LMU talks 3 Statistical significance Statistical significance has
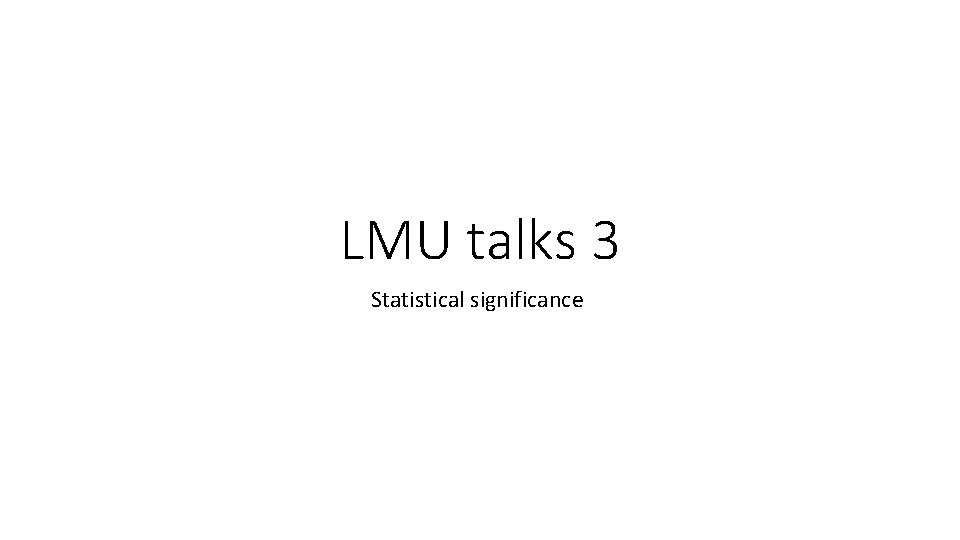
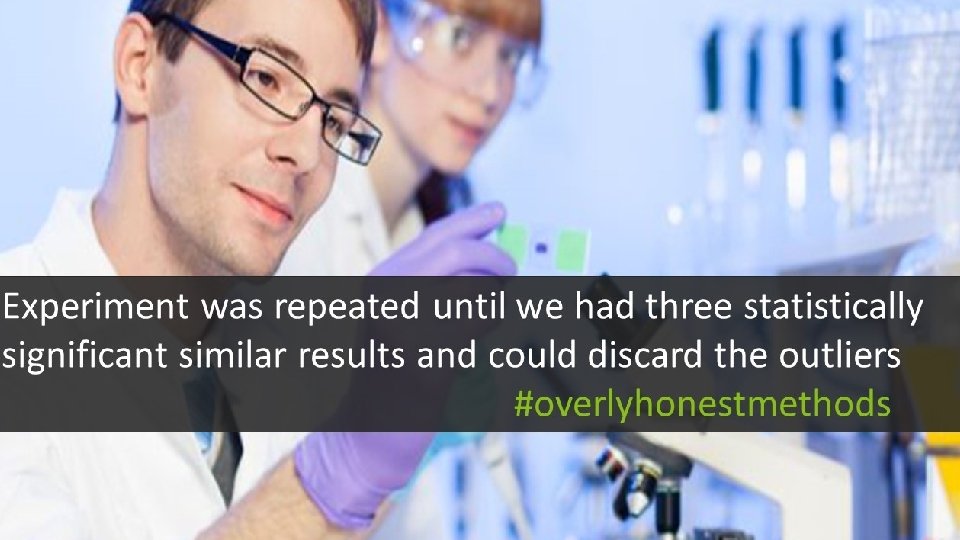
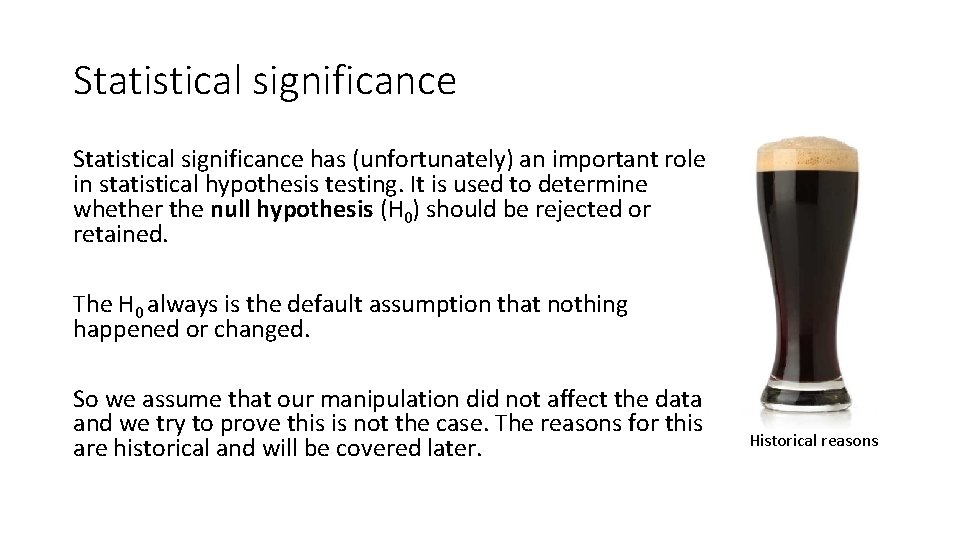
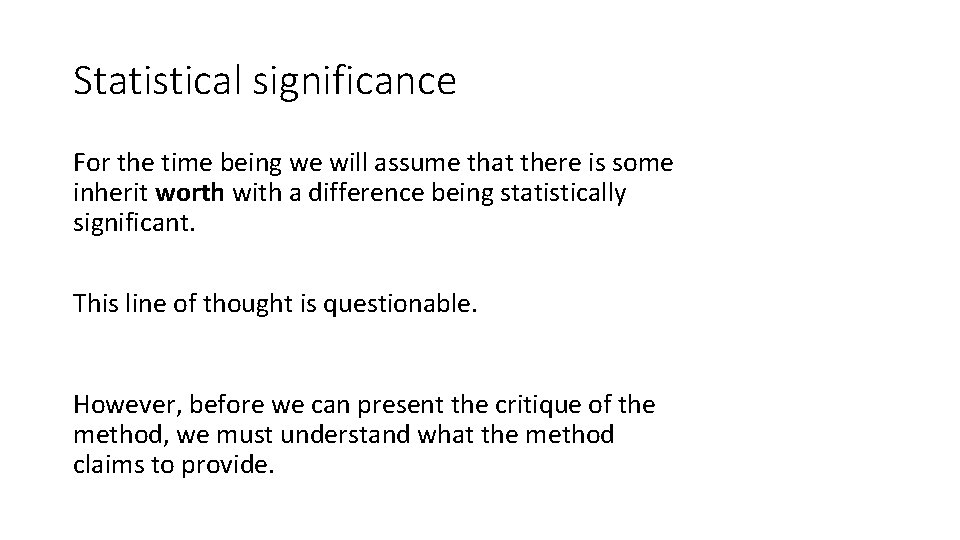
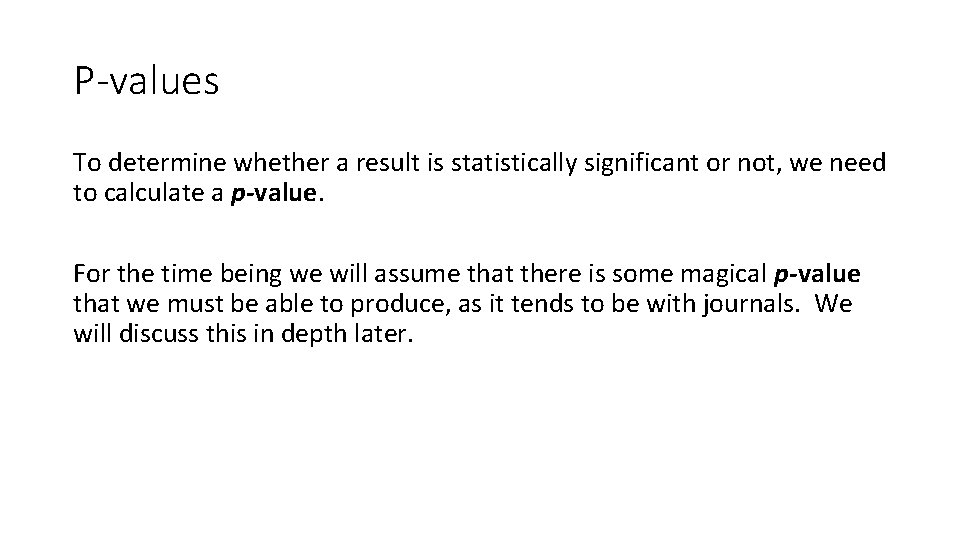
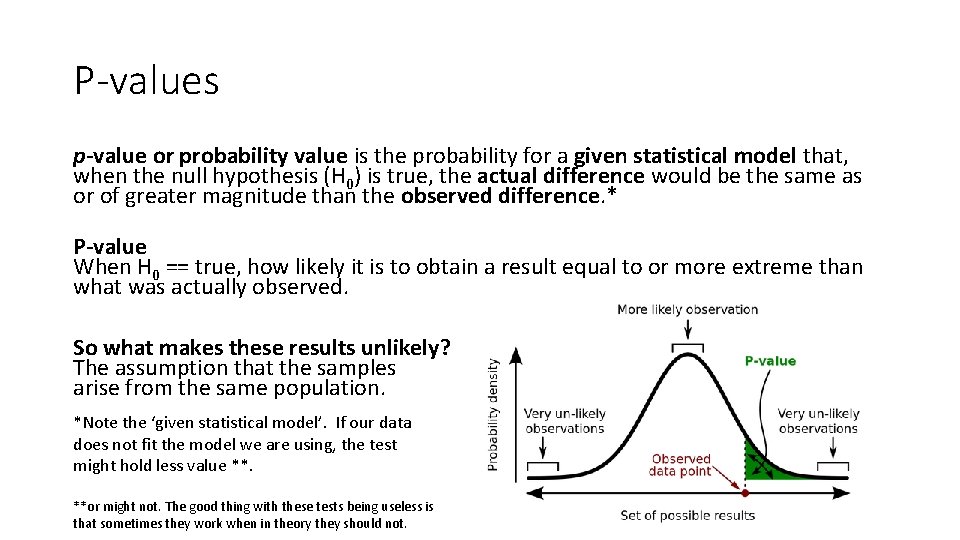
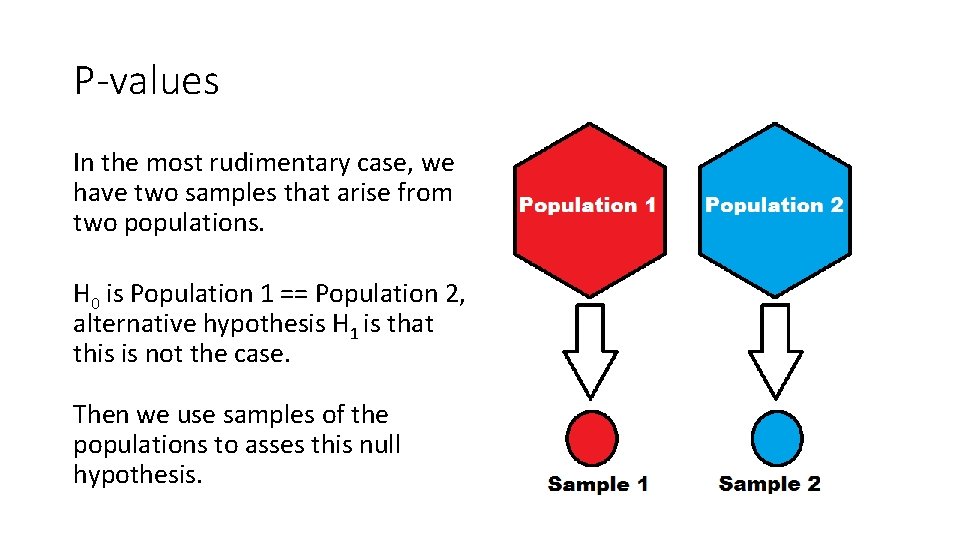
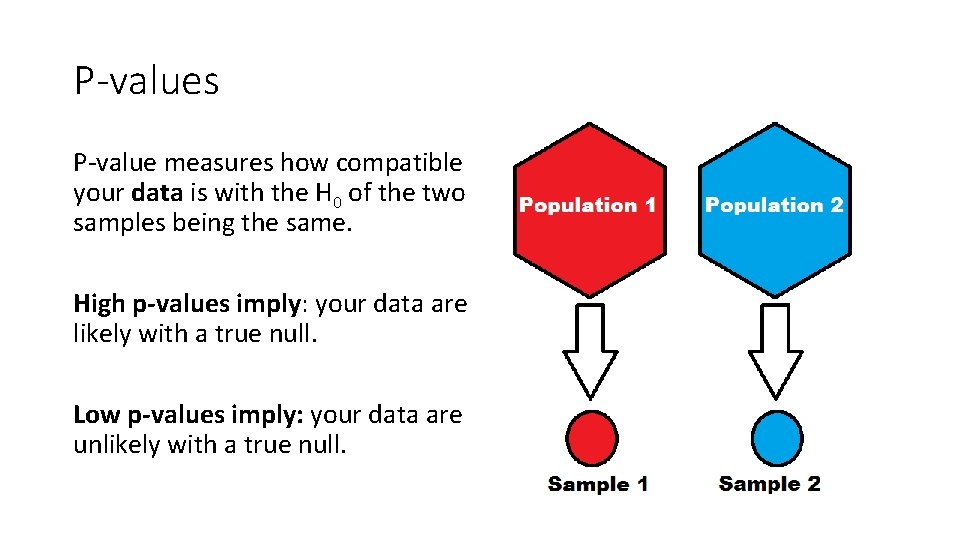
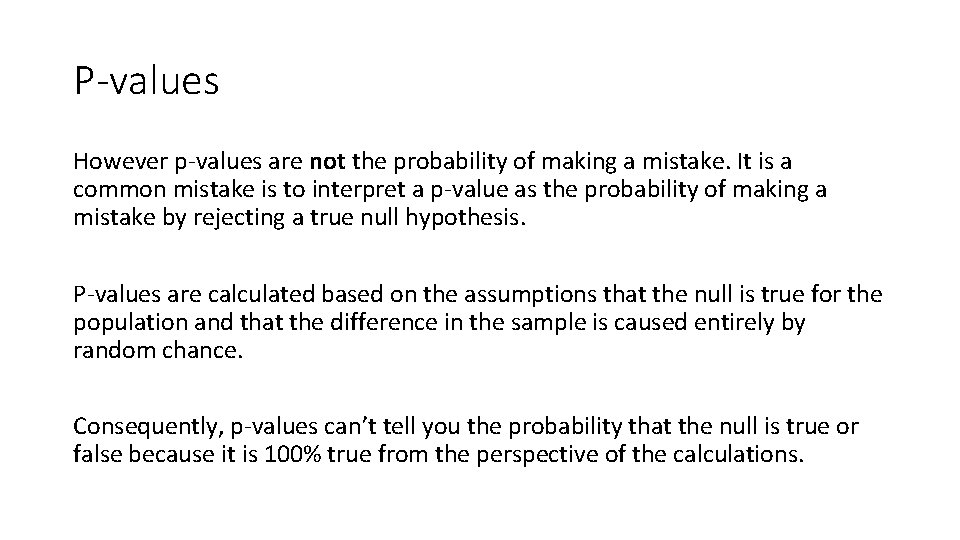
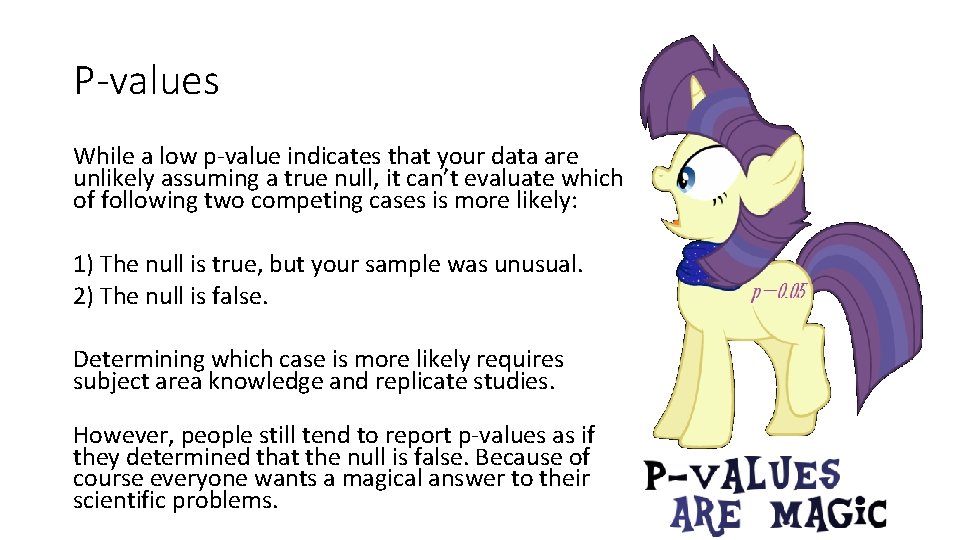
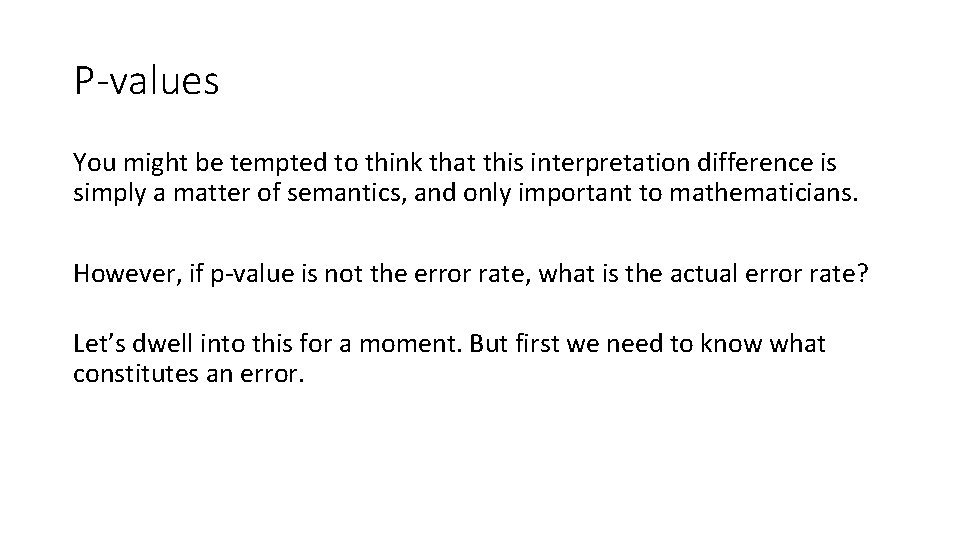
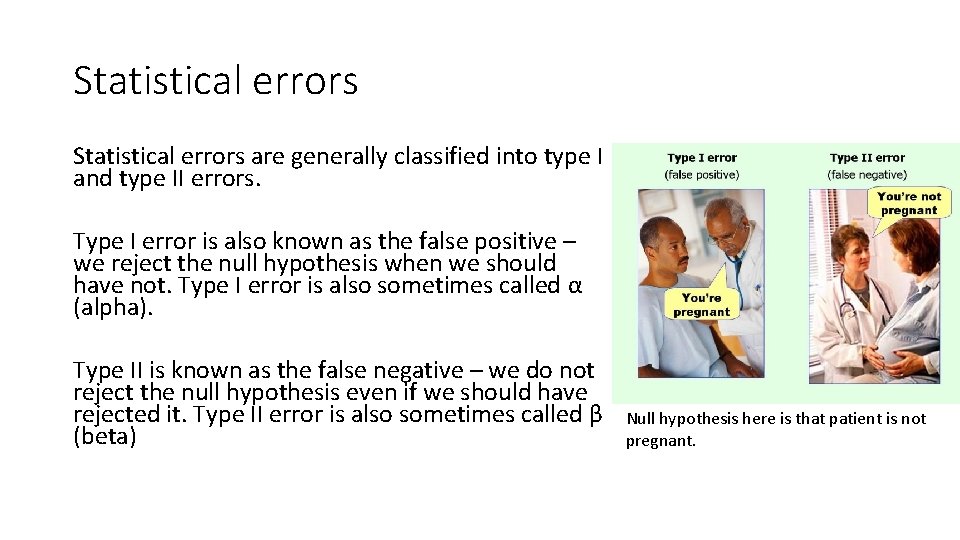
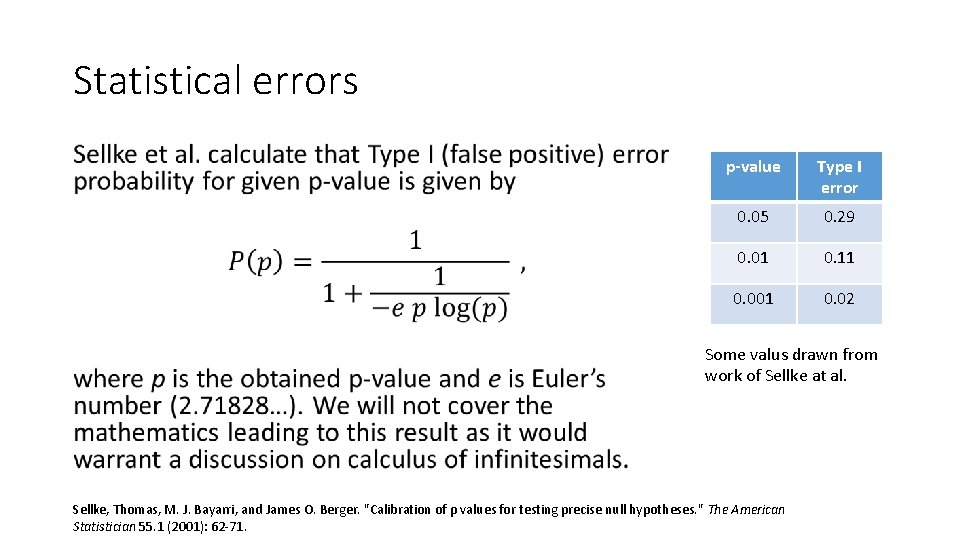
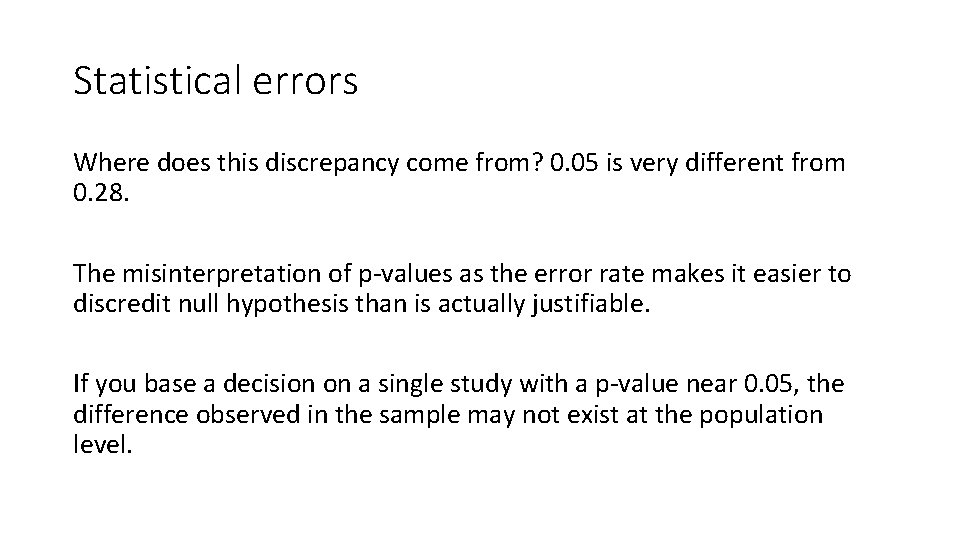
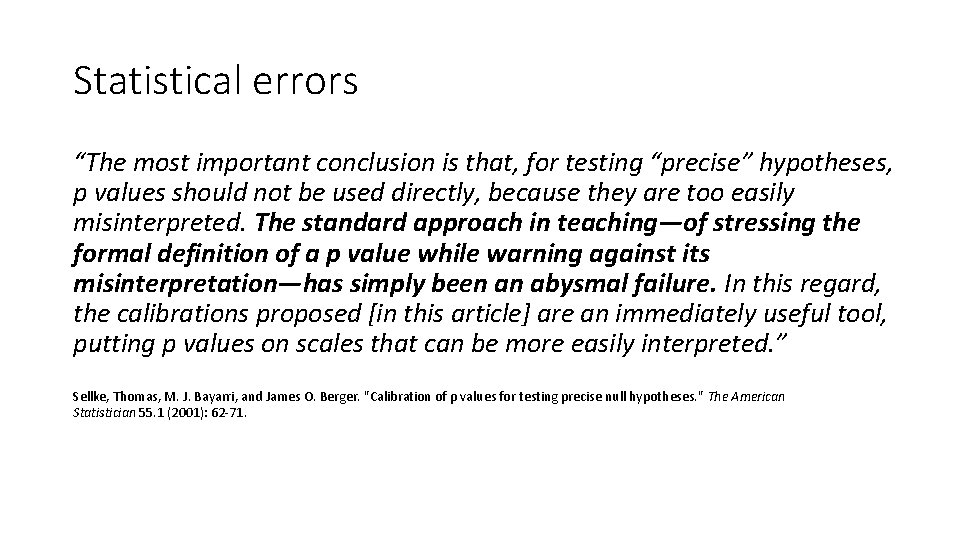
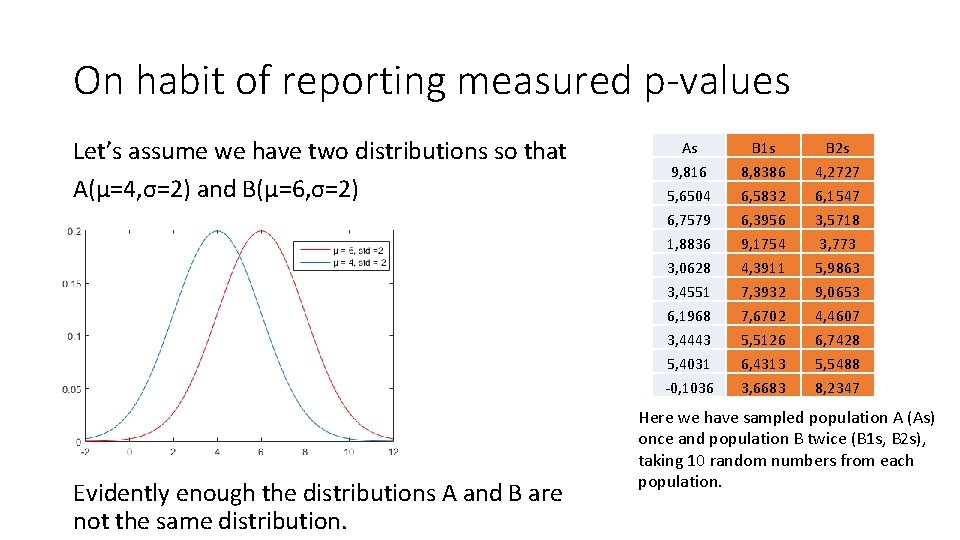
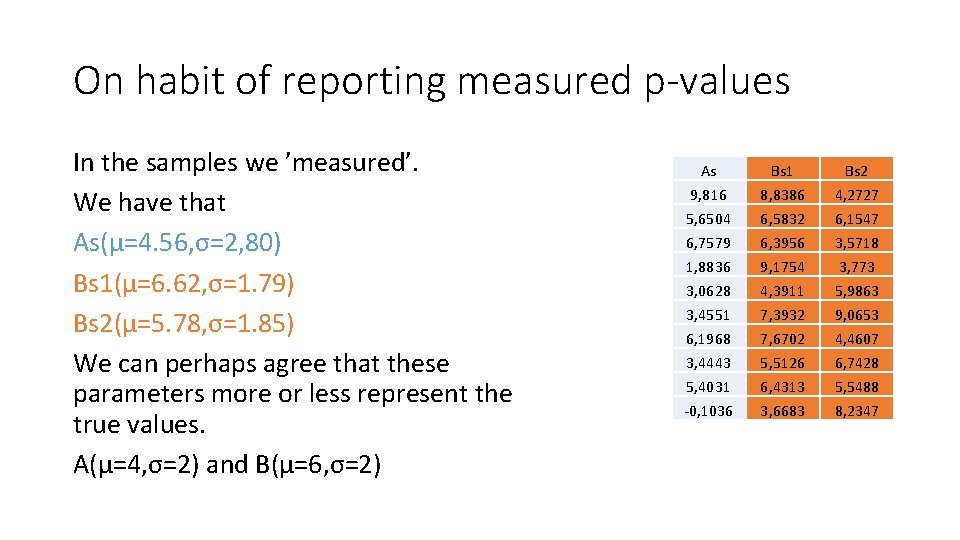
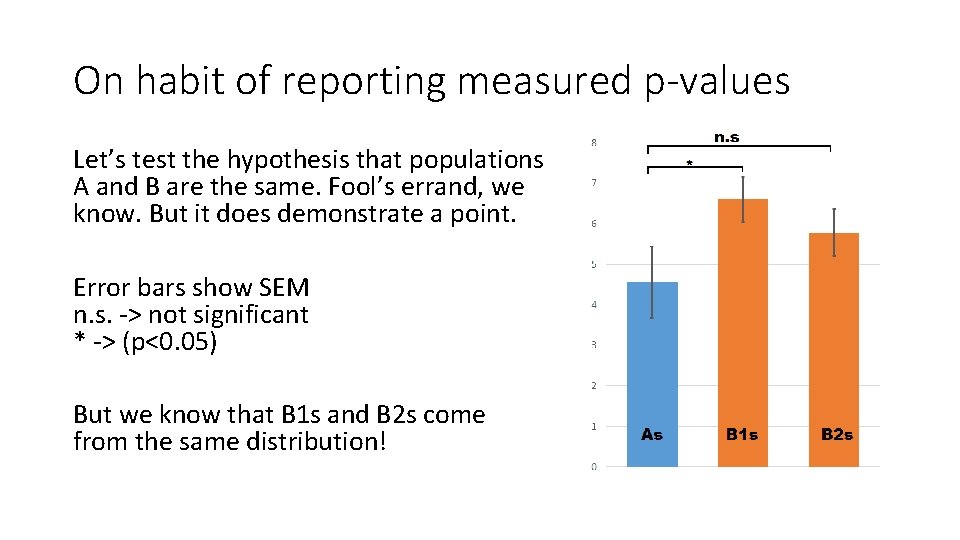
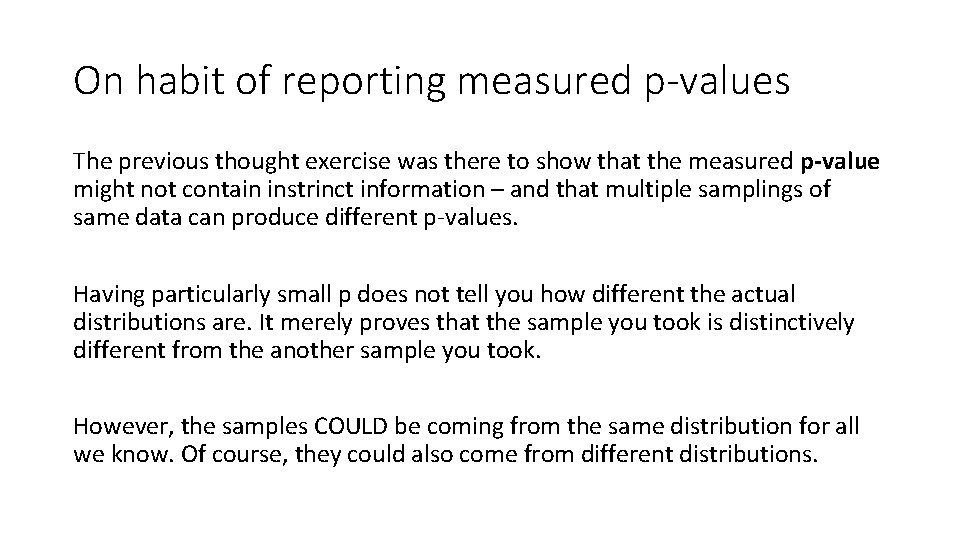
