A Forecast Evaluation Tool FET for CPC Operational
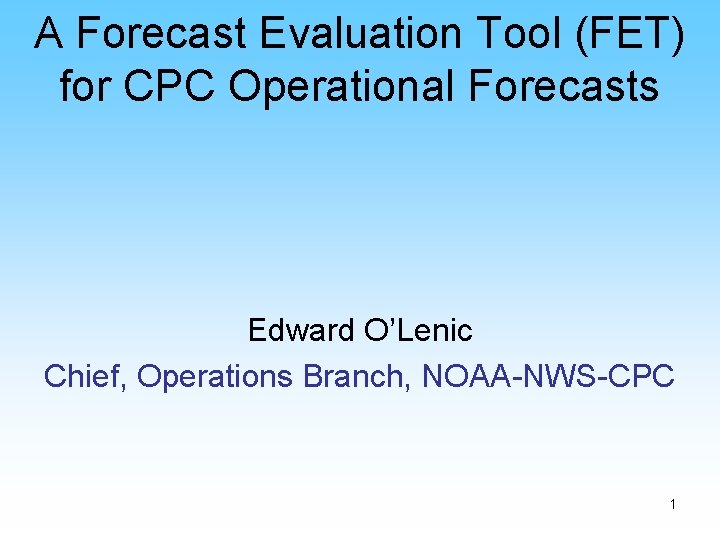
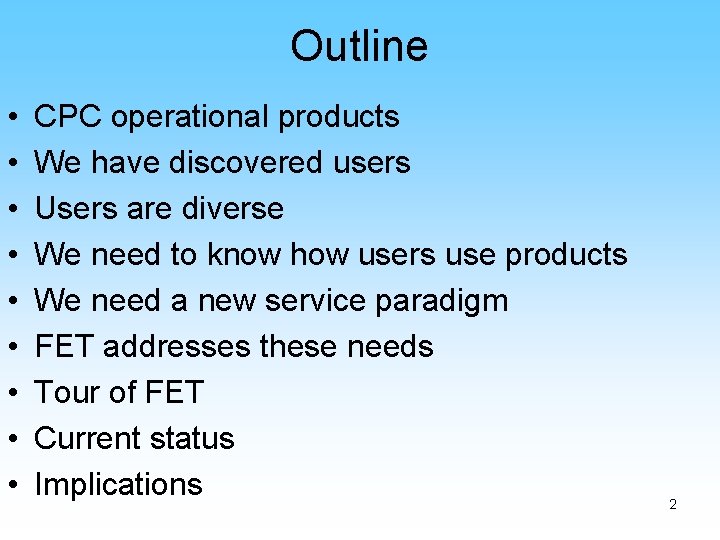
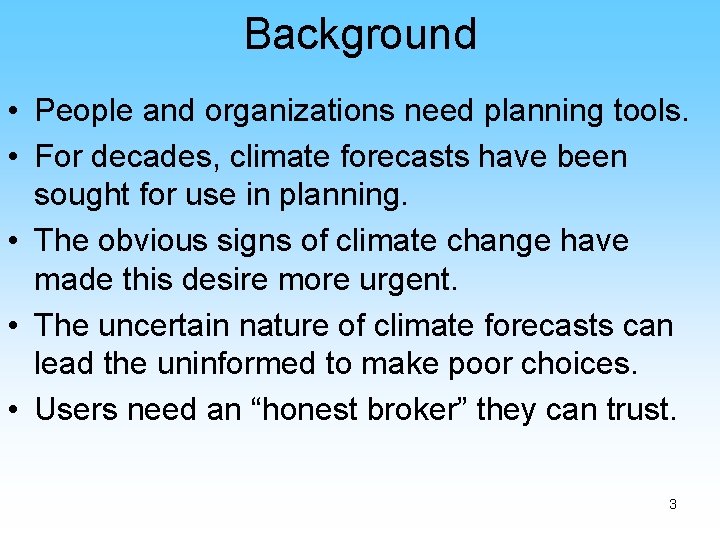
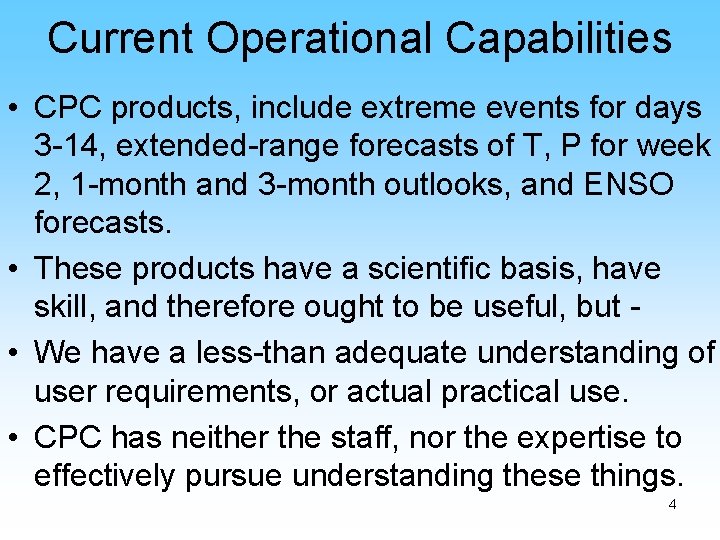
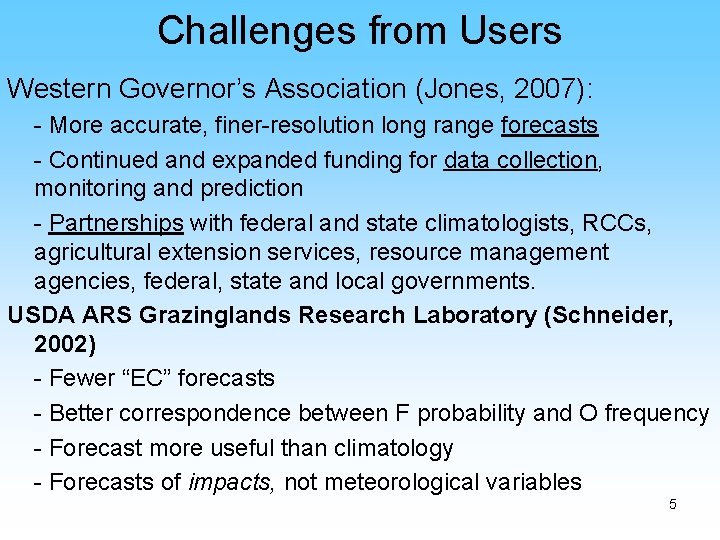
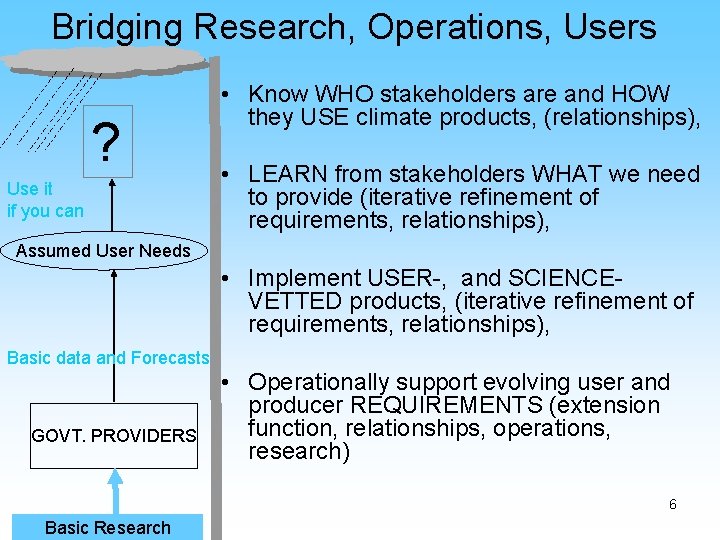
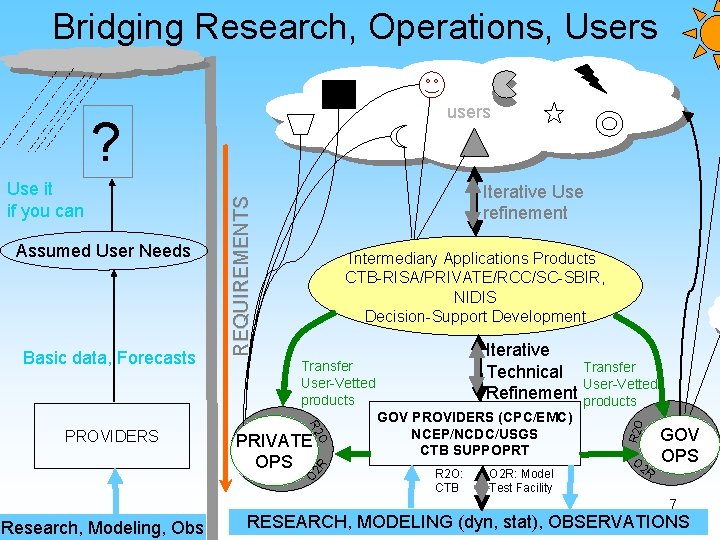
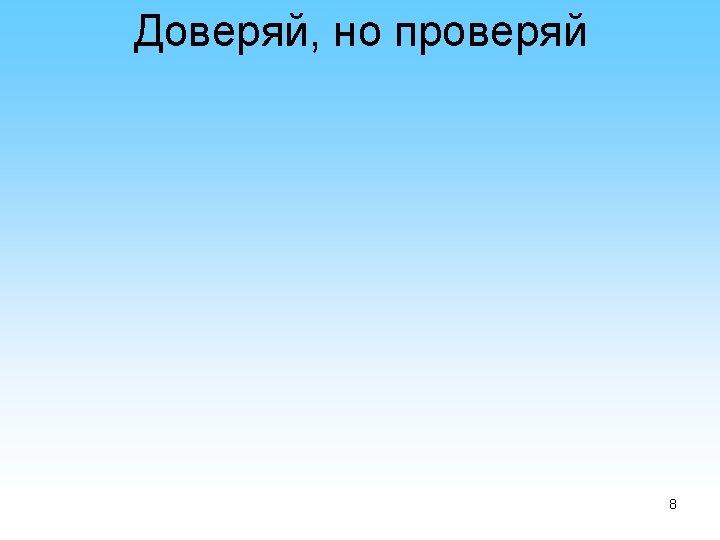
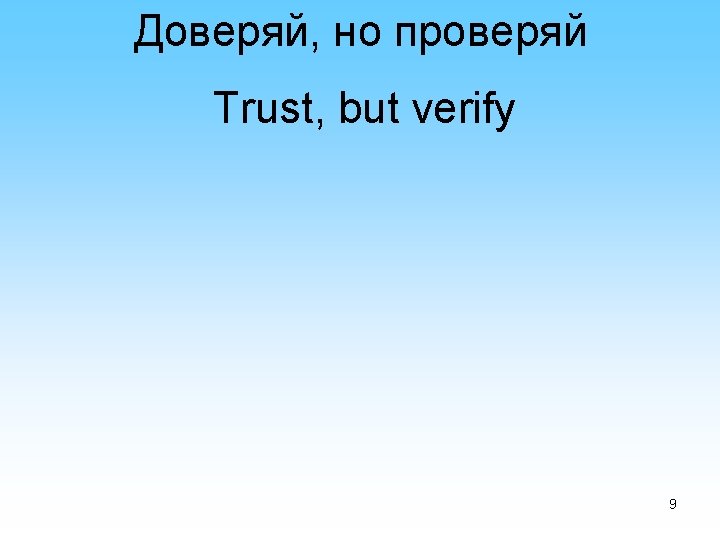
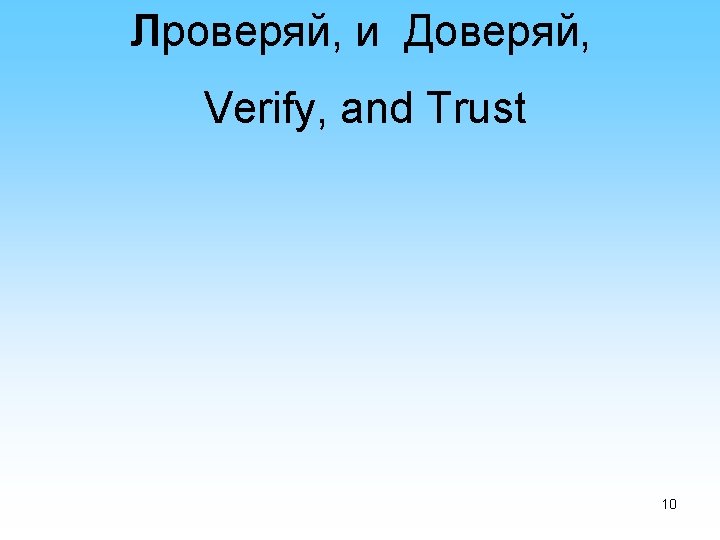
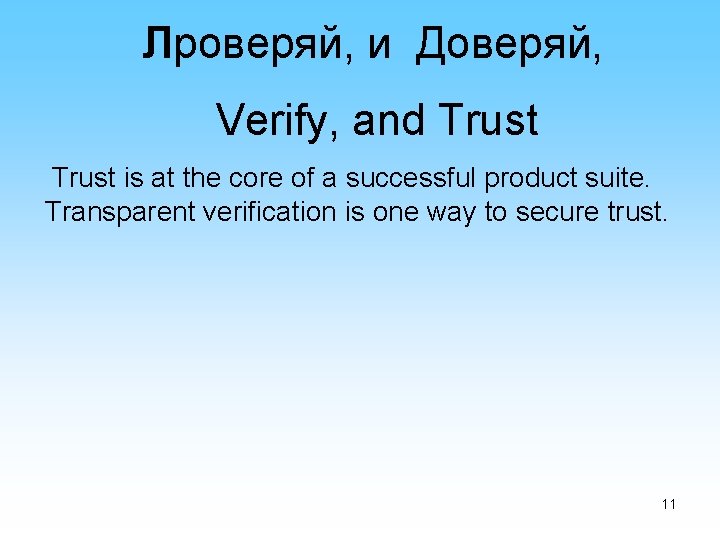
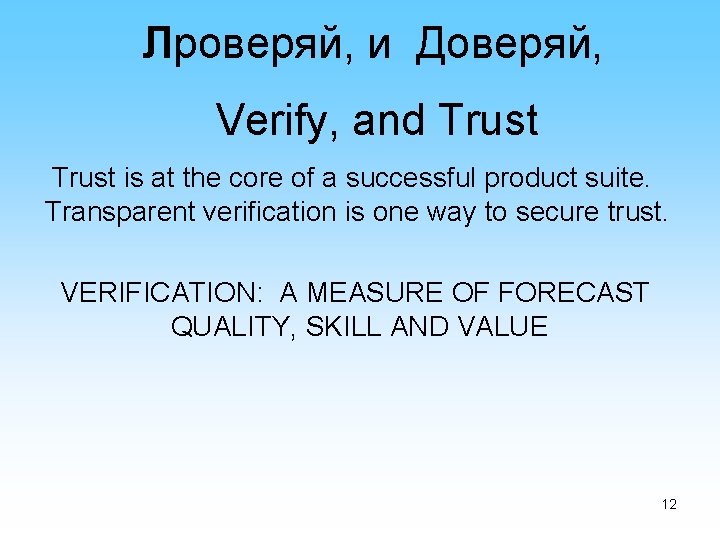
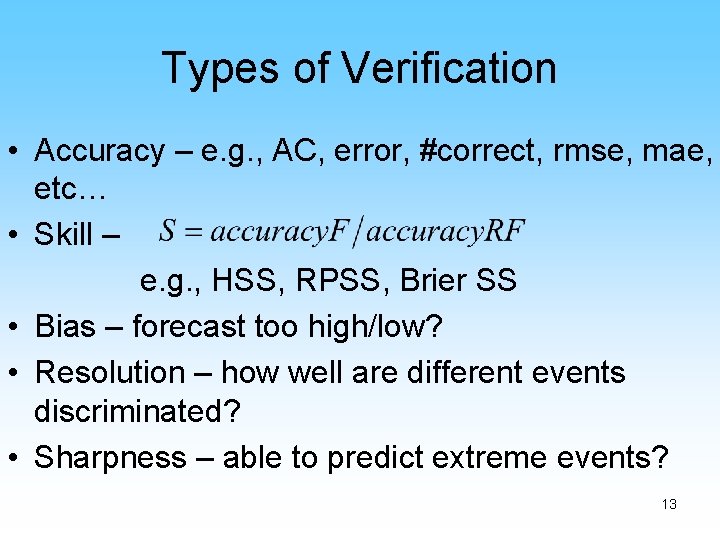
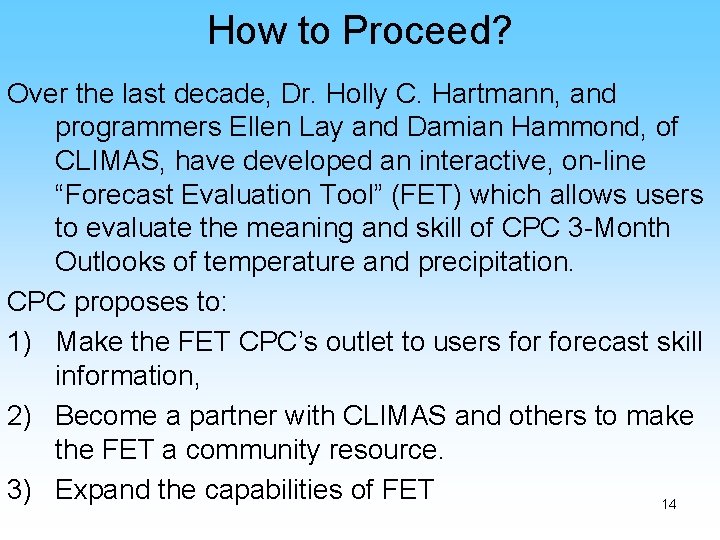
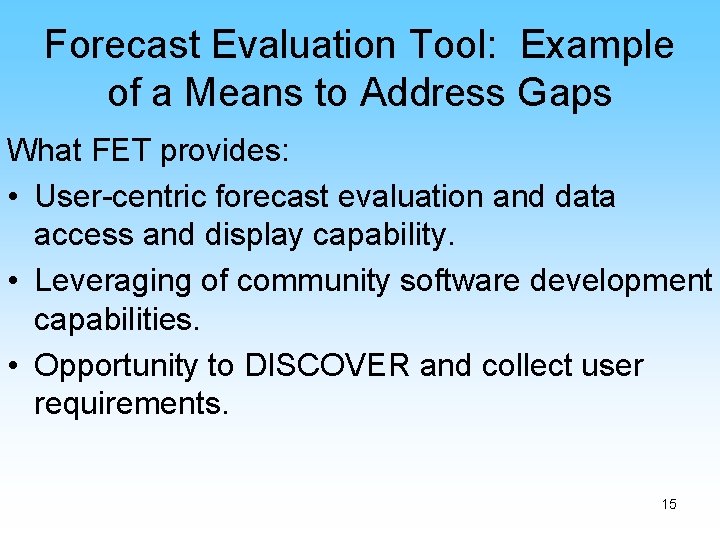
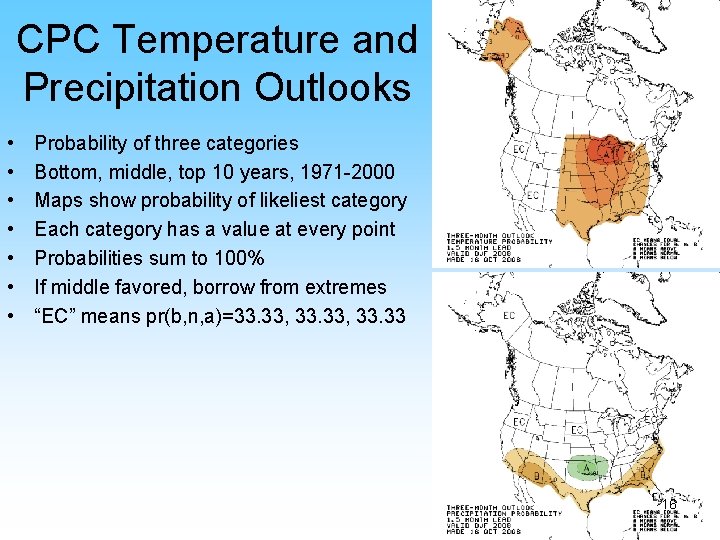
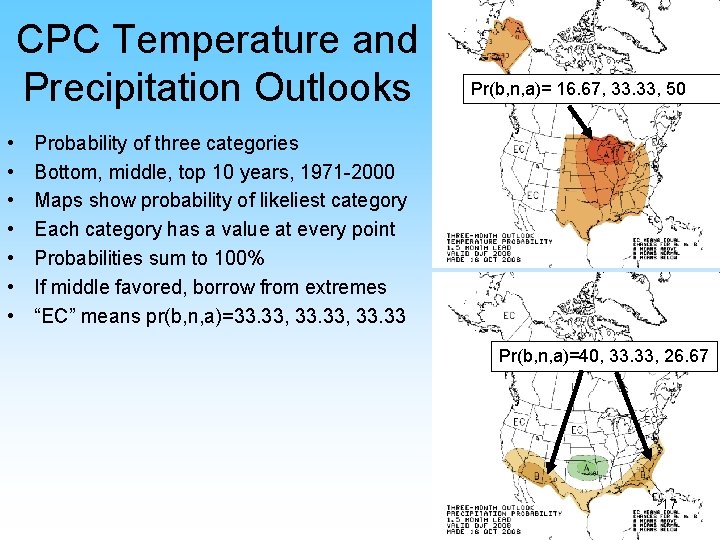
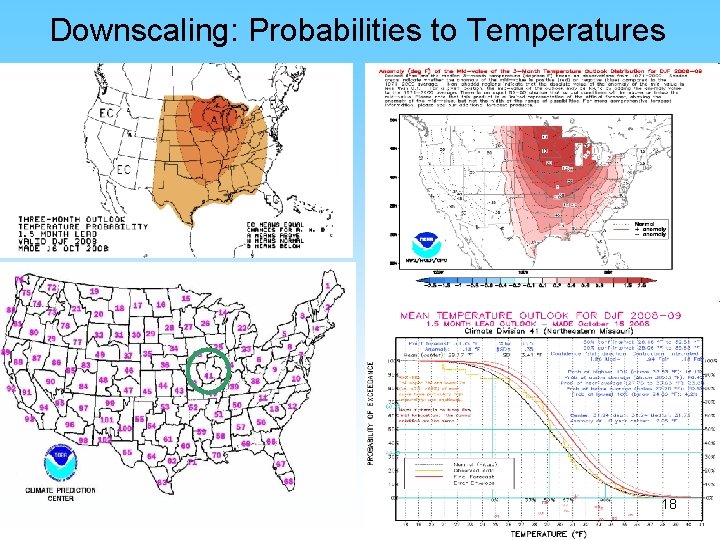
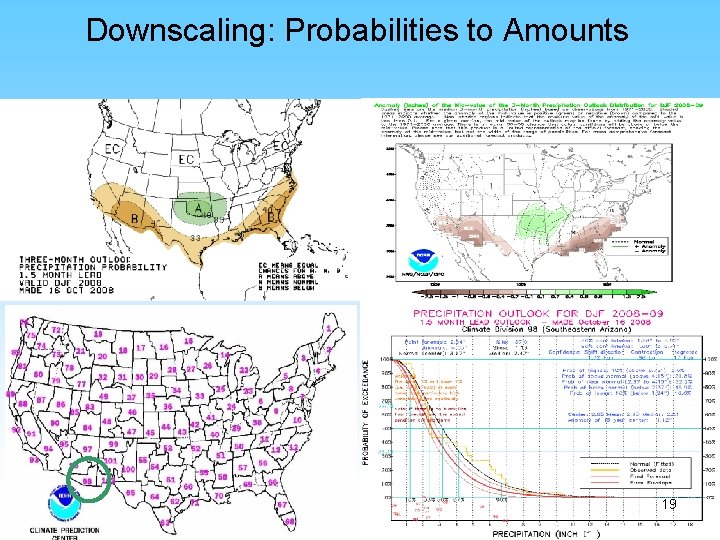
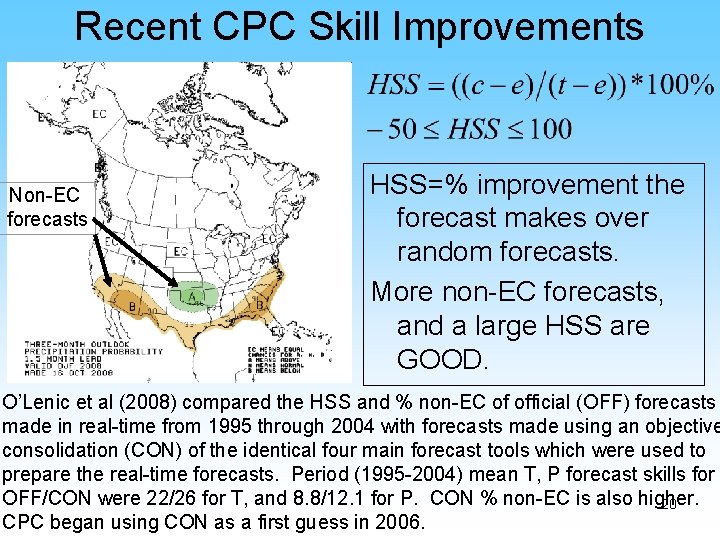
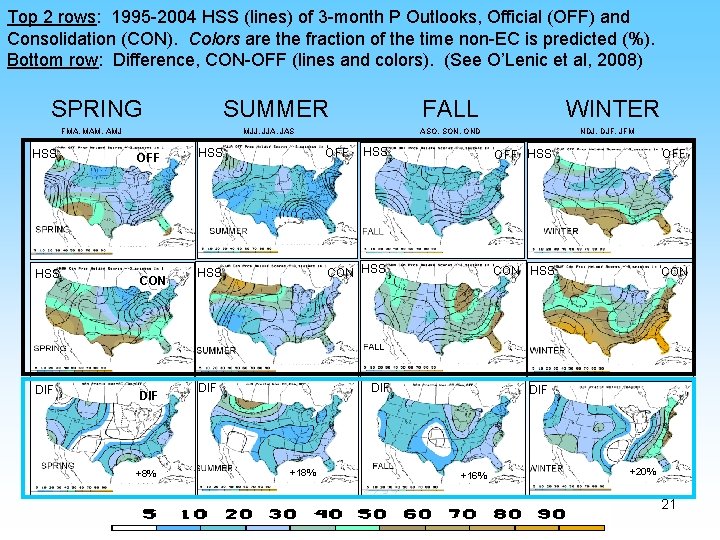
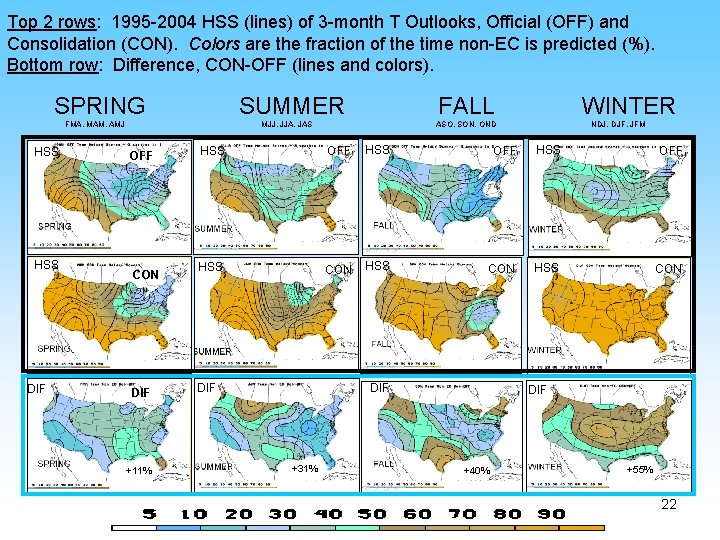
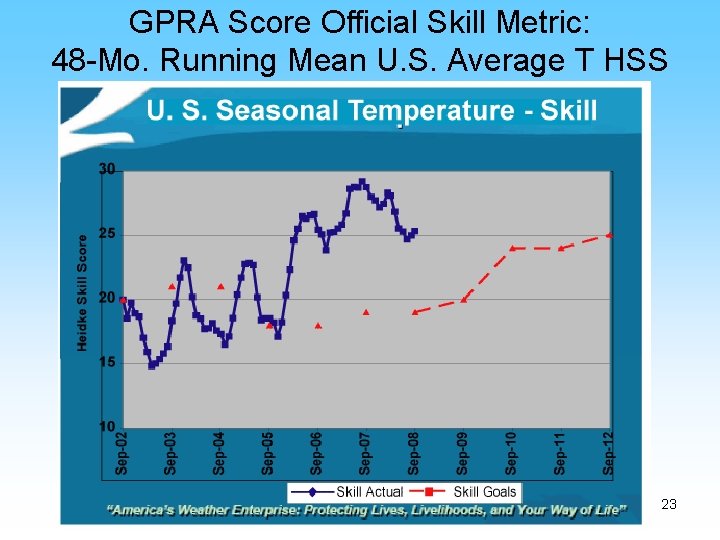
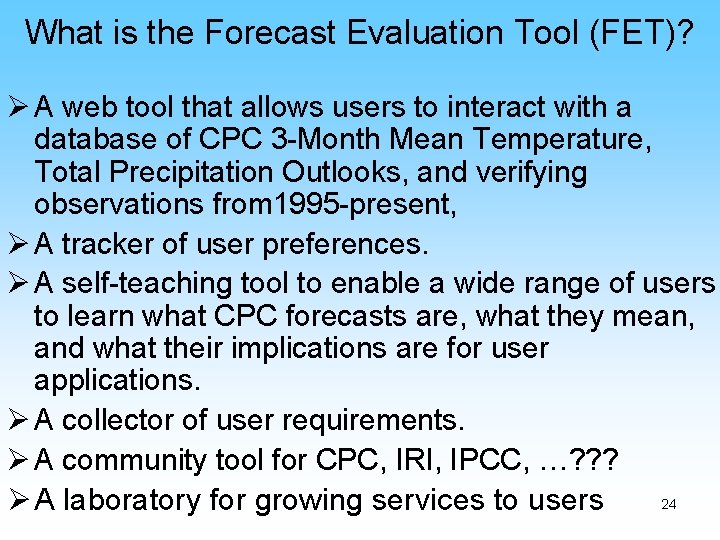
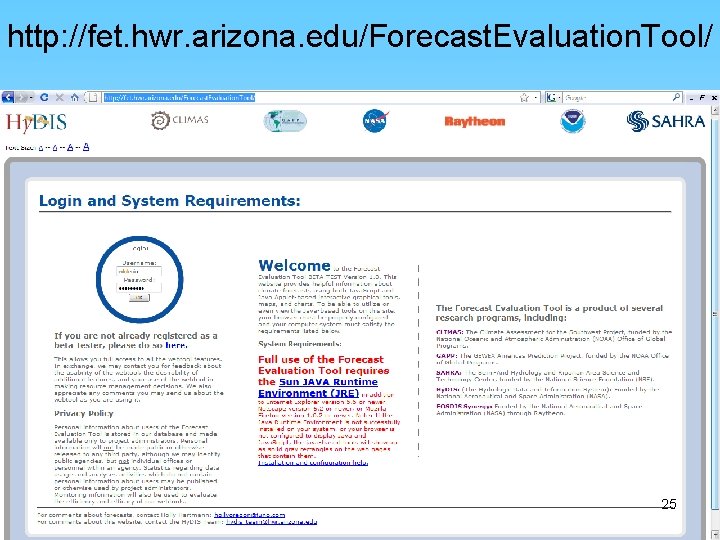
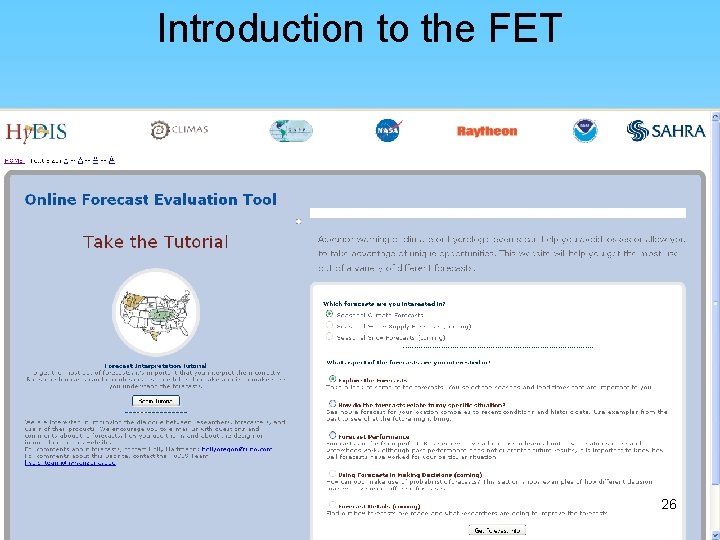
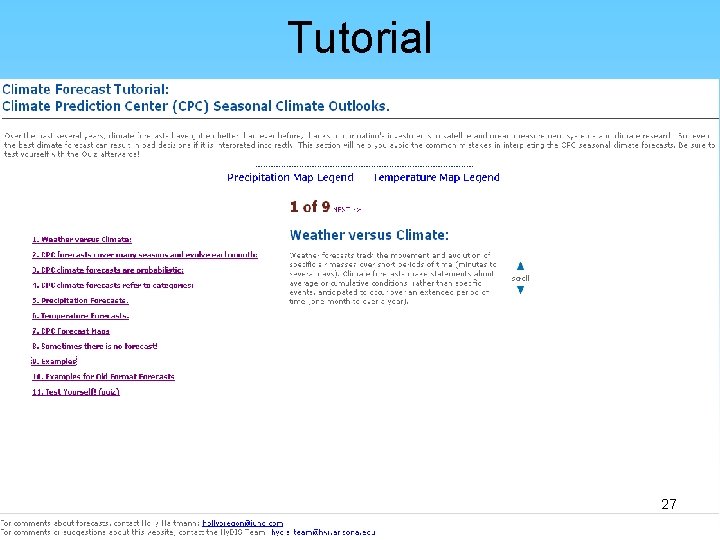
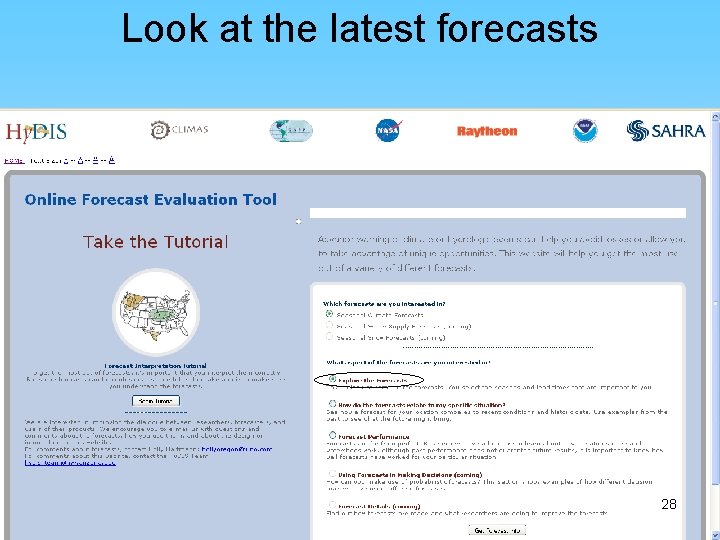
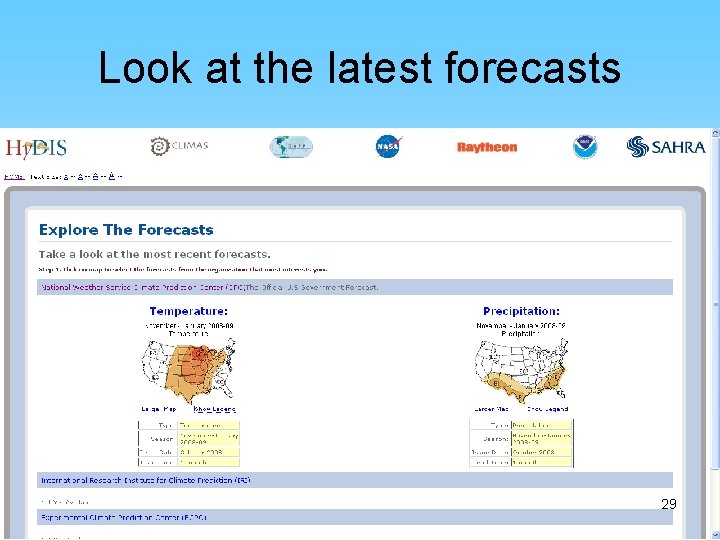
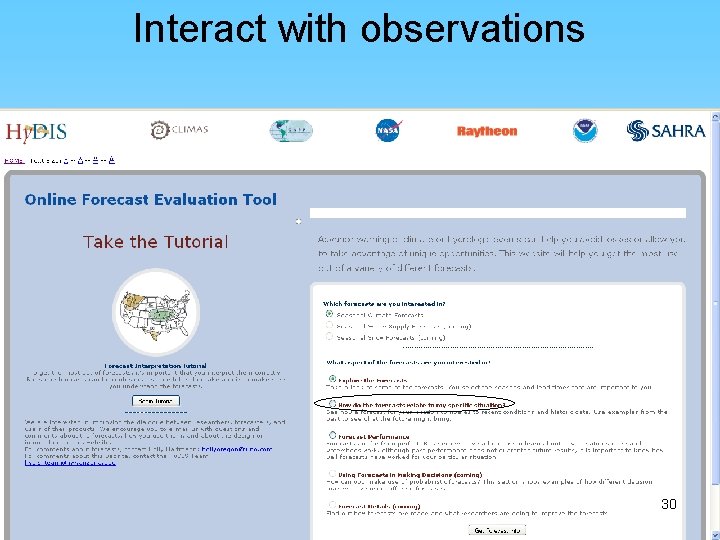
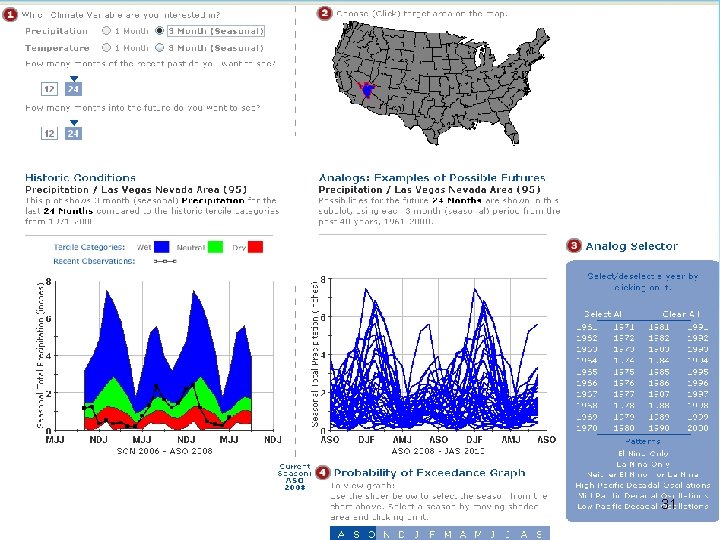
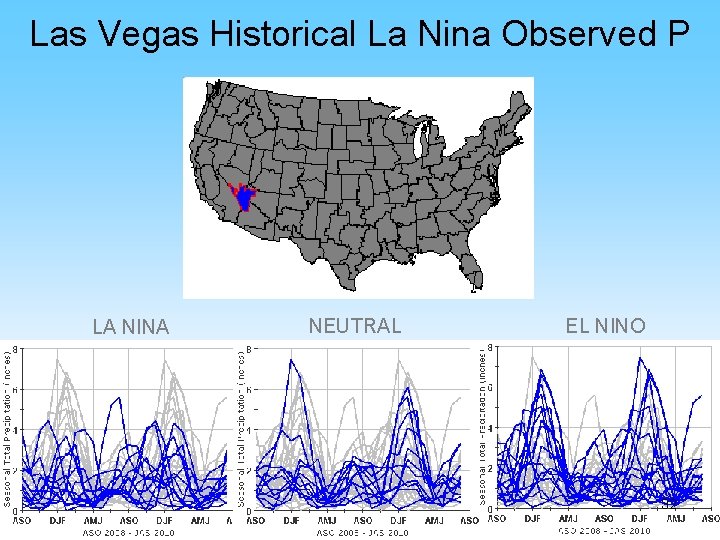
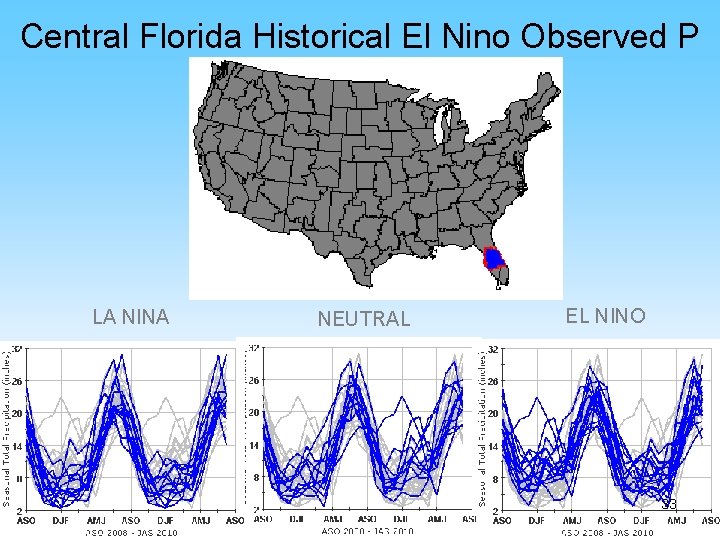
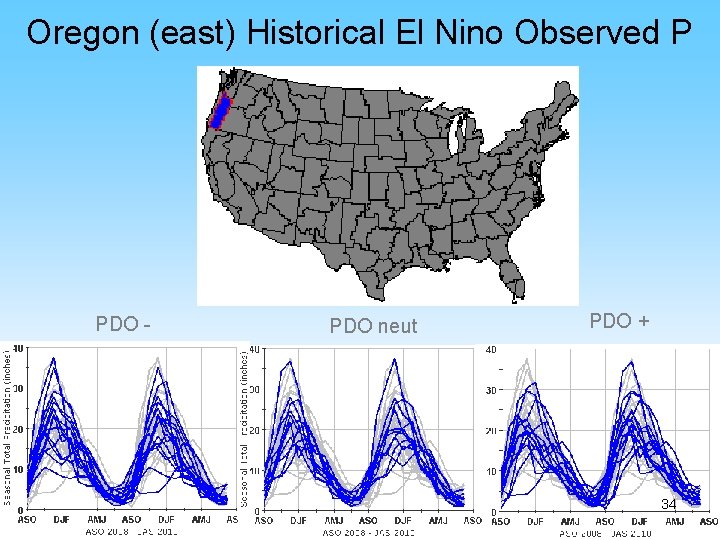
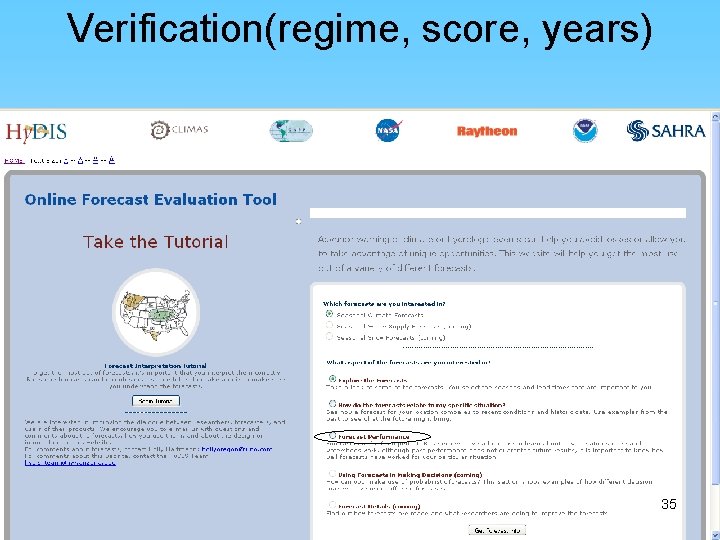
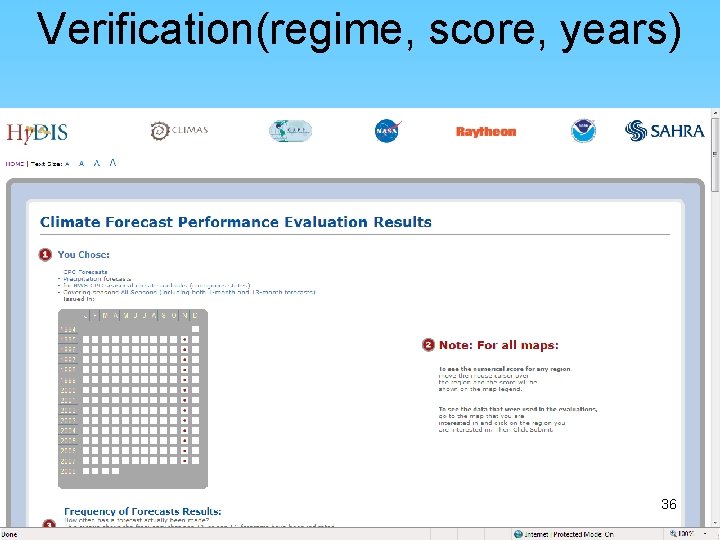
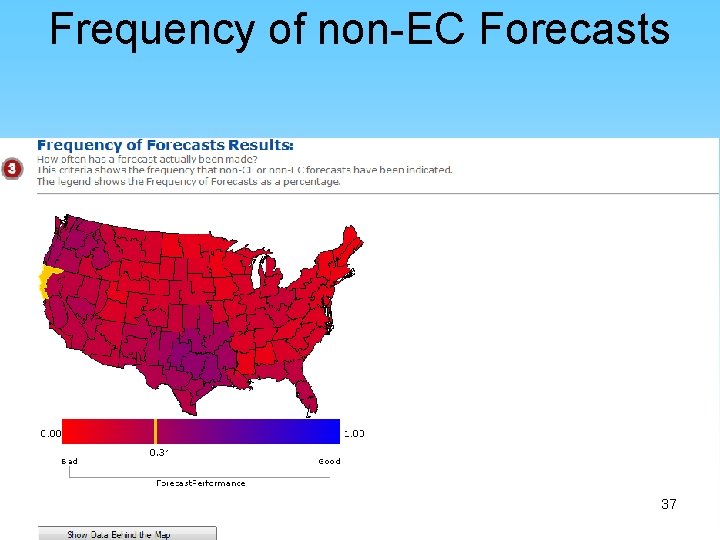
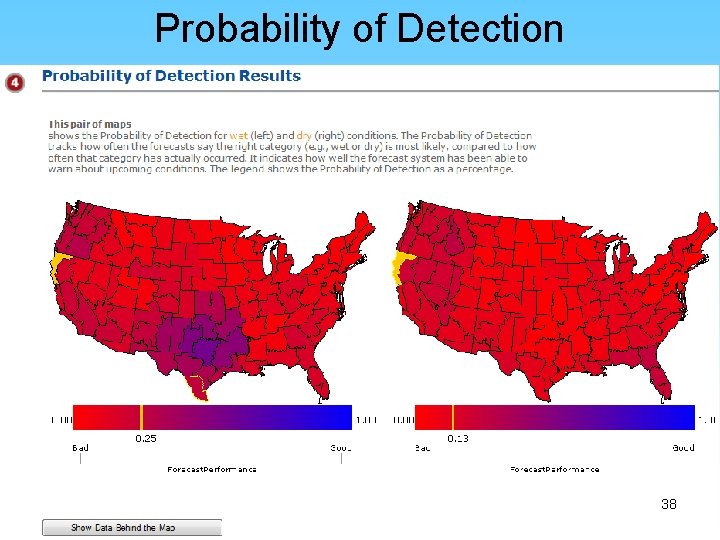
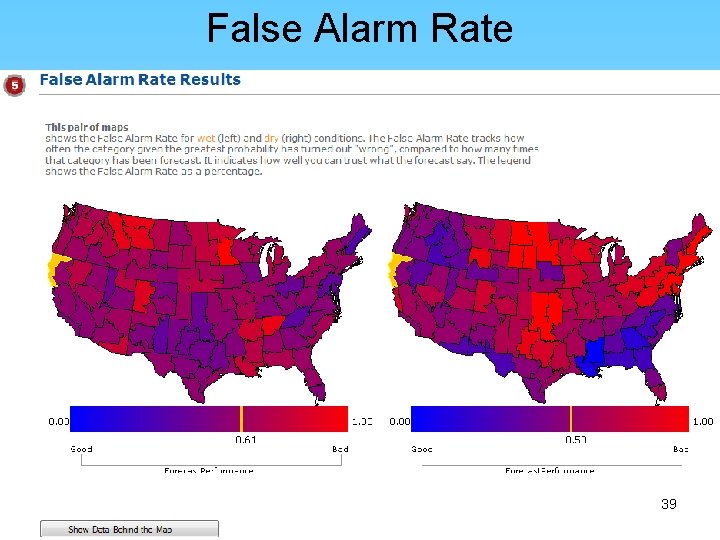
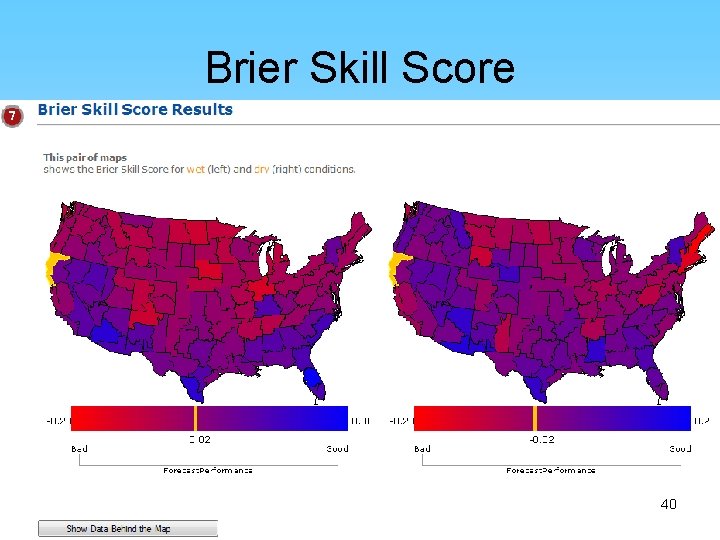
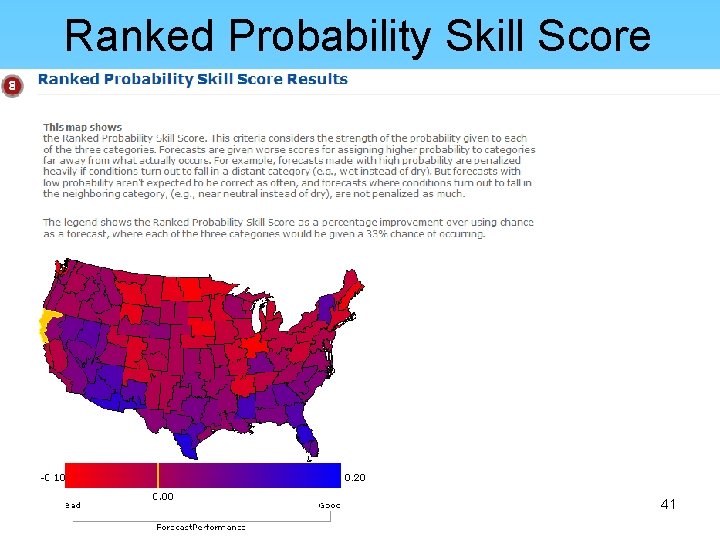
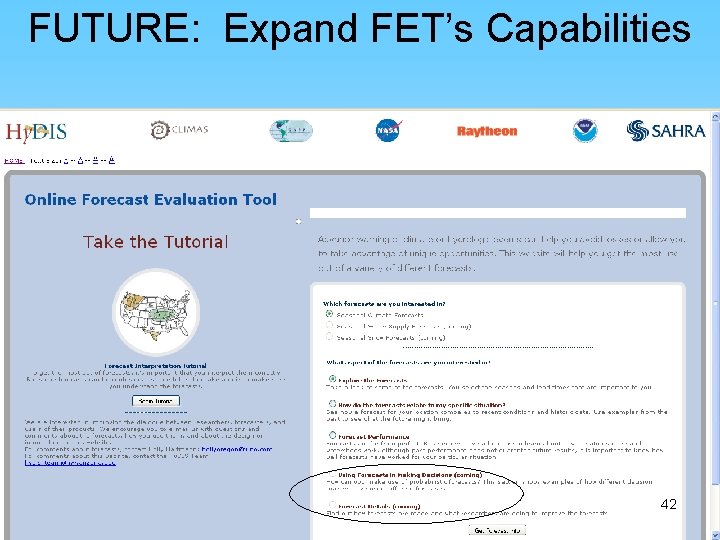
