Exploring Fitness and Free Energy Landscapes of Proteins
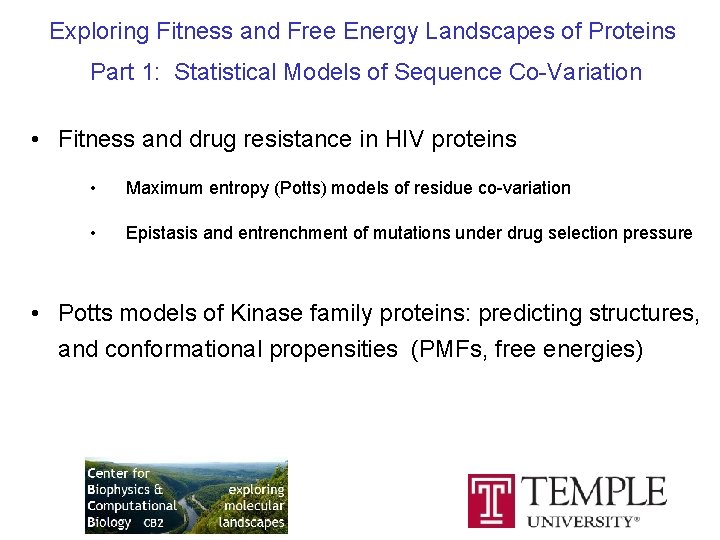
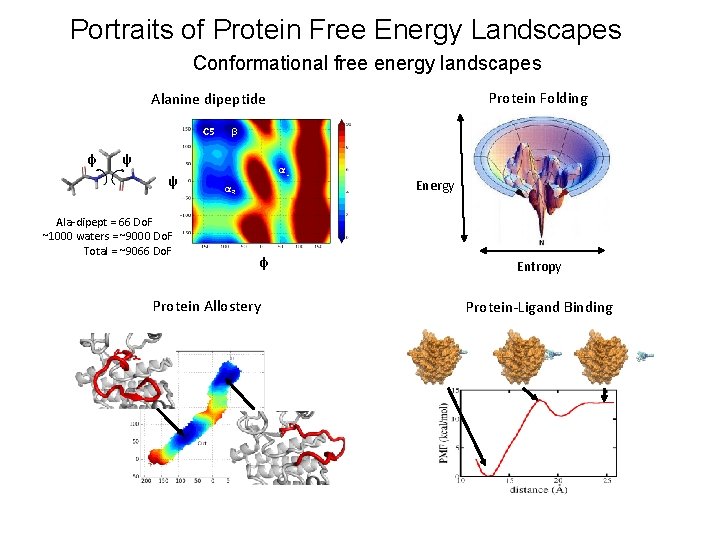
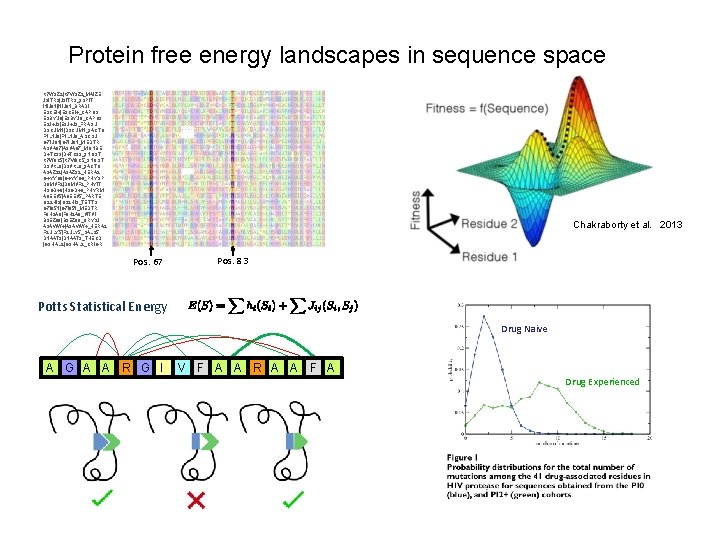
![ar. Xiv: 1207. 2484 v 1 [q-bio. QM] ar. Xiv: 1207. 2484 v 1 [q-bio. QM]](https://slidetodoc.com/presentation_image_h/0aecad485edd5e56de5f54ba42c397a0/image-4.jpg)
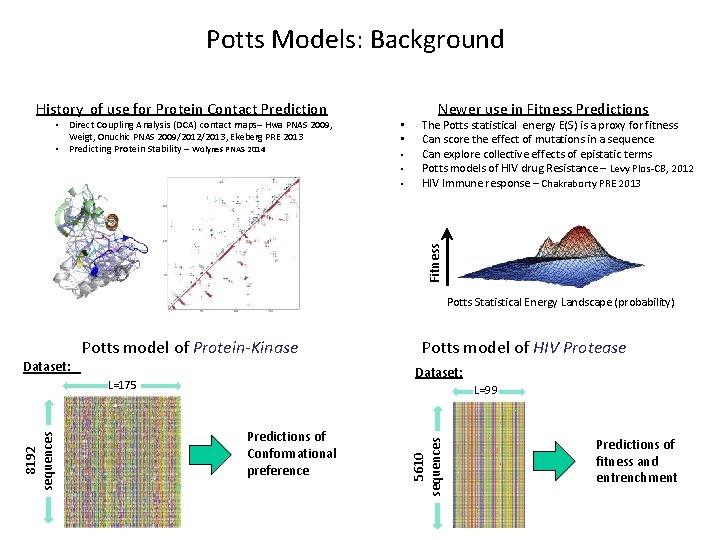
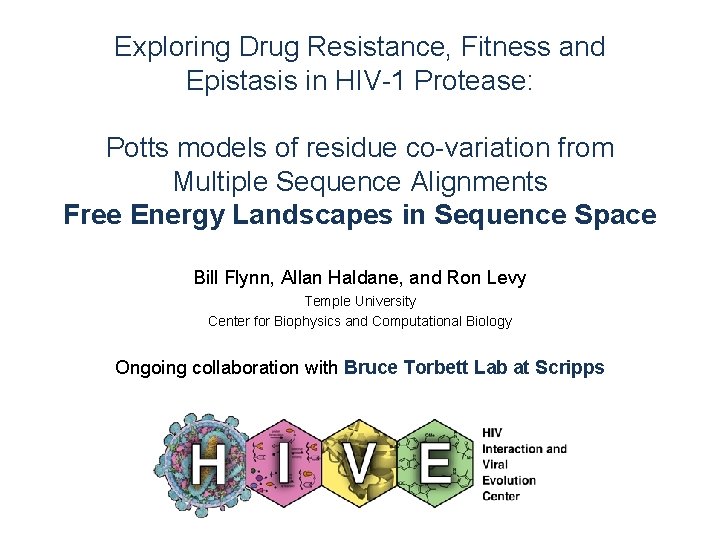
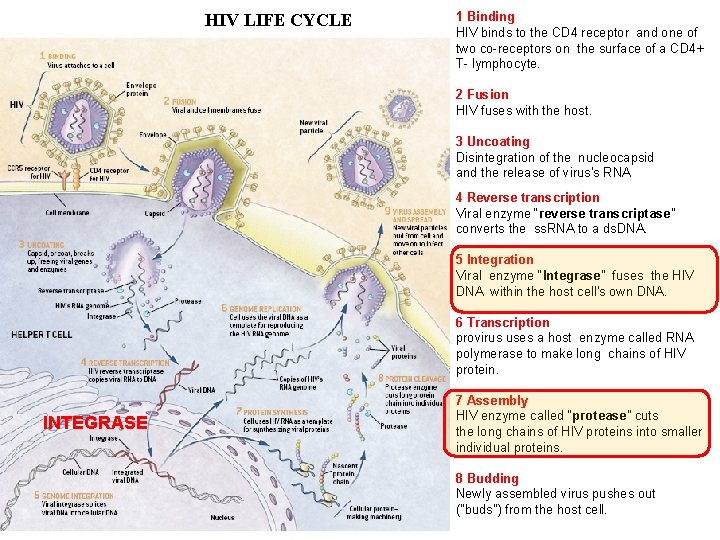
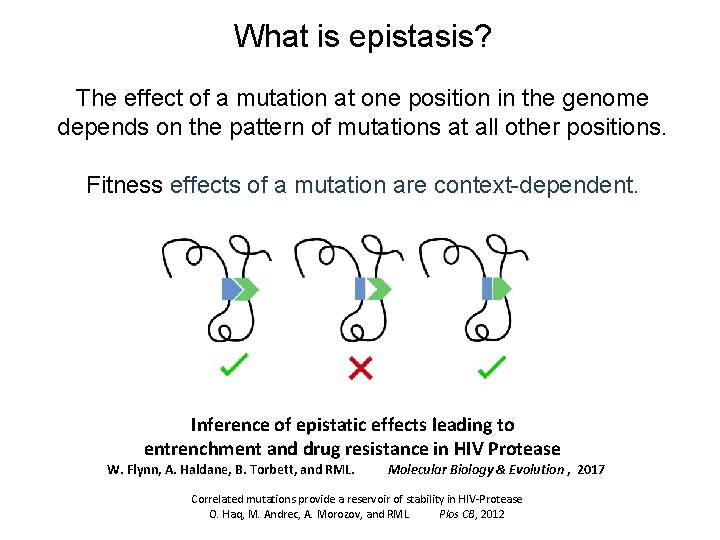
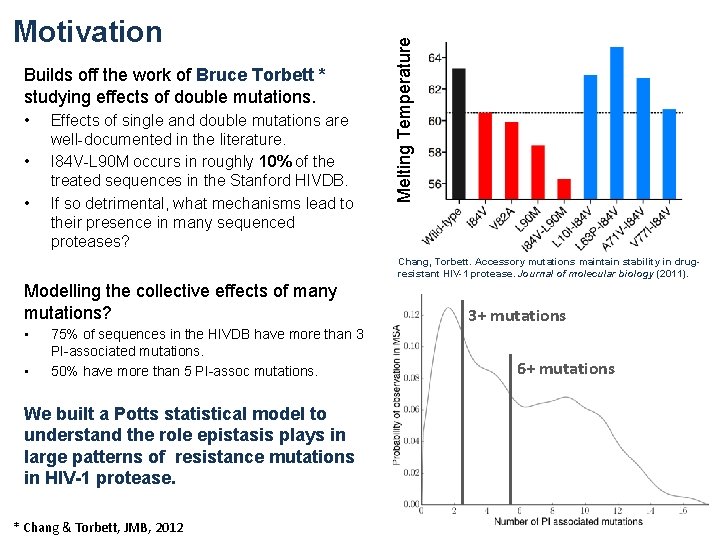
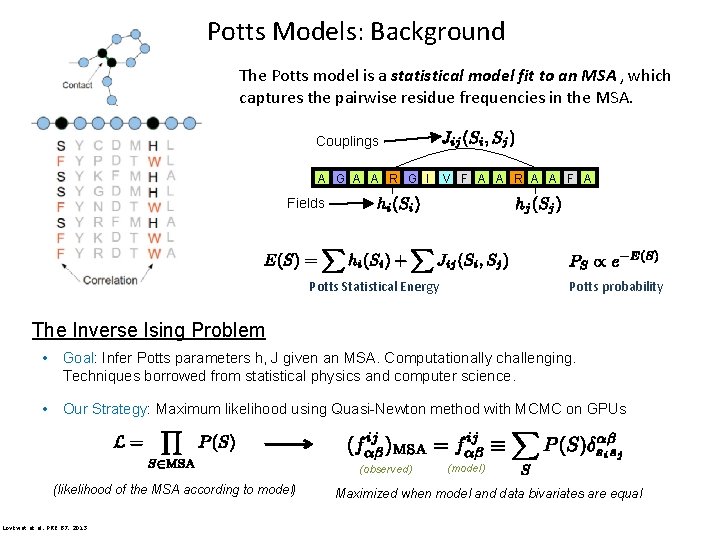
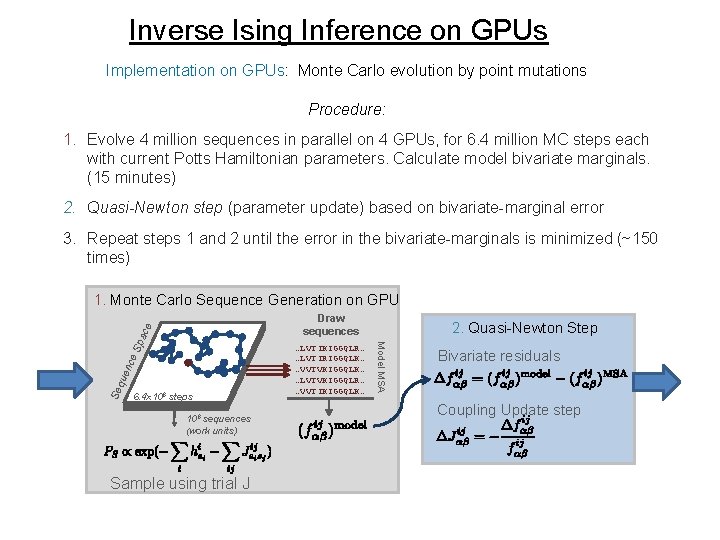
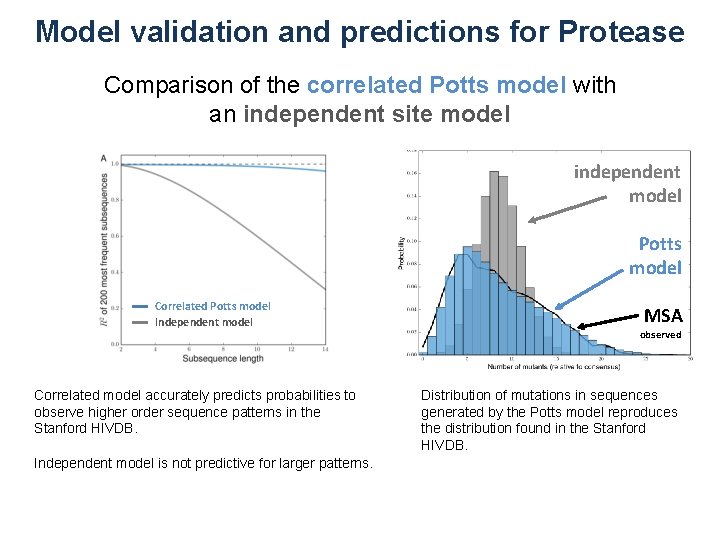
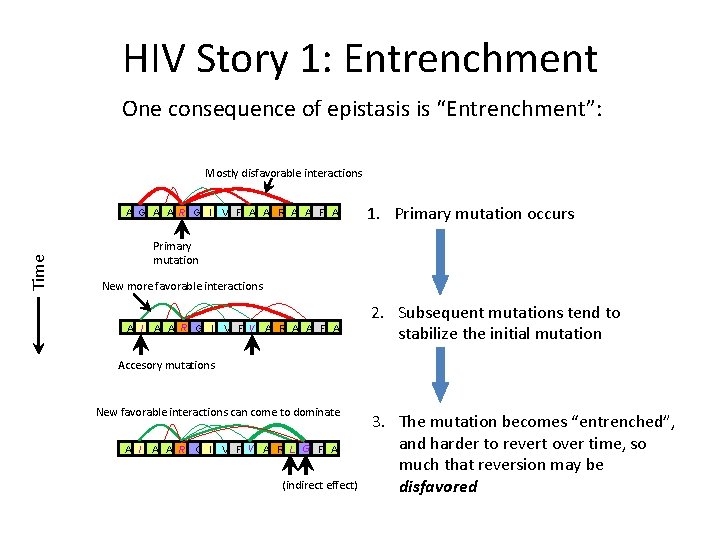
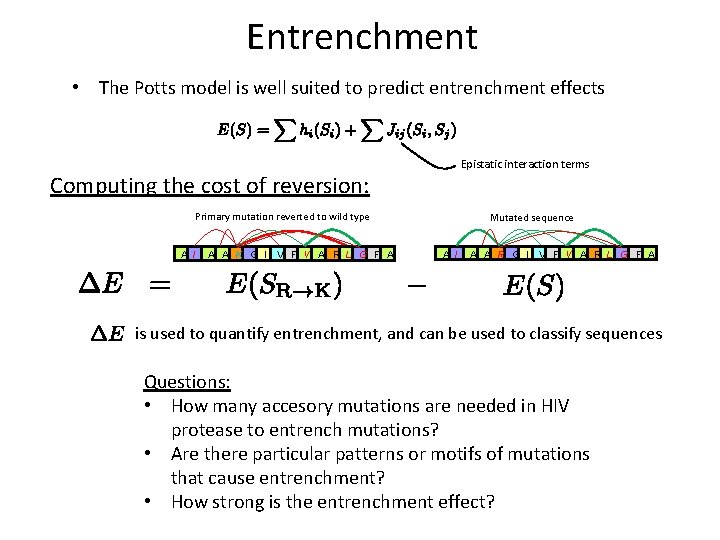
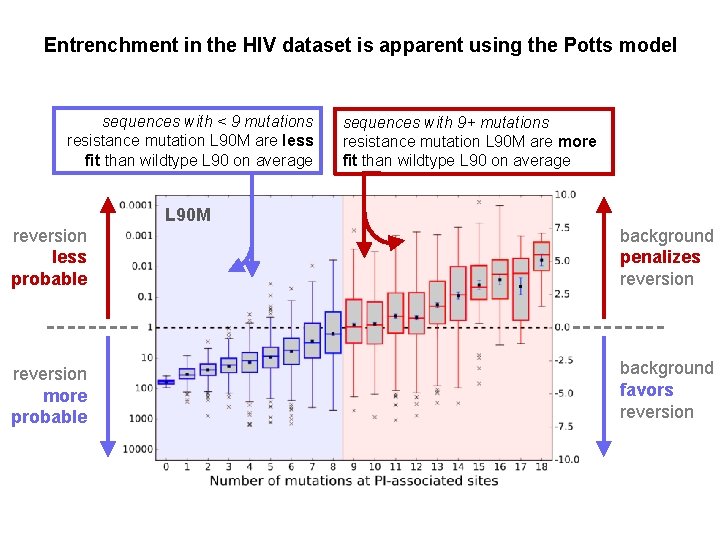
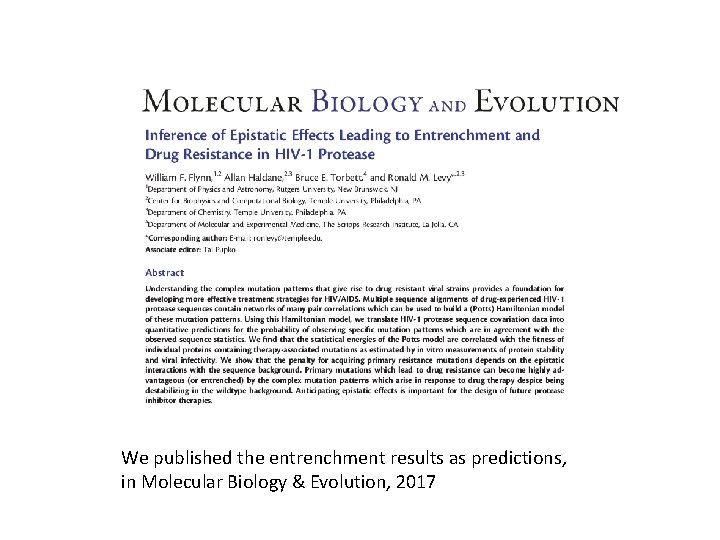
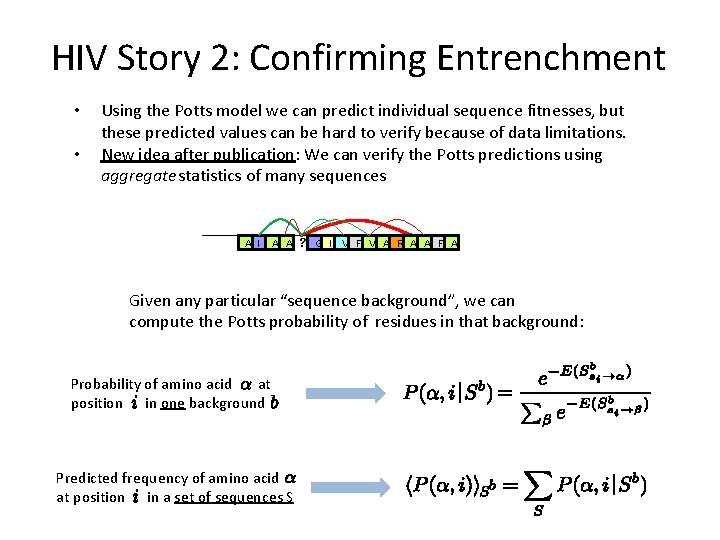
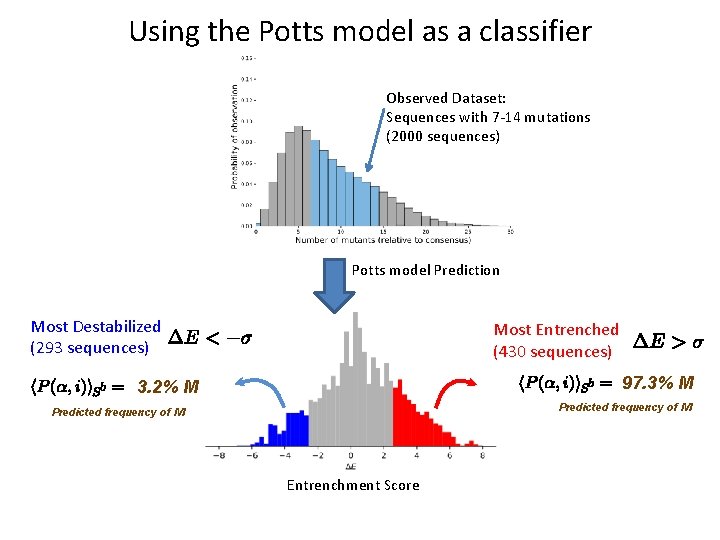
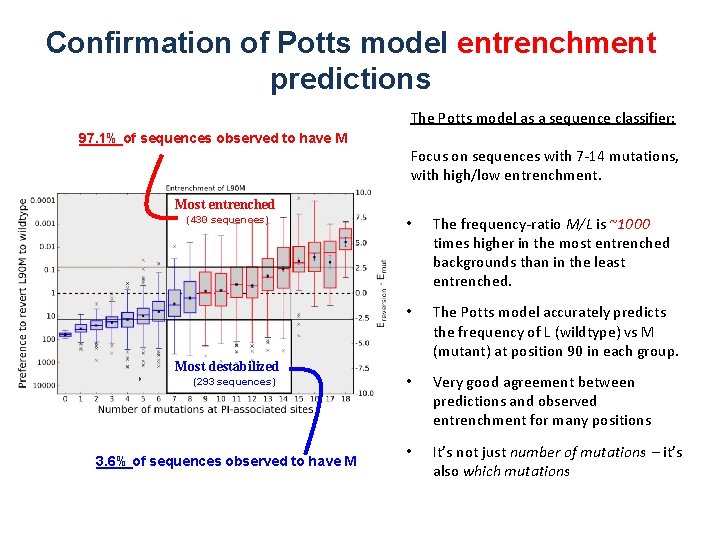
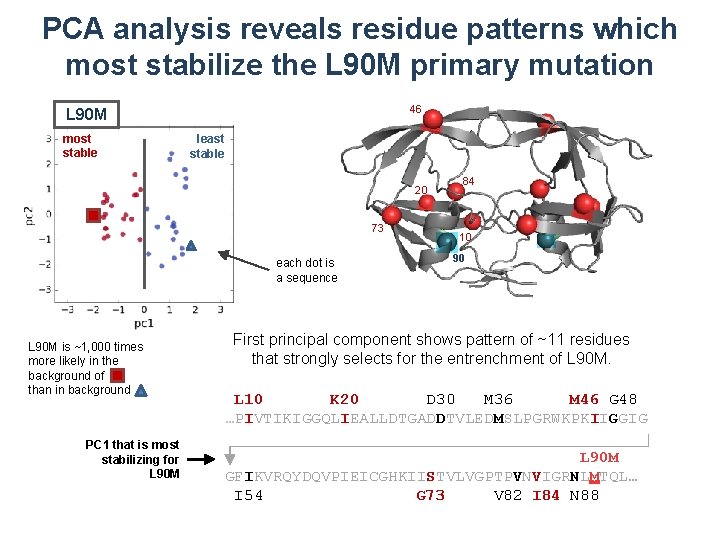
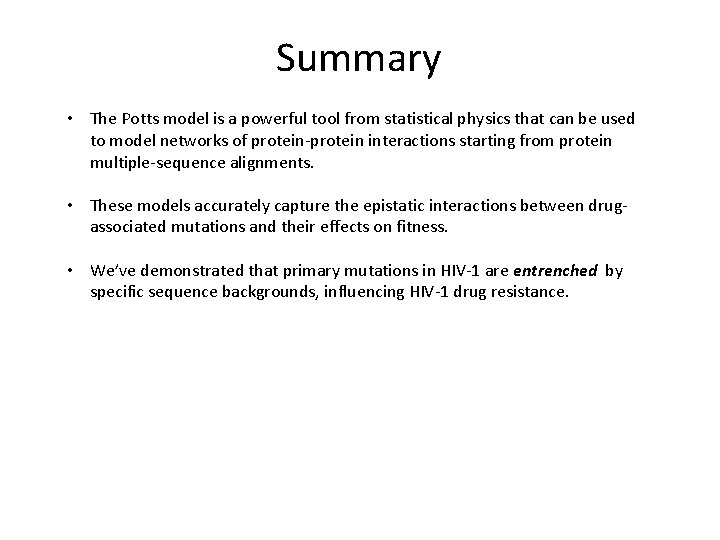
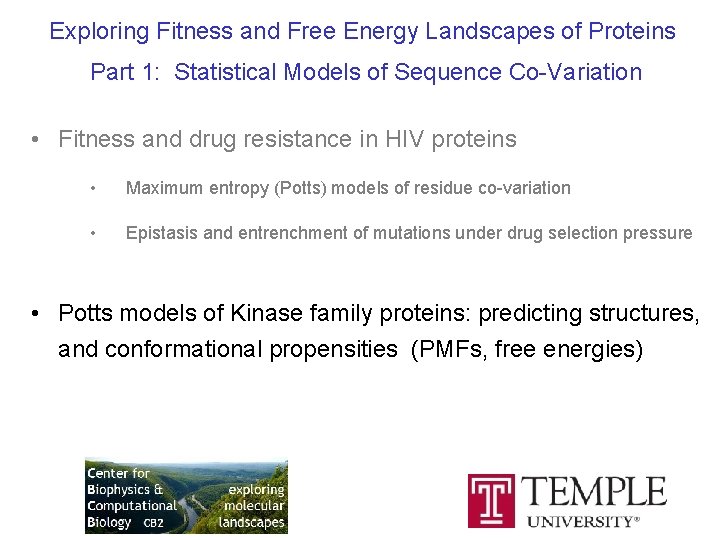
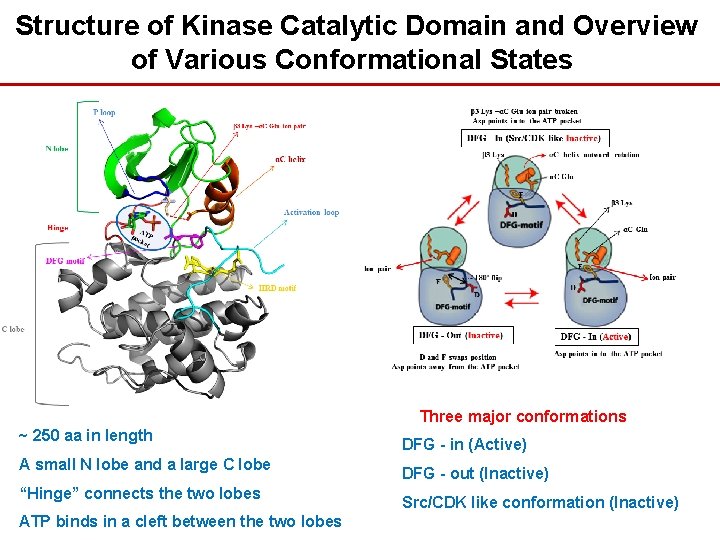
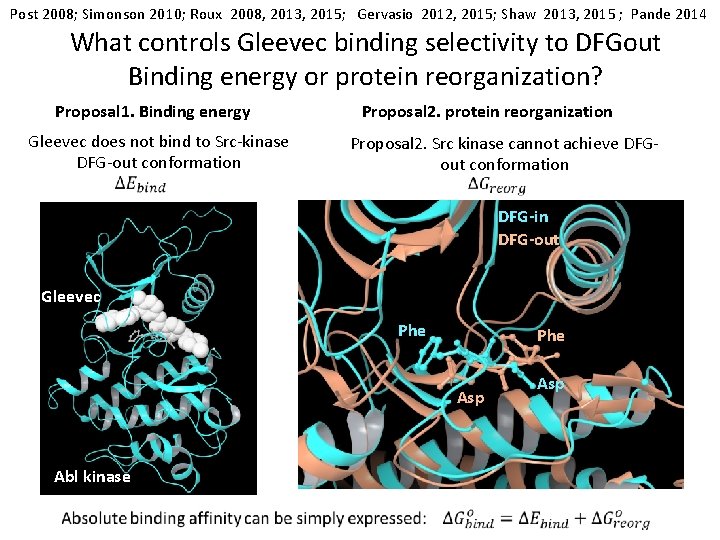
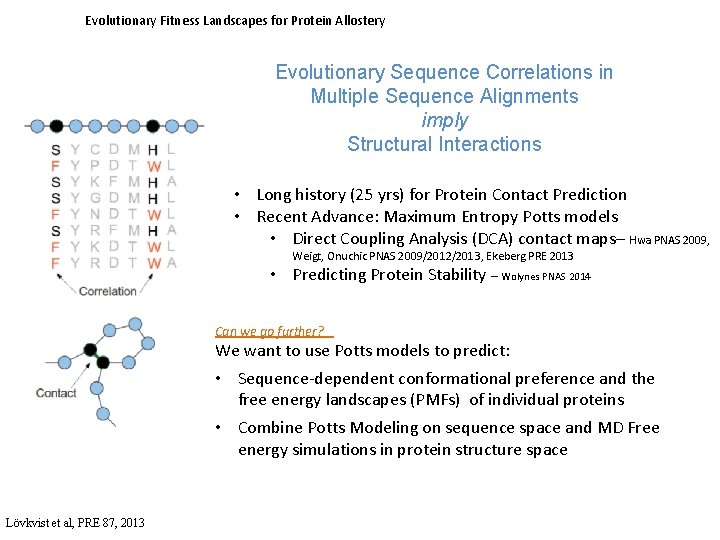
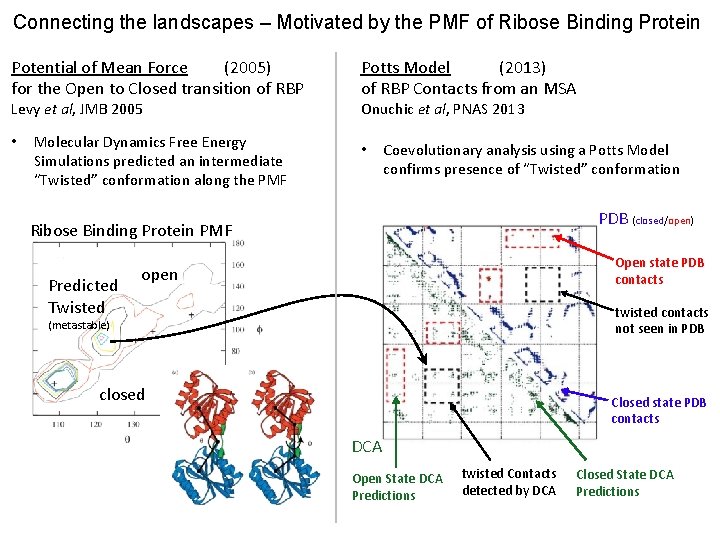
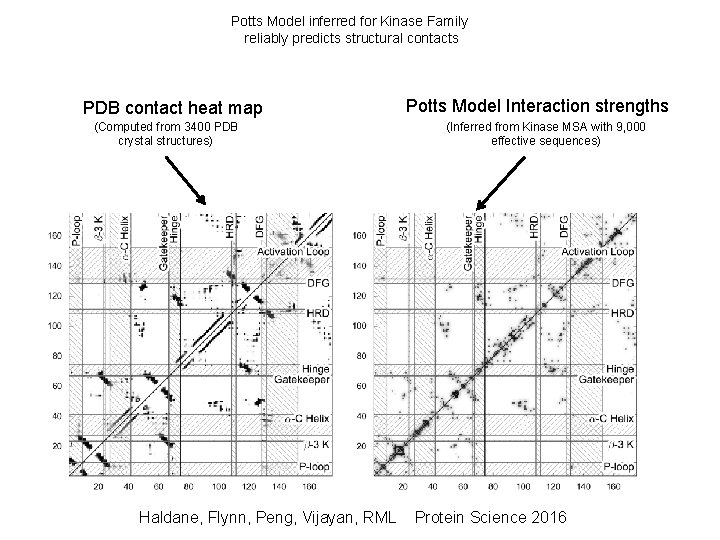
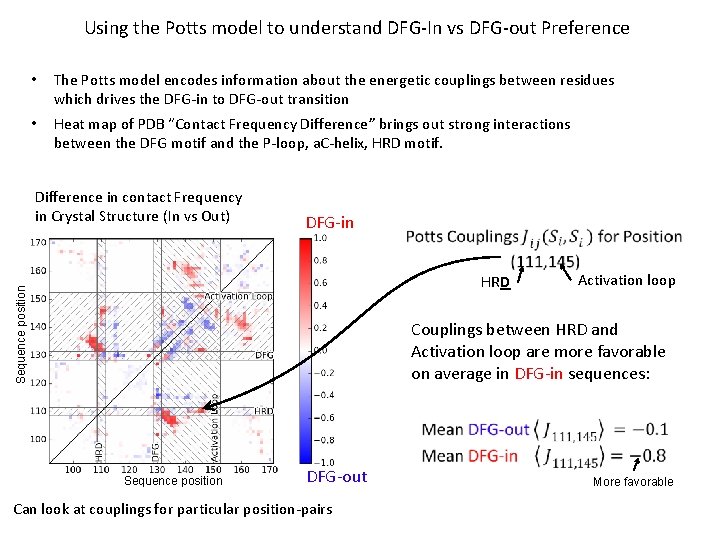
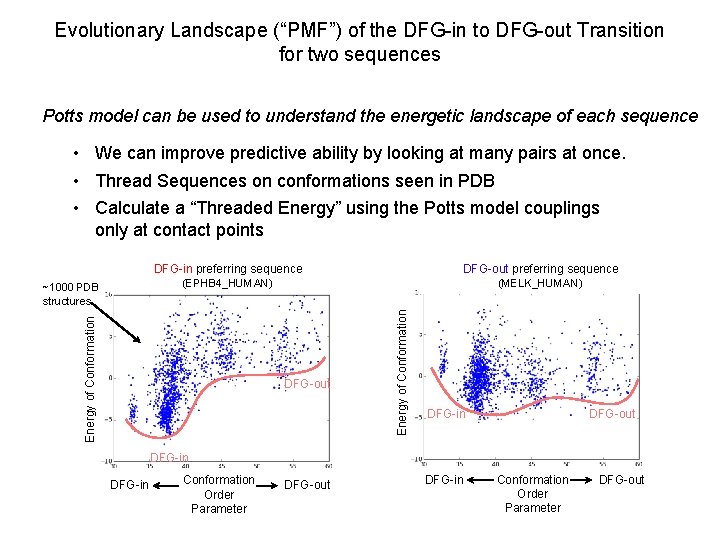
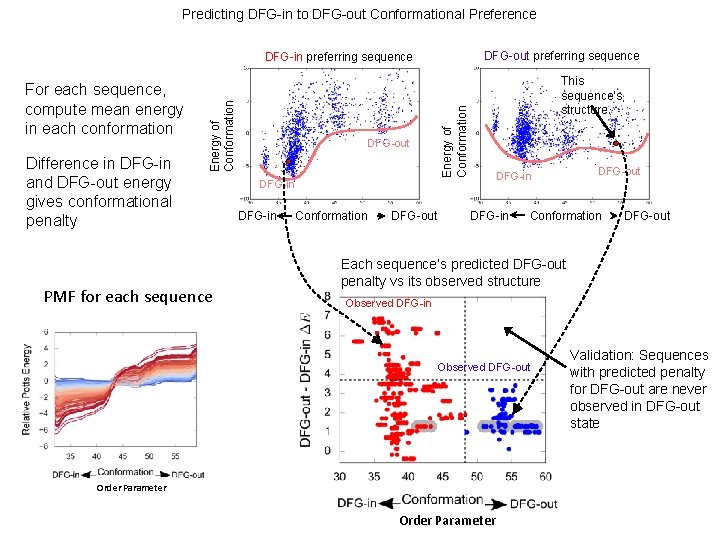
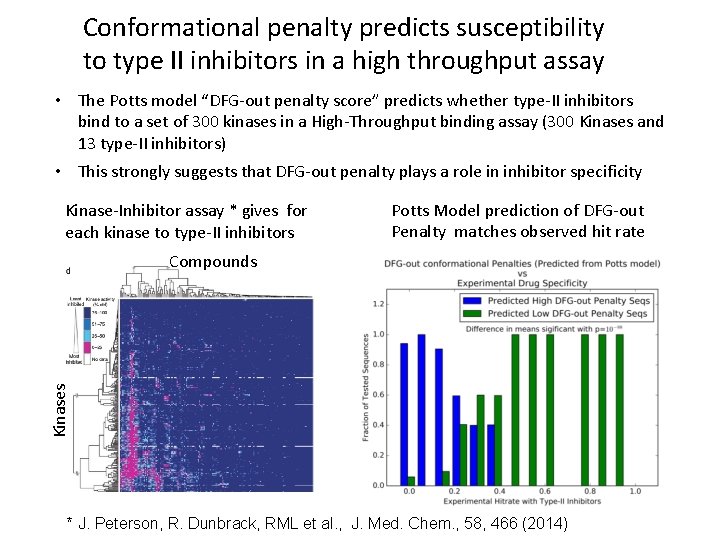
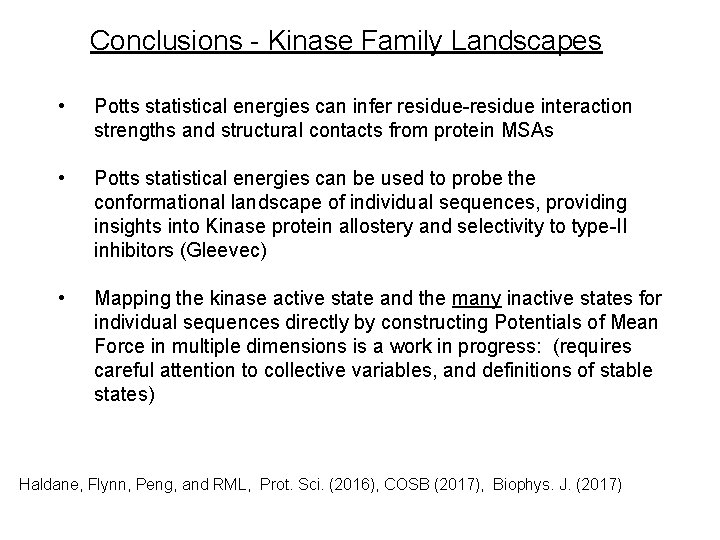