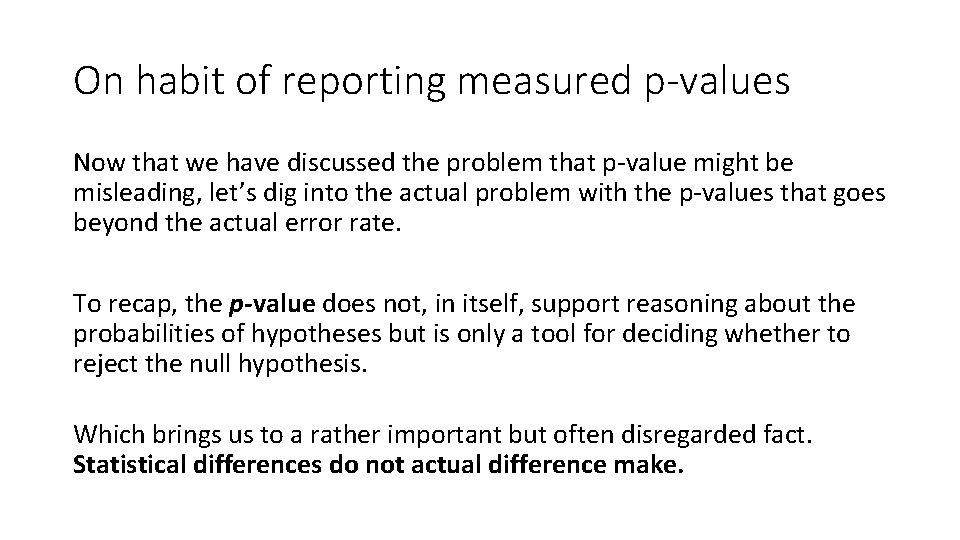
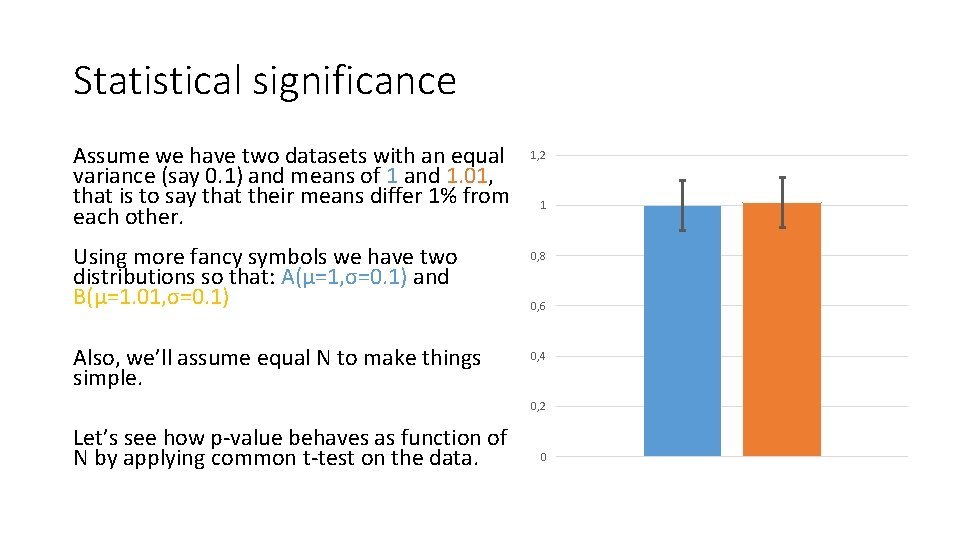
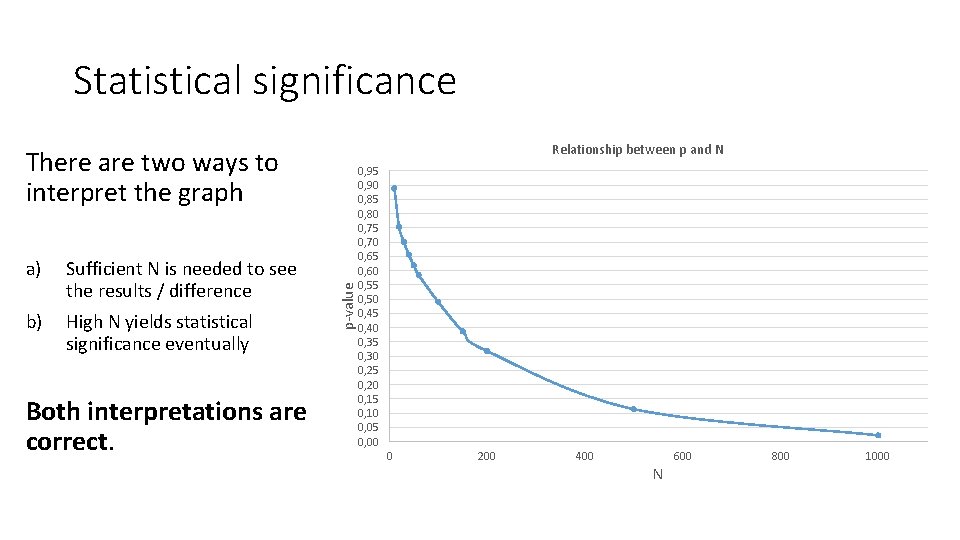
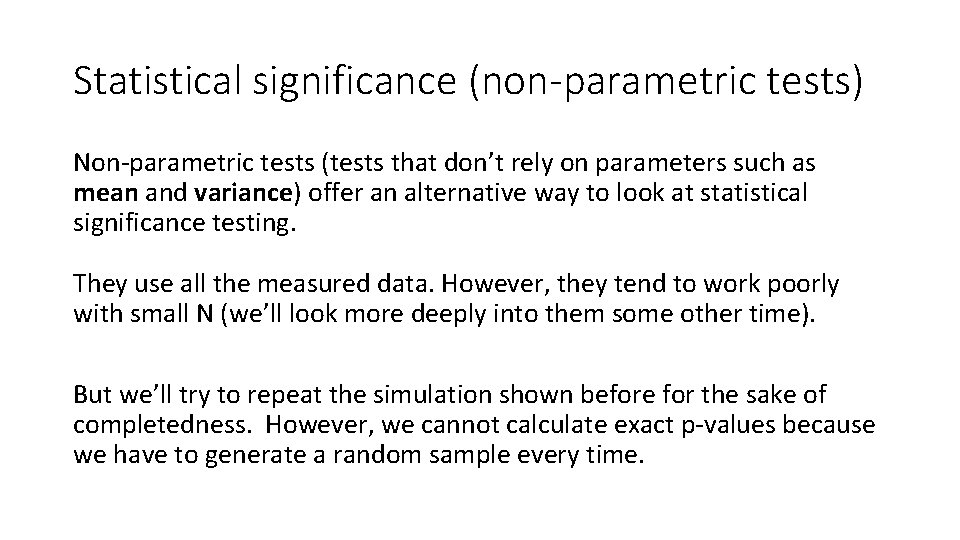
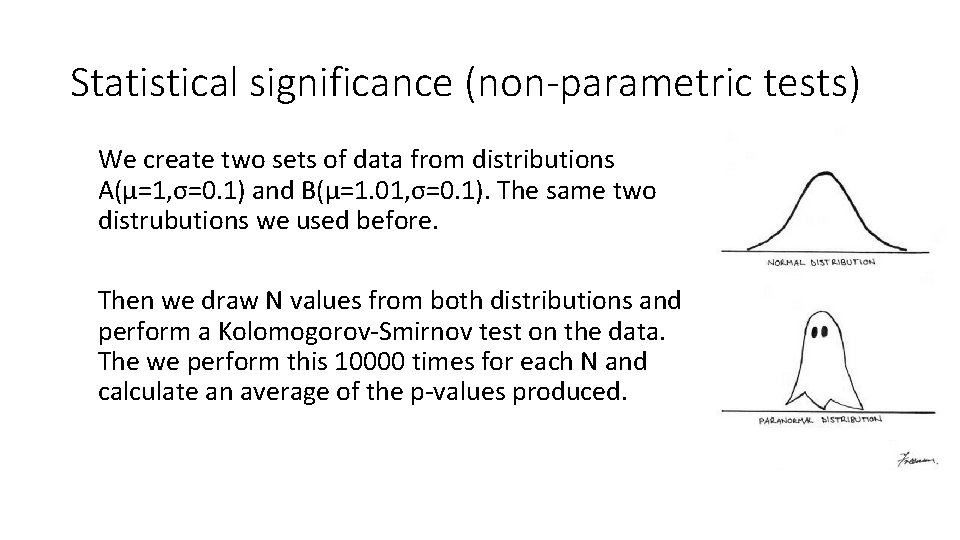
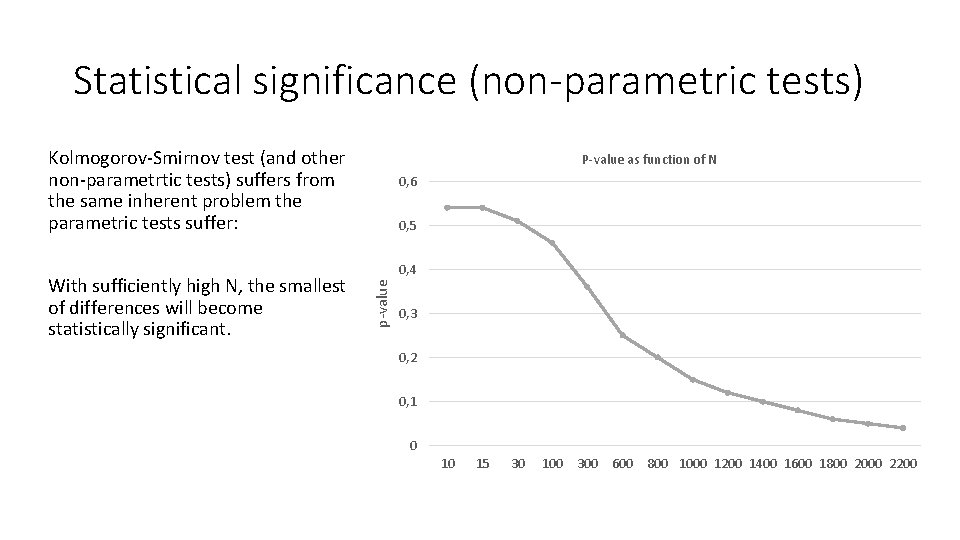
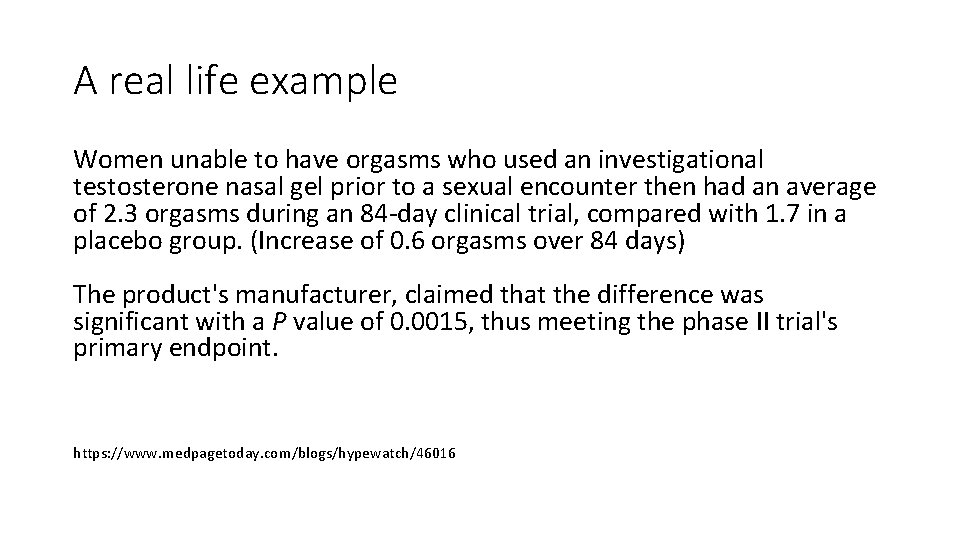
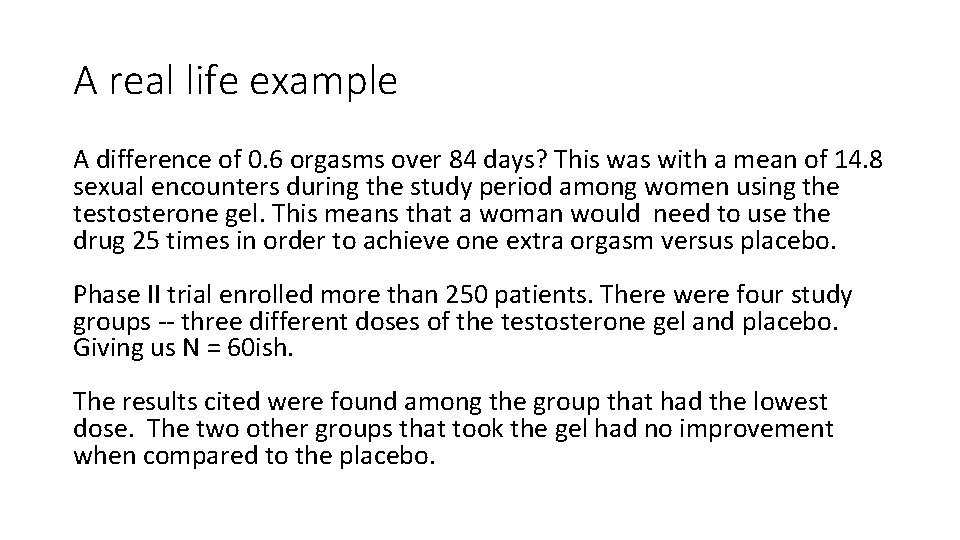
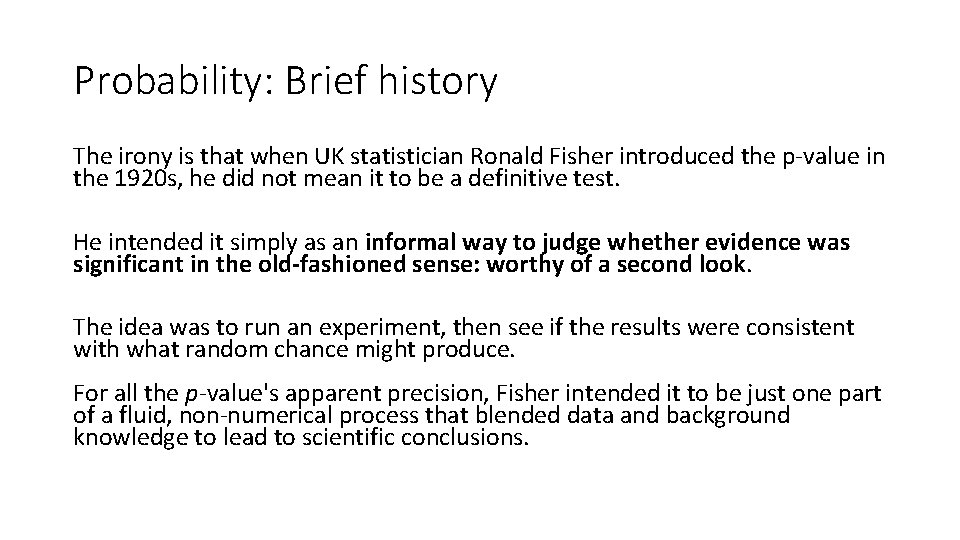
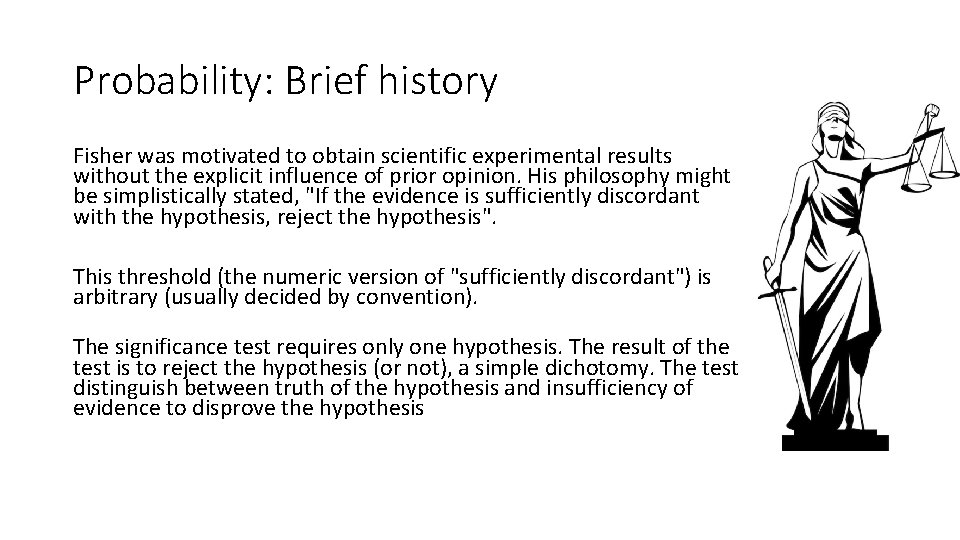
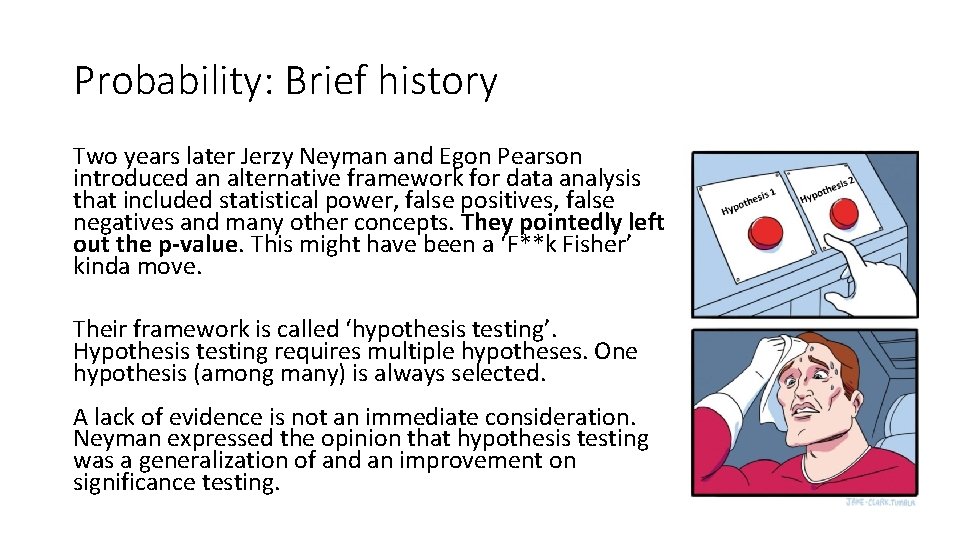
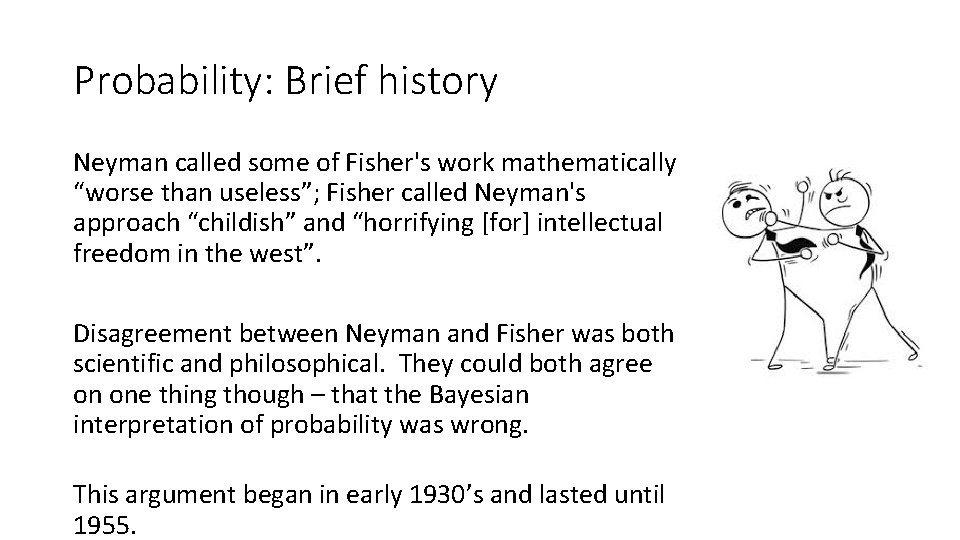
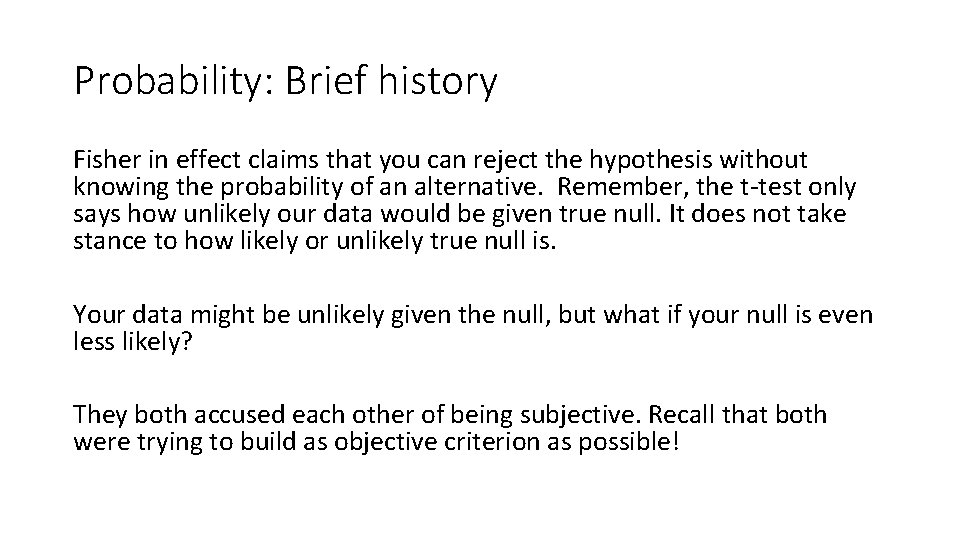
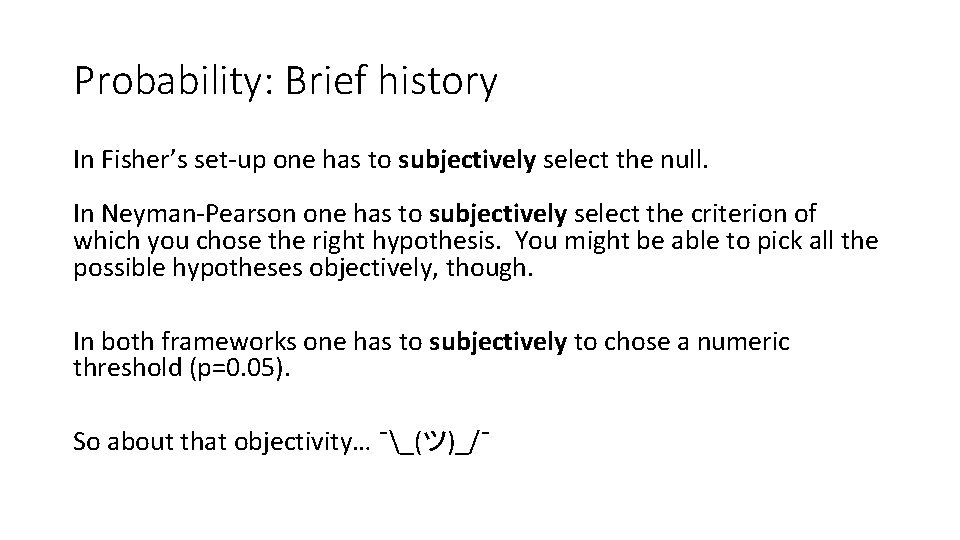
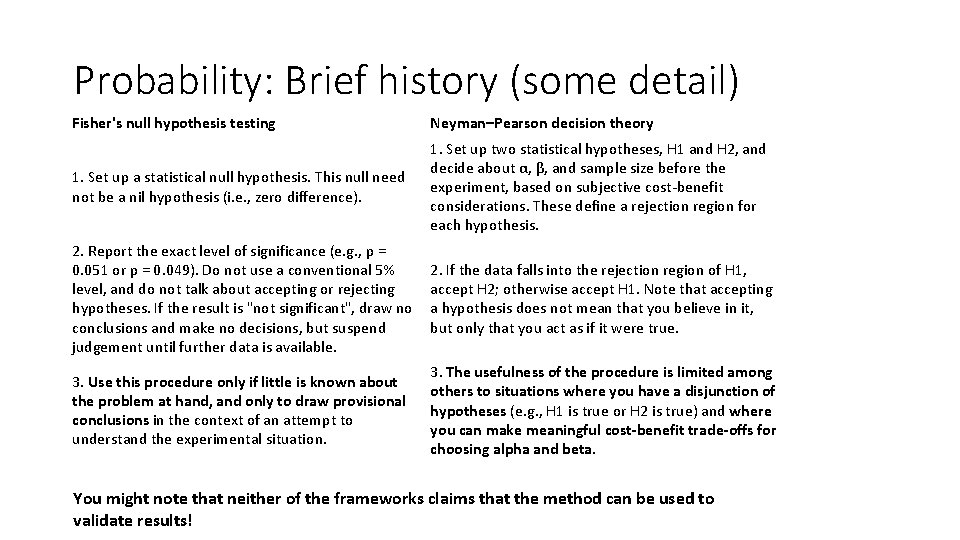
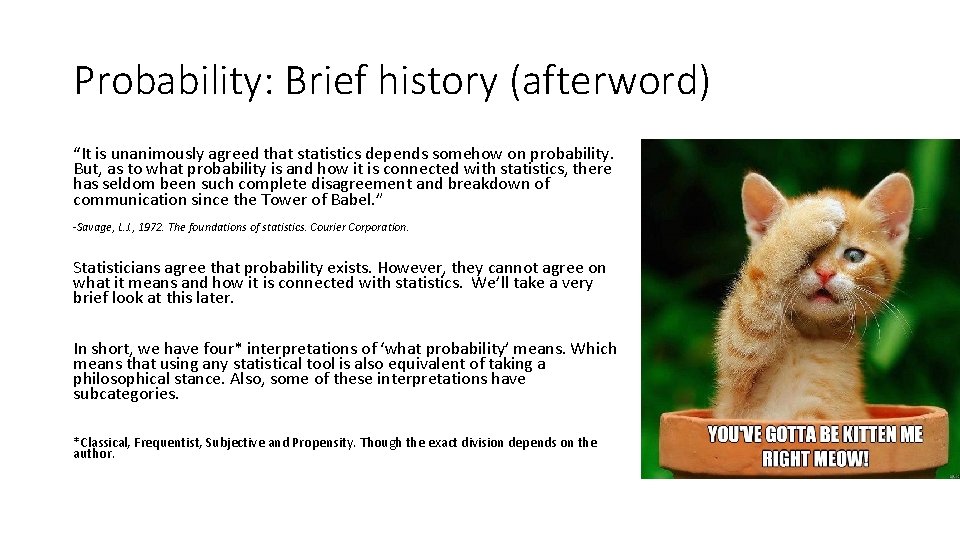
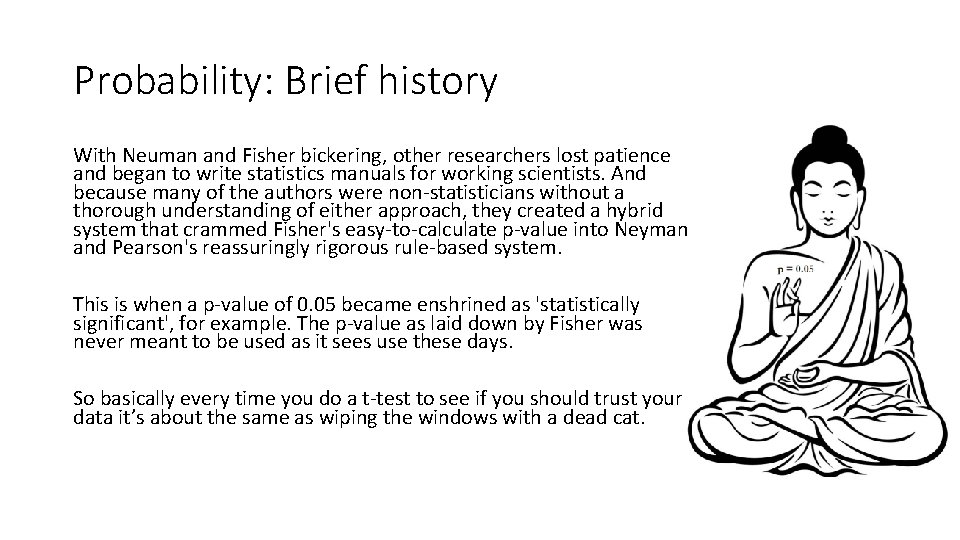
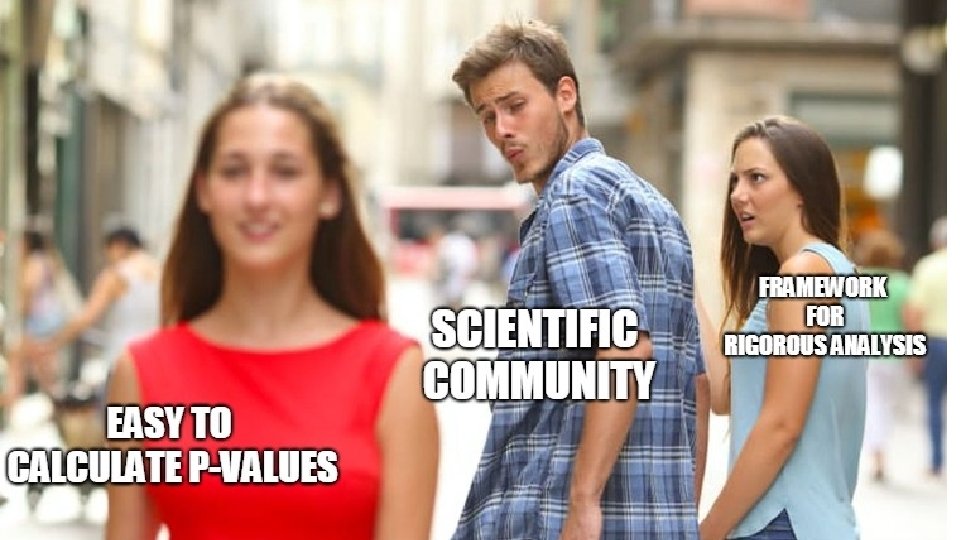
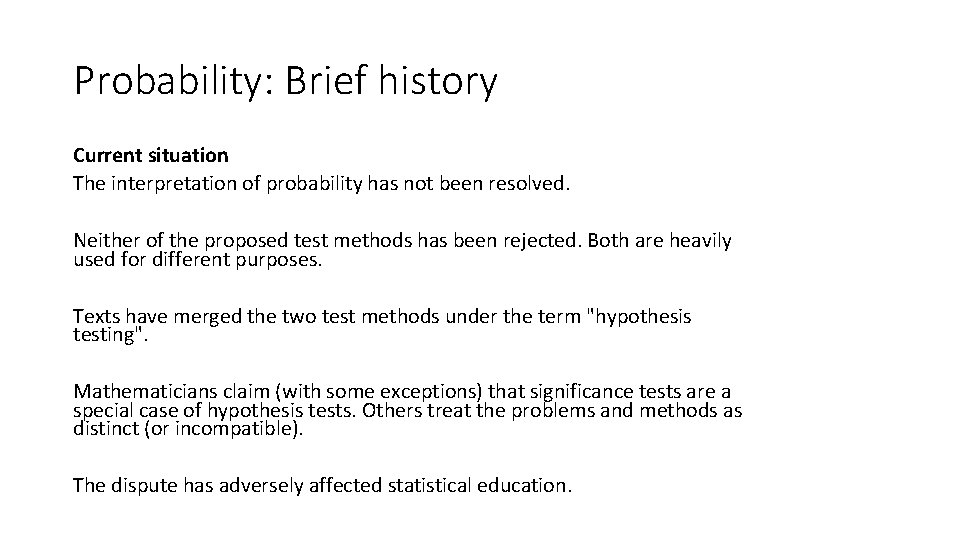
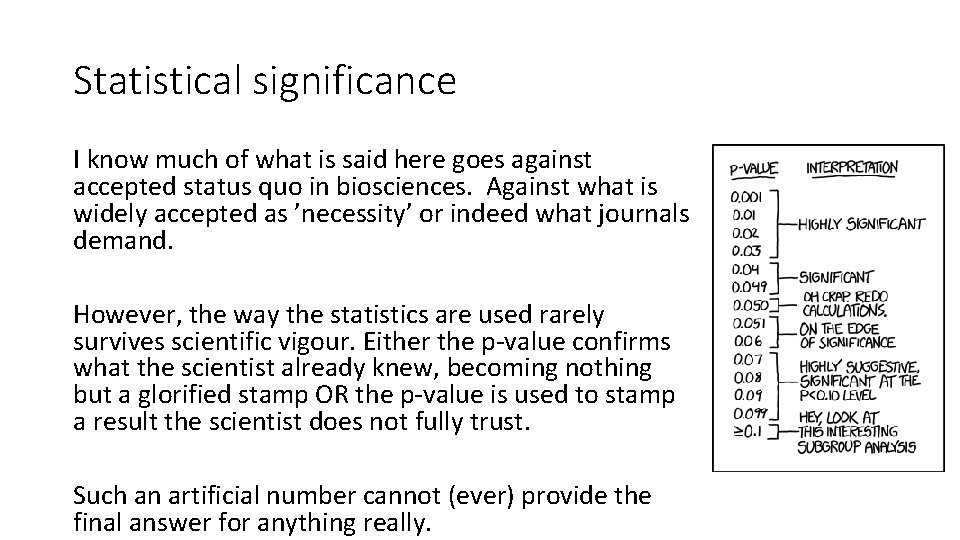
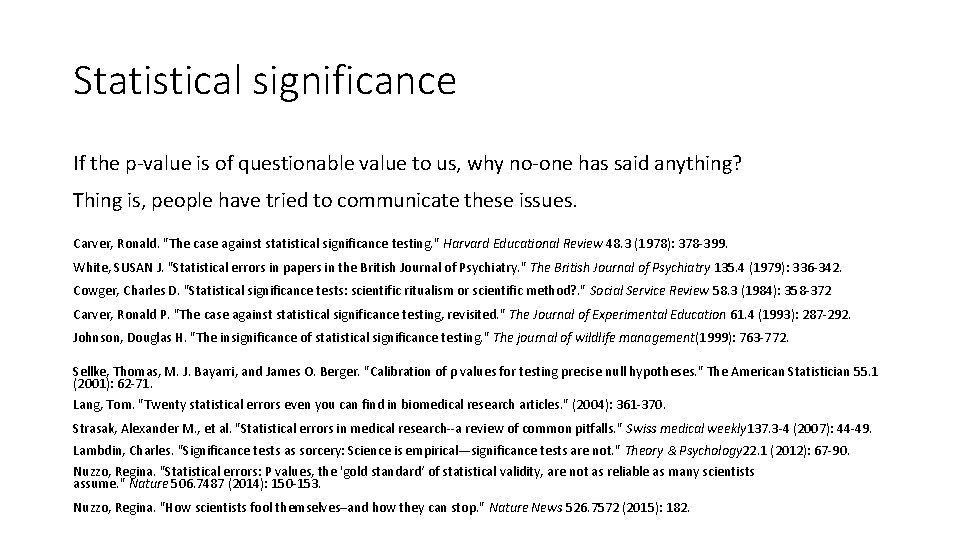
