Progress in Cloudy Microwave Satellite Data Assimilation at
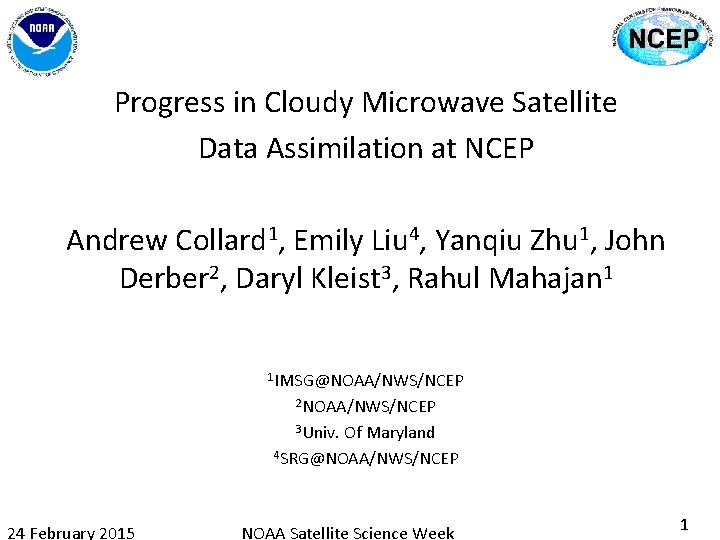
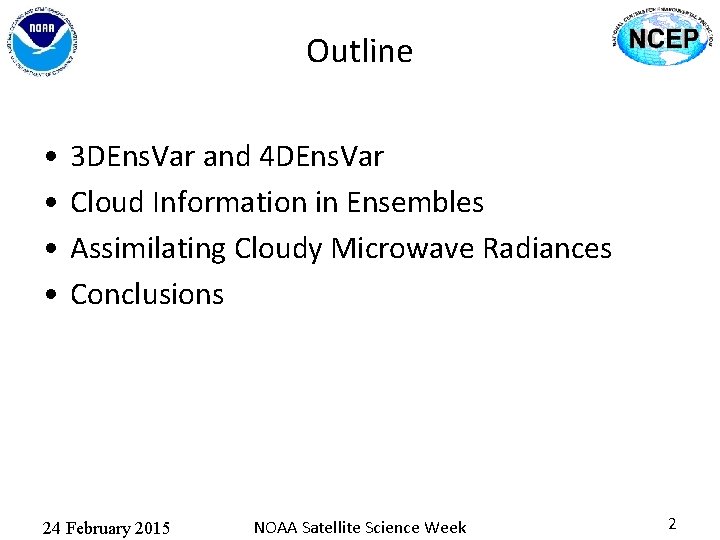
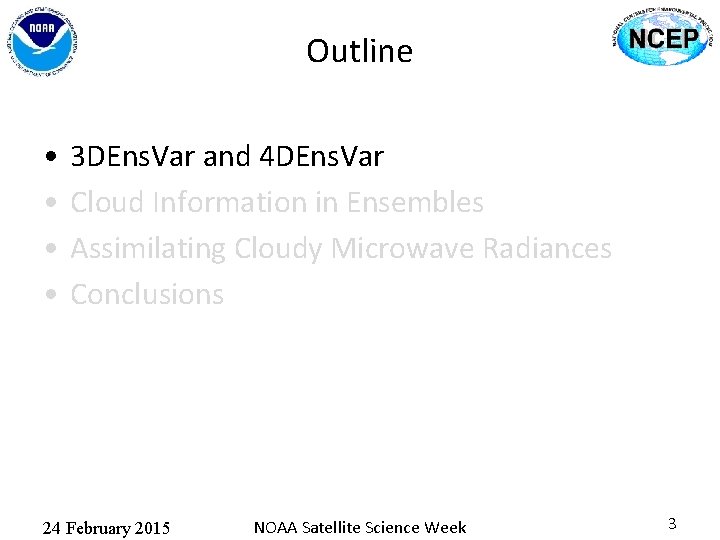
![GSI Hybrid [3 D] En. Var (ignoring preconditioning for simplicity) • Incorporate ensemble perturbations GSI Hybrid [3 D] En. Var (ignoring preconditioning for simplicity) • Incorporate ensemble perturbations](https://slidetodoc.com/presentation_image/98db1cd83da3b669995e3fc425c2b181/image-4.jpg)
![Hybrid 4 D-Ensemble-Var [H-4 DENSV] The 4 DENSV cost function can be easily expanded Hybrid 4 D-Ensemble-Var [H-4 DENSV] The 4 DENSV cost function can be easily expanded](https://slidetodoc.com/presentation_image/98db1cd83da3b669995e3fc425c2b181/image-5.jpg)
![Hybrid 4 D-Ensemble-Var [H-4 DENSV] The 4 DENSV cost function can be easily expanded Hybrid 4 D-Ensemble-Var [H-4 DENSV] The 4 DENSV cost function can be easily expanded](https://slidetodoc.com/presentation_image/98db1cd83da3b669995e3fc425c2b181/image-6.jpg)
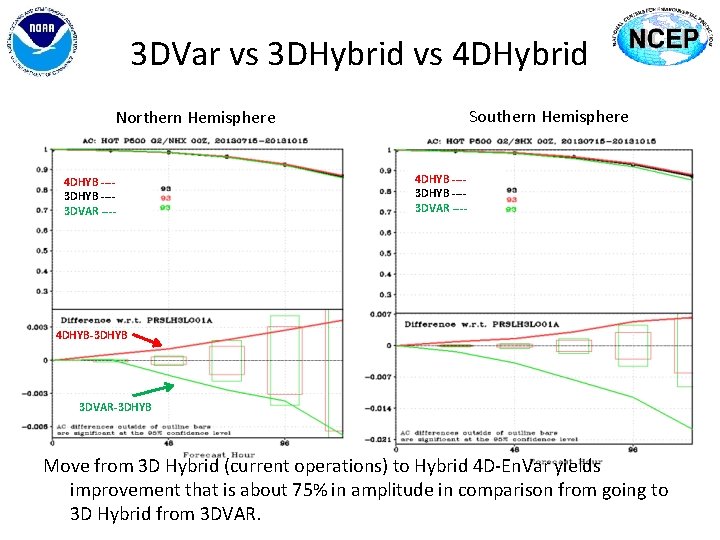
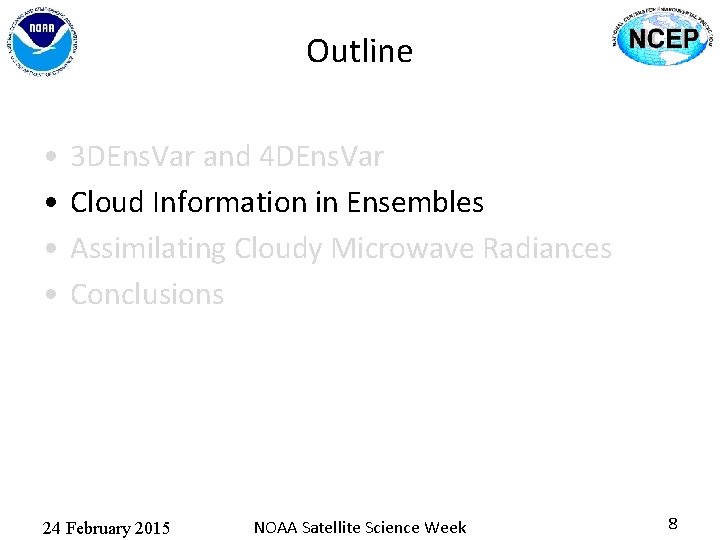
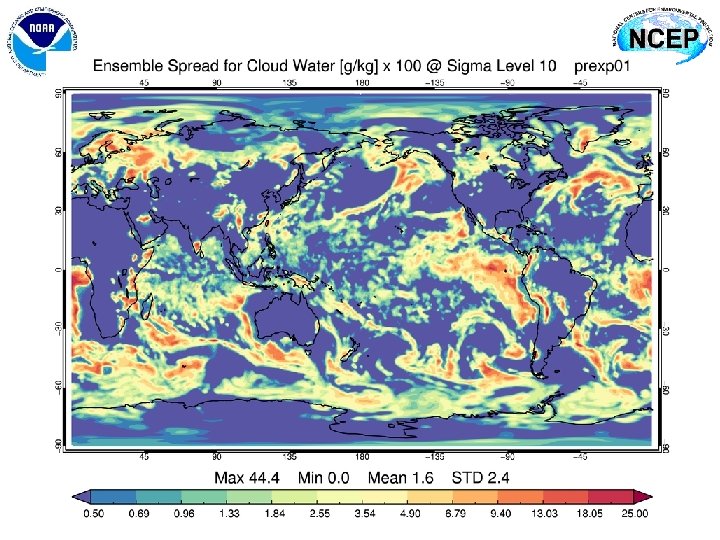
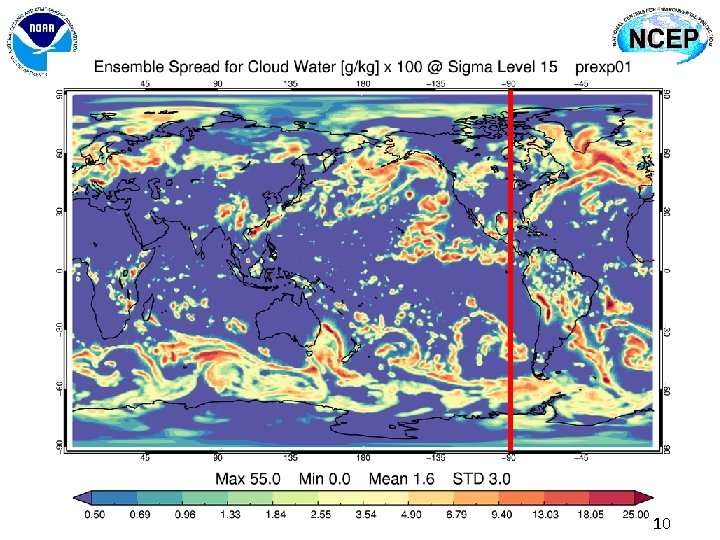
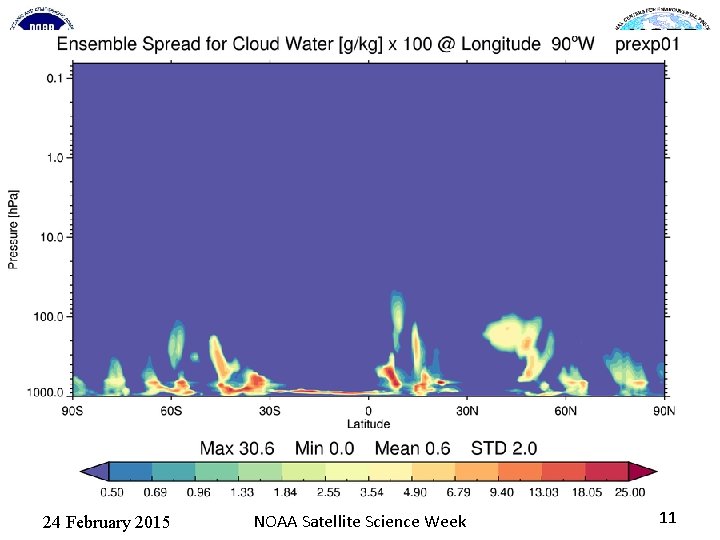
