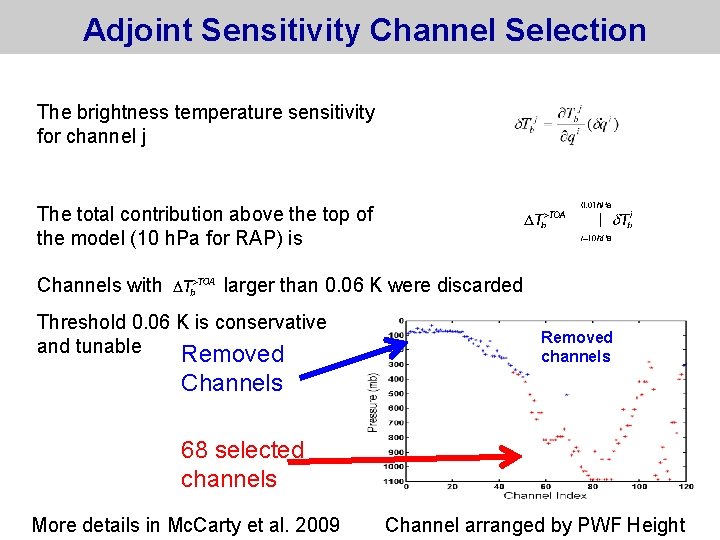
Adjoint Sensitivity Channel Selection The brightness temperature sensitivity for channel j The total contribution above the top of the model (10 h. Pa for RAP) is Channels with larger than 0. 06 K were discarded Threshold 0. 06 K is conservative and tunable Removed channels Channels 68 selected channels More details in Mc. Carty et al. 2009 Channel arranged by PWF Height
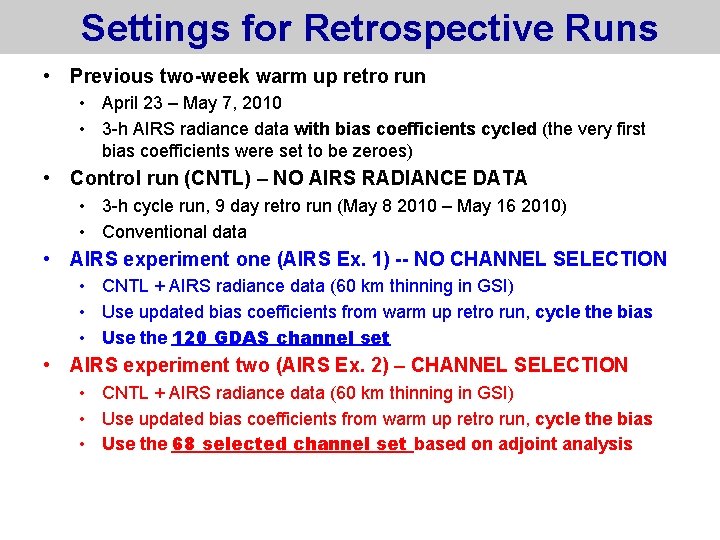
Settings for Retrospective Runs • Previous two-week warm up retro run • April 23 – May 7, 2010 • 3 -h AIRS radiance data with bias coefficients cycled (the very first bias coefficients were set to be zeroes) • Control run (CNTL) – NO AIRS RADIANCE DATA • 3 -h cycle run, 9 day retro run (May 8 2010 – May 16 2010) • Conventional data • AIRS experiment one (AIRS Ex. 1) -- NO CHANNEL SELECTION • CNTL + AIRS radiance data (60 km thinning in GSI) • Use updated bias coefficients from warm up retro run, cycle the bias • Use the 120 GDAS channel set • AIRS experiment two (AIRS Ex. 2) – CHANNEL SELECTION • CNTL + AIRS radiance data (60 km thinning in GSI) • Use updated bias coefficients from warm up retro run, cycle the bias • Use the 68 selected channel set based on adjoint analysis
