Logistics Network Configuration Designing Managing the Supply Chain
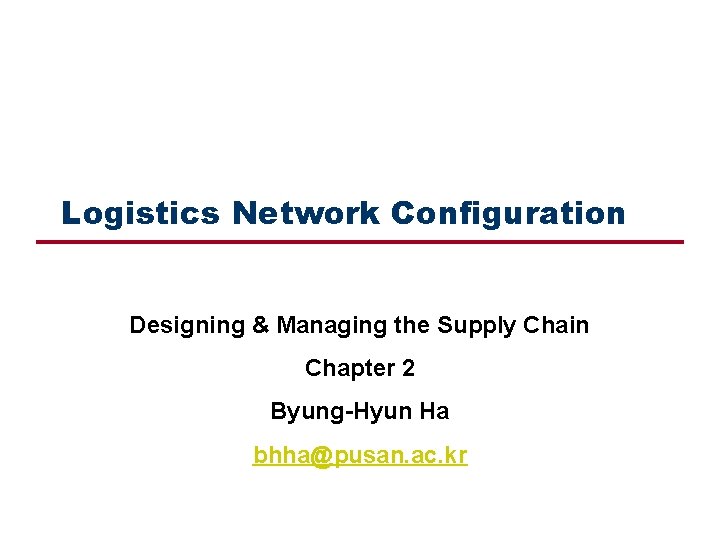
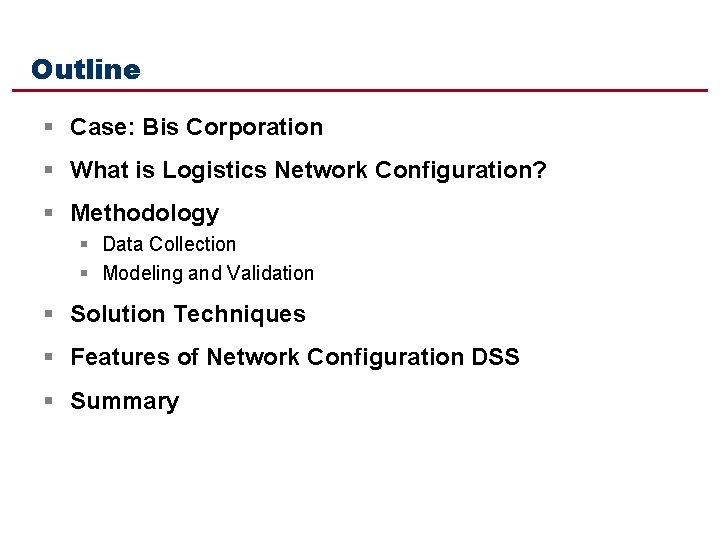
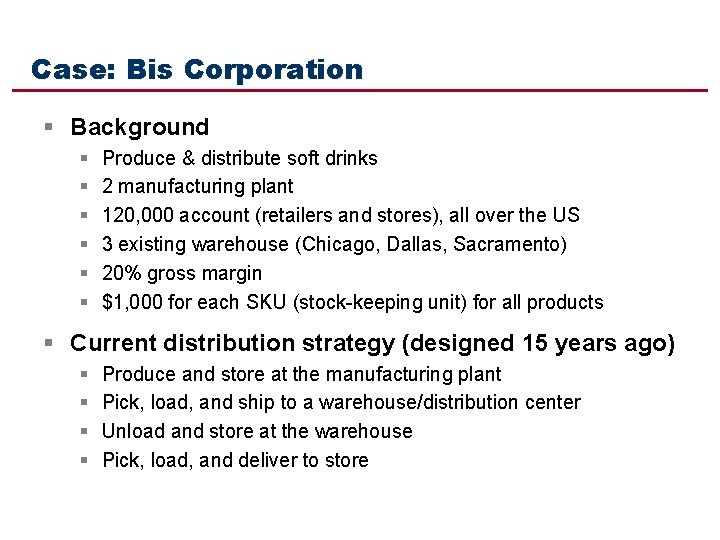
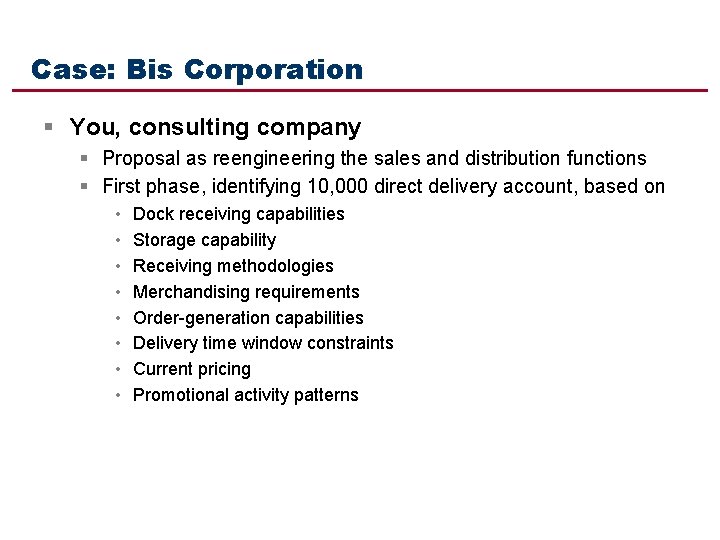
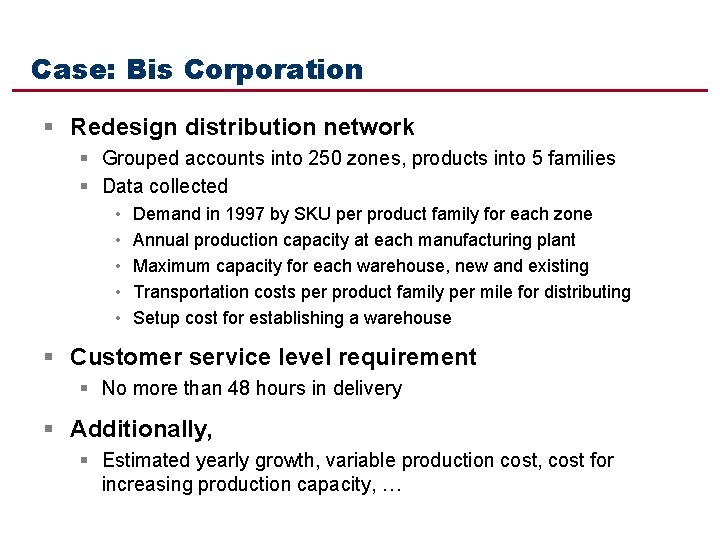
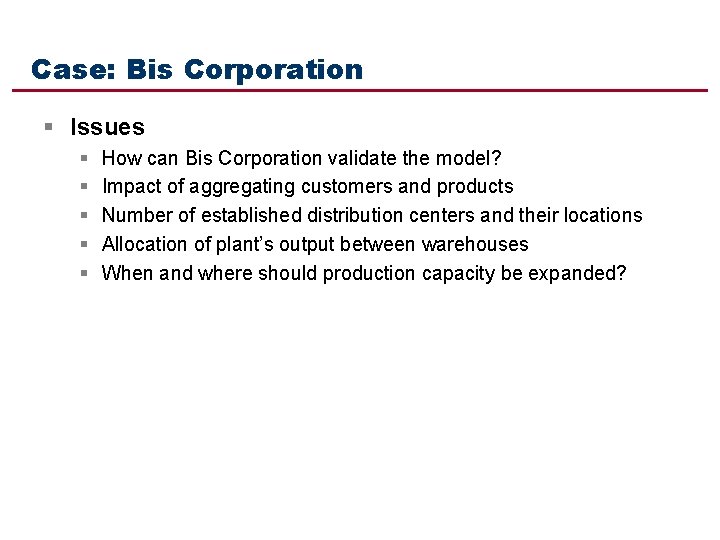
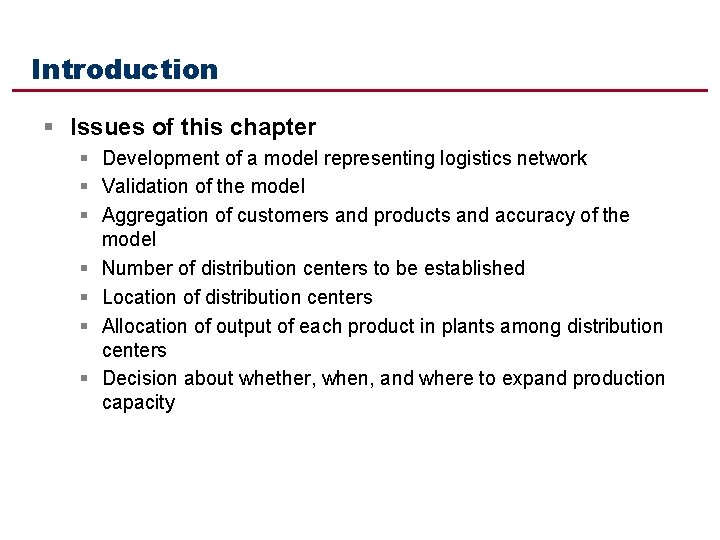
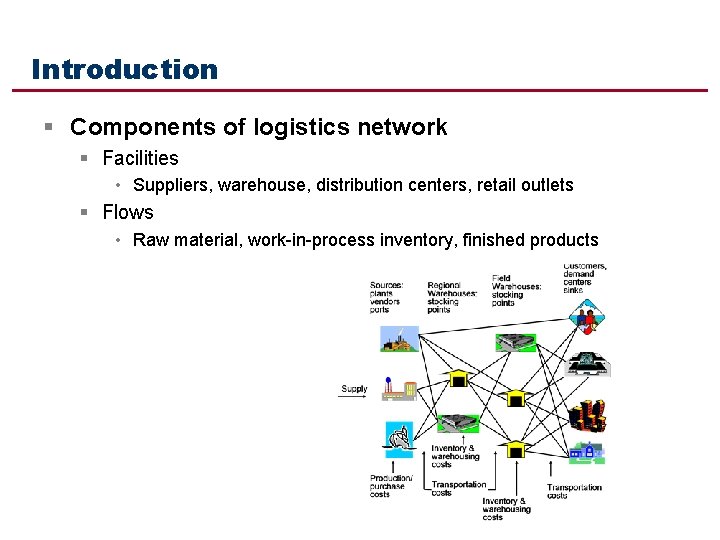
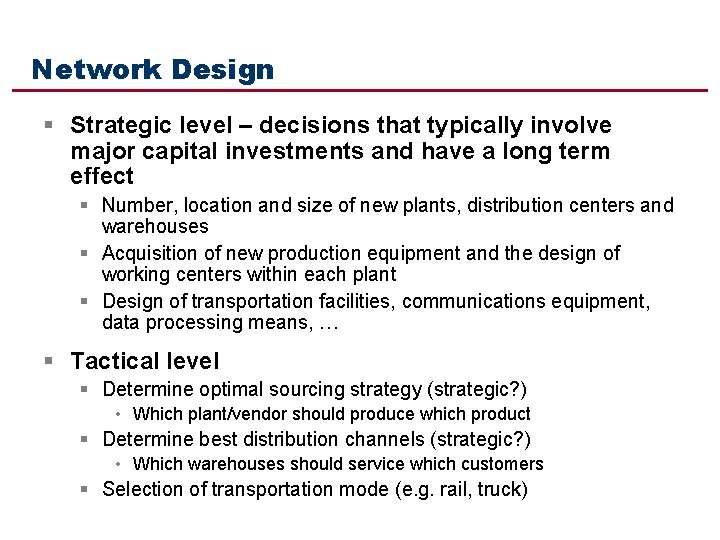
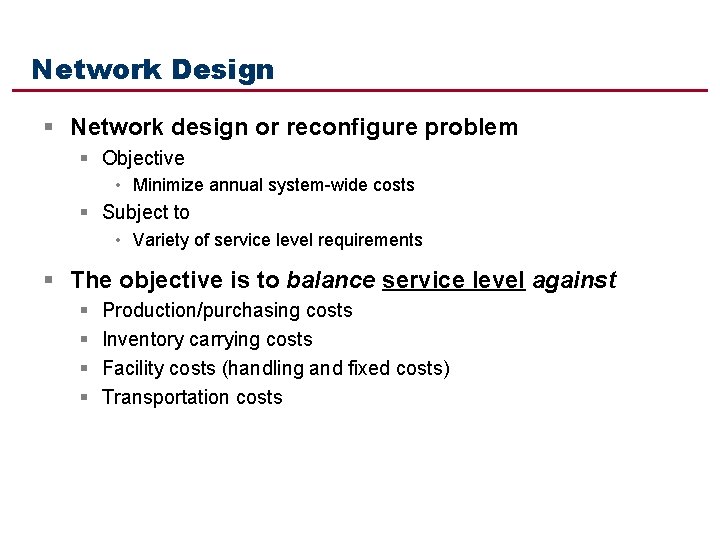
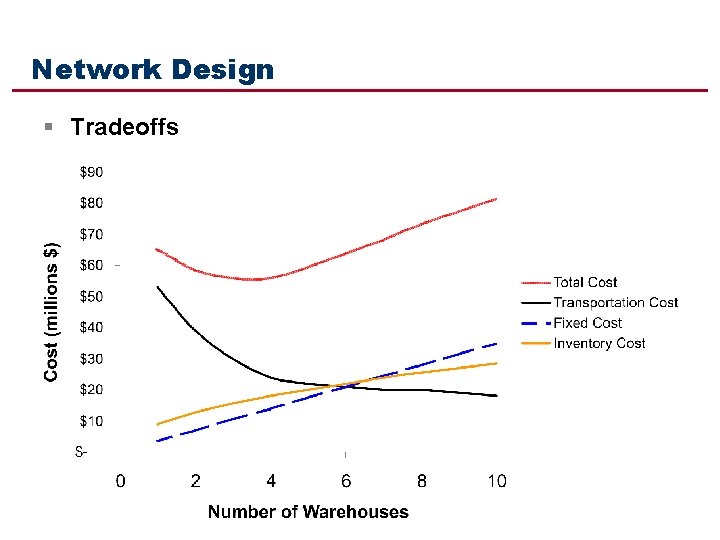
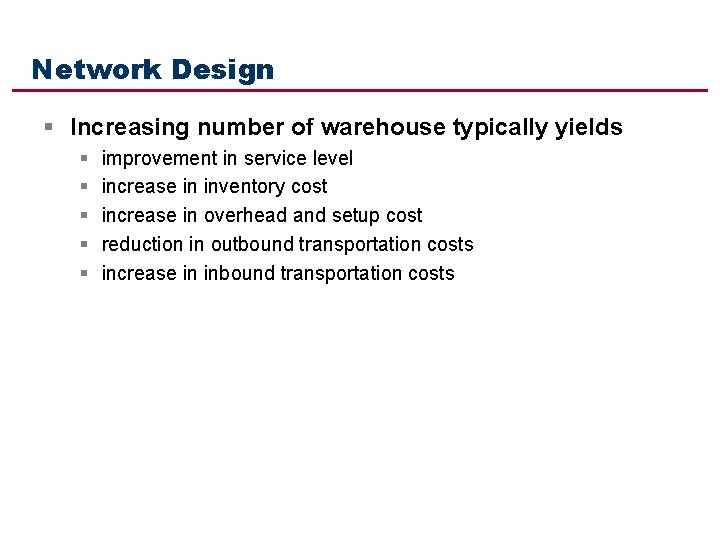
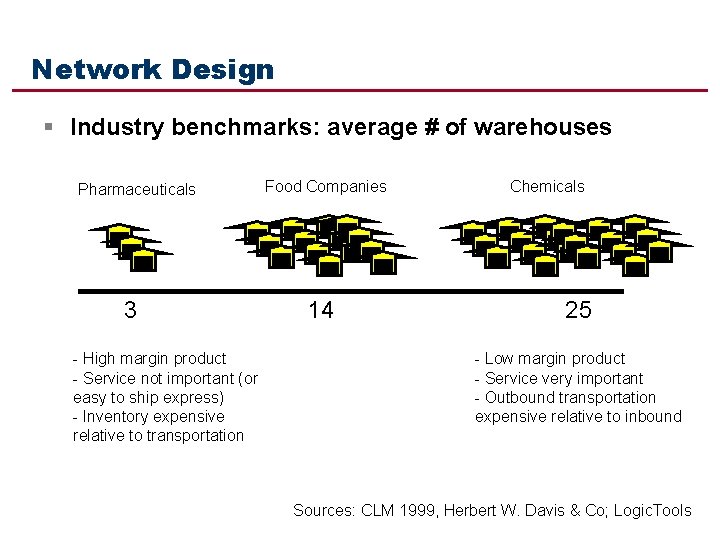
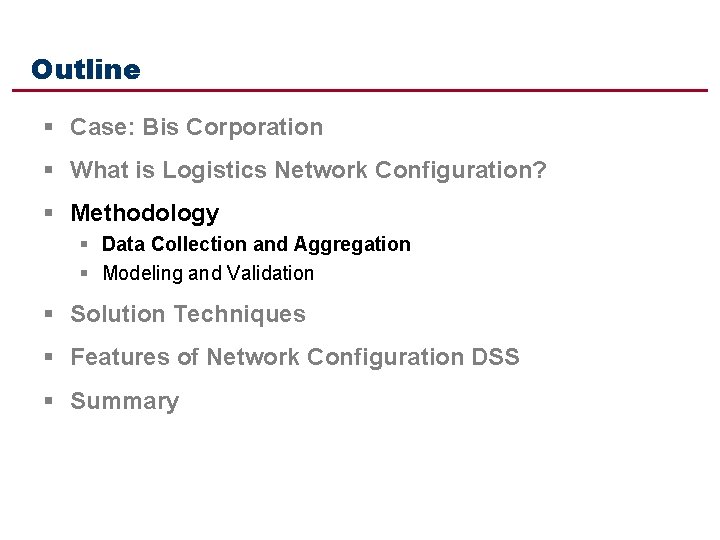
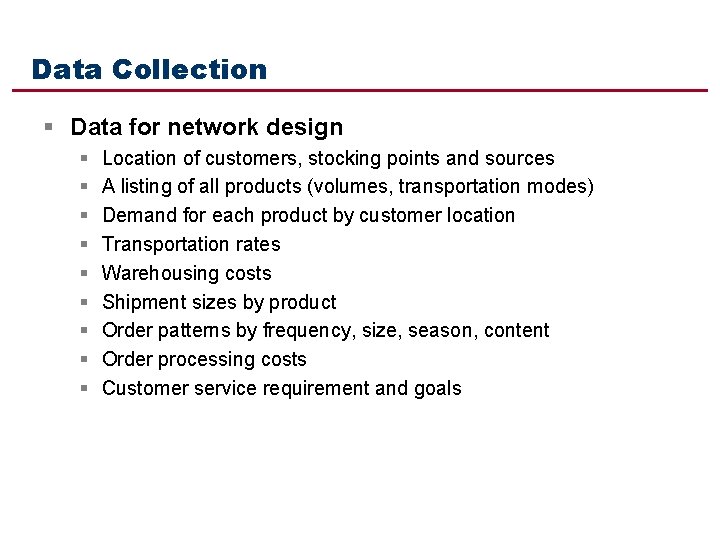
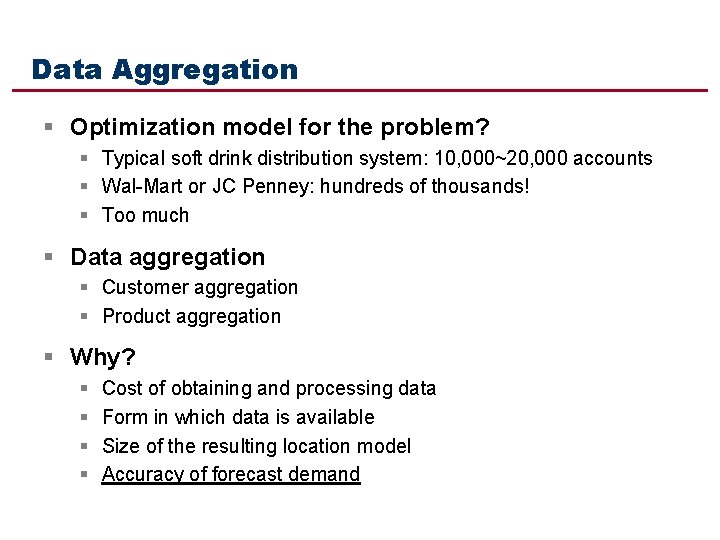
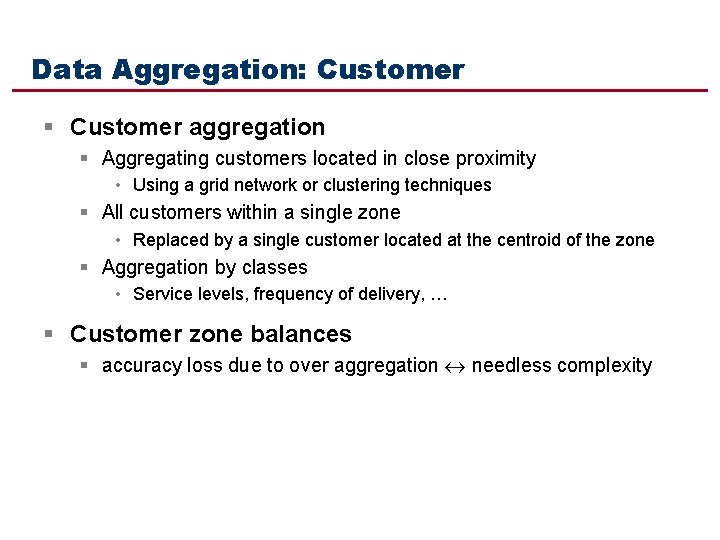
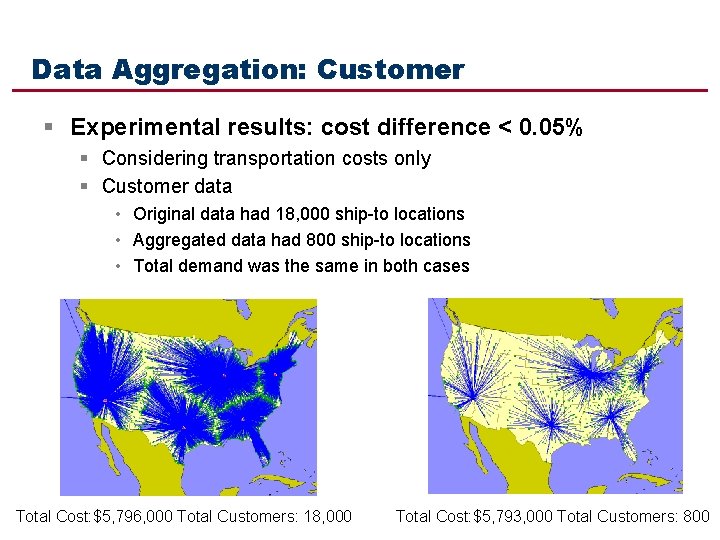
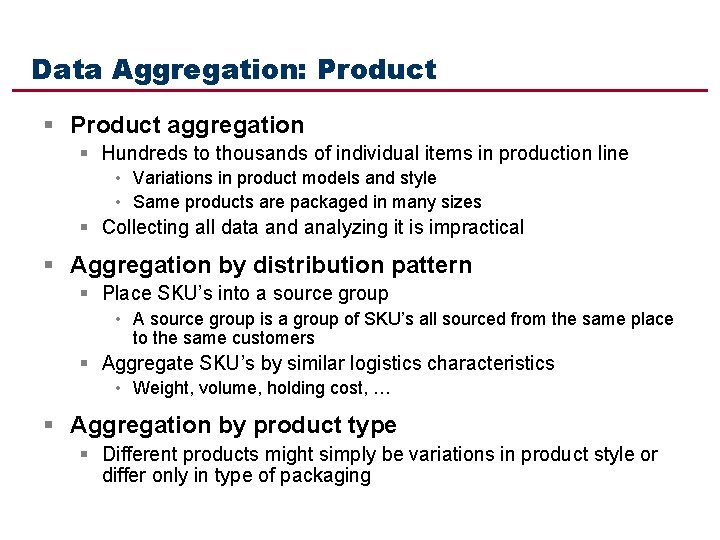
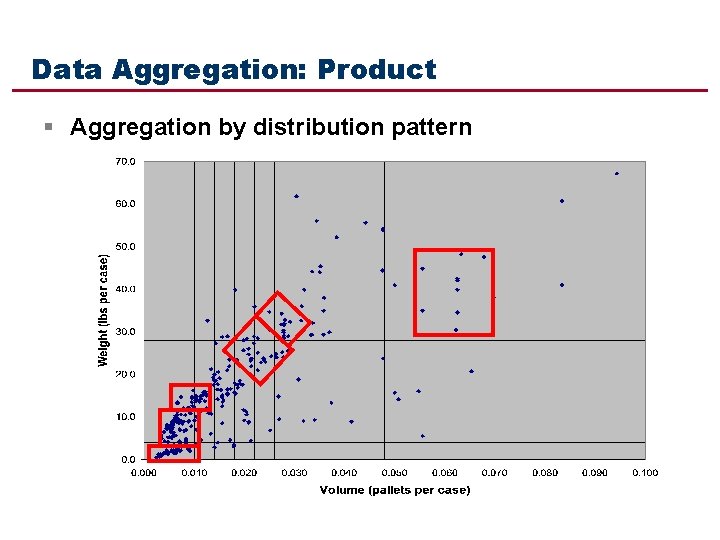
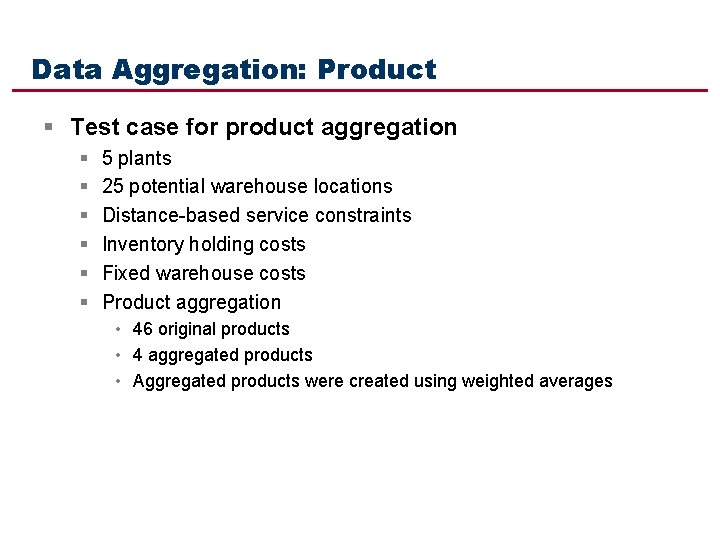
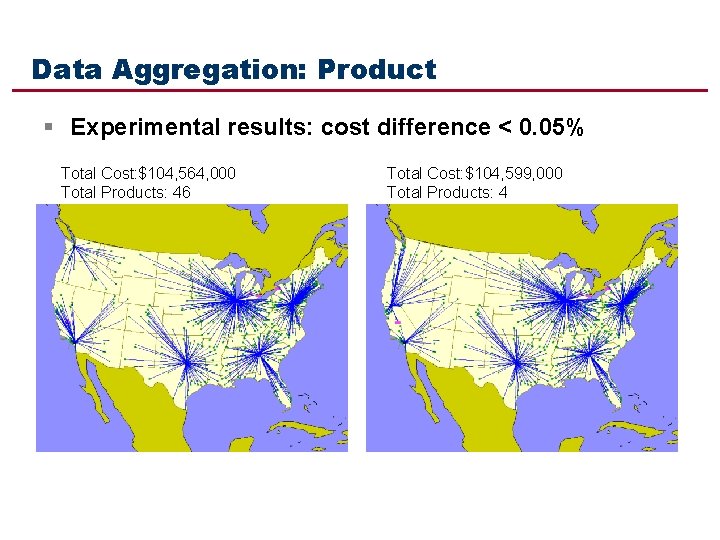
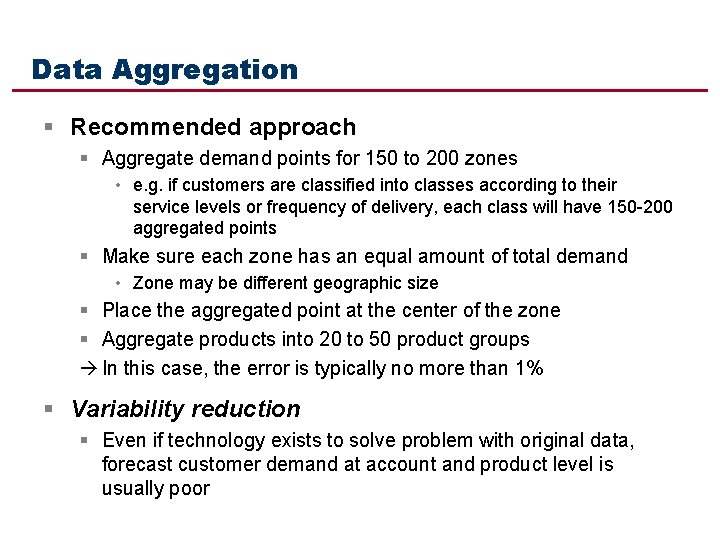
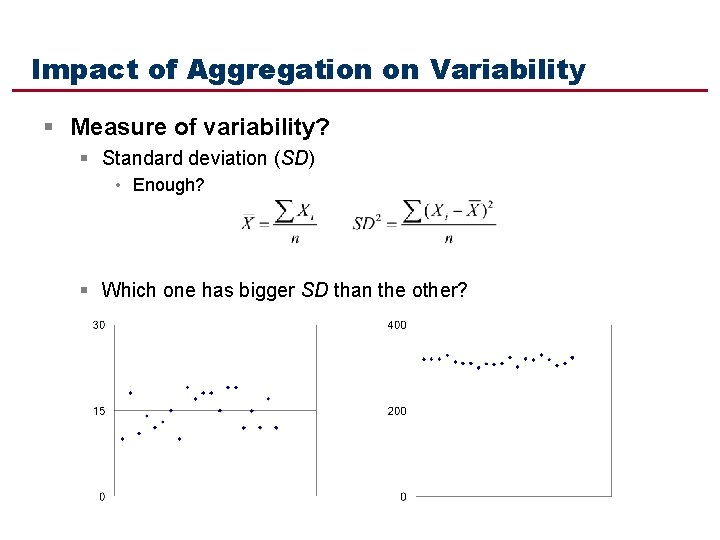
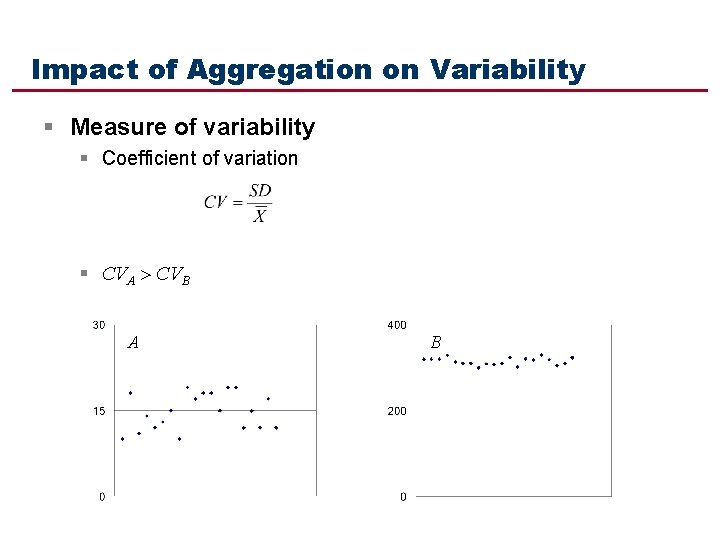
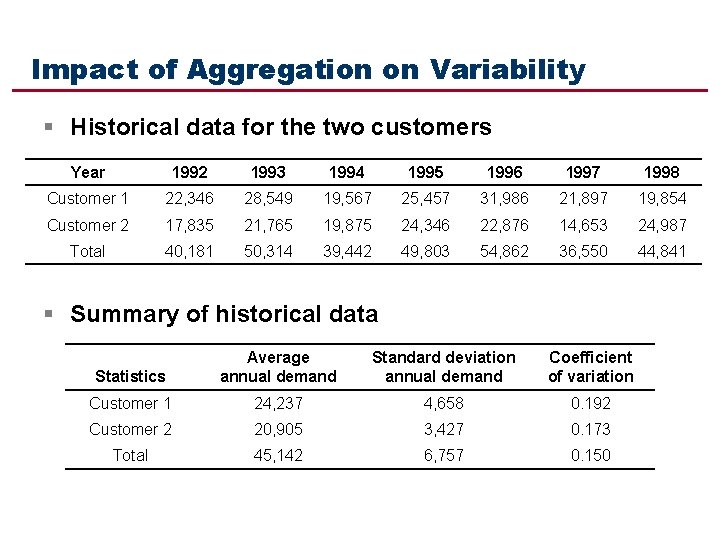
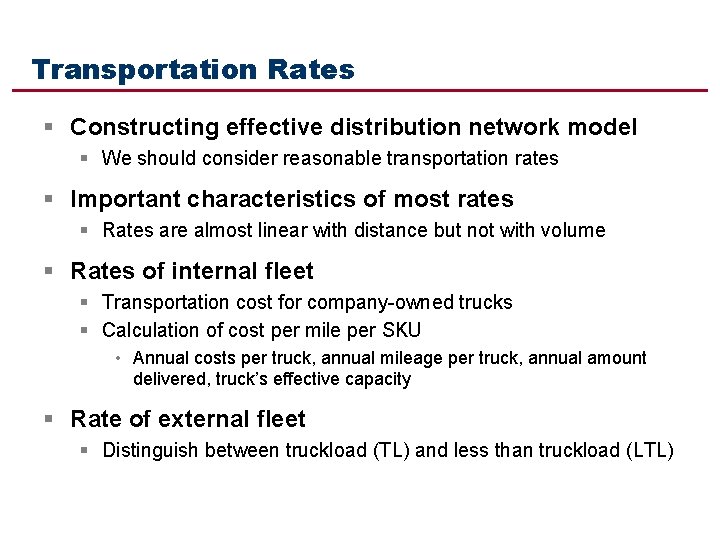
