WHAT IS DATA MINING Data Mining is An
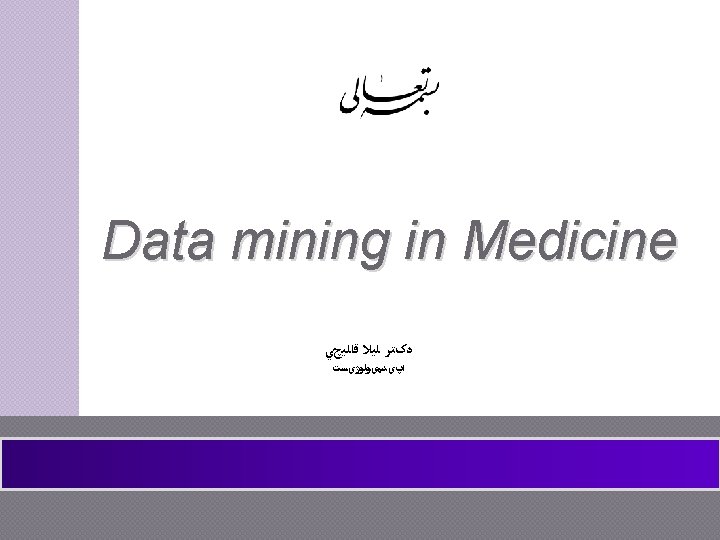
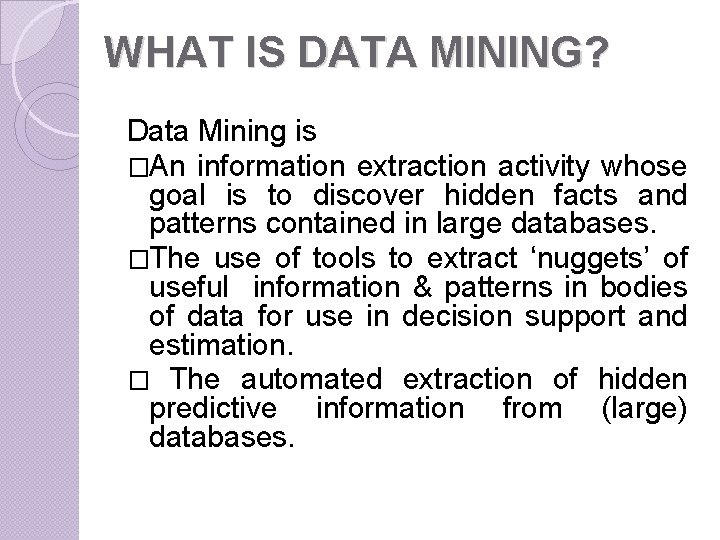
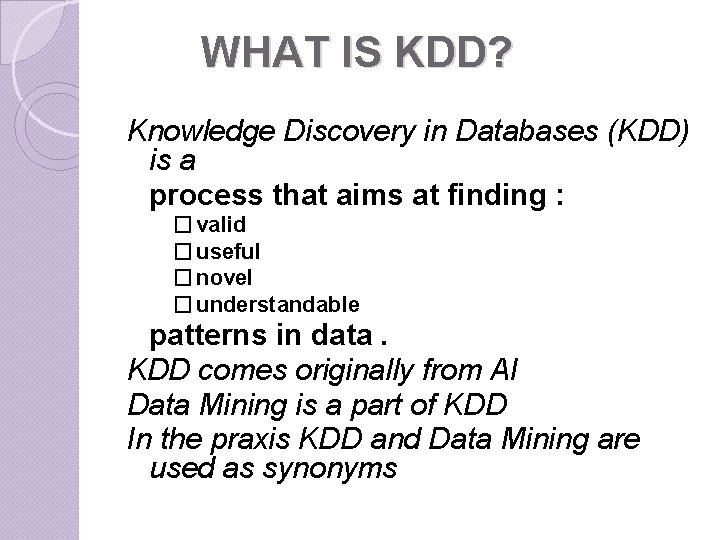
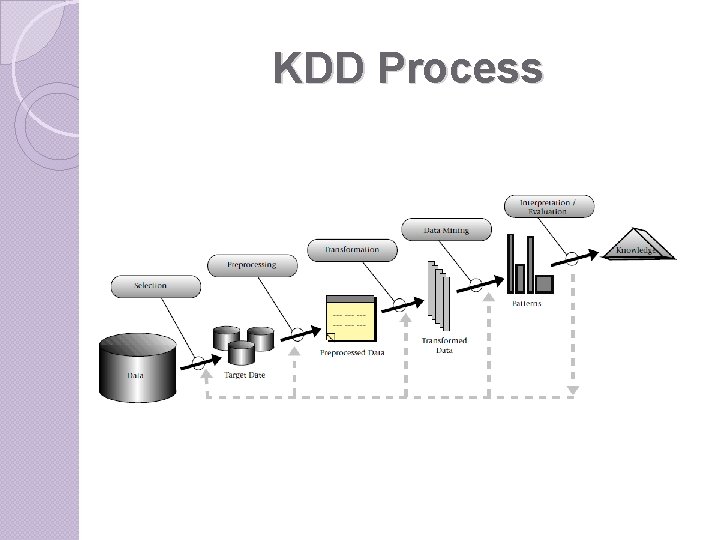
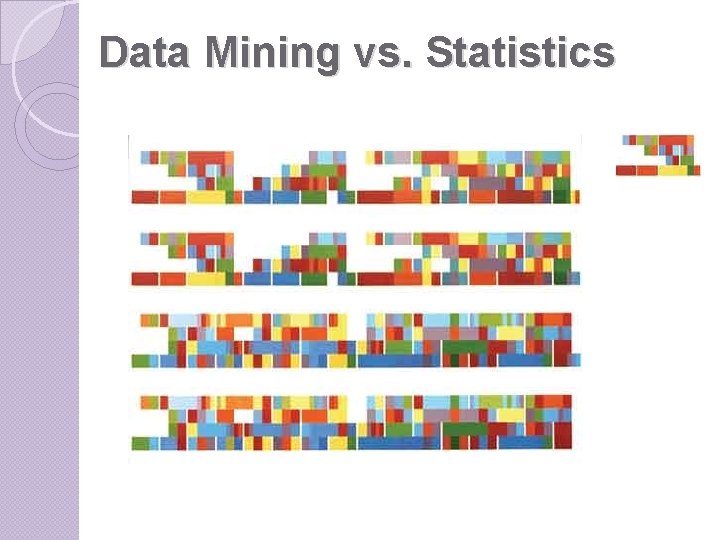
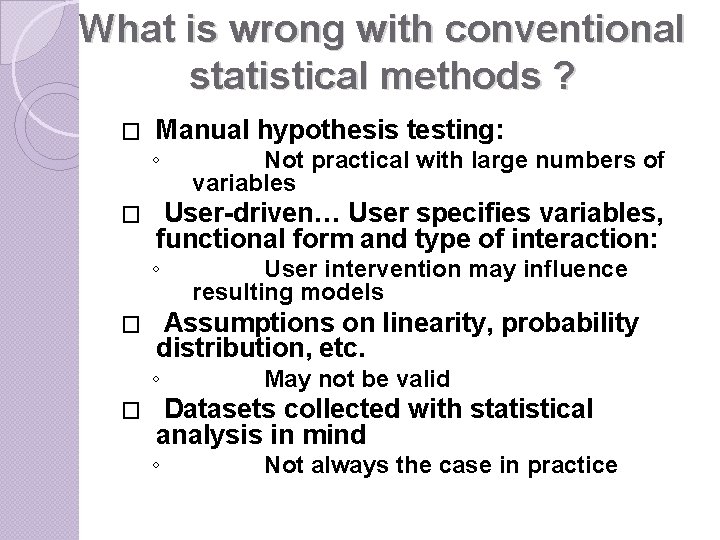
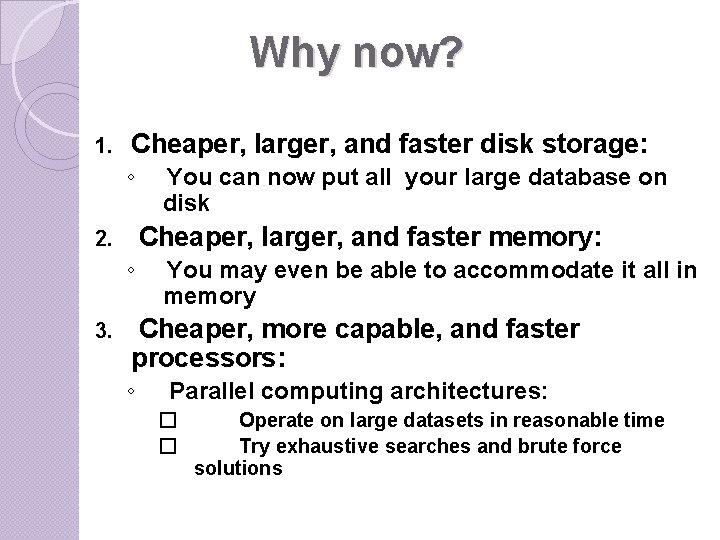
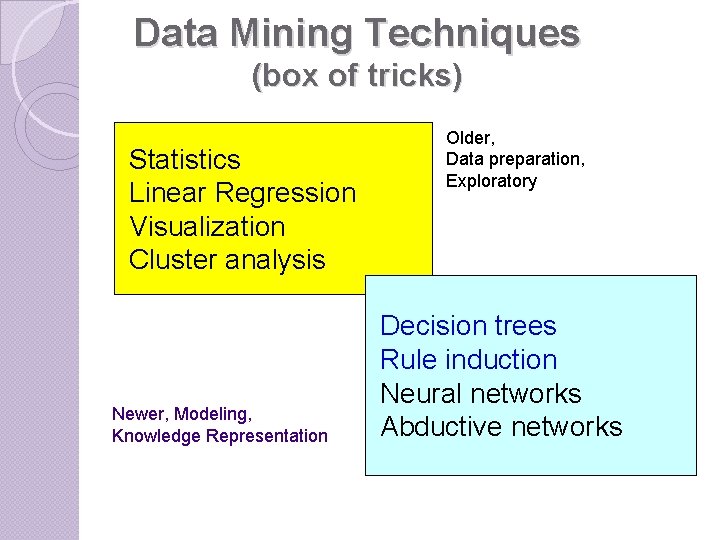
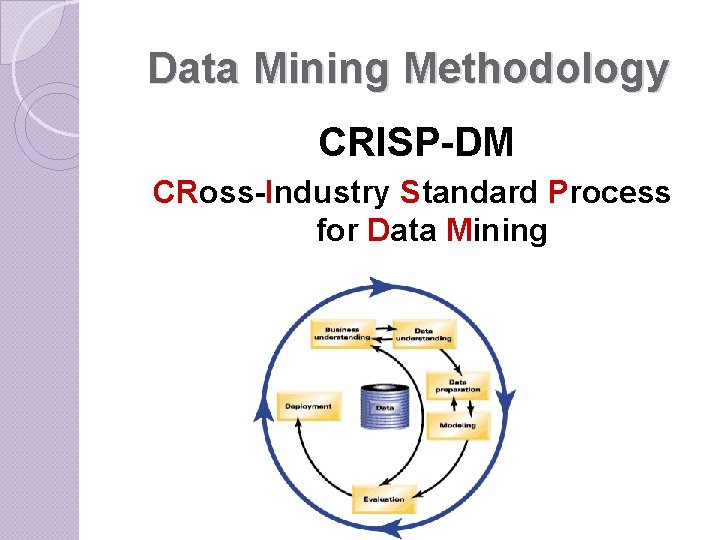
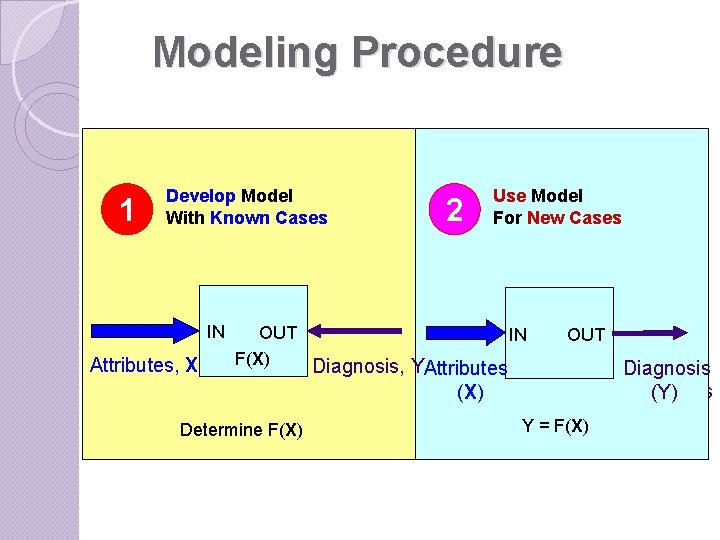
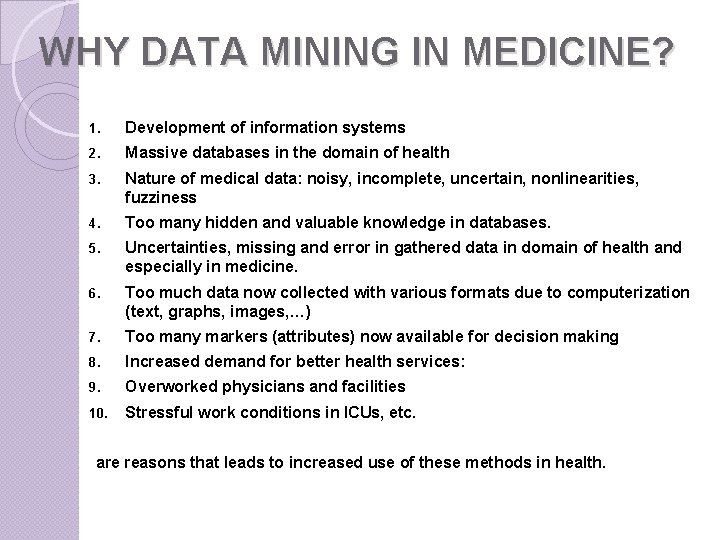
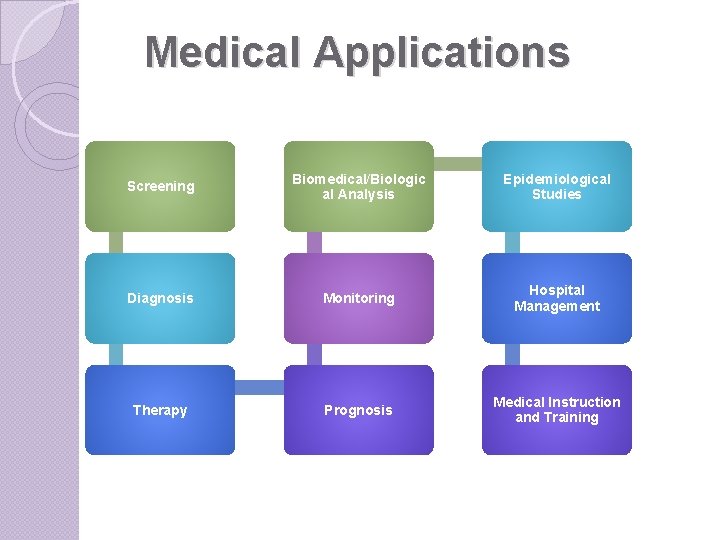
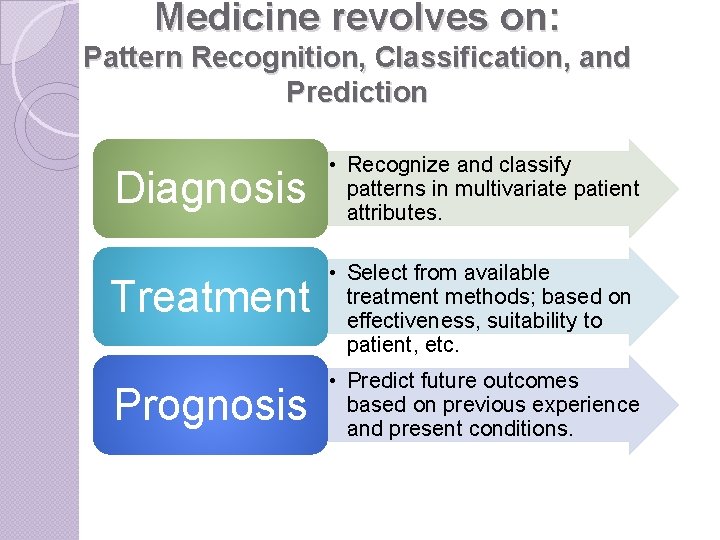
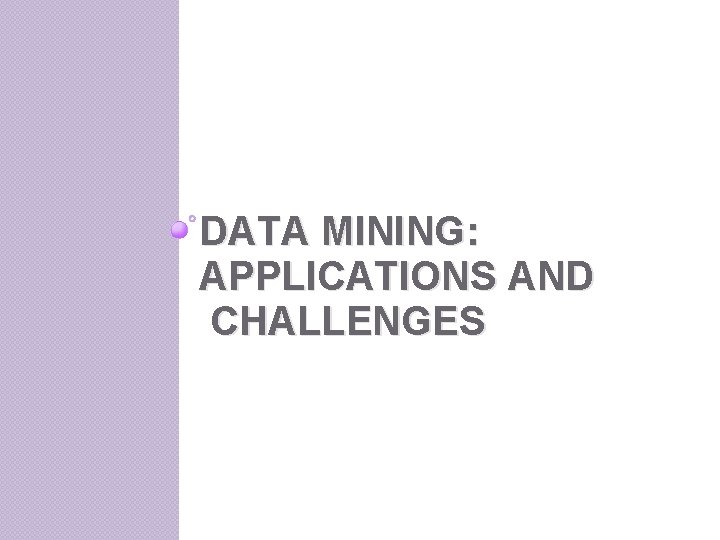
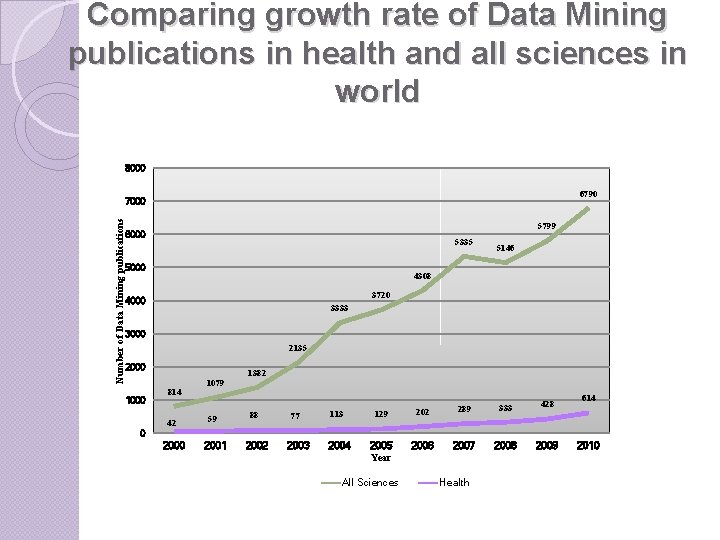