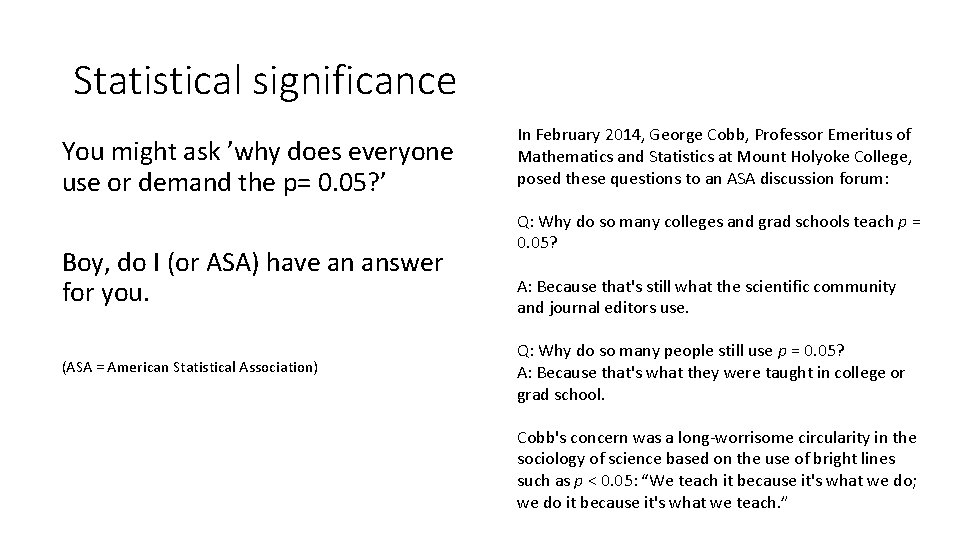
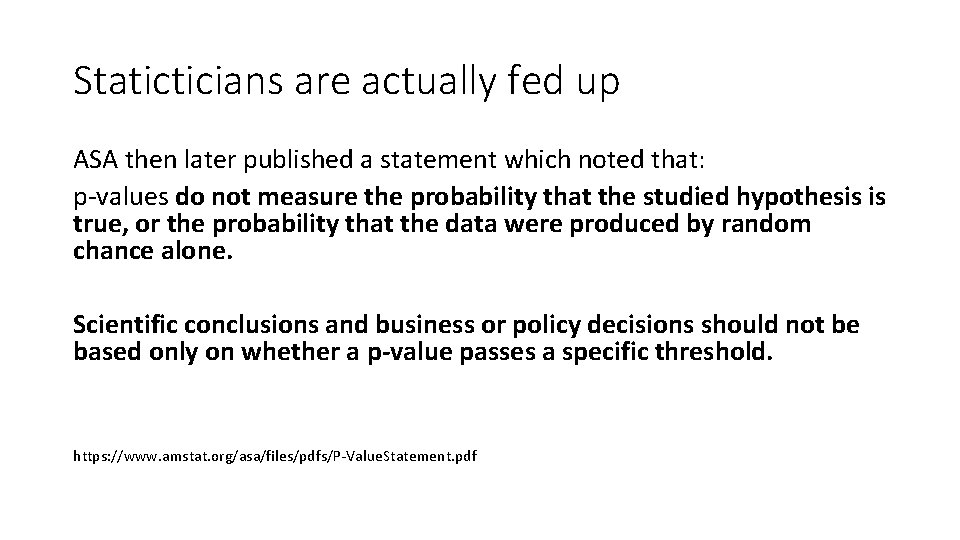
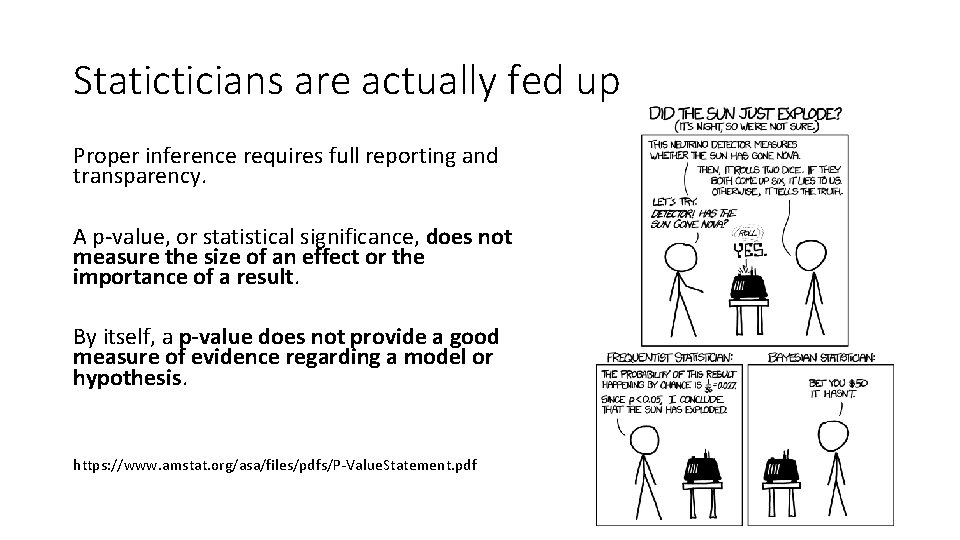
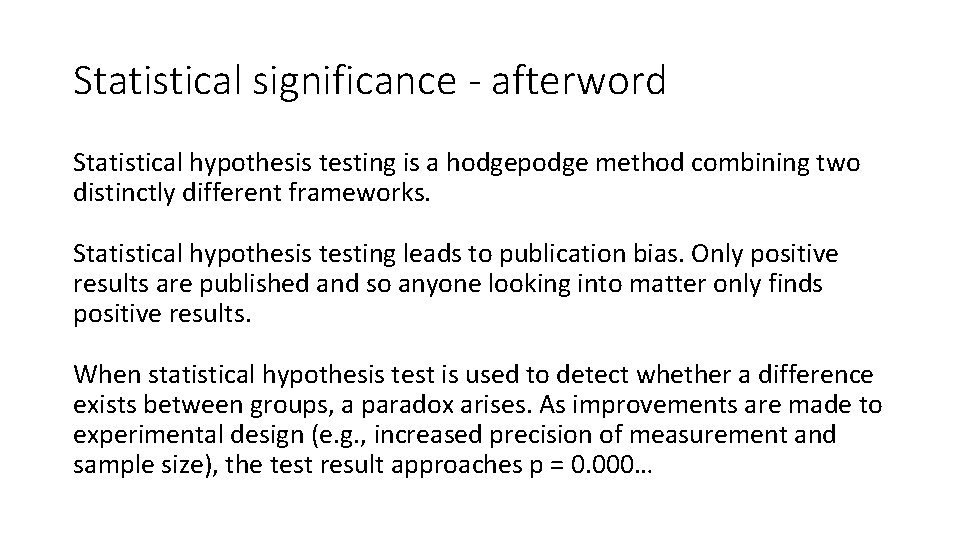
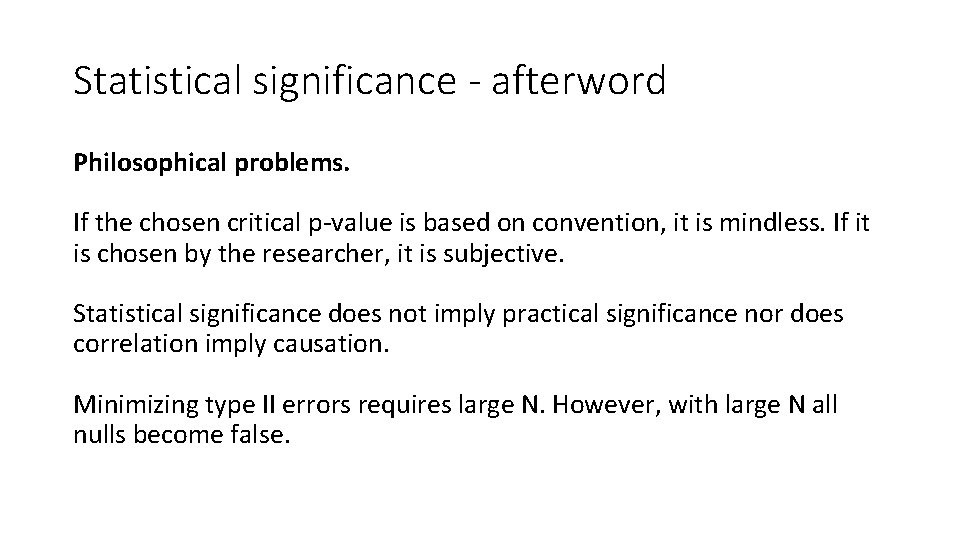
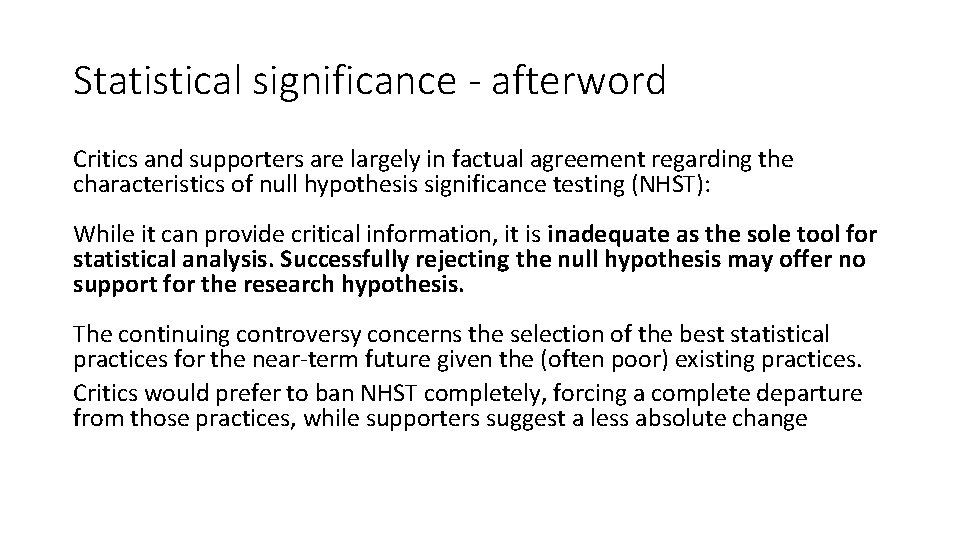
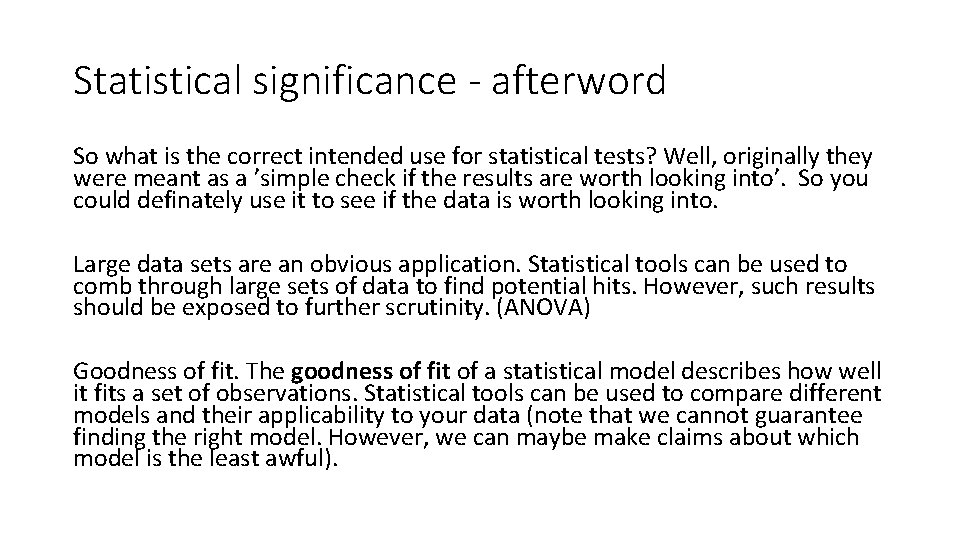
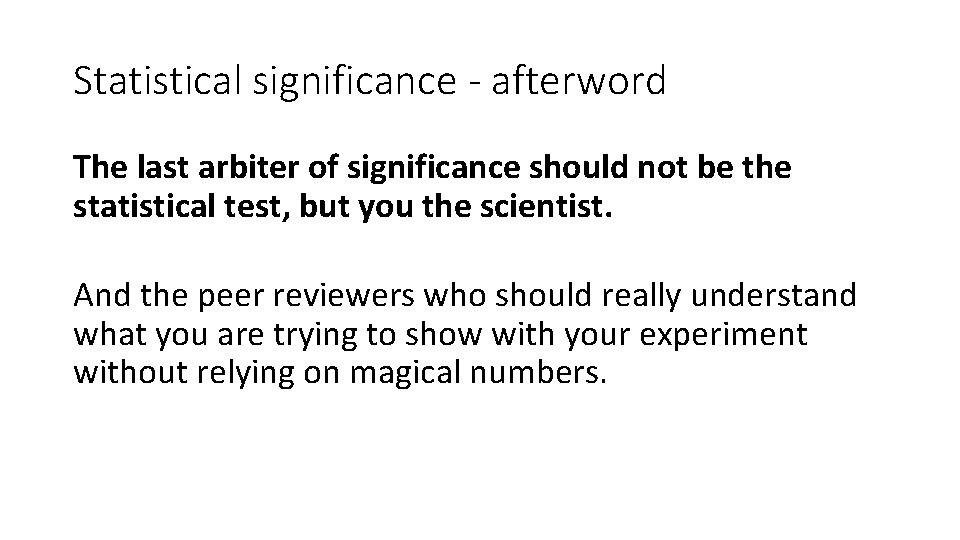
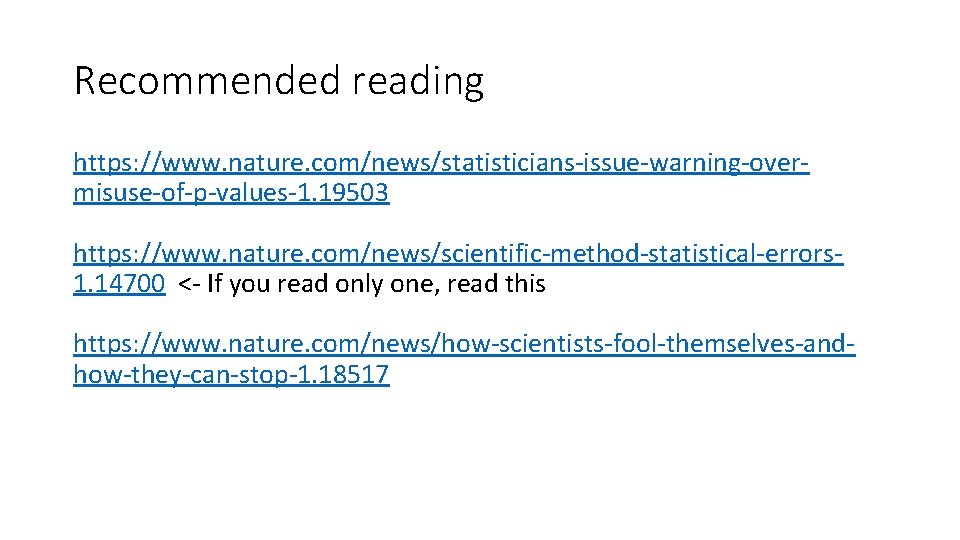
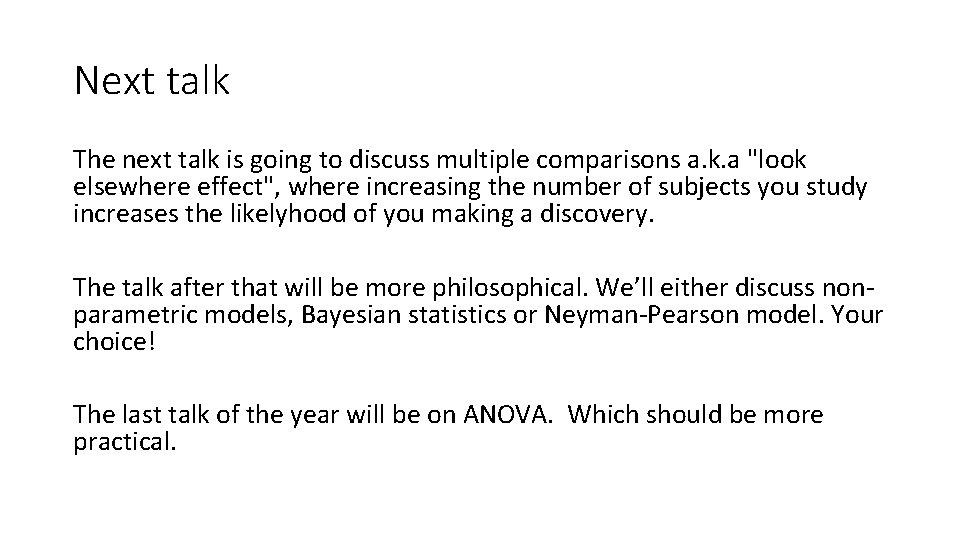
- Slides: 50
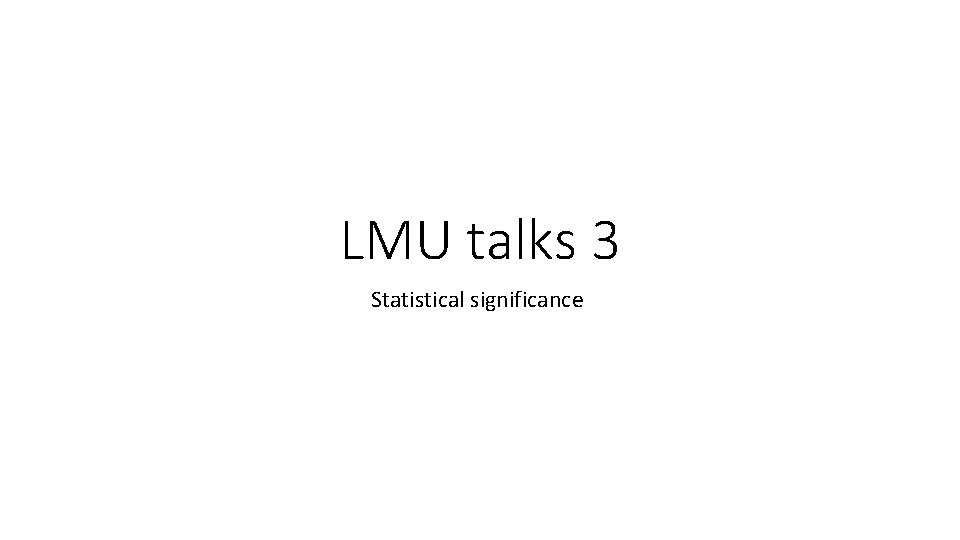
LMU talks 3 Statistical significance
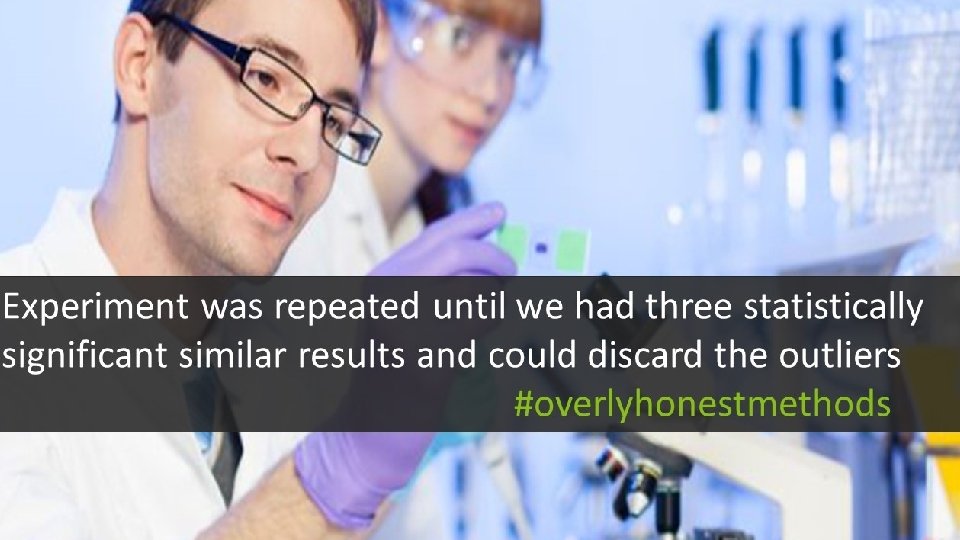
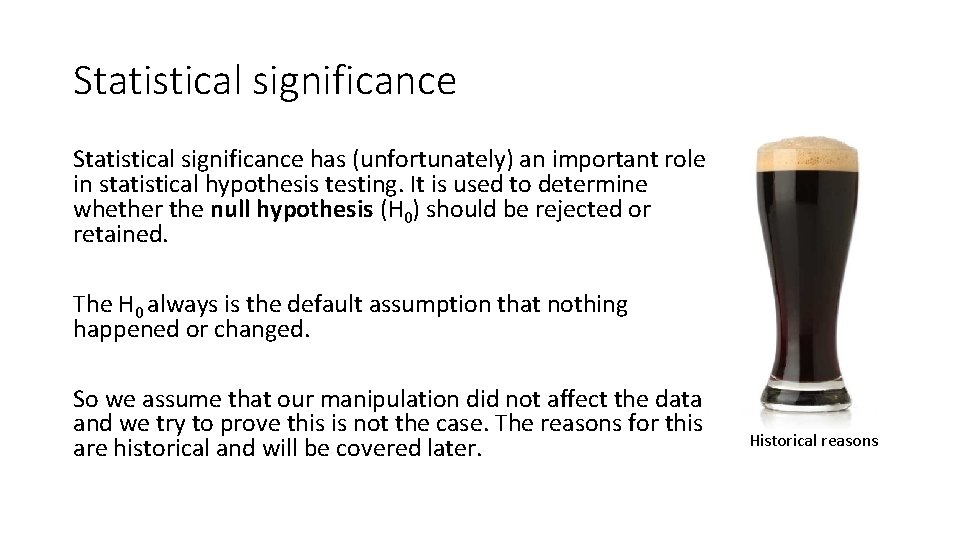
Statistical significance has (unfortunately) an important role in statistical hypothesis testing. It is used to determine whether the null hypothesis (H 0) should be rejected or retained. The H 0 always is the default assumption that nothing happened or