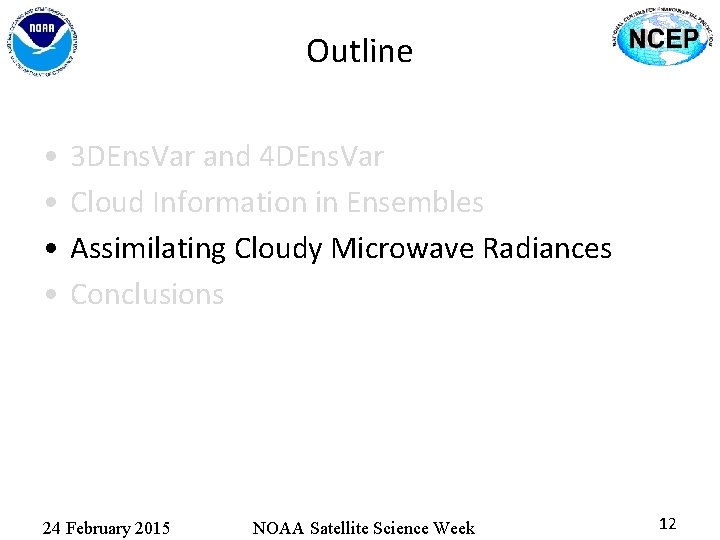
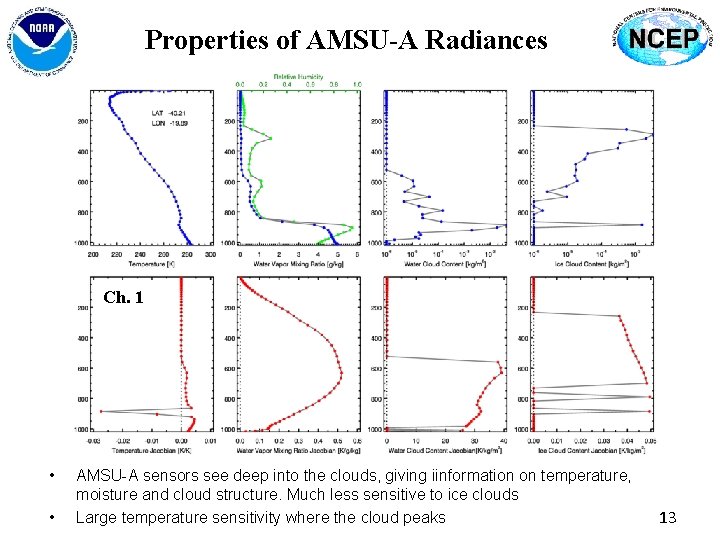
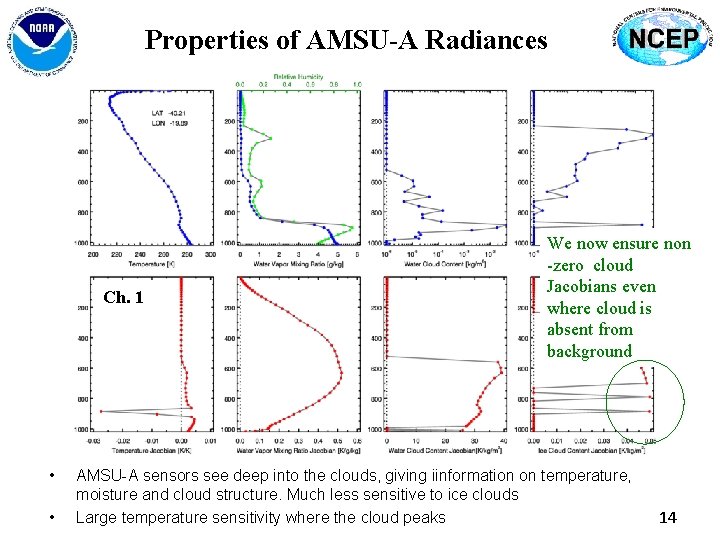
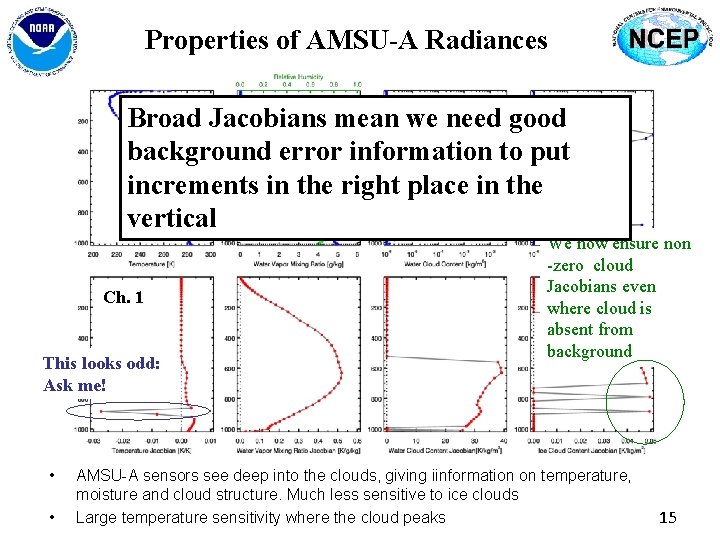
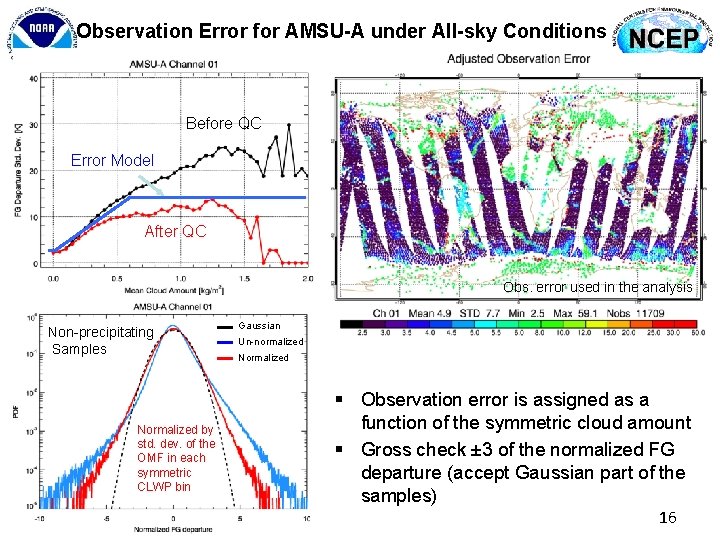
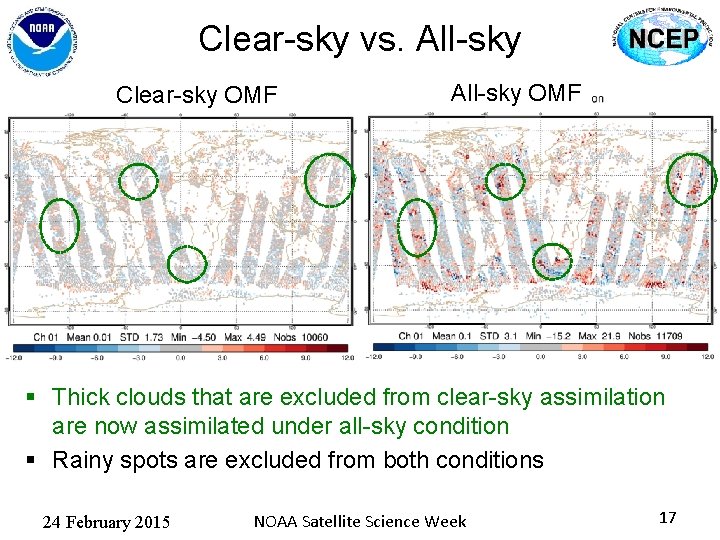
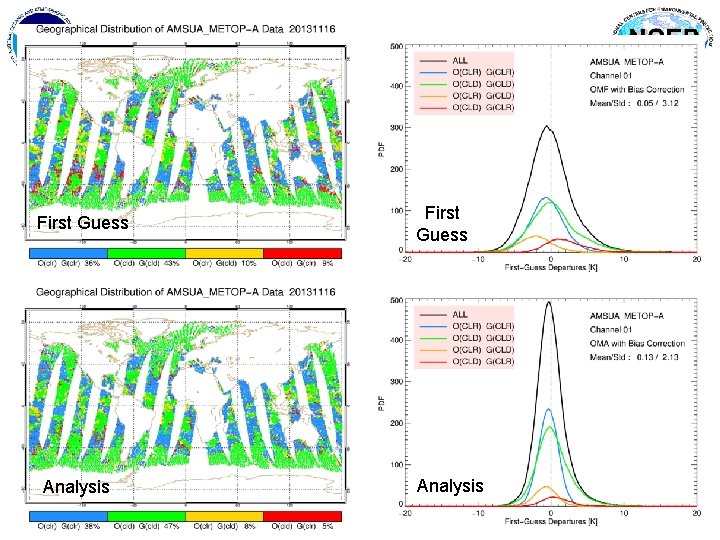
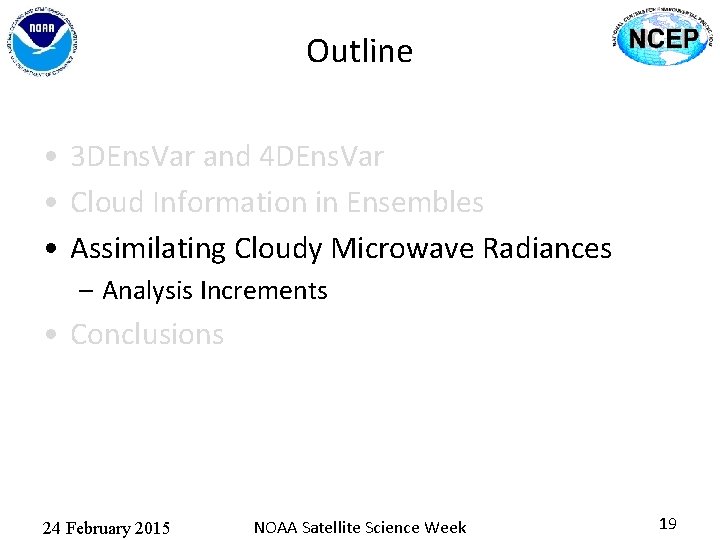
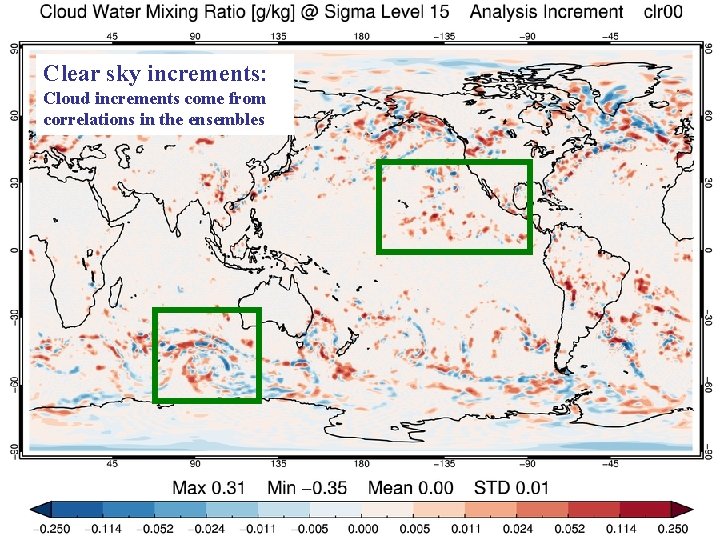
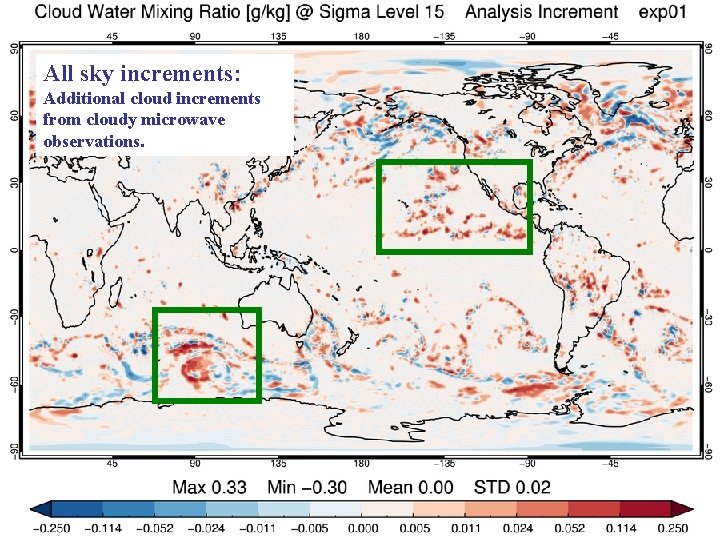
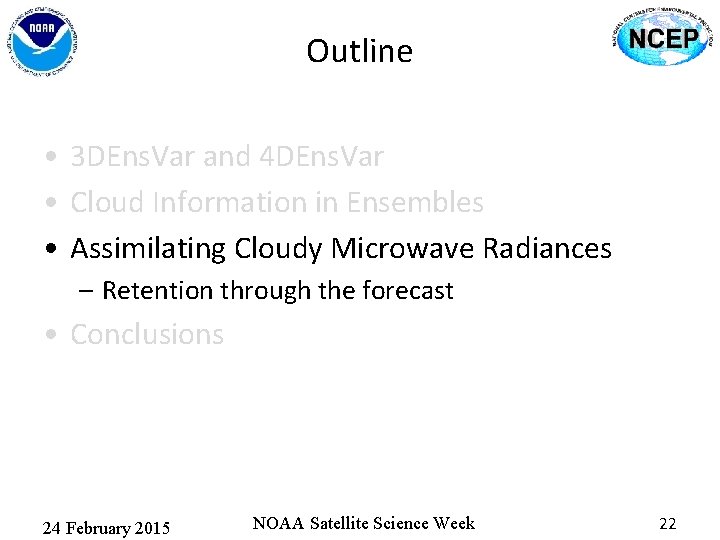
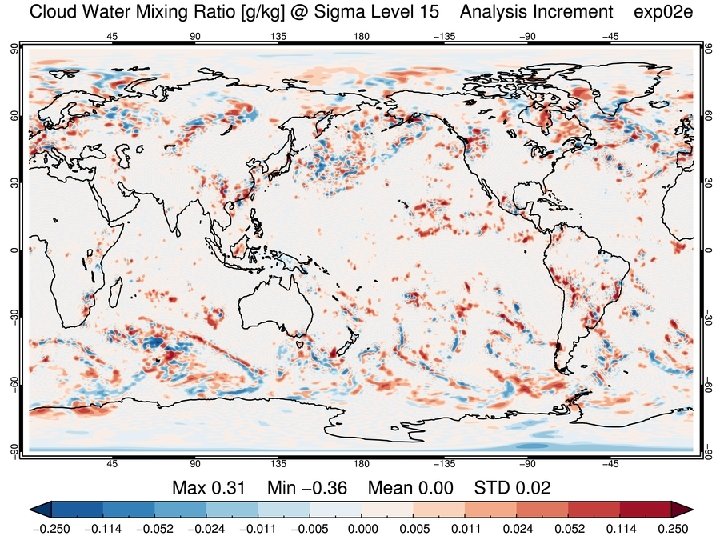
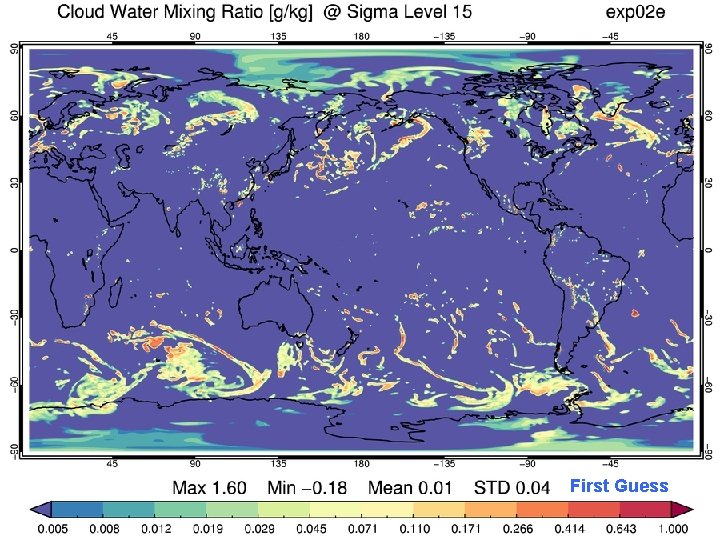
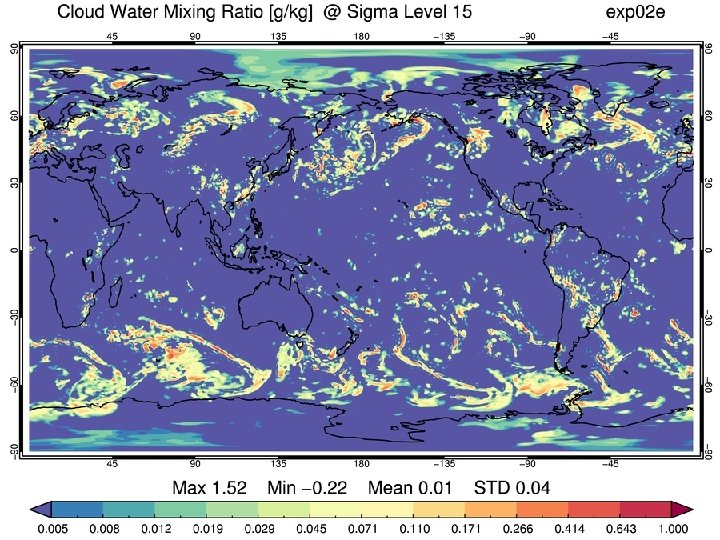
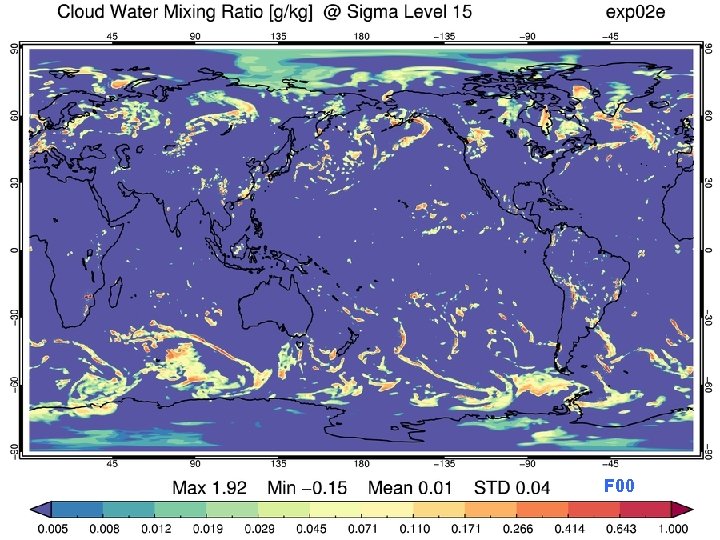
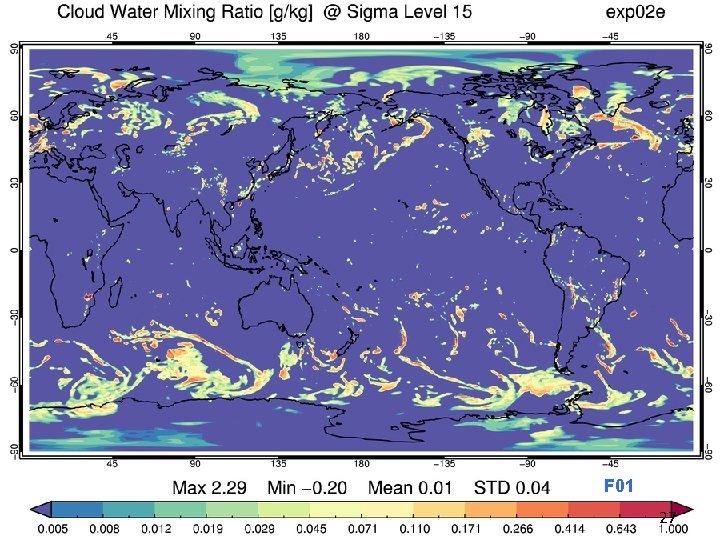
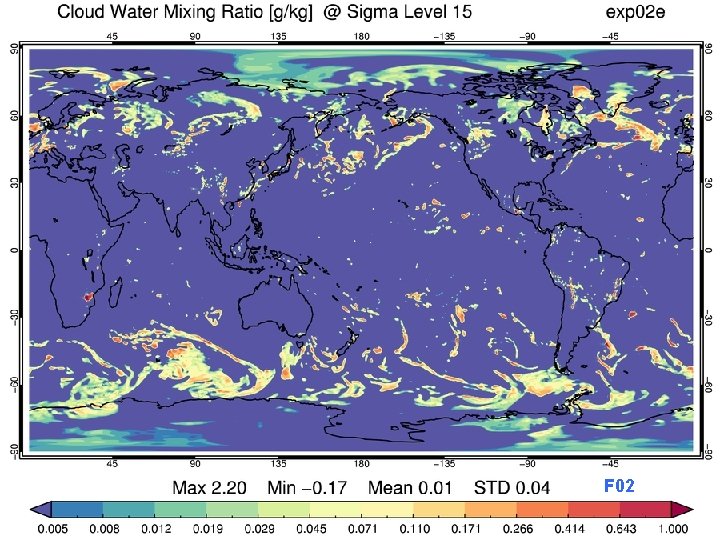
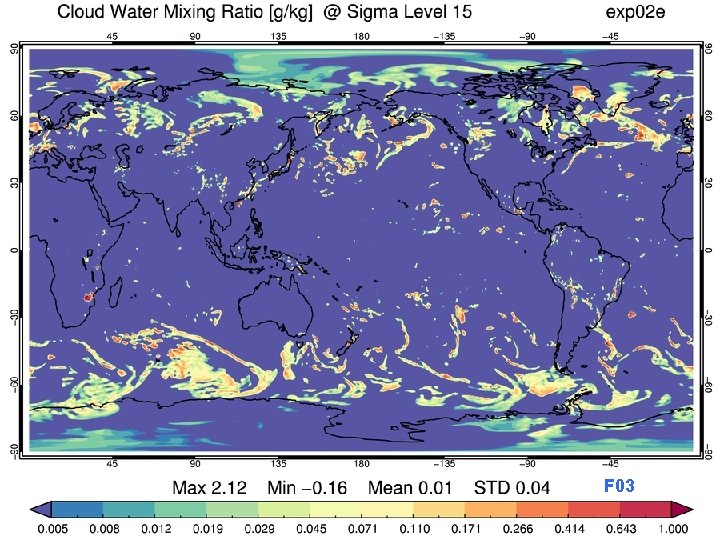
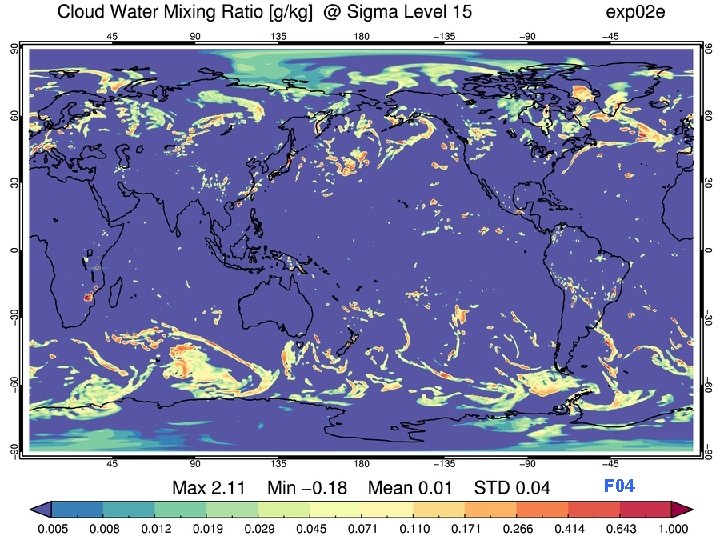
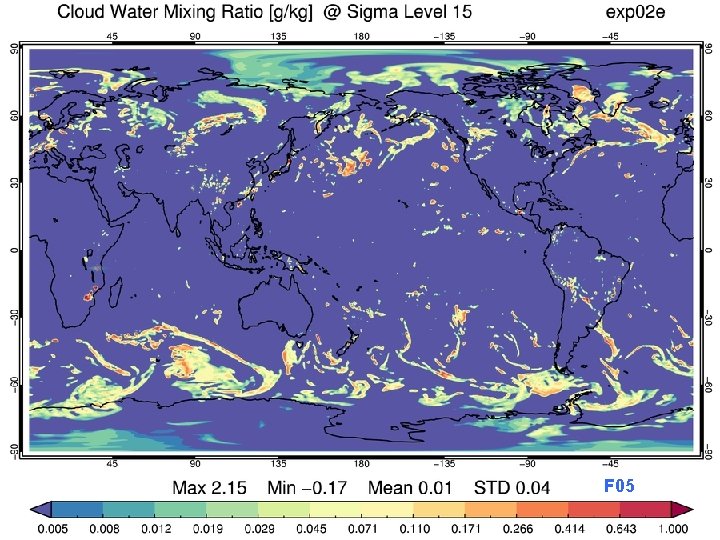
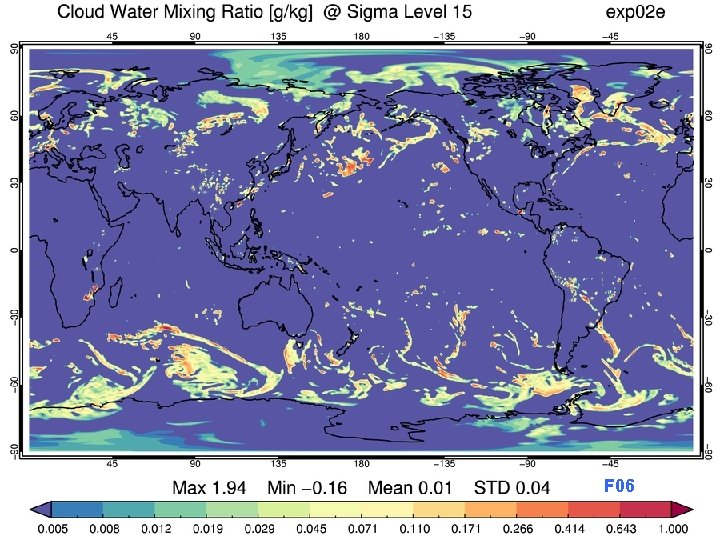
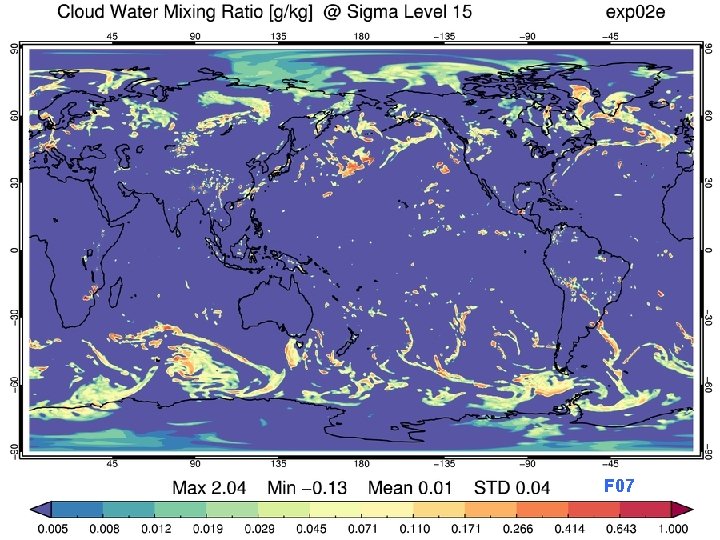
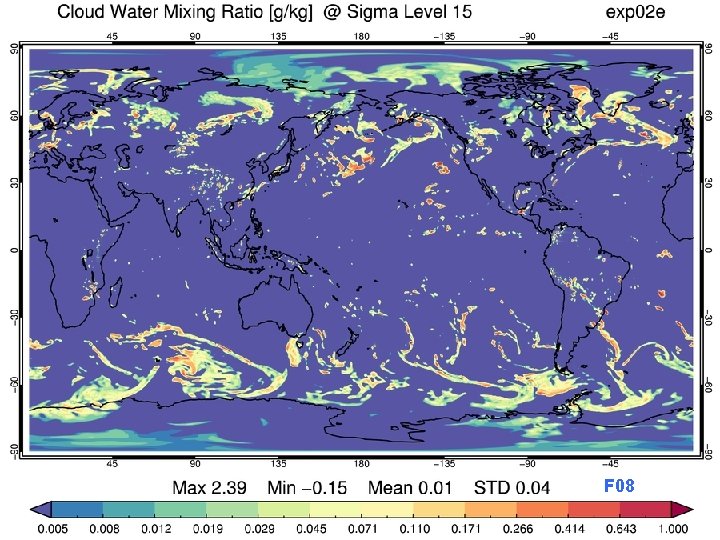
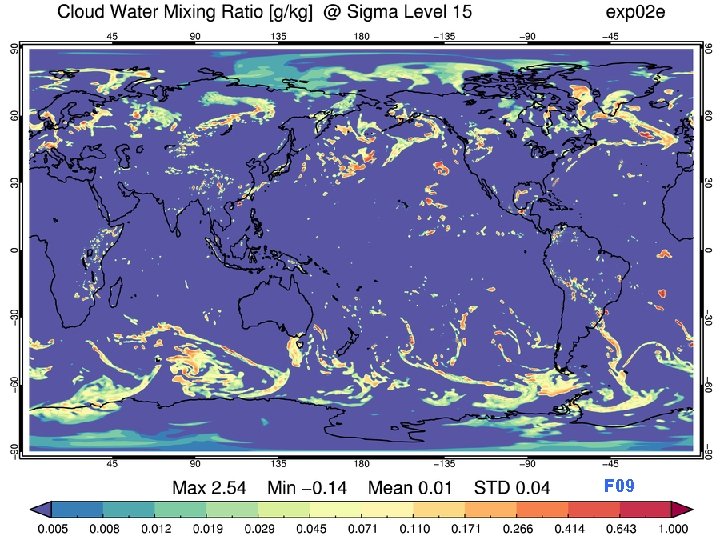
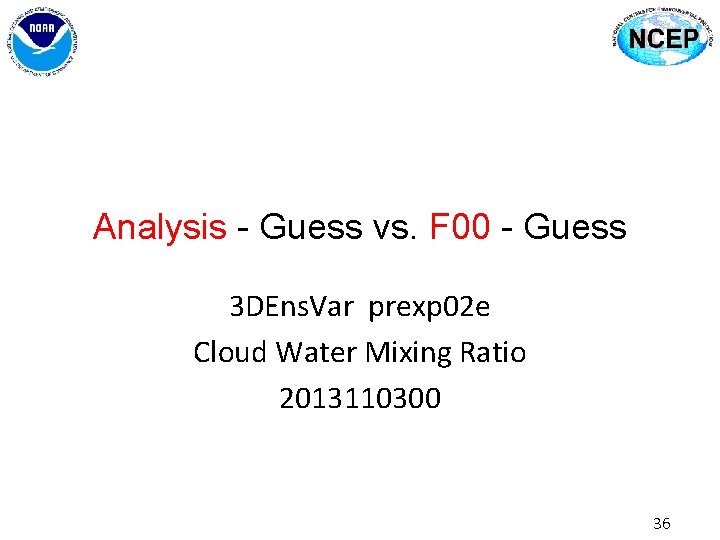
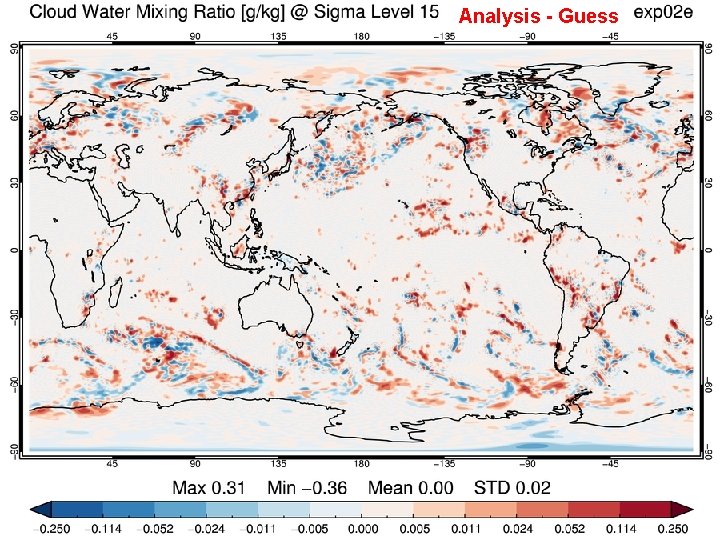
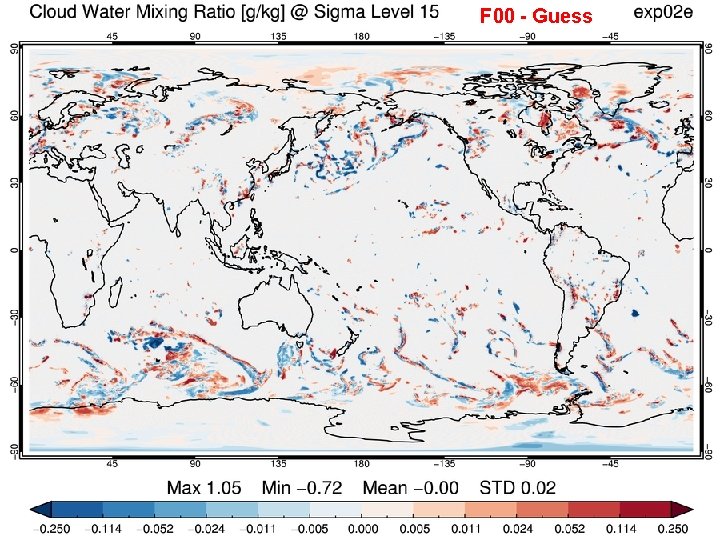
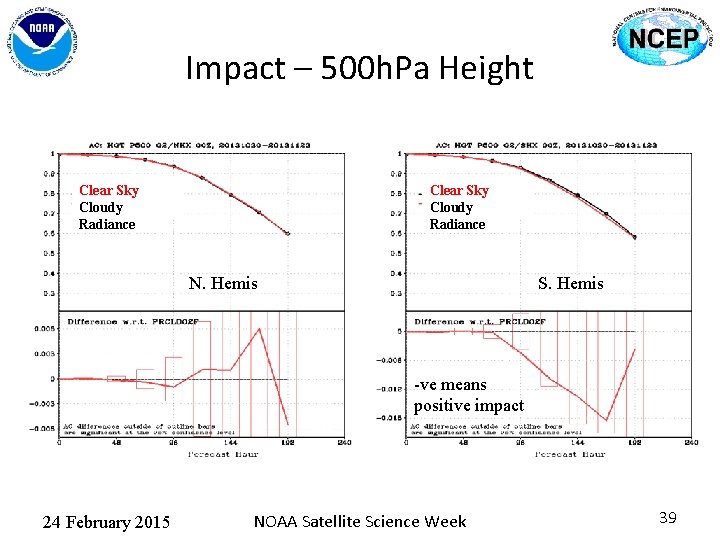
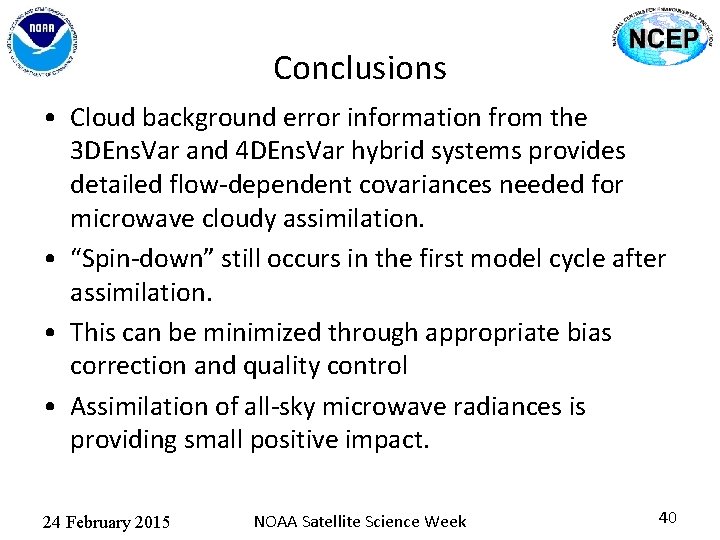
