DATA ASSIMILATION AND MODEL ERROR ESTIMATION Dusanka Zupanski
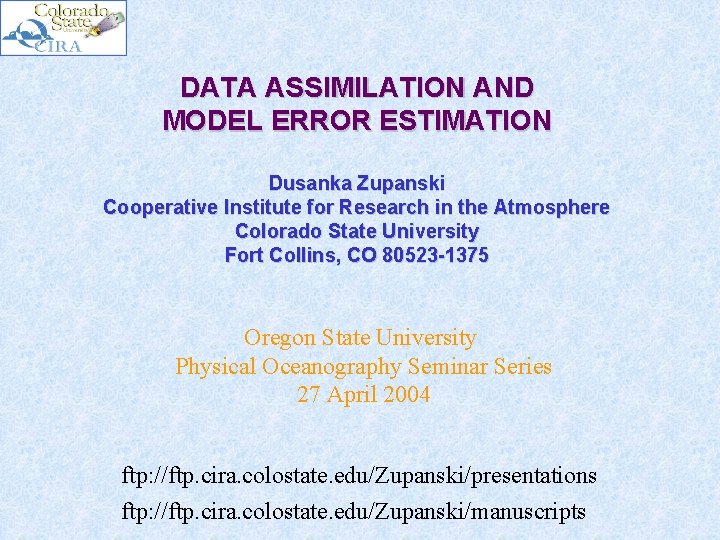
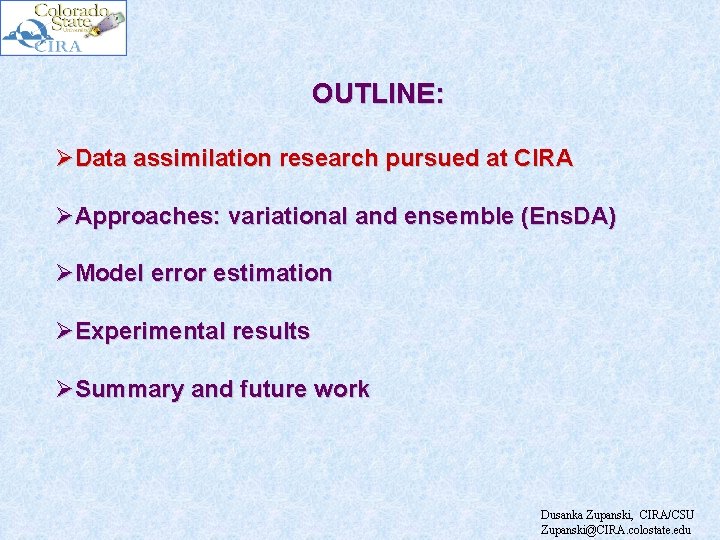
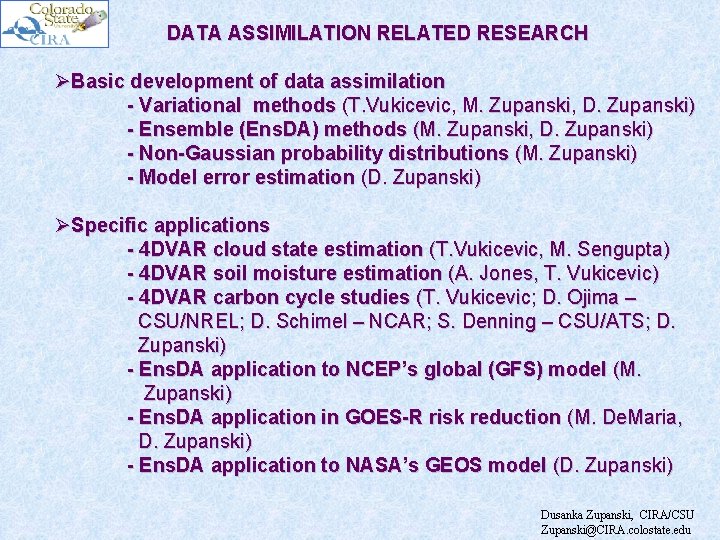
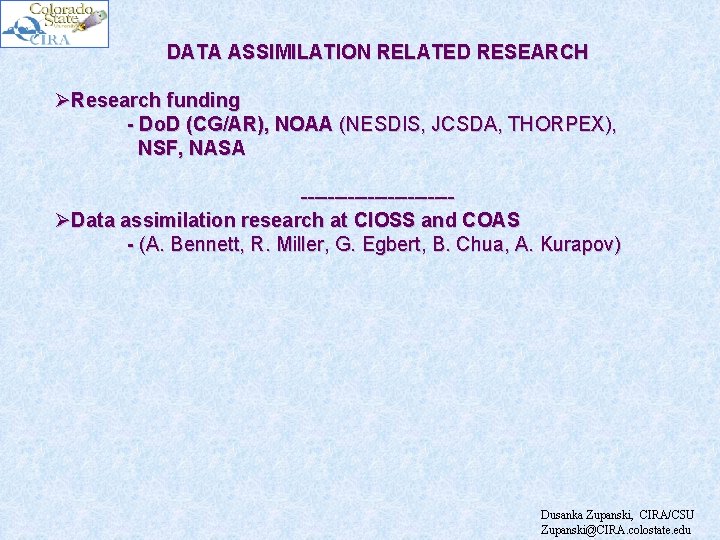
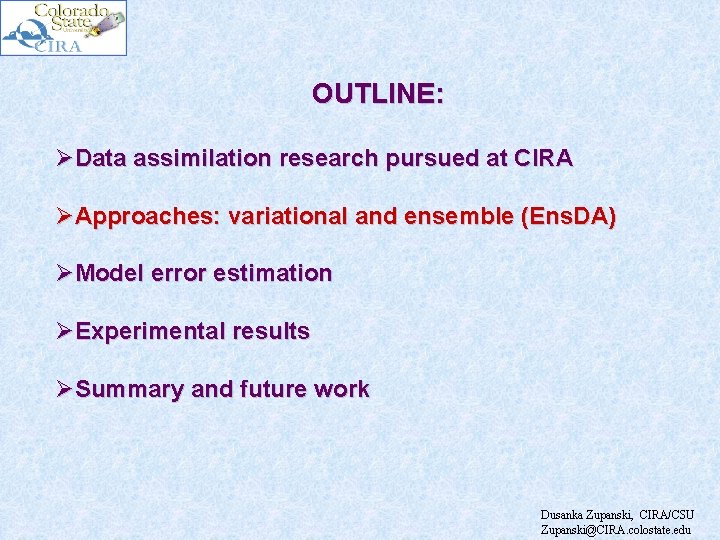
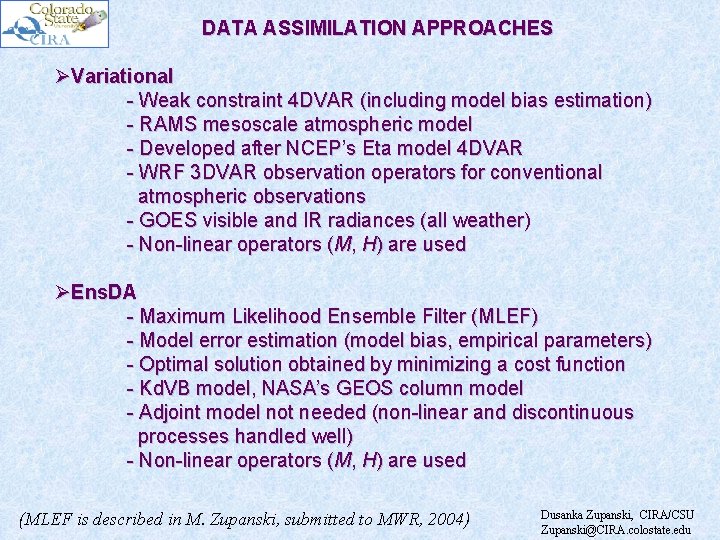
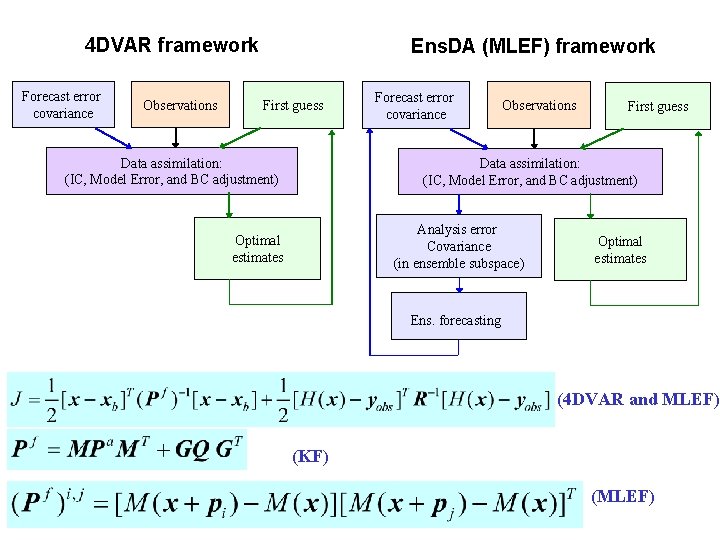
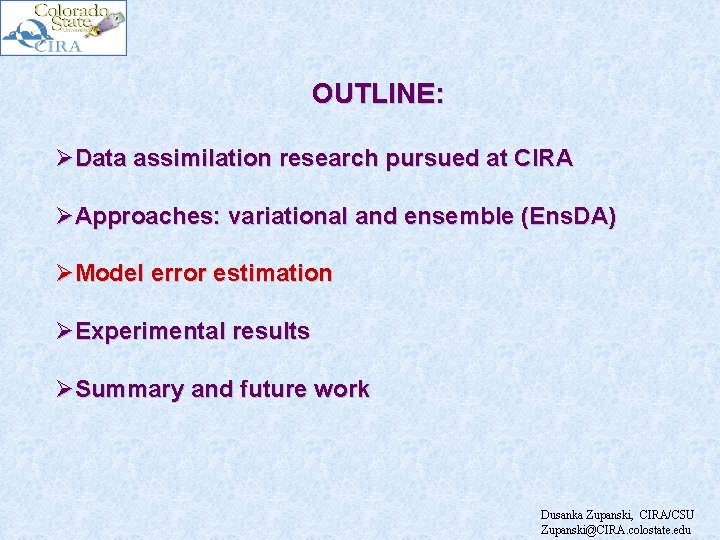
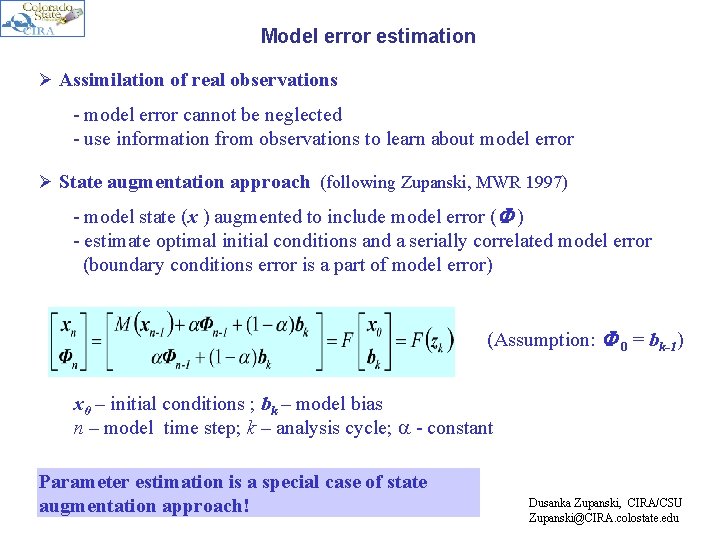
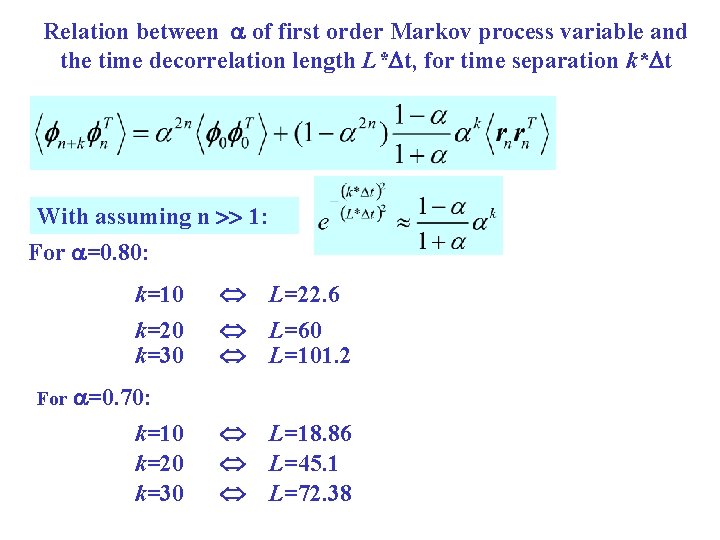
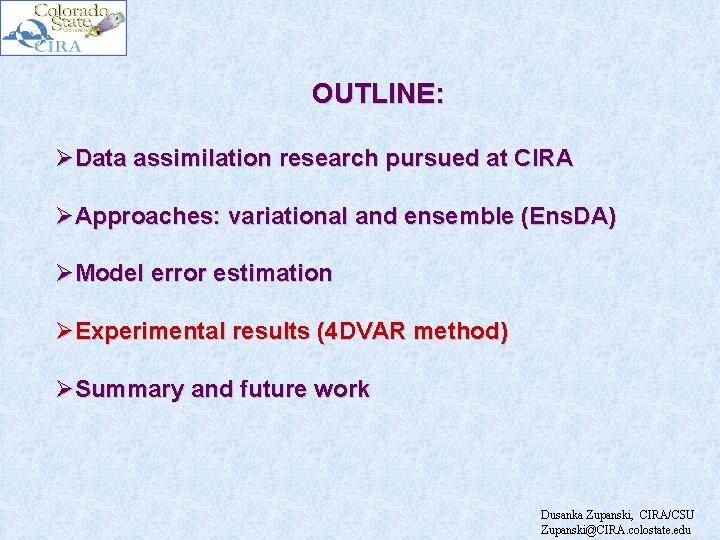
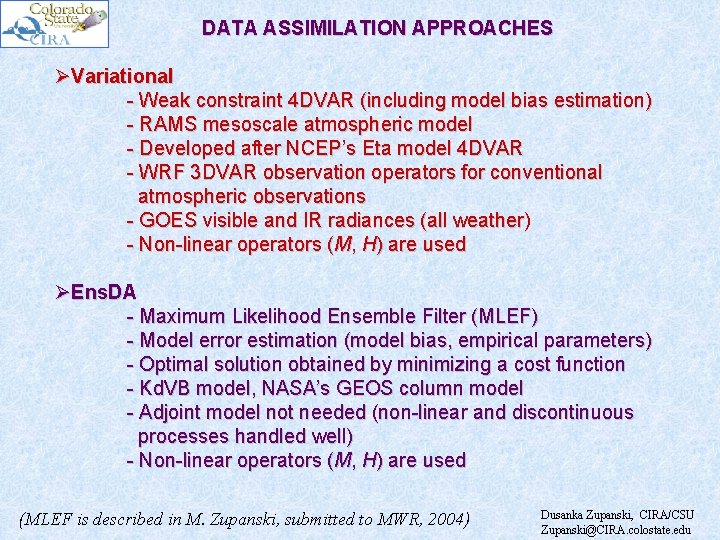
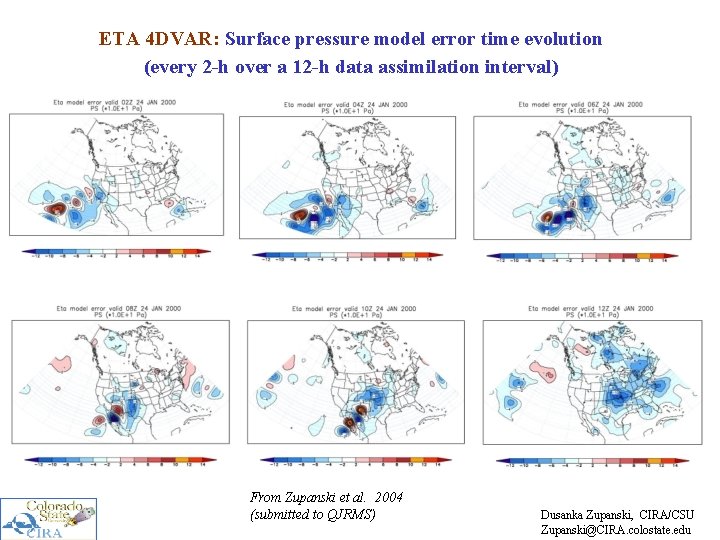
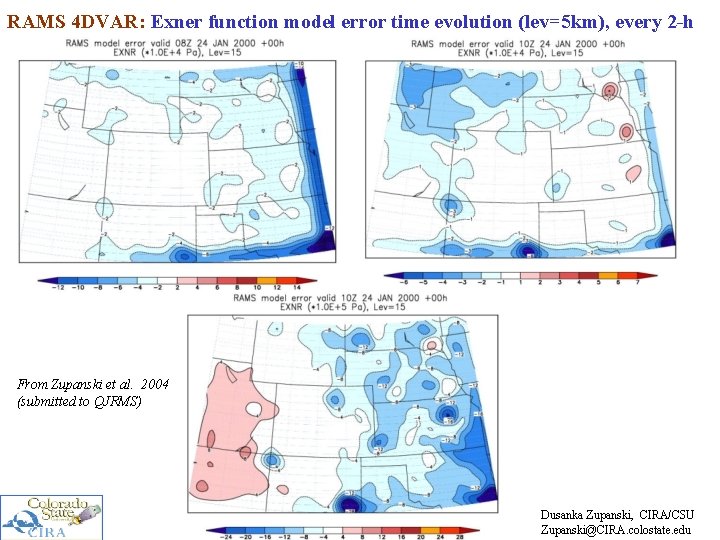
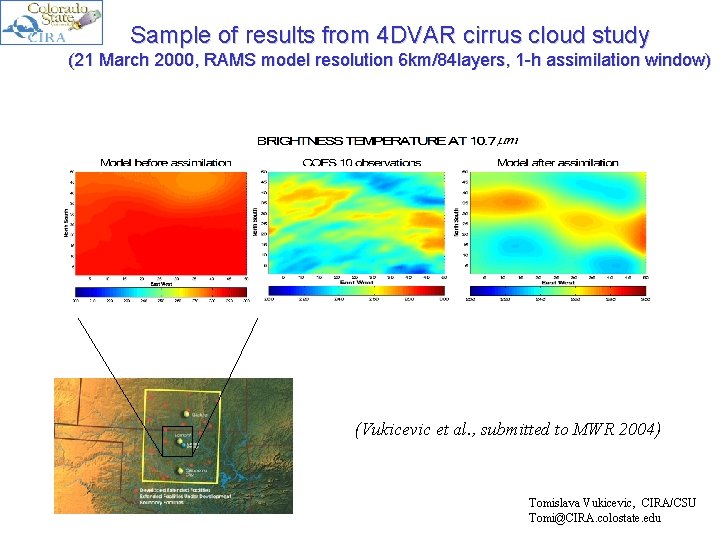
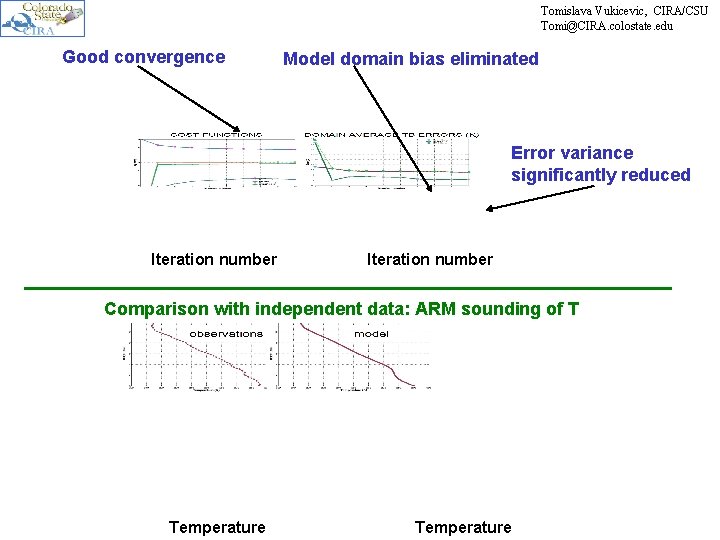
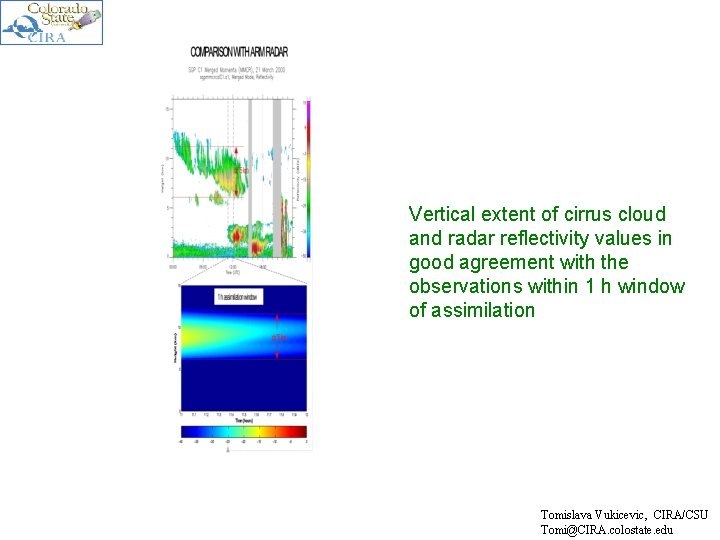
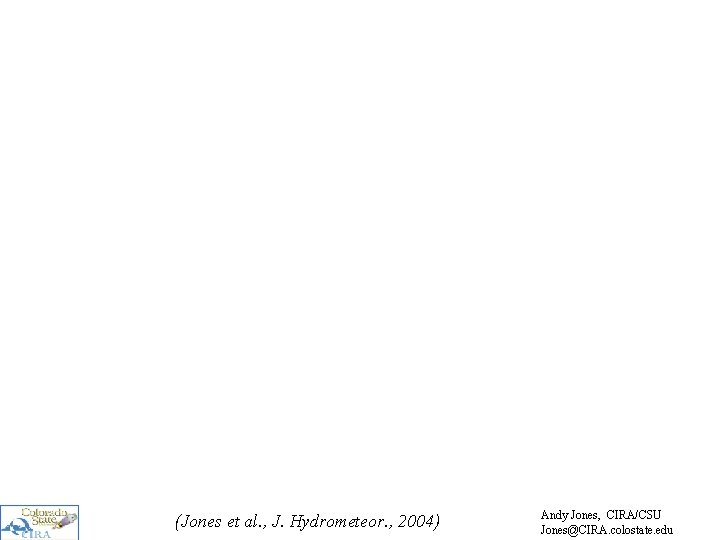
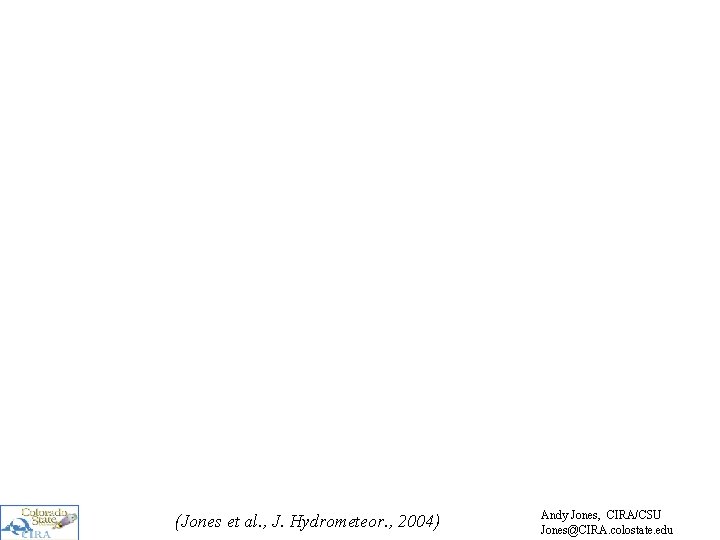
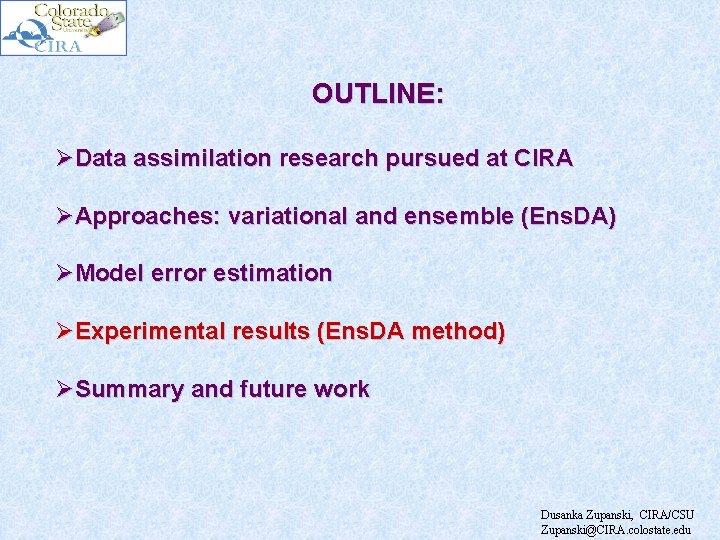
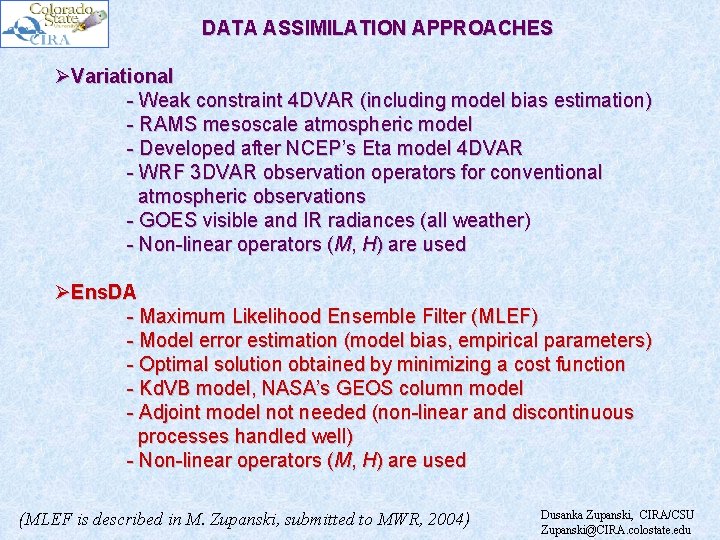
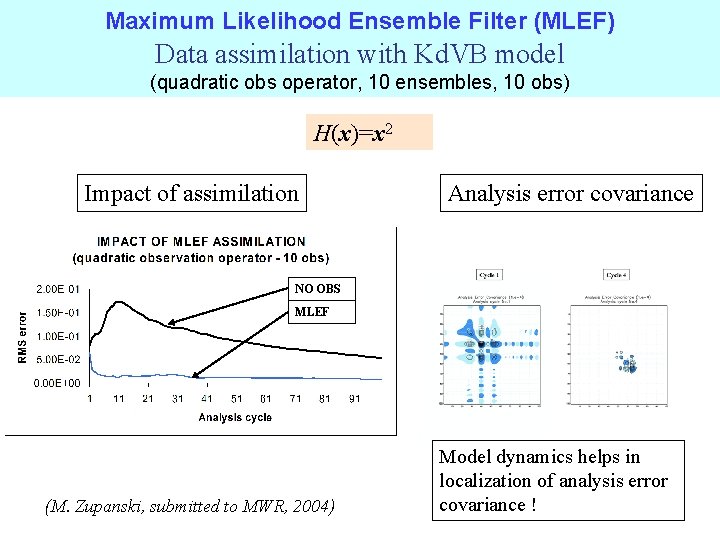
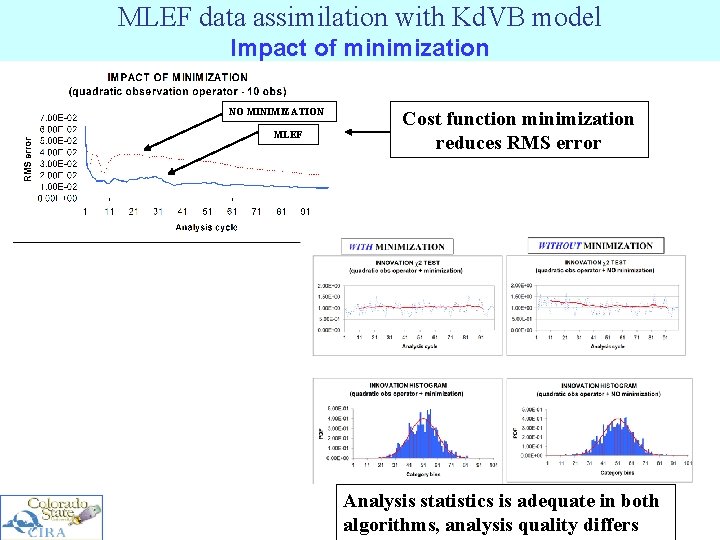
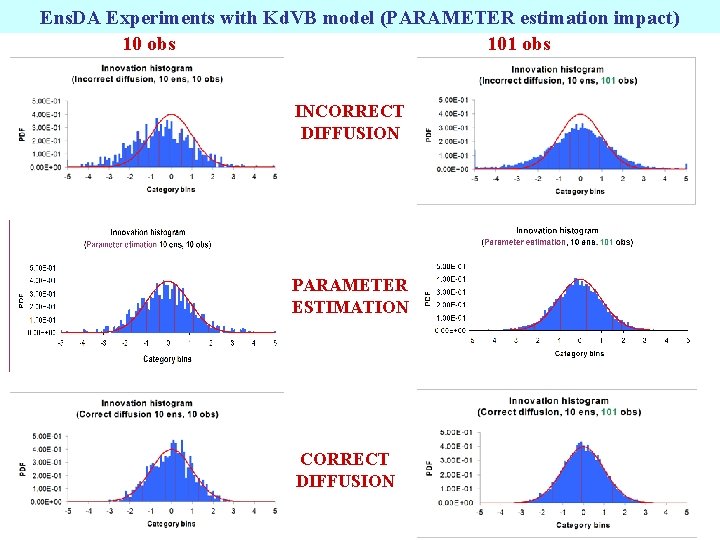
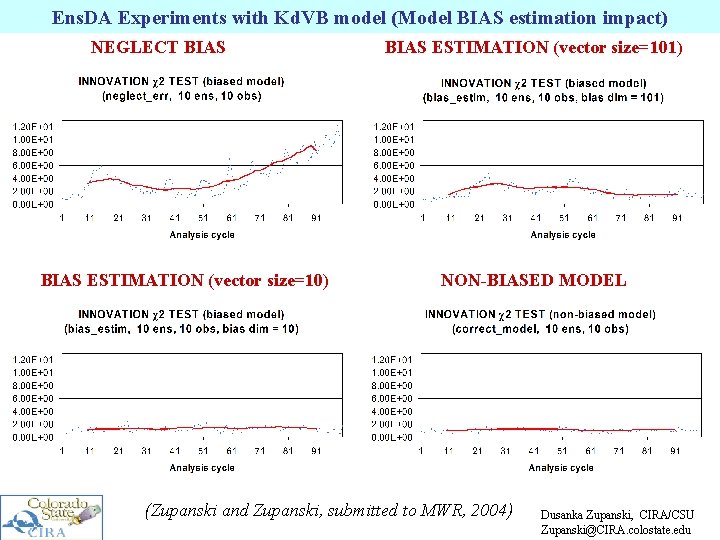
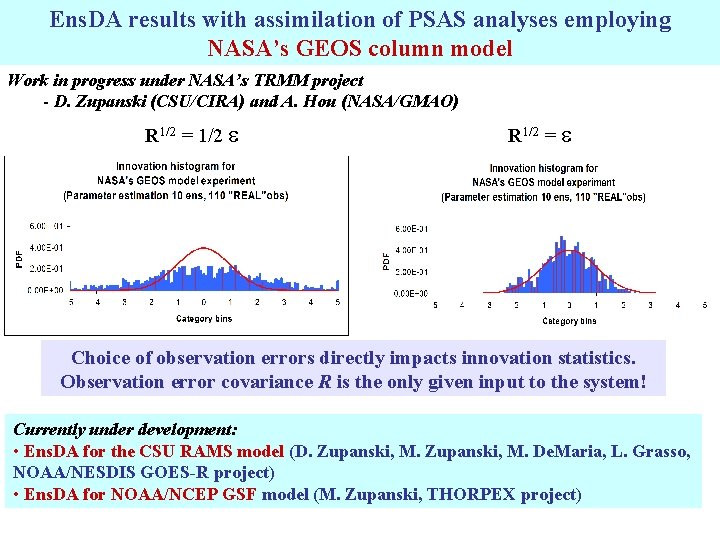
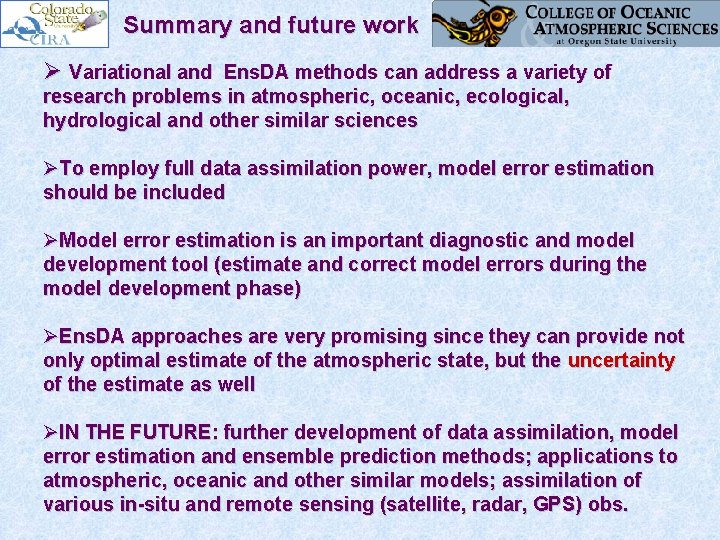
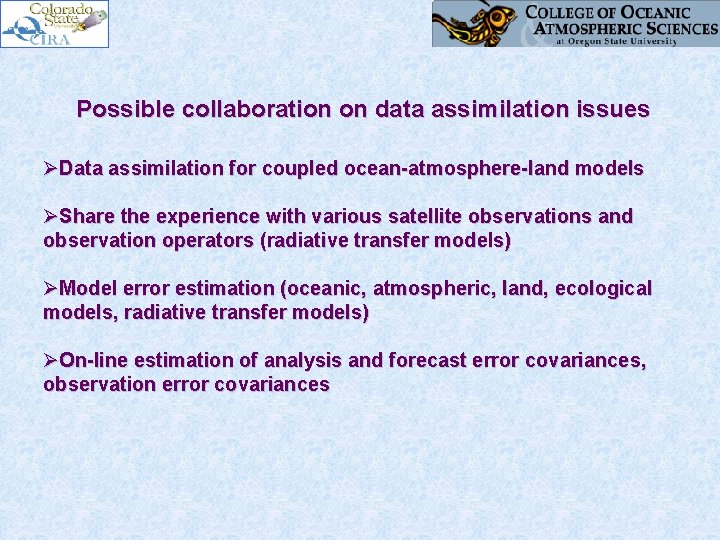
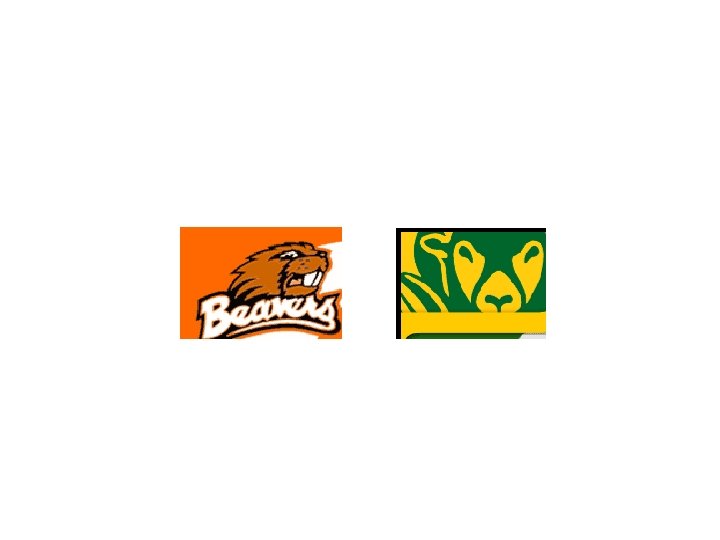
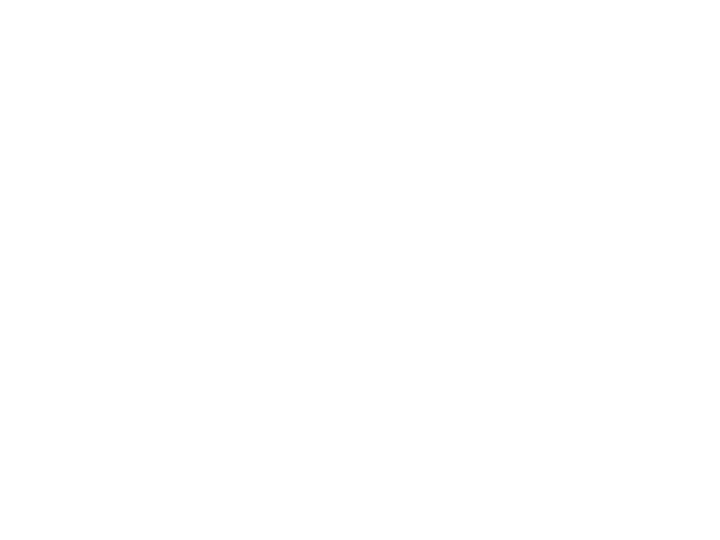
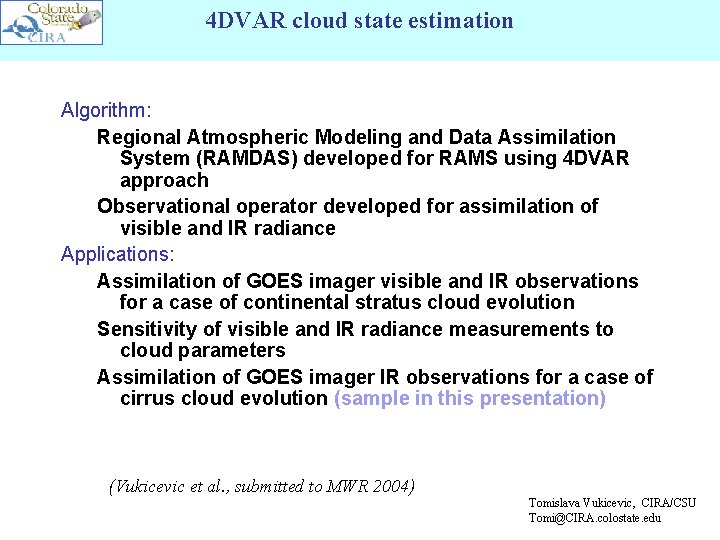
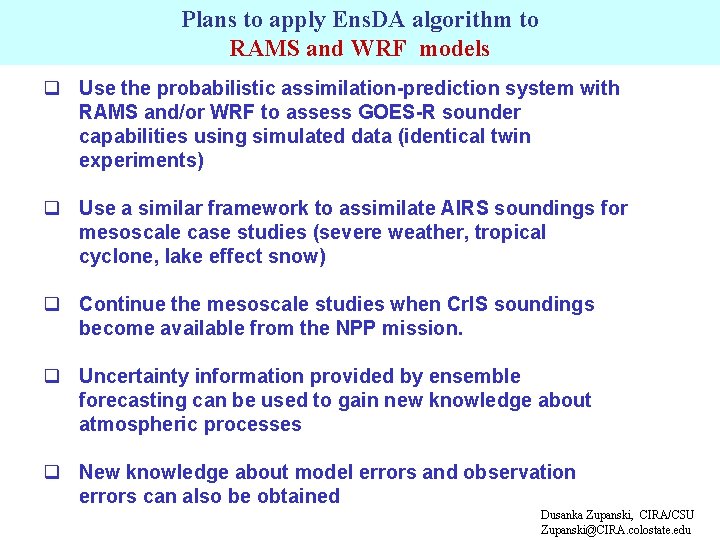
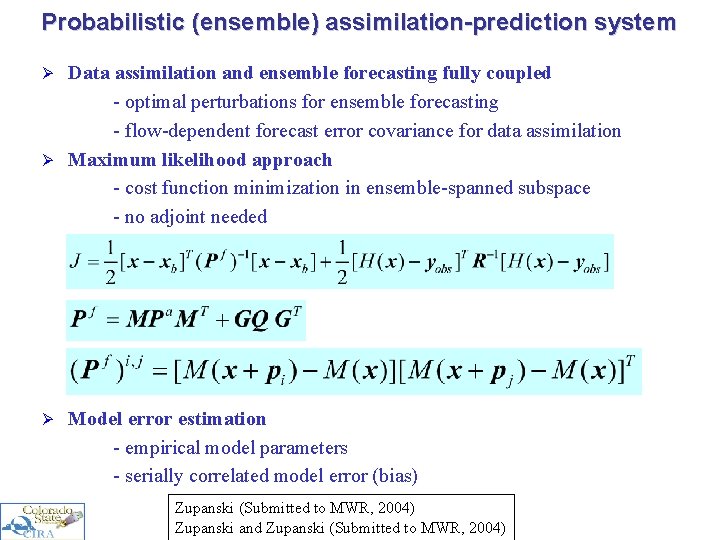
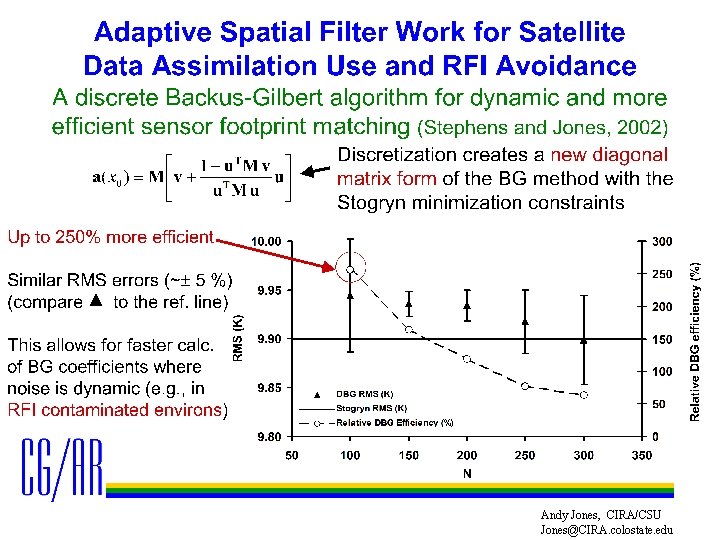
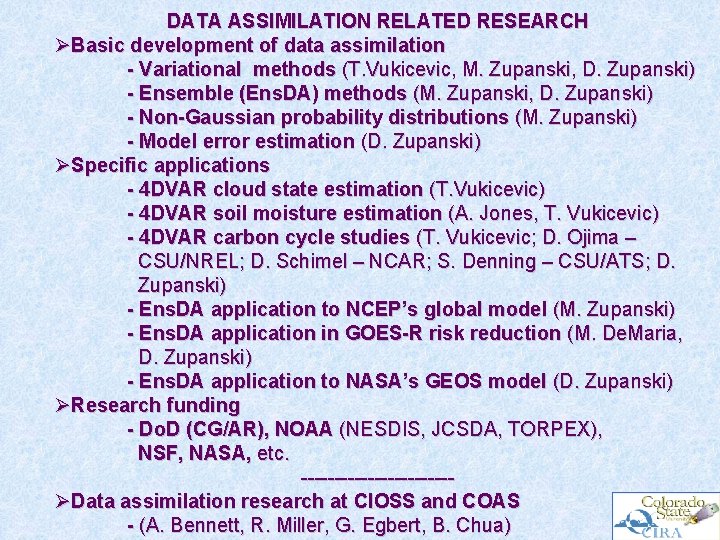
- Slides: 35
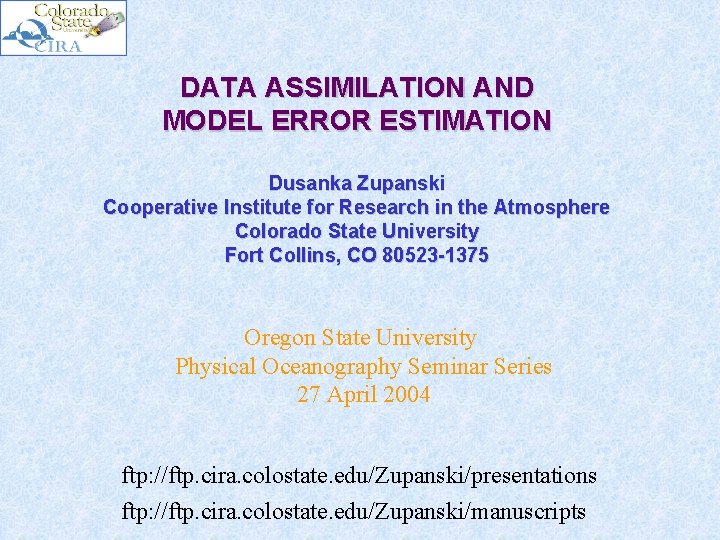
