Estimating Intervention Effect using Recurrent Events Tina XU
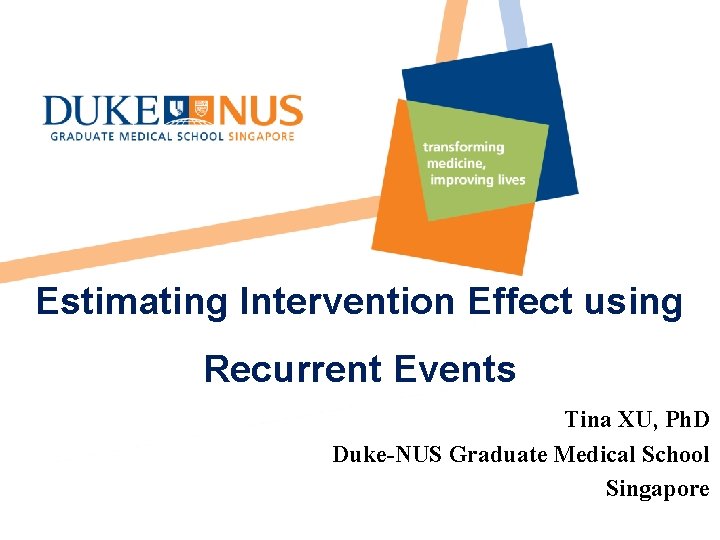
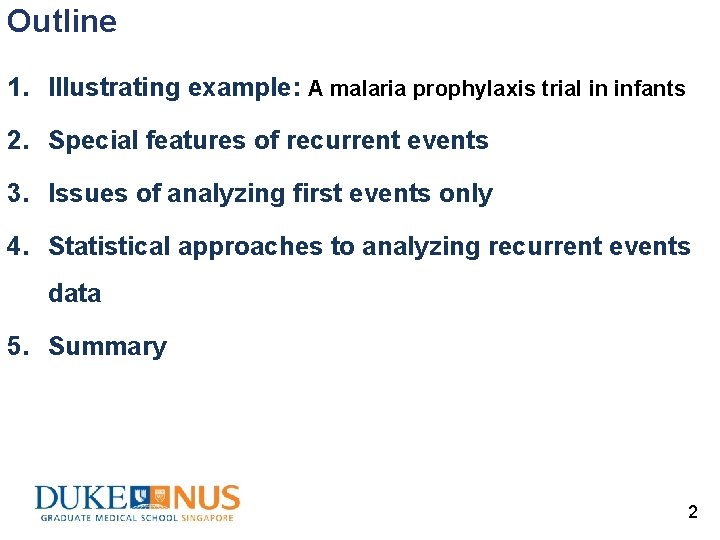
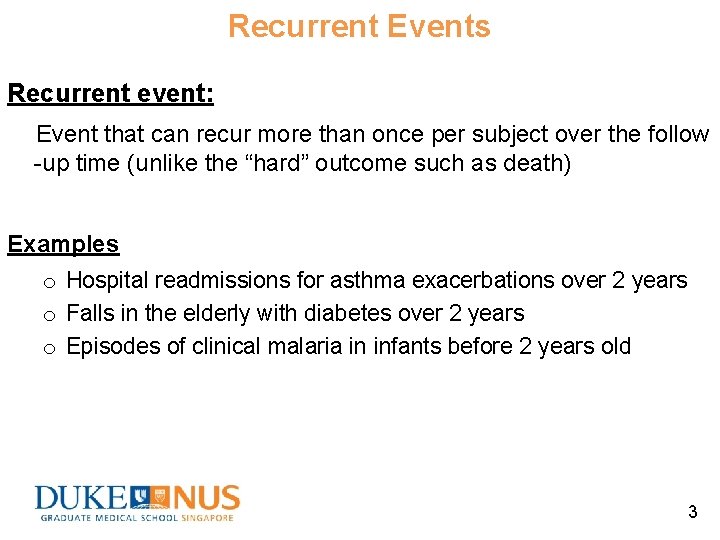
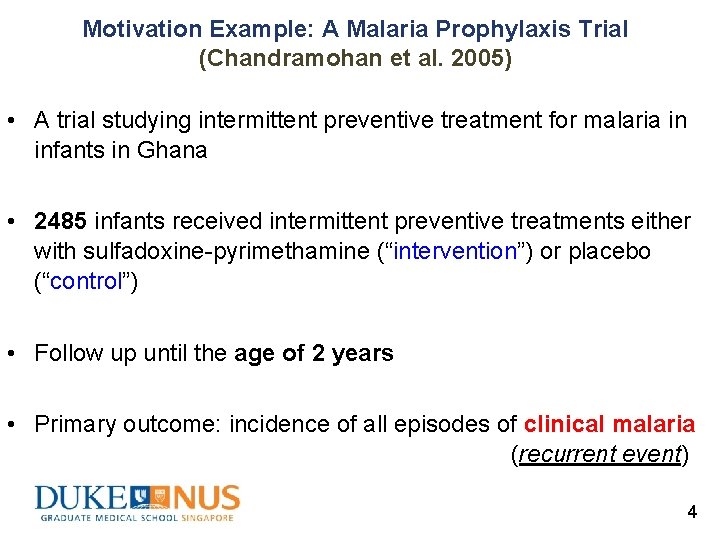
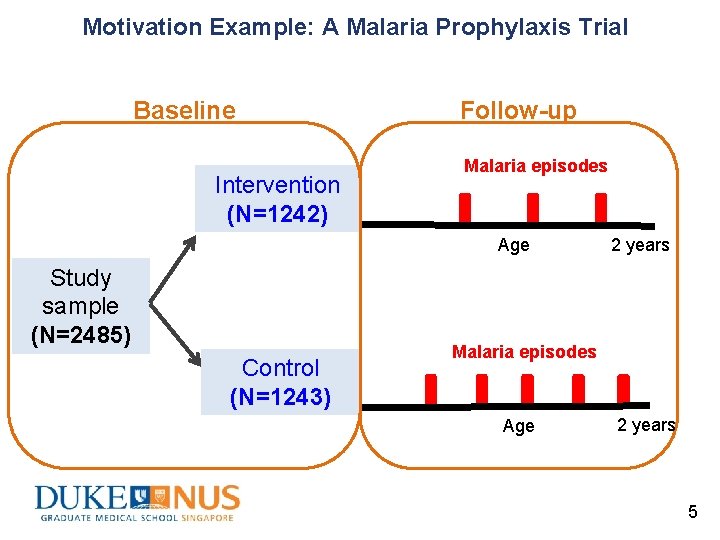
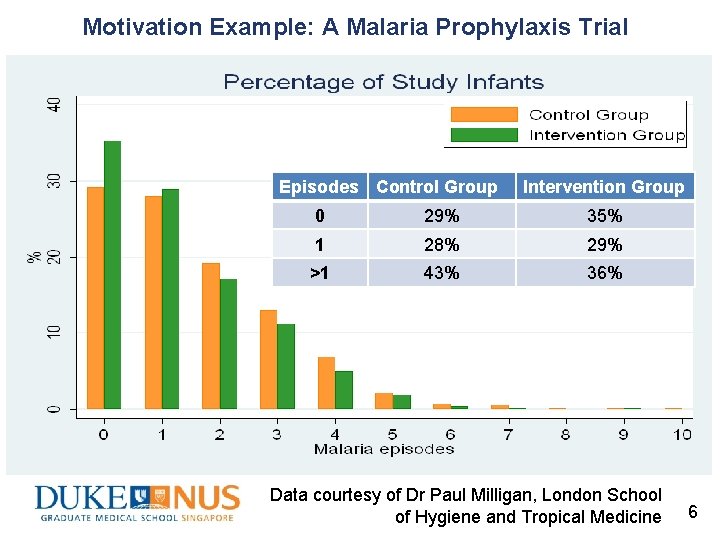
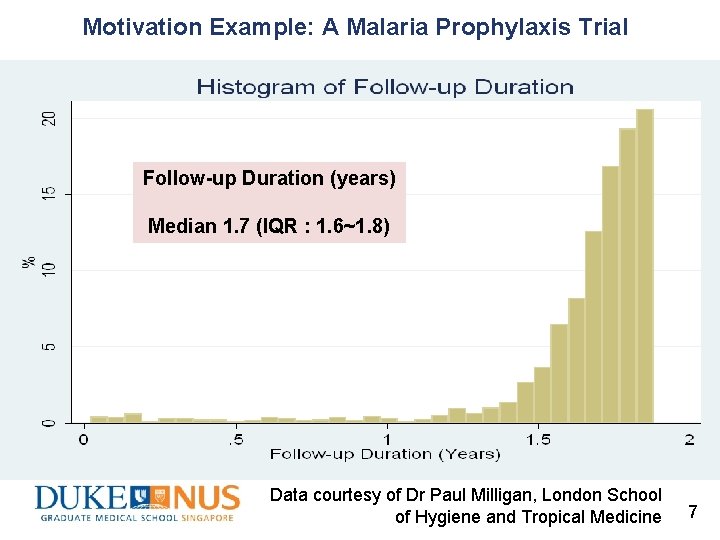
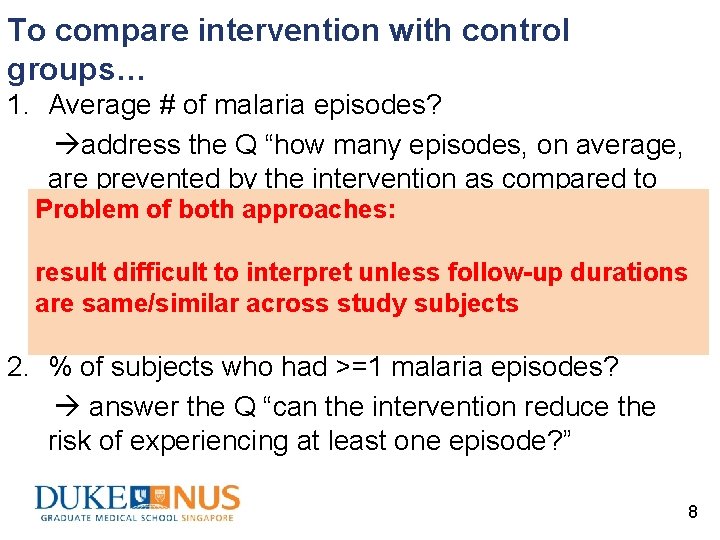
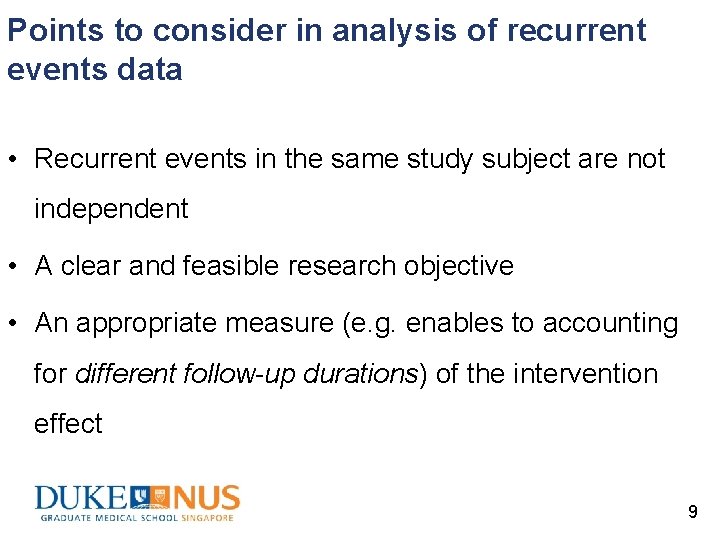
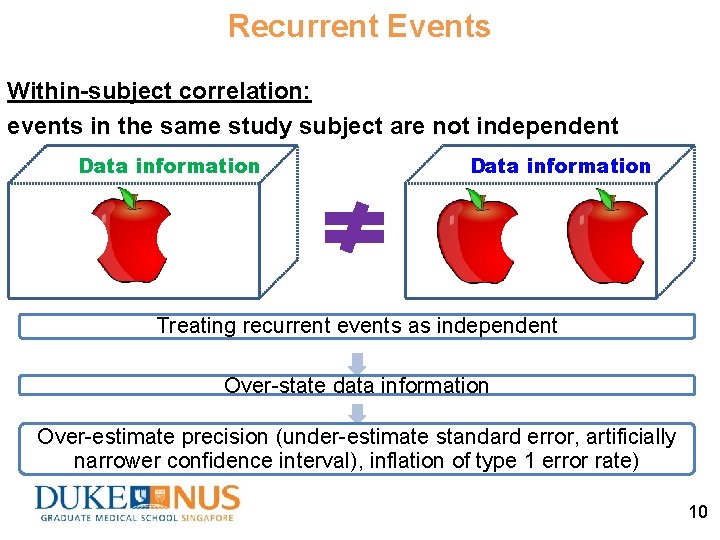
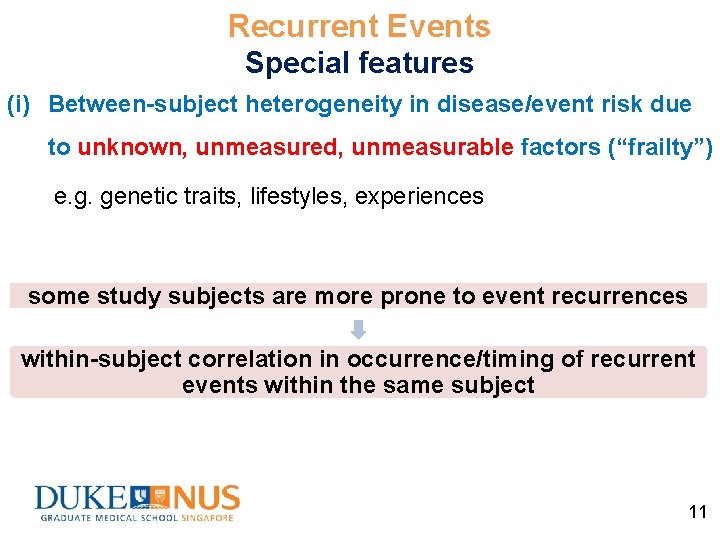
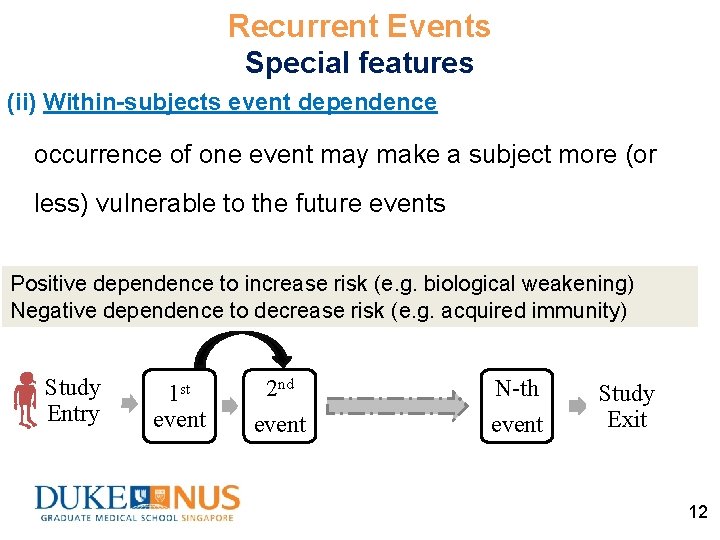
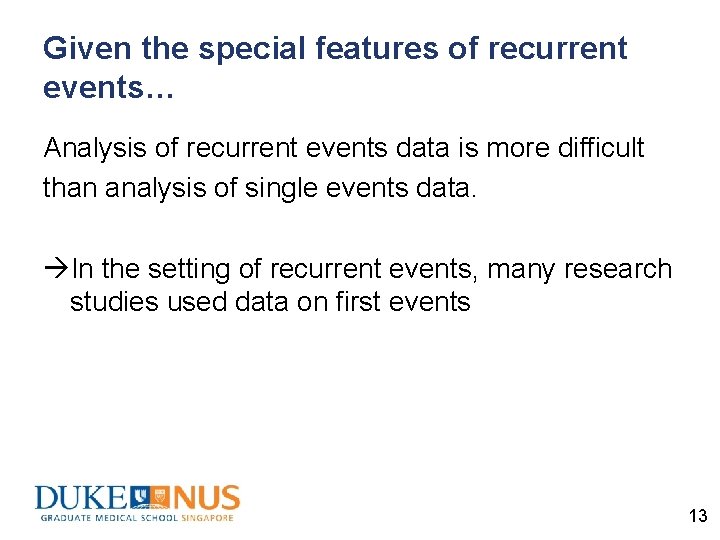
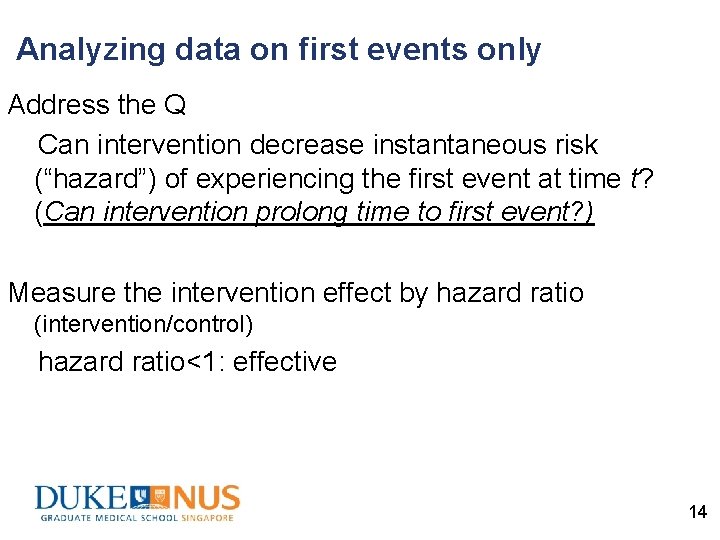
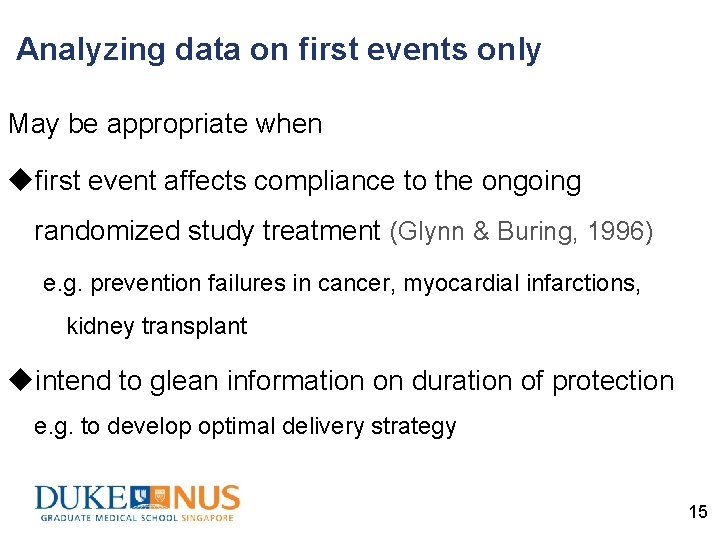
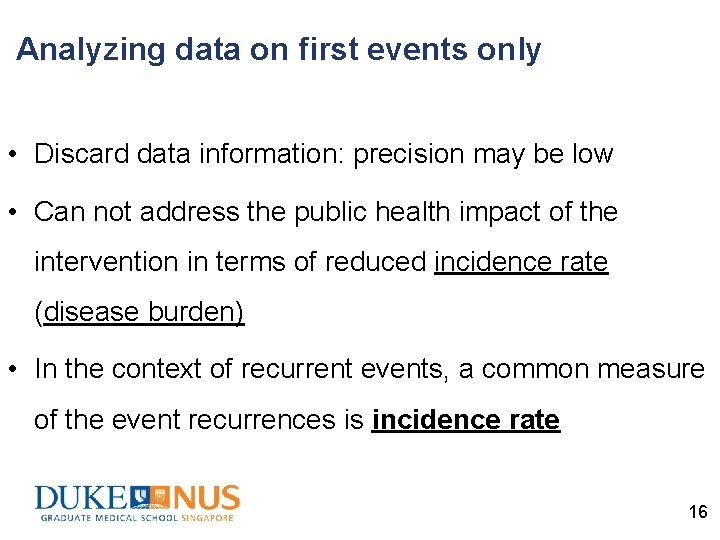
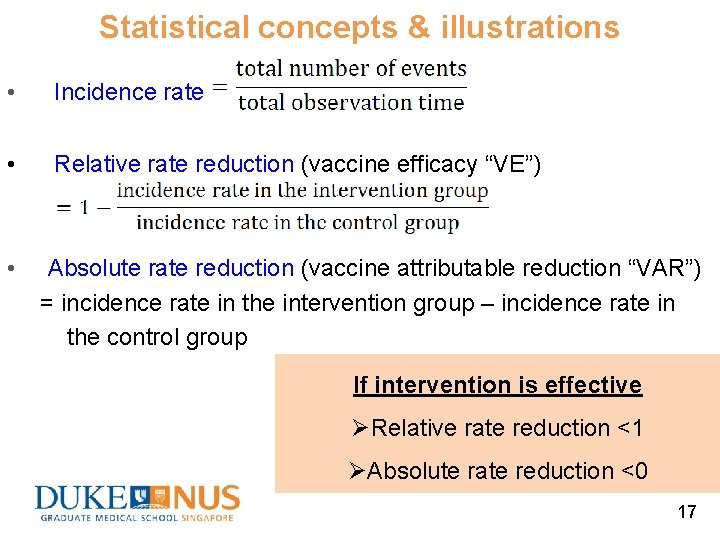
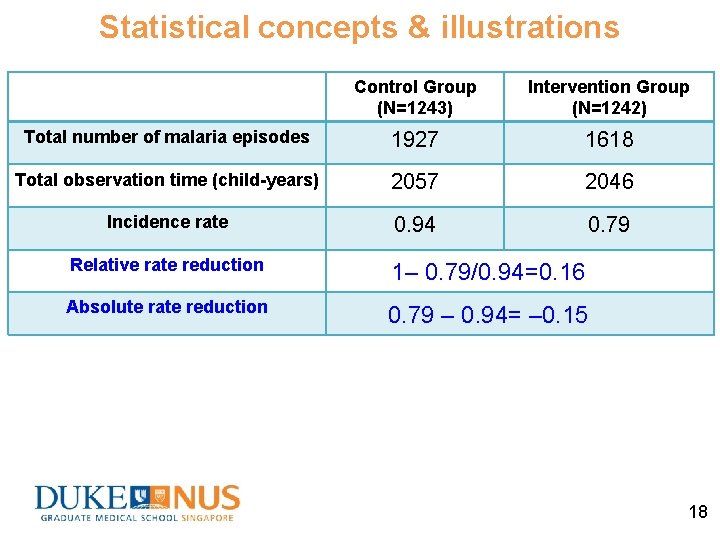
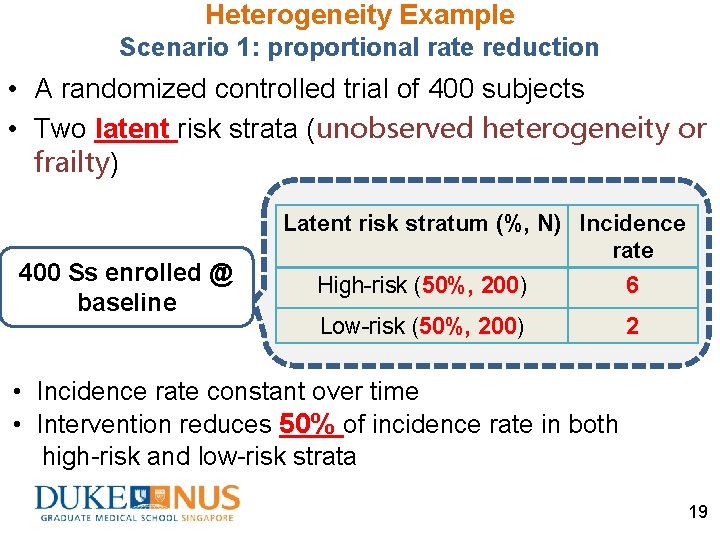
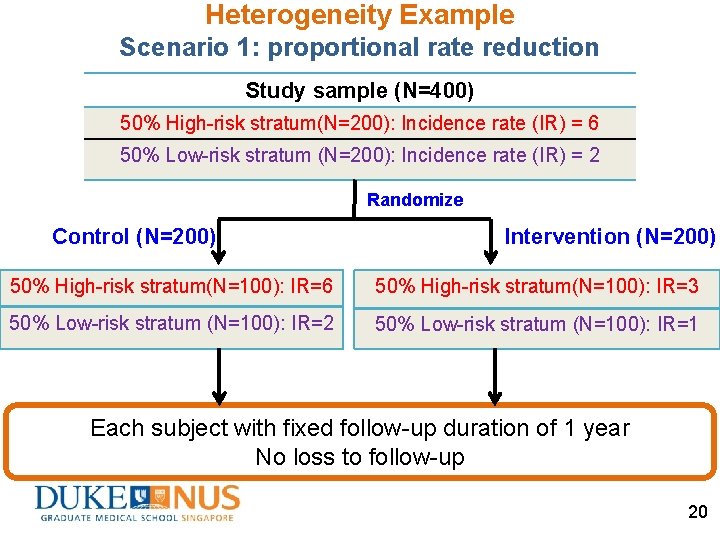
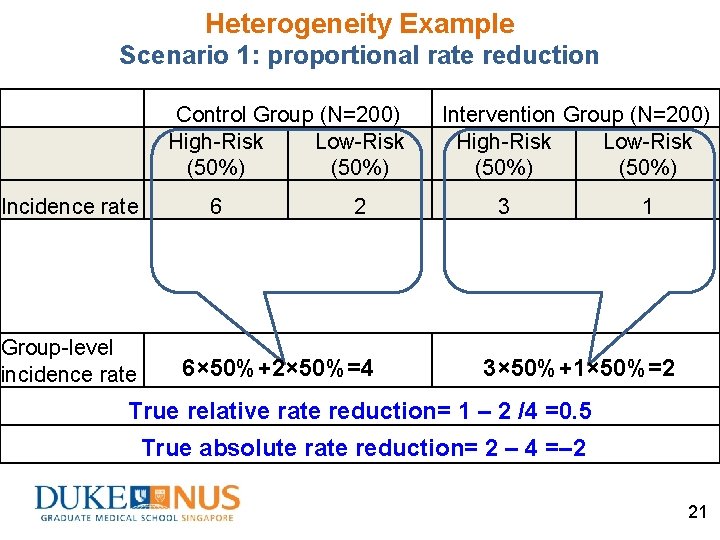
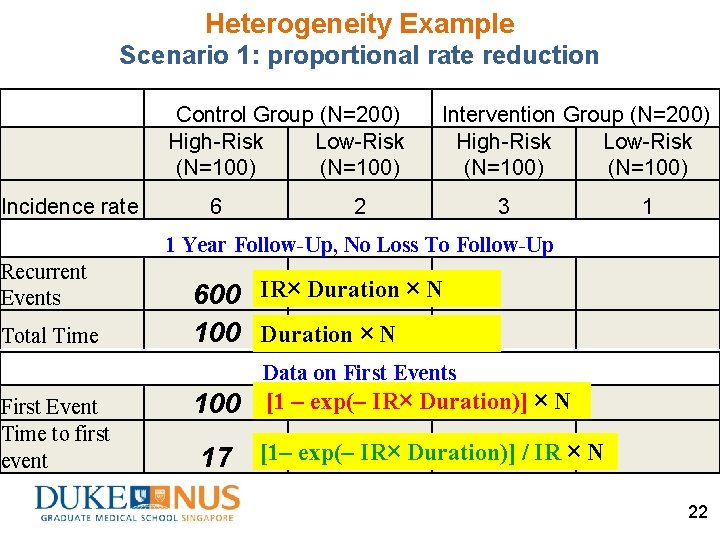
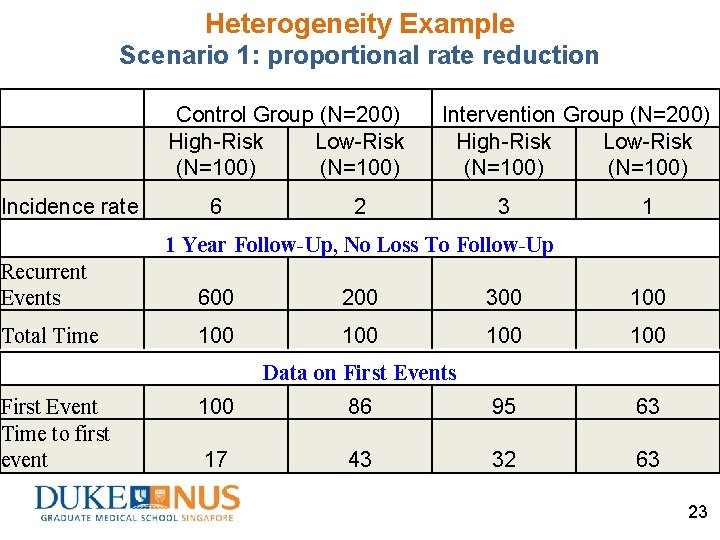
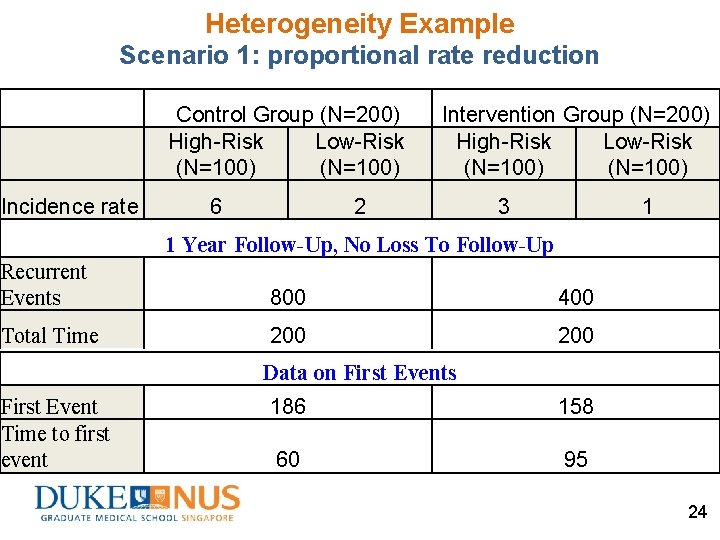
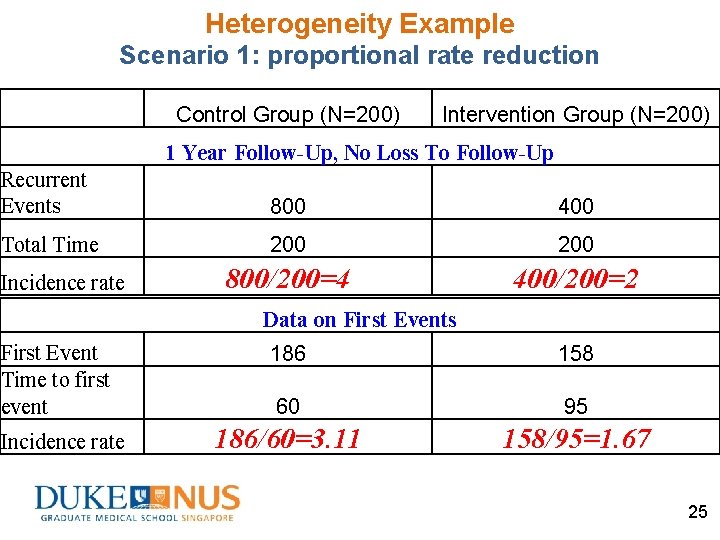
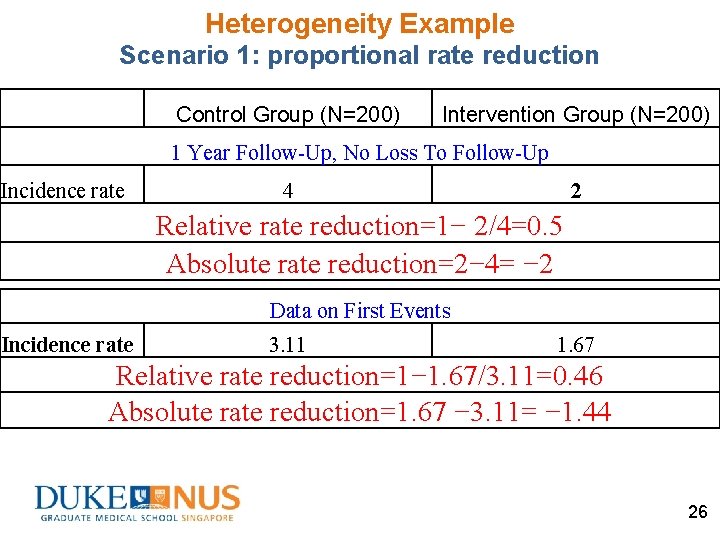
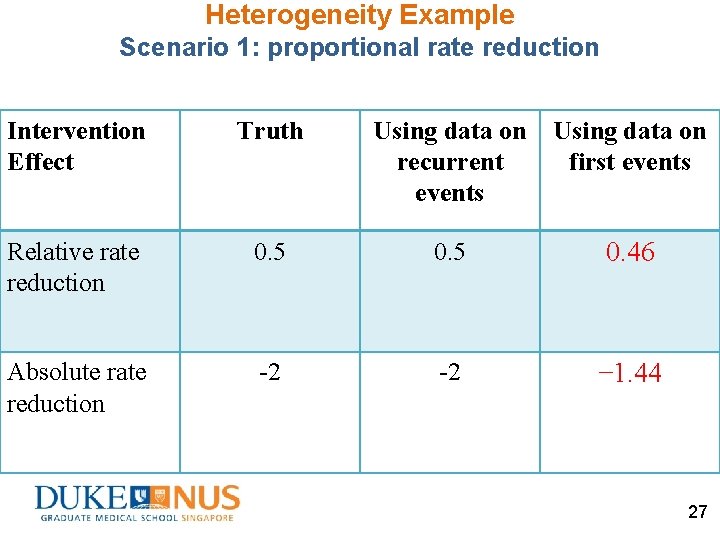
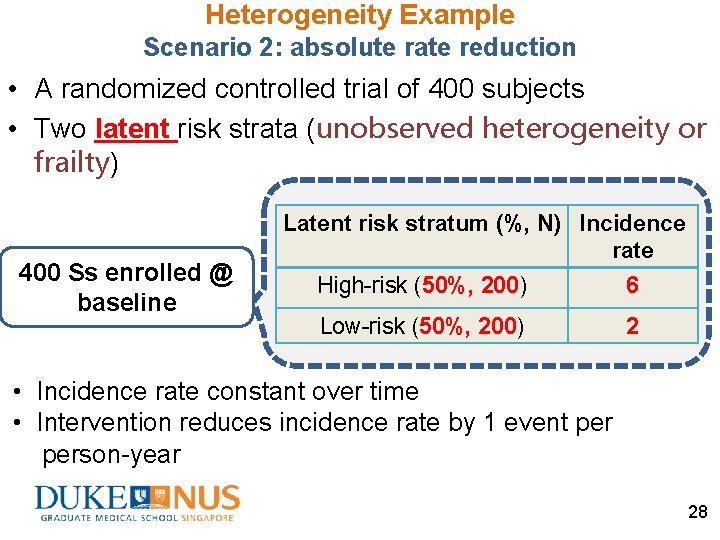
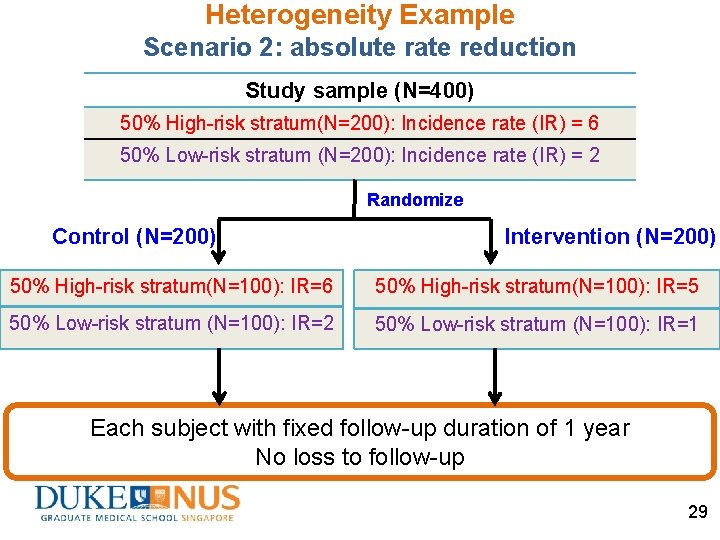
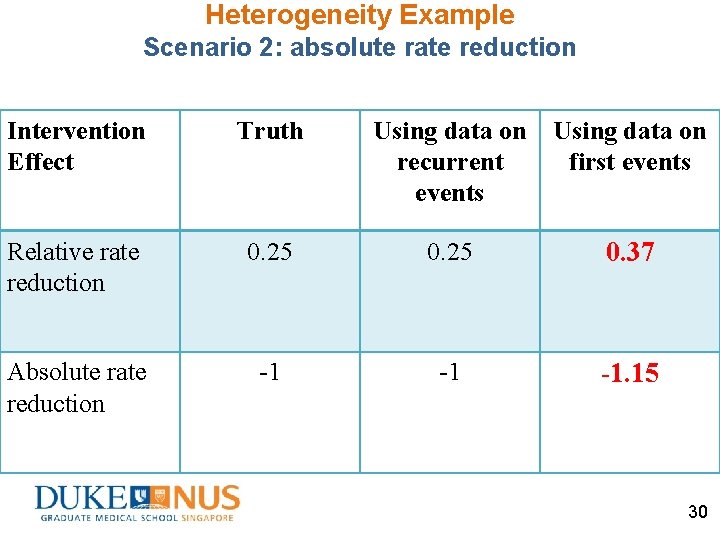
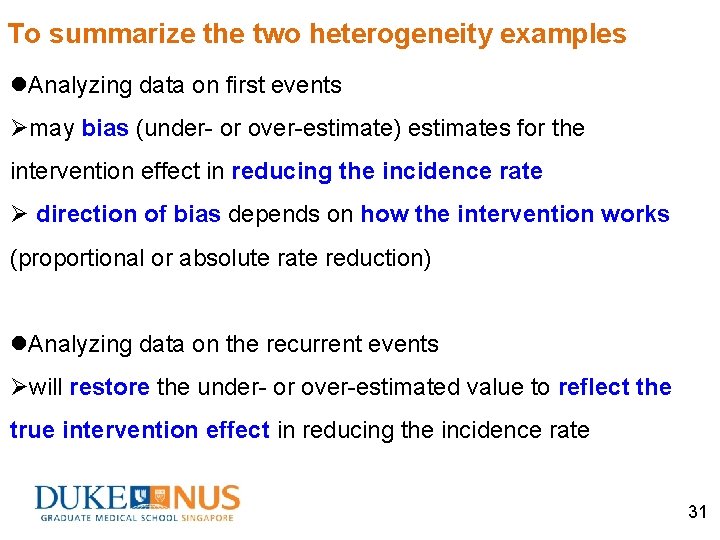
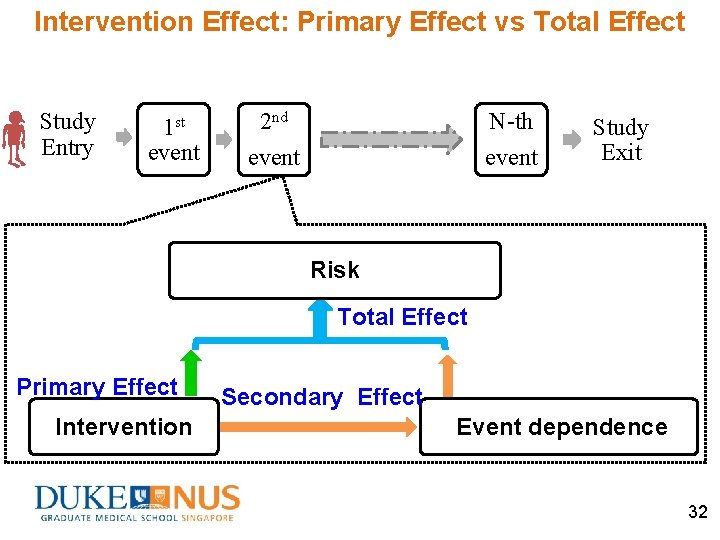
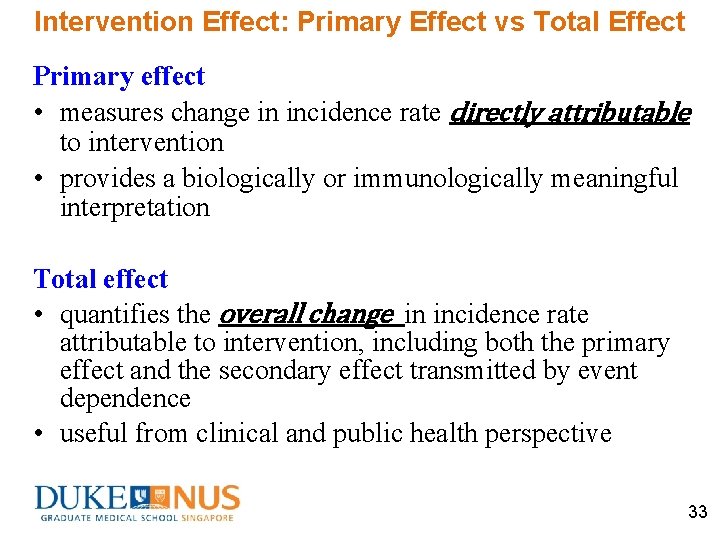
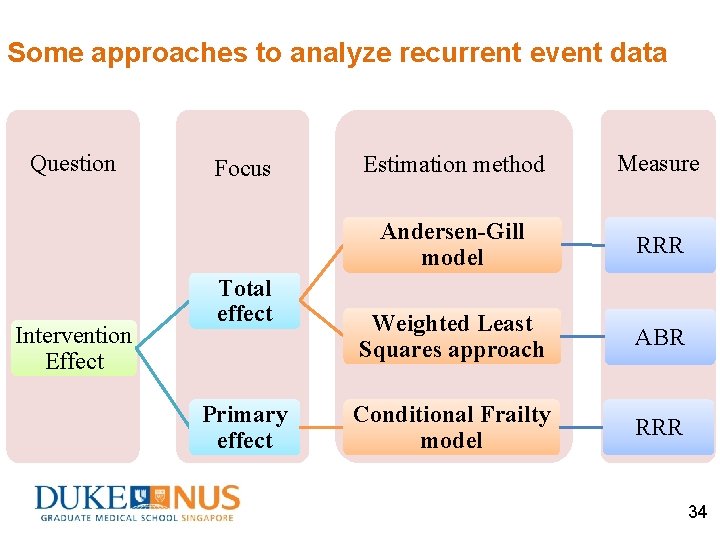
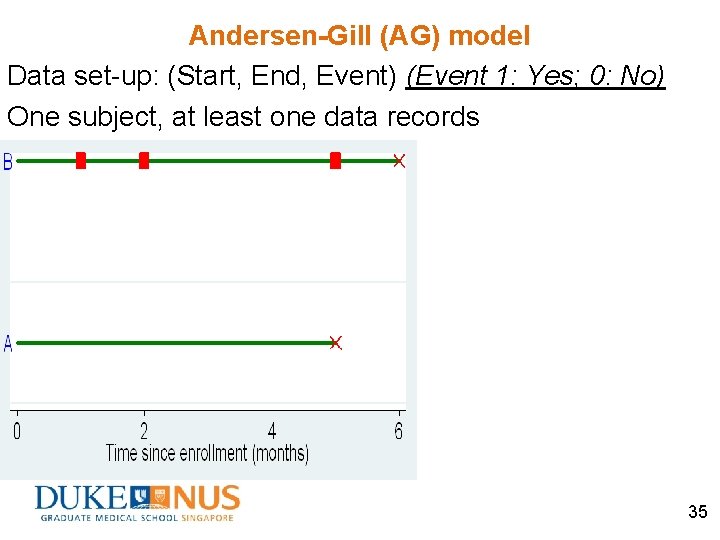
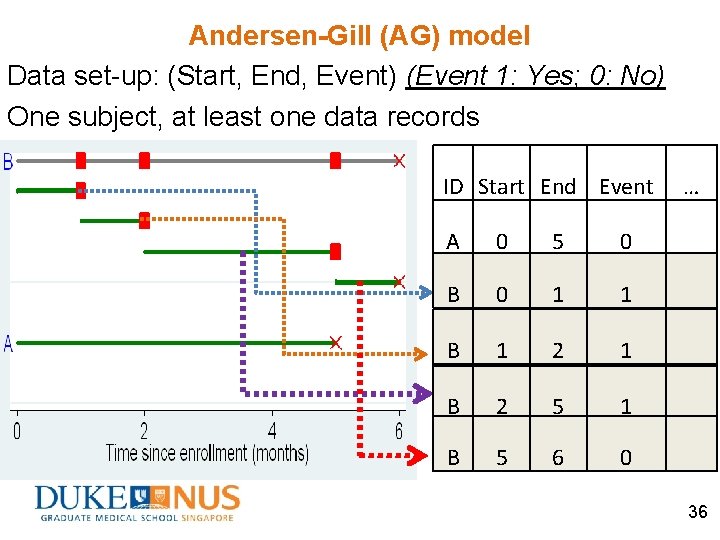
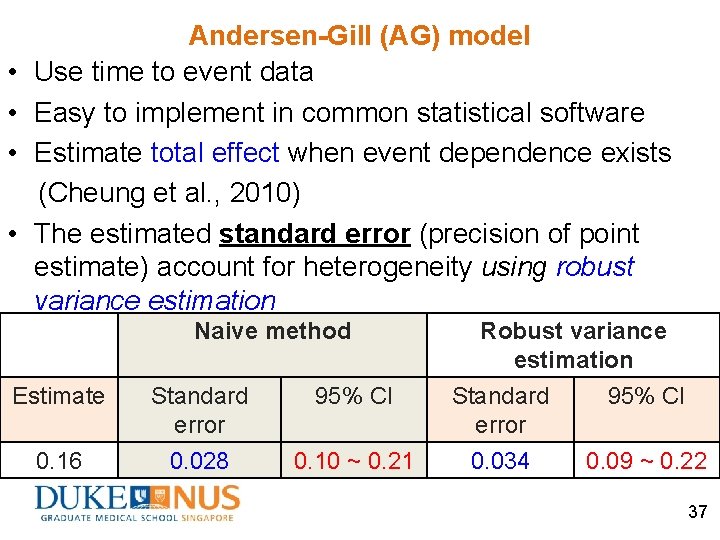
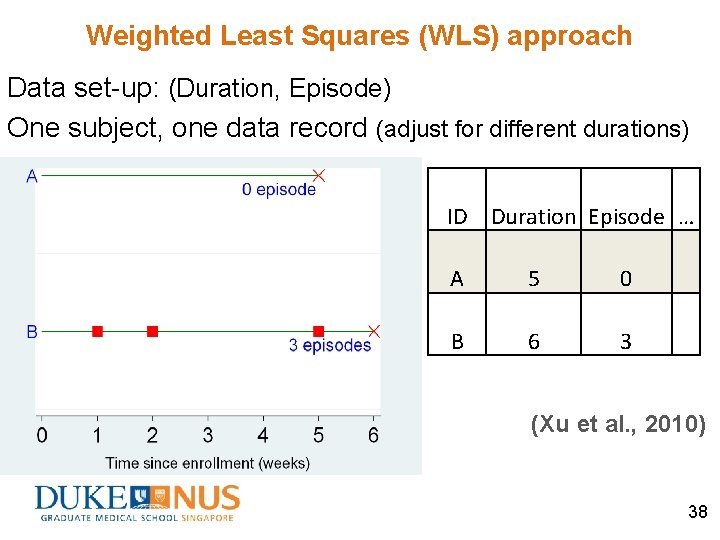
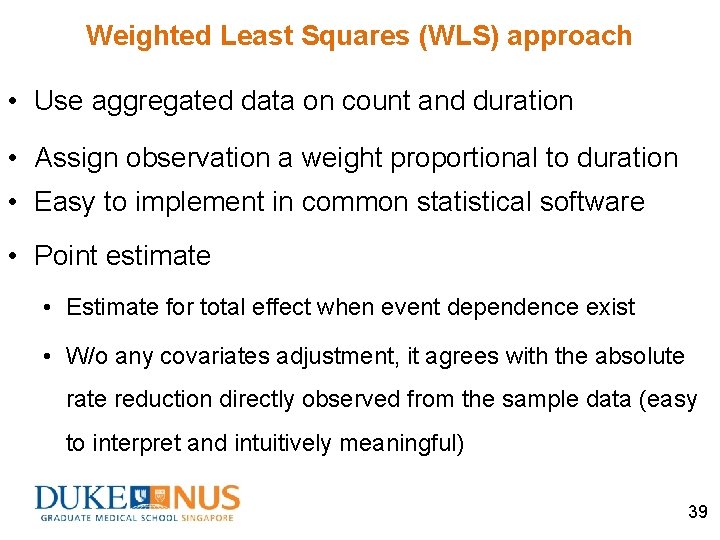
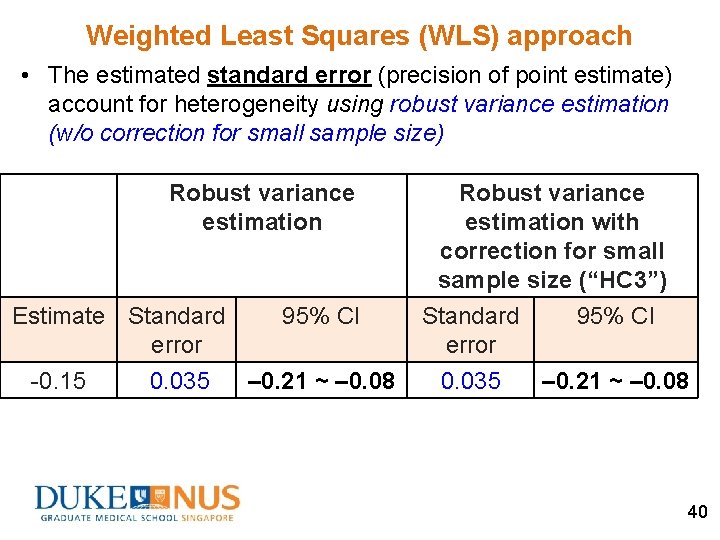
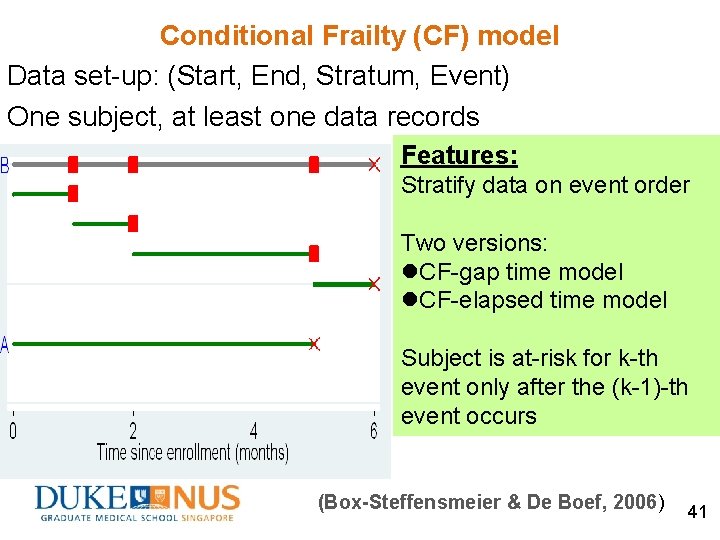
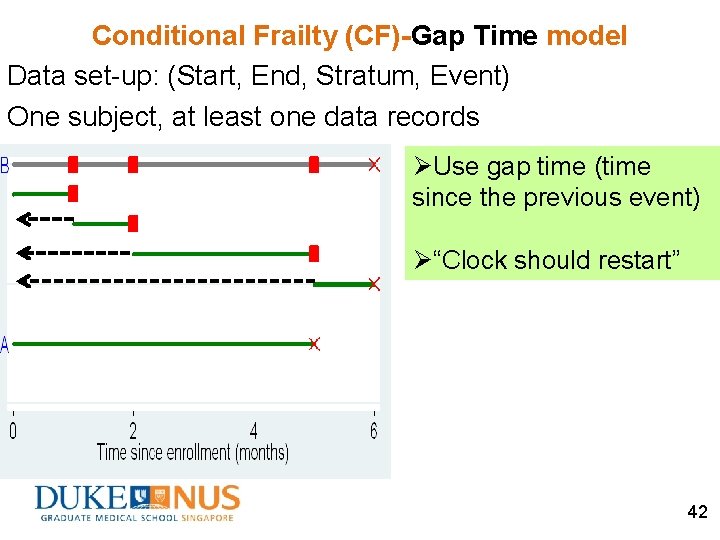
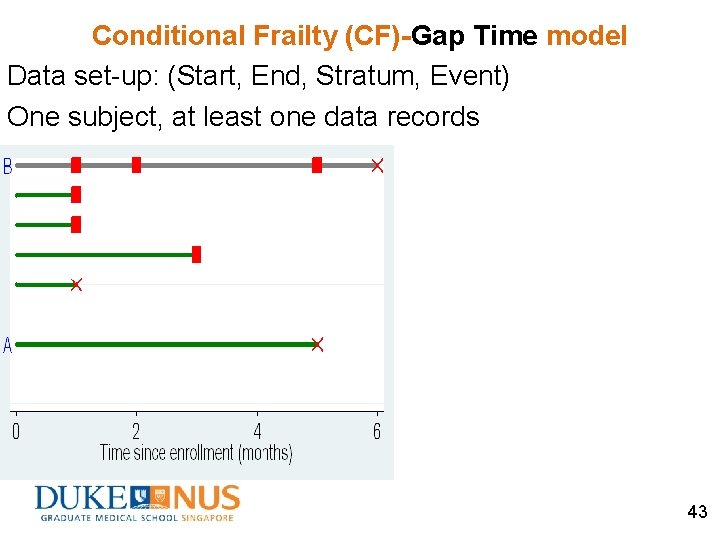
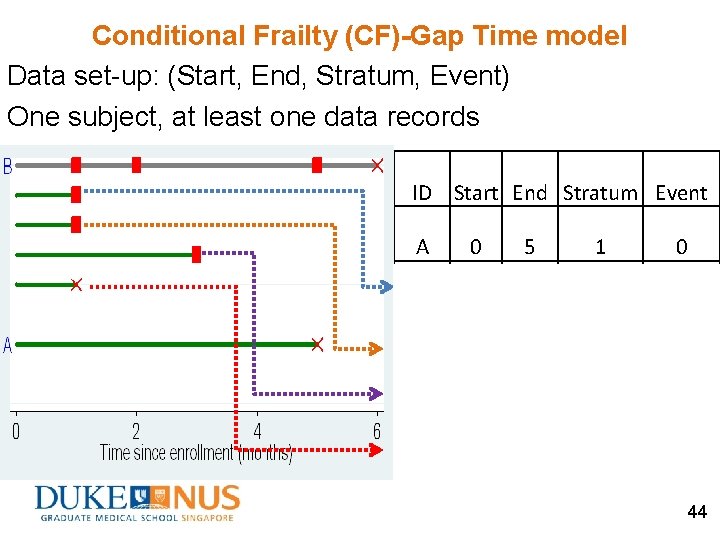
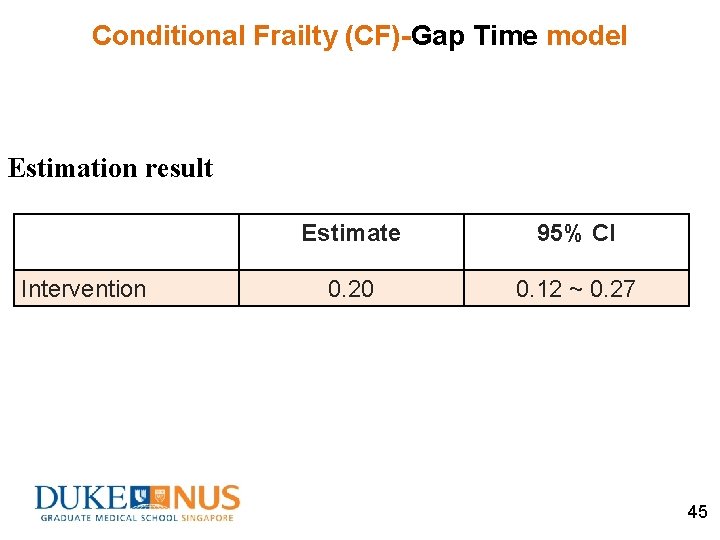
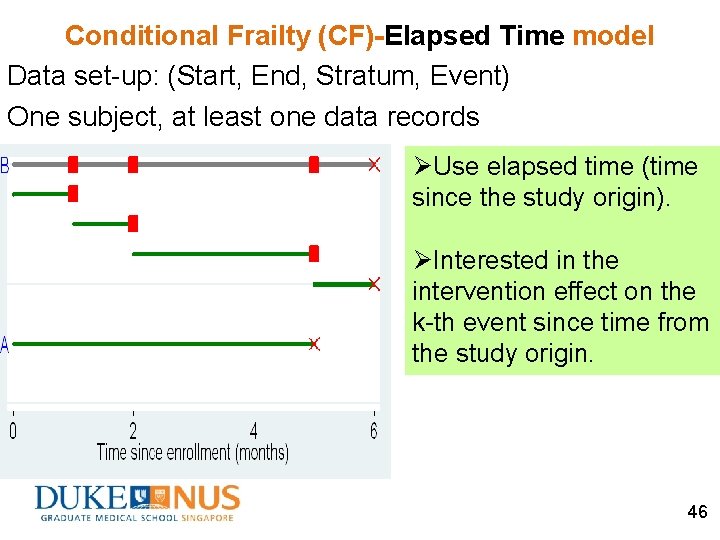
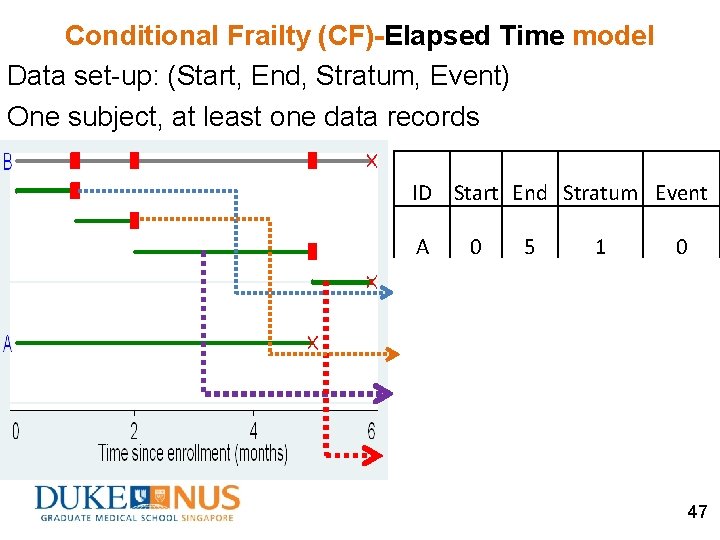
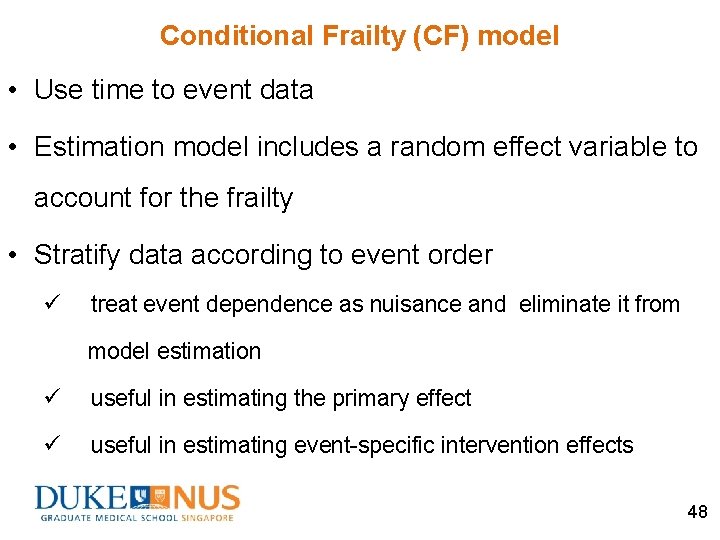
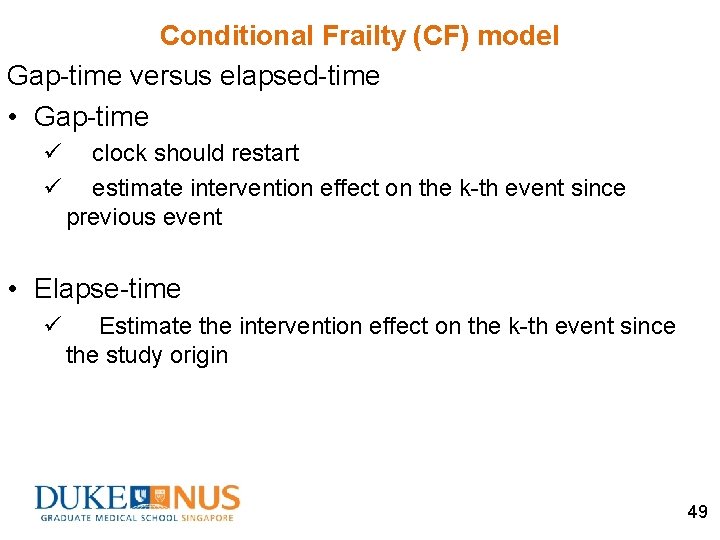
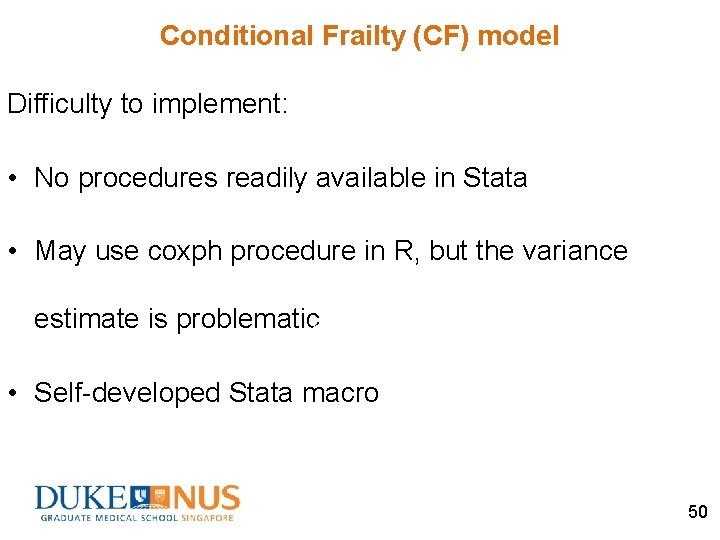
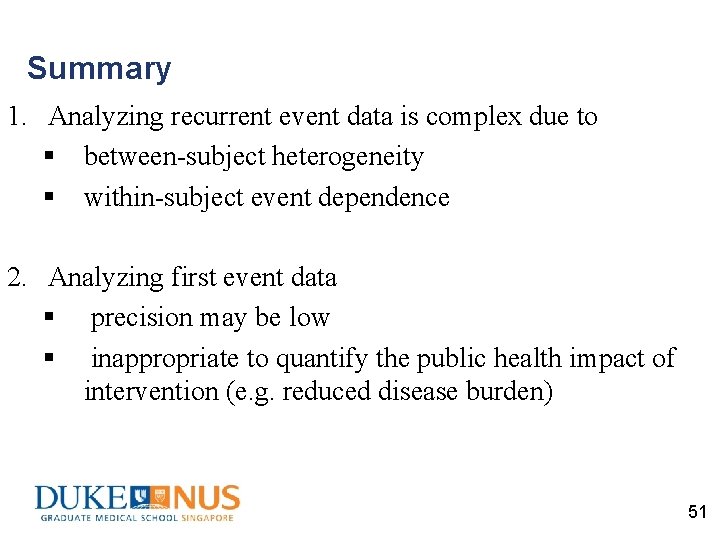
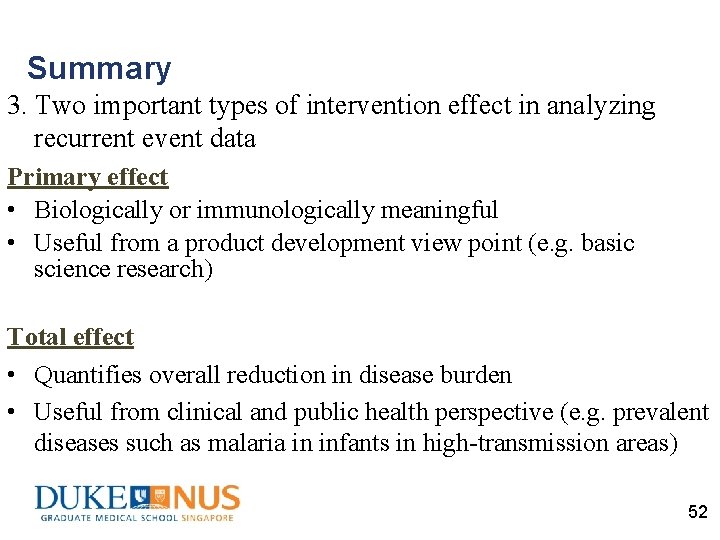
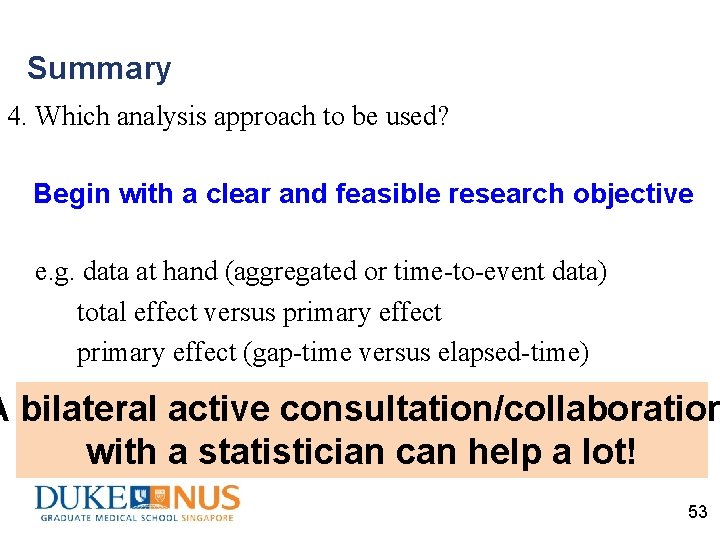
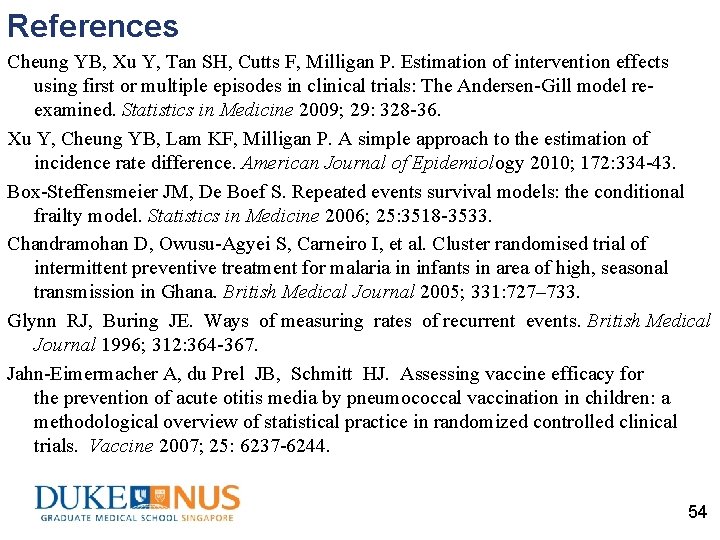
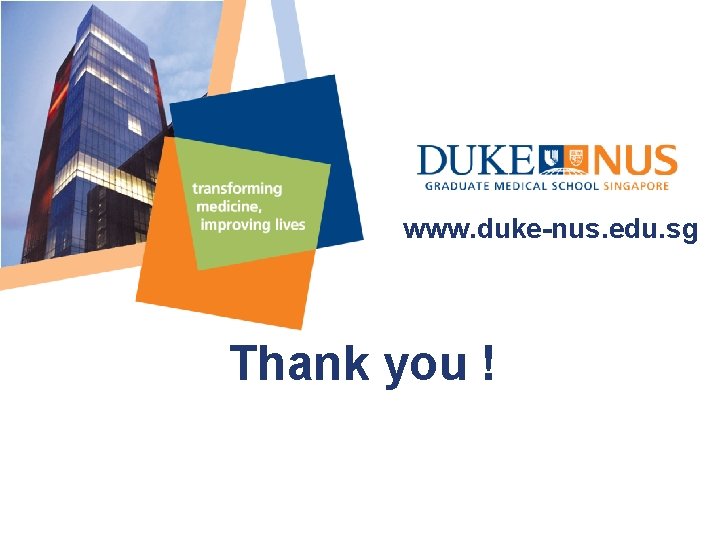
- Slides: 55
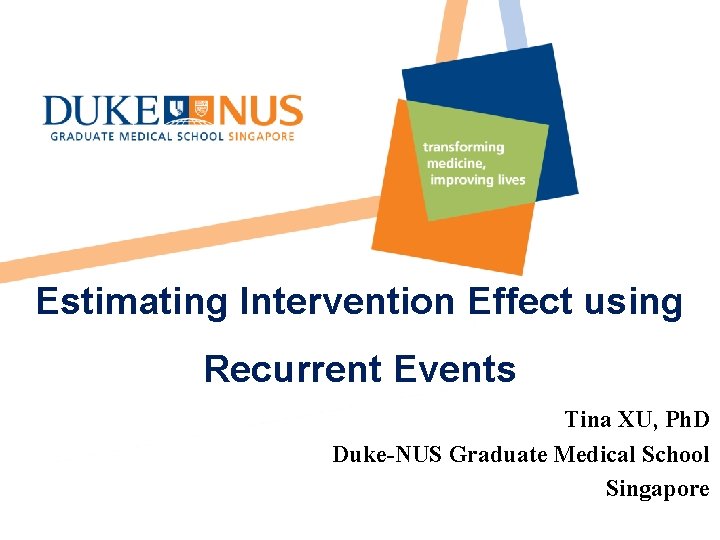
Estimating Intervention Effect using Recurrent Events Tina XU, Ph. D Duke-NUS Graduate Medical School Singapore
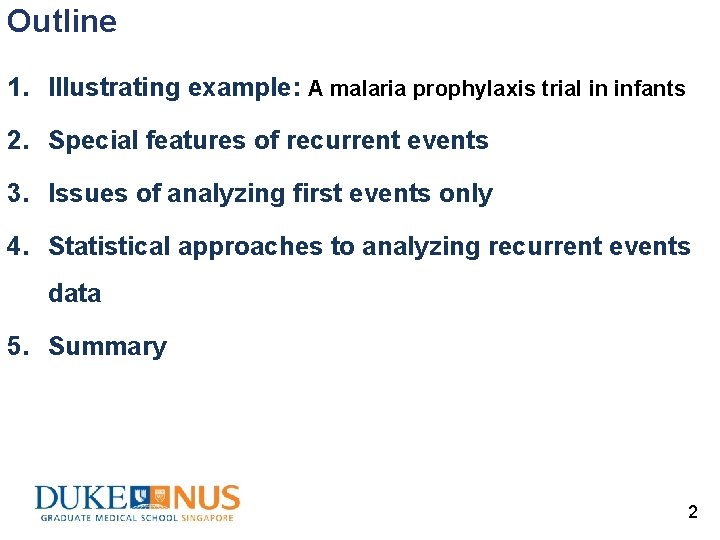
Outline 1. Illustrating example: A malaria prophylaxis trial in infants 2. Special features of recurrent events 3. Issues of analyzing first events only 4. Statistical approaches to analyzing recurrent events data 5. Summary 2
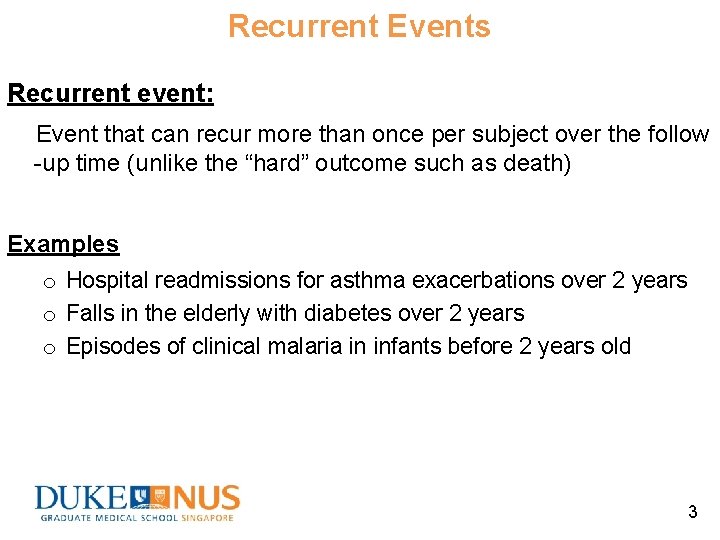