- Slides: 40
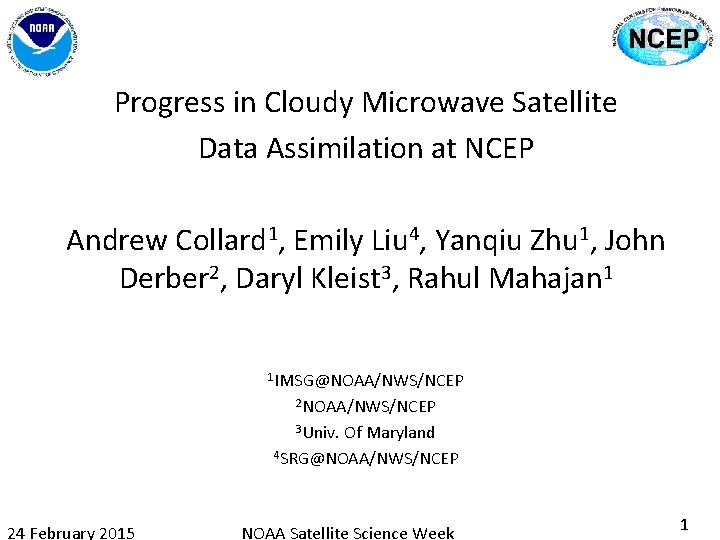
Progress in Cloudy Microwave Satellite Data Assimilation at NCEP Andrew Collard 1, Emily Liu 4, Yanqiu Zhu 1, John Derber 2, Daryl Kleist 3, Rahul Mahajan 1 1 IMSG@NOAA/NWS/NCEP 2 NOAA/NWS/NCEP 3 Univ. Of Maryland 4 SRG@NOAA/NWS/NCEP 24 February 2015 NOAA Satellite Science Week 1
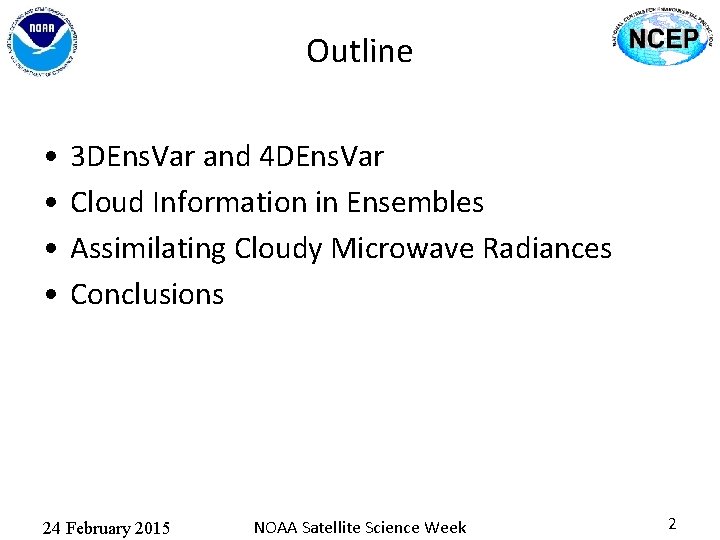
Outline • • 3 DEns. Var and 4 DEns. Var Cloud Information in Ensembles Assimilating Cloudy Microwave Radiances Conclusions 24 February 2015 NOAA Satellite Science Week 2
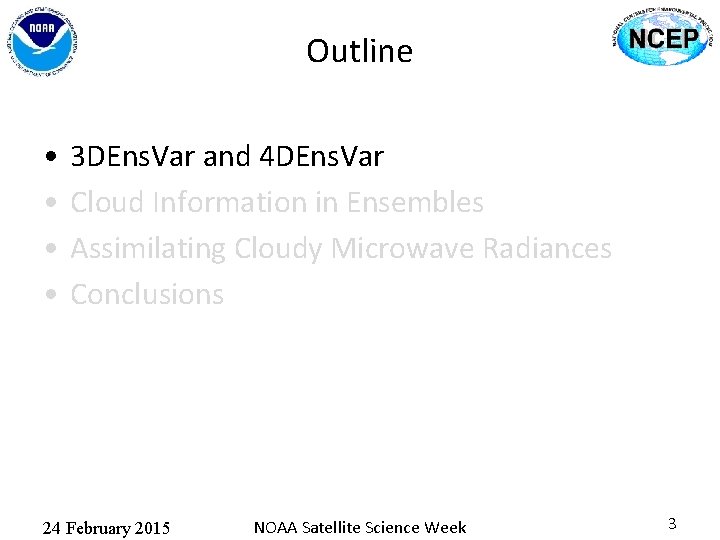
Outline • • 3 DEns. Var and 4 DEns. Var Cloud Information in Ensembles Assimilating Cloudy Microwave Radiances Conclusions 24 February 2015 NOAA Satellite Science Week 3
![GSI Hybrid 3 D En Var ignoring preconditioning for simplicity Incorporate ensemble perturbations GSI Hybrid [3 D] En. Var (ignoring preconditioning for simplicity) • Incorporate ensemble perturbations](https://slidetodoc.com/presentation_image/98db1cd83da3b669995e3fc425c2b181/image-4.jpg)
