A merged disease ontology for Clin Gen Chris
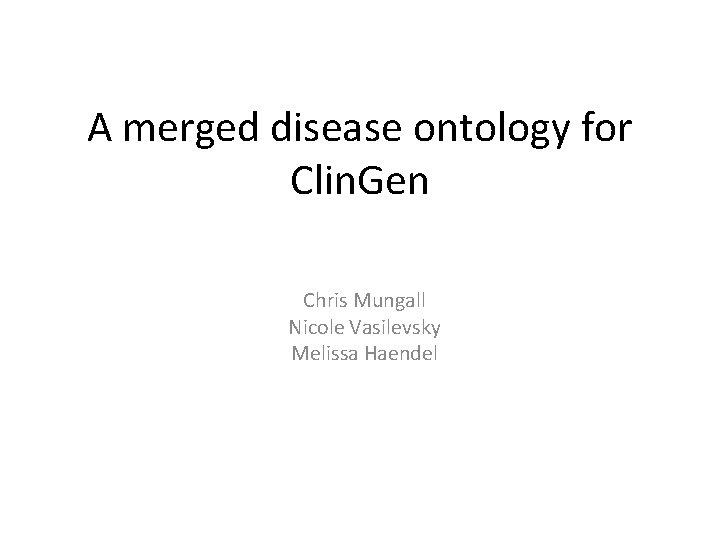
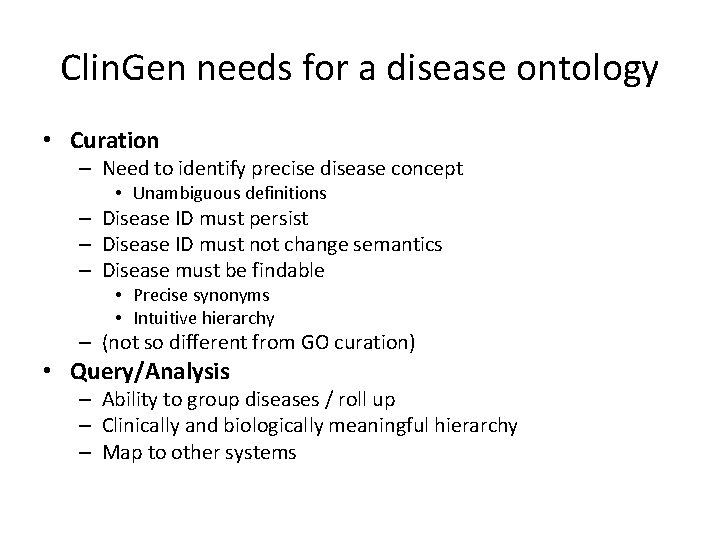
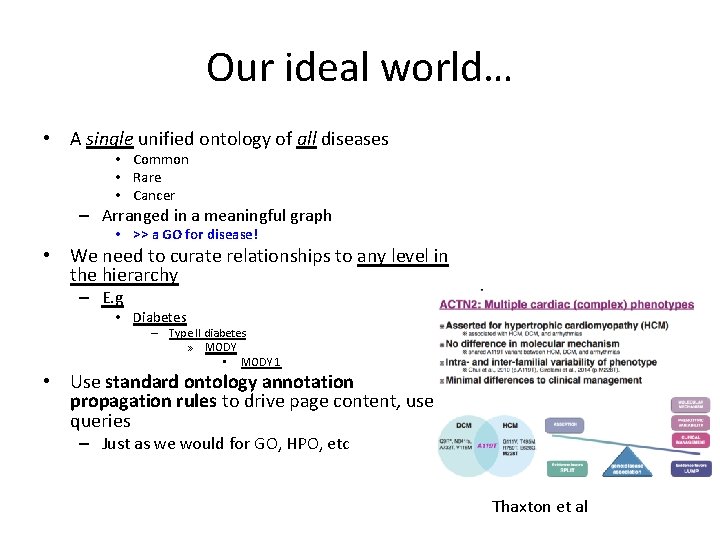
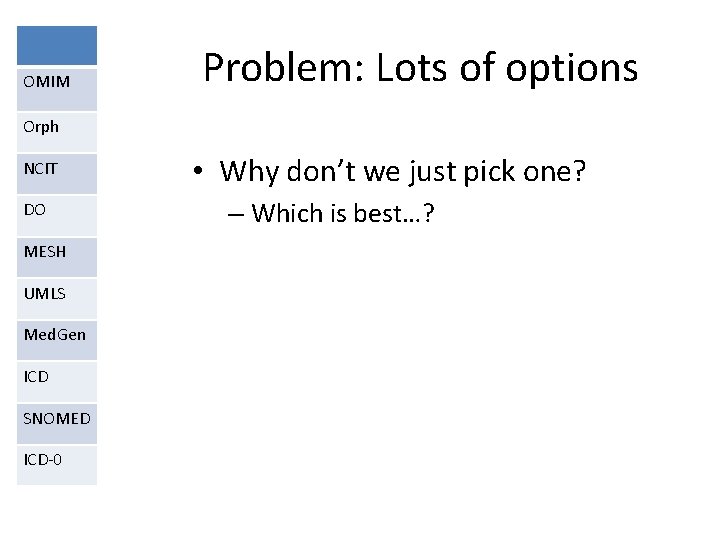
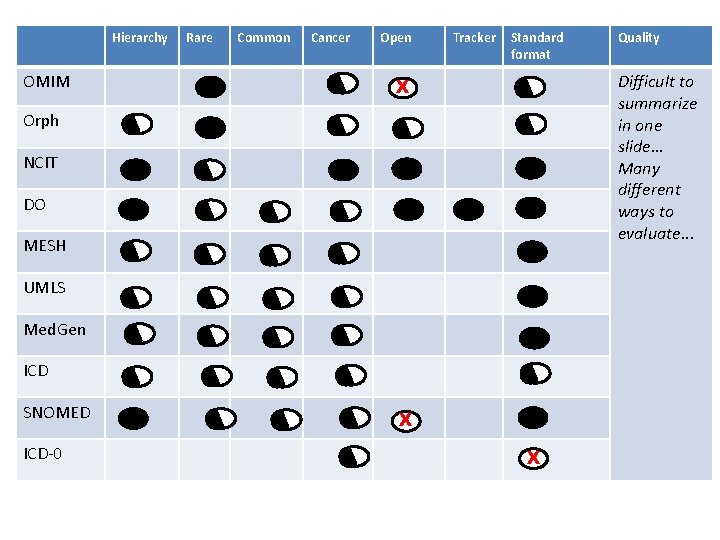
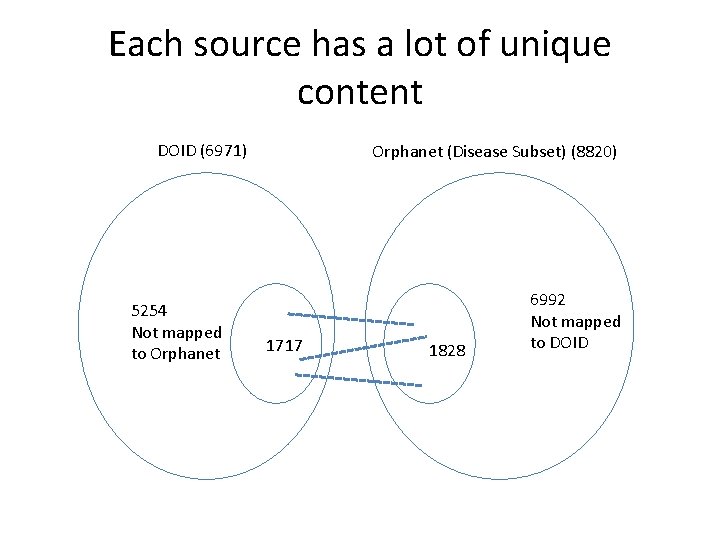
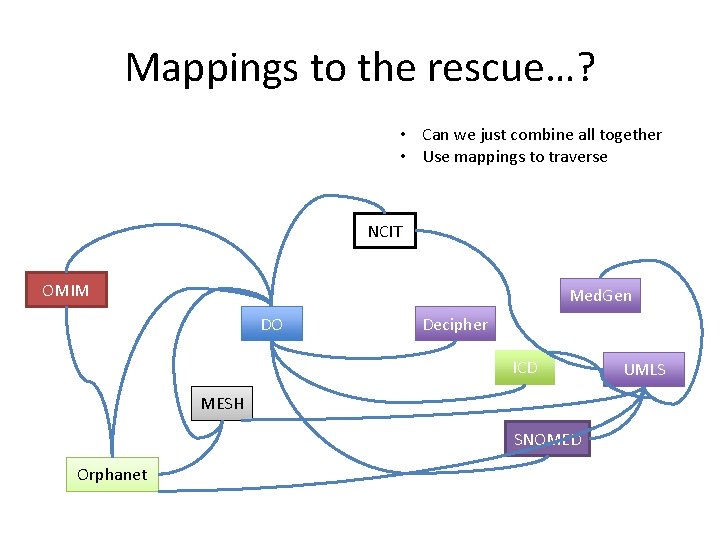
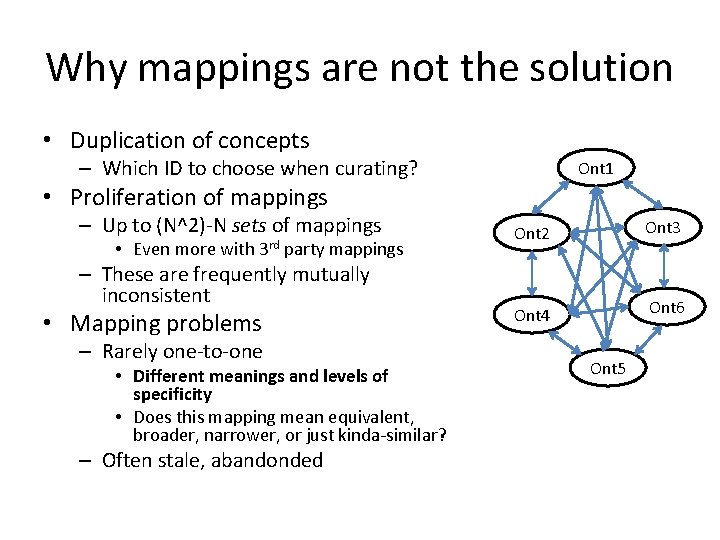
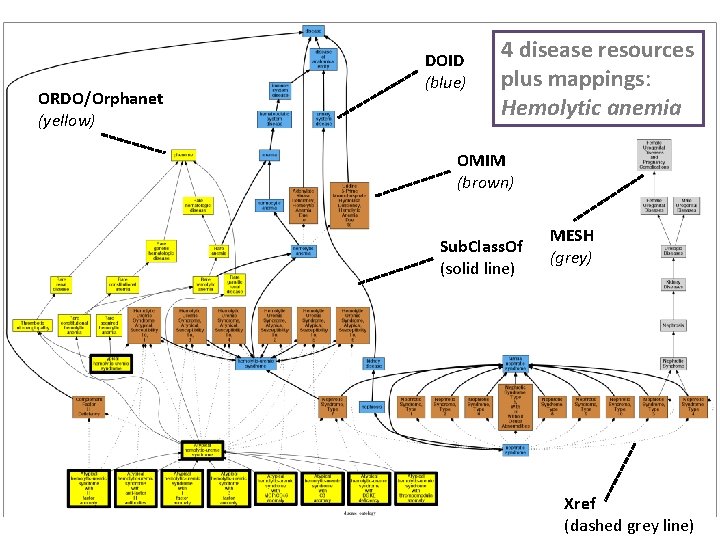
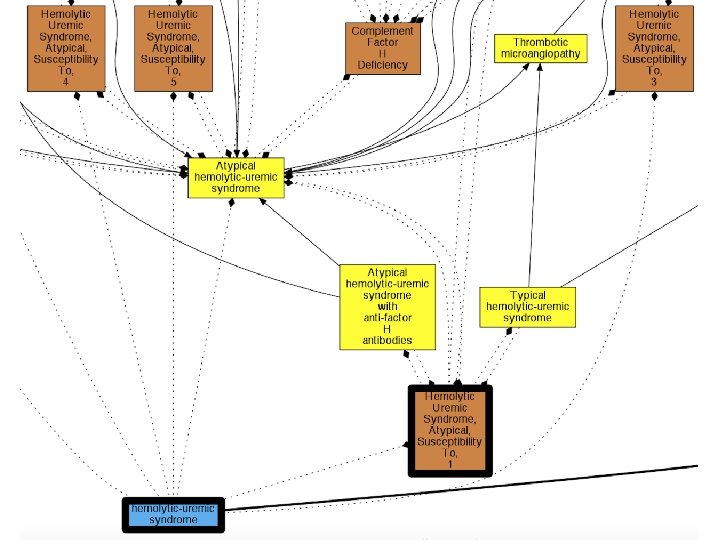
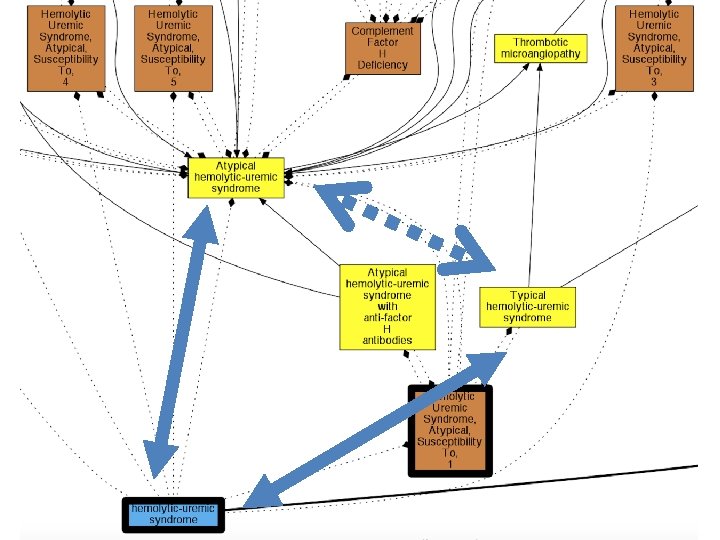
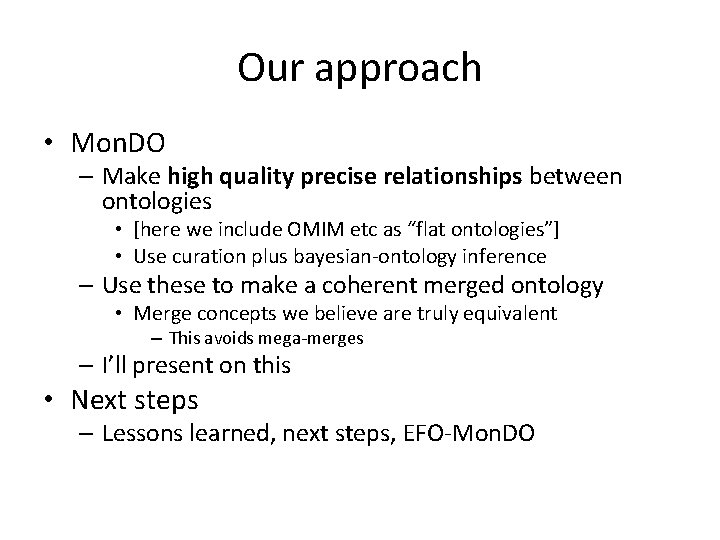
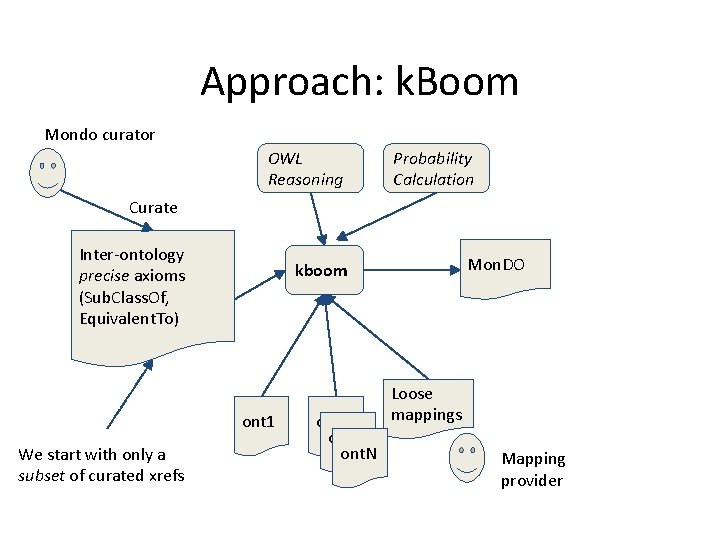
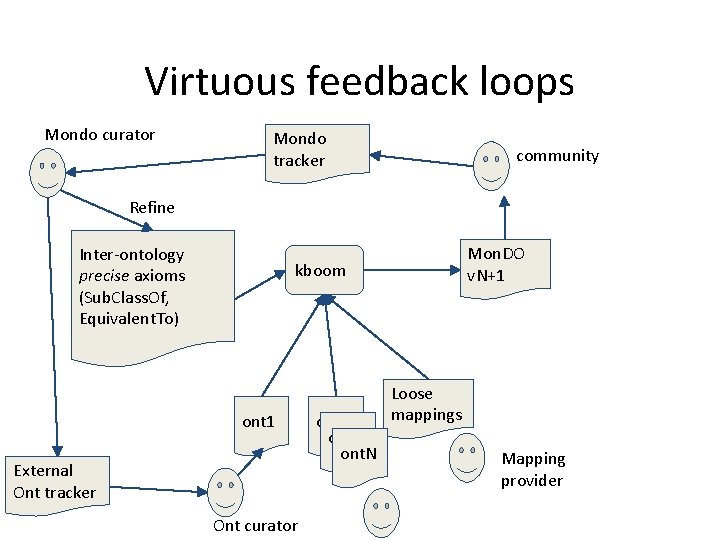
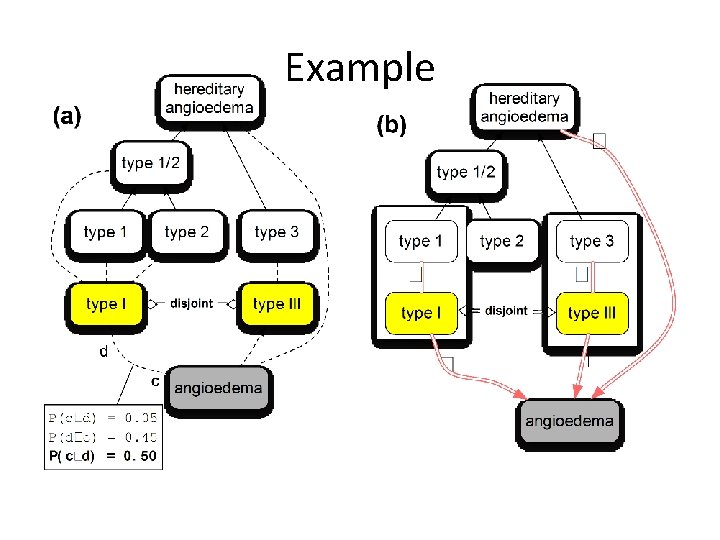
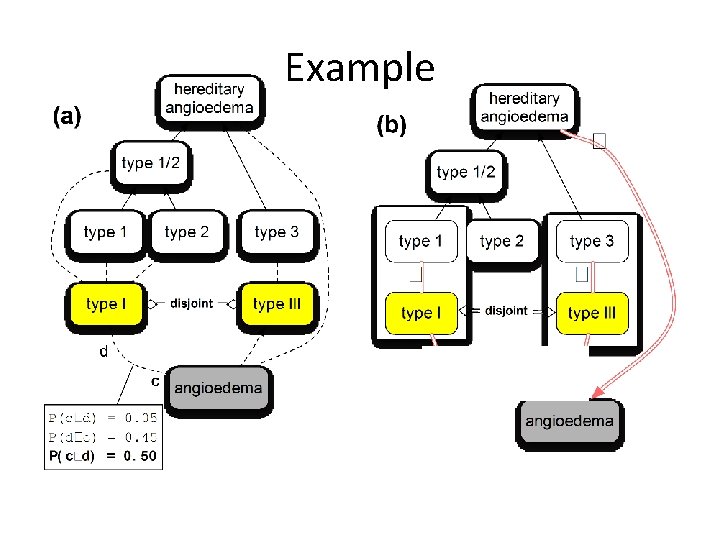
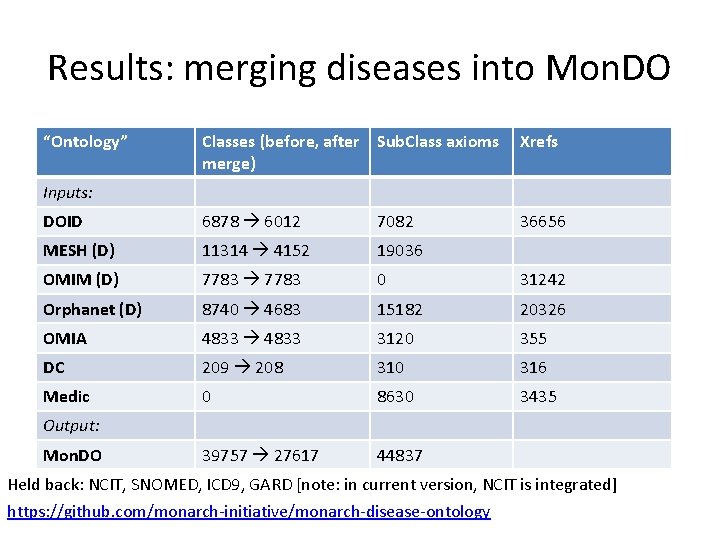
