DSS for Integrated Water Resources Management IWRM DM
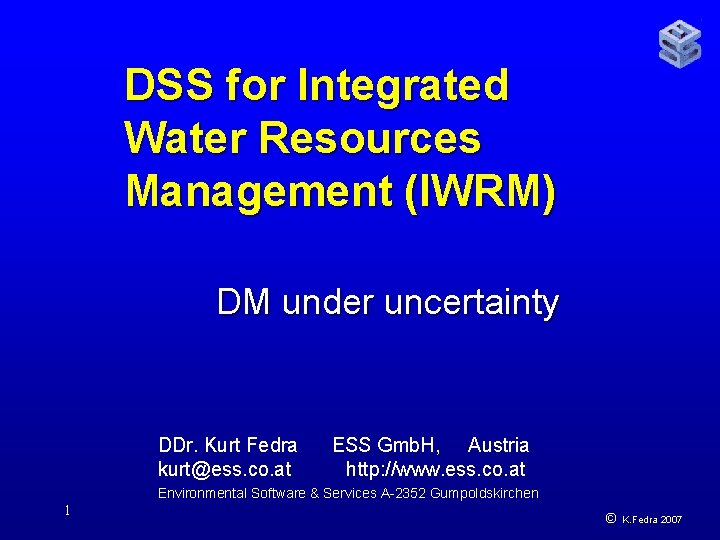
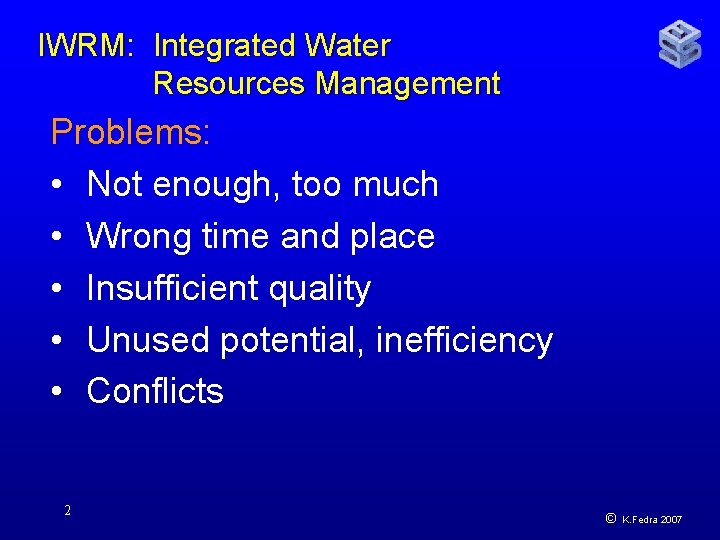
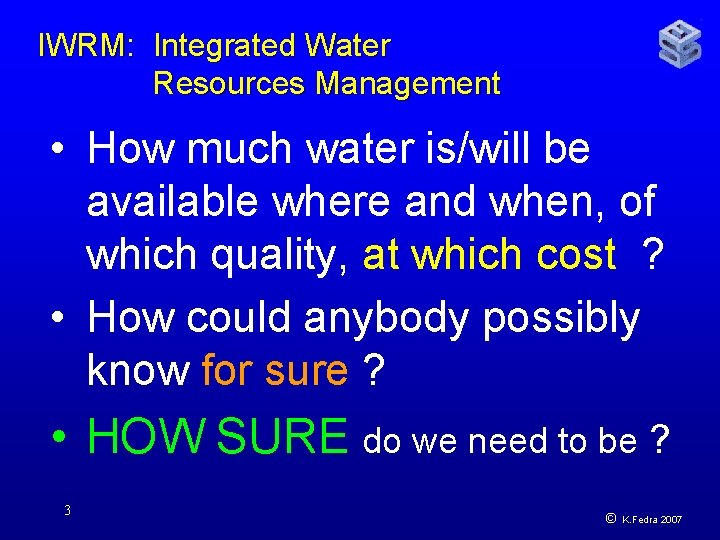
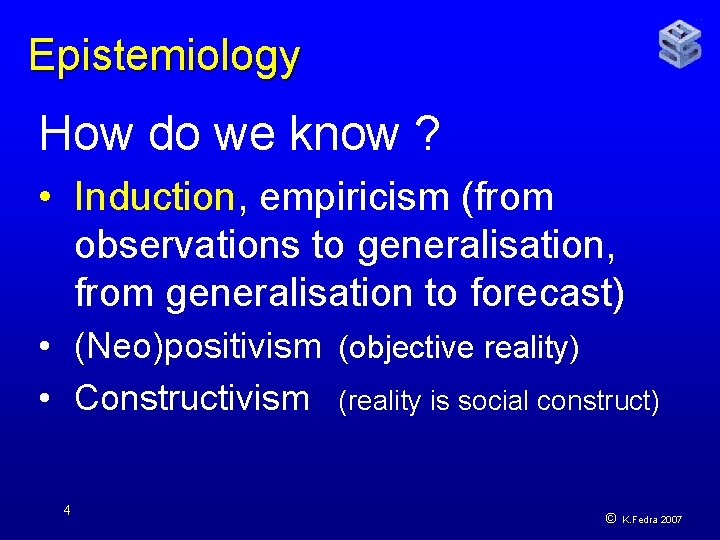
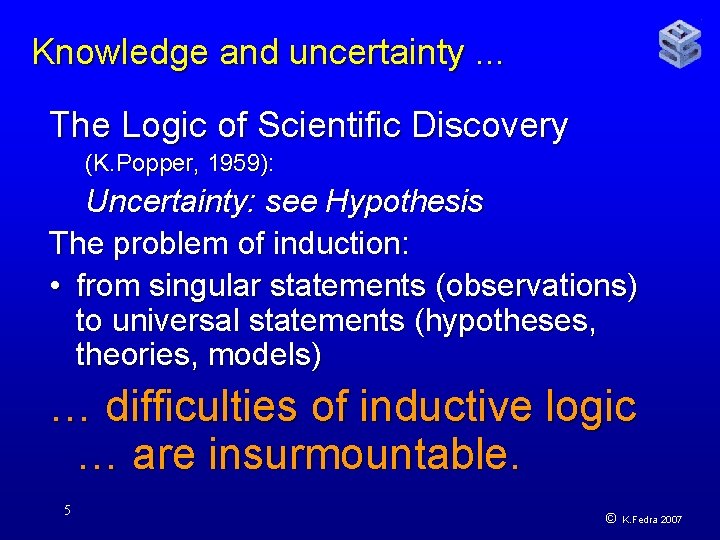
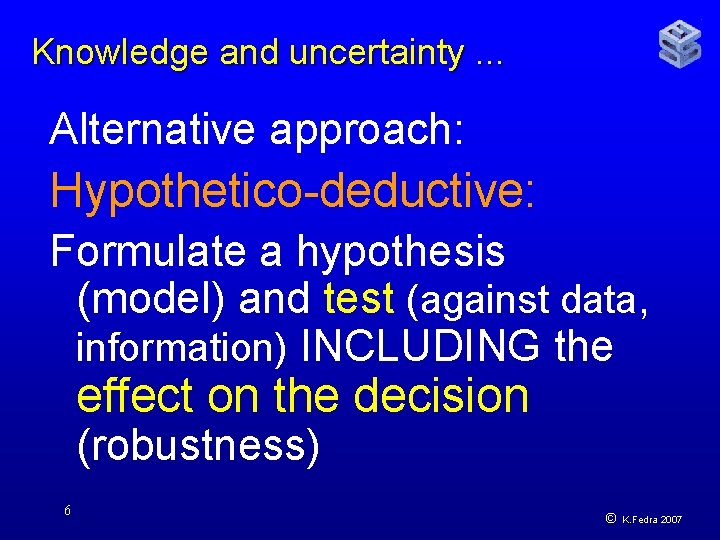
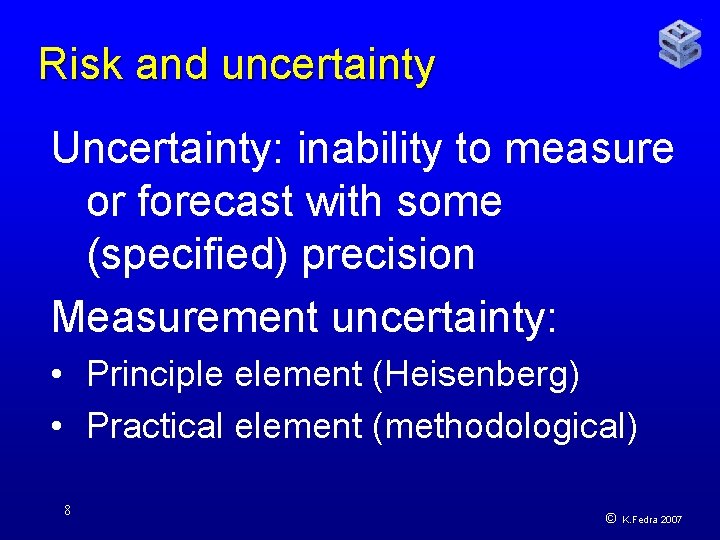
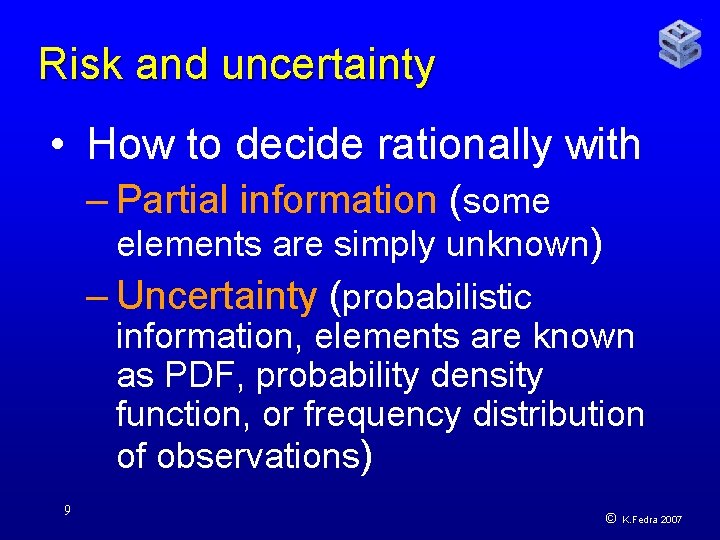
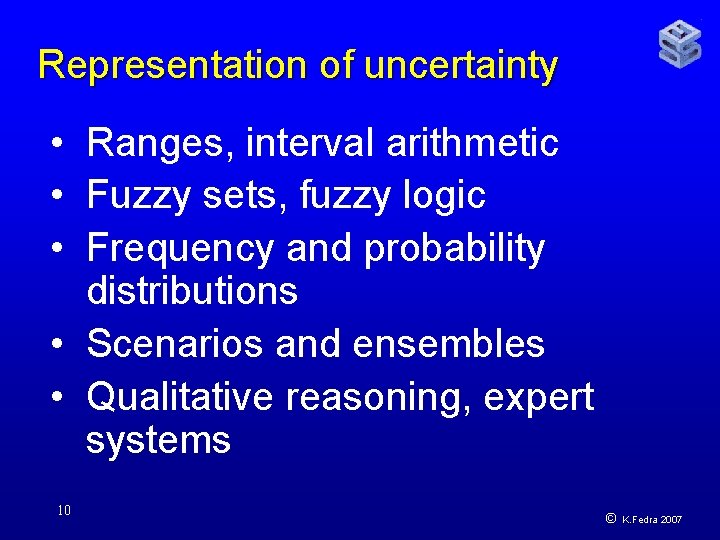
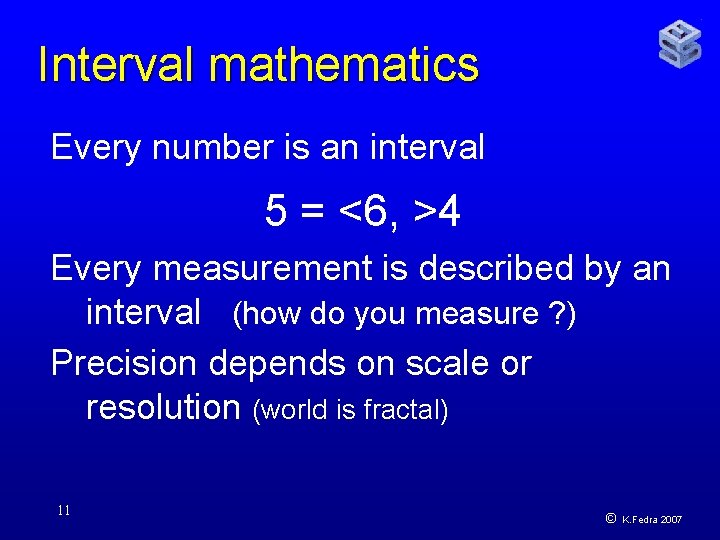
![Interval mathematics [a, b] + [c, d] = [a + c, b + d] Interval mathematics [a, b] + [c, d] = [a + c, b + d]](https://slidetodoc.com/presentation_image_h/c7478d8b5511e29420c47ada713d6fd7/image-11.jpg)
![Interval mathematics Ranges, interval arithmetic X X*Y 13 =5 = 15 = [4, 6] Interval mathematics Ranges, interval arithmetic X X*Y 13 =5 = 15 = [4, 6]](https://slidetodoc.com/presentation_image_h/c7478d8b5511e29420c47ada713d6fd7/image-12.jpg)
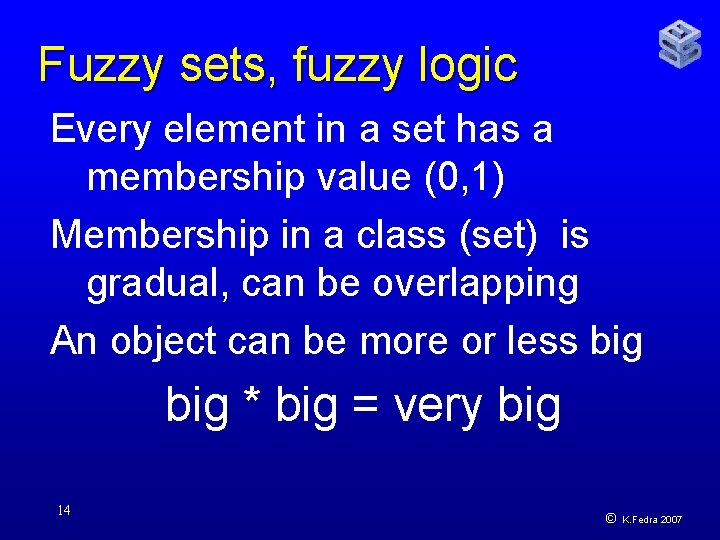
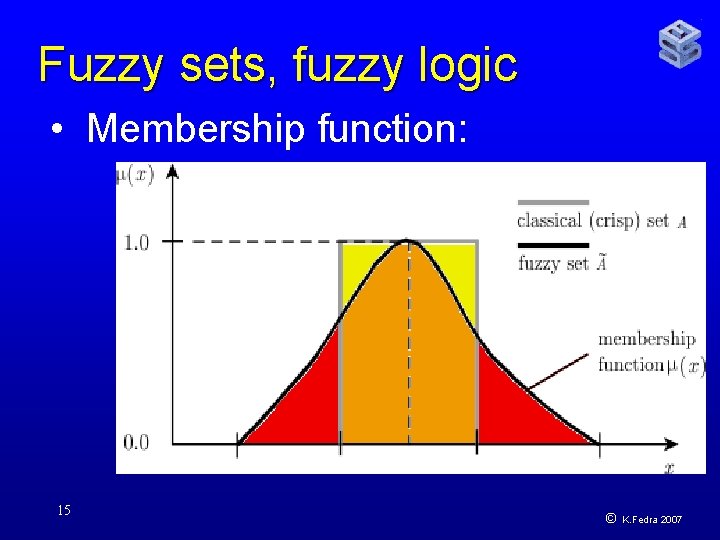
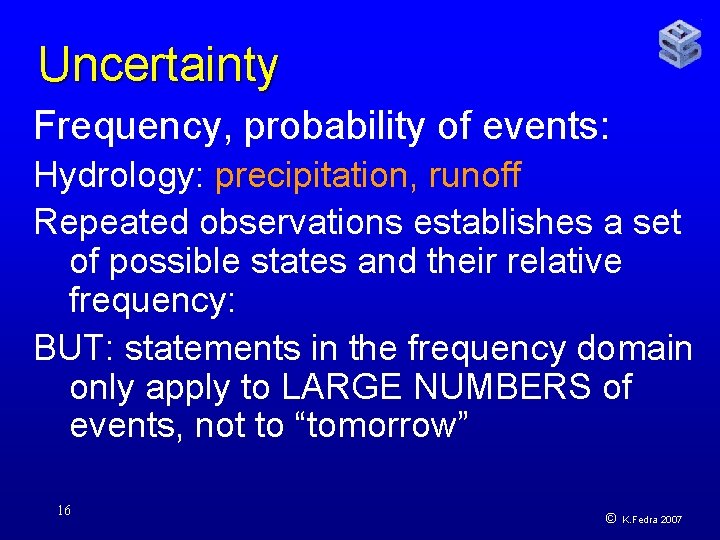
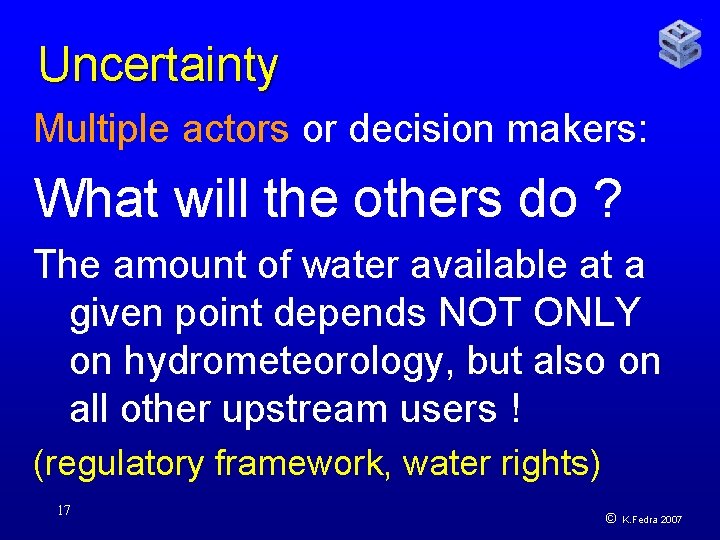
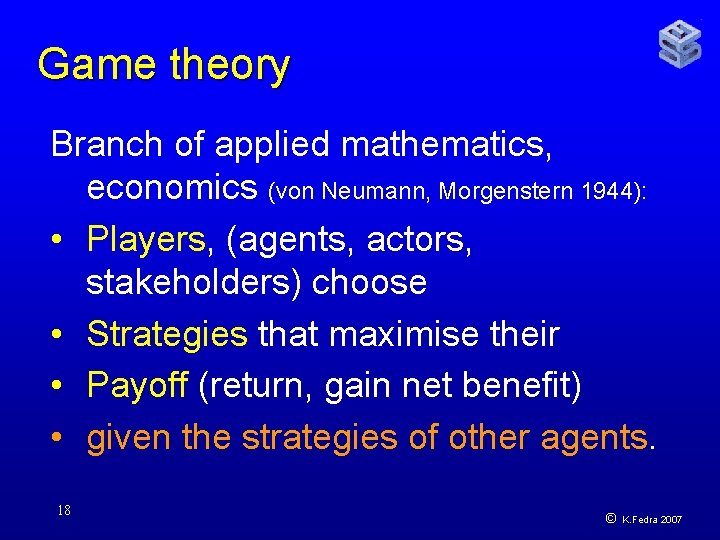
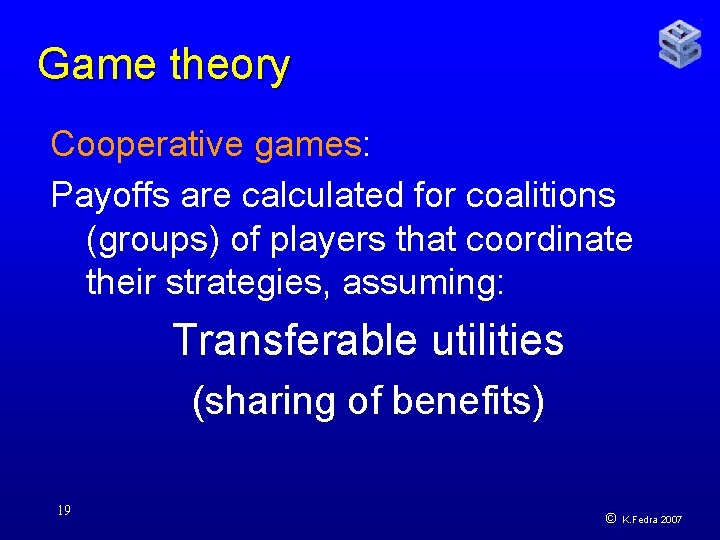
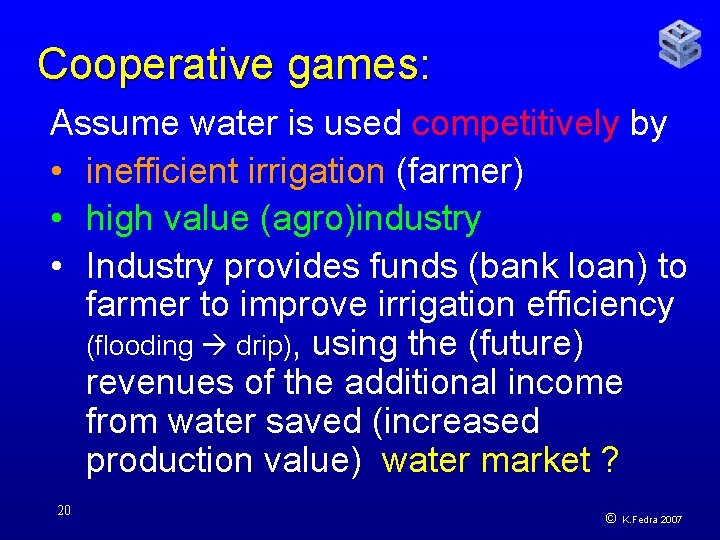
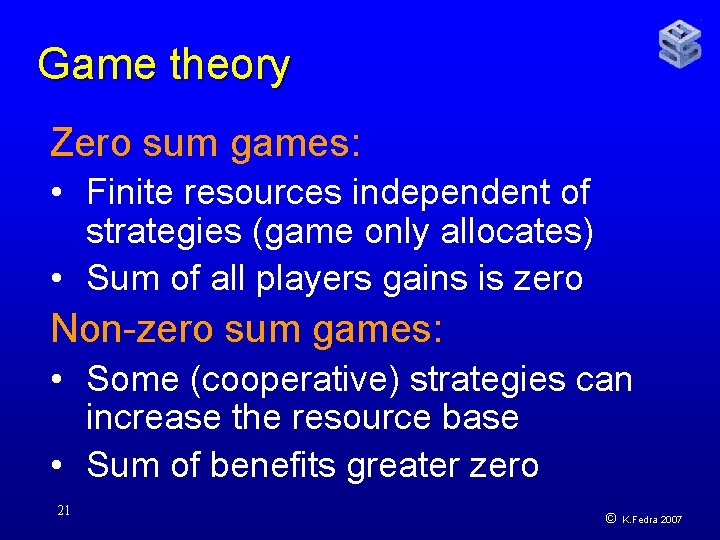
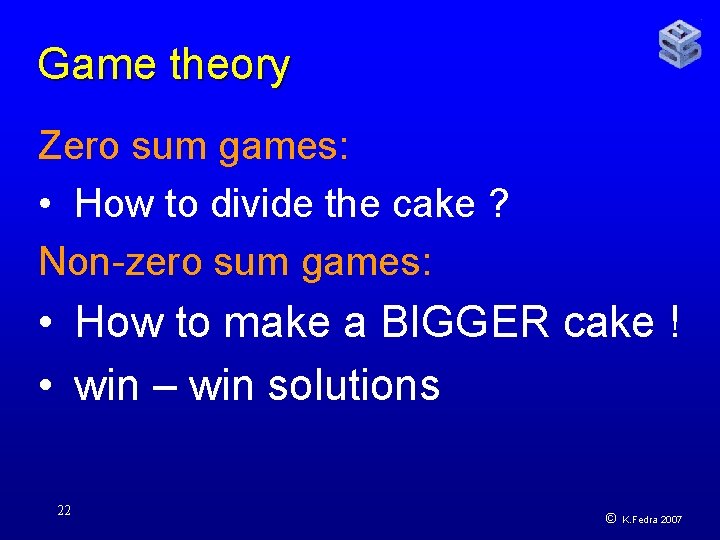
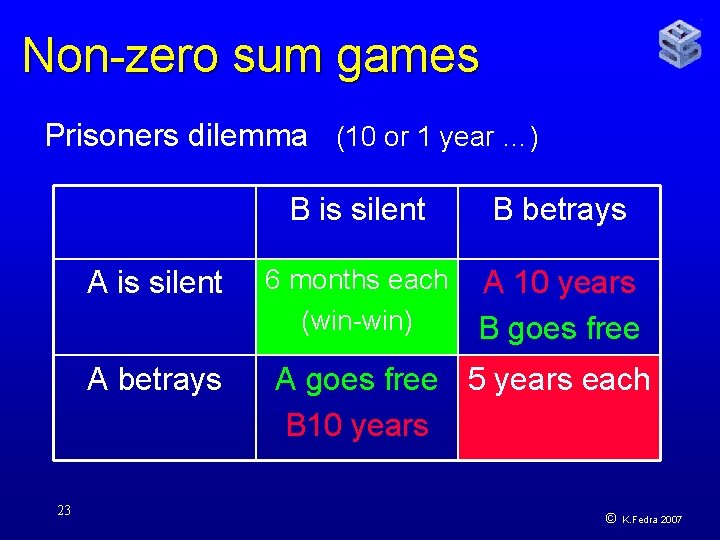
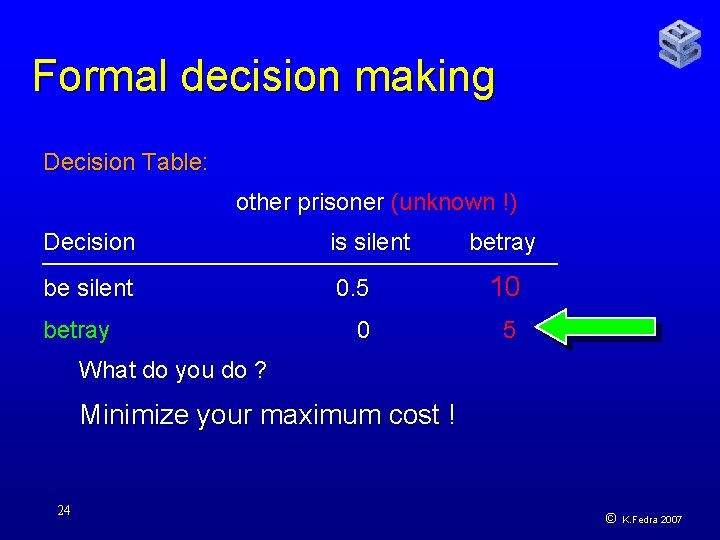
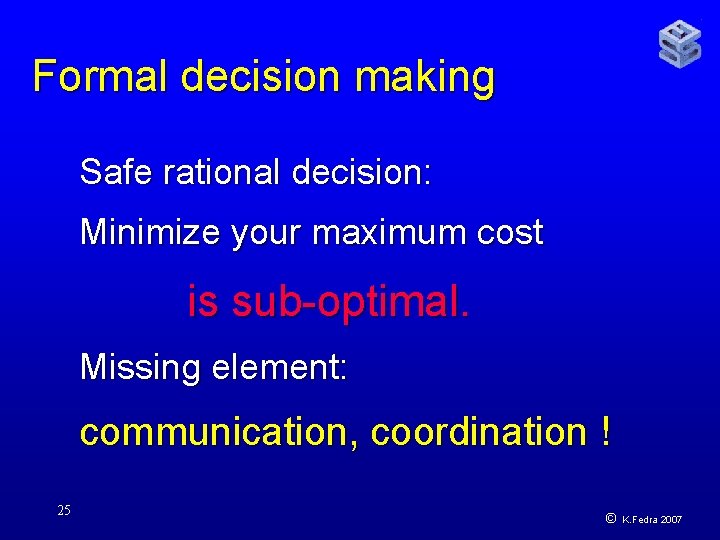
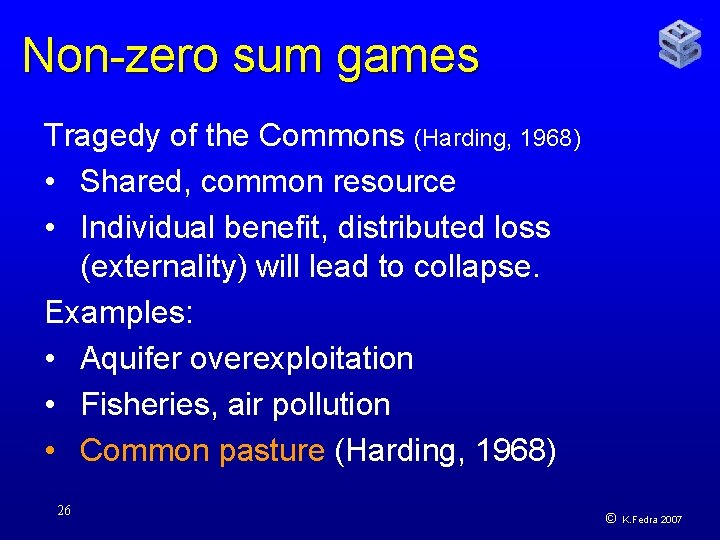
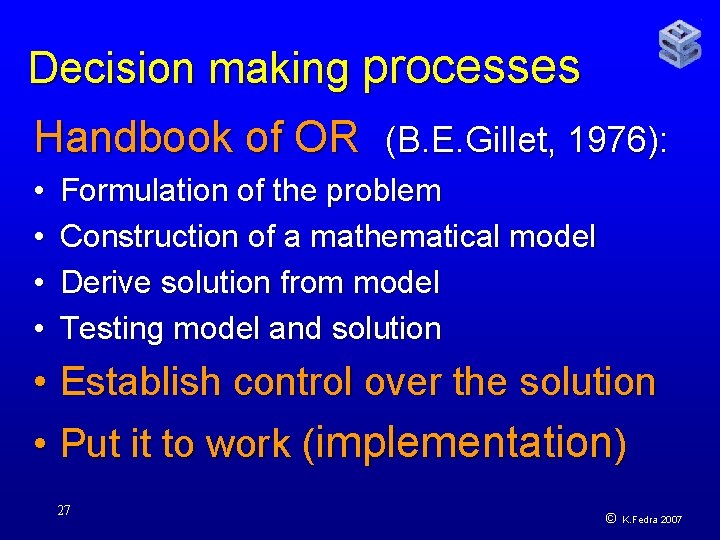
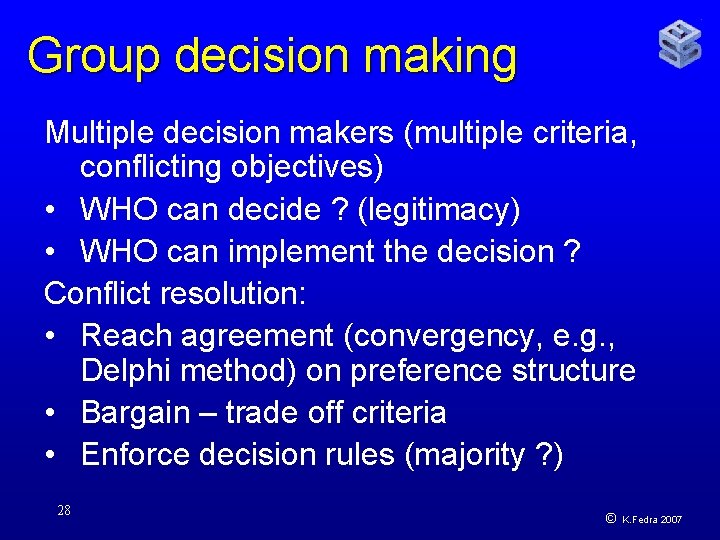
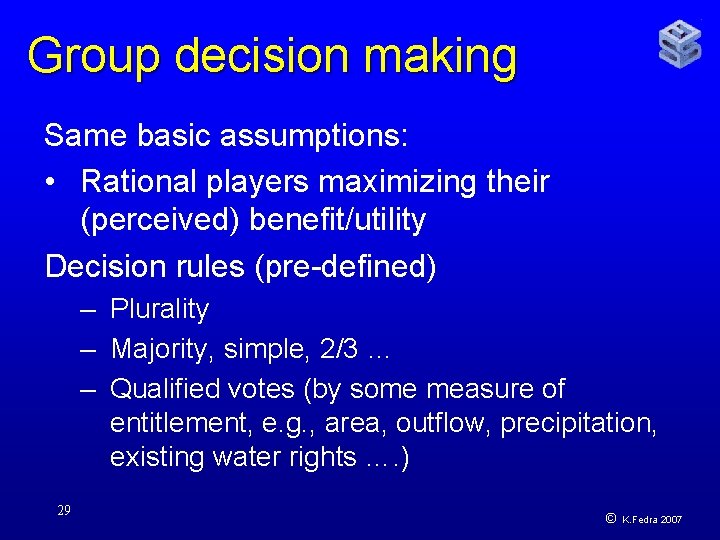
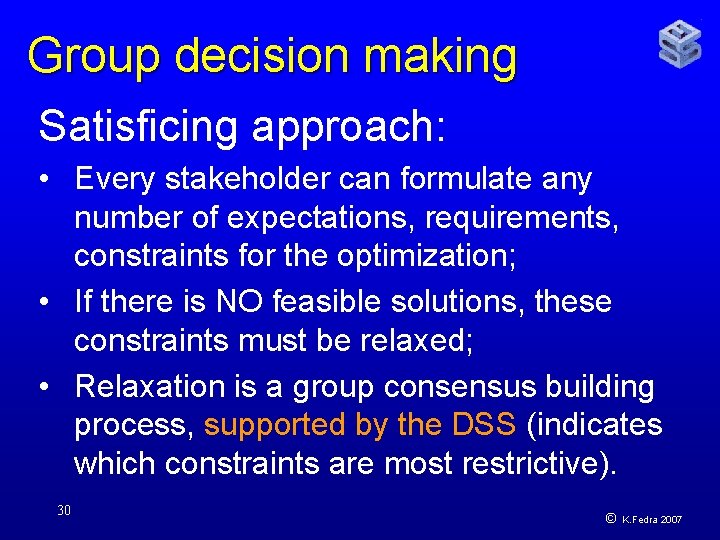
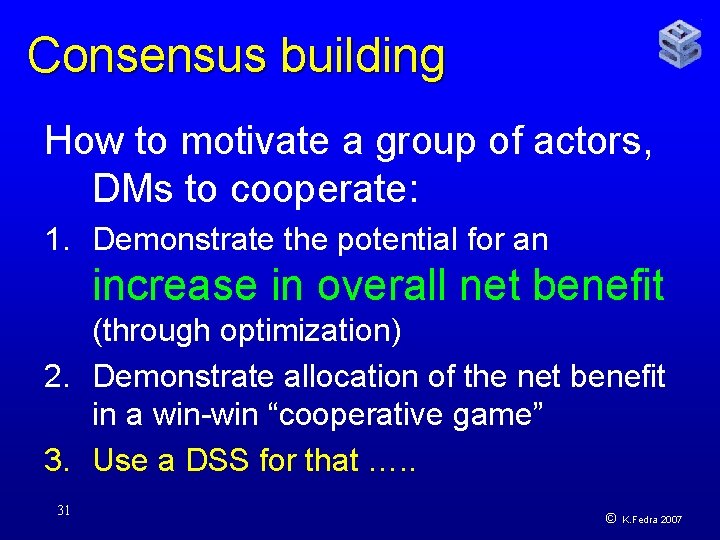
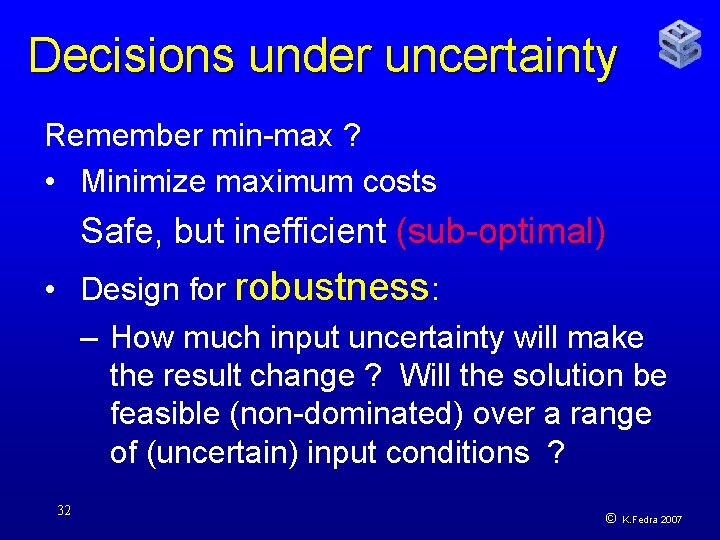