changed. So we assume that our manipulation did not affect the data and we try to prove this is not the case. The reasons for this are historical and will be covered later. Historical reasons
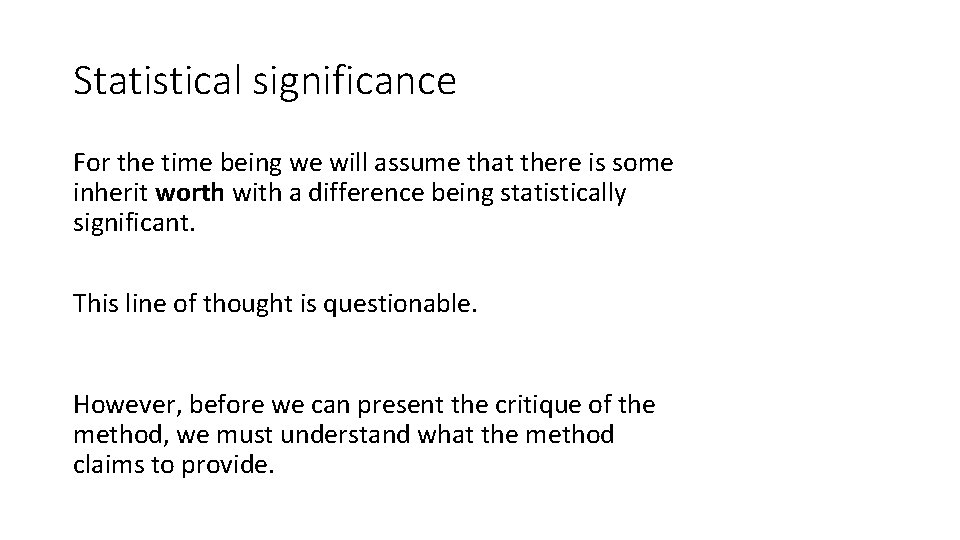
Statistical significance For the time being we will assume that there is some inherit worth with a difference being statistically significant. This line of thought is questionable. However, before we can present the critique of the method, we must understand what the method claims to provide.
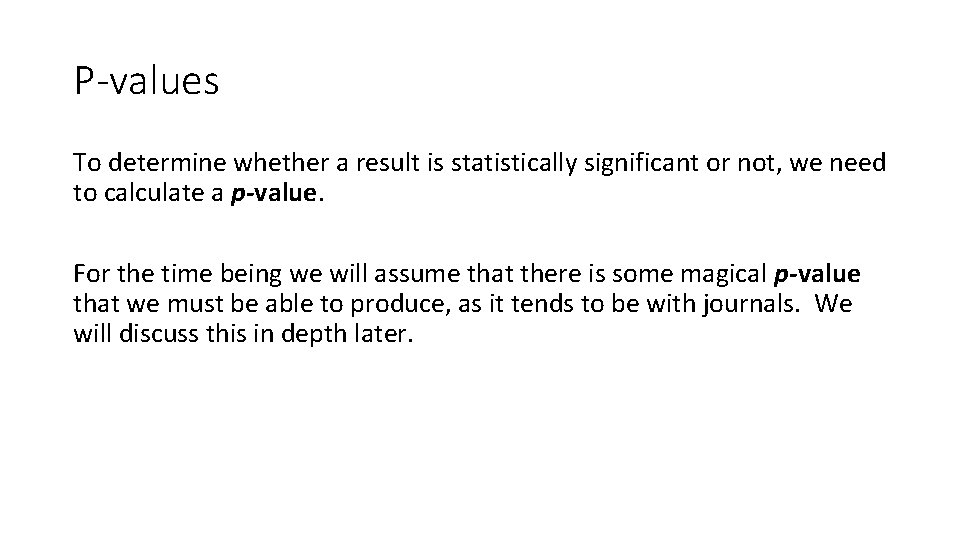
P-values To determine whether a result is statistically significant or not, we need to calculate a p-value. For the time being we will assume that there is some magical p-value that we must be able to produce, as it tends to be with journals. We will discuss this in depth later.
