Data collection and analysis Jrn Vatn NTNU 1
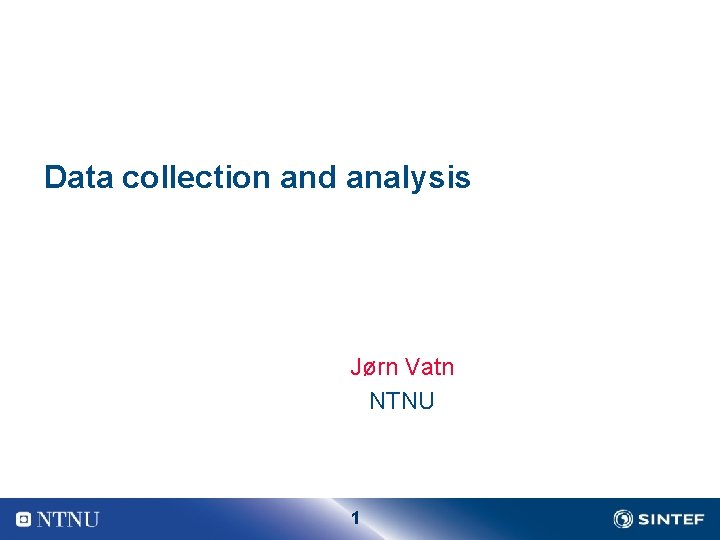
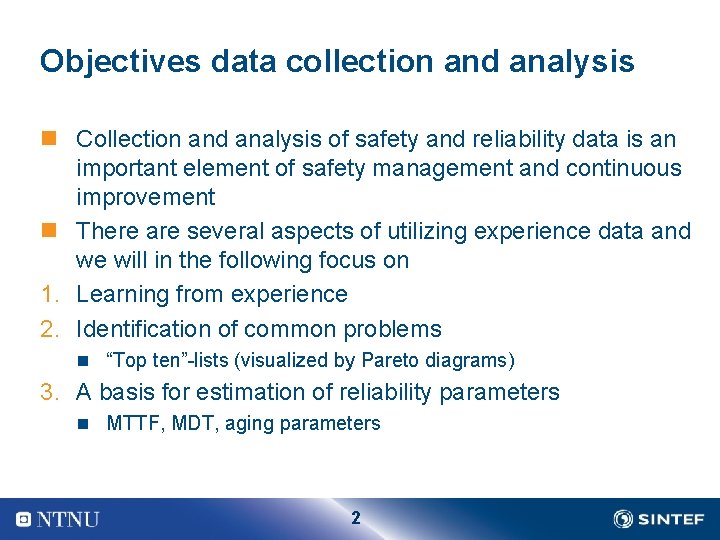
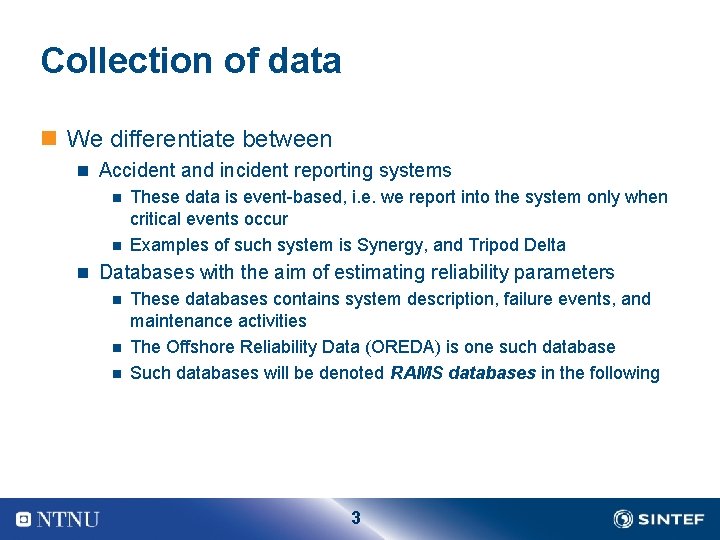
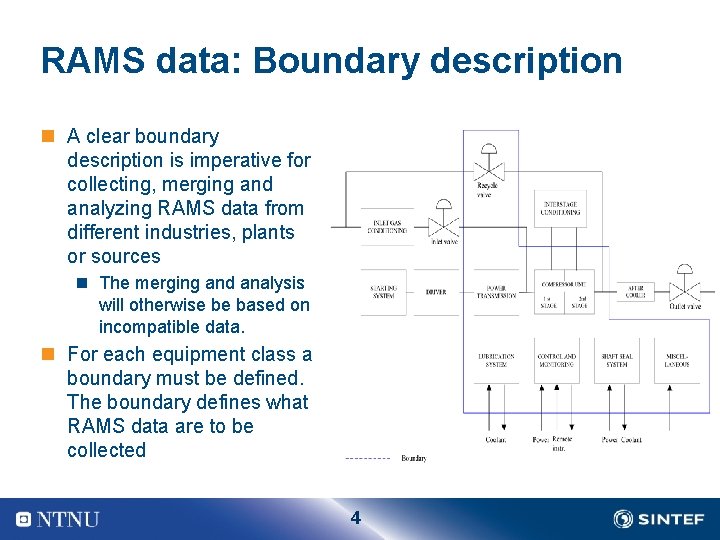
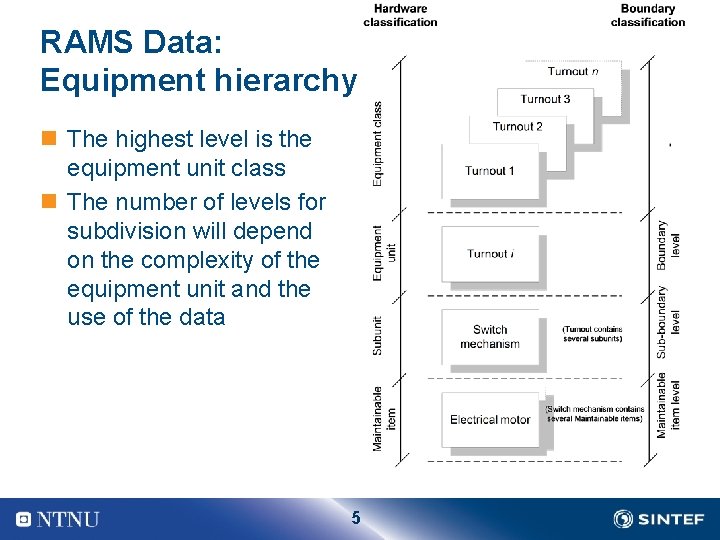
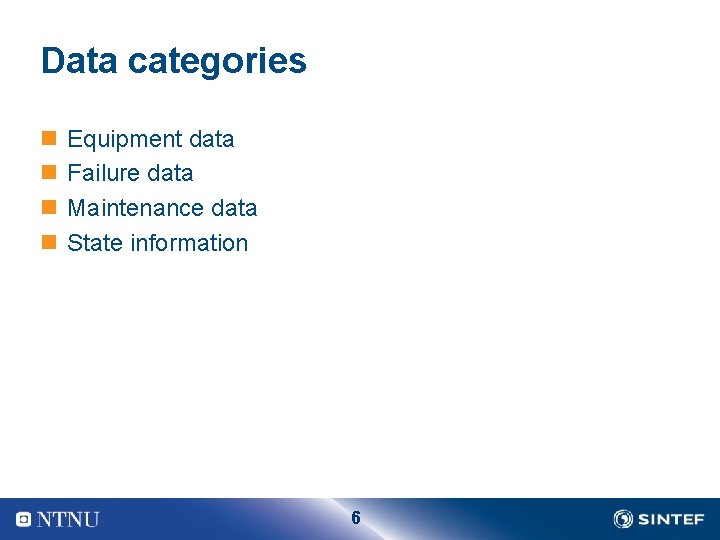
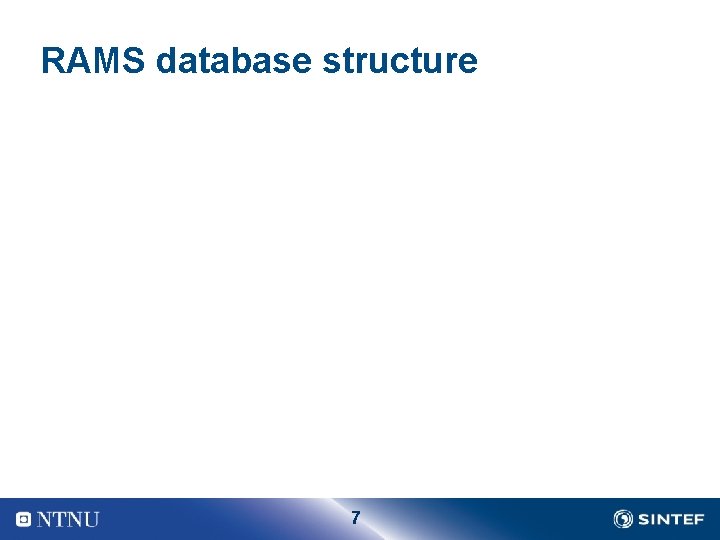
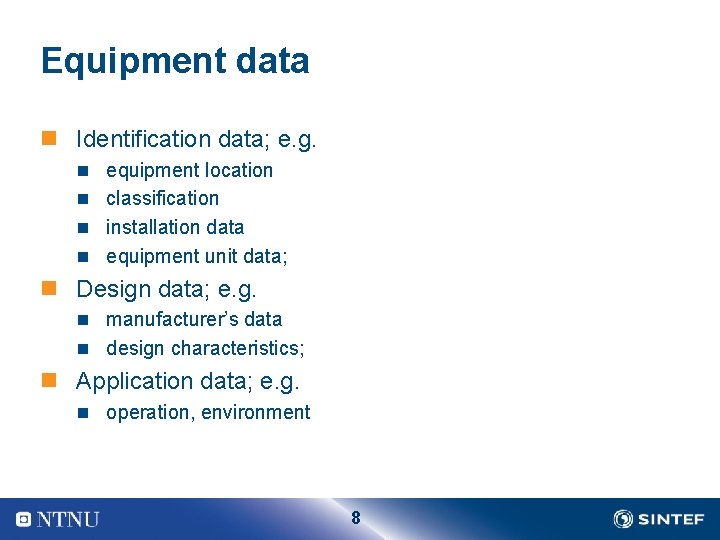
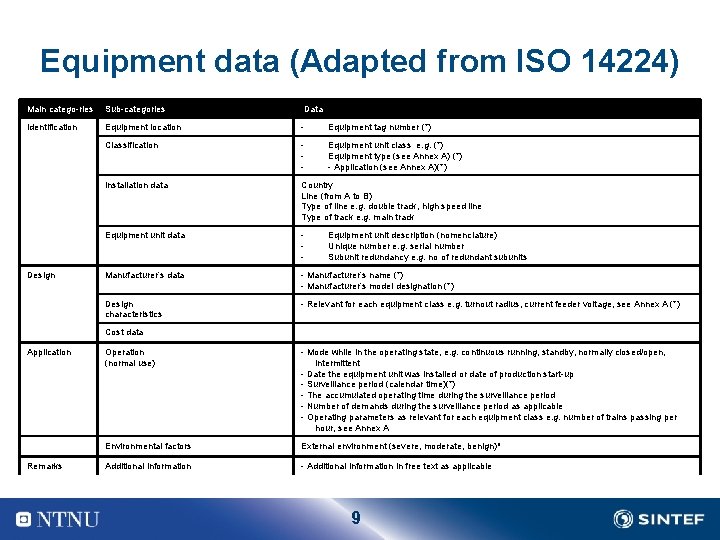
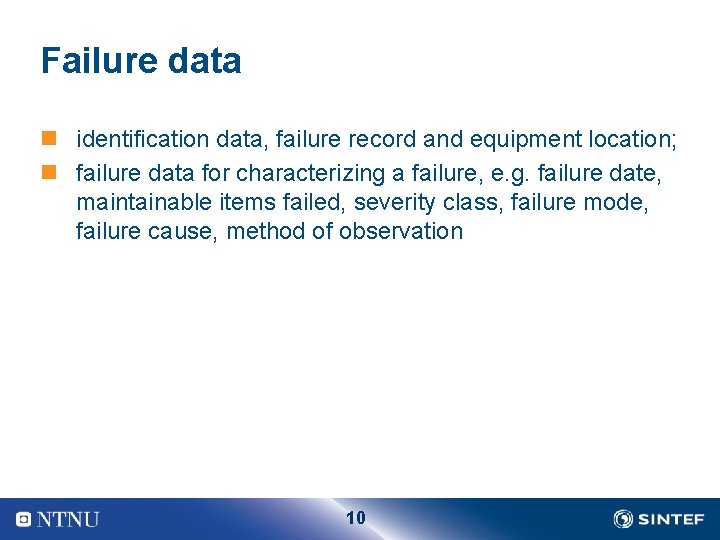
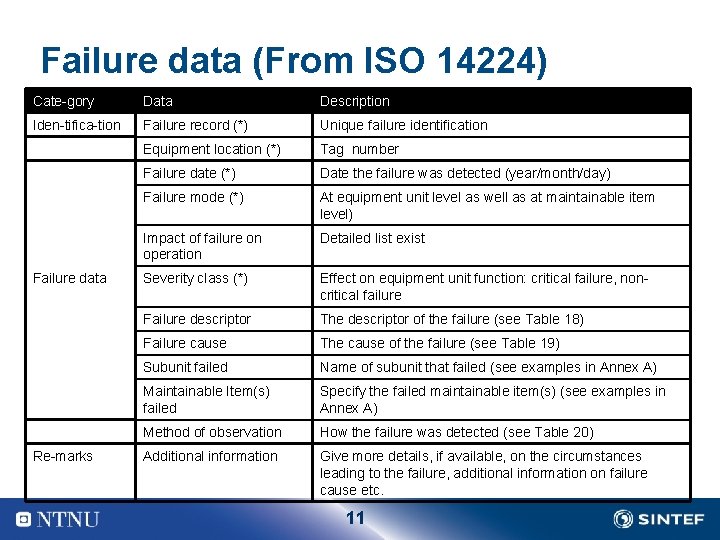
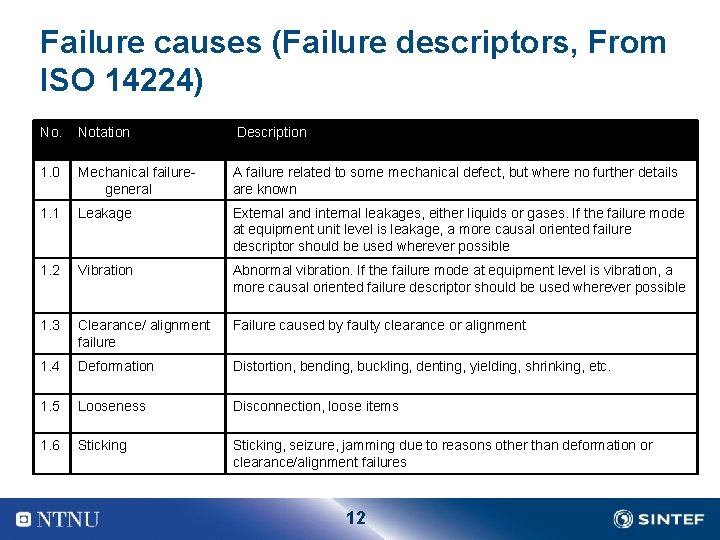
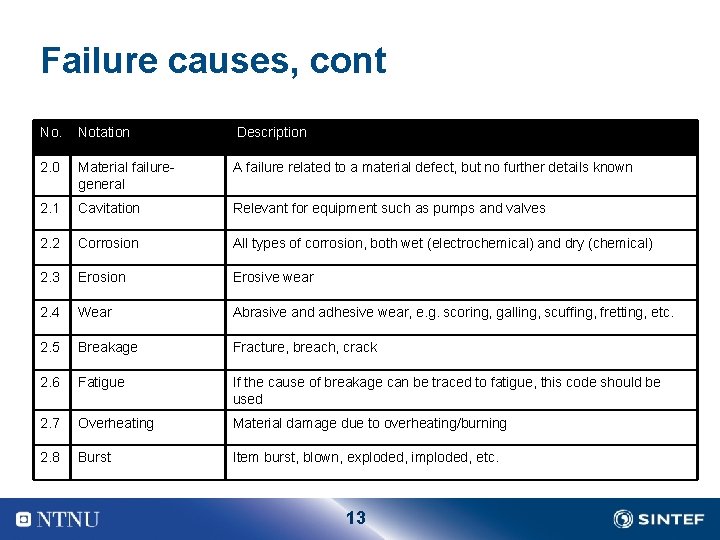
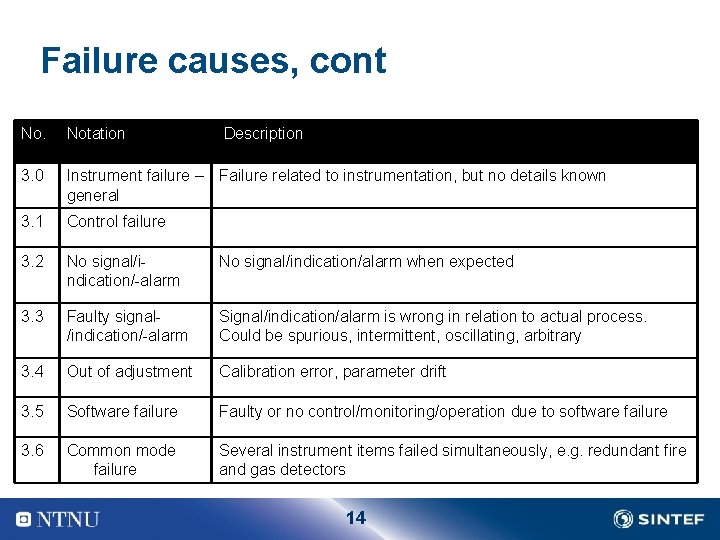
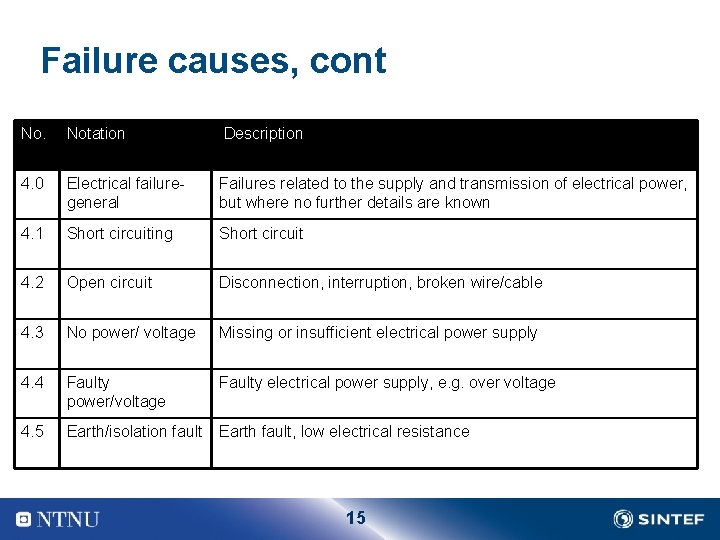
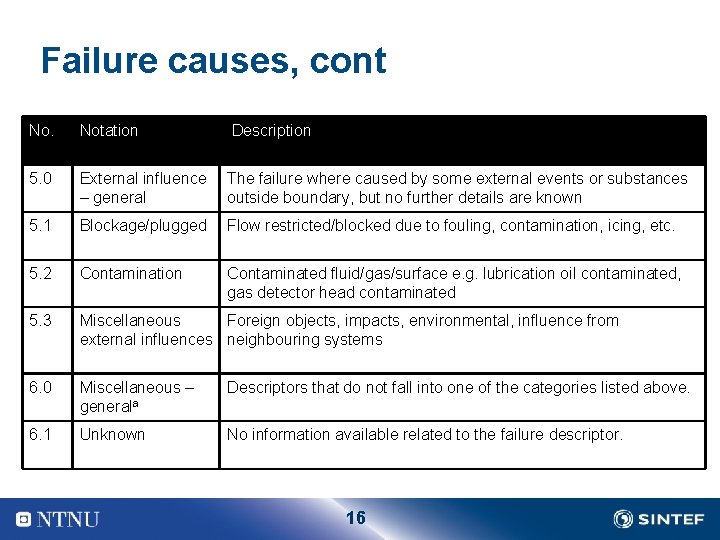
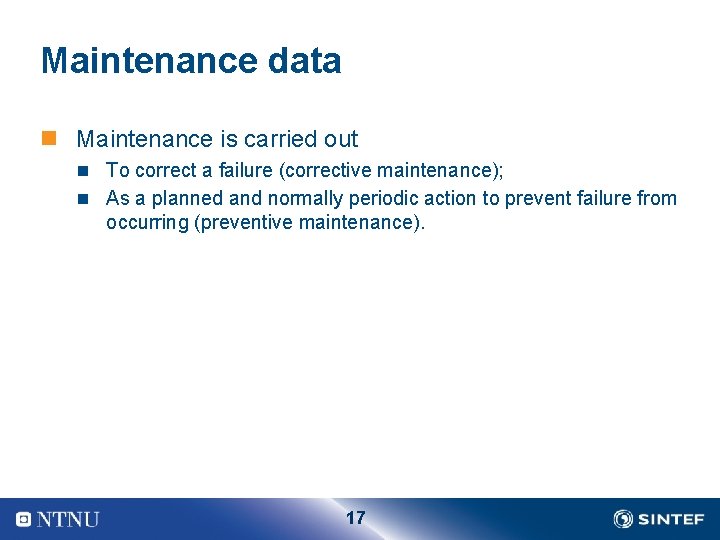
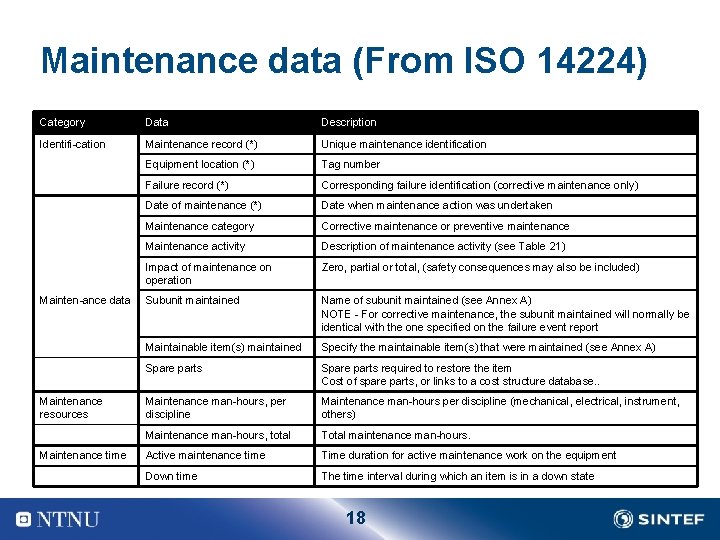
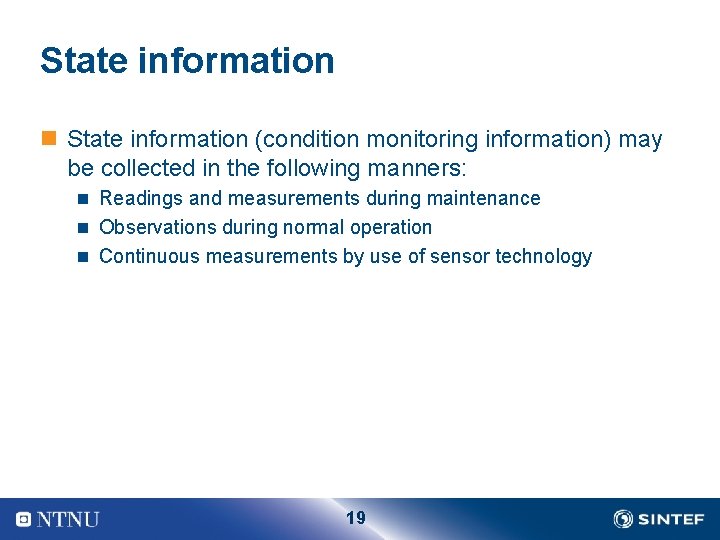
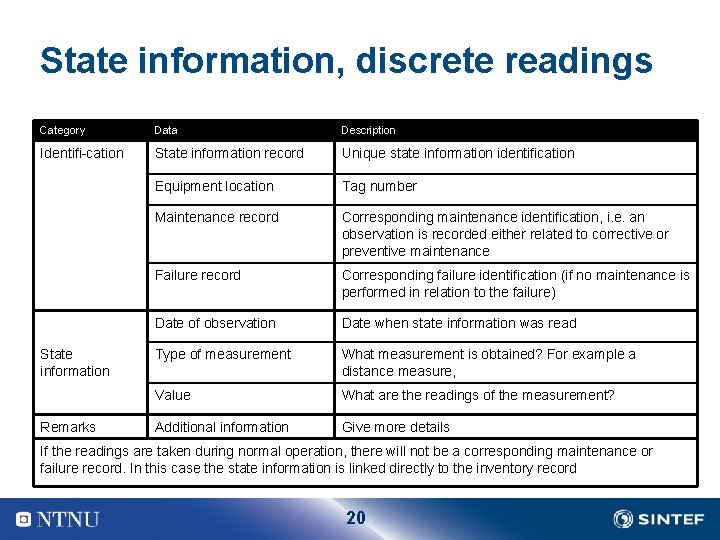
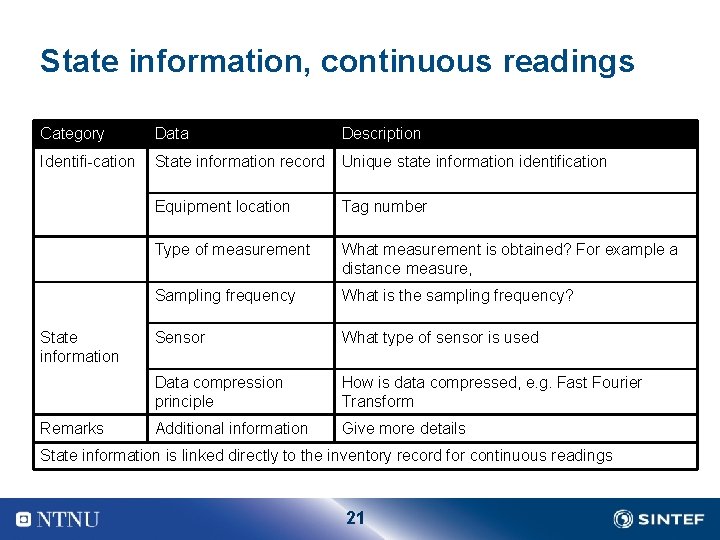
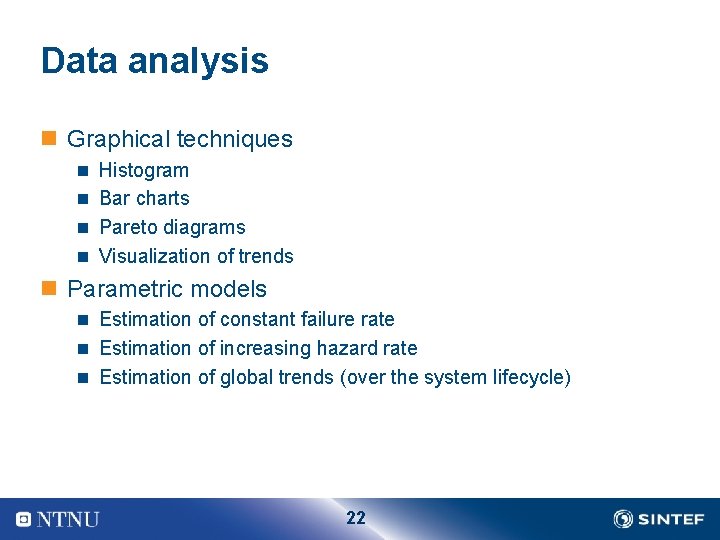
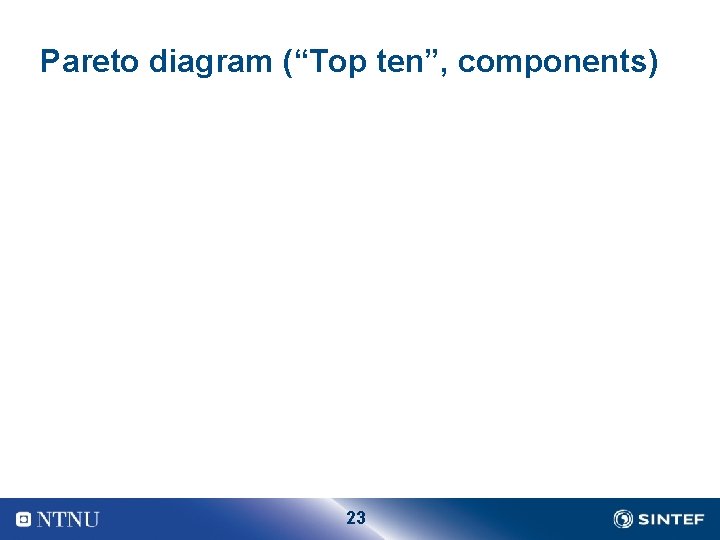
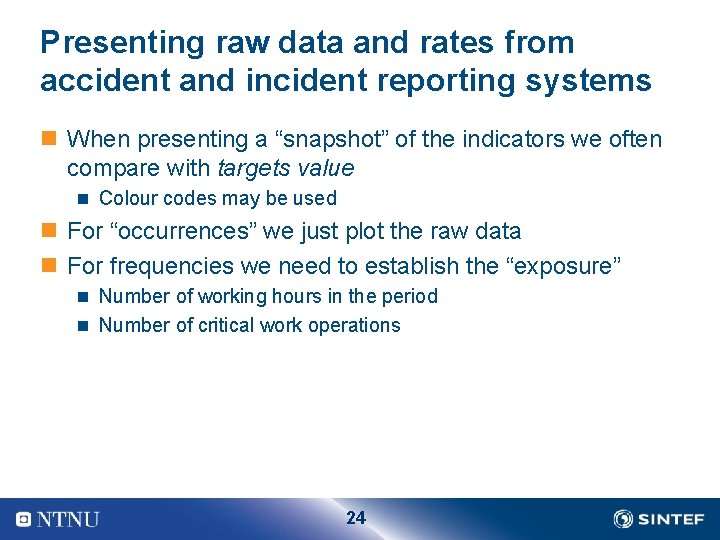
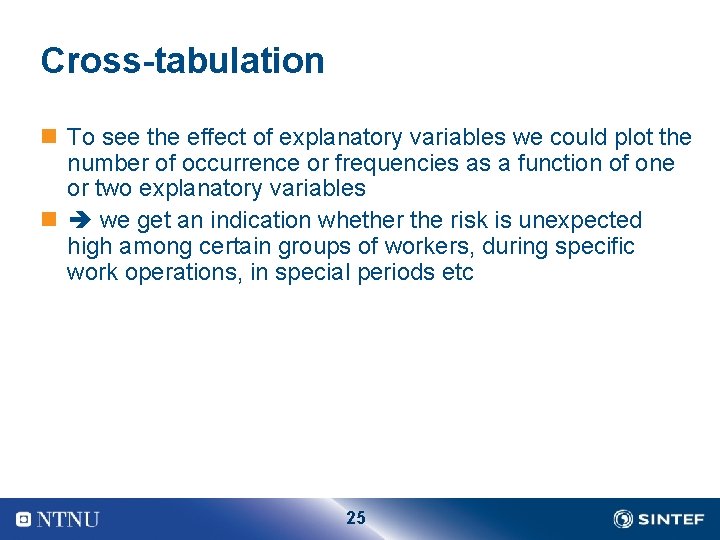
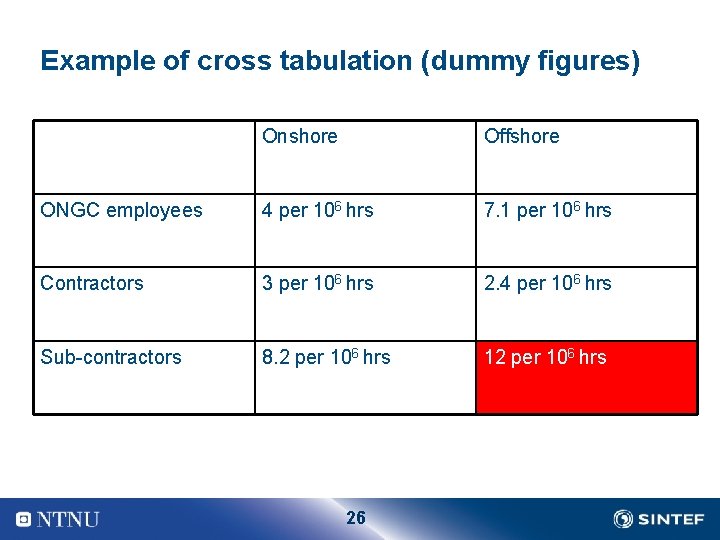
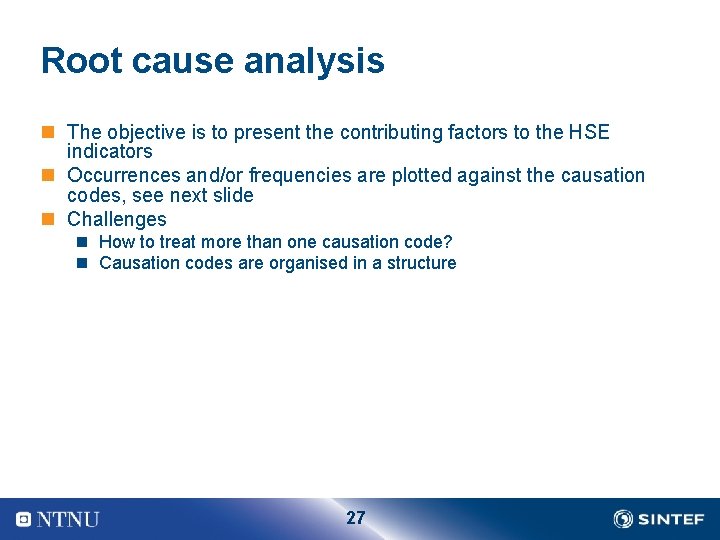
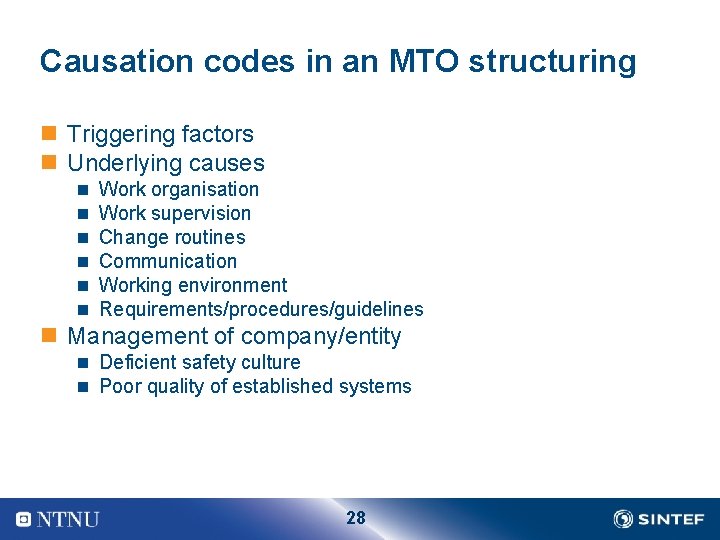
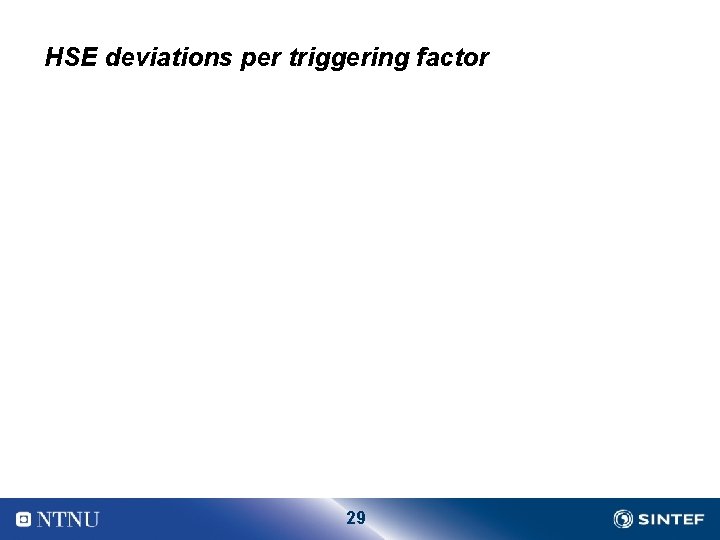
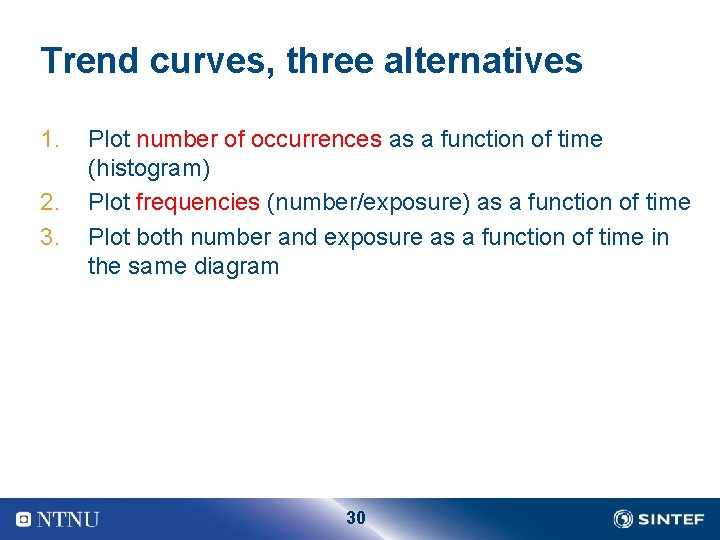
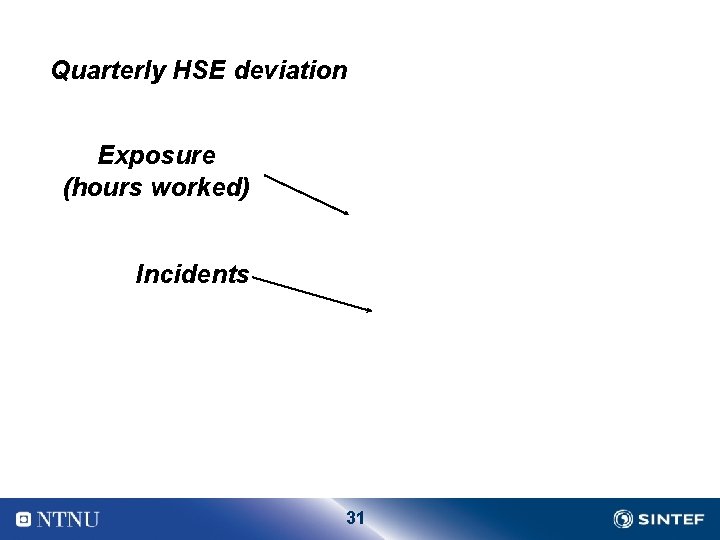
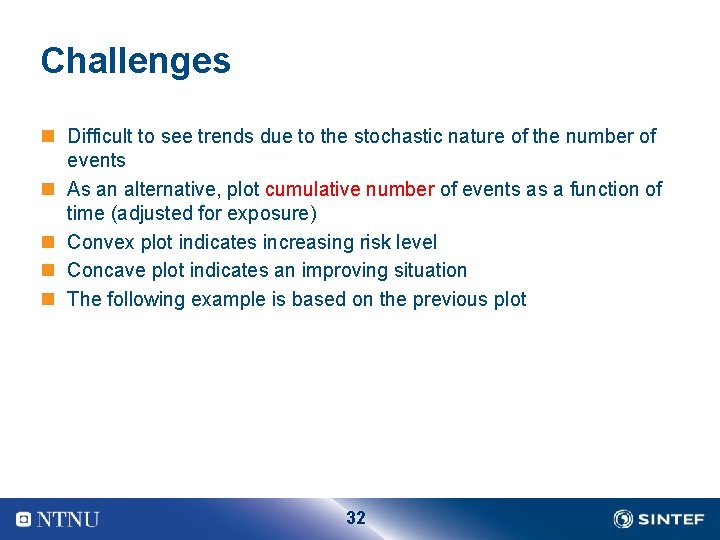
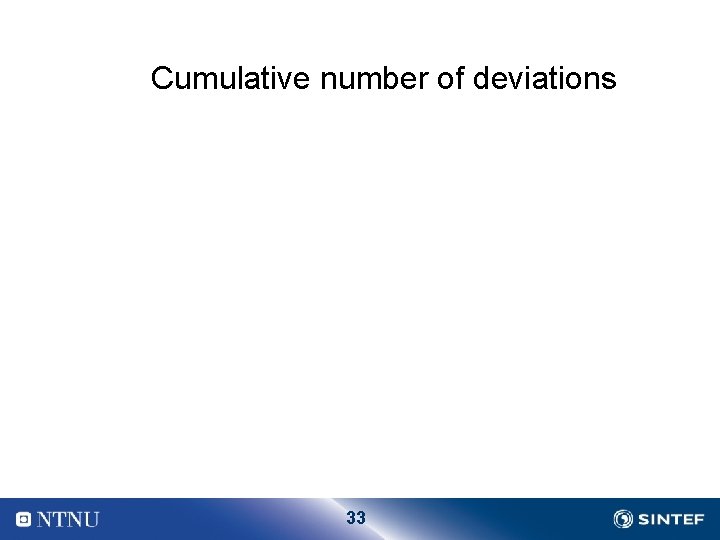
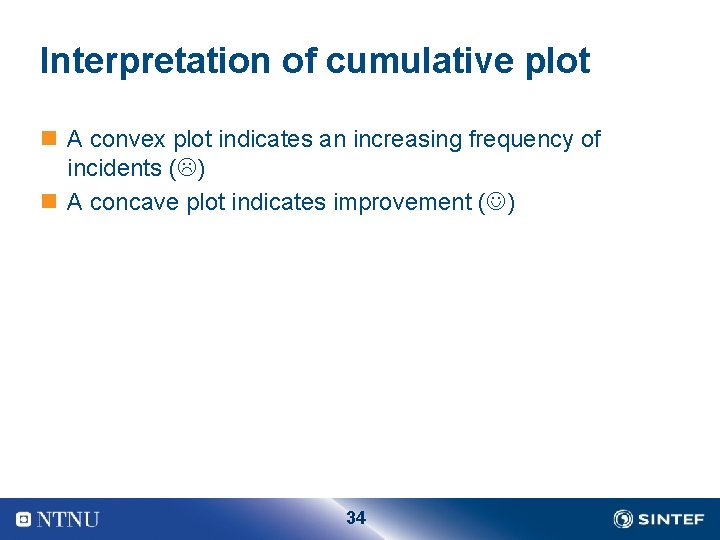
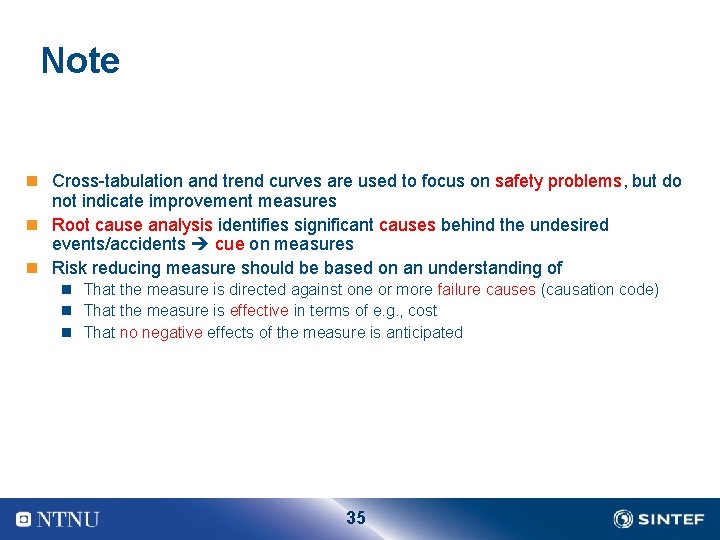
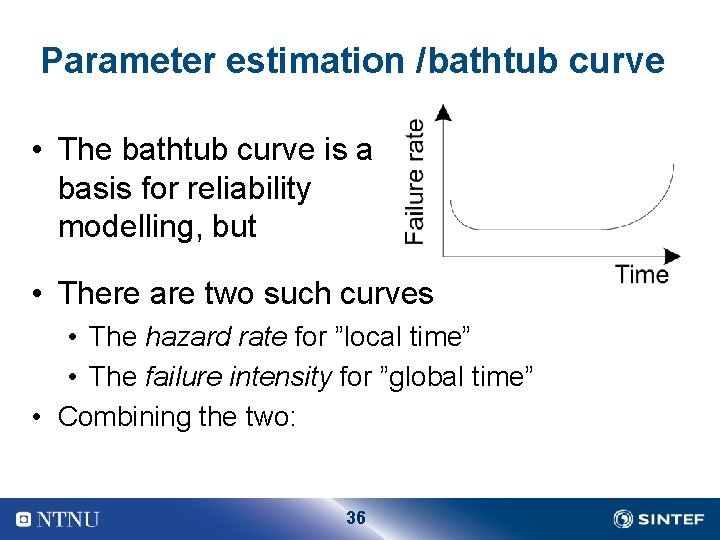
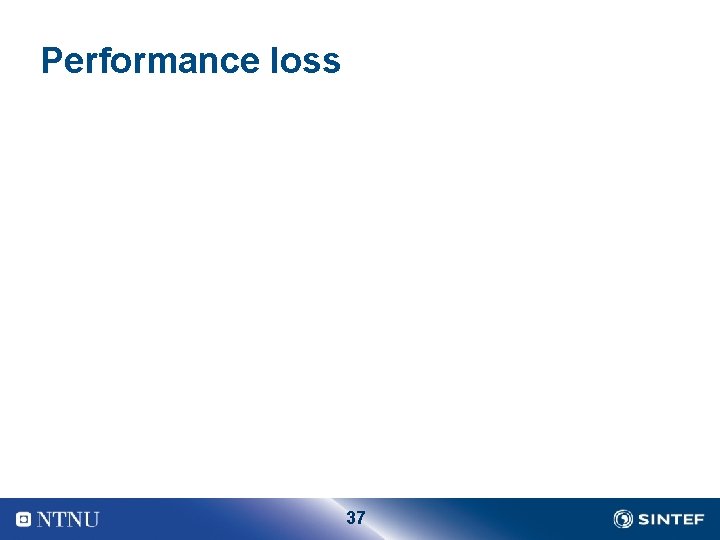
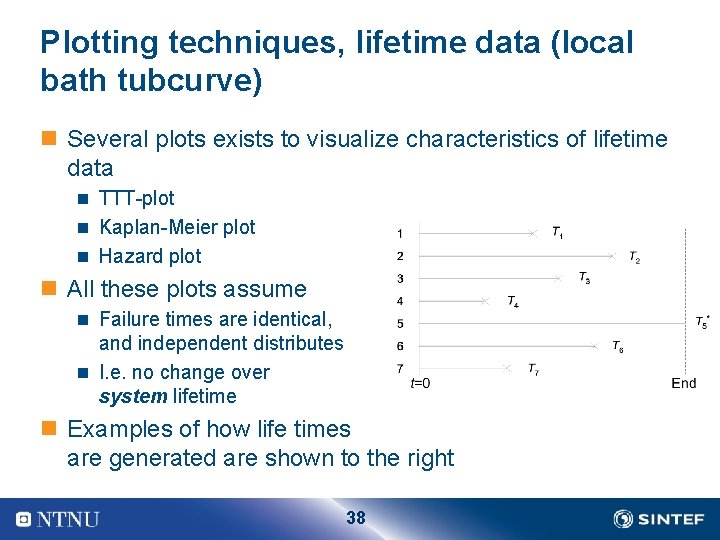
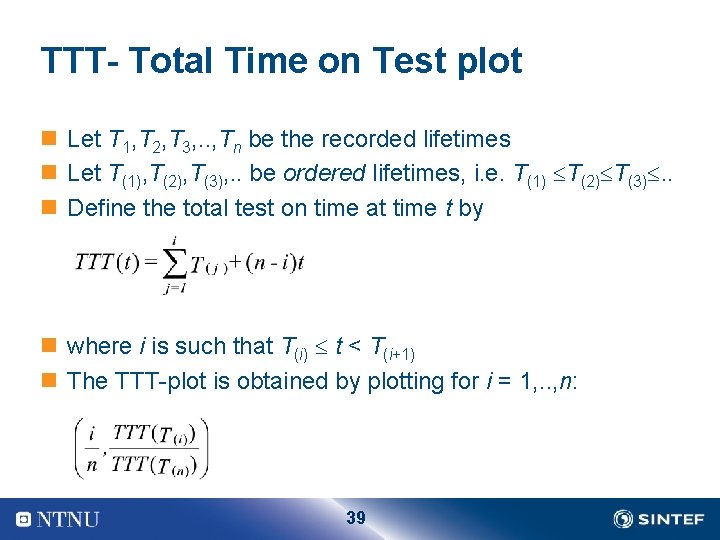
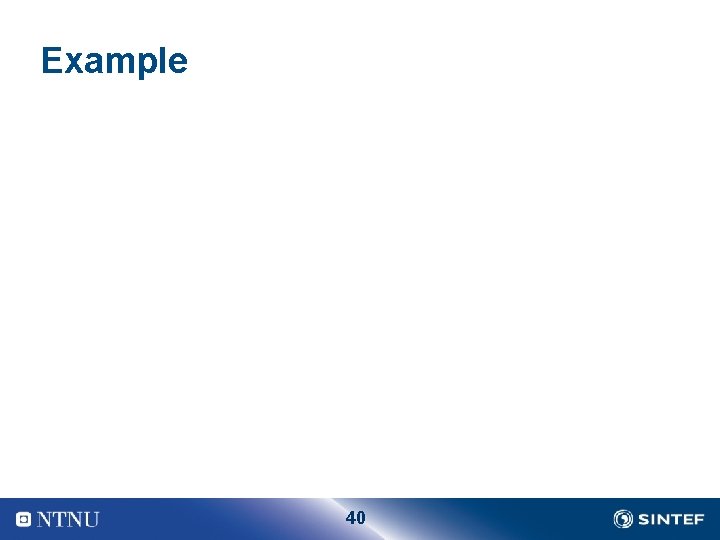
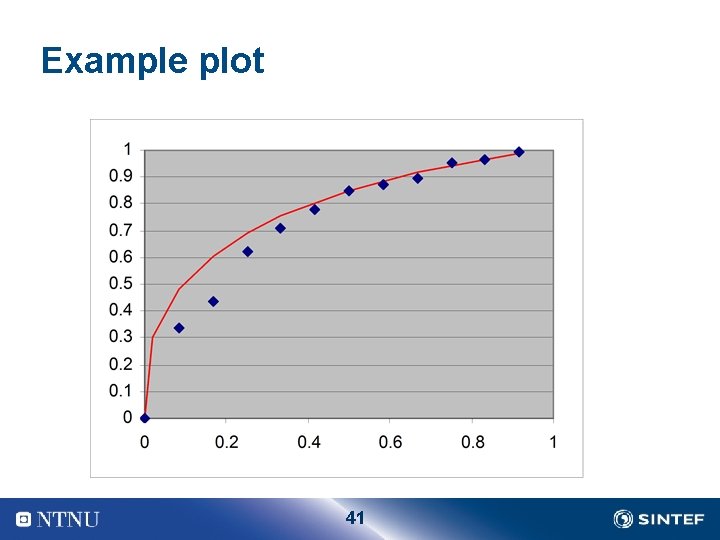
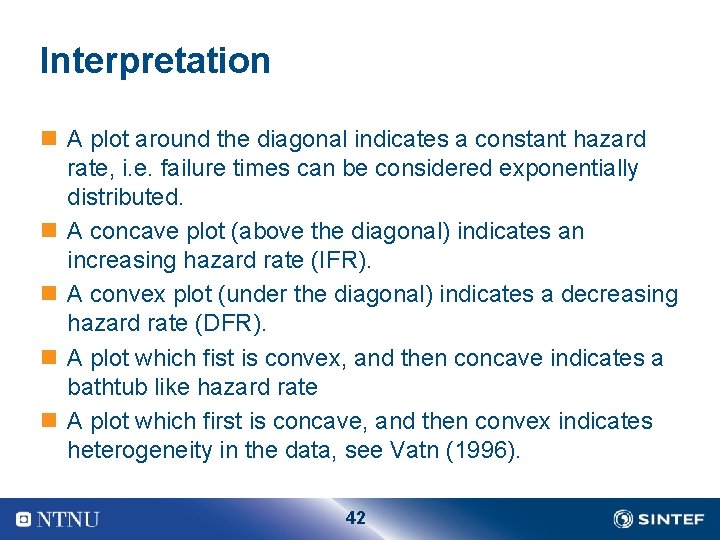
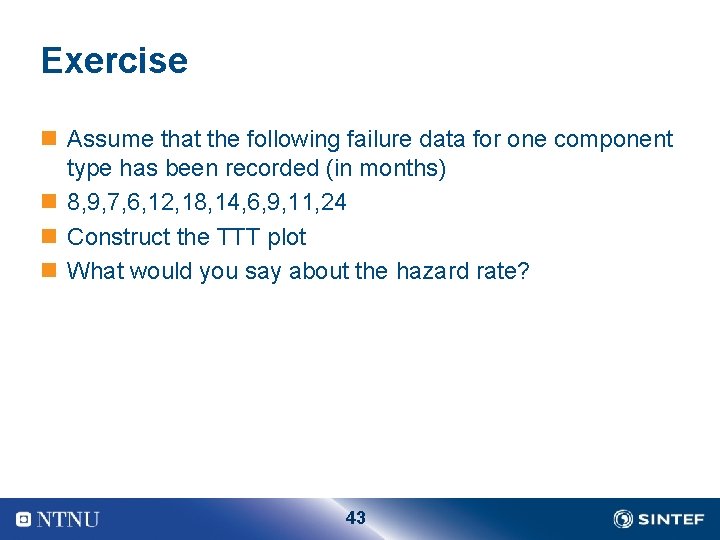
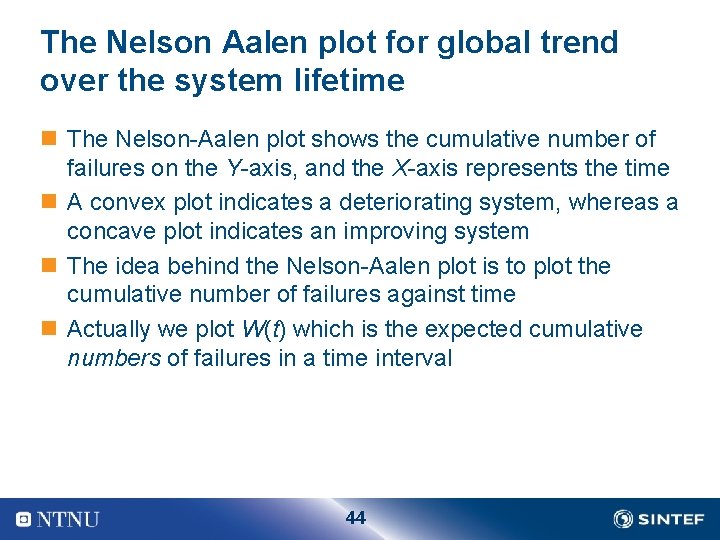
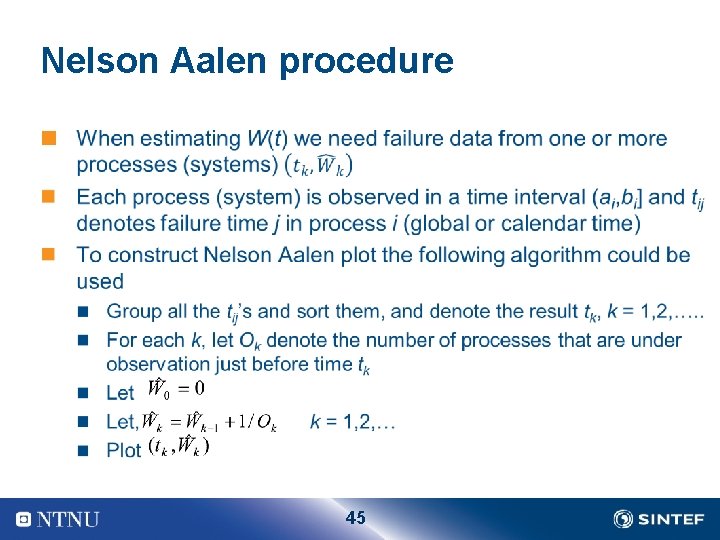
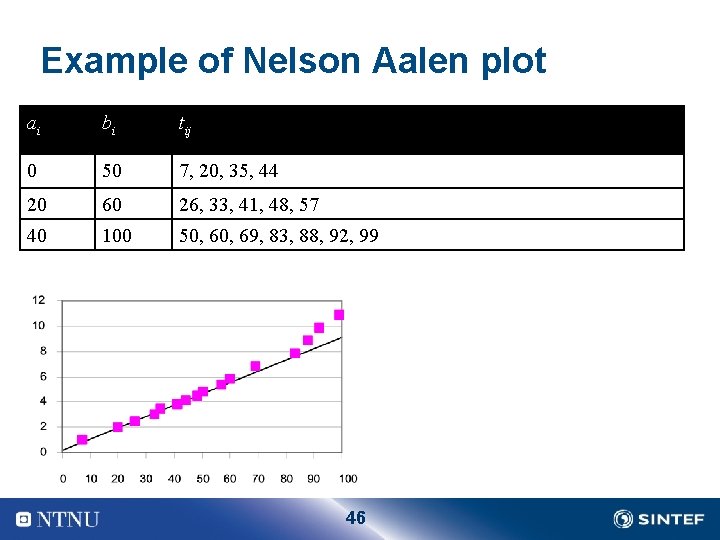
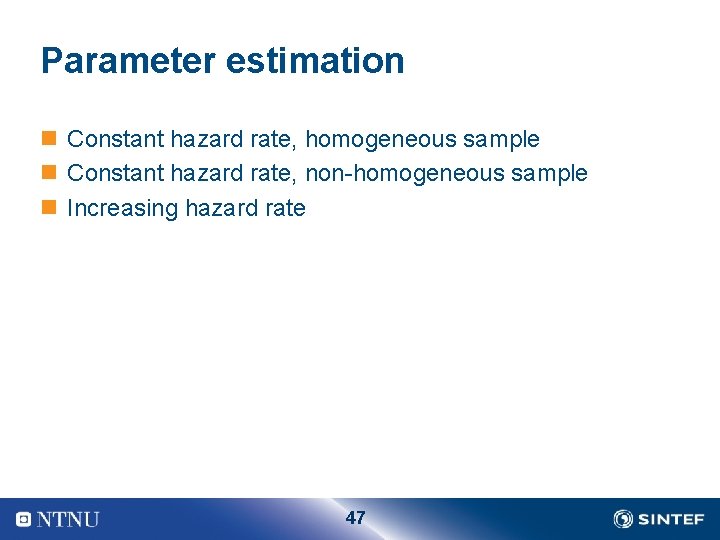
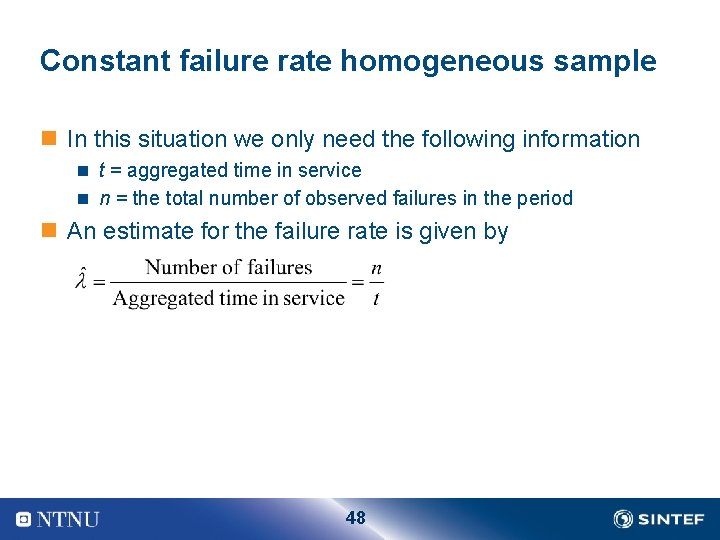
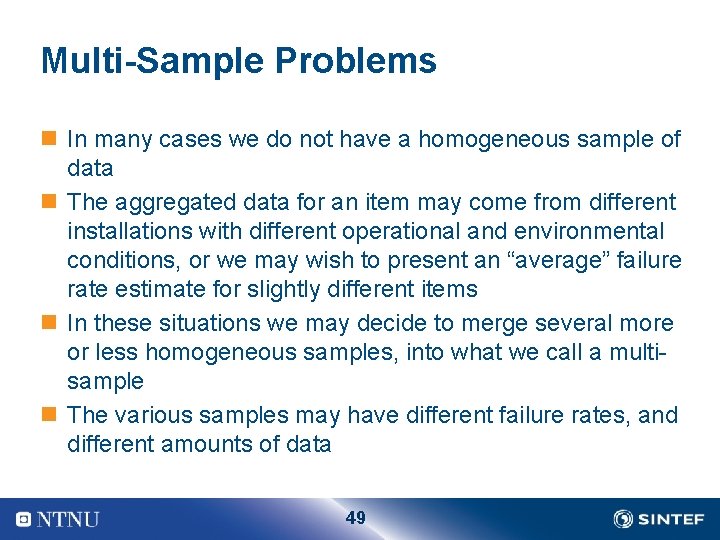
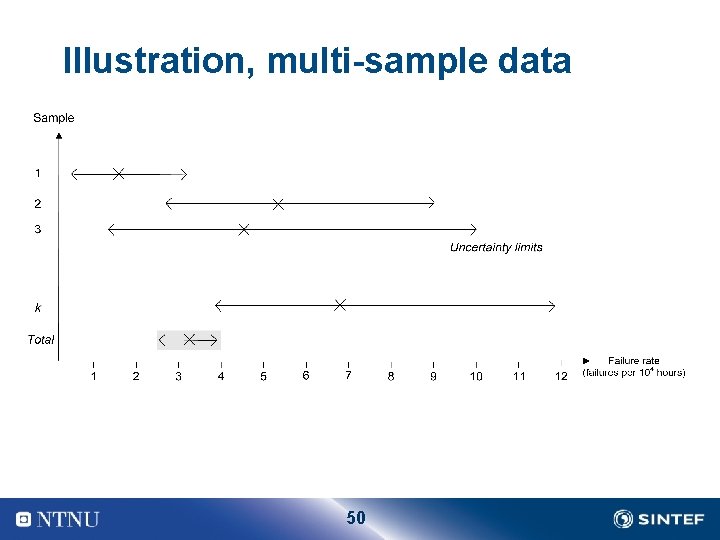
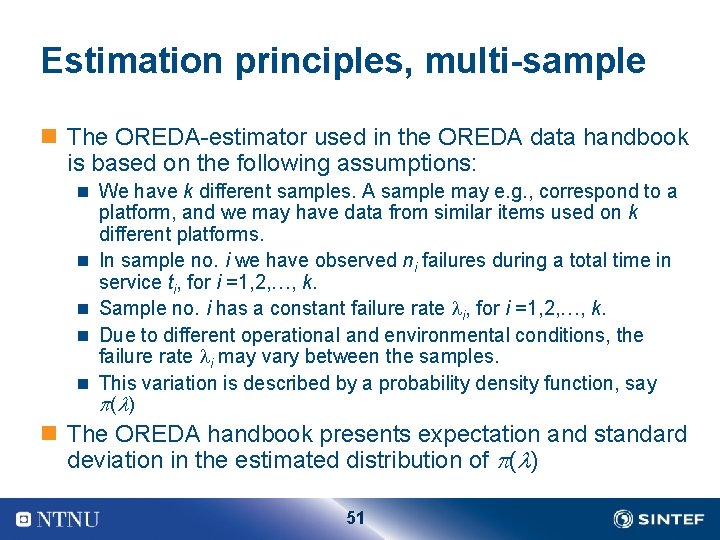
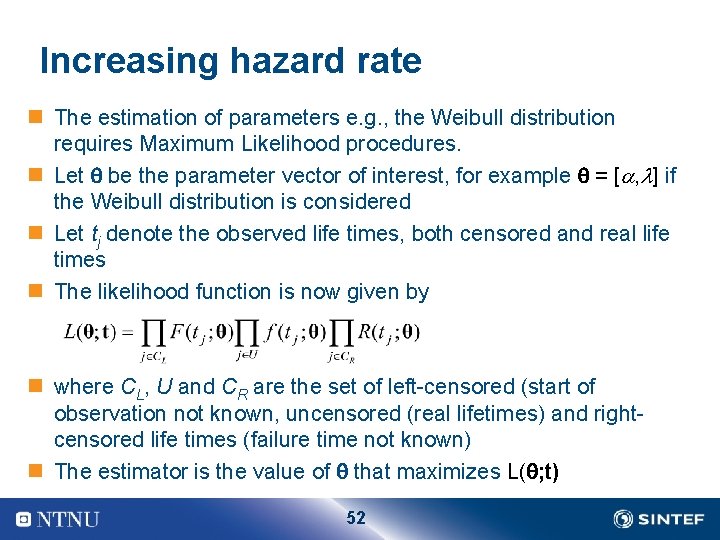
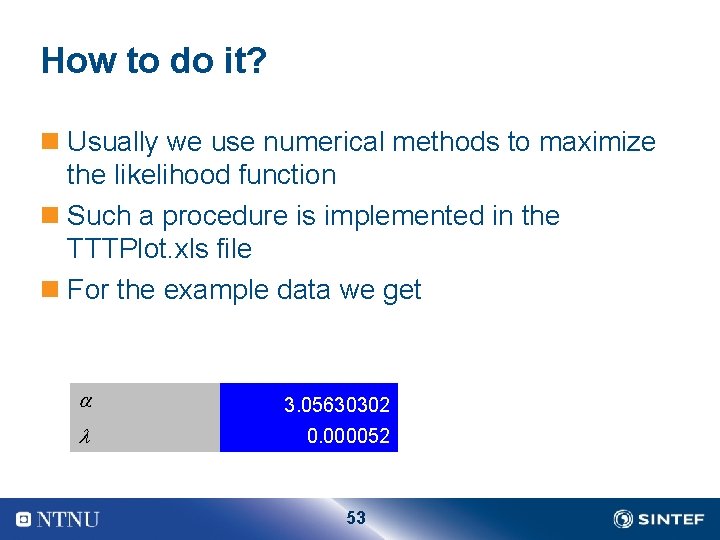
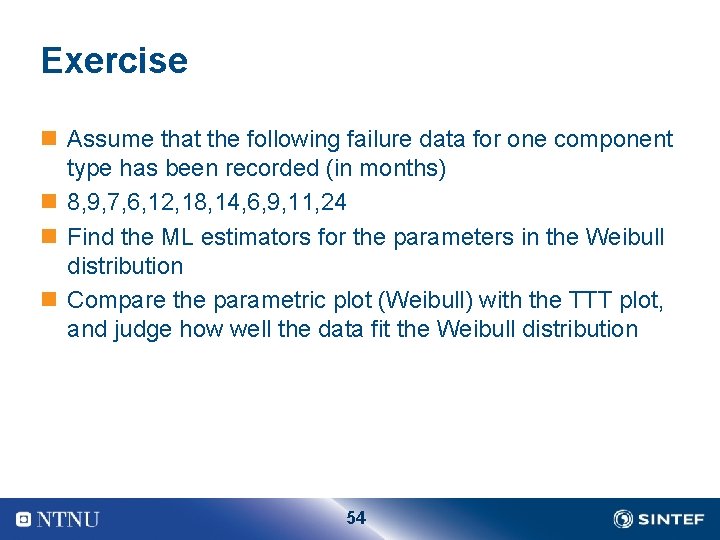
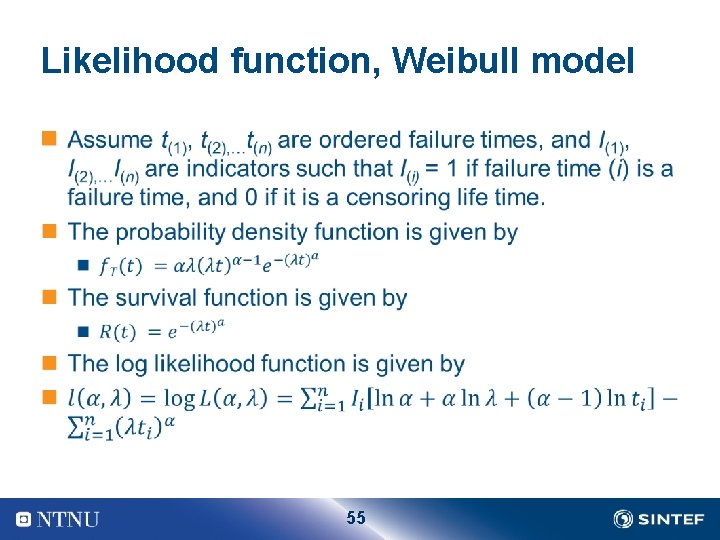
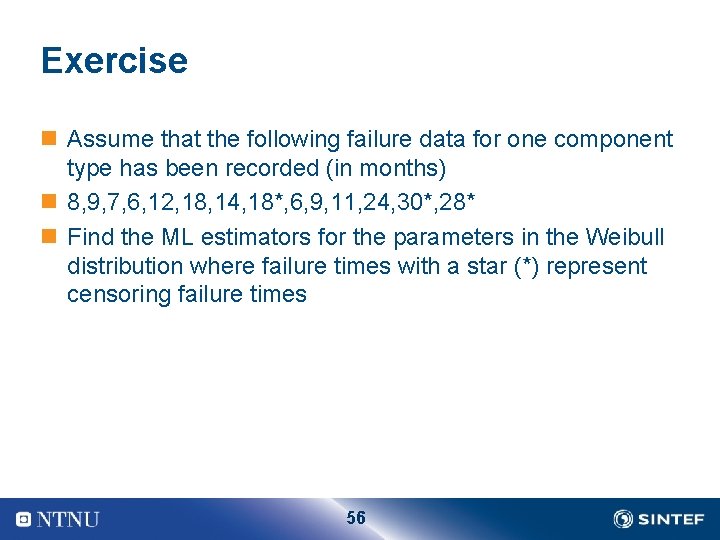
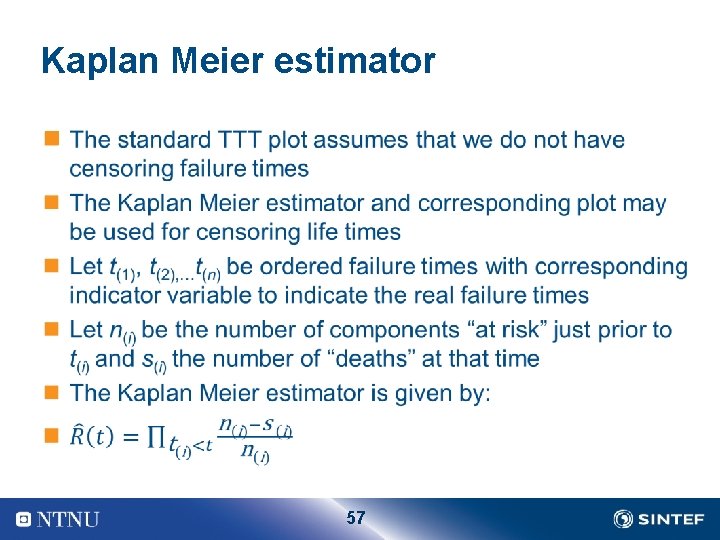
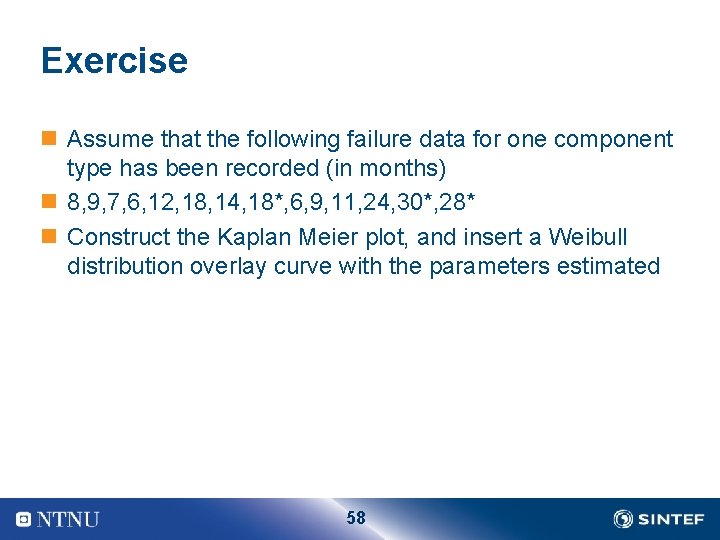
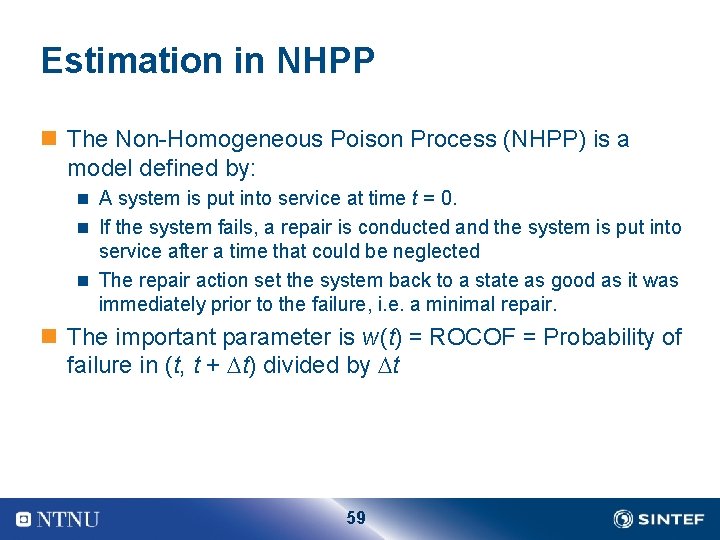
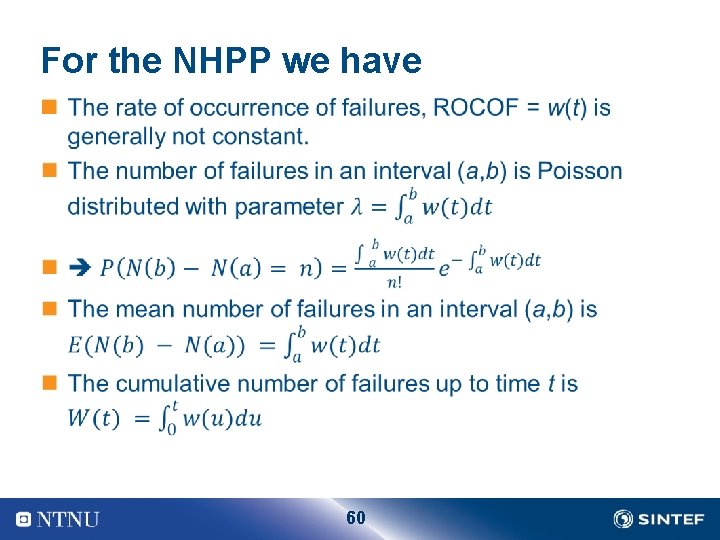
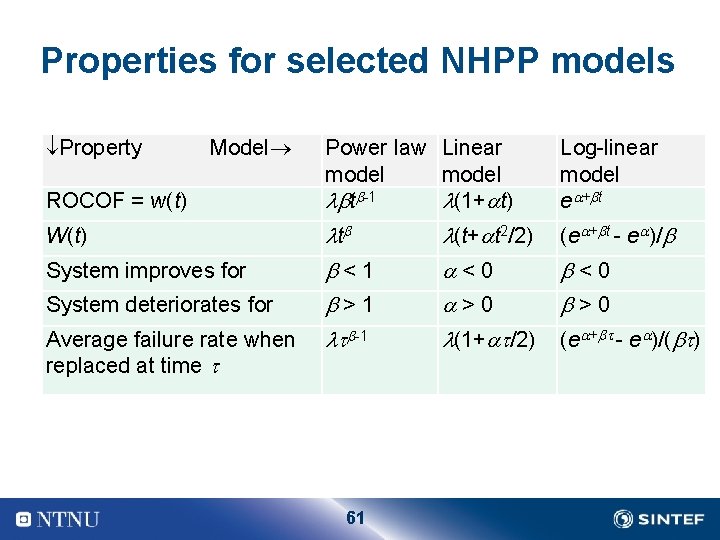
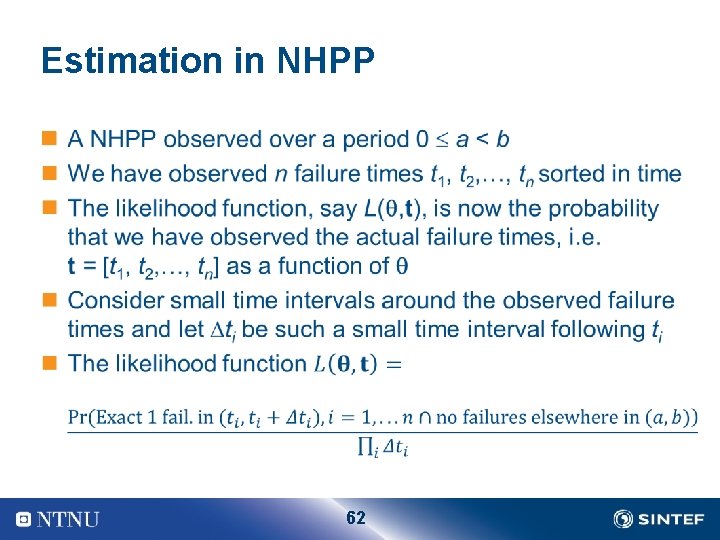
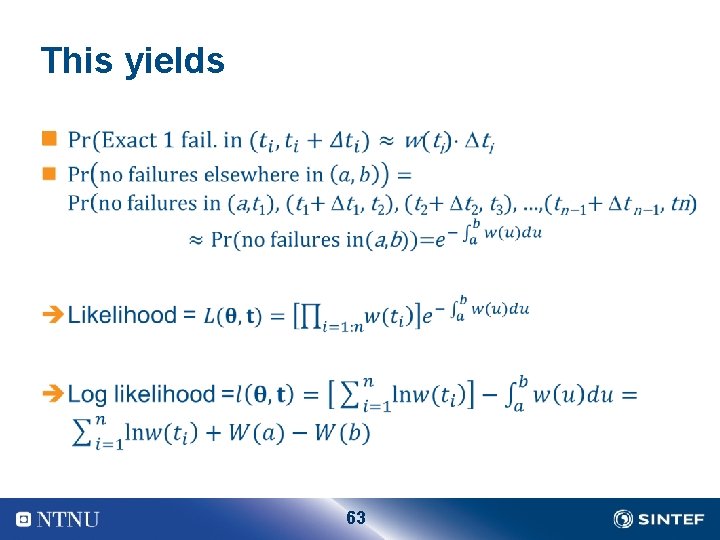
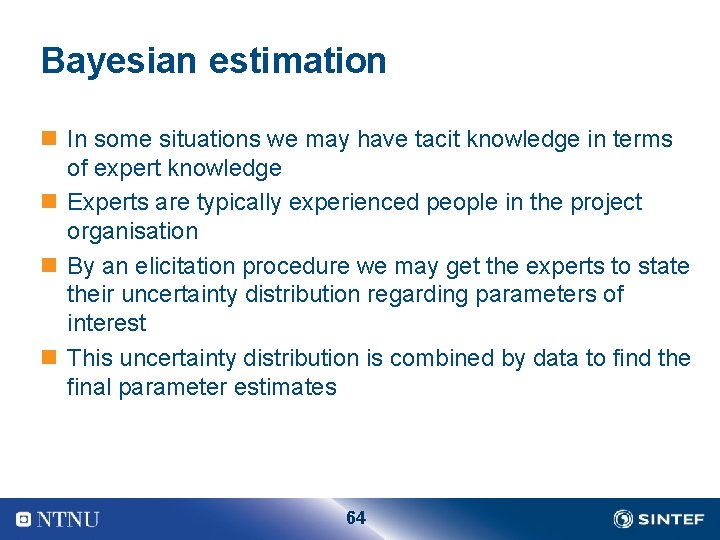
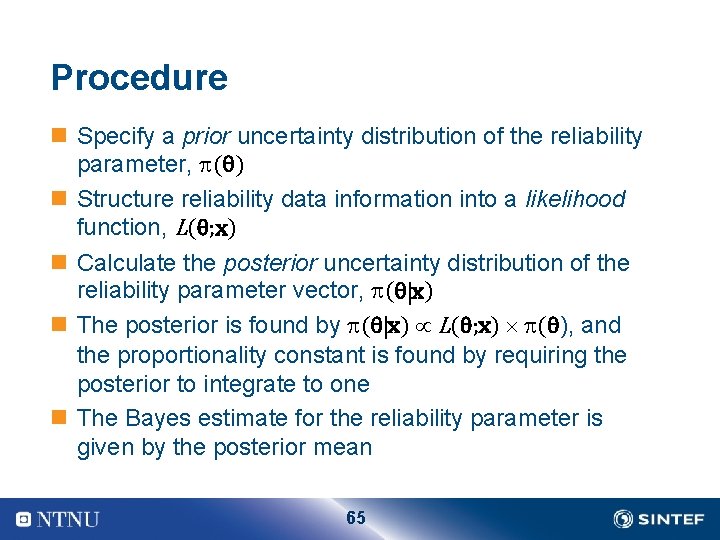
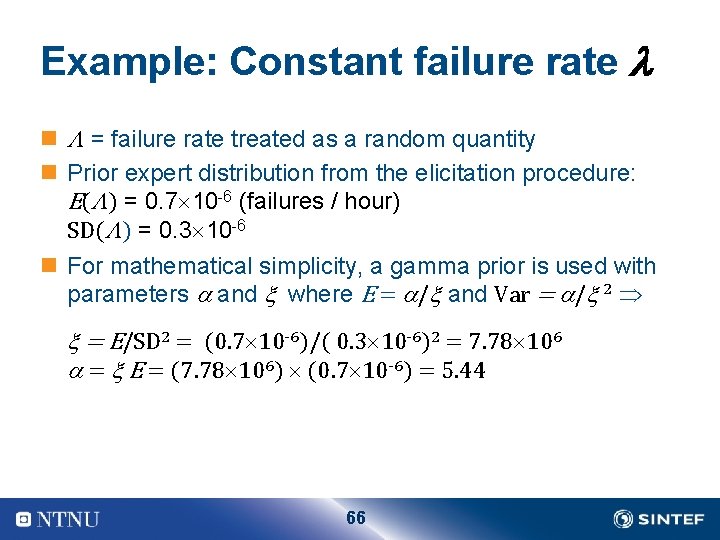
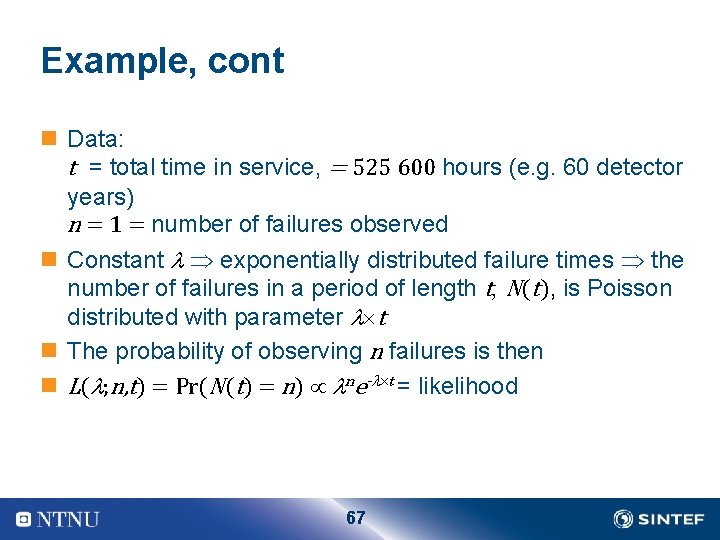
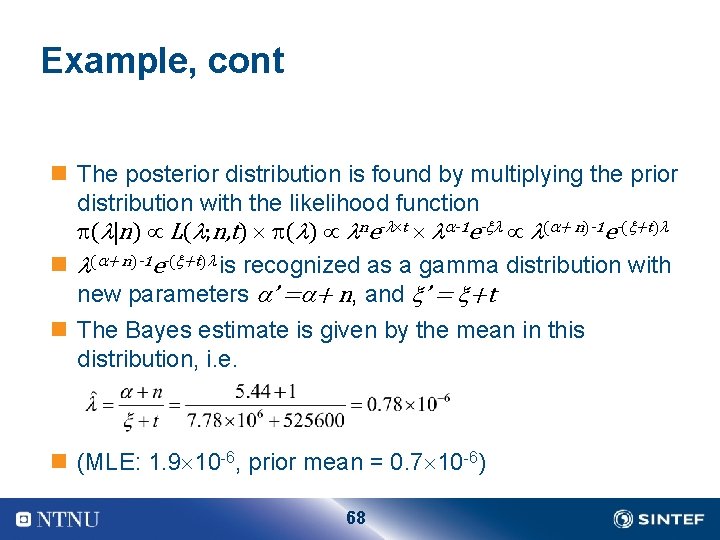
- Slides: 68
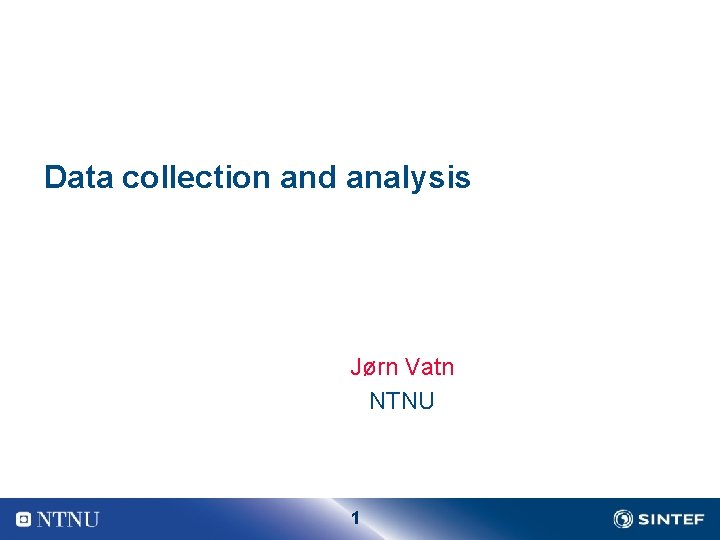
Data collection and analysis Jørn Vatn NTNU 1
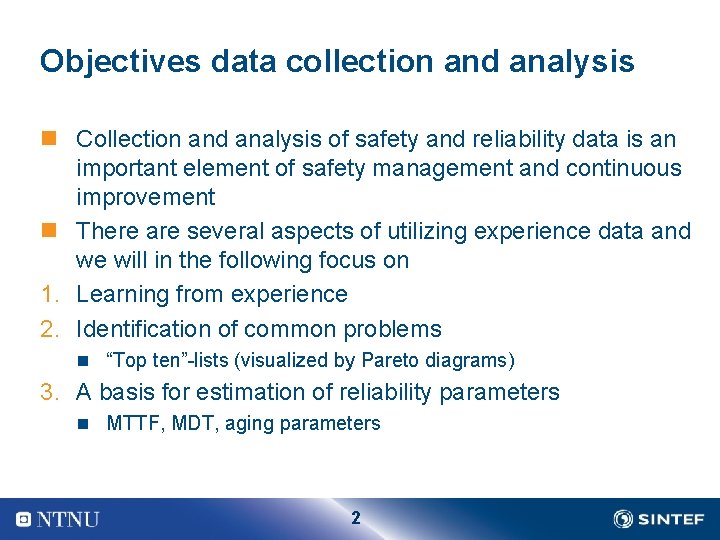
Objectives data collection and analysis n Collection and analysis of safety and reliability data is an important element of safety management and continuous improvement n There are several aspects of utilizing experience data and we will in the following focus on 1. Learning from experience 2. Identification of common problems n “Top ten” lists (visualized by Pareto diagrams) 3. A basis for estimation of reliability parameters n MTTF, MDT, aging parameters 2
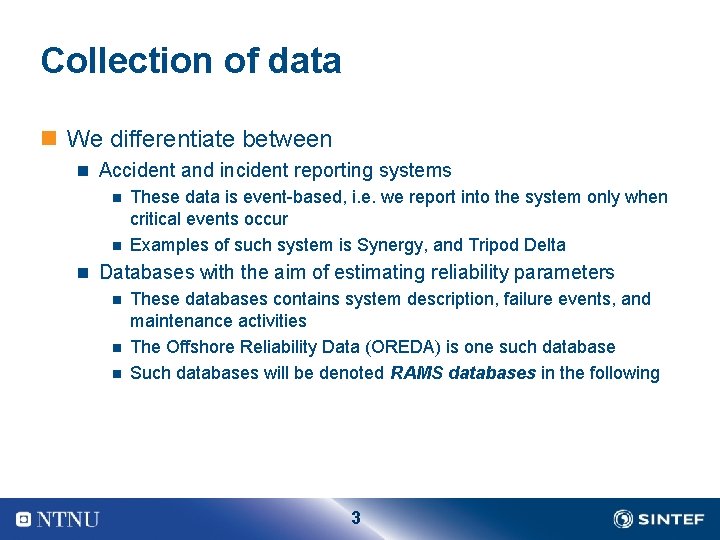
Collection of data n We differentiate between n Accident and incident reporting systems n These data is event based, i. e. we report into the system only when critical events occur n Examples of such system is Synergy, and Tripod Delta n Databases with the aim of estimating reliability parameters n These databases contains system description, failure events, and maintenance activities n The Offshore Reliability Data (OREDA) is one such database n Such databases will be denoted RAMS databases in the following 3