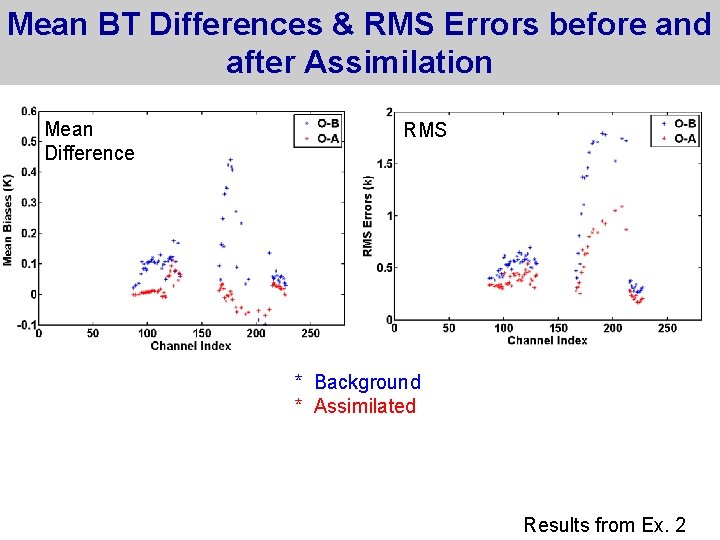
Mean BT Differences & RMS Errors before and after Assimilation Mean Difference RMS * Background * Assimilated Results from Ex. 2
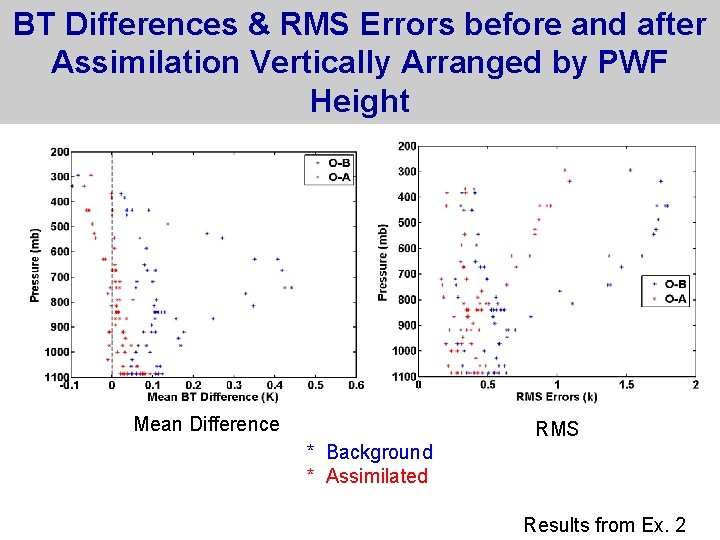
BT Differences & RMS Errors before and after Assimilation Vertically Arranged by PWF Height Mean Difference RMS * Background * Assimilated Results from Ex. 2
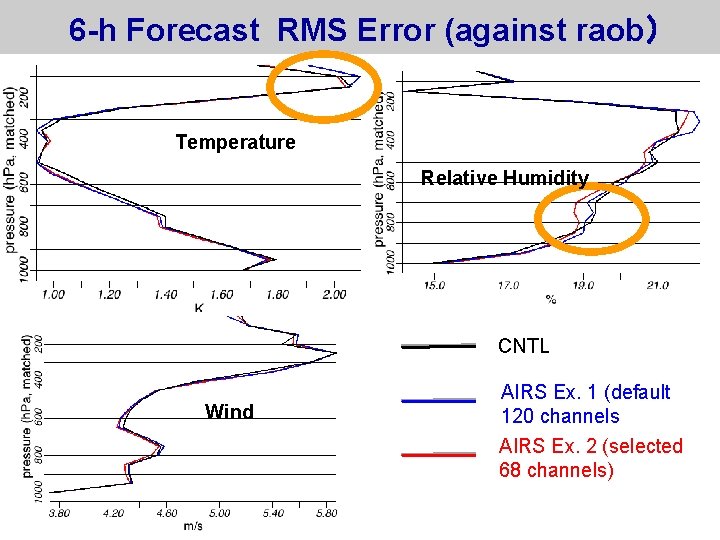
6 -h Forecast RMS Error (against raob) Temperature Relative Humidity CNTL Wind AIRS Ex. 1 (default 120 channels AIRS Ex. 2 (selected 68 channels)
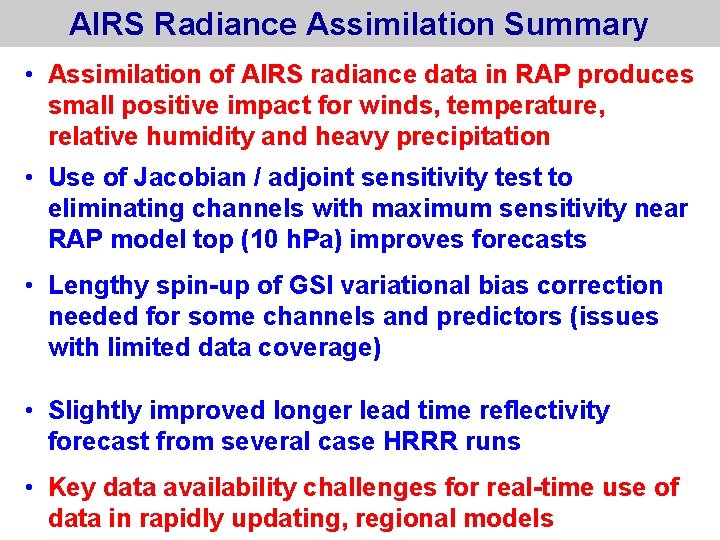
AIRS Radiance Assimilation Summary • Assimilation of AIRS radiance data in RAP produces small positive impact for winds, temperature, relative humidity and heavy precipitation • Use of Jacobian / adjoint sensitivity test to eliminating channels with maximum sensitivity near RAP model top (10 h. Pa) improves forecasts • Lengthy spin-up of GSI variational bias correction needed for some channels and predictors (issues with limited data coverage) • Slightly improved longer lead time reflectivity forecast from several case HRRR runs • Key data availability challenges for real-time use of data in rapidly updating, regional models