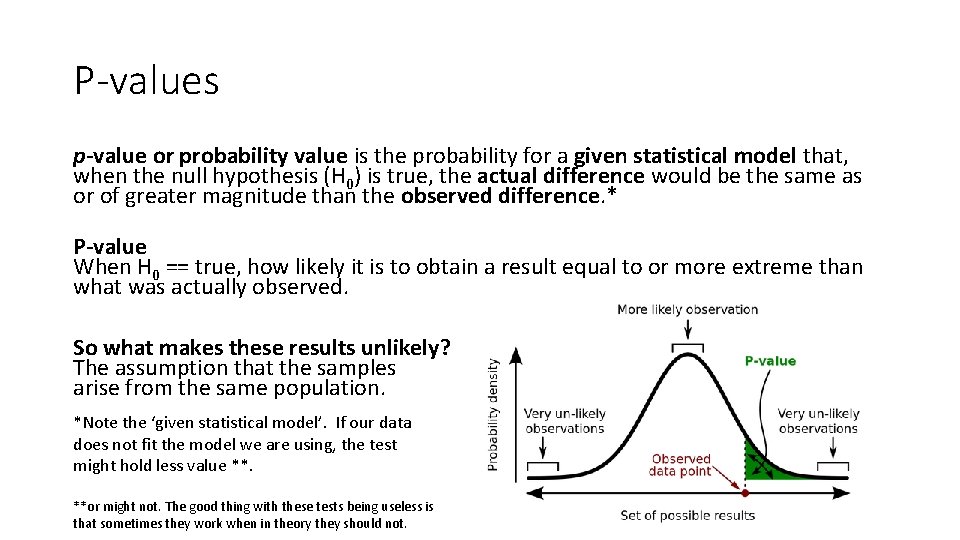
P-values p-value or probability value is the probability for a given statistical model that, when the null hypothesis (H 0) is true, the actual difference would be the same as or of greater magnitude than the observed difference. * P-value When H 0 == true, how likely it is to obtain a result equal to or more extreme than what was actually observed. So what makes these results unlikely? The assumption that the samples arise from the same population. *Note the ‘given statistical model’. If our data does not fit the model we are using, the test might hold less value **. **or might not. The good thing with these tests being useless is that sometimes they work when in theory they should not.
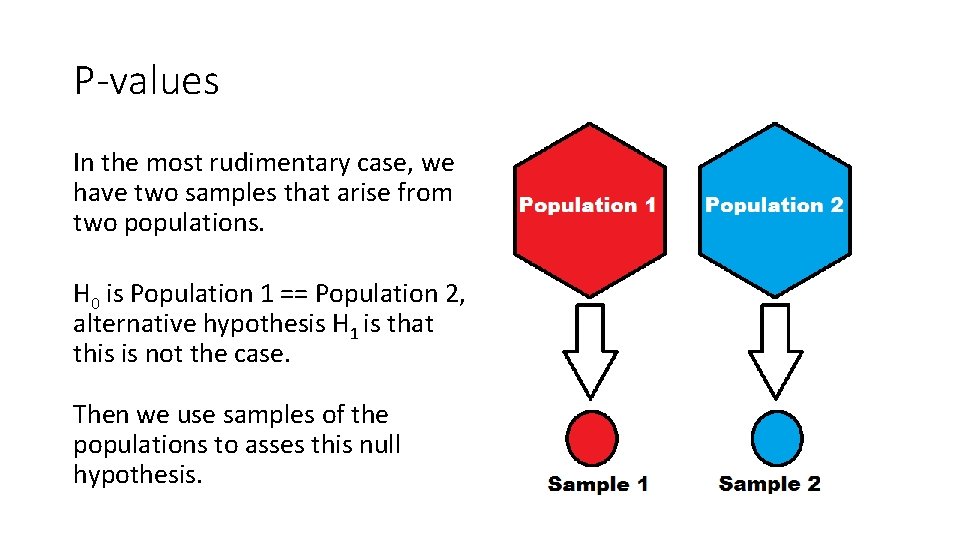
P-values In the most rudimentary case, we have two samples that arise from two populations. H 0 is Population 1 == Population 2, alternative hypothesis H 1 is that this is not the case. Then we use samples of the populations to asses this null hypothesis.
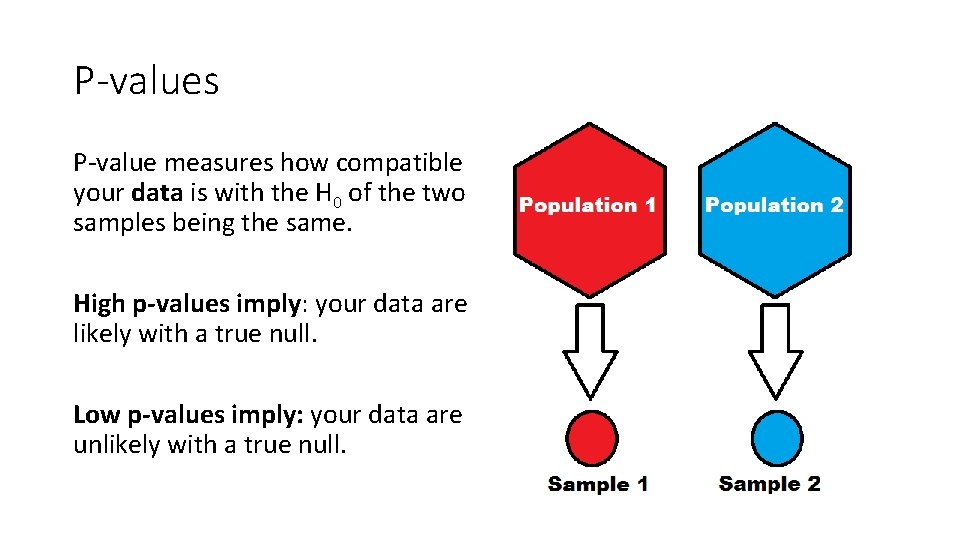
P-values P-value measures how compatible your data is with the H 0 of the two samples being the same. High p-values imply: your data are likely with a true null. Low p-values imply: your data are unlikely with a true null.
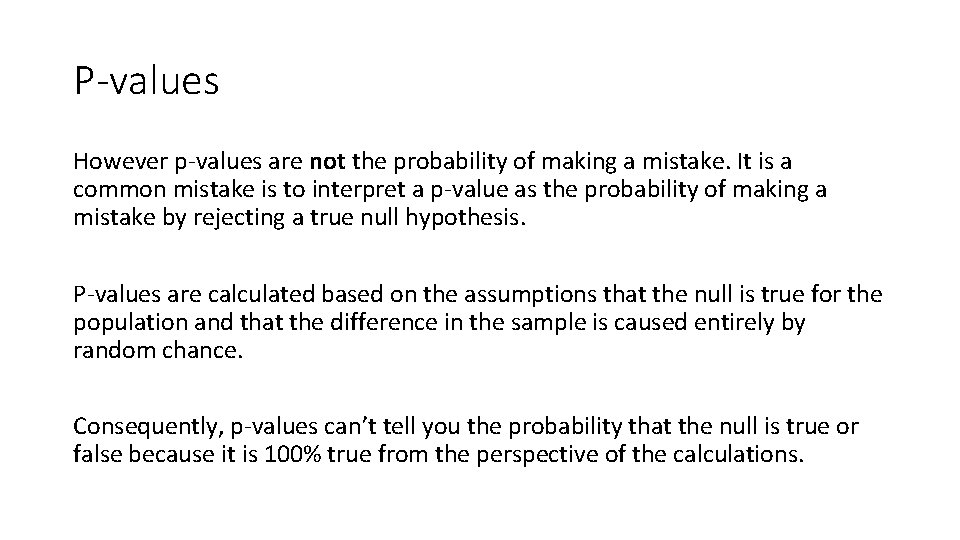
P-values However p-values are not the probability of making a mistake. It is a common mistake is to interpret a p-value as the probability of making a mistake by rejecting a true null hypothesis. P-values are calculated based on the assumptions that the null is true for the population and that the difference in the sample is caused entirely by random chance. Consequently, p-values can’t tell you the probability that the null is true or false because it is 100% true from the perspective of the calculations.
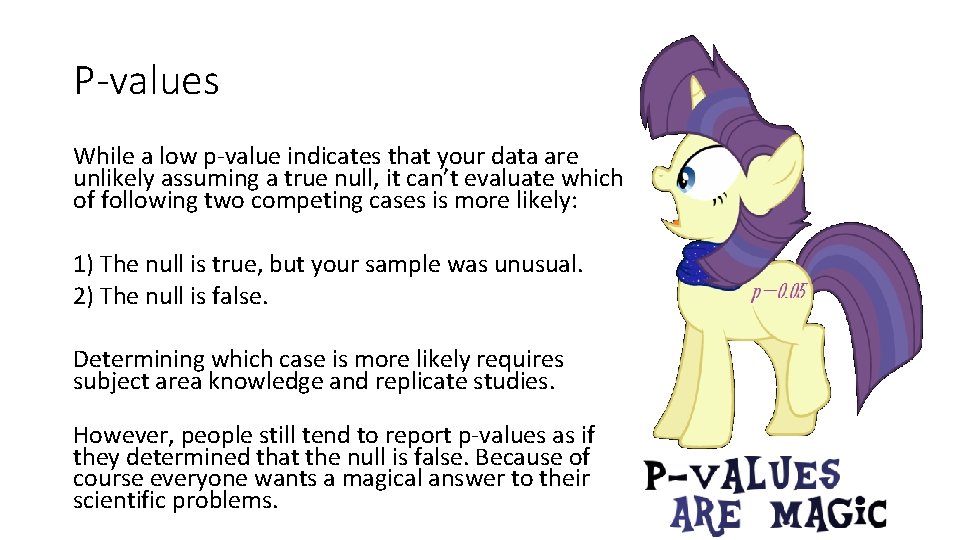
P-values While a low p-value indicates that your data are unlikely assuming a true null, it can’t evaluate which of following two competing cases is more likely: 1) The null is true, but your sample was unusual. 2) The null is false. Determining which case is more likely requires subject area knowledge and replicate studies. However, people still tend to report p-values as if they determined that the null is false. Because of course everyone wants a magical answer to their scientific problems.
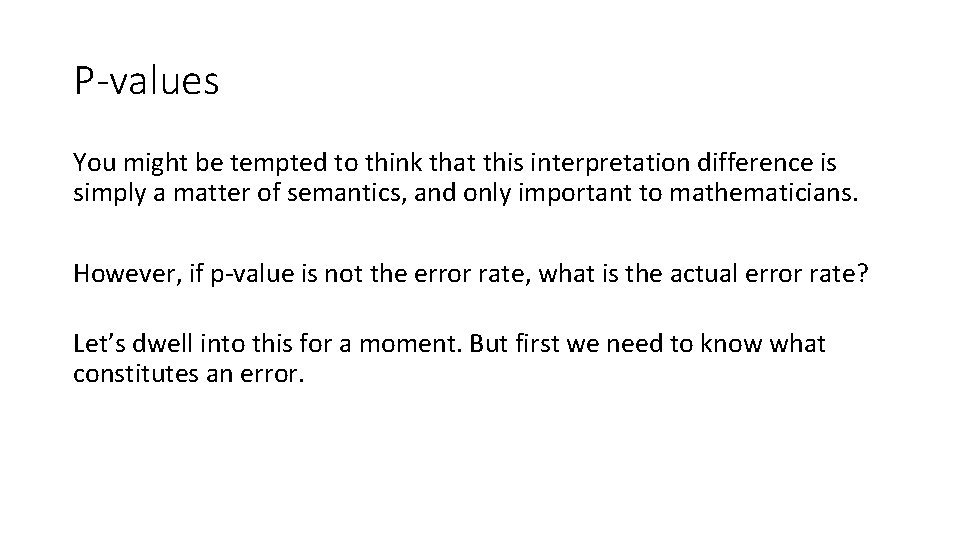
P-values You might be tempted to think that this interpretation difference is simply a matter of semantics, and only important to mathematicians. However, if p-value is not the error rate, what is the actual error rate? Let’s dwell into this for a moment. But first we need to know what constitutes an error.
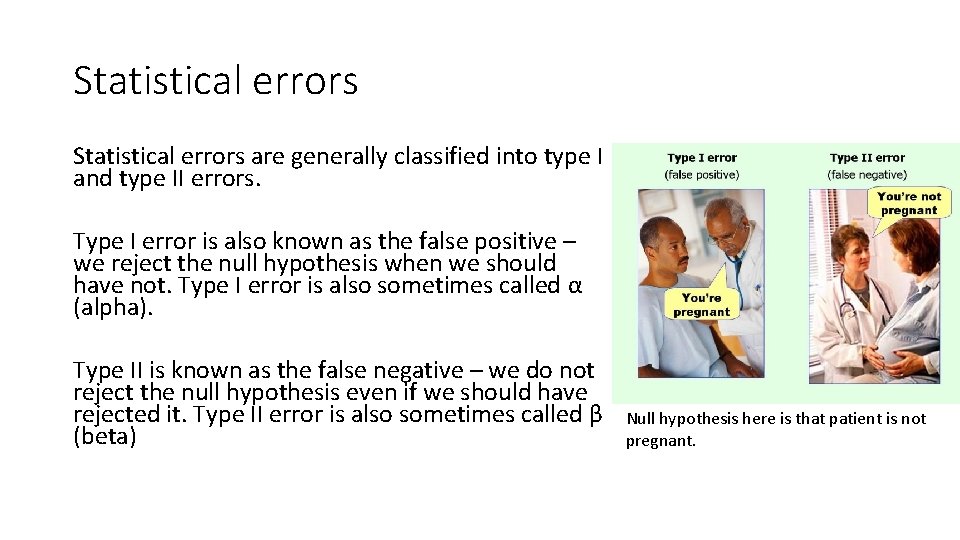
Statistical errors are generally classified into type I and type II errors. Type I error is also known as the false positive – we reject the null hypothesis when we should have not. Type I error is also sometimes called α (alpha). Type II is known as the false negative – we do not reject the null hypothesis even if we should have rejected it. Type II error is also sometimes called β (beta) Null hypothesis here is that patient is not pregnant.
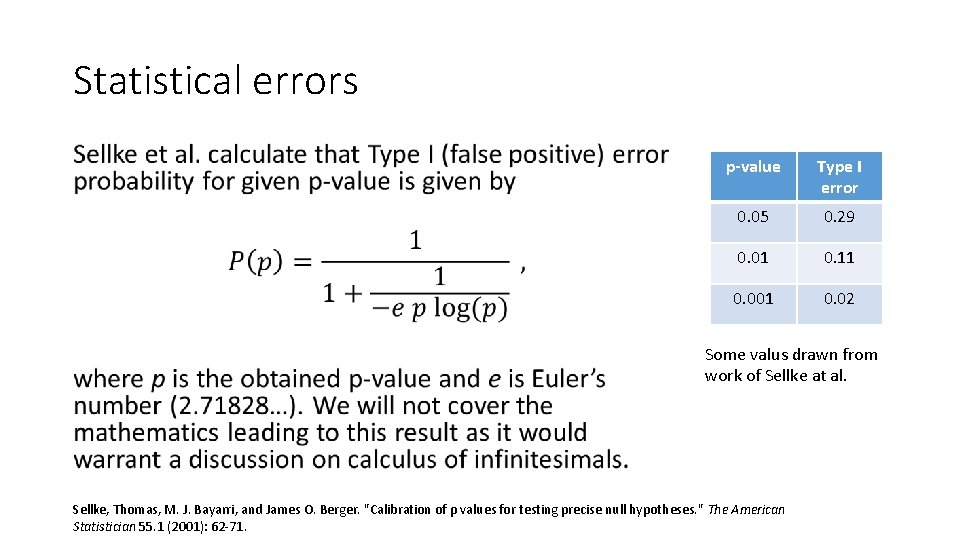
Statistical errors • p-value Type I error 0. 05 0. 29 0. 01 0. 11 0. 001 0. 02 Some valus drawn from work of Sellke at al. Sellke, Thomas, M. J. Bayarri, and James O. Berger. "Calibration of ρ values for testing precise null hypotheses. " The American Statistician 55. 1 (2001): 62 -71.
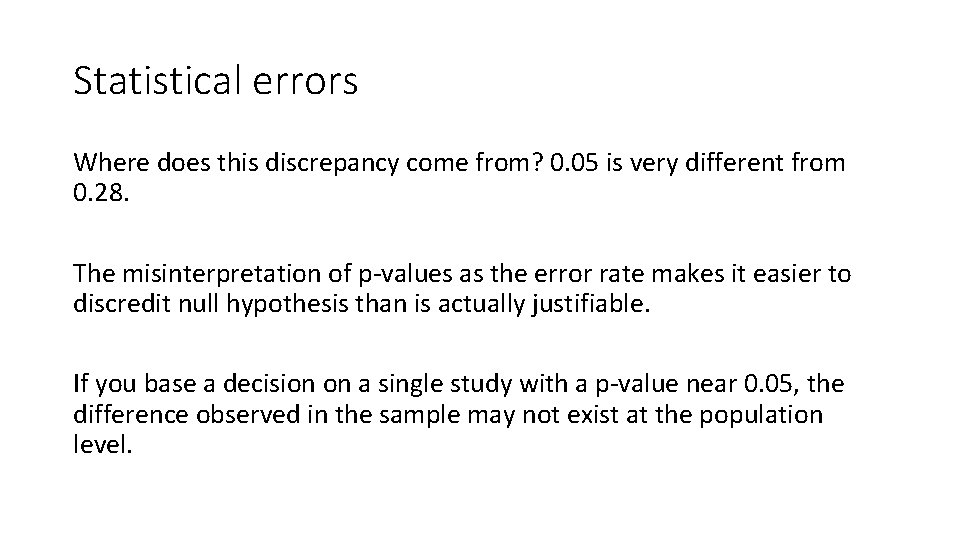
Statistical errors Where does this discrepancy come from? 0. 05 is very different from 0. 28. The misinterpretation of p-values as the error rate makes it easier to discredit null hypothesis than is actually justifiable. If you base a decision on a single study with a p-value near 0. 05, the difference observed in the sample may not exist at the population level.
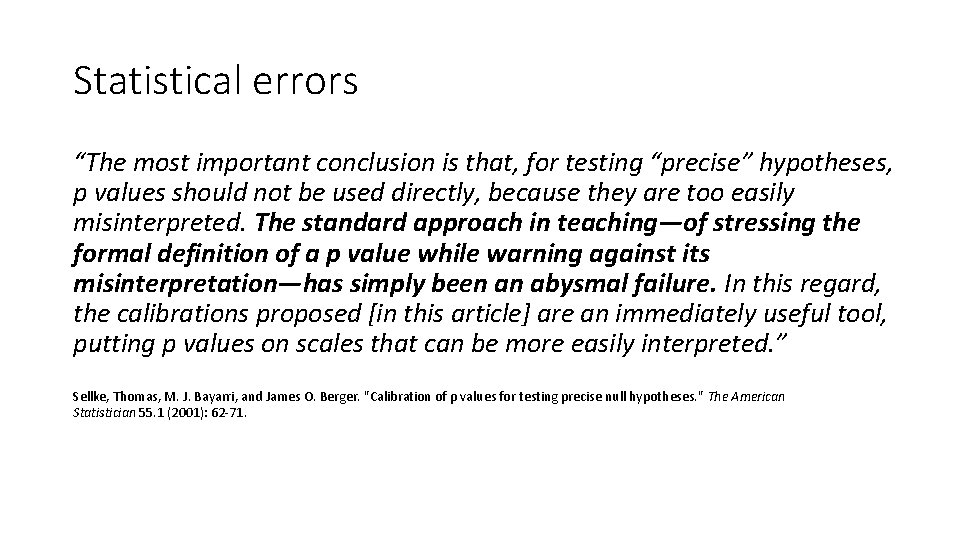
Statistical errors “The most important conclusion is that, for testing “precise” hypotheses, p values should not be used directly, because they are too easily misinterpreted. The standard approach in teaching—of stressing the formal definition of a p value while warning against its misinterpretation—has simply been an abysmal failure. In this regard, the calibrations proposed [in this article] are an immediately useful tool, putting p values on scales that can be more easily interpreted. ” Sellke, Thomas, M. J. Bayarri, and James O. Berger. "Calibration of ρ values for testing precise null hypotheses. " The American Statistician 55. 1 (2001): 62 -71.
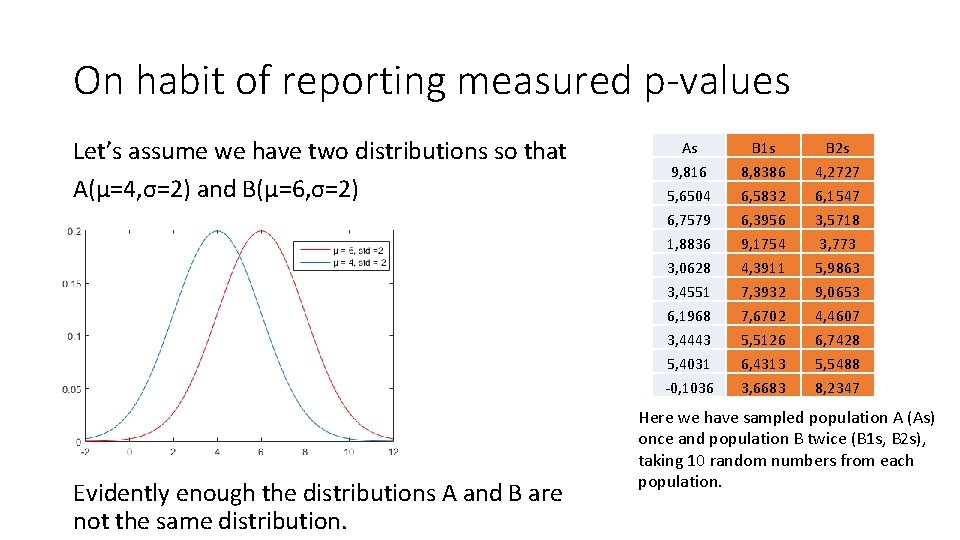
On habit of reporting measured p-values Let’s assume we have two distributions so that A(µ=4, σ=2) and B(µ=6, σ=2) Evidently enough the distributions A and B are not the same distribution. As 9, 816 5, 6504 6, 7579 1, 8836 3, 0628 3, 4551 6, 1968 3, 4443 5, 4031 -0, 1036 B 1 s 8, 8386 6, 5832 6, 3956 9, 1754 4, 3911 7, 3932 7, 6702 5, 5126 6, 4313 3, 6683 B 2 s 4, 2727 6, 1547 3, 5718 3, 773 5, 9863 9, 0653 4, 4607 6, 7428 5, 5488 8, 2347 Here we have sampled population A (As) once and population B twice (B 1 s, B 2 s), taking 10 random numbers from each population.
