SPAMMERS ON TWITTER 1 1 TWITTER GAMES HOW
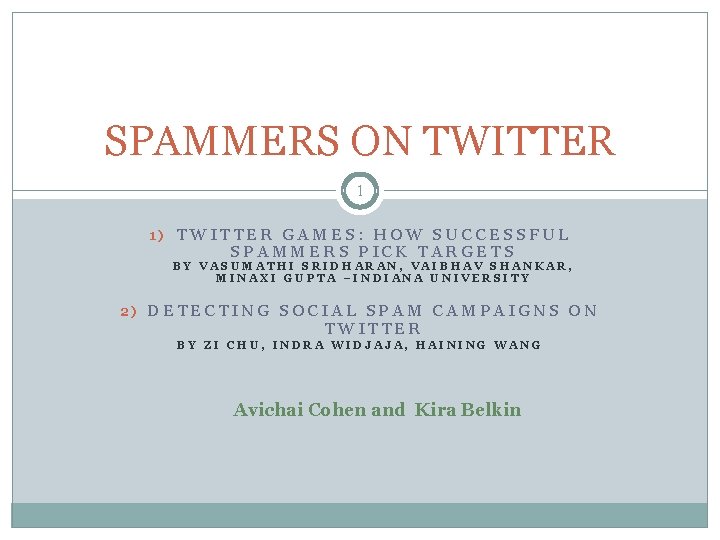
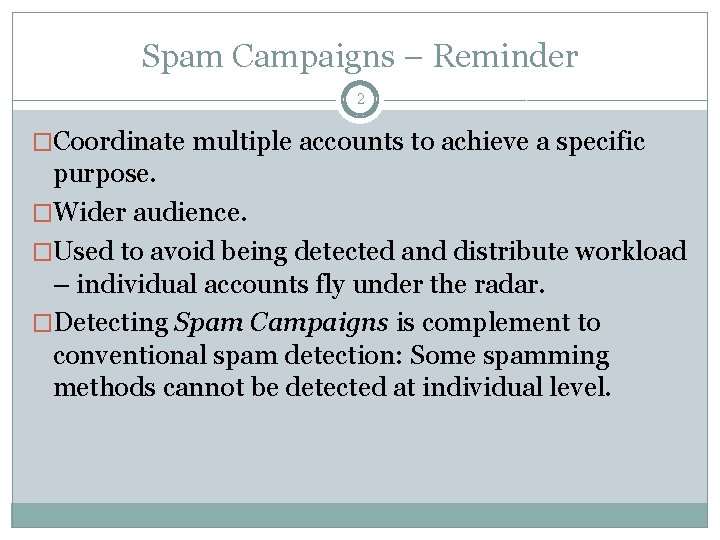
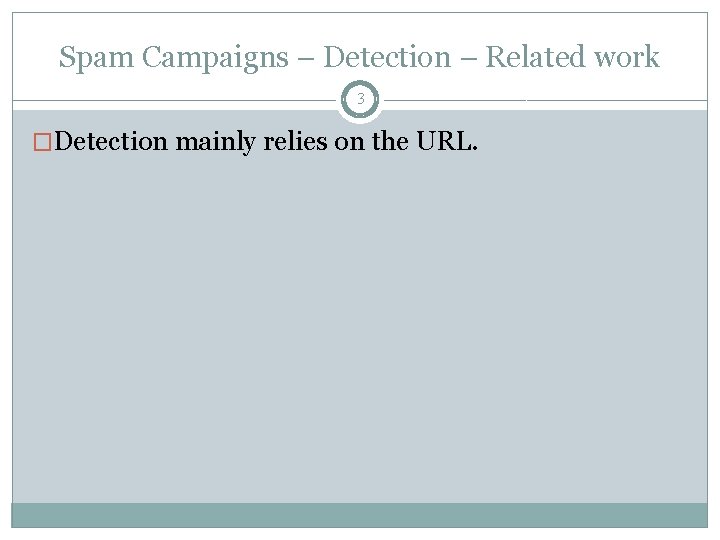