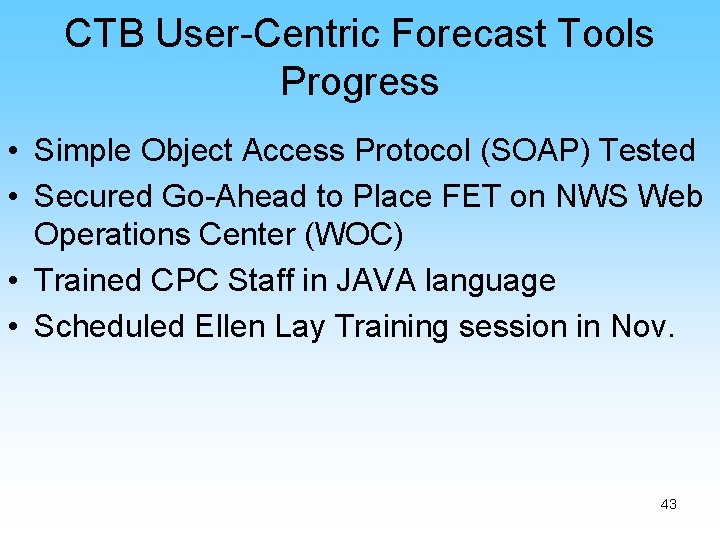
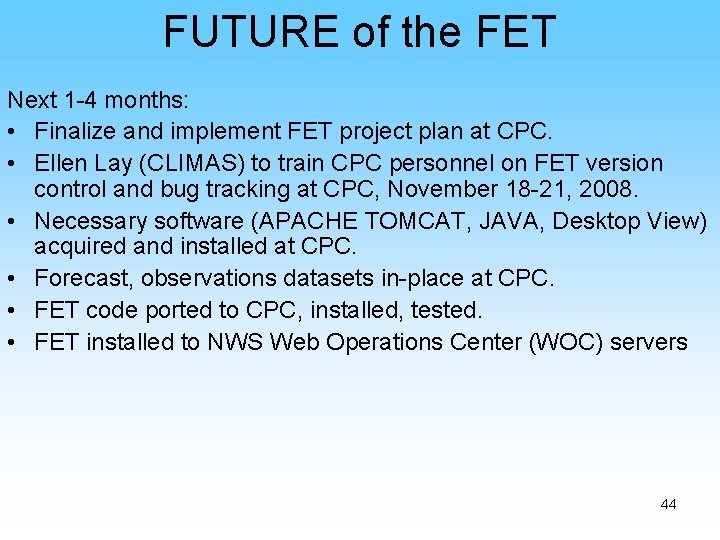
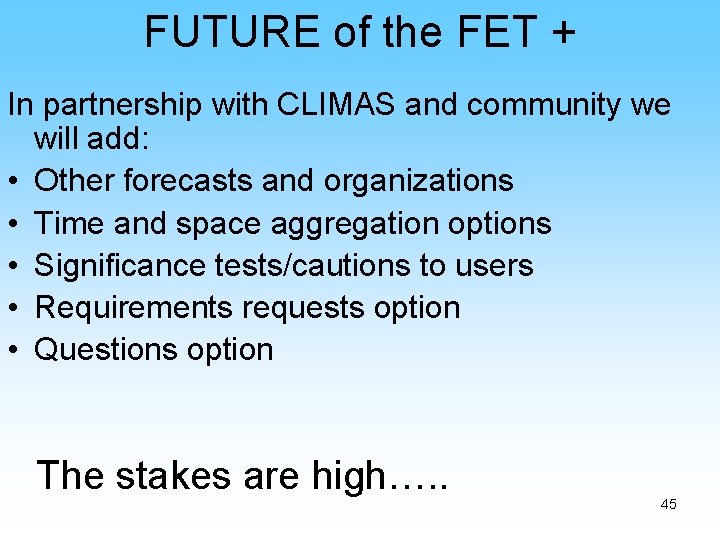
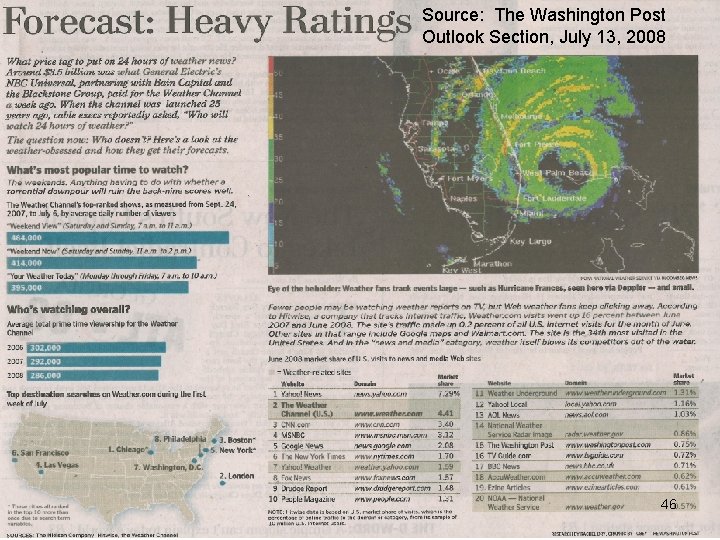
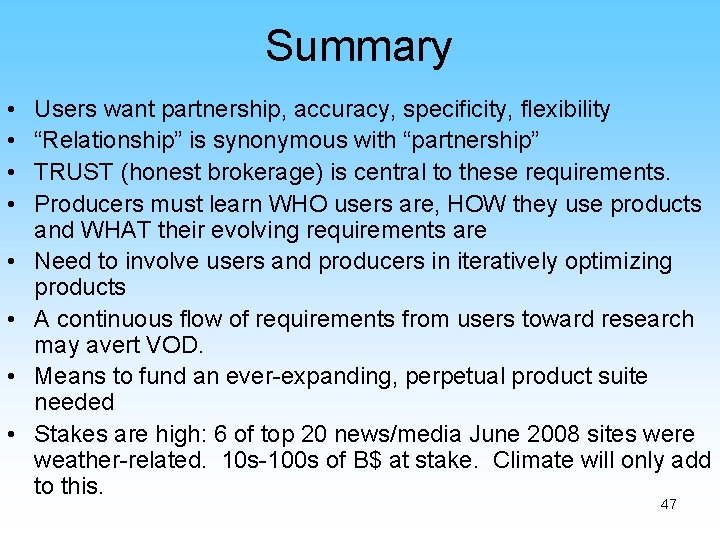
- Slides: 47
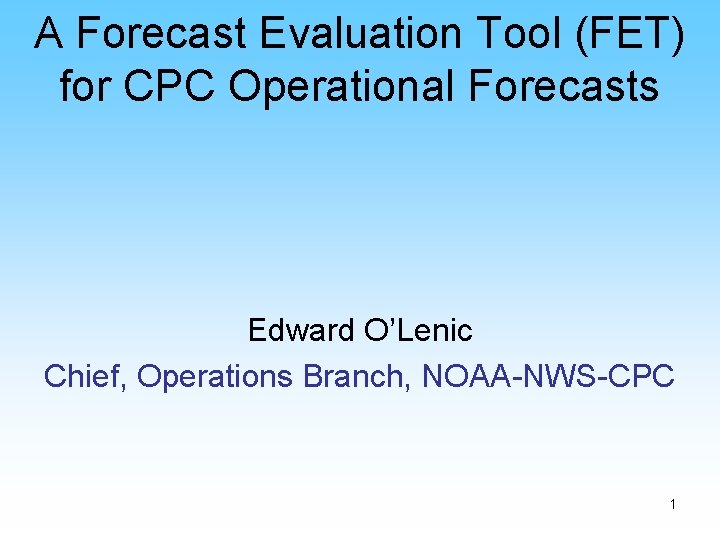
A Forecast Evaluation Tool (FET) for CPC Operational Forecasts Edward O’Lenic Chief, Operations Branch, NOAA-NWS-CPC 1
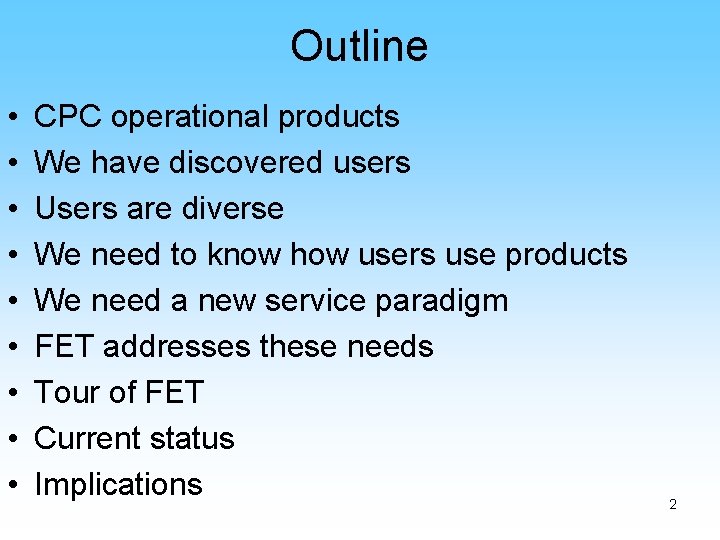
Outline • • • CPC operational products We have discovered users Users are diverse We need to know how users use products We need a new service paradigm FET addresses these needs Tour of FET Current status Implications 2
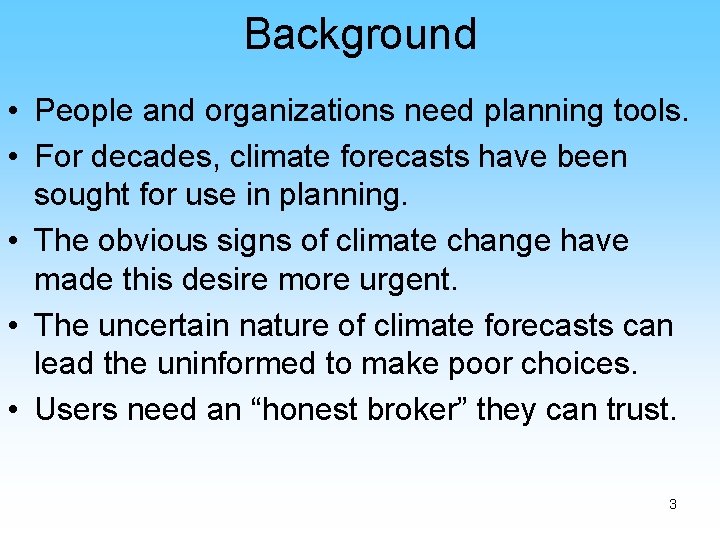
Background • People and organizations need planning tools. • For decades, climate forecasts have been sought for use in planning. • The obvious signs of climate change have made this desire more urgent. • The uncertain nature of climate forecasts can lead the uninformed to make poor choices. • Users need an “honest broker” they can trust. 3
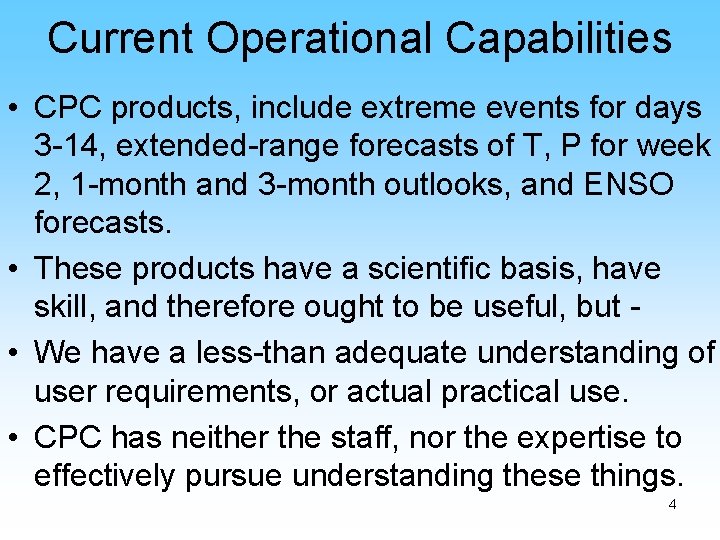
Current Operational Capabilities • CPC products, include extreme events for days 3 -14, extended-range forecasts of T, P for week 2, 1 -month and 3 -month outlooks, and ENSO forecasts. • These products have a scientific basis, have skill, and therefore ought to be useful, but • We have a less-than adequate understanding of user requirements, or actual practical use. • CPC has neither the staff, nor the expertise to effectively pursue understanding these things. 4
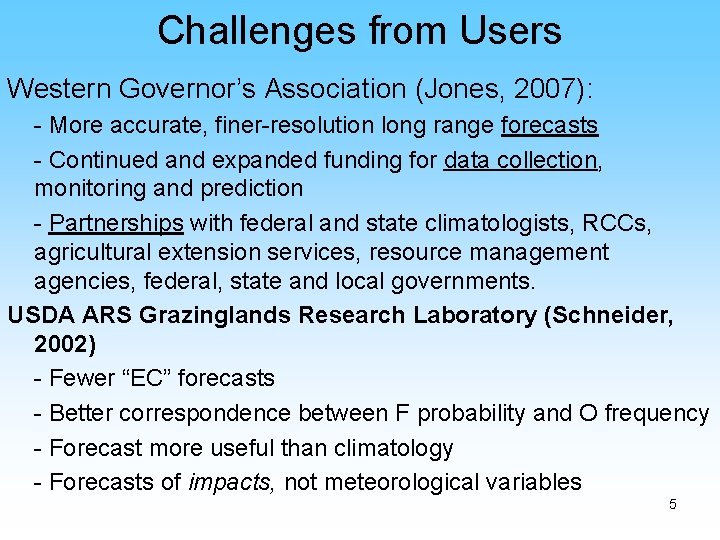