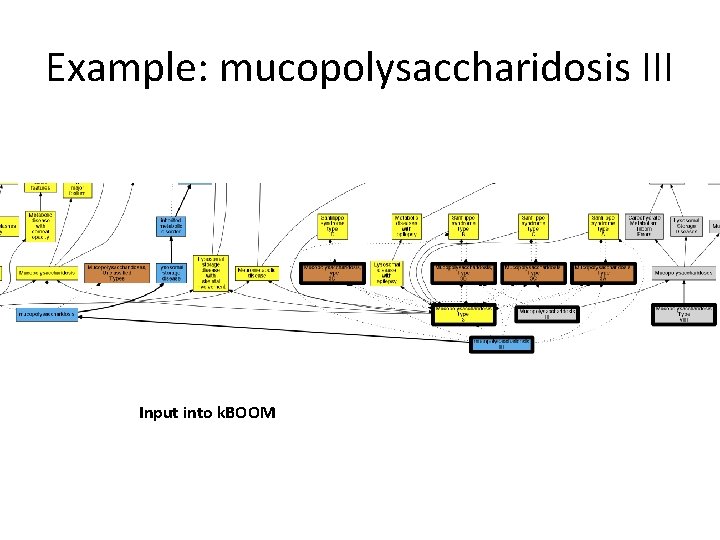
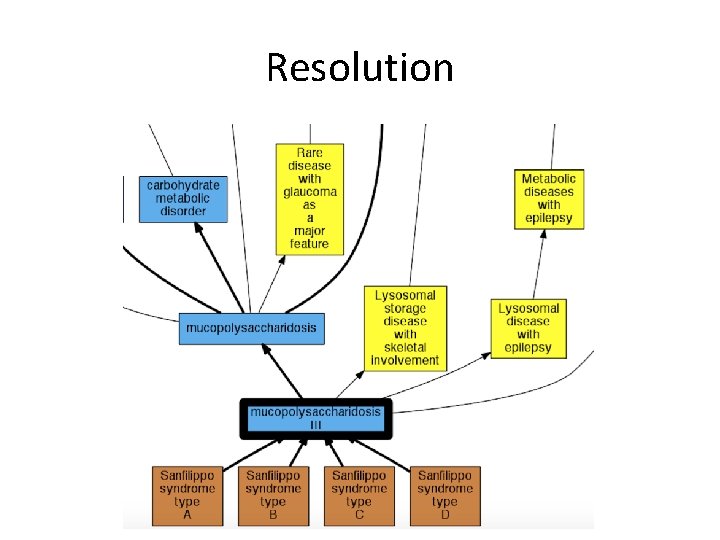
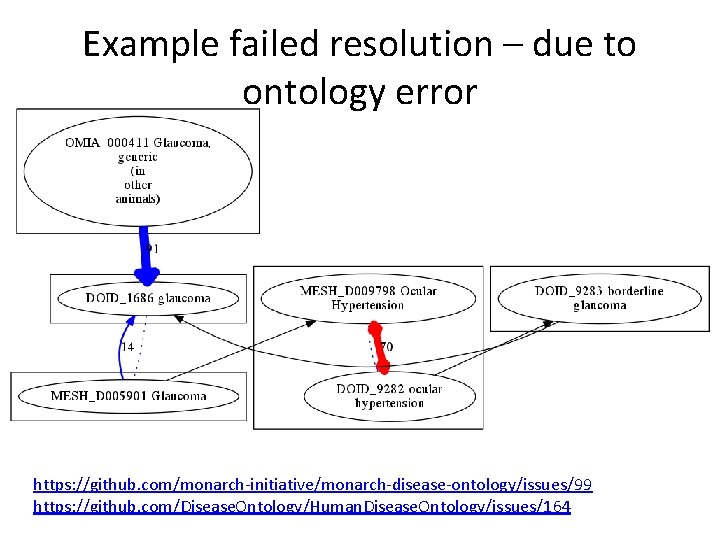
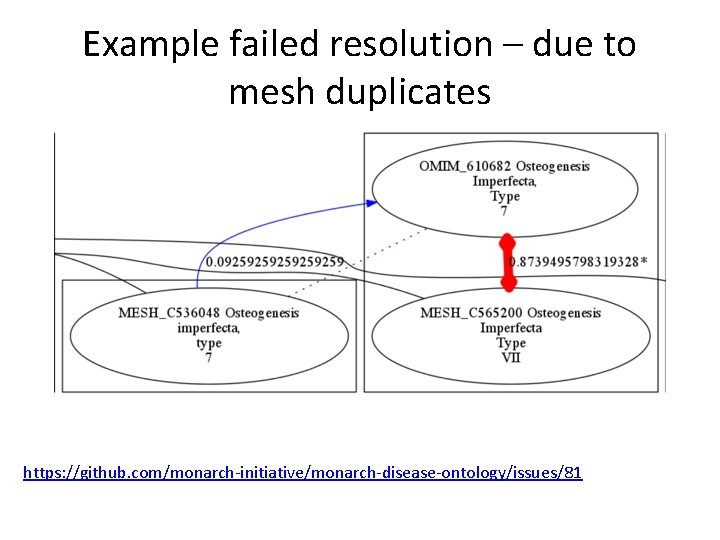
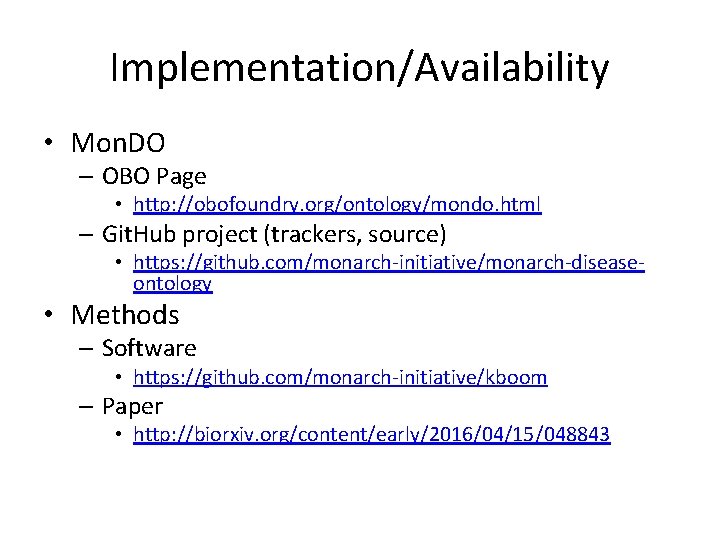
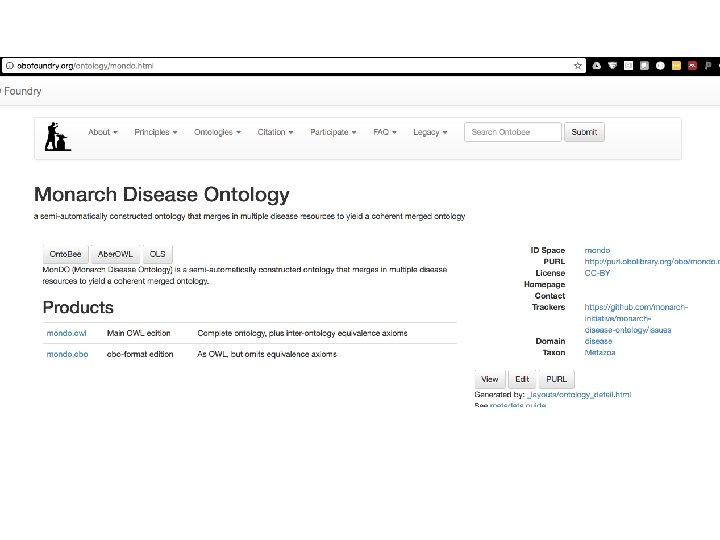
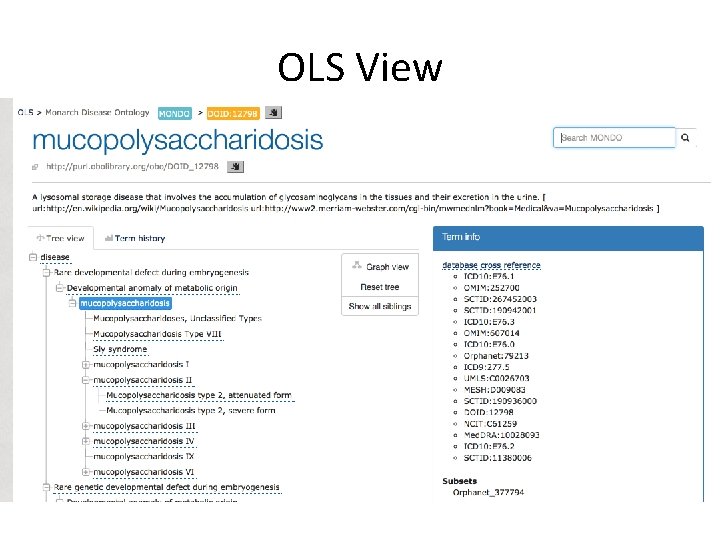
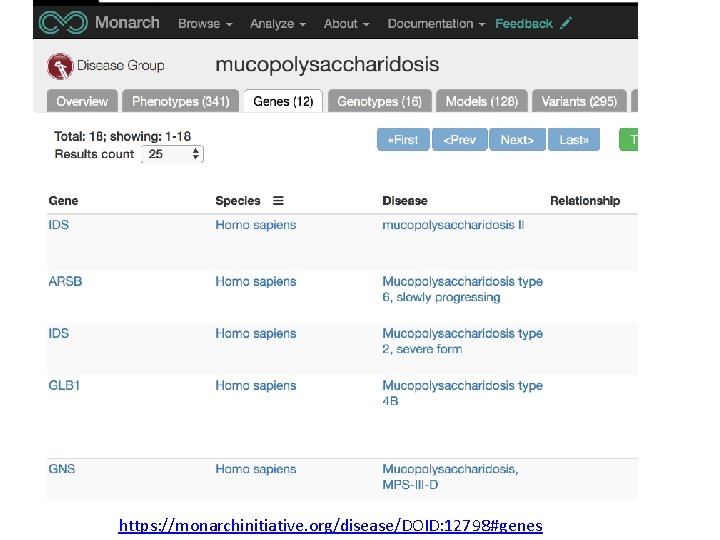
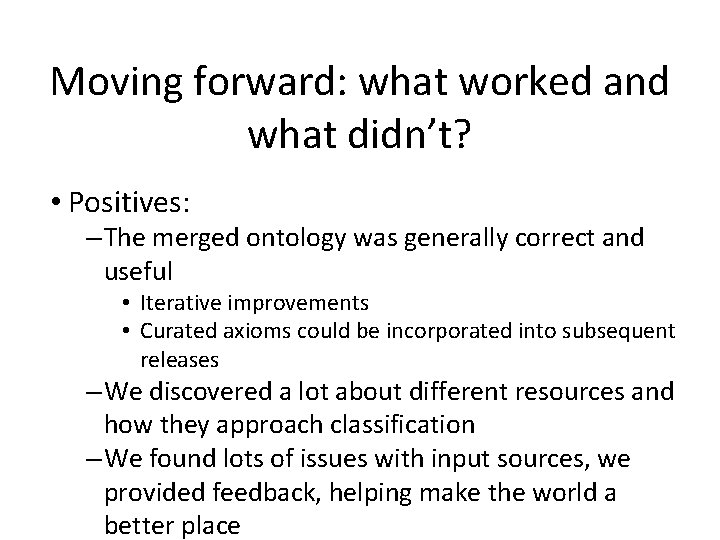
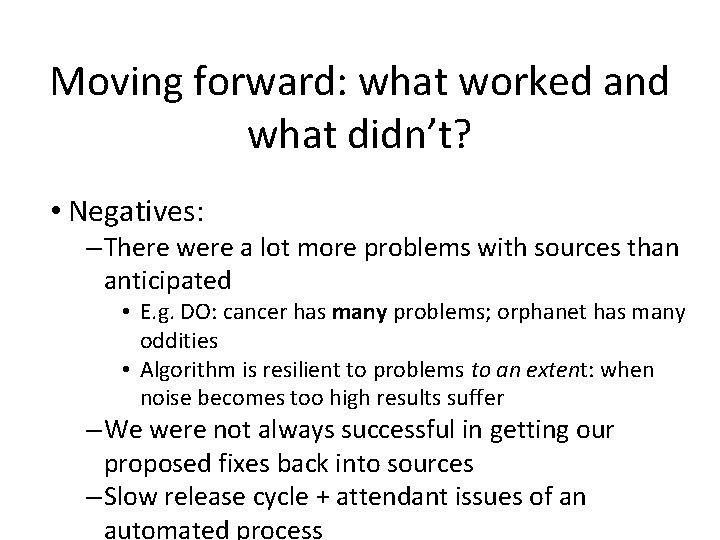
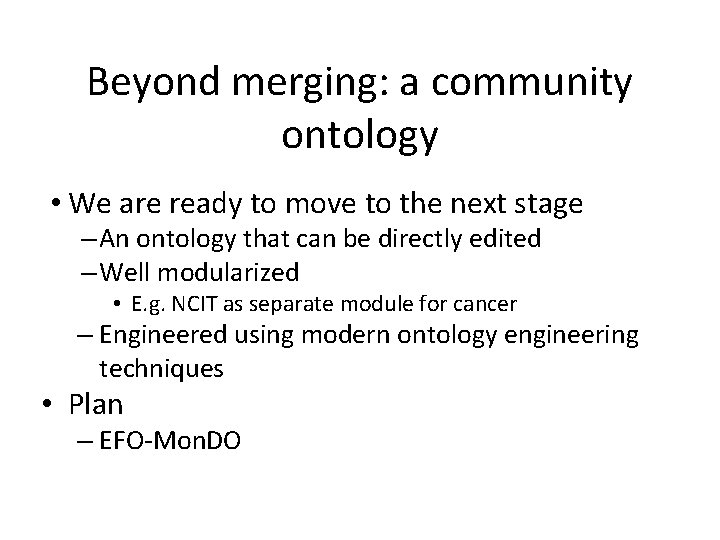
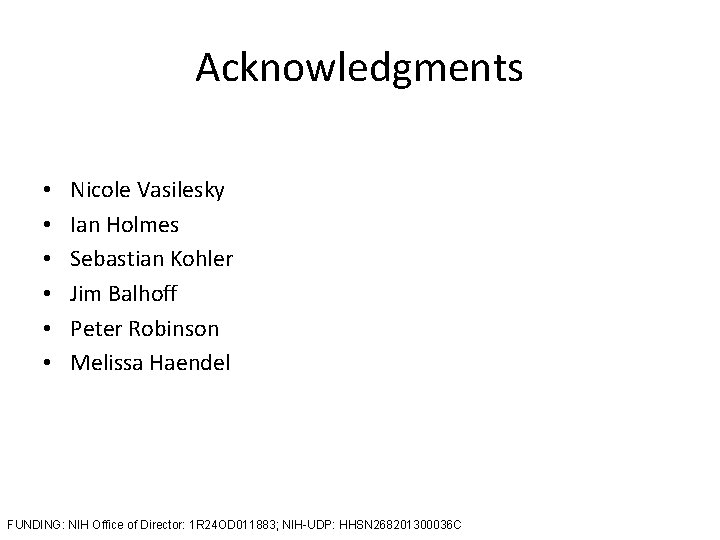
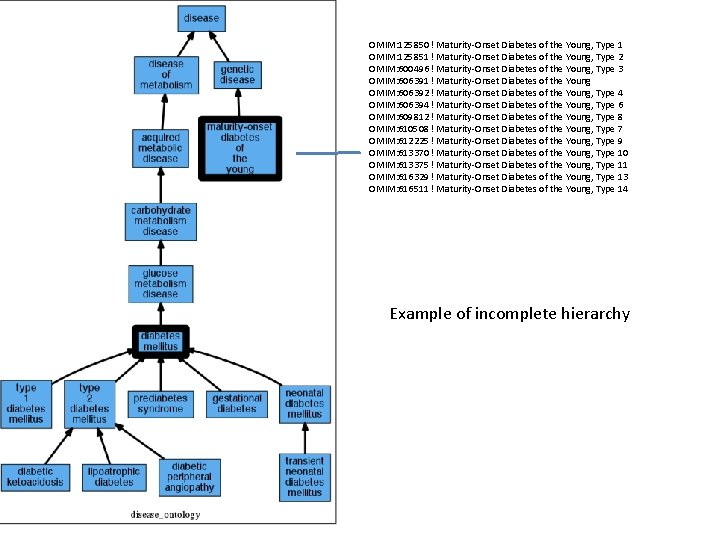
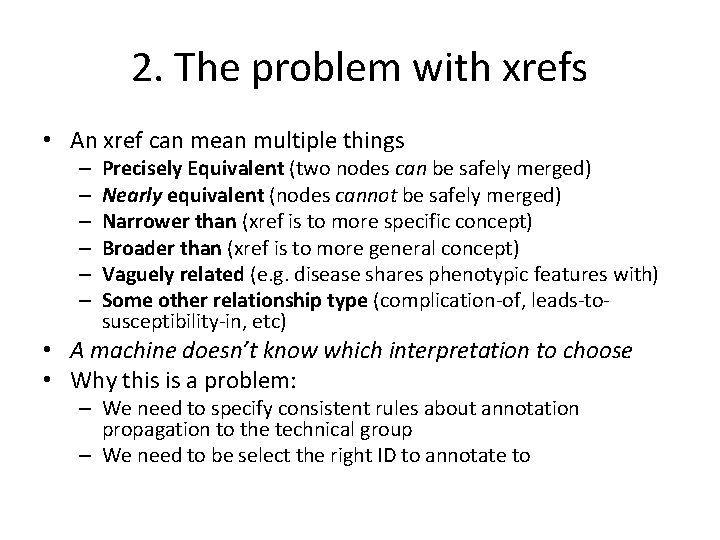
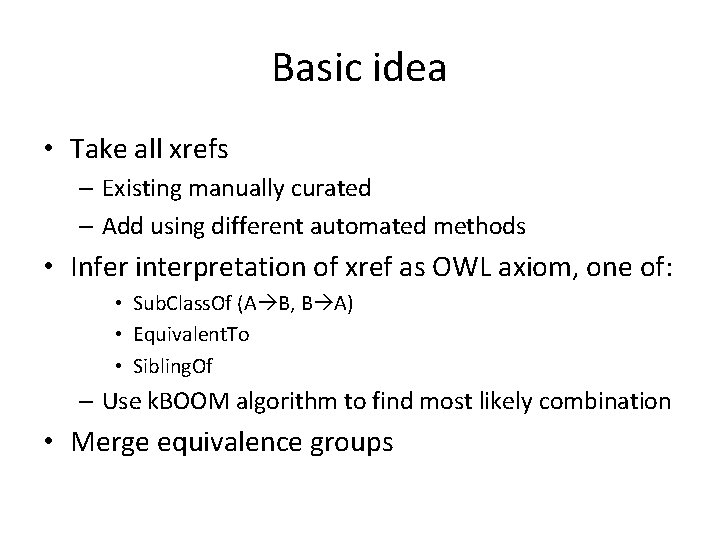
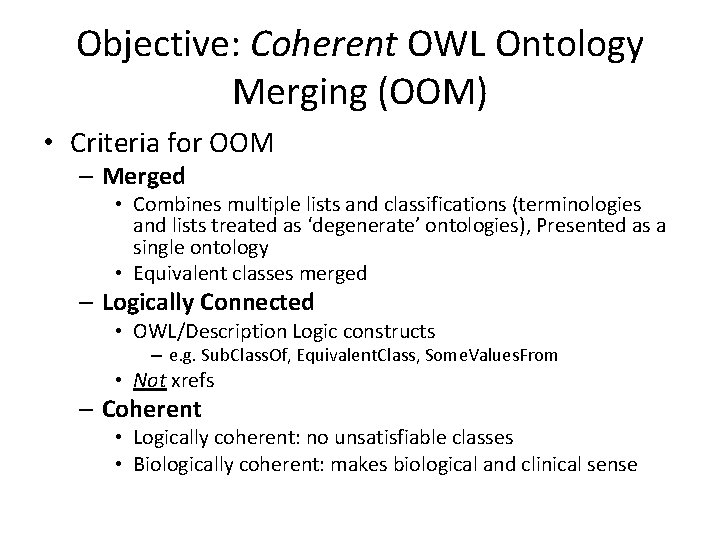
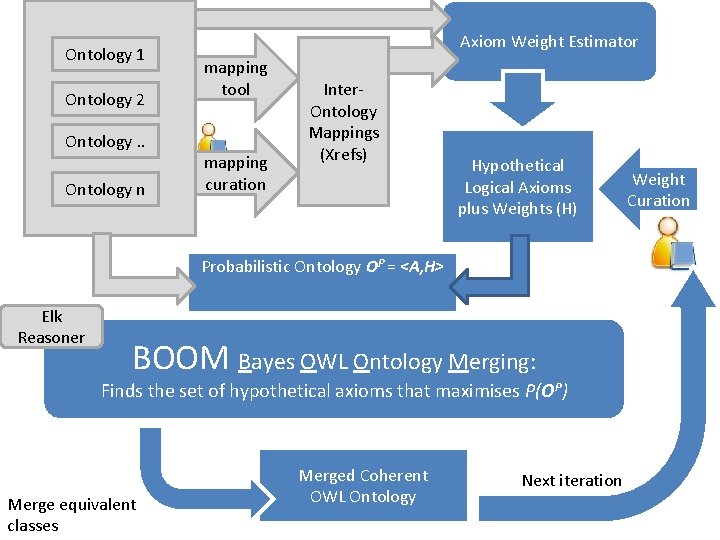
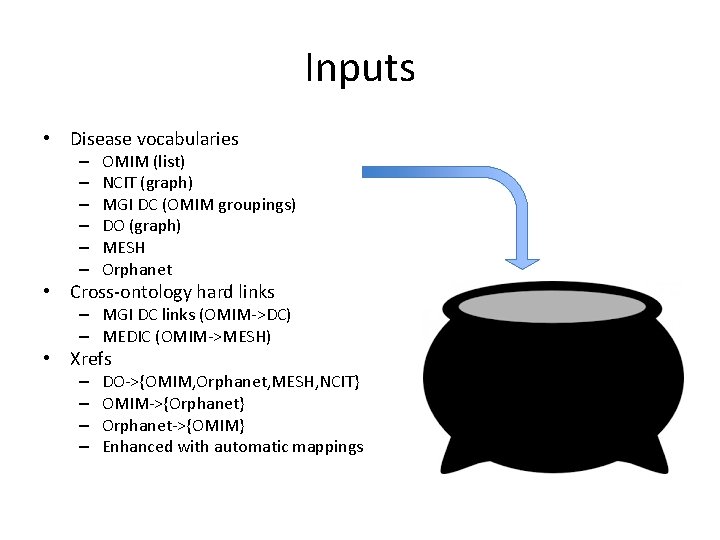
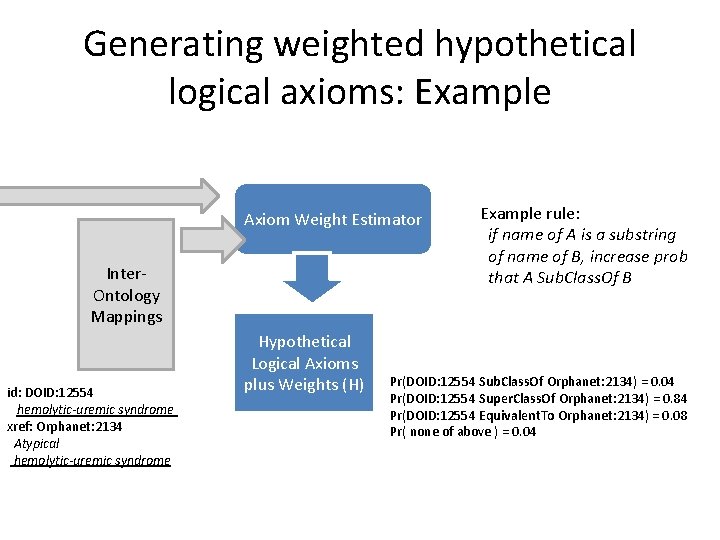
