Why and How to Replace Statistical Significance Tests
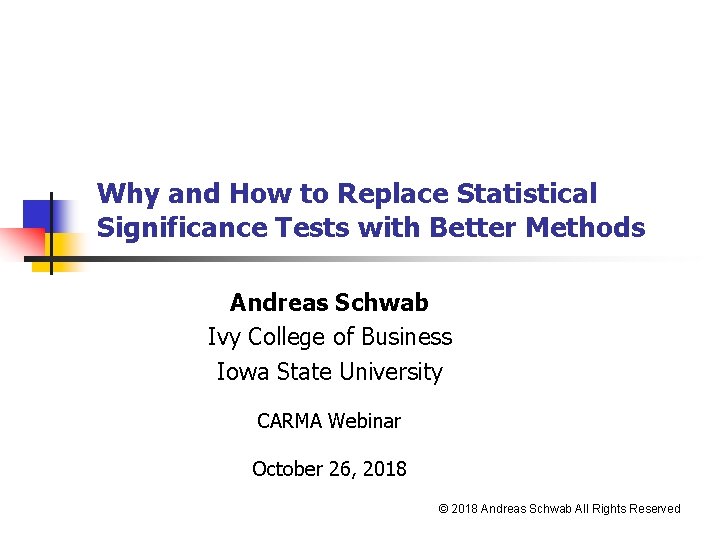
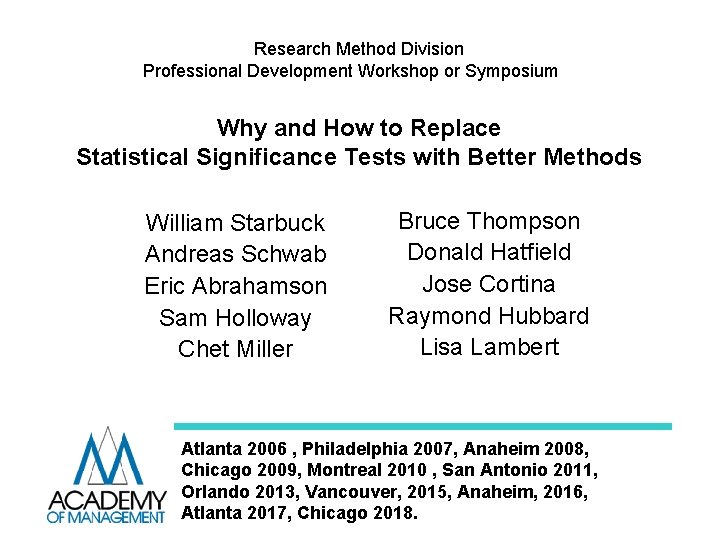
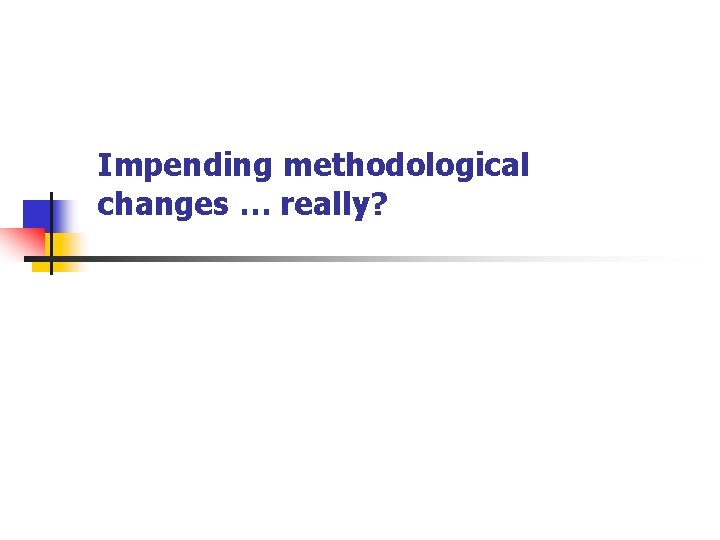
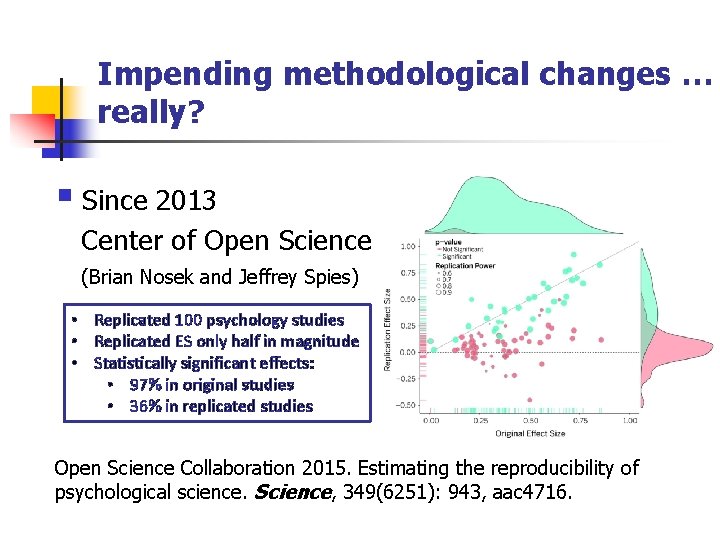
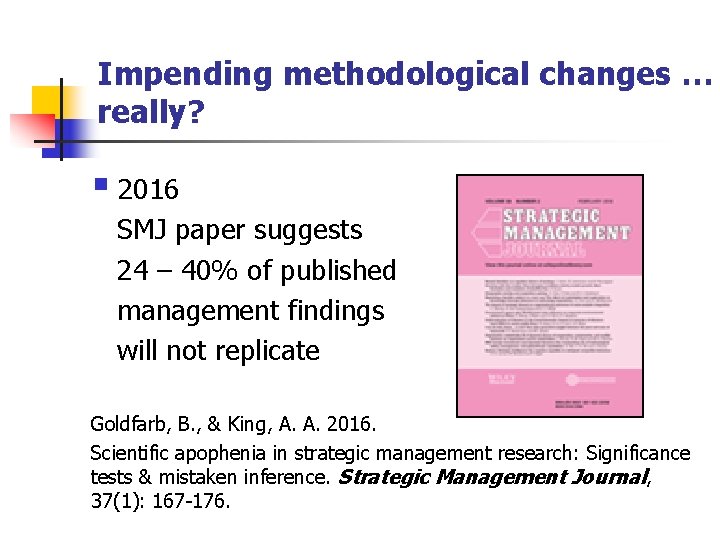
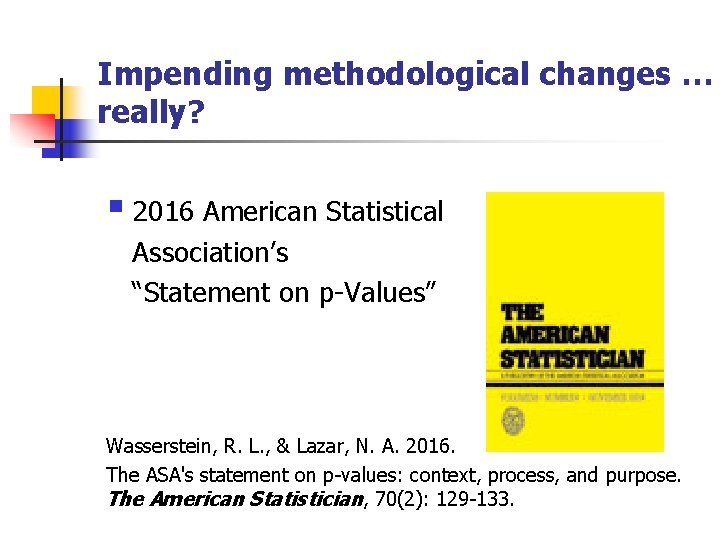
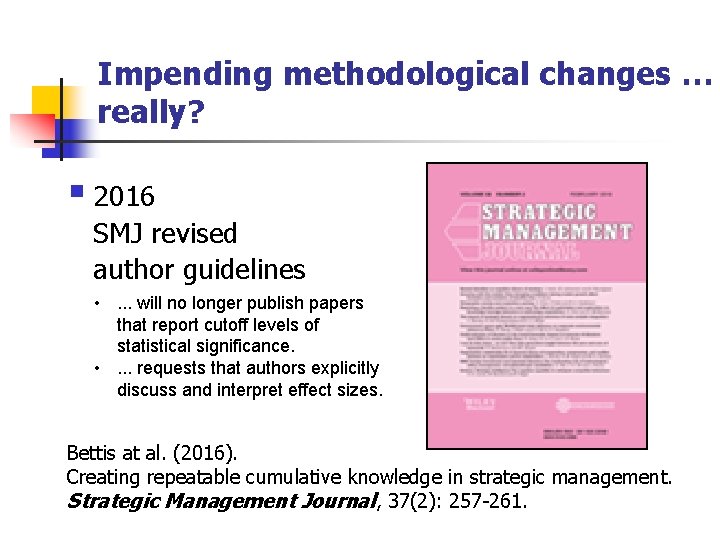
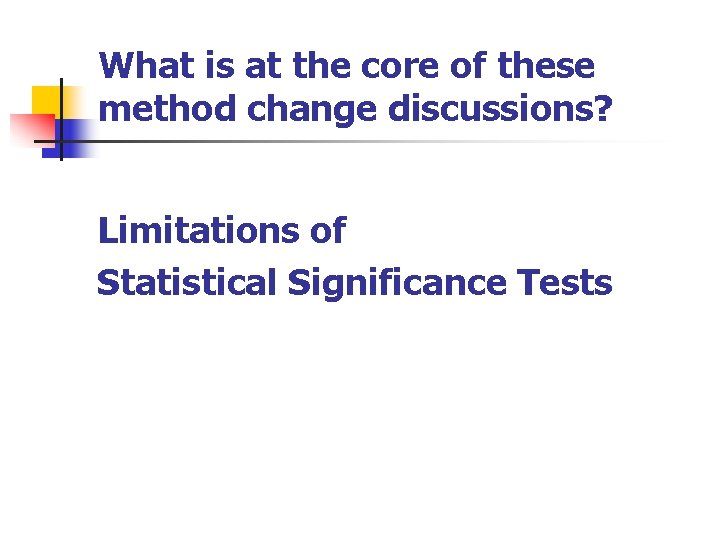
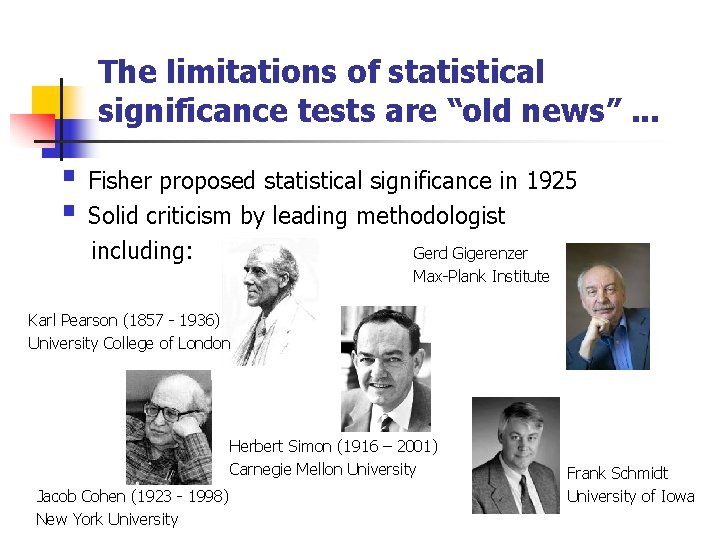
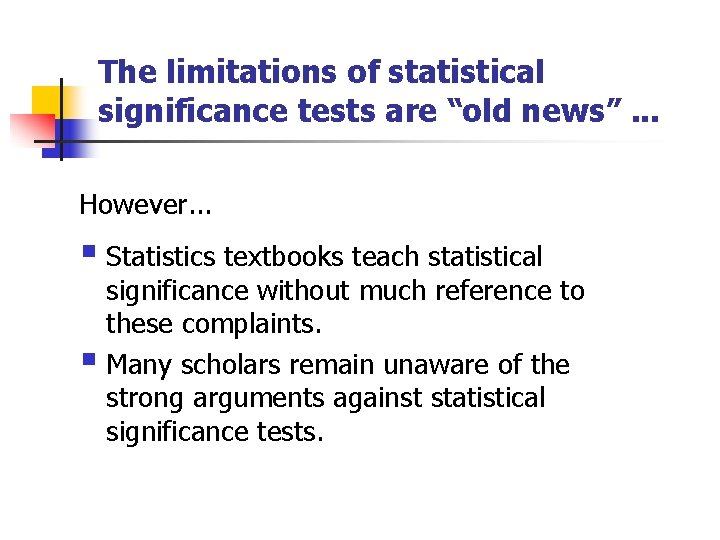
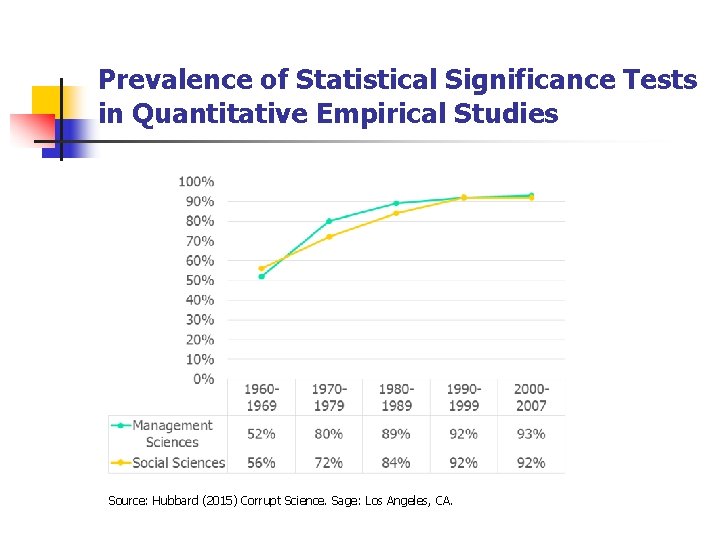
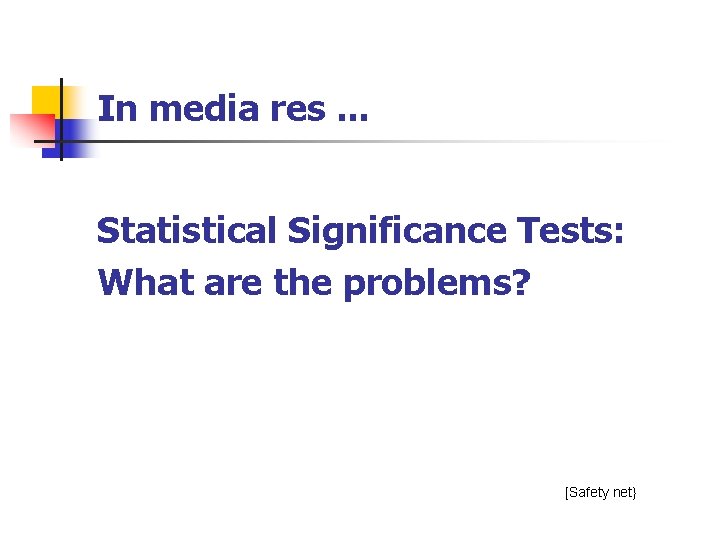
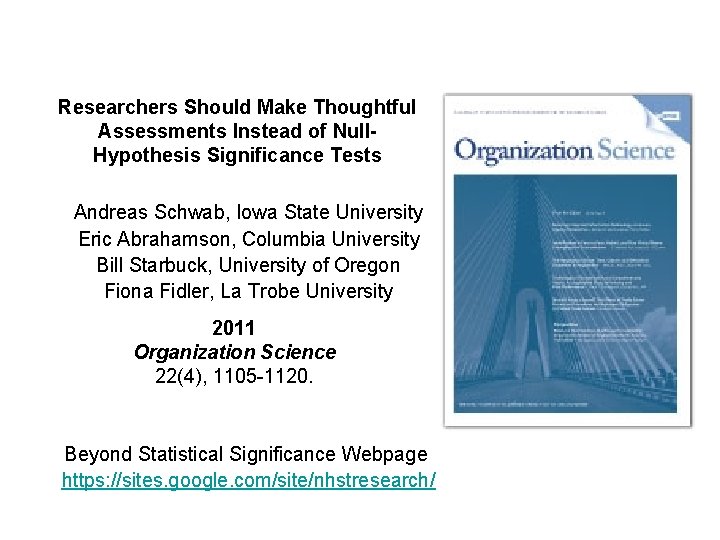
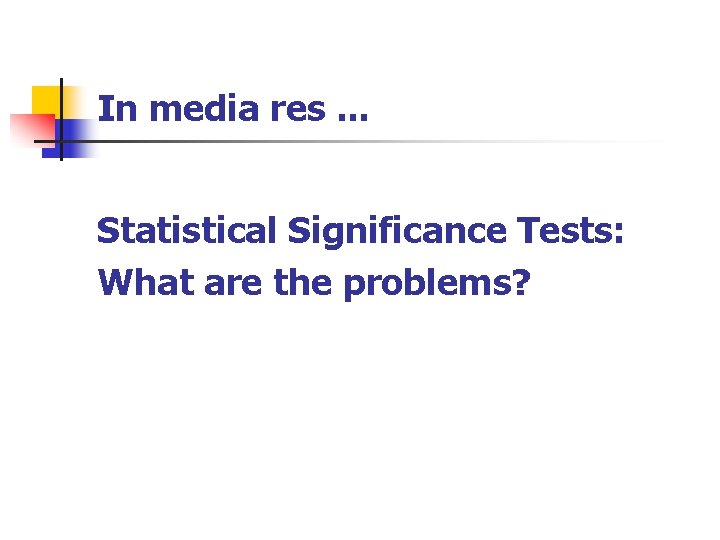
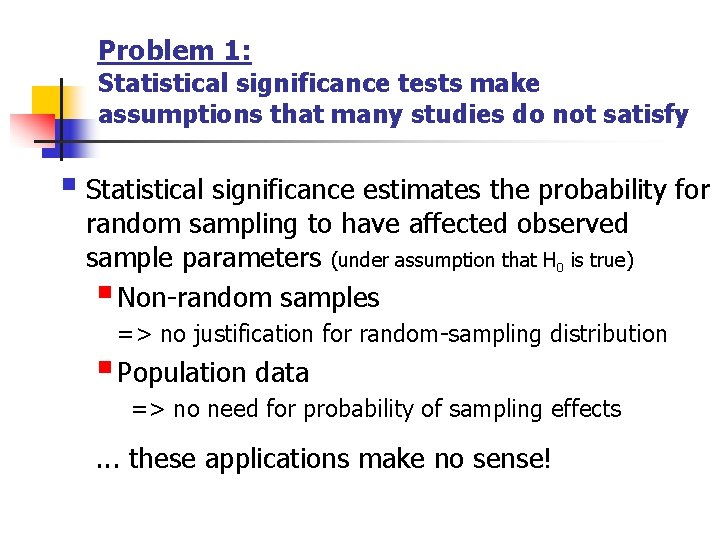
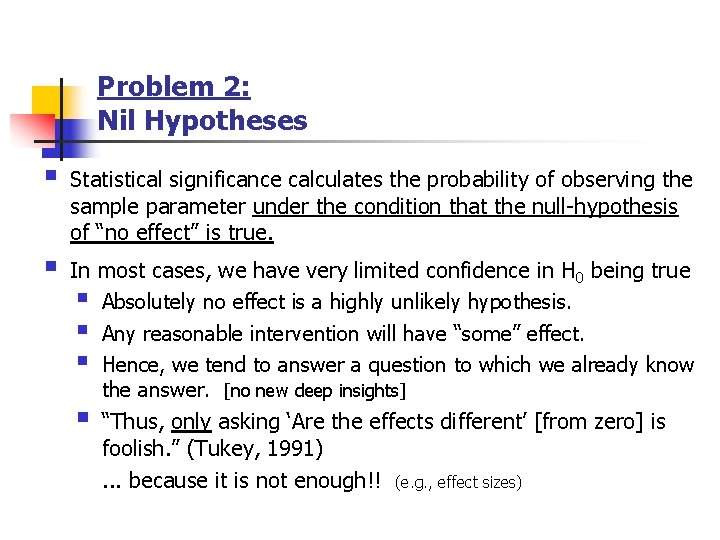
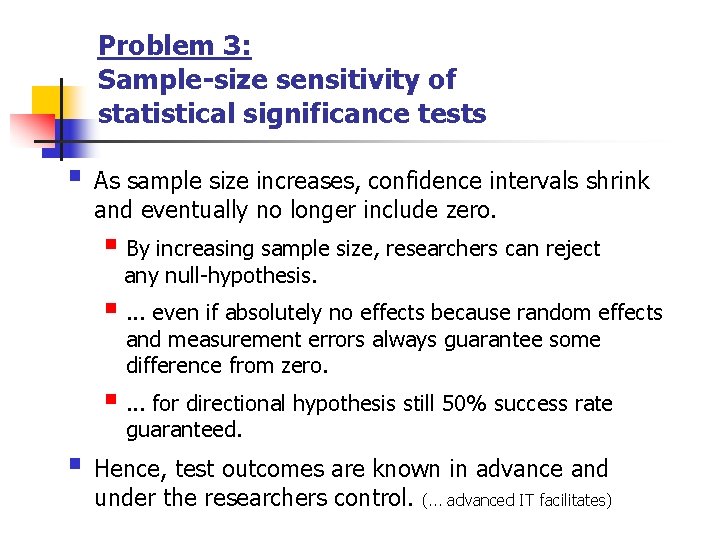
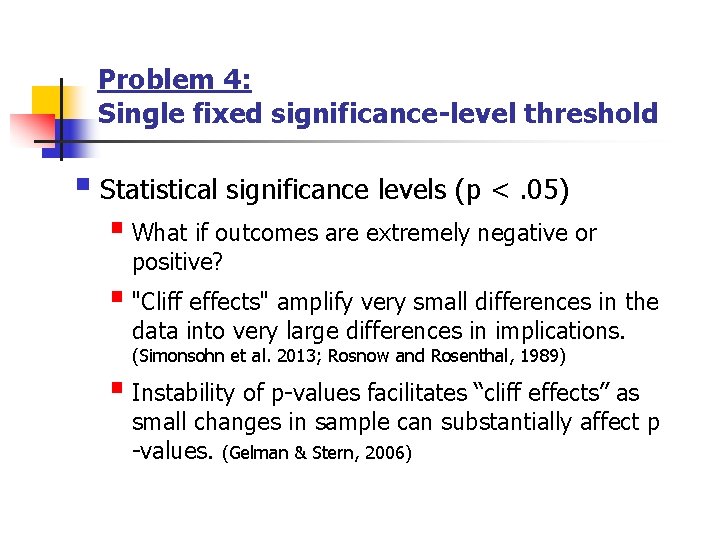
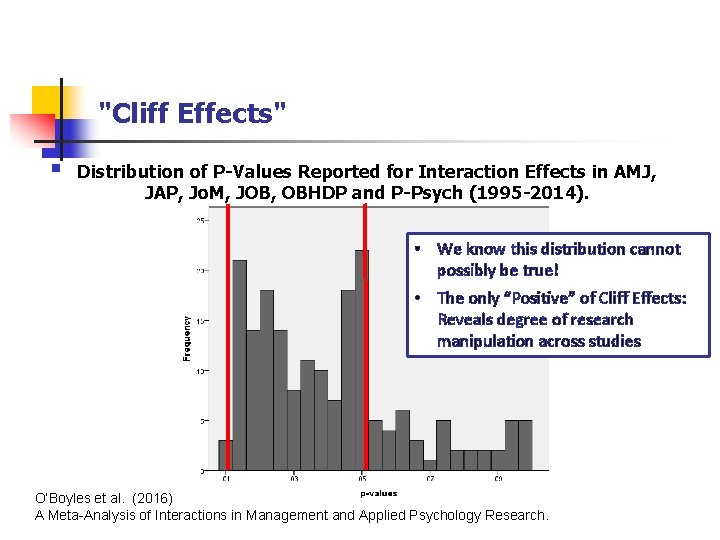
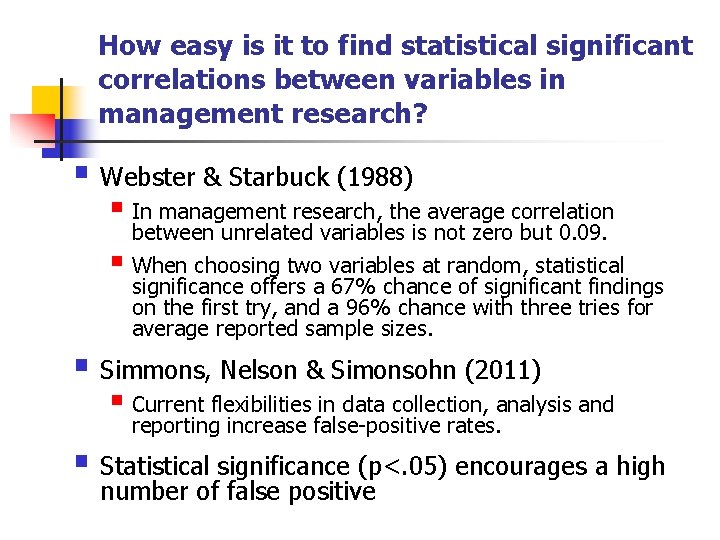
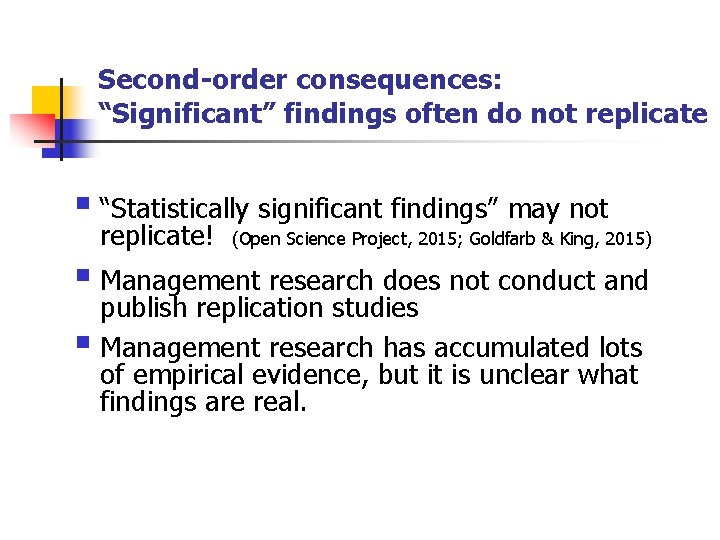
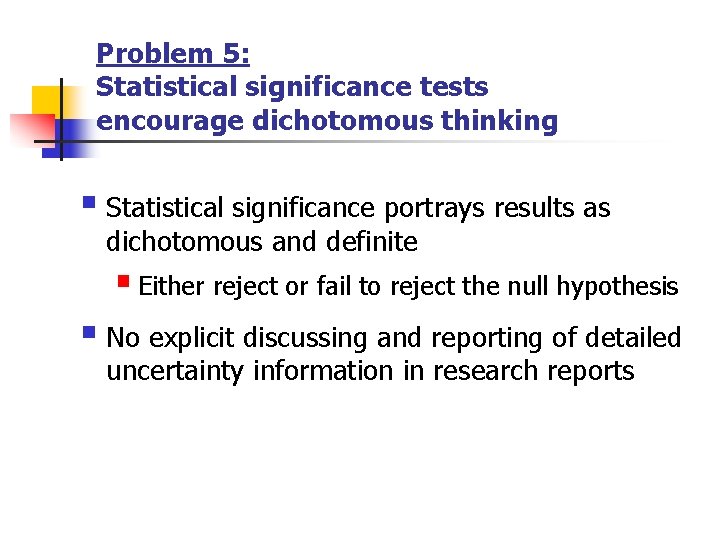
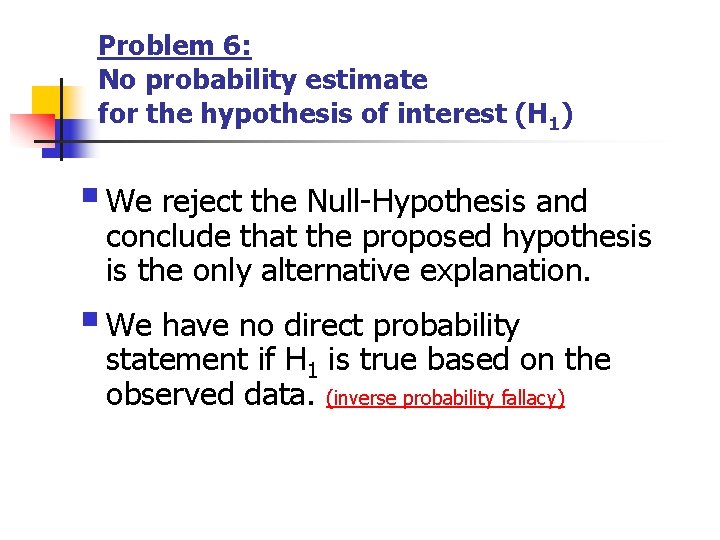