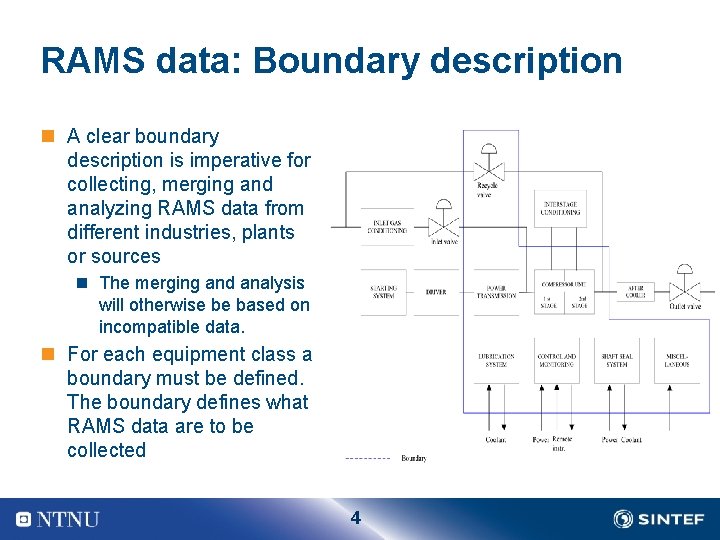
RAMS data: Boundary description n A clear boundary description is imperative for collecting, merging and analyzing RAMS data from different industries, plants or sources n The merging and analysis will otherwise be based on incompatible data. n For each equipment class a boundary must be defined. The boundary defines what RAMS data are to be collected 4
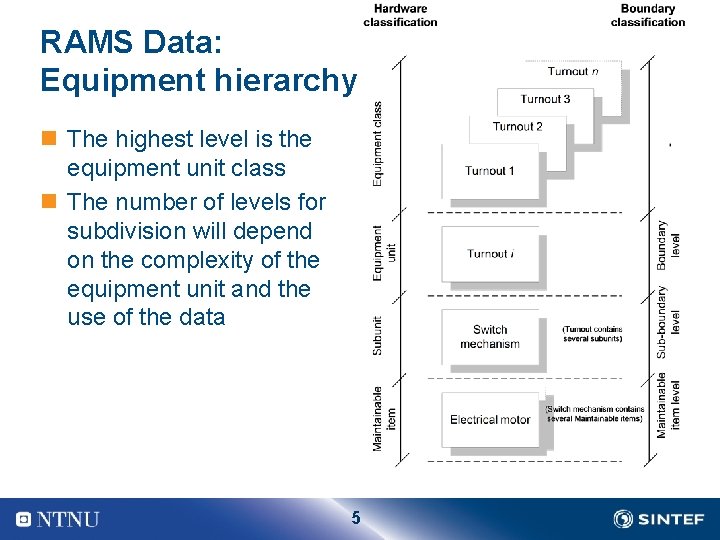
RAMS Data: Equipment hierarchy n The highest level is the equipment unit class n The number of levels for subdivision will depend on the complexity of the equipment unit and the use of the data 5
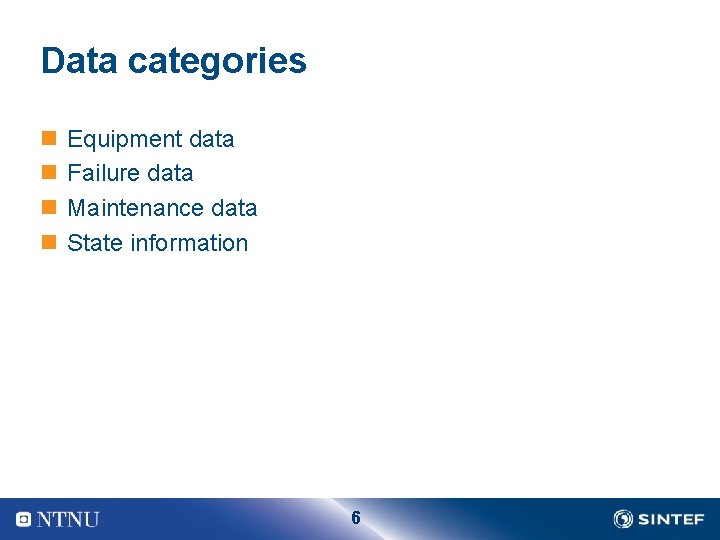
Data categories n n Equipment data Failure data Maintenance data State information 6
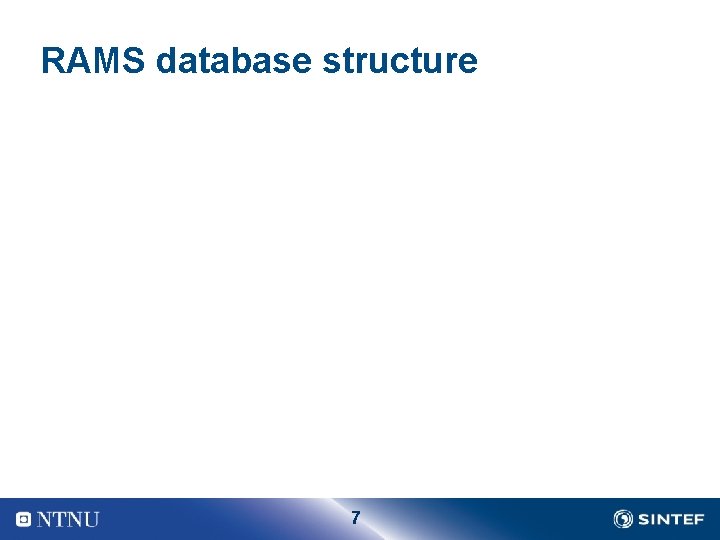
RAMS database structure 7
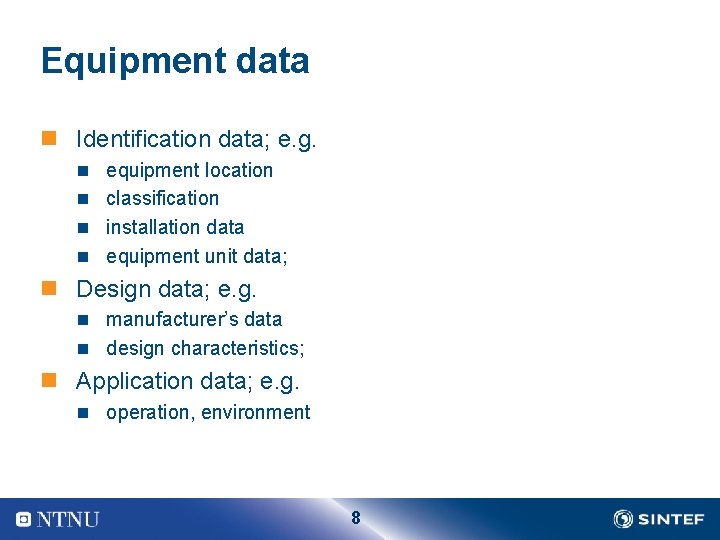
Equipment data n Identification data; e. g. n equipment location n classification n installation data n equipment unit data; n Design data; e. g. n manufacturer’s data n design characteristics; n Application data; e. g. n operation, environment 8
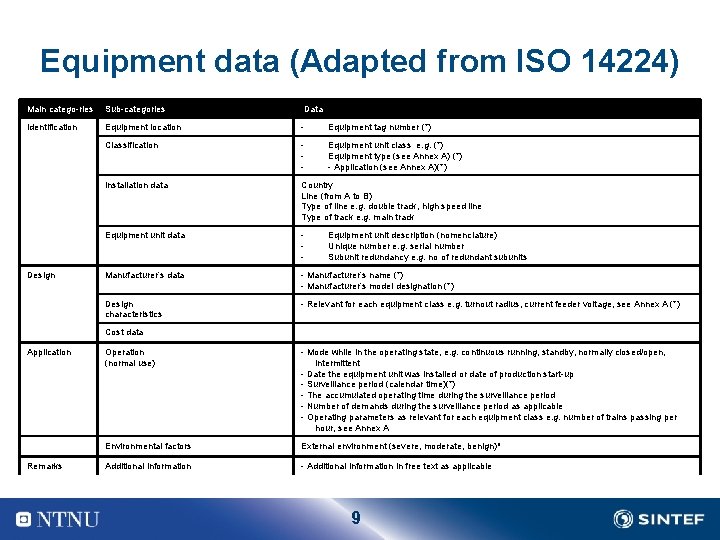
Equipment data (Adapted from ISO 14224) Main catego ries Sub categories Data Identification Equipment location Equipment tag number (*) Classification Equipment unit class e. g. (*) Equipment type (see Annex A) (*) Application (see Annex A)(*) Installation data Country Line (from A to B) Type of line e. g. double track, high speed line Type of track e. g. main track Equipment unit data Manufacturer’s data Manufacturer’s name (*) Manufacturer’s model designation (*) Design characteristics Relevant for each equipment class e. g. turnout radius, current feeder voltage, see Annex A (*) Design Equipment unit description (nomenclature) Unique number e. g. serial number Subunit redundancy e. g. no of redundant subunits Cost data Application Remarks Operation (normal use) Mode while in the operating state, e. g. continuous running, standby, normally closed/open, intermittent Date the equipment unit was installed or date of production start up Surveillance period (calendar time)(*) The accumulated operating time during the surveillance period Number of demands during the surveillance period as applicable Operating parameters as relevant for each equipment class e. g. number of trains passing per hour, see Annex A Environmental factors External environment (severe, moderate, benign)a Additional information in free text as applicable 9
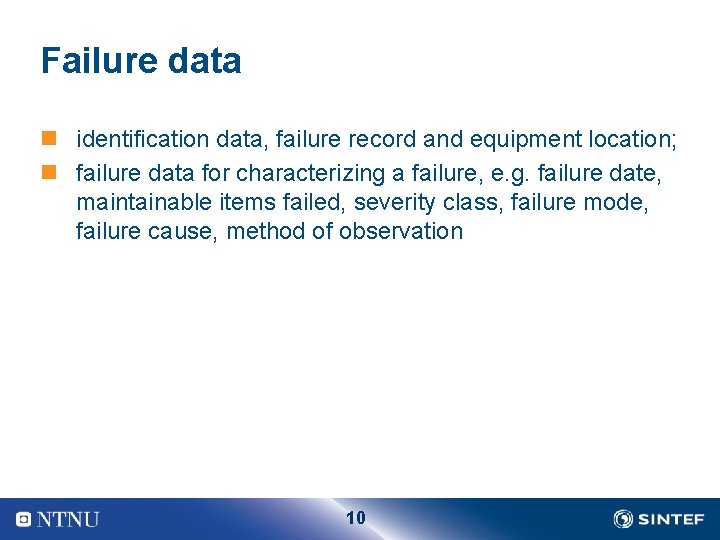
Failure data n identification data, failure record and equipment location; n failure data for characterizing a failure, e. g. failure date, maintainable items failed, severity class, failure mode, failure cause, method of observation 10
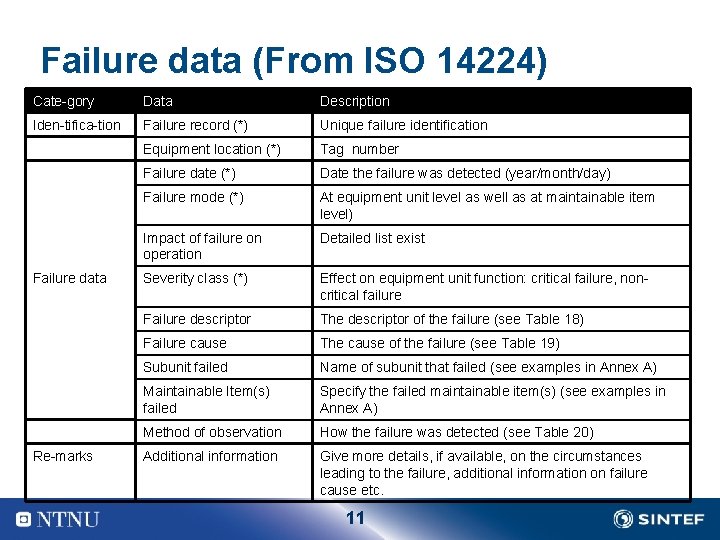
Failure data (From ISO 14224) Cate gory Data Description Iden tifica tion Failure record (*) Unique failure identification Equipment location (*) Tag number Failure date (*) Date the failure was detected (year/month/day) Failure mode (*) At equipment unit level as well as at maintainable item level) Impact of failure on operation Detailed list exist Severity class (*) Effect on equipment unit function: critical failure, non critical failure Failure descriptor The descriptor of the failure (see Table 18) Failure cause The cause of the failure (see Table 19) Subunit failed Name of subunit that failed (see examples in Annex A) Maintainable Item(s) failed Specify the failed maintainable item(s) (see examples in Annex A) Method of observation How the failure was detected (see Table 20) Additional information Give more details, if available, on the circumstances leading to the failure, additional information on failure cause etc. Failure data Re marks 11