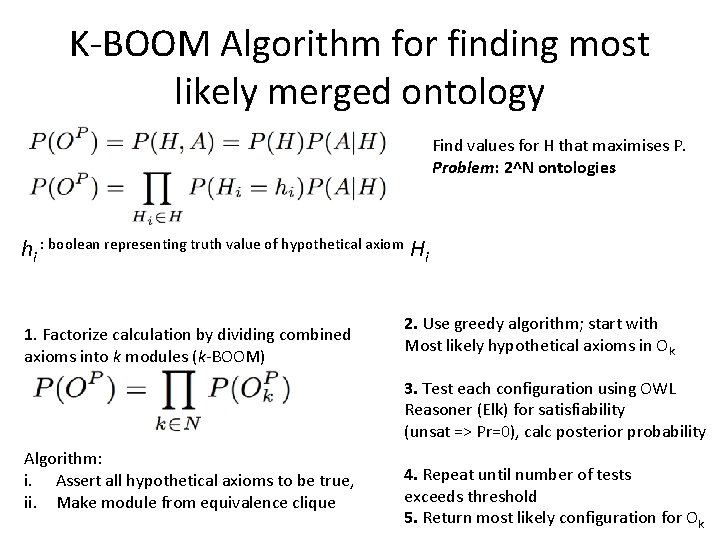
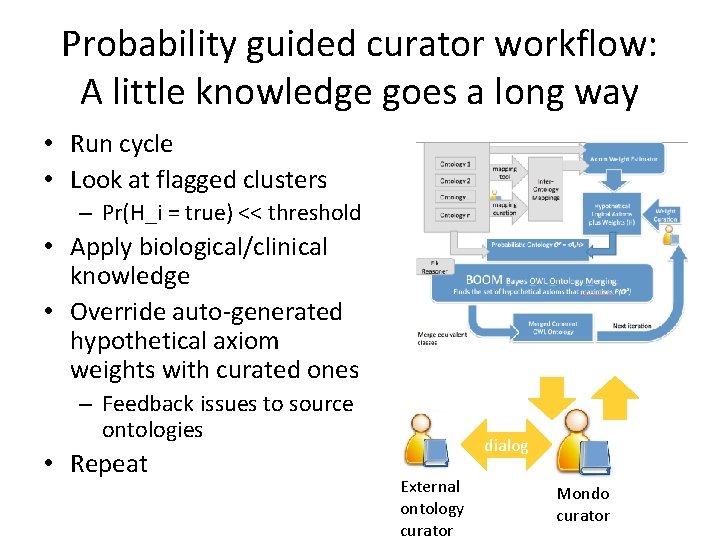
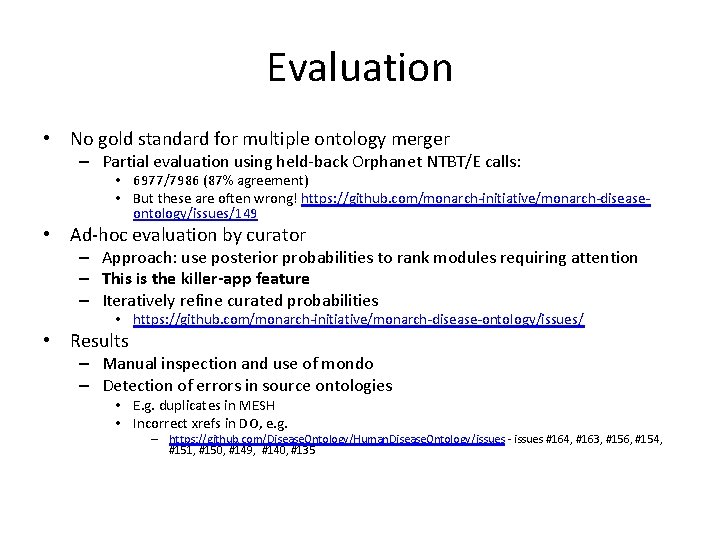
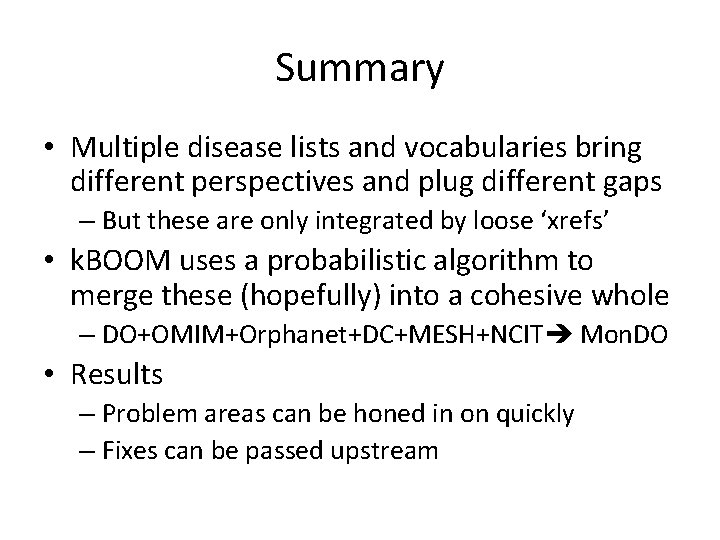
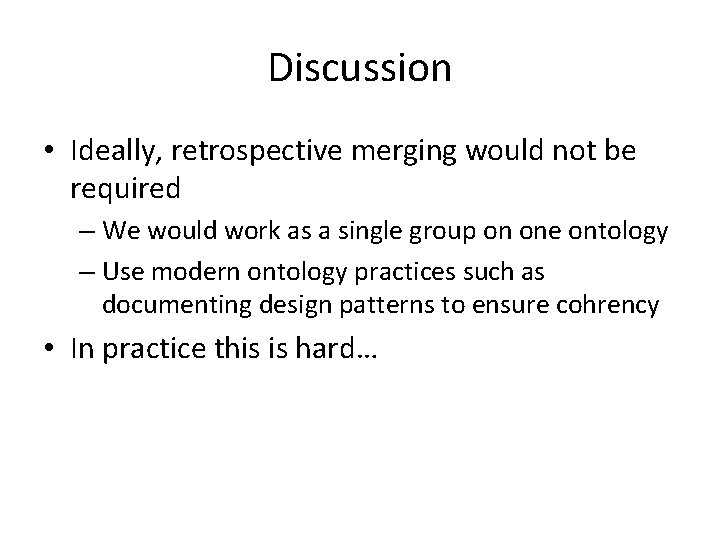
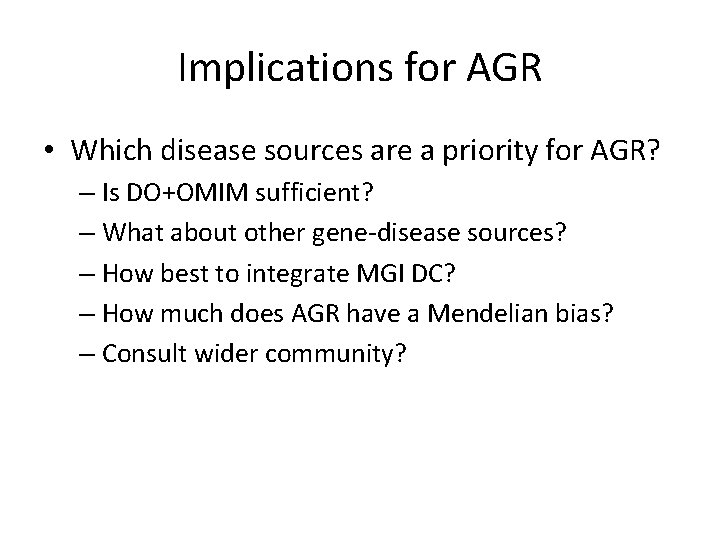
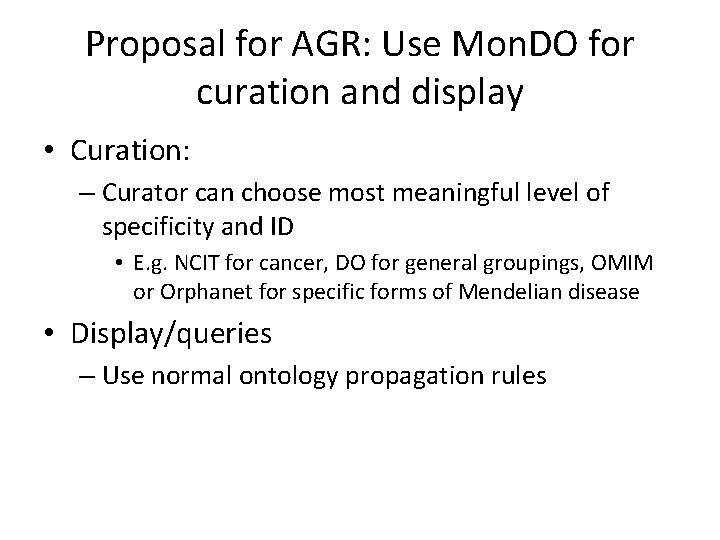
- Slides: 43
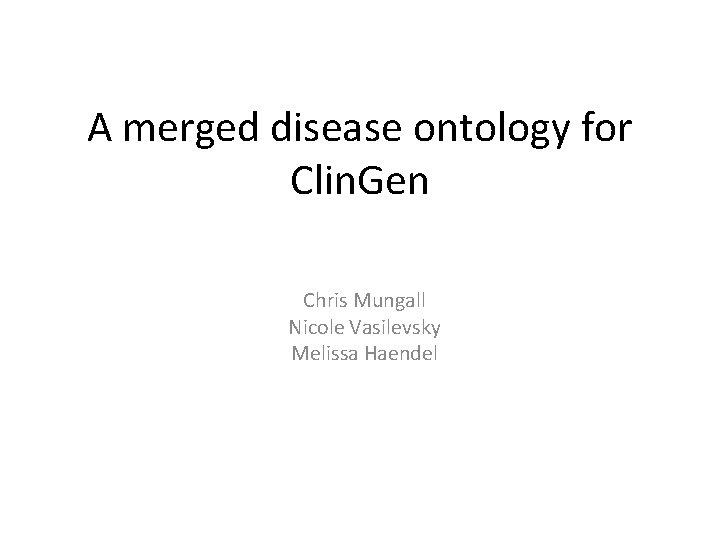
A merged disease ontology for Clin. Gen Chris Mungall Nicole Vasilevsky Melissa Haendel
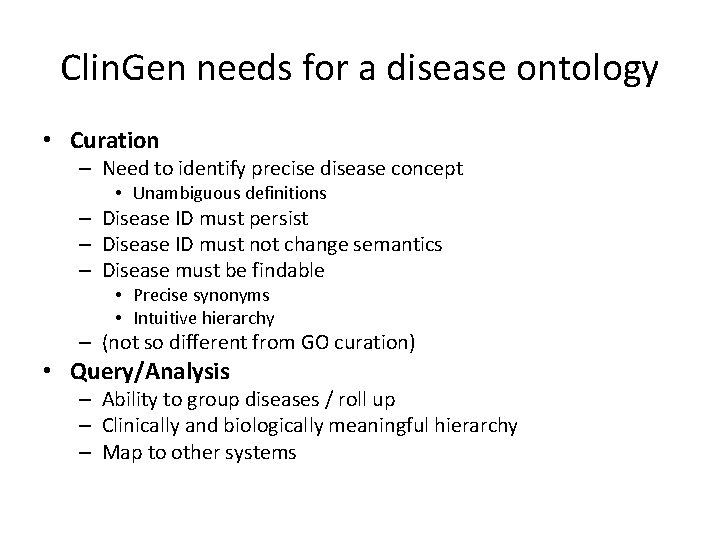
Clin. Gen needs for a disease ontology • Curation – Need to identify precise disease concept • Unambiguous definitions – Disease ID must persist – Disease ID must not change semantics – Disease must be findable • Precise synonyms • Intuitive hierarchy – (not so different