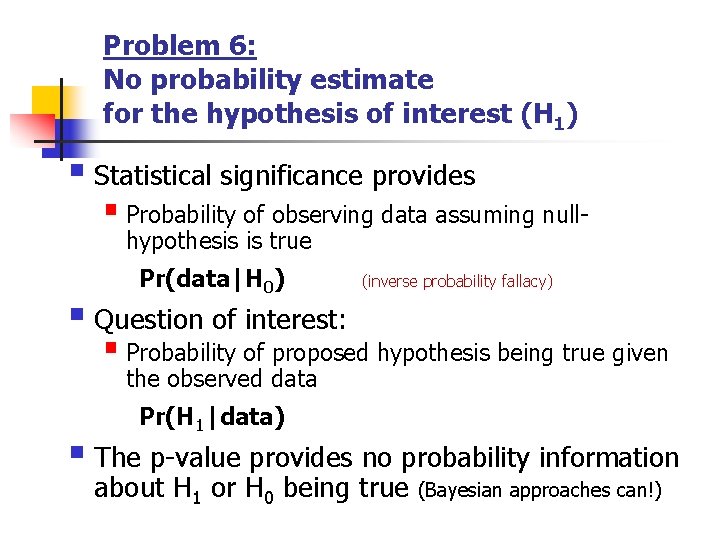
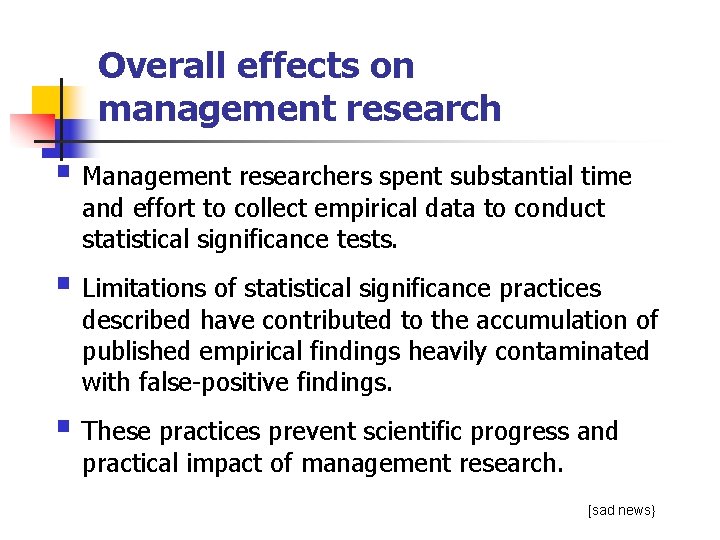
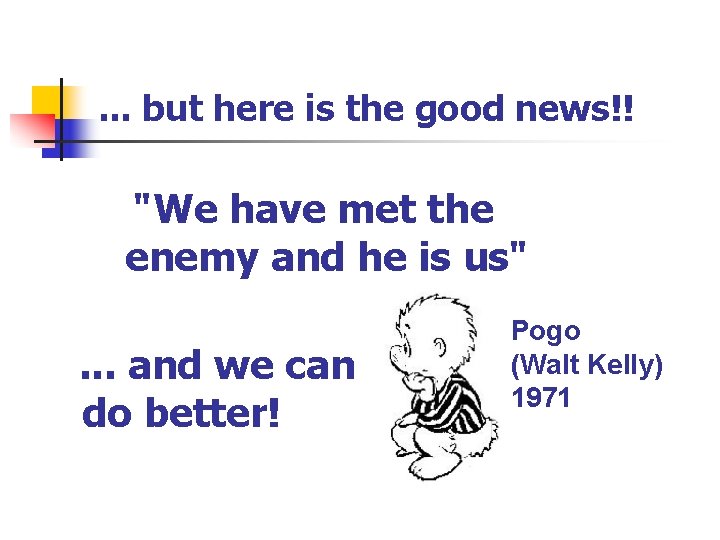
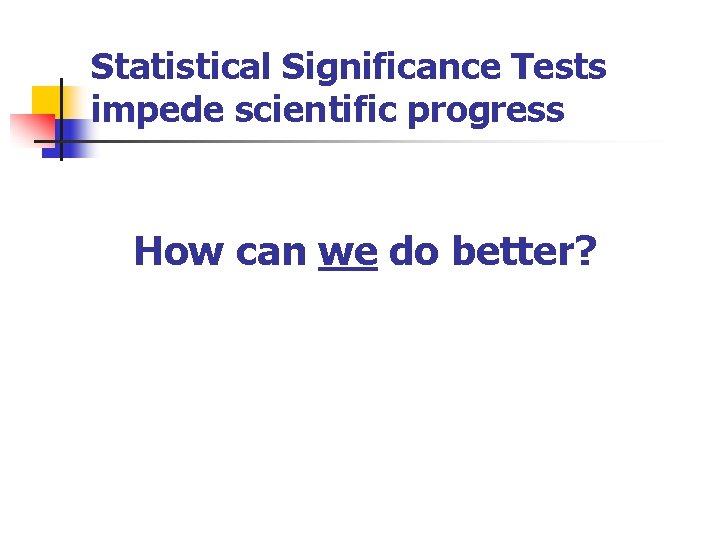
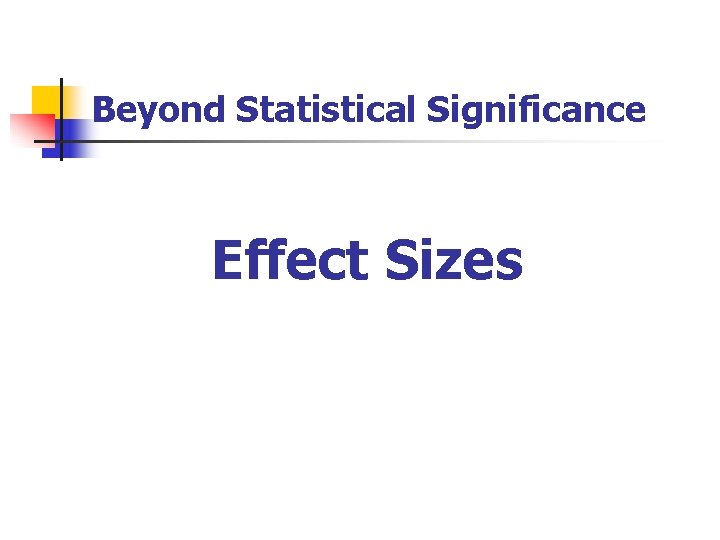
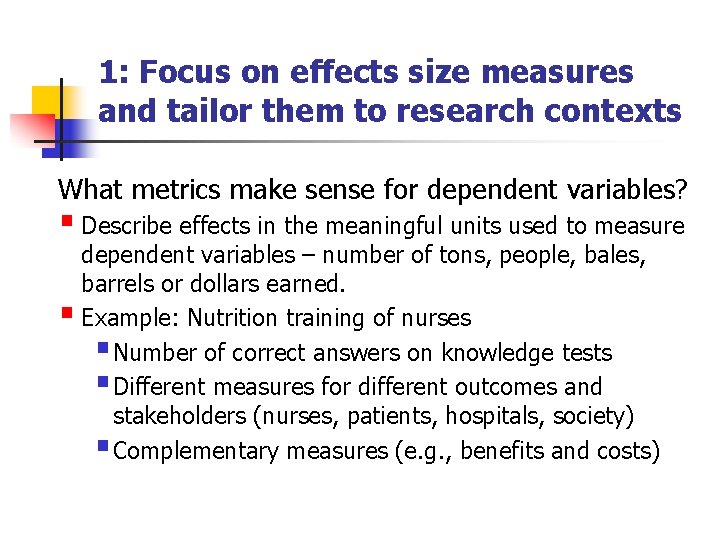
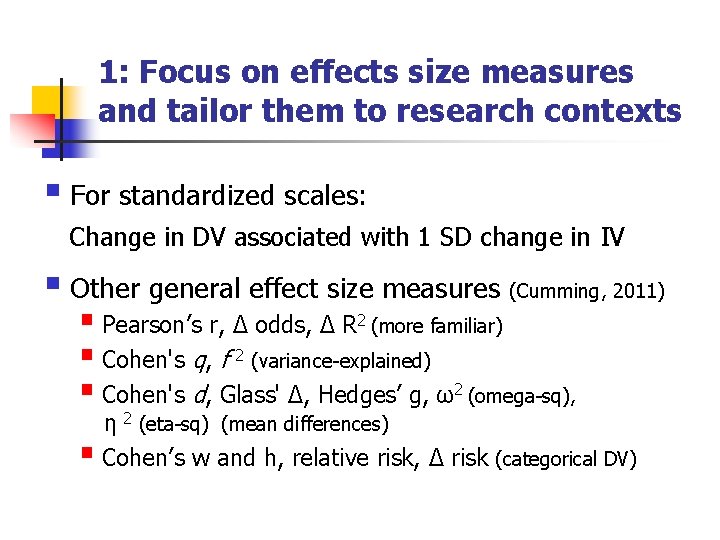
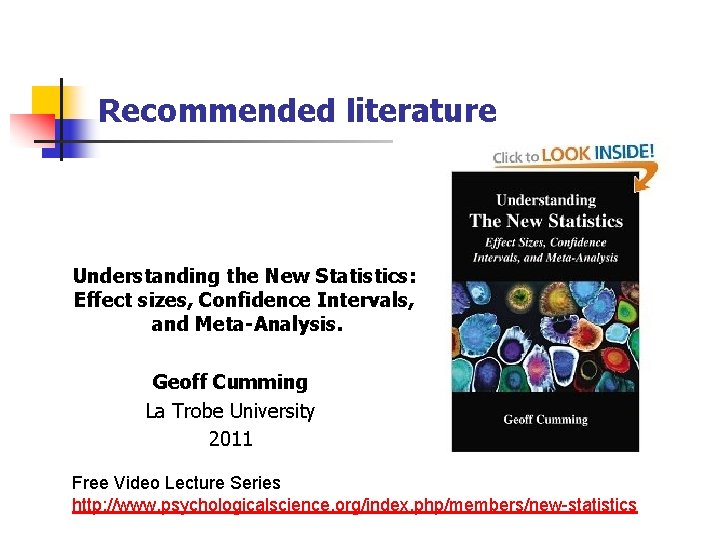
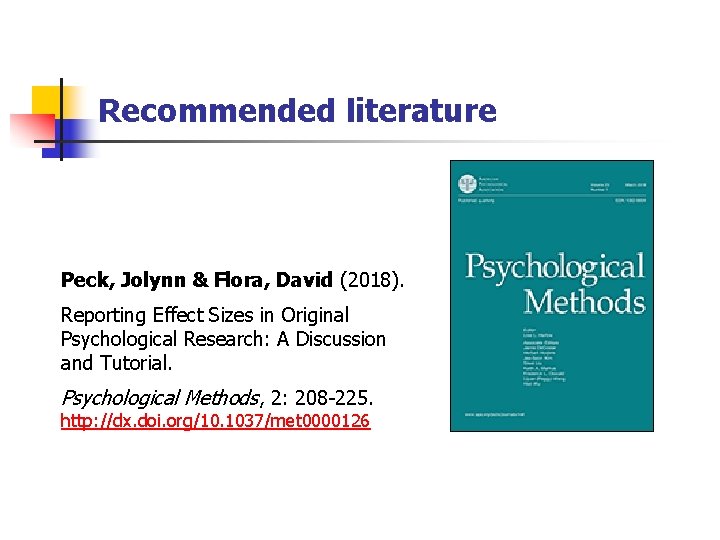
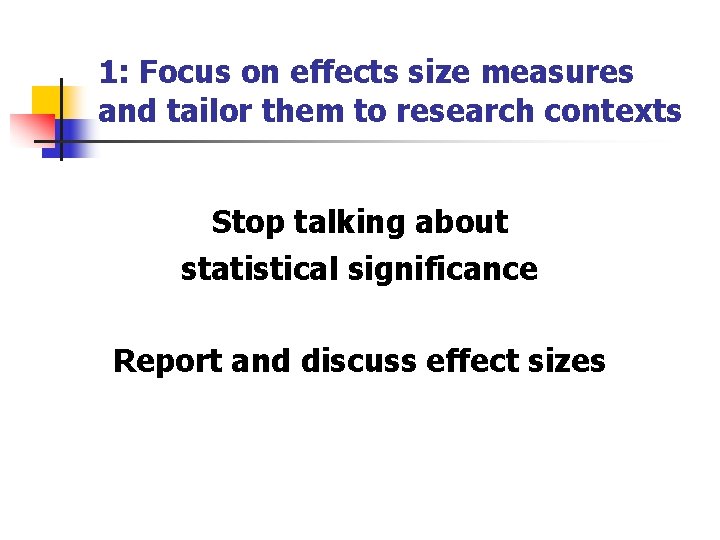
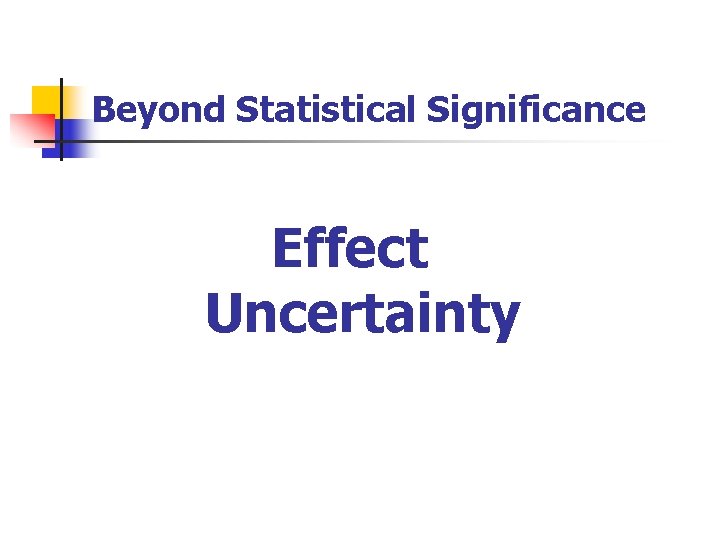
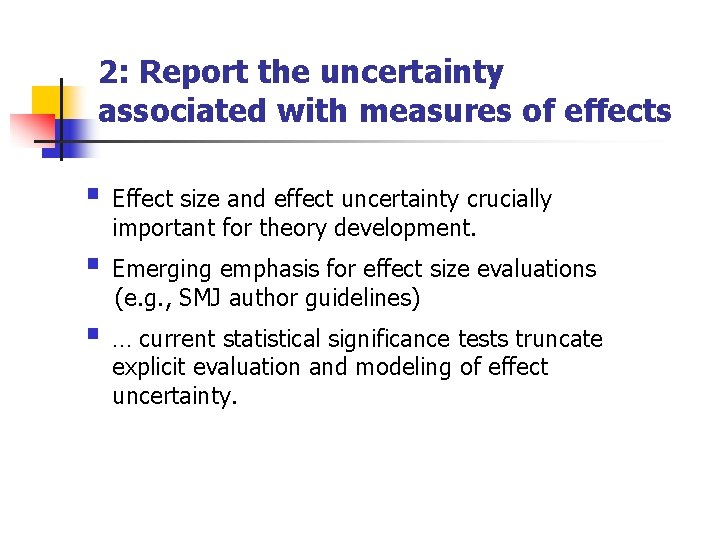
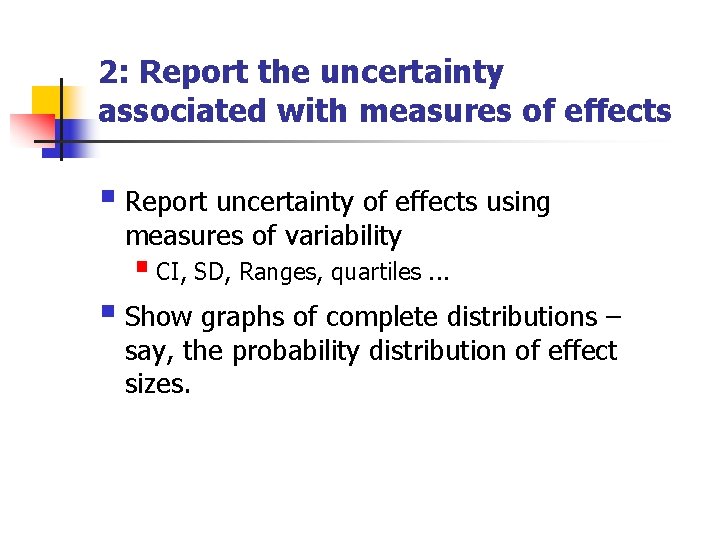
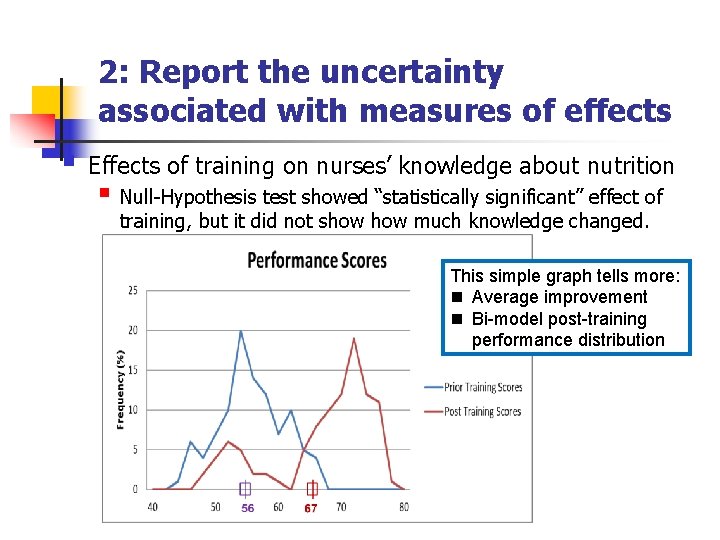
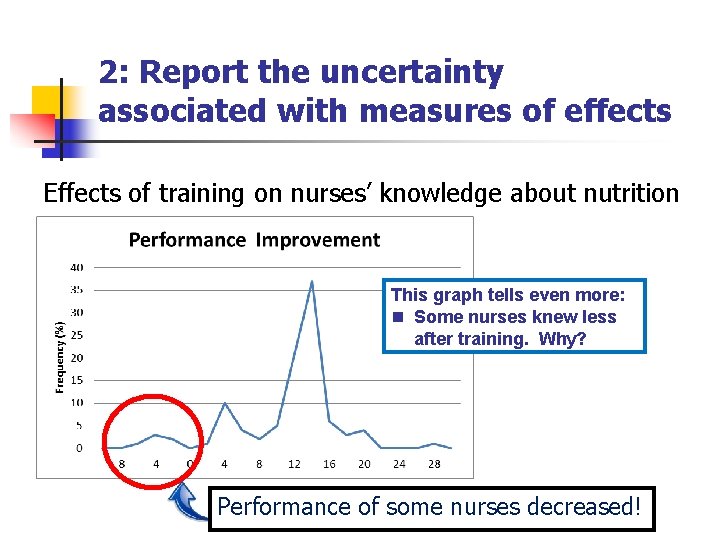
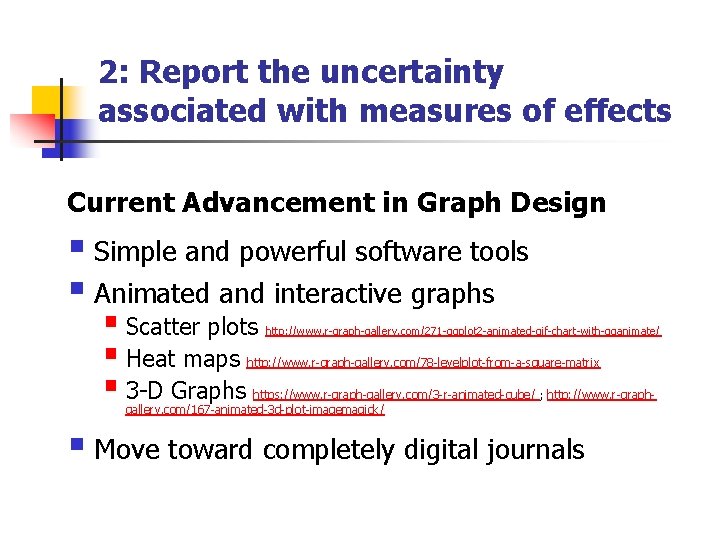
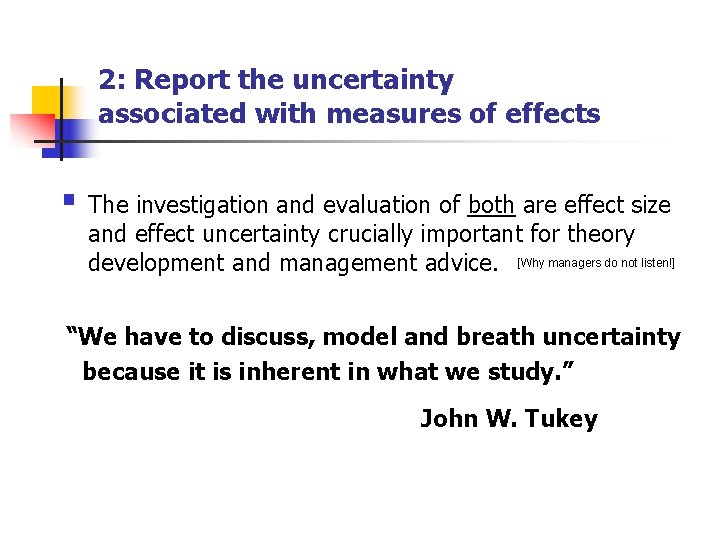
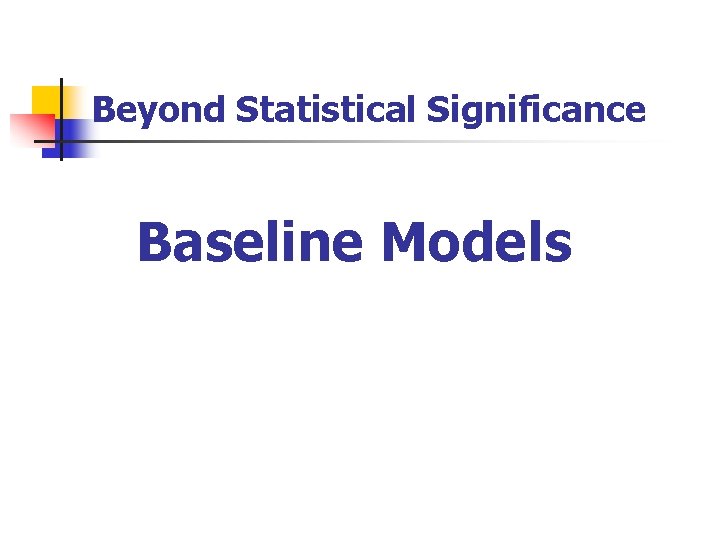
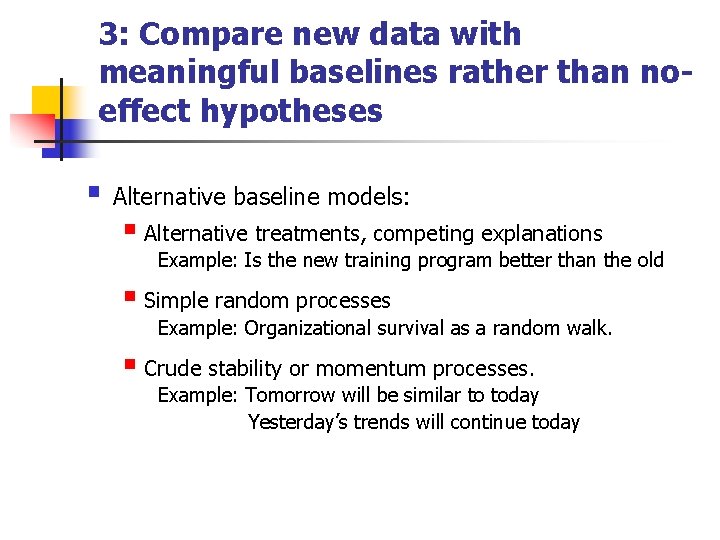
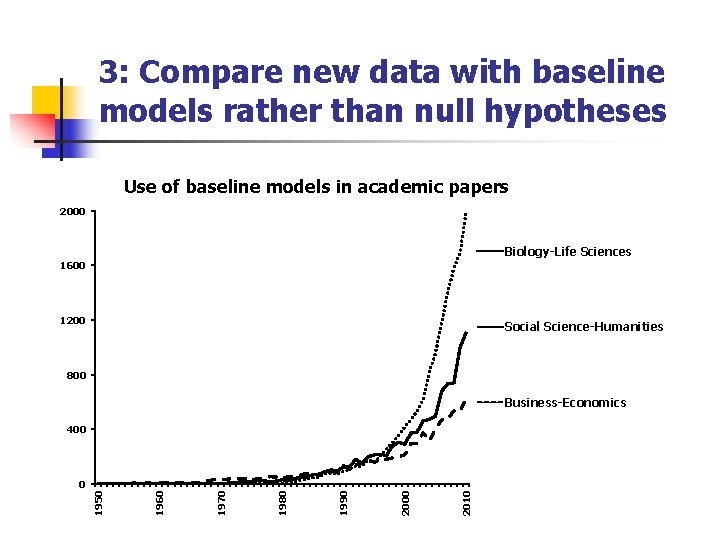
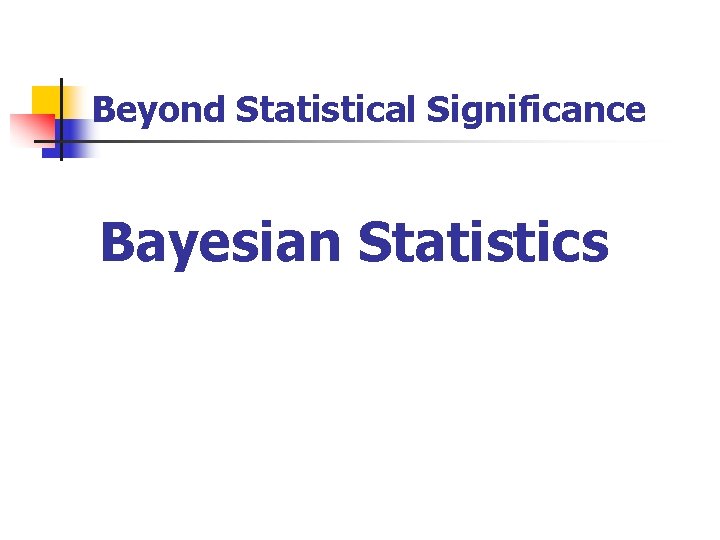
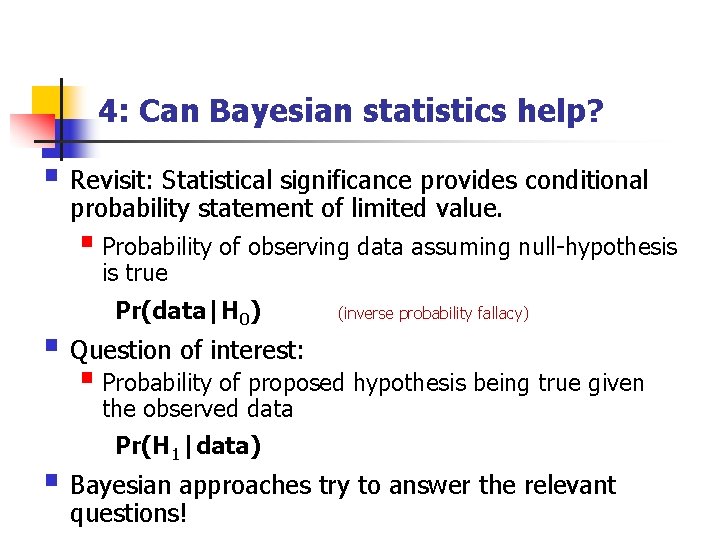
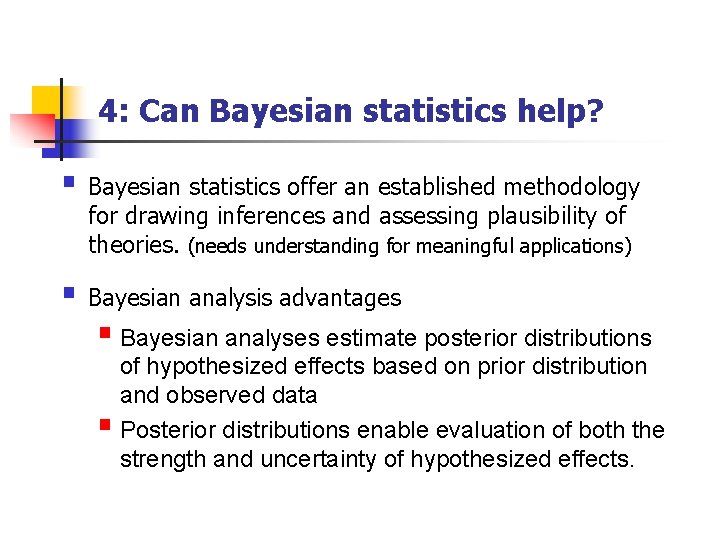
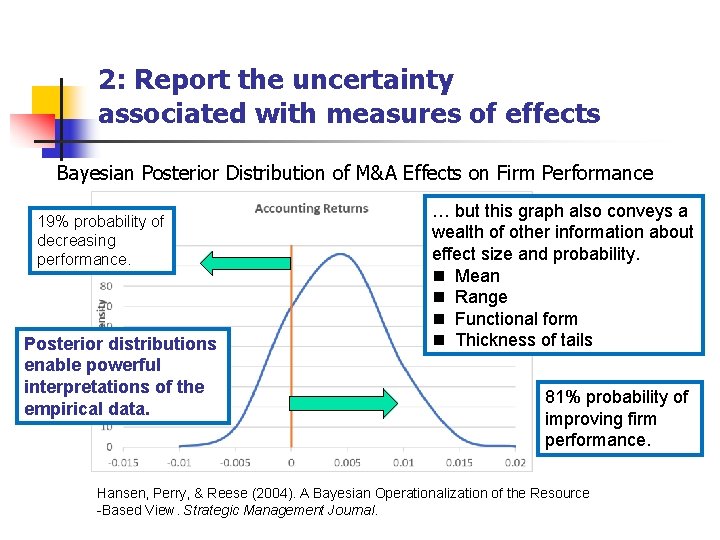