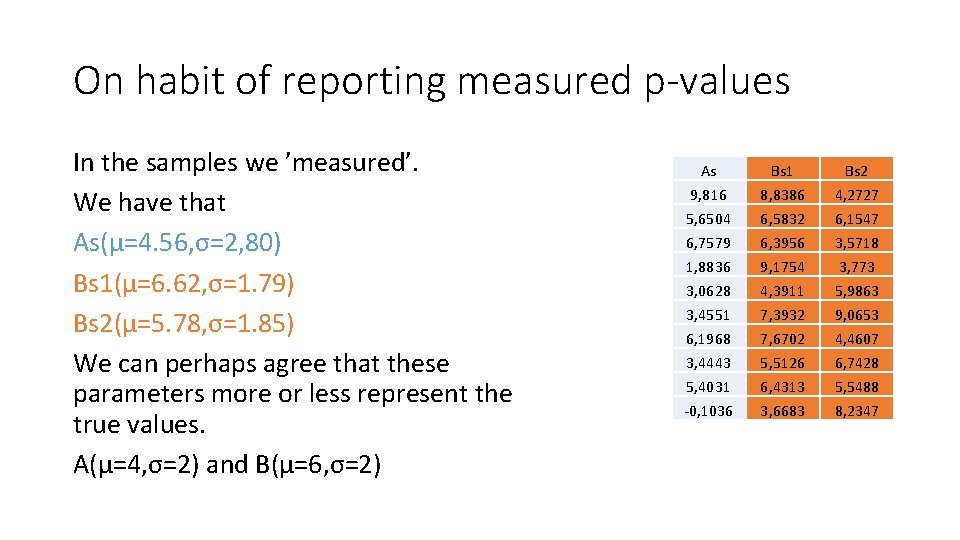
On habit of reporting measured p-values In the samples we ’measured’. We have that As(µ=4. 56, σ=2, 80) Bs 1(µ=6. 62, σ=1. 79) Bs 2(µ=5. 78, σ=1. 85) We can perhaps agree that these parameters more or less represent the true values. A(µ=4, σ=2) and B(µ=6, σ=2) As 9, 816 5, 6504 6, 7579 1, 8836 3, 0628 3, 4551 6, 1968 3, 4443 5, 4031 -0, 1036 Bs 1 8, 8386 6, 5832 6, 3956 9, 1754 4, 3911 7, 3932 7, 6702 5, 5126 6, 4313 3, 6683 Bs 2 4, 2727 6, 1547 3, 5718 3, 773 5, 9863 9, 0653 4, 4607 6, 7428 5, 5488 8, 2347
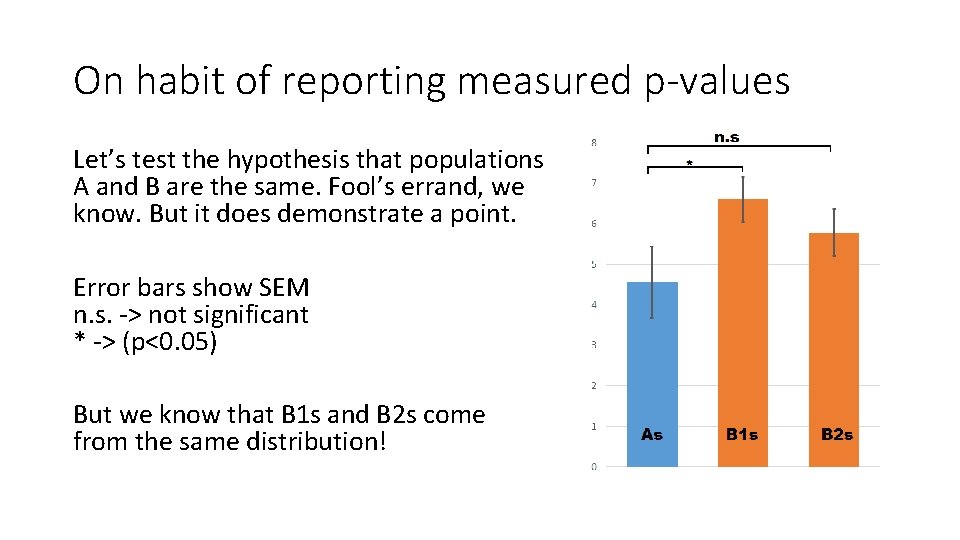
On habit of reporting measured p-values Let’s test the hypothesis that populations A and B are the same. Fool’s errand, we know. But it does demonstrate a point. Error bars show SEM n. s. -> not significant * -> (p<0. 05) But we know that B 1 s and B 2 s come from the same distribution!
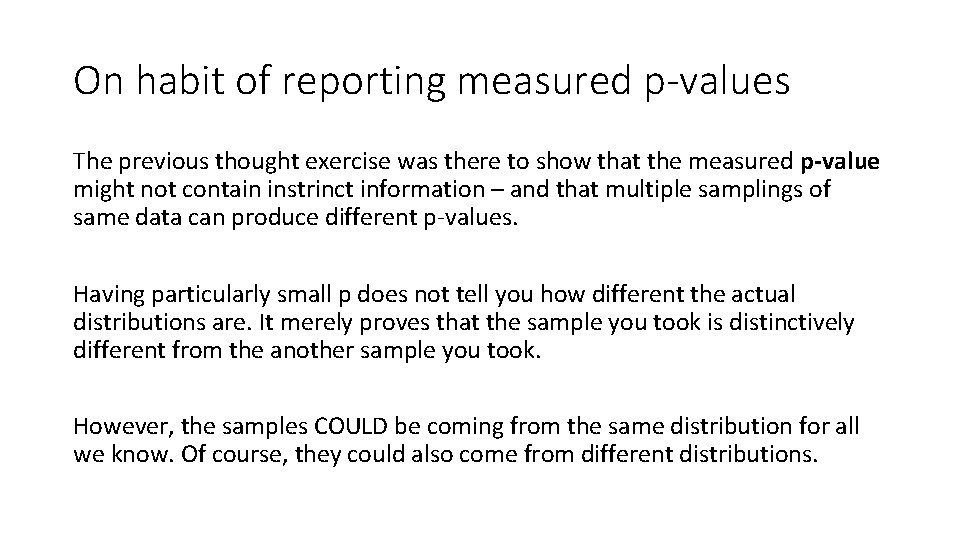
On habit of reporting measured p-values The previous thought exercise was there to show that the measured p-value might not contain instrinct information – and that multiple samplings of same data can produce different p-values. Having particularly small p does not tell you how different the actual distributions are. It merely proves that the sample you took is distinctively different from the another sample you took. However, the samples COULD be coming from the same distribution for all we know. Of course, they could also come from different distributions.
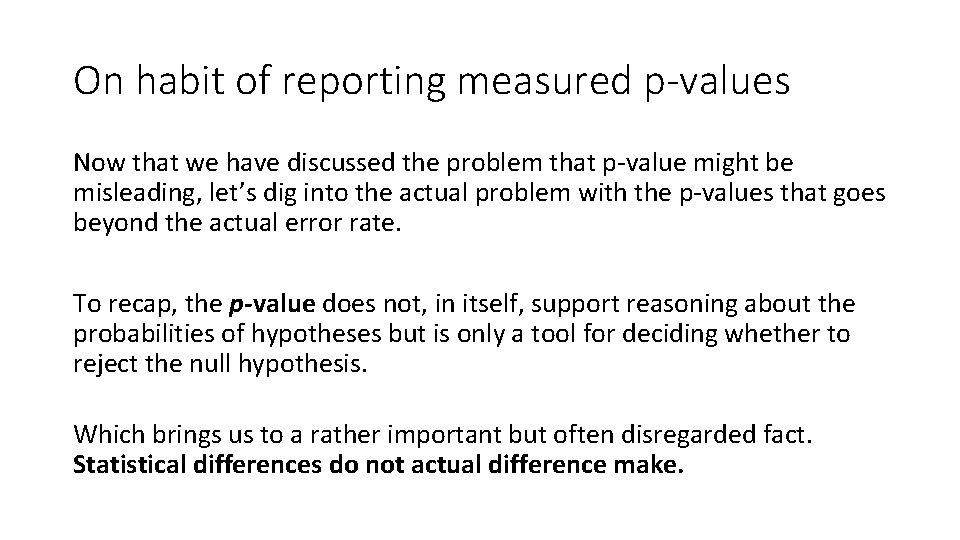
On habit of reporting measured p-values Now that we have discussed the problem that p-value might be misleading, let’s dig into the actual problem with the p-values that goes beyond the actual error rate. To recap, the p-value does not, in itself, support reasoning about the probabilities of hypotheses but is only a tool for deciding whether to reject the null hypothesis. Which brings us to a rather important but often disregarded fact. Statistical differences do not actual difference make.
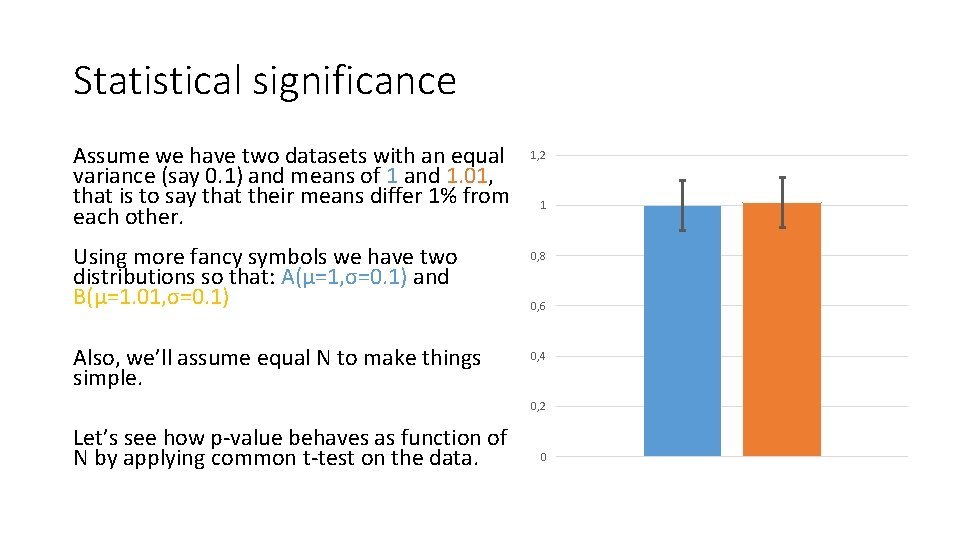
Statistical significance Assume we have two datasets with an equal variance (say 0. 1) and means of 1 and 1. 01, that is to say that their means differ 1% from each other. Using more fancy symbols we have two distributions so that: A(µ=1, σ=0. 1) and B(µ=1. 01, σ=0. 1) Also, we’ll assume equal N to make things simple. 1, 2 1 0, 8 0, 6 0, 4 0, 2 Let’s see how p-value behaves as function of N by applying common t-test on the data. 0
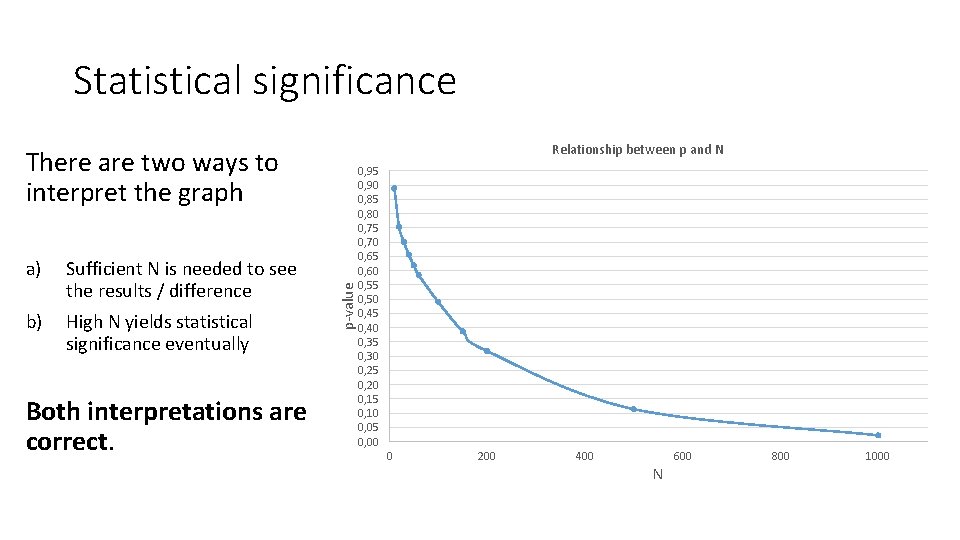
Statistical significance Relationship between p and N a) Sufficient N is needed to see the results / difference b) High N yields statistical significance eventually Both interpretations are correct. p-value There are two ways to interpret the graph 0, 95 0, 90 0, 85 0, 80 0, 75 0, 70 0, 65 0, 60 0, 55 0, 50 0, 45 0, 40 0, 35 0, 30 0, 25 0, 20 0, 15 0, 10 0, 05 0, 00 0 200 400 N 600 800 1000
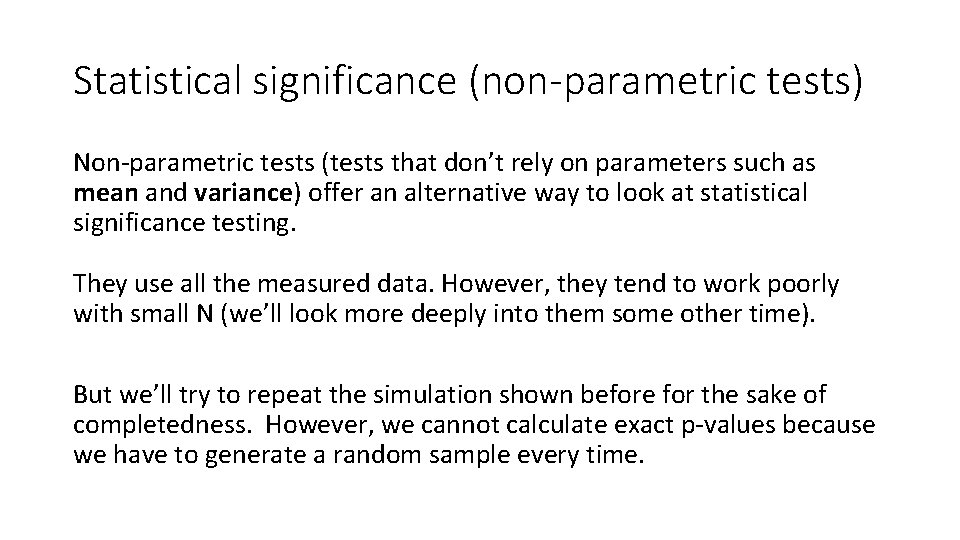
Statistical significance (non-parametric tests) Non-parametric tests (tests that don’t rely on parameters such as mean and variance) offer an alternative way to look at statistical significance testing. They use all the measured data. However, they tend to work poorly with small N (we’ll look more deeply into them some other time). But we’ll try to repeat the simulation shown before for the sake of completedness. However, we cannot calculate exact p-values because we have to generate a random sample every time.
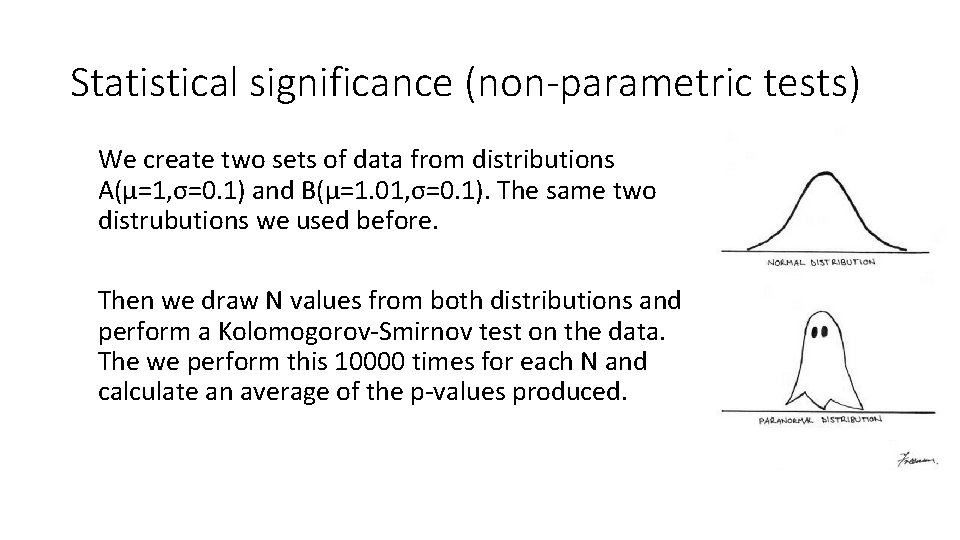
Statistical significance (non-parametric tests) We create two sets of data from distributions A(µ=1, σ=0. 1) and B(µ=1. 01, σ=0. 1). The same two distrubutions we used before. Then we draw N values from both distributions and perform a Kolomogorov-Smirnov test on the data. The we perform this 10000 times for each N and calculate an average of the p-values produced.
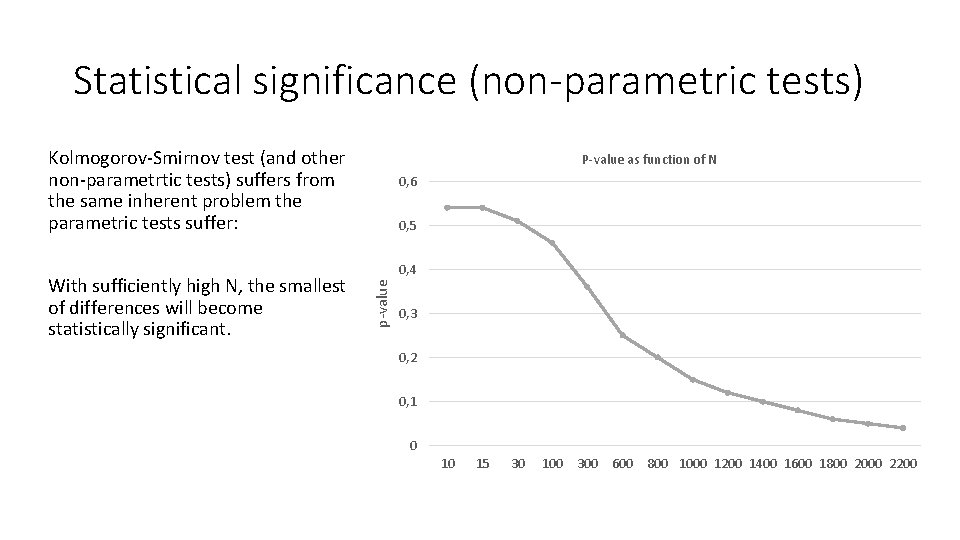
Statistical significance (non-parametric tests) Kolmogorov-Smirnov test (and other non-parametrtic tests) suffers from the same inherent problem the parametric tests suffer: 0, 6 0, 5 0, 4 p-value With sufficiently high N, the smallest of differences will become statistically significant. P-value as function of N 0, 3 0, 2 0, 1 0 10 15 30 100 300 600 800 1000 1200 1400 1600 1800 2000 2200
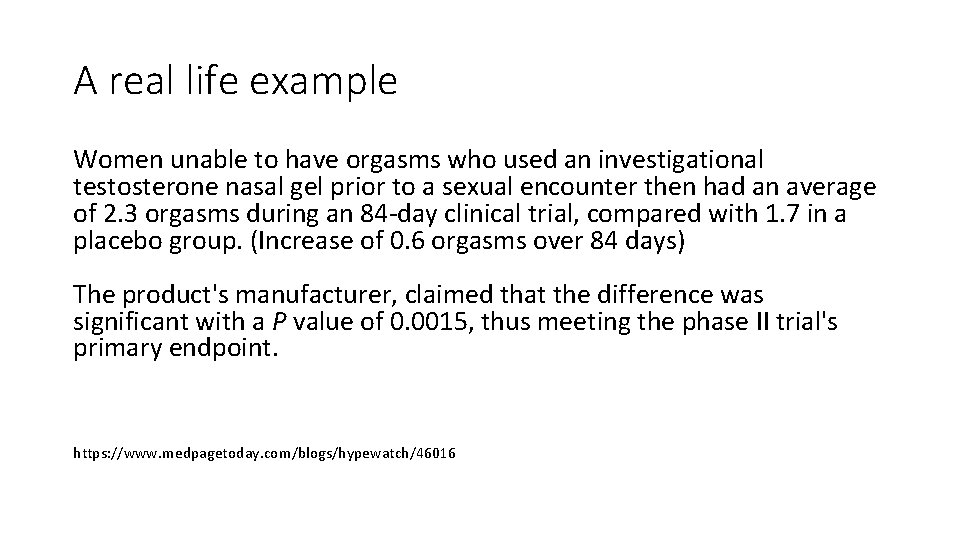
A real life example Women unable to have orgasms who used an investigational testosterone nasal gel prior to a sexual encounter then had an average of 2. 3 orgasms during an 84 -day clinical trial, compared with 1. 7 in a placebo group. (Increase of 0. 6 orgasms over 84 days) The product's manufacturer, claimed that the difference was significant with a P value of 0. 0015, thus meeting the phase II trial's primary endpoint. https: //www. medpagetoday. com/blogs/hypewatch/46016
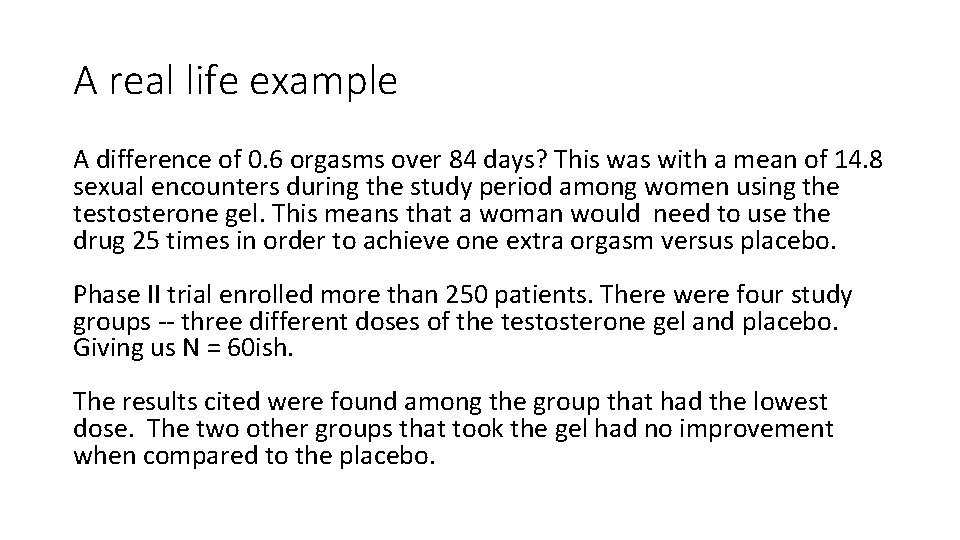
A real life example A difference of 0. 6 orgasms over 84 days? This was with a mean of 14. 8 sexual encounters during the study period among women using the testosterone gel. This means that a woman would need to use the drug 25 times in order to achieve one extra orgasm versus placebo. Phase II trial enrolled more than 250 patients. There were four study groups -- three different doses of the testosterone gel and placebo. Giving us N = 60 ish. The results cited were found among the group that had the lowest dose. The two other groups that took the gel had no improvement when compared to the placebo.
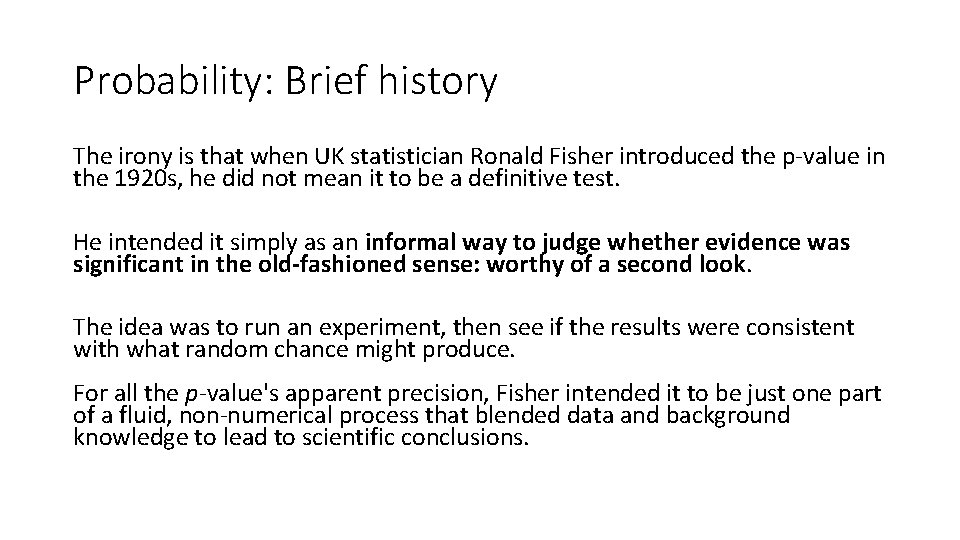
Probability: Brief history The irony is that when UK statistician Ronald Fisher introduced the p-value in the 1920 s, he did not mean it to be a definitive test. He intended it simply as an informal way to judge whether evidence was significant in the old-fashioned sense: worthy of a second look. The idea was to run an experiment, then see if the results were consistent with what random chance might produce. For all the p-value's apparent precision, Fisher intended it to be just one part of a fluid, non-numerical process that blended data and background knowledge to lead to scientific conclusions.