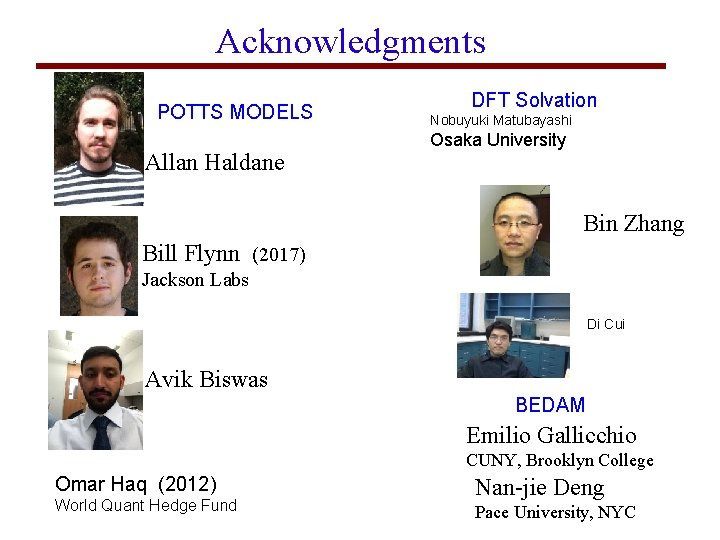
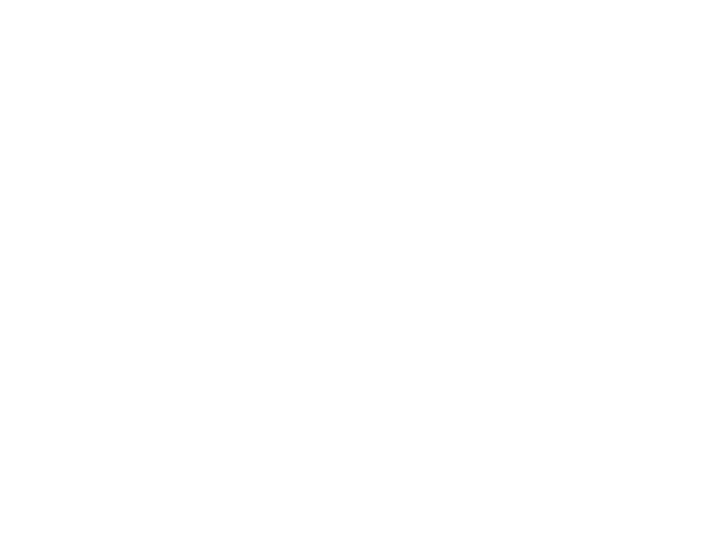
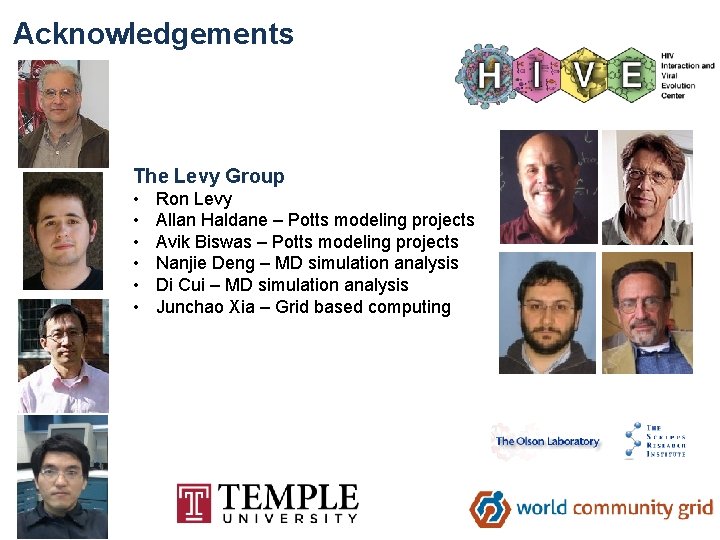
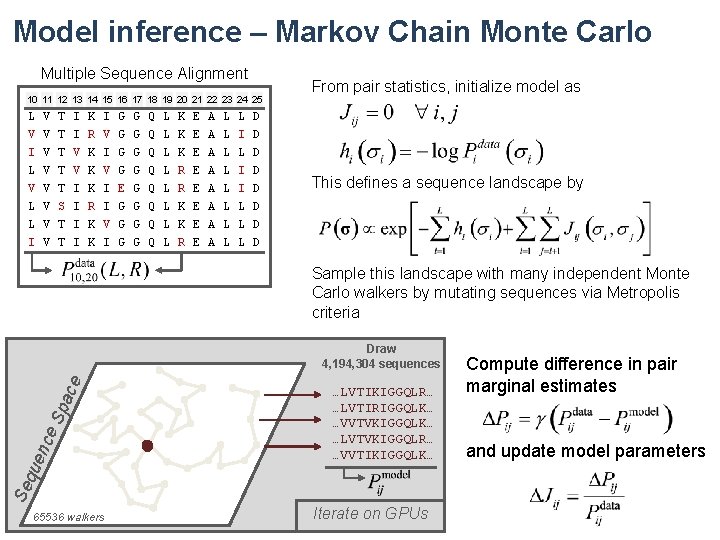
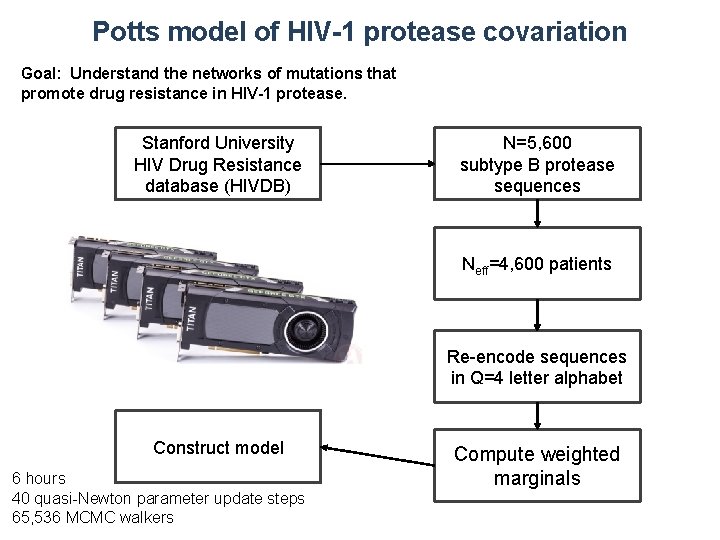
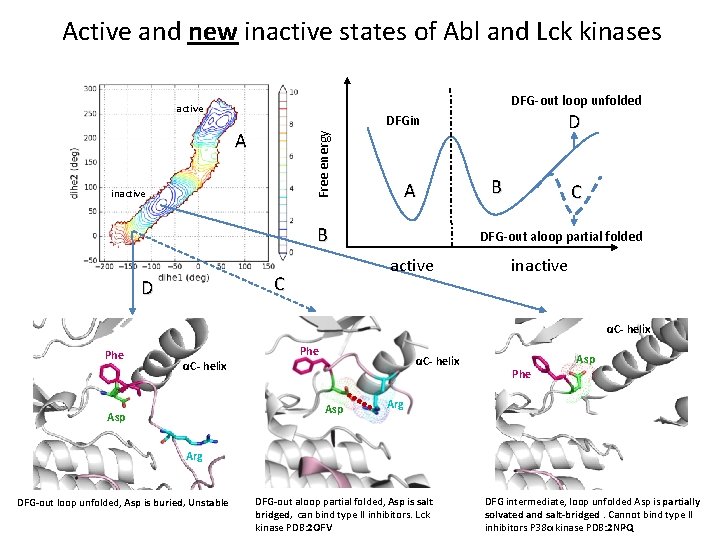
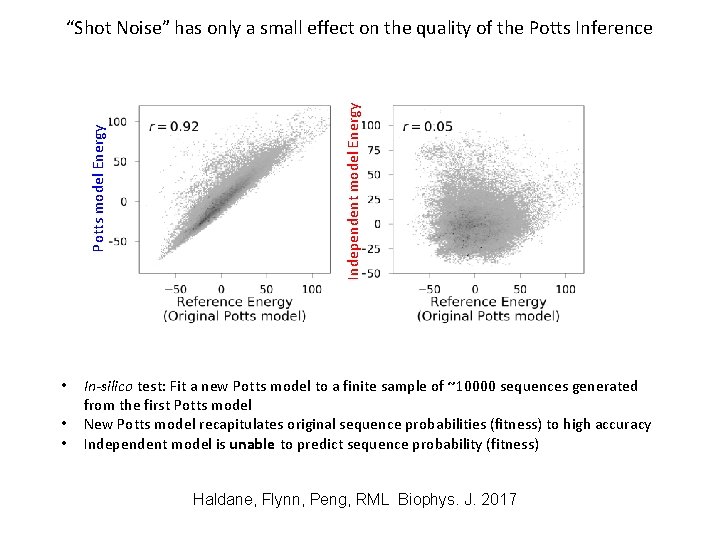
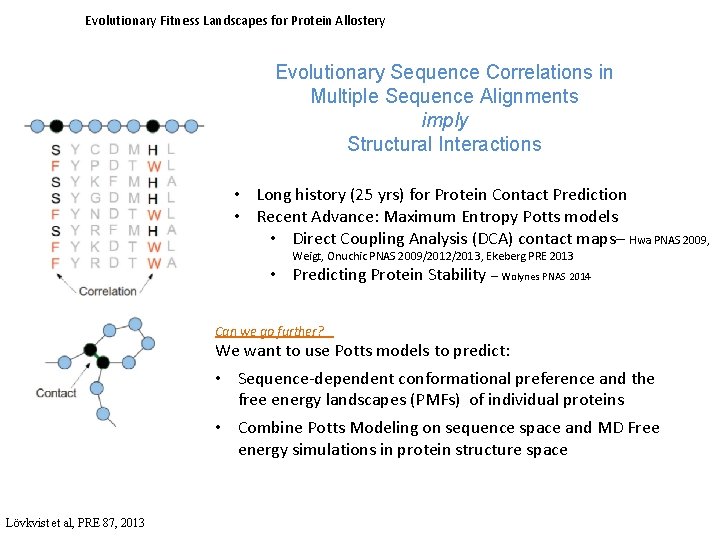
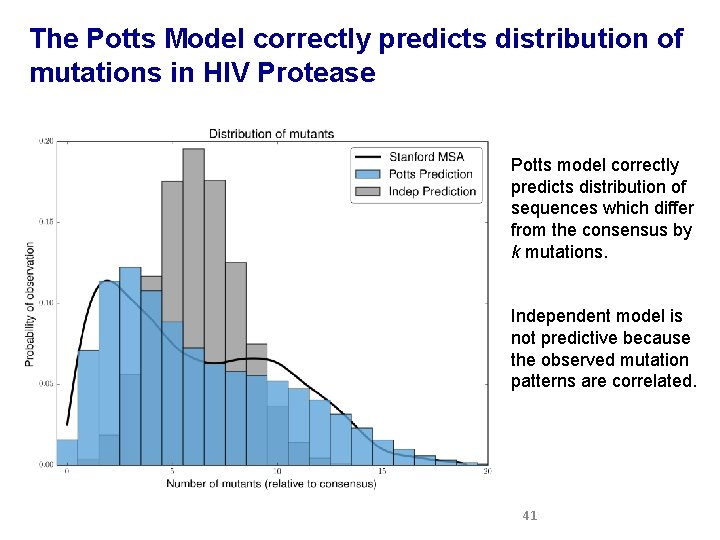
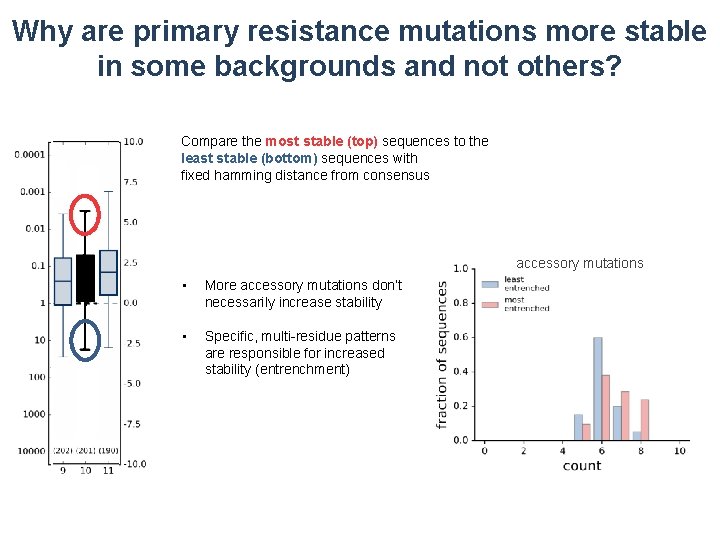
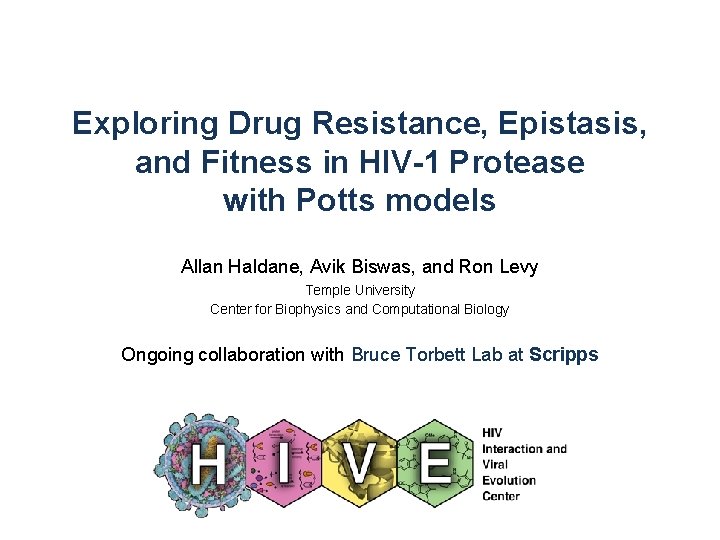
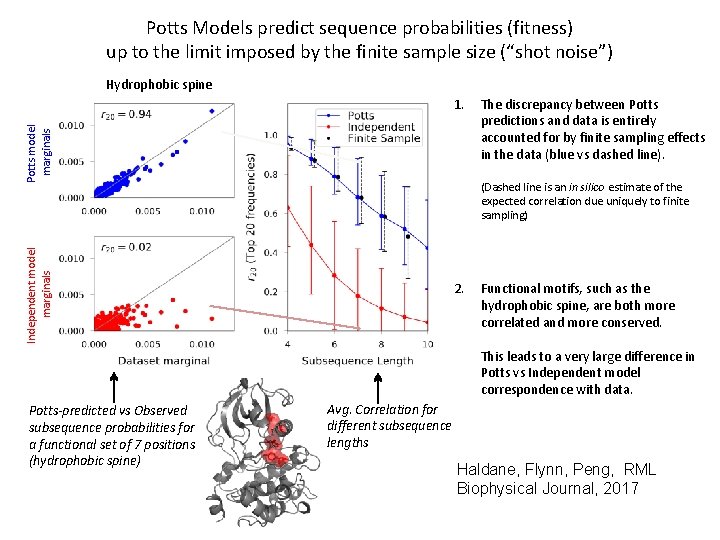
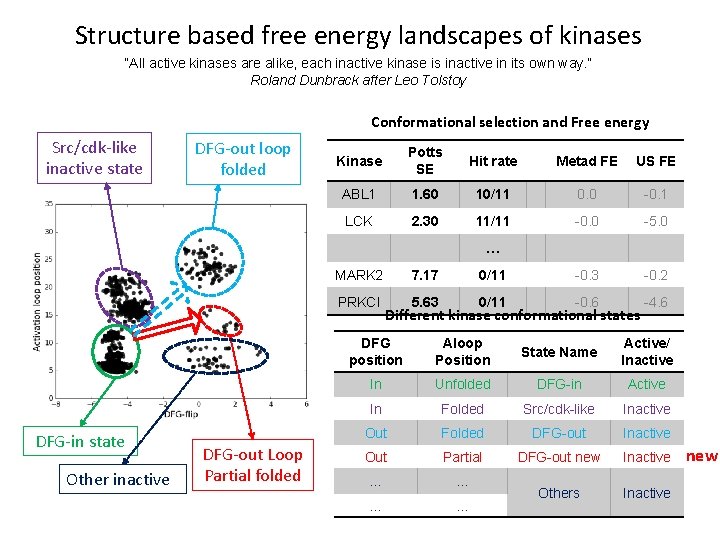
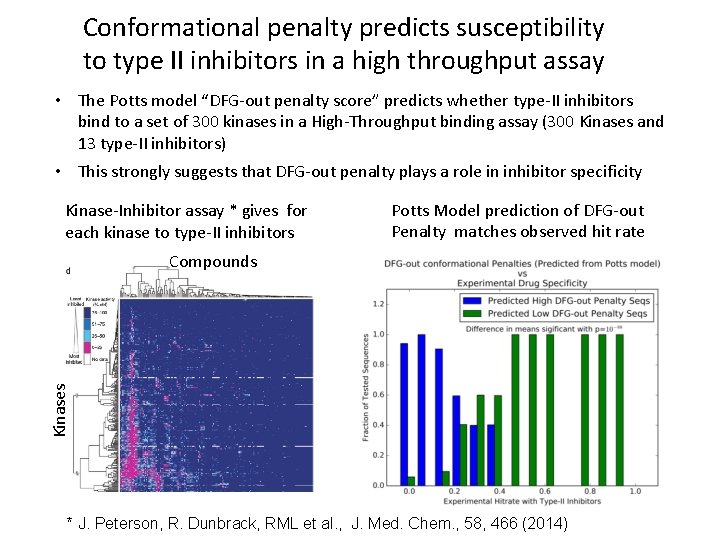
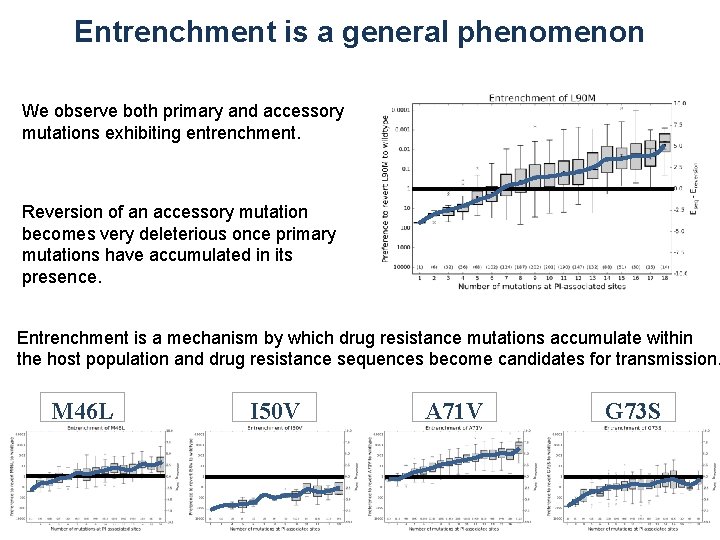
- Slides: 47
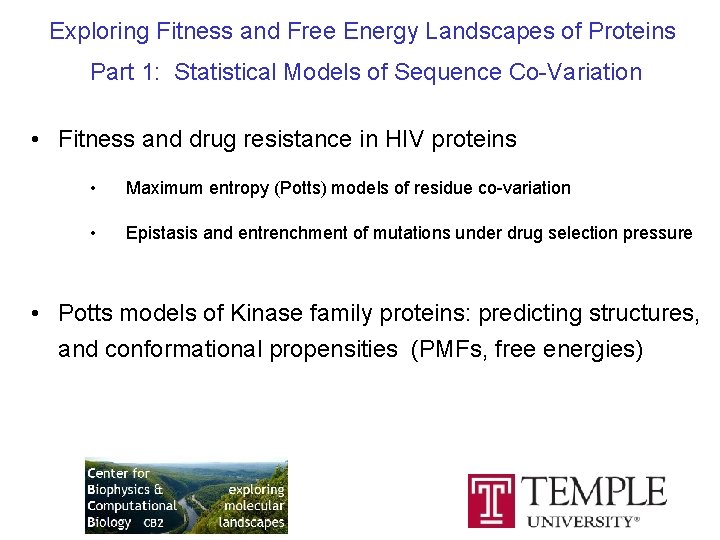
Exploring Fitness and Free Energy Landscapes of Proteins Part 1: Statistical Models of Sequence Co-Variation • Fitness and drug resistance in HIV proteins • Maximum entropy (Potts) models of residue co-variation • Epistasis and entrenchment of mutations under drug selection pressure • Potts models of Kinase family proteins: predicting structures, and conformational propensities (PMFs, free energies)
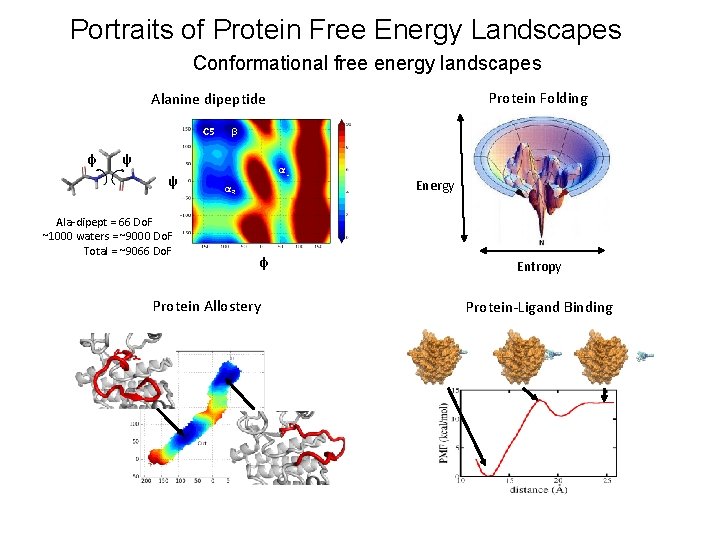
Portraits of Protein Free Energy Landscapes Conformational free energy landscapes Protein Folding Alanine dipeptide C 5 φ β ψ ψ Ala-dipept = 66 Do. F ~1000 waters = ~9000 Do. F Total = ~9066 Do. F αL αR φ Protein Allostery Energy Entropy Protein-Ligand Binding
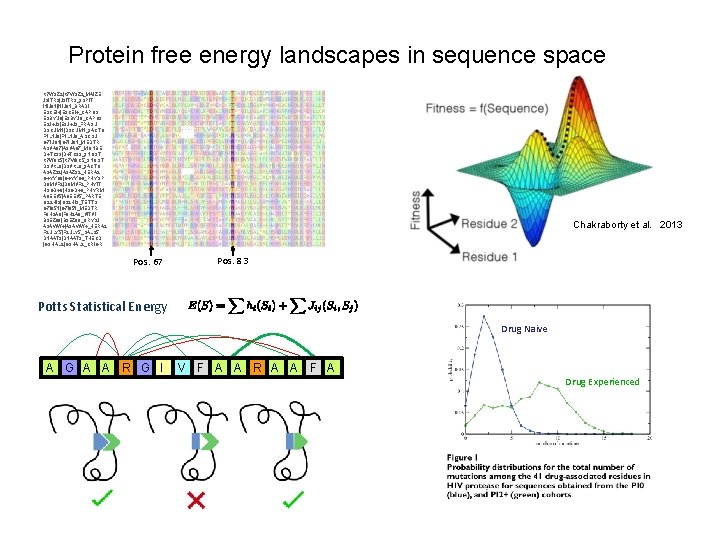