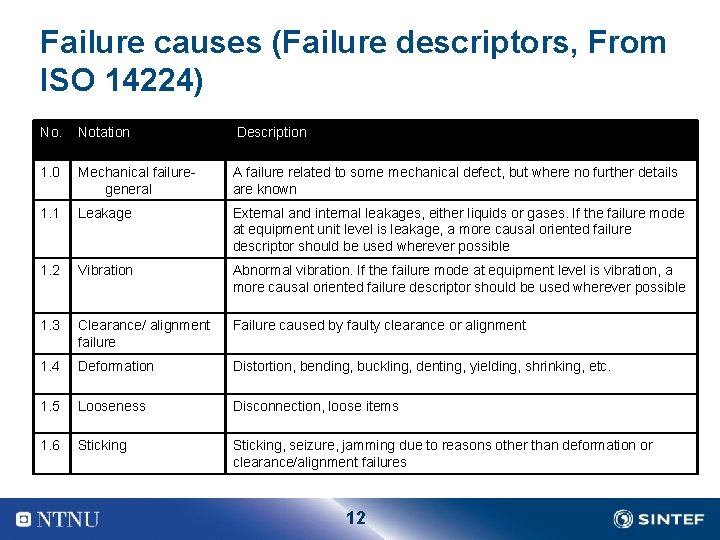
Failure causes (Failure descriptors, From ISO 14224) No. Notation Description 1. 0 Mechanical failure general A failure related to some mechanical defect, but where no further details are known 1. 1 Leakage External and internal leakages, either liquids or gases. If the failure mode at equipment unit level is leakage, a more causal oriented failure descriptor should be used wherever possible 1. 2 Vibration Abnormal vibration. If the failure mode at equipment level is vibration, a more causal oriented failure descriptor should be used wherever possible 1. 3 Clearance/ alignment failure Failure caused by faulty clearance or alignment 1. 4 Deformation Distortion, bending, buckling, denting, yielding, shrinking, etc. 1. 5 Looseness Disconnection, loose items 1. 6 Sticking, seizure, jamming due to reasons other than deformation or clearance/alignment failures 12
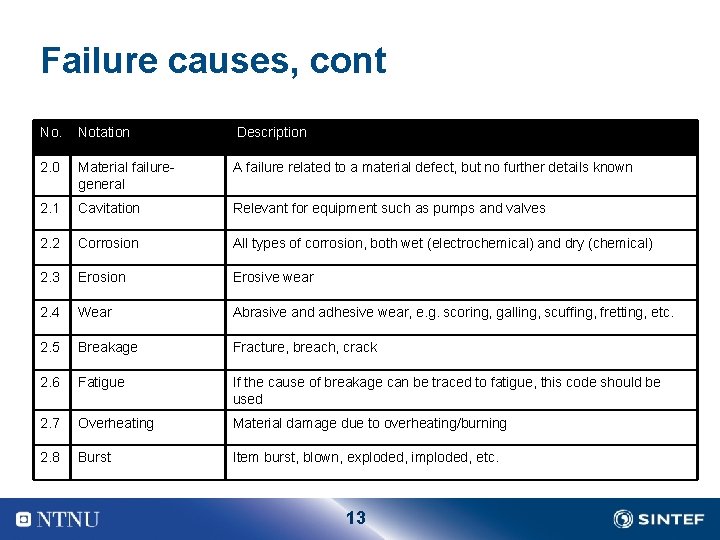
Failure causes, cont No. Notation Description 2. 0 Material failure general A failure related to a material defect, but no further details known 2. 1 Cavitation Relevant for equipment such as pumps and valves 2. 2 Corrosion All types of corrosion, both wet (electrochemical) and dry (chemical) 2. 3 Erosion Erosive wear 2. 4 Wear Abrasive and adhesive wear, e. g. scoring, galling, scuffing, fretting, etc. 2. 5 Breakage Fracture, breach, crack 2. 6 Fatigue If the cause of breakage can be traced to fatigue, this code should be used 2. 7 Overheating Material damage due to overheating/burning 2. 8 Burst Item burst, blown, exploded, imploded, etc. 13
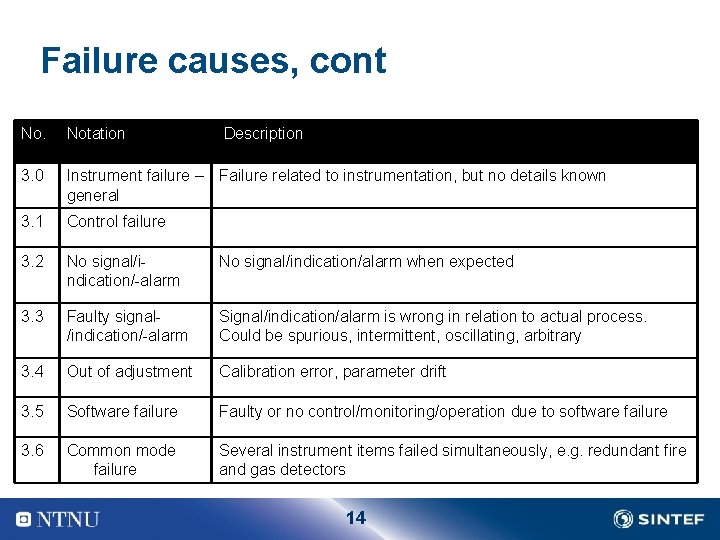
Failure causes, cont No. Notation Description 3. 0 Instrument failure – Failure related to instrumentation, but no details known general 3. 1 Control failure 3. 2 No signal/i ndication/ alarm No signal/indication/alarm when expected 3. 3 Faulty signal /indication/ alarm Signal/indication/alarm is wrong in relation to actual process. Could be spurious, intermittent, oscillating, arbitrary 3. 4 Out of adjustment Calibration error, parameter drift 3. 5 Software failure Faulty or no control/monitoring/operation due to software failure 3. 6 Common mode failure Several instrument items failed simultaneously, e. g. redundant fire and gas detectors 14
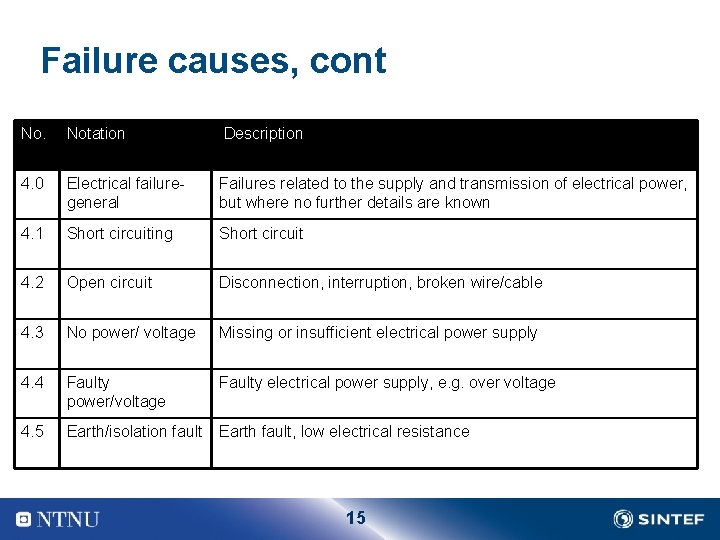
Failure causes, cont No. Notation Description 4. 0 Electrical failure general Failures related to the supply and transmission of electrical power, but where no further details are known 4. 1 Short circuiting Short circuit 4. 2 Open circuit Disconnection, interruption, broken wire/cable 4. 3 No power/ voltage Missing or insufficient electrical power supply 4. 4 Faulty power/voltage Faulty electrical power supply, e. g. over voltage 4. 5 Earth/isolation fault Earth fault, low electrical resistance 15
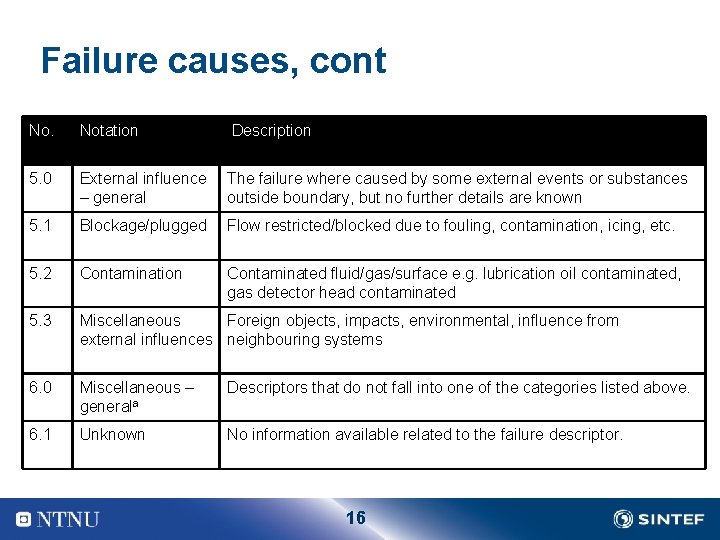
Failure causes, cont No. Notation Description 5. 0 External influence The failure where caused by some external events or substances – general outside boundary, but no further details are known 5. 1 Blockage/plugged Flow restricted/blocked due to fouling, contamination, icing, etc. 5. 2 Contamination Contaminated fluid/gas/surface e. g. lubrication oil contaminated, gas detector head contaminated 5. 3 Miscellaneous Foreign objects, impacts, environmental, influence from external influences neighbouring systems 6. 0 Miscellaneous – generala Descriptors that do not fall into one of the categories listed above. 6. 1 Unknown No information available related to the failure descriptor. 16