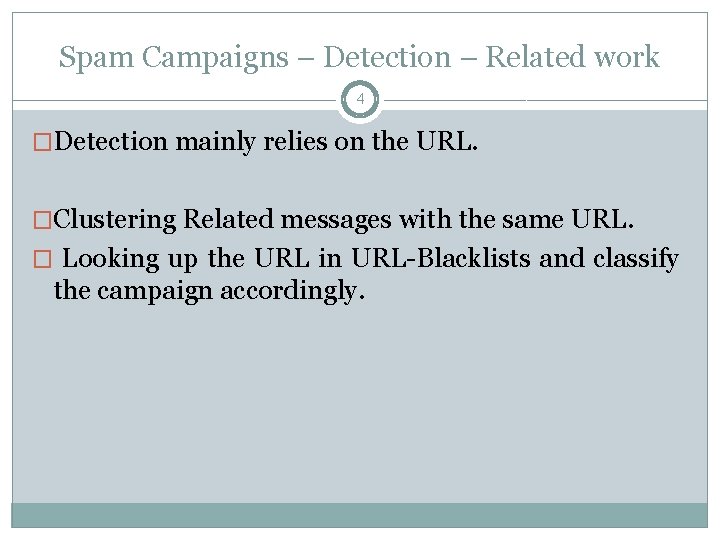
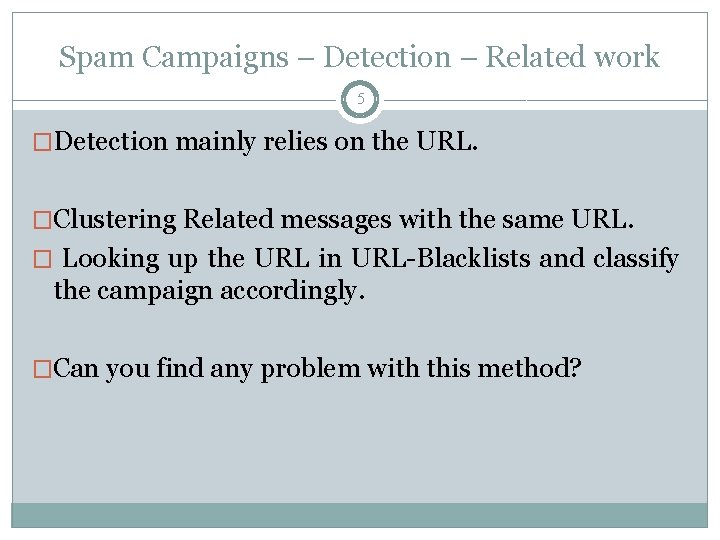
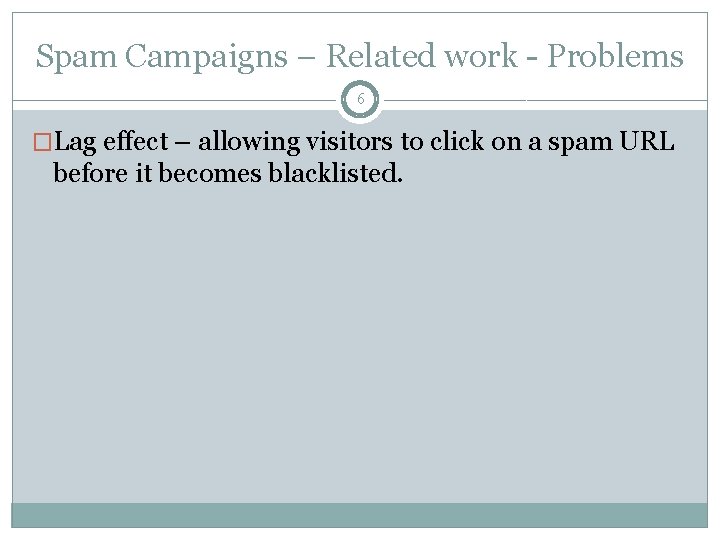