Challenges from Users Western Governor’s Association (Jones, 2007): - More accurate, finer-resolution long range forecasts - Continued and expanded funding for data collection, monitoring and prediction - Partnerships with federal and state climatologists, RCCs, agricultural extension services, resource management agencies, federal, state and local governments. USDA ARS Grazinglands Research Laboratory (Schneider, 2002) - Fewer “EC” forecasts - Better correspondence between F probability and O frequency - Forecast more useful than climatology - Forecasts of impacts, not meteorological variables 5
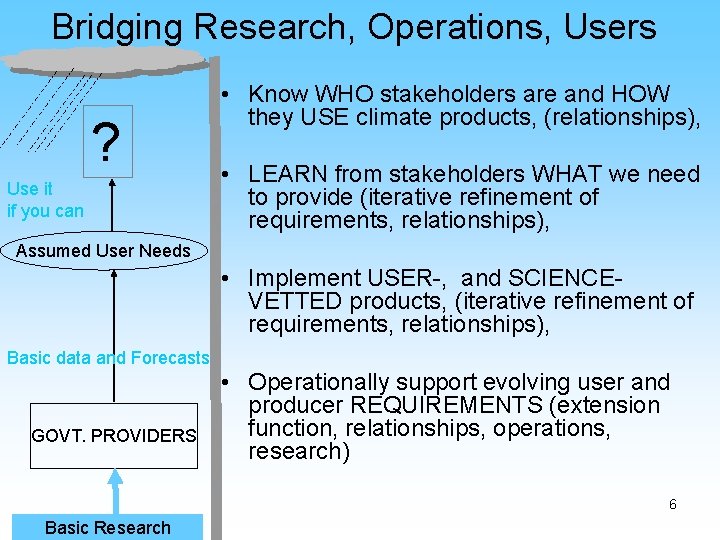
Bridging Research, Operations, Users ? Use it if you can • Know WHO stakeholders are and HOW they USE climate products, (relationships), • LEARN from stakeholders WHAT we need to provide (iterative refinement of requirements, relationships), Assumed User Needs • Implement USER-, and SCIENCEVETTED products, (iterative refinement of requirements, relationships), Basic data and Forecasts GOVT. PROVIDERS • Operationally support evolving user and producer REQUIREMENTS (extension function, relationships, operations, research) 6 Basic Research
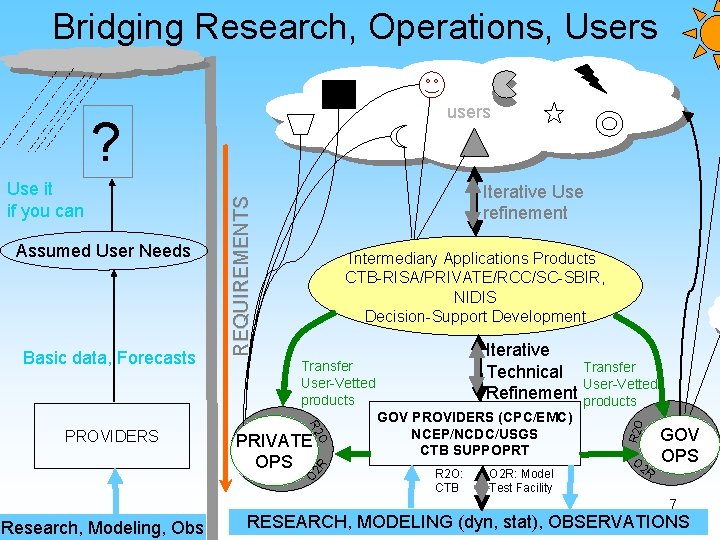
Bridging Research, Operations, Users users ? Basic data, Forecasts Iterative Technical Refinement Transfer User-Vetted products O R 2 PRIVATE OPS O 2 R PROVIDERS Intermediary Applications Products CTB-RISA/PRIVATE/RCC/SC-SBIR, NIDIS Decision-Support Development GOV PROVIDERS (CPC/EMC) NCEP/NCDC/USGS CTB SUPPOPRT R 2 O: CTB O 2 R: Model Test Facility Transfer User-Vetted products R 2 O Assumed User Needs Iterative Use refinement REQUIREMENTS Use it if you can O 2 R GOV OPS 7 Research, Modeling, Obs RESEARCH, MODELING (dyn, stat), OBSERVATIONS
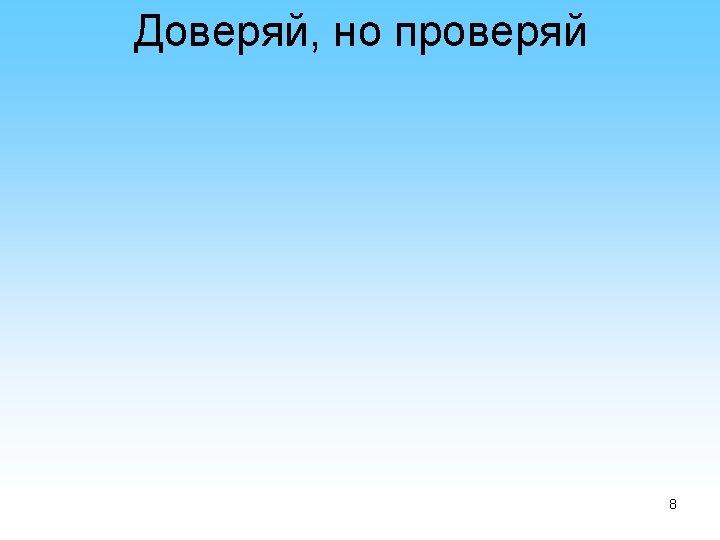
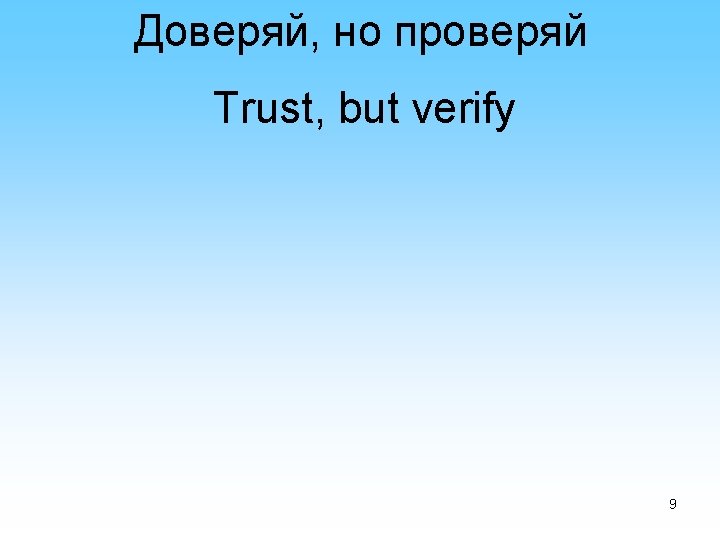
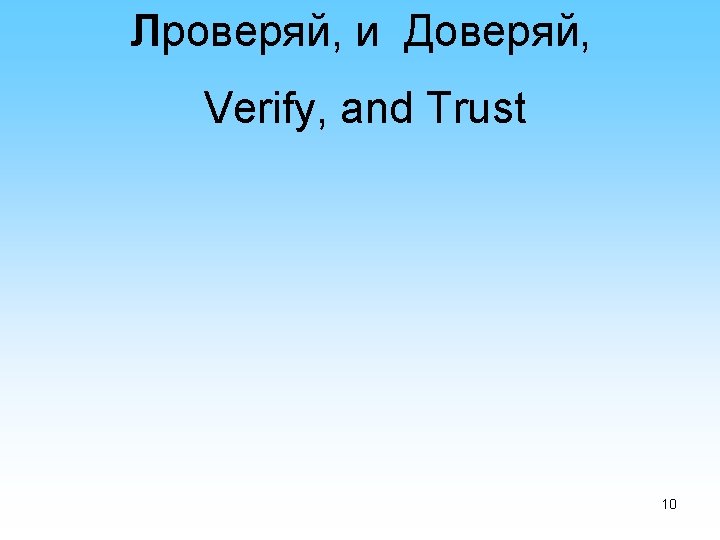
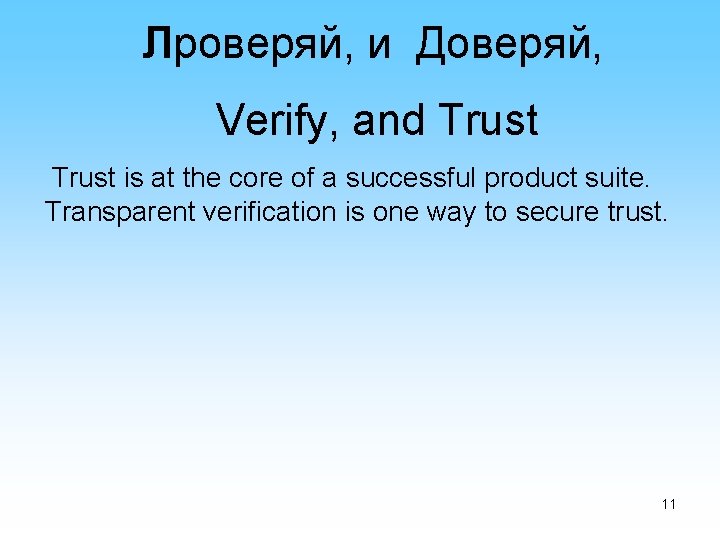