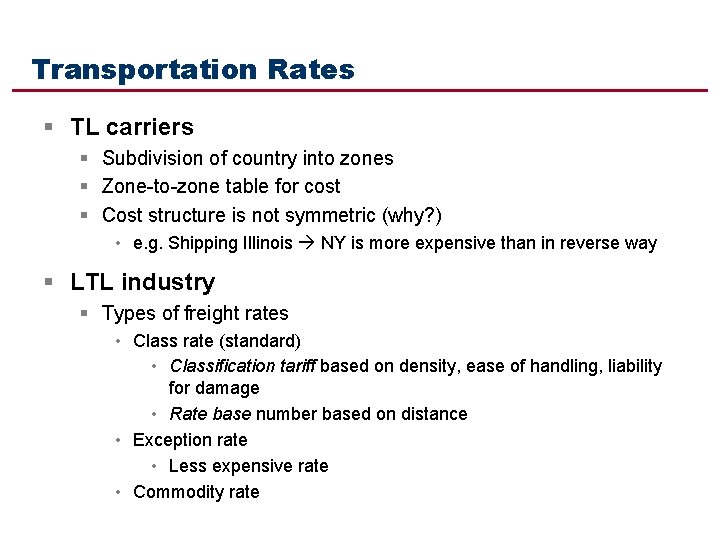
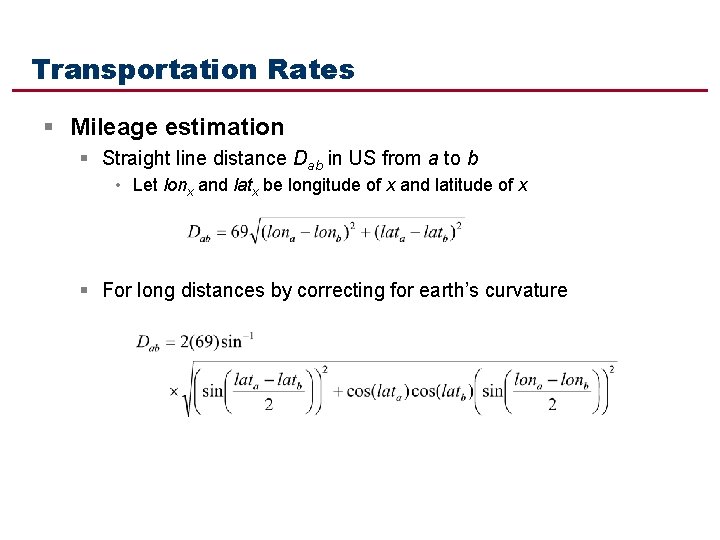
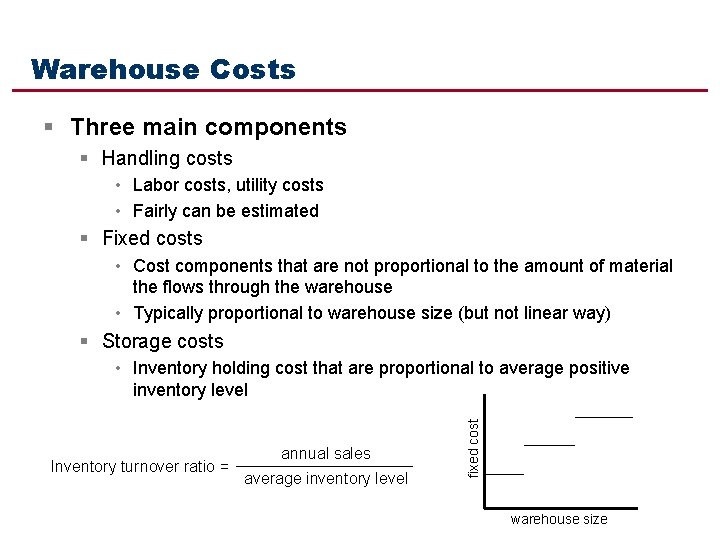
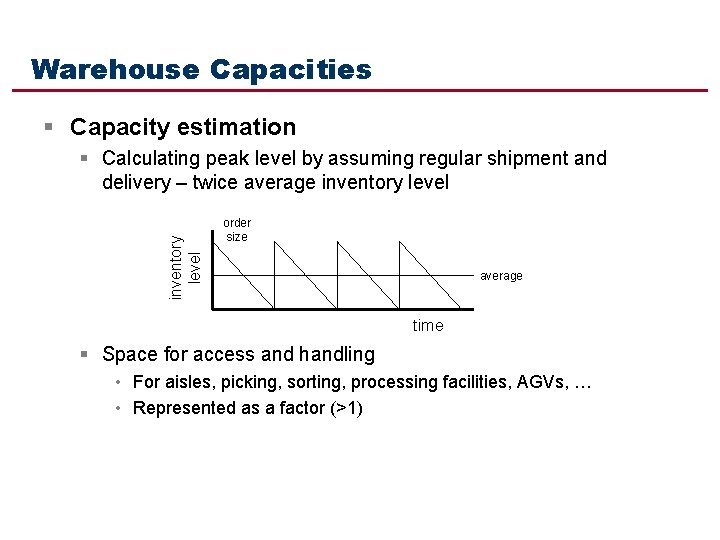
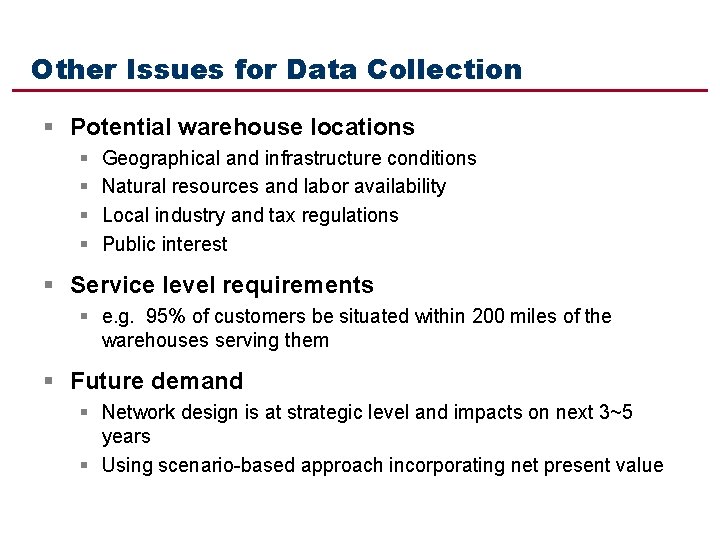
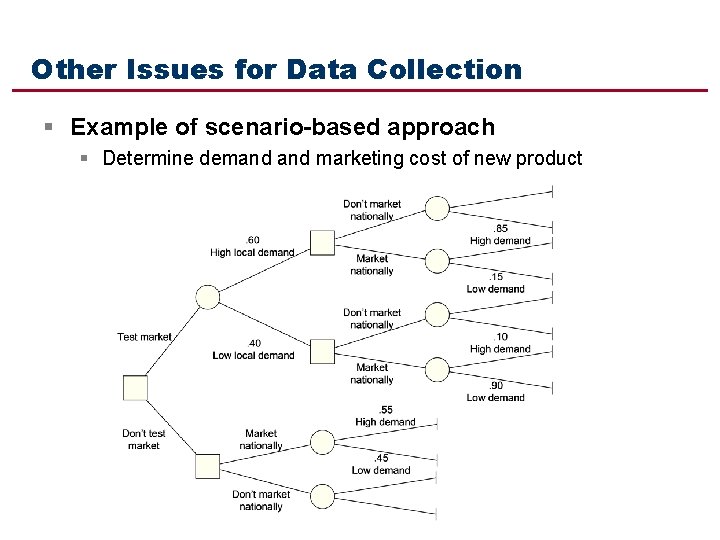
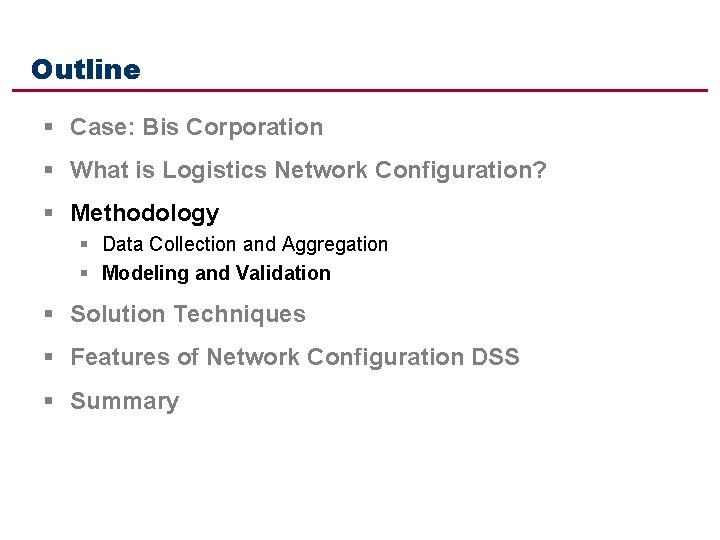
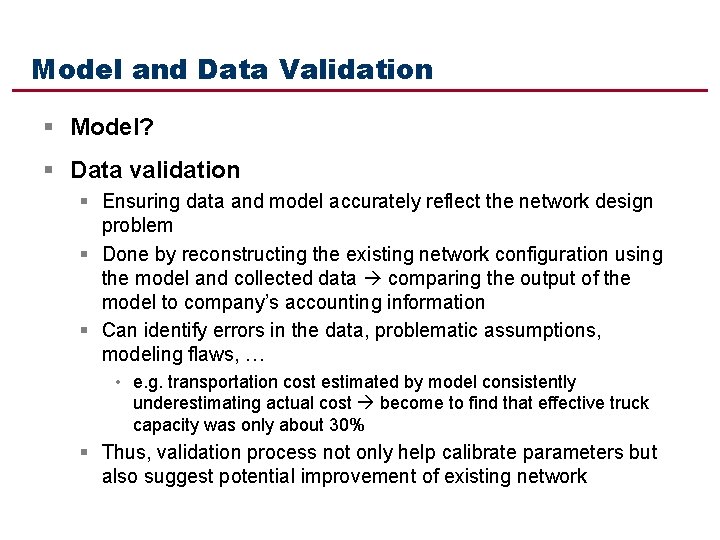
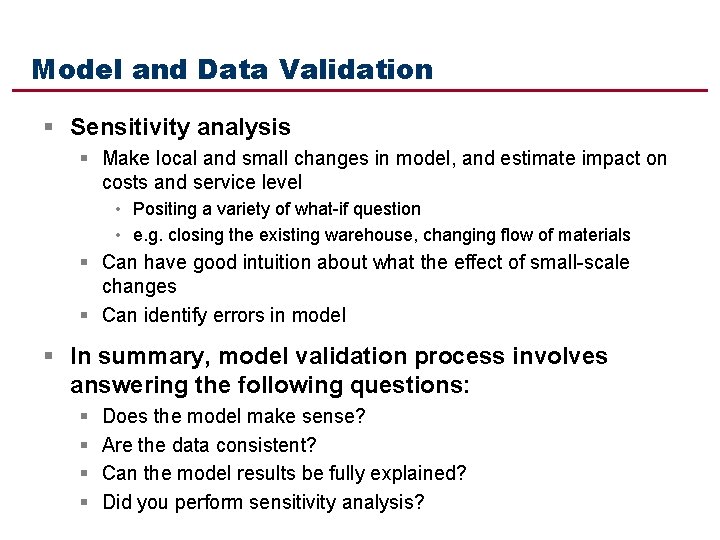
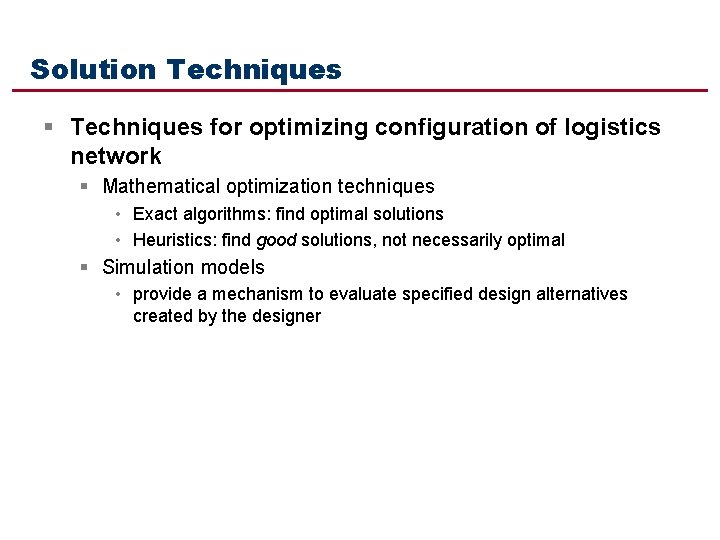
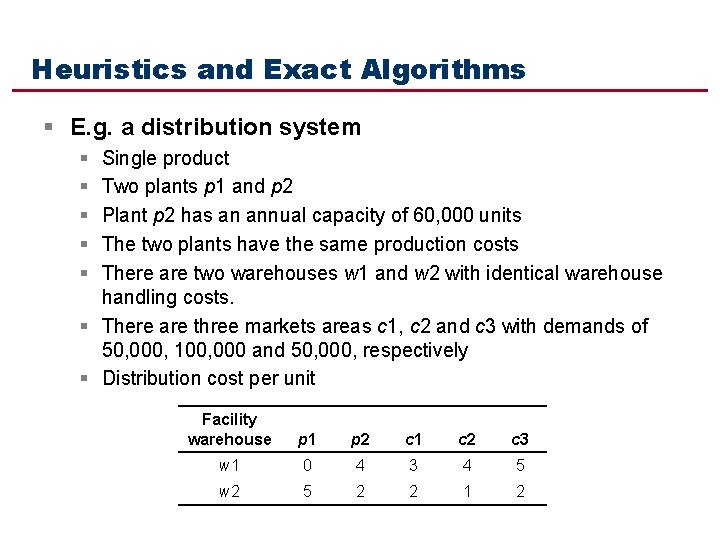
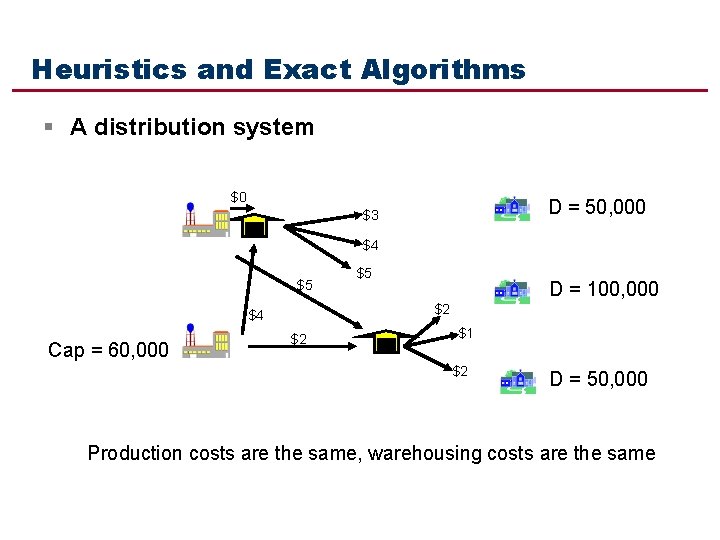
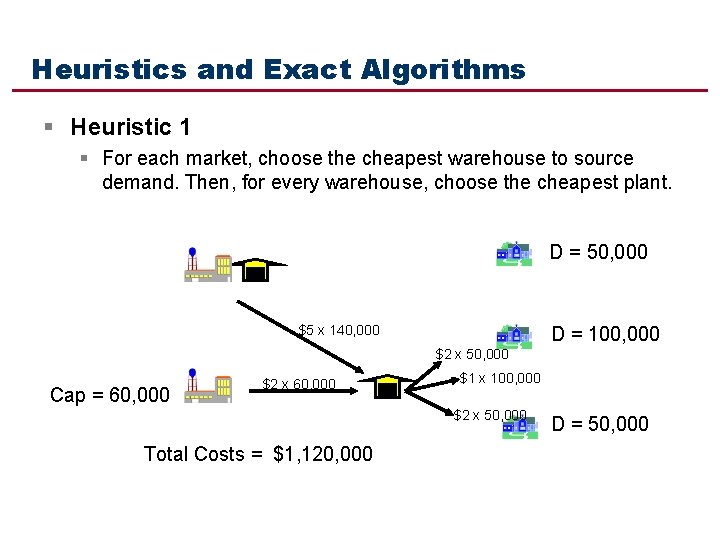
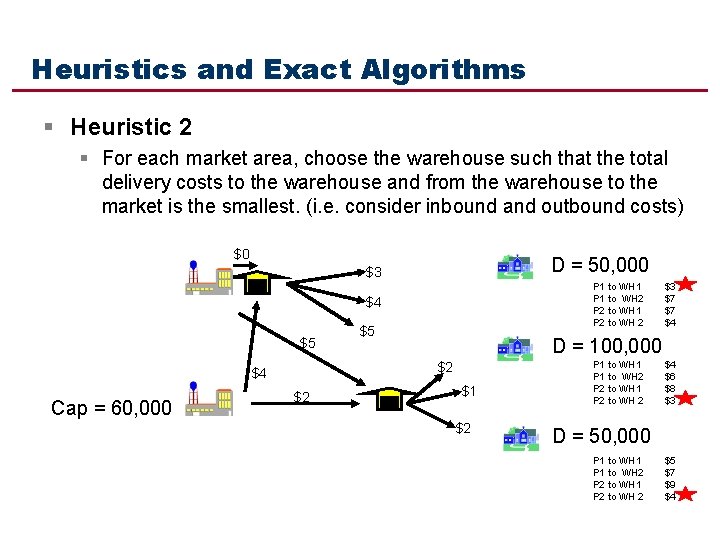
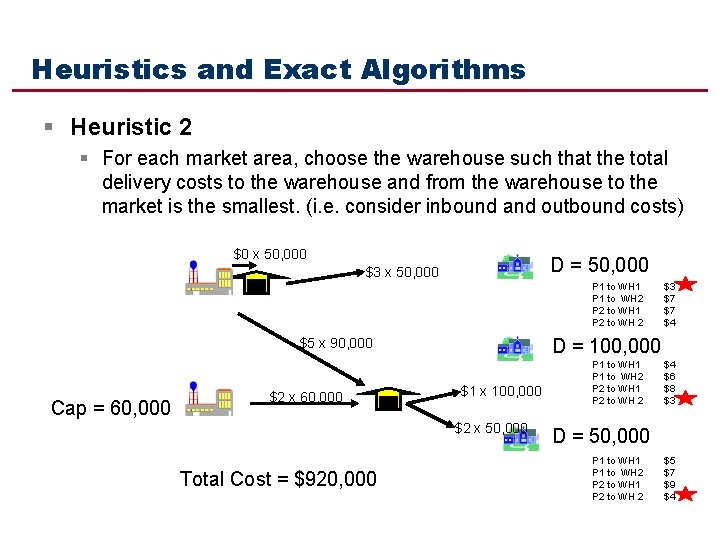
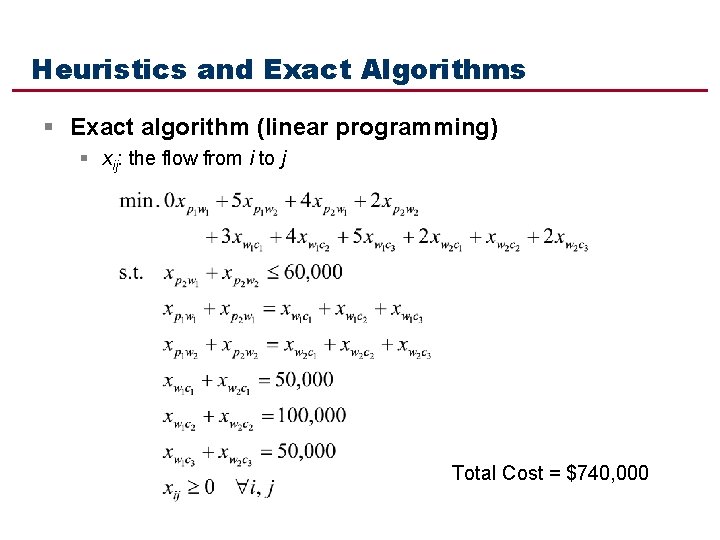
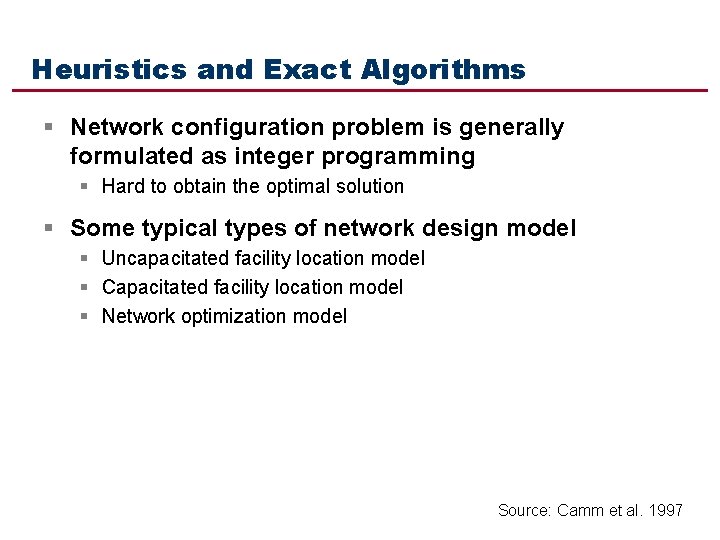
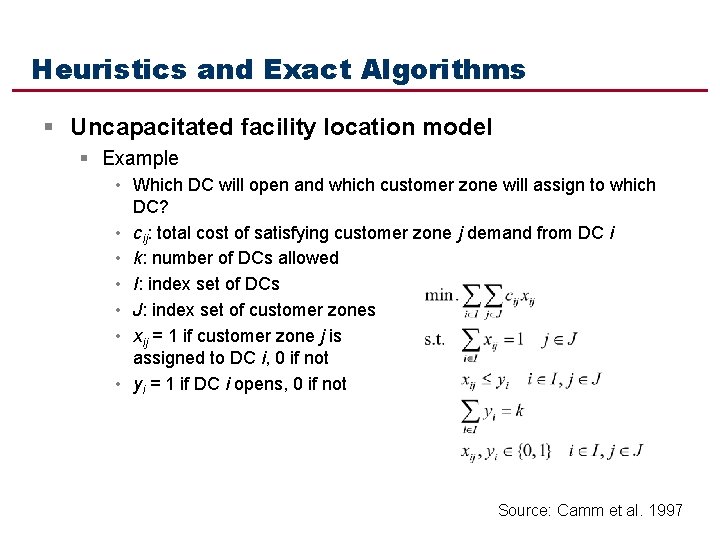
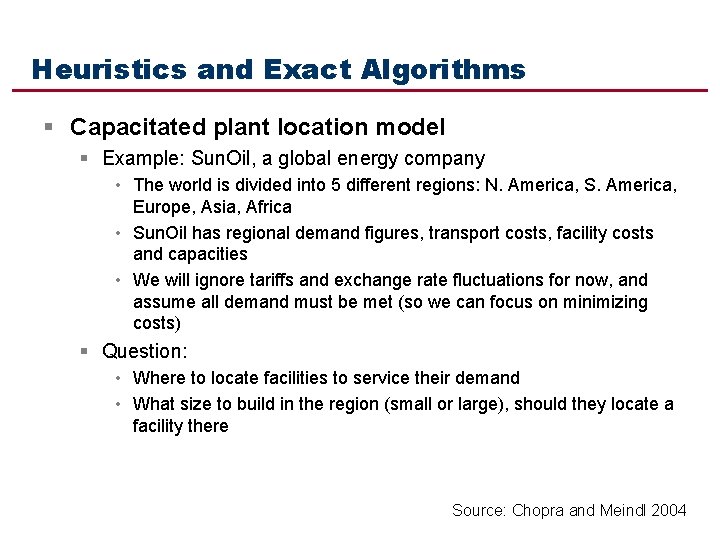
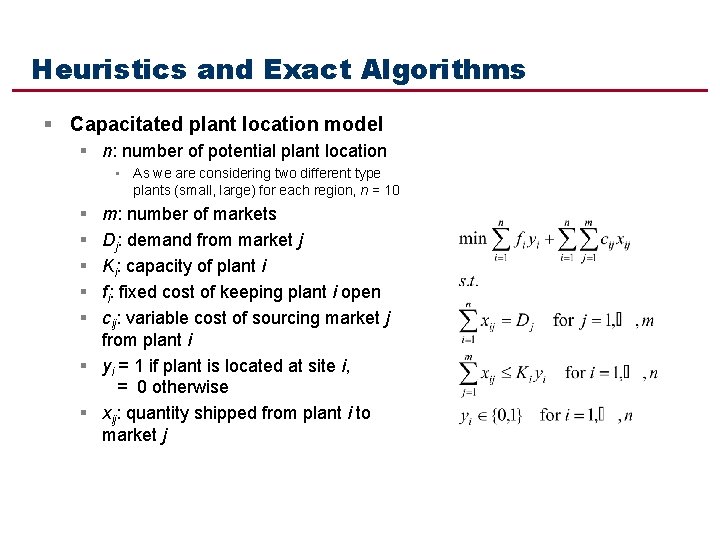
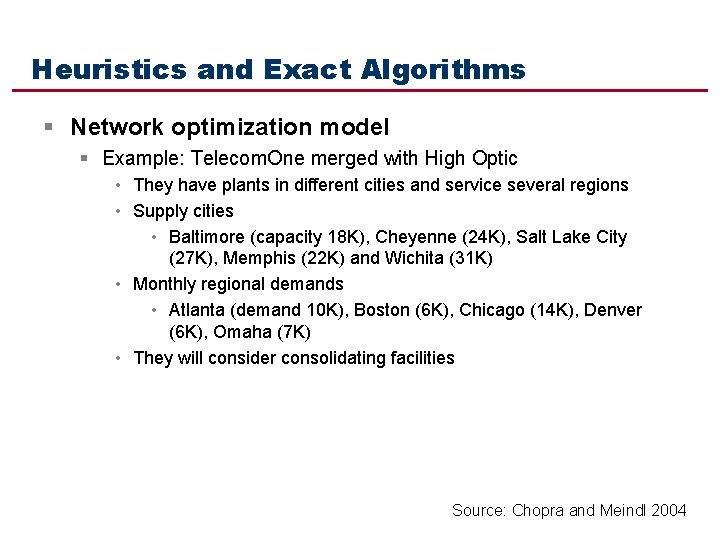
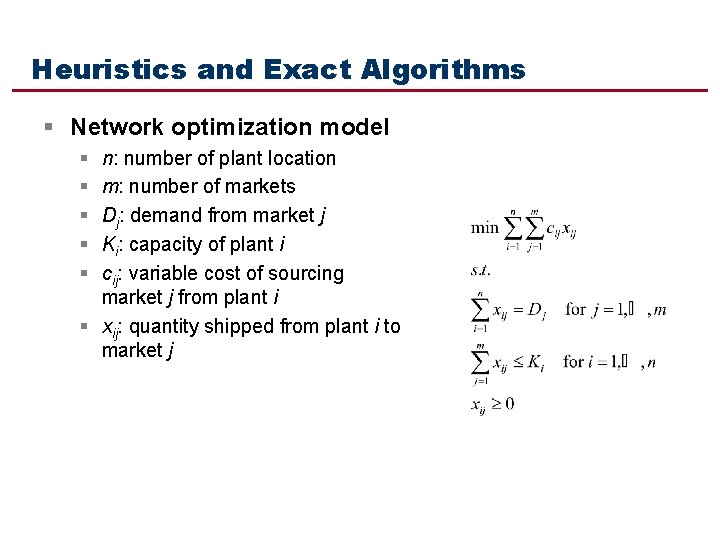
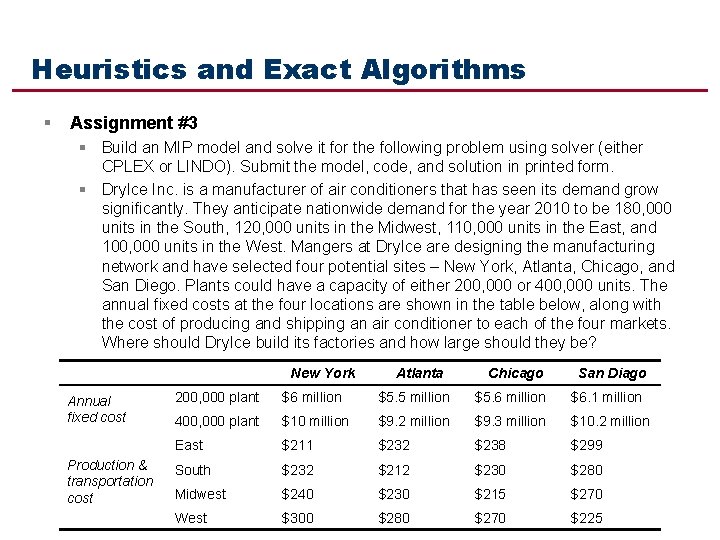
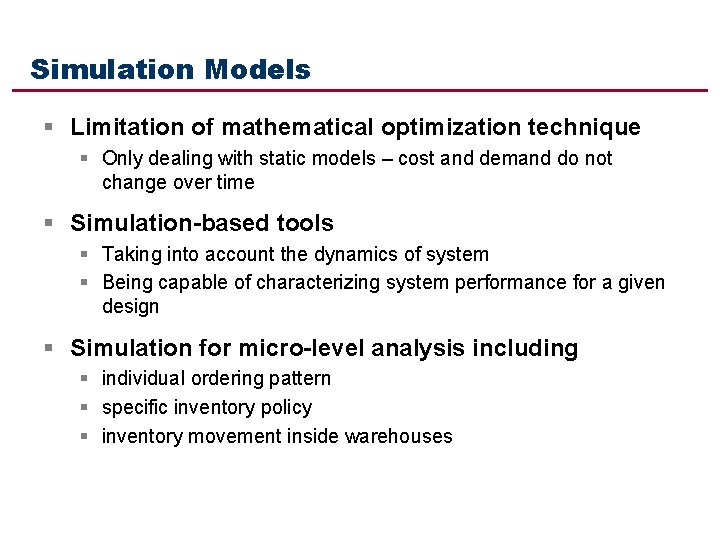
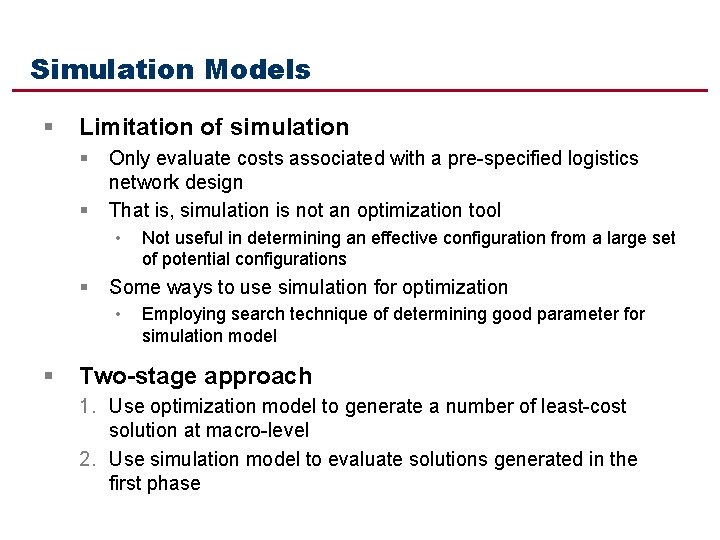
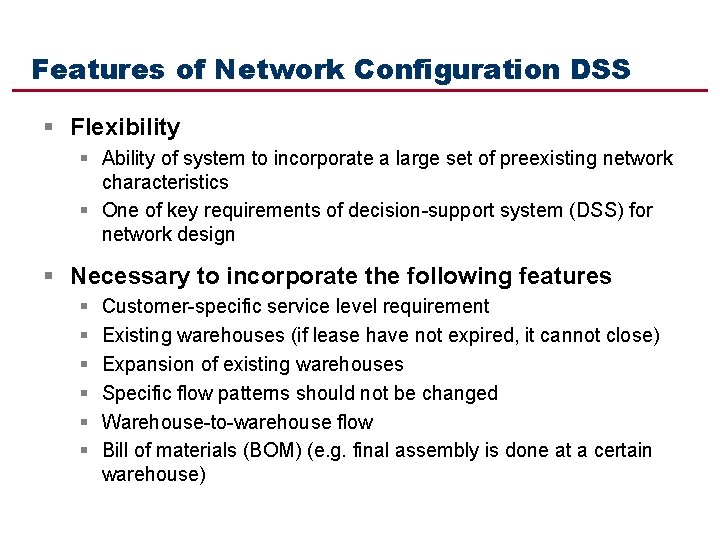
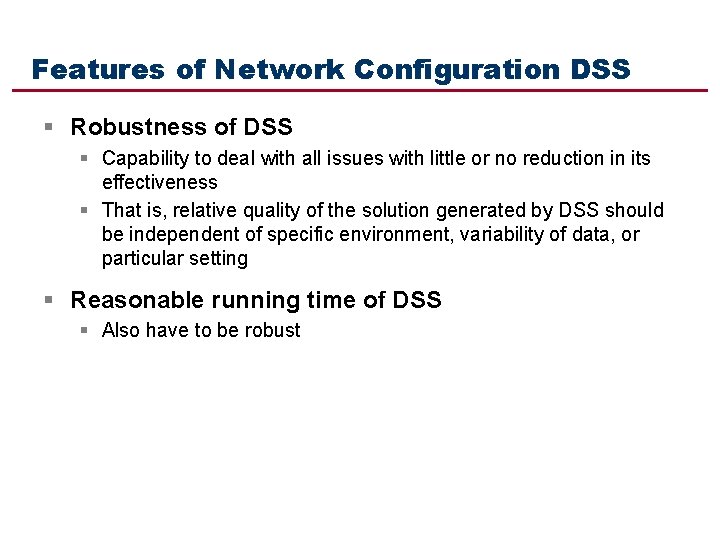
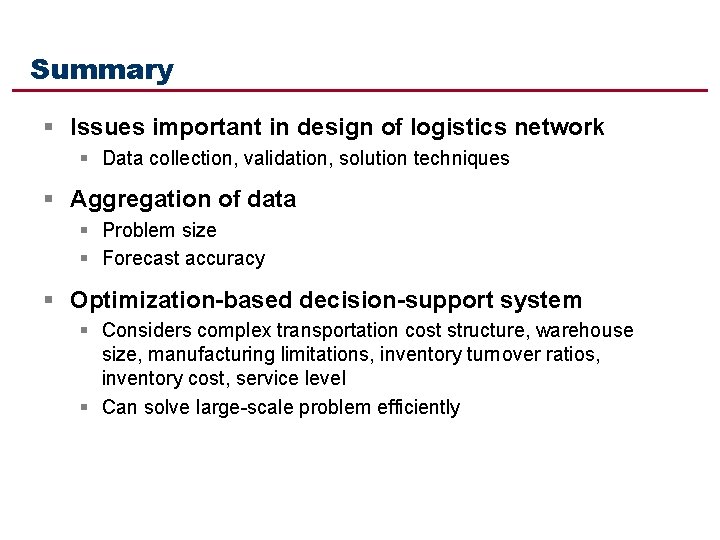
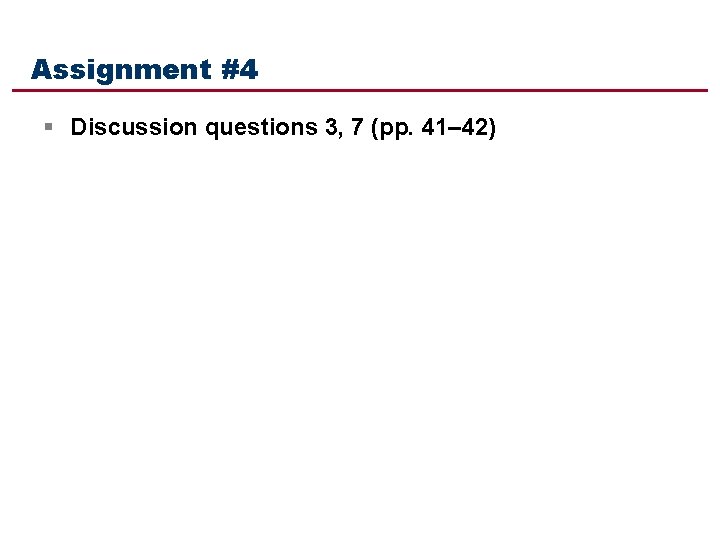