Protein free energy landscapes in sequence space K 7 W 9 Z 2|K 7 W 9 Z 2_MAIZE J 9 ITR 3|J 9 ITR 3_9 SPIT I 1 IJ 61|I 1 IJ 61_BRADI E 9 CEI 4|E 9 CEI 4_CAPO 3 E 9 BYU 8|E 9 BYU 8_CAPO 3 E 3 J 4 J 9|E 3 J 4 J 9_FRASU D 3 CUM 1|D 3 CUM 1_9 ACTO F 1 L 1 J 6|F 1 L 1 J 6_ASCSU G 7 IU 81|G 7 IU 81_MEDTR A 9 VA 07|A 9 VA 07_MONBE D 4 TC 93|D 4 TC 93_9 NOST K 7 W 6 C 5|K 7 W 6 C 5_9 NOST D 9 VKL 0|D 9 VKL 0_9 ACTO A 9 AZ 92|A 9 AZ 92_HERA 2 G 4 YYG 8|G 4 YYG 8_PHYSP D 0 MVF 2|D 0 MVF 2_PHYIT H 3 GD 48|H 3 GD 48_PHYRM A 0 EEV 5|A 0 EEV 5_PARTE Q 22 HI 9|Q 22 HI 9_TETTS G 7 I 651|G 7 I 651_MEDTR F 6 H 2 A 6|F 6 H 2 A 6_VITVI B 9 EZ 80|B 9 EZ 80_ORYSJ A 9 AWW 4|A 9 AWW 4_HERA 2 F 2 ULY 5|F 2 ULY 5_SALS 5 D 1 AAT 3|D 1 AAT 3_THECD |G 3 HAL 2_CRIGR Chakraborty et al. 2013 Pos. 67 Pos. 83 Potts Statistical Energy Drug Naive A G A A R G I V F A A R A A F A Drug Experienced
![ar Xiv 1207 2484 v 1 qbio QM ar. Xiv: 1207. 2484 v 1 [q-bio. QM]](https://slidetodoc.com/presentation_image_h/0aecad485edd5e56de5f54ba42c397a0/image-4.jpg)
ar. Xiv: 1207. 2484 v 1 [q-bio. QM]
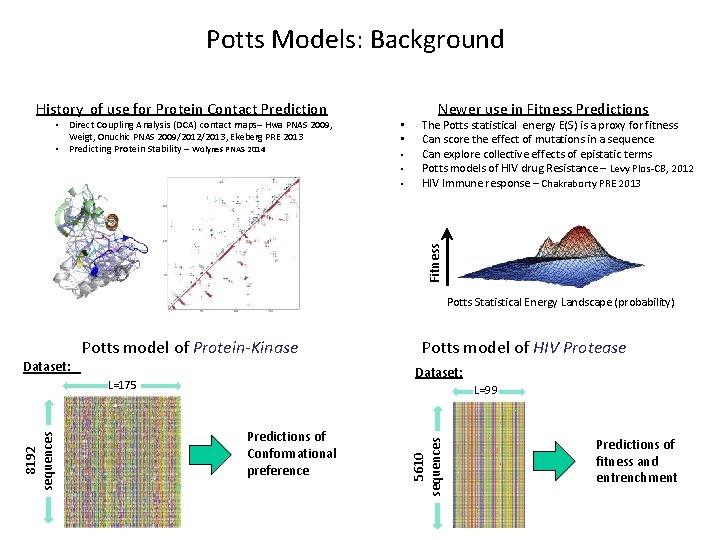
Potts Models: Background History of use for Protein Contact Prediction • • • Newer use in Fitness Predictions The Potts statistical energy E(S) is a proxy for fitness Can score the effect of mutations in a sequence Can explore collective effects of epistatic terms Potts models of HIV drug Resistance – Levy Plos-CB, 2012 HIV Immune response – Chakraborty PRE 2013 Fitness • Direct Coupling Analysis (DCA) contact maps– Hwa PNAS 