DATA ASSIMILATION AND MODEL ERROR ESTIMATION Dusanka Zupanski Cooperative Institute for Research in the Atmosphere Colorado State University Fort Collins, CO 80523 -1375 Oregon State University Physical Oceanography Seminar Series 27 April 2004 ftp: //ftp. cira. colostate. edu/Zupanski/presentations ftp: //ftp. cira. colostate. edu/Zupanski/manuscripts
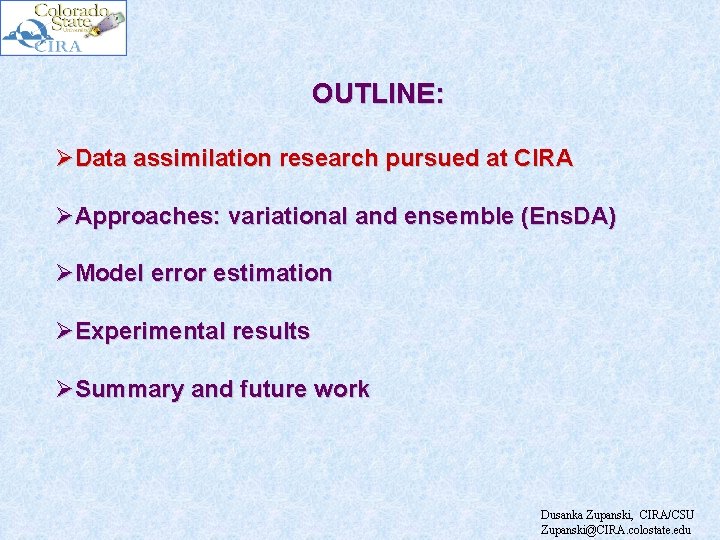
OUTLINE: ØData assimilation research pursued at CIRA ØApproaches: variational and ensemble (Ens. DA) ØModel error estimation ØExperimental results ØSummary and future work Dusanka Zupanski, CIRA/CSU Zupanski@CIRA. colostate. edu
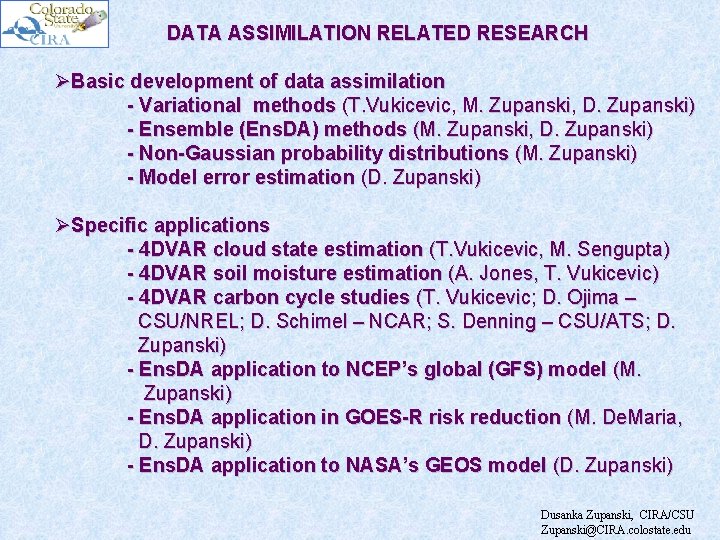
DATA ASSIMILATION RELATED RESEARCH ØBasic development of data assimilation - Variational methods (T. Vukicevic, M. Zupanski, D. Zupanski) - Ensemble (Ens. DA) methods (M. Zupanski, D. Zupanski) - Non-Gaussian probability distributions (M. Zupanski) - Model error estimation (D. Zupanski) ØSpecific applications - 4 DVAR cloud state estimation (T. Vukicevic, M. Sengupta) - 4 DVAR soil moisture estimation (A. Jones, T. Vukicevic) - 4 DVAR carbon cycle studies (T. Vukicevic; D. Ojima – CSU/NREL; D. Schimel – NCAR; S. Denning – CSU/ATS; D. Zupanski) - Ens. DA application to NCEP’s global (GFS) model (M. Zupanski) - Ens. DA application in GOES-R risk reduction (M. De. Maria, D. Zupanski) - Ens. DA application to NASA’s GEOS model (D. Zupanski) Dusanka Zupanski, CIRA/CSU Zupanski@CIRA. colostate. edu
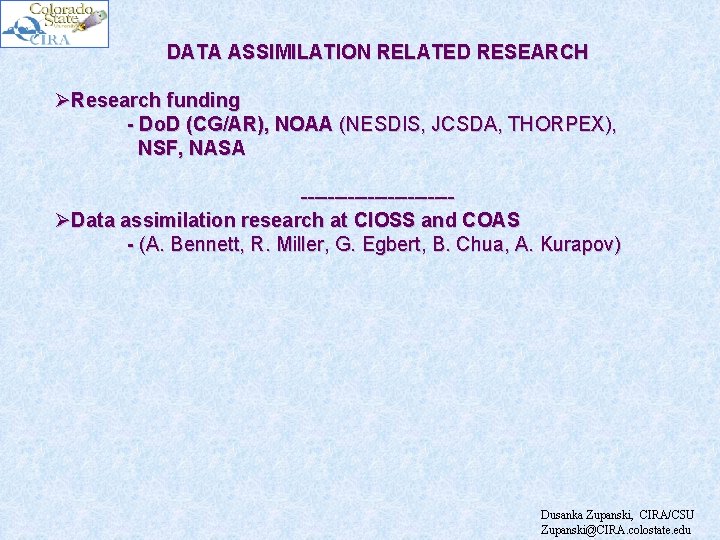
DATA ASSIMILATION RELATED RESEARCH ØResearch funding - Do. D (CG/AR), NOAA (NESDIS, JCSDA, THORPEX), NSF, NASA -----------ØData assimilation research at CIOSS and COAS - (A. Bennett, R. Miller, G. Egbert, B. Chua, A. Kurapov) Dusanka Zupanski, CIRA/CSU Zupanski@CIRA. colostate. edu
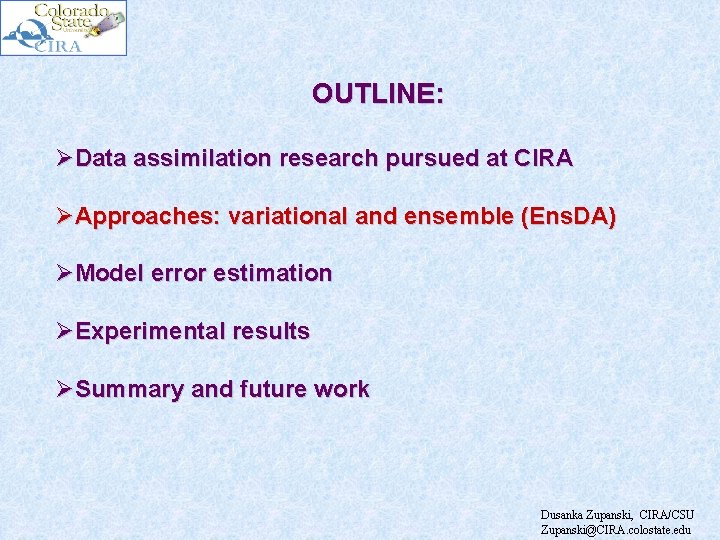