Recurrent Events Recurrent event: Event that can recur more than once per subject over the follow -up time (unlike the “hard” outcome such as death) Examples o Hospital readmissions for asthma exacerbations over 2 years o Falls in the elderly with diabetes over 2 years o Episodes of clinical malaria in infants before 2 years old 3
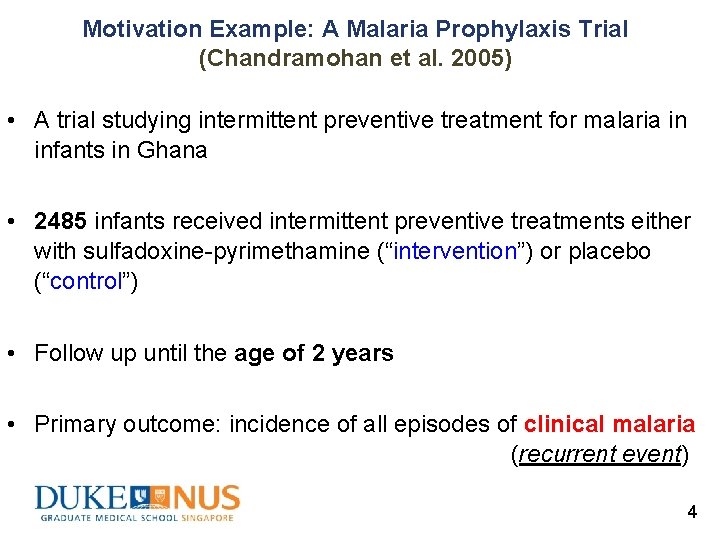
Motivation Example: A Malaria Prophylaxis Trial (Chandramohan et al. 2005) • A trial studying intermittent preventive treatment for malaria in infants in Ghana • 2485 infants received intermittent preventive treatments either with sulfadoxine-pyrimethamine (“intervention”) or placebo (“control”) • Follow up until the age of 2 years • Primary outcome: incidence of all episodes of clinical malaria (recurrent event) 4
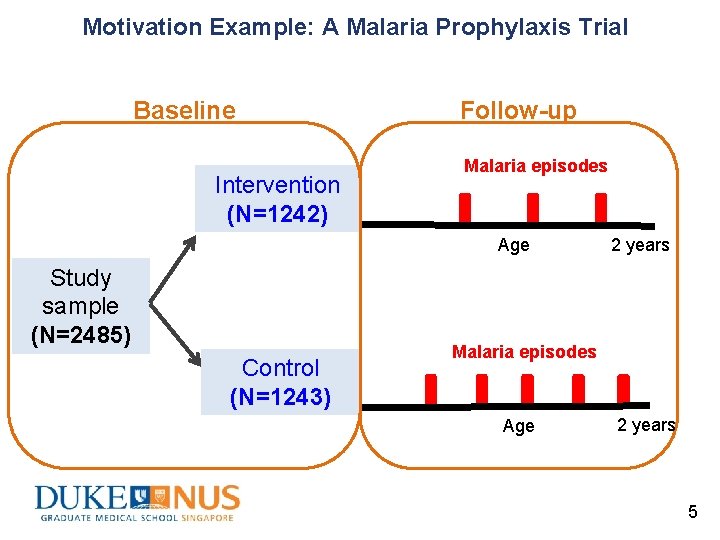
Motivation Example: A Malaria Prophylaxis Trial Baseline Follow-up Malaria episodes Intervention (N=1242) Age Study sample (N=2485) 2 years Malaria episodes Control (N=1243) Age 2 years 5
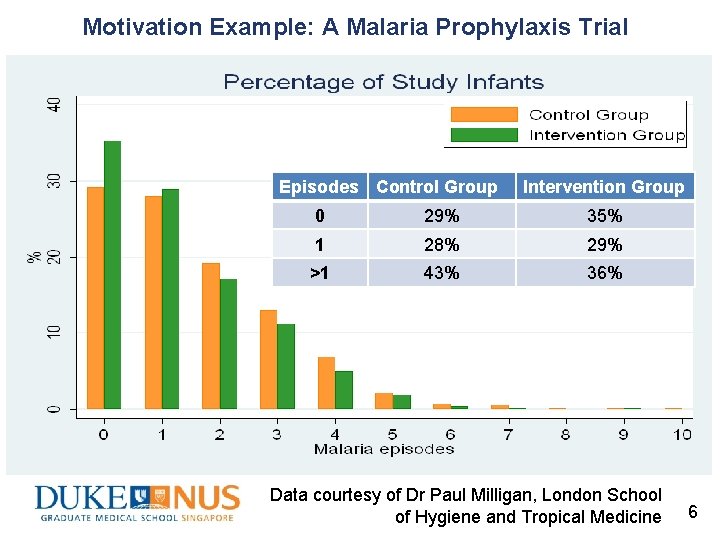
Motivation Example: A Malaria Prophylaxis Trial Episodes Control Group Intervention Group 0 29% 35% 1 28% 29% >1 43% 36% Data courtesy of Dr Paul Milligan, London School of Hygiene and Tropical Medicine 6
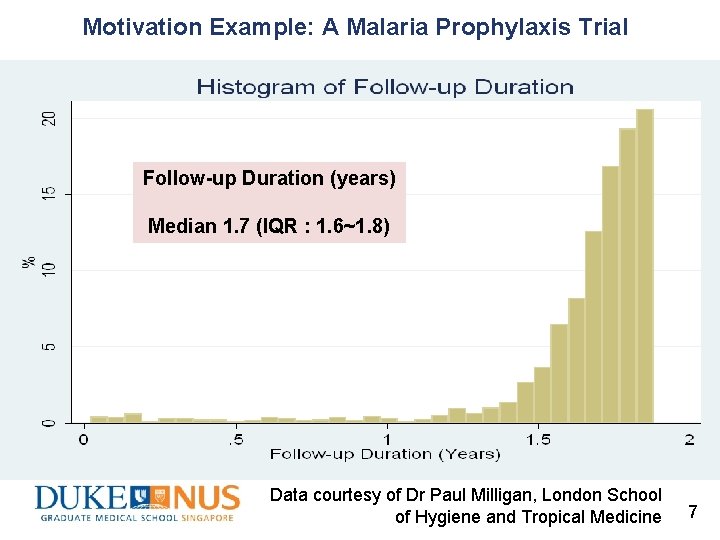
Motivation Example: A Malaria Prophylaxis Trial Follow-up Duration (years) Median 1. 7 (IQR : 1. 6~1. 8) Data courtesy of Dr Paul Milligan, London School of Hygiene and Tropical Medicine 7
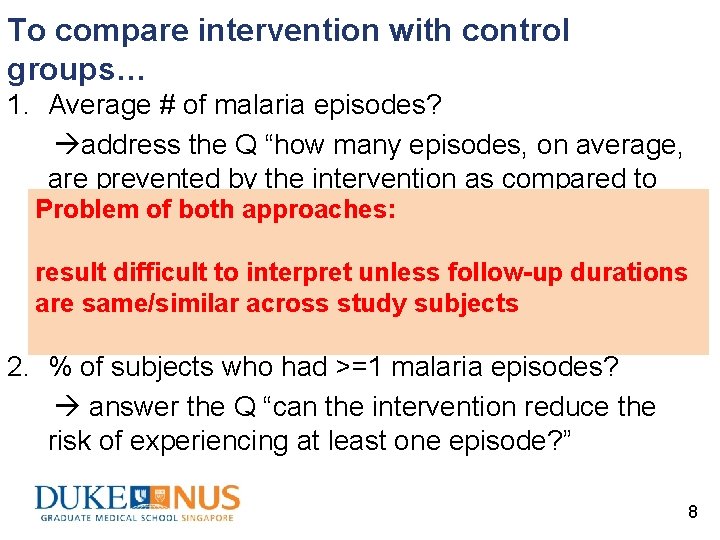
To compare intervention with control groups… 1. Average # of malaria episodes? address the Q “how many episodes, on average, are prevented by the intervention as compared to Problem of both approaches: the control? ” should be linked to a time period e. g. “over 2 result difficult to interpret unless follow-up durations years” are same/similar across study subjects 2. % of subjects who had >=1 malaria episodes? answer the Q “can the intervention reduce the risk of experiencing at least one episode? ” 8
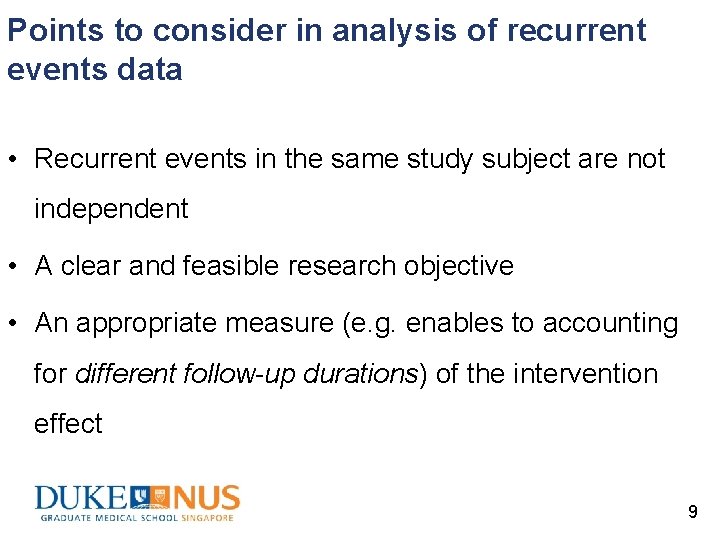
Points to consider in analysis of recurrent events data • Recurrent events in the same study subject are not independent • A clear and feasible research objective • An appropriate measure (e. g. enables to accounting for different follow-up durations) of the intervention effect 9
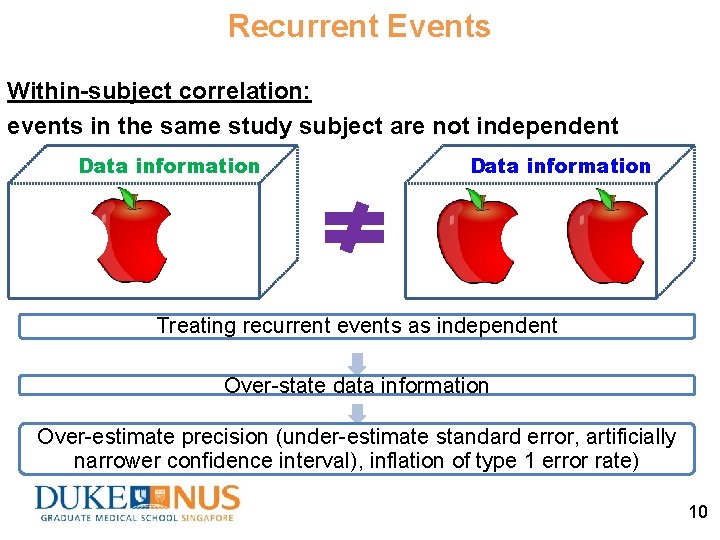
Recurrent Events Within-subject correlation: events in the same study subject are not independent Data information Treating recurrent events as independent Over-state data information Over-estimate precision (under-estimate standard error, artificially narrower confidence interval), inflation of type 1 error rate) 10
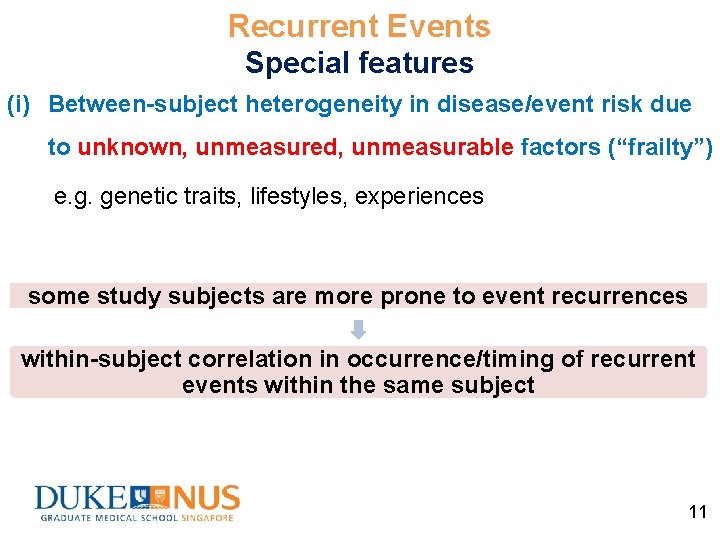
Recurrent Events Special features (i) Between-subject heterogeneity in disease/event risk due to unknown, unmeasured, unmeasurable factors (“frailty”) e. g. genetic traits, lifestyles, experiences some study subjects are more prone to event recurrences within-subject correlation in occurrence/timing of recurrent events within the same subject 11