2009, Weigt, Onuchic PNAS 2009/2012/2013, Ekeberg PRE 2013 • Predicting Protein Stability – Wolynes PNAS 2014 Potts Statistical Energy Landscape (probability) Potts model of Protein-Kinase Dataset: L=175 L=99 Predictions of Conformational preference 5610 sequences 8192 sequences Potts model of HIV Protease Predictions of fitness and entrenchment
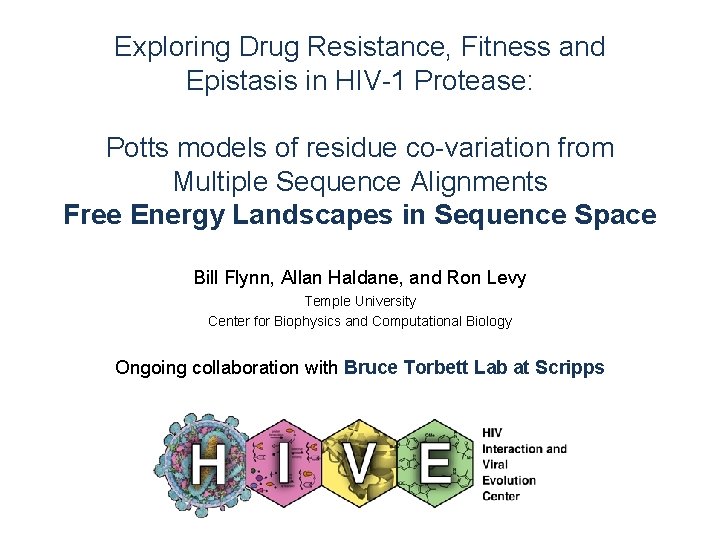
Exploring Drug Resistance, Fitness and Epistasis in HIV-1 Protease: Potts models of residue co-variation from Multiple Sequence Alignments Free Energy Landscapes in Sequence Space Bill Flynn, Allan Haldane, and Ron Levy Temple University Center for Biophysics and Computational Biology Ongoing collaboration with Bruce Torbett Lab at Scripps
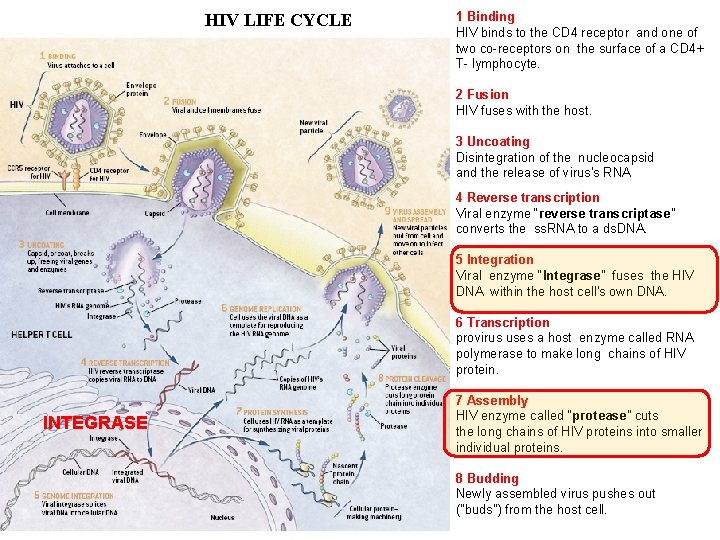
HIV LIFE CYCLE 1 Binding HIV binds to the CD 4 receptor and one of two co-receptors on the surface of a CD 4+ T- lymphocyte. 2 Fusion HIV fuses with the host. 3 Uncoating Disintegration of the nucleocapsid and the release of virus's RNA 4 Reverse transcription Viral enzyme “reverse transcriptase” converts the ss. RNA to a ds. DNA. 5 Integration Viral enzyme “Integrase" fuses the HIV DNA within the host cell's own DNA. 6 Transcription provirus uses a host enzyme called RNA polymerase to make long chains of HIV protein. INTEGRASE 7 Assembly HIV enzyme called “protease” cuts the long chains of HIV proteins into smaller individual proteins. 8 Budding Newly assembled virus pushes out ("buds") from the host cell.