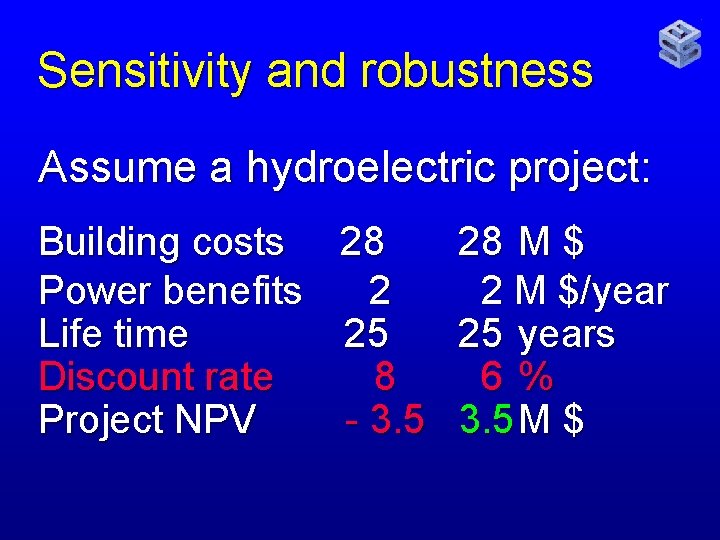
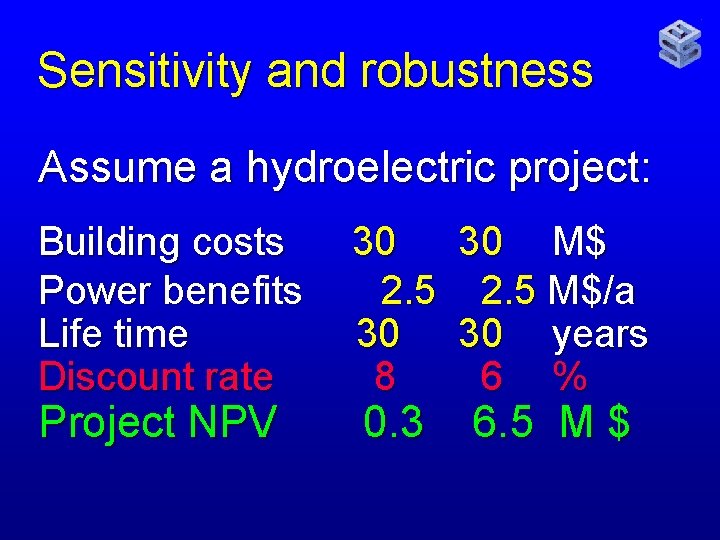
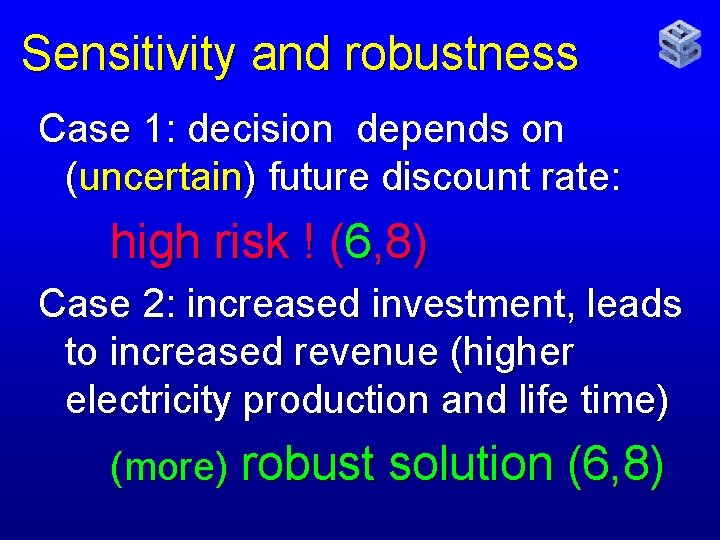
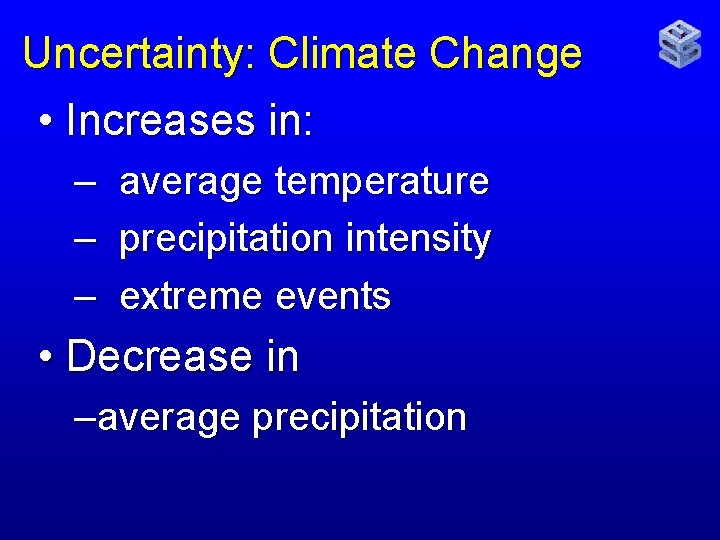
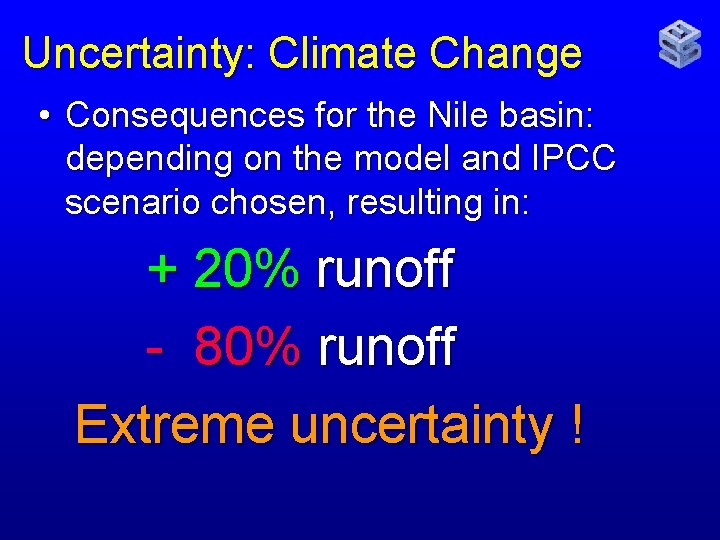
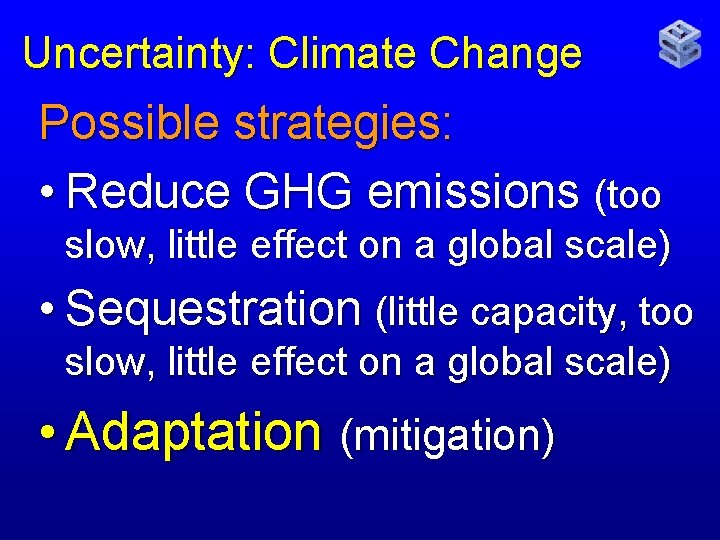
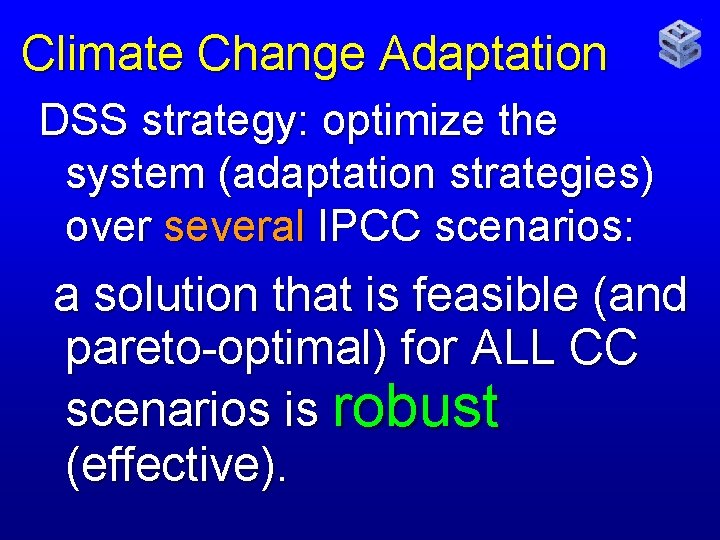
- Slides: 38
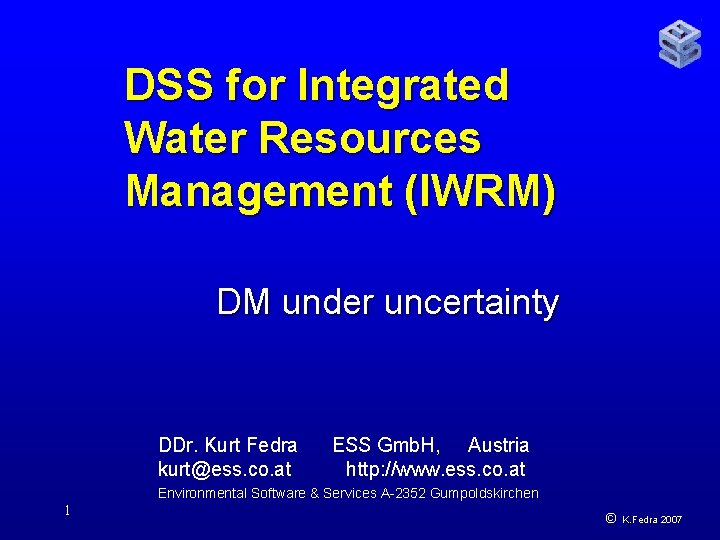
DSS for Integrated Water Resources Management (IWRM) DM under uncertainty DDr. Kurt Fedra kurt@ess. co. at ESS Gmb. H, Austria http: //www. ess. co. at Environmental Software & Services A-2352 Gumpoldskirchen 1 © K. Fedra 2007
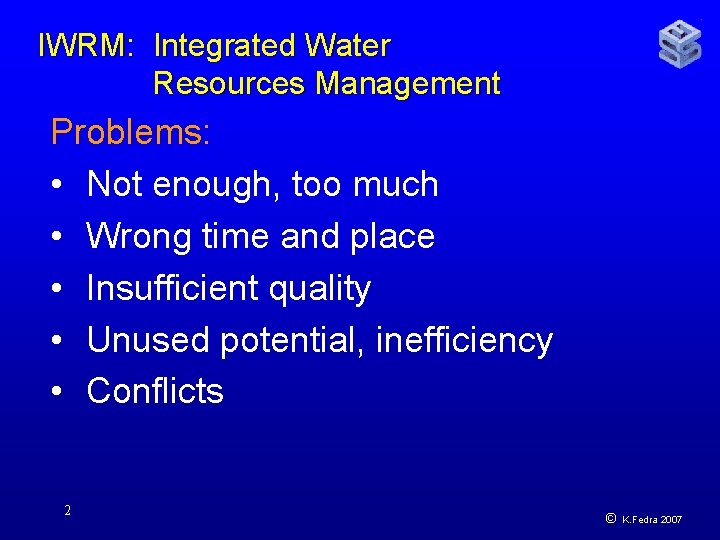
IWRM: Integrated Water Resources Management Problems: • Not enough, too much • Wrong time and place • Insufficient quality • Unused potential, inefficiency • Conflicts 2 © K. Fedra 2007
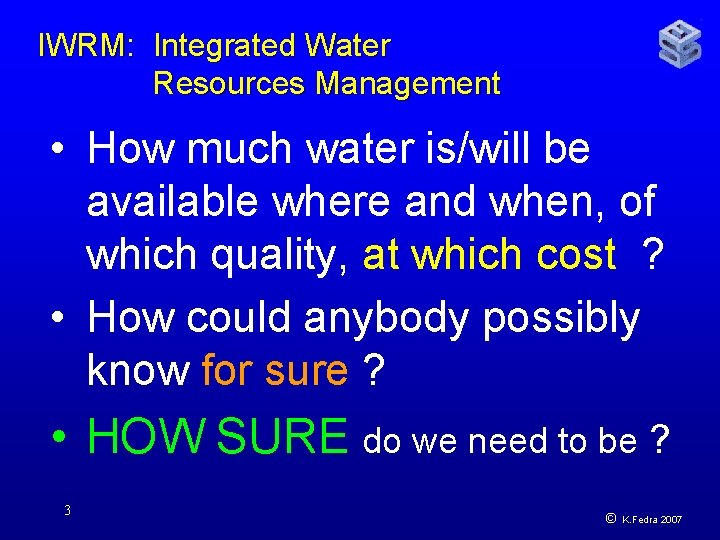
IWRM: Integrated Water Resources Management • How much water is/will be available where and when, of which quality, at which cost ? • How could anybody possibly know for sure ? • HOW SURE do we need to be ? 3 © K. Fedra 2007
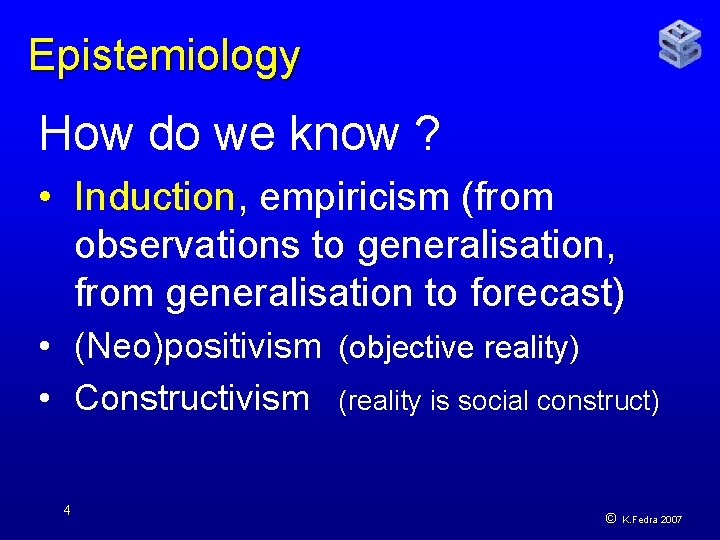
Epistemiology How do we know ? • Induction, empiricism (from observations to generalisation, from generalisation to forecast) • (Neo)positivism (objective reality) • Constructivism (reality is social construct) 4 © K. Fedra 2007
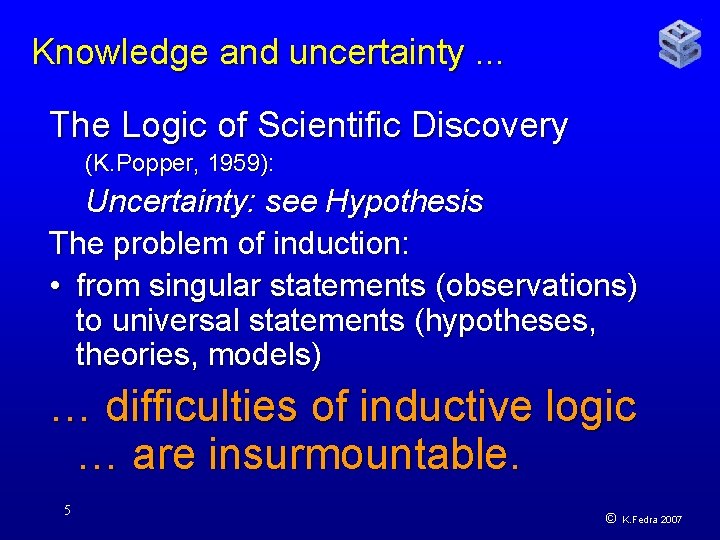
Knowledge and uncertainty. . . The Logic of Scientific Discovery (K. Popper, 1959): Uncertainty: see Hypothesis The problem of induction: • from singular statements (observations) to universal statements (hypotheses, theories, models) … difficulties of inductive logic … are insurmountable. 5 © K. Fedra 2007
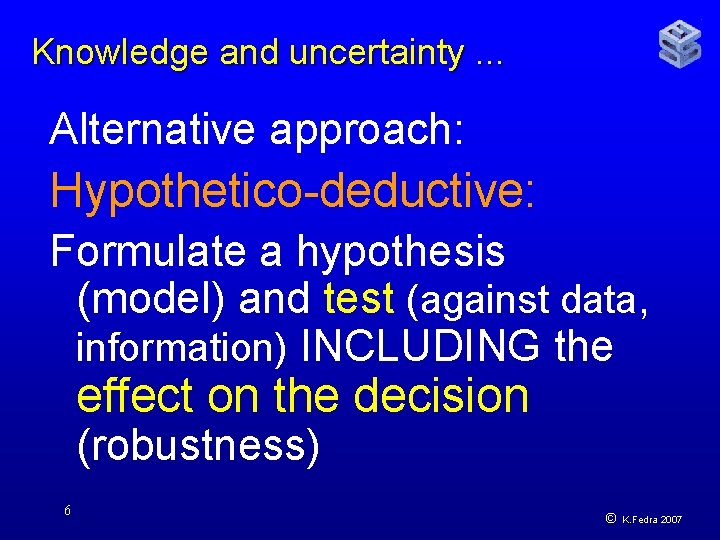