GSI Hybrid [3 D] En. Var (ignoring preconditioning for simplicity) • Incorporate ensemble perturbations directly into variational cost function through extended control variable – Lorenc (2003), Buehner (2005), Wang et. al. (2007), etc. bf & be: weighting coefficients for fixed and ensemble covariance respectively xt’: (total increment) sum of increment from fixed/static B (xf’) and ensemble B ak: extended control variable; : ensemble perturbations - analogous to the weights in the LETKF formulation L: correlation matrix [effectively the localization of ensemble perturbations] T: operator mapping from ensemble grid to analysis grid
![Hybrid 4 DEnsembleVar H4 DENSV The 4 DENSV cost function can be easily expanded Hybrid 4 D-Ensemble-Var [H-4 DENSV] The 4 DENSV cost function can be easily expanded](https://slidetodoc.com/presentation_image/98db1cd83da3b669995e3fc425c2b181/image-5.jpg)
Hybrid 4 D-Ensemble-Var [H-4 DENSV] The 4 DENSV cost function can be easily expanded to include a static contribution Where the 4 D increment is prescribed exclusively through linear combinations of the 4 D ensemble perturbations plus static contribution Here, the static contribution is considered time-invariant (i. e. from 3 DVARFGAT). Weighting parameters exist just as in the other hybrid variants. Again, no TLM or ADJ (so this is NOT 4 DVar)!