- Slides: 56
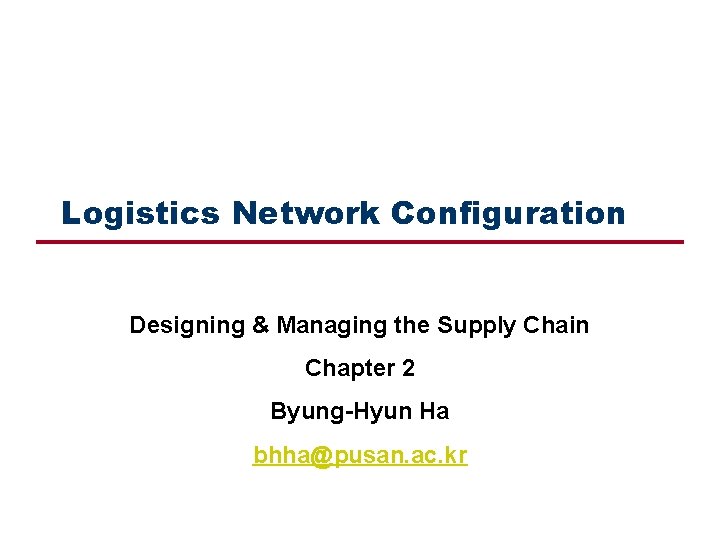
Logistics Network Configuration Designing & Managing the Supply Chain Chapter 2 Byung-Hyun Ha bhha@pusan. ac. kr
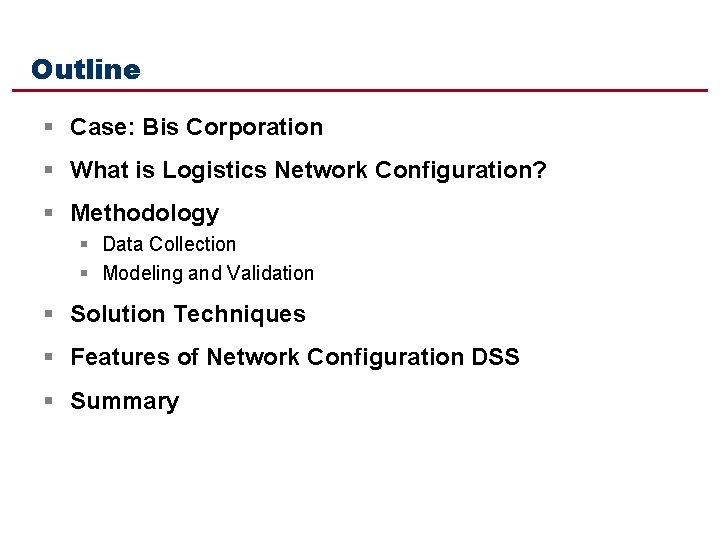
Outline § Case: Bis Corporation § What is Logistics Network Configuration? § Methodology § Data Collection § Modeling and Validation § Solution Techniques § Features of Network Configuration DSS § Summary
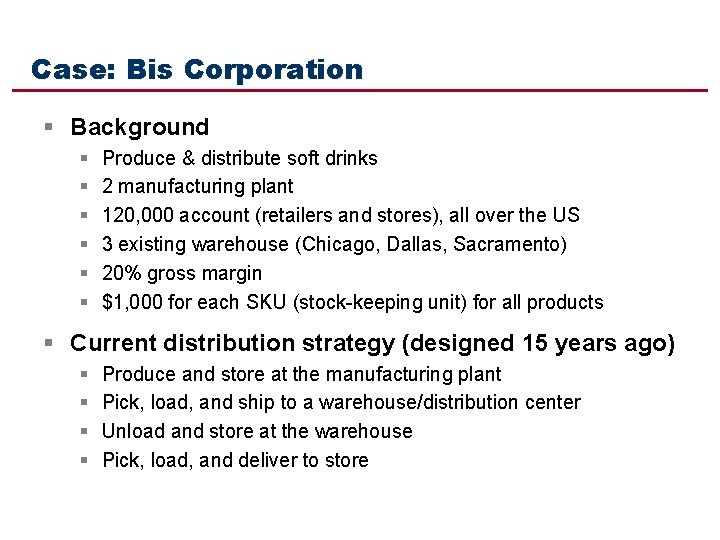
Case: Bis Corporation § Background § § § Produce & distribute soft drinks 2 manufacturing plant 120, 000 account (retailers and stores), all over the US 3 existing warehouse (Chicago, Dallas, Sacramento) 20% gross margin $1, 000 for each SKU (stock-keeping unit) for all products § Current distribution strategy (designed 15 years ago) § § Produce and store at the manufacturing plant Pick, load, and ship to a warehouse/distribution center Unload and store at the warehouse Pick, load, and deliver to store
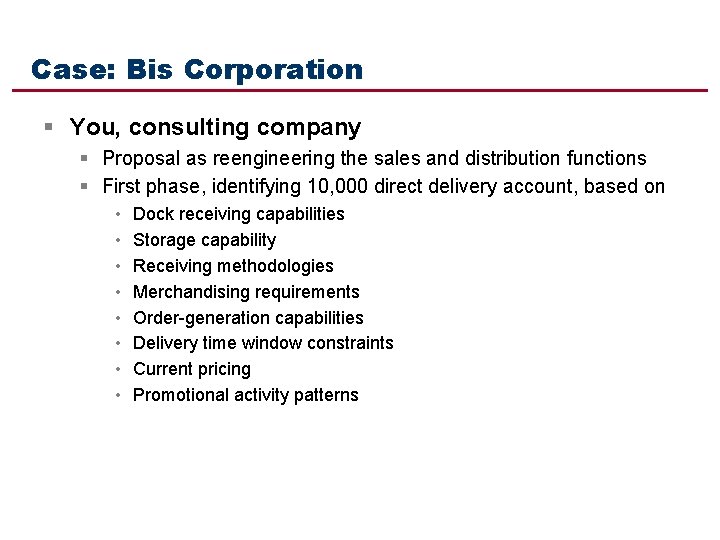
Case: Bis Corporation § You, consulting company § Proposal as reengineering the sales and distribution functions § First phase, identifying 10, 000 direct delivery account, based