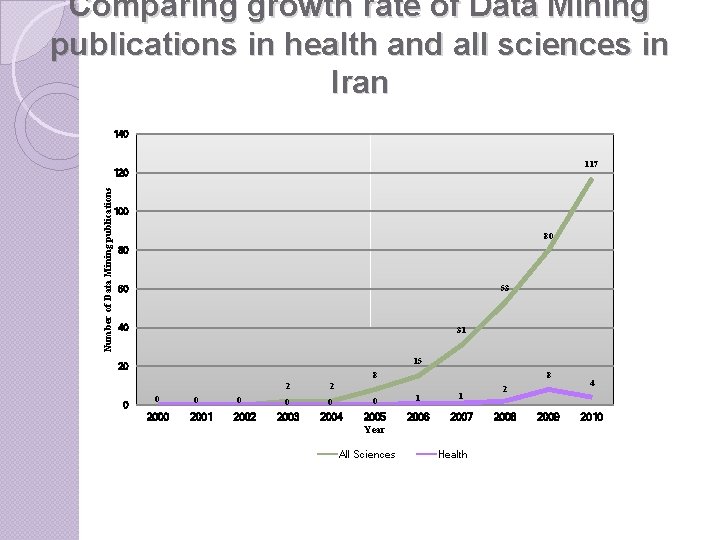
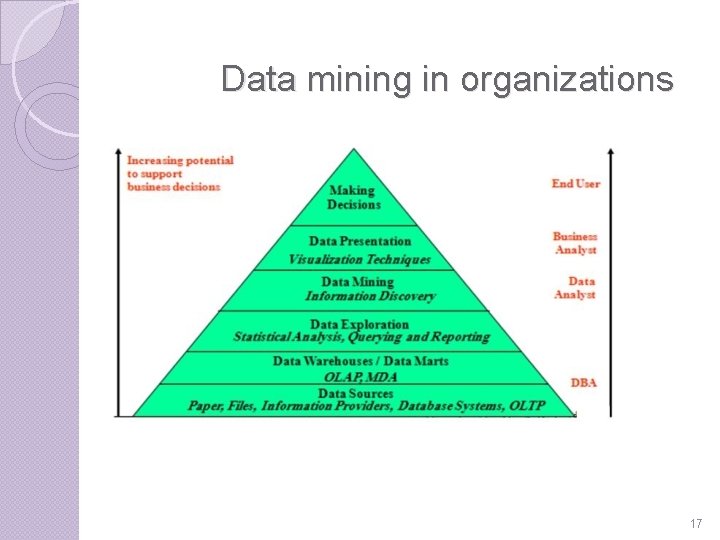
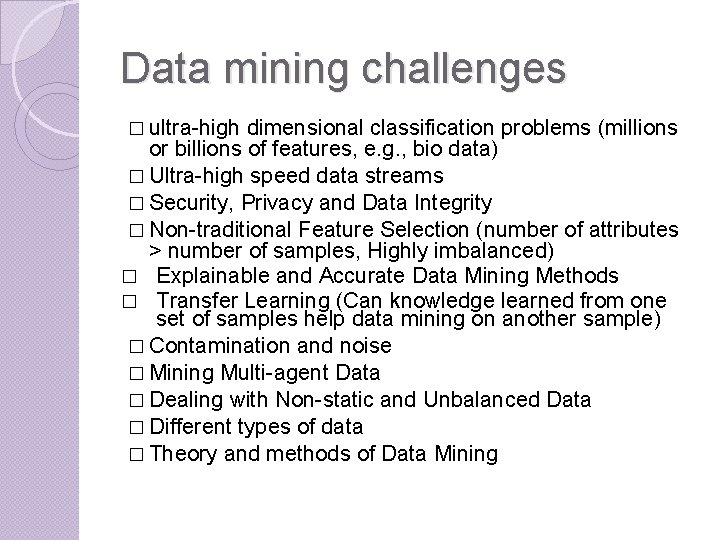
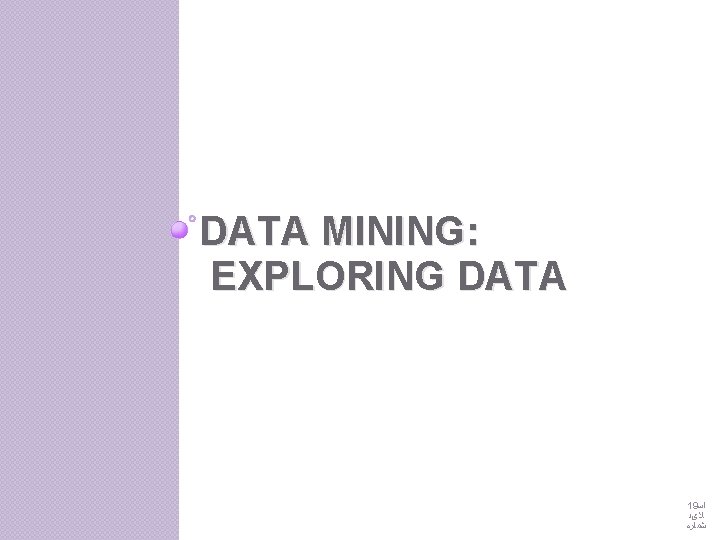
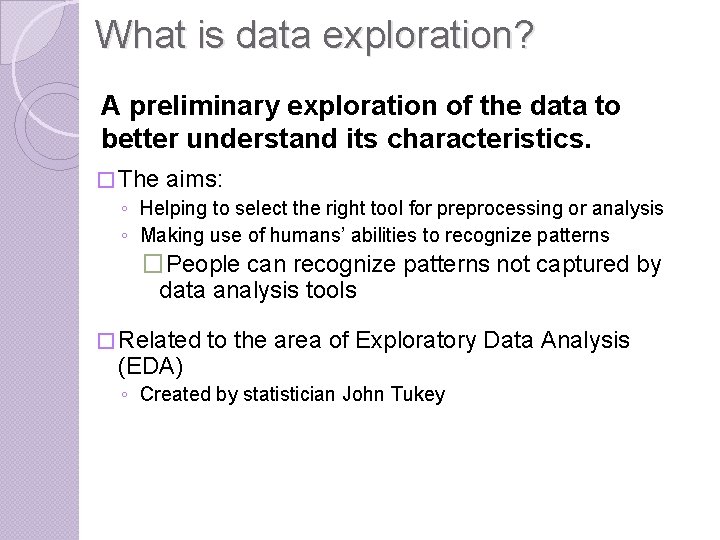
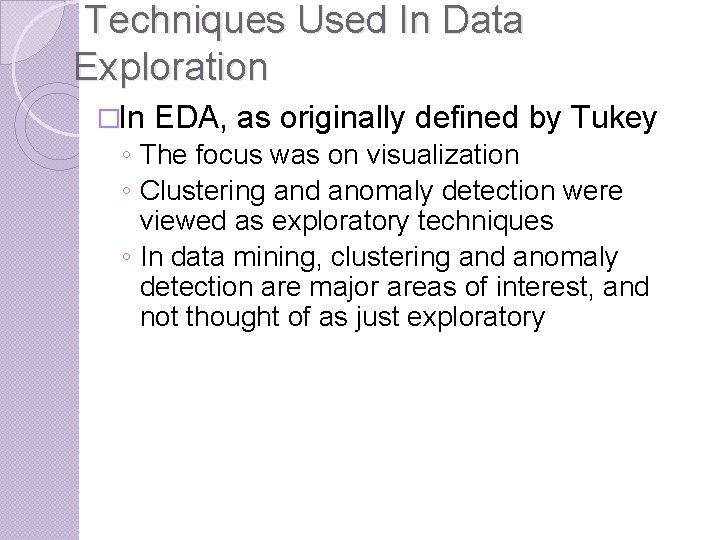
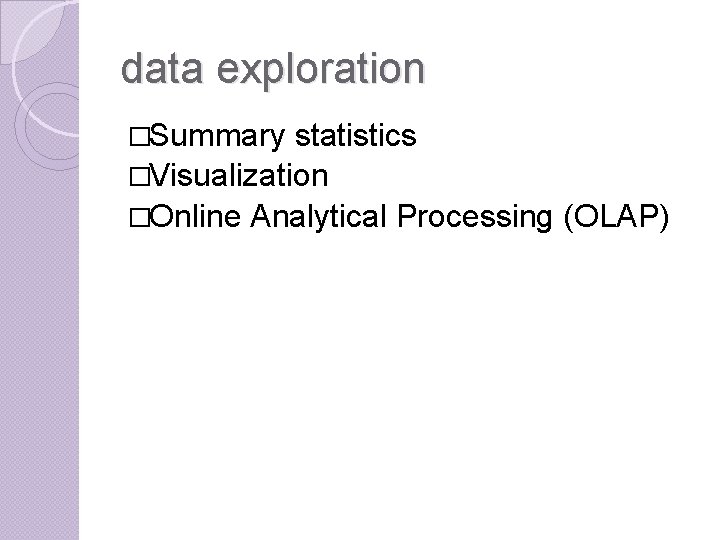
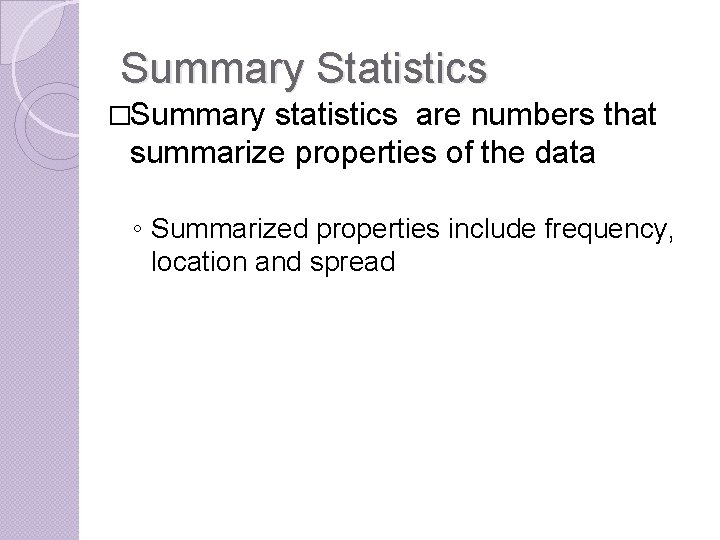
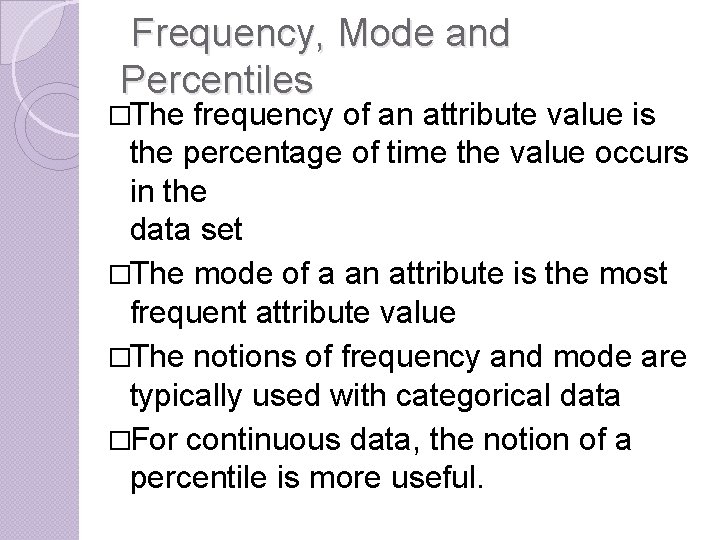
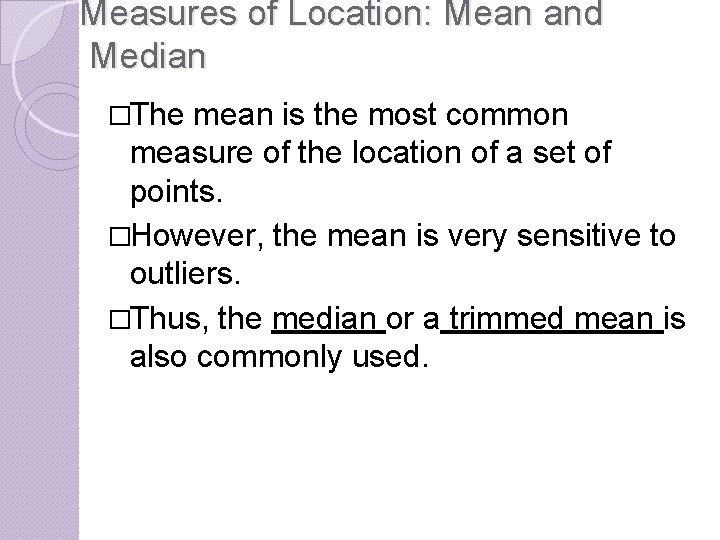
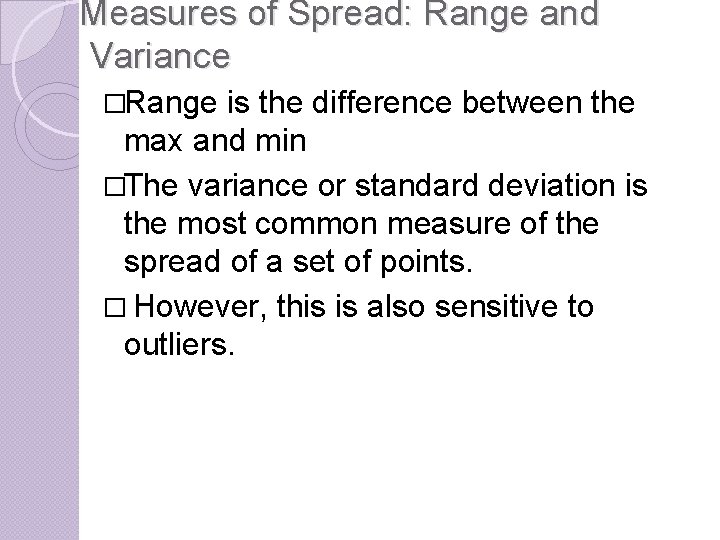
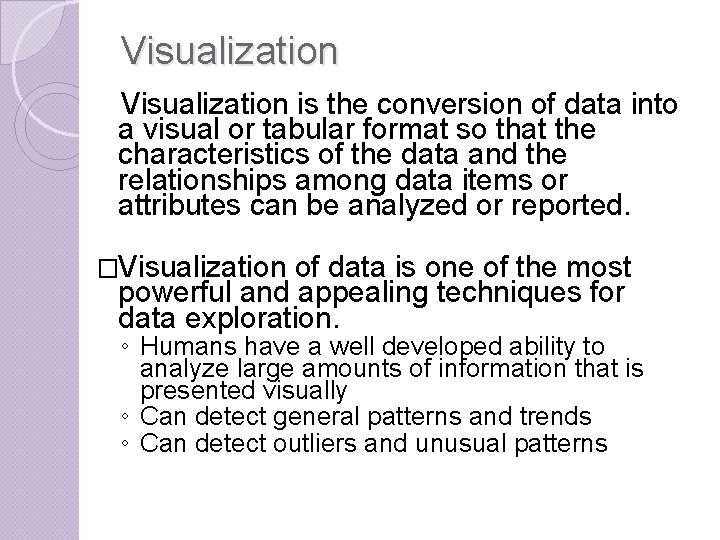
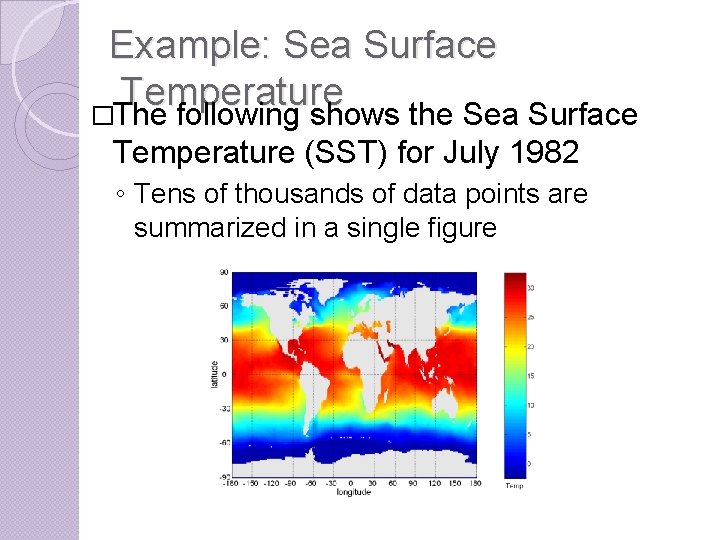
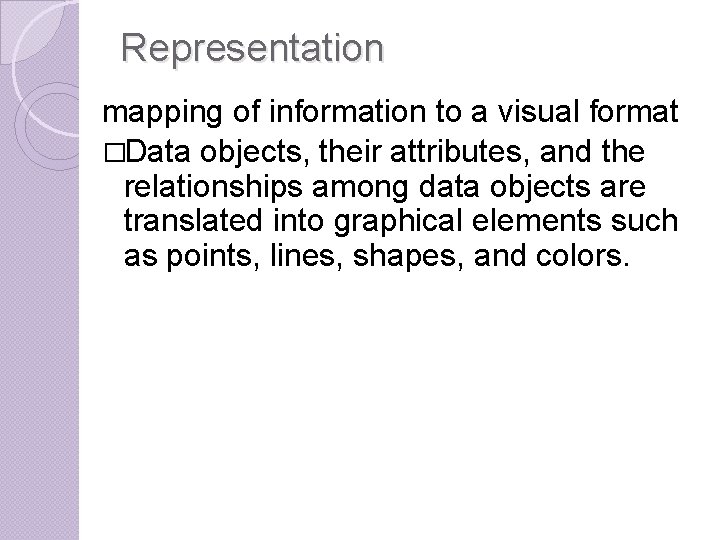
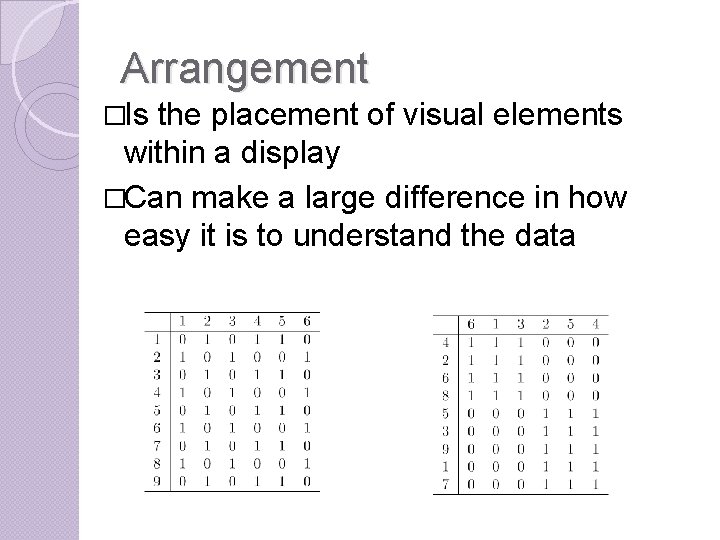
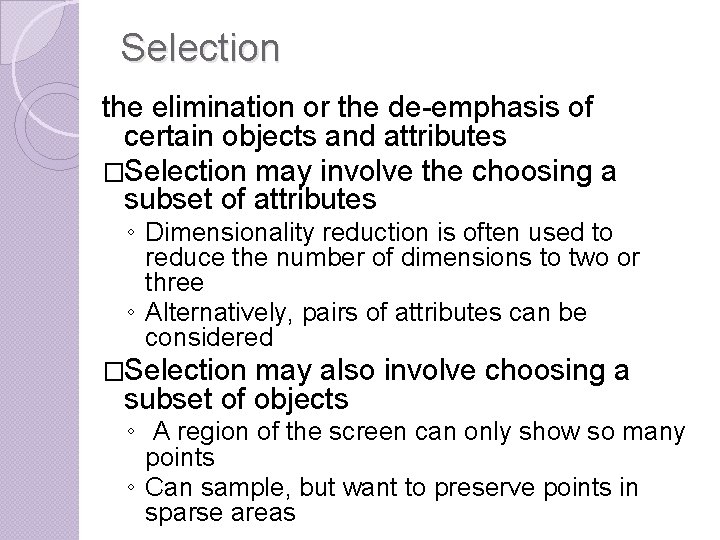