from GO curation) • Query/Analysis – Ability to group diseases / roll up – Clinically and biologically meaningful hierarchy – Map to other systems
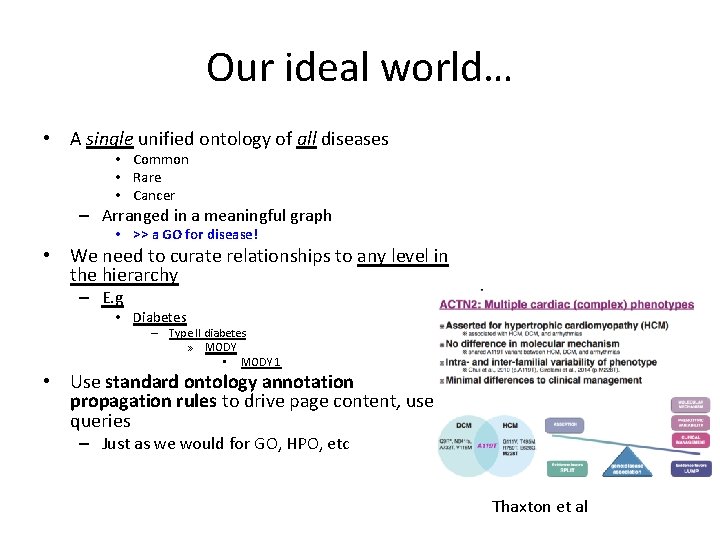
Our ideal world… • A single unified ontology of all diseases • Common • Rare • Cancer – Arranged in a meaningful graph • >> a GO for disease! • We need to curate relationships to any level in the hierarchy – E. g • Diabetes – Type II diabetes » MODY • MODY 1 • Use standard ontology annotation propagation rules to drive page content, user queries – Just as we would for GO, HPO, etc Thaxton et al
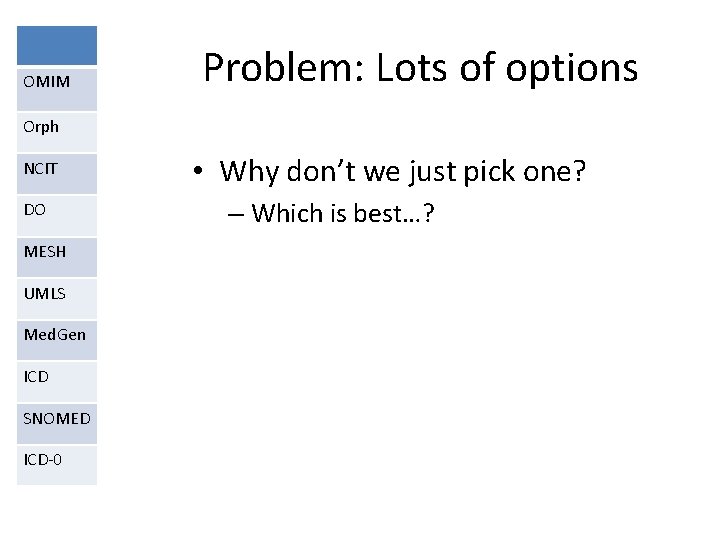
OMIM Problem: Lots of options Orph NCIT DO MESH UMLS Med. Gen ICD SNOMED ICD-0 • Why don’t we just pick one? – Which is best…?