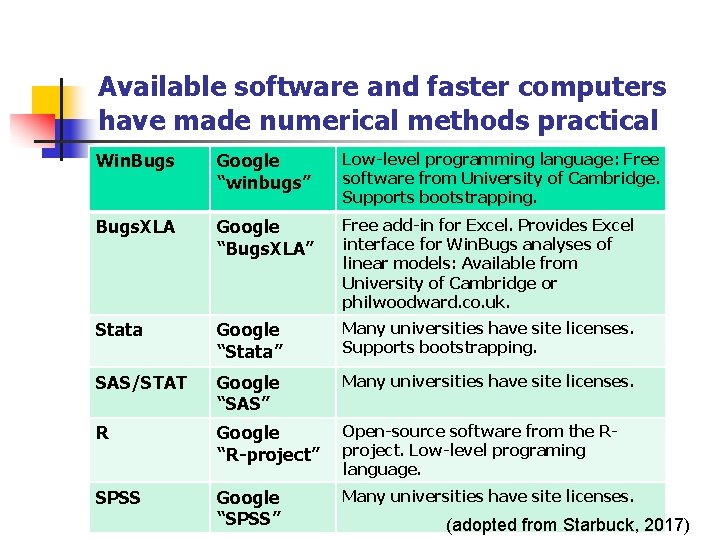
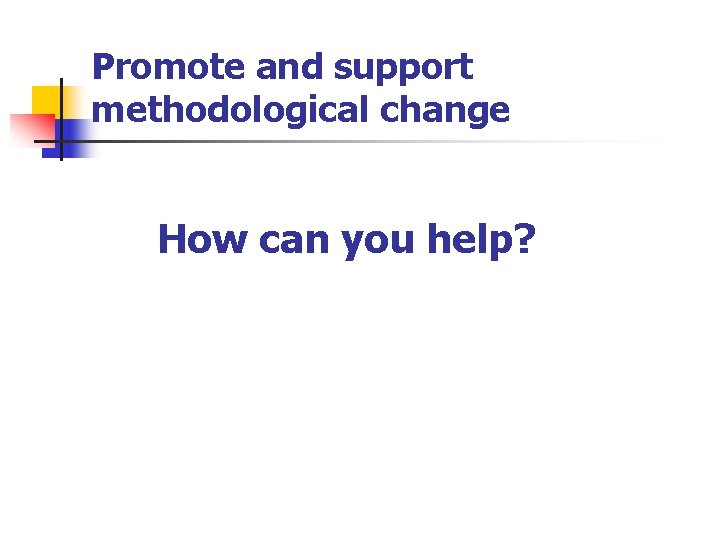
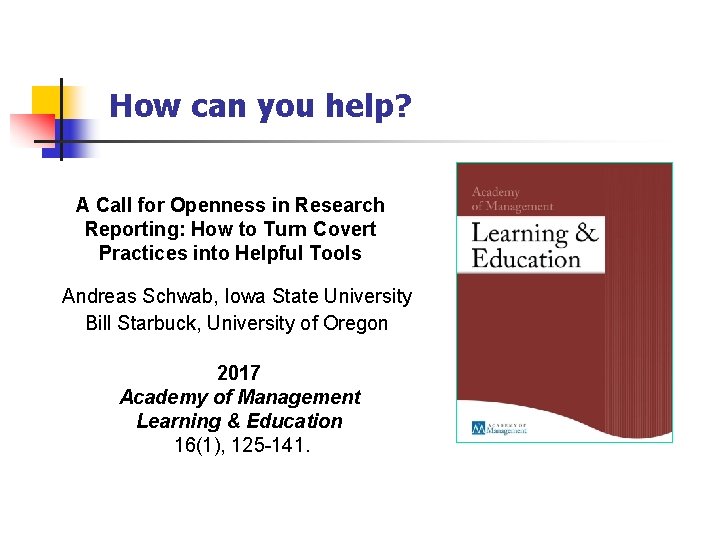
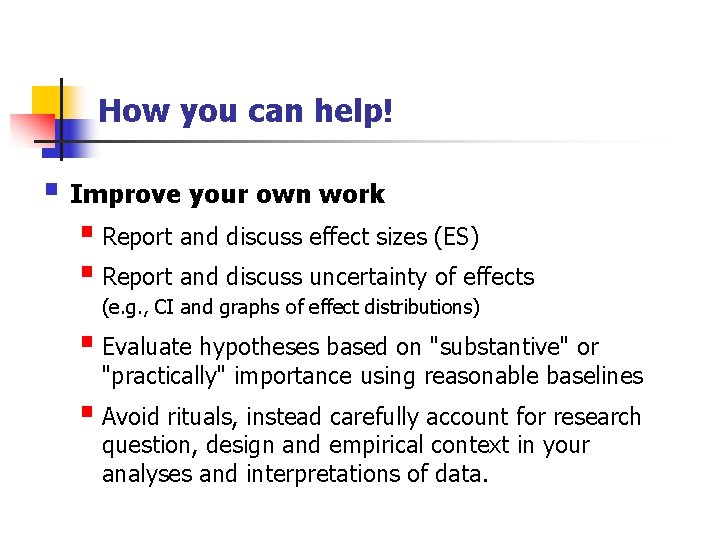
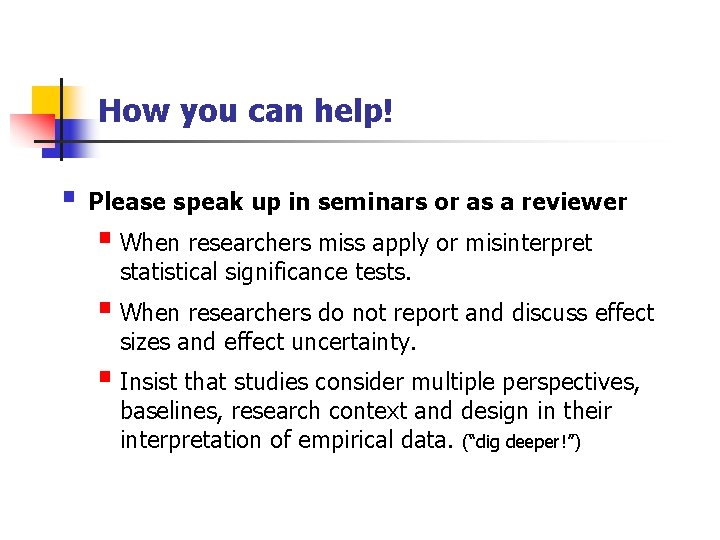
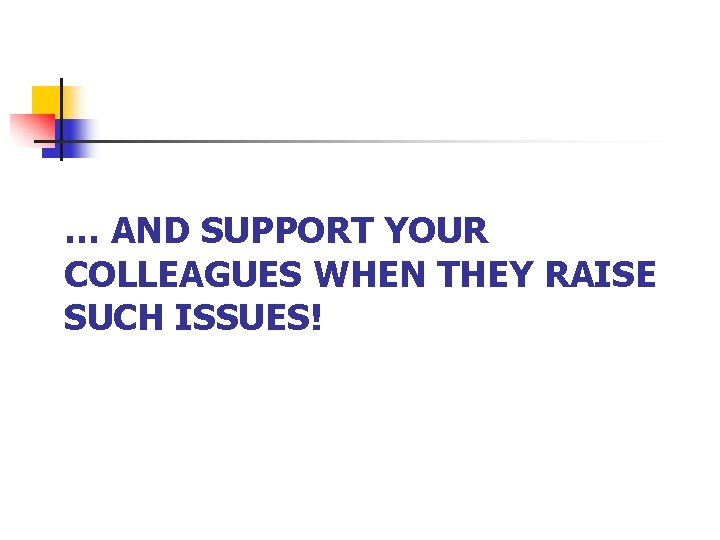
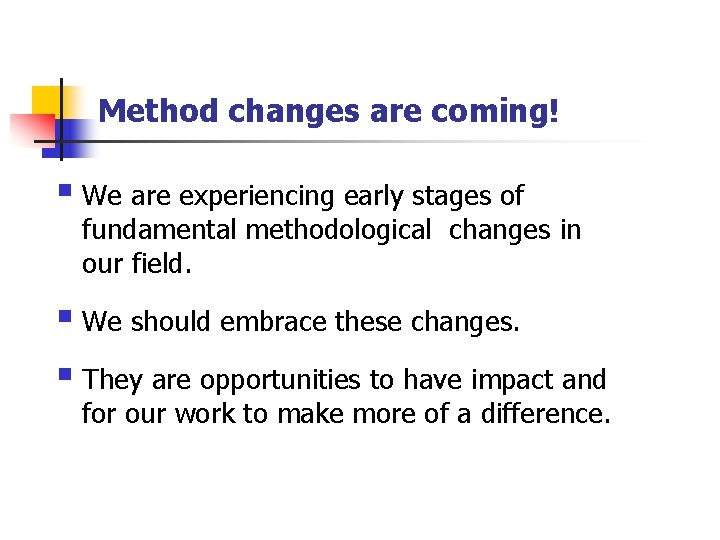
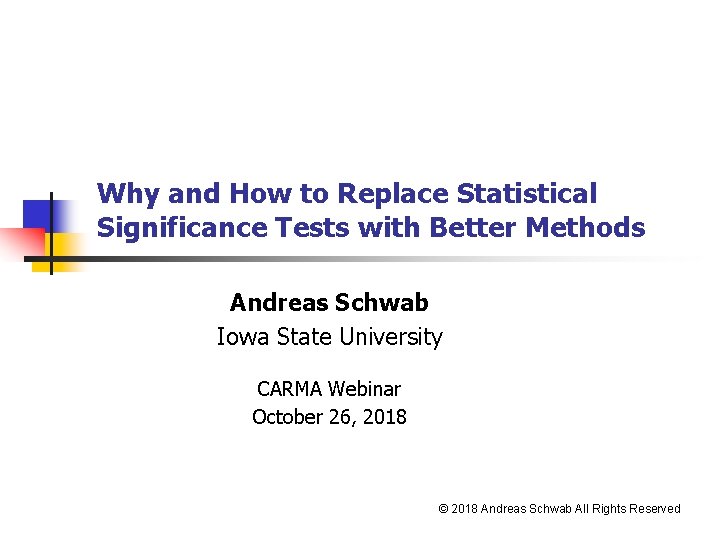
- Slides: 55
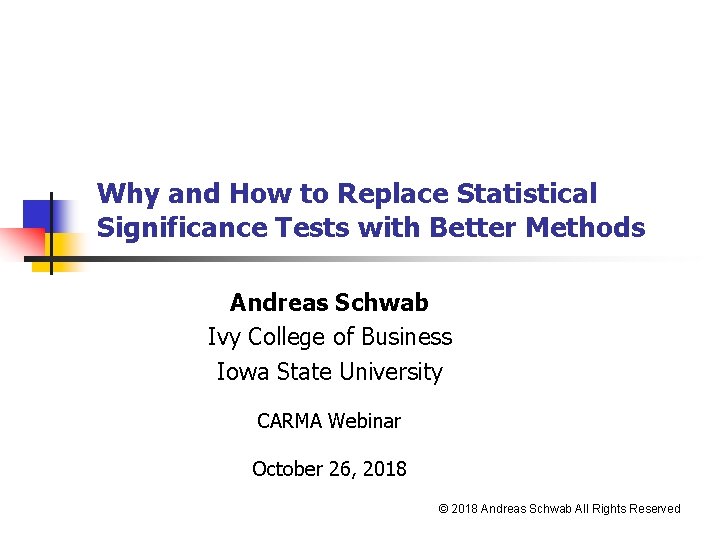
Why and How to Replace Statistical Significance Tests with Better Methods Andreas Schwab Ivy College of Business Iowa State University CARMA Webinar October 26, 2018 © 2018 Andreas Schwab All Rights Reserved
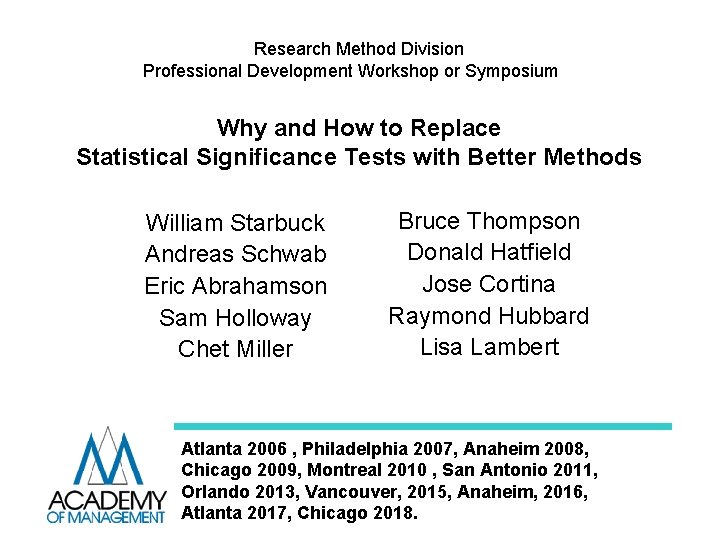
Research Method Division Professional Development Workshop or Symposium Why and How to Replace Statistical Significance Tests with Better Methods William Starbuck Andreas Schwab Eric Abrahamson Sam Holloway Chet Miller Bruce Thompson Donald Hatfield Jose Cortina Raymond Hubbard Lisa Lambert Atlanta 2006 , Philadelphia 2007, Anaheim 2008, Chicago 2009, Montreal 2010 , San Antonio 2011, Orlando 2013, Vancouver, 2015, Anaheim, 2016, Atlanta 2017, Chicago 2018.