on • • Dock receiving capabilities Storage capability Receiving methodologies Merchandising requirements Order-generation capabilities Delivery time window constraints Current pricing Promotional activity patterns
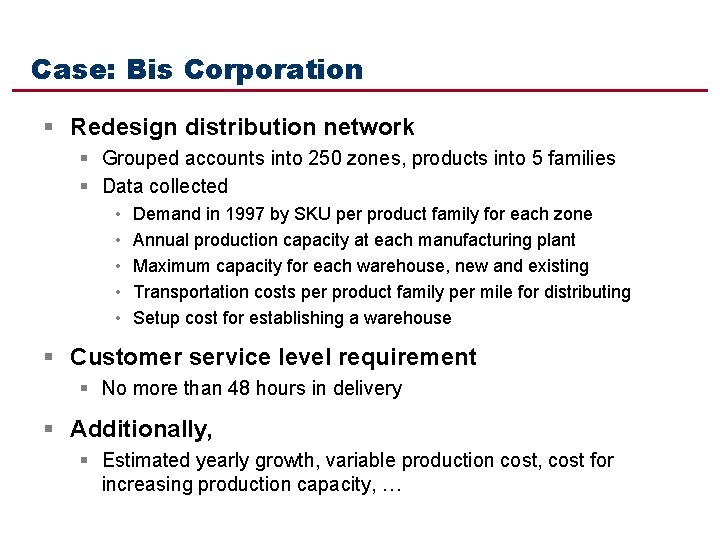
Case: Bis Corporation § Redesign distribution network § Grouped accounts into 250 zones, products into 5 families § Data collected • • • Demand in 1997 by SKU per product family for each zone Annual production capacity at each manufacturing plant Maximum capacity for each warehouse, new and existing Transportation costs per product family per mile for distributing Setup cost for establishing a warehouse § Customer service level requirement § No more than 48 hours in delivery § Additionally, § Estimated yearly growth, variable production cost, cost for increasing production capacity, …
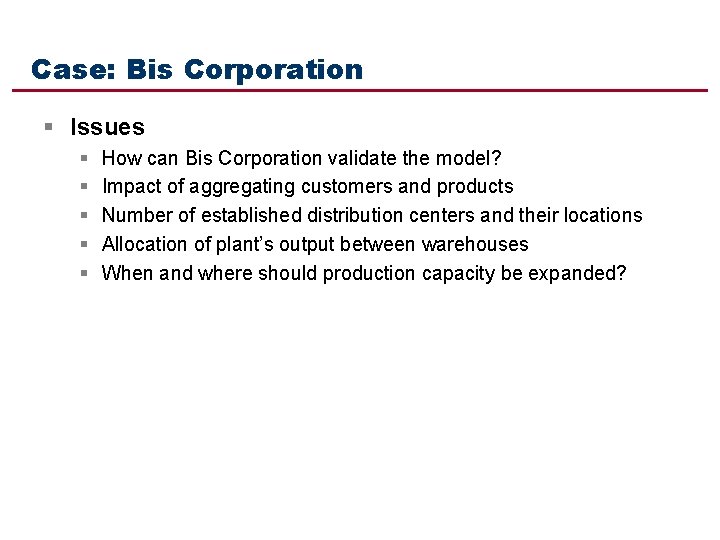
Case: Bis Corporation § Issues § § § How can Bis Corporation validate the model? Impact of aggregating customers and products Number of established distribution centers and their locations Allocation of plant’s output between warehouses When and where should production capacity be expanded?