Лроверяй, и Доверяй, Verify, and Trust is at the core of a successful product suite. Transparent verification is one way to secure trust. 11
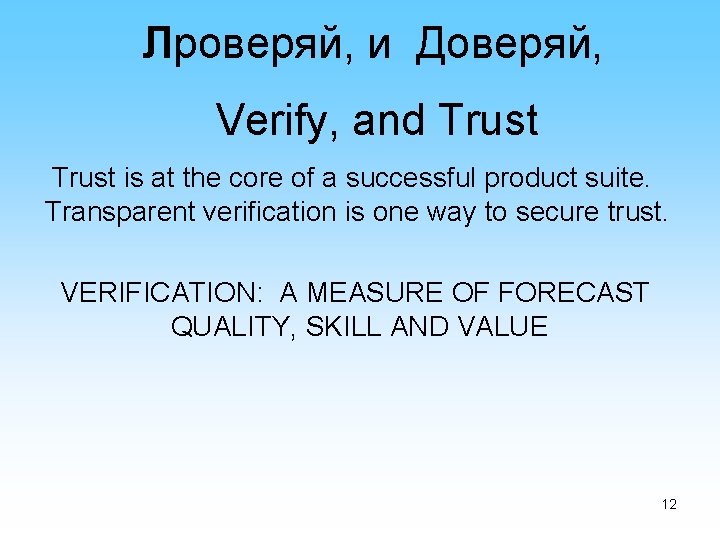
Лроверяй, и Доверяй, Verify, and Trust is at the core of a successful product suite. Transparent verification is one way to secure trust. VERIFICATION: A MEASURE OF FORECAST QUALITY, SKILL AND VALUE 12
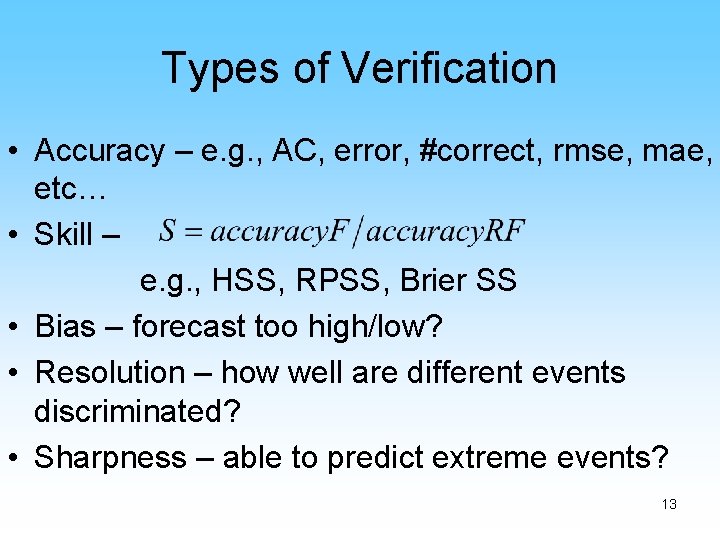
Types of Verification • Accuracy – e. g. , AC, error, #correct, rmse, mae, etc… • Skill – e. g. , HSS, RPSS, Brier SS • Bias – forecast too high/low? • Resolution – how well are different events discriminated? • Sharpness – able to predict extreme events? 13
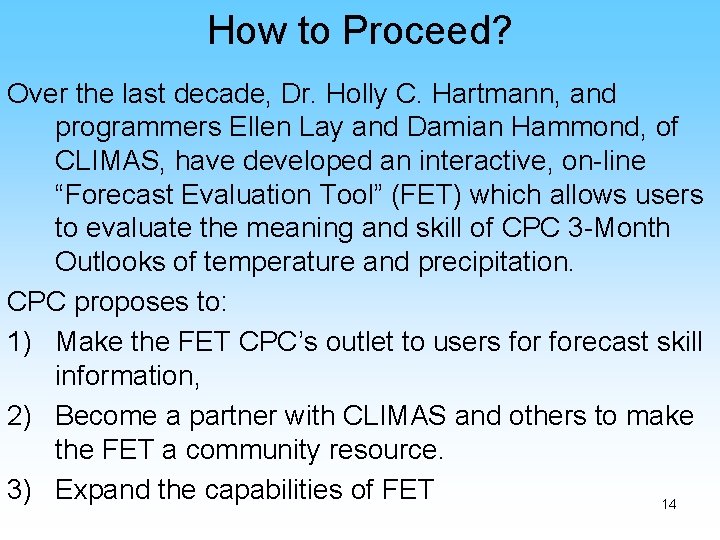
How to Proceed? Over the last decade, Dr. Holly C. Hartmann, and programmers Ellen Lay and Damian Hammond, of CLIMAS, have developed an interactive, on-line “Forecast Evaluation Tool” (FET) which allows users to evaluate the meaning and skill of CPC 3 -Month Outlooks of temperature and precipitation. CPC proposes to: 1) Make the FET CPC’s outlet to users forecast skill information, 2) Become a partner with CLIMAS and others to make the FET a community resource. 3) Expand the capabilities of FET 14
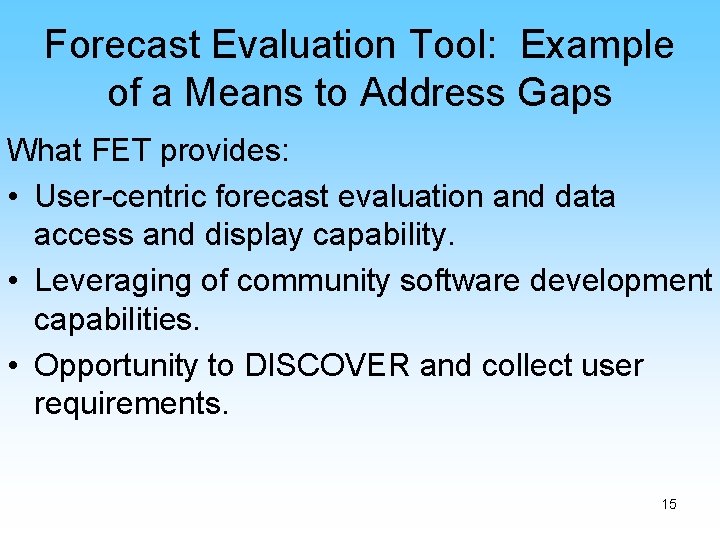
Forecast Evaluation Tool: Example of a Means to Address Gaps What FET provides: • User-centric forecast evaluation and data access and display capability. • Leveraging of community software development capabilities. • Opportunity to DISCOVER and collect user requirements. 15
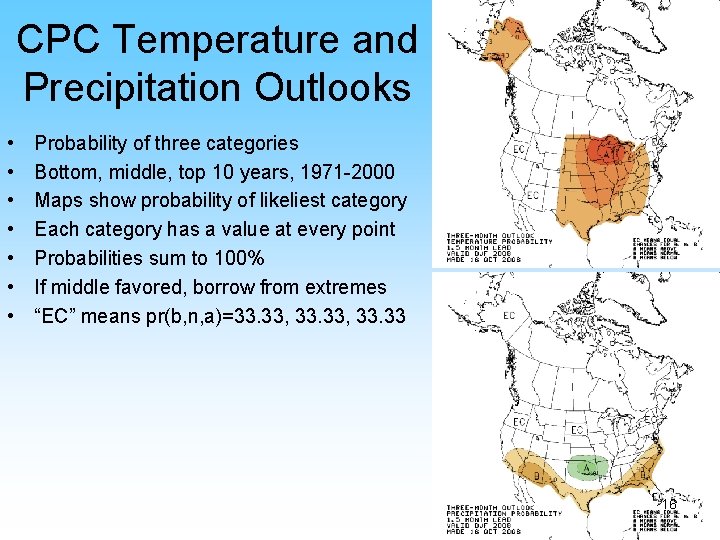
CPC Temperature and Precipitation Outlooks • • Probability of three categories Bottom, middle, top 10 years, 1971 -2000 Maps show probability of likeliest category Each category has a value at every point Probabilities sum to 100% If middle favored, borrow from extremes “EC” means pr(b, n, a)=33. 33, 33. 33 16