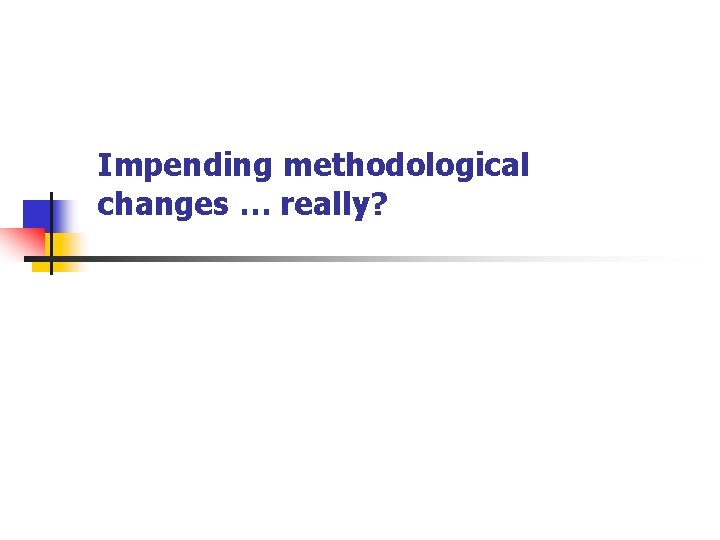
Impending methodological changes … really?
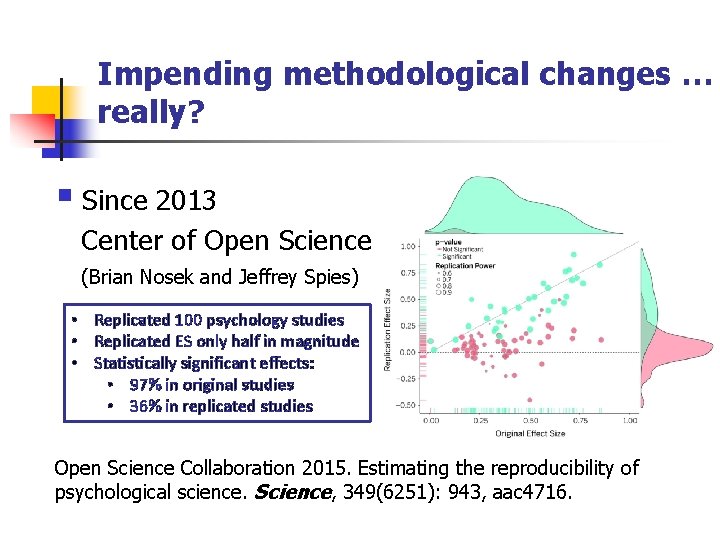
Impending methodological changes … really? § Since 2013 Center of Open Science (Brian Nosek and Jeffrey Spies) • Replicated 100 psychology studies • Replicated ES only half in magnitude • Statistically significant effects: • 97% in original studies • 36% in replicated studies Open Science Collaboration 2015. Estimating the reproducibility of psychological science. Science, 349(6251): 943, aac 4716.
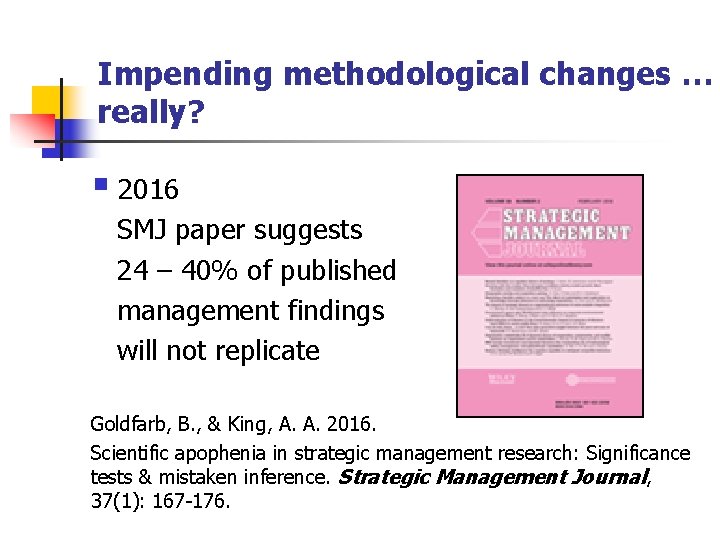
Impending methodological changes … really? § 2016 SMJ paper suggests 24 – 40% of published management findings will not replicate Goldfarb, B. , & King, A. A. 2016. Scientific apophenia in strategic management research: Significance tests & mistaken inference. Strategic Management Journal, 37(1): 167 -176.