Knowledge and uncertainty. . . Alternative approach: Hypothetico-deductive: Formulate a hypothesis (model) and test (against data, information) INCLUDING the effect on the decision (robustness) 6 © K. Fedra 2007
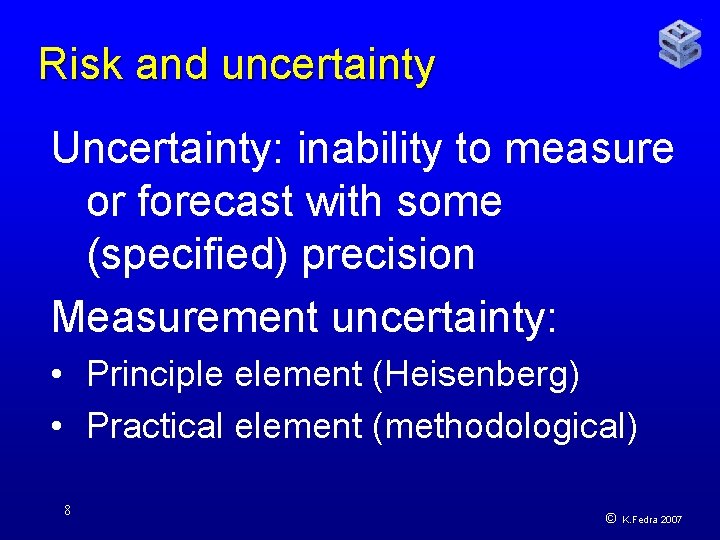
Risk and uncertainty Uncertainty: inability to measure or forecast with some (specified) precision Measurement uncertainty: • Principle element (Heisenberg) • Practical element (methodological) 8 © K. Fedra 2007
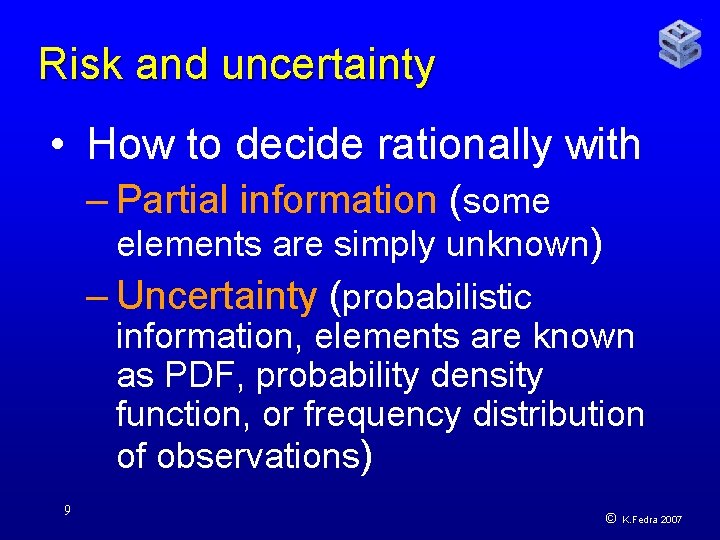
Risk and uncertainty • How to decide rationally with – Partial information (some elements are simply unknown) – Uncertainty (probabilistic information, elements are known as PDF, probability density function, or frequency distribution of observations) 9 © K. Fedra 2007
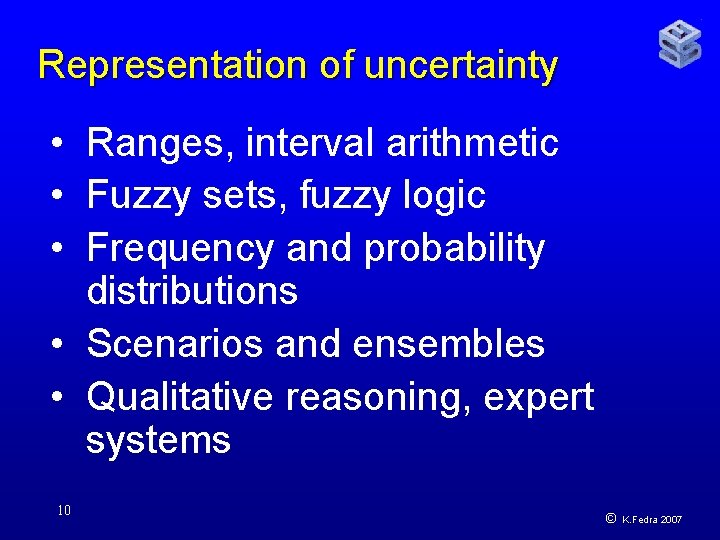
Representation of uncertainty • Ranges, interval arithmetic • Fuzzy sets, fuzzy logic • Frequency and probability distributions • Scenarios and ensembles • Qualitative reasoning, expert systems 10 © K. Fedra 2007
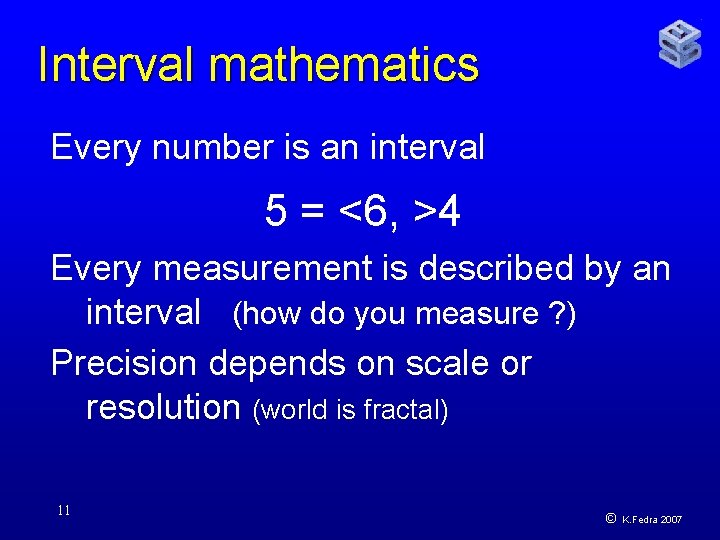
Interval mathematics Every number is an interval 5 = <6, >4 Every measurement is described by an interval (how do you measure ? ) Precision depends on scale or resolution (world is fractal) 11 © K. Fedra 2007
![Interval mathematics a b c d a c b d Interval mathematics [a, b] + [c, d] = [a + c, b + d]](https://slidetodoc.com/presentation_image_h/c7478d8b5511e29420c47ada713d6fd7/image-11.jpg)
Interval mathematics [a, b] + [c, d] = [a + c, b + d] [a, b] − [c, d] = [a − d, b −c] [a, b] × [c, d] = [min (ac, ad, bc, bd), max (ac, ad, bc, bd)] [a, b] / [c, d] = [min (a/c, a/d, b/c, b/d), max (a/c, a/d, b/c, b/d)] 12 © K. Fedra 2007