![Hybrid 4 DEnsembleVar H4 DENSV The 4 DENSV cost function can be easily expanded Hybrid 4 D-Ensemble-Var [H-4 DENSV] The 4 DENSV cost function can be easily expanded](https://slidetodoc.com/presentation_image/98db1cd83da3b669995e3fc425c2b181/image-6.jpg)
Hybrid 4 D-Ensemble-Var [H-4 DENSV] The 4 DENSV cost function can be easily expanded to include a static contribution Where the 4 D increment is prescribed exclusively through linear combinations of the 4 D ensemble perturbations plus static contribution Here, the static contribution is considered time-invariant (i. e. from 3 DVARFGAT). Weighting parameters exist just as in the other hybrid variants. Again, no TLM or ADJ (so this is NOT 4 DVar)!
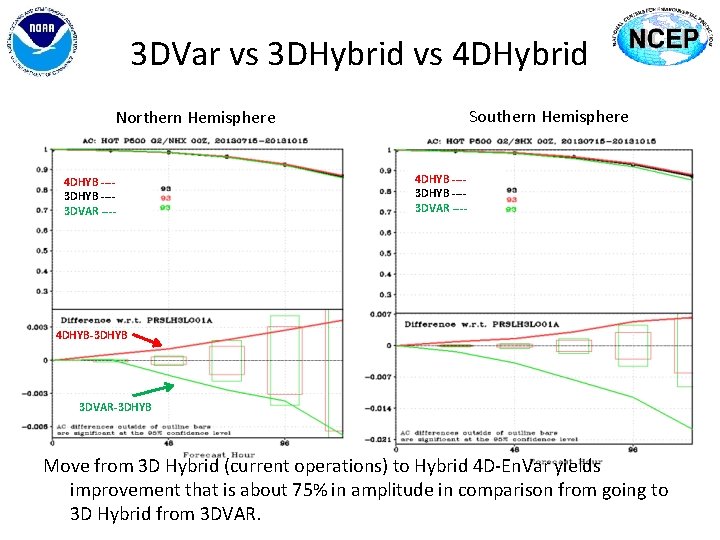
3 DVar vs 3 DHybrid vs 4 DHybrid Southern Hemisphere Northern Hemisphere 4 DHYB ---3 DHYB ---3 DVAR ---- 4 DHYB-3 DHYB 3 DVAR-3 DHYB Move from 3 D Hybrid (current operations) to Hybrid 4 D-En. Var yields improvement that is about 75% in amplitude in comparison from going to 3 D Hybrid from 3 DVAR.
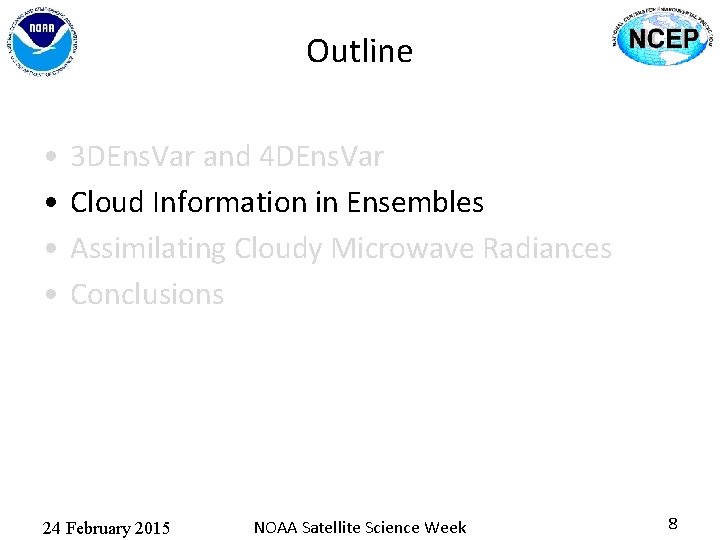
Outline • • 3 DEns. Var and 4 DEns. Var Cloud Information in Ensembles Assimilating Cloudy Microwave Radiances Conclusions 24 February 2015 NOAA Satellite Science Week 8
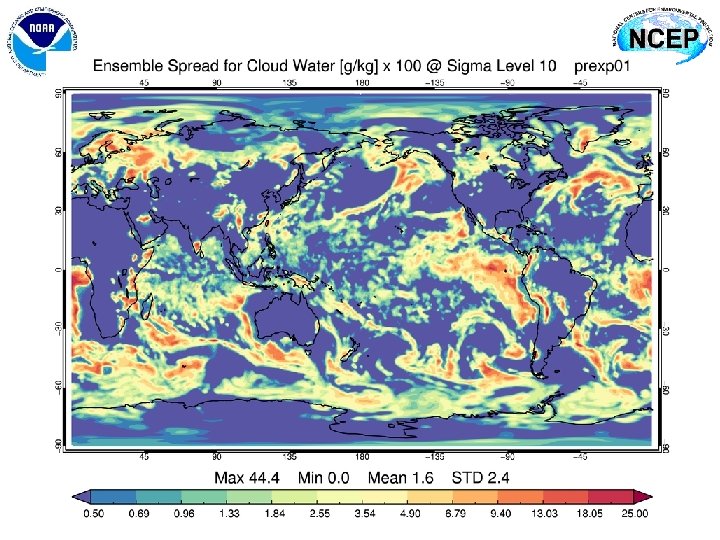
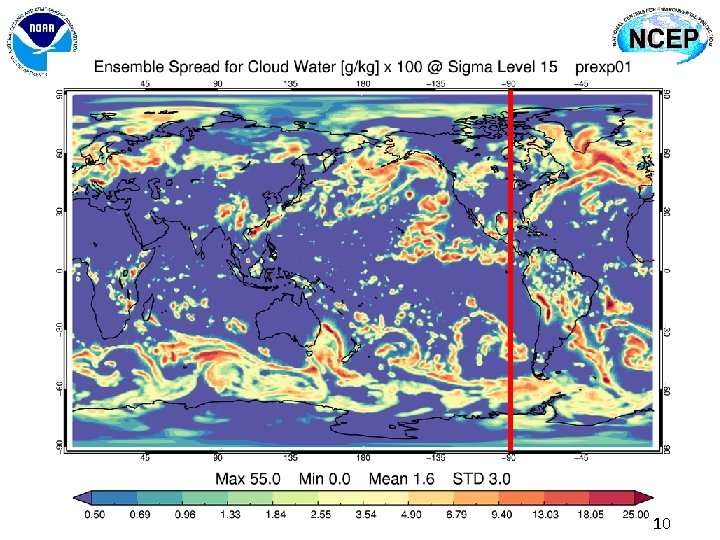
10
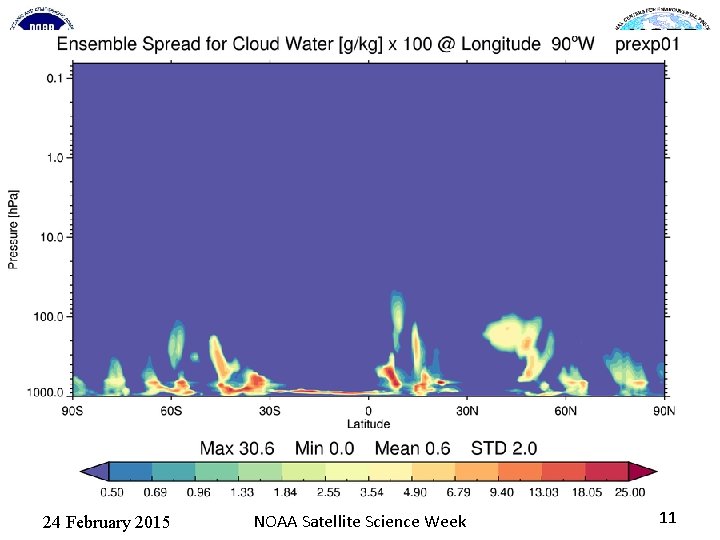
24 February 2015 NOAA Satellite Science Week 11
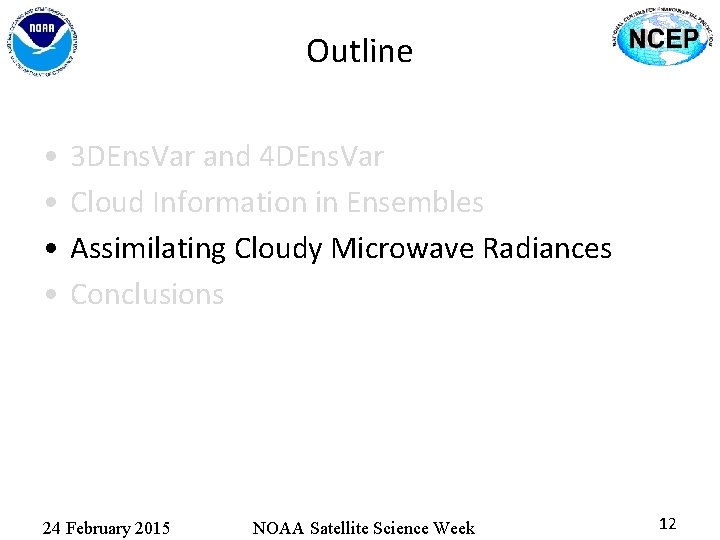
Outline • • 3 DEns. Var and 4 DEns. Var Cloud Information in Ensembles Assimilating Cloudy Microwave Radiances Conclusions 24 February 2015 NOAA Satellite Science Week 12
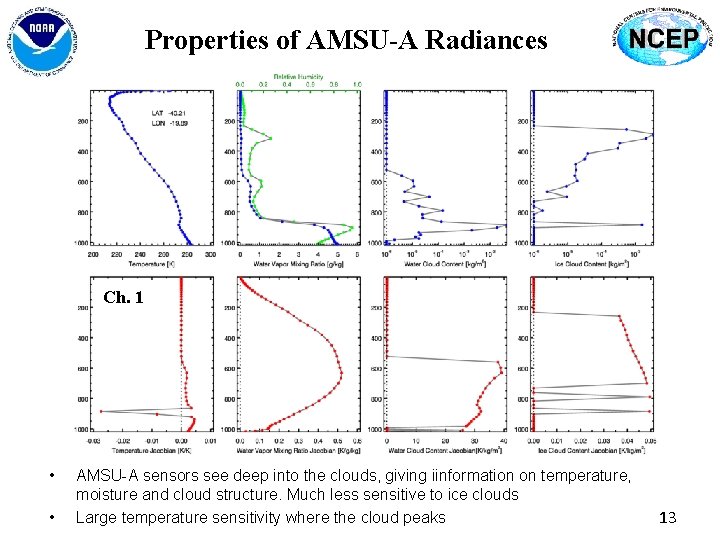
Properties of AMSU-A Radiances Ch. 1 • AMSU-A sensors see deep into the clouds, giving iinformation on temperature, moisture and cloud structure. Much less sensitive to ice clouds NOAA Satellite Science Week 24 2015 • February Large temperature sensitivity where the cloud peaks 13
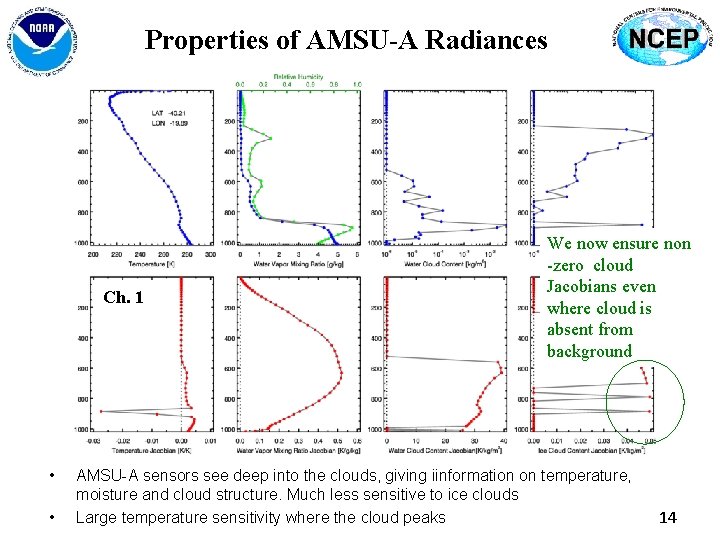