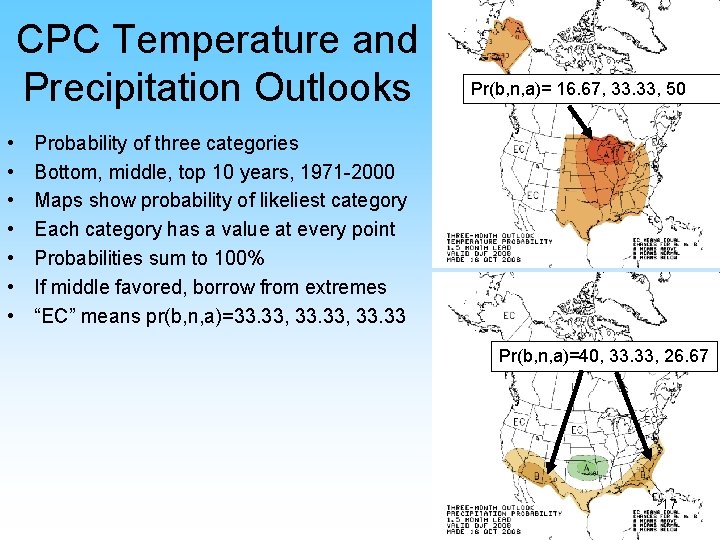
CPC Temperature and Precipitation Outlooks • • Pr(b, n, a)= 16. 67, 33. 33, 50 Probability of three categories Bottom, middle, top 10 years, 1971 -2000 Maps show probability of likeliest category Each category has a value at every point Probabilities sum to 100% If middle favored, borrow from extremes “EC” means pr(b, n, a)=33. 33, 33. 33 Pr(b, n, a)=40, 33. 33, 26. 67 17
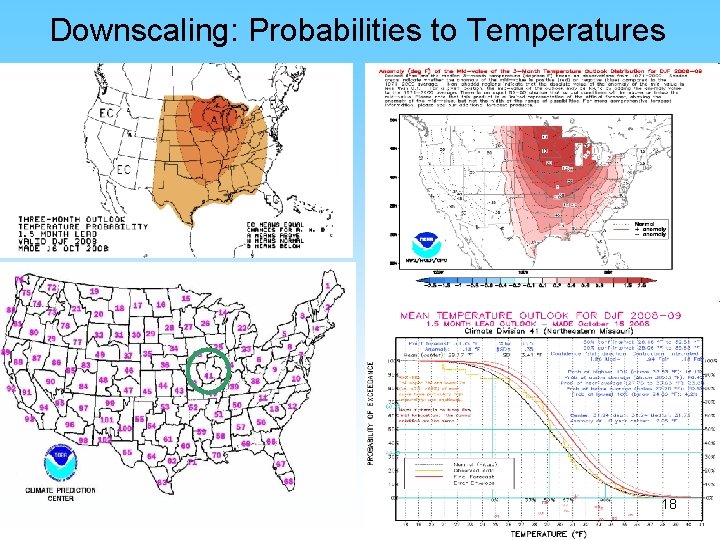
Downscaling: Probabilities to Temperatures 18
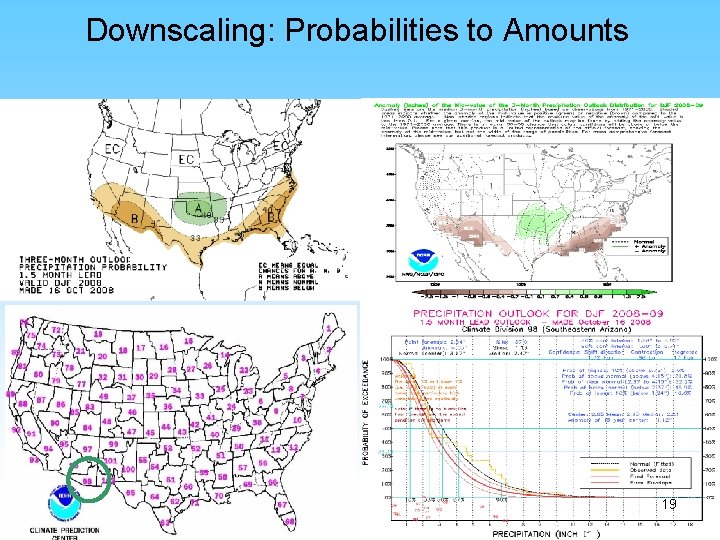
Downscaling: Probabilities to Amounts 19
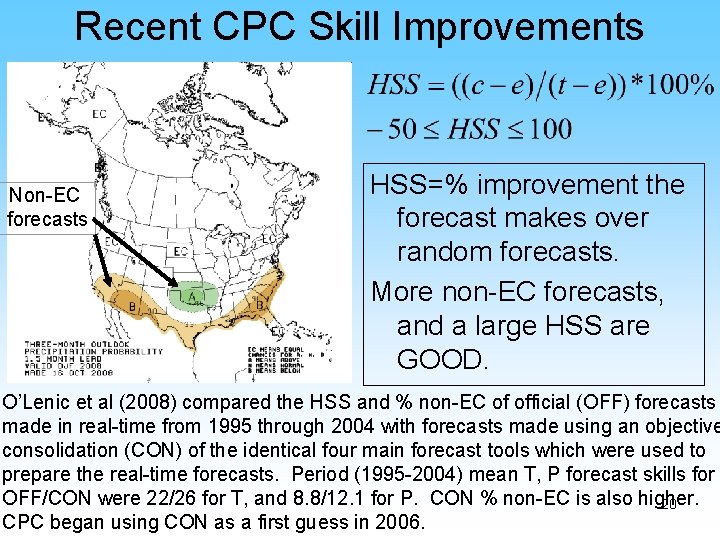
Recent CPC Skill Improvements Non-EC forecasts HSS=% improvement the forecast makes over random forecasts. More non-EC forecasts, and a large HSS are GOOD. O’Lenic et al (2008) compared the HSS and % non-EC of official (OFF) forecasts made in real-time from 1995 through 2004 with forecasts made using an objective consolidation (CON) of the identical four main forecast tools which were used to prepare the real-time forecasts. Period (1995 -2004) mean T, P forecast skills for OFF/CON were 22/26 for T, and 8. 8/12. 1 for P. CON % non-EC is also higher. 20 CPC began using CON as a first guess in 2006.
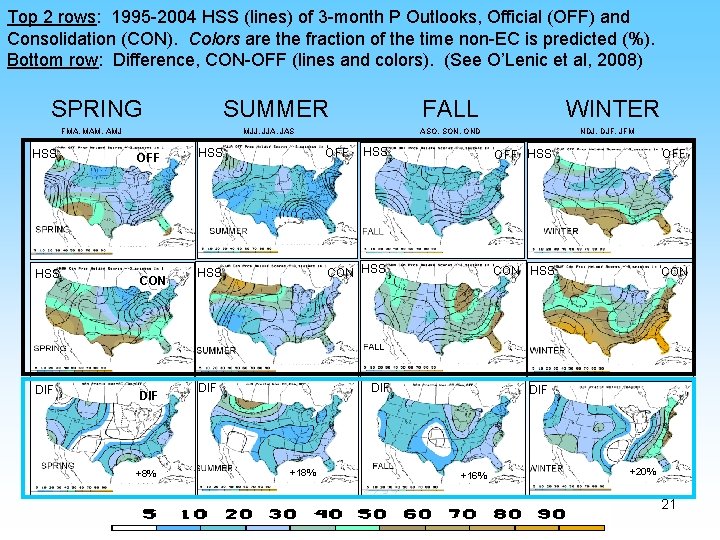
Top 2 rows: 1995 -2004 HSS (lines) of 3 -month P Outlooks, Official (OFF) and Consolidation (CON). Colors are the fraction of the time non-EC is predicted (%). Bottom row: Difference, CON-OFF (lines and colors). (See O’Lenic et al, 2008) SPRING SUMMER FMA, MAM, AMJ HSS DIF FALL MJJ, JJA, JAS OFF CON DIF +8% WINTER ASO, SON, OND NDJ, DJF, JFM HSS OFF HSS CON DIF +18% DIF +16% +20% 21
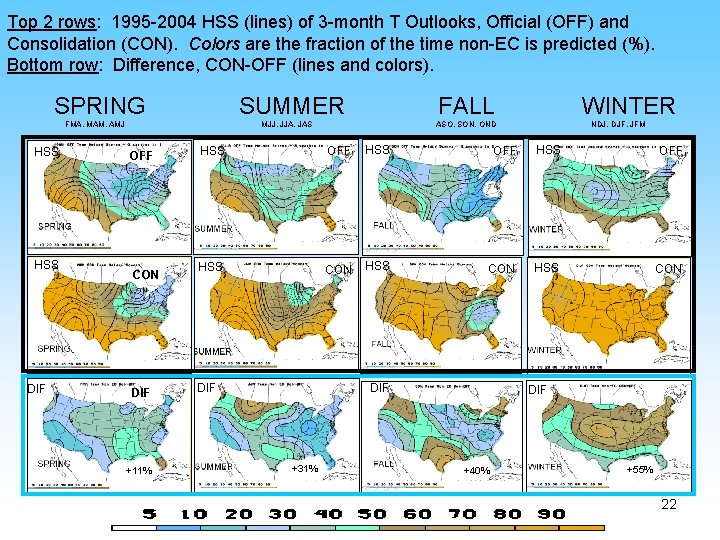