OUTLINE: ØData assimilation research pursued at CIRA ØApproaches: variational and ensemble (Ens. DA) ØModel error estimation ØExperimental results ØSummary and future work Dusanka Zupanski, CIRA/CSU Zupanski@CIRA. colostate. edu
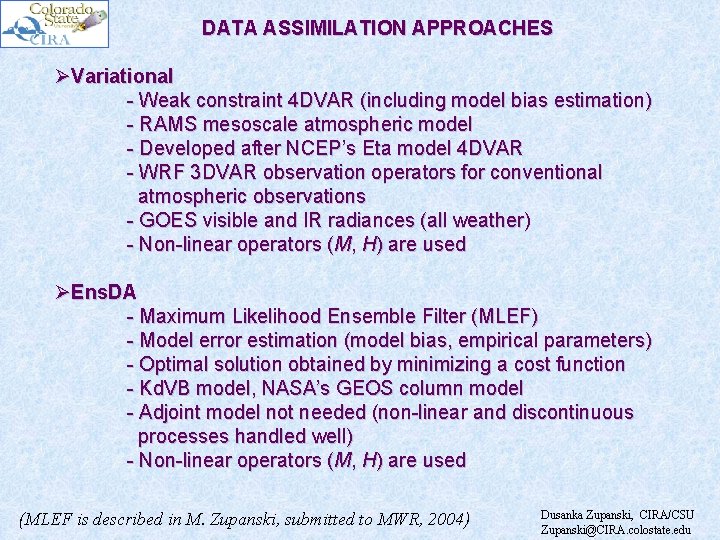
DATA ASSIMILATION APPROACHES ØVariational - Weak constraint 4 DVAR (including model bias estimation) - RAMS mesoscale atmospheric model - Developed after NCEP’s Eta model 4 DVAR - WRF 3 DVAR observation operators for conventional atmospheric observations - GOES visible and IR radiances (all weather) - Non-linear operators (M, H) are used ØEns. DA - Maximum Likelihood Ensemble Filter (MLEF) - Model error estimation (model bias, empirical parameters) - Optimal solution obtained by minimizing a cost function - Kd. VB model, NASA’s GEOS column model - Adjoint model not needed (non-linear and discontinuous processes handled well) - Non-linear operators (M, H) are used (MLEF is described in M. Zupanski, submitted to MWR, 2004) Dusanka Zupanski, CIRA/CSU Zupanski@CIRA. colostate. edu
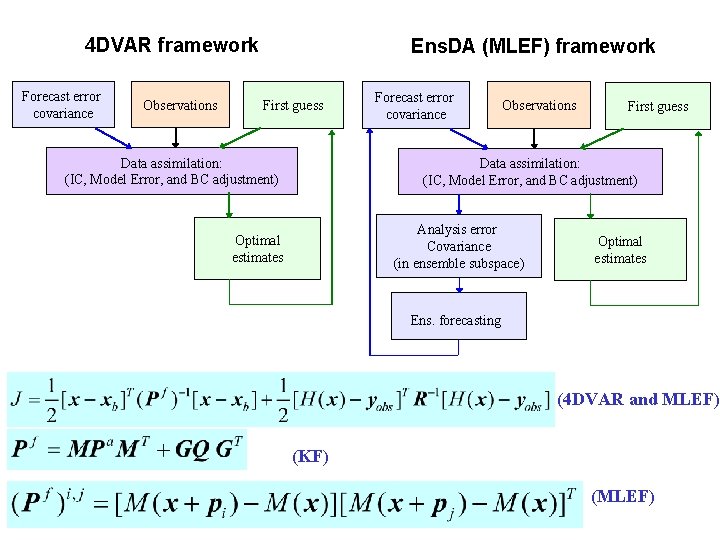
4 DVAR framework Forecast error covariance Observations Ens. DA (MLEF) framework First guess Data assimilation: (IC, Model Error, and BC adjustment) Forecast error covariance Observations First guess Data assimilation: (IC, Model Error, and BC adjustment) Analysis error Covariance (in ensemble subspace) Optimal estimates Ens. forecasting (4 DVAR and MLEF) (KF) (MLEF)
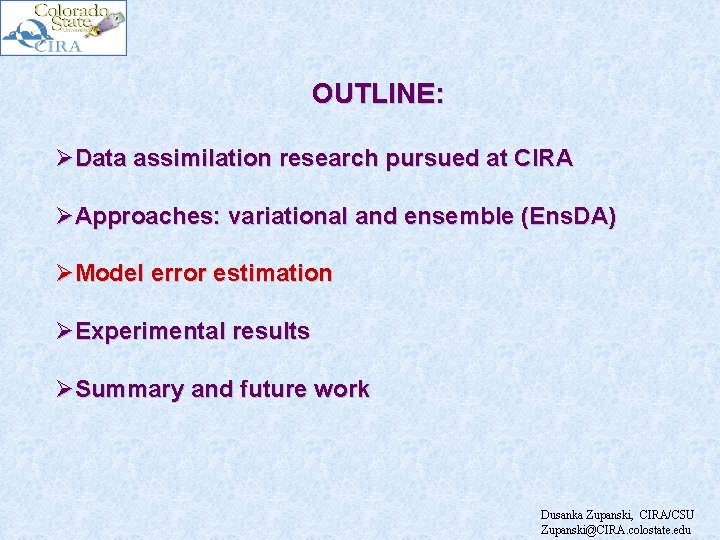
OUTLINE: ØData assimilation research pursued at CIRA ØApproaches: variational and ensemble (Ens. DA) ØModel error estimation ØExperimental results ØSummary and future work Dusanka Zupanski, CIRA/CSU Zupanski@CIRA. colostate. edu