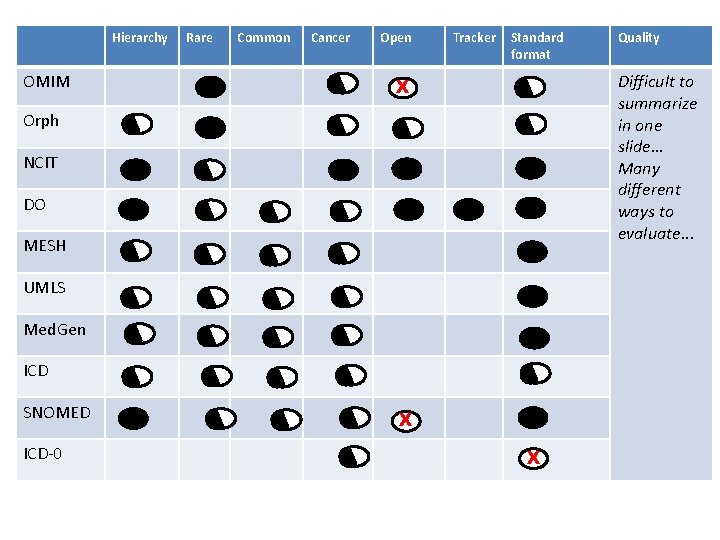
Hierarchy OMIM Rare Common Cancer Open Tracker Standard format Difficult to summarize in one slide… Many different ways to evaluate. . . X Orph NCIT DO MESH UMLS Med. Gen ICD SNOMED ICD-0 Quality X X
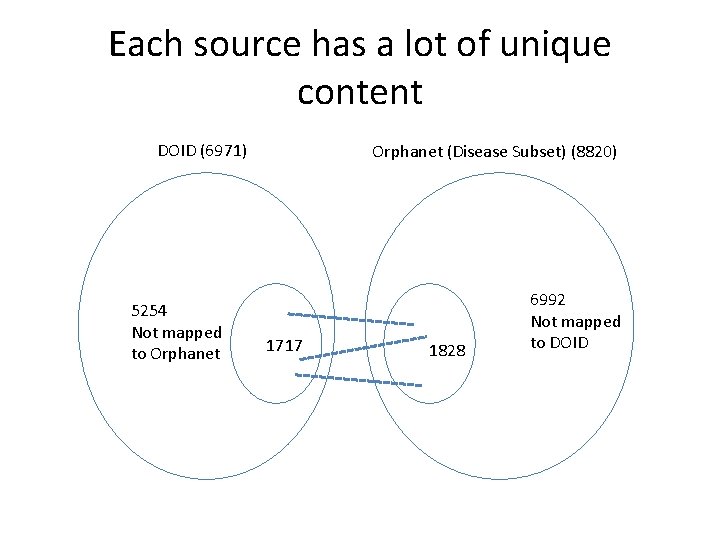
Each source has a lot of unique content DOID (6971) 5254 Not mapped to Orphanet (Disease Subset) (8820) 1717 1828 6992 Not mapped to DOID
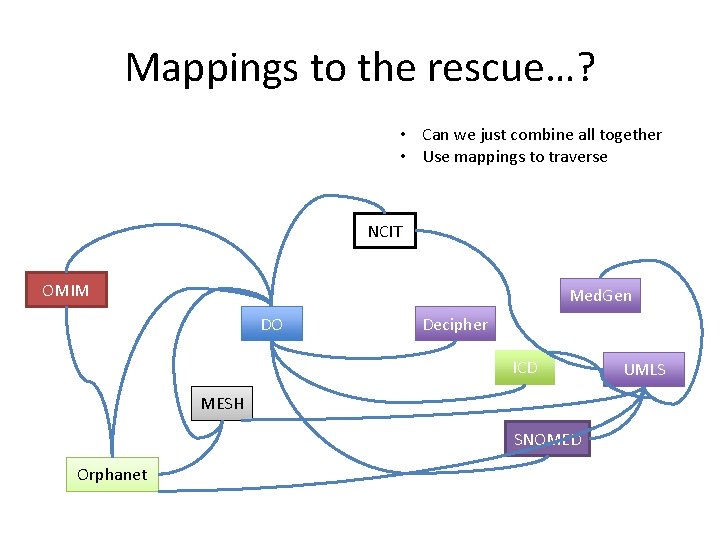
Mappings to the rescue…? • Can we just combine all together • Use mappings to traverse NCIT OMIM Med. Gen DO Decipher ICD MESH SNOMED Orphanet UMLS
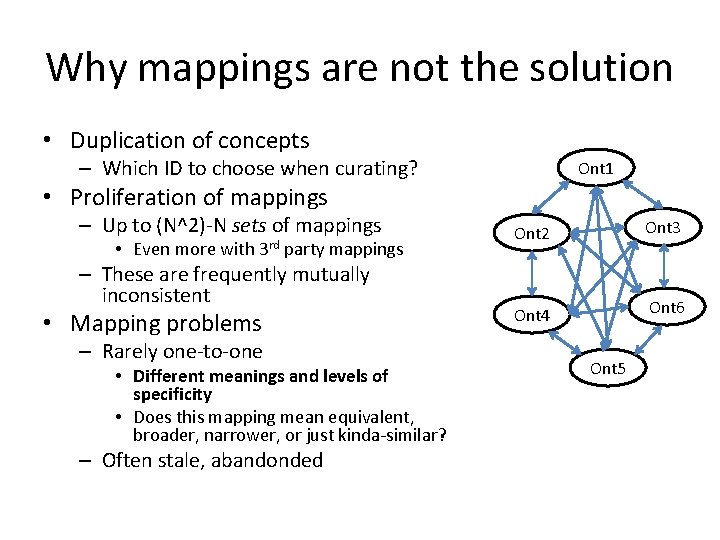
Why mappings are not the solution • Duplication of concepts – Which ID to choose when curating? Ont 1 • Proliferation of mappings – Up to (N^2)-N sets of mappings • Even more with 3 rd party mappings – These are frequently mutually inconsistent • Mapping problems – Rarely one-to-one • Different meanings and levels of specificity • Does this mapping mean equivalent, broader, narrower, or just kinda-similar? – Often stale, abandonded Ont 2 Ont 3 Ont 4 Ont 6 Ont 5
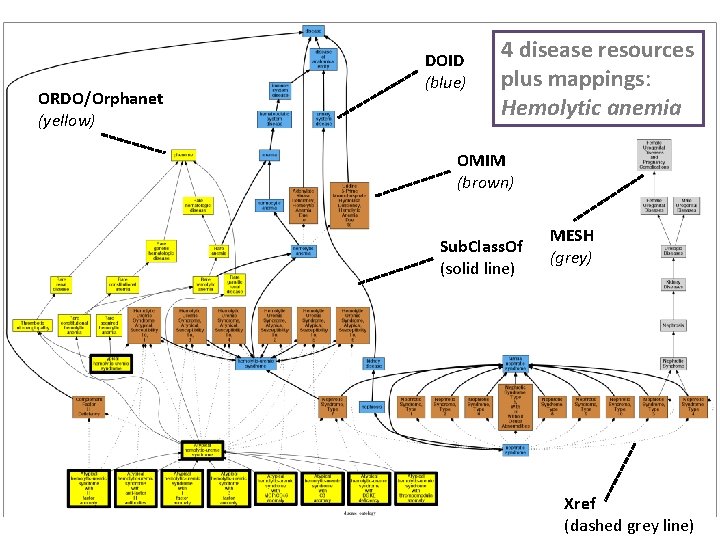
ORDO/Orphanet (yellow) DOID (blue) 4 disease resources plus mappings: Hemolytic anemia OMIM (brown) Sub. Class. Of (solid line) MESH (grey) Xref (dashed grey line)
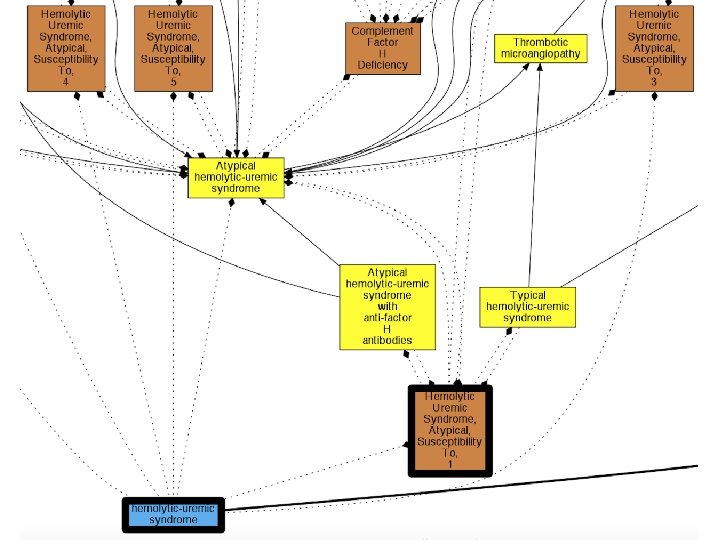
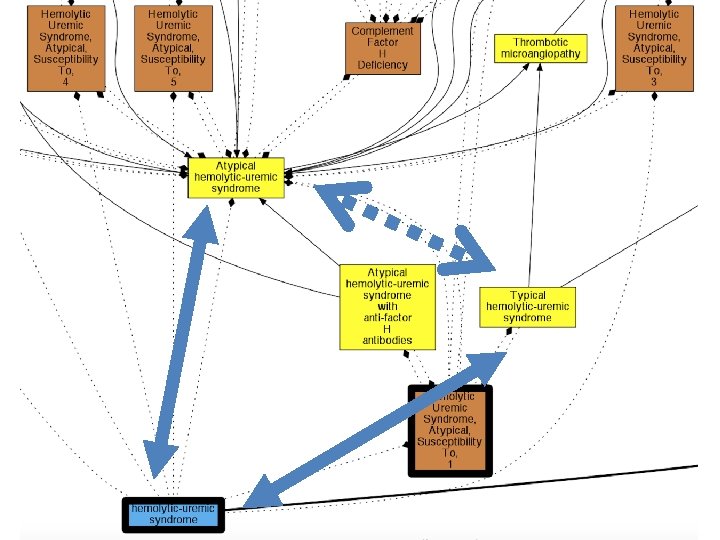
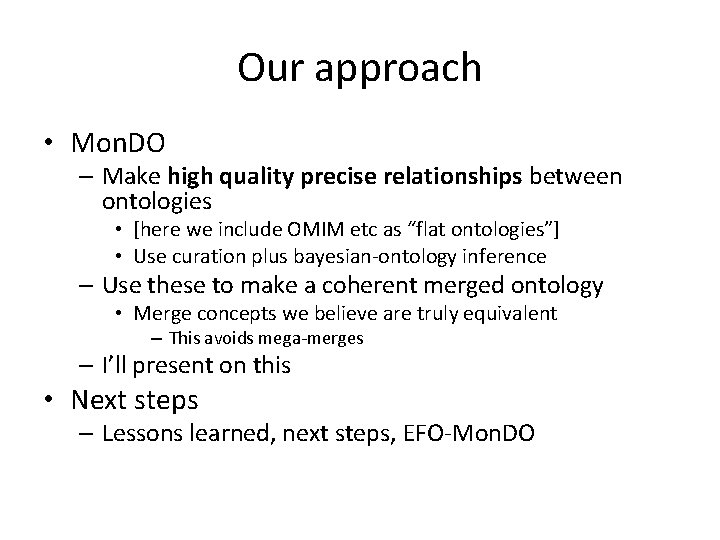
Our approach • Mon. DO – Make high quality precise relationships between ontologies • [here we include OMIM etc as “flat ontologies”] • Use curation plus bayesian-ontology inference – Use these to make a coherent merged ontology • Merge concepts we believe are truly equivalent – This avoids mega-merges – I’ll present on this • Next steps – Lessons learned, next steps, EFO-Mon. DO
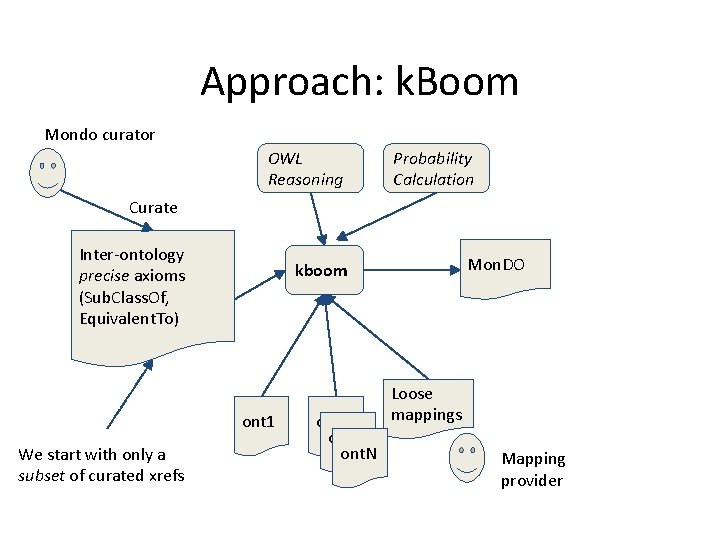
Approach: k. Boom Mondo curator OWL Reasoning Probability Calculation Curate Inter-ontology precise axioms (Sub. Class. Of, Equivalent. To) ont 1 We start with only a subset of curated xrefs Mon. DO kboom ont 2 ont. N Loose mappings Mapping provider
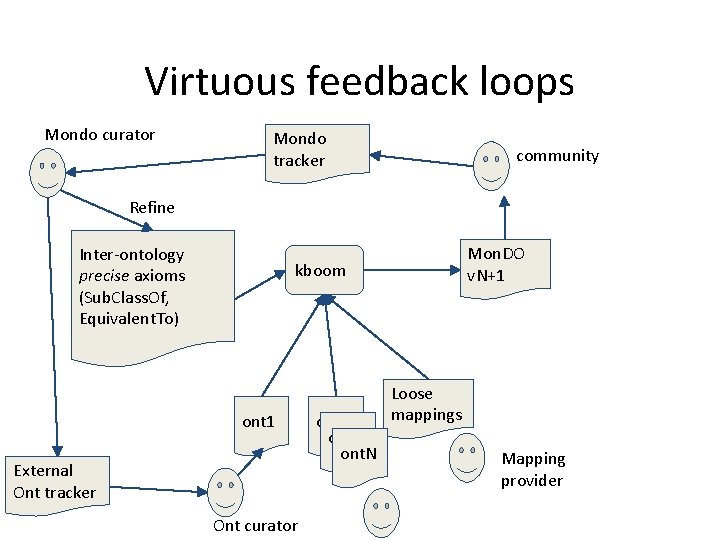
Virtuous feedback loops Mondo curator Mondo tracker community Refine Inter-ontology precise axioms (Sub. Class. Of, Equivalent. To) Mon. DO v. N+1 kboom ont 1 External Ont tracker Ont curator ont 2 ont. N Loose mappings Mapping provider
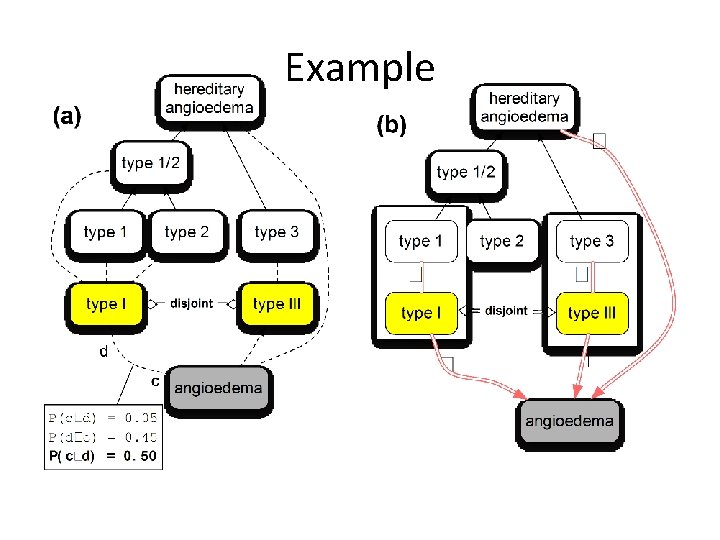
Example
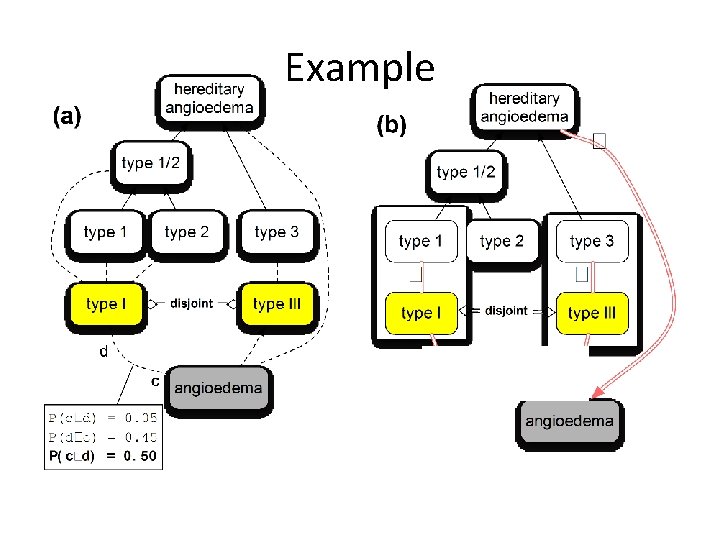
Example
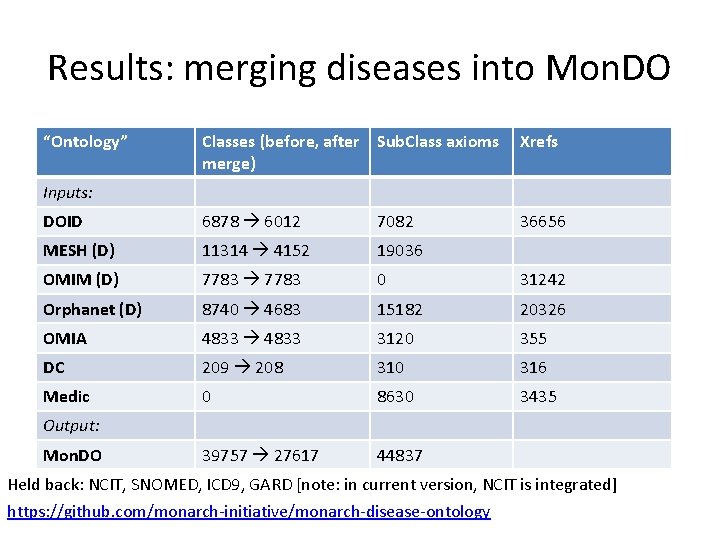
Results: merging diseases into Mon. DO “Ontology” Classes (before, after Sub. Class axioms merge) Xrefs DOID 6878 6012 7082 36656 MESH (D) 11314 4152 19036 OMIM (D) 7783 0 31242 Orphanet (D) 8740 4683 15182 20326 OMIA 4833 3120 355 DC 209 208 310 316 Medic 0 8630 3435 39757 27617 44837 Inputs: Output: Mon. DO Held back: NCIT, SNOMED, ICD 9, GARD [note: in current version, NCIT is integrated] https: //github. com/monarch-initiative/monarch-disease-ontology
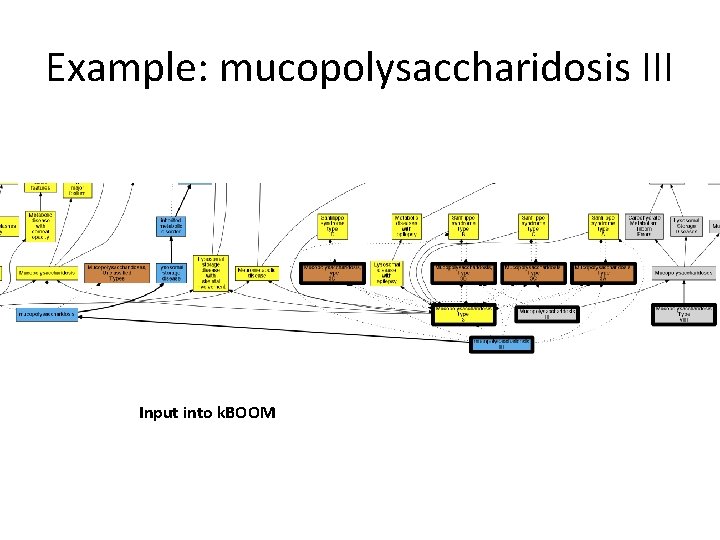
Example: mucopolysaccharidosis III Input into k. BOOM
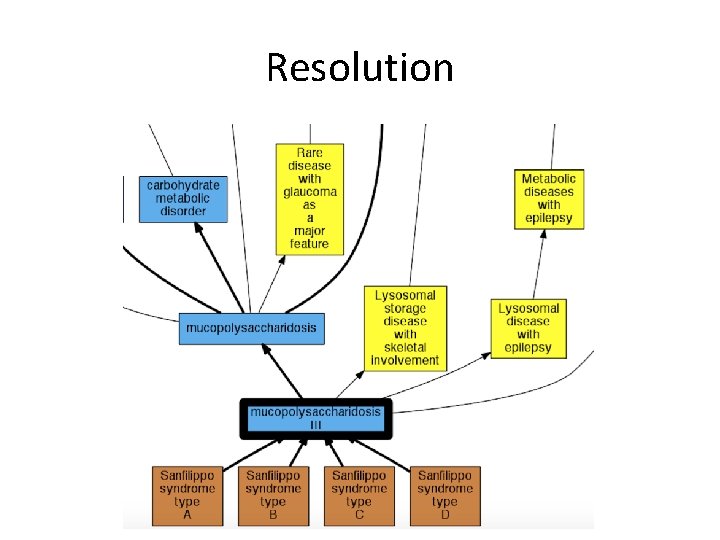
Resolution
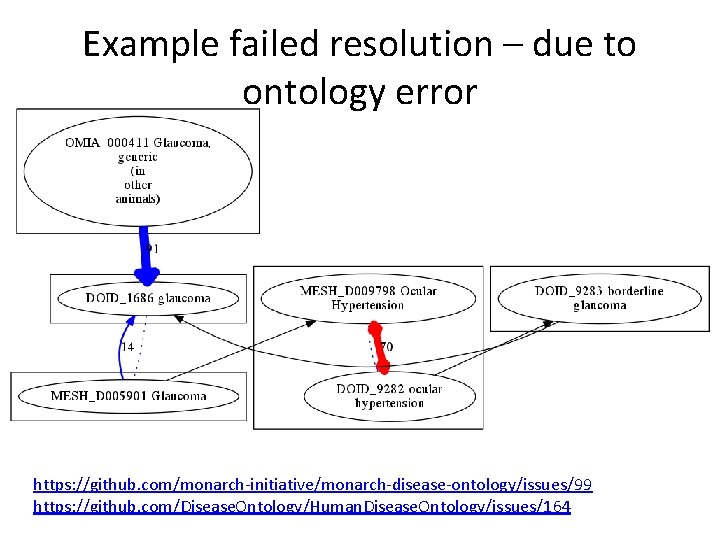
Example failed resolution – due to ontology error https: //github. com/monarch-initiative/monarch-disease-ontology/issues/99 https: //github. com/Disease. Ontology/Human. Disease. Ontology/issues/164