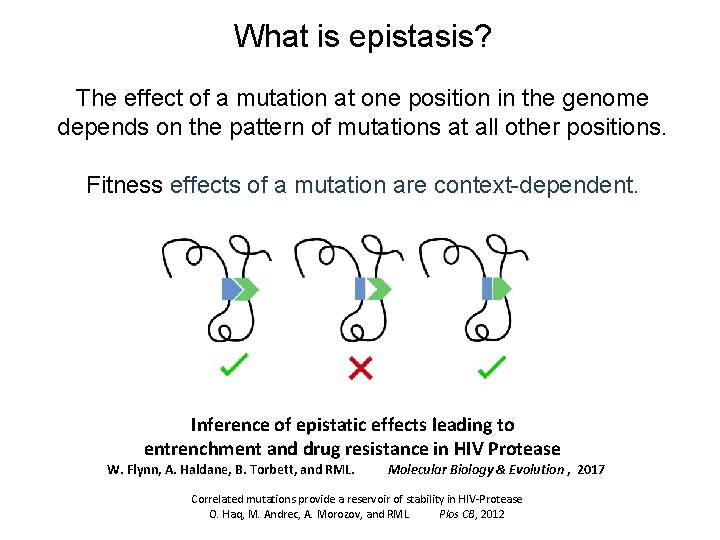
What is epistasis? The effect of a mutation at one position in the genome depends on the pattern of mutations at all other positions. Fitness effects of a mutation are context-dependent. Inference of epistatic effects leading to entrenchment and drug resistance in HIV Protease W. Flynn, A. Haldane, B. Torbett, and RML. Molecular Biology & Evolution , 2017 Correlated mutations provide a reservoir of stability in HIV-Protease O. Haq, M. Andrec, A. Morozov, and RML Plos CB, 2012
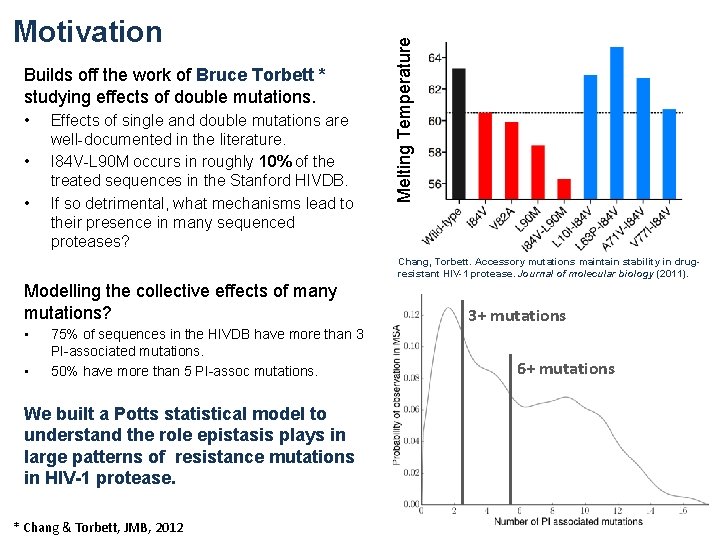
Builds off the work of Bruce Torbett * studying effects of double mutations. • • • Effects of single and double mutations are well-documented in the literature. I 84 V-L 90 M occurs in roughly 10% of the treated sequences in the Stanford HIVDB. If so detrimental, what mechanisms lead to their presence in many sequenced proteases? Melting Temperature Motivation Chang, Torbett. Accessory mutations maintain stability in drugresistant HIV-1 protease. Journal of molecular biology (2011). Modelling the collective effects of many mutations? • • 75% of sequences in the HIVDB have more than 3 PI-associated mutations. 50% have more than 5 PI-assoc mutations. We built a Potts statistical model to understand the role epistasis plays in large patterns of resistance mutations in HIV-1 protease. * Chang & Torbett, JMB, 2012 3+ mutations 6+ mutations
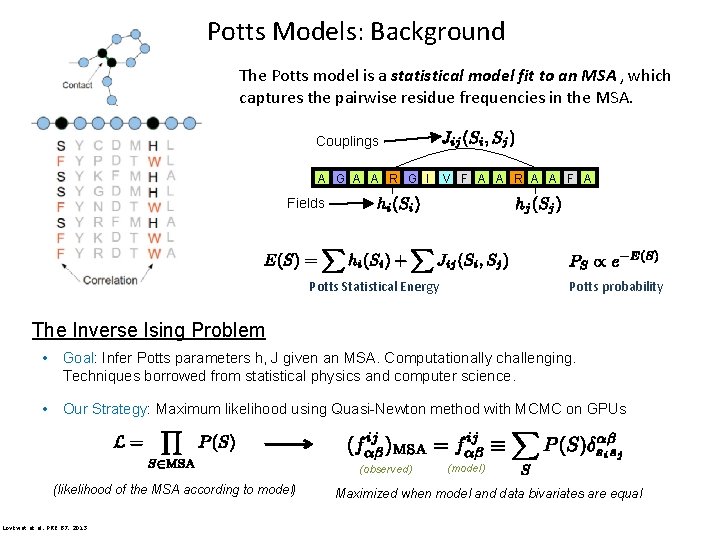
Potts Models: Background The Potts model is a statistical model fit to an MSA , which captures the pairwise residue frequencies in the MSA. Couplings A G A A R G I V F A A R A A Fields Potts Statistical Energy Potts probability The Inverse Ising Problem • Goal: Infer Potts parameters h, J given an MSA. Computationally challenging. Techniques borrowed from statistical physics and computer science. • Our Strategy: Maximum likelihood using Quasi-Newton method with MCMC on GPUs (observed) (likelihood of the MSA according to model) Lövkvist et al, PRE 87, 2013 (model) Maximized when model and data bivariates are equal
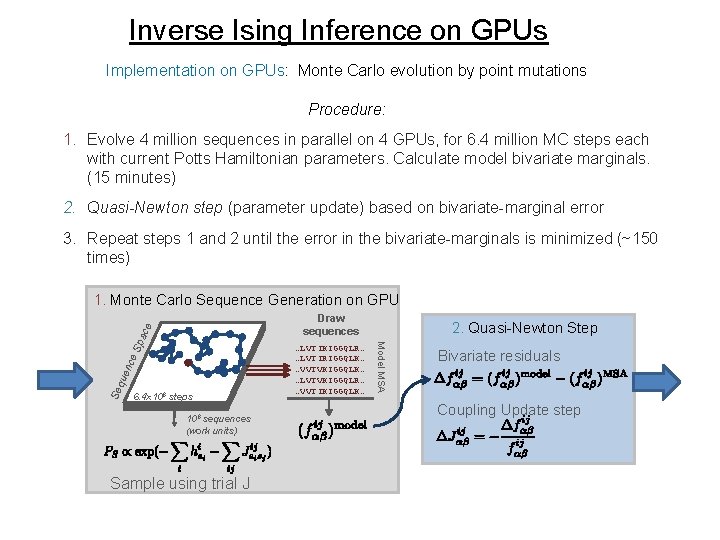
Inverse Ising Inference on GPUs Implementation on GPUs: Monte Carlo evolution by point mutations Procedure: 1. Evolve 4 million sequences in parallel on 4 GPUs, for 6. 4 million MC steps each with current Potts Hamiltonian parameters. Calculate model bivariate marginals. (15 minutes) 2. Quasi-Newton step (parameter update) based on bivariate-marginal error 3. Repeat steps 1 and 2 until the error in the bivariate-marginals is minimized (~150 times) 1. Monte Carlo Sequence Generation on GPU nce Se que 6. 4 x 106 steps 106 sequences (work units) Sample using trial J …LVTIKIGGQLR… …LVTIRIGGQLK… …VVTVKIGGQLK… …LVTVKIGGQLR… …VVTIKIGGQLK… 2. Quasi-Newton Step Model MSA Sp ace Draw sequences Bivariate residuals Coupling Update step
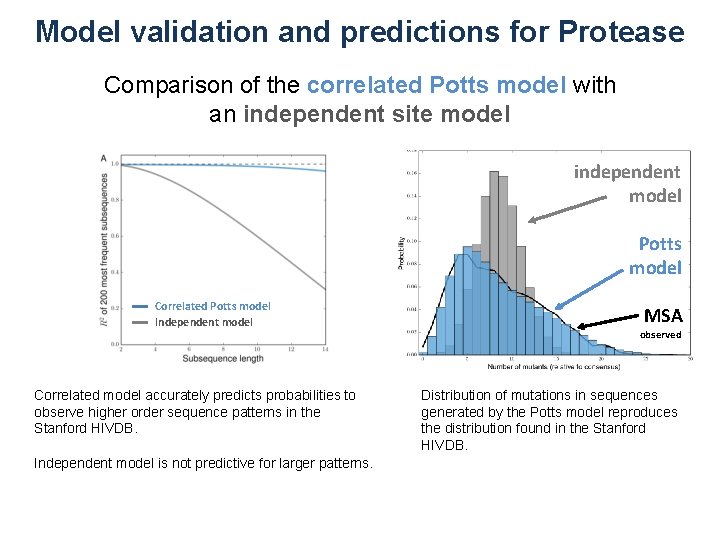
Model validation and predictions for Protease Comparison of the correlated Potts model with an independent site model independent model Potts model Correlated Potts model Independent model Correlated model accurately predicts probabilities to observe higher order sequence patterns in the Stanford HIVDB. Independent model is not predictive for larger patterns. MSA observed Distribution of mutations in sequences generated by the Potts model reproduces the distribution found in the Stanford HIVDB.
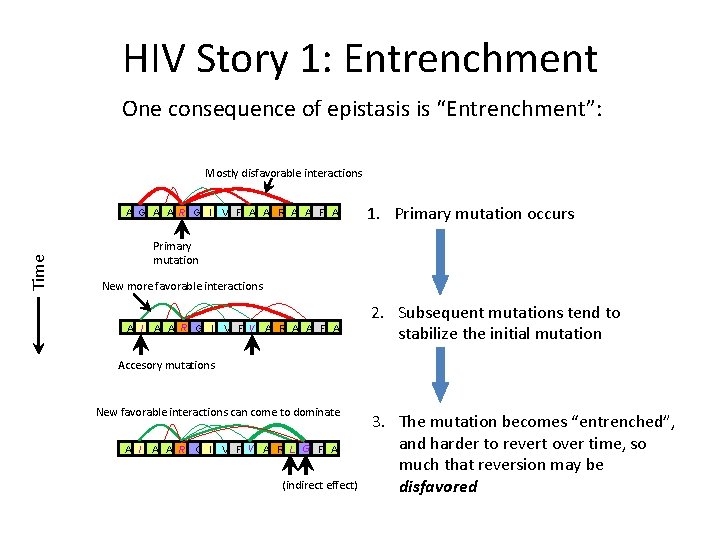
HIV Story 1: Entrenchment One consequence of epistasis is “Entrenchment”: Mostly disfavorable interactions Time A G A A R G I V F A A R A A F A 1. Primary mutation occurs Primary mutation New more favorable interactions A I A A R G I V F V A R A A F A 2. Subsequent mutations tend to stabilize the initial mutation Accesory mutations New favorable interactions can come to dominate A I A A R G I V F V A R L G F A (indirect effect) 3. The mutation becomes “entrenched”, and harder to revert over time, so much that reversion may be disfavored
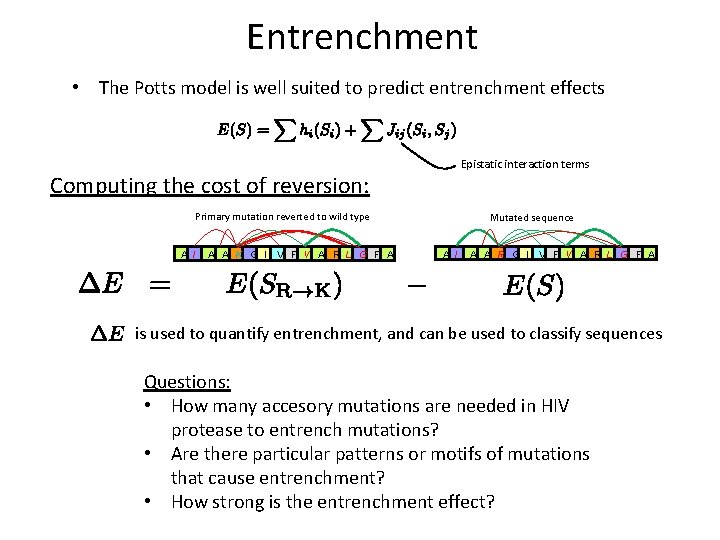
Entrenchment • The Potts model is well suited to predict entrenchment effects Epistatic interaction terms Computing the cost of reversion: Primary mutation reverted to wild type A I A A K G I V F V A R L G F A Mutated sequence A I A A R G I V F V A R L G F A is used to quantify entrenchment, and can be used to classify sequences Questions: • How many accesory mutations are needed in HIV protease to entrench mutations? • Are there particular patterns or motifs of mutations that cause entrenchment? • How strong is the entrenchment effect?