![Interval mathematics Ranges interval arithmetic X XY 13 5 15 4 6 Interval mathematics Ranges, interval arithmetic X X*Y 13 =5 = 15 = [4, 6]](https://slidetodoc.com/presentation_image_h/c7478d8b5511e29420c47ada713d6fd7/image-12.jpg)
Interval mathematics Ranges, interval arithmetic X X*Y 13 =5 = 15 = [4, 6] = [8, 24] Y=3 Y = [2, 4] © K. Fedra 2007
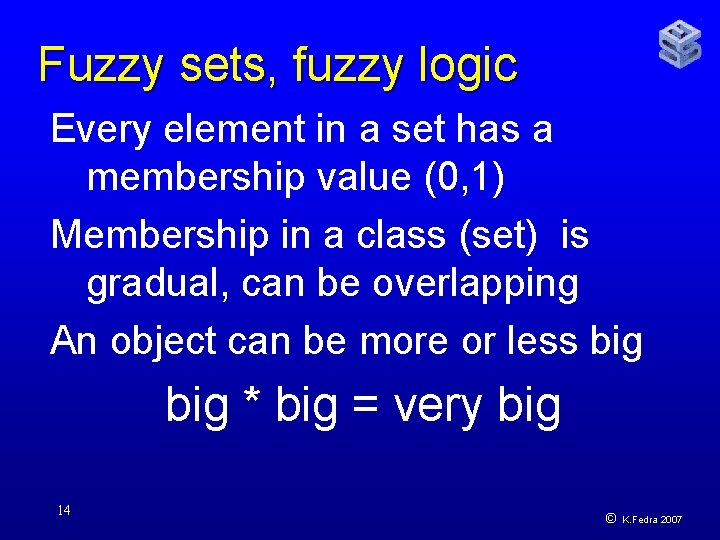
Fuzzy sets, fuzzy logic Every element in a set has a membership value (0, 1) Membership in a class (set) is gradual, can be overlapping An object can be more or less big * big = very big 14 © K. Fedra 2007
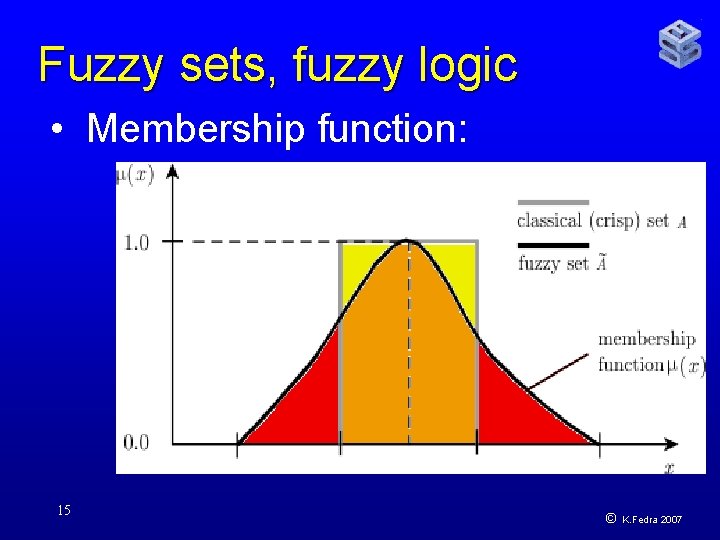
Fuzzy sets, fuzzy logic • Membership function: 15 © K. Fedra 2007
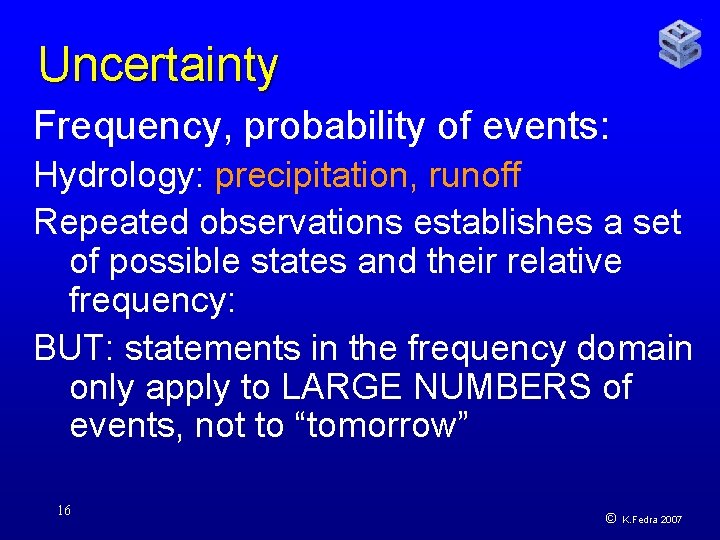
Uncertainty Frequency, probability of events: Hydrology: precipitation, runoff Repeated observations establishes a set of possible states and their relative frequency: BUT: statements in the frequency domain only apply to LARGE NUMBERS of events, not to “tomorrow” 16 © K. Fedra 2007
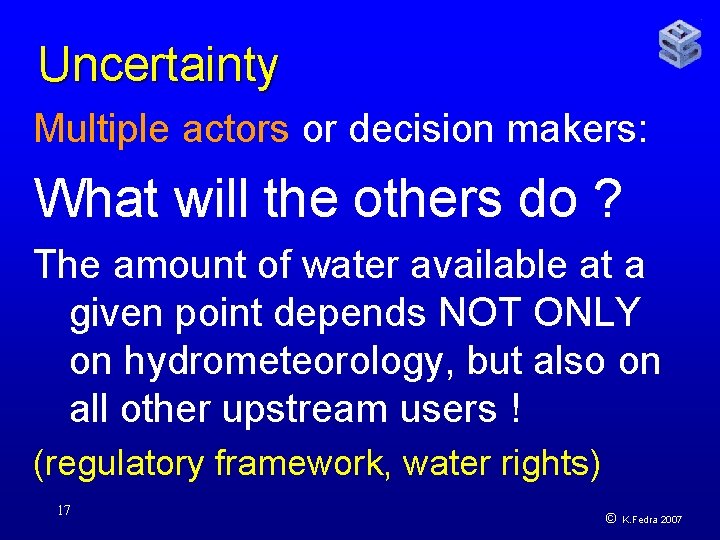
Uncertainty Multiple actors or decision makers: What will the others do ? The amount of water available at a given point depends NOT ONLY on hydrometeorology, but also on all other upstream users ! (regulatory framework, water rights) 17 © K. Fedra 2007
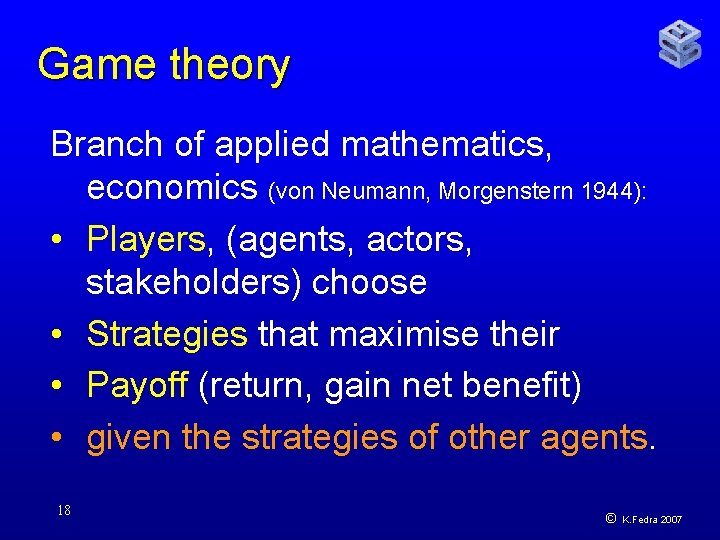
Game theory Branch of applied mathematics, economics (von Neumann, Morgenstern 1944): • Players, (agents, actors, stakeholders) choose • Strategies that maximise their • Payoff (return, gain net benefit) • given the strategies of other agents. 18 © K. Fedra 2007
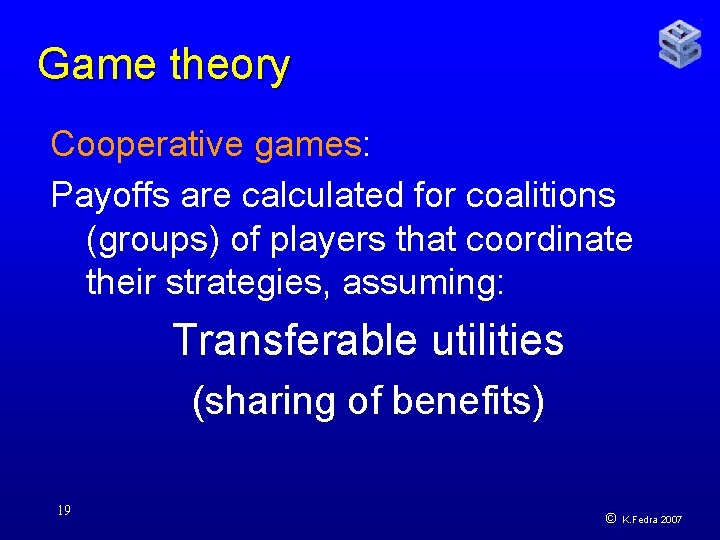