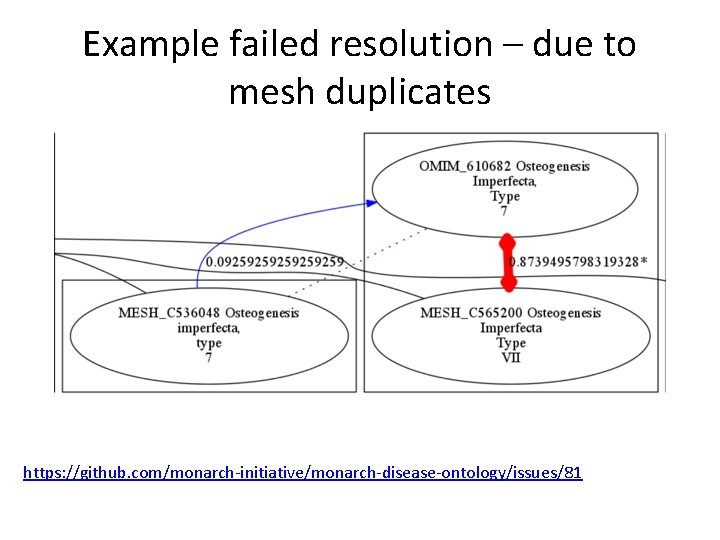
Example failed resolution – due to mesh duplicates https: //github. com/monarch-initiative/monarch-disease-ontology/issues/81
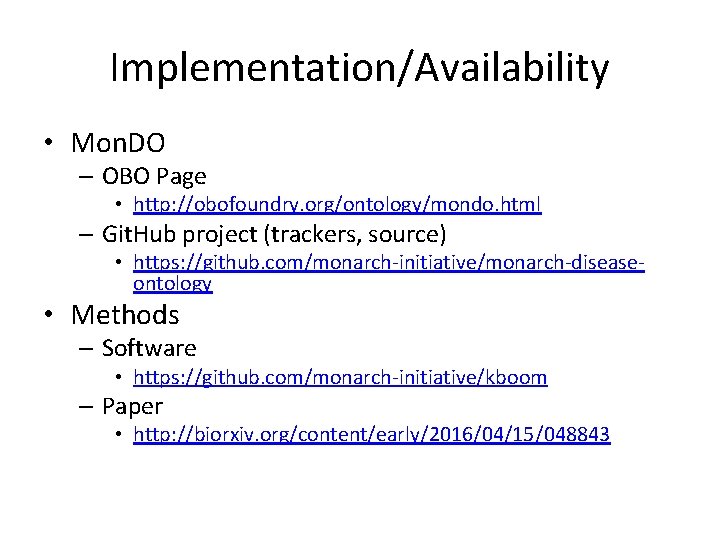
Implementation/Availability • Mon. DO – OBO Page • http: //obofoundry. org/ontology/mondo. html – Git. Hub project (trackers, source) • https: //github. com/monarch-initiative/monarch-diseaseontology • Methods – Software • https: //github. com/monarch-initiative/kboom – Paper • http: //biorxiv. org/content/early/2016/04/15/048843
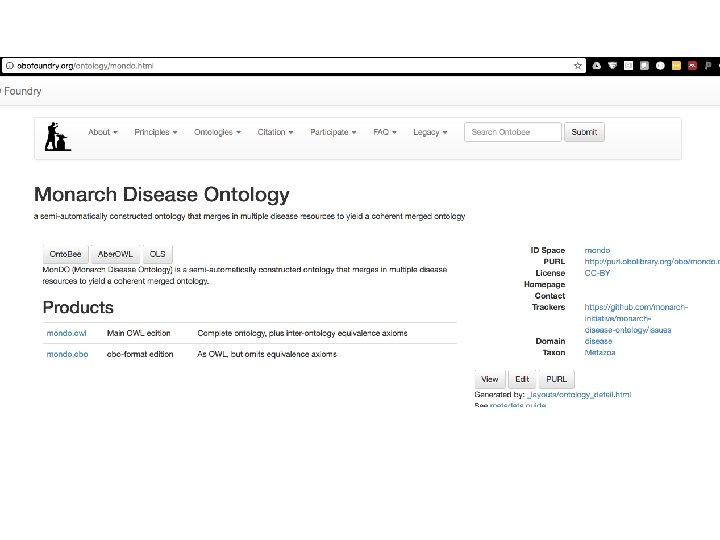
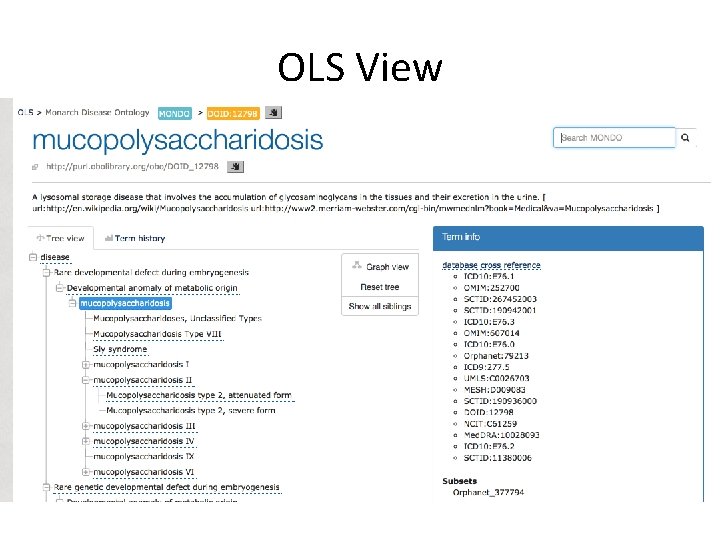
OLS View
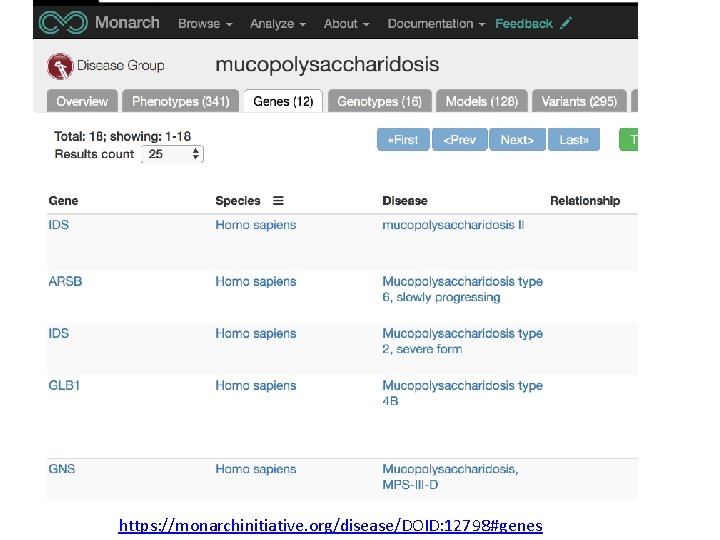
https: //monarchinitiative. org/disease/DOID: 12798#genes
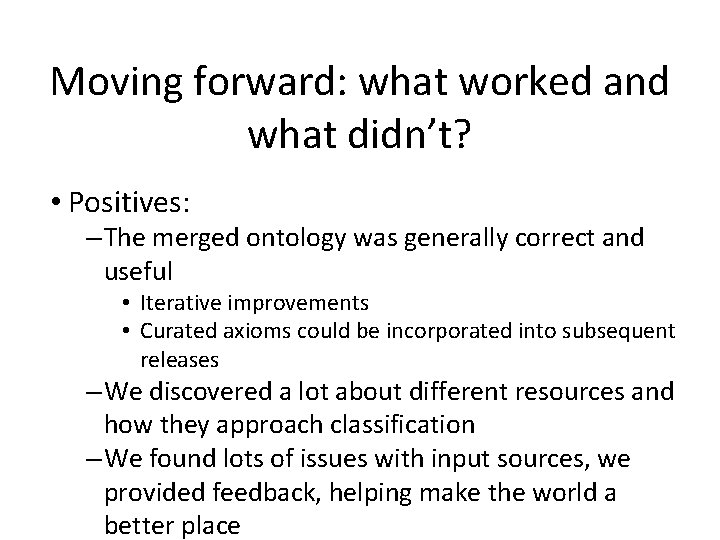
Moving forward: what worked and what didn’t? • Positives: – The merged ontology was generally correct and useful • Iterative improvements • Curated axioms could be incorporated into subsequent releases – We discovered a lot about different resources and how they approach classification – We found lots of issues with input sources, we provided feedback, helping make the world a better place
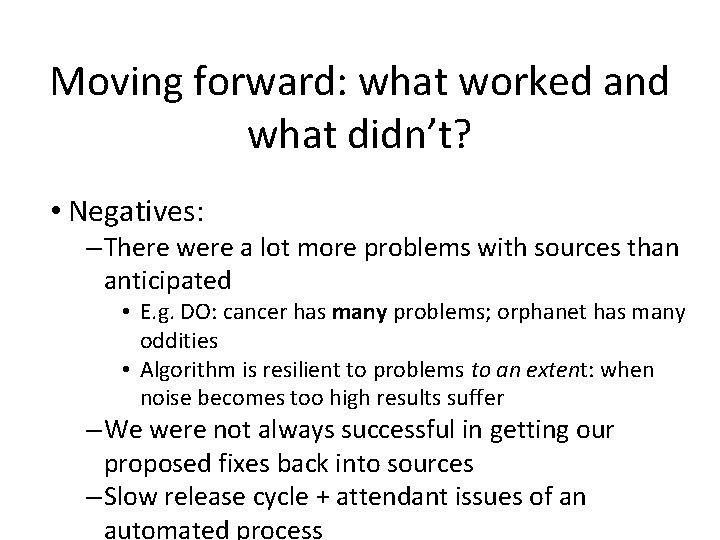
Moving forward: what worked and what didn’t? • Negatives: – There were a lot more problems with sources than anticipated • E. g. DO: cancer has many problems; orphanet has many oddities • Algorithm is resilient to problems to an extent: when noise becomes too high results suffer – We were not always successful in getting our proposed fixes back into sources – Slow release cycle + attendant issues of an automated process
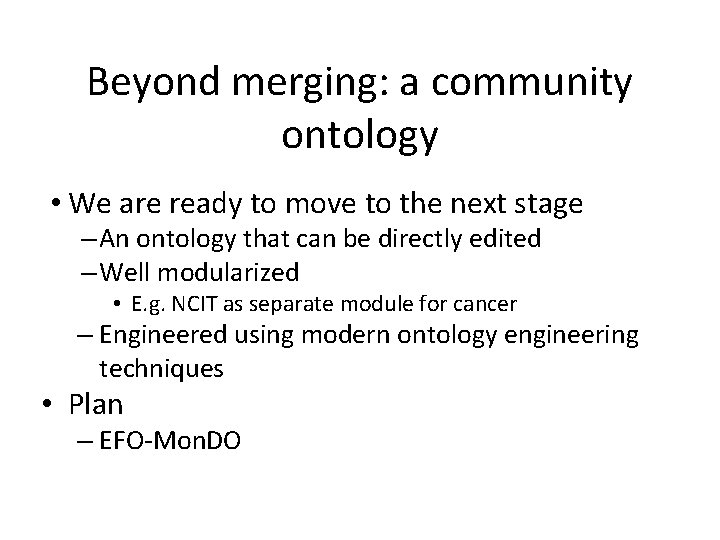
Beyond merging: a community ontology • We are ready to move to the next stage – An ontology that can be directly edited – Well modularized • E. g. NCIT as separate module for cancer – Engineered using modern ontology engineering techniques • Plan – EFO-Mon. DO
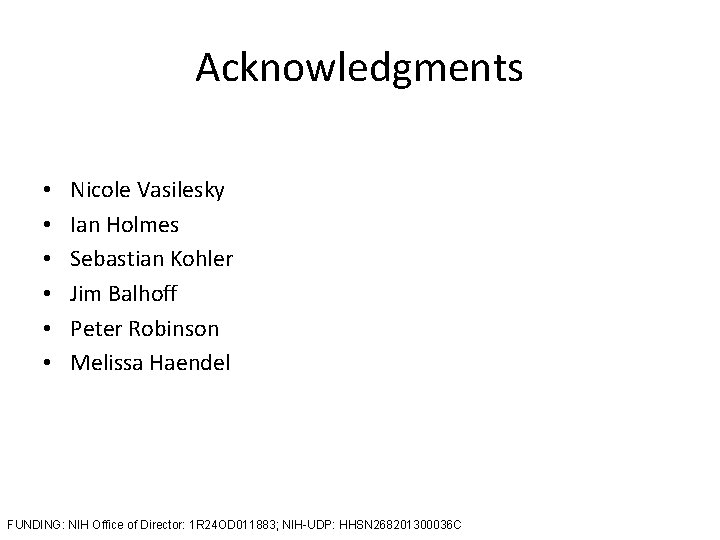
Acknowledgments • • • Nicole Vasilesky Ian Holmes Sebastian Kohler Jim Balhoff Peter Robinson Melissa Haendel FUNDING: NIH Office of Director: 1 R 24 OD 011883; NIH-UDP: HHSN 268201300036 C
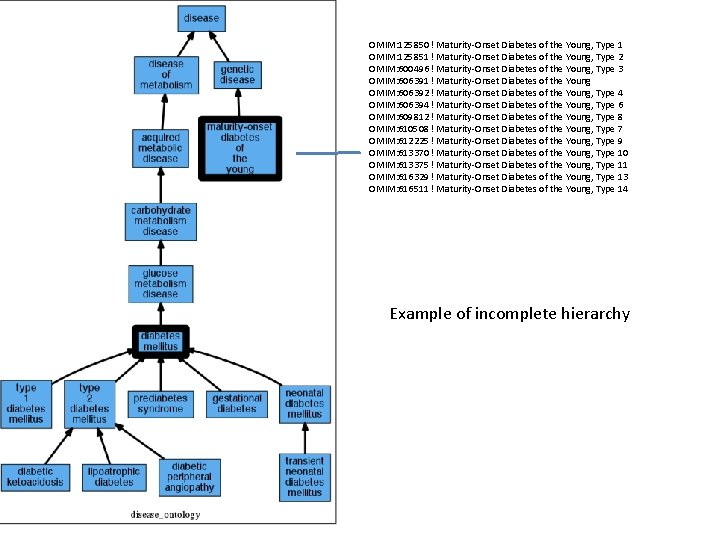
OMIM: 125850 ! Maturity-Onset Diabetes of the Young, Type 1 OMIM: 125851 ! Maturity-Onset Diabetes of the Young, Type 2 OMIM: 600496 ! Maturity-Onset Diabetes of the Young, Type 3 OMIM: 606391 ! Maturity-Onset Diabetes of the Young OMIM: 606392 ! Maturity-Onset Diabetes of the Young, Type 4 OMIM: 606394 ! Maturity-Onset Diabetes of the Young, Type 6 OMIM: 609812 ! Maturity-Onset Diabetes of the Young, Type 8 OMIM: 610508 ! Maturity-Onset Diabetes of the Young, Type 7 OMIM: 612225 ! Maturity-Onset Diabetes of the Young, Type 9 OMIM: 613370 ! Maturity-Onset Diabetes of the Young, Type 10 OMIM: 613375 ! Maturity-Onset Diabetes of the Young, Type 11 OMIM: 616329 ! Maturity-Onset Diabetes of the Young, Type 13 OMIM: 616511 ! Maturity-Onset Diabetes of the Young, Type 14 Example of incomplete hierarchy
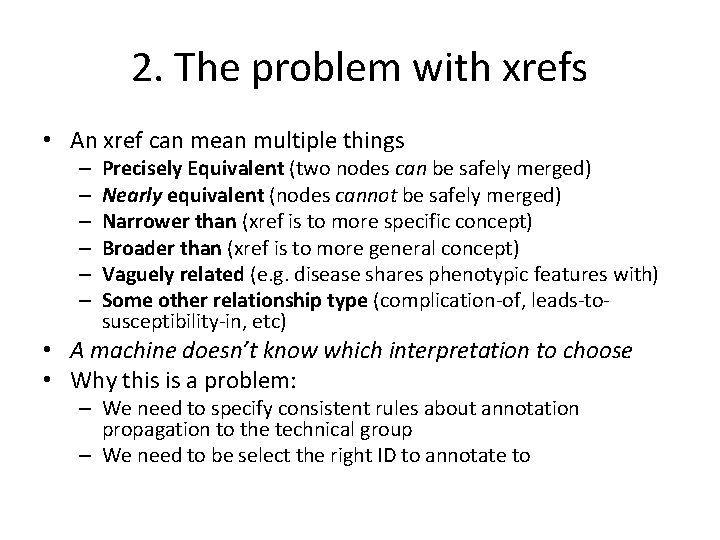