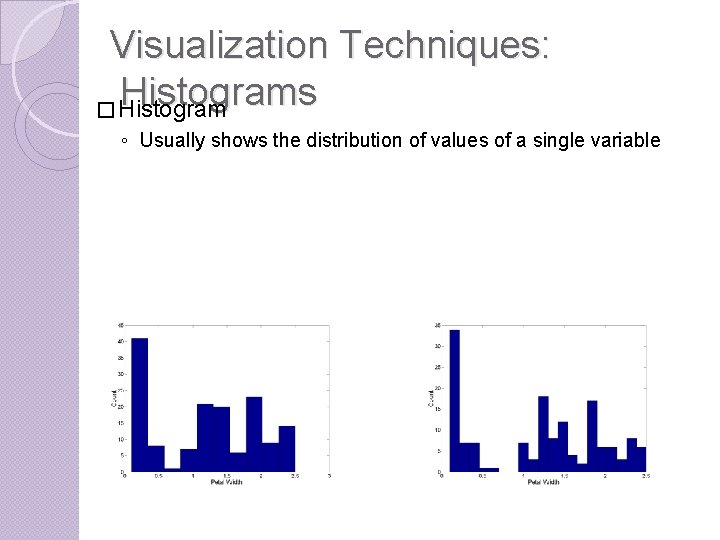
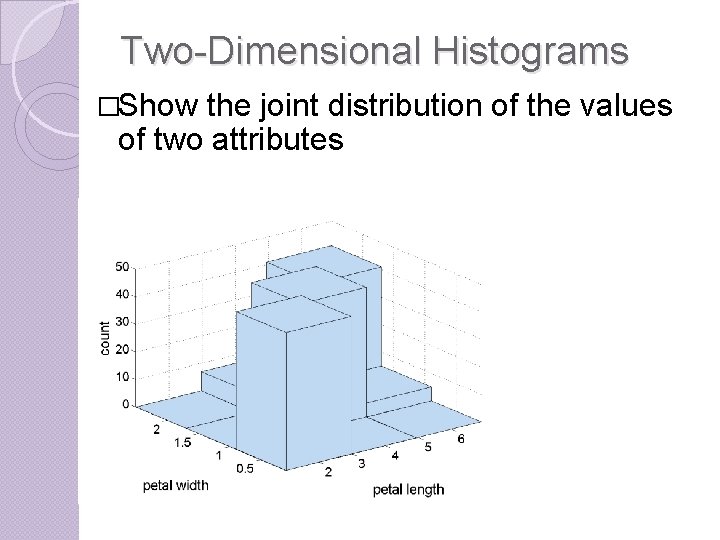
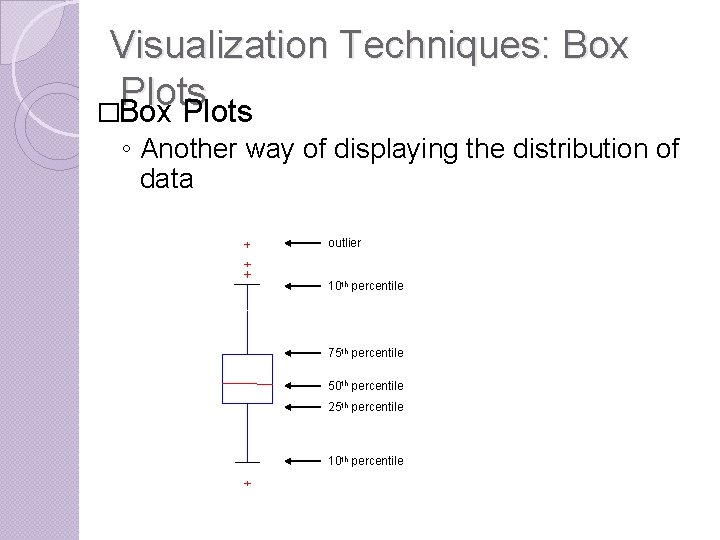
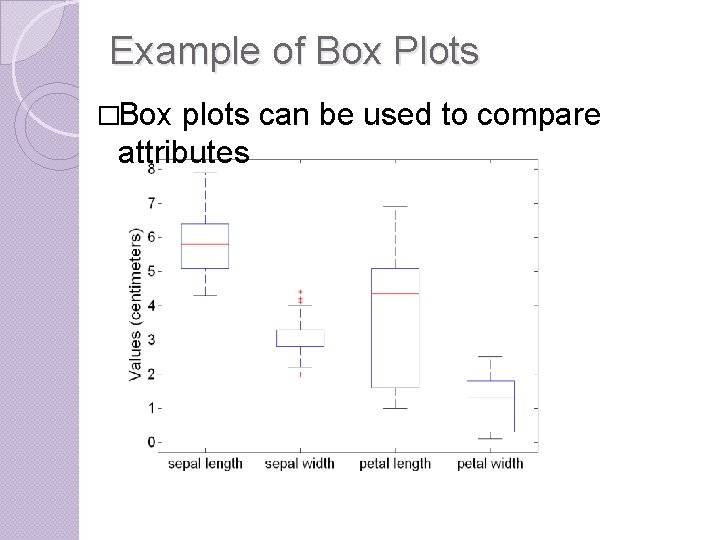
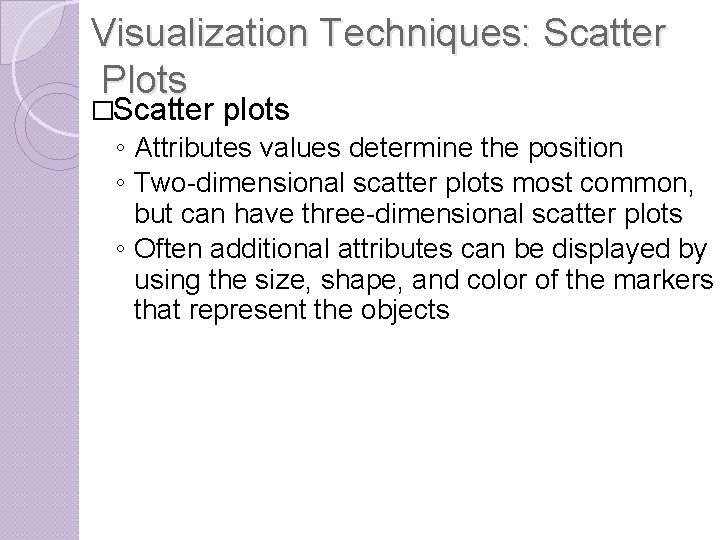
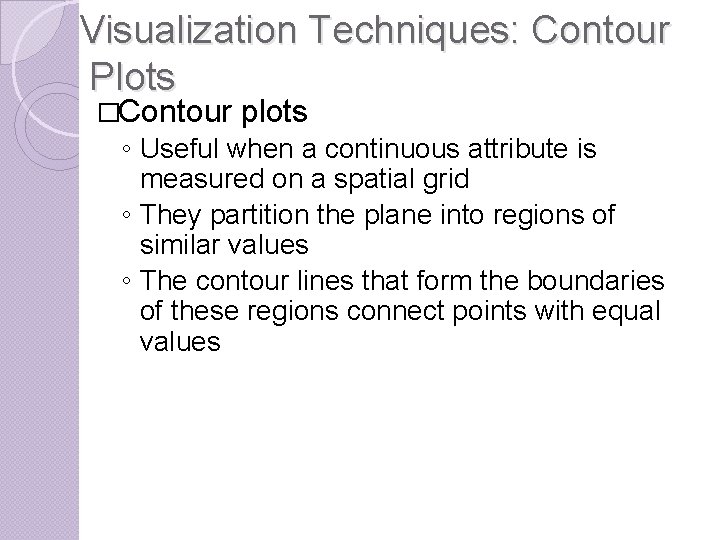
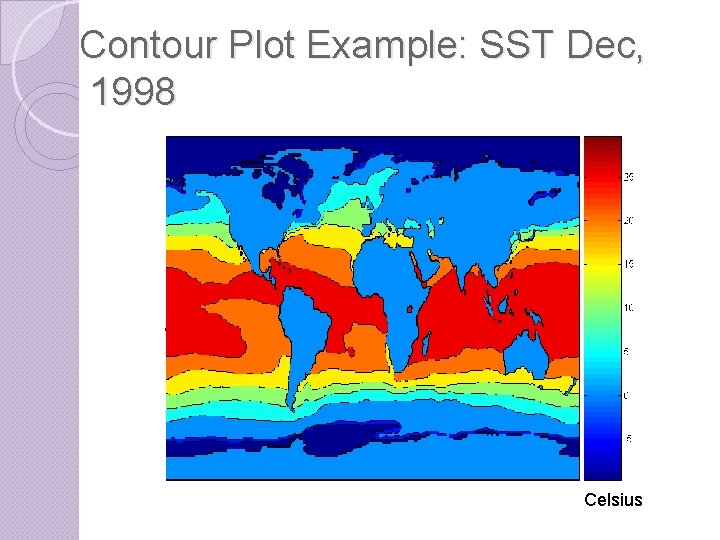
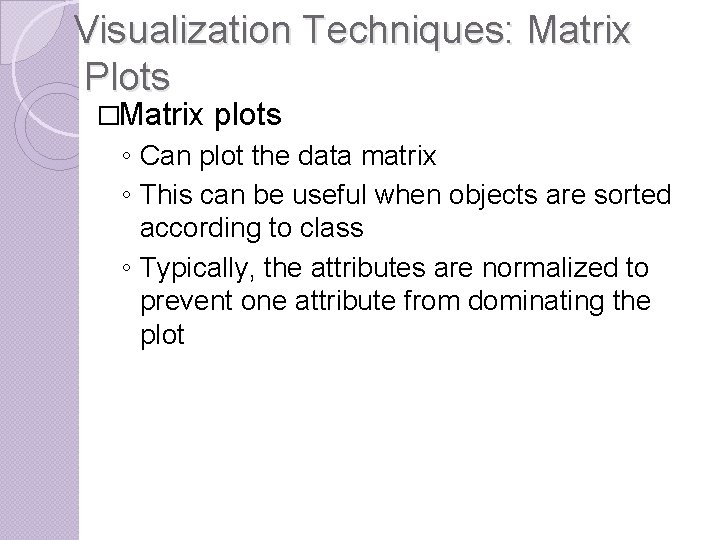
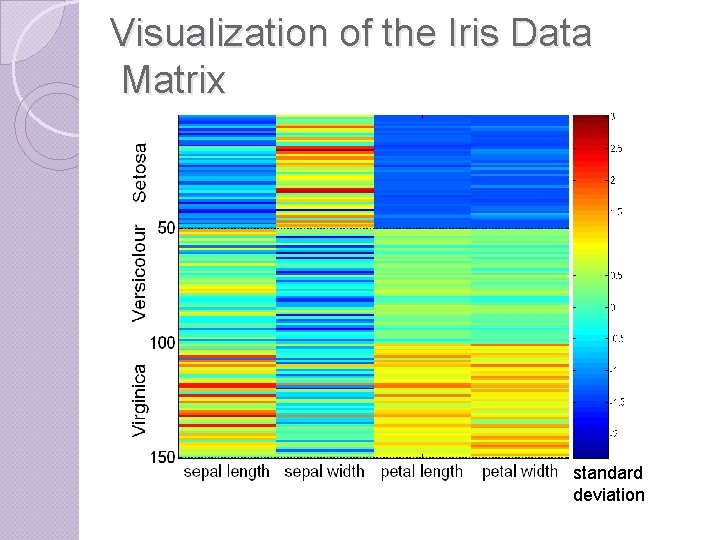
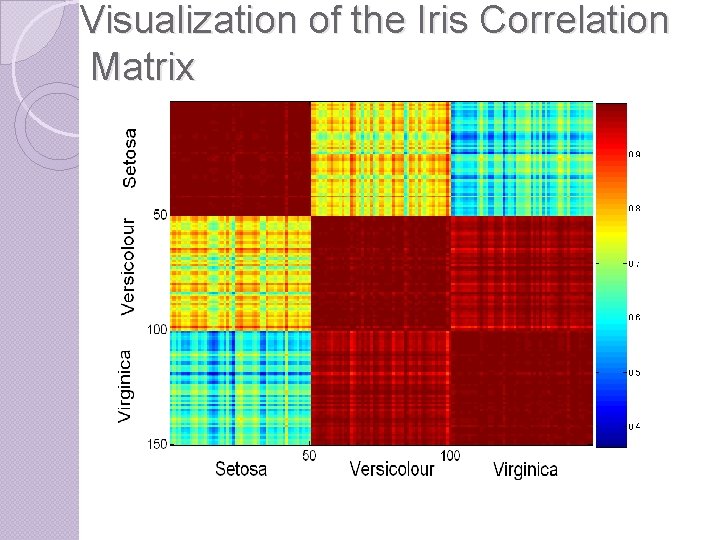
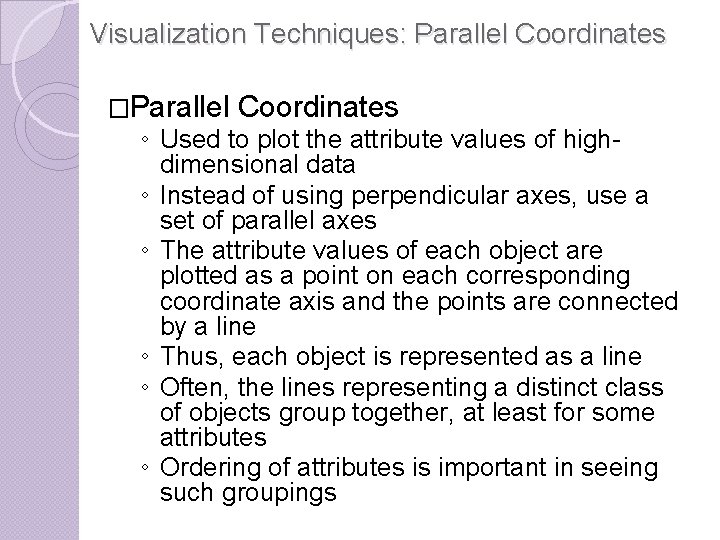
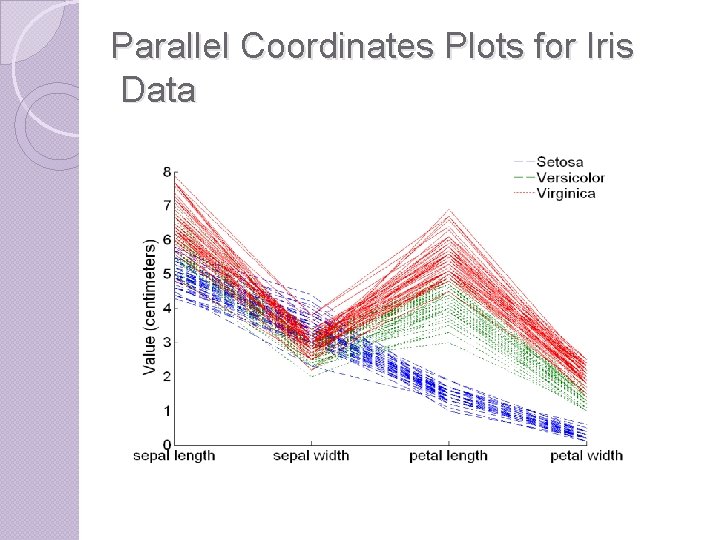
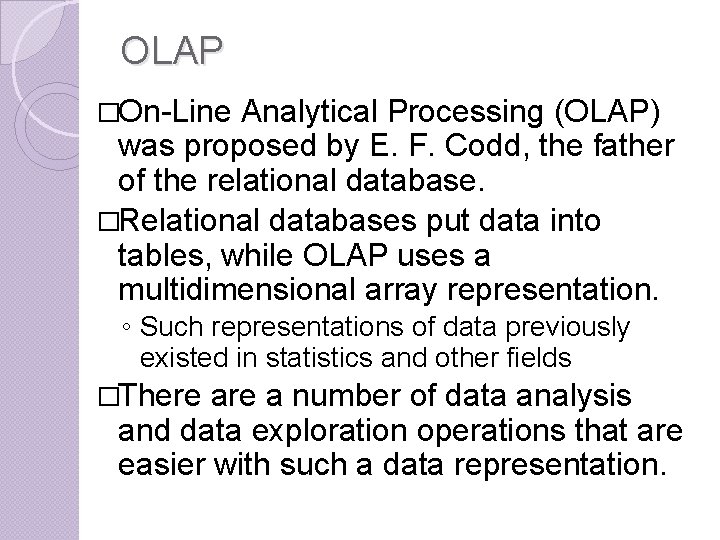
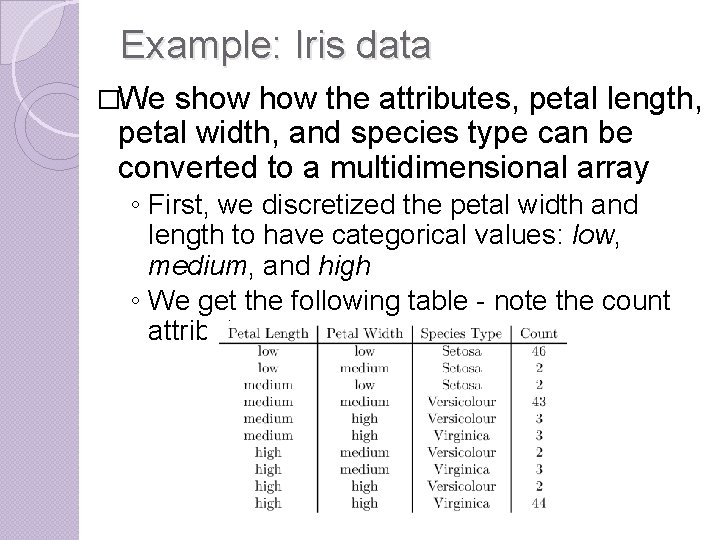
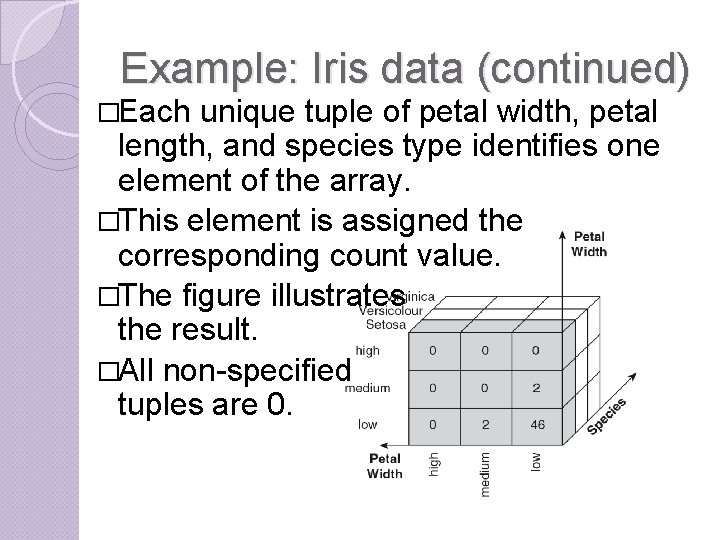
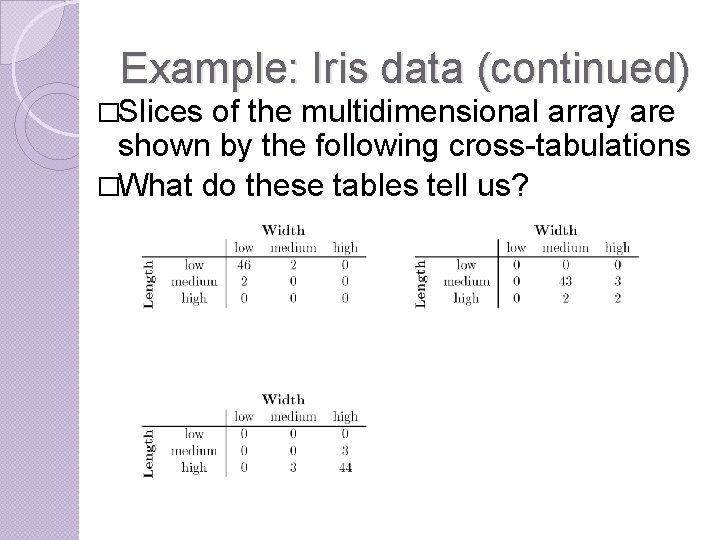