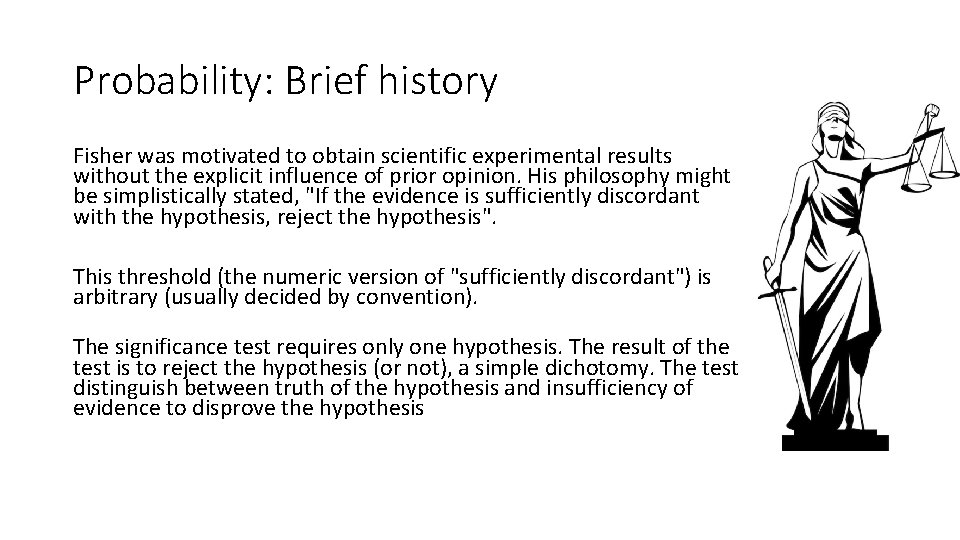
Probability: Brief history Fisher was motivated to obtain scientific experimental results without the explicit influence of prior opinion. His philosophy might be simplistically stated, "If the evidence is sufficiently discordant with the hypothesis, reject the hypothesis". This threshold (the numeric version of "sufficiently discordant") is arbitrary (usually decided by convention). The significance test requires only one hypothesis. The result of the test is to reject the hypothesis (or not), a simple dichotomy. The test distinguish between truth of the hypothesis and insufficiency of evidence to disprove the hypothesis
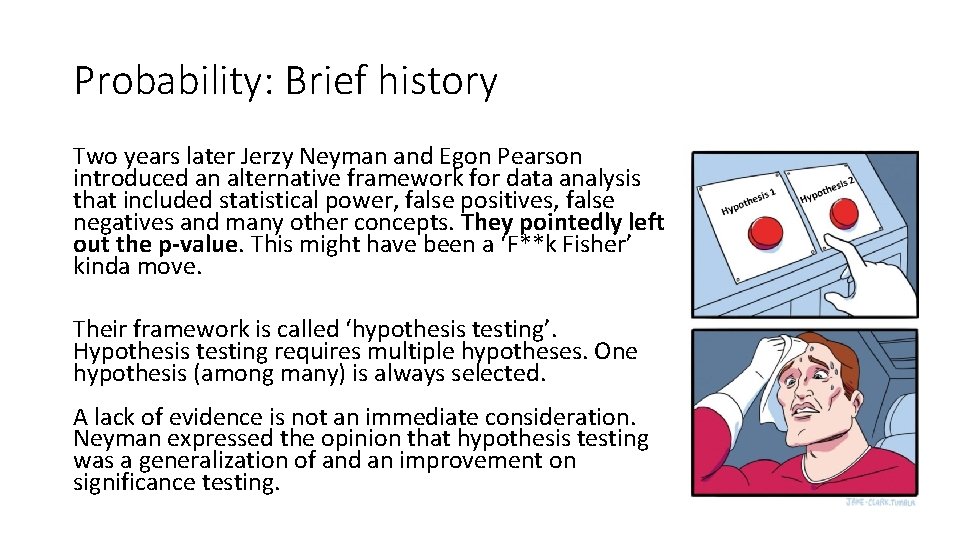
Probability: Brief history Two years later Jerzy Neyman and Egon Pearson introduced an alternative framework for data analysis that included statistical power, false positives, false negatives and many other concepts. They pointedly left out the p-value. This might have been a ‘F**k Fisher’ kinda move. Their framework is called ‘hypothesis testing’. Hypothesis testing requires multiple hypotheses. One hypothesis (among many) is always selected. A lack of evidence is not an immediate consideration. Neyman expressed the opinion that hypothesis testing was a generalization of and an improvement on significance testing.
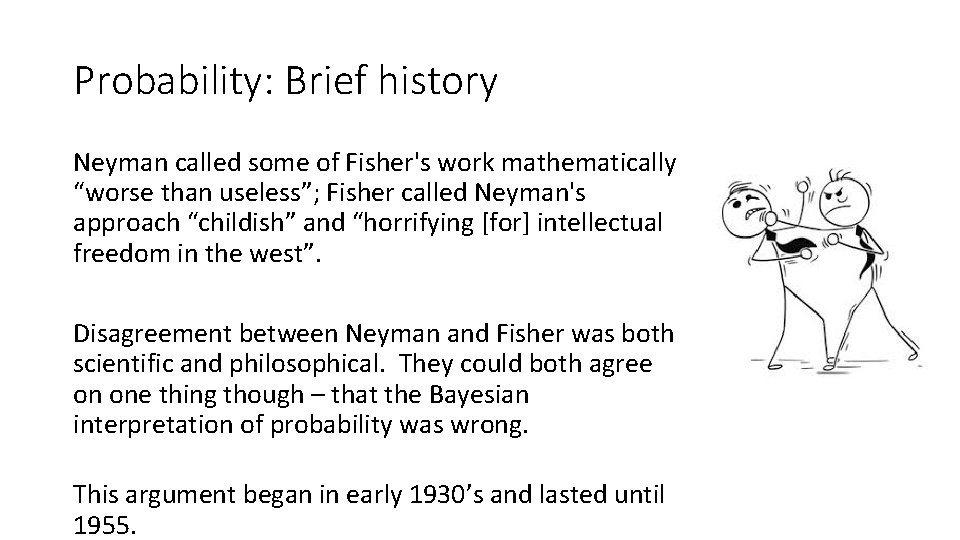
Probability: Brief history Neyman called some of Fisher's work mathematically “worse than useless”; Fisher called Neyman's approach “childish” and “horrifying [for] intellectual freedom in the west”. Disagreement between Neyman and Fisher was both scientific and philosophical. They could both agree on one thing though – that the Bayesian interpretation of probability was wrong. This argument began in early 1930’s and lasted until 1955.
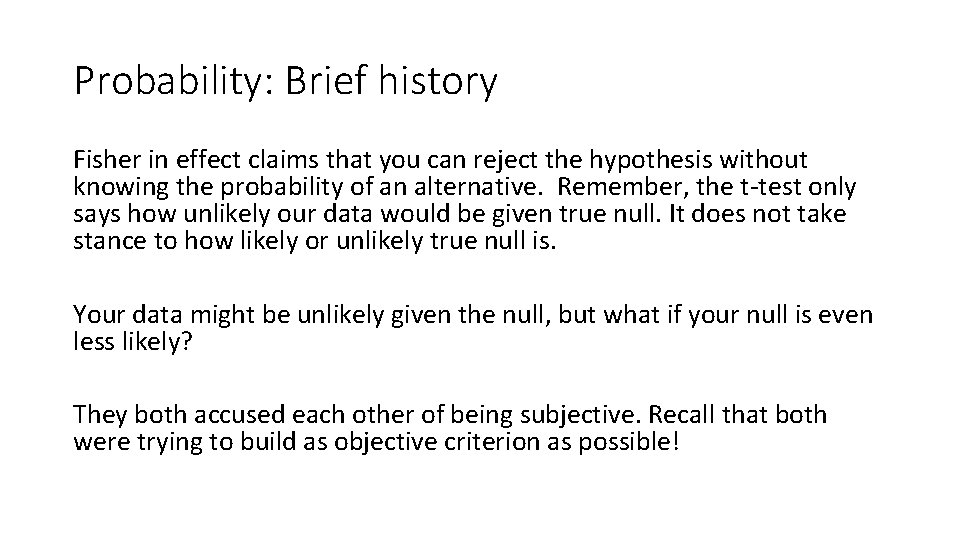
Probability: Brief history Fisher in effect claims that you can reject the hypothesis without knowing the probability of an alternative. Remember, the t-test only says how unlikely our data would be given true null. It does not take stance to how likely or unlikely true null is. Your data might be unlikely given the null, but what if your null is even less likely? They both accused each other of being subjective. Recall that both were trying to build as objective criterion as possible!
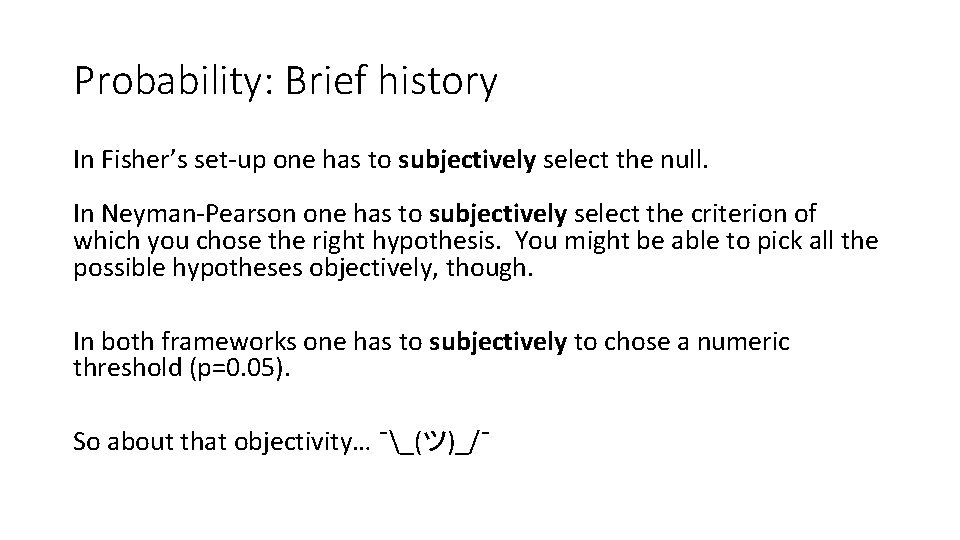
Probability: Brief history In Fisher’s set-up one has to subjectively select the null. In Neyman-Pearson one has to subjectively select the criterion of which you chose the right hypothesis. You might be able to pick all the possible hypotheses objectively, though. In both frameworks one has to subjectively to chose a numeric threshold (p=0. 05). So about that objectivity… ¯_(ツ)_/¯
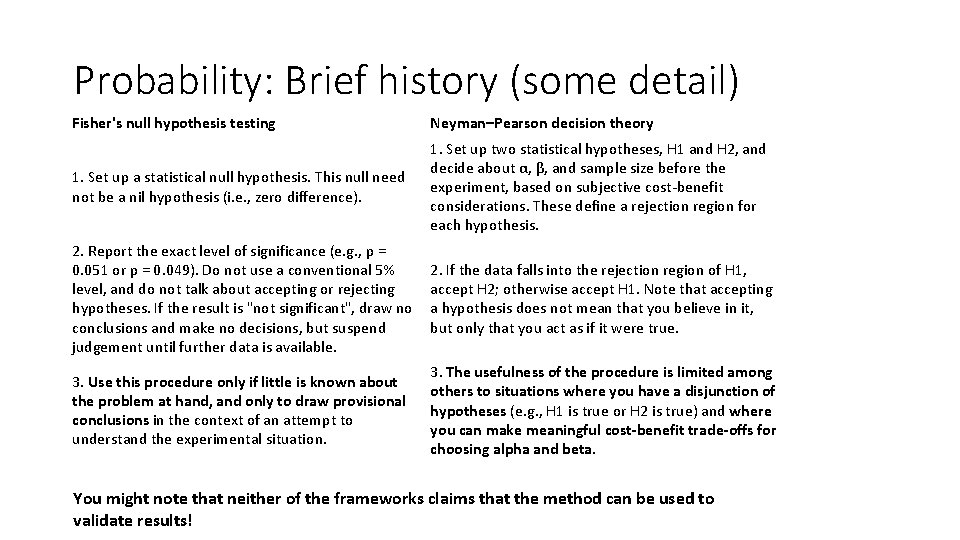
Probability: Brief history (some detail) Fisher's null hypothesis testing Neyman–Pearson decision theory 1. Set up a statistical null hypothesis. This null need not be a nil hypothesis (i. e. , zero difference). 1. Set up two statistical hypotheses, H 1 and H 2, and decide about α, β, and sample size before the experiment, based on subjective cost-benefit considerations. These define a rejection region for each hypothesis. 2. Report the exact level of significance (e. g. , p = 0. 051 or p = 0. 049). Do not use a conventional 5% level, and do not talk about accepting or rejecting hypotheses. If the result is "not significant", draw no conclusions and make no decisions, but suspend judgement until further data is available. 2. If the data falls into the rejection region of H 1, accept H 2; otherwise accept H 1. Note that accepting a hypothesis does not mean that you believe in it, but only that you act as if it were true. 3. Use this procedure only if little is known about the problem at hand, and only to draw provisional conclusions in the context of an attempt to understand the experimental situation. 3. The usefulness of the procedure is limited among others to situations where you have a disjunction of hypotheses (e. g. , H 1 is true or H 2 is true) and where you can make meaningful cost-benefit trade-offs for choosing alpha and beta. You might note that neither of the frameworks claims that the method can be used to validate results!
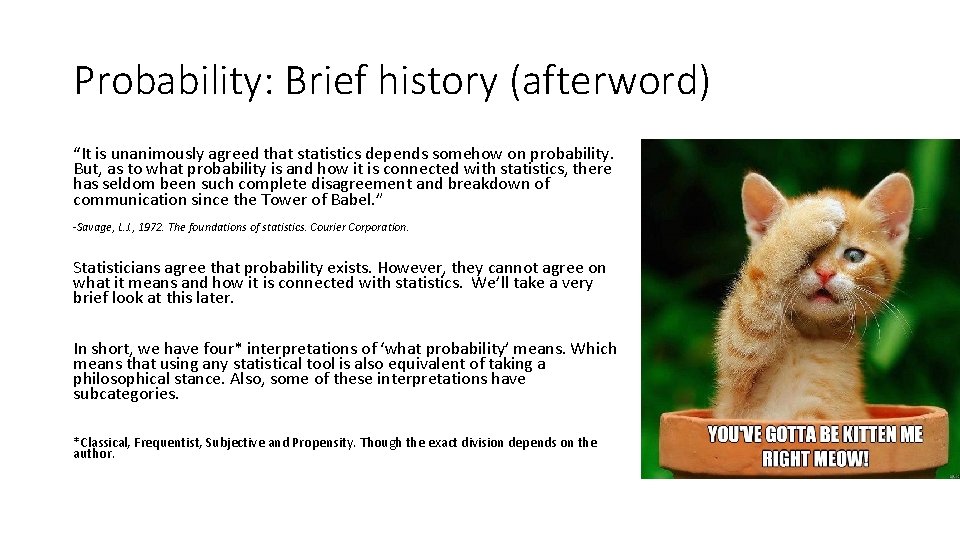
Probability: Brief history (afterword) “It is unanimously agreed that statistics depends somehow on probability. But, as to what probability is and how it is connected with statistics, there has seldom been such complete disagreement and breakdown of communication since the Tower of Babel. ” -Savage, L. J. , 1972. The foundations of statistics. Courier Corporation. Statisticians agree that probability exists. However, they cannot agree on what it means and how it is connected with statistics. We’ll take a very brief look at this later. In short, we have four* interpretations of ‘what probability’ means. Which means that using any statistical tool is also equivalent of taking a philosophical stance. Also, some of these interpretations have subcategories. *Classical, Frequentist, Subjective and Propensity. Though the exact division depends on the author.
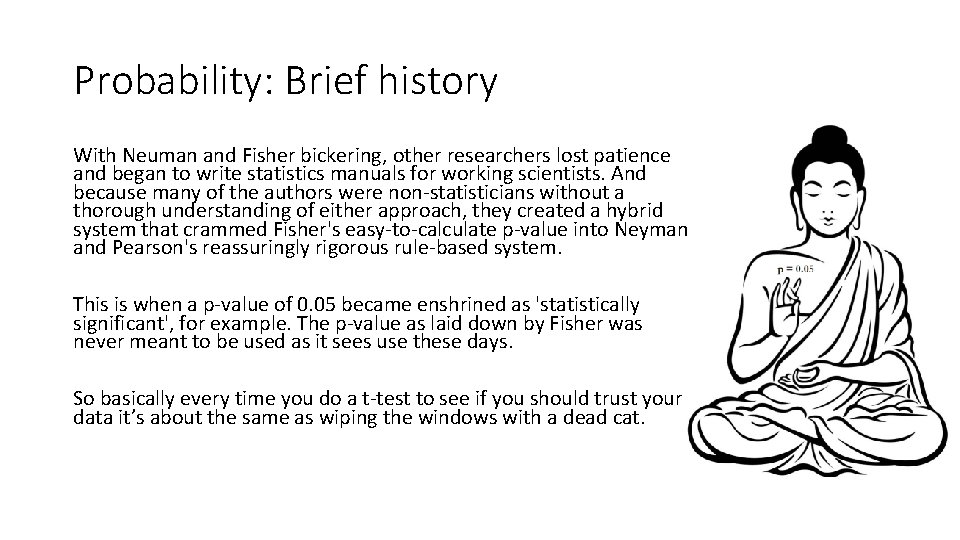
Probability: Brief history With Neuman and Fisher bickering, other researchers lost patience and began to write statistics manuals for working scientists. And because many of the authors were non-statisticians without a thorough understanding of either approach, they created a hybrid system that crammed Fisher's easy-to-calculate p-value into Neyman and Pearson's reassuringly rigorous rule-based system. This is when a p-value of 0. 05 became enshrined as 'statistically significant', for example. The p-value as laid down by Fisher was never meant to be used as it sees use these days. So basically every time you do a t-test to see if you should trust your data it’s about the same as wiping the windows with a dead cat.
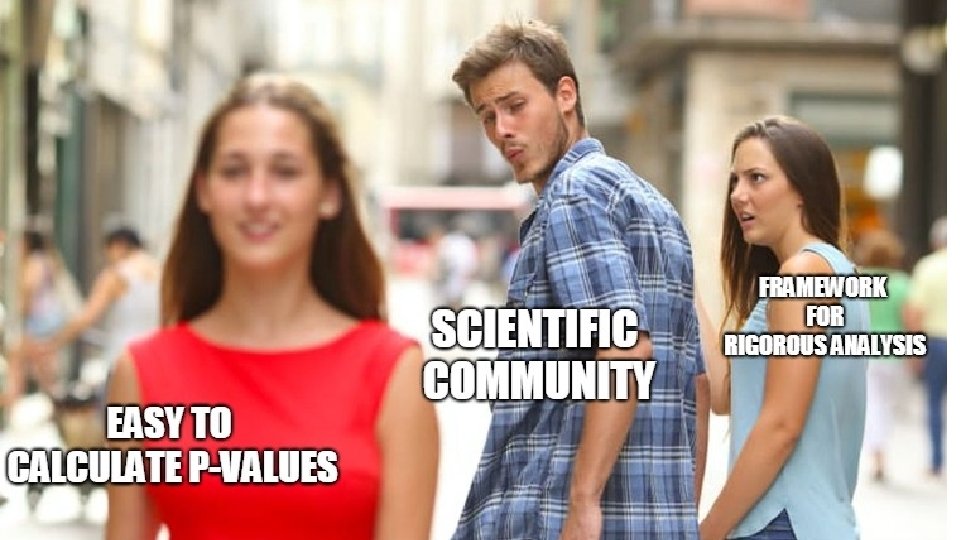
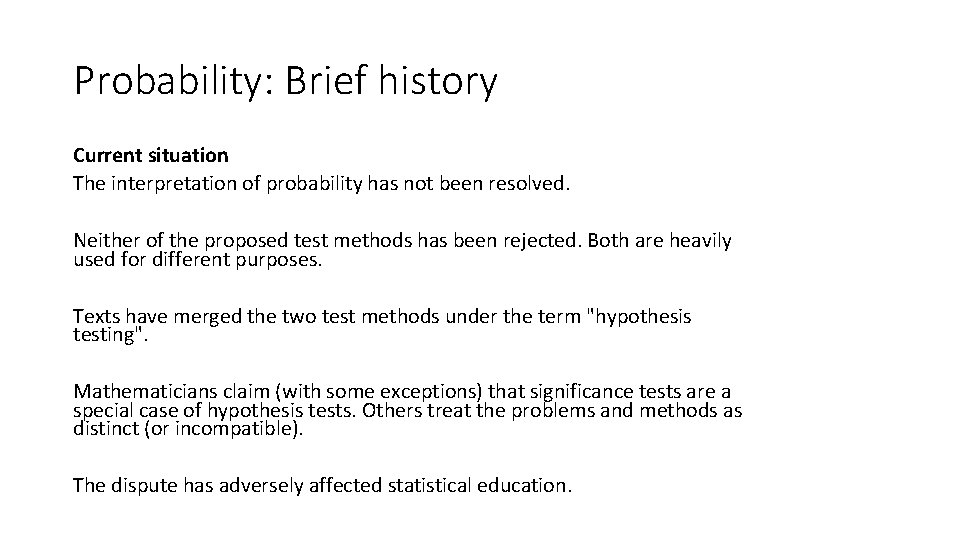
Probability: Brief history Current situation The interpretation of probability has not been resolved. Neither of the proposed test methods has been rejected. Both are heavily used for different purposes. Texts have merged the two test methods under the term "hypothesis testing". Mathematicians claim (with some exceptions) that significance tests are a special case of hypothesis tests. Others treat the problems and methods as distinct (or incompatible). The dispute has adversely affected statistical education.
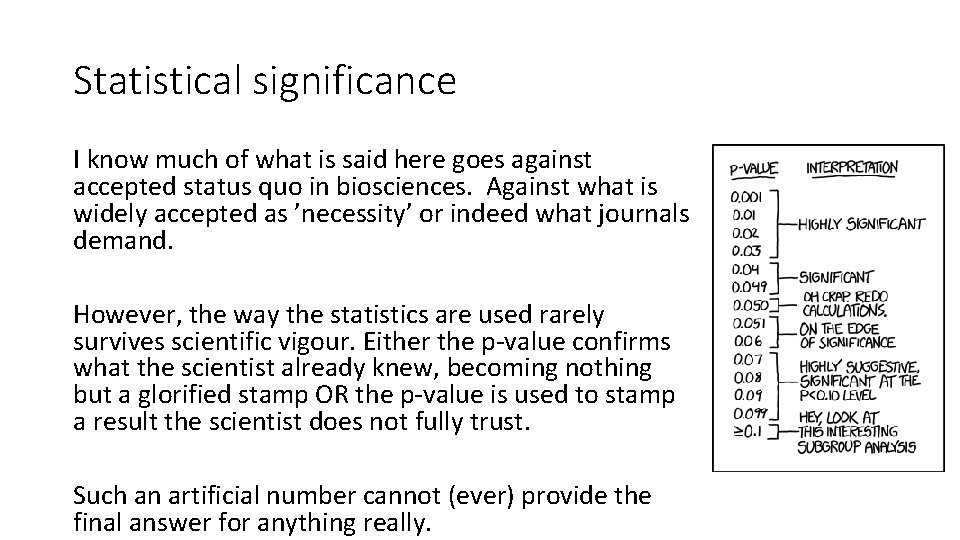
Statistical significance I know much of what is said here goes against accepted status quo in biosciences. Against what is widely accepted as ’necessity’ or indeed what journals demand. However, the way the statistics are used rarely survives scientific vigour. Either the p-value confirms what the scientist already knew, becoming nothing but a glorified stamp OR the p-value is used to stamp a result the scientist does not fully trust. Such an artificial number cannot (ever) provide the final answer for anything really.