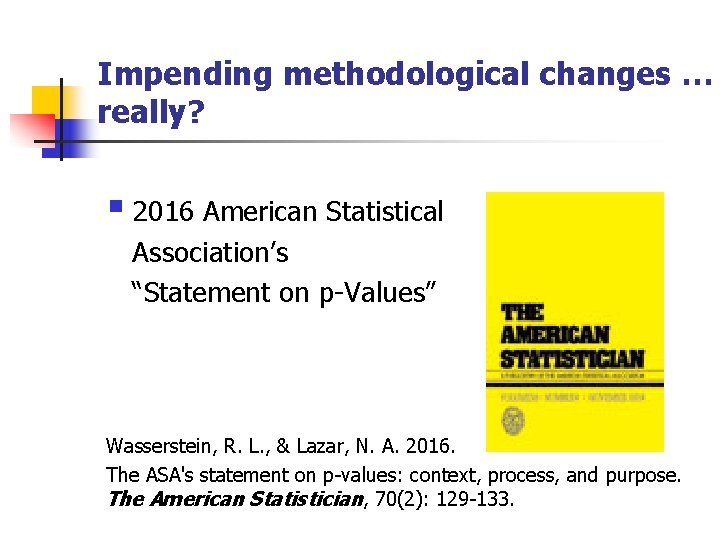
Impending methodological changes … really? § 2016 American Statistical Association’s “Statement on p-Values” Wasserstein, R. L. , & Lazar, N. A. 2016. The ASA's statement on p-values: context, process, and purpose. The American Statistician, 70(2): 129 -133.
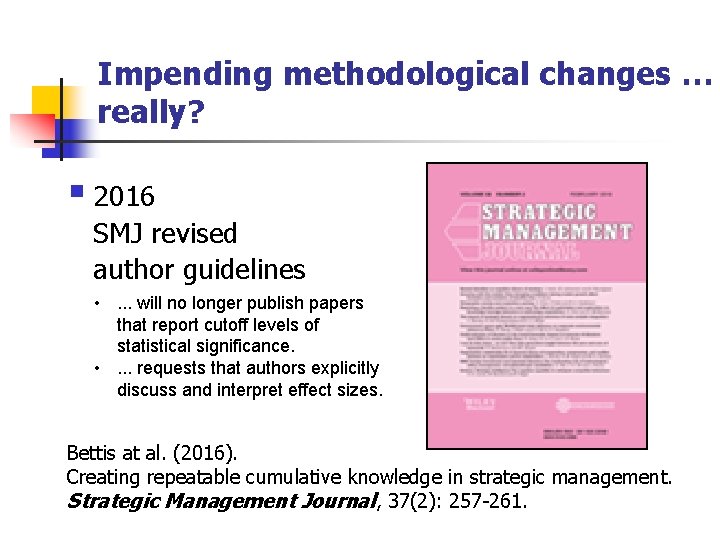
Impending methodological changes … really? § 2016 SMJ revised author guidelines • . . . will no longer publish papers that report cutoff levels of statistical significance. • . . . requests that authors explicitly discuss and interpret effect sizes. Bettis at al. (2016). Creating repeatable cumulative knowledge in strategic management. Strategic Management Journal, 37(2): 257 -261.
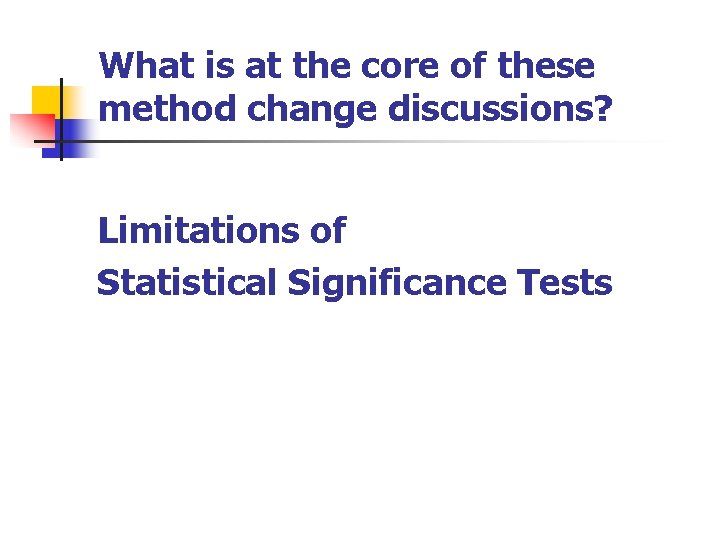
What is at the core of these method change discussions? Limitations of Statistical Significance Tests
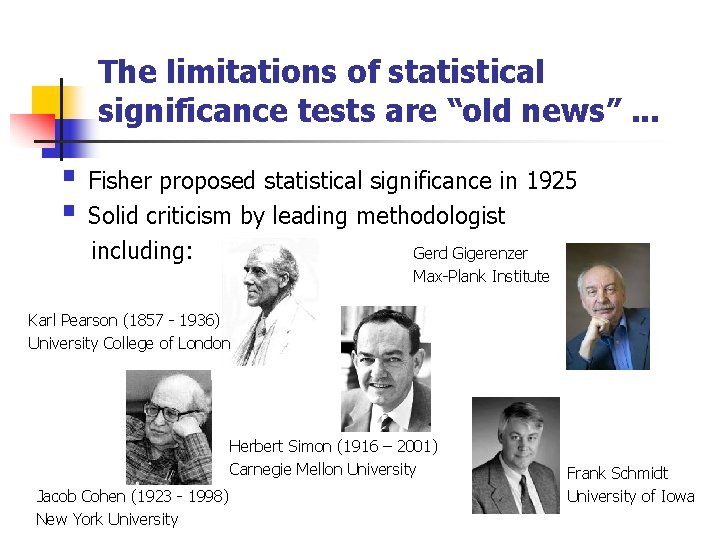
The limitations of statistical significance tests are “old news”. . . § Fisher proposed statistical significance in 1925 § Solid criticism by leading methodologist including: Gerd Gigerenzer Max-Plank Institute Karl Pearson (1857 - 1936) University College of London Herbert Simon (1916 – 2001) Carnegie Mellon University Jacob Cohen (1923 - 1998) New York University Frank Schmidt University of Iowa
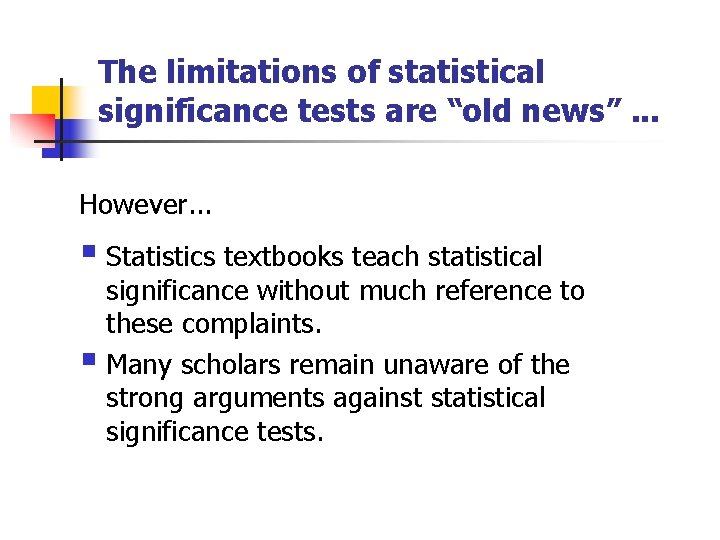
The limitations of statistical significance tests are “old news”. . . However. . . § Statistics textbooks teach statistical significance without much reference to these complaints. § Many scholars remain unaware of the strong arguments against statistical significance tests.
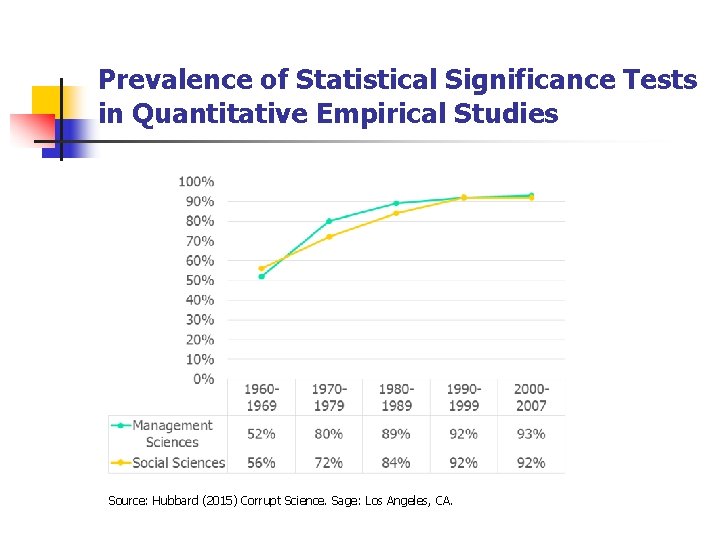
Prevalence of Statistical Significance Tests in Quantitative Empirical Studies Source: Hubbard (2015) Corrupt Science. Sage: Los Angeles, CA.
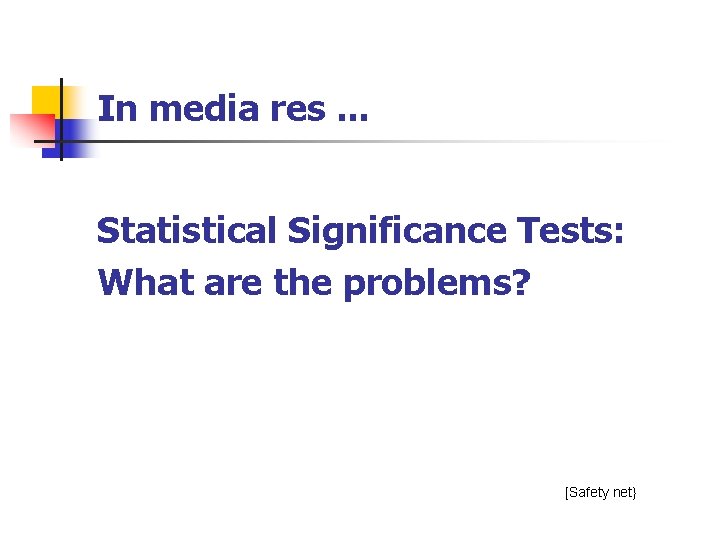
In media res. . . Statistical Significance Tests: What are the problems? [Safety net}
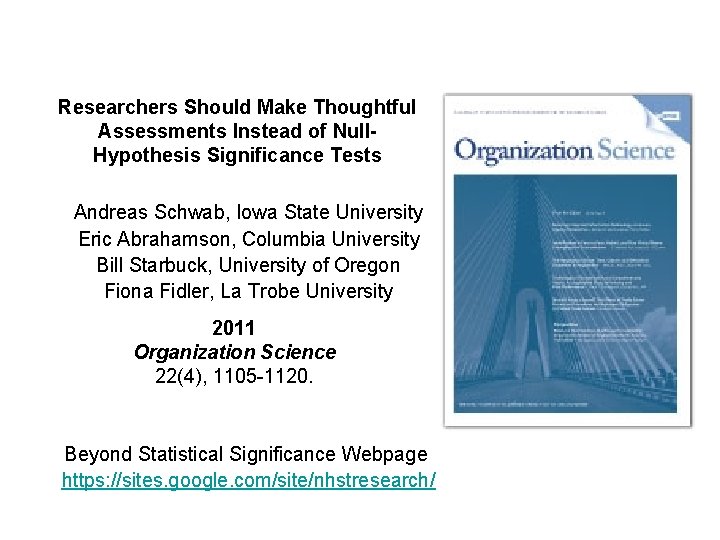
Researchers Should Make Thoughtful Assessments Instead of Null. Hypothesis Significance Tests Andreas Schwab, Iowa State University Eric Abrahamson, Columbia University Bill Starbuck, University of Oregon Fiona Fidler, La Trobe University 2011 Organization Science 22(4), 1105 -1120. Beyond Statistical Significance Webpage https: //sites. google. com/site/nhstresearch/
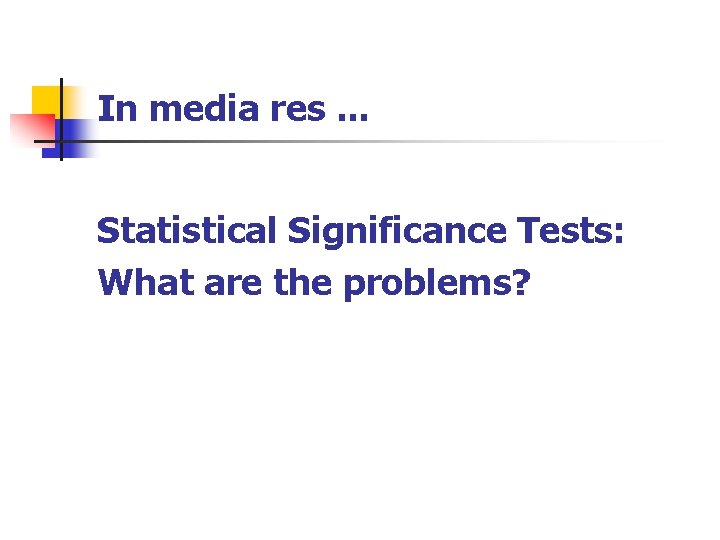
In media res. . . Statistical Significance Tests: What are the problems?
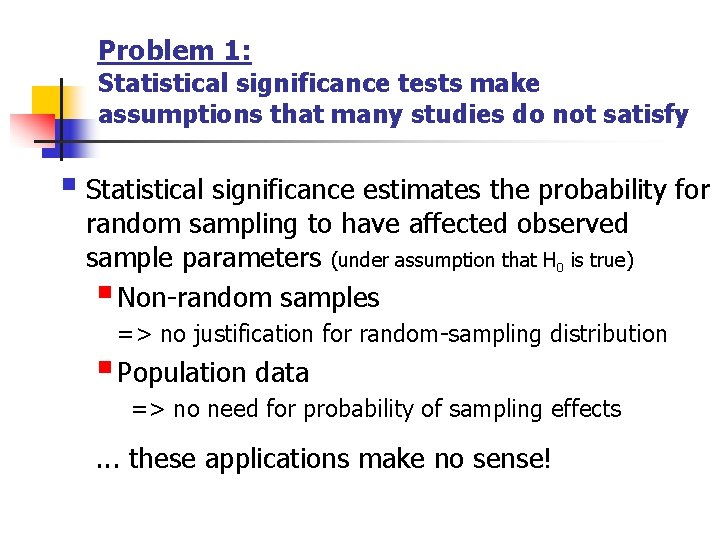
Problem 1: Statistical significance tests make assumptions that many studies do not satisfy § Statistical significance estimates the probability for random sampling to have affected observed sample parameters (under assumption that H 0 is true) § Non-random samples => no justification for random-sampling distribution § Population data => no need for probability of sampling effects . . . these applications make no sense!
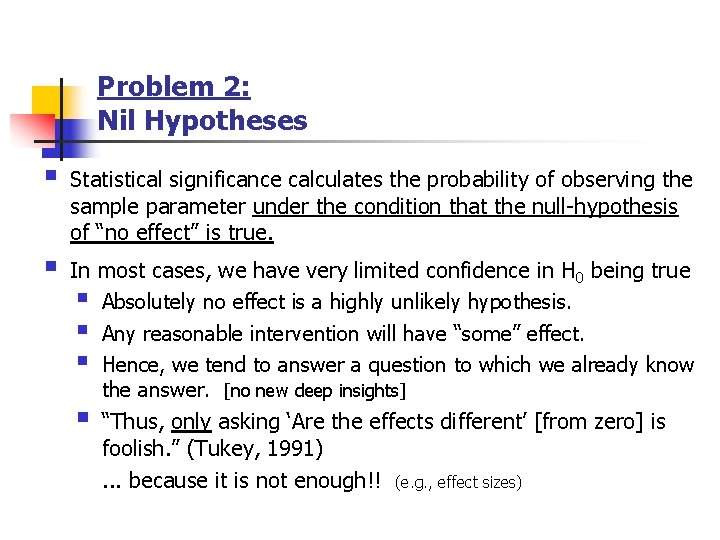
Problem 2: Nil Hypotheses § Statistical significance calculates the probability of observing the sample parameter under the condition that the null-hypothesis of “no effect” is true. § In most cases, we have very limited confidence in H 0 being true § Absolutely no effect is a highly unlikely hypothesis. § Any reasonable intervention will have “some” effect. § Hence, we tend to answer a question to which we already know the answer. [no new deep insights] § “Thus, only asking ‘Are the effects different’ [from zero] is foolish. ” (Tukey, 1991). . . because it is not enough!! (e. g. , effect sizes)
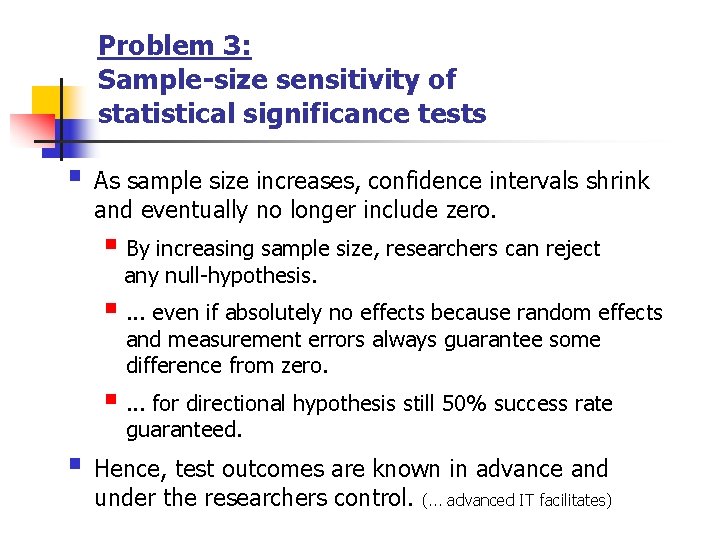
Problem 3: Sample-size sensitivity of statistical significance tests § As sample size increases, confidence intervals shrink and eventually no longer include zero. § By increasing sample size, researchers can reject any null-hypothesis. §. . . even if absolutely no effects because random effects and measurement errors always guarantee some difference from zero. §. . . for directional hypothesis still 50% success rate guaranteed. § Hence, test outcomes are known in advance and under the researchers control. (. . . advanced IT facilitates)
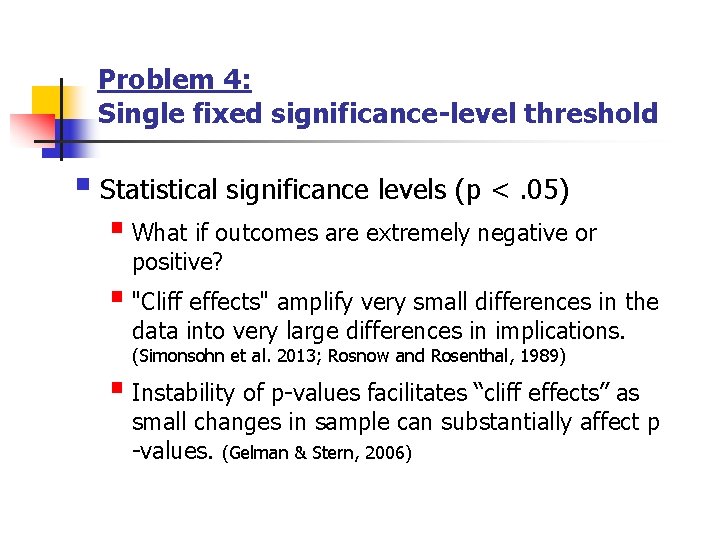
Problem 4: Single fixed significance-level threshold § Statistical significance levels (p <. 05) § What if outcomes are extremely negative or positive? § "Cliff effects" amplify very small differences in the data into very large differences in implications. (Simonsohn et al. 2013; Rosnow and Rosenthal, 1989) § Instability of p-values facilitates “cliff effects” as small changes in sample can substantially affect p -values. (Gelman & Stern, 2006)
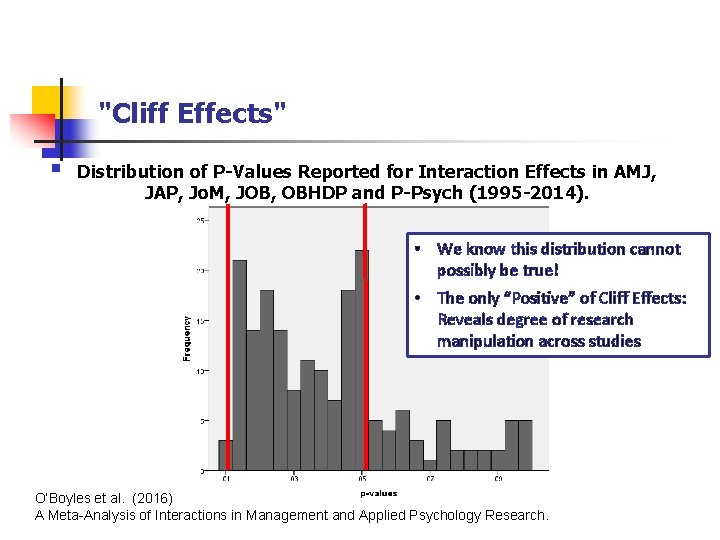
"Cliff Effects" § Distribution of P-Values Reported for Interaction Effects in AMJ, JAP, Jo. M, JOB, OBHDP and P-Psych (1995 -2014). • We know this distribution cannot possibly be true! • The only “Positive” of Cliff Effects: Reveals degree of research manipulation across studies O’Boyles et al. (2016) What Moderates Moderators? A Meta-Analysis of Interactions in Management and Applied Psychology Research.