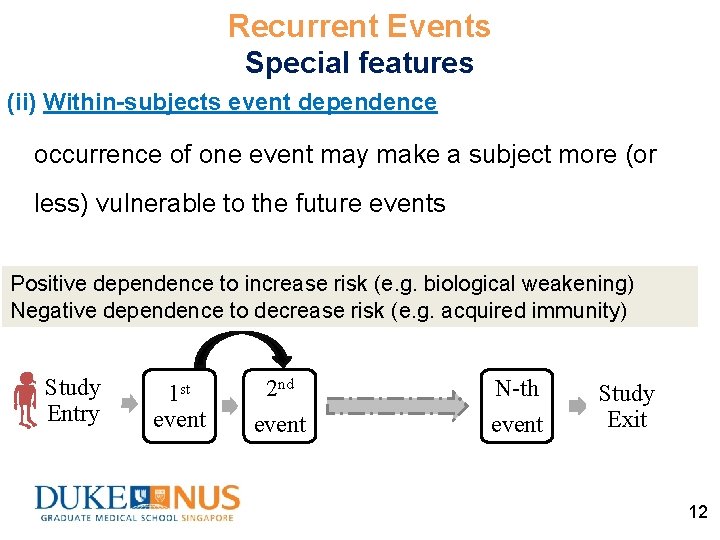
Recurrent Events Special features (ii) Within-subjects event dependence occurrence of one event may make a subject more (or less) vulnerable to the future events Positive dependence to increase risk (e. g. biological weakening) Negative dependence to decrease risk (e. g. acquired immunity) Study Entry 1 st event 2 nd event N-th event Study Exit 12
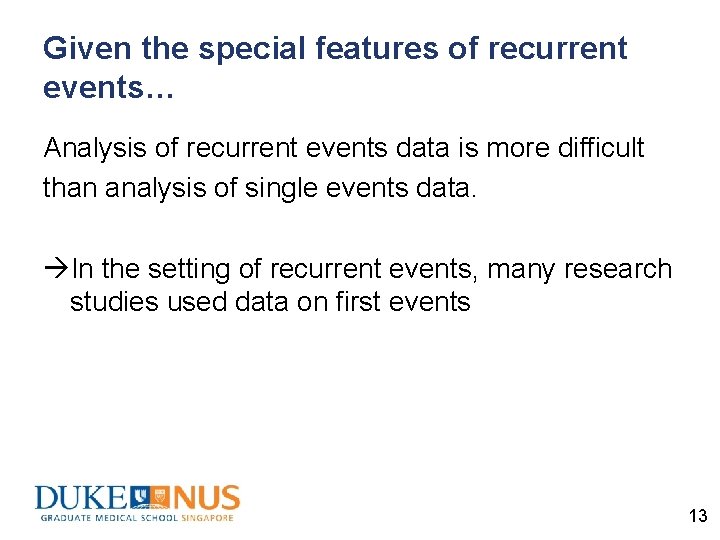
Given the special features of recurrent events… Analysis of recurrent events data is more difficult than analysis of single events data. In the setting of recurrent events, many research studies used data on first events 13
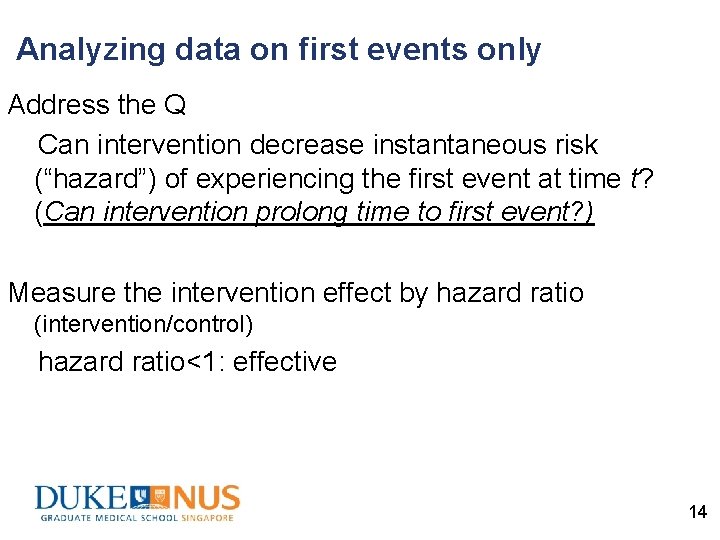
Analyzing data on first events only Address the Q Can intervention decrease instantaneous risk (“hazard”) of experiencing the first event at time t? (Can intervention prolong time to first event? ) Measure the intervention effect by hazard ratio (intervention/control) hazard ratio<1: effective 14
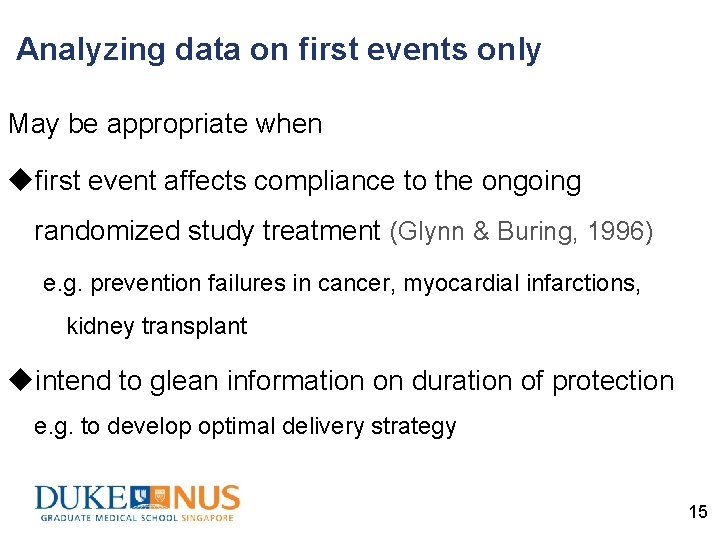
Analyzing data on first events only May be appropriate when ufirst event affects compliance to the ongoing randomized study treatment (Glynn & Buring, 1996) e. g. prevention failures in cancer, myocardial infarctions, kidney transplant uintend to glean information on duration of protection e. g. to develop optimal delivery strategy 15
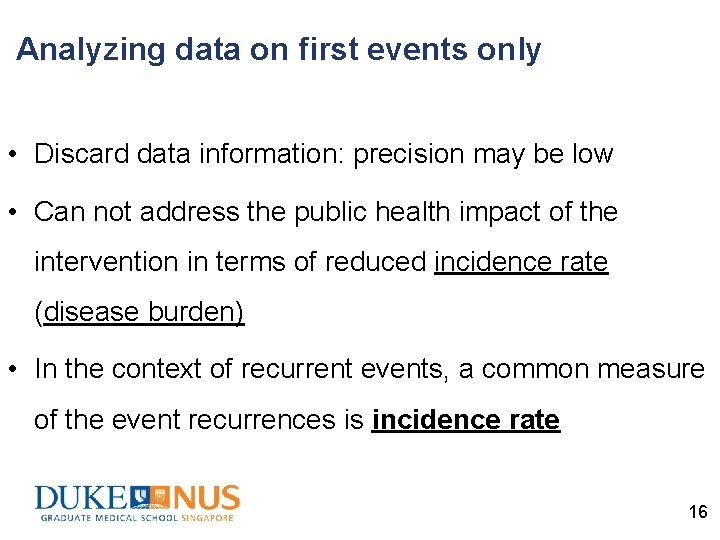
Analyzing data on first events only • Discard data information: precision may be low • Can not address the public health impact of the intervention in terms of reduced incidence rate (disease burden) • In the context of recurrent events, a common measure of the event recurrences is incidence rate 16
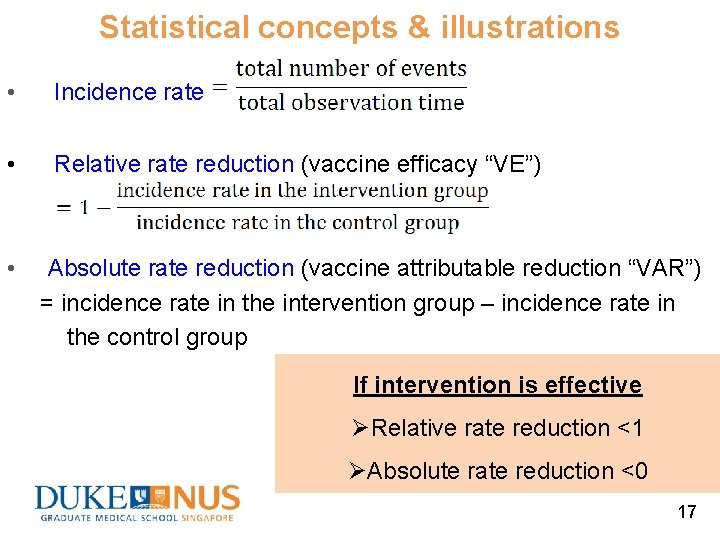
Statistical concepts & illustrations • Incidence rate • Relative rate reduction (vaccine efficacy “VE”) • Absolute rate reduction (vaccine attributable reduction “VAR”) = incidence rate in the intervention group – incidence rate in the control group If intervention is effective Ø Relative rate reduction <1 ØAbsolute rate reduction <0 17
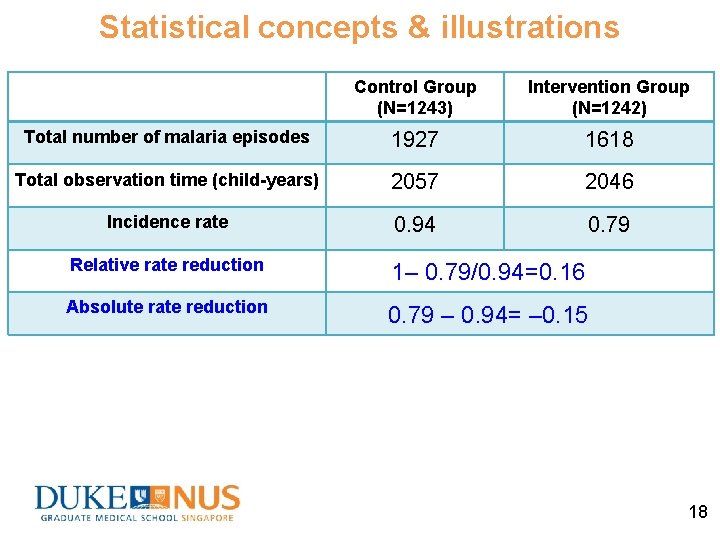
Statistical concepts & illustrations Control Group (N=1243) Intervention Group (N=1242) Total number of malaria episodes 1927 1618 Total observation time (child-years) 2057 2046 Incidence rate 0. 94 0. 79 Relative rate reduction 1– 0. 79/0. 94=0. 16 Absolute rate reduction 0. 79 – 0. 94= – 0. 15 18
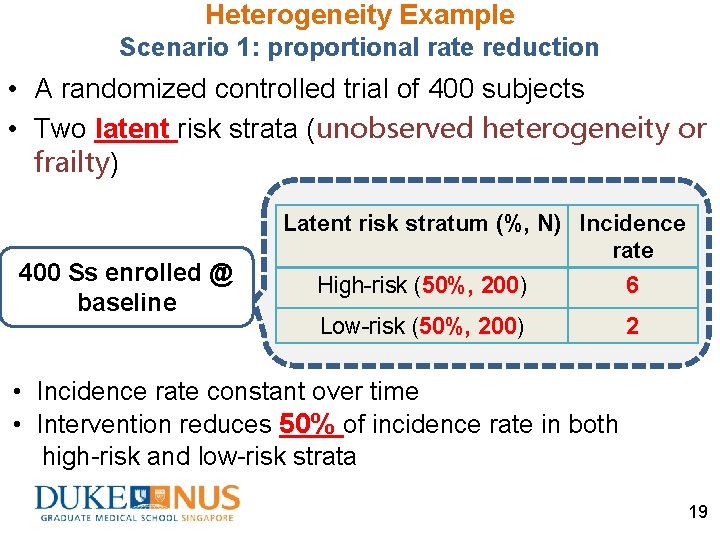
Heterogeneity Example Scenario 1: proportional rate reduction • A randomized controlled trial of 400 subjects • Two latent risk strata (unobserved heterogeneity or frailty) 400 Ss enrolled @ baseline Latent risk stratum (%, N) Incidence rate High-risk (50%, 200) 6 Low-risk (50%, 200) 2 • Incidence rate constant over time • Intervention reduces 50% of incidence rate in both high-risk and low-risk strata 19