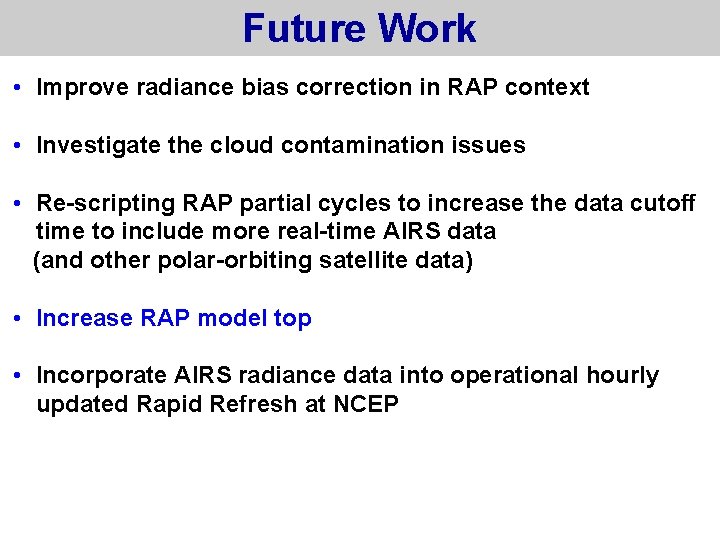
Future Work • Improve radiance bias correction in RAP context • Investigate the cloud contamination issues • Re-scripting RAP partial cycles to increase the data cutoff time to include more real-time AIRS data (and other polar-orbiting satellite data) • Increase RAP model top • Incorporate AIRS radiance data into operational hourly updated Rapid Refresh at NCEP
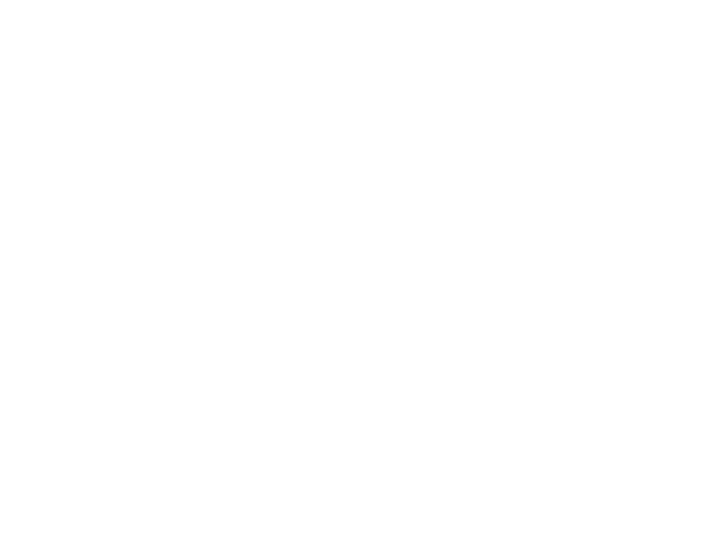
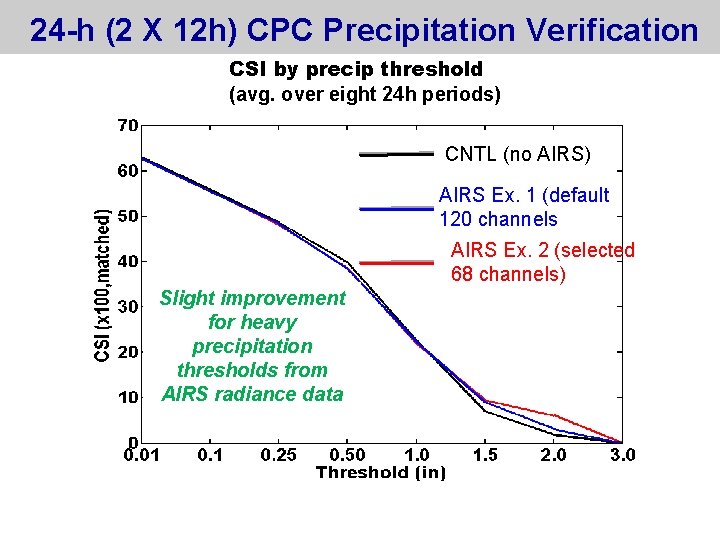
24 -h (2 X 12 h) CPC Precipitation Verification CSI by precip threshold (avg. over eight 24 h periods) CNTL (no AIRS) AIRS Ex. 1 (default 120 channels AIRS Ex. 2 (selected 68 channels) Slight improvement for heavy precipitation thresholds from AIRS radiance data
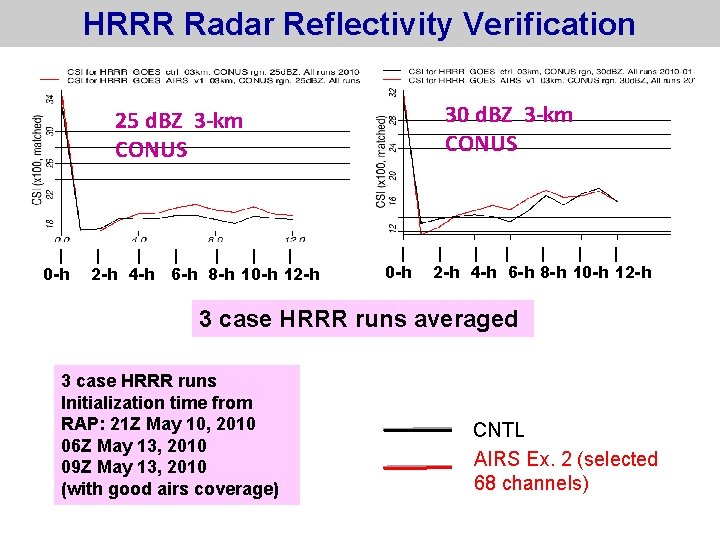
HRRR Radar Reflectivity Verification 30 d. BZ 3 -km CONUS 25 d. BZ 3 -km CONUS | 0 -h | | | 2 -h 4 -h 6 -h 8 -h 10 -h 12 -h 3 case HRRR runs averaged 3 case HRRR runs Initialization time from RAP: 21 Z May 10, 2010 06 Z May 13, 2010 09 Z May 13, 2010 (with good airs coverage) CNTL AIRS Ex. 2 (selected 68 channels)