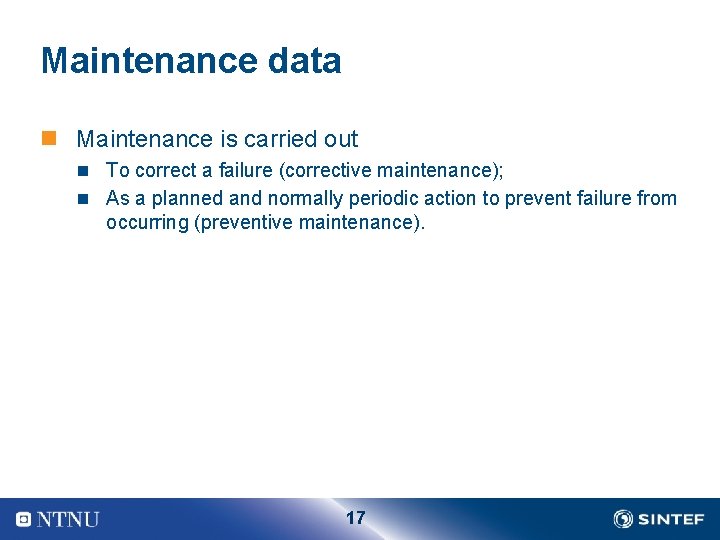
Maintenance data n Maintenance is carried out n To correct a failure (corrective maintenance); n As a planned and normally periodic action to prevent failure from occurring (preventive maintenance). 17
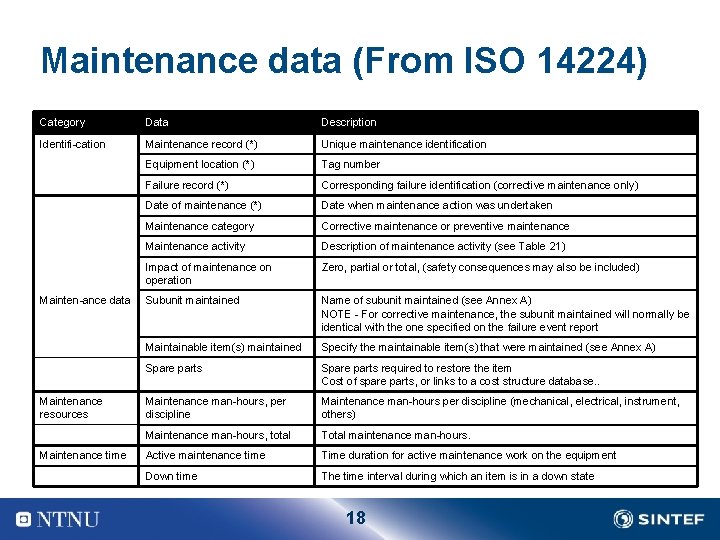
Maintenance data (From ISO 14224) Category Data Description Identifi cation Maintenance record (*) Unique maintenance identification Equipment location (*) Tag number Failure record (*) Corresponding failure identification (corrective maintenance only) Date of maintenance (*) Date when maintenance action was undertaken Maintenance category Corrective maintenance or preventive maintenance Maintenance activity Description of maintenance activity (see Table 21) Impact of maintenance on operation Zero, partial or total, (safety consequences may also be included) Subunit maintained Name of subunit maintained (see Annex A) NOTE For corrective maintenance, the subunit maintained will normally be identical with the one specified on the failure event report Maintainable item(s) maintained Specify the maintainable item(s) that were maintained (see Annex A) Spare parts required to restore the item Cost of spare parts, or links to a cost structure database. . Maintenance man hours, per discipline Maintenance man hours per discipline (mechanical, electrical, instrument, others) Maintenance man hours, total Total maintenance man hours. Active maintenance time Time duration for active maintenance work on the equipment Down time The time interval during which an item is in a down state Mainten ance data Maintenance resources Maintenance time 18
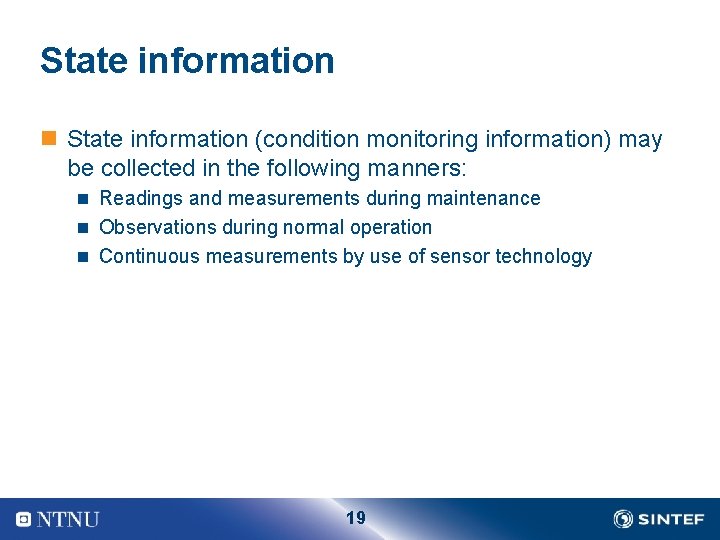
State information n State information (condition monitoring information) may be collected in the following manners: n Readings and measurements during maintenance n Observations during normal operation n Continuous measurements by use of sensor technology 19
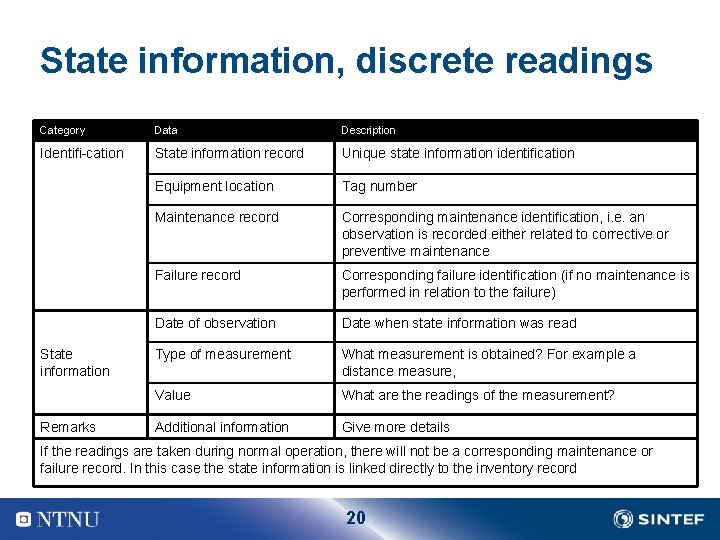
State information, discrete readings Category Data Description Identifi cation State information record Unique state information identification Equipment location Tag number Maintenance record Corresponding maintenance identification, i. e. an observation is recorded either related to corrective or preventive maintenance Failure record Corresponding failure identification (if no maintenance is performed in relation to the failure) Date of observation Date when state information was read Type of measurement What measurement is obtained? For example a distance measure, Value What are the readings of the measurement? Additional information Give more details State information Remarks If the readings are taken during normal operation, there will not be a corresponding maintenance or failure record. In this case the state information is linked directly to the inventory record 20
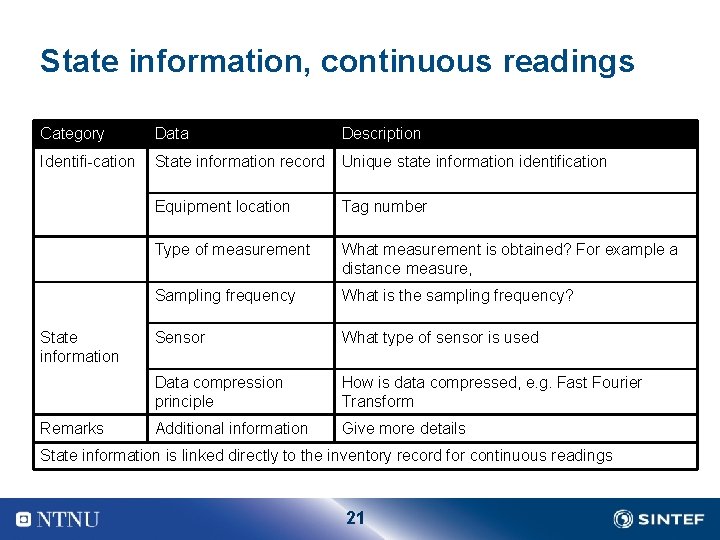
State information, continuous readings Category Data Description Identifi cation State information record Unique state information identification Equipment location Tag number Type of measurement What measurement is obtained? For example a distance measure, Sampling frequency What is the sampling frequency? Sensor What type of sensor is used Data compression principle How is data compressed, e. g. Fast Fourier Transform Additional information Give more details State information Remarks State information is linked directly to the inventory record for continuous readings 21
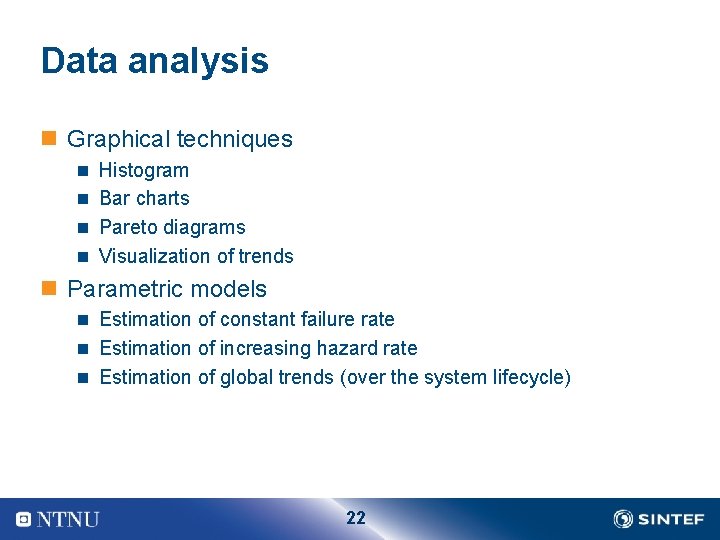