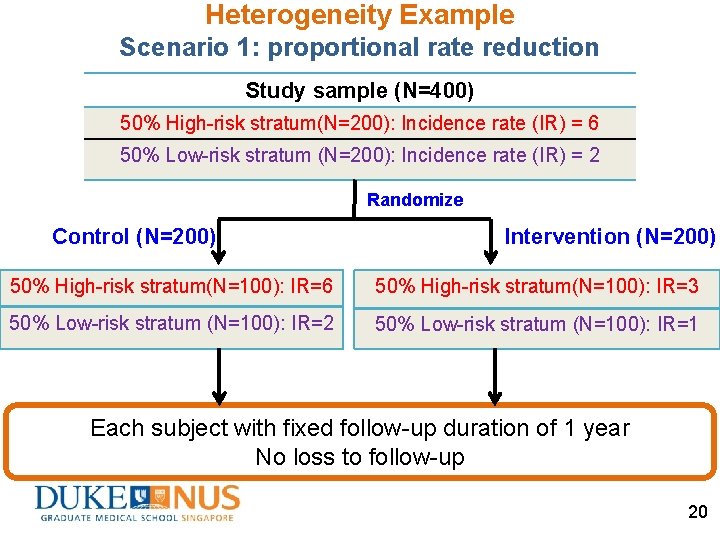
Heterogeneity Example Scenario 1: proportional rate reduction Study sample (N=400) 50% High-risk stratum(N=200): Incidence rate (IR) = 6 50% Low-risk stratum (N=200): Incidence rate (IR) = 2 Randomize Control (N=200) Intervention (N=200) 50% High-risk stratum(N=100): IR=6 50% High-risk stratum(N=100): IR=3 50% Low-risk stratum (N=100): IR=2 50% Low-risk stratum (N=100): IR=1 Each subject with fixed follow-up duration of 1 year No loss to follow-up 20
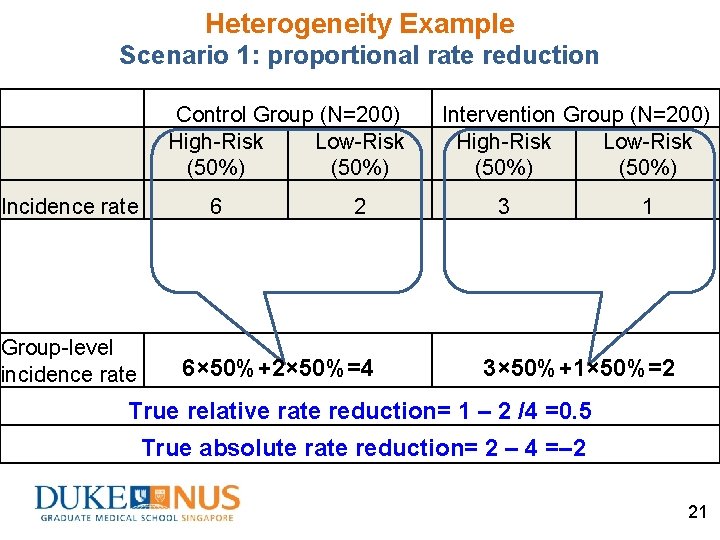
Heterogeneity Example Scenario 1: proportional rate reduction Control Group (N=200) High-Risk Low-Risk (50%) Incidence rate Group-level incidence rate 6 2 6× 50%+2× 50%=4 Intervention Group (N=200) High-Risk Low-Risk (50%) 3 1 3× 50%+1× 50%=2 True relative rate reduction= 1 – 2 /4 =0. 5 True absolute rate reduction= 2 – 4 =– 2 21
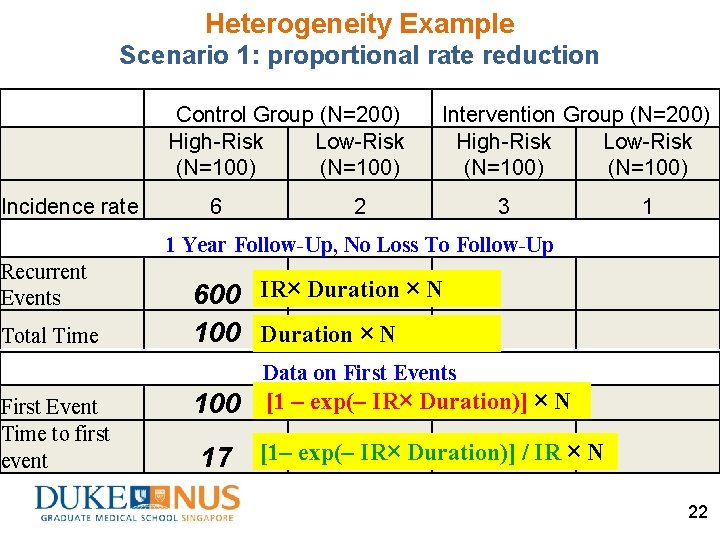
Heterogeneity Example Scenario 1: proportional rate reduction Control Group (N=200) High-Risk Low-Risk (N=100) Incidence rate 6 Intervention Group (N=200) High-Risk Low-Risk (N=100) 2 3 1 1 Year Follow-Up, No Loss To Follow-Up Recurrent Events Total Time 600 IR× Duration × N 100 Duration × N Data on First Events First Event Time to first event 100 [1 – exp(– IR× Duration)] × N 17 [1– exp(– IR× Duration)] / IR × N 22
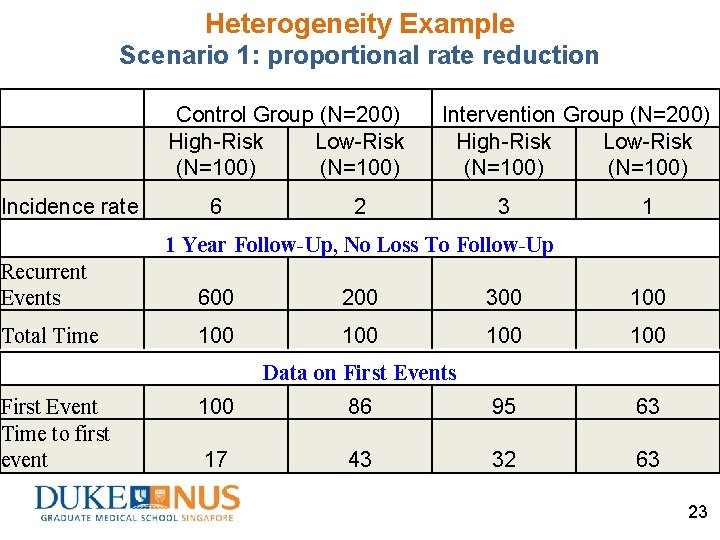
Heterogeneity Example Scenario 1: proportional rate reduction Control Group (N=200) High-Risk Low-Risk (N=100) Incidence rate 6 Intervention Group (N=200) High-Risk Low-Risk (N=100) 2 3 1 1 Year Follow-Up, No Loss To Follow-Up Recurrent Events 600 200 300 100 Total Time 100 100 95 63 32 63 Data on First Events First Event Time to first event 100 86 17 43 23
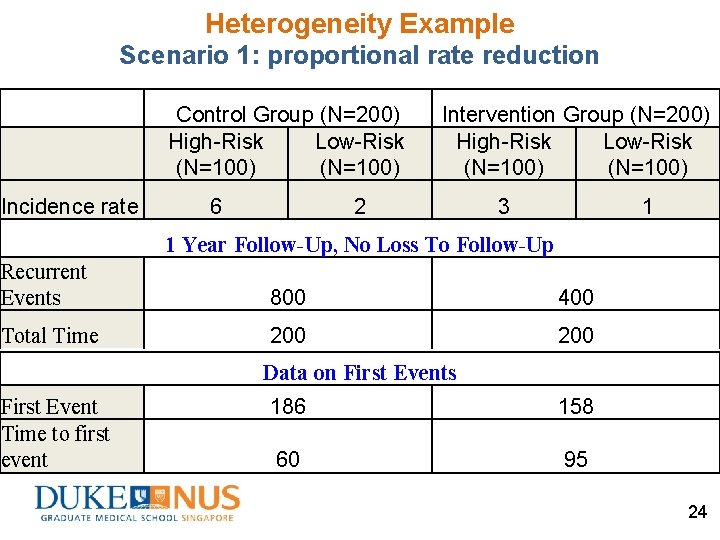
Heterogeneity Example Scenario 1: proportional rate reduction Control Group (N=200) High-Risk Low-Risk (N=100) Incidence rate 6 Intervention Group (N=200) High-Risk Low-Risk (N=100) 2 3 1 1 Year Follow-Up, No Loss To Follow-Up Recurrent Events 800 400 Total Time 200 Data on First Events First Event Time to first event 186 158 60 95 24
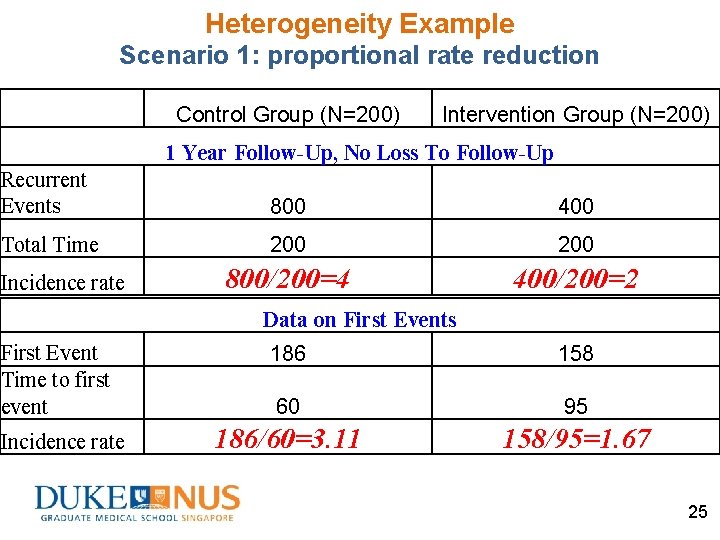
Heterogeneity Example Scenario 1: proportional rate reduction Control Group (N=200) Intervention Group (N=200) 1 Year Follow-Up, No Loss To Follow-Up Recurrent Events 800 400 Total Time 200 800/200=4 400/200=2 Incidence rate Data on First Events First Event Time to first event Incidence rate 186 158 60 95 186/60=3. 11 158/95=1. 67 25
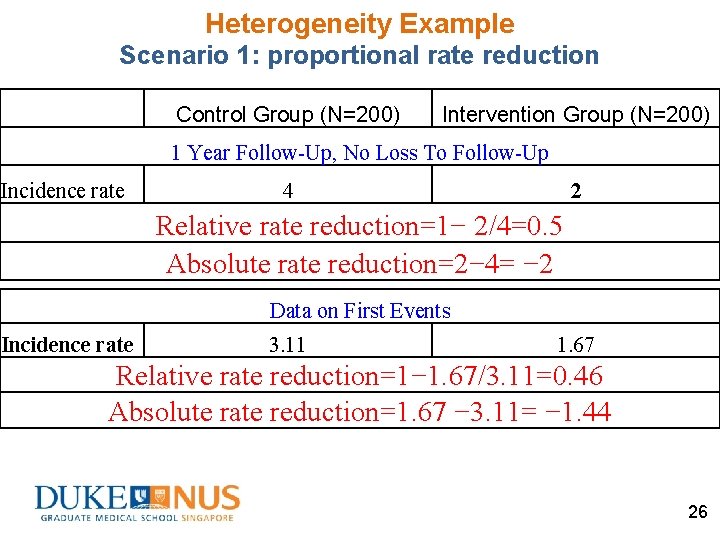
Heterogeneity Example Scenario 1: proportional rate reduction Control Group (N=200) Intervention Group (N=200) 1 Year Follow-Up, No Loss To Follow-Up Incidence rate 4 2 Relative rate reduction=1− 2/4=0. 5 Absolute rate reduction=2− 4= − 2 Data on First Events Incidence rate 3. 11 1. 67 Relative rate reduction=1− 1. 67/3. 11=0. 46 Absolute rate reduction=1. 67 − 3. 11= − 1. 44 26