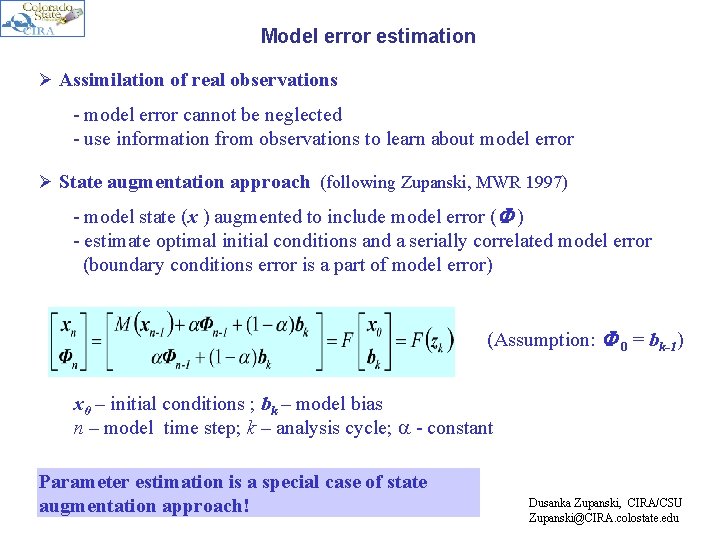
Model error estimation Ø Assimilation of real observations - model error cannot be neglected - use information from observations to learn about model error Ø State augmentation approach (following Zupanski, MWR 1997) - model state (x ) augmented to include model error ( ) - estimate optimal initial conditions and a serially correlated model error (boundary conditions error is a part of model error) (Assumption: 0 = bk-1) x 0 – initial conditions ; bk – model bias n – model time step; k – analysis cycle; a - constant Parameter estimation is a special case of state augmentation approach! Dusanka Zupanski, CIRA/CSU Zupanski@CIRA. colostate. edu
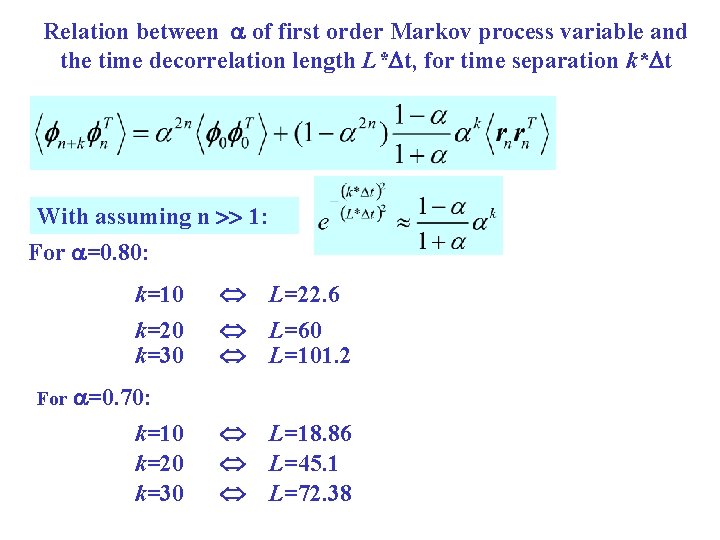
Relation between of first order Markov process variable and the time decorrelation length L* t, for time separation k* t With assuming n 1: For =0. 80: k=10 k=20 k=30 L=22. 6 L=60 L=101. 2 L=18. 86 L=45. 1 L=72. 38 For =0. 70: k=10 k=20 k=30
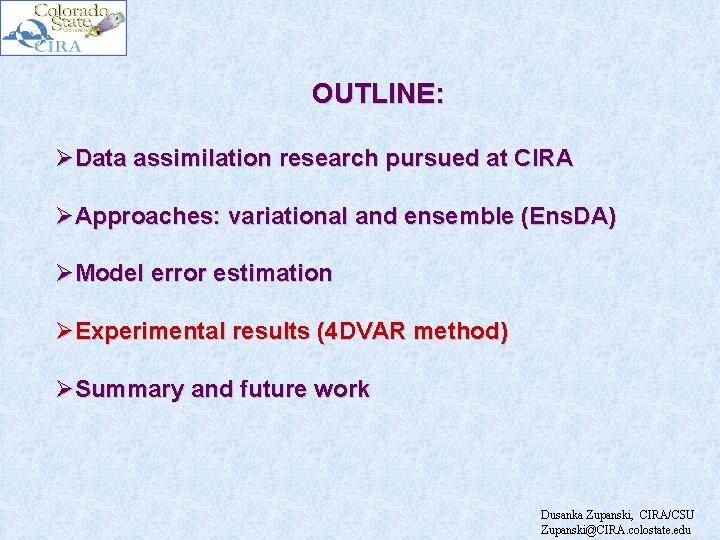
OUTLINE: ØData assimilation research pursued at CIRA ØApproaches: variational and ensemble (Ens. DA) ØModel error estimation ØExperimental results (4 DVAR method) ØSummary and future work Dusanka Zupanski, CIRA/CSU Zupanski@CIRA. colostate. edu
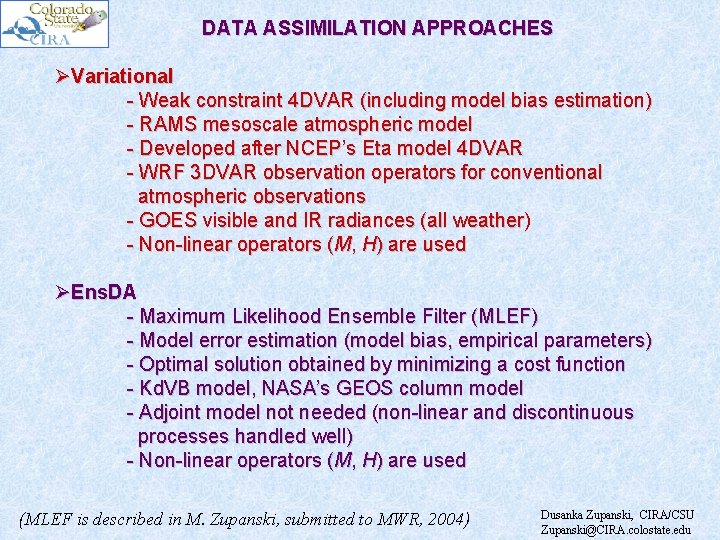
DATA ASSIMILATION APPROACHES ØVariational - Weak constraint 4 DVAR (including model bias estimation) - RAMS mesoscale atmospheric model - Developed after NCEP’s Eta model 4 DVAR - WRF 3 DVAR observation operators for conventional atmospheric observations - GOES visible and IR radiances (all weather) - Non-linear operators (M, H) are used ØEns. DA - Maximum Likelihood Ensemble Filter (MLEF) - Model error estimation (model bias, empirical parameters) - Optimal solution obtained by minimizing a cost function - Kd. VB model, NASA’s GEOS column model - Adjoint model not needed (non-linear and discontinuous processes handled well) - Non-linear operators (M, H) are used (MLEF is described in M. Zupanski, submitted to MWR, 2004) Dusanka Zupanski, CIRA/CSU Zupanski@CIRA. colostate. edu
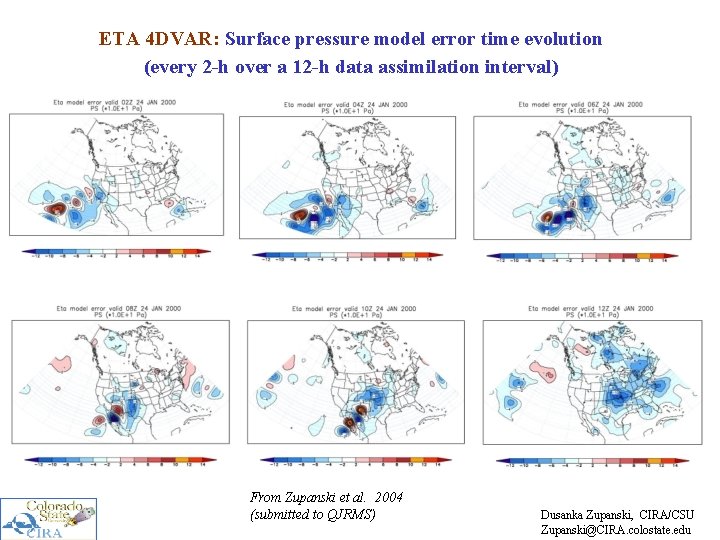
ETA 4 DVAR: Surface pressure model error time evolution (every 2 -h over a 12 -h data assimilation interval) From Zupanski et al. 2004 (submitted to QJRMS) Dusanka Zupanski, CIRA/CSU Zupanski@CIRA. colostate. edu
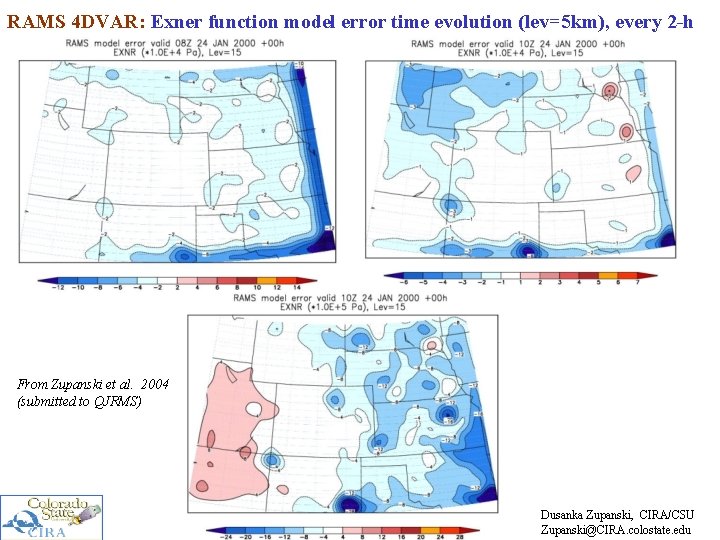
RAMS 4 DVAR: Exner function model error time evolution (lev=5 km), every 2 -h From Zupanski et al. 2004 (submitted to QJRMS) Dusanka Zupanski, CIRA/CSU Zupanski@CIRA. colostate. edu
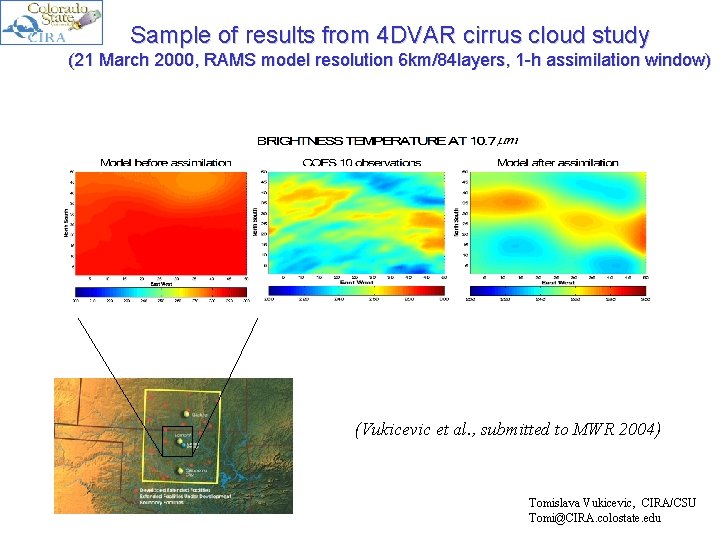
Sample of results from 4 DVAR cirrus cloud study (21 March 2000, RAMS model resolution 6 km/84 layers, 1 -h assimilation window) (Vukicevic et al. , submitted to MWR 2004) Tomislava Vukicevic, CIRA/CSU Tomi@CIRA. colostate. edu
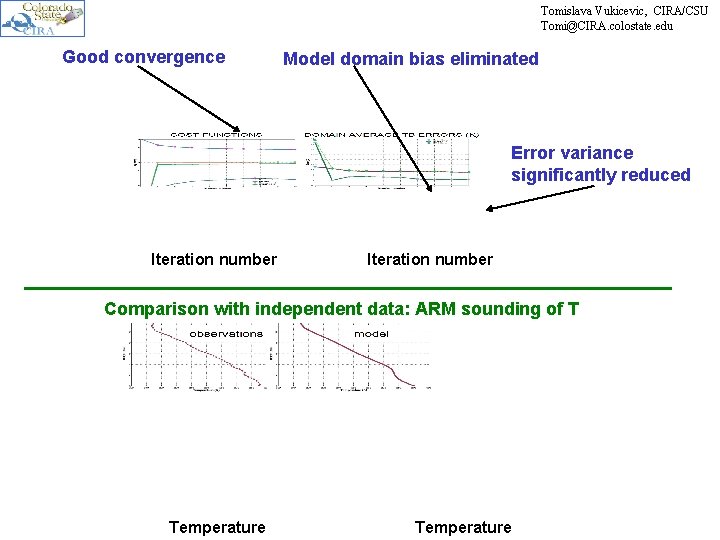
Tomislava Vukicevic, CIRA/CSU Tomi@CIRA. colostate. edu Good convergence Model domain bias eliminated Error variance significantly reduced Iteration number Comparison with independent data: ARM sounding of T Temperature
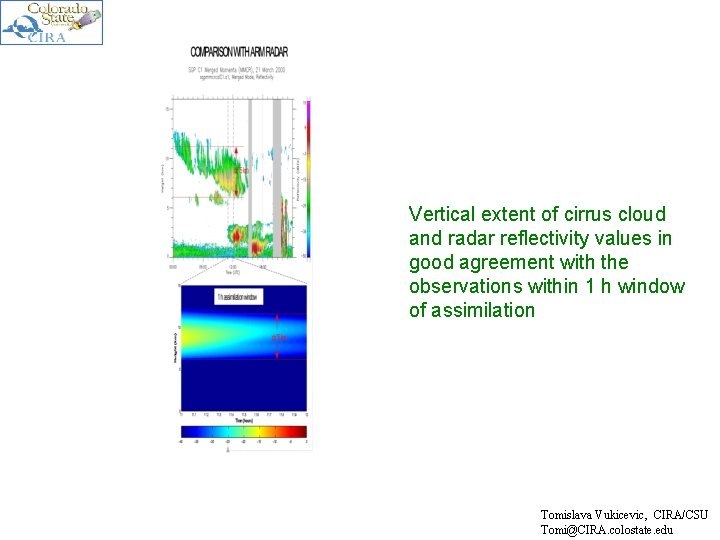
Vertical extent of cirrus cloud and radar reflectivity values in good agreement with the observations within 1 h window of assimilation Tomislava Vukicevic, CIRA/CSU Tomi@CIRA. colostate. edu
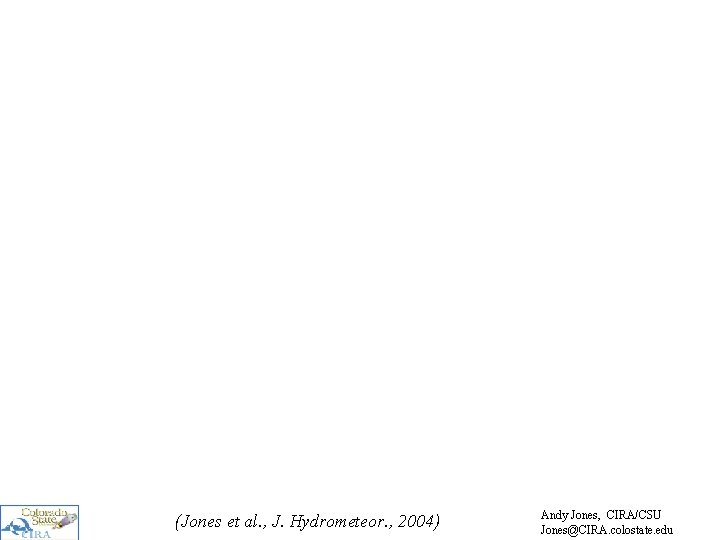