Data analysis n Graphical techniques n Histogram n Bar charts n Pareto diagrams n Visualization of trends n Parametric models n Estimation of constant failure rate n Estimation of increasing hazard rate n Estimation of global trends (over the system lifecycle) 22
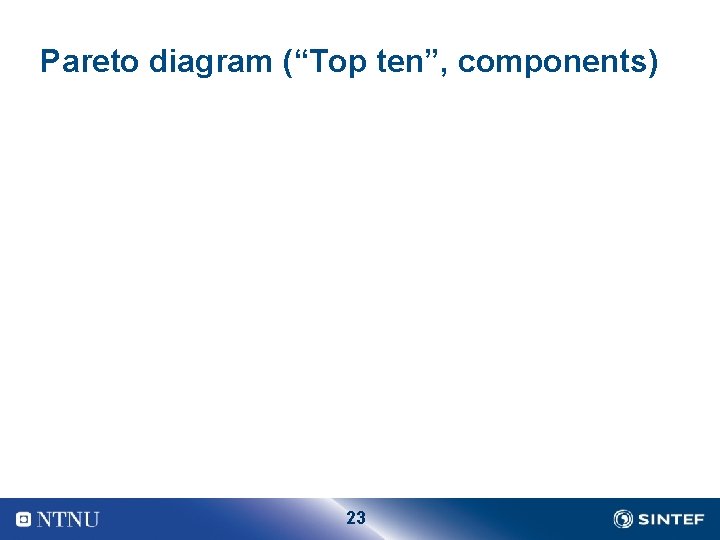
Pareto diagram (“Top ten”, components) 23
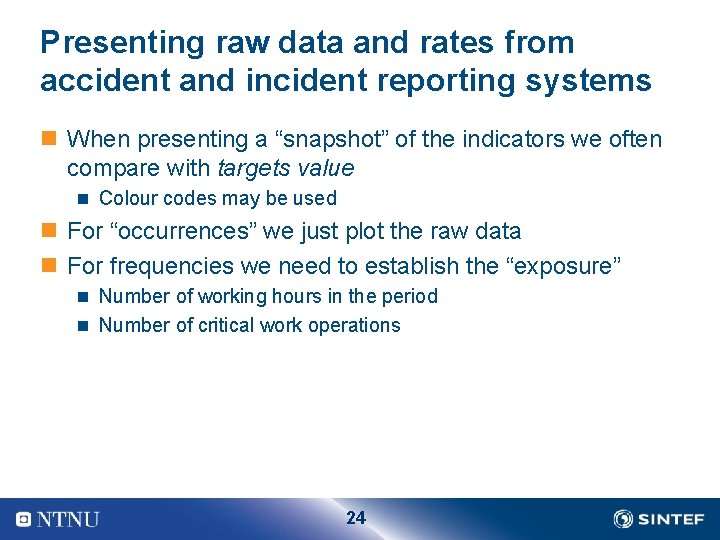
Presenting raw data and rates from accident and incident reporting systems n When presenting a “snapshot” of the indicators we often compare with targets value n Colour codes may be used n For “occurrences” we just plot the raw data n For frequencies we need to establish the “exposure” n Number of working hours in the period n Number of critical work operations 24
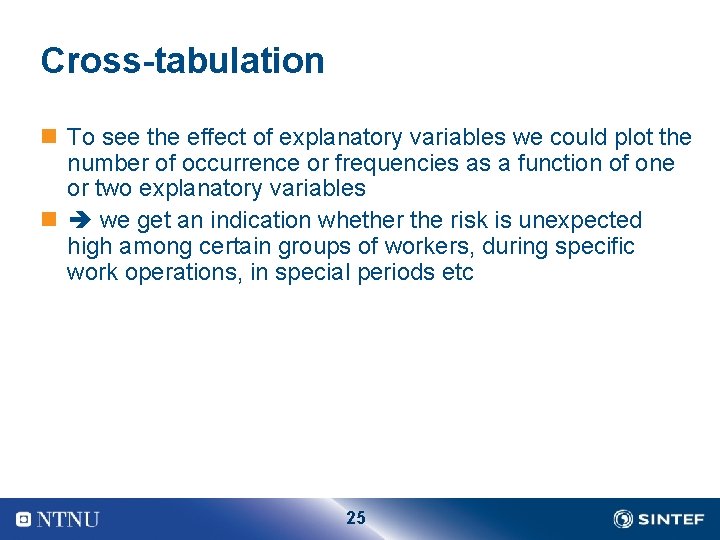
Cross-tabulation n To see the effect of explanatory variables we could plot the number of occurrence or frequencies as a function of one or two explanatory variables n we get an indication whether the risk is unexpected high among certain groups of workers, during specific work operations, in special periods etc 25
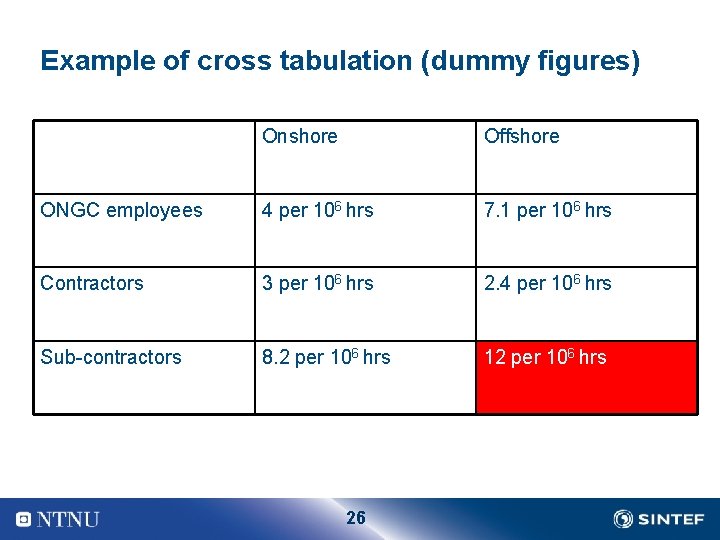
Example of cross tabulation (dummy figures) Onshore Offshore ONGC employees 4 per 106 hrs 7. 1 per 106 hrs Contractors 3 per 106 hrs 2. 4 per 106 hrs Sub contractors 8. 2 per 106 hrs 12 per 106 hrs 26
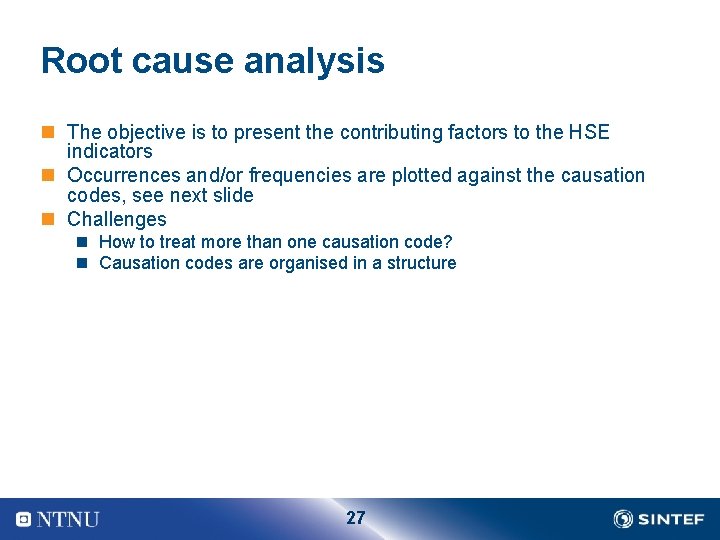
Root cause analysis n The objective is to present the contributing factors to the HSE indicators n Occurrences and/or frequencies are plotted against the causation codes, see next slide n Challenges n How to treat more than one causation code? n Causation codes are organised in a structure 27
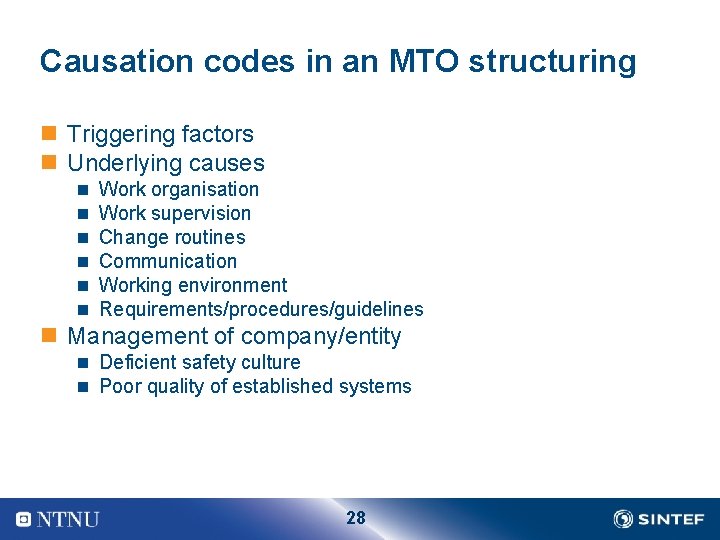
Causation codes in an MTO structuring n Triggering factors n Underlying causes n n n Work organisation Work supervision Change routines Communication Working environment Requirements/procedures/guidelines n Management of company/entity n Deficient safety culture n Poor quality of established systems 28
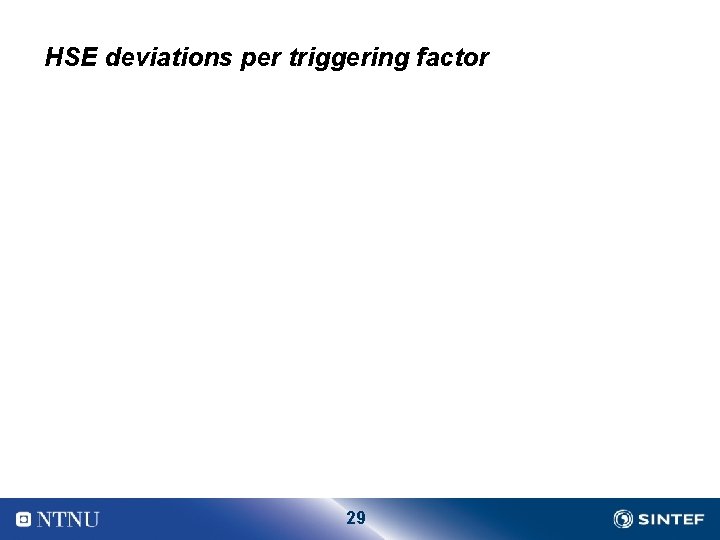
HSE deviations per triggering factor 29
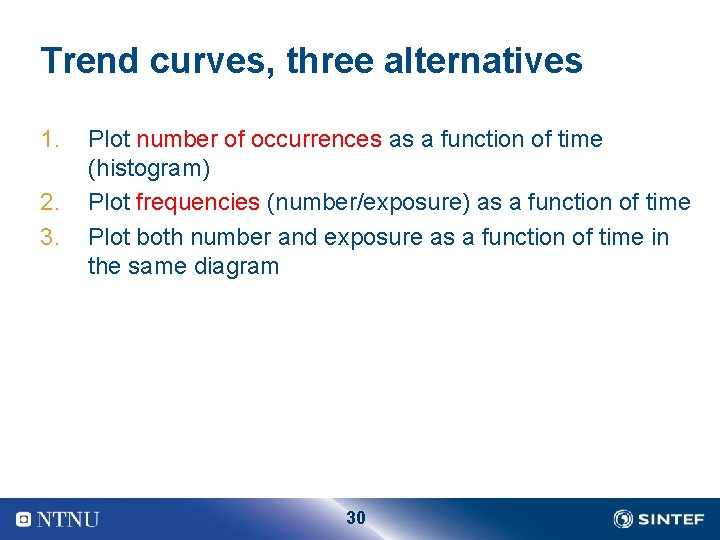
Trend curves, three alternatives 1. 2. 3. Plot number of occurrences as a function of time (histogram) Plot frequencies (number/exposure) as a function of time Plot both number and exposure as a function of time in the same diagram 30
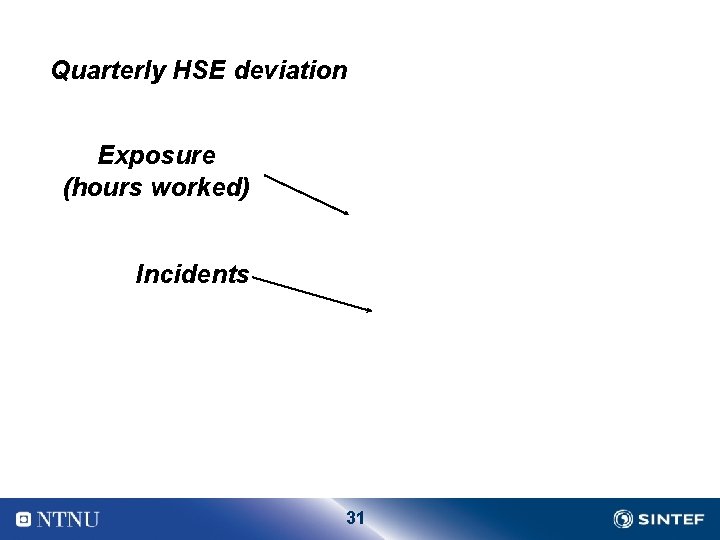
Quarterly HSE deviation Exposure (hours worked) Incidents 31
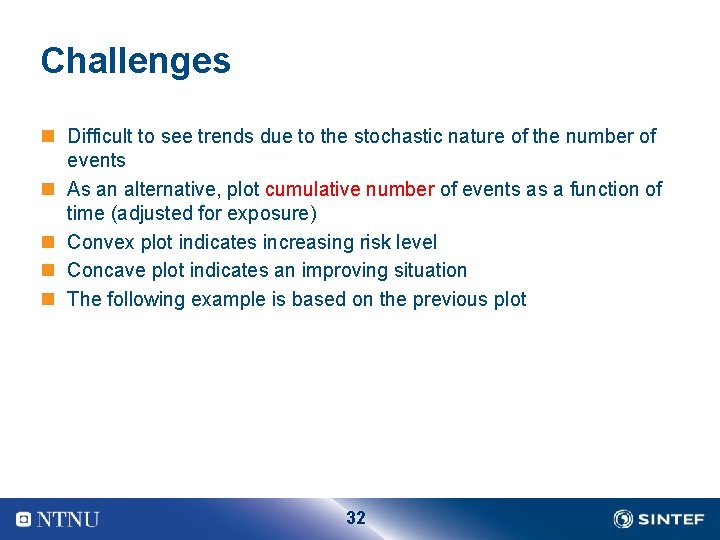
Challenges n Difficult to see trends due to the stochastic nature of the number of events n As an alternative, plot cumulative number of events as a function of time (adjusted for exposure) n Convex plot indicates increasing risk level n Concave plot indicates an improving situation n The following example is based on the previous plot 32
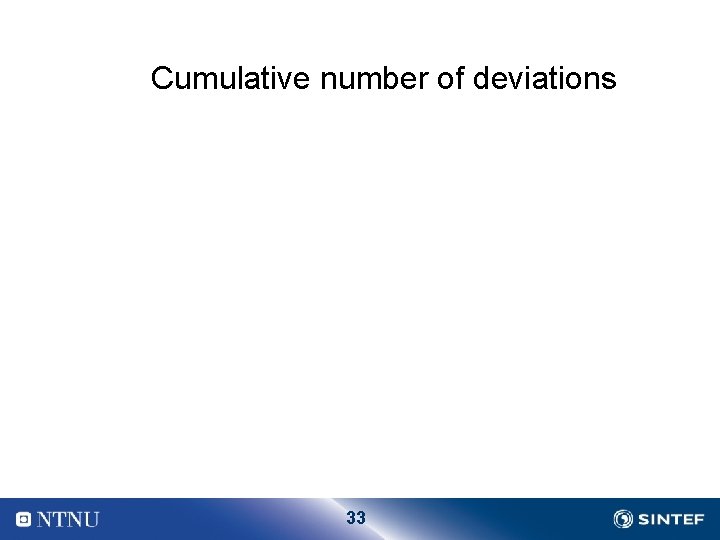
Cumulative number of deviations 33
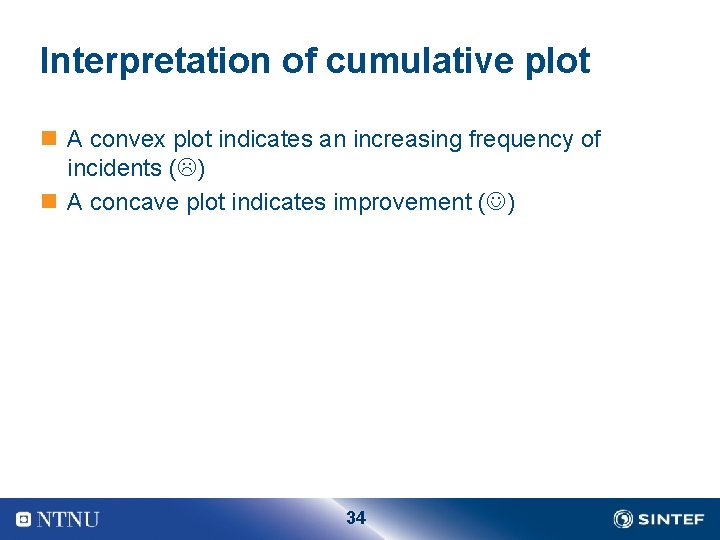
Interpretation of cumulative plot n A convex plot indicates an increasing frequency of incidents ( ) n A concave plot indicates improvement ( ) 34
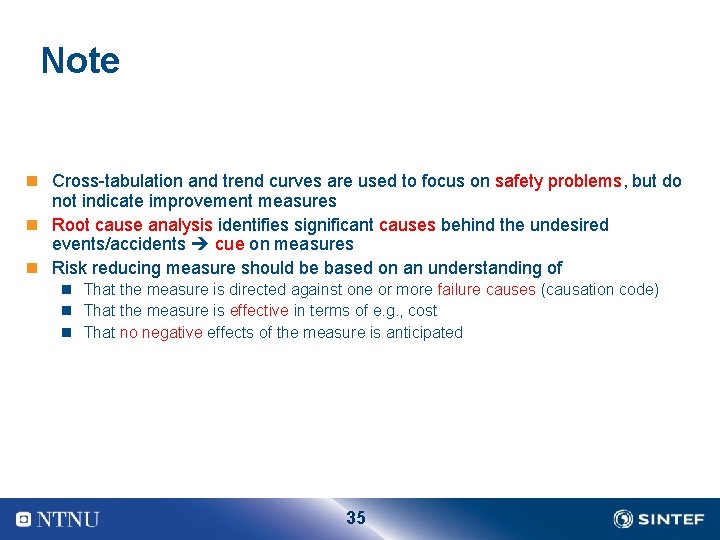
Note n Cross tabulation and trend curves are used to focus on safety problems, but do not indicate improvement measures n Root cause analysis identifies significant causes behind the undesired events/accidents cue on measures n Risk reducing measure should be based on an understanding of n That the measure is directed against one or more failure causes (causation code) n That the measure is effective in terms of e. g. , cost n That no negative effects of the measure is anticipated 35
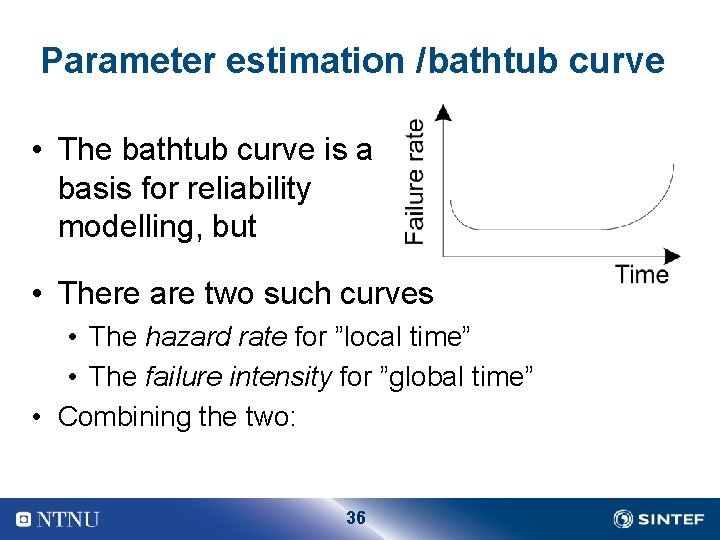