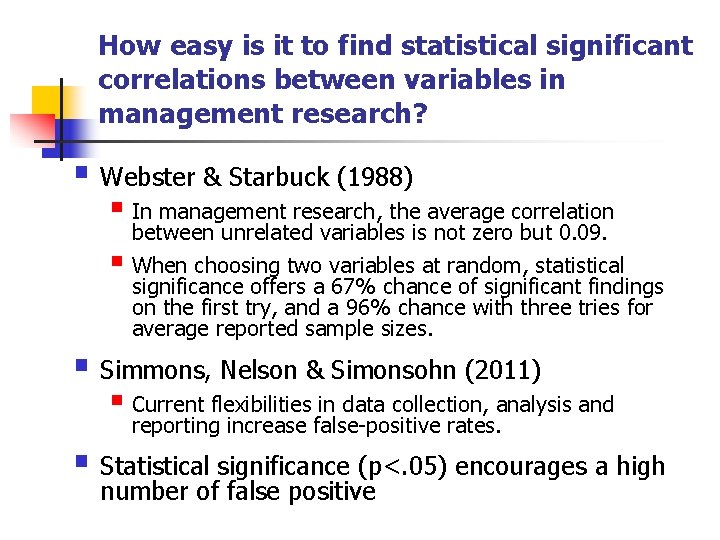
How easy is it to find statistical significant correlations between variables in management research? § Webster & Starbuck (1988) § In management research, the average correlation § between unrelated variables is not zero but 0. 09. When choosing two variables at random, statistical significance offers a 67% chance of significant findings on the first try, and a 96% chance with three tries for average reported sample sizes. § Simmons, Nelson & Simonsohn (2011) § Current flexibilities in data collection, analysis and reporting increase false-positive rates. § Statistical significance (p<. 05) encourages a high number of false positive
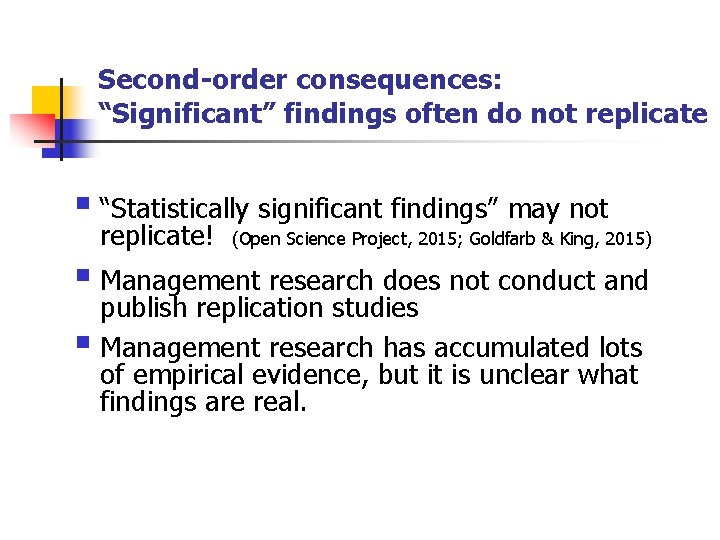
Second-order consequences: “Significant” findings often do not replicate § “Statistically significant findings” may not replicate! (Open Science Project, 2015; Goldfarb & King, 2015) § Management research does not conduct and publish replication studies § Management research has accumulated lots of empirical evidence, but it is unclear what findings are real.
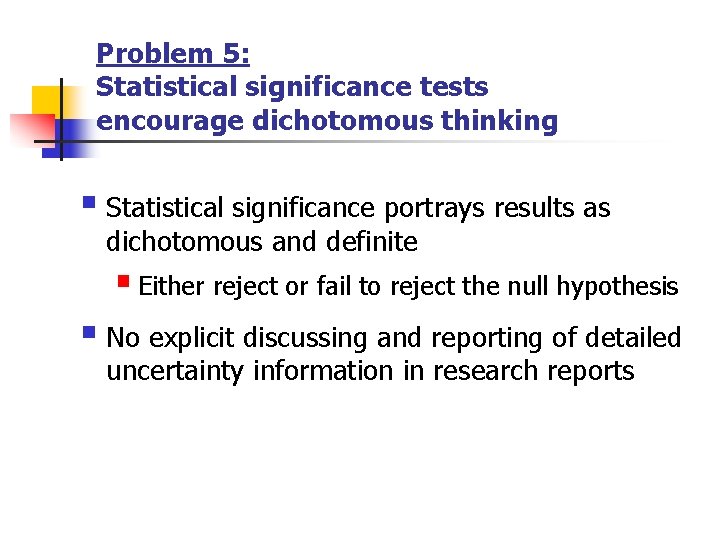
Problem 5: Statistical significance tests encourage dichotomous thinking § Statistical significance portrays results as dichotomous and definite § Either reject or fail to reject the null hypothesis § No explicit discussing and reporting of detailed uncertainty information in research reports
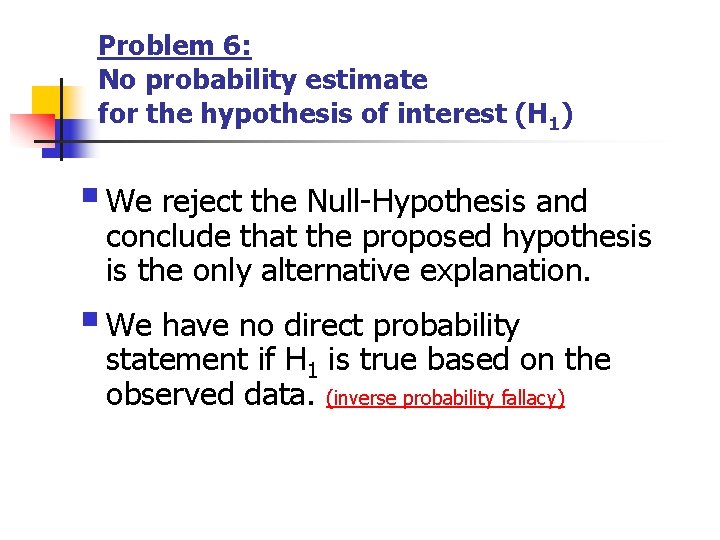
Problem 6: No probability estimate for the hypothesis of interest (H 1) § We reject the Null-Hypothesis and conclude that the proposed hypothesis is the only alternative explanation. § We have no direct probability statement if H 1 is true based on the observed data. (inverse probability fallacy)
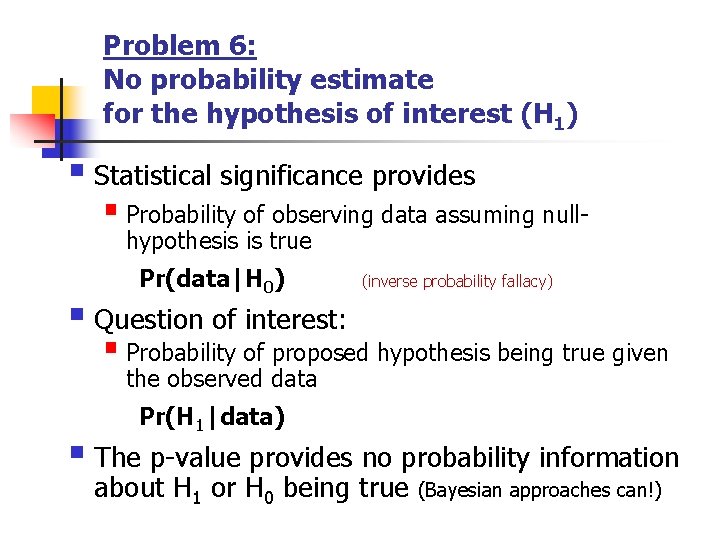
Problem 6: No probability estimate for the hypothesis of interest (H 1) § Statistical significance provides § Probability of observing data assuming nullhypothesis is true Pr(data|H 0) (inverse probability fallacy) § Question of interest: § Probability of proposed hypothesis being true given the observed data Pr(H 1|data) § The p-value provides no probability information about H 1 or H 0 being true (Bayesian approaches can!)
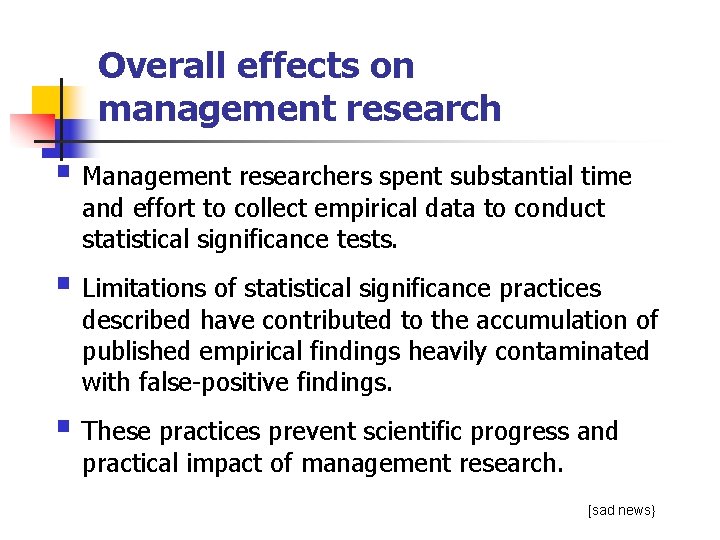
Overall effects on management research § Management researchers spent substantial time and effort to collect empirical data to conduct statistical significance tests. § Limitations of statistical significance practices described have contributed to the accumulation of published empirical findings heavily contaminated with false-positive findings. § These practices prevent scientific progress and practical impact of management research. [sad news}
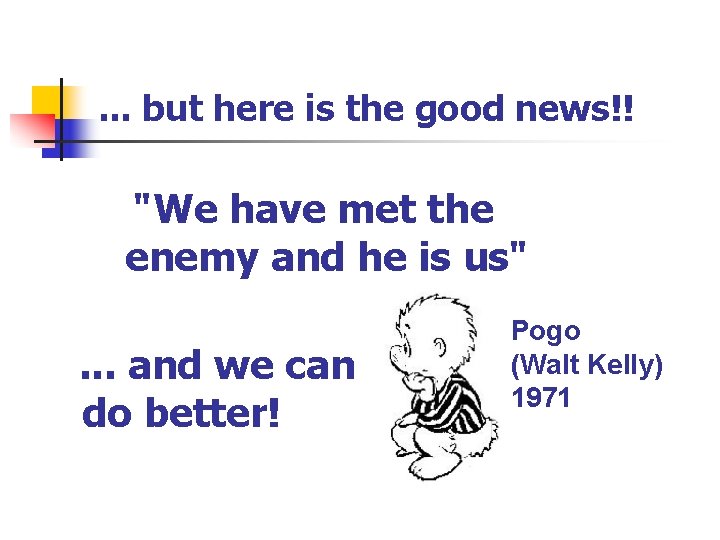
. . . but here is the good news!! "We have met the enemy and he is us". . . and we can do better! Pogo (Walt Kelly) 1971
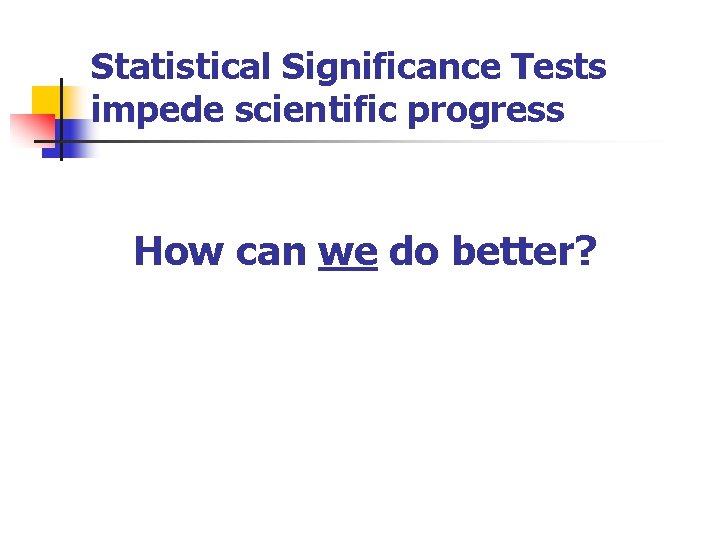
Statistical Significance Tests impede scientific progress How can we do better?