(Jones et al. , J. Hydrometeor. , 2004) Andy Jones, CIRA/CSU Jones@CIRA. colostate. edu
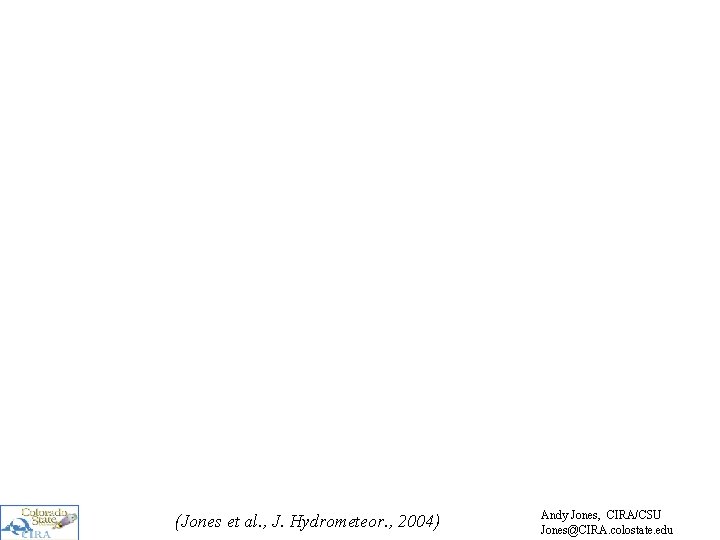
(Jones et al. , J. Hydrometeor. , 2004) Andy Jones, CIRA/CSU Jones@CIRA. colostate. edu
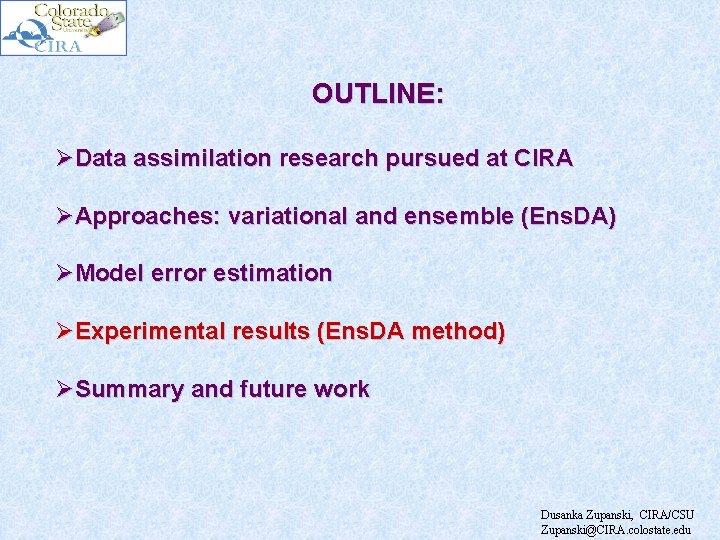
OUTLINE: ØData assimilation research pursued at CIRA ØApproaches: variational and ensemble (Ens. DA) ØModel error estimation ØExperimental results (Ens. DA method) ØSummary and future work Dusanka Zupanski, CIRA/CSU Zupanski@CIRA. colostate. edu
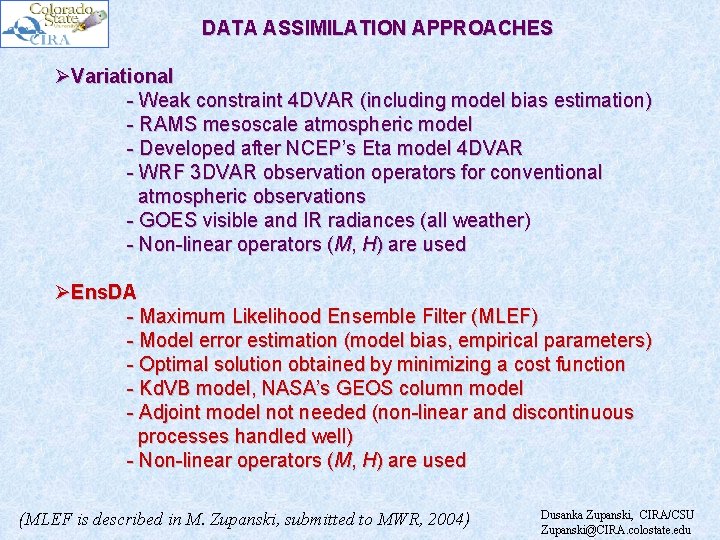
DATA ASSIMILATION APPROACHES ØVariational - Weak constraint 4 DVAR (including model bias estimation) - RAMS mesoscale atmospheric model - Developed after NCEP’s Eta model 4 DVAR - WRF 3 DVAR observation operators for conventional atmospheric observations - GOES visible and IR radiances (all weather) - Non-linear operators (M, H) are used ØEns. DA - Maximum Likelihood Ensemble Filter (MLEF) - Model error estimation (model bias, empirical parameters) - Optimal solution obtained by minimizing a cost function - Kd. VB model, NASA’s GEOS column model - Adjoint model not needed (non-linear and discontinuous processes handled well) - Non-linear operators (M, H) are used (MLEF is described in M. Zupanski, submitted to MWR, 2004) Dusanka Zupanski, CIRA/CSU Zupanski@CIRA. colostate. edu
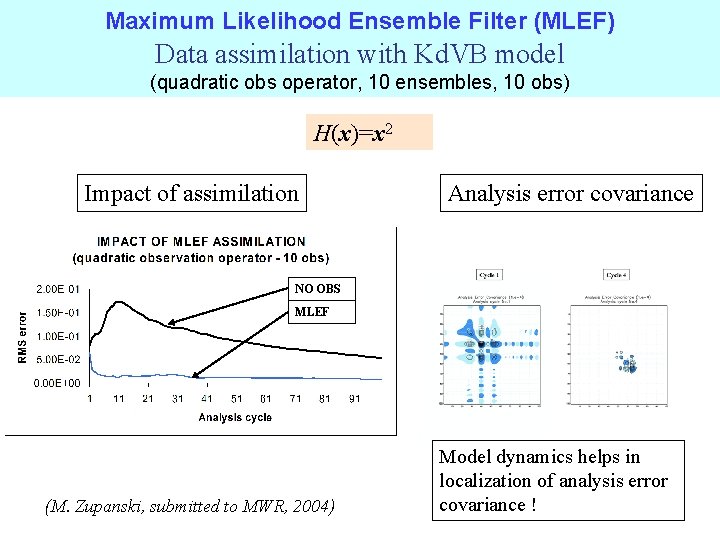
Maximum Likelihood Ensemble Filter (MLEF) Data assimilation with Kd. VB model (quadratic obs operator, 10 ensembles, 10 obs) H(x)=x 2 Impact of assimilation Analysis error covariance NO OBS MLEF (M. Zupanski, submitted to MWR, 2004) Model dynamics helps in localization of analysis error covariance !