2. The problem with xrefs • An xref can mean multiple things – – – Precisely Equivalent (two nodes can be safely merged) Nearly equivalent (nodes cannot be safely merged) Narrower than (xref is to more specific concept) Broader than (xref is to more general concept) Vaguely related (e. g. disease shares phenotypic features with) Some other relationship type (complication-of, leads-tosusceptibility-in, etc) • A machine doesn’t know which interpretation to choose • Why this is a problem: – We need to specify consistent rules about annotation propagation to the technical group – We need to be select the right ID to annotate to
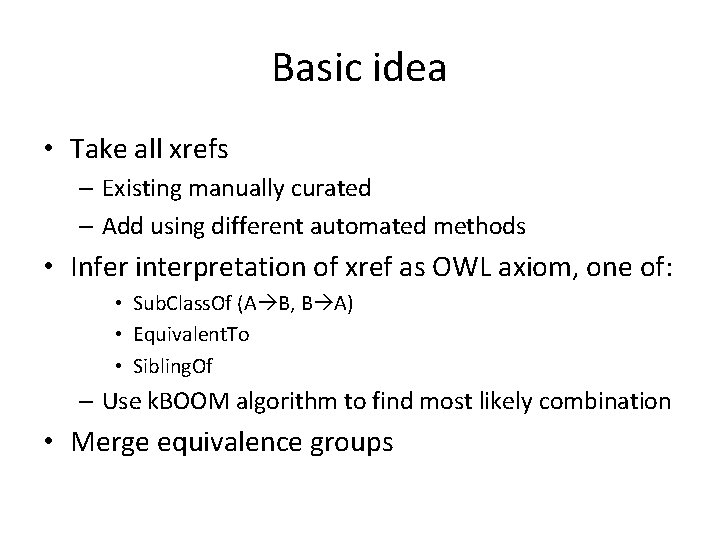
Basic idea • Take all xrefs – Existing manually curated – Add using different automated methods • Infer interpretation of xref as OWL axiom, one of: • Sub. Class. Of (A B, B A) • Equivalent. To • Sibling. Of – Use k. BOOM algorithm to find most likely combination • Merge equivalence groups
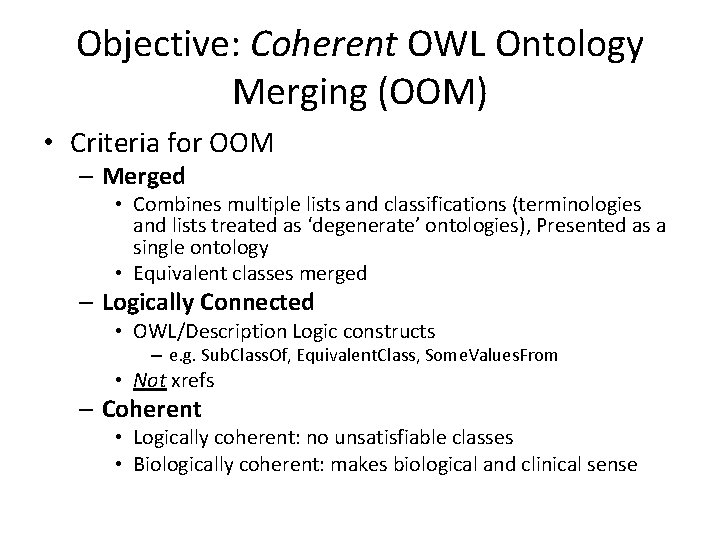
Objective: Coherent OWL Ontology Merging (OOM) • Criteria for OOM – Merged • Combines multiple lists and classifications (terminologies and lists treated as ‘degenerate’ ontologies), Presented as a single ontology • Equivalent classes merged – Logically Connected • OWL/Description Logic constructs – e. g. Sub. Class. Of, Equivalent. Class, Some. Values. From • Not xrefs – Coherent • Logically coherent: no unsatisfiable classes • Biologically coherent: makes biological and clinical sense
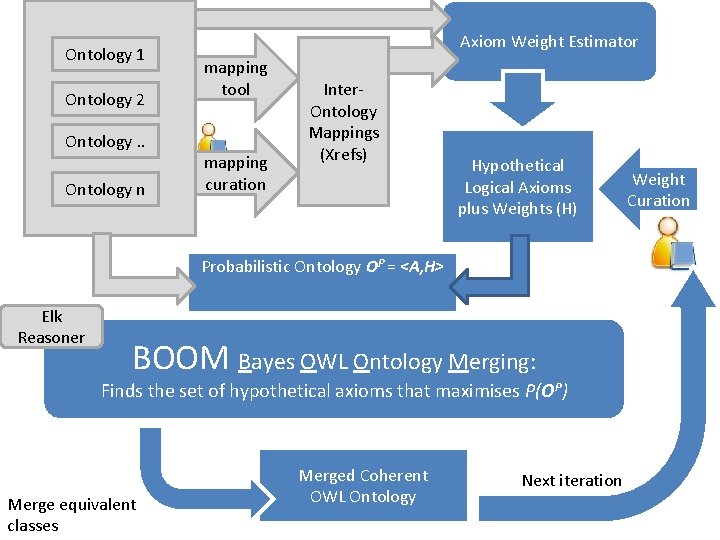
Ontology 1 Ontology 2 Ontology. . Ontology n Axiom Weight Estimator mapping tool mapping curation Inter. Ontology Mappings (Xrefs) Hypothetical Logical Axioms plus Weights (H) Probabilistic Ontology OP = <A, H> Elk Reasoner BOOM Bayes OWL Ontology Merging: Finds the set of hypothetical axioms that maximises P(OP) Merge equivalent classes Merged Coherent OWL Ontology Next iteration Weight Curation
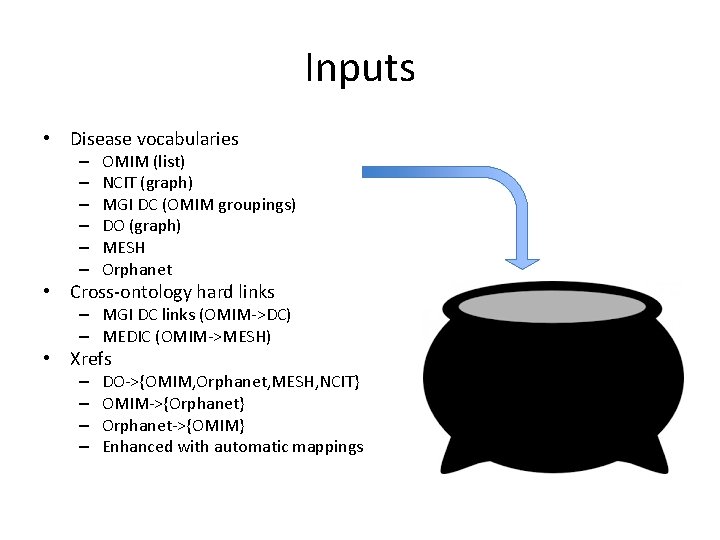
Inputs • Disease vocabularies – – – OMIM (list) NCIT (graph) MGI DC (OMIM groupings) DO (graph) MESH Orphanet • Cross-ontology hard links – MGI DC links (OMIM->DC) – MEDIC (OMIM->MESH) • Xrefs – – DO->{OMIM, Orphanet, MESH, NCIT} OMIM->{Orphanet} Orphanet->{OMIM} Enhanced with automatic mappings
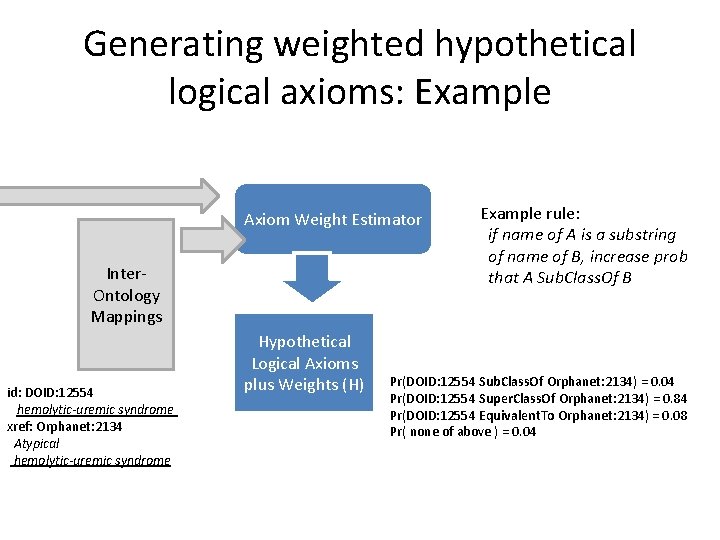
Generating weighted hypothetical logical axioms: Example Axiom Weight Estimator Inter. Ontology Mappings id: DOID: 12554 hemolytic-uremic syndrome xref: Orphanet: 2134 Atypical hemolytic-uremic syndrome Hypothetical Logical Axioms plus Weights (H) Example rule: if name of A is a substring of name of B, increase prob that A Sub. Class. Of B Pr(DOID: 12554 Sub. Class. Of Orphanet: 2134) = 0. 04 Pr(DOID: 12554 Super. Class. Of Orphanet: 2134) = 0. 84 Pr(DOID: 12554 Equivalent. To Orphanet: 2134) = 0. 08 Pr( none of above ) = 0. 04
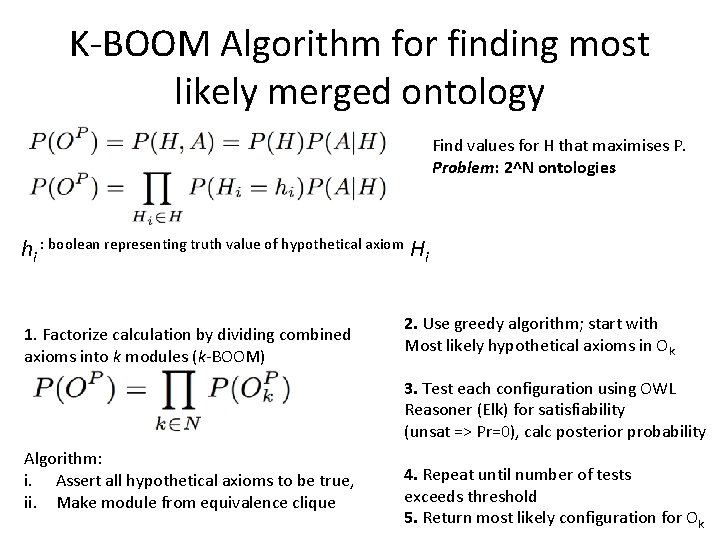
K-BOOM Algorithm for finding most likely merged ontology Find values for H that maximises P. Problem: 2^N ontologies hi : boolean representing truth value of hypothetical axiom Hi 1. Factorize calculation by dividing combined axioms into k modules (k-BOOM) 2. Use greedy algorithm; start with Most likely hypothetical axioms in Ok 3. Test each configuration using OWL Reasoner (Elk) for satisfiability (unsat => Pr=0), calc posterior probability Algorithm: i. Assert all hypothetical axioms to be true, ii. Make module from equivalence clique 4. Repeat until number of tests exceeds threshold 5. Return most likely configuration for Ok
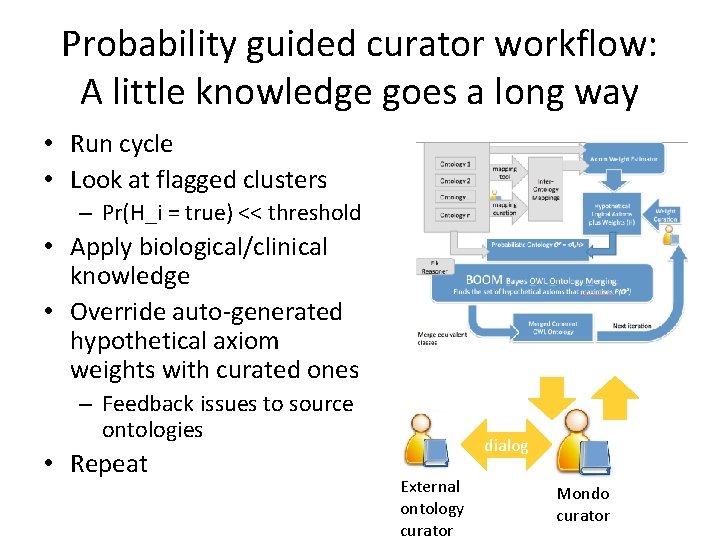