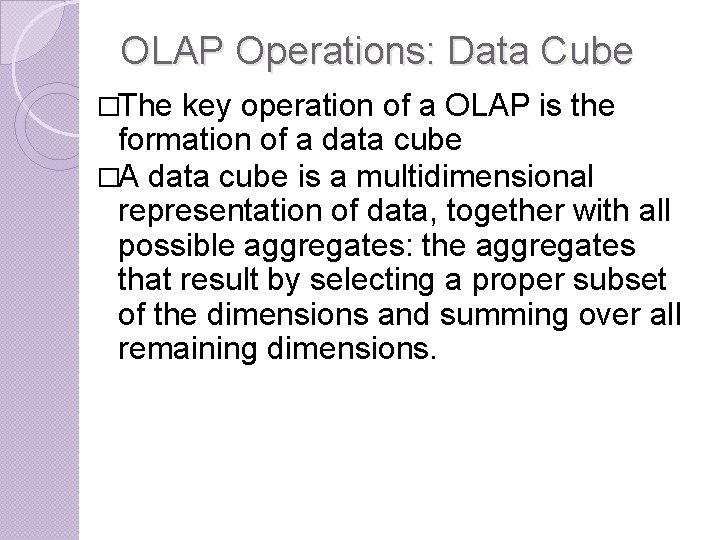
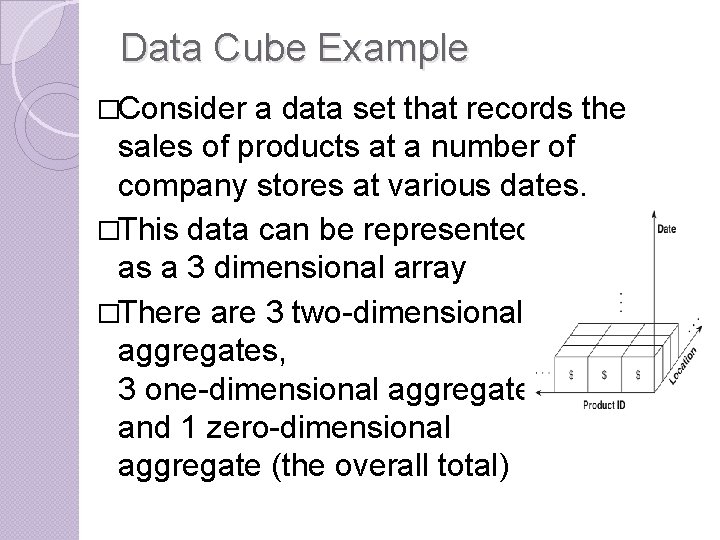
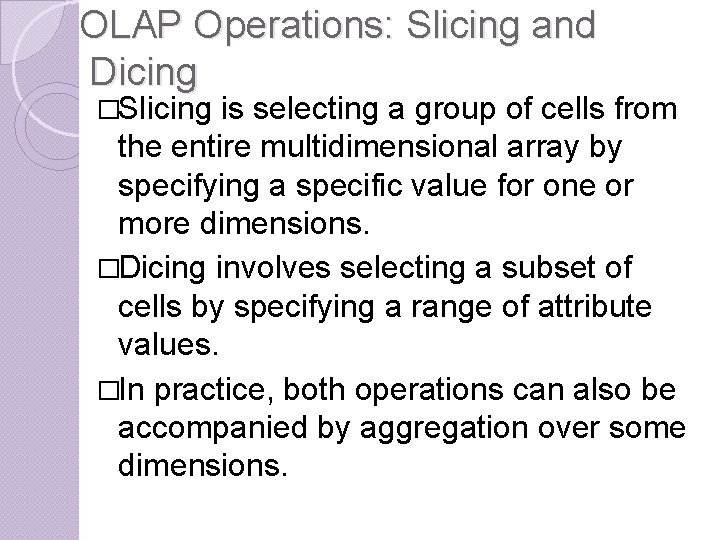
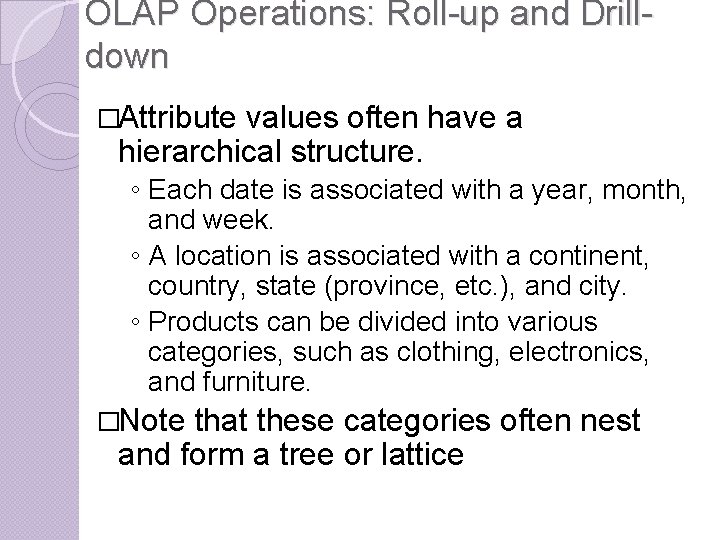
- Slides: 51
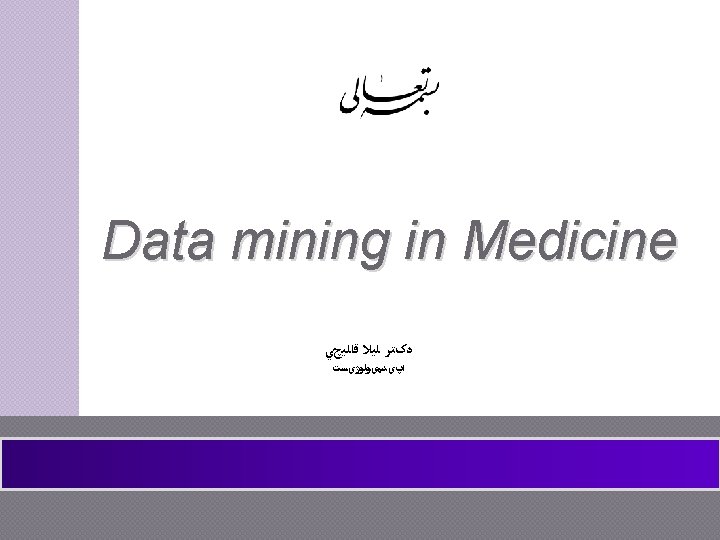
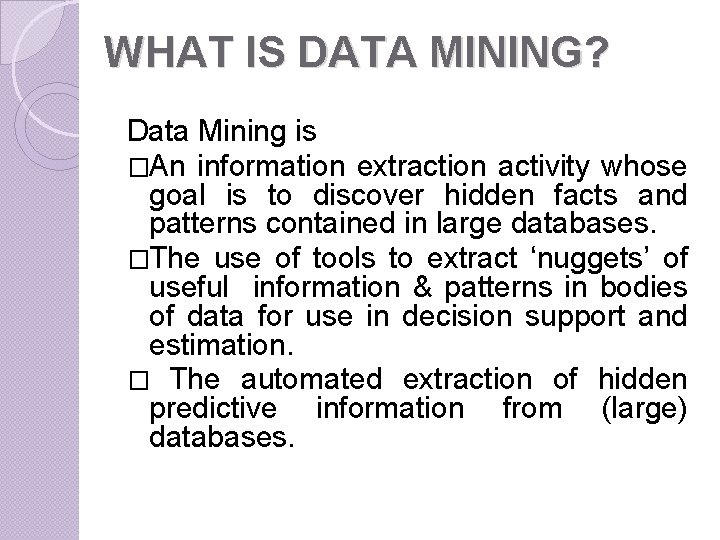
WHAT IS DATA MINING? Data Mining is �An information extraction activity whose goal is to discover hidden facts and patterns contained in large databases. �The use of tools to extract ‘nuggets’ of useful information & patterns in bodies of data for use in decision support and estimation. � The automated extraction of hidden predictive information from (large) databases.