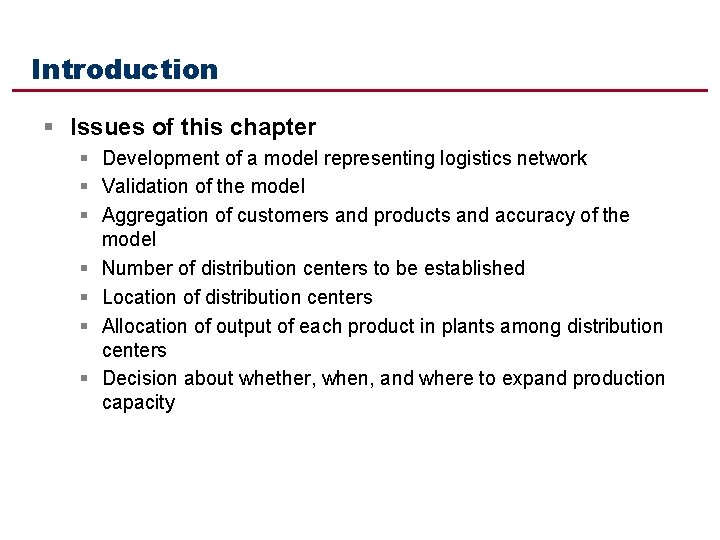
Introduction § Issues of this chapter § Development of a model representing logistics network § Validation of the model § Aggregation of customers and products and accuracy of the model § Number of distribution centers to be established § Location of distribution centers § Allocation of output of each product in plants among distribution centers § Decision about whether, when, and where to expand production capacity
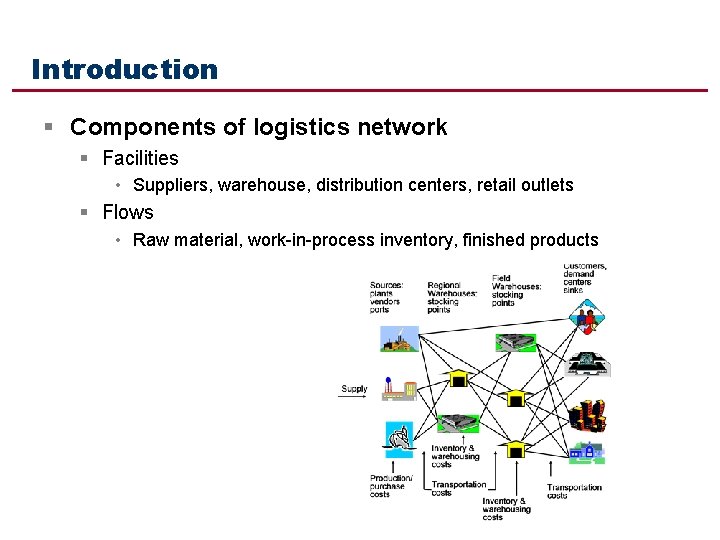
Introduction § Components of logistics network § Facilities • Suppliers, warehouse, distribution centers, retail outlets § Flows • Raw material, work-in-process inventory, finished products
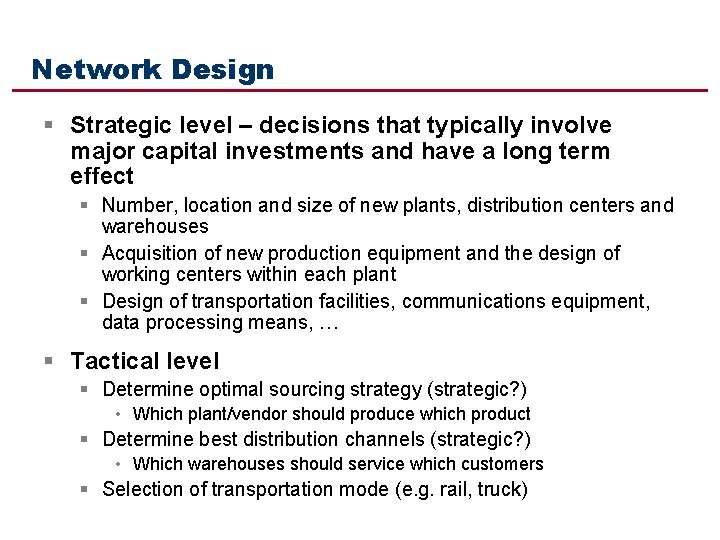
Network Design § Strategic level – decisions that typically involve major capital investments and have a long term effect § Number, location and size of new plants, distribution centers and warehouses § Acquisition of new production equipment and the design of working centers within each plant § Design of transportation facilities, communications equipment, data processing means, … § Tactical level § Determine optimal sourcing strategy (strategic? ) • Which plant/vendor should produce which product § Determine best distribution channels (strategic? ) • Which warehouses should service which customers § Selection of transportation mode (e. g. rail, truck)
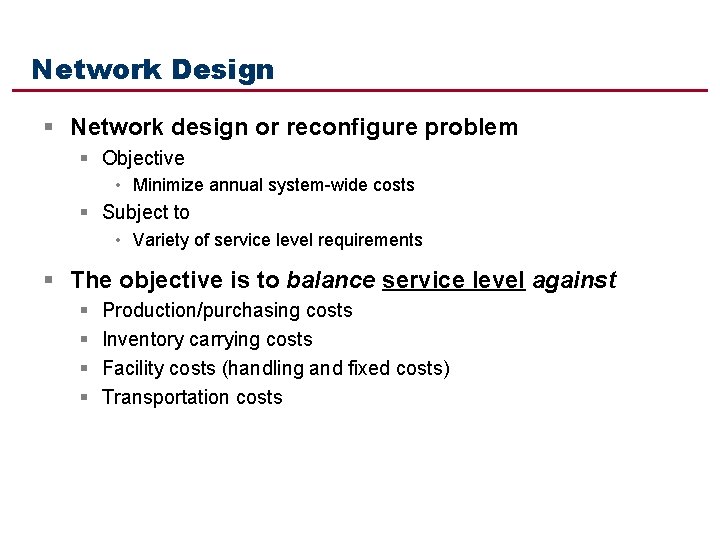
Network Design § Network design or reconfigure problem § Objective • Minimize annual system-wide costs § Subject to • Variety of service level requirements § The objective is to balance service level against § § Production/purchasing costs Inventory carrying costs Facility costs (handling and fixed costs) Transportation costs
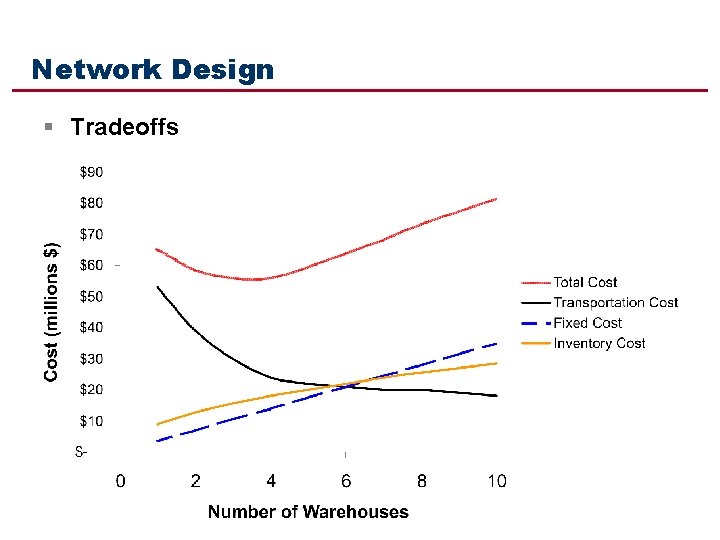
Network Design § Tradeoffs
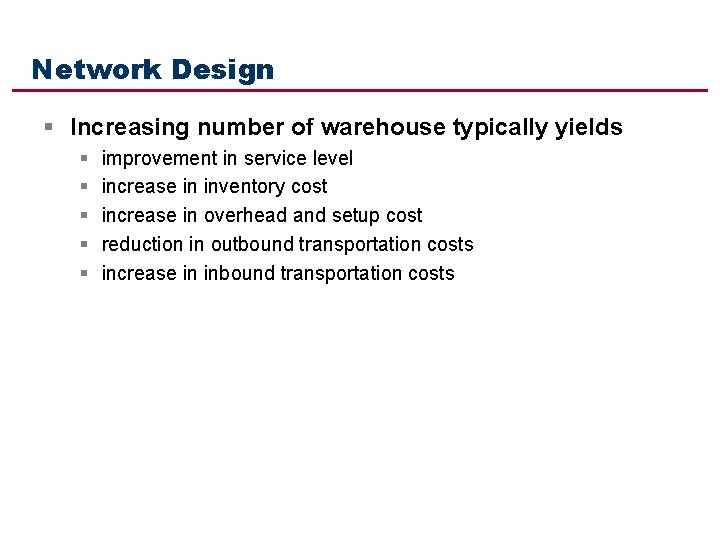
Network Design § Increasing number of warehouse typically yields § § § improvement in service level increase in inventory cost increase in overhead and setup cost reduction in outbound transportation costs increase in inbound transportation costs
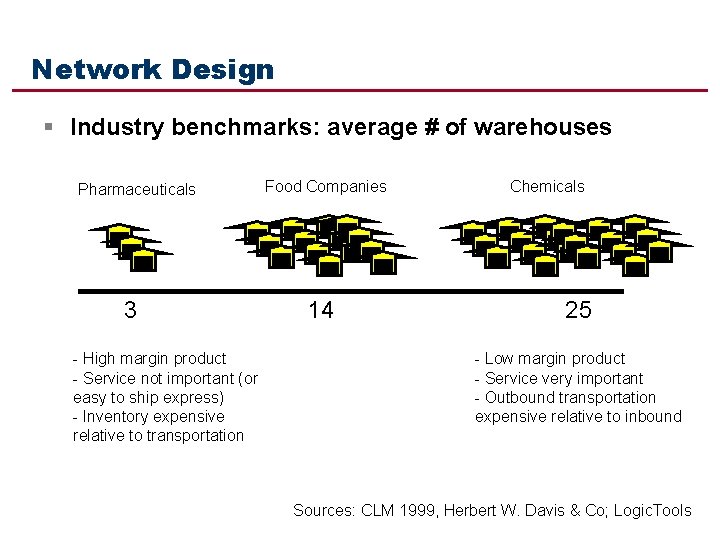
Network Design § Industry benchmarks: average # of warehouses Pharmaceuticals 3 - High margin product - Service not important (or easy to ship express) - Inventory expensive relative to transportation Food Companies 14 Chemicals 25 - Low margin product - Service very important - Outbound transportation expensive relative to inbound Sources: CLM 1999, Herbert W. Davis & Co; Logic. Tools
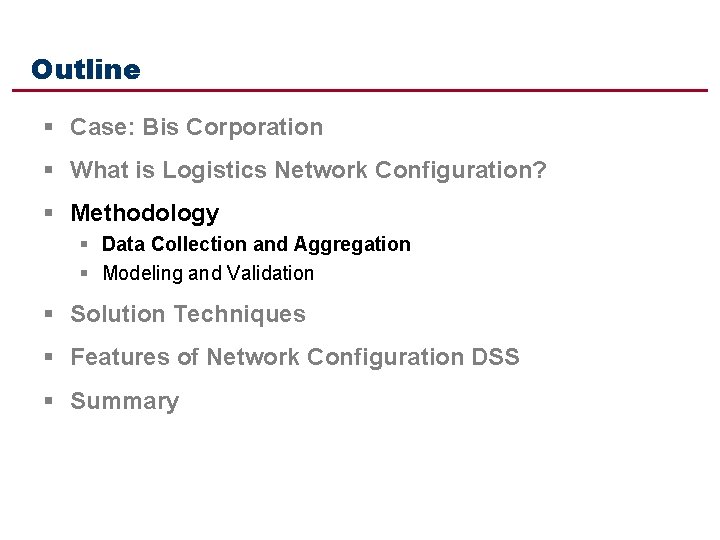
Outline § Case: Bis Corporation § What is Logistics Network Configuration? § Methodology § Data Collection and Aggregation § Modeling and Validation § Solution Techniques § Features of Network Configuration DSS § Summary
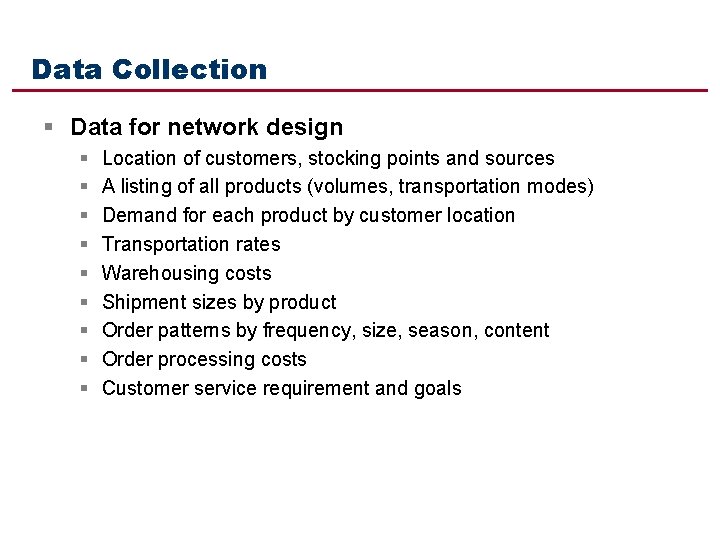
Data Collection § Data for network design § § § § § Location of customers, stocking points and sources A listing of all products (volumes, transportation modes) Demand for each product by customer location Transportation rates Warehousing costs Shipment sizes by product Order patterns by frequency, size, season, content Order processing costs Customer service requirement and goals
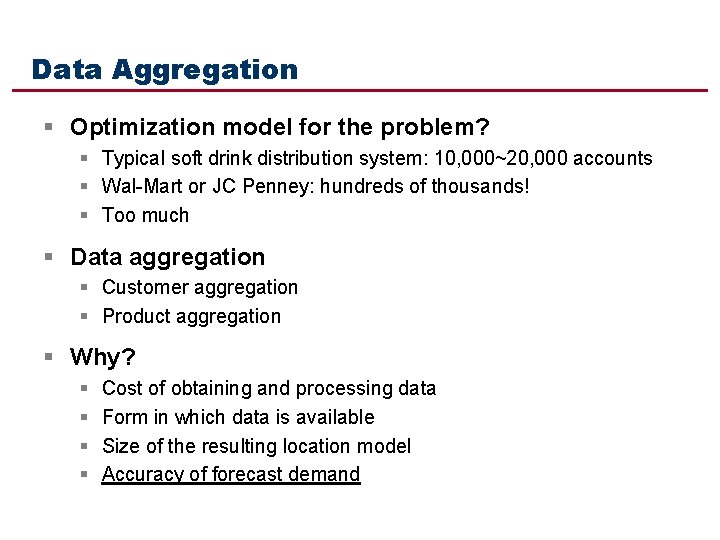
Data Aggregation § Optimization model for the problem? § Typical soft drink distribution system: 10, 000~20, 000 accounts § Wal-Mart or JC Penney: hundreds of thousands! § Too much § Data aggregation § Customer aggregation § Product aggregation § Why? § § Cost of obtaining and processing data Form in which data is available Size of the resulting location model Accuracy of forecast demand
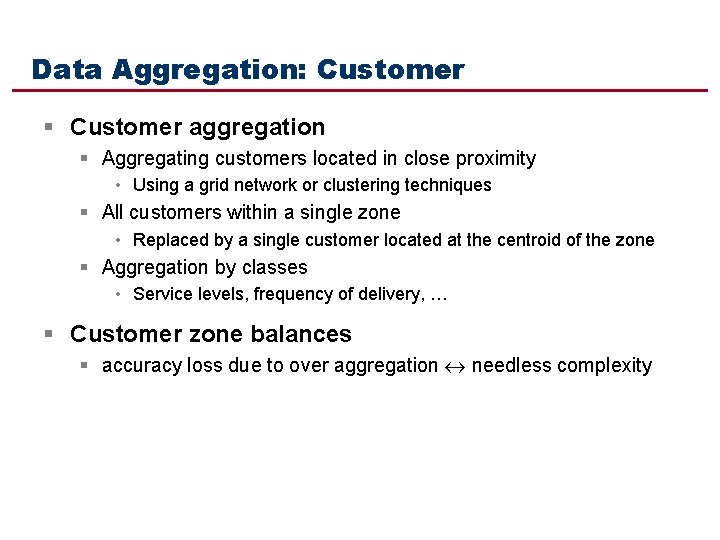
Data Aggregation: Customer § Customer aggregation § Aggregating customers located in close proximity • Using a grid network or clustering techniques § All customers within a single zone • Replaced by a single customer located at the centroid of the zone § Aggregation by classes • Service levels, frequency of delivery, … § Customer zone balances § accuracy loss due to over aggregation needless complexity
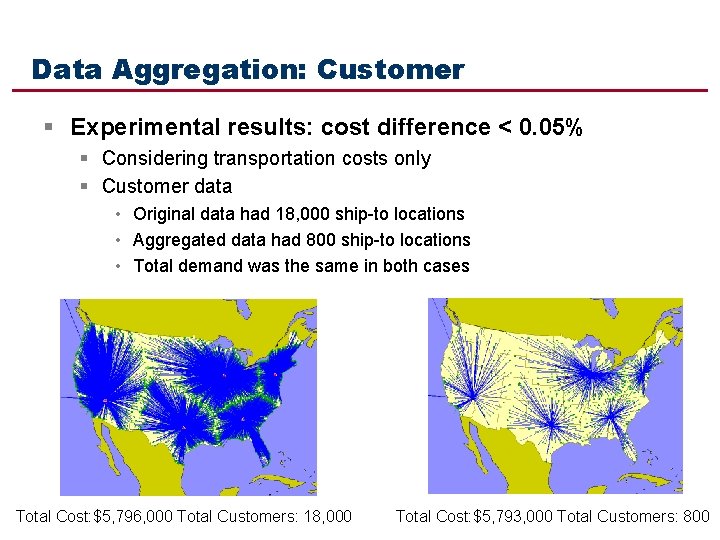
Data Aggregation: Customer § Experimental results: cost difference < 0. 05% § Considering transportation costs only § Customer data • Original data had 18, 000 ship-to locations • Aggregated data had 800 ship-to locations • Total demand was the same in both cases Total Cost: $5, 796, 000 Total Customers: 18, 000 Total Cost: $5, 793, 000 Total Customers: 800
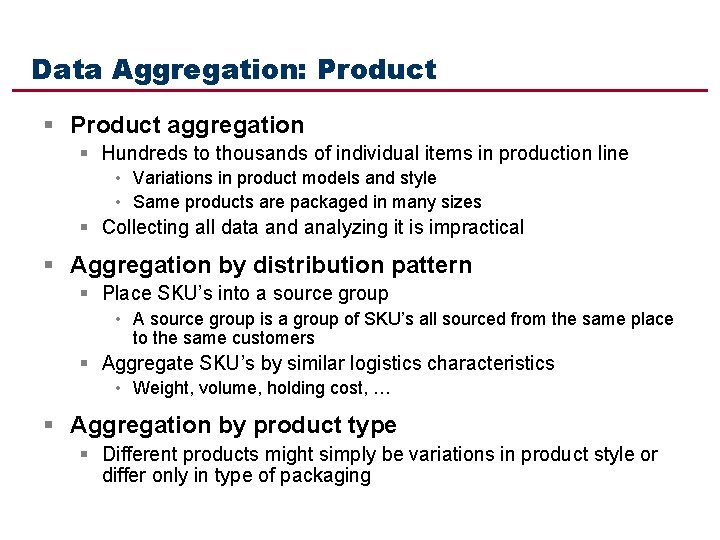
Data Aggregation: Product § Product aggregation § Hundreds to thousands of individual items in production line • Variations in product models and style • Same products are packaged in many sizes § Collecting all data and analyzing it is impractical § Aggregation by distribution pattern § Place SKU’s into a source group • A source group is a group of SKU’s all sourced from the same place to the same customers § Aggregate SKU’s by similar logistics characteristics • Weight, volume, holding cost, … § Aggregation by product type § Different products might simply be variations in product style or differ only in type of packaging
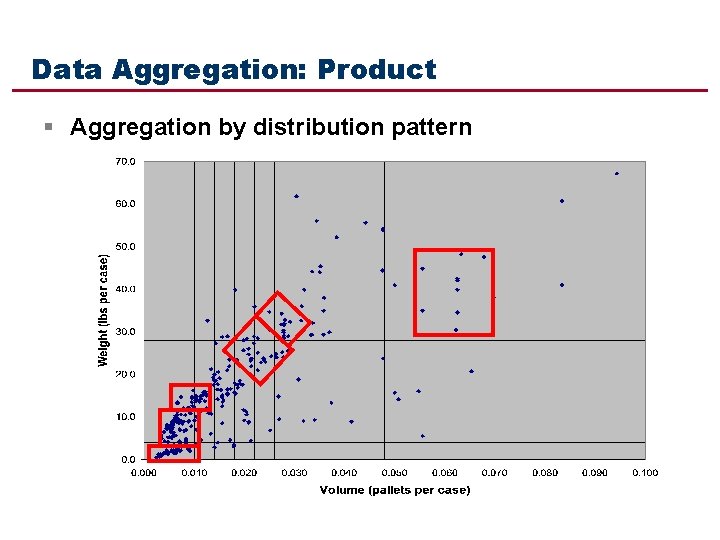
Data Aggregation: Product § Aggregation by distribution pattern
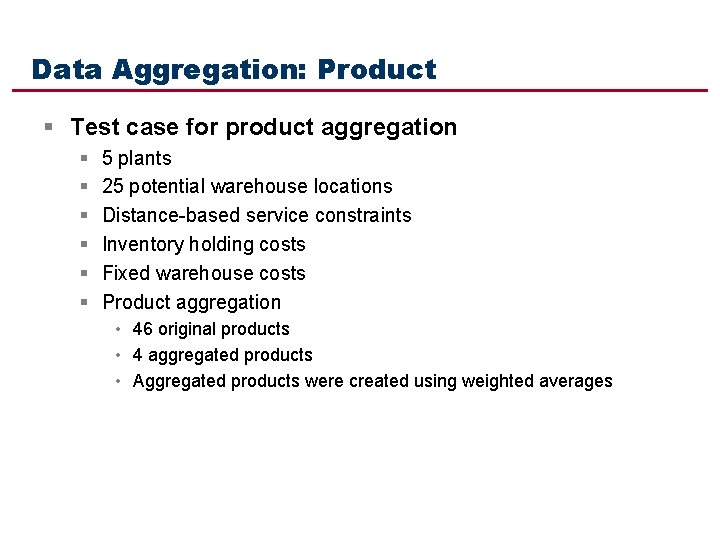
Data Aggregation: Product § Test case for product aggregation § § § 5 plants 25 potential warehouse locations Distance-based service constraints Inventory holding costs Fixed warehouse costs Product aggregation • 46 original products • 4 aggregated products • Aggregated products were created using weighted averages
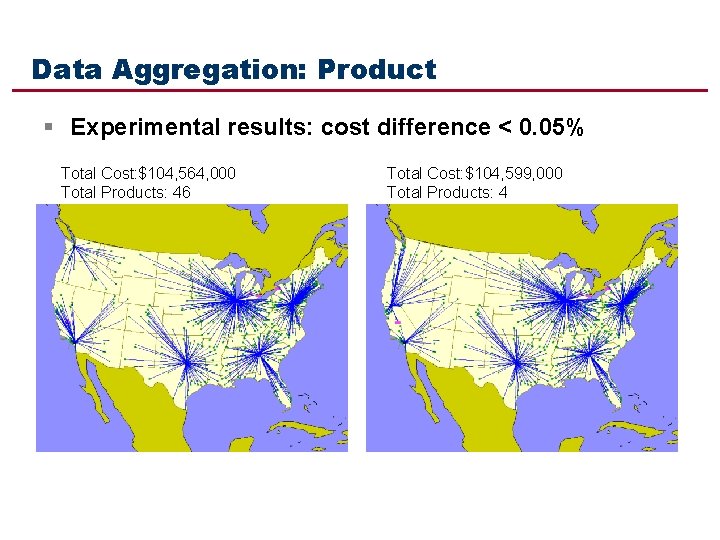
Data Aggregation: Product § Experimental results: cost difference < 0. 05% Total Cost: $104, 564, 000 Total Products: 46 Total Cost: $104, 599, 000 Total Products: 4
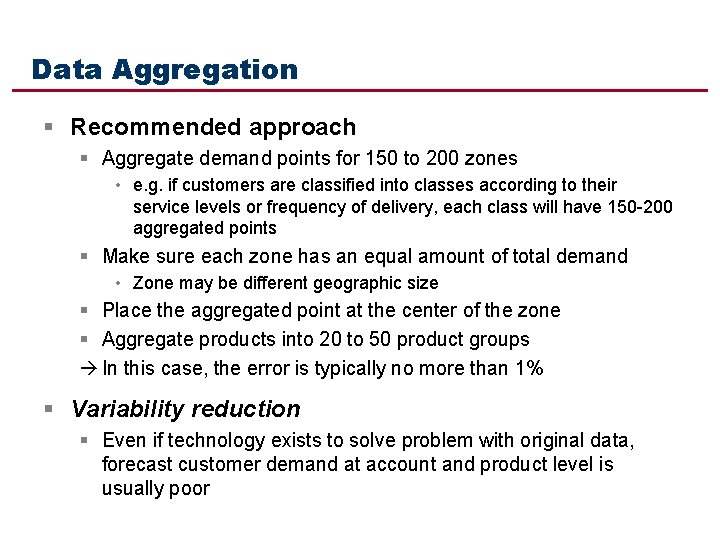