Game theory Cooperative games: Payoffs are calculated for coalitions (groups) of players that coordinate their strategies, assuming: Transferable utilities (sharing of benefits) 19 © K. Fedra 2007
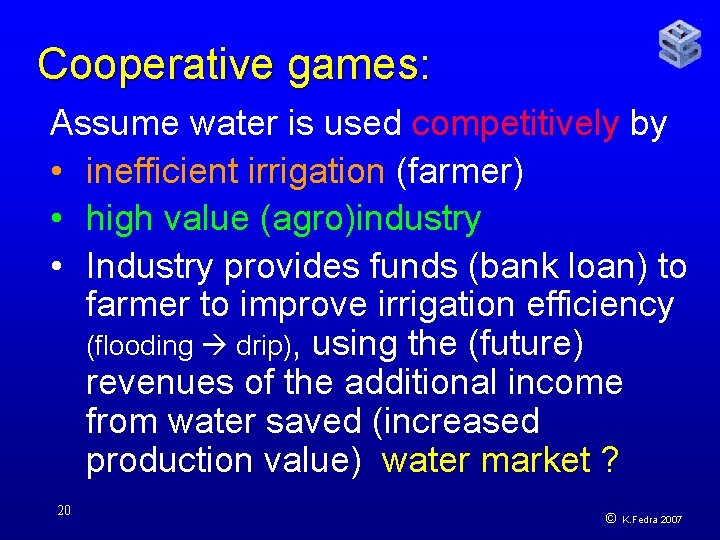
Cooperative games: Assume water is used competitively by • inefficient irrigation (farmer) • high value (agro)industry • Industry provides funds (bank loan) to farmer to improve irrigation efficiency (flooding drip), using the (future) revenues of the additional income from water saved (increased production value) water market ? 20 © K. Fedra 2007
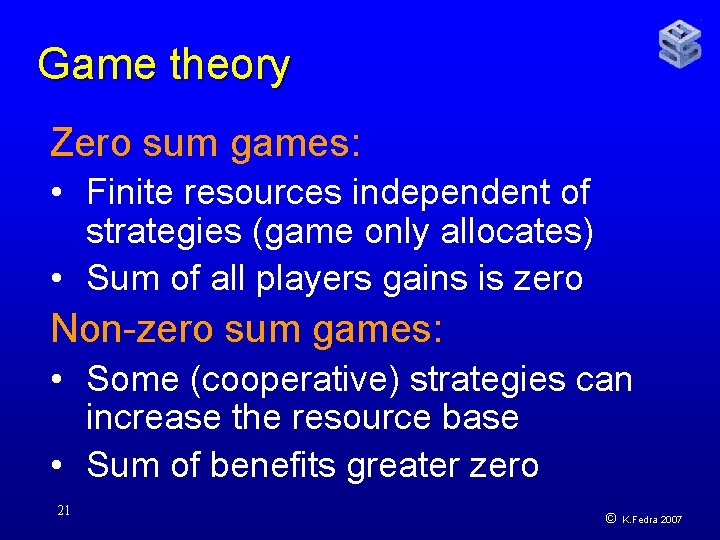
Game theory Zero sum games: • Finite resources independent of strategies (game only allocates) • Sum of all players gains is zero Non-zero sum games: • Some (cooperative) strategies can increase the resource base • Sum of benefits greater zero 21 © K. Fedra 2007
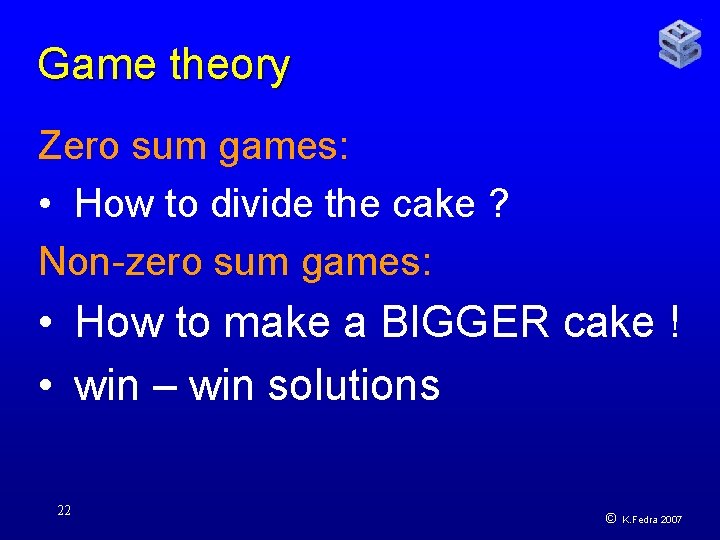
Game theory Zero sum games: • How to divide the cake ? Non-zero sum games: • How to make a BIGGER cake ! • win – win solutions 22 © K. Fedra 2007
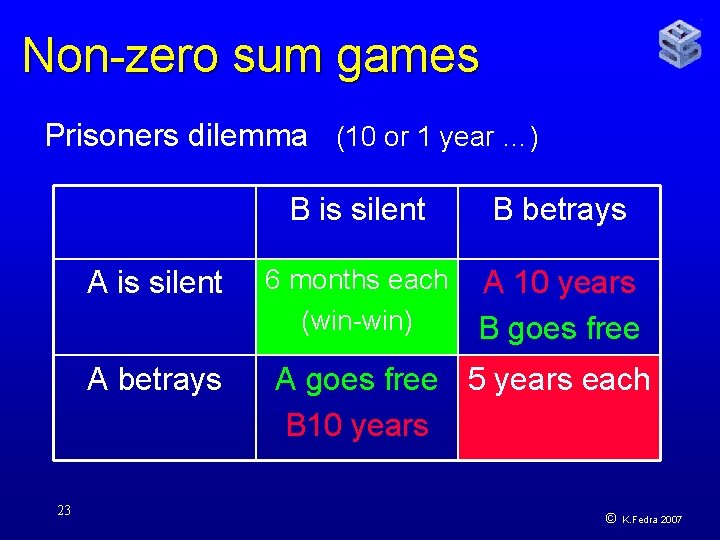
Non-zero sum games Prisoners dilemma (10 or 1 year …) A is silent A betrays 23 B is silent B betrays 6 months each (win-win) A 10 years B goes free A goes free 5 years each B 10 years © K. Fedra 2007
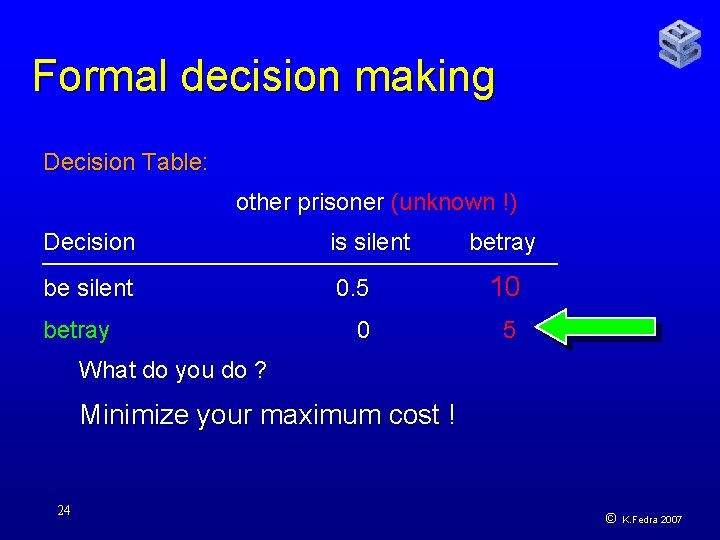
Formal decision making Decision Table: other prisoner (unknown !) Decision is silent be silent 0. 5 10 0 5 betray What do you do ? Minimize your maximum cost ! 24 © K. Fedra 2007
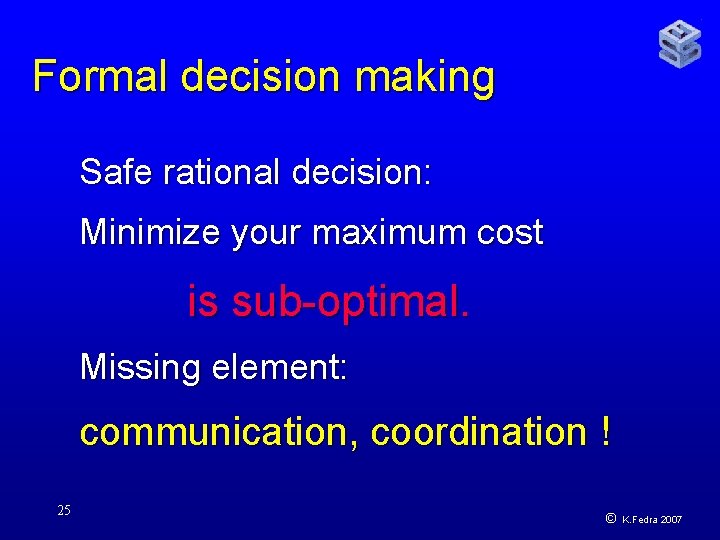
Formal decision making Safe rational decision: Minimize your maximum cost is sub-optimal. Missing element: communication, coordination ! 25 © K. Fedra 2007
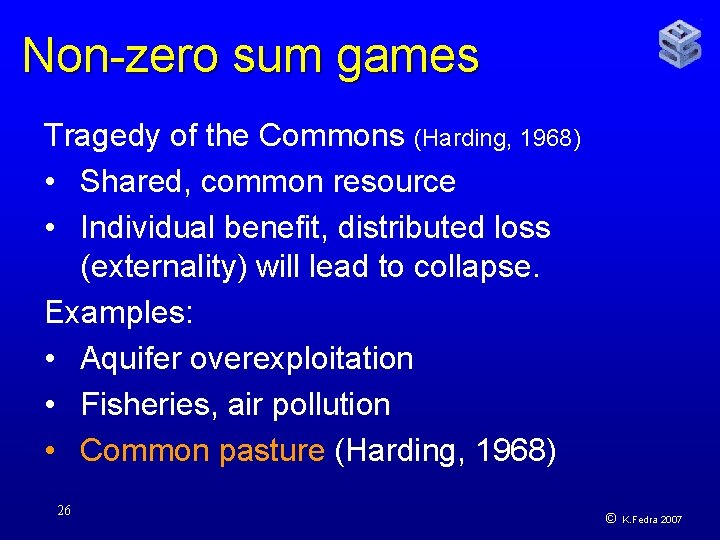