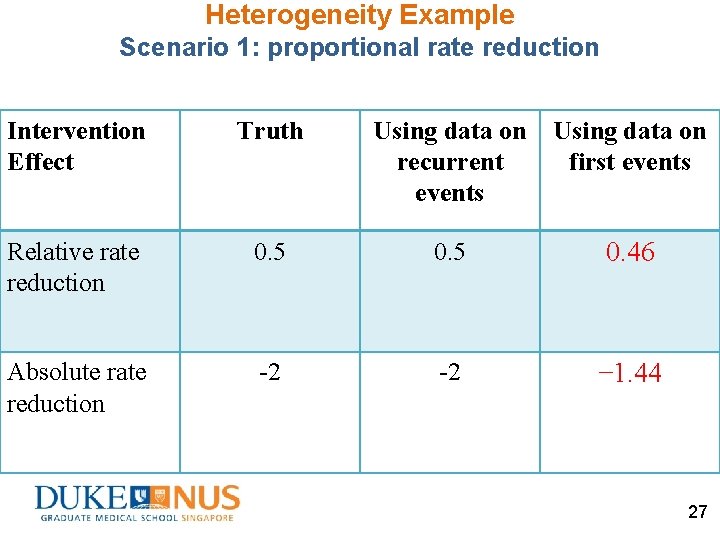
Heterogeneity Example Scenario 1: proportional rate reduction Intervention Effect Truth Using data on recurrent events Using data on first events Relative rate reduction 0. 5 0. 46 Absolute rate reduction -2 -2 − 1. 44 27
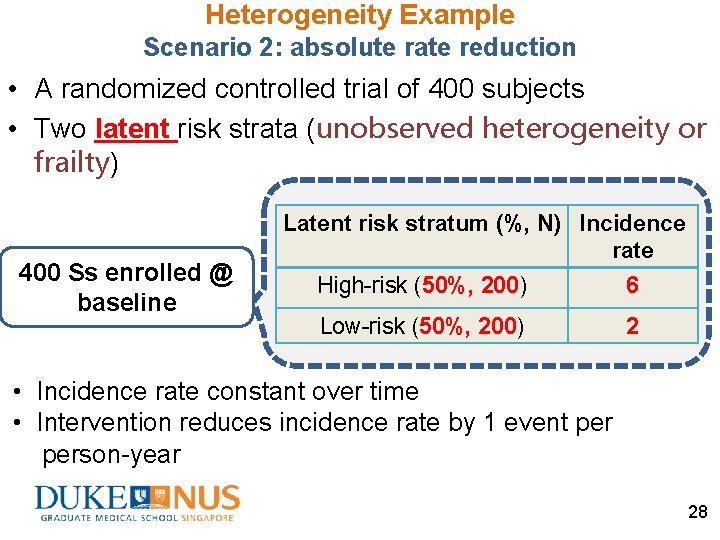
Heterogeneity Example Scenario 2: absolute rate reduction • A randomized controlled trial of 400 subjects • Two latent risk strata (unobserved heterogeneity or frailty) 400 Ss enrolled @ baseline Latent risk stratum (%, N) Incidence rate High-risk (50%, 200) 6 Low-risk (50%, 200) 2 • Incidence rate constant over time • Intervention reduces incidence rate by 1 event person-year 28
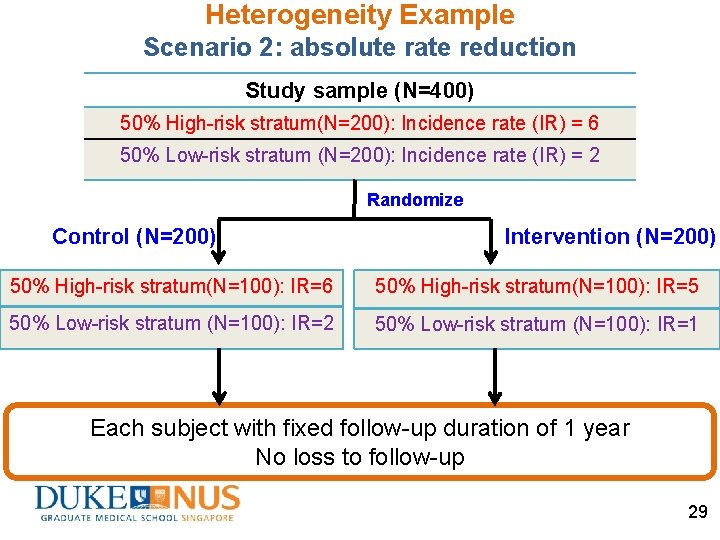
Heterogeneity Example Scenario 2: absolute rate reduction Study sample (N=400) 50% High-risk stratum(N=200): Incidence rate (IR) = 6 50% Low-risk stratum (N=200): Incidence rate (IR) = 2 Randomize Control (N=200) Intervention (N=200) 50% High-risk stratum(N=100): IR=6 50% High-risk stratum(N=100): IR=5 50% Low-risk stratum (N=100): IR=2 50% Low-risk stratum (N=100): IR=1 Each subject with fixed follow-up duration of 1 year No loss to follow-up 29
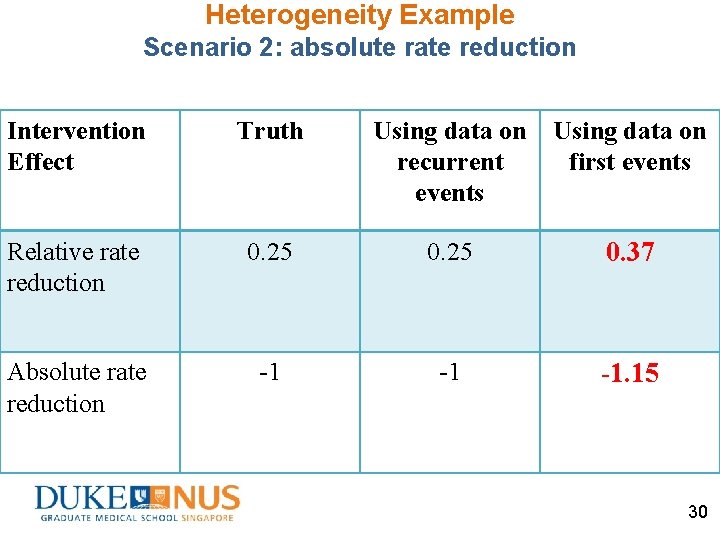
Heterogeneity Example Scenario 2: absolute rate reduction Intervention Effect Truth Using data on recurrent events Using data on first events Relative rate reduction 0. 25 0. 37 Absolute rate reduction -1 -1 -1. 15 30
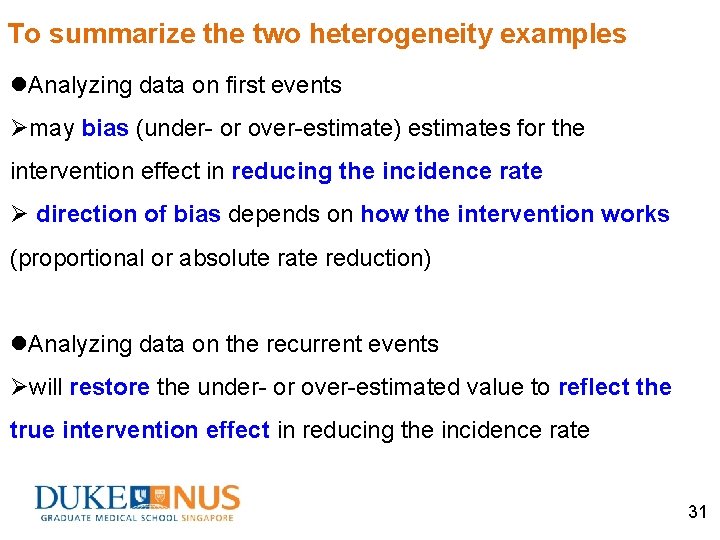
To summarize the two heterogeneity examples l. Analyzing data on first events Ømay bias (under- or over-estimate) estimates for the intervention effect in reducing the incidence rate Ø direction of bias depends on how the intervention works (proportional or absolute rate reduction) l. Analyzing data on the recurrent events Øwill restore the under- or over-estimated value to reflect the true intervention effect in reducing the incidence rate 31
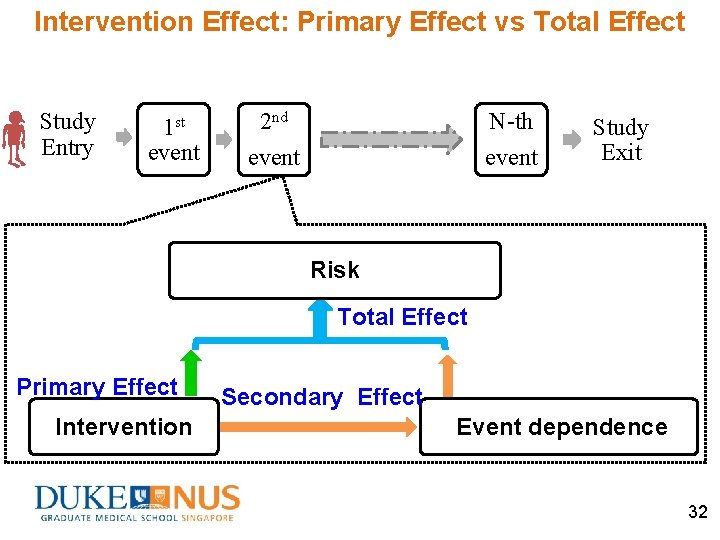
Intervention Effect: Primary Effect vs Total Effect Study Entry 1 st event 2 nd N-th event Study Exit Risk Total Effect Primary Effect Intervention Secondary Effect Event dependence 32
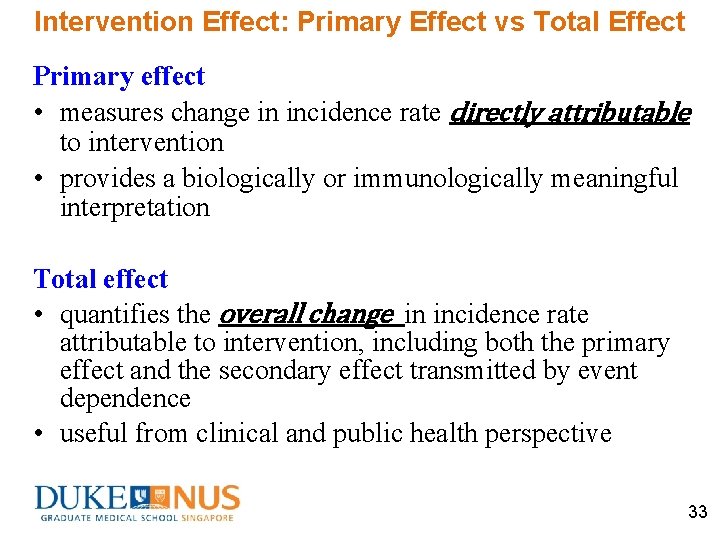
Intervention Effect: Primary Effect vs Total Effect Primary effect • measures change in incidence rate directly attributable to intervention • provides a biologically or immunologically meaningful interpretation Total effect • quantifies the overall change in incidence rate attributable to intervention, including both the primary effect and the secondary effect transmitted by event dependence • useful from clinical and public health perspective 33
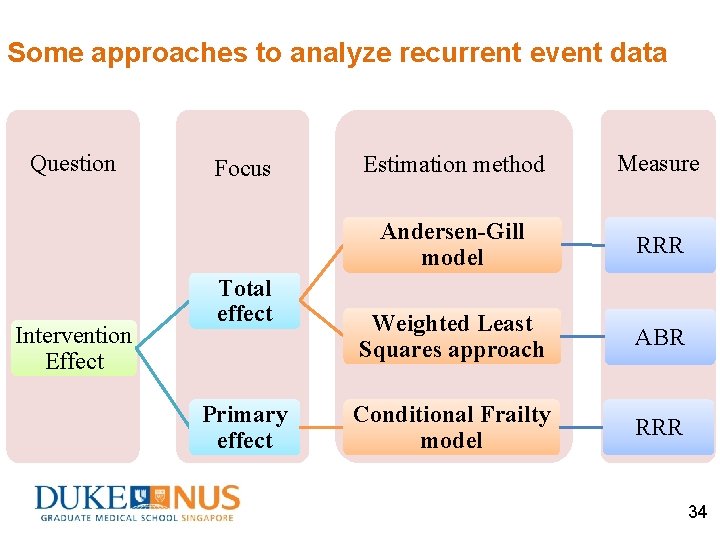
Some approaches to analyze recurrent event data Question Intervention Effect Focus Total effect Primary effect Estimation method Measure Andersen-Gill model RRR Weighted Least Squares approach ABR Conditional Frailty model RRR 34