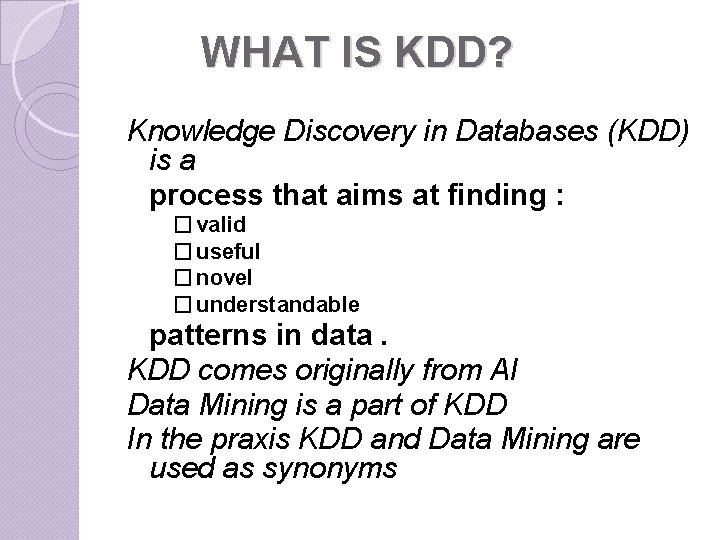
WHAT IS KDD? Knowledge Discovery in Databases (KDD) is a process that aims at finding : � valid � useful � novel � understandable patterns in data. KDD comes originally from AI Data Mining is a part of KDD In the praxis KDD and Data Mining are used as synonyms
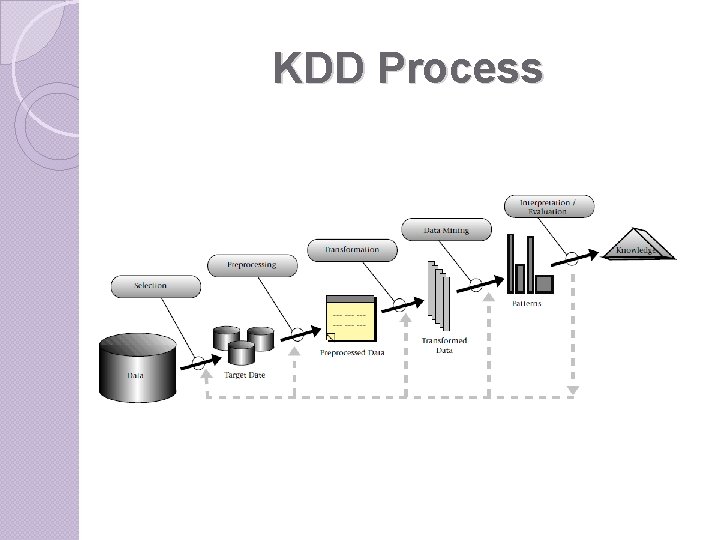
KDD Process
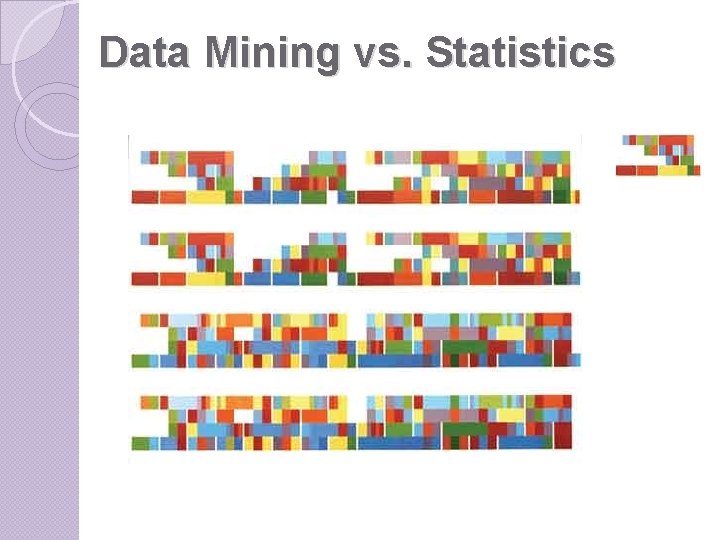
Data Mining vs. Statistics
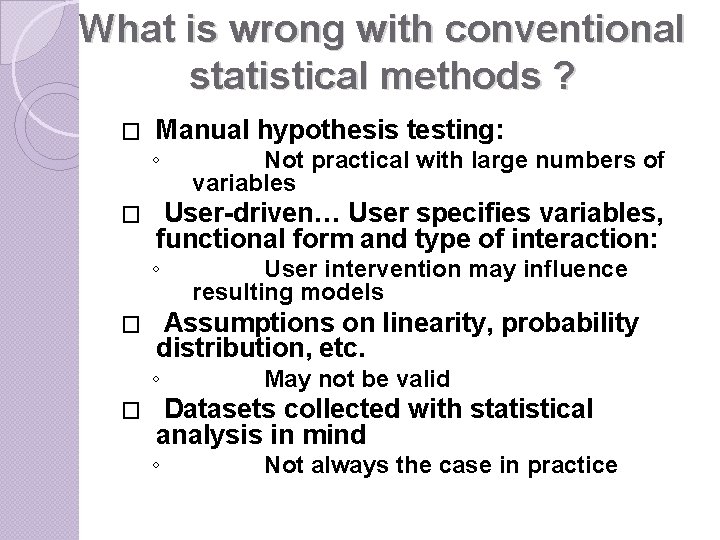
What is wrong with conventional statistical methods ? � Manual hypothesis testing: ◦ � User-driven… User specifies variables, functional form and type of interaction: ◦ � User intervention may influence resulting models Assumptions on linearity, probability distribution, etc. ◦ � Not practical with large numbers of variables May not be valid Datasets collected with statistical analysis in mind ◦ Not always the case in practice
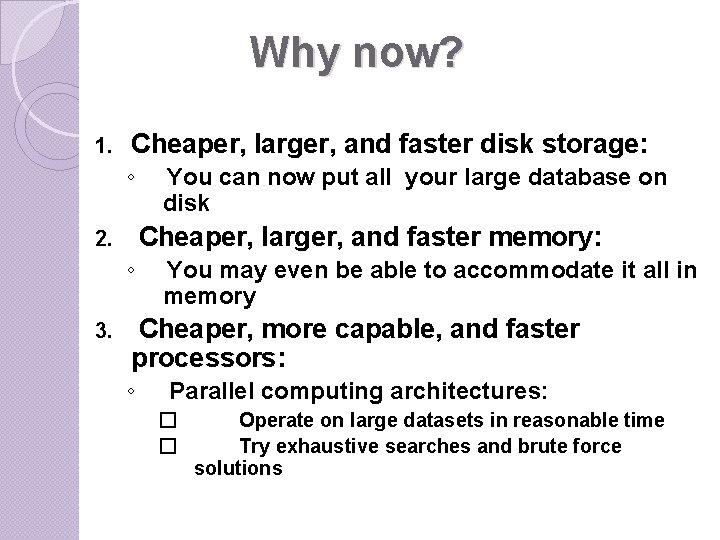
Why now? 1. Cheaper, larger, and faster disk storage: ◦ Cheaper, larger, and faster memory: 2. ◦ 3. You can now put all your large database on disk You may even be able to accommodate it all in memory Cheaper, more capable, and faster processors: ◦ Parallel computing architectures: � � Operate on large datasets in reasonable time Try exhaustive searches and brute force solutions
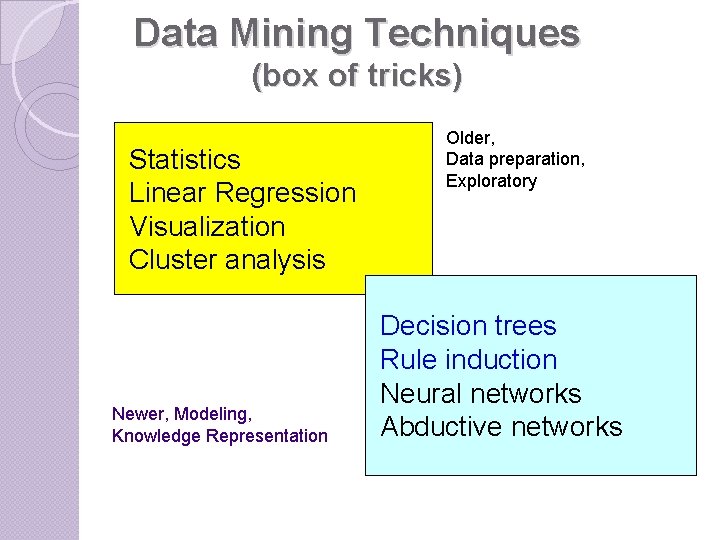
Data Mining Techniques (box of tricks) Statistics Linear Regression Visualization Cluster analysis Newer, Modeling, Knowledge Representation Older, Data preparation, Exploratory Decision trees Rule induction Neural networks Abductive networks
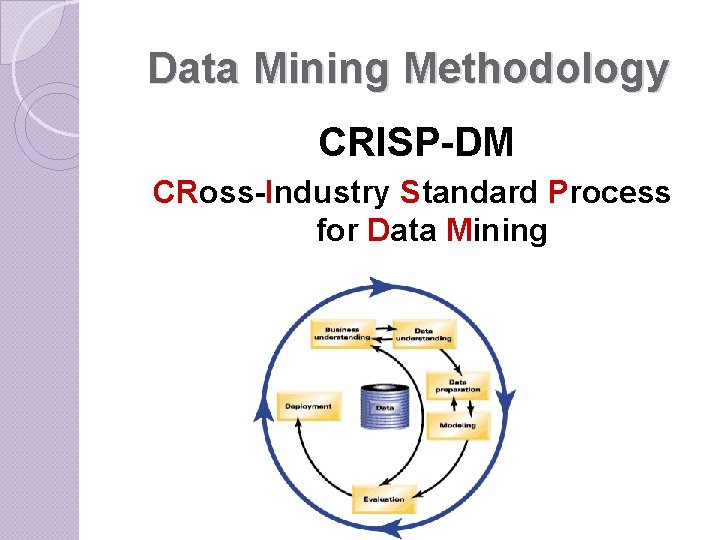
Data Mining Methodology CRISP-DM CRoss-Industry Standard Process for Data Mining
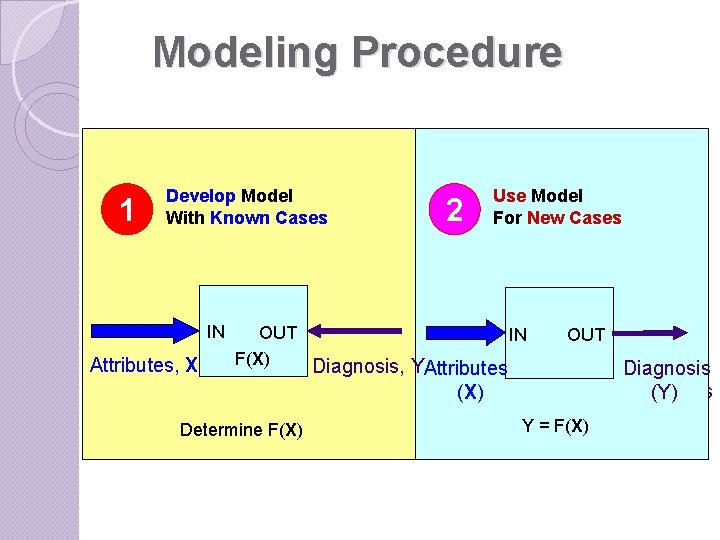
Modeling Procedure 1 Develop Model With Known Cases IN Attributes, X 2 Use Model For New Cases OUT IN F(X) Diagnosis, YAttributes OUT (X) Determine F(X) Y = F(X) Rock Diagnosis Properties (Y)
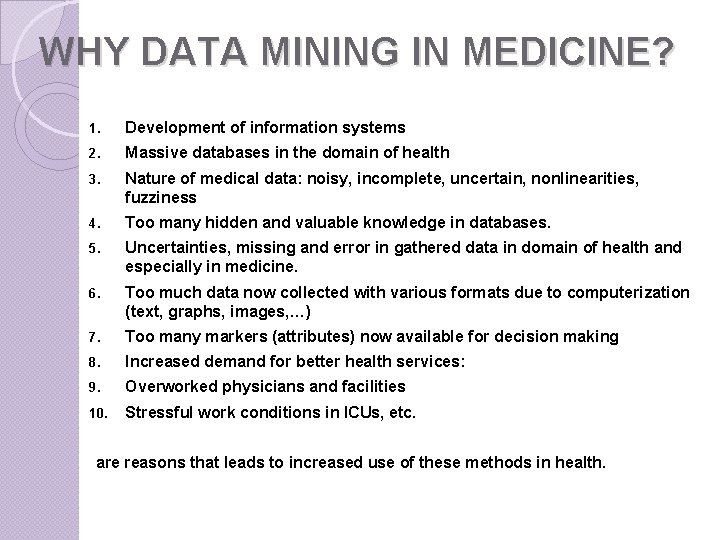
WHY DATA MINING IN MEDICINE? 1. Development of information systems 2. Massive databases in the domain of health 3. Nature of medical data: noisy, incomplete, uncertain, nonlinearities, fuzziness 4. Too many hidden and valuable knowledge in databases. 5. Uncertainties, missing and error in gathered data in domain of health and especially in medicine. 6. Too much data now collected with various formats due to computerization (text, graphs, images, …) 7. Too many markers (attributes) now available for decision making 8. Increased demand for better health services: 9. Overworked physicians and facilities 10. Stressful work conditions in ICUs, etc. are reasons that leads to increased use of these methods in health.
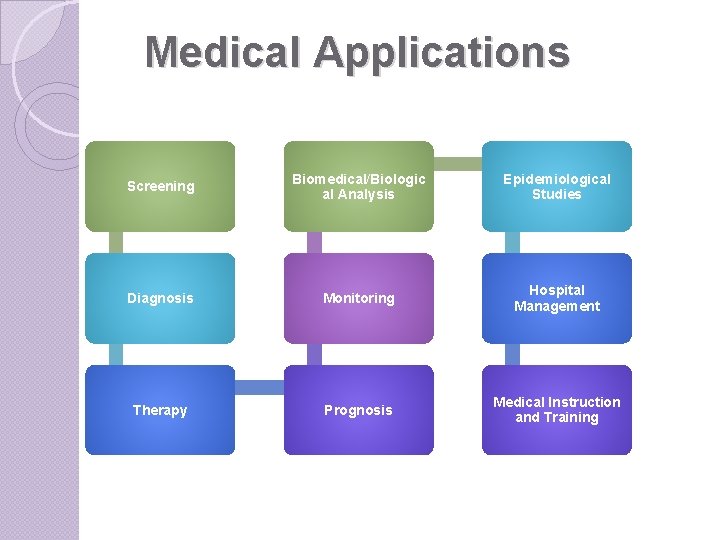
Medical Applications Screening Biomedical/Biologic al Analysis Epidemiological Studies Diagnosis Monitoring Hospital Management Therapy Prognosis Medical Instruction and Training
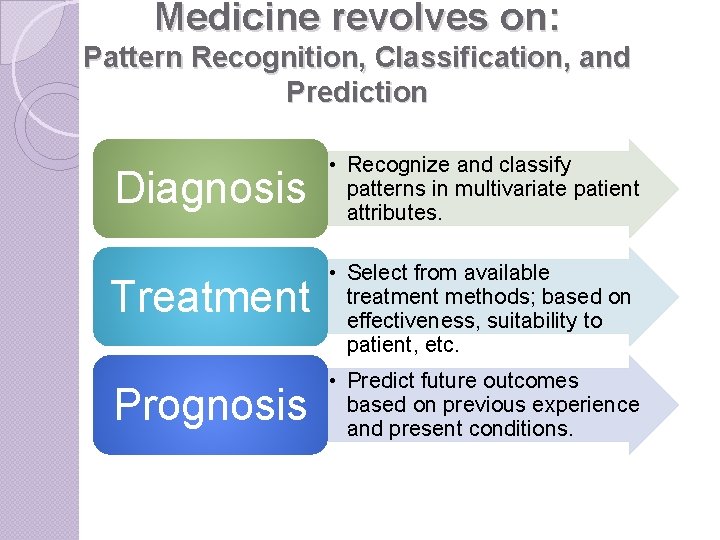
Medicine revolves on: Pattern Recognition, Classification, and Prediction Diagnosis Treatment Prognosis • Recognize and classify patterns in multivariate patient attributes. • Select from available treatment methods; based on effectiveness, suitability to patient, etc. • Predict future outcomes based on previous experience and present conditions.