Non-zero sum games Tragedy of the Commons (Harding, 1968) • Shared, common resource • Individual benefit, distributed loss (externality) will lead to collapse. Examples: • Aquifer overexploitation • Fisheries, air pollution • Common pasture (Harding, 1968) 26 © K. Fedra 2007
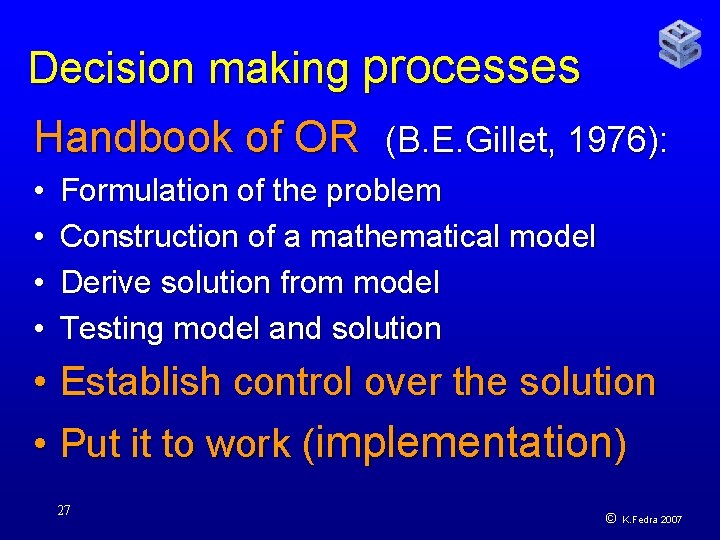
Decision making processes Handbook of OR (B. E. Gillet, 1976): • • Formulation of the problem Construction of a mathematical model Derive solution from model Testing model and solution • Establish control over the solution • Put it to work (implementation) 27 © K. Fedra 2007
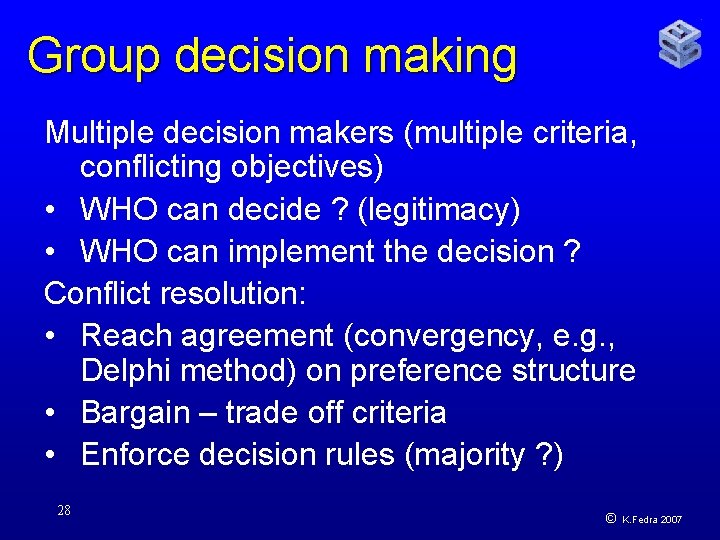
Group decision making Multiple decision makers (multiple criteria, conflicting objectives) • WHO can decide ? (legitimacy) • WHO can implement the decision ? Conflict resolution: • Reach agreement (convergency, e. g. , Delphi method) on preference structure • Bargain – trade off criteria • Enforce decision rules (majority ? ) 28 © K. Fedra 2007
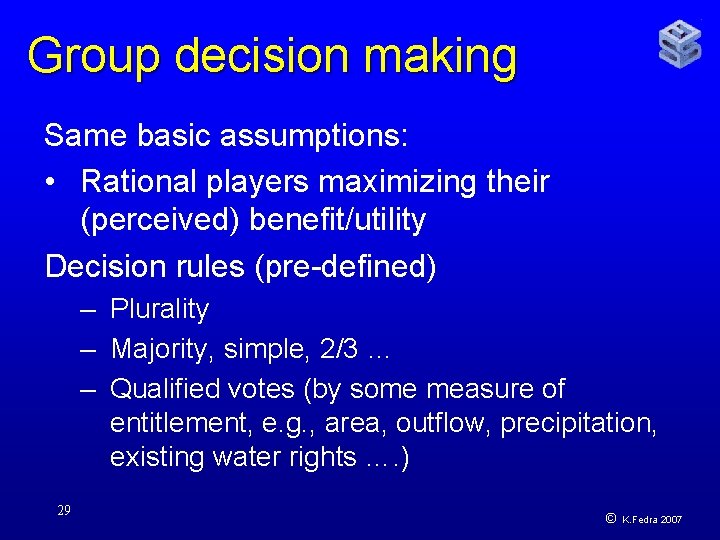
Group decision making Same basic assumptions: • Rational players maximizing their (perceived) benefit/utility Decision rules (pre-defined) – Plurality – Majority, simple, 2/3 … – Qualified votes (by some measure of entitlement, e. g. , area, outflow, precipitation, existing water rights …. ) 29 © K. Fedra 2007
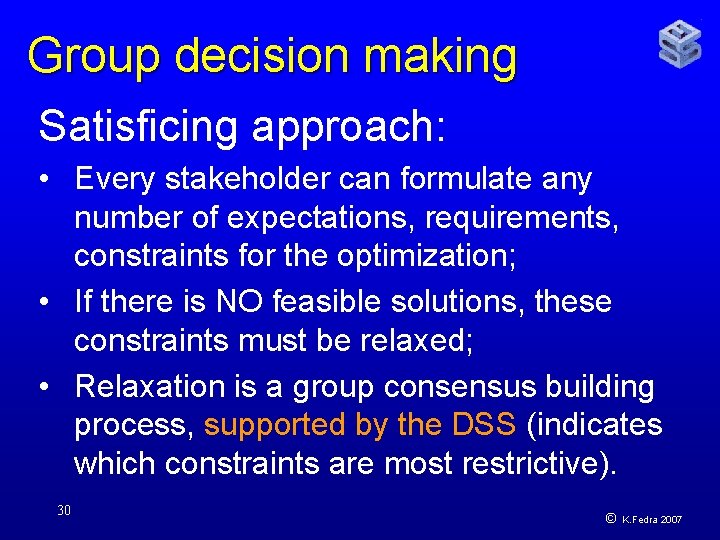
Group decision making Satisficing approach: • Every stakeholder can formulate any number of expectations, requirements, constraints for the optimization; • If there is NO feasible solutions, these constraints must be relaxed; • Relaxation is a group consensus building process, supported by the DSS (indicates which constraints are most restrictive). 30 © K. Fedra 2007