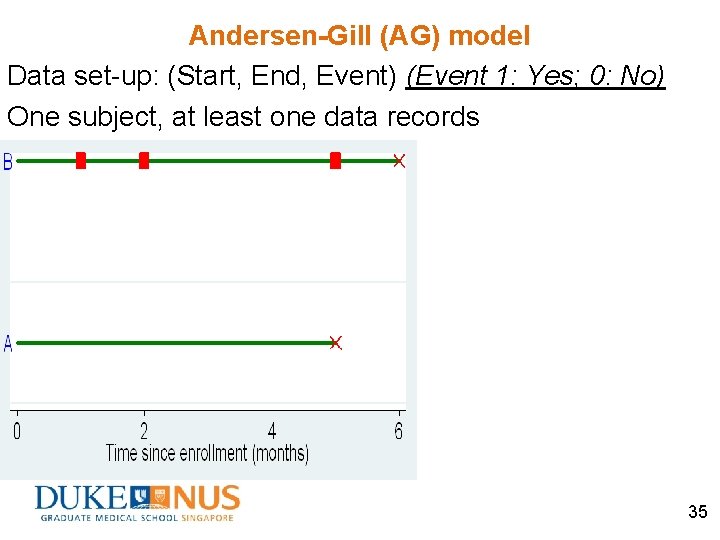
Andersen-Gill (AG) model Data set-up: (Start, End, Event) (Event 1: Yes; 0: No) One subject, at least one data records 35
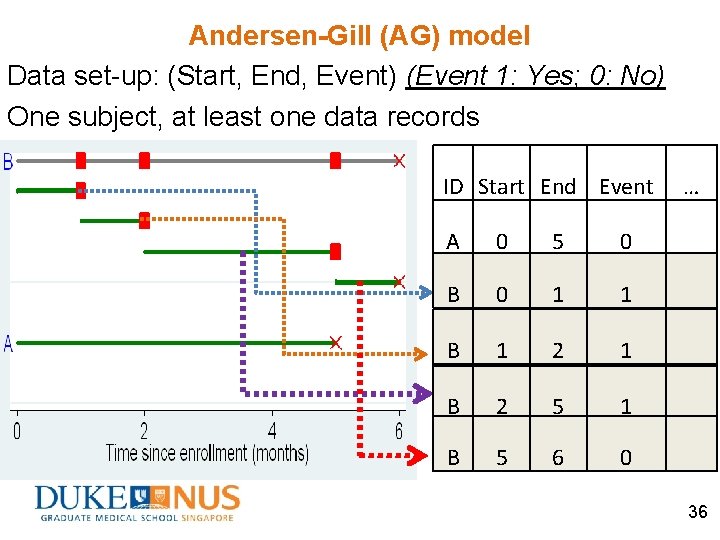
Andersen-Gill (AG) model Data set-up: (Start, End, Event) (Event 1: Yes; 0: No) One subject, at least one data records ID Start End Event A 0 5 0 B 0 1 1 B 1 2 1 B 2 5 1 B 5 6 0 … 36
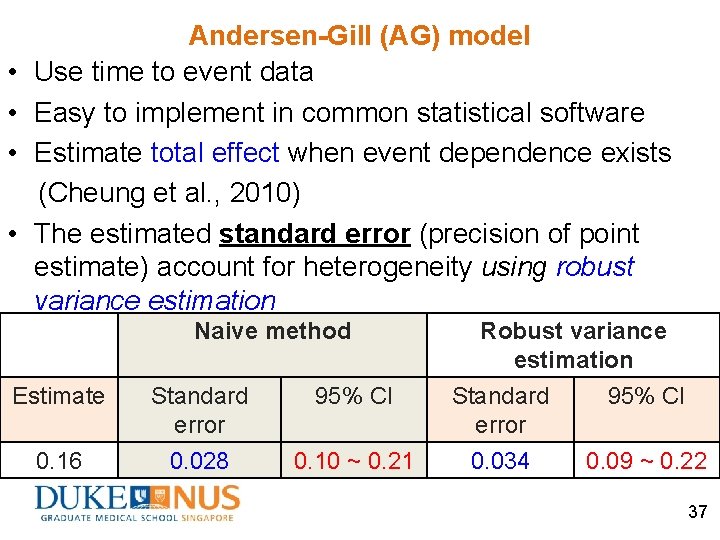
• • Andersen-Gill (AG) model Use time to event data Easy to implement in common statistical software Estimate total effect when event dependence exists (Cheung et al. , 2010) The estimated standard error (precision of point estimate) account for heterogeneity using robust variance estimation Naive method Estimate 0. 16 Standard error 0. 028 Robust variance estimation 95% CI 0. 10 ~ 0. 21 Standard error 0. 034 95% CI 0. 09 ~ 0. 22 37
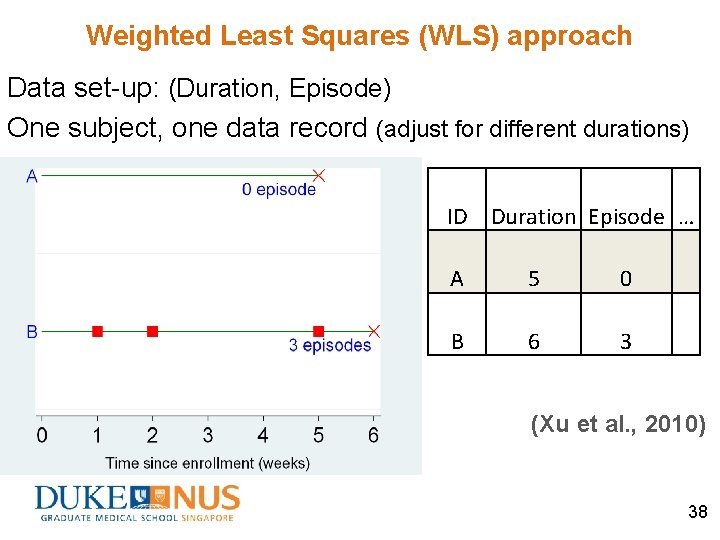
Weighted Least Squares (WLS) approach Data set-up: (Duration, Episode) One subject, one data record (adjust for different durations) ID Duration Episode … A 5 0 B 6 3 (Xu et al. , 2010) 38
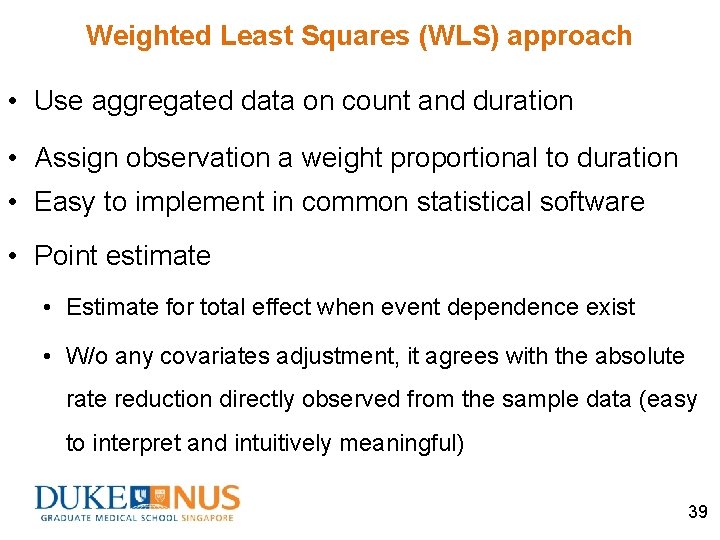
Weighted Least Squares (WLS) approach • Use aggregated data on count and duration • Assign observation a weight proportional to duration • Easy to implement in common statistical software • Point estimate • Estimate for total effect when event dependence exist • W/o any covariates adjustment, it agrees with the absolute rate reduction directly observed from the sample data (easy to interpret and intuitively meaningful) 39
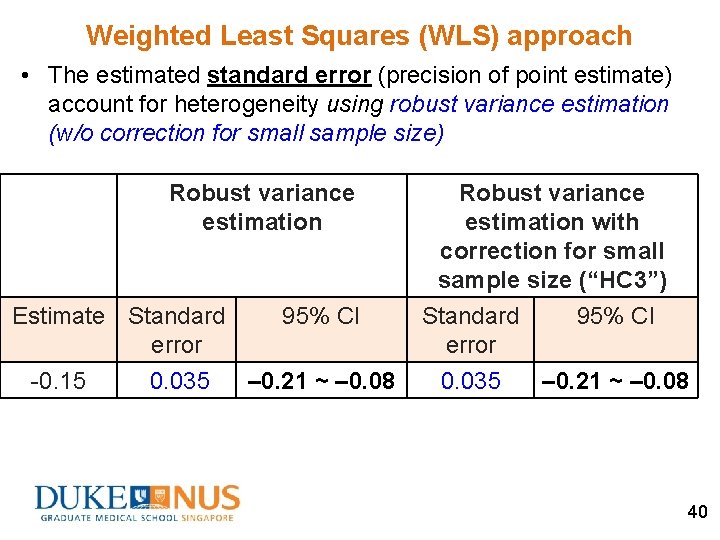
Weighted Least Squares (WLS) approach • The estimated standard error (precision of point estimate) account for heterogeneity using robust variance estimation (w/o correction for small sample size) Robust variance estimation Estimate Standard error -0. 15 0. 035 95% CI – 0. 21 ~ – 0. 08 Robust variance estimation with correction for small sample size (“HC 3”) Standard 95% CI error 0. 035 – 0. 21 ~ – 0. 08 40
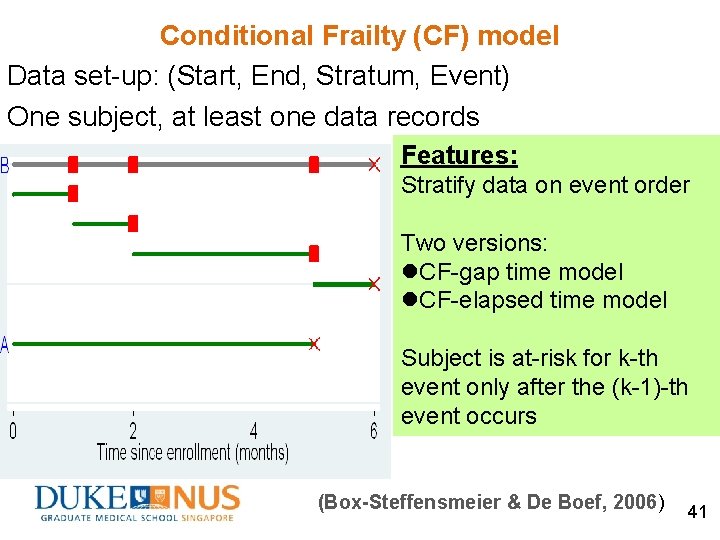
Conditional Frailty (CF) model Data set-up: (Start, End, Stratum, Event) One subject, at least one data records Features: Stratify data on event order Two versions: l. CF-gap time model l. CF-elapsed time model Subject is at-risk for k-th event only after the (k-1)-th event occurs (Box-Steffensmeier & De Boef, 2006) 41
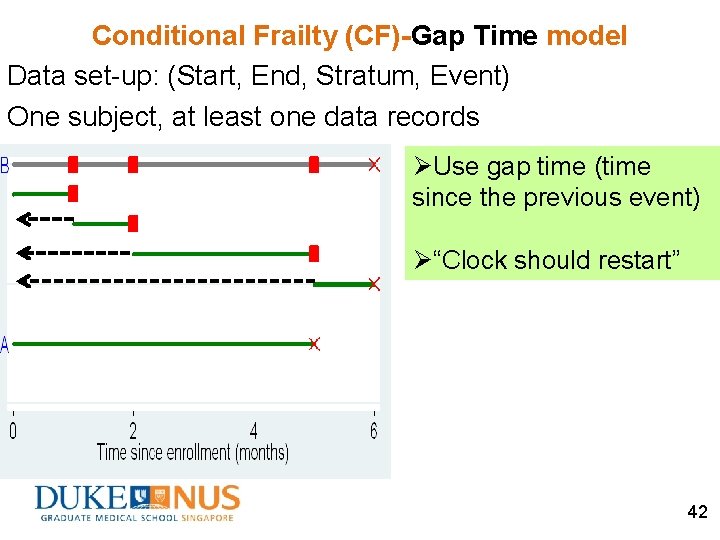
Conditional Frailty (CF)-Gap Time model Data set-up: (Start, End, Stratum, Event) One subject, at least one data records ØUse gap time (time since the previous event) Ø“Clock should restart” 42
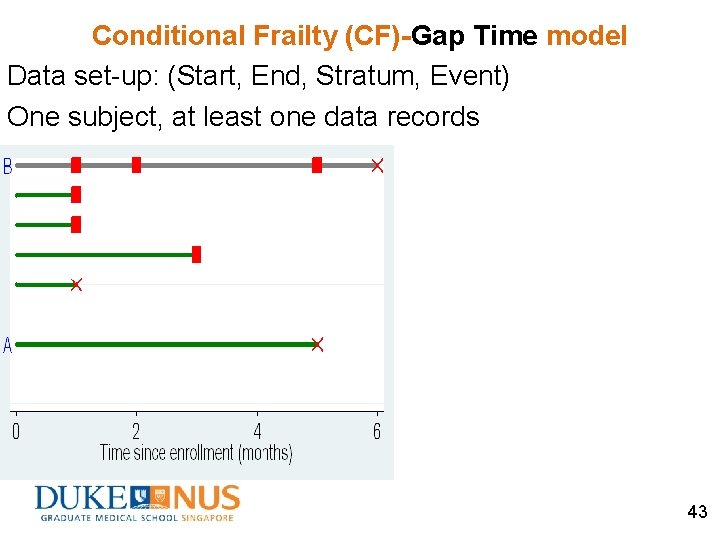
Conditional Frailty (CF)-Gap Time model Data set-up: (Start, End, Stratum, Event) One subject, at least one data records 43
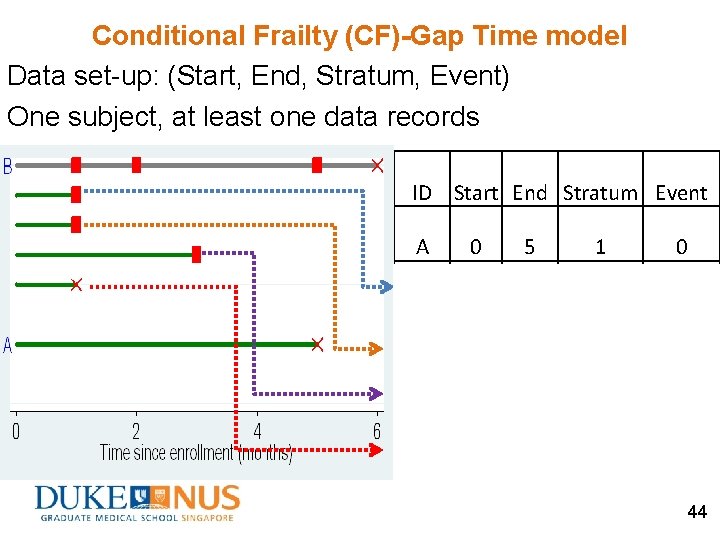