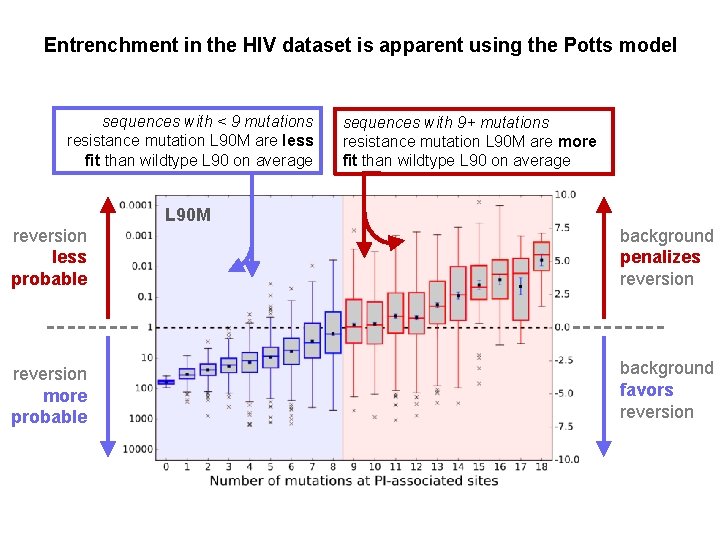
Entrenchment in the HIV dataset is apparent using the Potts model sequences with < 9 mutations resistance mutation L 90 M are less fit than wildtype L 90 on average sequences with 9+ mutations resistance mutation L 90 M are more fit than wildtype L 90 on average L 90 M reversion less probable background penalizes reversion more probable background favors reversion
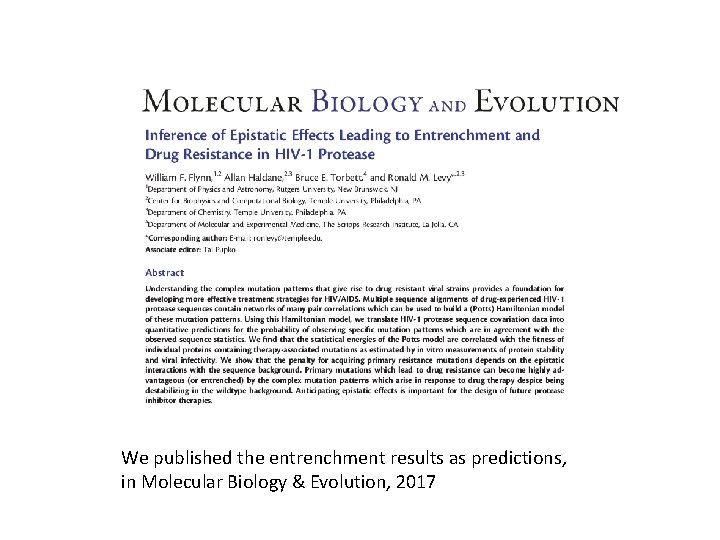
We published the entrenchment results as predictions, in Molecular Biology & Evolution, 2017
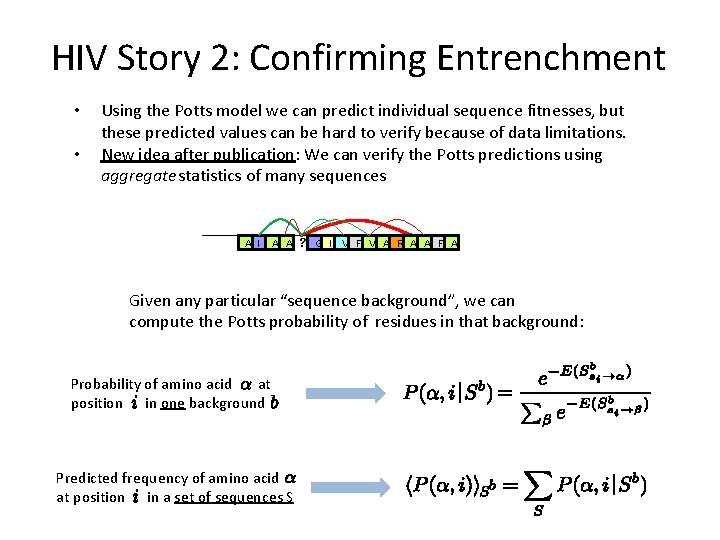
HIV Story 2: Confirming Entrenchment • • Using the Potts model we can predict individual sequence fitnesses, but these predicted values can be hard to verify because of data limitations. New idea after publication: We can verify the Potts predictions using aggregate statistics of many sequences A I A A ? G I V F V A R A A F A Given any particular “sequence background”, we can compute the Potts probability of residues in that background: Probability of amino acid at position in one background Predicted frequency of amino acid at position in a set of sequences S
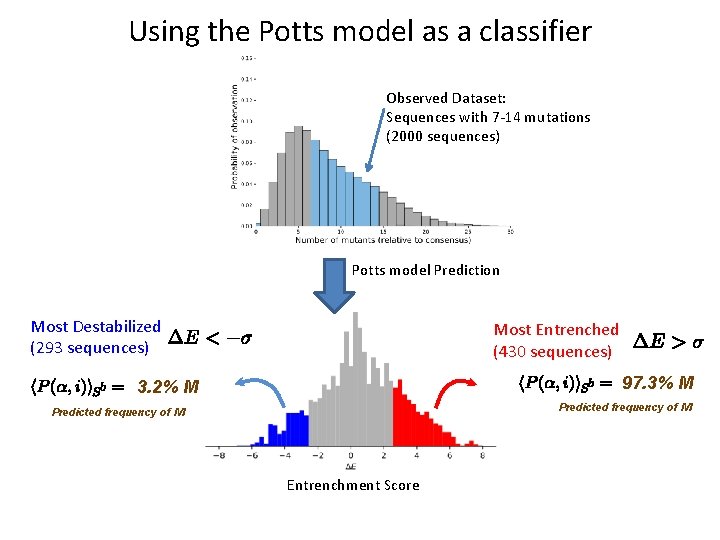
Using the Potts model as a classifier Observed Dataset: Sequences with 7 -14 mutations (2000 sequences) Potts model Prediction Most Destabilized (293 sequences) Most Entrenched (430 sequences) 97. 3% M 3. 2% M Predicted frequency of M Entrenchment Score
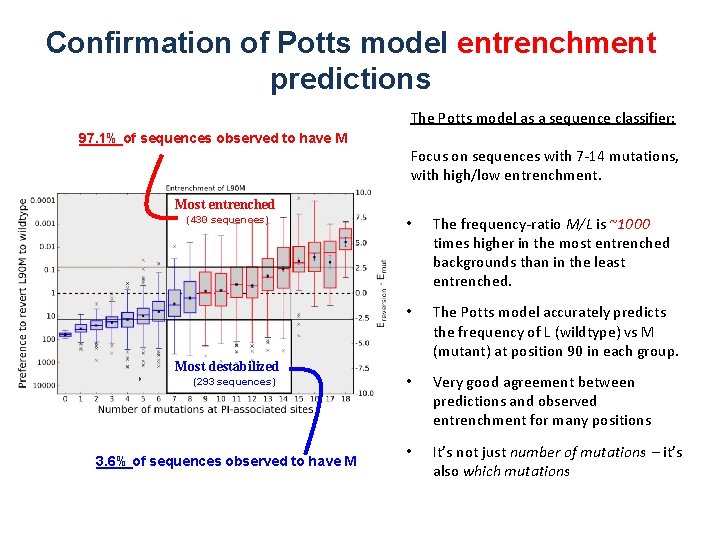
Confirmation of Potts model entrenchment predictions The Potts model as a sequence classifier: 97. 1% of sequences observed to have M Focus on sequences with 7 -14 mutations, with high/low entrenchment. Most entrenched (430 sequences) Most destabilized (293 sequences) 3. 6% of sequences observed to have M • The frequency-ratio M/L is ~1000 times higher in the most entrenched backgrounds than in the least entrenched. • The Potts model accurately predicts the frequency of L (wildtype) vs M (mutant) at position 90 in each group. • Very good agreement between predictions and observed entrenchment for many positions • It’s not just number of mutations – it’s also which mutations
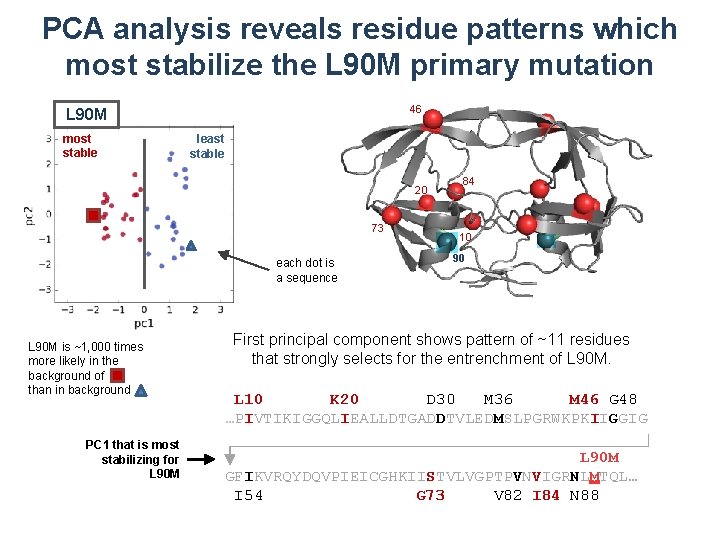
PCA analysis reveals residue patterns which most stabilize the L 90 M primary mutation 46 L 90 M most stable least stable 20 73 each dot is a sequence L 90 M is ~1, 000 times more likely in the background of than in background PC 1 that is most stabilizing for L 90 M 84 10 90 First principal component shows pattern of ~11 residues that strongly selects for the entrenchment of L 90 M. L 10 K 20 D 30 M 36 M 46 G 48 …PIVTIKIGGQLIEALLDTGADDTVLEDMSLPGRWKPKIIGGIG L 90 M GFIKVRQYDQVPIEICGHKIISTVLVGPTPVNVIGRNLMTQL… I 54 G 73 V 82 I 84 N 88
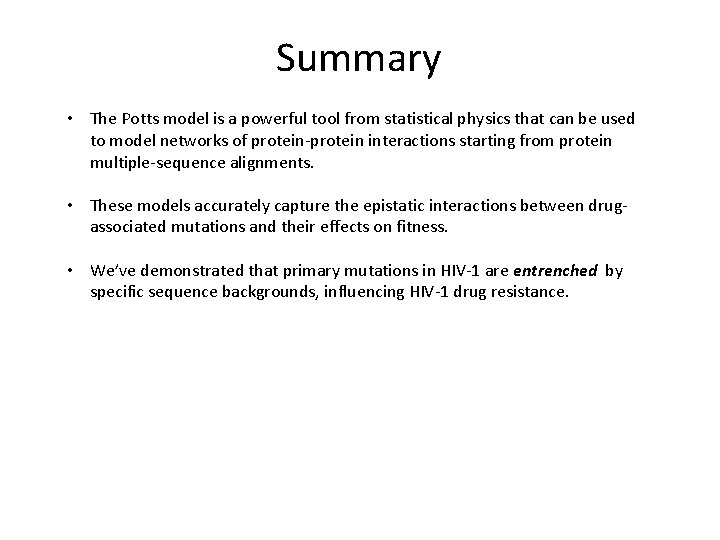
Summary • The Potts model is a powerful tool from statistical physics that can be used to model networks of protein-protein interactions starting from protein multiple-sequence alignments. • These models accurately capture the epistatic interactions between drugassociated mutations and their effects on fitness. • We’ve demonstrated that primary mutations in HIV-1 are entrenched by specific sequence backgrounds, influencing HIV-1 drug resistance.
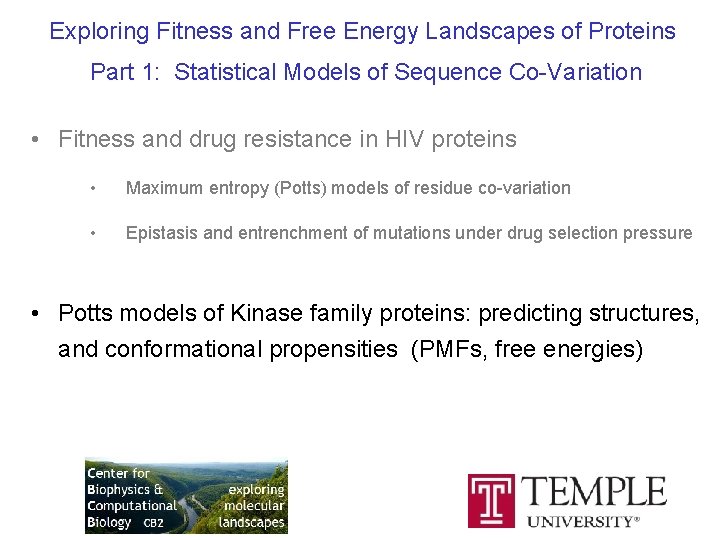
Exploring Fitness and Free Energy Landscapes of Proteins Part 1: Statistical Models of Sequence Co-Variation • Fitness and drug resistance in HIV proteins • Maximum entropy (Potts) models of residue co-variation • Epistasis and entrenchment of mutations under drug selection pressure • Potts models of Kinase family proteins: predicting structures, and conformational propensities (PMFs, free energies)
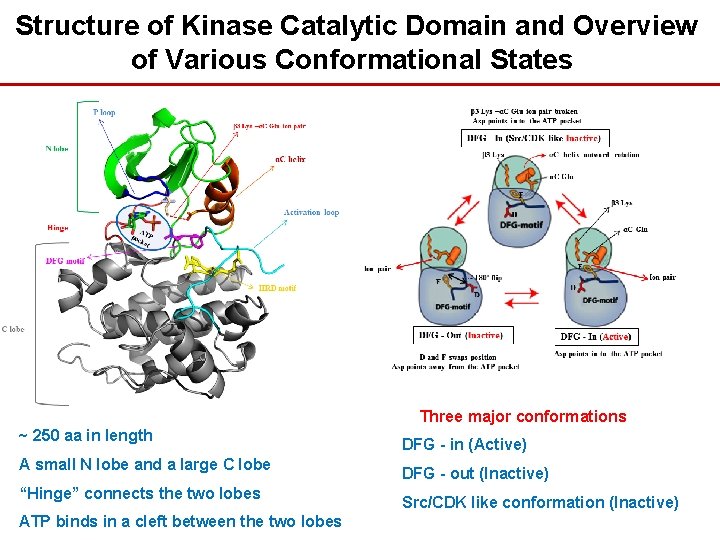
Structure of Kinase Catalytic Domain and Overview of Various Conformational States Three major conformations ~ 250 aa in length A small N lobe and a large C lobe “Hinge” connects the two lobes ATP binds in a cleft between the two lobes DFG - in (Active) DFG - out (Inactive) Src/CDK like conformation (Inactive)
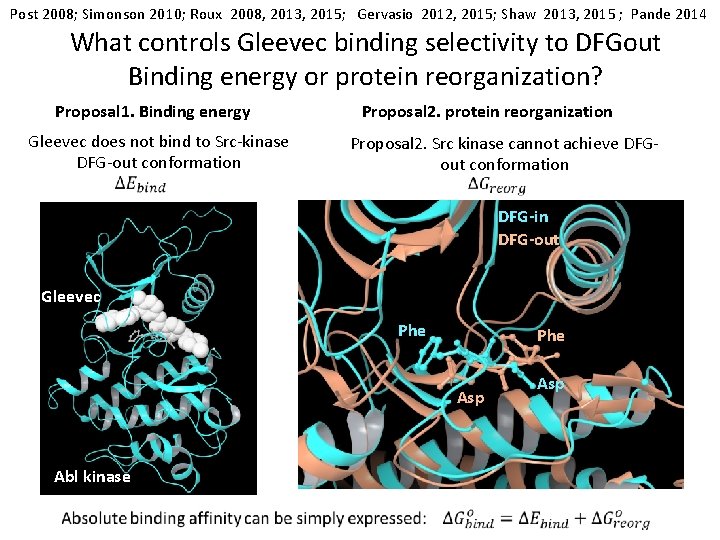
Post 2008; Simonson 2010; Roux 2008, 2013, 2015; Gervasio 2012, 2015; Shaw 2013, 2015 ; Pande 2014 What controls Gleevec binding selectivity to DFGout Binding energy or protein reorganization? Proposal 1. Binding energy Gleevec does not bind to Src-kinase DFG-out conformation Proposal 2. protein reorganization Proposal 2. Src kinase cannot achieve DFGout conformation DFG-in DFG-out Gleevec Phe Asp Abl kinase Asp
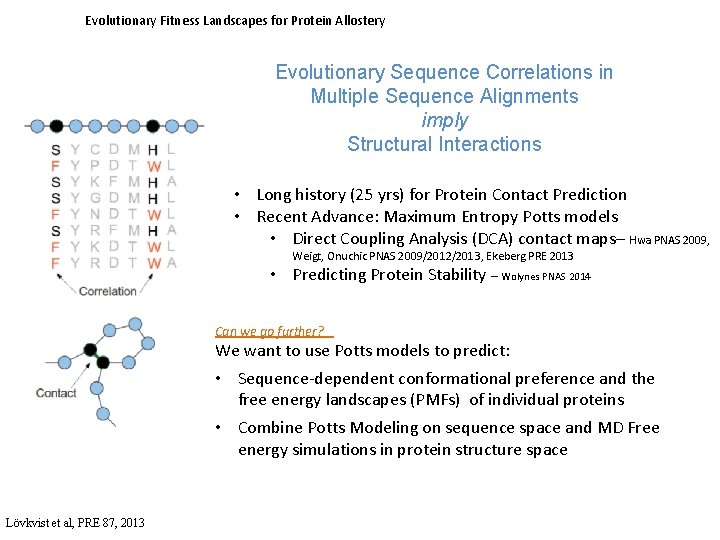
Evolutionary Fitness Landscapes for Protein Allostery Evolutionary Sequence Correlations in Multiple Sequence Alignments imply Structural Interactions • Long history (25 yrs) for Protein Contact Prediction • Recent Advance: Maximum Entropy Potts models • Direct Coupling Analysis (DCA) contact maps– Hwa PNAS 2009, Weigt, Onuchic PNAS 2009/2012/2013, Ekeberg PRE 2013 • Predicting Protein Stability – Wolynes PNAS 2014 Can we go further? We want to use Potts models to predict: • Sequence-dependent conformational preference and the free energy landscapes (PMFs) of individual proteins • Combine Potts Modeling on sequence space and MD Free energy simulations in protein structure space Lövkvist et al, PRE 87, 2013
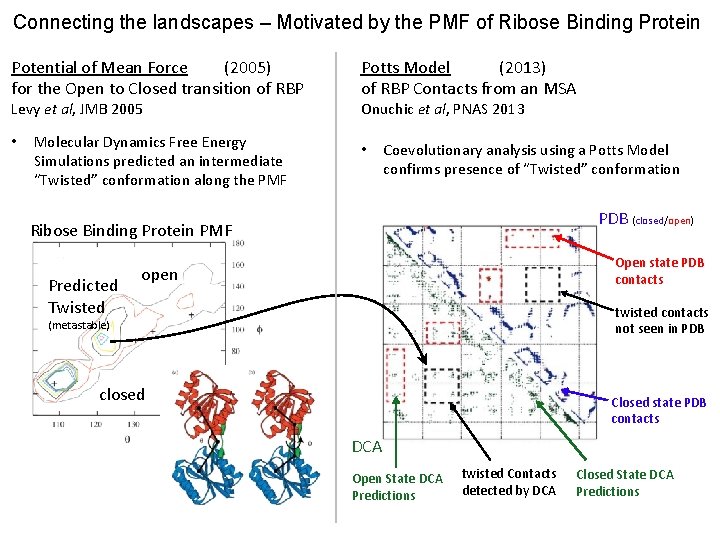
Connecting the landscapes – Motivated by the PMF of Ribose Binding Protein Potential of Mean Force (2005) for the Open to Closed transition of RBP Levy et al, JMB 2005 • Molecular Dynamics Free Energy Simulations predicted an intermediate “Twisted” conformation along the PMF Potts Model (2013) of RBP Contacts from an MSA Onuchic et al, PNAS 2013 • Coevolutionary analysis using a Potts Model confirms presence of “Twisted” conformation PDB (closed/open) Ribose Binding Protein PMF Predicted Twisted Open state PDB contacts open twisted contacts not seen in PDB (metastable) closed Closed state PDB contacts DCA Open State DCA Predictions twisted Contacts detected by DCA Closed State DCA Predictions
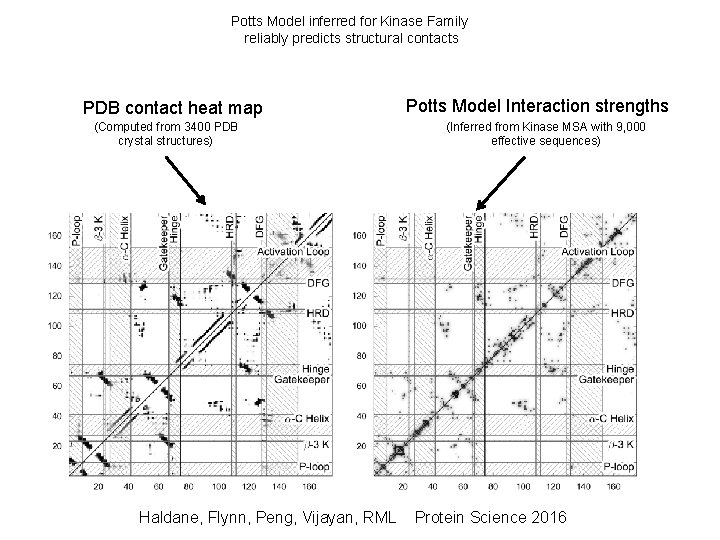