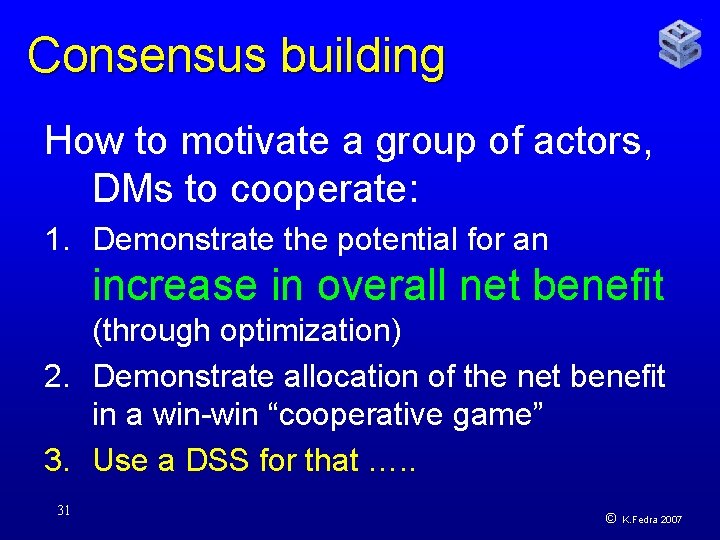
Consensus building How to motivate a group of actors, DMs to cooperate: 1. Demonstrate the potential for an increase in overall net benefit (through optimization) 2. Demonstrate allocation of the net benefit in a win-win “cooperative game” 3. Use a DSS for that …. . 31 © K. Fedra 2007
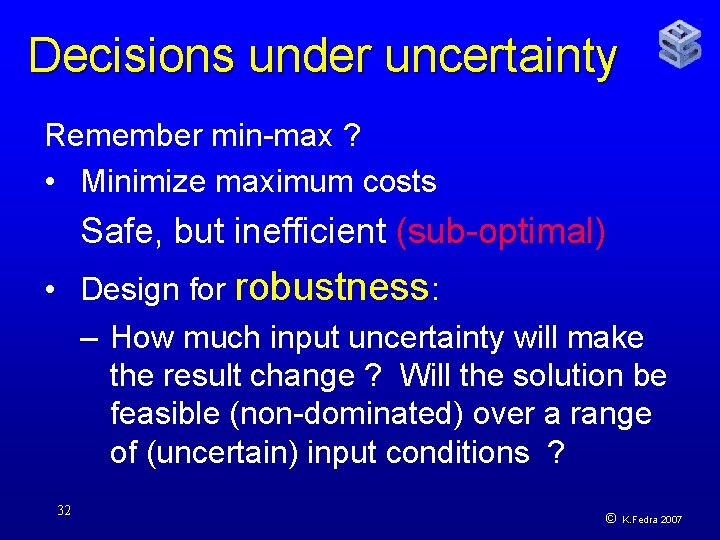
Decisions under uncertainty Remember min-max ? • Minimize maximum costs Safe, but inefficient (sub-optimal) • Design for robustness: – How much input uncertainty will make the result change ? Will the solution be feasible (non-dominated) over a range of (uncertain) input conditions ? 32 © K. Fedra 2007
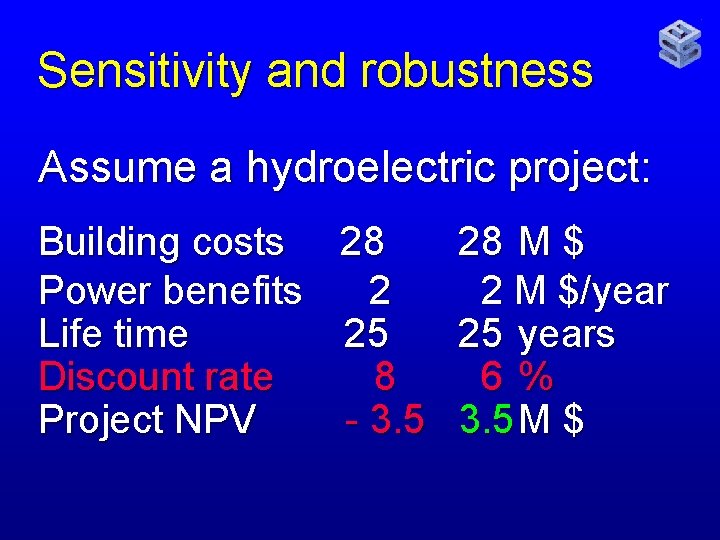
Sensitivity and robustness Assume a hydroelectric project: Building costs 28 Power benefits 2 Life time 25 Discount rate 8 Project NPV - 3. 5 28 M $ 2 M $/year 25 years 6% 3. 5 M $
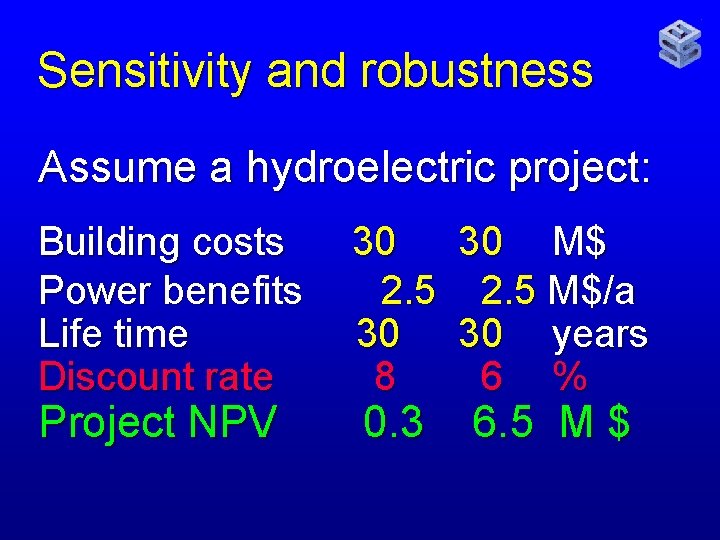
Sensitivity and robustness Assume a hydroelectric project: Building costs Power benefits Life time Discount rate Project NPV 30 2. 5 30 8 0. 3 30 M$ 2. 5 M$/a 30 years 6 % 6. 5 M $
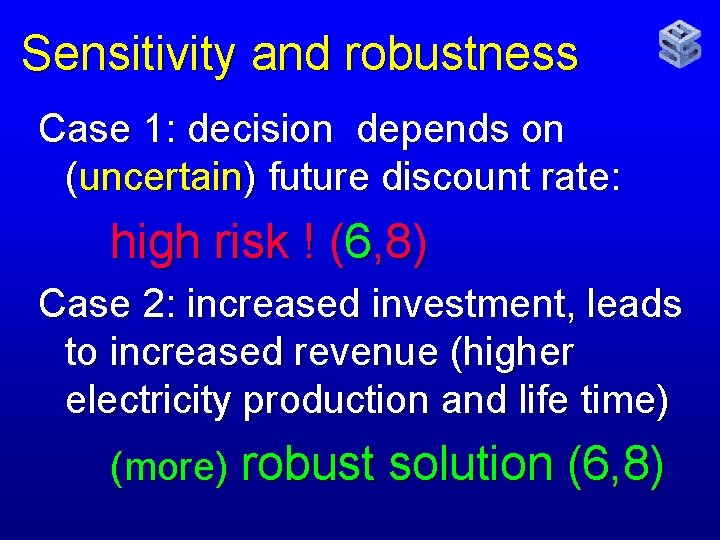
Sensitivity and robustness Case 1: decision depends on (uncertain) future discount rate: high risk ! (6, 8) Case 2: increased investment, leads to increased revenue (higher electricity production and life time) (more) robust solution (6, 8)
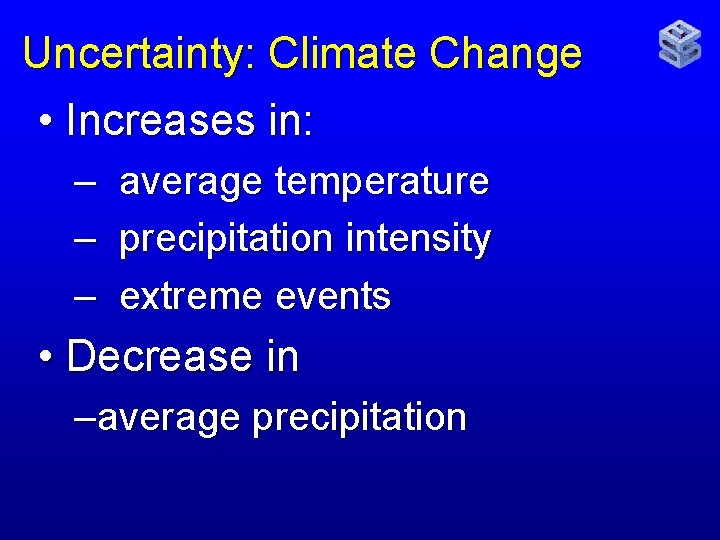
Uncertainty: Climate Change • Increases in: – – – average temperature precipitation intensity extreme events • Decrease in –average precipitation
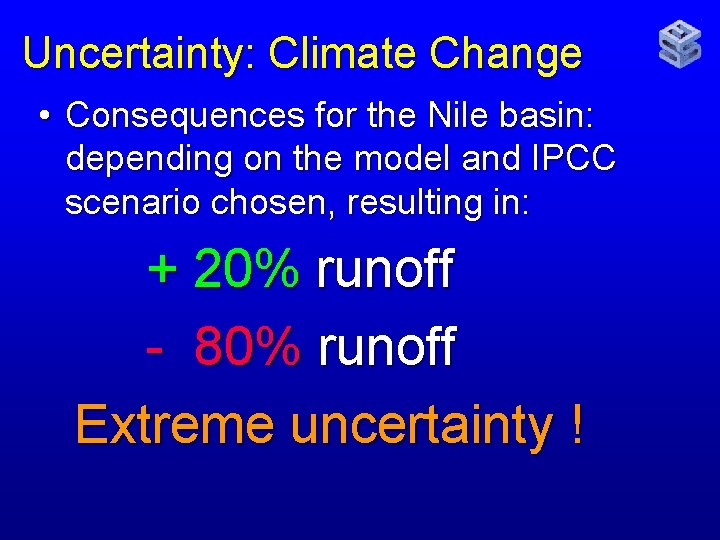