Data Aggregation § Recommended approach § Aggregate demand points for 150 to 200 zones • e. g. if customers are classified into classes according to their service levels or frequency of delivery, each class will have 150 -200 aggregated points § Make sure each zone has an equal amount of total demand • Zone may be different geographic size § Place the aggregated point at the center of the zone § Aggregate products into 20 to 50 product groups In this case, the error is typically no more than 1% § Variability reduction § Even if technology exists to solve problem with original data, forecast customer demand at account and product level is usually poor
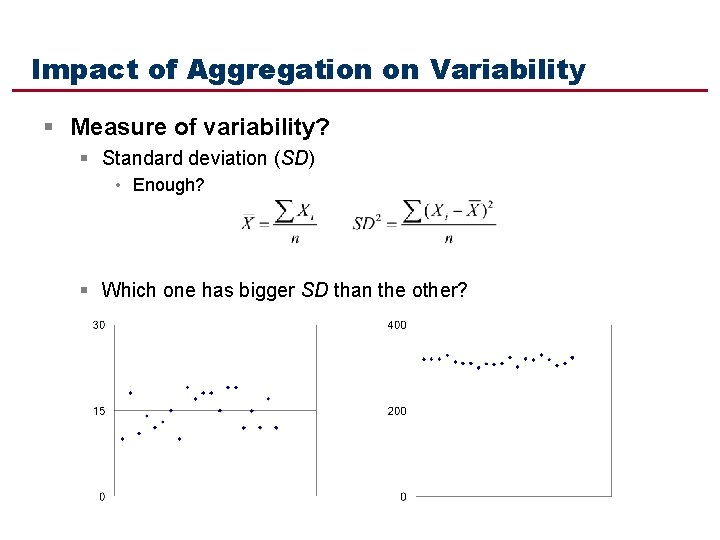
Impact of Aggregation on Variability § Measure of variability? § Standard deviation (SD) • Enough? § Which one has bigger SD than the other?
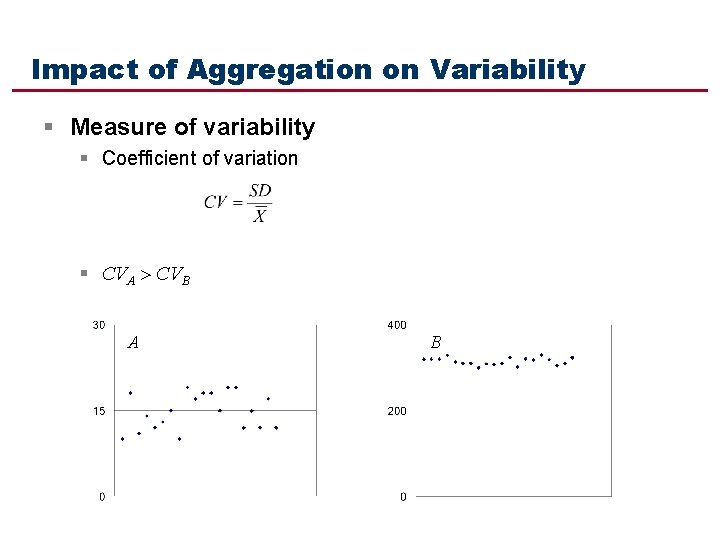
Impact of Aggregation on Variability § Measure of variability § Coefficient of variation § CVA CVB A B
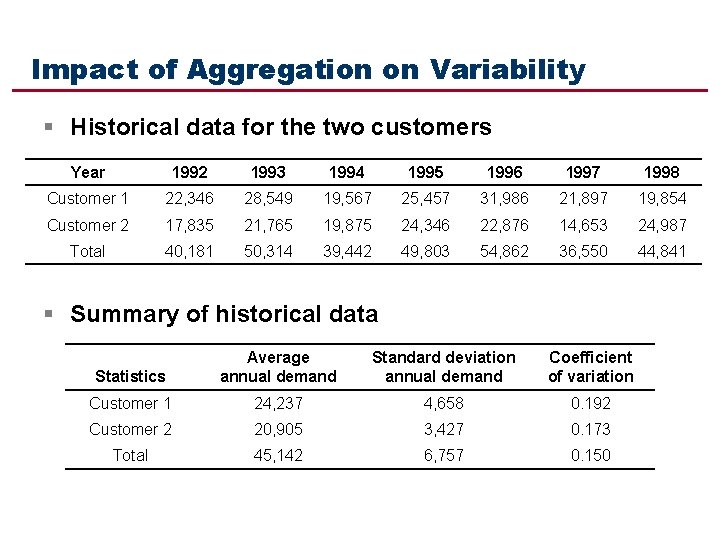
Impact of Aggregation on Variability § Historical data for the two customers Year 1992 1993 1994 1995 1996 1997 1998 Customer 1 22, 346 28, 549 19, 567 25, 457 31, 986 21, 897 19, 854 Customer 2 17, 835 21, 765 19, 875 24, 346 22, 876 14, 653 24, 987 Total 40, 181 50, 314 39, 442 49, 803 54, 862 36, 550 44, 841 § Summary of historical data Statistics Average annual demand Standard deviation annual demand Coefficient of variation Customer 1 24, 237 4, 658 0. 192 Customer 2 20, 905 3, 427 0. 173 Total 45, 142 6, 757 0. 150
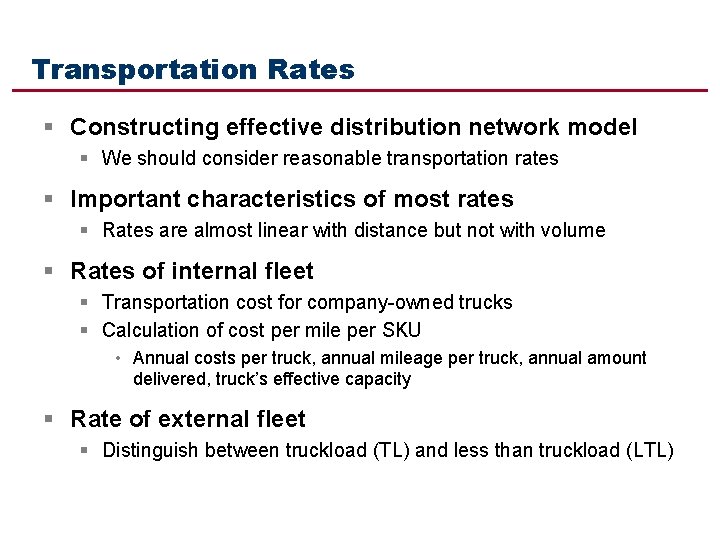
Transportation Rates § Constructing effective distribution network model § We should consider reasonable transportation rates § Important characteristics of most rates § Rates are almost linear with distance but not with volume § Rates of internal fleet § Transportation cost for company-owned trucks § Calculation of cost per mile per SKU • Annual costs per truck, annual mileage per truck, annual amount delivered, truck’s effective capacity § Rate of external fleet § Distinguish between truckload (TL) and less than truckload (LTL)
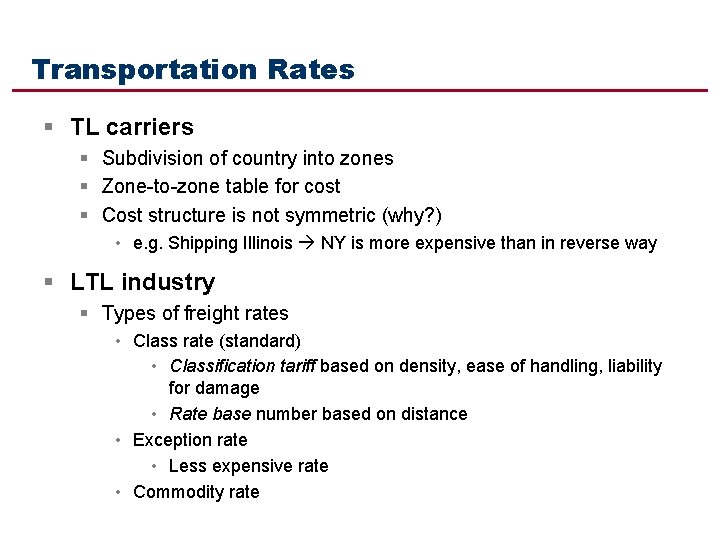
Transportation Rates § TL carriers § Subdivision of country into zones § Zone-to-zone table for cost § Cost structure is not symmetric (why? ) • e. g. Shipping Illinois NY is more expensive than in reverse way § LTL industry § Types of freight rates • Class rate (standard) • Classification tariff based on density, ease of handling, liability for damage • Rate base number based on distance • Exception rate • Less expensive rate • Commodity rate
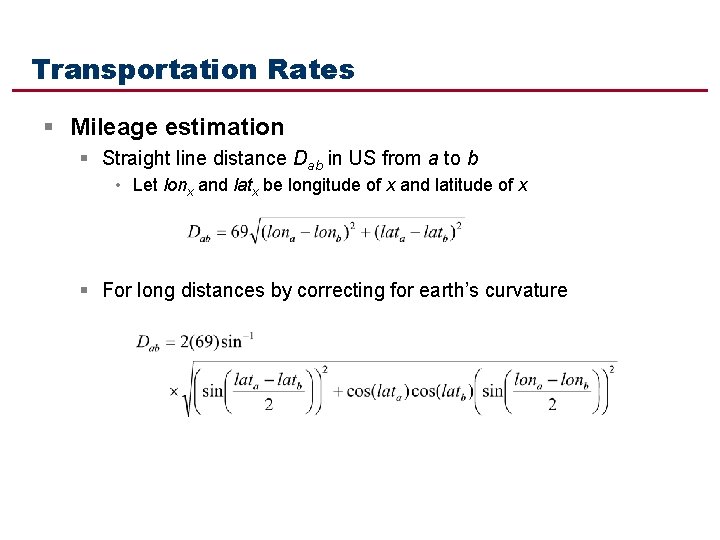
Transportation Rates § Mileage estimation § Straight line distance Dab in US from a to b • Let lonx and latx be longitude of x and latitude of x § For long distances by correcting for earth’s curvature
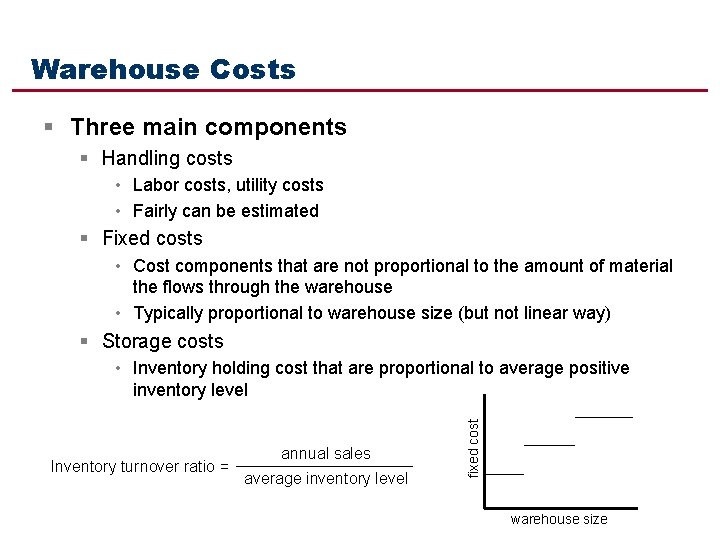
Warehouse Costs § Three main components § Handling costs • Labor costs, utility costs • Fairly can be estimated § Fixed costs • Cost components that are not proportional to the amount of material the flows through the warehouse • Typically proportional to warehouse size (but not linear way) § Storage costs Inventory turnover ratio = annual sales average inventory level fixed cost • Inventory holding cost that are proportional to average positive inventory level warehouse size
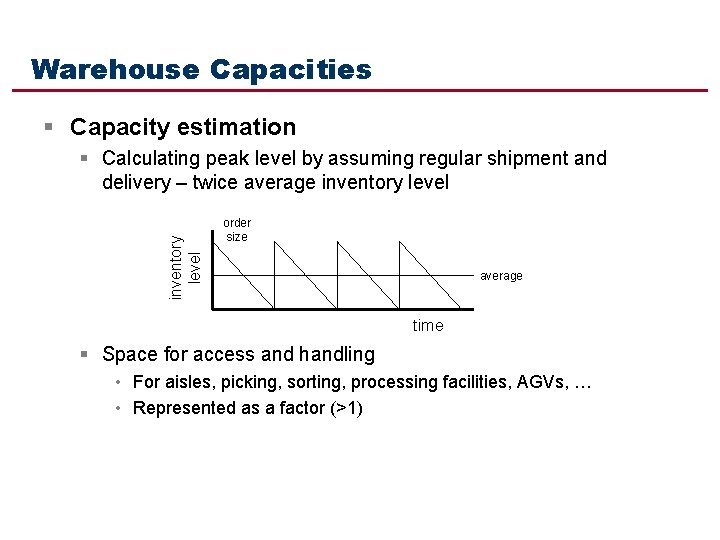
Warehouse Capacities § Capacity estimation inventory level § Calculating peak level by assuming regular shipment and delivery – twice average inventory level order size average time § Space for access and handling • For aisles, picking, sorting, processing facilities, AGVs, … • Represented as a factor (>1)
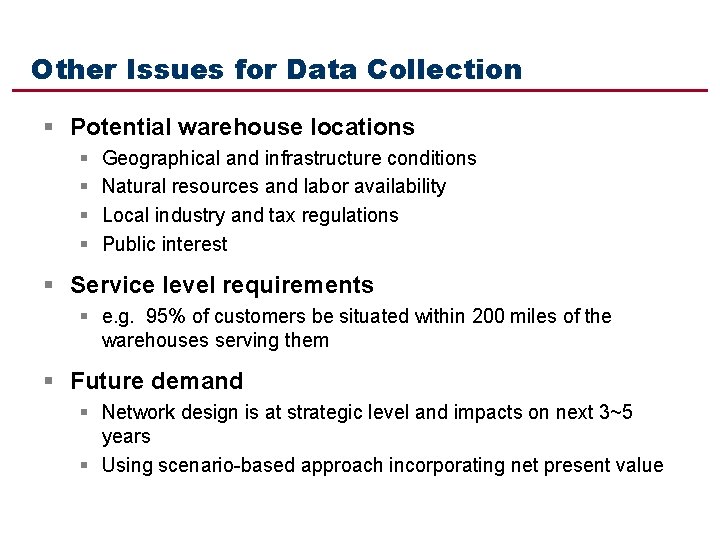
Other Issues for Data Collection § Potential warehouse locations § § Geographical and infrastructure conditions Natural resources and labor availability Local industry and tax regulations Public interest § Service level requirements § e. g. 95% of customers be situated within 200 miles of the warehouses serving them § Future demand § Network design is at strategic level and impacts on next 3~5 years § Using scenario-based approach incorporating net present value
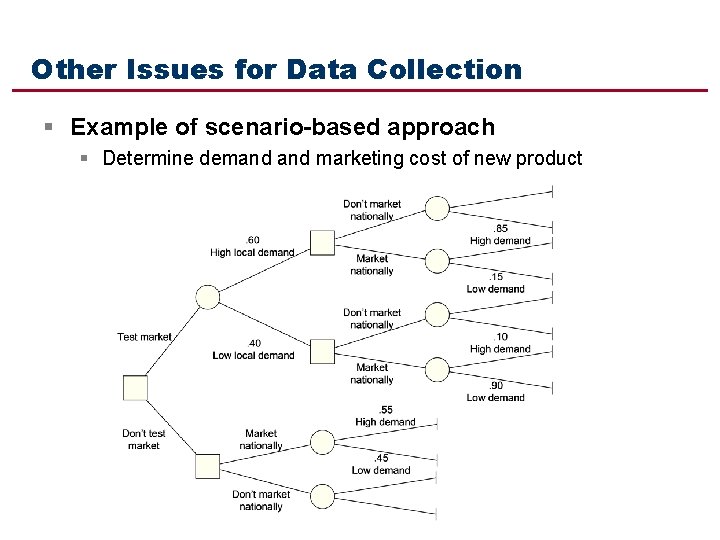
Other Issues for Data Collection § Example of scenario-based approach § Determine demand marketing cost of new product
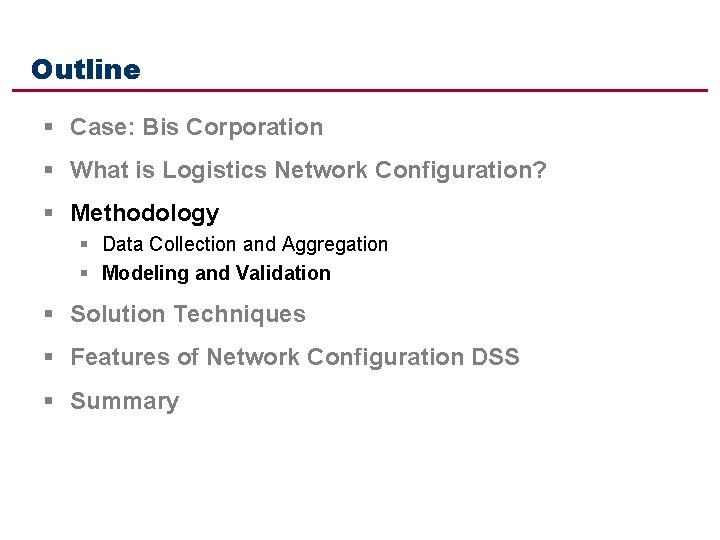
Outline § Case: Bis Corporation § What is Logistics Network Configuration? § Methodology § Data Collection and Aggregation § Modeling and Validation § Solution Techniques § Features of Network Configuration DSS § Summary
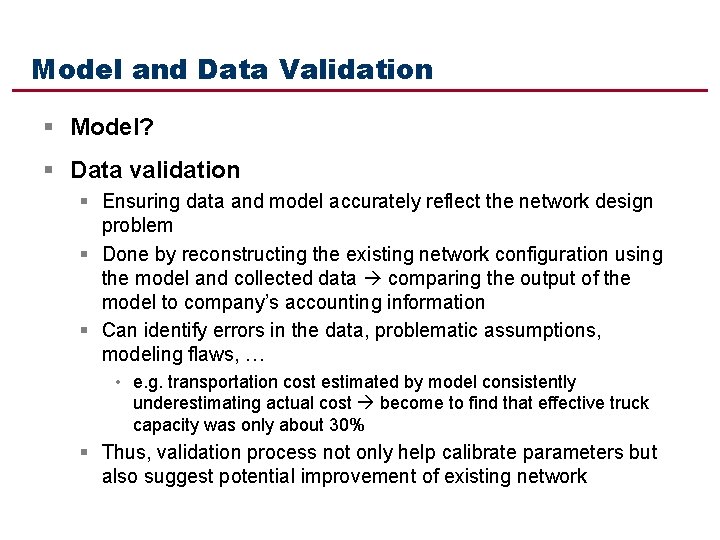
Model and Data Validation § Model? § Data validation § Ensuring data and model accurately reflect the network design problem § Done by reconstructing the existing network configuration using the model and collected data comparing the output of the model to company’s accounting information § Can identify errors in the data, problematic assumptions, modeling flaws, … • e. g. transportation cost estimated by model consistently underestimating actual cost become to find that effective truck capacity was only about 30% § Thus, validation process not only help calibrate parameters but also suggest potential improvement of existing network
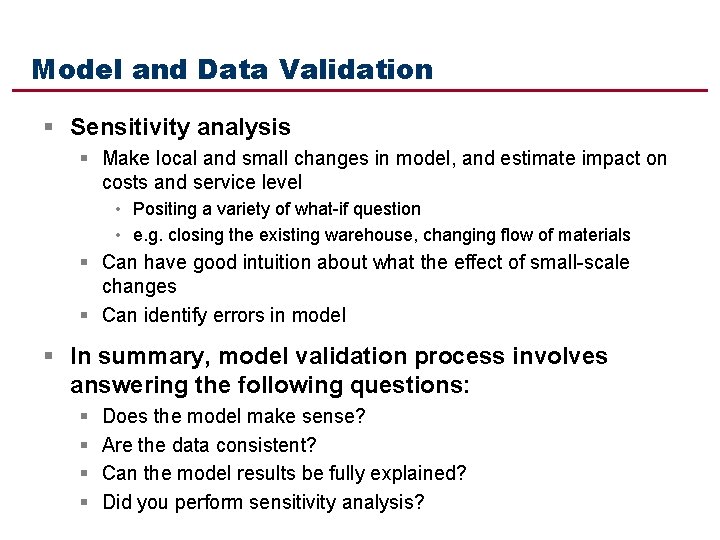
Model and Data Validation § Sensitivity analysis § Make local and small changes in model, and estimate impact on costs and service level • Positing a variety of what-if question • e. g. closing the existing warehouse, changing flow of materials § Can have good intuition about what the effect of small-scale changes § Can identify errors in model § In summary, model validation process involves answering the following questions: § § Does the model make sense? Are the data consistent? Can the model results be fully explained? Did you perform sensitivity analysis?