Potts Model inferred for Kinase Family reliably predicts structural contacts PDB contact heat map (Computed from 3400 PDB crystal structures) Haldane, Flynn, Peng, Vijayan, RML Potts Model Interaction strengths (Inferred from Kinase MSA with 9, 000 effective sequences) Protein Science 2016
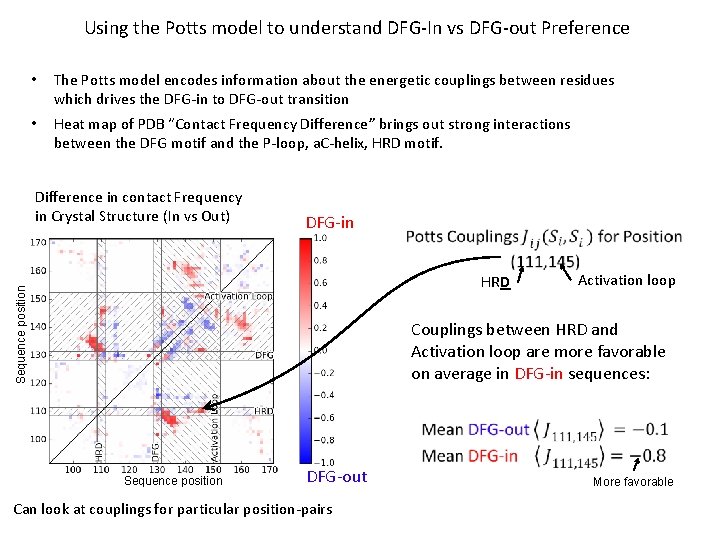
Using the Potts model to understand DFG-In vs DFG-out Preference • The Potts model encodes information about the energetic couplings between residues which drives the DFG-in to DFG-out transition • Heat map of PDB “Contact Frequency Difference” brings out strong interactions between the DFG motif and the P-loop, a. C-helix, HRD motif. Difference in contact Frequency in Crystal Structure (In vs Out) DFG-in Sequence position HRD Activation loop Couplings between HRD and Activation loop are more favorable on average in DFG-in sequences: Sequence position DFG-out Can look at couplings for particular position-pairs More favorable
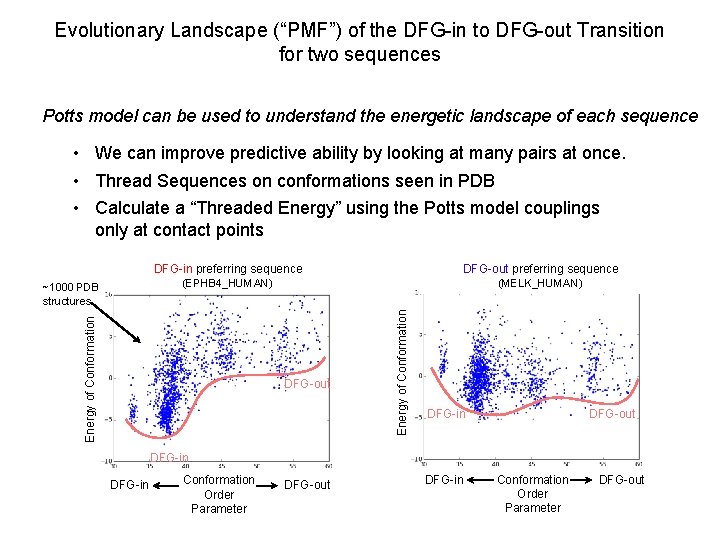
Evolutionary Landscape (“PMF”) of the DFG-in to DFG-out Transition for two sequences Potts model can be used to understand the energetic landscape of each sequence • We can improve predictive ability by looking at many pairs at once. • Thread Sequences on conformations seen in PDB • Calculate a “Threaded Energy” using the Potts model couplings only at contact points (MELK_HUMAN) DFG-out Energy of Conformation DFG-out preferring sequence (EPHB 4_HUMAN) Energy of Conformation ~1000 PDB structures DFG-in preferring sequence DFG-in DFG-out DFG-in Conformation Order Parameter DFG-out
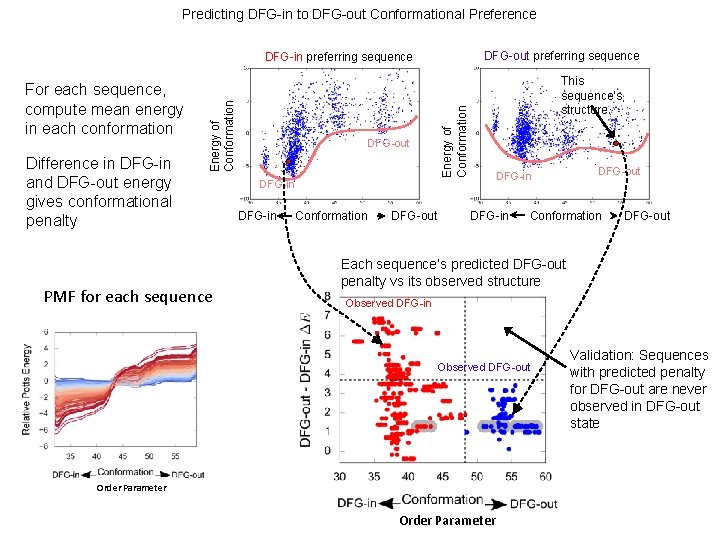
Predicting DFG-in to DFG-out Conformational Preference DFG-out preferring sequence Difference in DFG-in and DFG-out energy gives conformational penalty PMF for each sequence DFG-out This sequence’s structure Energy of Conformation For each sequence, compute mean energy in each conformation Energy of Conformation DFG-in preferring sequence DFG-in Conformation DFG-out DFG-in DFG-out Conformation DFG-out Each sequence’s predicted DFG-out penalty vs its observed structure Observed DFG-in Observed DFG-out Order Parameter Validation: Sequences with predicted penalty for DFG-out are never observed in DFG-out state
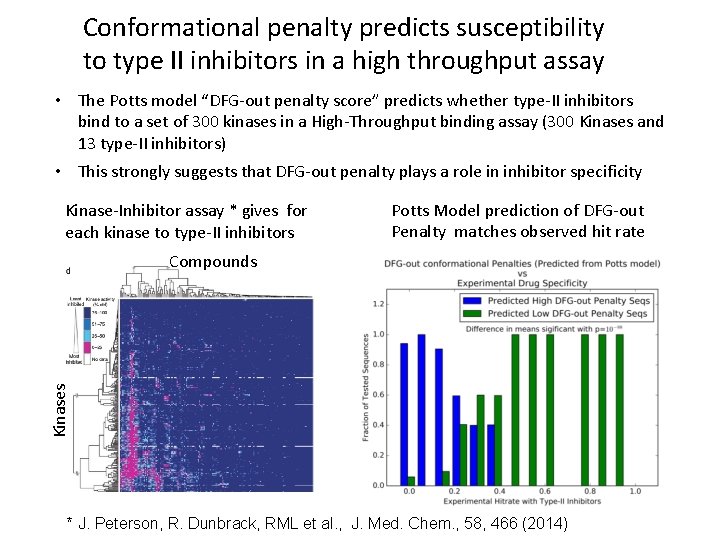
Conformational penalty predicts susceptibility to type II inhibitors in a high throughput assay • The Potts model “DFG-out penalty score” predicts whether type-II inhibitors bind to a set of 300 kinases in a High-Throughput binding assay (300 Kinases and 13 type-II inhibitors) • This strongly suggests that DFG-out penalty plays a role in inhibitor specificity Kinase-Inhibitor assay * gives for each kinase to type-II inhibitors Potts Model prediction of DFG-out Penalty matches observed hit rate Kinases Compounds * J. Peterson, R. Dunbrack, RML et al. , J. Med. Chem. , 58, 466 (2014)
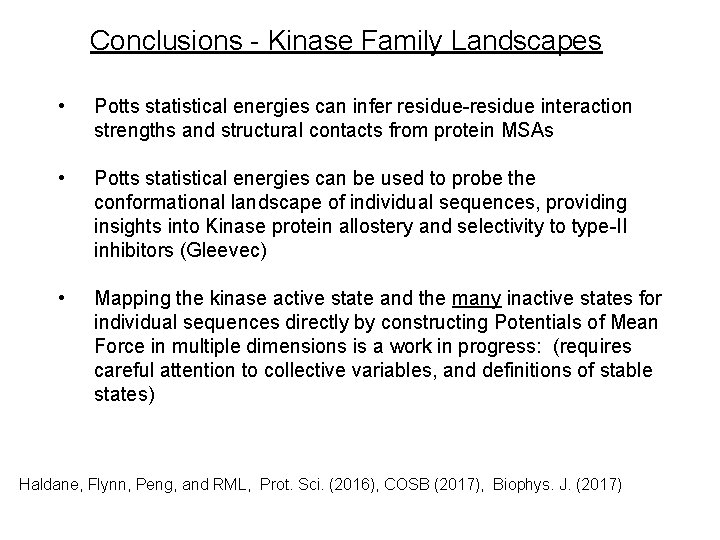
Conclusions - Kinase Family Landscapes • Potts statistical energies can infer residue-residue interaction strengths and structural contacts from protein MSAs • Potts statistical energies can be used to probe the conformational landscape of individual sequences, providing insights into Kinase protein allostery and selectivity to type-II inhibitors (Gleevec) • Mapping the kinase active state and the many inactive states for individual sequences directly by constructing Potentials of Mean Force in multiple dimensions is a work in progress: (requires careful attention to collective variables, and definitions of stable states) Haldane, Flynn, Peng, and RML, Prot. Sci. (2016), COSB (2017), Biophys. J. (2017)
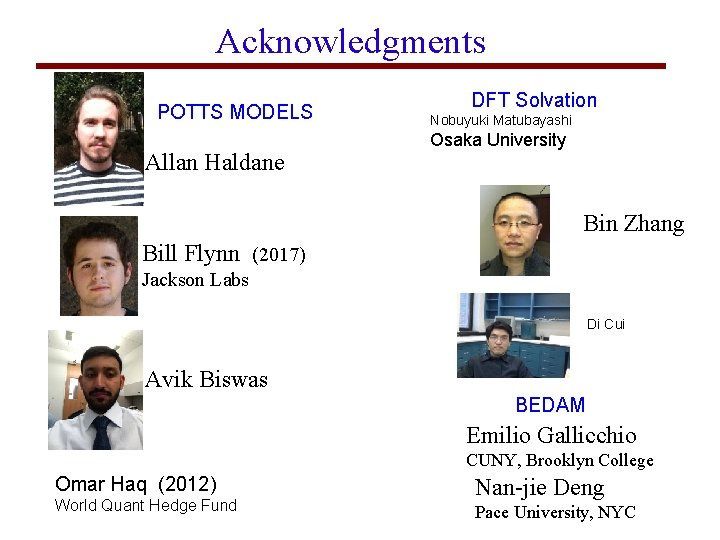
Acknowledgments POTTS MODELS Allan Haldane DFT Solvation Nobuyuki Matubayashi Osaka University Bin Zhang Bill Flynn (2017) Jackson Labs Di Cui Avik Biswas BEDAM Emilio Gallicchio CUNY, Brooklyn College Omar Haq (2012) World Quant Hedge Fund Nan-jie Deng Pace University, NYC
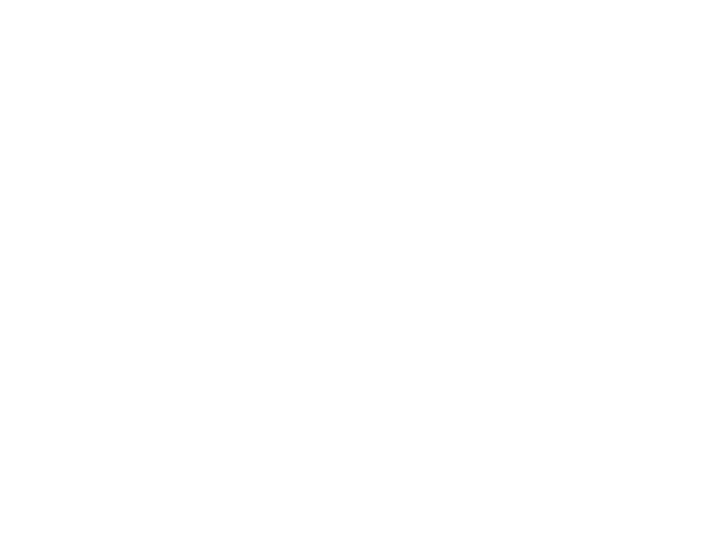
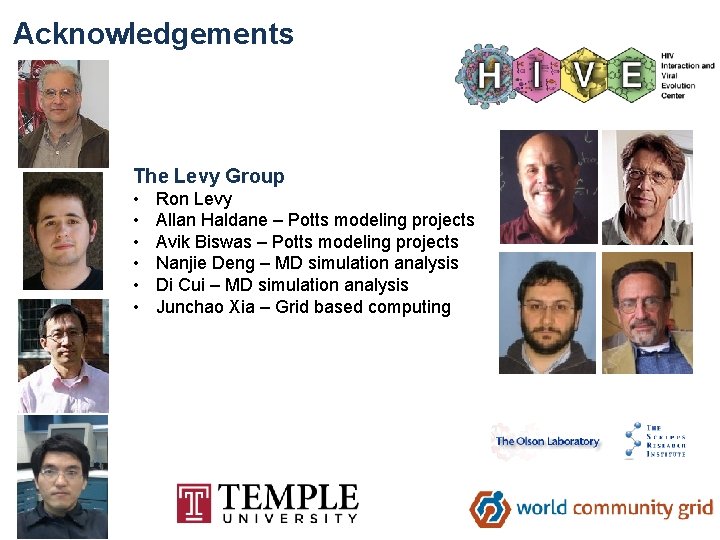
Acknowledgements The Levy Group • • • Ron Levy Allan Haldane – Potts modeling projects Avik Biswas – Potts modeling projects Nanjie Deng – MD simulation analysis Di Cui – MD simulation analysis Junchao Xia – Grid based computing
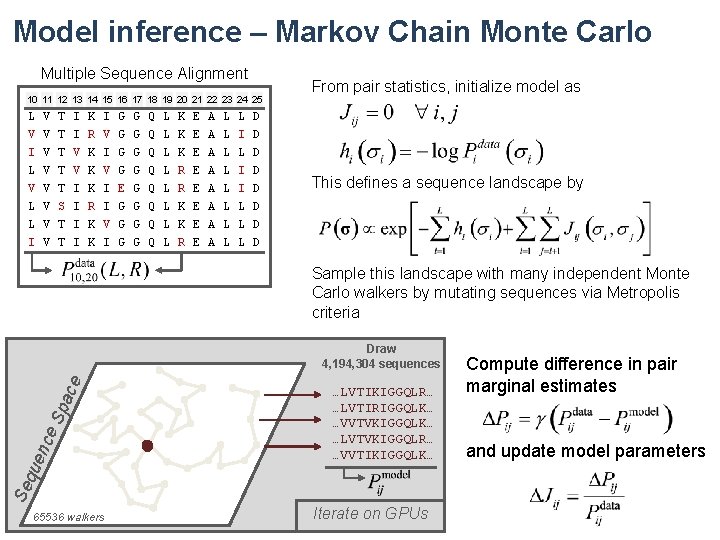
Model inference – Markov Chain Monte Carlo Multiple Sequence Alignment 10 11 12 13 14 15 16 17 18 19 20 21 22 23 24 25 From pair statistics, initialize model as L V T I K I G G Q L K E A L L D V I L V L L I V V V V T T S T T I V V I I R K K K R K K V I I V I G G G E G G G G G Q Q Q Q L L L L K K R R K K R E E E E A A A A L L L L I I L L L D D D D This defines a sequence landscape by Sample this landscape with many independent Monte Carlo walkers by mutating sequences via Metropolis criteria Se que nce S pac e Draw 4, 194, 304 sequences 65536 walkers …LVTIKIGGQLR… …LVTIRIGGQLK… …VVTVKIGGQLK… …LVTVKIGGQLR… …VVTIKIGGQLK… Iterate on GPUs Compute difference in pair marginal estimates and update model parameters
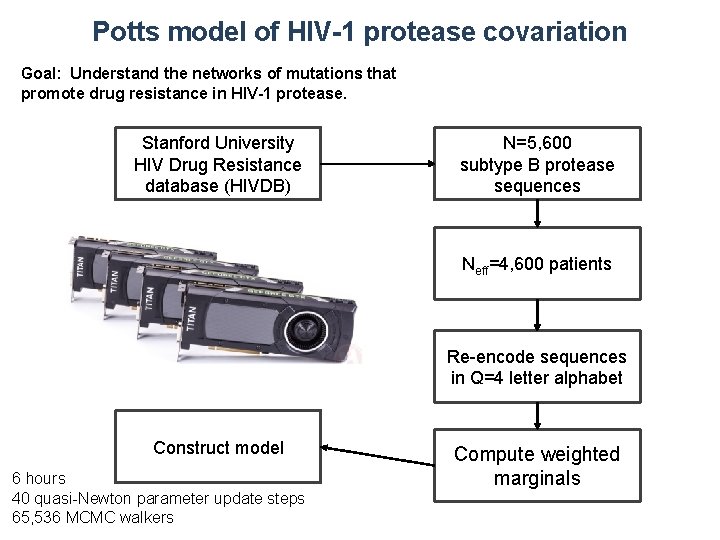
Potts model of HIV-1 protease covariation Goal: Understand the networks of mutations that promote drug resistance in HIV-1 protease. Stanford University HIV Drug Resistance database (HIVDB) N=5, 600 subtype B protease sequences Neff=4, 600 patients Re-encode sequences in Q=4 letter alphabet Construct model 6 hours 40 quasi-Newton parameter update steps 65, 536 MCMC walkers Compute weighted marginals
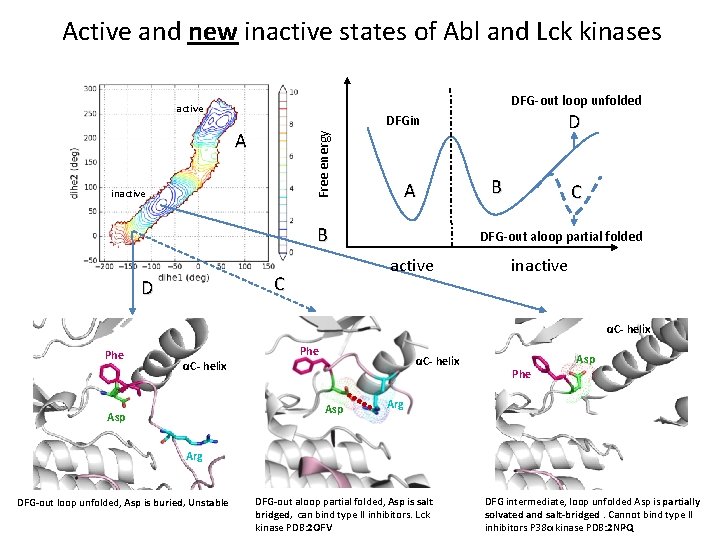
Active and new inactive states of Abl and Lck kinases DFG-out loop unfolded active Free energy A inactive A B B C DFG-out aloop partial folded active C D D DFGin inactive αC- helix Phe αC- helix Asp Asp Phe Arg DFG-out loop unfolded, Asp is buried, Unstable DFG-out aloop partial folded, Asp is salt bridged, can bind type II inhibitors. Lck kinase PDB: 2 OFV DFG intermediate, loop unfolded Asp is partially solvated and salt-bridged. Cannot bind type II inhibitors P 38α kinase PDB: 2 NPQ
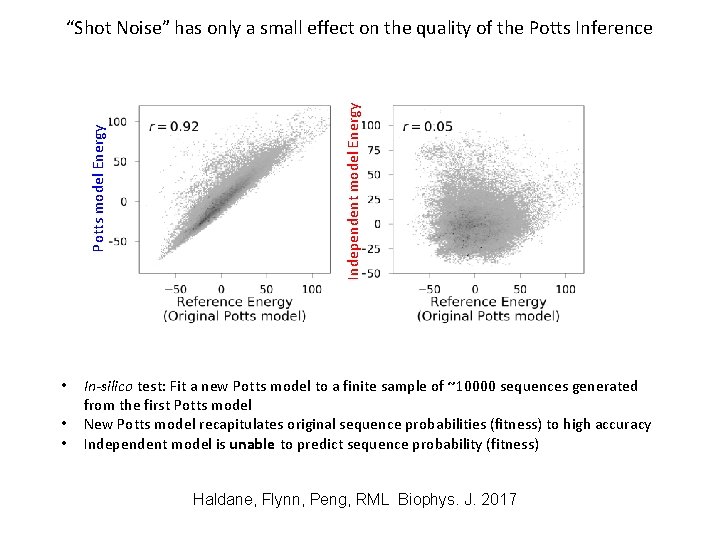
• • • Independent model Energy Potts model Energy “Shot Noise” has only a small effect on the quality of the Potts Inference In-silico test: Fit a new Potts model to a finite sample of ~10000 sequences generated from the first Potts model New Potts model recapitulates original sequence probabilities (fitness) to high accuracy Independent model is unable to predict sequence probability (fitness) Haldane, Flynn, Peng, RML Biophys. J. 2017