Uncertainty: Climate Change • Consequences for the Nile basin: depending on the model and IPCC scenario chosen, resulting in: + 20% runoff - 80% runoff Extreme uncertainty !
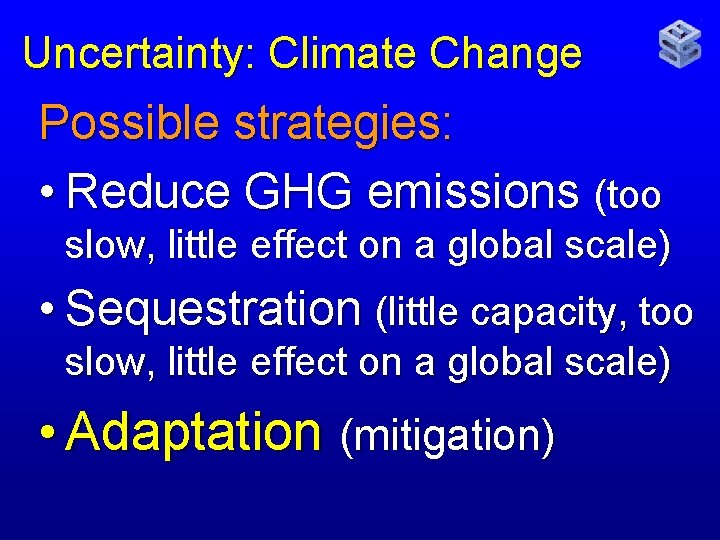
Uncertainty: Climate Change Possible strategies: • Reduce GHG emissions (too slow, little effect on a global scale) • Sequestration (little capacity, too slow, little effect on a global scale) • Adaptation (mitigation)
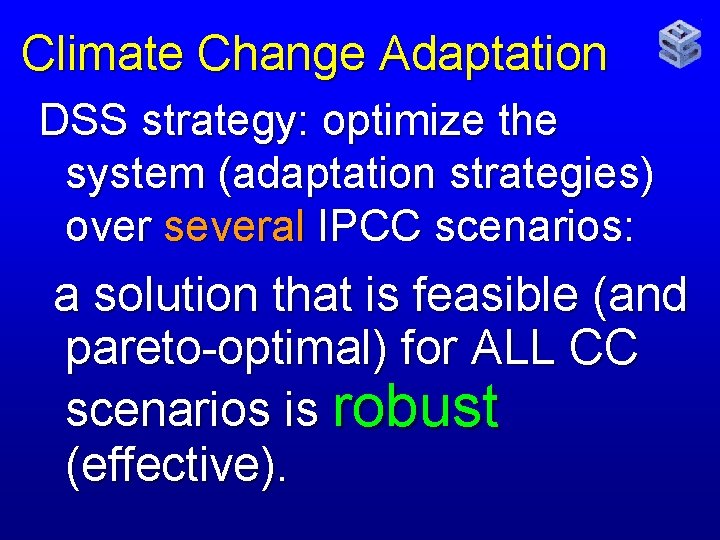
Climate Change Adaptation DSS strategy: optimize the system (adaptation strategies) over several IPCC scenarios: a solution that is feasible (and pareto-optimal) for ALL CC scenarios is robust (effective).
Water and water and water water
Iwrm toolbox
Iwrm adalah
What is iwrm
Dss risk management framework
"dss management"
National water informatics centre
Example of mis and dss
"dss management"
Data management subsystem in dss
Characteristics of a decision support system
Transformed resources and transforming resources
Fixed resources definition
Renewable vs nonrenewable resources worksheet
Fspos vägledning för kontinuitetshantering
Novell typiska drag
Tack för att ni lyssnade bild
Returpilarna
Varför kallas perioden 1918-1939 för mellankrigstiden?
En lathund för arbete med kontinuitetshantering
Kassaregister ideell förening
Tidbok för yrkesförare
Anatomi organ reproduksi
Densitet vatten
Datorkunskap för nybörjare
Stig kerman
Hur skriver man en debattartikel
Autokratiskt ledarskap
Nyckelkompetenser för livslångt lärande
Påbyggnader för flakfordon
Formel för lufttryck
Publik sektor
Jag har nigit för nymånens skära
Presentera för publik crossboss
Jiddisch
Bat mitza
Klassificeringsstruktur för kommunala verksamheter
Epiteltyper
Claes martinsson