Parameter estimation /bathtub curve • The bathtub curve is a basis for reliability modelling, but • There are two such curves • The hazard rate for ”local time” • The failure intensity for ”global time” • Combining the two: 36
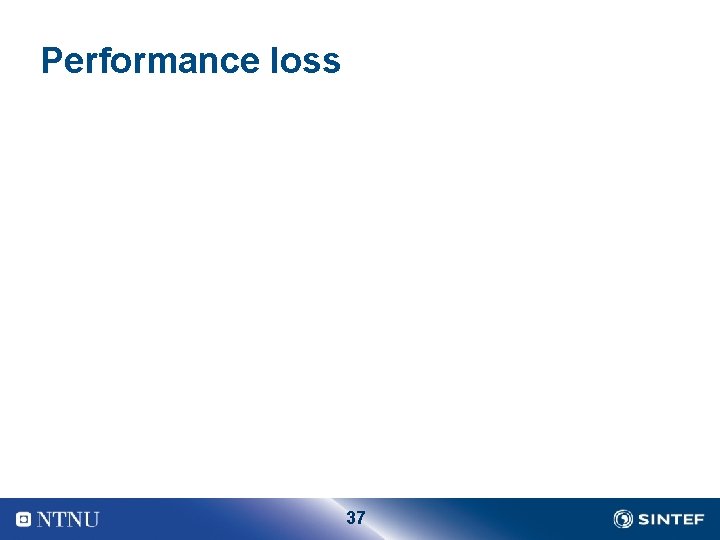
Performance loss 37
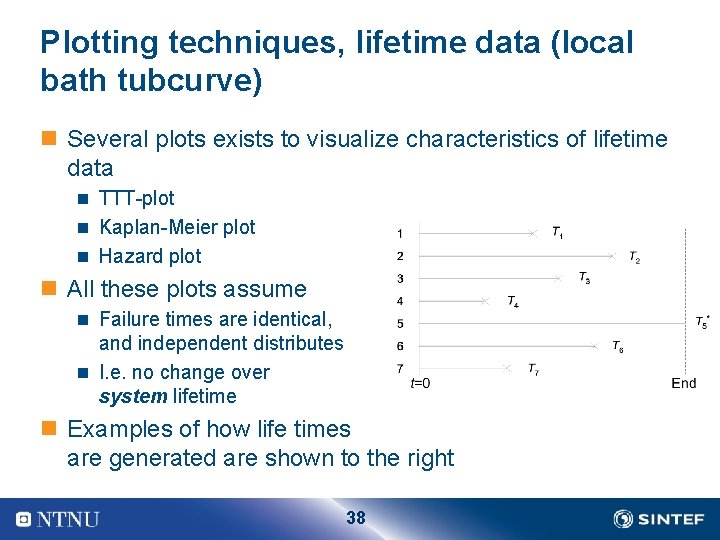
Plotting techniques, lifetime data (local bath tubcurve) n Several plots exists to visualize characteristics of lifetime data n TTT plot n Kaplan Meier plot n Hazard plot n All these plots assume n Failure times are identical, and independent distributes n I. e. no change over system lifetime n Examples of how life times are generated are shown to the right 38
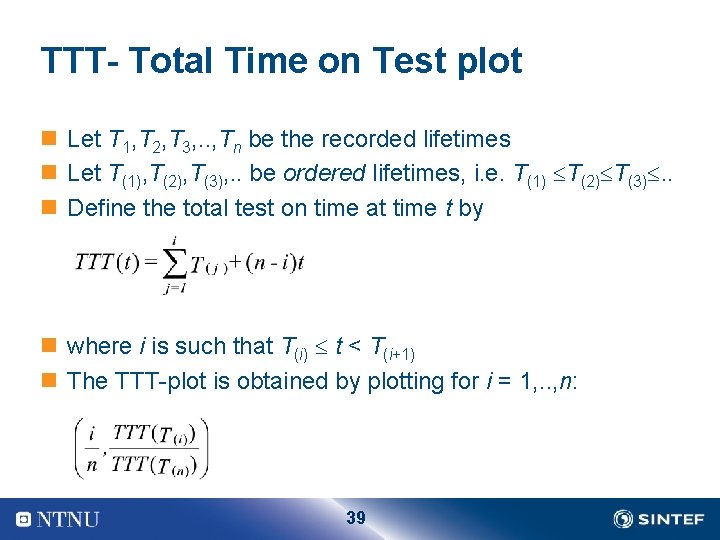
TTT- Total Time on Test plot n Let T 1, T 2, T 3, . . , Tn be the recorded lifetimes n Let T(1), T(2), T(3), . . be ordered lifetimes, i. e. T(1) T(2) T(3). . n Define the total test on time at time t by n where i is such that T(i) t < T(i+1) n The TTT plot is obtained by plotting for i = 1, . . , n: 39
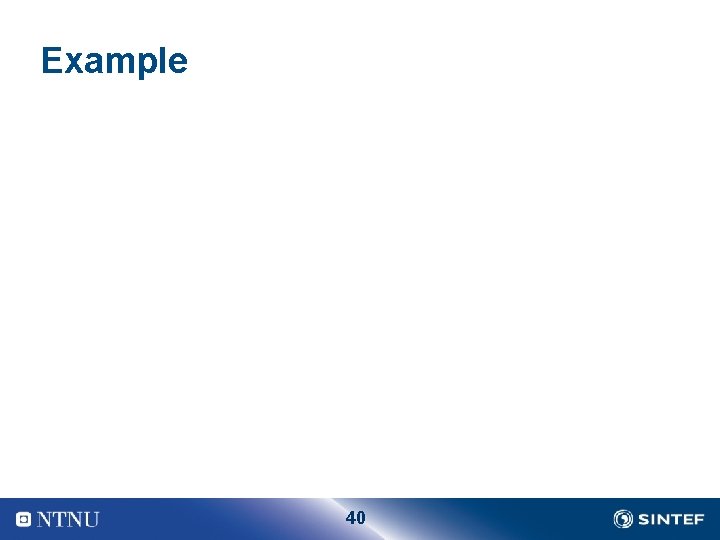
Example 40
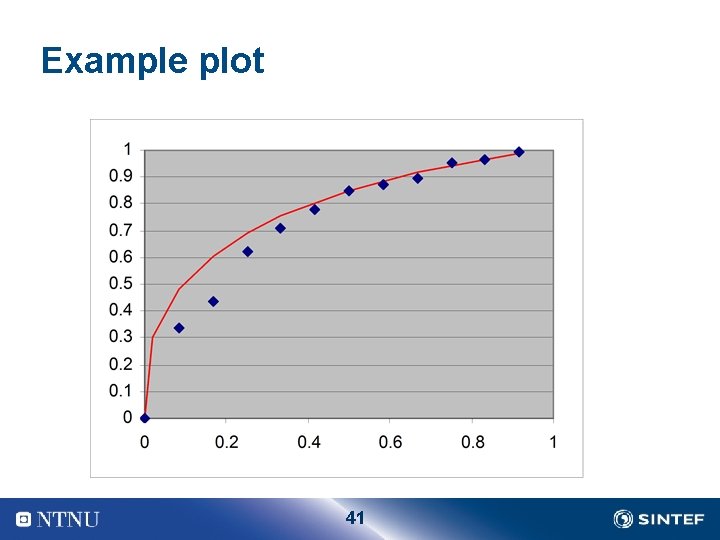
Example plot 41
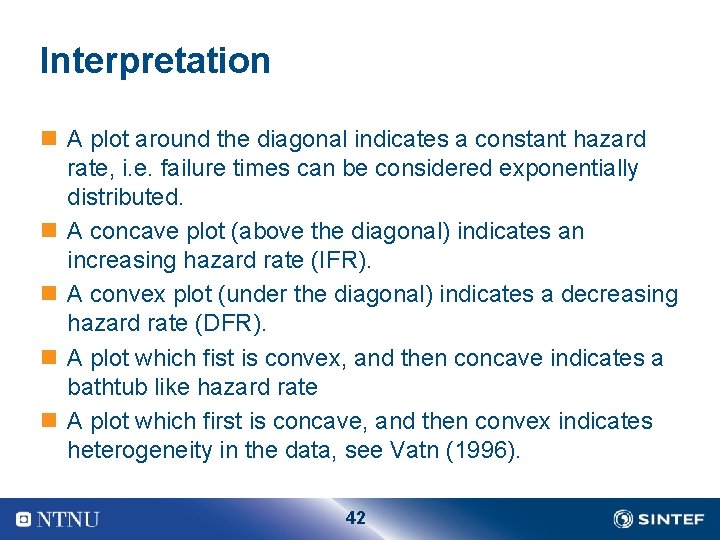
Interpretation n A plot around the diagonal indicates a constant hazard rate, i. e. failure times can be considered exponentially distributed. n A concave plot (above the diagonal) indicates an increasing hazard rate (IFR). n A convex plot (under the diagonal) indicates a decreasing hazard rate (DFR). n A plot which fist is convex, and then concave indicates a bathtub like hazard rate n A plot which first is concave, and then convex indicates heterogeneity in the data, see Vatn (1996). 42
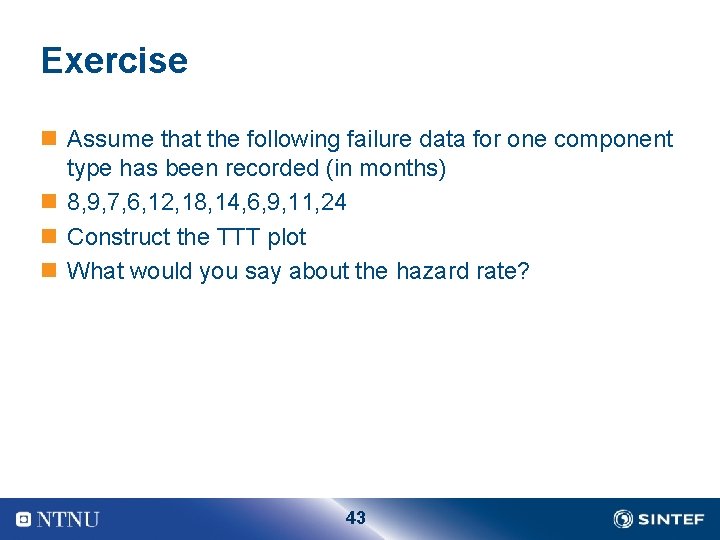
Exercise n Assume that the following failure data for one component type has been recorded (in months) n 8, 9, 7, 6, 12, 18, 14, 6, 9, 11, 24 n Construct the TTT plot n What would you say about the hazard rate? 43
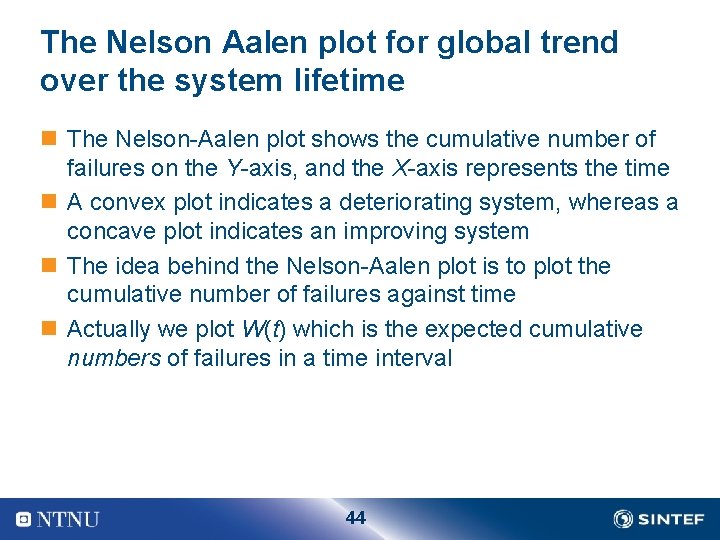
The Nelson Aalen plot for global trend over the system lifetime n The Nelson Aalen plot shows the cumulative number of failures on the Y axis, and the X axis represents the time n A convex plot indicates a deteriorating system, whereas a concave plot indicates an improving system n The idea behind the Nelson Aalen plot is to plot the cumulative number of failures against time n Actually we plot W(t) which is the expected cumulative numbers of failures in a time interval 44
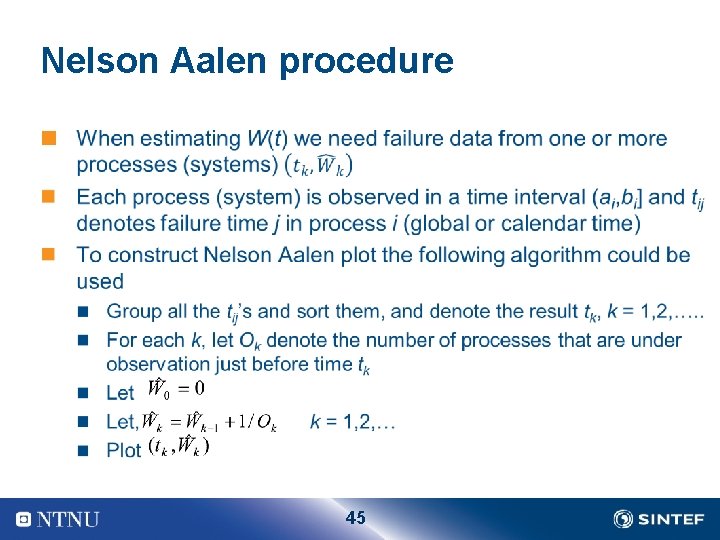
Nelson Aalen procedure n 45
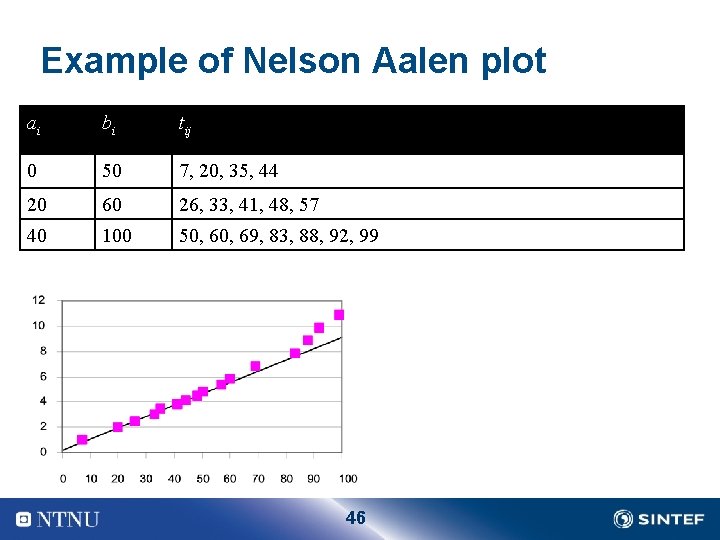
Example of Nelson Aalen plot ai bi tij 0 50 7, 20, 35, 44 20 60 26, 33, 41, 48, 57 40 100 50, 69, 83, 88, 92, 99 46
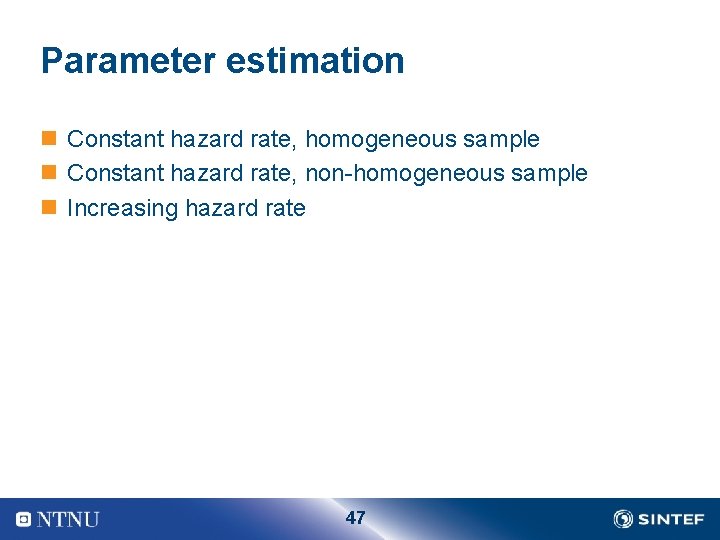
Parameter estimation n Constant hazard rate, homogeneous sample n Constant hazard rate, non homogeneous sample n Increasing hazard rate 47
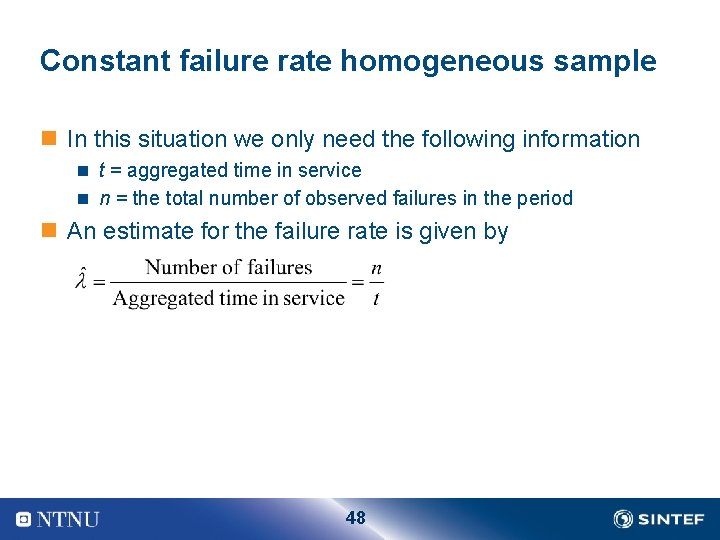
Constant failure rate homogeneous sample n In this situation we only need the following information n t = aggregated time in service n n = the total number of observed failures in the period n An estimate for the failure rate is given by 48
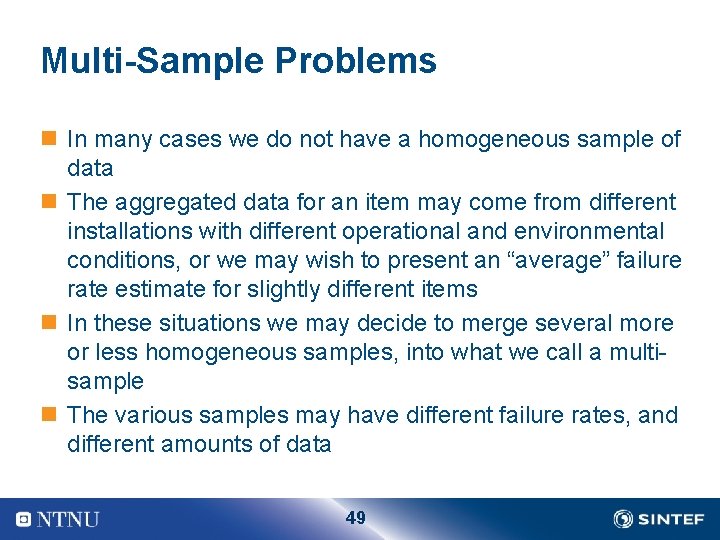
Multi-Sample Problems n In many cases we do not have a homogeneous sample of data n The aggregated data for an item may come from different installations with different operational and environmental conditions, or we may wish to present an “average” failure rate estimate for slightly different items n In these situations we may decide to merge several more or less homogeneous samples, into what we call a multi sample n The various samples may have different failure rates, and different amounts of data 49
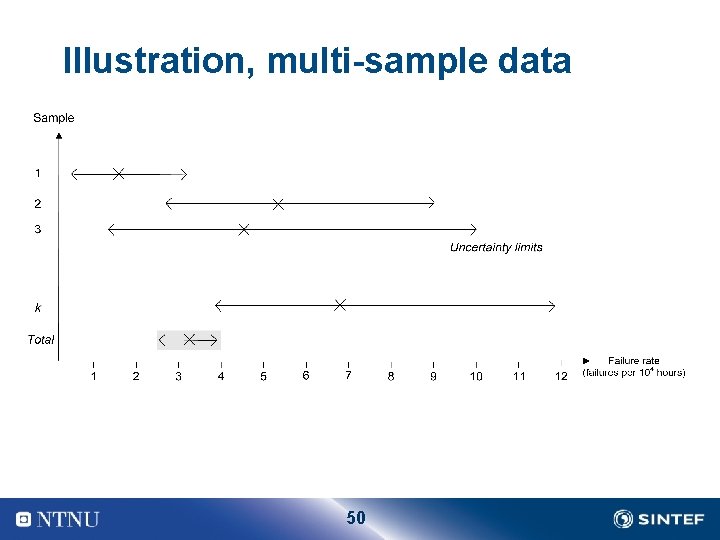
Illustration, multi-sample data 50
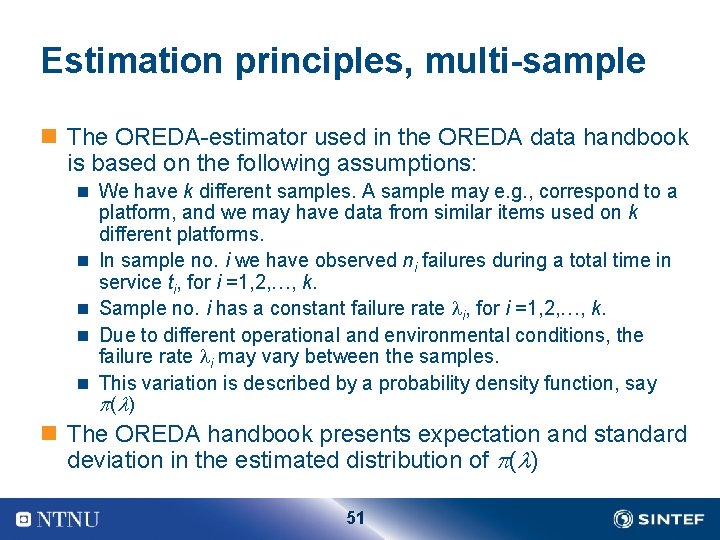