Properties of AMSU-A Radiances Ch. 1 We now ensure non -zero cloud Jacobians even where cloud is absent from background • AMSU-A sensors see deep into the clouds, giving iinformation on temperature, moisture and cloud structure. Much less sensitive to ice clouds NOAA Satellite Science Week 24 2015 • February Large temperature sensitivity where the cloud peaks 14
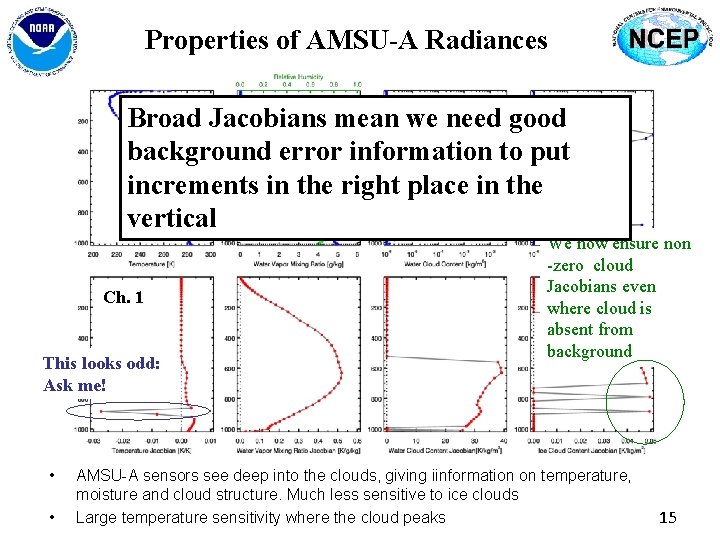
Properties of AMSU-A Radiances Broad Jacobians mean we need good background error information to put increments in the right place in the vertical Ch. 1 This looks odd: Ask me! We now ensure non -zero cloud Jacobians even where cloud is absent from background • AMSU-A sensors see deep into the clouds, giving iinformation on temperature, moisture and cloud structure. Much less sensitive to ice clouds NOAA Satellite Science Week 24 2015 • February Large temperature sensitivity where the cloud peaks 15
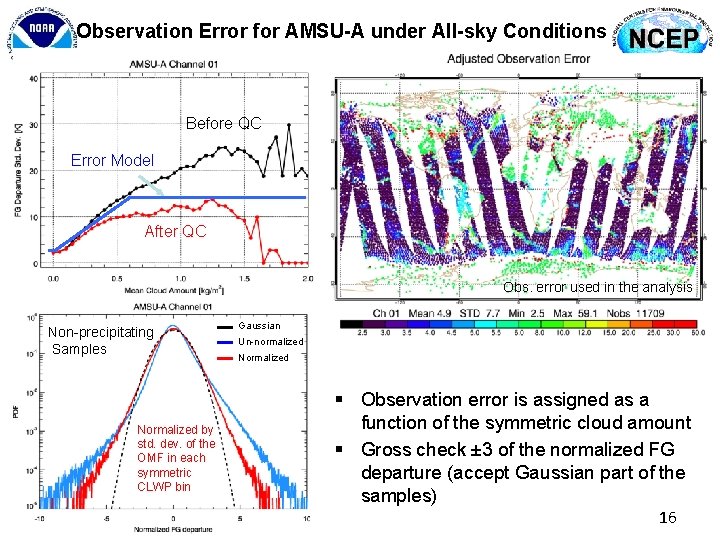
Observation Error for AMSU-A under All-sky Conditions Before QC Error Model After QC Obs. error used in the analysis Non-precipitating Samples Normalized by std. dev. of the OMF in each symmetric CLWP bin Gaussian Un-normalized Normalized § Observation error is assigned as a function of the symmetric cloud amount § Gross check ± 3 of the normalized FG departure (accept Gaussian part of the samples) 16
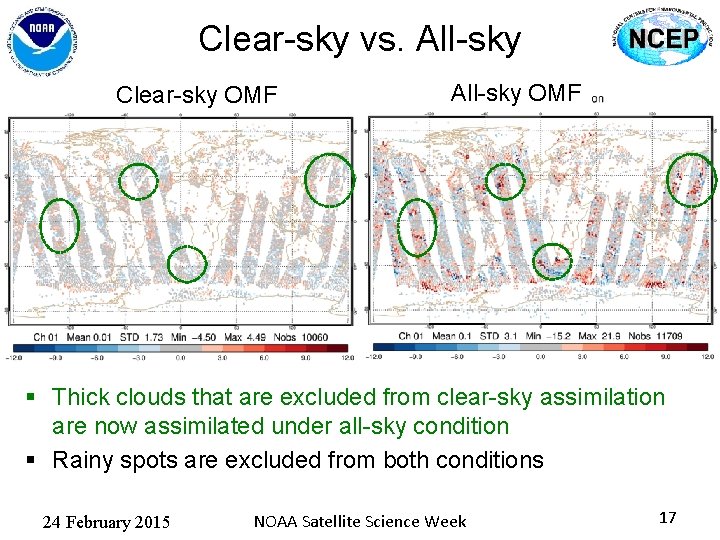
Clear-sky vs. All-sky Clear-sky OMF All-sky OMF § Thick clouds that are excluded from clear-sky assimilation are now assimilated under all-sky condition § Rainy spots are excluded from both conditions 24 February 2015 NOAA Satellite Science Week 17
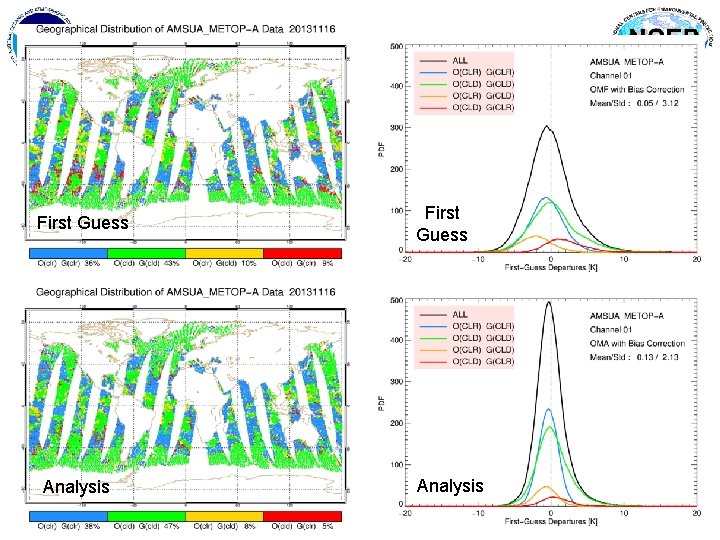
First Guess Analysis
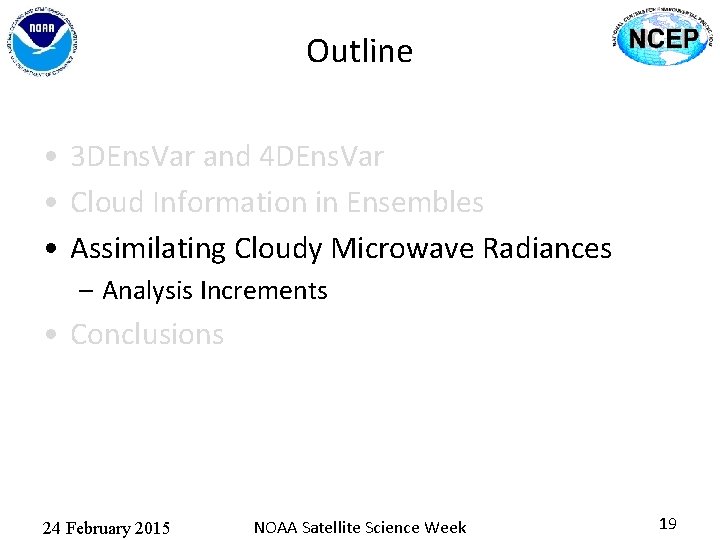
Outline • 3 DEns. Var and 4 DEns. Var • Cloud Information in Ensembles • Assimilating Cloudy Microwave Radiances – Analysis Increments • Conclusions 24 February 2015 NOAA Satellite Science Week 19
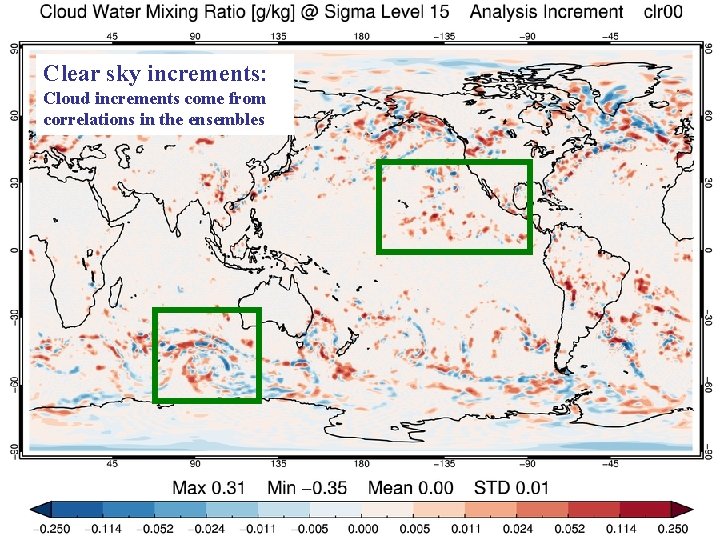
Clear sky increments: Cloud increments come from correlations in the ensembles
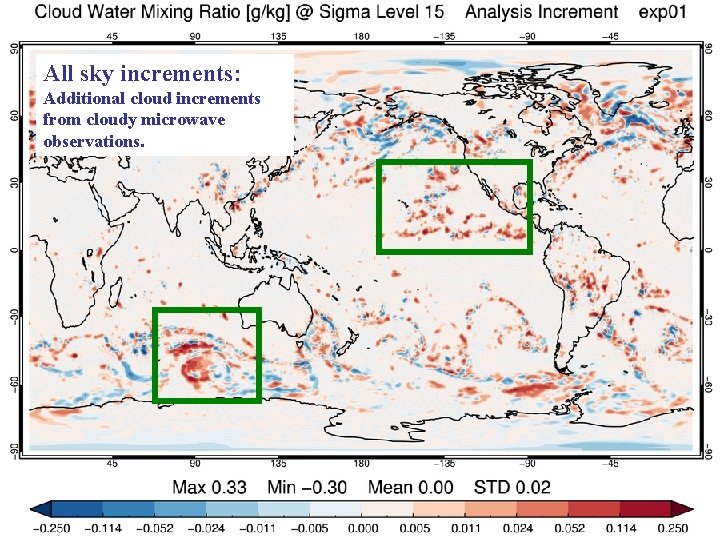
All sky increments: Additional cloud increments from cloudy microwave observations.