Top 2 rows: 1995 -2004 HSS (lines) of 3 -month T Outlooks, Official (OFF) and Consolidation (CON). Colors are the fraction of the time non-EC is predicted (%). Bottom row: Difference, CON-OFF (lines and colors). SPRING SUMMER FMA, MAM, AMJ HSS DIF FALL MJJ, JJA, JAS OFF CON DIF +11% WINTER ASO, SON, OND NDJ, DJF, JFM HSS OFF HSS CON DIF +31% DIF +40% +55% 22
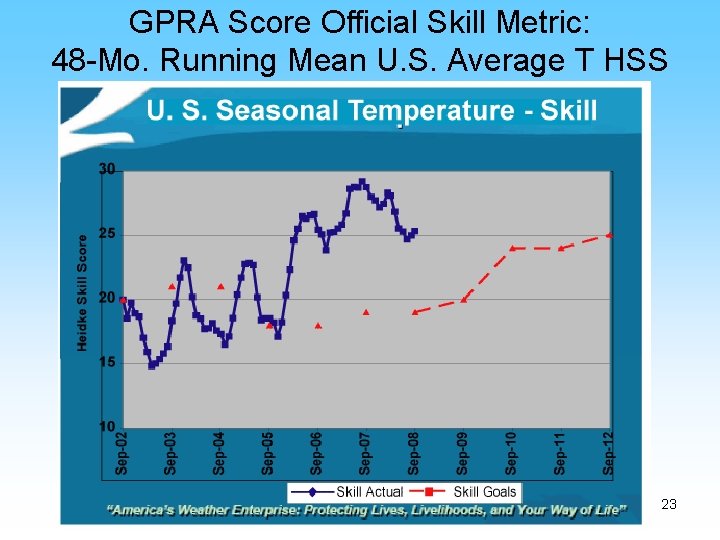
GPRA Score Official Skill Metric: 48 -Mo. Running Mean U. S. Average T HSS 23
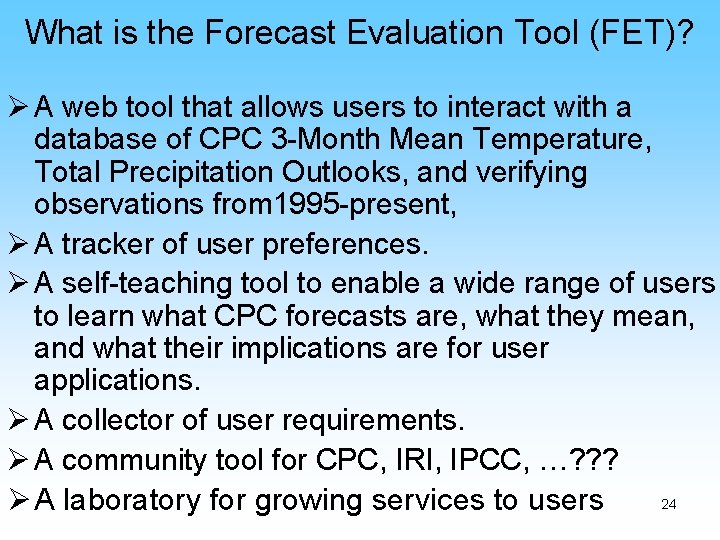
What is the Forecast Evaluation Tool (FET)? Ø A web tool that allows users to interact with a database of CPC 3 -Month Mean Temperature, Total Precipitation Outlooks, and verifying observations from 1995 -present, Ø A tracker of user preferences. Ø A self-teaching tool to enable a wide range of users to learn what CPC forecasts are, what they mean, and what their implications are for user applications. Ø A collector of user requirements. Ø A community tool for CPC, IRI, IPCC, …? ? ? 24 Ø A laboratory for growing services to users
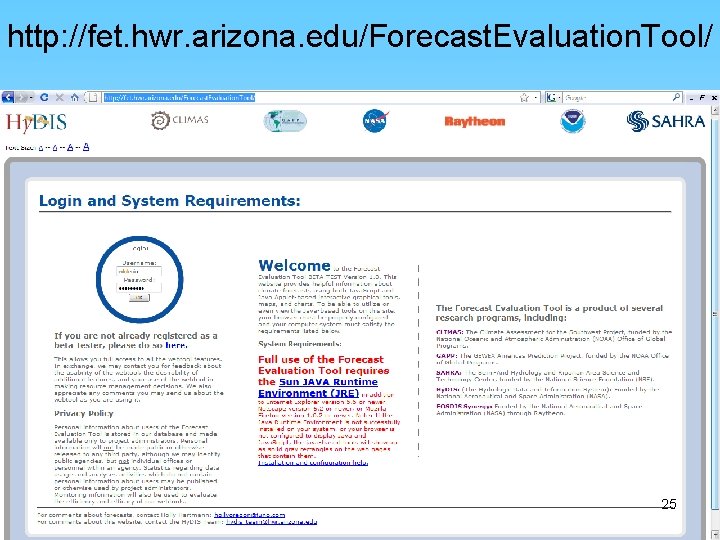
http: //fet. hwr. arizona. edu/Forecast. Evaluation. Tool/ 25
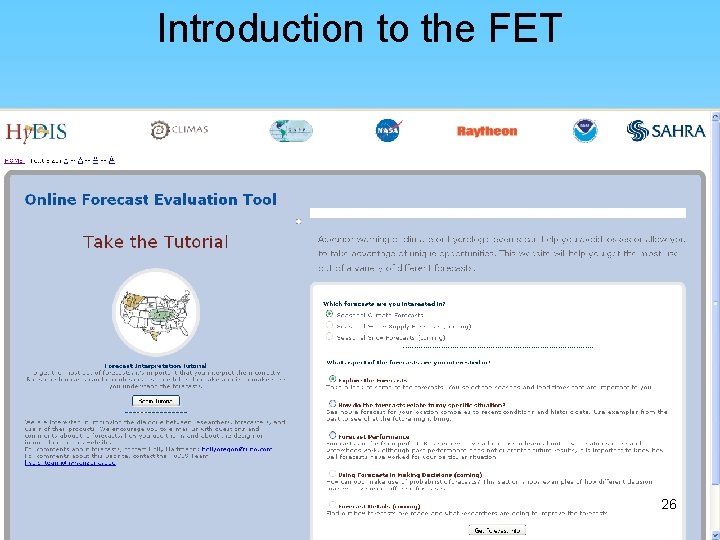
Introduction to the FET 26
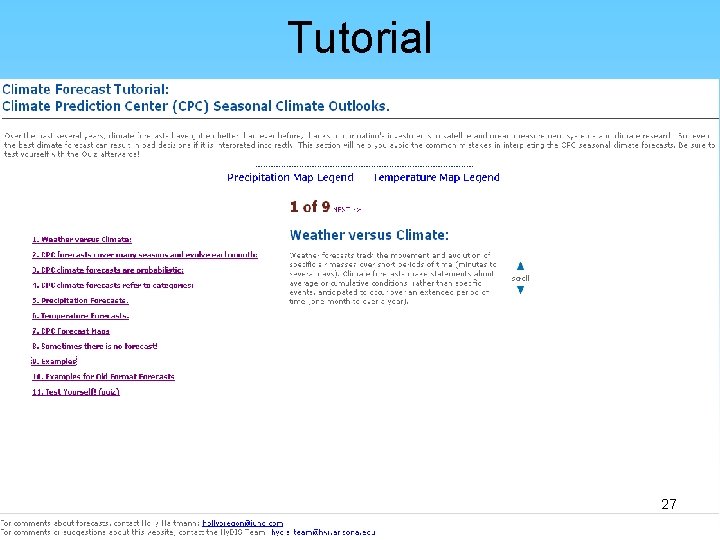
Tutorial 27
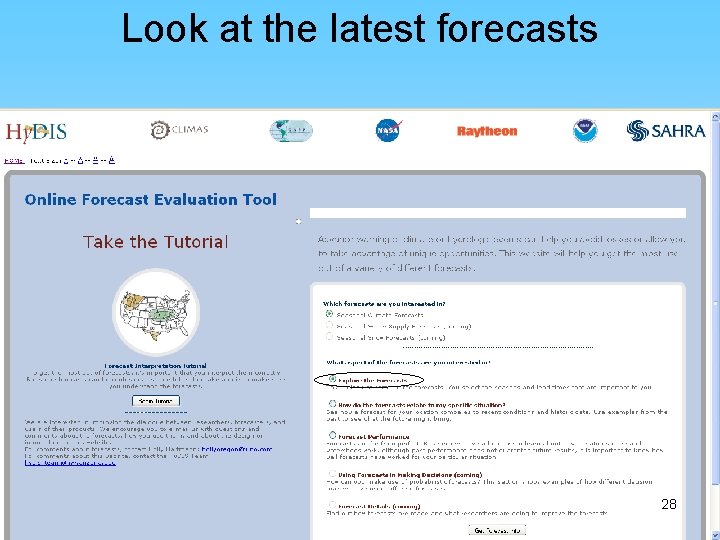
Look at the latest forecasts 28
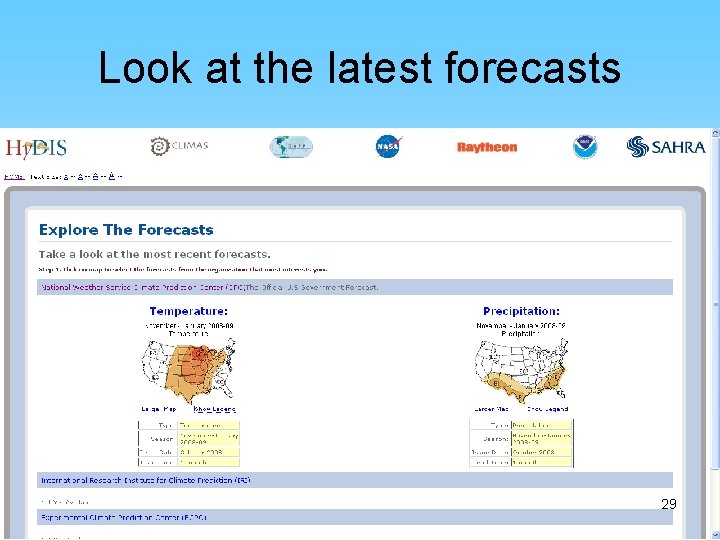
Look at the latest forecasts 29
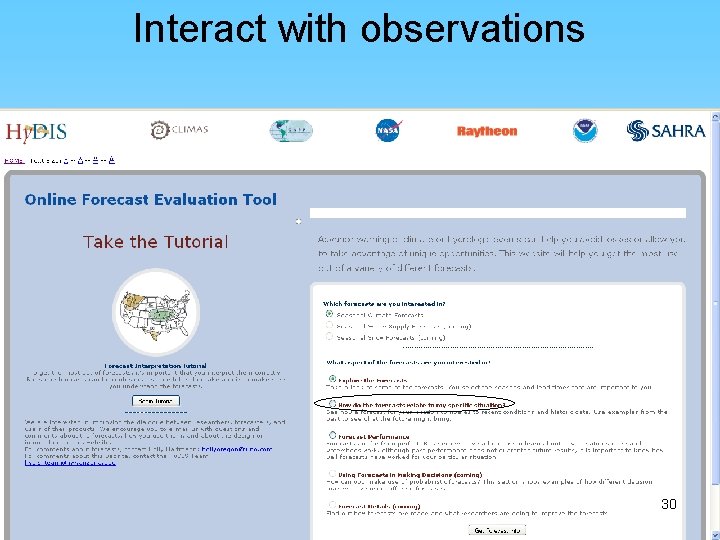
Interact with observations 30
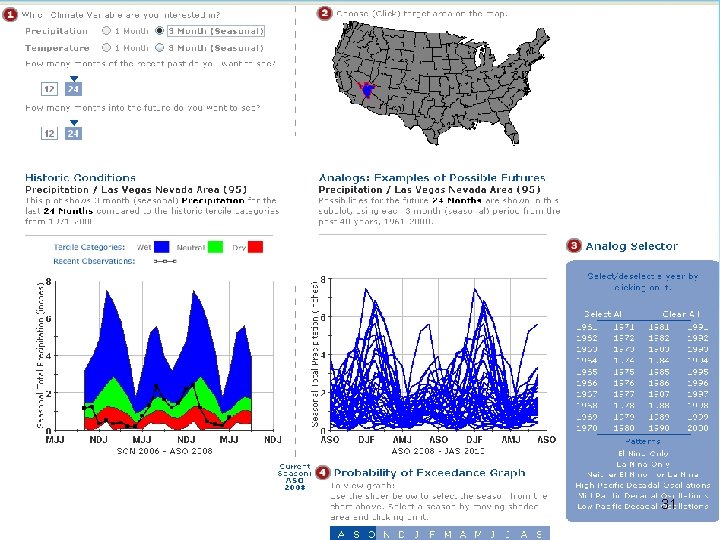
31
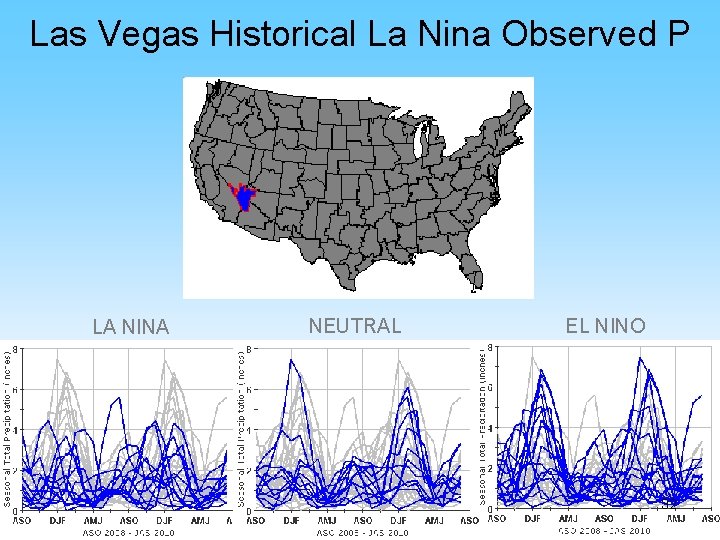
Las Vegas Historical La Nina Observed P LA NINA NEUTRAL EL NINO 32
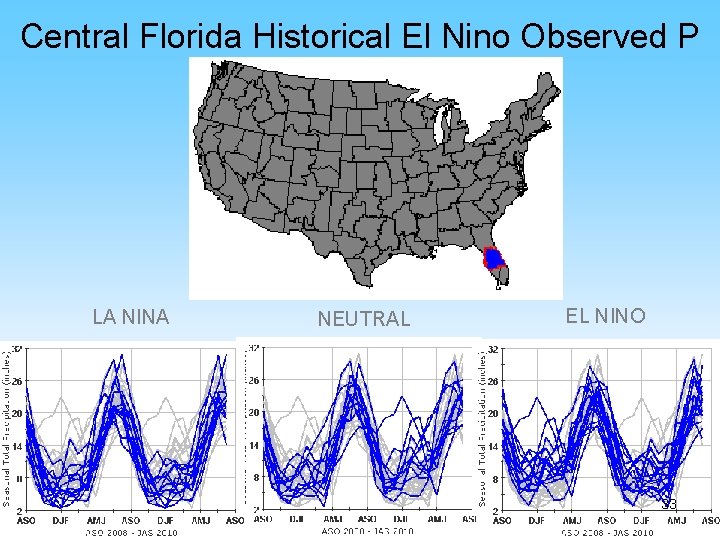
Central Florida Historical El Nino Observed P LA NINA NEUTRAL EL NINO 33