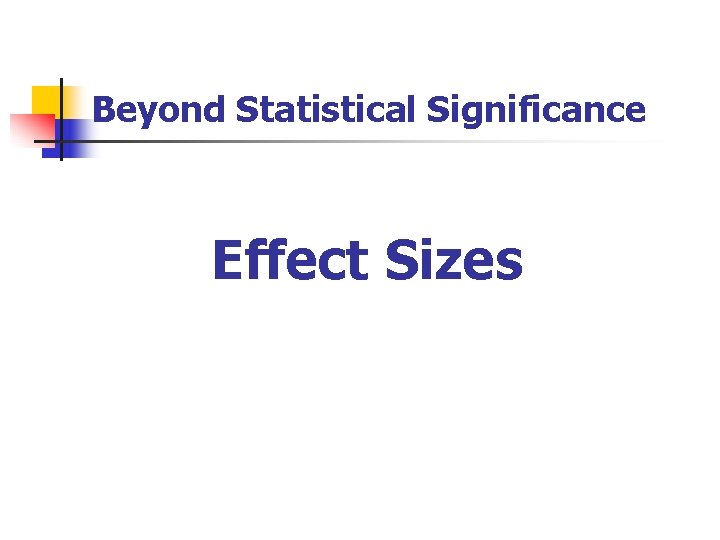
Beyond Statistical Significance Effect Sizes
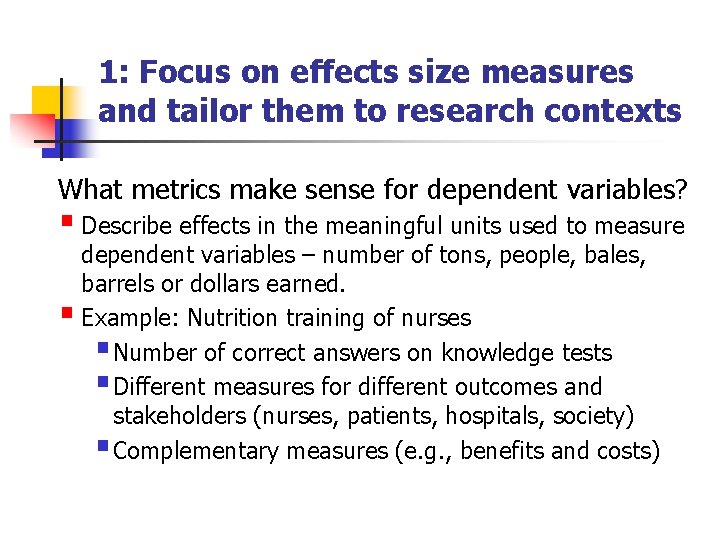
1: Focus on effects size measures and tailor them to research contexts What metrics make sense for dependent variables? § Describe effects in the meaningful units used to measure dependent variables – number of tons, people, bales, barrels or dollars earned. § Example: Nutrition training of nurses § Number of correct answers on knowledge tests § Different measures for different outcomes and stakeholders (nurses, patients, hospitals, society) § Complementary measures (e. g. , benefits and costs)
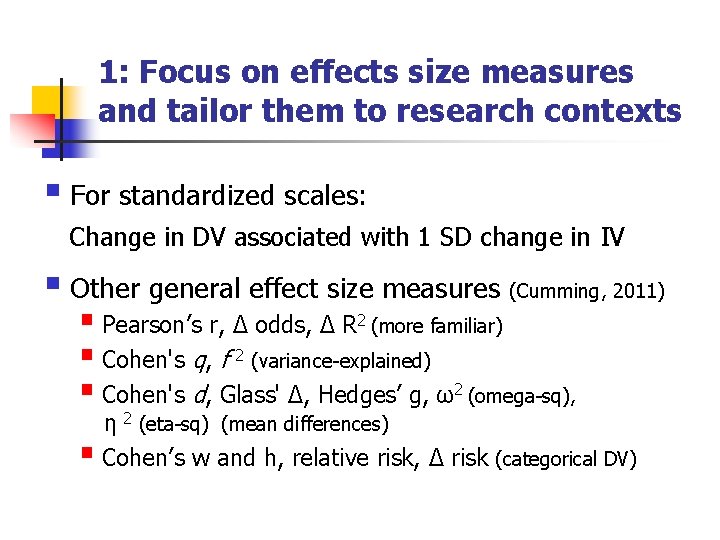
1: Focus on effects size measures and tailor them to research contexts § For standardized scales: Change in DV associated with 1 SD change in IV § Other general effect size measures (Cumming, 2011) § Pearson’s r, ∆ odds, ∆ R 2 (more familiar) § Cohen's q, f 2 (variance-explained) § Cohen's d, Glass' ∆, Hedges’ g, ω2 (omega-sq), η 2 (eta-sq) (mean differences) § Cohen’s w and h, relative risk, ∆ risk (categorical DV)
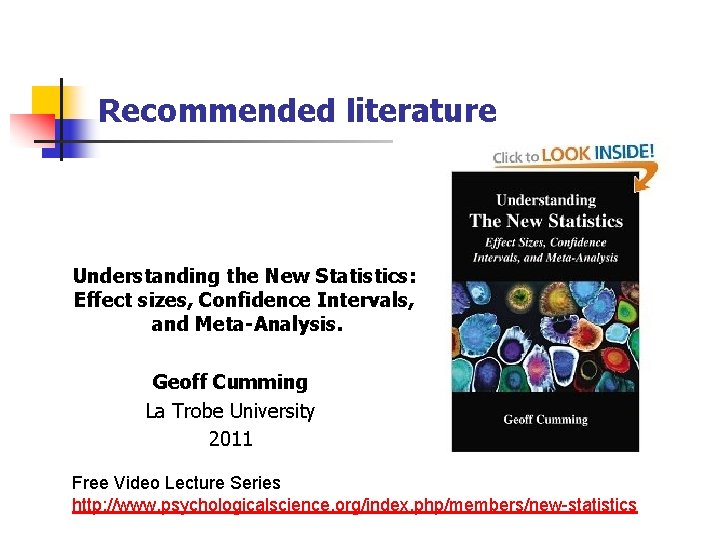
Recommended literature Understanding the New Statistics: Effect sizes, Confidence Intervals, and Meta-Analysis. Geoff Cumming La Trobe University 2011 Free Video Lecture Series http: //www. psychologicalscience. org/index. php/members/new-statistics
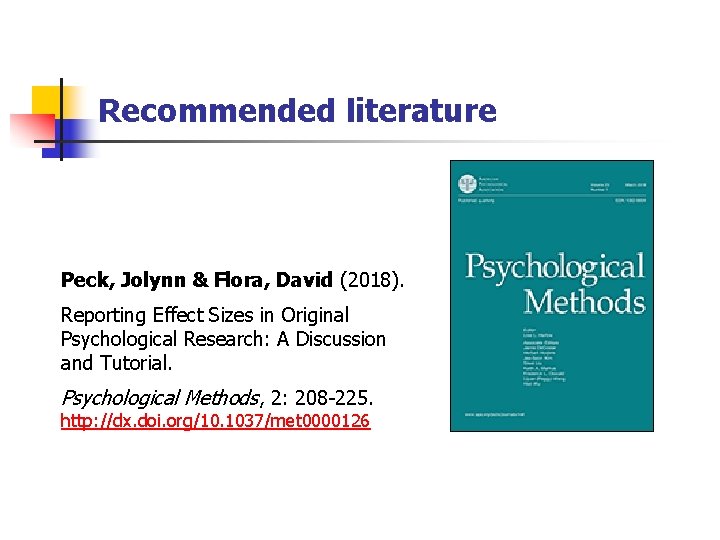
Recommended literature Peck, Jolynn & Flora, David (2018). Reporting Effect Sizes in Original Psychological Research: A Discussion and Tutorial. Psychological Methods, 2: 208 -225. http: //dx. doi. org/10. 1037/met 0000126
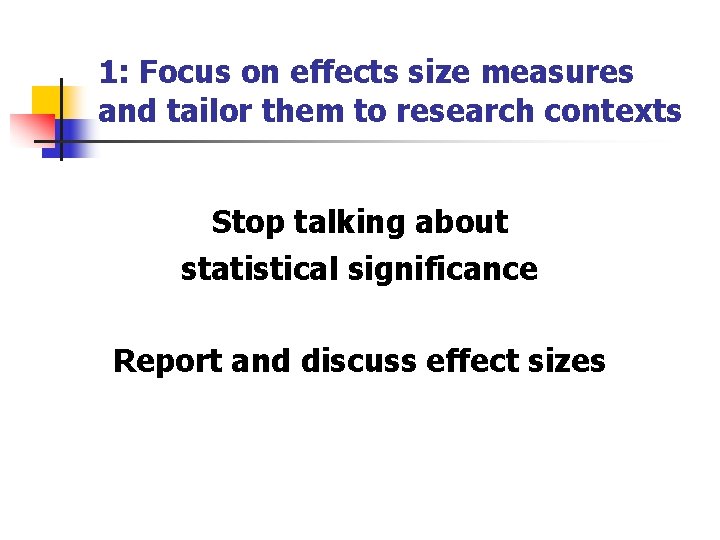
1: Focus on effects size measures and tailor them to research contexts Stop talking about statistical significance Report and discuss effect sizes
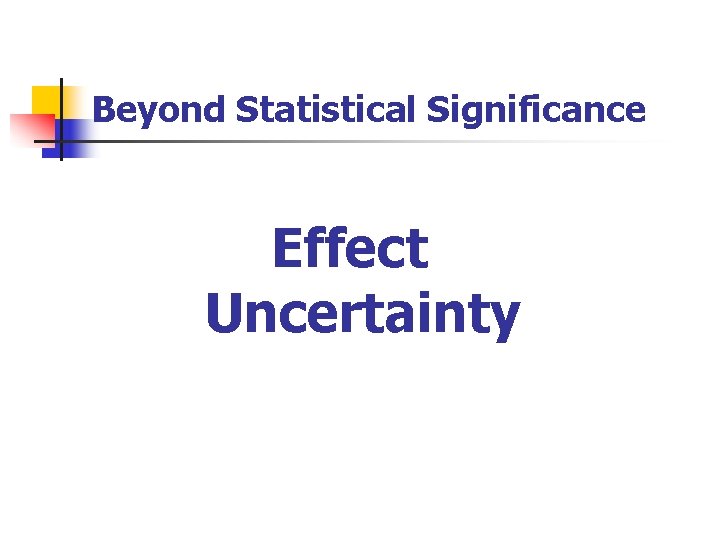
Beyond Statistical Significance Effect Uncertainty
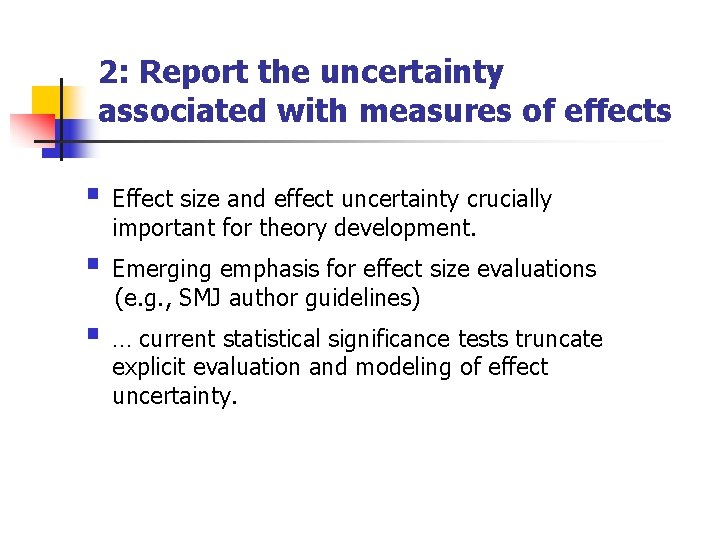
2: Report the uncertainty associated with measures of effects § Effect size and effect uncertainty crucially important for theory development. § Emerging emphasis for effect size evaluations (e. g. , SMJ author guidelines) § … current statistical significance tests truncate explicit evaluation and modeling of effect uncertainty.
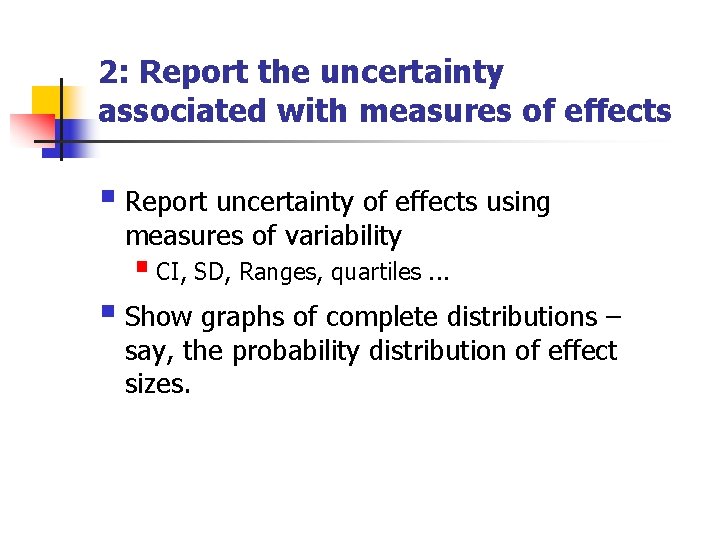
2: Report the uncertainty associated with measures of effects § Report uncertainty of effects using measures of variability § CI, SD, Ranges, quartiles. . . § Show graphs of complete distributions – say, the probability distribution of effect sizes.
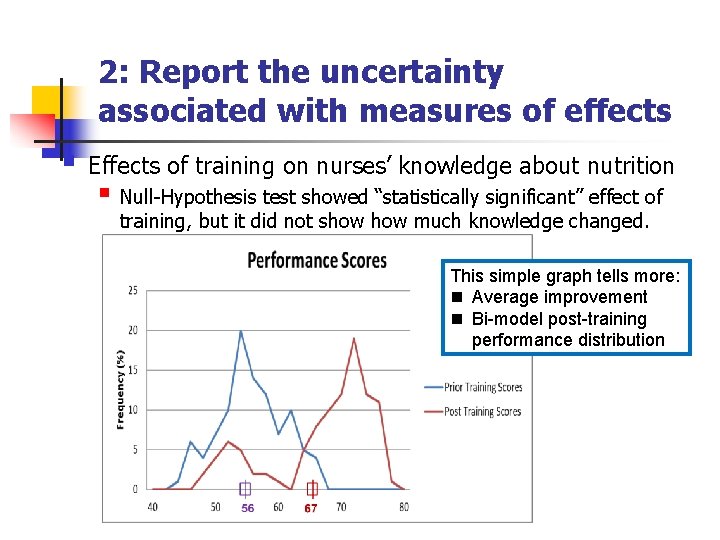
2: Report the uncertainty associated with measures of effects § Effects of training on nurses’ knowledge about nutrition § Null-Hypothesis test showed “statistically significant” effect of training, but it did not show much knowledge changed. This simple graph tells more: n Average improvement n Bi-model post-training performance distribution
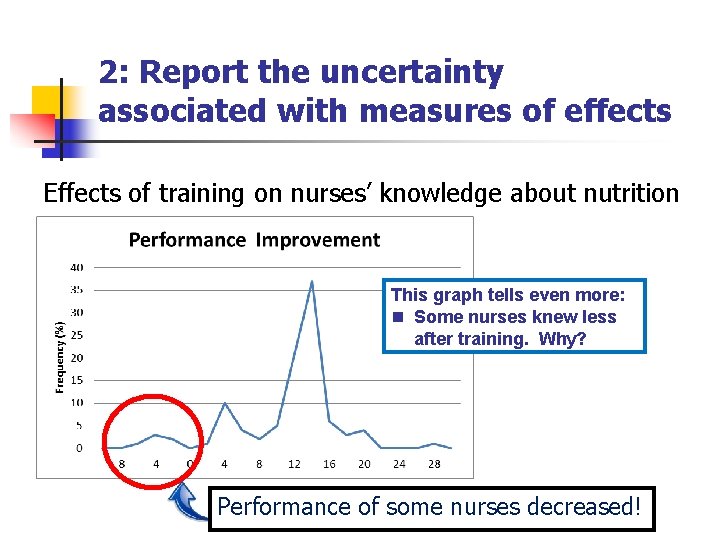
2: Report the uncertainty associated with measures of effects Effects of training on nurses’ knowledge about nutrition This graph tells even more: n Some nurses knew less after training. Why? Performance of some nurses decreased!
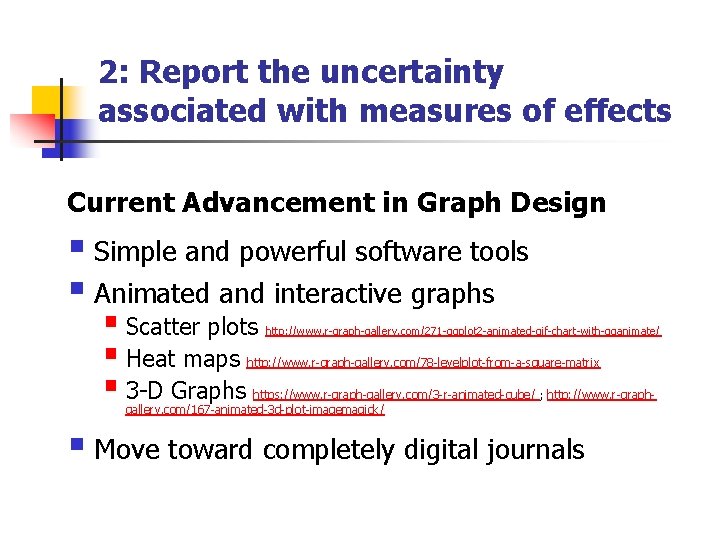
2: Report the uncertainty associated with measures of effects Current Advancement in Graph Design § Simple and powerful software tools § Animated and interactive graphs § Scatter plots § Heat maps § 3 -D Graphs http: //www. r-graph-gallery. com/271 -ggplot 2 -animated-gif-chart-with-gganimate/ http: //www. r-graph-gallery. com/78 -levelplot-from-a-square-matrix https: //www. r-graph-gallery. com/3 -r-animated-cube/ ; http: //www. r-graphgallery. com/167 -animated-3 d-plot-imagemagick/ § Move toward completely digital journals
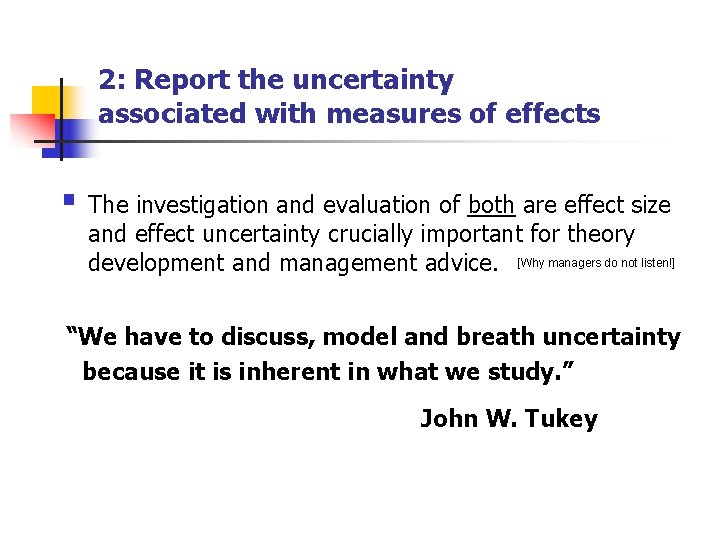
2: Report the uncertainty associated with measures of effects § The investigation and evaluation of both are effect size and effect uncertainty crucially important for theory development and management advice. [Why managers do not listen!] “We have to discuss, model and breath uncertainty because it is inherent in what we study. ” John W. Tukey
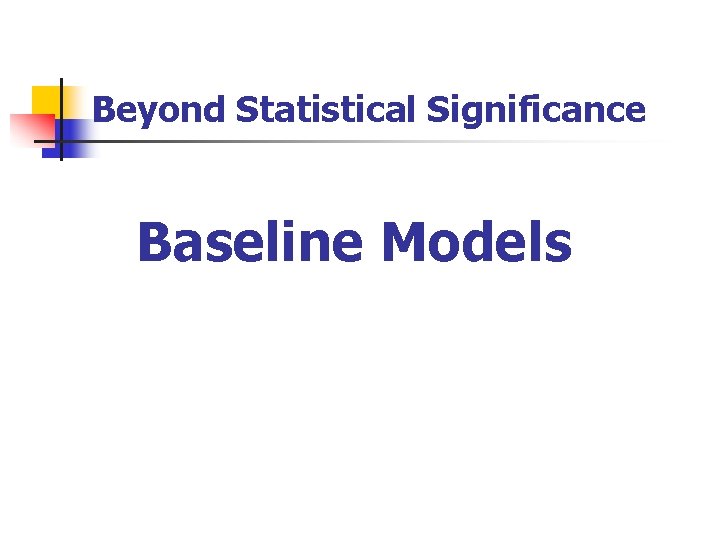
Beyond Statistical Significance Baseline Models
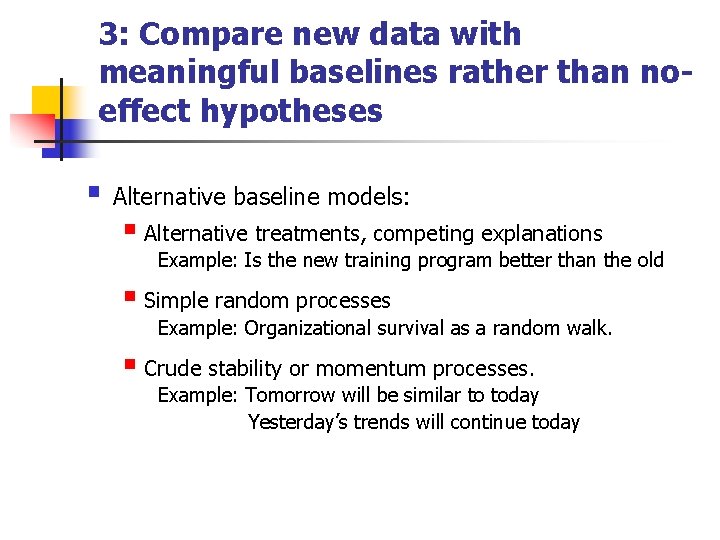
3: Compare new data with meaningful baselines rather than noeffect hypotheses § Alternative baseline models: § Alternative treatments, competing explanations Example: Is the new training program better than the old § Simple random processes Example: Organizational survival as a random walk. § Crude stability or momentum processes. Example: Tomorrow will be similar to today Yesterday’s trends will continue today
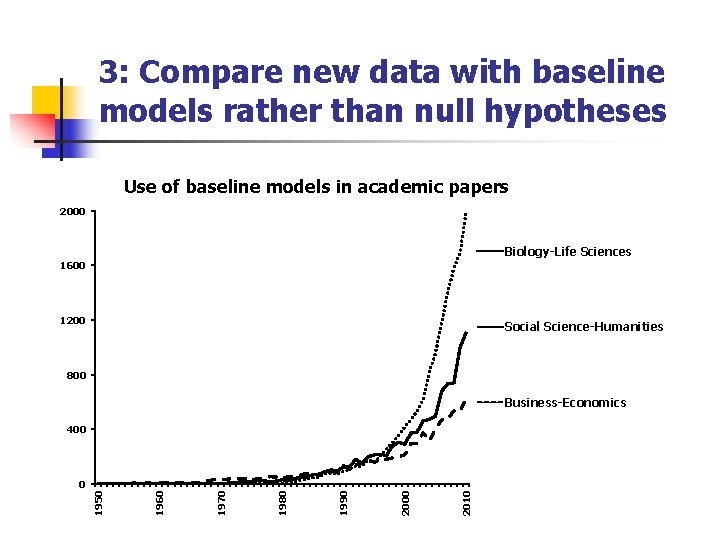
3: Compare new data with baseline models rather than null hypotheses Use of baseline models in academic papers 2000 Biology-Life Sciences 1600 1200 Social Science-Humanities 800 Business-Economics 400 2010 2000 1990 1980 1970 1960 1950 0
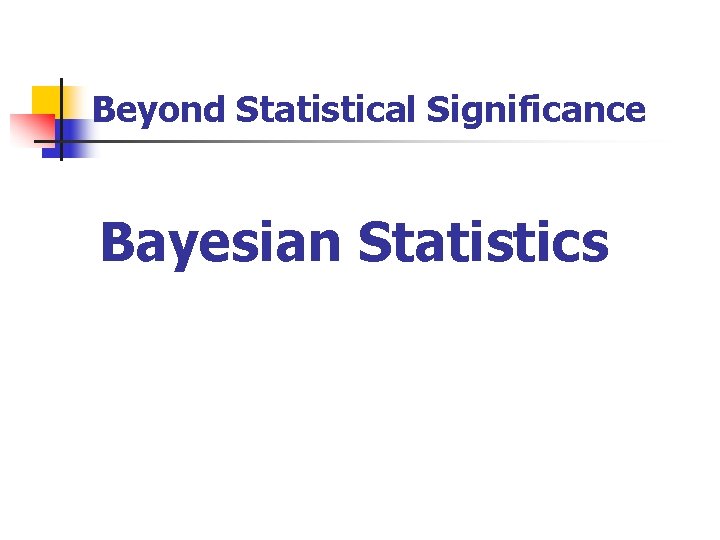
Beyond Statistical Significance Bayesian Statistics
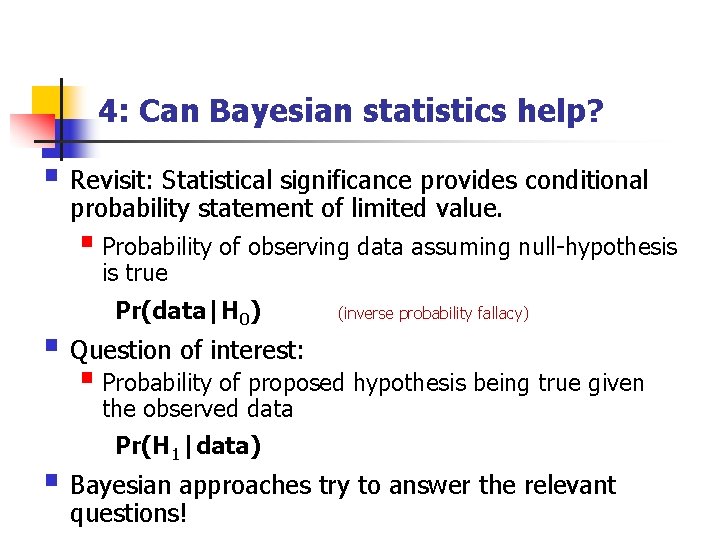
4: Can Bayesian statistics help? § Revisit: Statistical significance provides conditional probability statement of limited value. § Probability of observing data assuming null-hypothesis is true Pr(data|H 0) (inverse probability fallacy) § Question of interest: § Probability of proposed hypothesis being true given the observed data Pr(H 1|data) § Bayesian approaches try to answer the relevant questions!