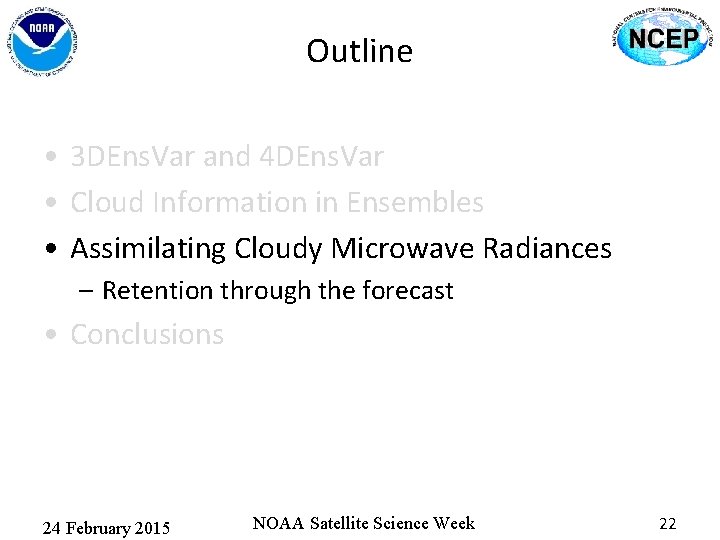
Outline • 3 DEns. Var and 4 DEns. Var • Cloud Information in Ensembles • Assimilating Cloudy Microwave Radiances – Retention through the forecast • Conclusions 24 February 2015 NOAA Satellite Science Week 22
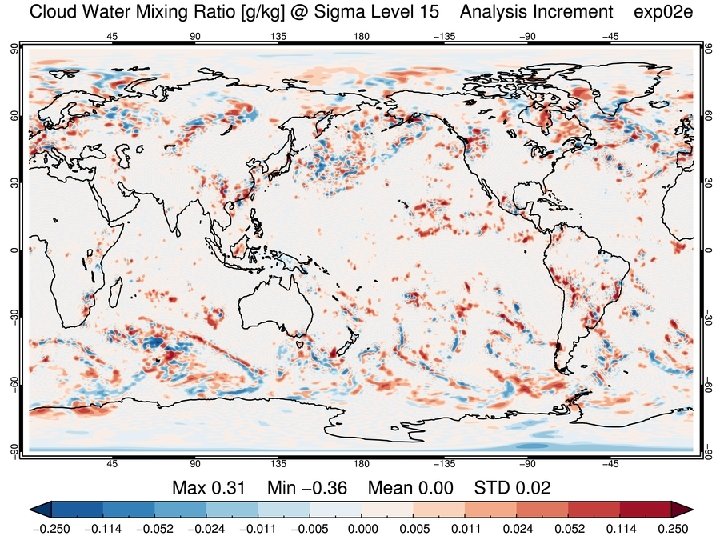
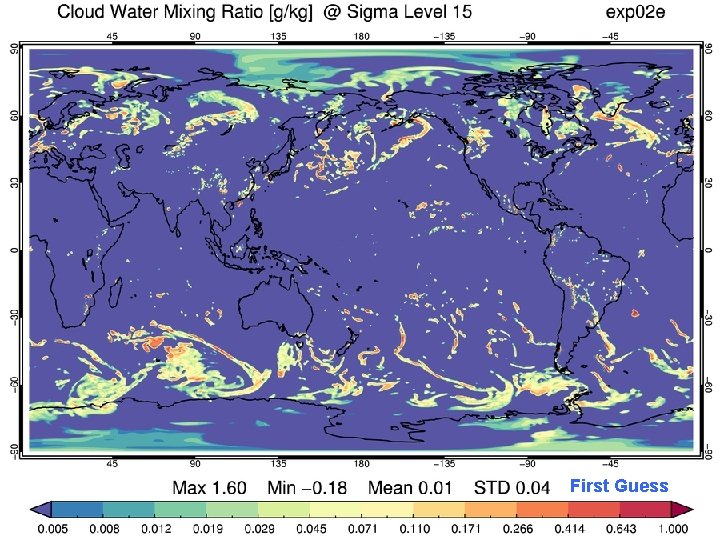
First Guess
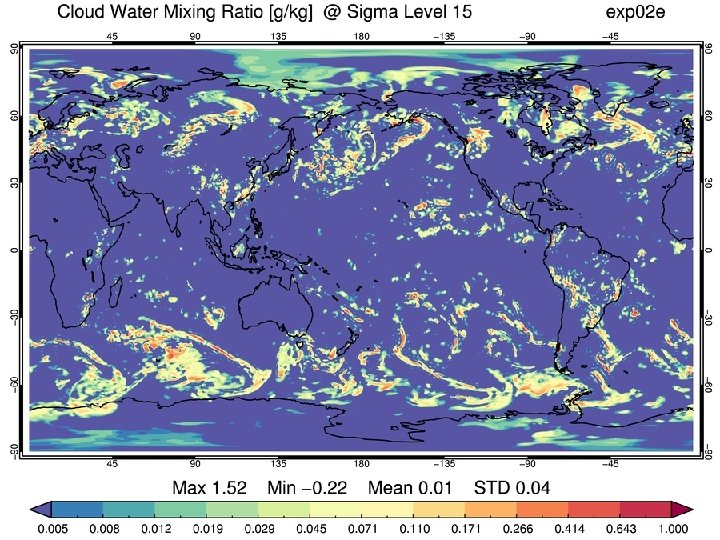
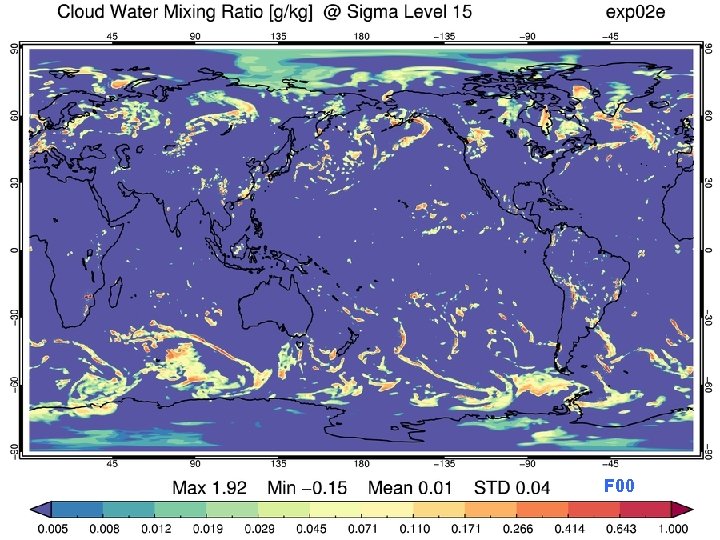
F 00
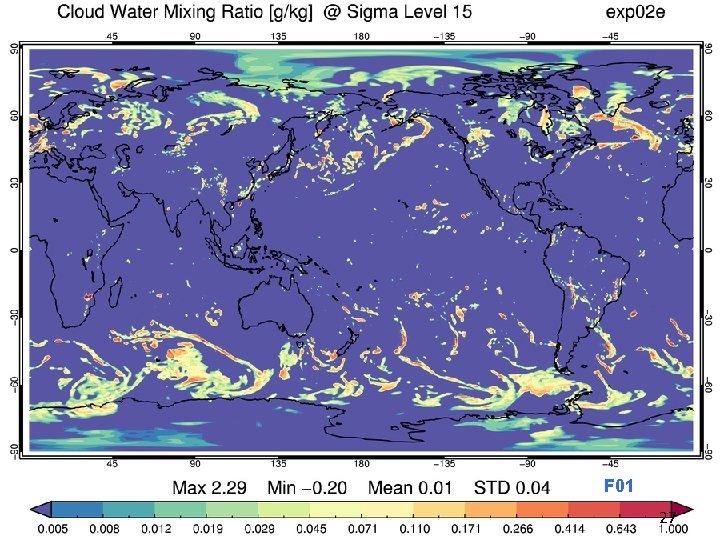
F 01 27
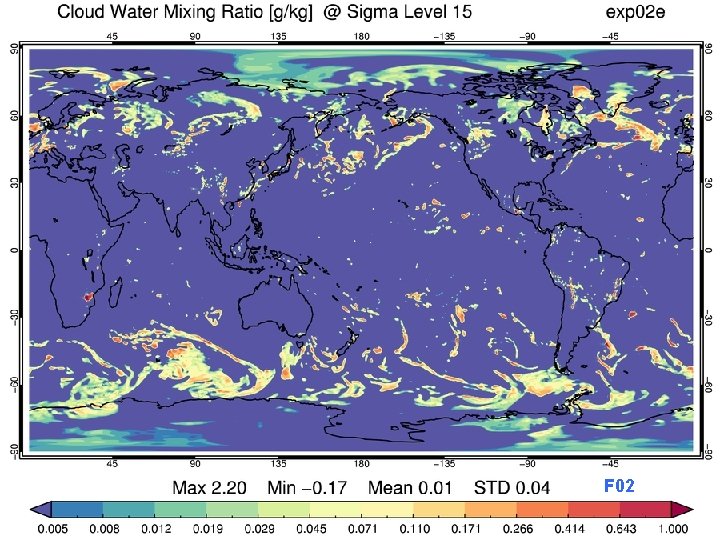
F 02
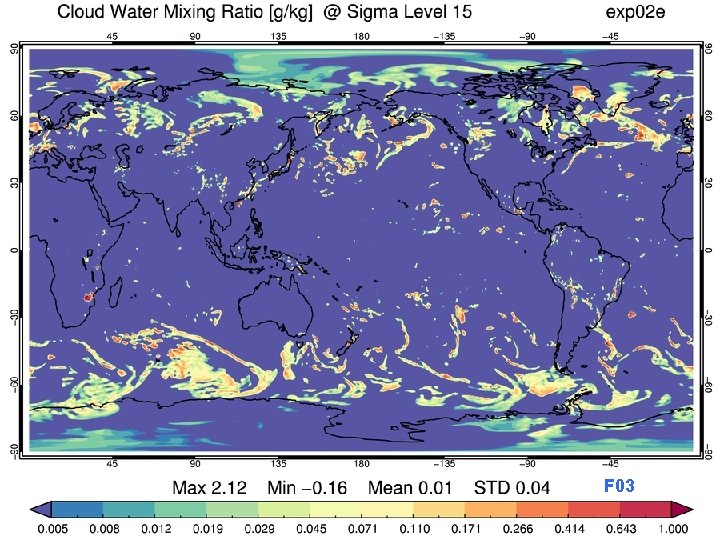
F 03
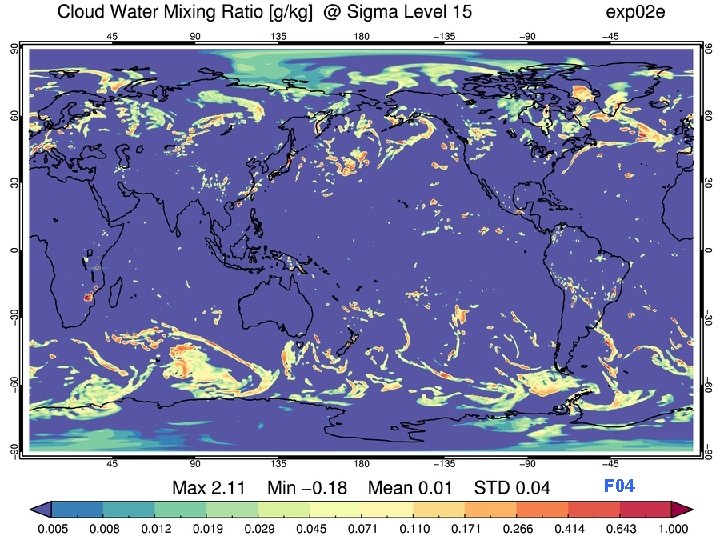
F 04
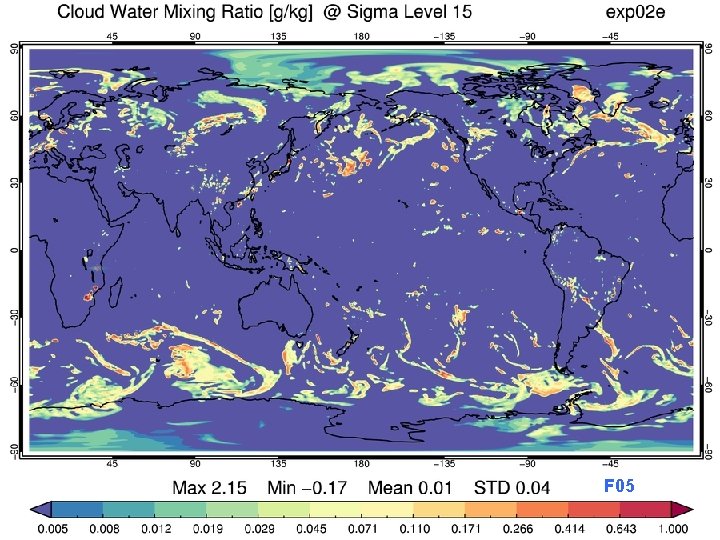
F 05
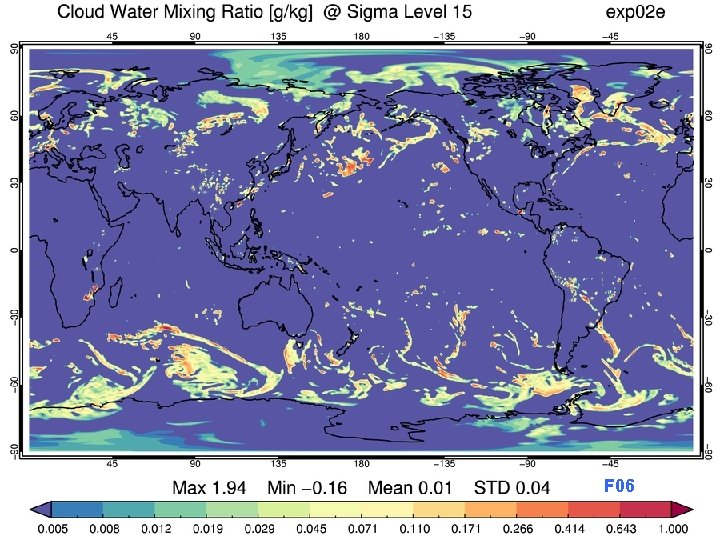
F 06
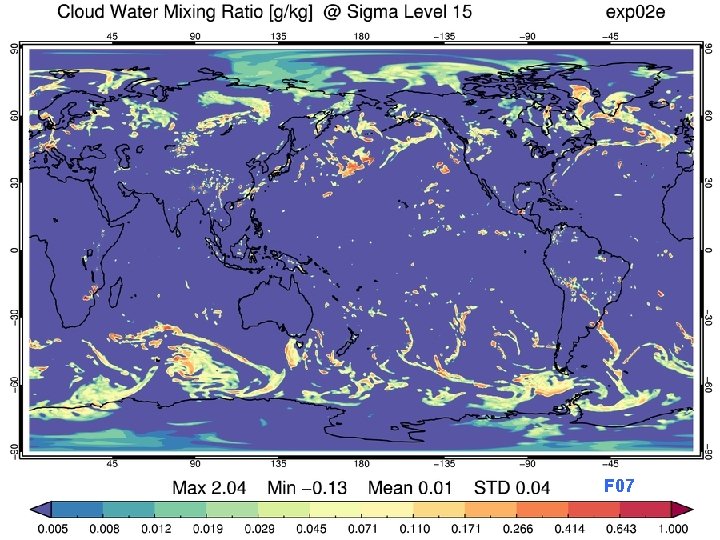
F 07
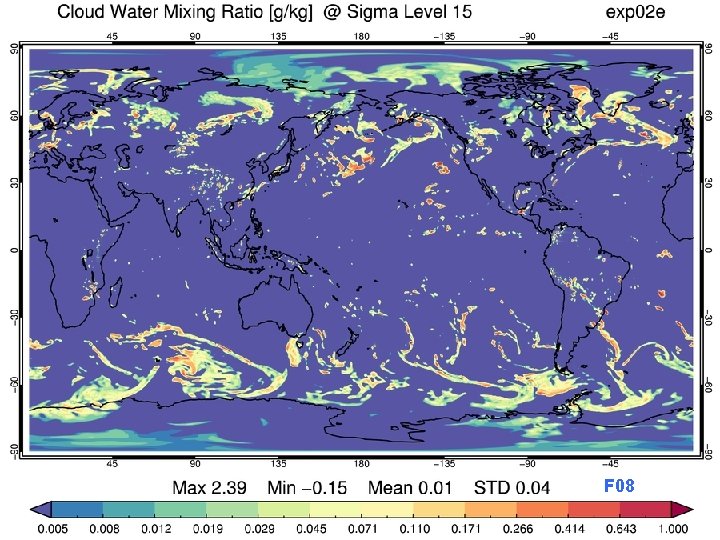
F 08
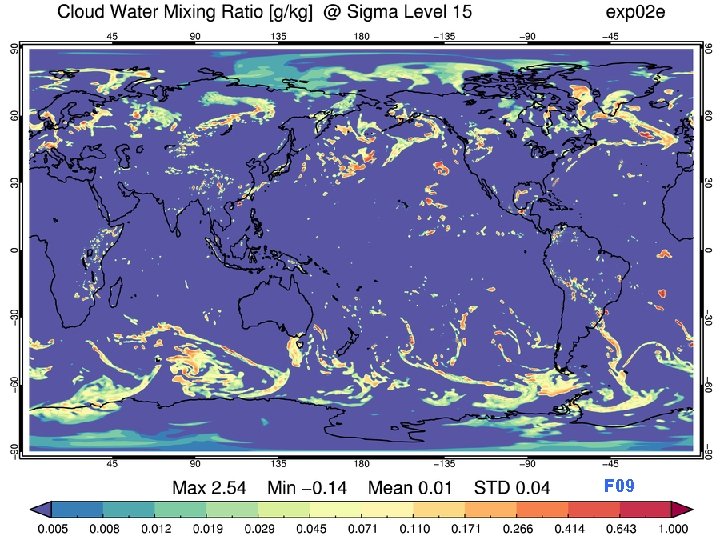
F 09
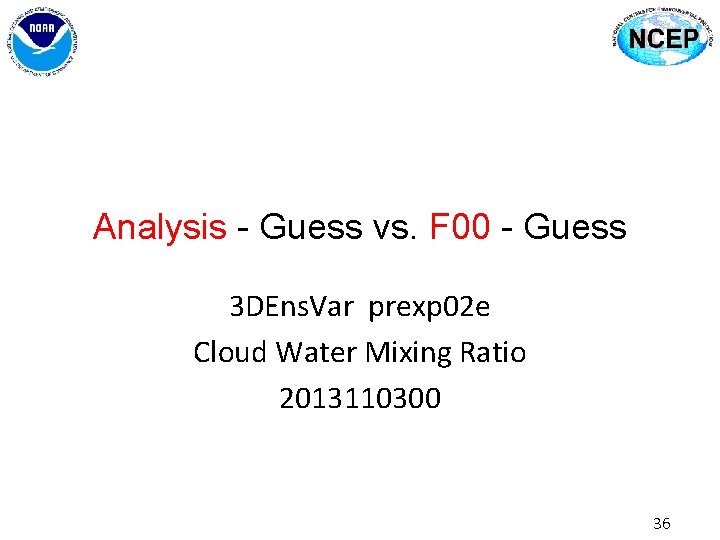
Analysis - Guess vs. F 00 - Guess 3 DEns. Var prexp 02 e Cloud Water Mixing Ratio 2013110300 36
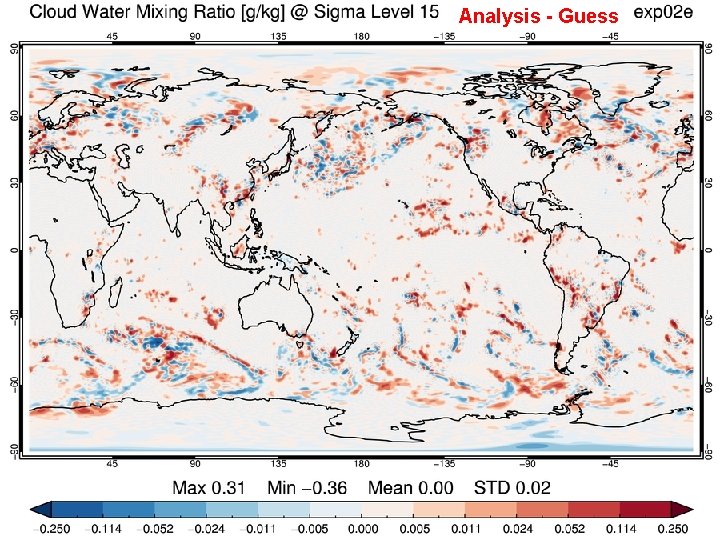
Analysis - Guess
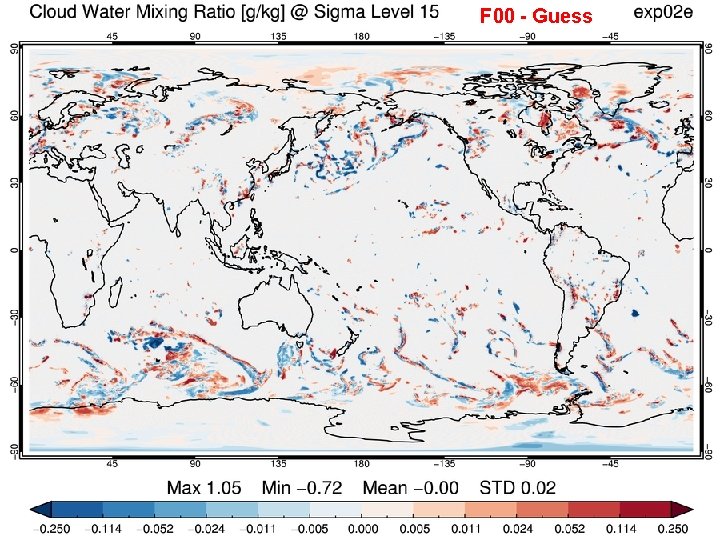
F 00 - Guess
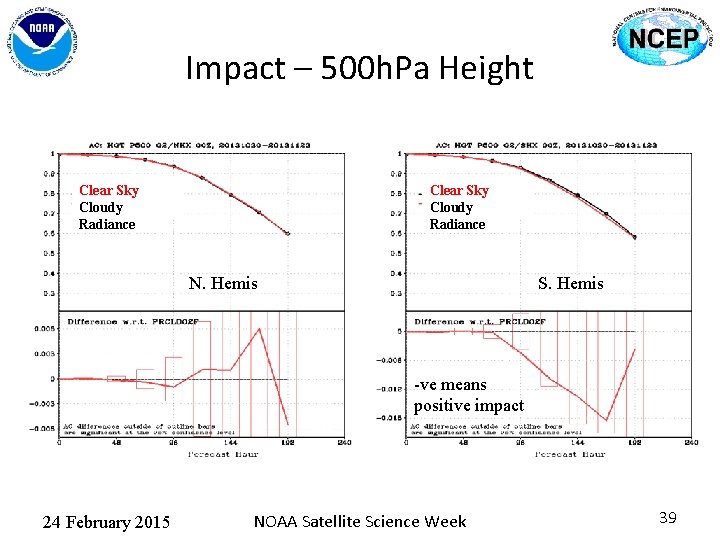
Impact – 500 h. Pa Height Clear Sky Cloudy Radiance N. Hemis S. Hemis -ve means positive impact 24 February 2015 NOAA Satellite Science Week 39
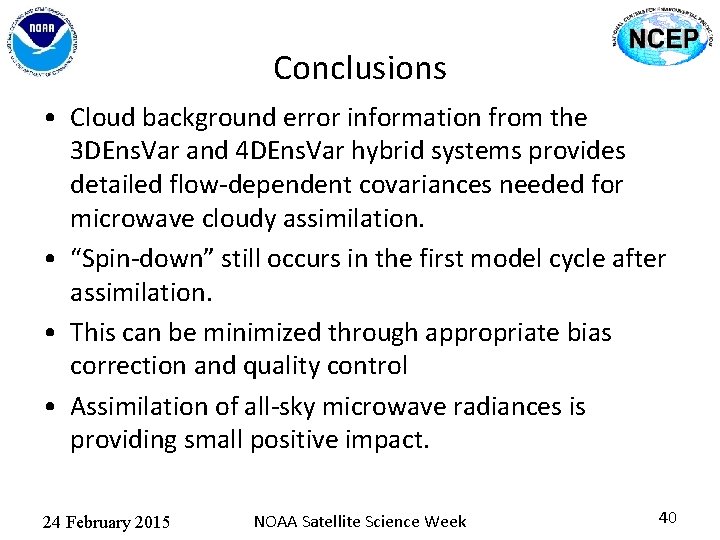