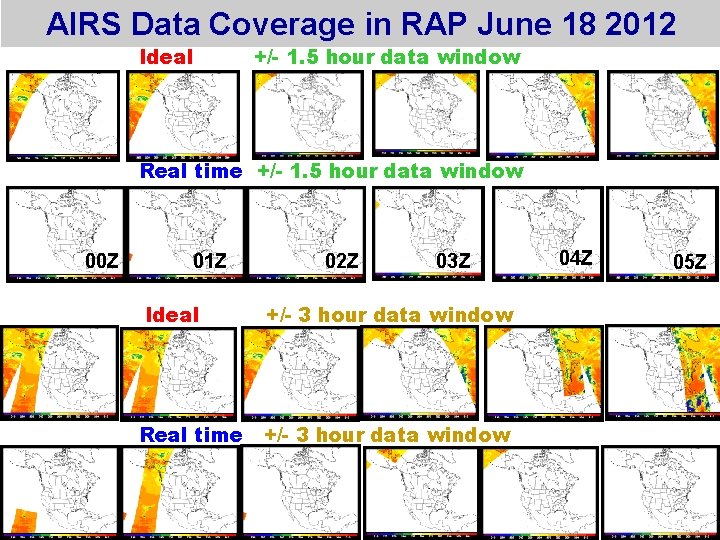
AIRS Data Coverage in RAP June 18 2012 Ideal +/- 1. 5 hour data window Real time +/- 1. 5 hour data window 00 Z 01 Z 02 Z 03 Z Ideal +/- 3 hour data window Real time +/- 3 hour data window 04 Z 05 Z
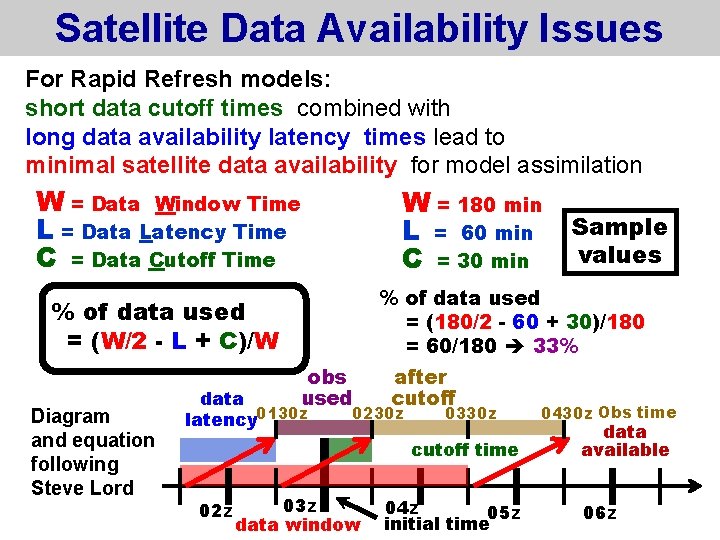
Satellite Data Availability Issues For Rapid Refresh models: short data cutoff times combined with long data availability latency times lead to minimal satellite data availability for model assimilation W = Data Window Time L = Data Latency Time C = Data Cutoff Time W = 180 min L = 60 min C = 30 min % of data used = (180/2 - 60 + 30)/180 = 60/180 33% % of data used = (W/2 - L + C)/W Diagram and equation following Steve Lord obs used data latency 0130 z after cutoff 0230 z 0330 z cutoff time 02 z Sample values 03 z data window 04 z 05 z initial time 0430 z Obs time data available 06 z
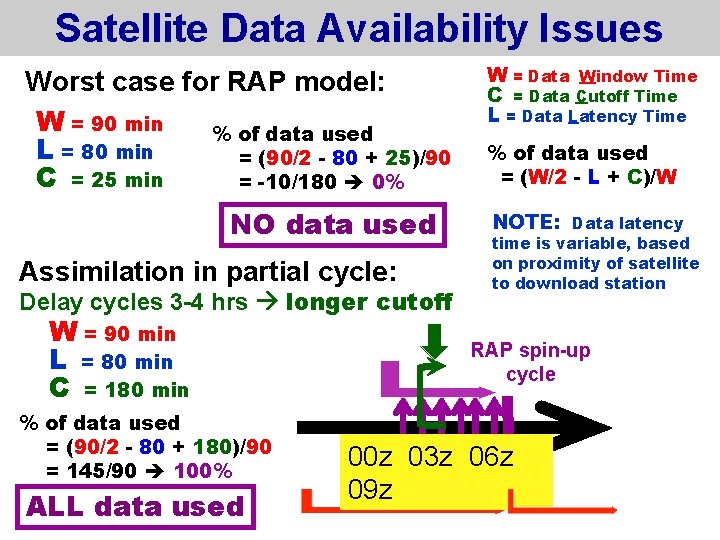