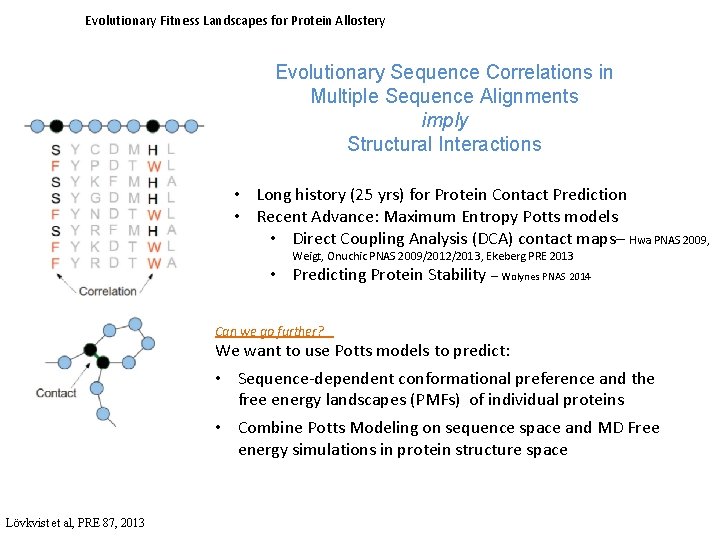
Evolutionary Fitness Landscapes for Protein Allostery Evolutionary Sequence Correlations in Multiple Sequence Alignments imply Structural Interactions • Long history (25 yrs) for Protein Contact Prediction • Recent Advance: Maximum Entropy Potts models • Direct Coupling Analysis (DCA) contact maps– Hwa PNAS 2009, Weigt, Onuchic PNAS 2009/2012/2013, Ekeberg PRE 2013 • Predicting Protein Stability – Wolynes PNAS 2014 Can we go further? We want to use Potts models to predict: • Sequence-dependent conformational preference and the free energy landscapes (PMFs) of individual proteins • Combine Potts Modeling on sequence space and MD Free energy simulations in protein structure space Lövkvist et al, PRE 87, 2013
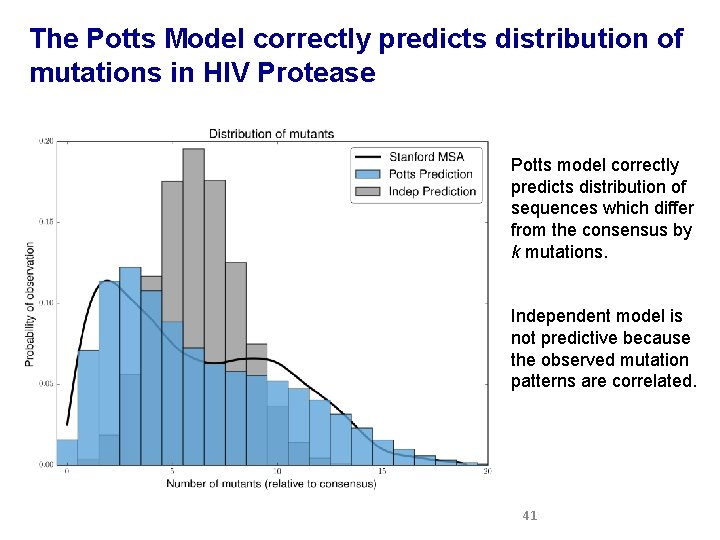
The Potts Model correctly predicts distribution of mutations in HIV Protease Potts model correctly predicts distribution of sequences which differ from the consensus by k mutations. Independent model is not predictive because the observed mutation patterns are correlated. 41
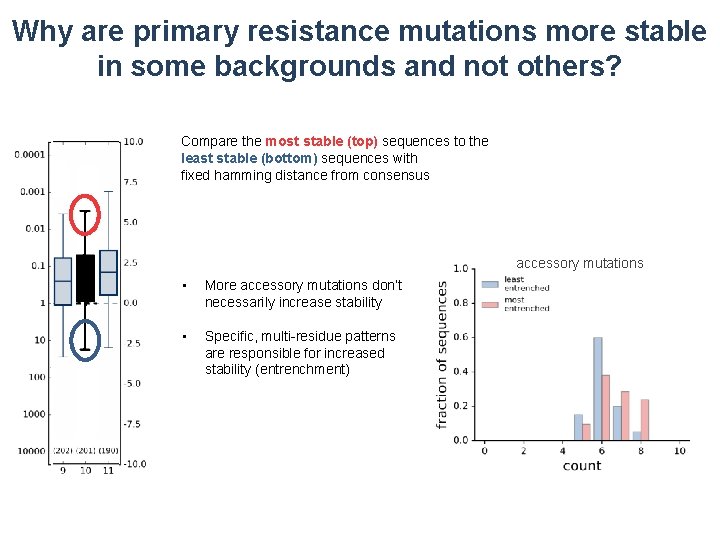
Why are primary resistance mutations more stable in some backgrounds and not others? Compare the most stable (top) sequences to the least stable (bottom) sequences with fixed hamming distance from consensus accessory mutations • More accessory mutations don’t necessarily increase stability • Specific, multi-residue patterns are responsible for increased stability (entrenchment)
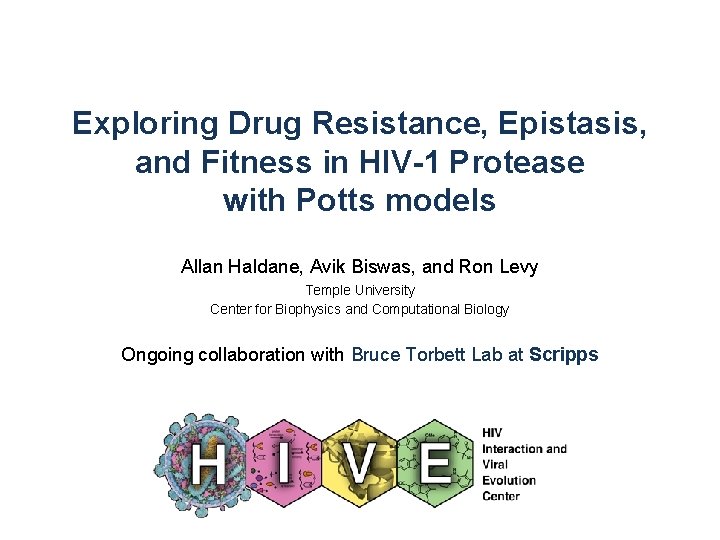
Exploring Drug Resistance, Epistasis, and Fitness in HIV-1 Protease with Potts models Allan Haldane, Avik Biswas, and Ron Levy Temple University Center for Biophysics and Computational Biology Ongoing collaboration with Bruce Torbett Lab at Scripps
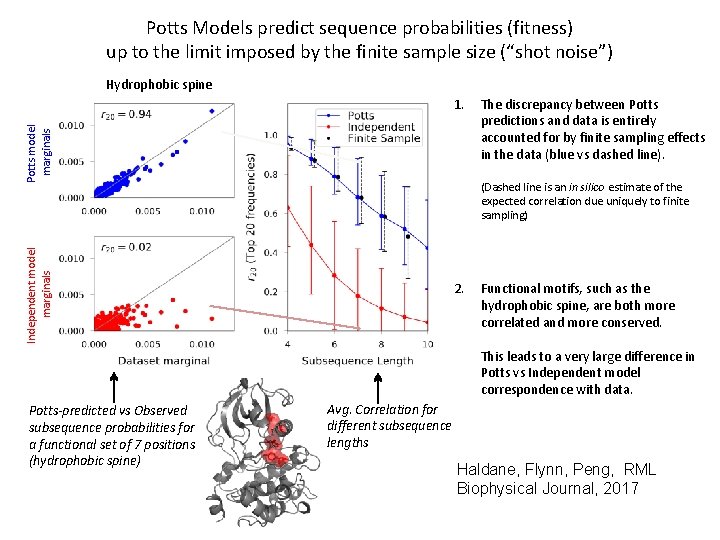
Potts Models predict sequence probabilities (fitness) up to the limit imposed by the finite sample size (“shot noise”) Hydrophobic spine Potts model marginals 1. The discrepancy between Potts predictions and data is entirely accounted for by finite sampling effects in the data (blue vs dashed line). Independent model marginals (Dashed line is an in silico estimate of the expected correlation due uniquely to finite sampling) 2. Functional motifs, such as the hydrophobic spine, are both more correlated and more conserved. This leads to a very large difference in Potts vs Independent model correspondence with data. Potts-predicted vs Observed subsequence probabilities for a functional set of 7 positions (hydrophobic spine) Avg. Correlation for different subsequence lengths Haldane, Flynn, Peng, RML Biophysical Journal, 2017
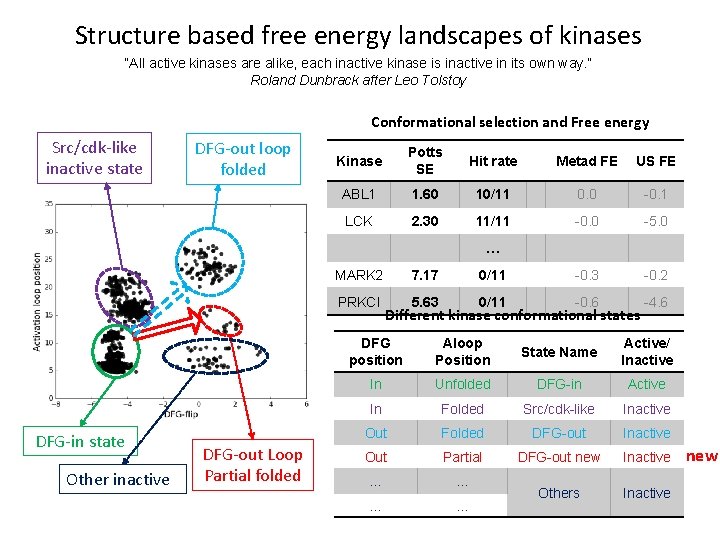
Structure based free energy landscapes of kinases “All active kinases are alike, each inactive kinase is inactive in its own way. ” Roland Dunbrack after Leo Tolstoy Conformational selection and Free energy Src/cdk-like inactive state DFG-out loop folded Kinase Potts SE Hit rate Metad FE US FE ABL 1 1. 60 10/11 0. 0 -0. 1 LCK 2. 30 11/11 -0. 0 -5. 0 … MARK 2 PRKCI DFG-in state Other inactive DFG-out Loop Partial folded 7. 17 0/11 -0. 3 -0. 2 5. 63 0/11 -0. 6 -4. 6 Different kinase conformational states DFG position Aloop Position State Name Active/ Inactive In Unfolded DFG-in Active In Folded Src/cdk-like Inactive Out Folded DFG-out Inactive Out Partial DFG-out new Inactive … … Others Inactive new
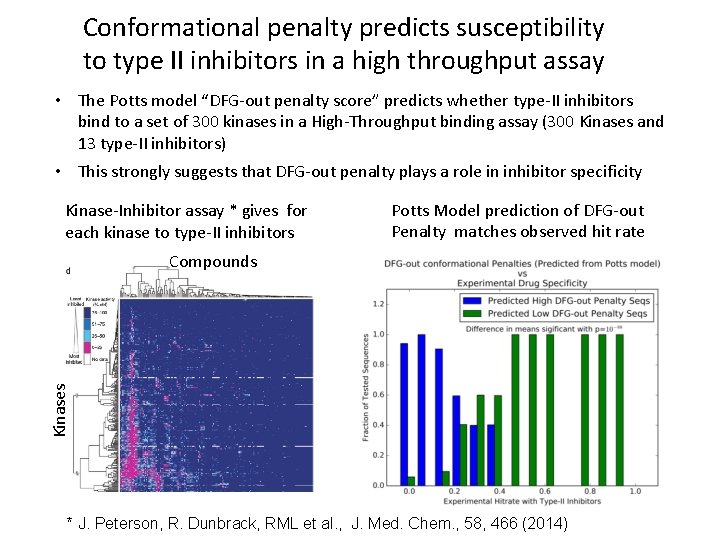
Conformational penalty predicts susceptibility to type II inhibitors in a high throughput assay • The Potts model “DFG-out penalty score” predicts whether type-II inhibitors bind to a set of 300 kinases in a High-Throughput binding assay (300 Kinases and 13 type-II inhibitors) • This strongly suggests that DFG-out penalty plays a role in inhibitor specificity Kinase-Inhibitor assay * gives for each kinase to type-II inhibitors Potts Model prediction of DFG-out Penalty matches observed hit rate Kinases Compounds * J. Peterson, R. Dunbrack, RML et al. , J. Med. Chem. , 58, 466 (2014)
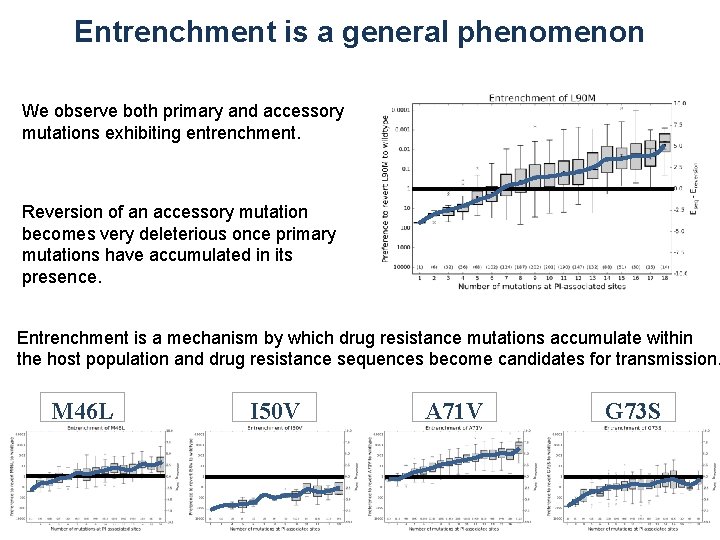
Entrenchment is a general phenomenon We observe both primary and accessory mutations exhibiting entrenchment. Reversion of an accessory mutation becomes very deleterious once primary mutations have accumulated in its presence. Entrenchment is a mechanism by which drug resistance mutations accumulate within the host population and drug resistance sequences become candidates for transmission. M 46 L I 50 V A 71 V G 73 S
Helmholtz free energy and gibbs free energy
Gibbs free energy equation
How to calculate gibbs free energy
Delta g = rt ln(q/k)
Skills related
Landscapes and landforms booklet
Landscapes and landforms
Morraine glacier
Uk physical landscapes
Fpm landscapes
Sand dunes landforms
Limestone
What makes landscapes distinctive
Coastal landscapes a level geography
Limestone landscapes
Enchanted definition
Eterogeneizzazione
Energy energy transfer and general energy analysis
Energy energy transfer and general energy analysis
A story of an hour summary
Optimistic poem
Section 8-1 carbohydrates fats and proteins answer key
Protein domains and motifs
Organic compounds such as proteins and starches are too
Hbv foods
Section 8-1 carbohydrates fats and proteins answer key
Salting in and salting out of proteins
What are the functions of proteins
Dna rna and proteins study guide answers
Functions and importance of proteins
What is the connection between genes and proteins
Integral and peripheral proteins
Amidomalonate synthesis mechanism
Ribosome factory part or worker
Integral and peripheral proteins
Difference between affinity and ion exchange chromatography
Golgi endoplasmic reticulum
Daily nutritional requirements chart pdf
Microfilament function
Energy fitness orla
The allocation map
Free-free absorption
Channel vs carrier proteins
Globular vs fibrous proteins
All enzymes are globular proteins
Protein building blocks
Denatured protein
What are derived proteins