Conclusions • Cloud background error information from the 3 DEns. Var and 4 DEns. Var hybrid systems provides detailed flow-dependent covariances needed for microwave cloudy assimilation. • “Spin-down” still occurs in the first model cycle after assimilation. • This can be minimized through appropriate bias correction and quality control • Assimilation of all-sky microwave radiances is providing small positive impact. 24 February 2015 NOAA Satellite Science Week 40
Satellite microwave transmission
Physical progress and financial progress
Jedi data assimilation
Data assimilation
Data assimilation tutorial
Data assimilation
Data assimilation
Data assimilation
Data assimilation
Data assimilation
Windy
Alliteration simile metaphor personification onomatopoeia
Windy cloudy rainy sunny
Bayes net toolbox for matlab
Grey cloudy discharge
Grey cloudy discharge
What is a mixture in chemistry
Examples of suspension
Glomerulonephrotis
Glaucoma classification
Characteristics of normal urine
Nso satellite office
Ap psychology unit 7
Regressive assimilation examples
Progressive assimilation
Linking vowel to vowel examples
Examples of carbohydrase
Example of assimilation in child development
Dissimilation rules
Assimilation vs accommodation
Example of phonology
Phonetics
Phonological processes
Ch
Assimilation linguistics
4 parts of digestive system
Regressive assimilation examples
Elision examples phonetics
Assimilation ap psychology
Assimilation in phonology
Nitrifying bacteria convert _____ to _____.