Conditional Frailty (CF)-Gap Time model Data set-up: (Start, End, Stratum, Event) One subject, at least one data records ID Start End Stratum Event A 0 5 1 0 B 0 1 1 1 B 0 1 2 1 B 0 3 3 1 B 0 1 4 0 44
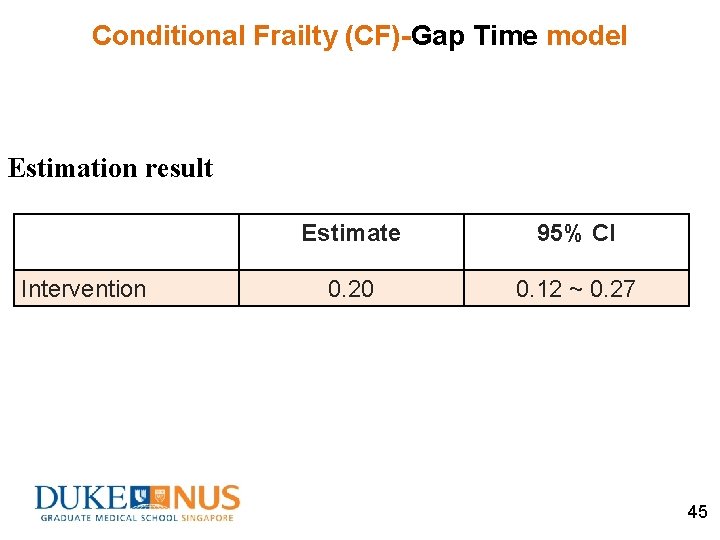
Conditional Frailty (CF)-Gap Time model Estimation result Intervention Estimate 95% CI 0. 20 0. 12 ~ 0. 27 45
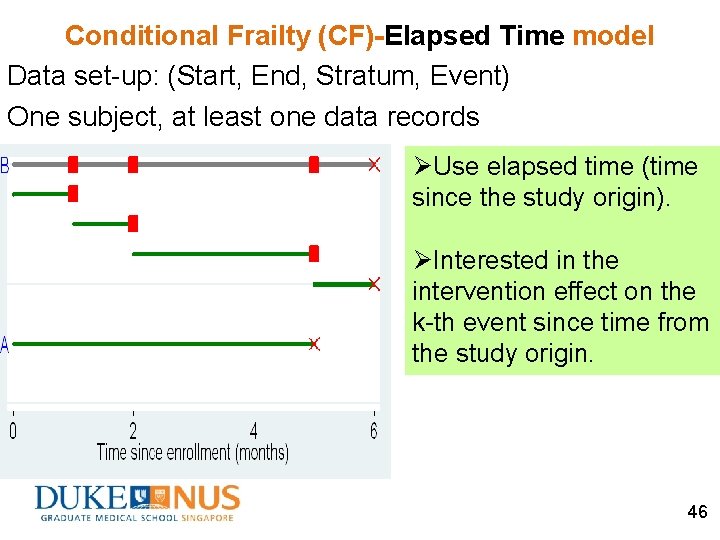
Conditional Frailty (CF)-Elapsed Time model Data set-up: (Start, End, Stratum, Event) One subject, at least one data records ØUse elapsed time (time since the study origin). ØInterested in the intervention effect on the k-th event since time from the study origin. 46
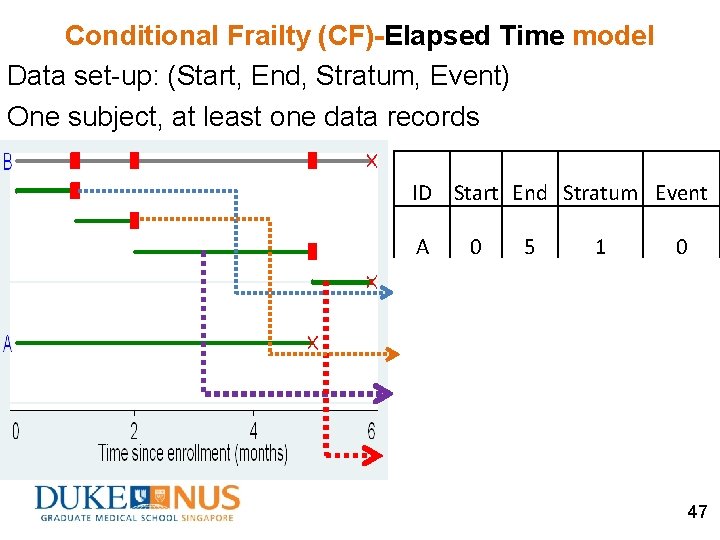
Conditional Frailty (CF)-Elapsed Time model Data set-up: (Start, End, Stratum, Event) One subject, at least one data records ID Start End Stratum Event A 0 5 1 0 B 0 1 1 1 B 1 2 2 1 B 2 5 3 1 B 5 6 4 0 47
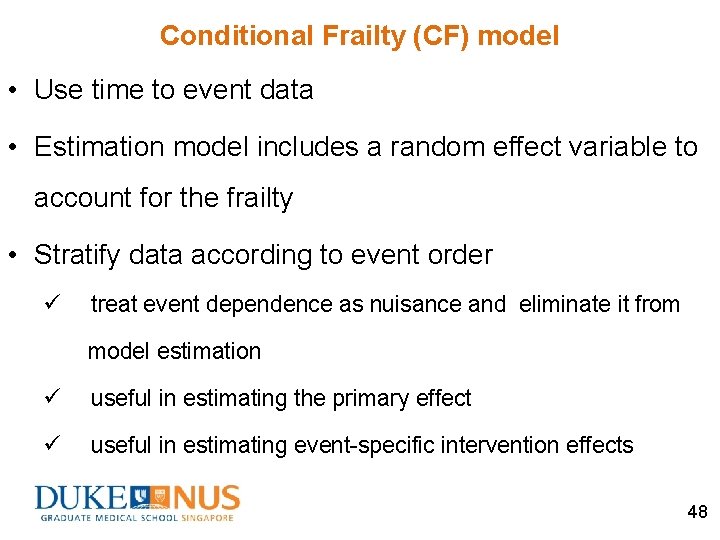
Conditional Frailty (CF) model • Use time to event data • Estimation model includes a random effect variable to account for the frailty • Stratify data according to event order ü treat event dependence as nuisance and eliminate it from model estimation ü useful in estimating the primary effect ü useful in estimating event-specific intervention effects 48
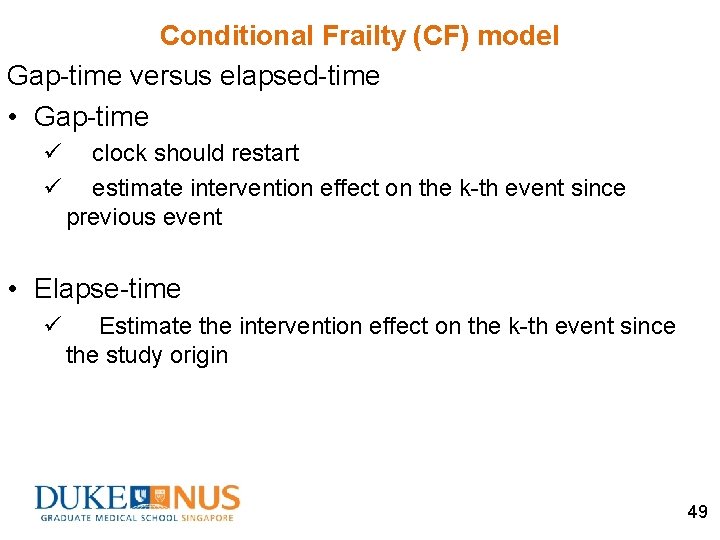
Conditional Frailty (CF) model Gap-time versus elapsed-time • Gap-time ü ü clock should restart estimate intervention effect on the k-th event since previous event • Elapse-time ü Estimate the intervention effect on the k-th event since the study origin 49
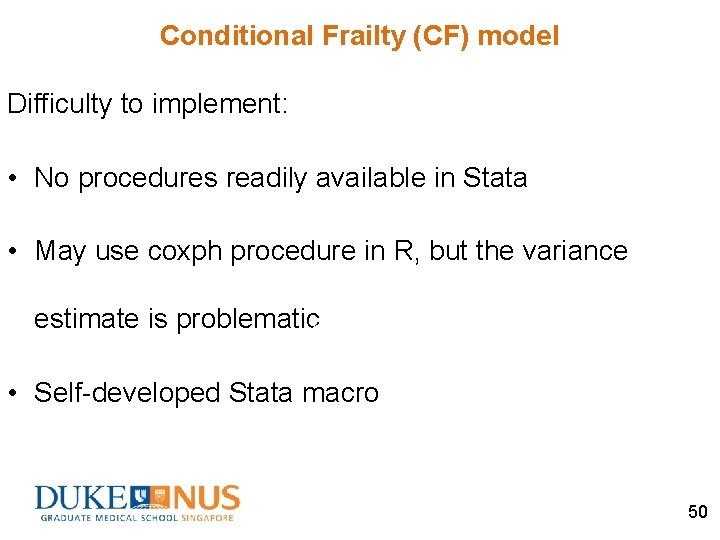
Conditional Frailty (CF) model Difficulty to implement: • No procedures readily available in Stata • May use coxph procedure in R, but the variance estimate is problematic • Self-developed Stata macro 50
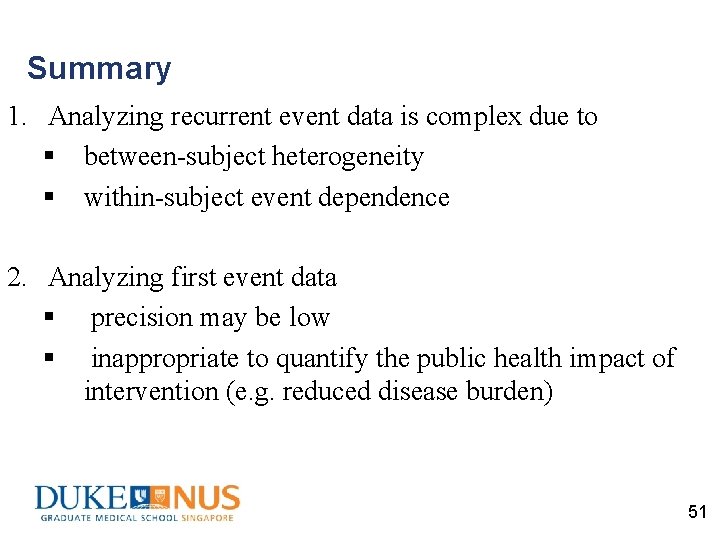
Summary 1. Analyzing recurrent event data is complex due to § between-subject heterogeneity § within-subject event dependence 2. Analyzing first event data § precision may be low § inappropriate to quantify the public health impact of intervention (e. g. reduced disease burden) 51
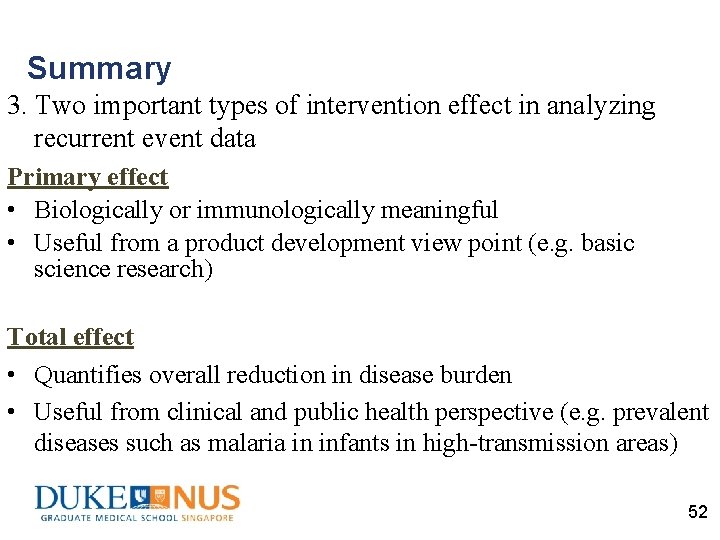
Summary 3. Two important types of intervention effect in analyzing recurrent event data Primary effect • Biologically or immunologically meaningful • Useful from a product development view point (e. g. basic science research) Total effect • Quantifies overall reduction in disease burden • Useful from clinical and public health perspective (e. g. prevalent diseases such as malaria in infants in high-transmission areas) 52
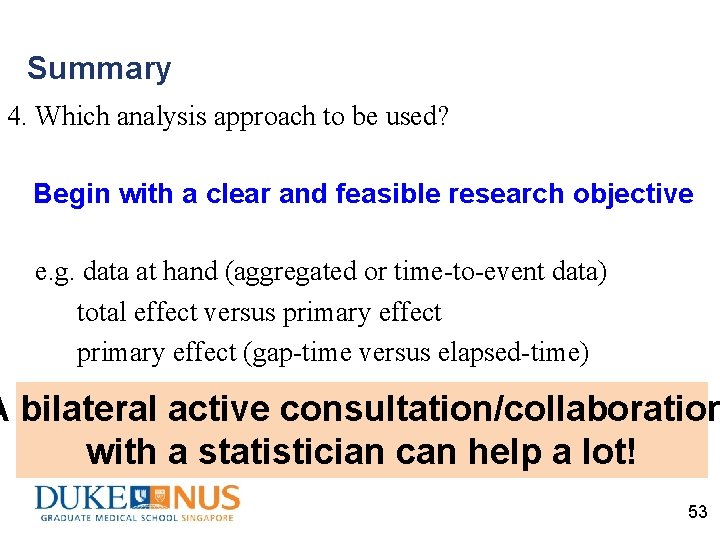
Summary 4. Which analysis approach to be used? Begin with a clear and feasible research objective e. g. data at hand (aggregated or time-to-event data) total effect versus primary effect (gap-time versus elapsed-time) A bilateral active consultation/collaboration with a statistician can help a lot! 53