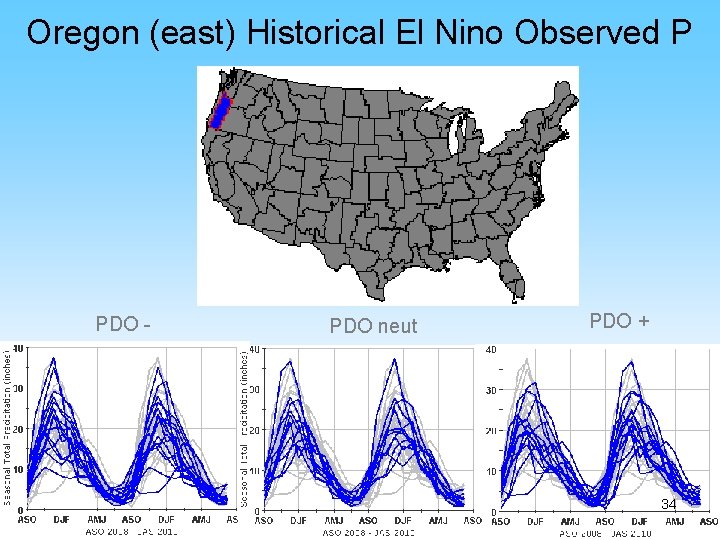
Oregon (east) Historical El Nino Observed P PDO - PDO neut PDO + 34
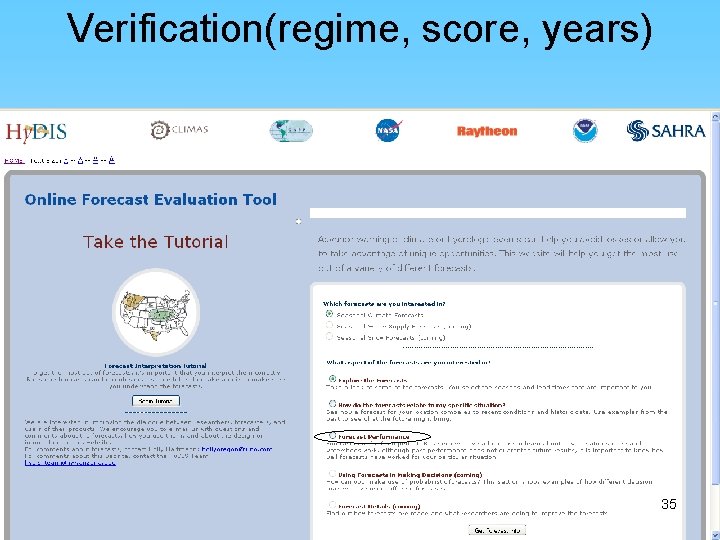
Verification(regime, score, years) 35
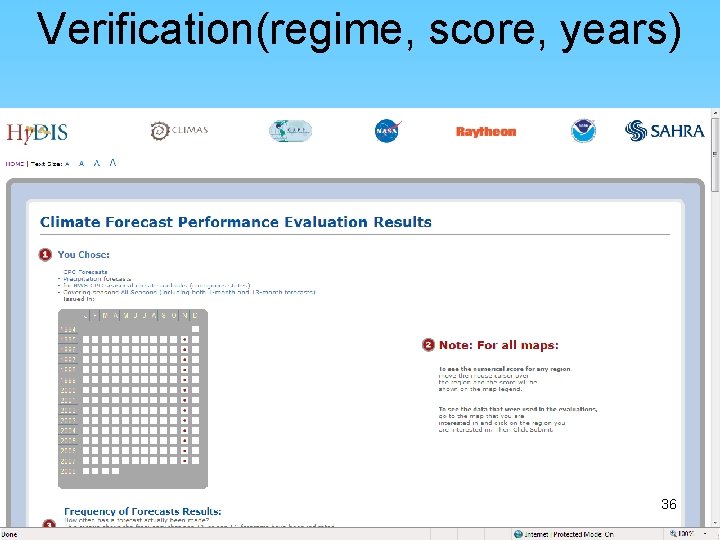
Verification(regime, score, years) 36
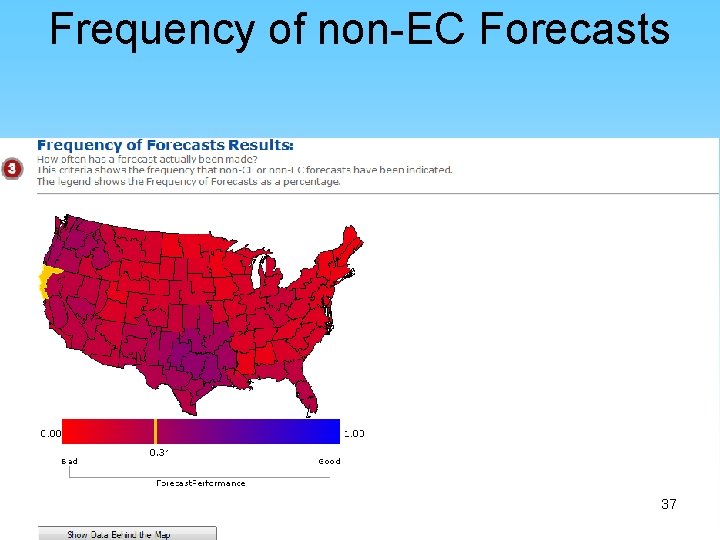
Frequency of non-EC Forecasts 37
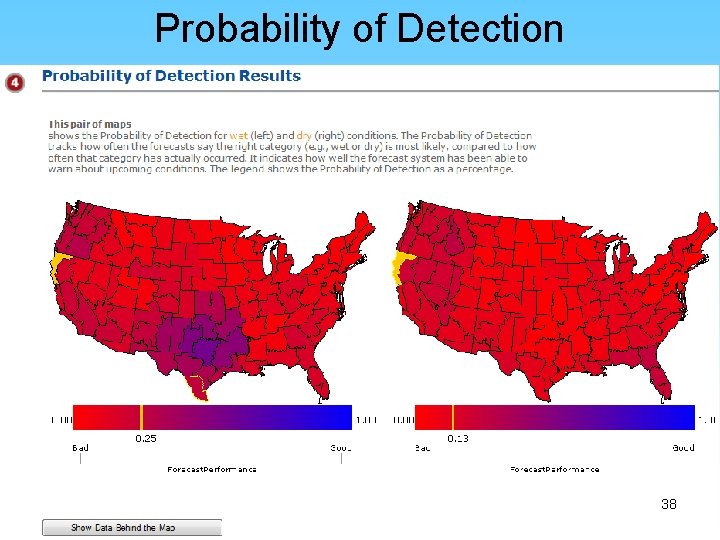
Probability of Detection 38
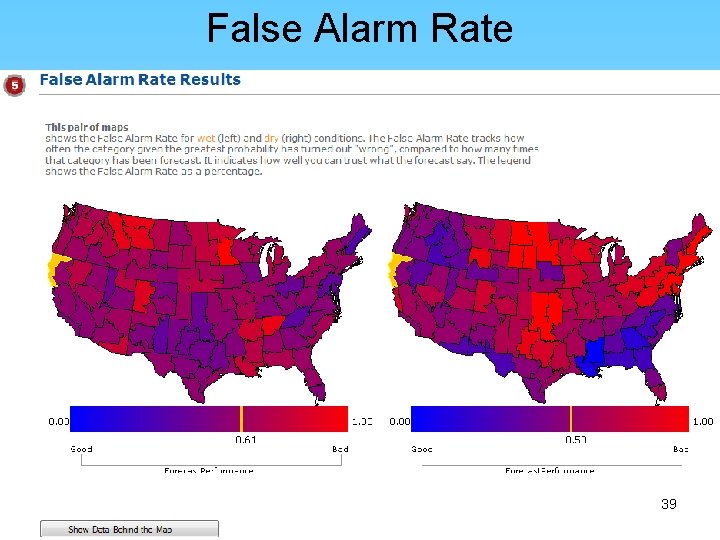
False Alarm Rate 39
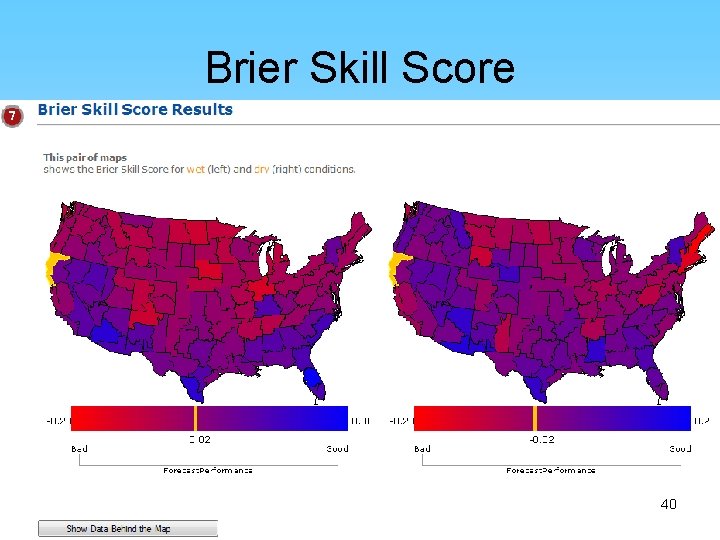
Brier Skill Score 40
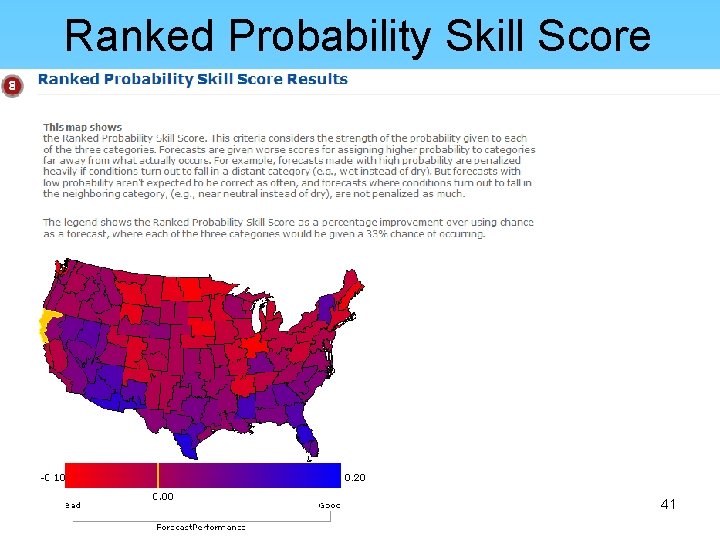
Ranked Probability Skill Score 41
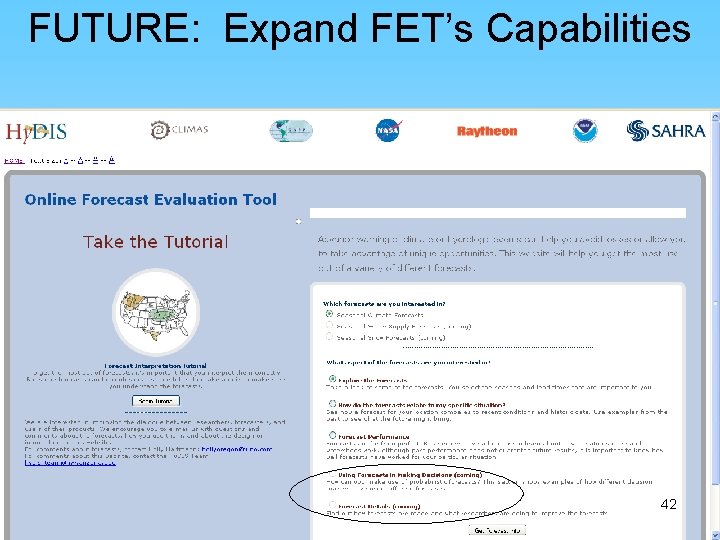
FUTURE: Expand FET’s Capabilities 42
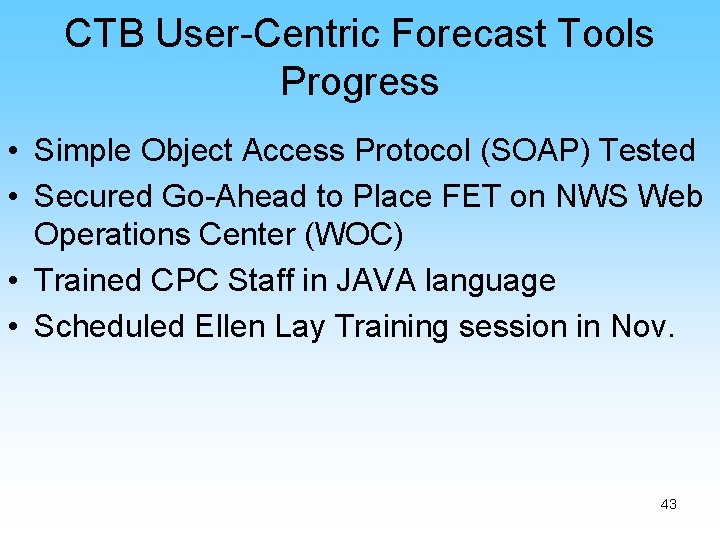
CTB User-Centric Forecast Tools Progress • Simple Object Access Protocol (SOAP) Tested • Secured Go-Ahead to Place FET on NWS Web Operations Center (WOC) • Trained CPC Staff in JAVA language • Scheduled Ellen Lay Training session in Nov. 43
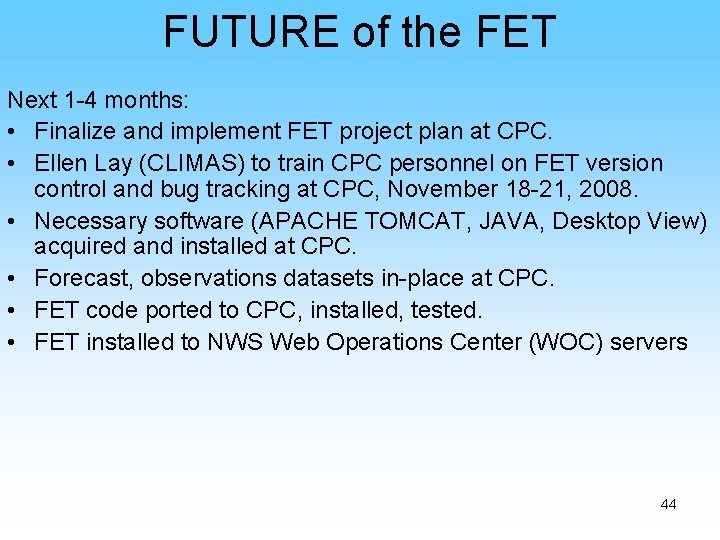
FUTURE of the FET Next 1 -4 months: • Finalize and implement FET project plan at CPC. • Ellen Lay (CLIMAS) to train CPC personnel on FET version control and bug tracking at CPC, November 18 -21, 2008. • Necessary software (APACHE TOMCAT, JAVA, Desktop View) acquired and installed at CPC. • Forecast, observations datasets in-place at CPC. • FET code ported to CPC, installed, tested. • FET installed to NWS Web Operations Center (WOC) servers 44
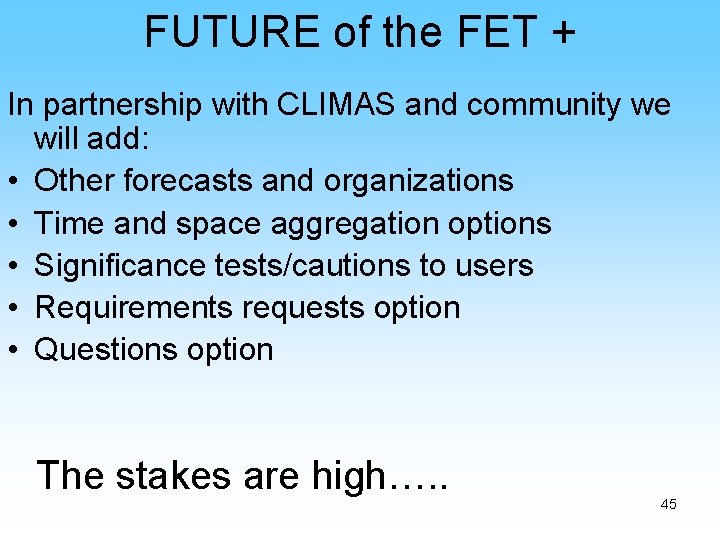
FUTURE of the FET + In partnership with CLIMAS and community we will add: • Other forecasts and organizations • Time and space aggregation options • Significance tests/cautions to users • Requirements requests option • Questions option The stakes are high…. . 45