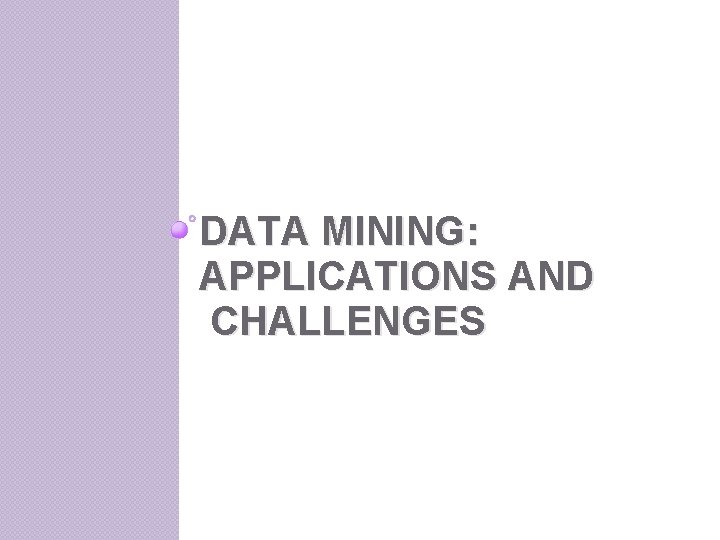
DATA MINING: APPLICATIONS AND CHALLENGES
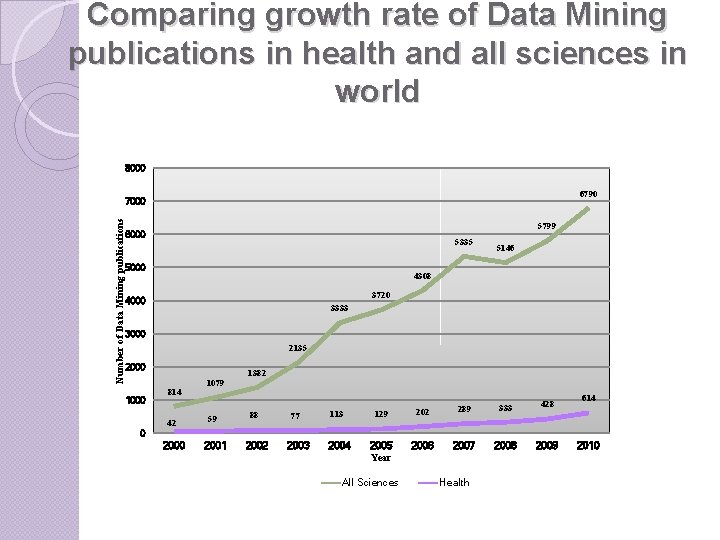
Comparing growth rate of Data Mining publications in health and all sciences in world 8000 6790 Number of Data Mining publications 7000 5799 6000 5335 5000 5146 4308 3720 4000 3333 3000 2135 2000 1382 814 42 1079 59 88 77 113 129 202 289 333 428 2005 Year 2006 2007 2008 2009 614 0 2001 2002 2003 2004 All Sciences Slide No. 15 2010 Health 2 nd Medical Informatics Seminar
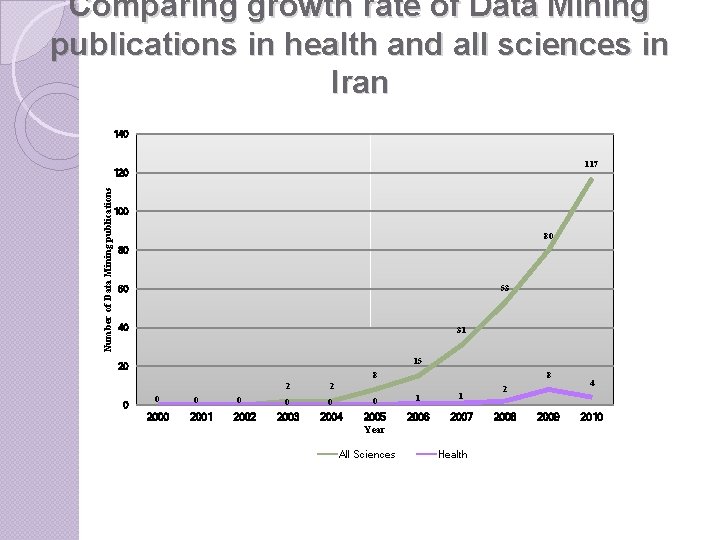
Comparing growth rate of Data Mining publications in health and all sciences in Iran 140 117 Number of Data Mining publications 120 100 80 80 53 60 40 31 15 20 0 8 0 2000 0 2001 0 2002 8 2 2 0 0 0 1 1 2003 2004 2005 Year 2006 2007 All Sciences Slide No. 16 2 2008 2009 4 2010 Health 2 nd Medical Informatics Seminar
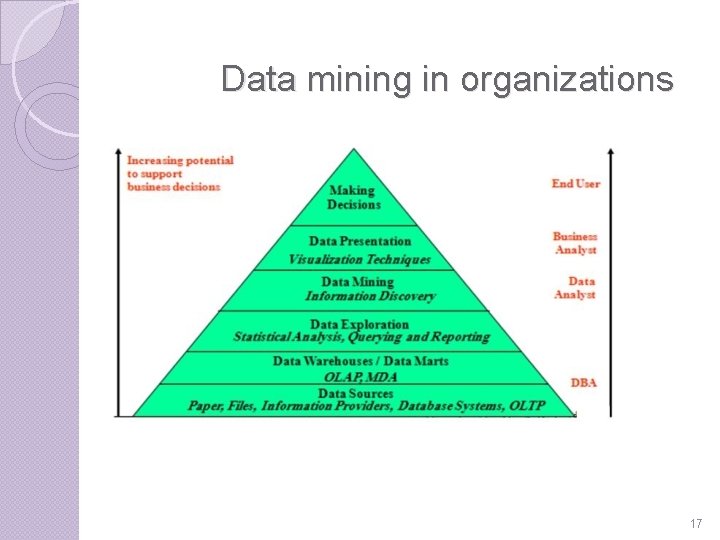
Data mining in organizations 17
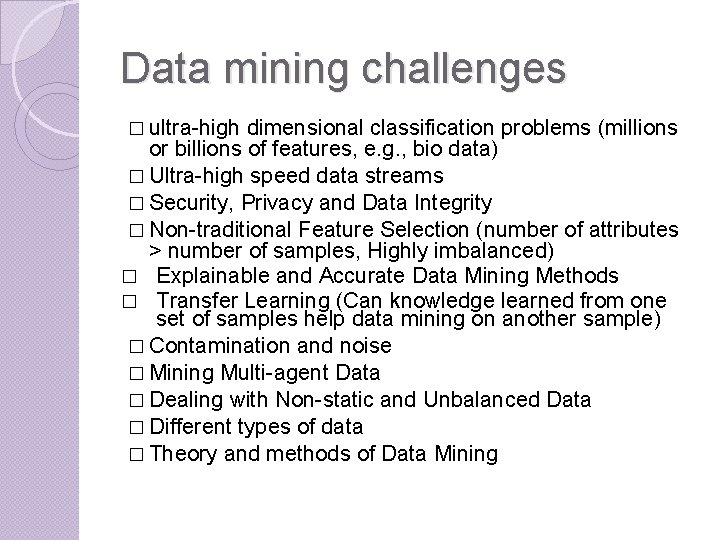
Data mining challenges � ultra-high dimensional classification problems (millions or billions of features, e. g. , bio data) � Ultra-high speed data streams � Security, Privacy and Data Integrity � Non-traditional Feature Selection (number of attributes > number of samples, Highly imbalanced) � Explainable and Accurate Data Mining Methods � Transfer Learning (Can knowledge learned from one set of samples help data mining on another sample) � Contamination and noise � Mining Multi-agent Data � Dealing with Non-static and Unbalanced Data � Different types of data � Theory and methods of Data Mining
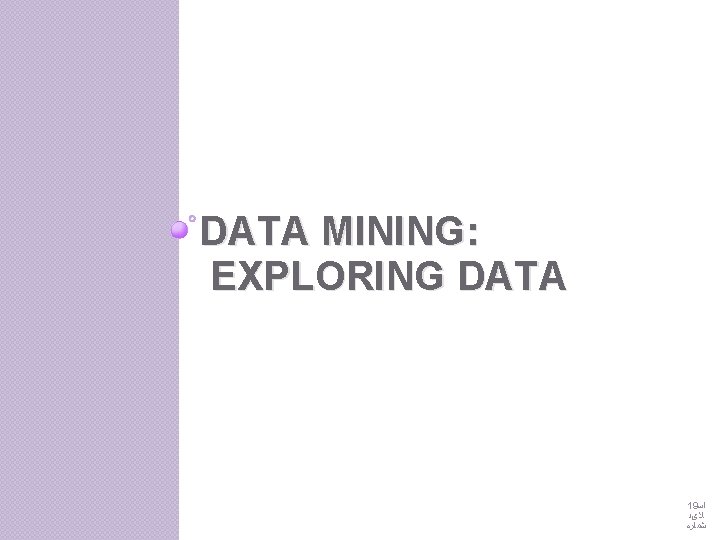
DATA MINING: EXPLORING DATA 19 ﺍﺳ ﻼیﺪ ﺷﻤﺎﺭﻩ
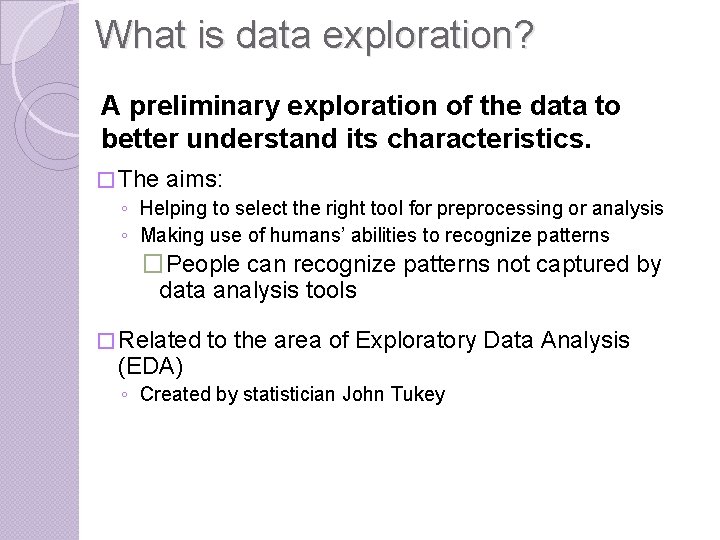
What is data exploration? A preliminary exploration of the data to better understand its characteristics. � The aims: ◦ Helping to select the right tool for preprocessing or analysis ◦ Making use of humans’ abilities to recognize patterns �People can recognize patterns not captured by data analysis tools � Related (EDA) to the area of Exploratory Data Analysis ◦ Created by statistician John Tukey
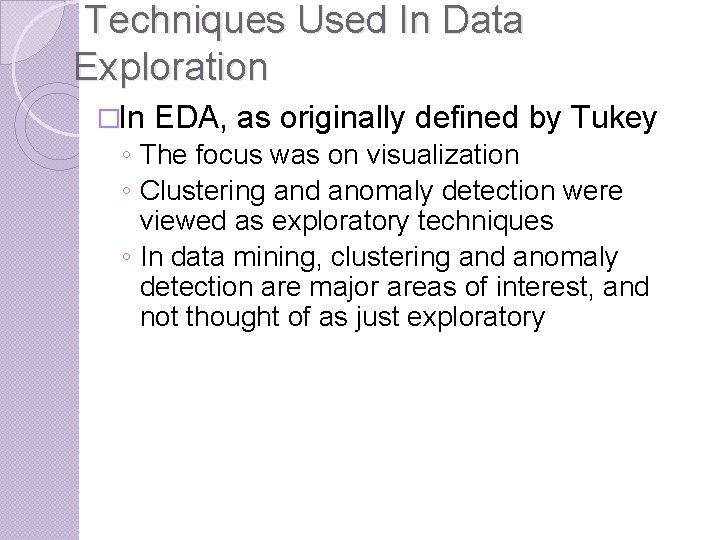
Techniques Used In Data Exploration �In EDA, as originally defined by Tukey ◦ The focus was on visualization ◦ Clustering and anomaly detection were viewed as exploratory techniques ◦ In data mining, clustering and anomaly detection are major areas of interest, and not thought of as just exploratory
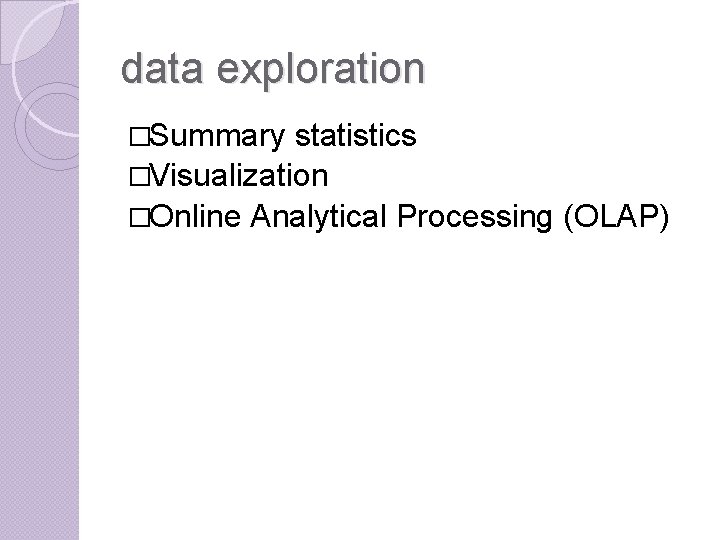
data exploration �Summary statistics �Visualization �Online Analytical Processing (OLAP)
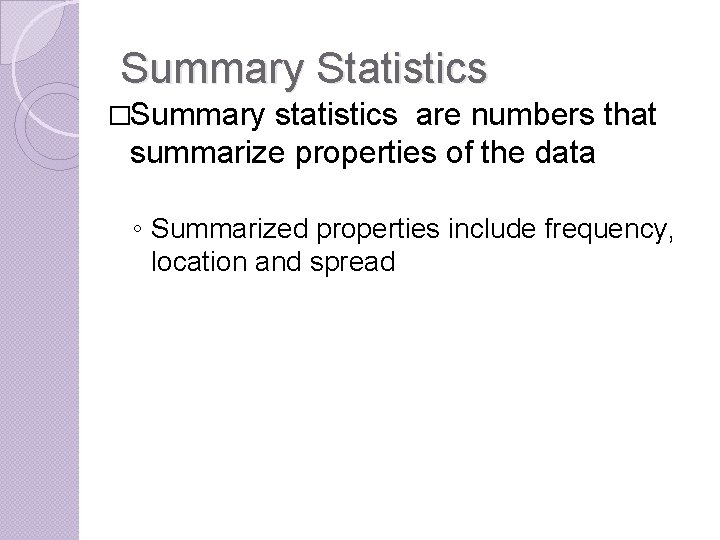
Summary Statistics �Summary statistics are numbers that summarize properties of the data ◦ Summarized properties include frequency, location and spread
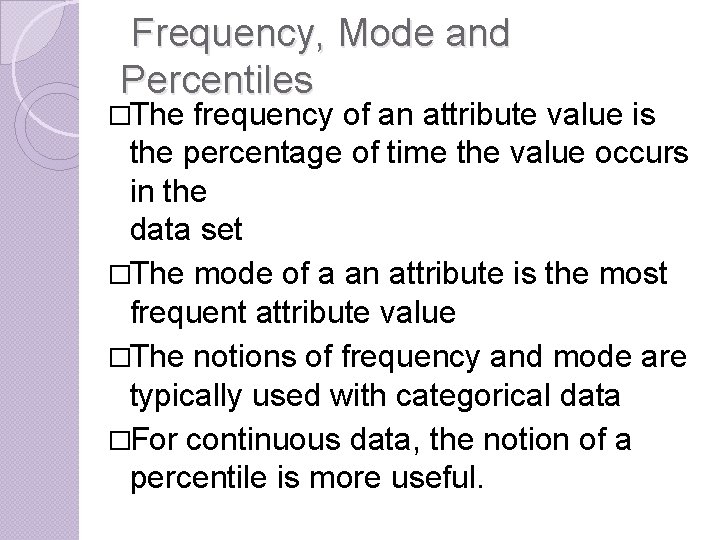
Frequency, Mode and Percentiles �The frequency of an attribute value is the percentage of time the value occurs in the data set �The mode of a an attribute is the most frequent attribute value �The notions of frequency and mode are typically used with categorical data �For continuous data, the notion of a percentile is more useful.
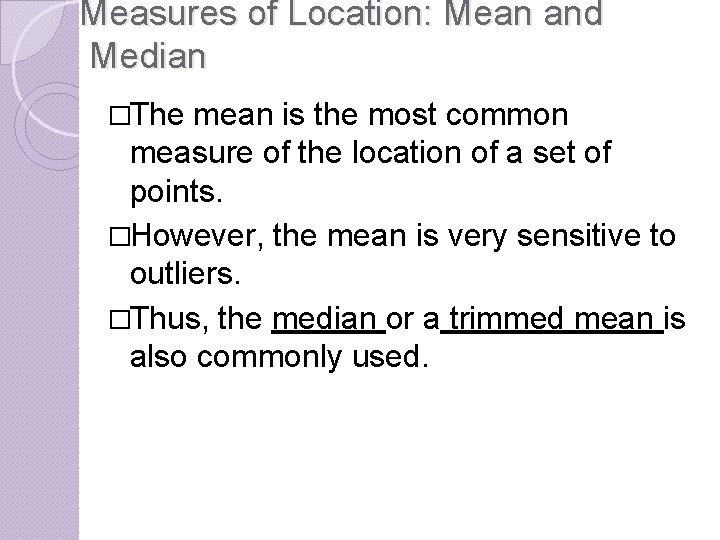
Measures of Location: Mean and Median �The mean is the most common measure of the location of a set of points. �However, the mean is very sensitive to outliers. �Thus, the median or a trimmed mean is also commonly used.
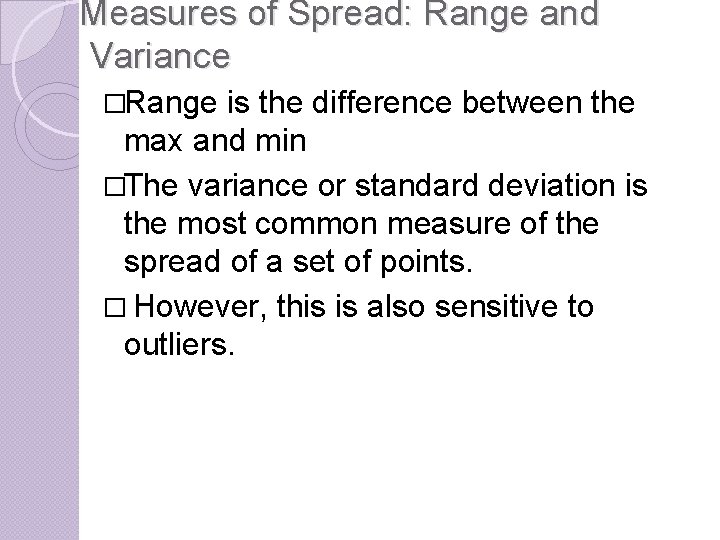
Measures of Spread: Range and Variance �Range is the difference between the max and min �The variance or standard deviation is the most common measure of the spread of a set of points. � However, this is also sensitive to outliers.
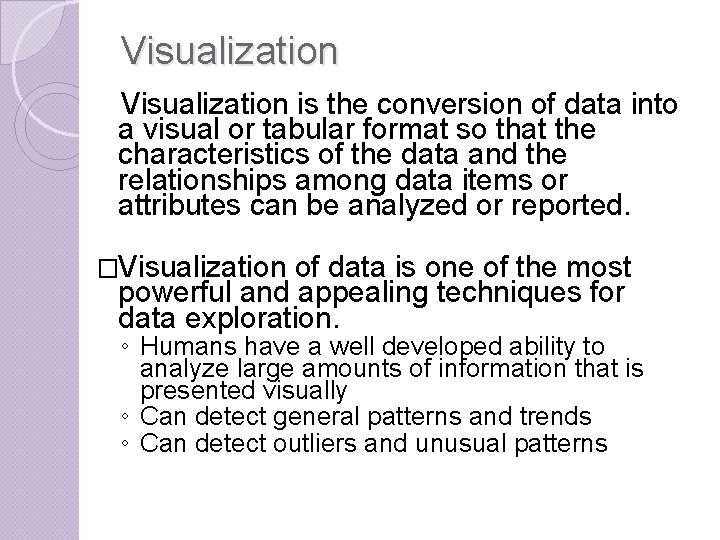
Visualization is the conversion of data into a visual or tabular format so that the characteristics of the data and the relationships among data items or attributes can be analyzed or reported. �Visualization of data is one of the most powerful and appealing techniques for data exploration. ◦ Humans have a well developed ability to analyze large amounts of information that is presented visually ◦ Can detect general patterns and trends ◦ Can detect outliers and unusual patterns
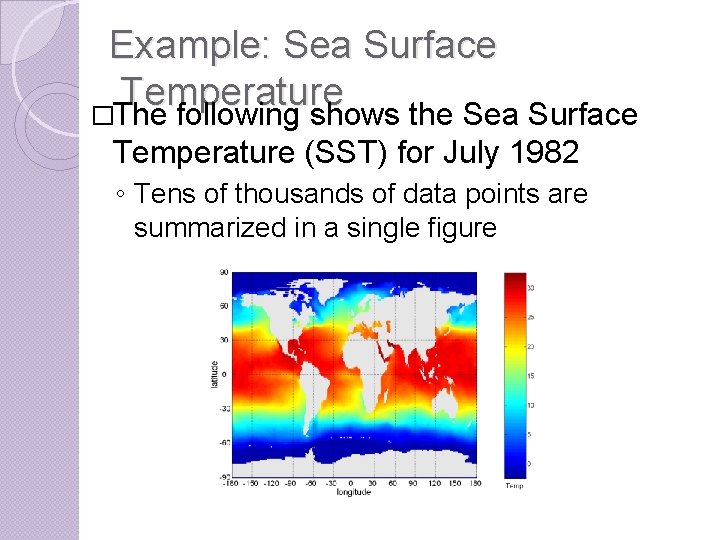
Example: Sea Surface Temperature �The following shows the Sea Surface Temperature (SST) for July 1982 ◦ Tens of thousands of data points are summarized in a single figure
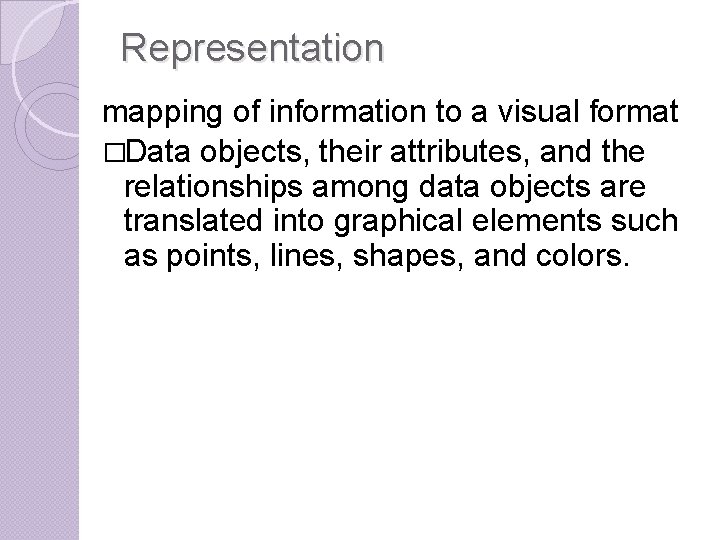
Representation mapping of information to a visual format �Data objects, their attributes, and the relationships among data objects are translated into graphical elements such as points, lines, shapes, and colors.