Estimation principles, multi-sample n The OREDA estimator used in the OREDA data handbook is based on the following assumptions: n We have k different samples. A sample may e. g. , correspond to a n n platform, and we may have data from similar items used on k different platforms. In sample no. i we have observed ni failures during a total time in service ti, for i =1, 2, …, k. Sample no. i has a constant failure rate i, for i =1, 2, …, k. Due to different operational and environmental conditions, the failure rate i may vary between the samples. This variation is described by a probability density function, say ( ) n The OREDA handbook presents expectation and standard deviation in the estimated distribution of ( ) 51
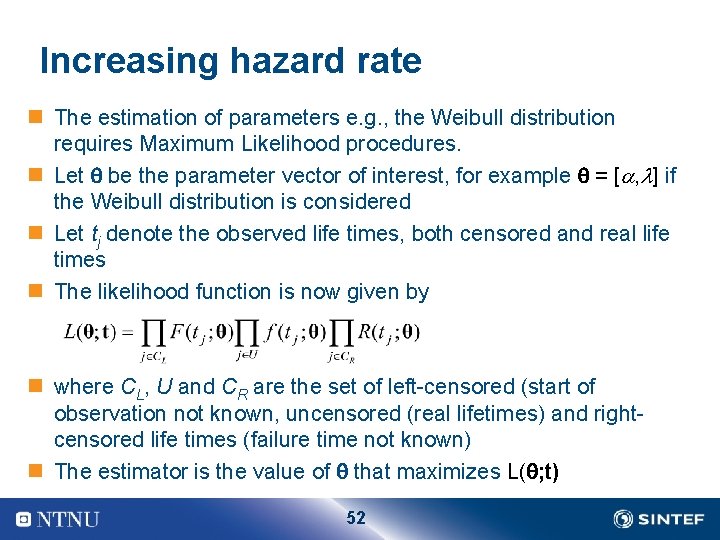
Increasing hazard rate n The estimation of parameters e. g. , the Weibull distribution requires Maximum Likelihood procedures. n Let be the parameter vector of interest, for example = [ , ] if the Weibull distribution is considered n Let tj denote the observed life times, both censored and real life times n The likelihood function is now given by n where CL, U and CR are the set of left censored (start of observation not known, uncensored (real lifetimes) and right censored life times (failure time not known) n The estimator is the value of that maximizes L( ; t) 52
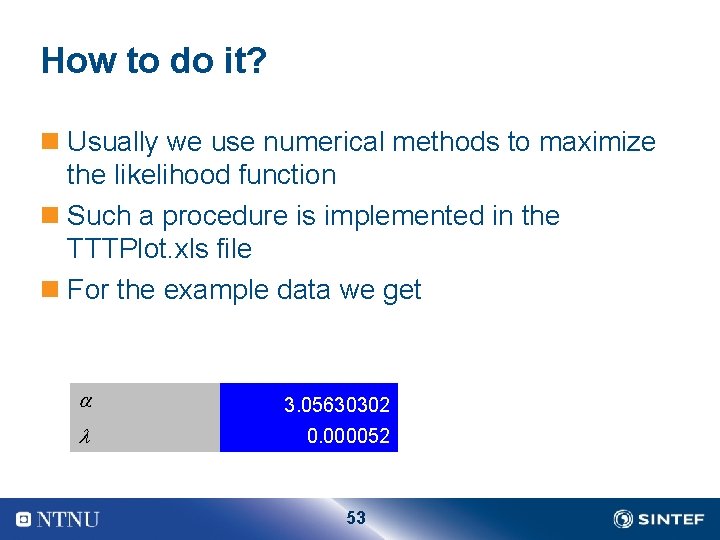
How to do it? n Usually we use numerical methods to maximize the likelihood function n Such a procedure is implemented in the TTTPlot. xls file n For the example data we get 3. 05630302 0. 000052 53
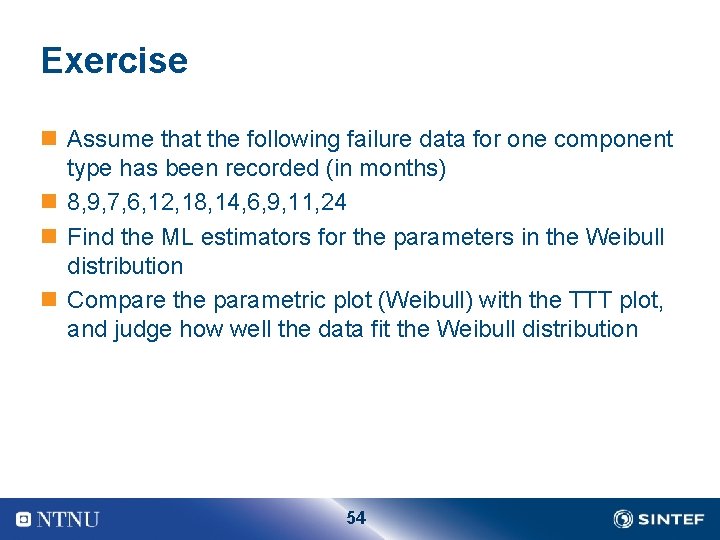
Exercise n Assume that the following failure data for one component type has been recorded (in months) n 8, 9, 7, 6, 12, 18, 14, 6, 9, 11, 24 n Find the ML estimators for the parameters in the Weibull distribution n Compare the parametric plot (Weibull) with the TTT plot, and judge how well the data fit the Weibull distribution 54
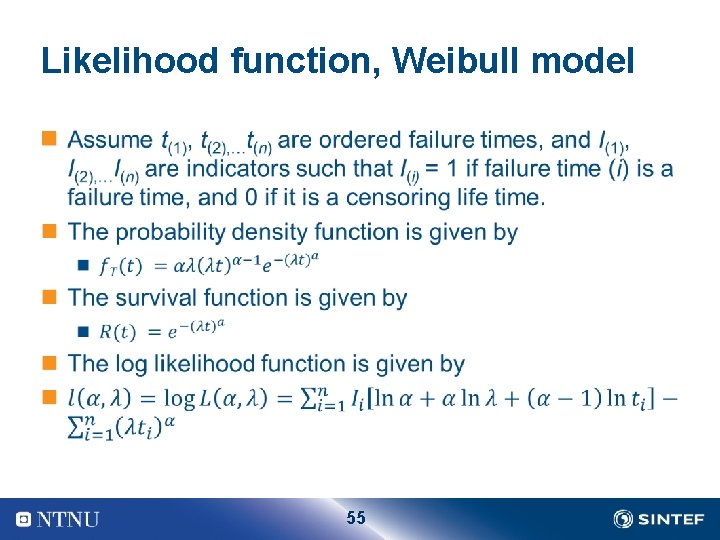
Likelihood function, Weibull model n 55
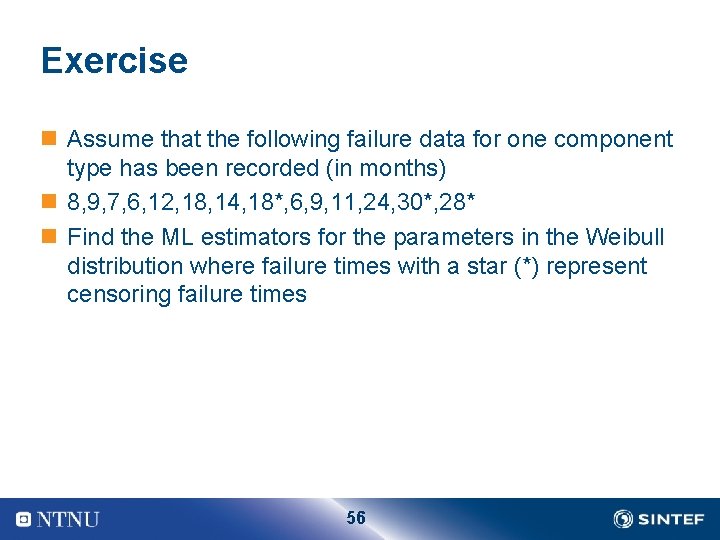
Exercise n Assume that the following failure data for one component type has been recorded (in months) n 8, 9, 7, 6, 12, 18, 14, 18*, 6, 9, 11, 24, 30*, 28* n Find the ML estimators for the parameters in the Weibull distribution where failure times with a star (*) represent censoring failure times 56
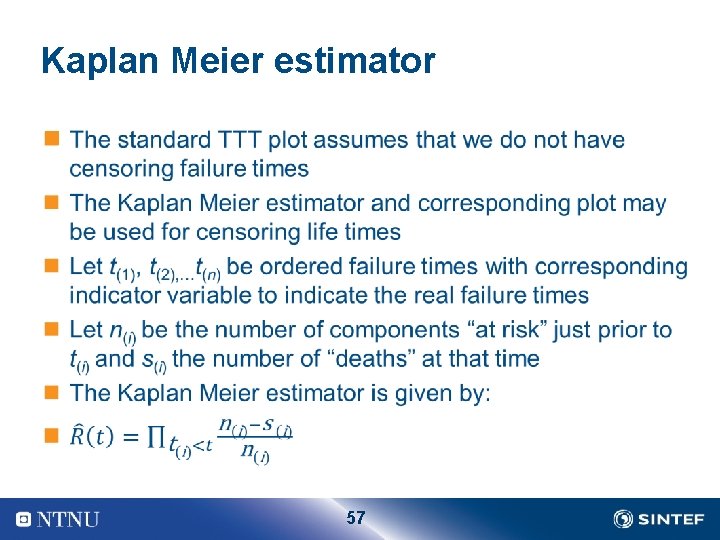
Kaplan Meier estimator n 57
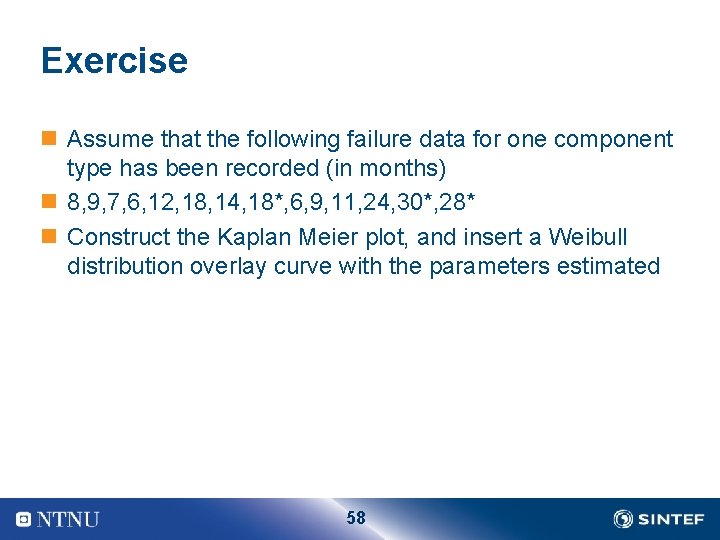
Exercise n Assume that the following failure data for one component type has been recorded (in months) n 8, 9, 7, 6, 12, 18, 14, 18*, 6, 9, 11, 24, 30*, 28* n Construct the Kaplan Meier plot, and insert a Weibull distribution overlay curve with the parameters estimated 58
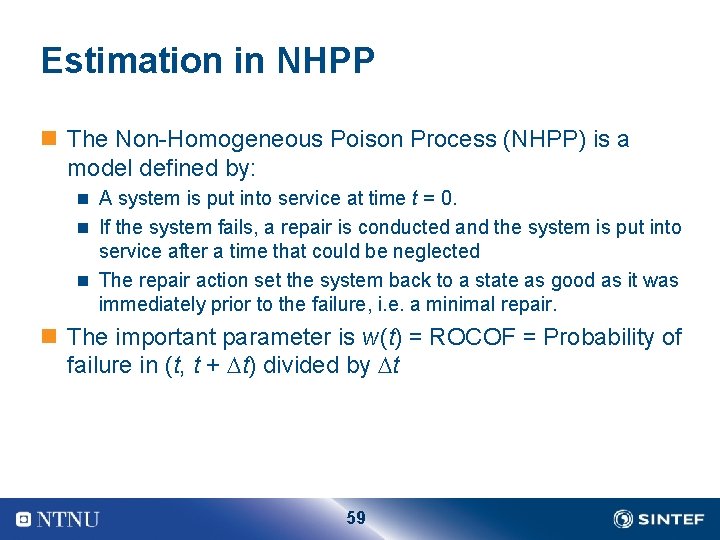
Estimation in NHPP n The Non Homogeneous Poison Process (NHPP) is a model defined by: n A system is put into service at time t = 0. n If the system fails, a repair is conducted and the system is put into service after a time that could be neglected n The repair action set the system back to a state as good as it was immediately prior to the failure, i. e. a minimal repair. n The important parameter is w(t) = ROCOF = Probability of failure in (t, t + t) divided by t 59
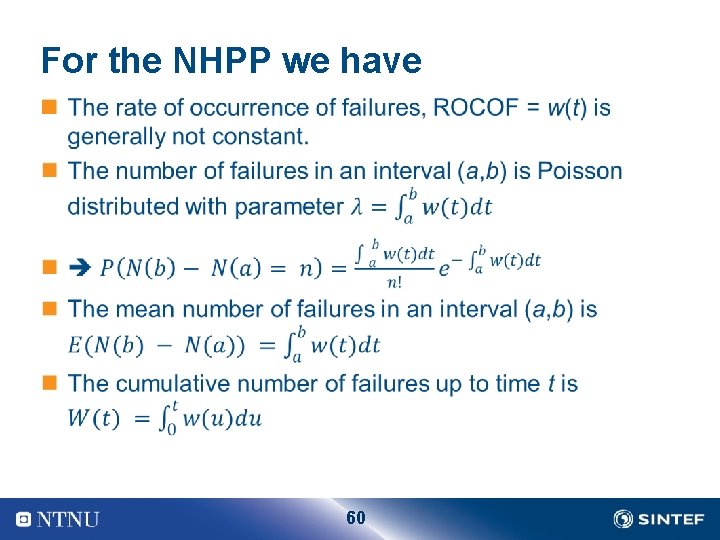
For the NHPP we have n 60
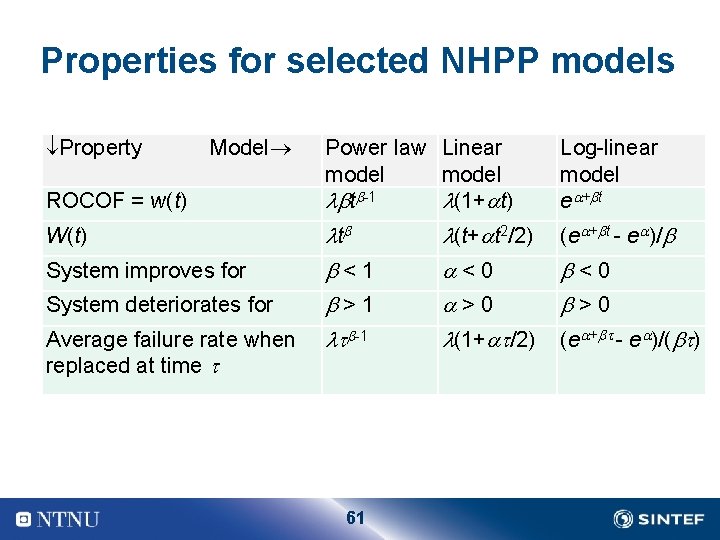
Properties for selected NHPP models Property Model ROCOF = w(t) W(t) System improves for System deteriorates for Average failure rate when replaced at time Power law Linear model t 1 (1+ t) Log linear model e + t t < 1 > 1 (e + t e )/ 1 61 (t+ t 2/2) < 0 > 0 (1+ /2) < 0 > 0 (e + e )/( )
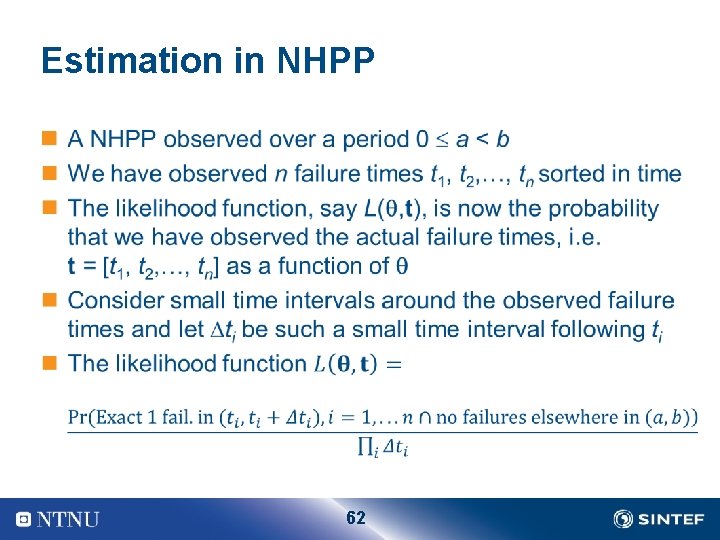
Estimation in NHPP n 62
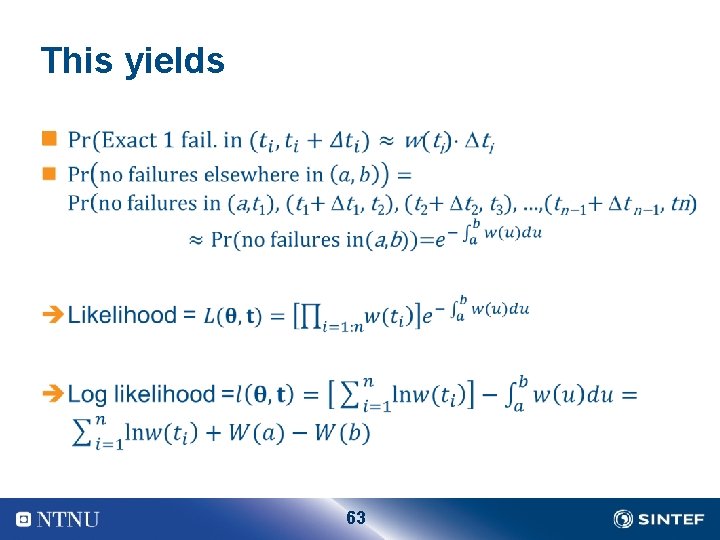