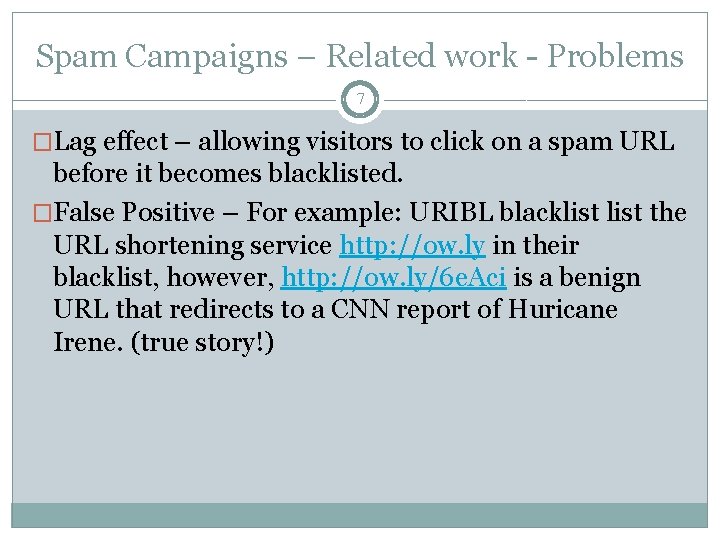
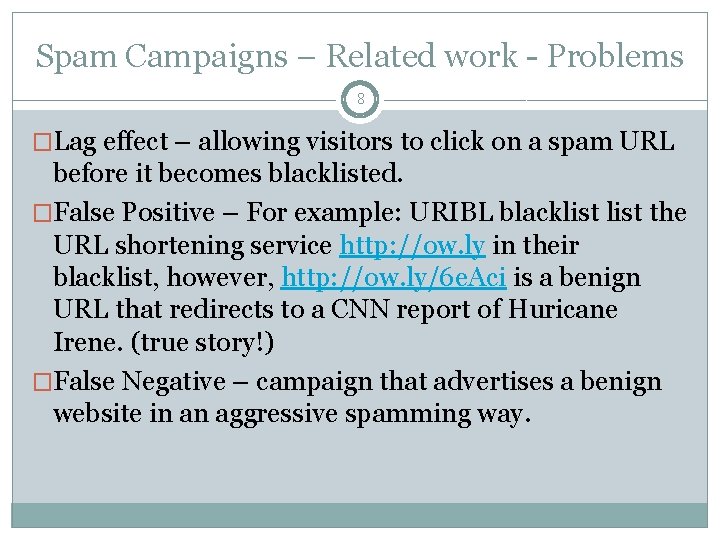
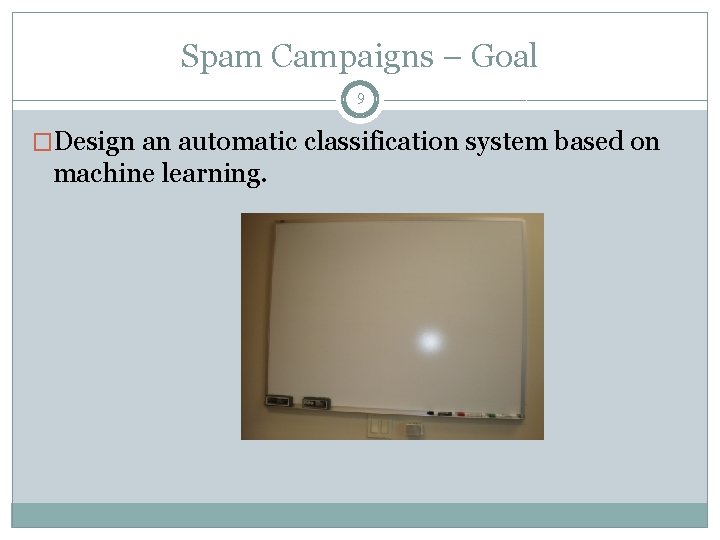
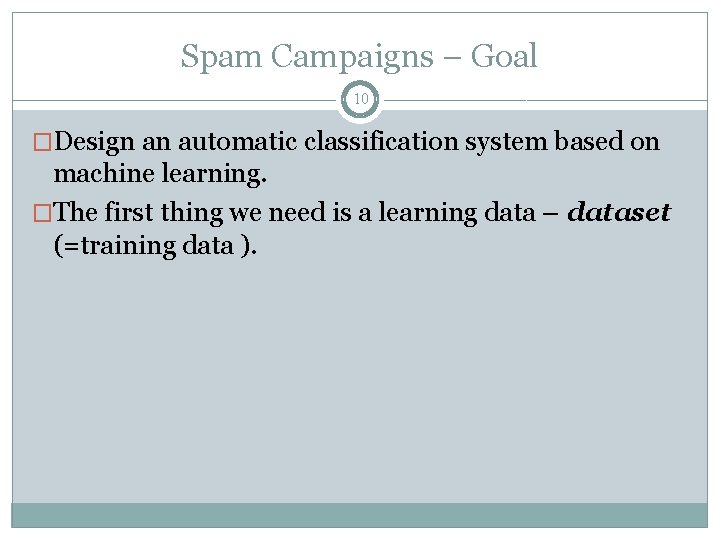