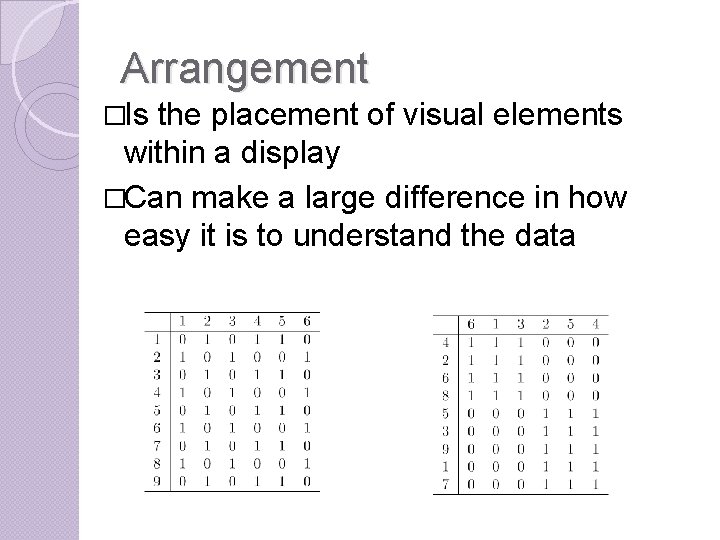
Arrangement �Is the placement of visual elements within a display �Can make a large difference in how easy it is to understand the data
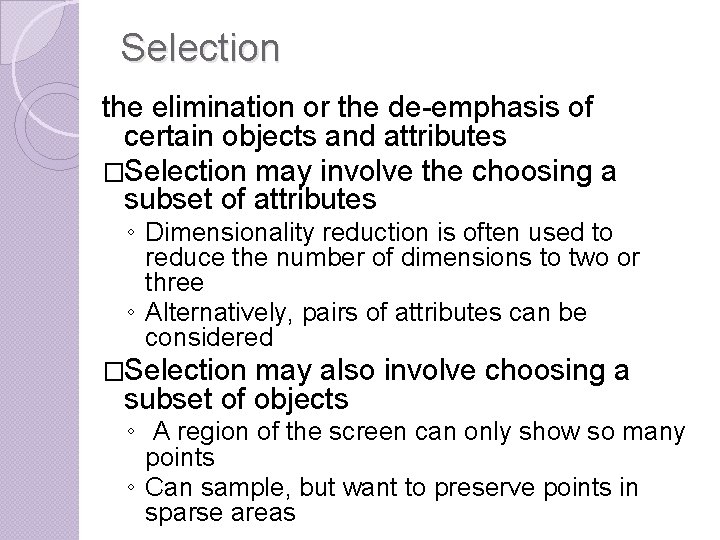
Selection the elimination or the de-emphasis of certain objects and attributes �Selection may involve the choosing a subset of attributes ◦ Dimensionality reduction is often used to reduce the number of dimensions to two or three ◦ Alternatively, pairs of attributes can be considered �Selection may also involve choosing a subset of objects ◦ A region of the screen can only show so many points ◦ Can sample, but want to preserve points in sparse areas
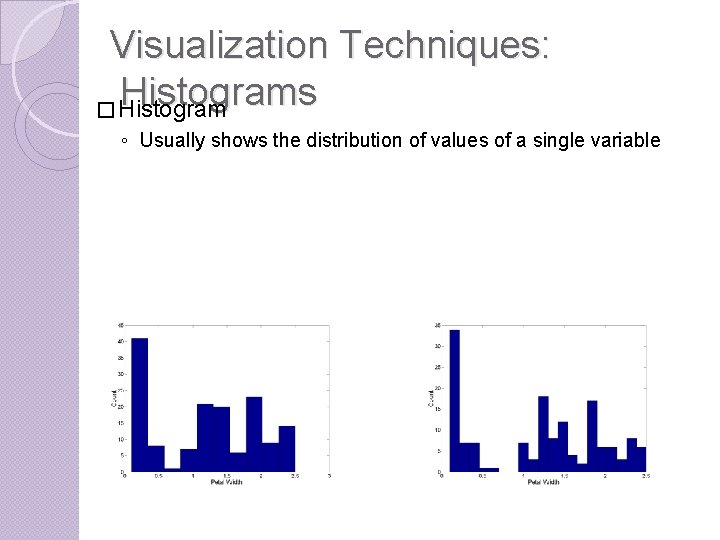
Visualization Techniques: Histograms � Histogram ◦ Usually shows the distribution of values of a single variable
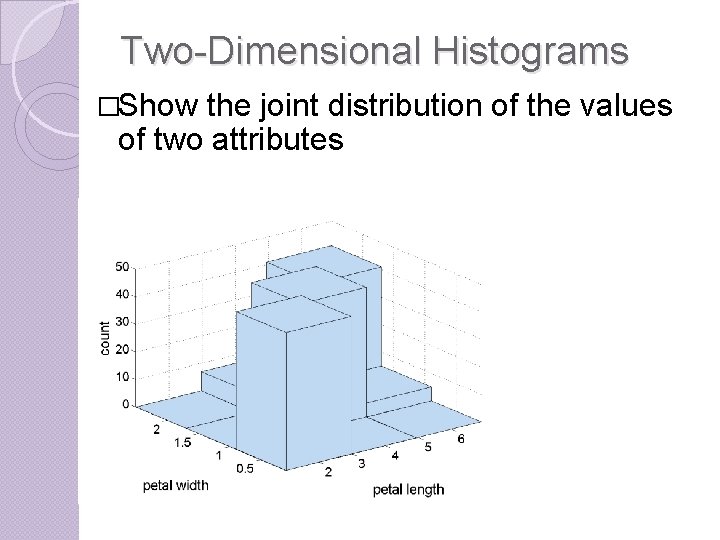
Two-Dimensional Histograms �Show the joint distribution of the values of two attributes
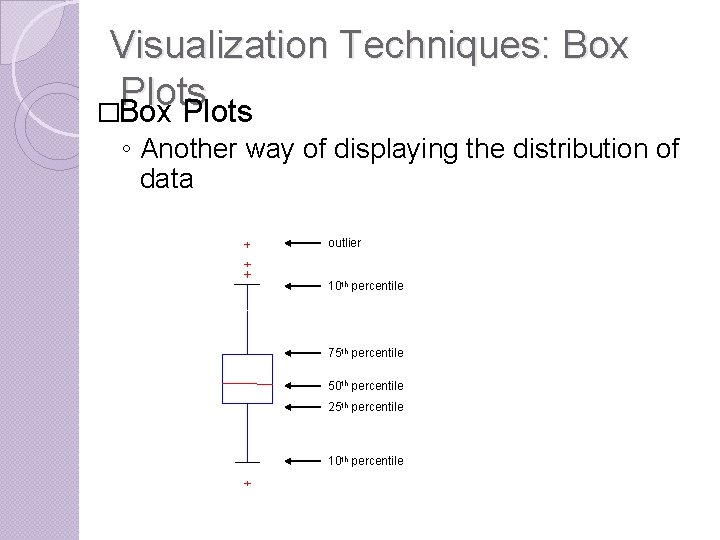
Visualization Techniques: Box Plots �Box Plots ◦ Another way of displaying the distribution of data outlier 10 th percentile 75 th percentile 50 th percentile 25 th percentile 10 th percentile
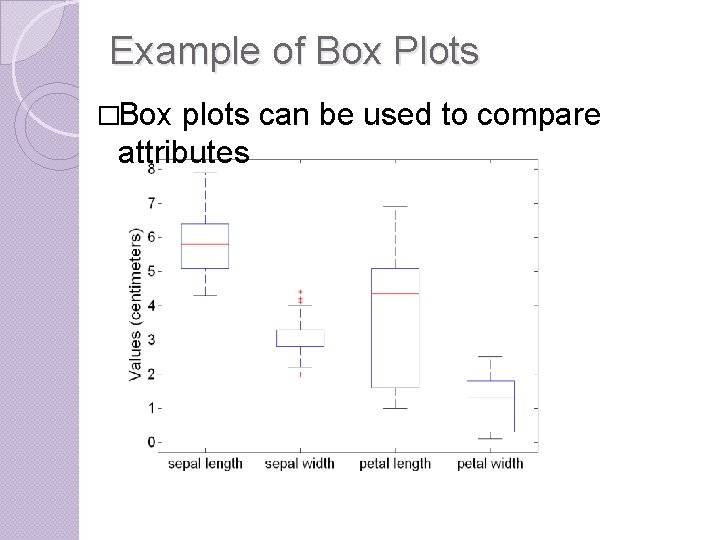
Example of Box Plots �Box plots can be used to compare attributes
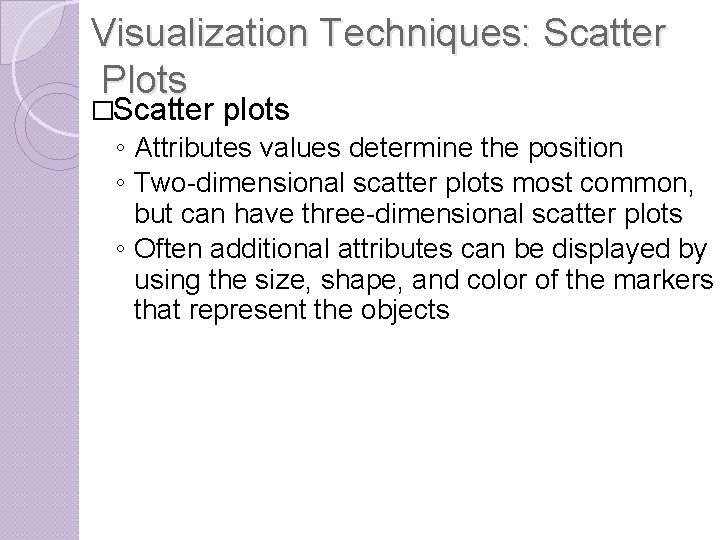
Visualization Techniques: Scatter Plots �Scatter plots ◦ Attributes values determine the position ◦ Two-dimensional scatter plots most common, but can have three-dimensional scatter plots ◦ Often additional attributes can be displayed by using the size, shape, and color of the markers that represent the objects
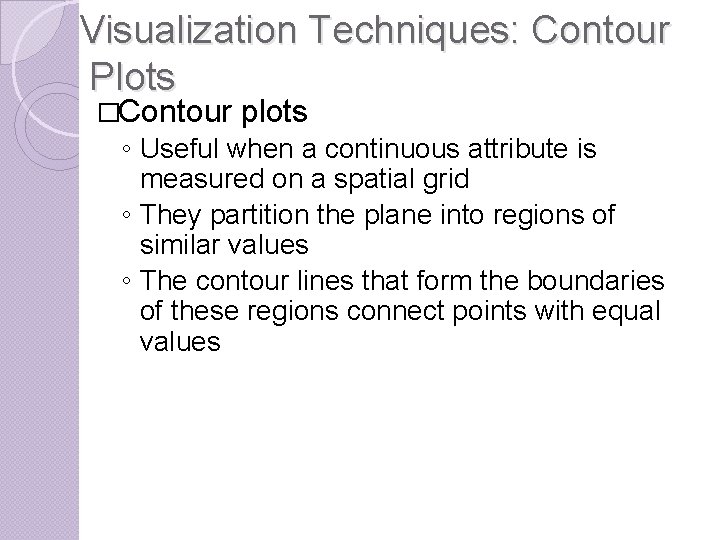
Visualization Techniques: Contour Plots �Contour plots ◦ Useful when a continuous attribute is measured on a spatial grid ◦ They partition the plane into regions of similar values ◦ The contour lines that form the boundaries of these regions connect points with equal values
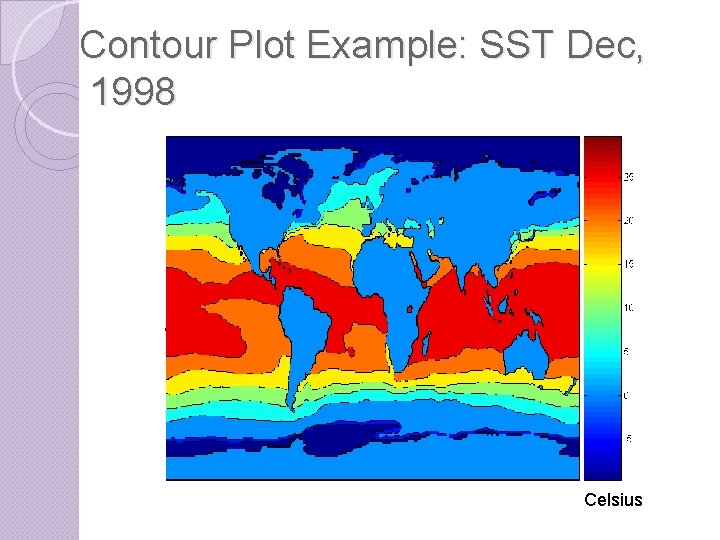
Contour Plot Example: SST Dec, 1998 Celsius
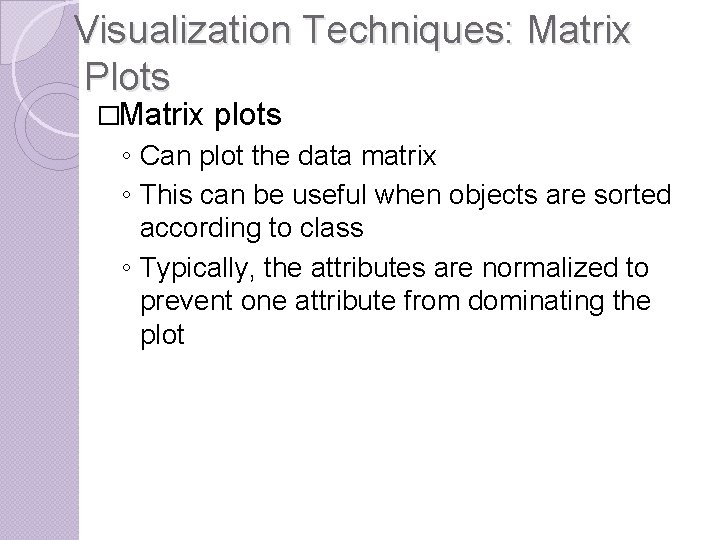
Visualization Techniques: Matrix Plots �Matrix plots ◦ Can plot the data matrix ◦ This can be useful when objects are sorted according to class ◦ Typically, the attributes are normalized to prevent one attribute from dominating the plot
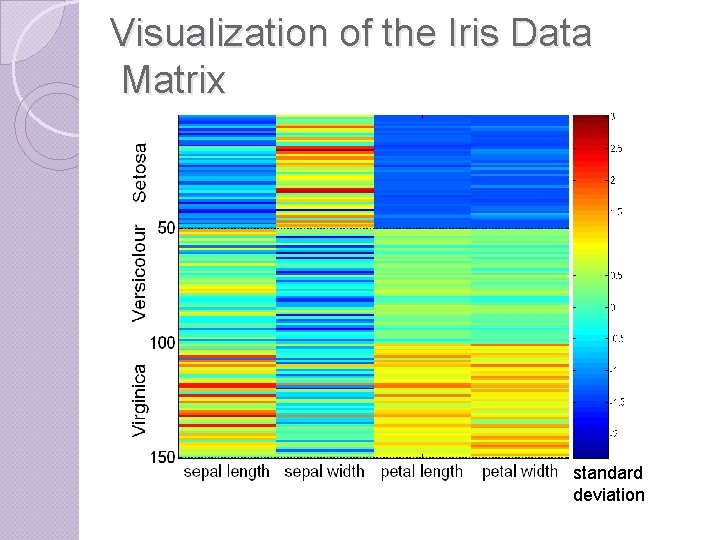
Visualization of the Iris Data Matrix standard deviation
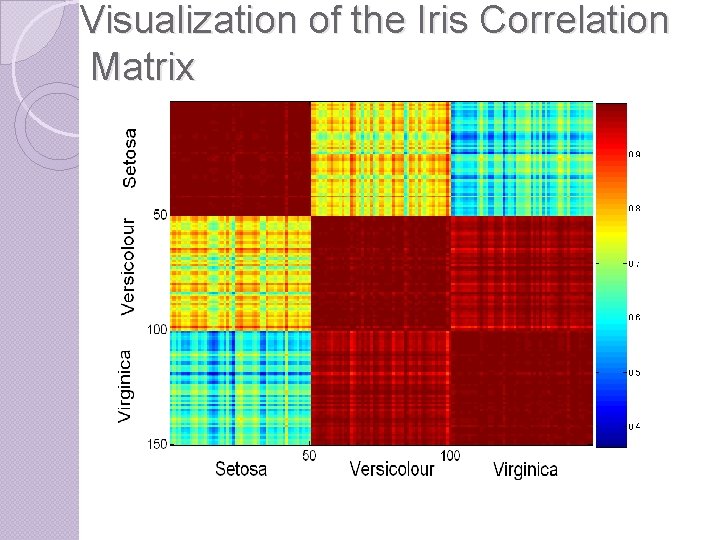
Visualization of the Iris Correlation Matrix
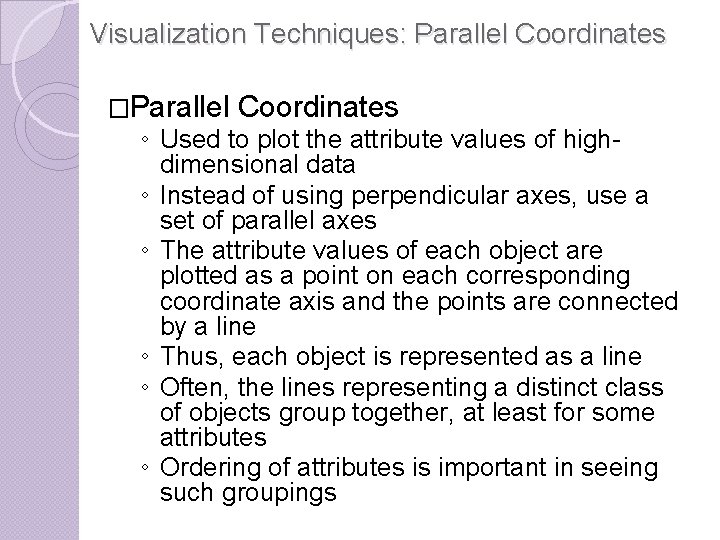
Visualization Techniques: Parallel Coordinates �Parallel Coordinates ◦ Used to plot the attribute values of highdimensional data ◦ Instead of using perpendicular axes, use a set of parallel axes ◦ The attribute values of each object are plotted as a point on each corresponding coordinate axis and the points are connected by a line ◦ Thus, each object is represented as a line ◦ Often, the lines representing a distinct class of objects group together, at least for some attributes ◦ Ordering of attributes is important in seeing such groupings
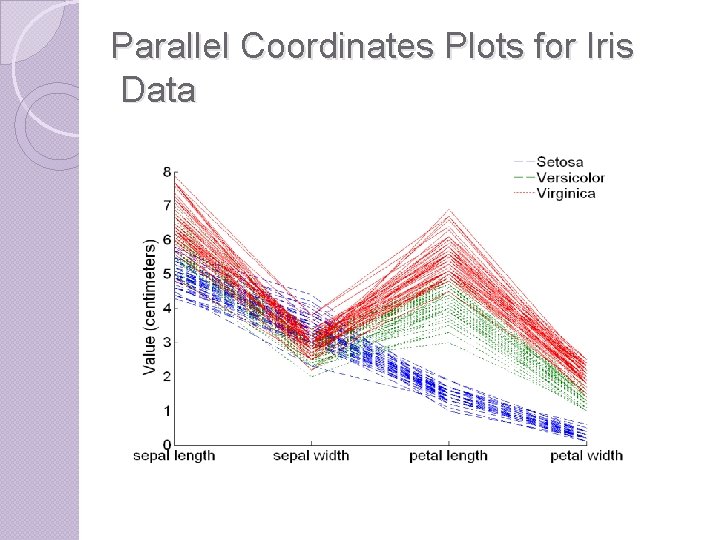
Parallel Coordinates Plots for Iris Data
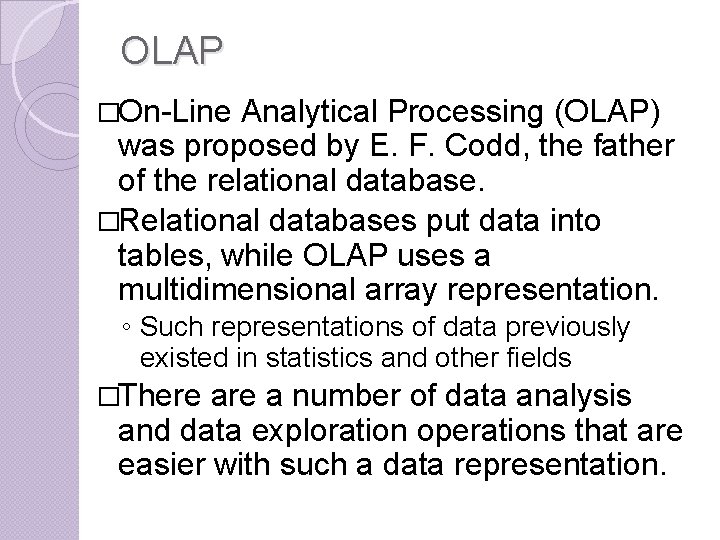
OLAP �On-Line Analytical Processing (OLAP) was proposed by E. F. Codd, the father of the relational database. �Relational databases put data into tables, while OLAP uses a multidimensional array representation. ◦ Such representations of data previously existed in statistics and other fields �There a number of data analysis and data exploration operations that are easier with such a data representation.
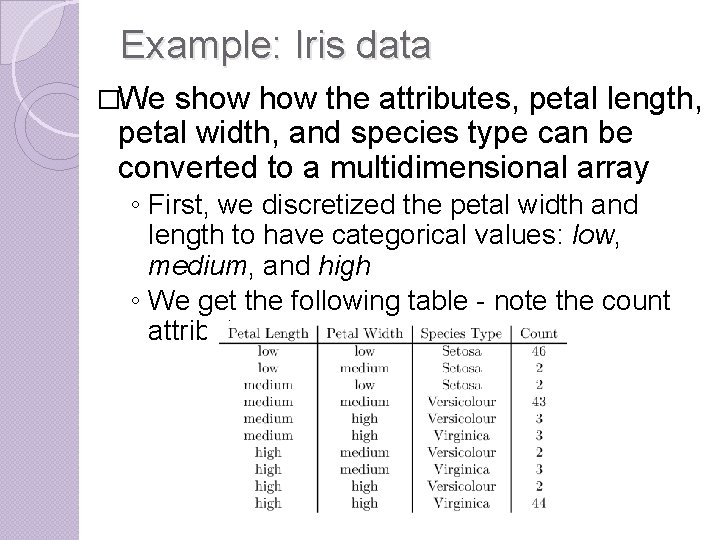