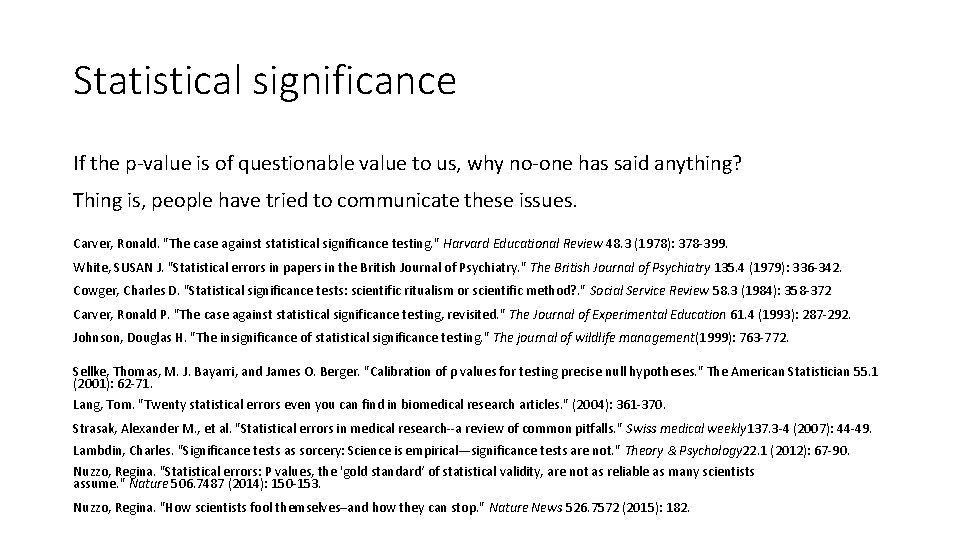
Statistical significance If the p-value is of questionable value to us, why no-one has said anything? Thing is, people have tried to communicate these issues. Carver, Ronald. "The case against statistical significance testing. " Harvard Educational Review 48. 3 (1978): 378 -399. White, SUSAN J. "Statistical errors in papers in the British Journal of Psychiatry. " The British Journal of Psychiatry 135. 4 (1979): 336 -342. Cowger, Charles D. "Statistical significance tests: scientific ritualism or scientific method? . " Social Service Review 58. 3 (1984): 358 -372 Carver, Ronald P. "The case against statistical significance testing, revisited. " The Journal of Experimental Education 61. 4 (1993): 287 -292. Johnson, Douglas H. "The insignificance of statistical significance testing. " The journal of wildlife management(1999): 763 -772. Sellke, Thomas, M. J. Bayarri, and James O. Berger. "Calibration of ρ values for testing precise null hypotheses. " The American Statistician 55. 1 (2001): 62 -71. Lang, Tom. "Twenty statistical errors even you can find in biomedical research articles. " (2004): 361 -370. Strasak, Alexander M. , et al. "Statistical errors in medical research--a review of common pitfalls. " Swiss medical weekly 137. 3 -4 (2007): 44 -49. Lambdin, Charles. "Significance tests as sorcery: Science is empirical—significance tests are not. " Theory & Psychology 22. 1 (2012): 67 -90. Nuzzo, Regina. "Statistical errors: P values, the 'gold standard‘ of statistical validity, are not as reliable as many scientists assume. " Nature 506. 7487 (2014): 150 -153. Nuzzo, Regina. "How scientists fool themselves–and how they can stop. " Nature News 526. 7572 (2015): 182.
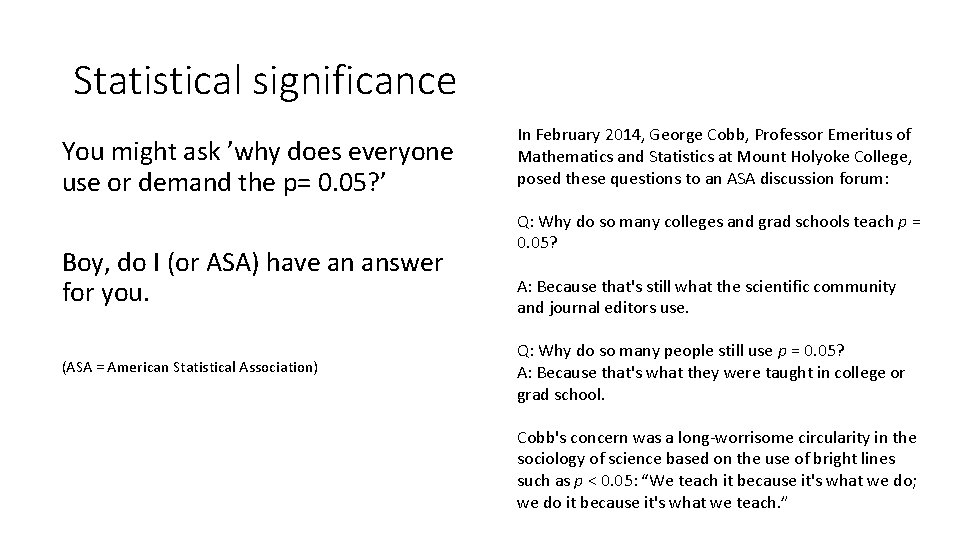
Statistical significance You might ask ’why does everyone use or demand the p= 0. 05? ’ Boy, do I (or ASA) have an answer for you. (ASA = American Statistical Association) In February 2014, George Cobb, Professor Emeritus of Mathematics and Statistics at Mount Holyoke College, posed these questions to an ASA discussion forum: Q: Why do so many colleges and grad schools teach p = 0. 05? A: Because that's still what the scientific community and journal editors use. Q: Why do so many people still use p = 0. 05? A: Because that's what they were taught in college or grad school. Cobb's concern was a long-worrisome circularity in the sociology of science based on the use of bright lines such as p < 0. 05: “We teach it because it's what we do; we do it because it's what we teach. ”
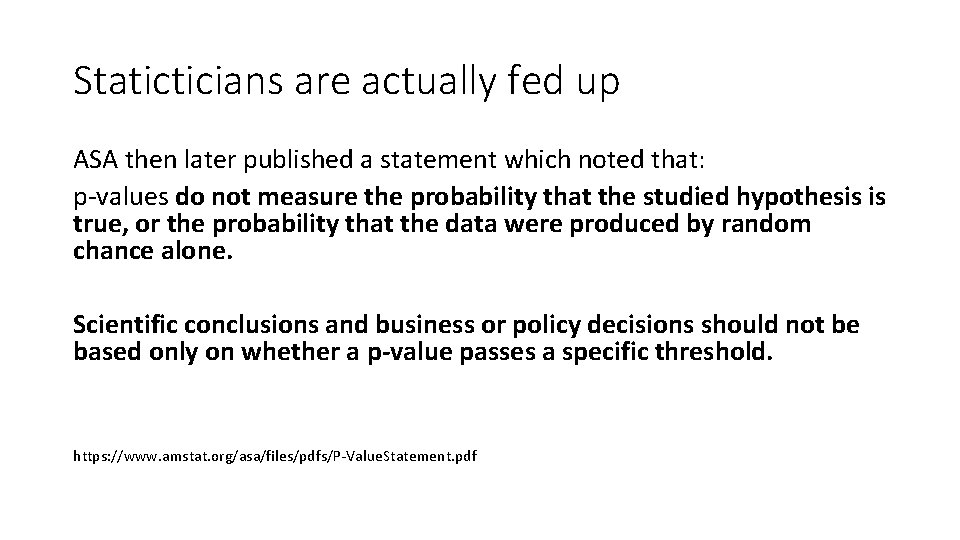
Staticticians are actually fed up ASA then later published a statement which noted that: p-values do not measure the probability that the studied hypothesis is true, or the probability that the data were produced by random chance alone. Scientific conclusions and business or policy decisions should not be based only on whether a p-value passes a specific threshold. https: //www. amstat. org/asa/files/pdfs/P-Value. Statement. pdf
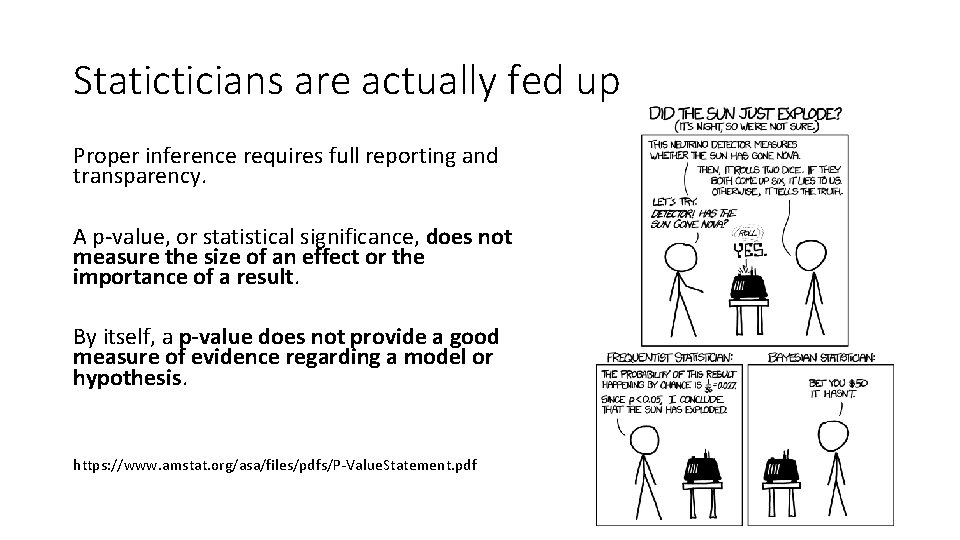
Staticticians are actually fed up Proper inference requires full reporting and transparency. A p-value, or statistical significance, does not measure the size of an effect or the importance of a result. By itself, a p-value does not provide a good measure of evidence regarding a model or hypothesis. https: //www. amstat. org/asa/files/pdfs/P-Value. Statement. pdf
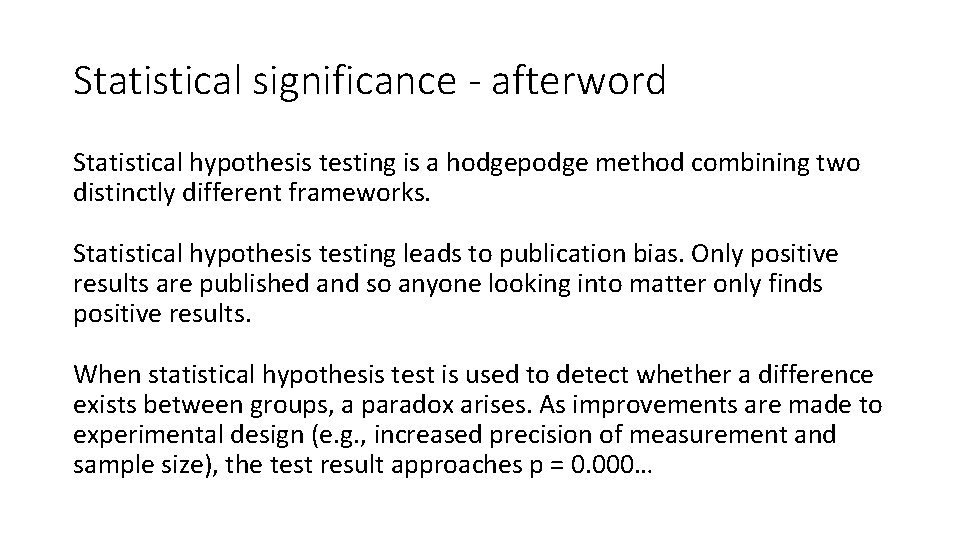
Statistical significance - afterword Statistical hypothesis testing is a hodgepodge method combining two distinctly different frameworks. Statistical hypothesis testing leads to publication bias. Only positive results are published and so anyone looking into matter only finds positive results. When statistical hypothesis test is used to detect whether a difference exists between groups, a paradox arises. As improvements are made to experimental design (e. g. , increased precision of measurement and sample size), the test result approaches p = 0. 000…
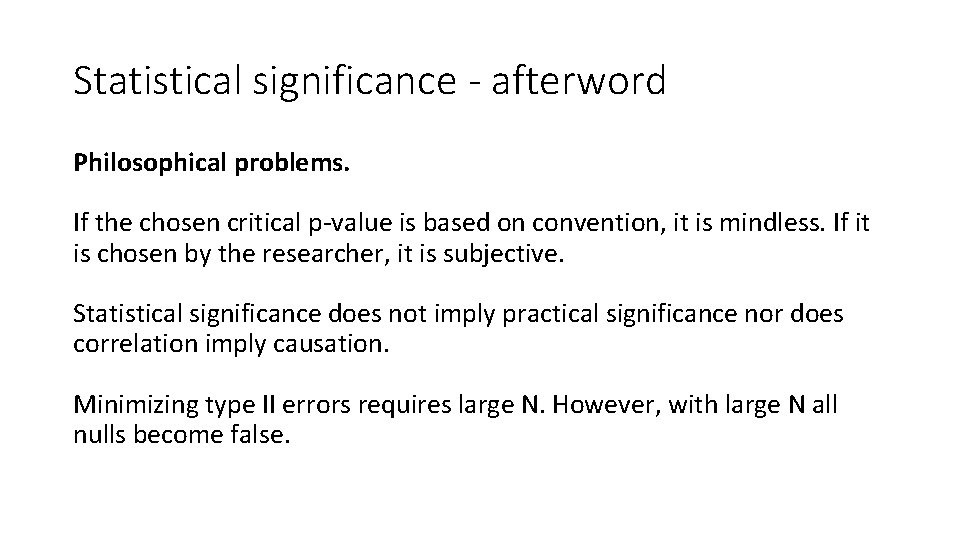
Statistical significance - afterword Philosophical problems. If the chosen critical p-value is based on convention, it is mindless. If it is chosen by the researcher, it is subjective. Statistical significance does not imply practical significance nor does correlation imply causation. Minimizing type II errors requires large N. However, with large N all nulls become false.
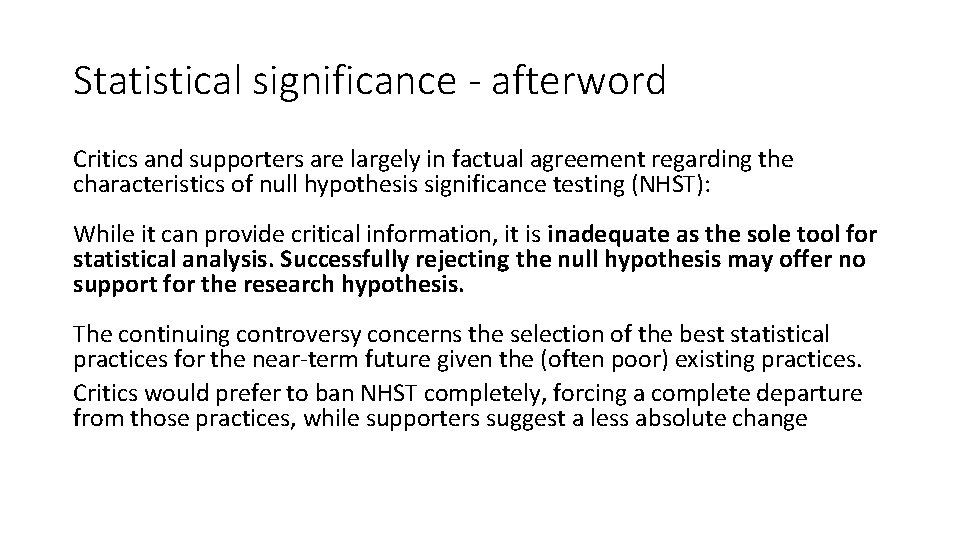
Statistical significance - afterword Critics and supporters are largely in factual agreement regarding the characteristics of null hypothesis significance testing (NHST): While it can provide critical information, it is inadequate as the sole tool for statistical analysis. Successfully rejecting the null hypothesis may offer no support for the research hypothesis. The continuing controversy concerns the selection of the best statistical practices for the near-term future given the (often poor) existing practices. Critics would prefer to ban NHST completely, forcing a complete departure from those practices, while supporters suggest a less absolute change
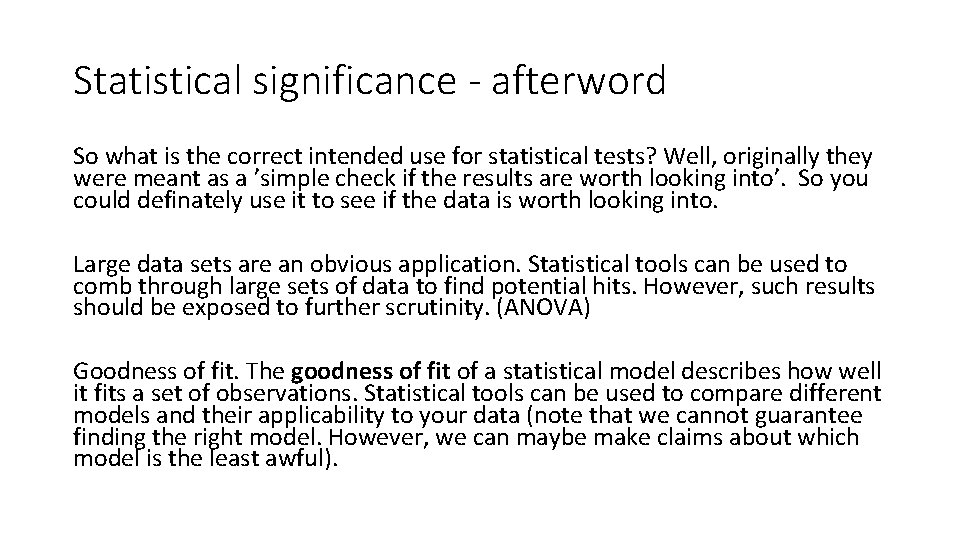
Statistical significance - afterword So what is the correct intended use for statistical tests? Well, originally they were meant as a ’simple check if the results are worth looking into’. So you could definately use it to see if the data is worth looking into. Large data sets are an obvious application. Statistical tools can be used to comb through large sets of data to find potential hits. However, such results should be exposed to further scrutinity. (ANOVA) Goodness of fit. The goodness of fit of a statistical model describes how well it fits a set of observations. Statistical tools can be used to compare different models and their applicability to your data (note that we cannot guarantee finding the right model. However, we can maybe make claims about which model is the least awful).
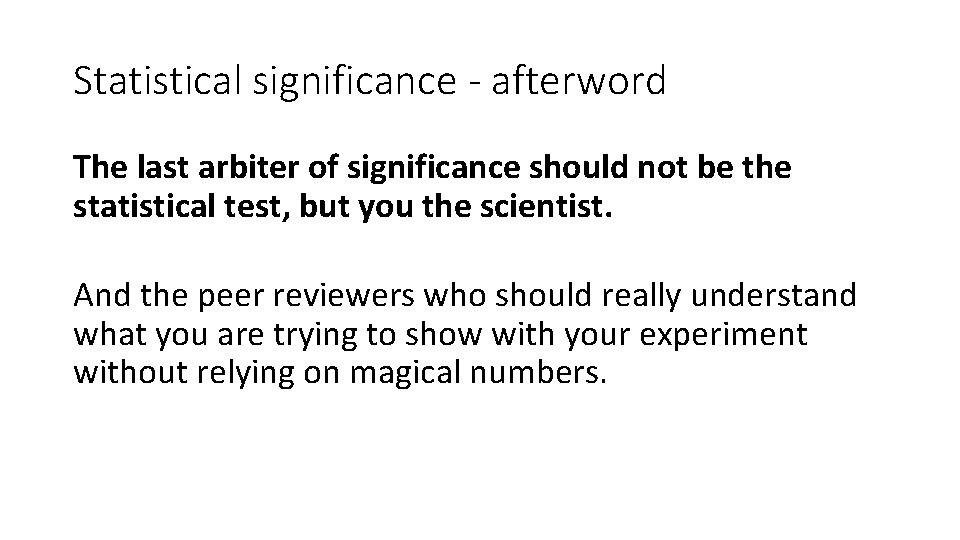
Statistical significance - afterword The last arbiter of significance should not be the statistical test, but you the scientist. And the peer reviewers who should really understand what you are trying to show with your experiment without relying on magical numbers.
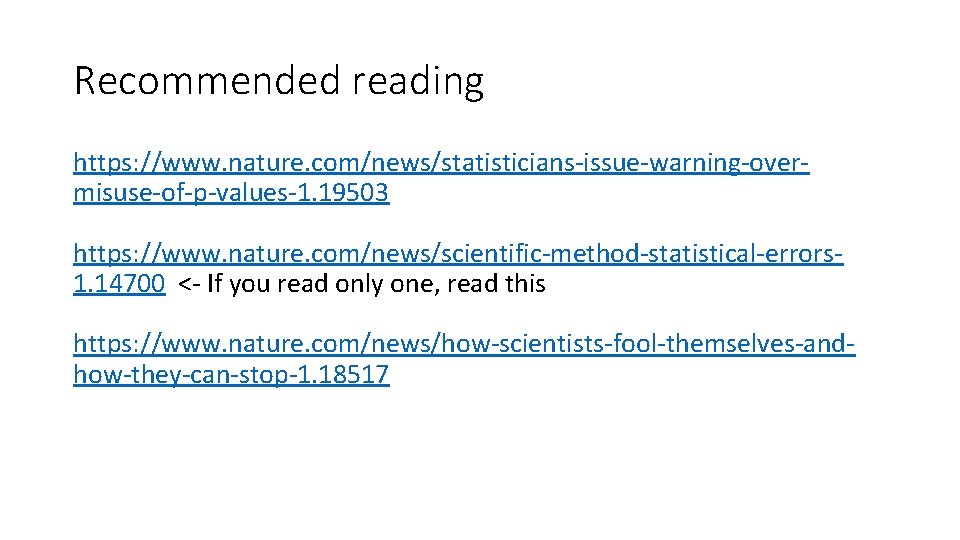
Recommended reading https: //www. nature. com/news/statisticians-issue-warning-overmisuse-of-p-values-1. 19503 https: //www. nature. com/news/scientific-method-statistical-errors 1. 14700 <- If you read only one, read this https: //www. nature. com/news/how-scientists-fool-themselves-andhow-they-can-stop-1. 18517
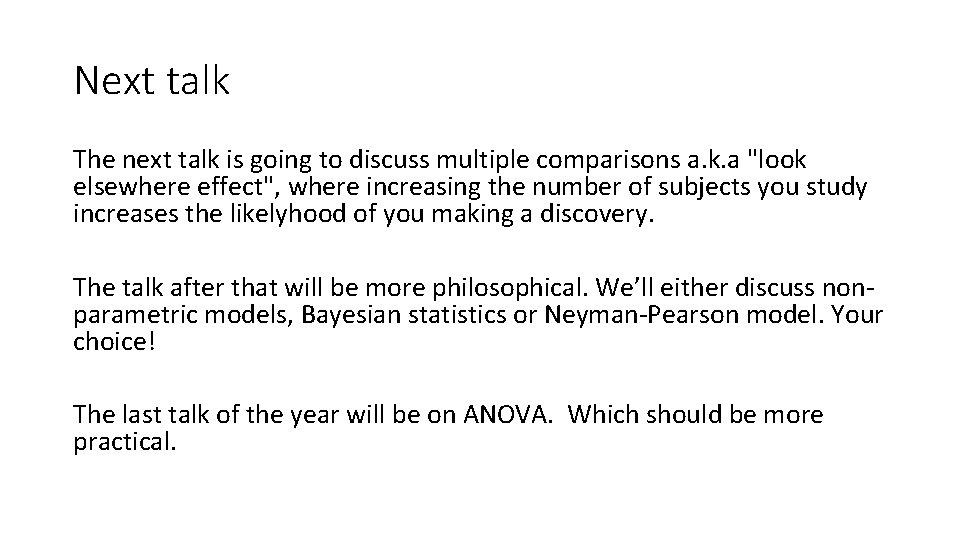
Next talk The next talk is going to discuss multiple comparisons a. k. a "look elsewhere effect", where increasing the number of subjects you study increases the likelyhood of you making a discovery. The talk after that will be more philosophical. We’ll either discuss nonparametric models, Bayesian statistics or Neyman-Pearson model. Your choice! The last talk of the year will be on ANOVA. Which should be more practical.