Probability guided curator workflow: A little knowledge goes a long way • Run cycle • Look at flagged clusters – Pr(H_i = true) << threshold • Apply biological/clinical knowledge • Override auto-generated hypothetical axiom weights with curated ones – Feedback issues to source ontologies • Repeat dialog External ontology curator Mondo curator
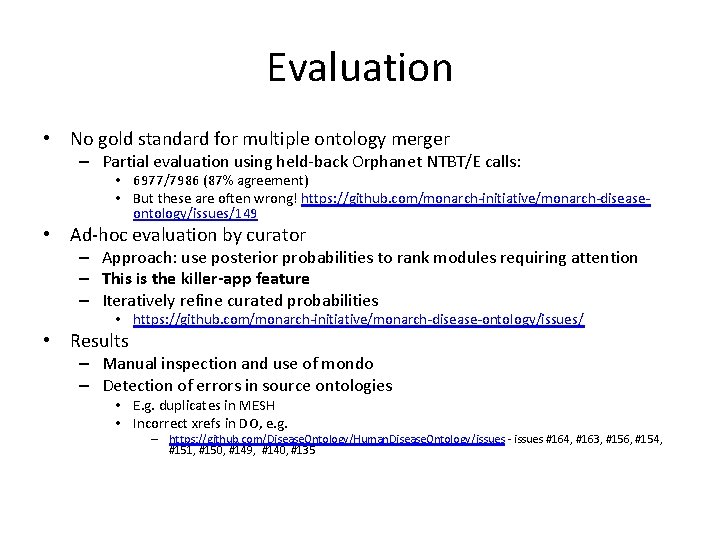
Evaluation • No gold standard for multiple ontology merger – Partial evaluation using held-back Orphanet NTBT/E calls: • 6977/7986 (87% agreement) • But these are often wrong! https: //github. com/monarch-initiative/monarch-diseaseontology/issues/149 • Ad-hoc evaluation by curator – Approach: use posterior probabilities to rank modules requiring attention – This is the killer-app feature – Iteratively refine curated probabilities • https: //github. com/monarch-initiative/monarch-disease-ontology/issues/ • Results – Manual inspection and use of mondo – Detection of errors in source ontologies • E. g. duplicates in MESH • Incorrect xrefs in DO, e. g. – https: //github. com/Disease. Ontology/Human. Disease. Ontology/issues - issues #164, #163, #156, #154, #151, #150, #149, #140, #135
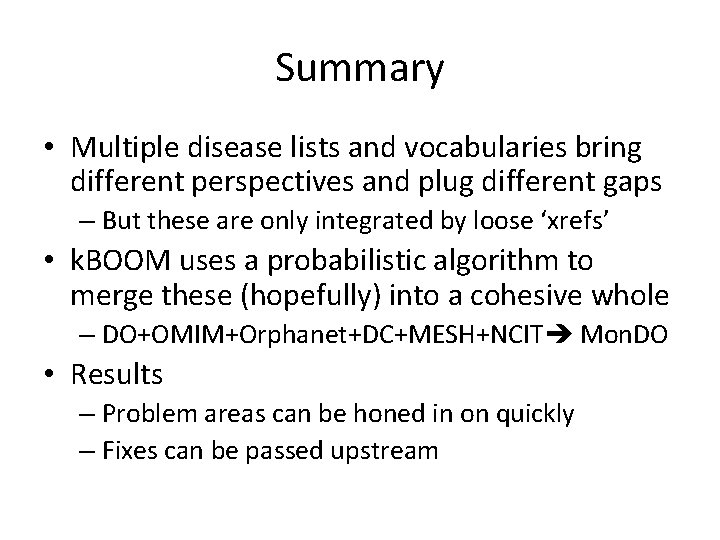
Summary • Multiple disease lists and vocabularies bring different perspectives and plug different gaps – But these are only integrated by loose ‘xrefs’ • k. BOOM uses a probabilistic algorithm to merge these (hopefully) into a cohesive whole – DO+OMIM+Orphanet+DC+MESH+NCIT Mon. DO • Results – Problem areas can be honed in on quickly – Fixes can be passed upstream
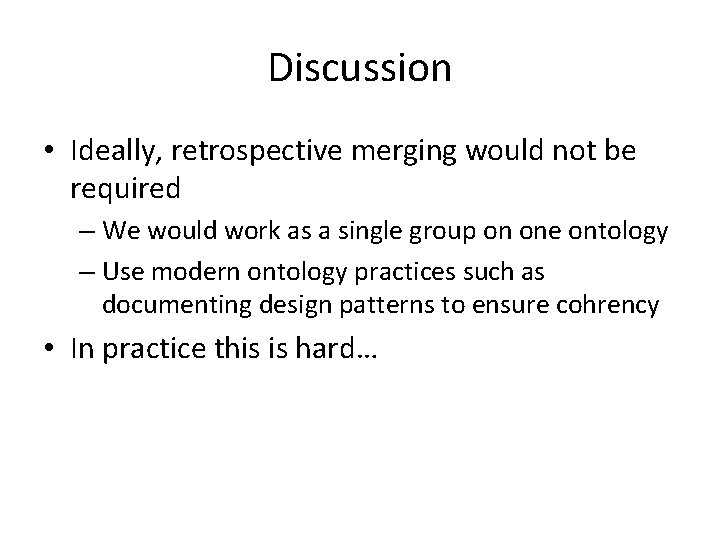
Discussion • Ideally, retrospective merging would not be required – We would work as a single group on one ontology – Use modern ontology practices such as documenting design patterns to ensure cohrency • In practice this is hard…
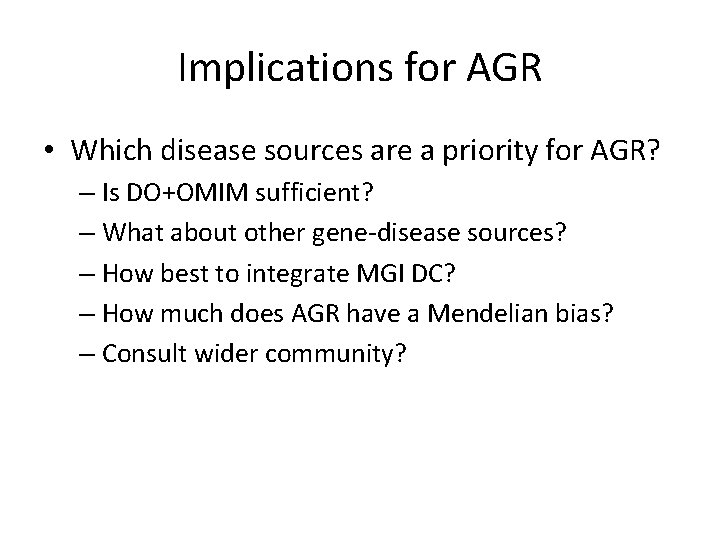
Implications for AGR • Which disease sources are a priority for AGR? – Is DO+OMIM sufficient? – What about other gene-disease sources? – How best to integrate MGI DC? – How much does AGR have a Mendelian bias? – Consult wider community?
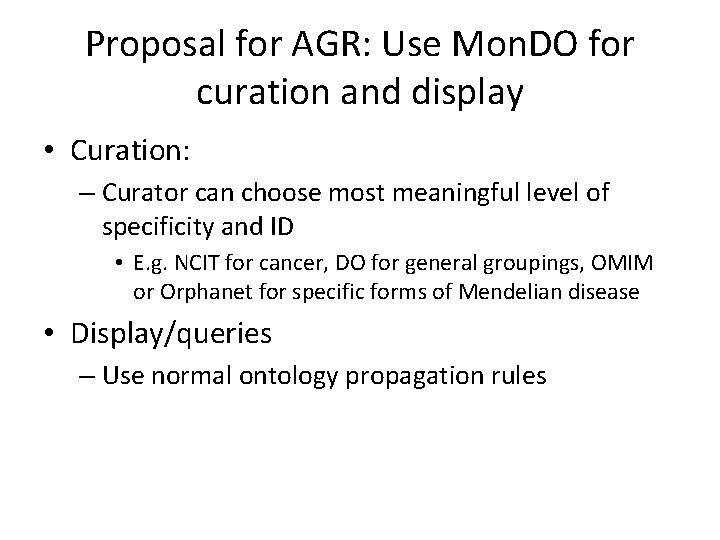
Proposal for AGR: Use Mon. DO for curation and display • Curation: – Curator can choose most meaningful level of specificity and ID • E. g. NCIT for cancer, DO for general groupings, OMIM or Orphanet for specific forms of Mendelian disease • Display/queries – Use normal ontology propagation rules
Suggested upper merged ontology
Clin gen
Clin gen
Research card
Clin chest med
Clin var
Northern renaissance thinkers merged humanist ideas with
Tertiary line of the ikebana representing earth
Ffa history timeline
Communicable disease and non communicable disease
Gene ontology project
How to identify epistemology in research
Resources events agents
Basic formal ontology
Ontology alignment
Ontology alignment
Gene ontology project
Ontology meaning
Ontology editors
Pizza ontology download
Financial industry business ontology
Ontology
Provo ontology
Protege owl tutorial
Ontology kurssi
Types of ontology
Business ontology
Ontology creation
Dolce ontology
Barry smith buffalo
Ontology 101
Football ontology
Ontology epistemology axiology
Dorsal recumbent position
Fibo ontology
What is meant by gene ontology?
Ontology rdf
Metu class
What is an ontologist
Vivo ontology
Schema .org
Ontology vs epistemology
Rdf schema example
Business model ontology