This yields n 63
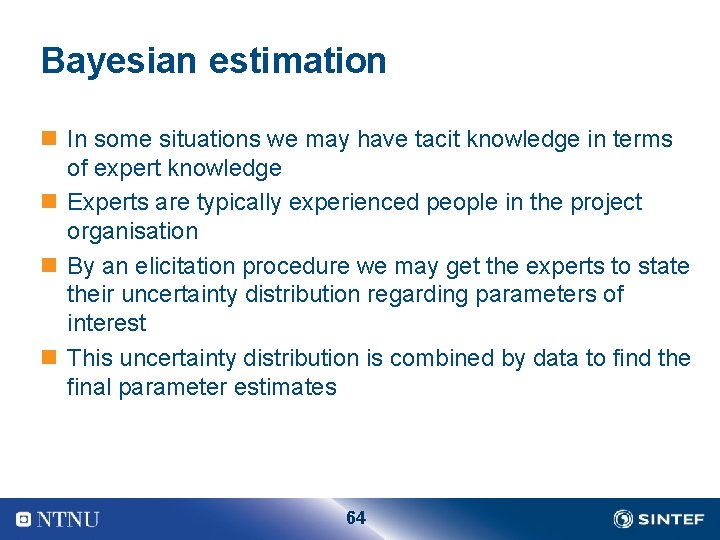
Bayesian estimation n In some situations we may have tacit knowledge in terms of expert knowledge n Experts are typically experienced people in the project organisation n By an elicitation procedure we may get the experts to state their uncertainty distribution regarding parameters of interest n This uncertainty distribution is combined by data to find the final parameter estimates 64
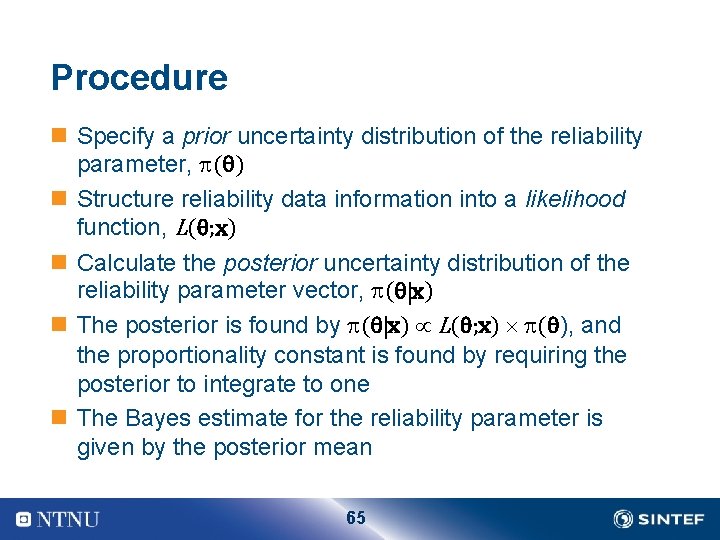
Procedure n Specify a prior uncertainty distribution of the reliability parameter, ( ) n Structure reliability data information into a likelihood function, L( ; x) n Calculate the posterior uncertainty distribution of the reliability parameter vector, ( x) n The posterior is found by ( x) L( ; x) ( ), and the proportionality constant is found by requiring the posterior to integrate to one n The Bayes estimate for the reliability parameter is given by the posterior mean 65
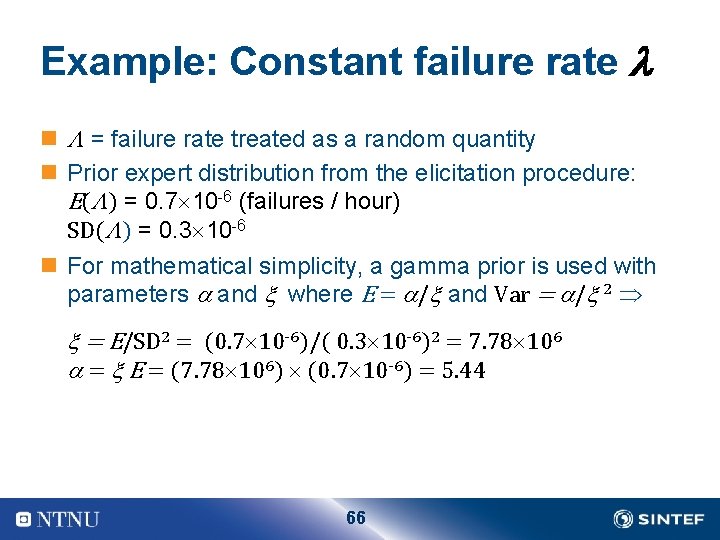
Example: Constant failure rate n = failure rate treated as a random quantity n Prior expert distribution from the elicitation procedure: E( ) = 0. 7 10 6 (failures / hour) SD( ) = 0. 3 10 6 n For mathematical simplicity, a gamma prior is used with parameters and where E = / and Var = / 2 = E/SD 2 = (0. 7 10 -6)/( 0. 3 10 -6)2 = 7. 78 106 = E = (7. 78 106) (0. 7 10 -6) = 5. 44 66
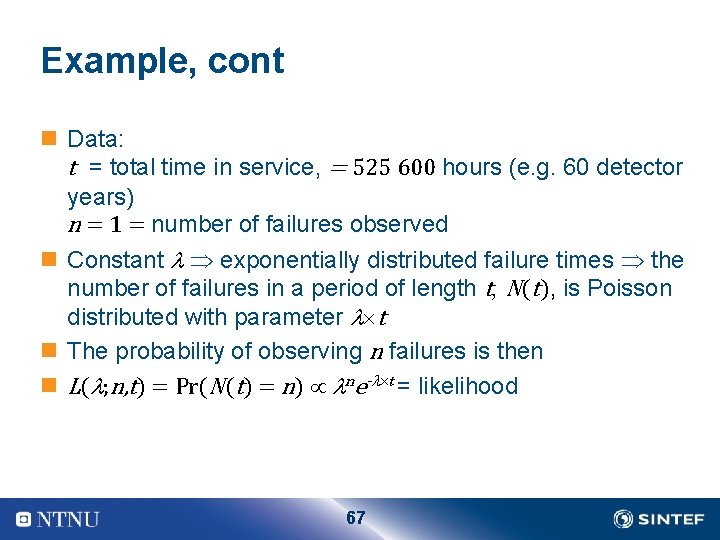
Example, cont n Data: t = total time in service, = 525 600 hours (e. g. 60 detector years) n = 1 = number of failures observed n Constant exponentially distributed failure times the number of failures in a period of length t, N(t), is Poisson distributed with parameter t n The probability of observing n failures is then n L( ; n, t) = Pr(N(t) = n) ne- t = likelihood 67
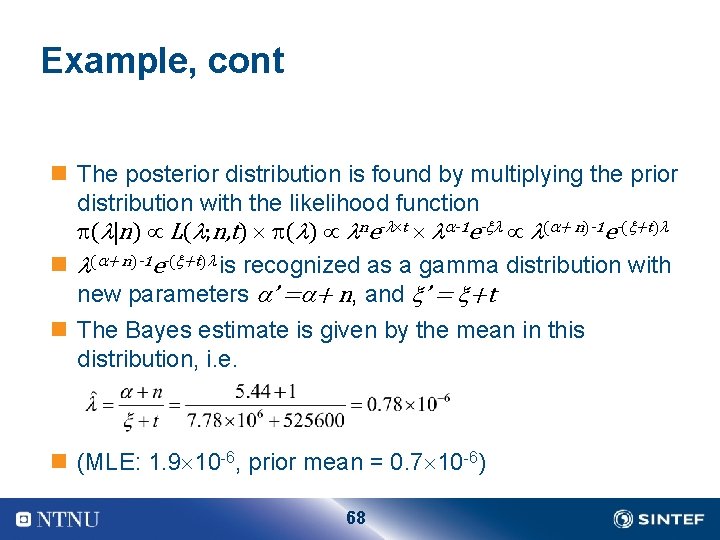
Example, cont n The posterior distribution is found by multiplying the prior distribution with the likelihood function ( n) L( ; n, t) ( ) ne- t -1 e- ( + n)-1 e-( +t) n ( + n)-1 e-( +t) is recognized as a gamma distribution with new parameters ’ = + n, and ’ = +t n The Bayes estimate is given by the mean in this distribution, i. e. n (MLE: 1. 9 10 6, prior mean = 0. 7 10 6) 68
Jørn vatn
Jørn vatn
Jørn vatn
Data collection procedure
Collection and analysis of rate data
Data collection management and analysis
Data analysis research example
Landsat collection 1 vs collection 2
Documentary collection definition
Collection analysis dissemination of data
The terms external secondary data and syndicated
Fire and arson investigation ppt
Database development adalah
Methods of data organization and presentation
Interrelated collection of data
Data entry in gis
Data are raw facts.
What is the primary data
Data preparation and basic data analysis
Data acquisition and data analysis
Ntnu chemical engineering
Opptakskontoret ntnu
Math ntnu
Ntnu stoffkartotek
Ssiim
Ntnu forkurs realfag
Folk.ntnu.no
Bygg og anlegg ntnu
Timeplan medisin ntnu
Ntnu powerpoint template
Realfagsbiblioteket ntnu
Math
Ntnu eurocard
Ntnu
Paga ntnu
Ntnu.no
Rakesh kumar ntnu
Ntnu
Olga2000
Math ntnu
Ntnu.no
Ntnu.no
Ntnu
Ntnu vpn
Ntnu semesterplan
Asdfghjkløæ
Itslearning ntnu
Trondheim university erasmus
Ntnu
Ntnu stoffkartotek
Idi qa
Ntnu chemdraw
Mmi ntnu
Ntnu matlab
Qualitative and quantitative research
Tollgate review
Priority improvement areas
Finding answers through data collection
Sampoorna tc request
Data collection proposal
It is part where you indicate the research instrument
Research instruments example
Define data collection method
Phase 2 data
Ace curriculum autism
Pantry audits
Self report method of data collection
Https://pfs.ine.es/iriaportal/
Network data collection at slac