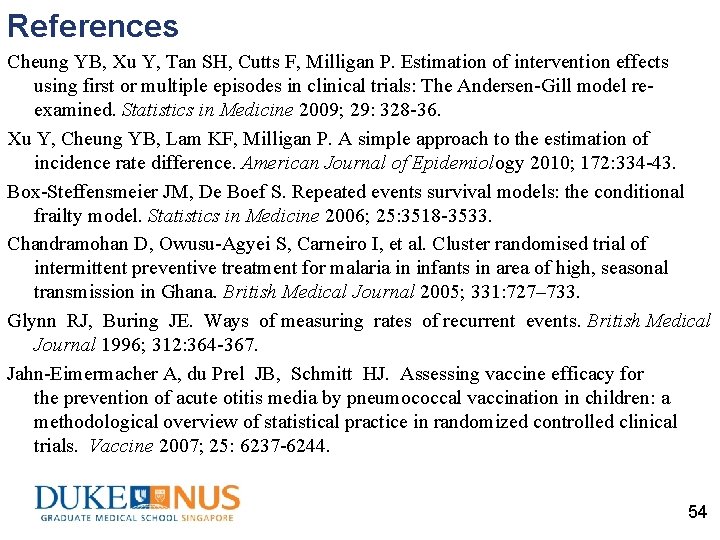
References Cheung YB, Xu Y, Tan SH, Cutts F, Milligan P. Estimation of intervention effects using first or multiple episodes in clinical trials: The Andersen-Gill model reexamined. Statistics in Medicine 2009; 29: 328 -36. Xu Y, Cheung YB, Lam KF, Milligan P. A simple approach to the estimation of incidence rate difference. American Journal of Epidemiology 2010; 172: 334 -43. Box-Steffensmeier JM, De Boef S. Repeated events survival models: the conditional frailty model. Statistics in Medicine 2006; 25: 3518 -3533. Chandramohan D, Owusu-Agyei S, Carneiro I, et al. Cluster randomised trial of intermittent preventive treatment for malaria in infants in area of high, seasonal transmission in Ghana. British Medical Journal 2005; 331: 727– 733. Glynn RJ, Buring JE. Ways of measuring rates of recurrent events. British Medical Journal 1996; 312: 364 -367. Jahn-Eimermacher A, du Prel JB, Schmitt HJ. Assessing vaccine efficacy for the prevention of acute otitis media by pneumococcal vaccination in children: a methodological overview of statistical practice in randomized controlled clinical trials. Vaccine 2007; 25: 6237 -6244. 54
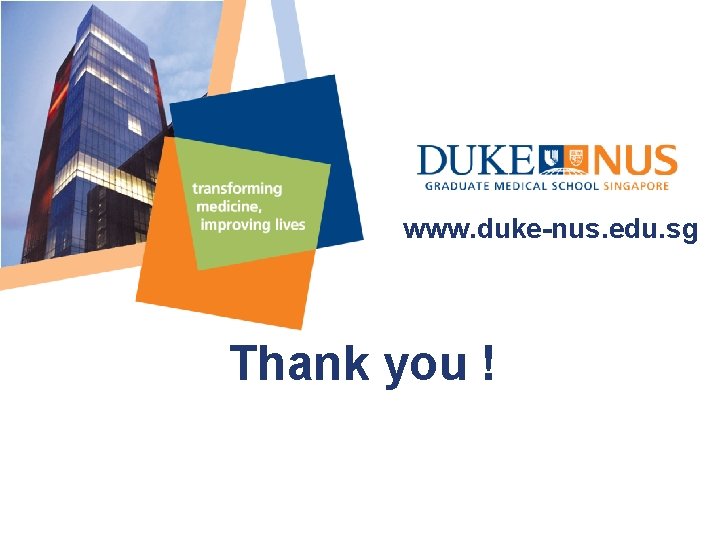
www. duke-nus. edu. sg Thank you !
Mutually exclusive events vs not mutually exclusive events
Vocal cord positions
Visualizing and understanding recurrent networks
Branches of radial artery
Arytenoid cartilage
Lstm andrew ng
Recurrent strokes
Recurrent stroke causes
Webers syndrome
Extensions of recurrent neural network language model
Cs 7643 github
Cs 231 n
A recurrent bert-based model for question generation
Rnn
Recurrent neural network based language model
6 elements of hair design
Coarcotation
A recurrent image in a literary work is the
Part 135 recurrent training
Wegner grossman theory
Epiglottoplexy
Recurrent connection
Disease of external nose
Lateral malleolar artery
Recurrent yolo
Simple recurrent network
Bohr effect and haldane effect
Bottleneck effect
Roy's identity
Cohens effect size
Moment of force
Kumar is producing the photoelectric effect by using
Cause and effect connectives examples
Using adverb clauses to show cause and effect
Types of information systems
Front-end rounding
Mcaces mii
Estimation of degradation function
Gao cost estimating and assessment guide 2020
Estimation with decimals
Tendering and estimating
Falguni aggarwal
Dr nabil issa
Concrete weights and measures
Estimating avogadro's number lab
Electrical wiring estimating and costing
Cost analysis and estimating for engineering and management
Common critical values
12-1 estimating limits graphically
Agile estimating and planning by mike cohn
Determining time of death worksheet
Estimating with percents
Estimating limit values from tables
Estimating limits numerically
Estimate whole numbers
Hurdle principle