Satellite Data Availability Issues Worst case for RAP model: W = 90 min % of data used L = 80 min = (90/2 - 80 + 25)/90 C = 25 min = -10/180 0% NO data used Assimilation in partial cycle: Delay cycles 3 -4 hrs longer cutoff W = 90 min L = 80 min C = 180 min % of data used = (90/2 - 80 + 180)/90 = 145/90 100% ALL data used W = Data Window Time C = Data Cutoff Time L = Data Latency Time % of data used = (W/2 - L + C)/W NOTE: Data latency time is variable, based on proximity of satellite to download station RAP spin-up cycle 00 z 03 z 06 z 09 z
Jedi data assimilation
Data assimilation
Data assimilation tutorial
Data assimilation
Data assimilation
Data assimilation
Data assimilation
Data assimilation
Bilkent registrar's office
Airs accreditation
Ahca incident reporting system
Alliance of information and referral systems
Airs taxonomy
Cirs certification
Cfia airs tool
Radiance equation
Total radiance
Reflectance vs radiance
Total radiance
Irradiance to radiance conversion
Spherical harmonics lighting
Radiance map
Precomputed radiance transfer
Ap psychology unit 7 cognition
Progressive assimilation
Regressive assimilation examples
Assimilation of voice examples
Ingestion digestion absorption assimilation egestion order
Example of assimilation in child development