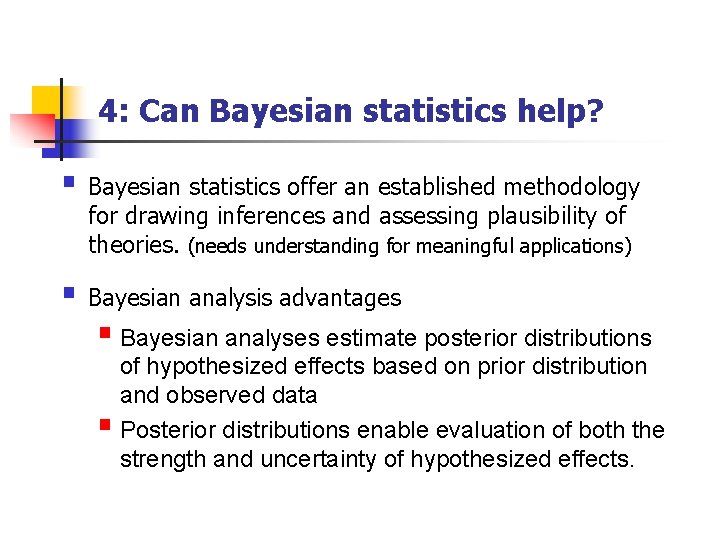
4: Can Bayesian statistics help? § Bayesian statistics offer an established methodology for drawing inferences and assessing plausibility of theories. (needs understanding for meaningful applications) § Bayesian analysis advantages § Bayesian analyses estimate posterior distributions of hypothesized effects based on prior distribution and observed data § Posterior distributions enable evaluation of both the strength and uncertainty of hypothesized effects.
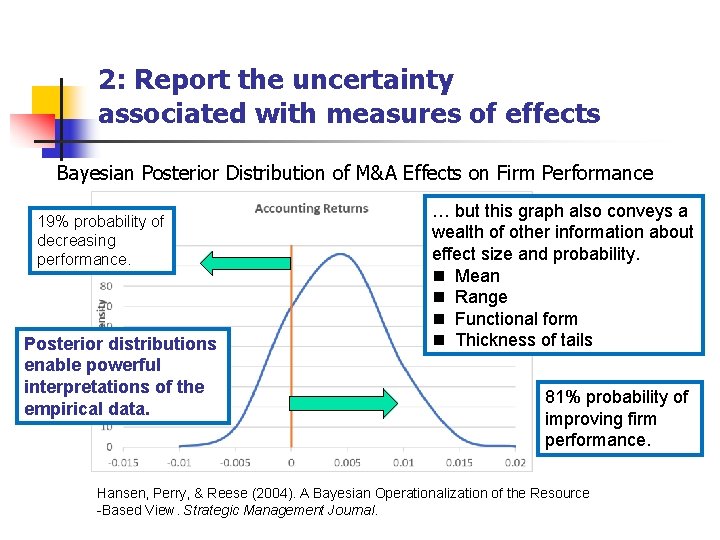
2: Report the uncertainty associated with measures of effects Bayesian Posterior Distribution of M&A Effects on Firm Performance 19% probability of decreasing performance. Posterior distributions enable powerful interpretations of the empirical data. … but this graph also conveys a wealth of other information about effect size and probability. n Mean n Range n Functional form n Thickness of tails 81% probability of improving firm performance. Hansen, Perry, & Reese (2004). A Bayesian Operationalization of the Resource -Based View. Strategic Management Journal.
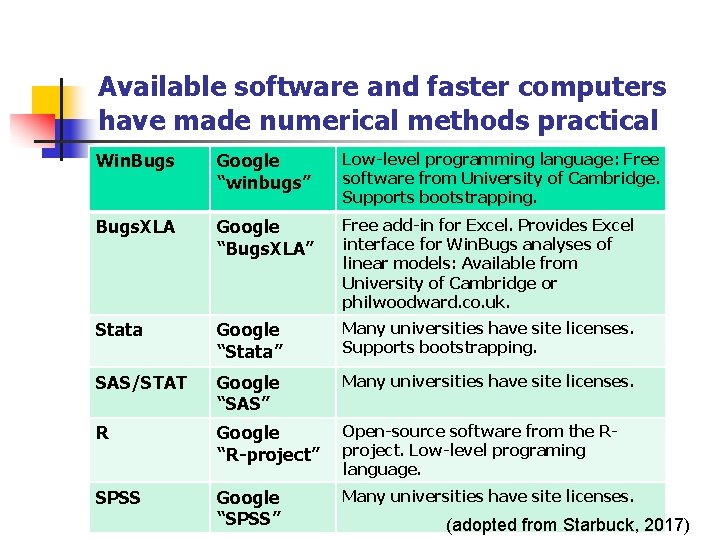
Available software and faster computers have made numerical methods practical Win. Bugs Google “winbugs” Low-level programming language: Free software from University of Cambridge. Supports bootstrapping. Bugs. XLA Google “Bugs. XLA” Free add-in for Excel. Provides Excel interface for Win. Bugs analyses of linear models: Available from University of Cambridge or philwoodward. co. uk. Stata Google “Stata” Many universities have site licenses. Supports bootstrapping. SAS/STAT Google “SAS” Many universities have site licenses. R Google “R-project” Open-source software from the Rproject. Low-level programing language. SPSS Google “SPSS” Many universities have site licenses. (adopted from Starbuck, 2017)
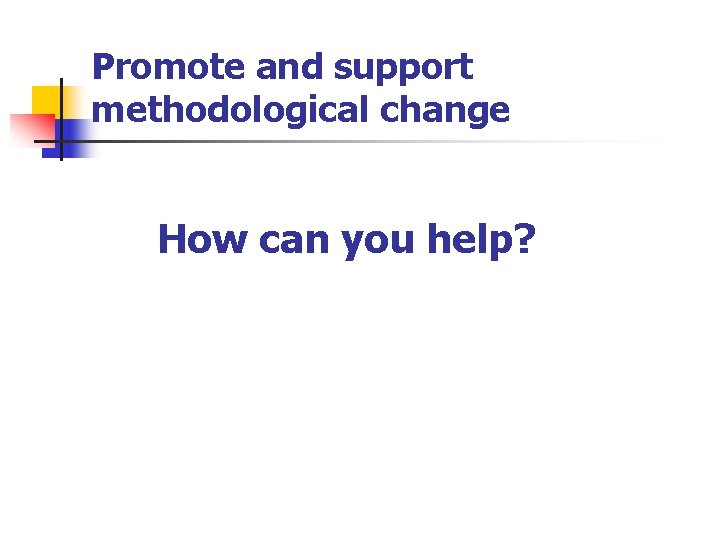
Promote and support methodological change How can you help?
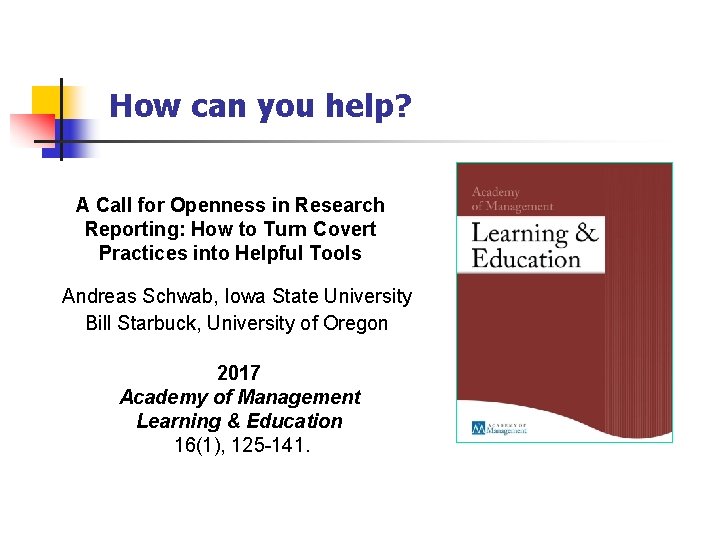
How can you help? A Call for Openness in Research Reporting: How to Turn Covert Practices into Helpful Tools Andreas Schwab, Iowa State University Bill Starbuck, University of Oregon 2017 Academy of Management Learning & Education 16(1), 125 -141.
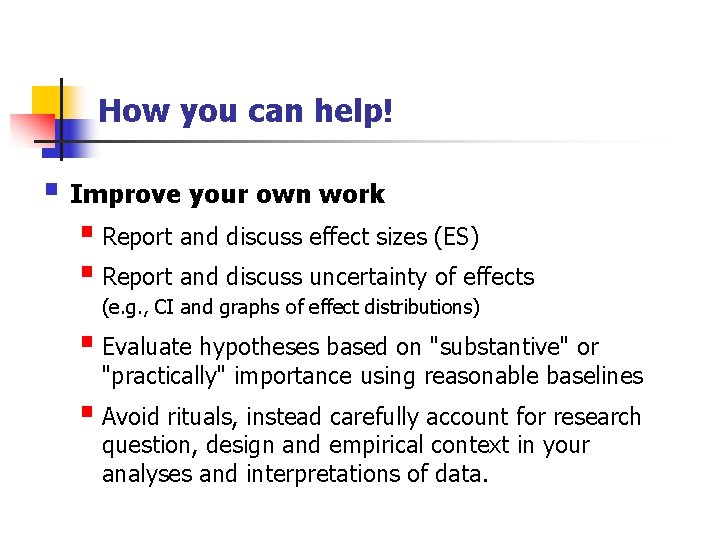
How you can help! § Improve your own work § Report and discuss effect sizes (ES) § Report and discuss uncertainty of effects (e. g. , CI and graphs of effect distributions) § Evaluate hypotheses based on "substantive" or "practically" importance using reasonable baselines § Avoid rituals, instead carefully account for research question, design and empirical context in your analyses and interpretations of data.
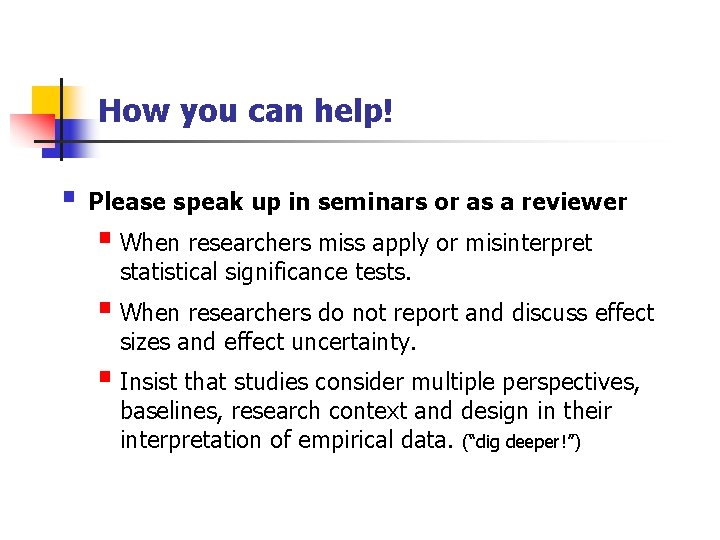
How you can help! § Please speak up in seminars or as a reviewer § When researchers miss apply or misinterpret statistical significance tests. § When researchers do not report and discuss effect sizes and effect uncertainty. § Insist that studies consider multiple perspectives, baselines, research context and design in their interpretation of empirical data. (“dig deeper!”)
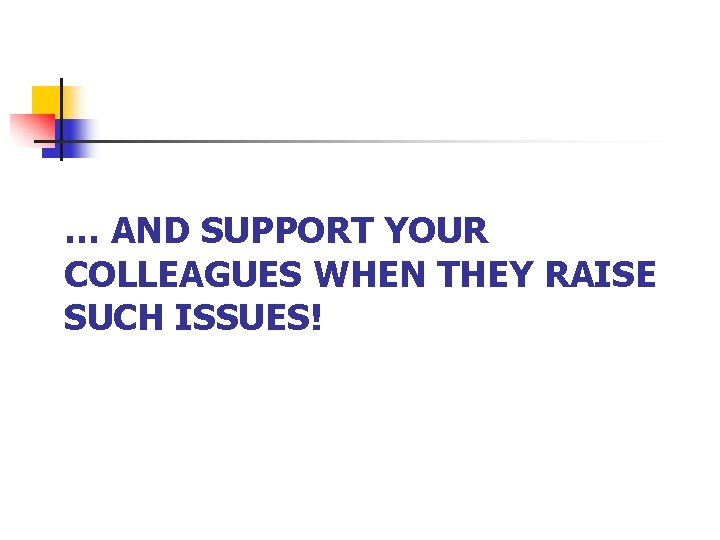
… AND SUPPORT YOUR COLLEAGUES WHEN THEY RAISE SUCH ISSUES!
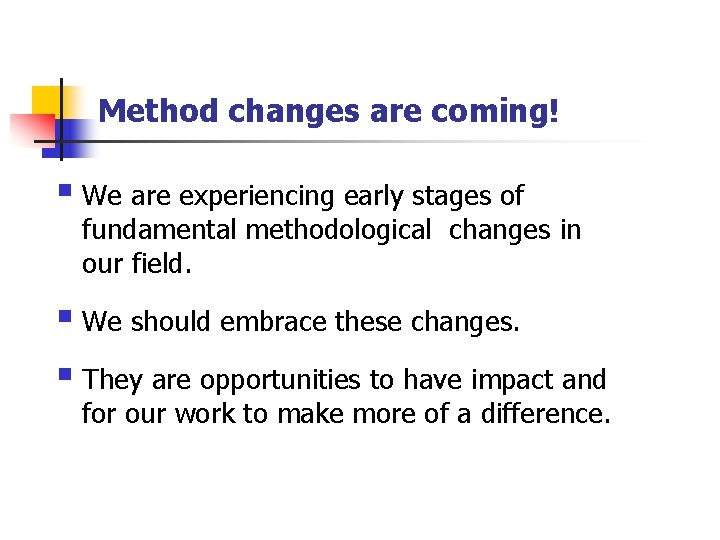
Method changes are coming! § We are experiencing early stages of fundamental methodological changes in our field. § We should embrace these changes. § They are opportunities to have impact and for our work to make more of a difference.
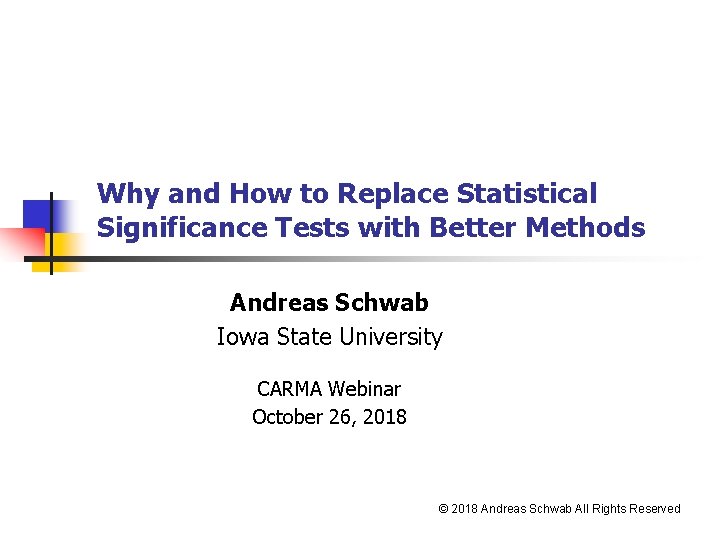
Why and How to Replace Statistical Significance Tests with Better Methods Andreas Schwab Iowa State University CARMA Webinar October 26, 2018 © 2018 Andreas Schwab All Rights Reserved
Iq intelligence
Hey hey bye bye
Univariate analysis tests
T test ordinal data
Tutor webinar
Yyxyxx
Statistical test table psychology
Types of significance tests
Statistical significance p value
Retire statistical significance
A b testing 101
Dont ask why why why
Gedit keyboard shortcuts
Replace crda with core/wireless-regdb
Theme of who can replace a man
Cold fusion 9
Replace recursion with iteration
Ca datacom
Please replace
A metaphorical phrase used to replace a concrete noun
A metaphorical phrase used to replace a concrete noun
Create or replace procedure add_dept
Can technology replace social engineering
Create or replace procedure add_dept
Ruc 1
Pl/sql assignment
L
Multiplication sign symbol
Replace consultoria
Mensa candidate
Geometry boot camp answers
Nêu sự khác biệt giữa lệnh find và replace
Bicarbonate deficit replacement
Manage 3. hali
Shrek ordinary world
Parametric and non parametric test
City and guilds open assess
Chapter 24 diagnostic tests and specimen collection
Chapter 20 more about tests and intervals
Act 1 test romeo and juliet
Test of details
Family and friends 2 tests
Gl progress test sample papers
Biochemical data, medical tests, and procedures (bd)
Physical fitness grade 9
Projective test advantages and disadvantages
Advantage of focus groups
Family and friends tests
Chapter 23 specimen collection and diagnostic testing
List and describe 3 tests of cerebellar function
Leeds pathology tests and tubes
Statistical symbols and meanings
Chebyshev's inequality
Probability and statistical inference 9th solution pdf
6 sigma percentile
Distinguish between synchronous and statistical tdm.