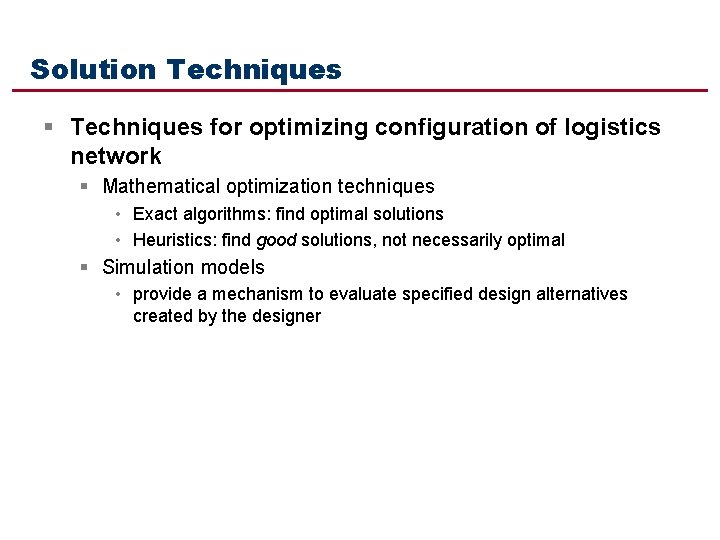
Solution Techniques § Techniques for optimizing configuration of logistics network § Mathematical optimization techniques • Exact algorithms: find optimal solutions • Heuristics: find good solutions, not necessarily optimal § Simulation models • provide a mechanism to evaluate specified design alternatives created by the designer
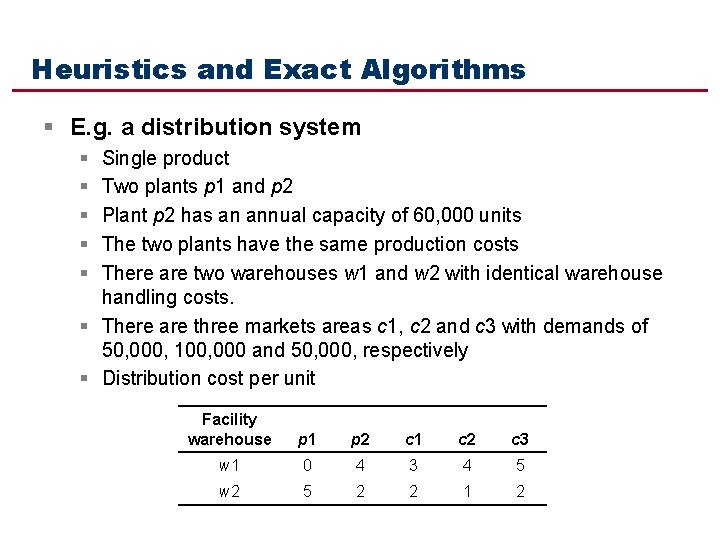
Heuristics and Exact Algorithms § E. g. a distribution system § § § Single product Two plants p 1 and p 2 Plant p 2 has an annual capacity of 60, 000 units The two plants have the same production costs There are two warehouses w 1 and w 2 with identical warehouse handling costs. § There are three markets areas c 1, c 2 and c 3 with demands of 50, 000, 100, 000 and 50, 000, respectively § Distribution cost per unit Facility warehouse p 1 p 2 c 1 c 2 c 3 w 1 0 4 3 4 5 w 2 5 2 2 1 2
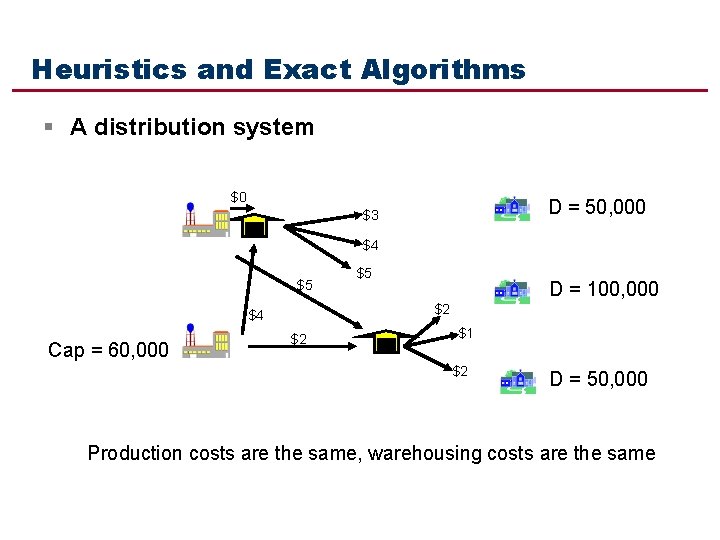
Heuristics and Exact Algorithms § A distribution system $0 D = 50, 000 $3 $4 $5 D = 100, 000 $2 $4 Cap = 60, 000 $5 $2 $1 $2 D = 50, 000 Production costs are the same, warehousing costs are the same
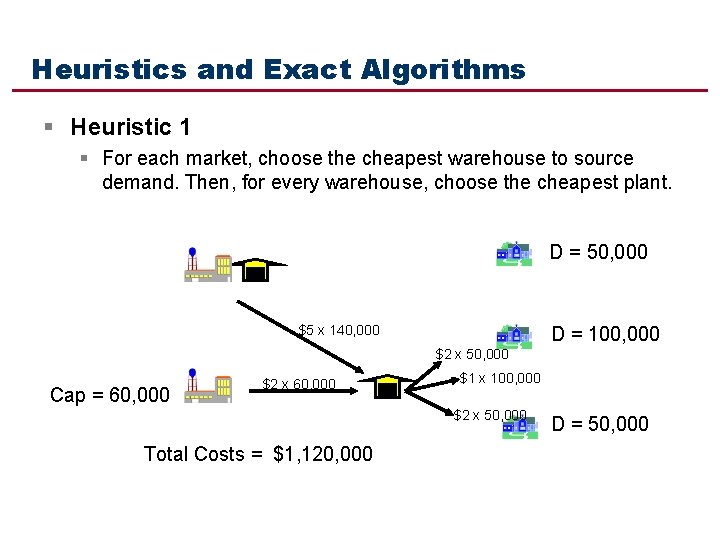
Heuristics and Exact Algorithms § Heuristic 1 § For each market, choose the cheapest warehouse to source demand. Then, for every warehouse, choose the cheapest plant. D = 50, 000 $5 x 140, 000 D = 100, 000 $2 x 50, 000 Cap = 60, 000 $2 x 60, 000 $1 x 100, 000 $2 x 50, 000 Total Costs = $1, 120, 000 D = 50, 000
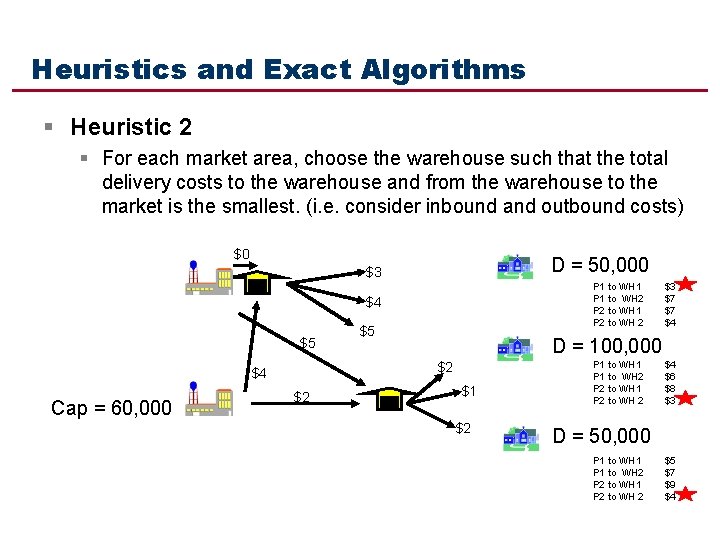
Heuristics and Exact Algorithms § Heuristic 2 § For each market area, choose the warehouse such that the total delivery costs to the warehouse and from the warehouse to the market is the smallest. (i. e. consider inbound and outbound costs) $0 D = 50, 000 $3 P 1 to WH 1 P 1 to WH 2 P 2 to WH 1 P 2 to WH 2 $4 $5 D = 100, 000 $2 $4 Cap = 60, 000 $5 $2 $3 $7 $7 $4 $1 $2 P 1 to WH 1 P 1 to WH 2 P 2 to WH 1 P 2 to WH 2 $4 $6 $8 $3 D = 50, 000 P 1 to WH 1 P 1 to WH 2 P 2 to WH 1 P 2 to WH 2 $5 $7 $9 $4
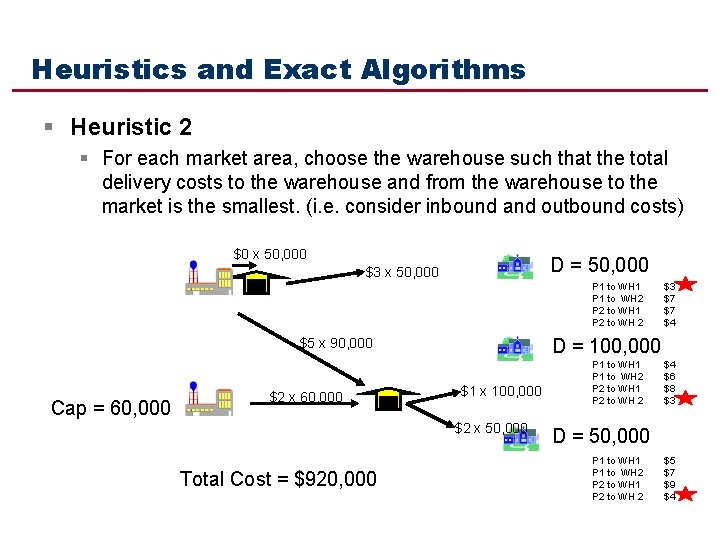
Heuristics and Exact Algorithms § Heuristic 2 § For each market area, choose the warehouse such that the total delivery costs to the warehouse and from the warehouse to the market is the smallest. (i. e. consider inbound and outbound costs) $0 x 50, 000 D = 50, 000 $3 x 50, 000 P 1 to WH 1 P 1 to WH 2 P 2 to WH 1 P 2 to WH 2 $5 x 90, 000 Cap = 60, 000 $2 x 60, 000 D = 100, 000 $1 x 100, 000 $2 x 50, 000 Total Cost = $920, 000 $3 $7 $7 $4 P 1 to WH 1 P 1 to WH 2 P 2 to WH 1 P 2 to WH 2 $4 $6 $8 $3 D = 50, 000 P 1 to WH 1 P 1 to WH 2 P 2 to WH 1 P 2 to WH 2 $5 $7 $9 $4
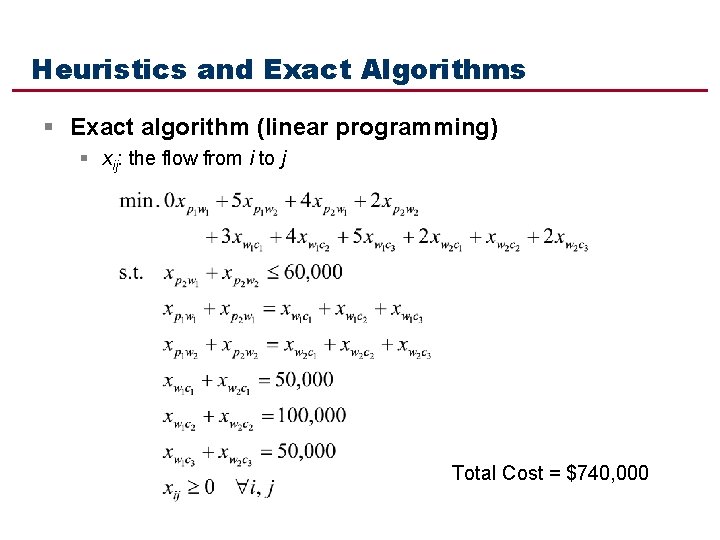
Heuristics and Exact Algorithms § Exact algorithm (linear programming) § xij: the flow from i to j Total Cost = $740, 000
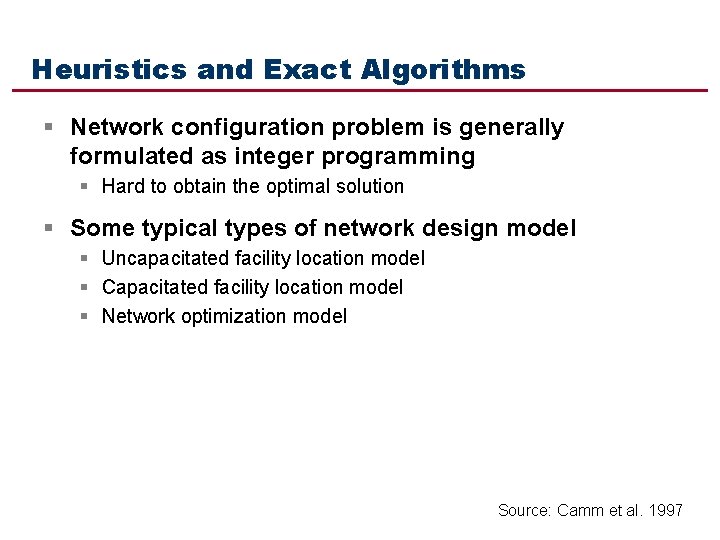
Heuristics and Exact Algorithms § Network configuration problem is generally formulated as integer programming § Hard to obtain the optimal solution § Some typical types of network design model § Uncapacitated facility location model § Capacitated facility location model § Network optimization model Source: Camm et al. 1997
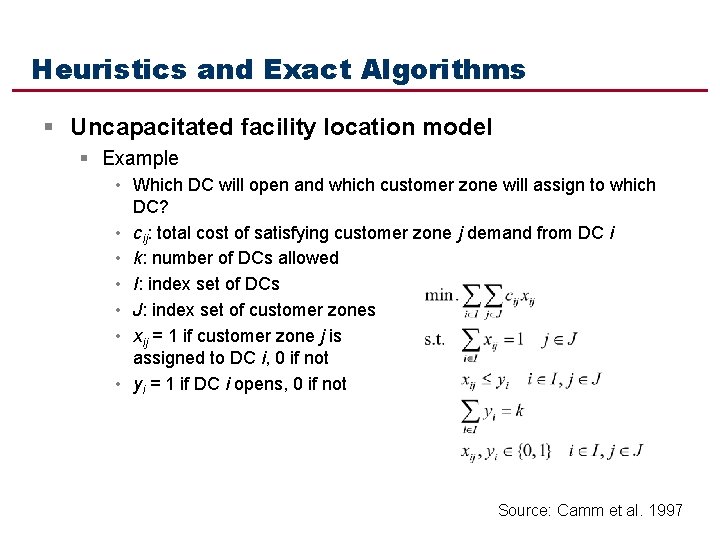
Heuristics and Exact Algorithms § Uncapacitated facility location model § Example • Which DC will open and which customer zone will assign to which DC? • cij: total cost of satisfying customer zone j demand from DC i • k: number of DCs allowed • I: index set of DCs • J: index set of customer zones • xij = 1 if customer zone j is assigned to DC i, 0 if not • yi = 1 if DC i opens, 0 if not Source: Camm et al. 1997
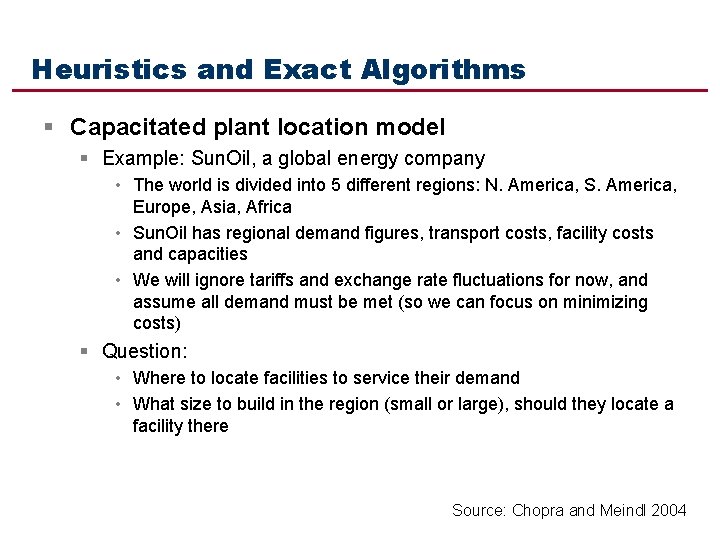
Heuristics and Exact Algorithms § Capacitated plant location model § Example: Sun. Oil, a global energy company • The world is divided into 5 different regions: N. America, S. America, Europe, Asia, Africa • Sun. Oil has regional demand figures, transport costs, facility costs and capacities • We will ignore tariffs and exchange rate fluctuations for now, and assume all demand must be met (so we can focus on minimizing costs) § Question: • Where to locate facilities to service their demand • What size to build in the region (small or large), should they locate a facility there Source: Chopra and Meindl 2004
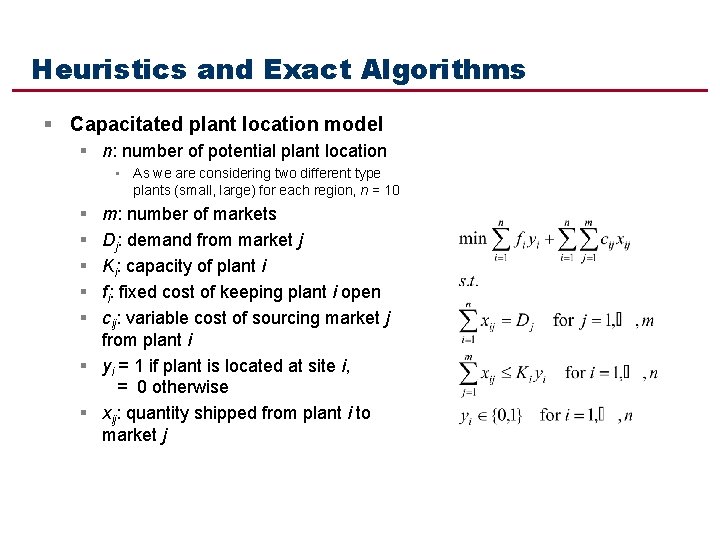
Heuristics and Exact Algorithms § Capacitated plant location model § n: number of potential plant location • As we are considering two different type plants (small, large) for each region, n = 10 § § § m: number of markets Dj: demand from market j Ki: capacity of plant i fi: fixed cost of keeping plant i open cij: variable cost of sourcing market j from plant i § yi = 1 if plant is located at site i, = 0 otherwise § xij: quantity shipped from plant i to market j
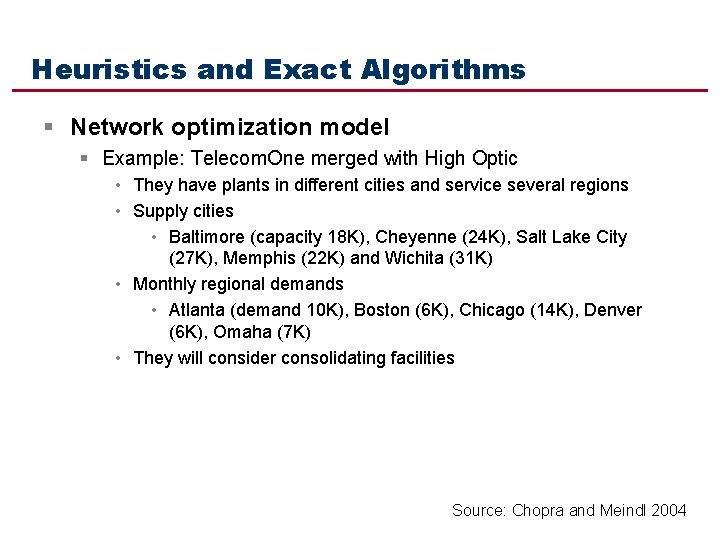
Heuristics and Exact Algorithms § Network optimization model § Example: Telecom. One merged with High Optic • They have plants in different cities and service several regions • Supply cities • Baltimore (capacity 18 K), Cheyenne (24 K), Salt Lake City (27 K), Memphis (22 K) and Wichita (31 K) • Monthly regional demands • Atlanta (demand 10 K), Boston (6 K), Chicago (14 K), Denver (6 K), Omaha (7 K) • They will consider consolidating facilities Source: Chopra and Meindl 2004
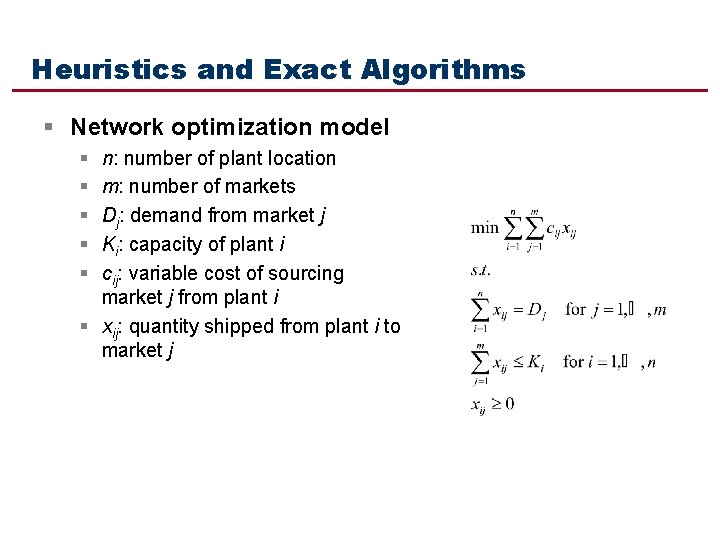
Heuristics and Exact Algorithms § Network optimization model § § § n: number of plant location m: number of markets Dj: demand from market j Ki: capacity of plant i cij: variable cost of sourcing market j from plant i § xij: quantity shipped from plant i to market j
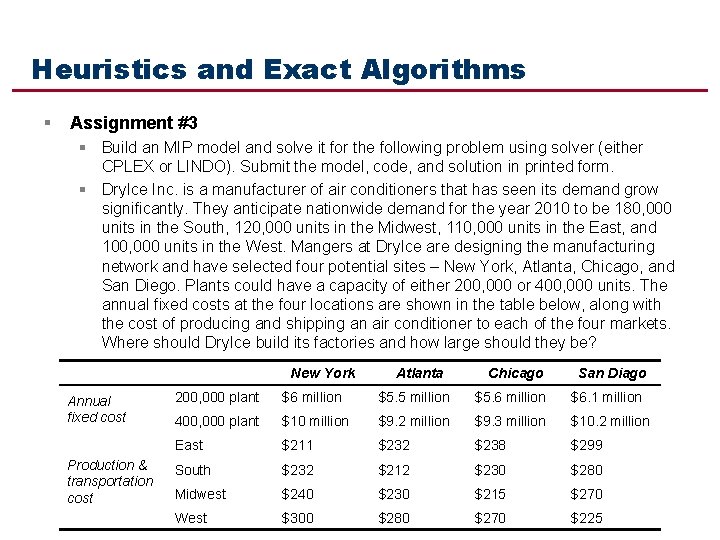