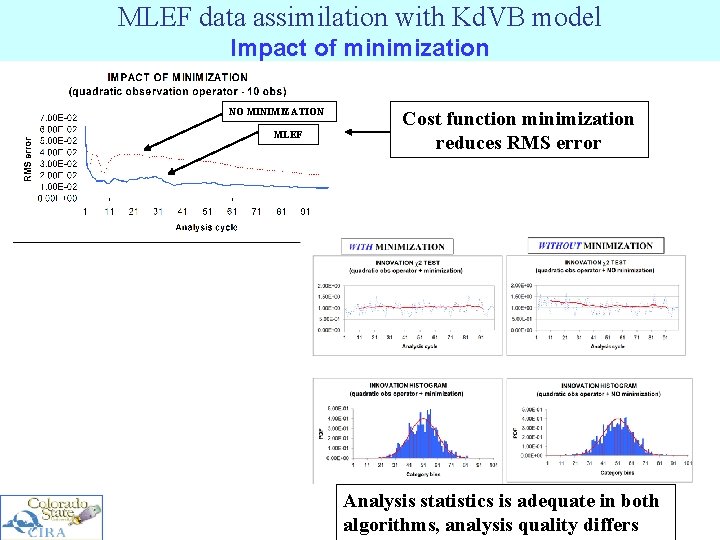
MLEF data assimilation with Kd. VB model Impact of minimization NO MINIMIZATION MLEF Cost function minimization reduces RMS error Analysis statistics is adequate in both algorithms, analysis quality differs
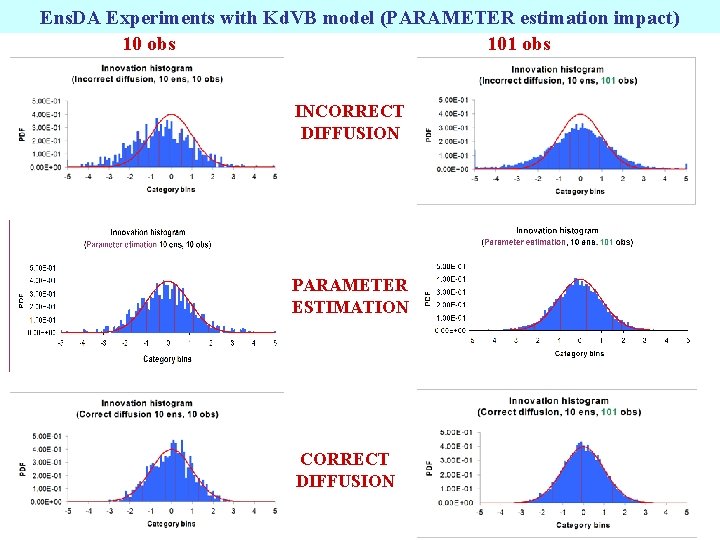
Ens. DA Experiments with Kd. VB model (PARAMETER estimation impact) 10 obs 101 obs INCORRECT DIFFUSION PARAMETER ESTIMATION CORRECT DIFFUSION
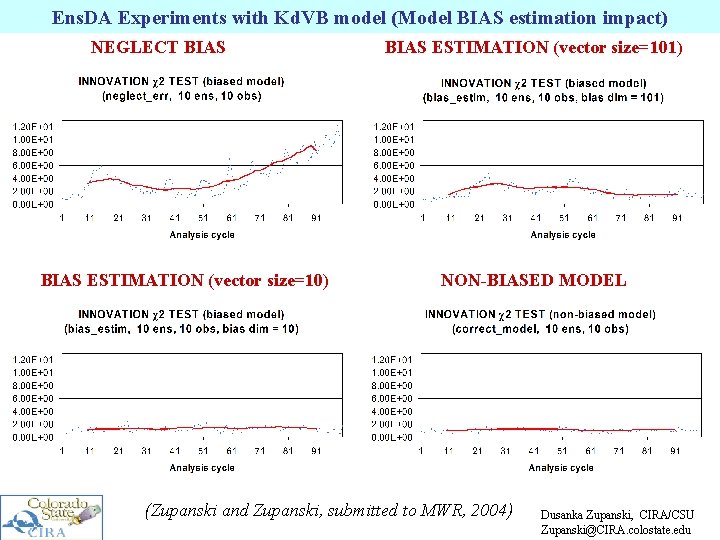
Ens. DA Experiments with Kd. VB model (Model BIAS estimation impact) NEGLECT BIAS ESTIMATION (vector size=10) BIAS ESTIMATION (vector size=101) NON-BIASED MODEL (Zupanski and Zupanski, submitted to MWR, 2004) Dusanka Zupanski, CIRA/CSU Zupanski@CIRA. colostate. edu
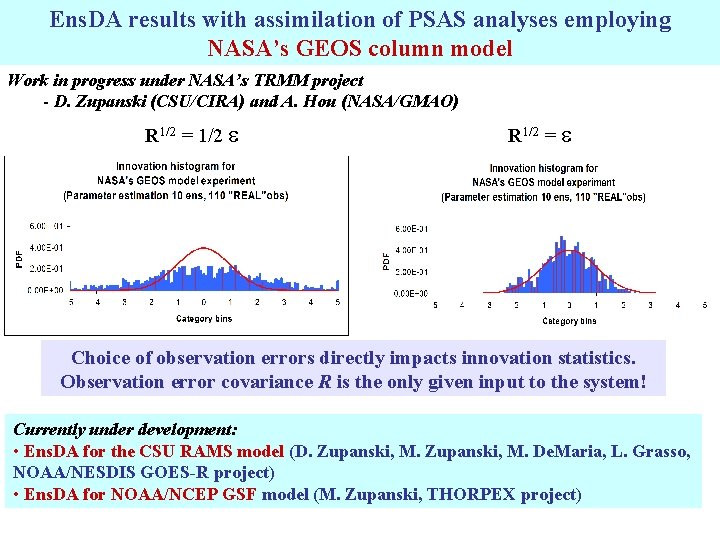
Ens. DA results with assimilation of PSAS analyses employing NASA’s GEOS column model Work in progress under NASA’s TRMM project - D. Zupanski (CSU/CIRA) and A. Hou (NASA/GMAO) R 1/2 = 1/2 e R 1/2 = e Choice of observation errors directly impacts innovation statistics. Observation error covariance R is the only given input to the system! Currently under development: • Ens. DA for the CSU RAMS model (D. Zupanski, M. De. Maria, L. Grasso, NOAA/NESDIS GOES-R project) • Ens. DA for NOAA/NCEP GSF model (M. Zupanski, THORPEX project)
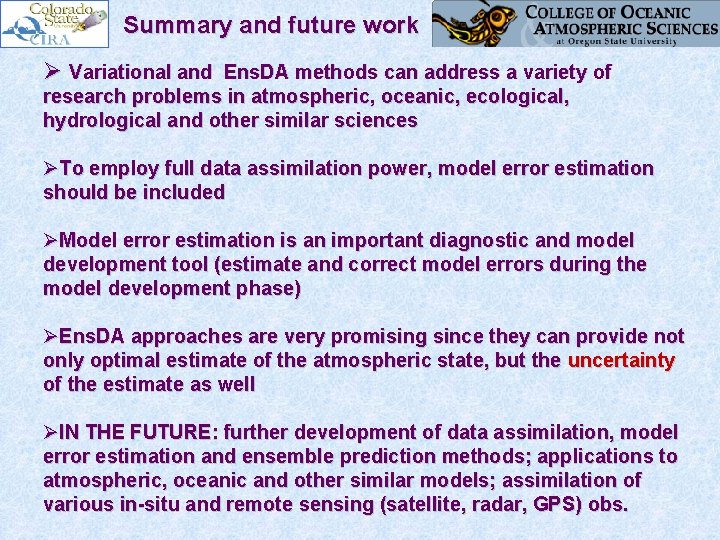
Summary and future work Ø Variational and Ens. DA methods can address a variety of research problems in atmospheric, oceanic, ecological, hydrological and other similar sciences ØTo employ full data assimilation power, model error estimation should be included ØModel error estimation is an important diagnostic and model development tool (estimate and correct model errors during the model development phase) ØEns. DA approaches are very promising since they can provide not only optimal estimate of the atmospheric state, but the uncertainty of the estimate as well ØIN THE FUTURE: further development of data assimilation, model error estimation and ensemble prediction methods; applications to atmospheric, oceanic and other similar models; assimilation of various in-situ and remote sensing (satellite, radar, GPS) obs.