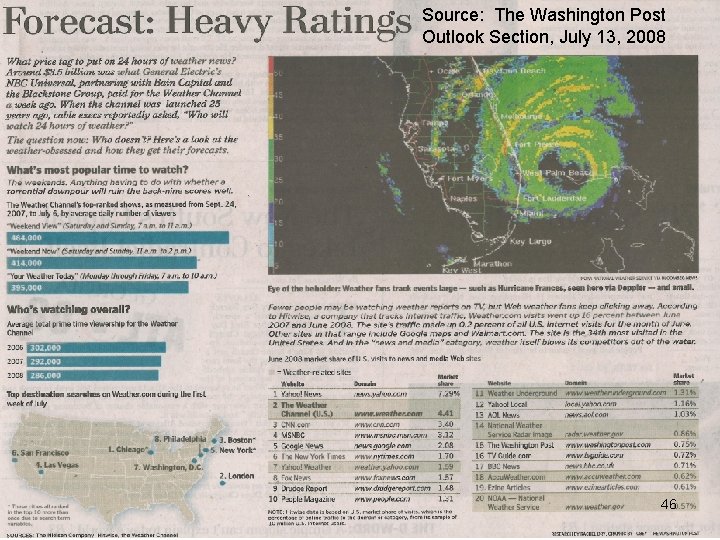
Source: The Washington Post Outlook Section, July 13, 2008 46
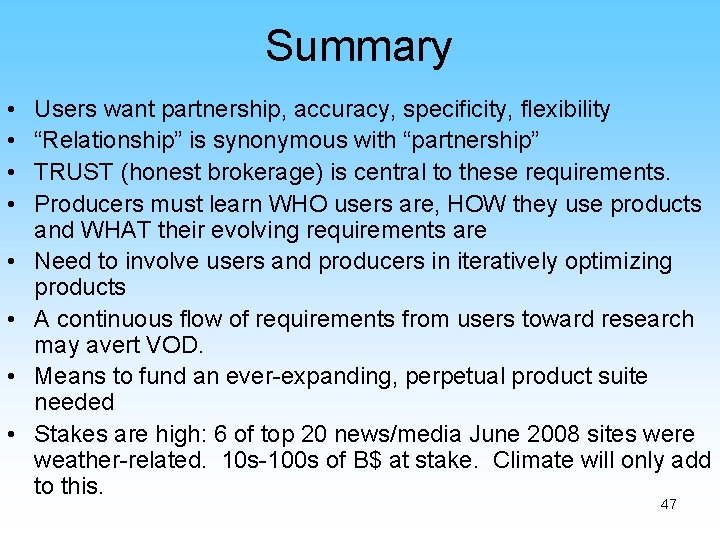
Summary • • Users want partnership, accuracy, specificity, flexibility “Relationship” is synonymous with “partnership” TRUST (honest brokerage) is central to these requirements. Producers must learn WHO users are, HOW they use products and WHAT their evolving requirements are Need to involve users and producers in iteratively optimizing products A continuous flow of requirements from users toward research may avert VOD. Means to fund an ever-expanding, perpetual product suite needed Stakes are high: 6 of top 20 news/media June 2008 sites were weather-related. 10 s-100 s of B$ at stake. Climate will only add to this. 47
Cpc long range forecast
Evaluation and control
Noaa corps cpc
Art 294 a 311 cpc
Artigo 606 cpc
Sarah-cpc
Art 610 cpc
Cpc cpma
Fraude contra credores cpc
Noaa corps cpc
Prazo para abertura de inventário novo cpc
Cpc cycle kelly
Nulla executio sine titulo
Importancia de la demanda
Artigo 775 cpc
Ipc vs cpc
Dr grace ng
Civil procedure 1908
Cpc monitoring
Reveiewed
Actualizar cpc
Art. 131 cpc
Prezentare google
Cpc 02
Commonality corollary example
Testamento particular novo cpc
Moodle cpc
Que es c.p.c. en contabilidad
Cpc assessment tools
Cpc vale do rio doce
Gmod surfing
Cpc pathology
Cpc cabi
Artigo 783 do cpc
Cpc pna
Wellbeing for school excellence evaluation support tool
Evaluation tool for instructional materials
Monitoring and evaluation tool sample deped
Instructional materials evaluation tool
Sip/aip based monitoring and evaluation instrument tool
Potter's tool is data cleaning tool
Fet examples
Amplification factor of jfet
Bjt and fet frequency response
Pch fet
Vp jfet
1nm transistor
Reconfigurable fet