Heuristics and Exact Algorithms § Assignment #3 § Build an MIP model and solve it for the following problem using solver (either CPLEX or LINDO). Submit the model, code, and solution in printed form. § Dry. Ice Inc. is a manufacturer of air conditioners that has seen its demand grow significantly. They anticipate nationwide demand for the year 2010 to be 180, 000 units in the South, 120, 000 units in the Midwest, 110, 000 units in the East, and 100, 000 units in the West. Mangers at Dry. Ice are designing the manufacturing network and have selected four potential sites – New York, Atlanta, Chicago, and San Diego. Plants could have a capacity of either 200, 000 or 400, 000 units. The annual fixed costs at the four locations are shown in the table below, along with the cost of producing and shipping an air conditioner to each of the four markets. Where should Dry. Ice build its factories and how large should they be? New York Annual fixed cost Production & transportation cost Atlanta Chicago San Diago 200, 000 plant $6 million $5. 5 million $5. 6 million $6. 1 million 400, 000 plant $10 million $9. 2 million $9. 3 million $10. 2 million East $211 $232 $238 $299 South $232 $212 $230 $280 Midwest $240 $230 $215 $270 West $300 $280 $270 $225
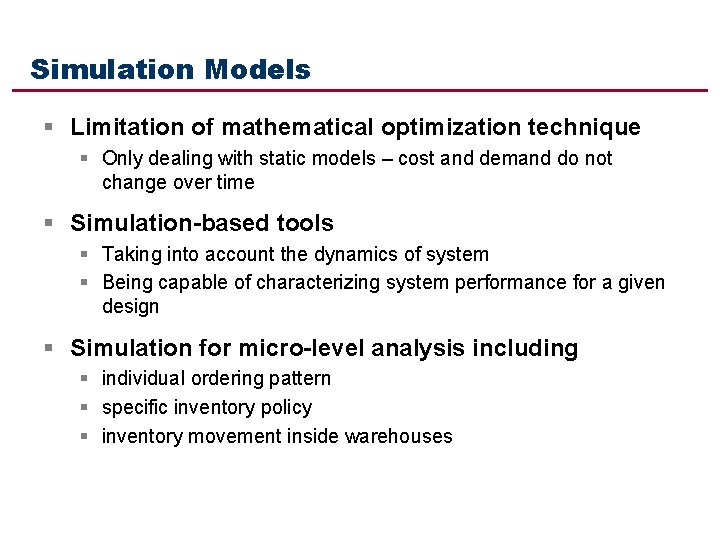
Simulation Models § Limitation of mathematical optimization technique § Only dealing with static models – cost and demand do not change over time § Simulation-based tools § Taking into account the dynamics of system § Being capable of characterizing system performance for a given design § Simulation for micro-level analysis including § individual ordering pattern § specific inventory policy § inventory movement inside warehouses
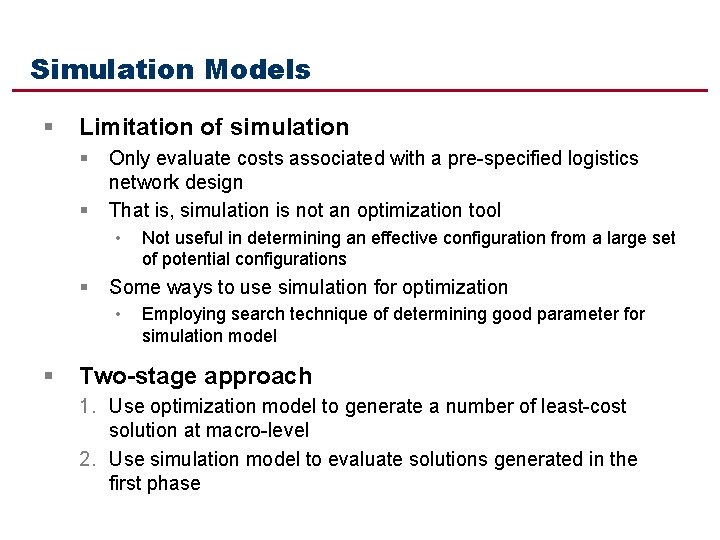
Simulation Models § Limitation of simulation § § Only evaluate costs associated with a pre-specified logistics network design That is, simulation is not an optimization tool • § Some ways to use simulation for optimization • § Not useful in determining an effective configuration from a large set of potential configurations Employing search technique of determining good parameter for simulation model Two-stage approach 1. Use optimization model to generate a number of least-cost solution at macro-level 2. Use simulation model to evaluate solutions generated in the first phase
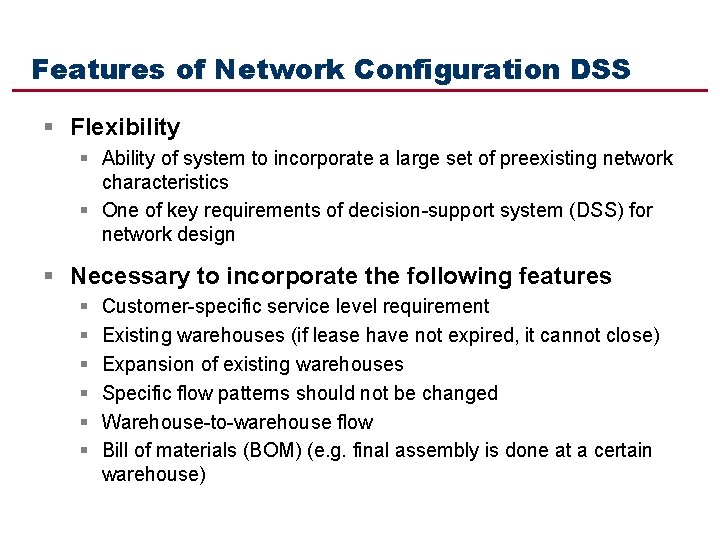
Features of Network Configuration DSS § Flexibility § Ability of system to incorporate a large set of preexisting network characteristics § One of key requirements of decision-support system (DSS) for network design § Necessary to incorporate the following features § § § Customer-specific service level requirement Existing warehouses (if lease have not expired, it cannot close) Expansion of existing warehouses Specific flow patterns should not be changed Warehouse-to-warehouse flow Bill of materials (BOM) (e. g. final assembly is done at a certain warehouse)
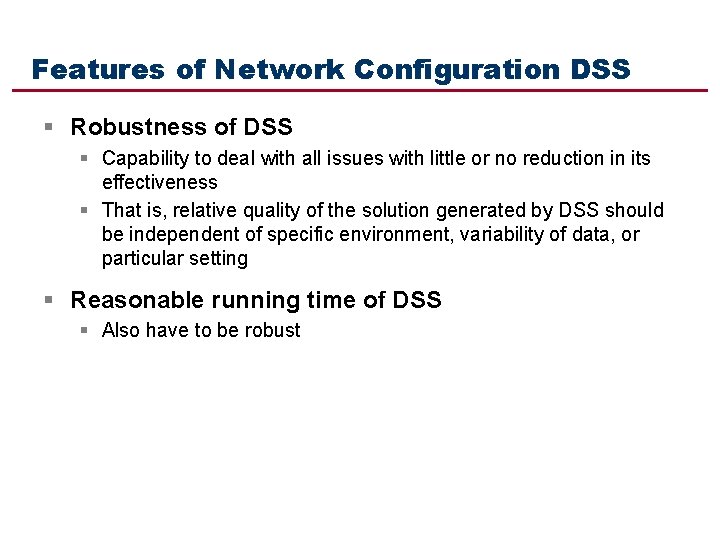
Features of Network Configuration DSS § Robustness of DSS § Capability to deal with all issues with little or no reduction in its effectiveness § That is, relative quality of the solution generated by DSS should be independent of specific environment, variability of data, or particular setting § Reasonable running time of DSS § Also have to be robust
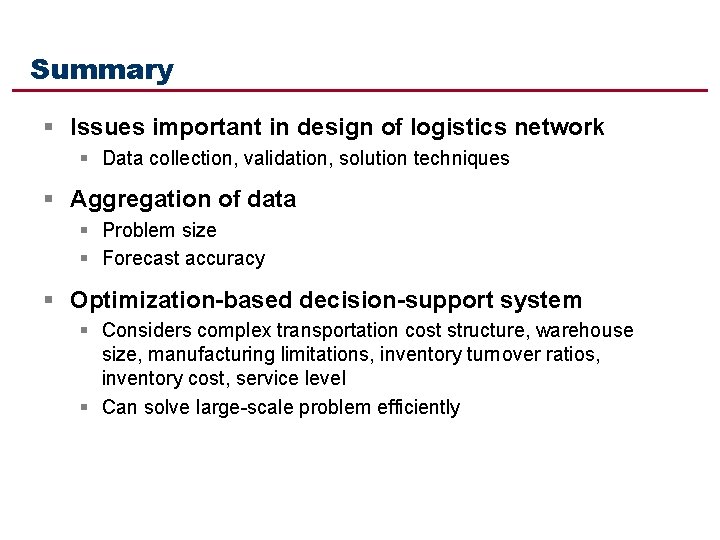
Summary § Issues important in design of logistics network § Data collection, validation, solution techniques § Aggregation of data § Problem size § Forecast accuracy § Optimization-based decision-support system § Considers complex transportation cost structure, warehouse size, manufacturing limitations, inventory turnover ratios, inventory cost, service level § Can solve large-scale problem efficiently
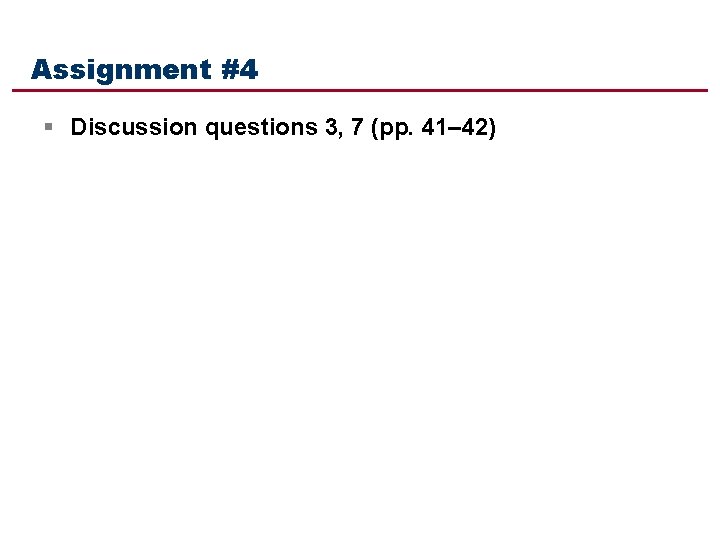
Assignment #4 § Discussion questions 3, 7 (pp. 41– 42)
Managing supply chains a logistics approach
Managing supply chains: a logistics approach
Managing supply chains a logistics approach
Managing supply chains a logistics approach
Data aggregation
Designing global supply chain networks
Difference between logistics and supply chain
Types of ecrm
International logistics and supply chain outsourcing
Ford mmog
Global logistics and supply chain management
Supply chain strategic planning
Component of physical distribution
A key to reducing lot size without increasing costs is to
Managing economies of scale in a supply chain
The required safety inventory
Managing economies of scale in a supply chain
Matching supply with demand
Eltonian pyramid
Framework for network design decisions
Facility network design
All shipments via central dc
Decision tree supply chain
Supply chain network analysis
Network design in the supply chain chapter 5
Supply chain management network design
Benefits of designing and managing service processes
Managing products and brands
Designing and managing services
Services are typically produced and consumed simultaneously
Designing and managing integrated marketing communications
Obero spm
Managing channel dynamics
What is a marketing channel system and value network
Designing and managing value networks
Types of jaycustomers
Designing and managing integrated marketing communications
High value deal seekers
Designing and managing integrated marketing channels
Amplified expressiveness
Designing and managing services
Designing and managing integrated marketing communications
Designing and managing integrated marketing communications
Absolute vs relative configuration
Absolute configuration
Electron configuration vs noble gas configuration
Absolute configuration vs relative configuration
Vender vs supplier
Designing a secure local area network
Design options for a distribution network
Adaptive logistics network
Iam logistics network
Logical network perimeter
Cisco configuration management
Oracle network environment
Network configuration optimization analysis
Enterprise network configuration