Example: Iris data �We show the attributes, petal length, petal width, and species type can be converted to a multidimensional array ◦ First, we discretized the petal width and length to have categorical values: low, medium, and high ◦ We get the following table - note the count attribute
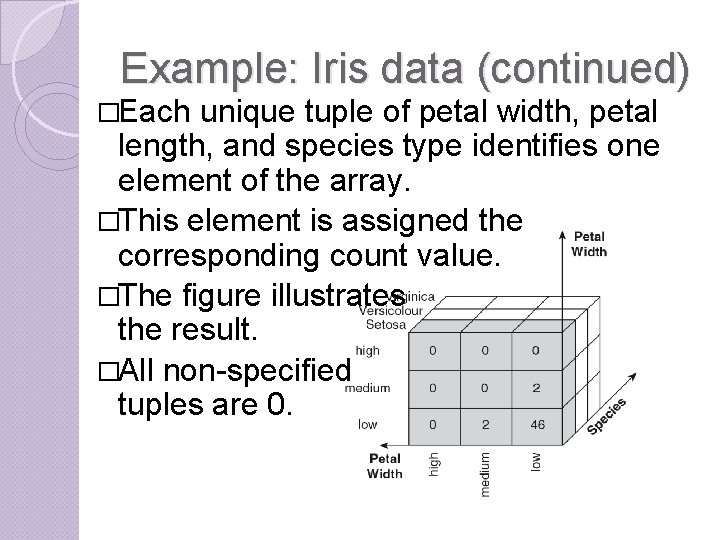
Example: Iris data (continued) �Each unique tuple of petal width, petal length, and species type identifies one element of the array. �This element is assigned the corresponding count value. �The figure illustrates the result. �All non-specified tuples are 0.
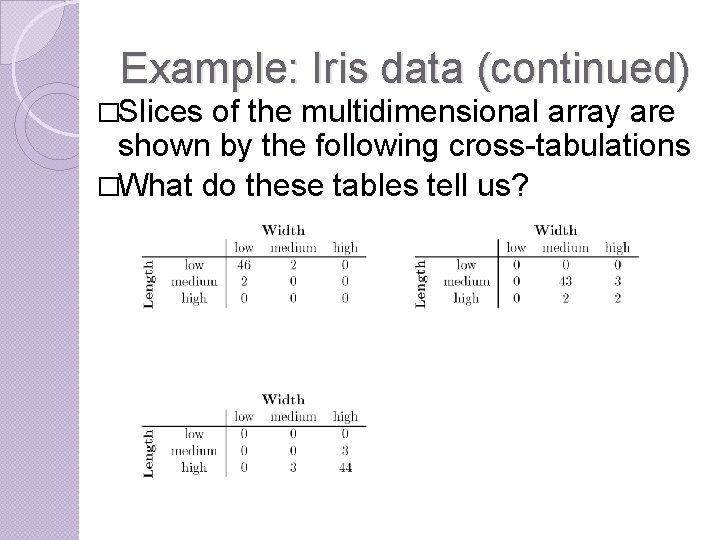
Example: Iris data (continued) �Slices of the multidimensional array are shown by the following cross-tabulations �What do these tables tell us?
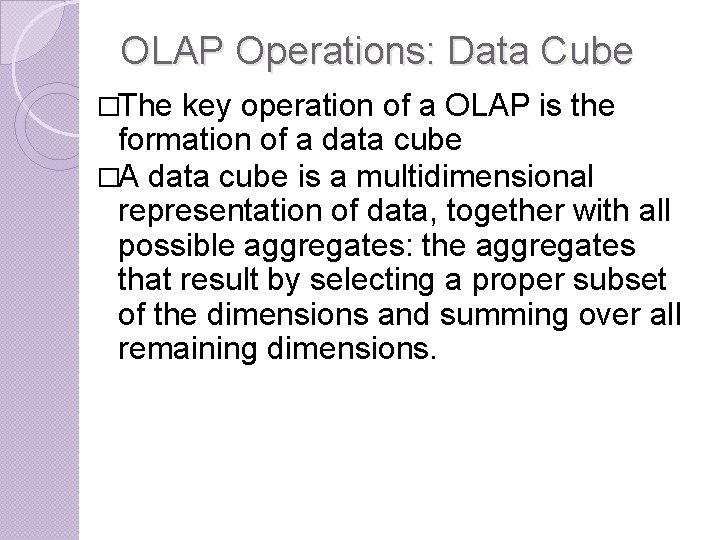
OLAP Operations: Data Cube �The key operation of a OLAP is the formation of a data cube �A data cube is a multidimensional representation of data, together with all possible aggregates: the aggregates that result by selecting a proper subset of the dimensions and summing over all remaining dimensions.
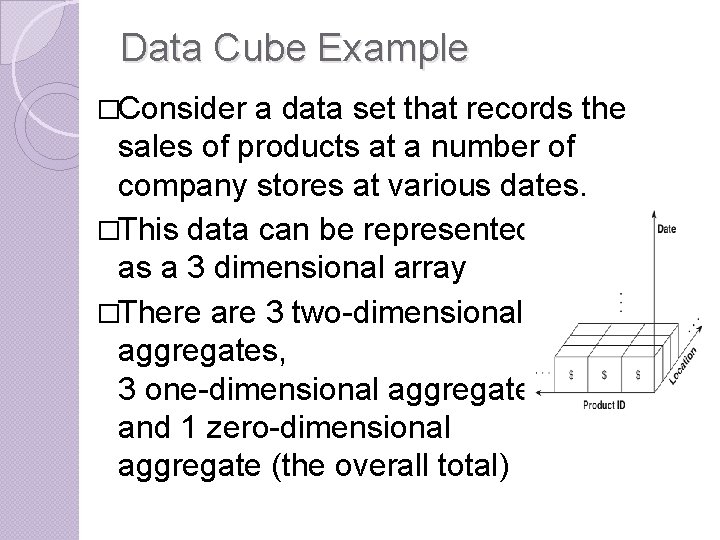
Data Cube Example �Consider a data set that records the sales of products at a number of company stores at various dates. �This data can be represented as a 3 dimensional array �There are 3 two-dimensional aggregates, 3 one-dimensional aggregates, and 1 zero-dimensional aggregate (the overall total)
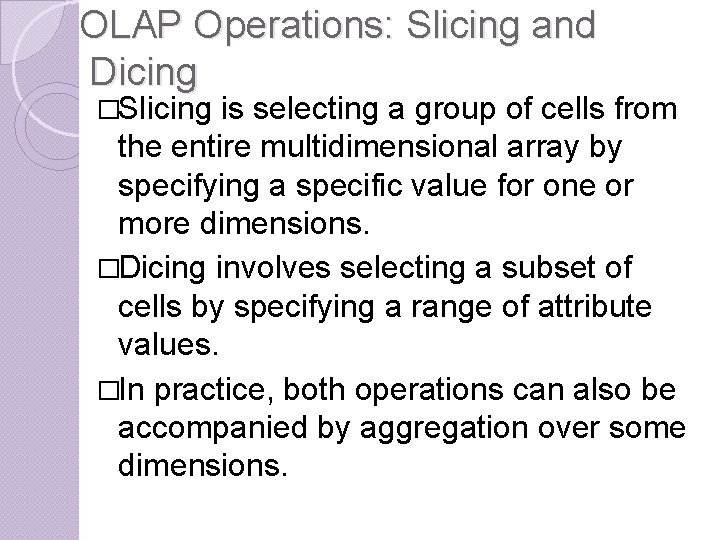
OLAP Operations: Slicing and Dicing �Slicing is selecting a group of cells from the entire multidimensional array by specifying a specific value for one or more dimensions. �Dicing involves selecting a subset of cells by specifying a range of attribute values. �In practice, both operations can also be accompanied by aggregation over some dimensions.
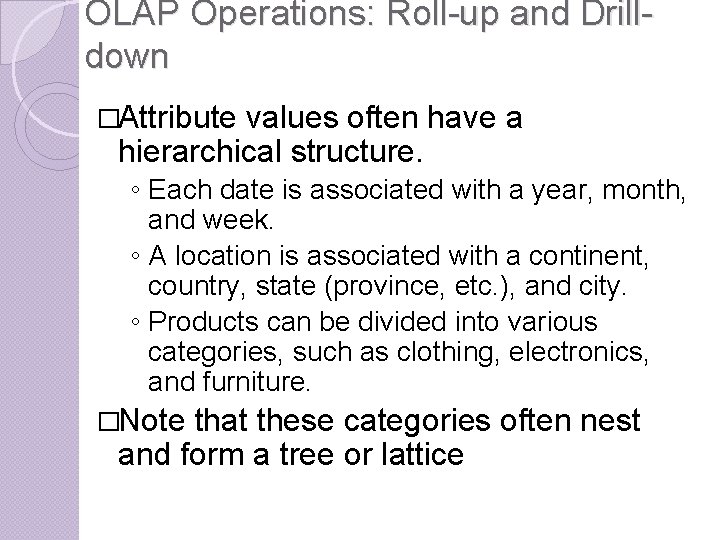
OLAP Operations: Roll-up and Drilldown �Attribute values often have a hierarchical structure. ◦ Each date is associated with a year, month, and week. ◦ A location is associated with a continent, country, state (province, etc. ), and city. ◦ Products can be divided into various categories, such as clothing, electronics, and furniture. �Note that these categories often nest and form a tree or lattice
Mining complex types of data in data mining
Mining multimedia databases in data mining
Strip mining vs open pit mining
Chapter 13 mineral resources and mining
Difference between strip mining and open pit mining
Difference between text mining and web mining
Data reduction in data mining
Data mining in data warehouse
What is missing data in data mining
Concept hierarchy generation for nominal data
Data reduction in data mining
Data reduction in data mining
Data cube technology in data mining
Data reduction in data mining
Data warehouse dan data mining
Data mining dan data warehouse
Data warehousing and data mining in crm
Descriptive mining of complex data objects
Olap
Noisy data in data mining
4 tier architecture of data warehouse
Markku roiha
Data compression in data mining
Introduction to data warehouse
Data warehouse dan data mining
Complex data types in data mining
Unsupervised learning in data mining
Motivation and importance of data mining
Data mining concepts and techniques slides
Reporting and query tools
Pump it up: data mining the water table
Sebutkan tahapan utama proses data mining!
Sebutkan 5 peran utama data mining
Olap stands for: *
Bloom filter for stream data mining
What are the steps in mining process?
Data mining midterm exam with solutions
Multidimensional space in data mining
Data mining roadmap
Pentaho weka
Spatial data mining applications
Walmart data mining
Data mining spss
Spss 14
Apriori algorithm
Objective of data mining
Emr data mining
Cur decomposition in data mining
Dss in data mining
6-8-10 square rule
Overfitting and pruning in data mining
Svd data mining