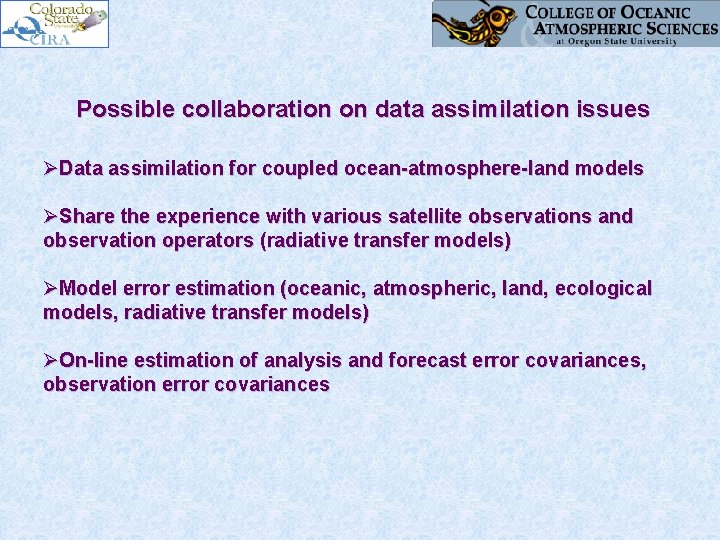
Possible collaboration on data assimilation issues ØData assimilation for coupled ocean-atmosphere-land models ØShare the experience with various satellite observations and observation operators (radiative transfer models) ØModel error estimation (oceanic, atmospheric, land, ecological models, radiative transfer models) ØOn-line estimation of analysis and forecast error covariances, observation error covariances
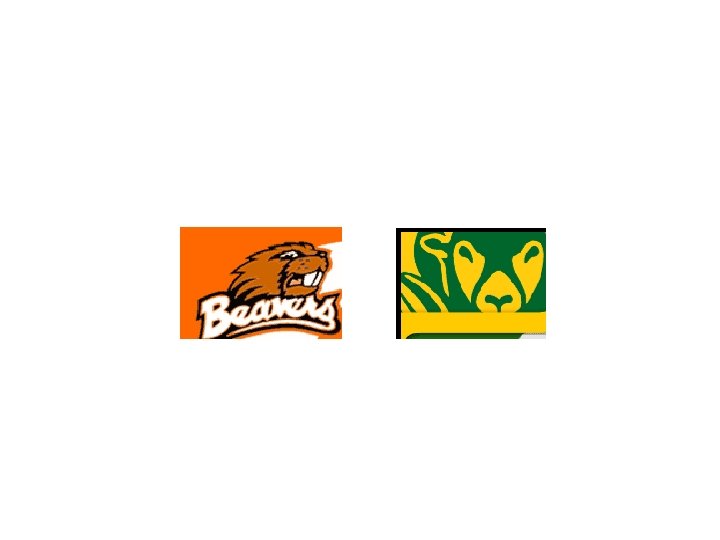
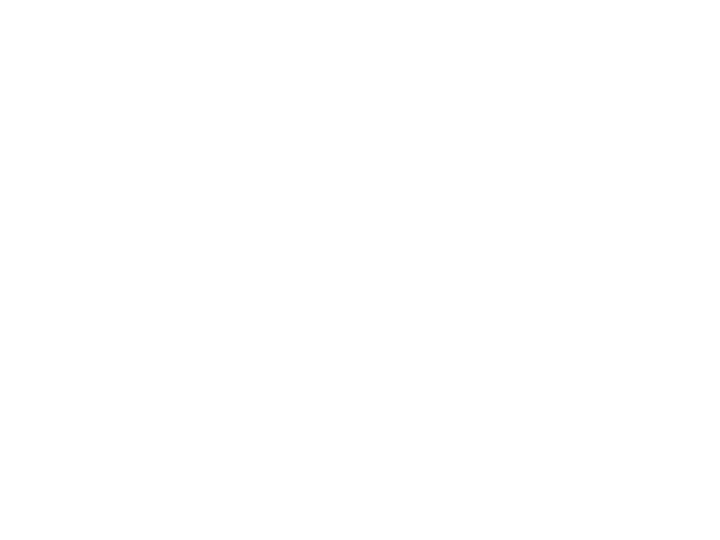
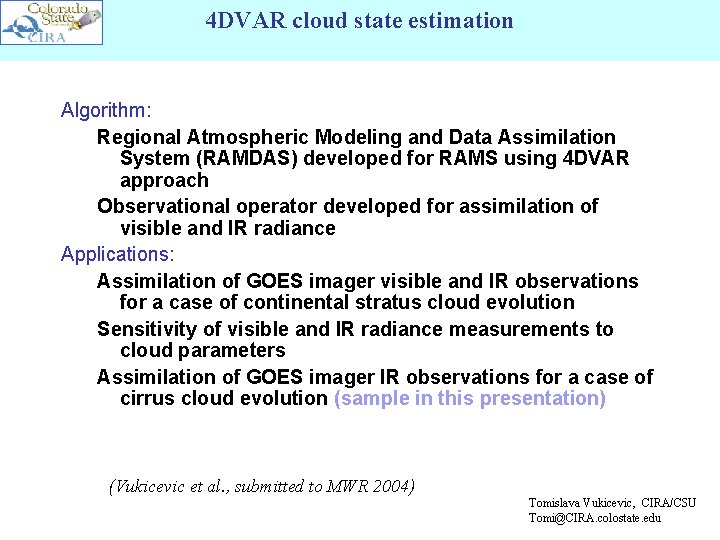
4 DVAR cloud state estimation Algorithm: Regional Atmospheric Modeling and Data Assimilation System (RAMDAS) developed for RAMS using 4 DVAR approach Observational operator developed for assimilation of visible and IR radiance Applications: Assimilation of GOES imager visible and IR observations for a case of continental stratus cloud evolution Sensitivity of visible and IR radiance measurements to cloud parameters Assimilation of GOES imager IR observations for a case of cirrus cloud evolution (sample in this presentation) (Vukicevic et al. , submitted to MWR 2004) Tomislava Vukicevic, CIRA/CSU Tomi@CIRA. colostate. edu
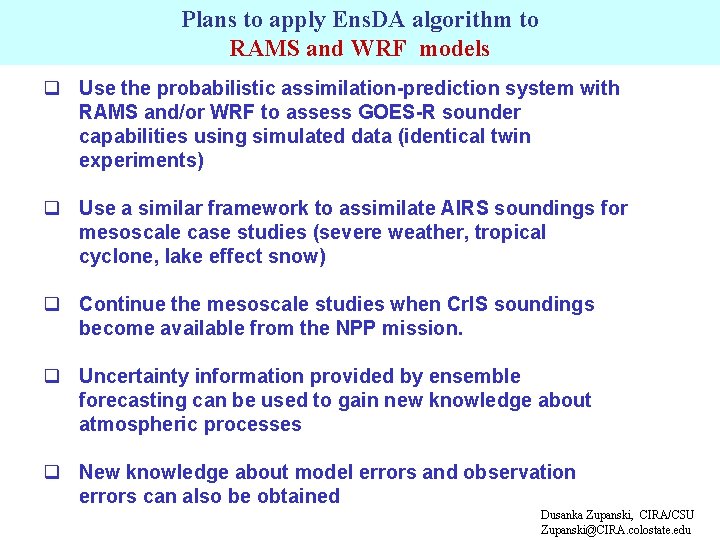
Plans to apply Ens. DA algorithm to RAMS and WRF models q Use the probabilistic assimilation-prediction system with RAMS and/or WRF to assess GOES-R sounder capabilities using simulated data (identical twin experiments) q Use a similar framework to assimilate AIRS soundings for mesoscale case studies (severe weather, tropical cyclone, lake effect snow) q Continue the mesoscale studies when Cr. IS soundings become available from the NPP mission. q Uncertainty information provided by ensemble forecasting can be used to gain new knowledge about atmospheric processes q New knowledge about model errors and observation errors can also be obtained Dusanka Zupanski, CIRA/CSU Zupanski@CIRA. colostate. edu
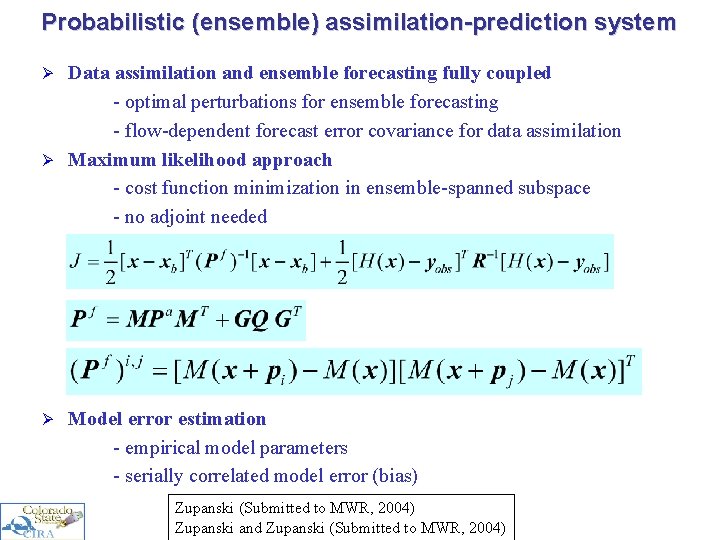
Probabilistic (ensemble) assimilation-prediction system Data assimilation and ensemble forecasting fully coupled - optimal perturbations for ensemble forecasting - flow-dependent forecast error covariance for data assimilation Ø Maximum likelihood approach - cost function minimization in ensemble-spanned subspace - no adjoint needed Ø Ø Model error estimation - empirical model parameters - serially correlated model error (bias) Zupanski (Submitted to MWR, 2004) Zupanski and Zupanski (Submitted to MWR, 2004)
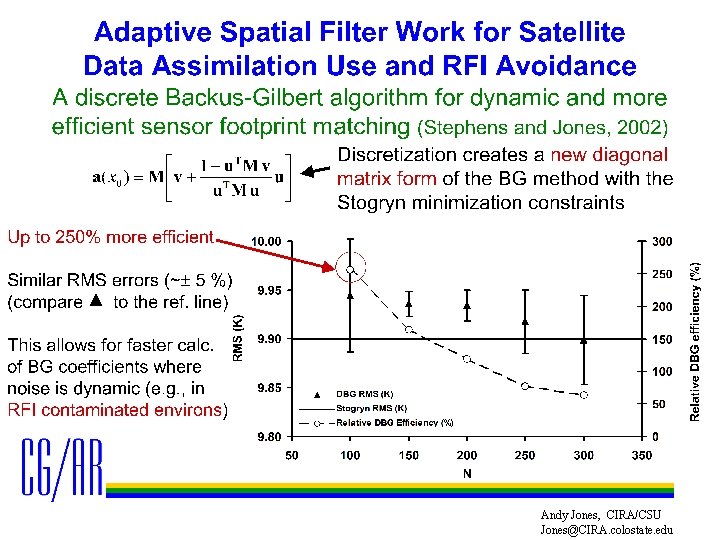
Andy Jones, CIRA/CSU Jones@CIRA. colostate. edu
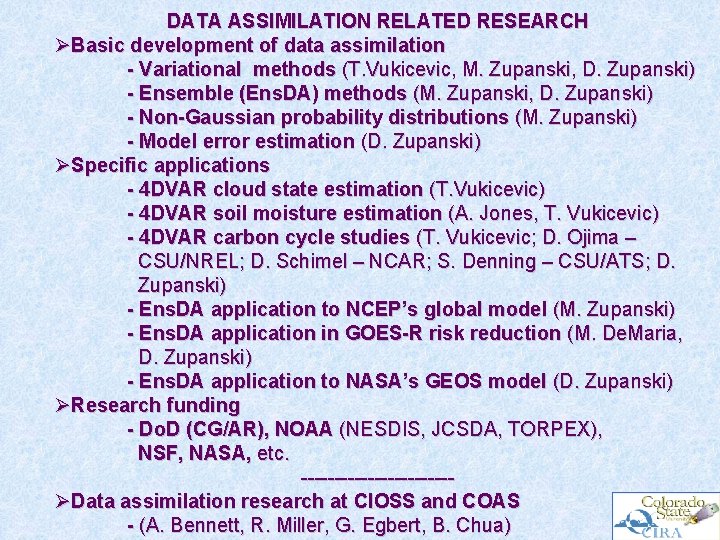
DATA ASSIMILATION RELATED RESEARCH ØBasic development of data assimilation - Variational methods (T. Vukicevic, M. Zupanski, D. Zupanski) - Ensemble (Ens. DA) methods (M. Zupanski, D. Zupanski) - Non-Gaussian probability distributions (M. Zupanski) - Model error estimation (D. Zupanski) ØSpecific applications - 4 DVAR cloud state estimation (T. Vukicevic) - 4 DVAR soil moisture estimation (A. Jones, T. Vukicevic) - 4 DVAR carbon cycle studies (T. Vukicevic; D. Ojima – CSU/NREL; D. Schimel – NCAR; S. Denning – CSU/ATS; D. Zupanski) - Ens. DA application to NCEP’s global model (M. Zupanski) - Ens. DA application in GOES-R risk reduction (M. De. Maria, D. Zupanski) - Ens. DA application to NASA’s GEOS model (D. Zupanski) ØResearch funding - Do. D (CG/AR), NOAA (NESDIS, JCSDA, TORPEX), NSF, NASA, etc. -----------ØData assimilation research at CIOSS and COAS - (A. Bennett, R. Miller, G. Egbert, B. Chua)
Dusanka zupanski
Dusanka zupanski
Dusanka zupanski
Dusanka boskovic
Dušanka janežič
Jedi data assimilation
Data assimilation
Data assimilation tutorial
Data assimilation
Data assimilation
Data assimilation
Data assimilation
Data assimilation
Alternating series estimation theorem
Type of errors in statistics
Random and systematic errors examples
Round off vs truncation error
Crc example
Volume uncertainty
4 parts of digestive system
Absorption and assimilation
Pluralism and assimilation
Allotment and assimilation
Type 2 vs type 1 error
Cdmvt formula
What is a null hypothesis
Power swries
Error sistematico y error aleatorio
H
Validez interna y externa
Error absolut i error relatiu
Invertery
During error reporting, icmp always reports error messages
Cognition ap psych definition
Elision examples phonetics
Stress shift examples