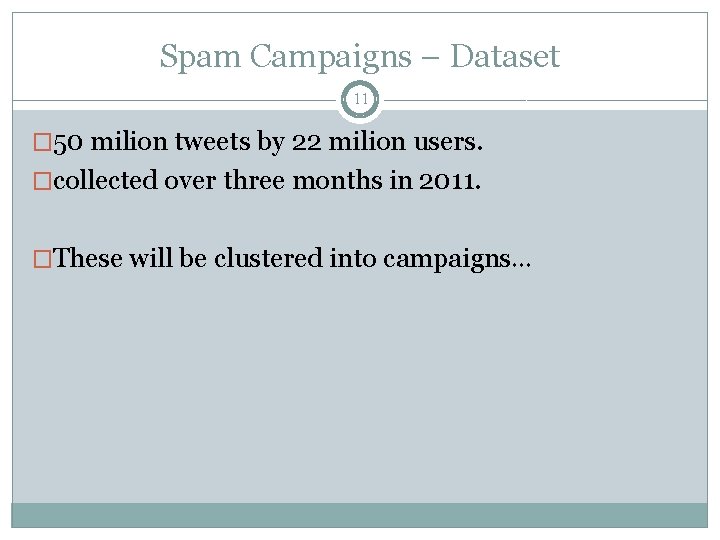
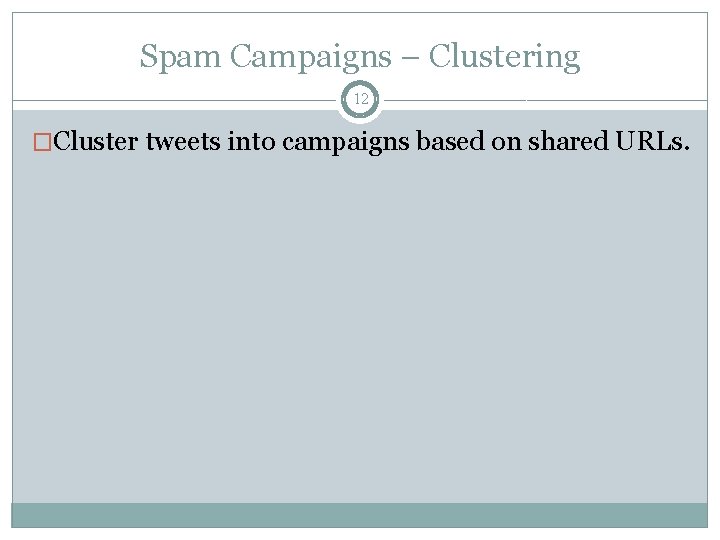
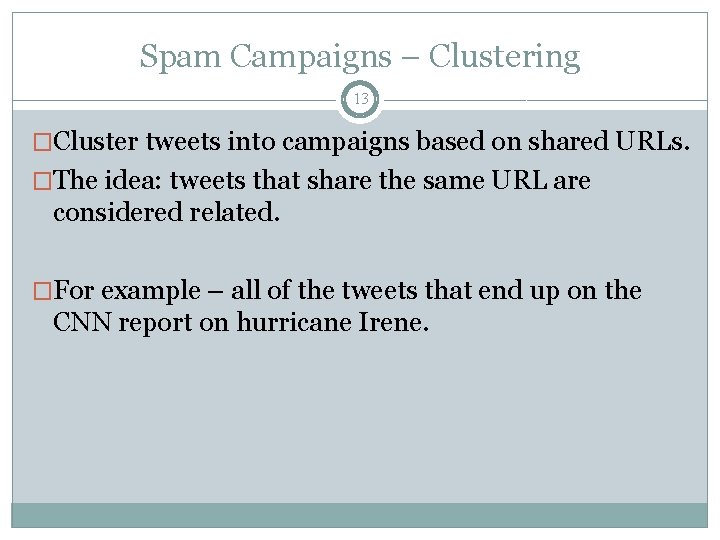
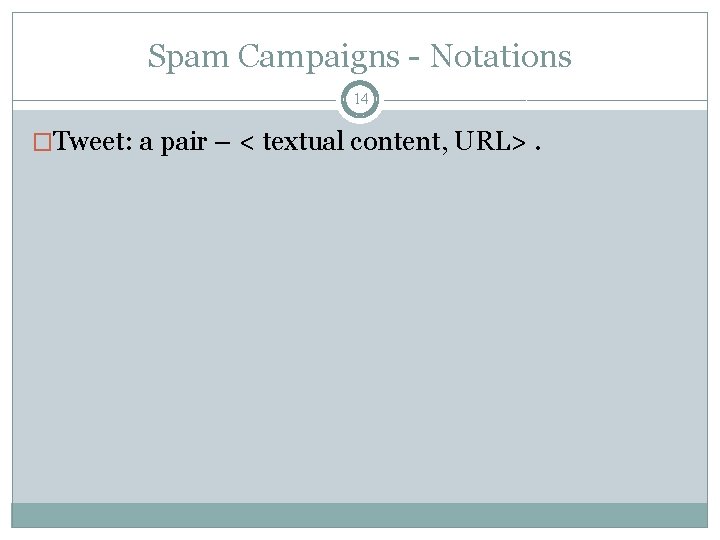
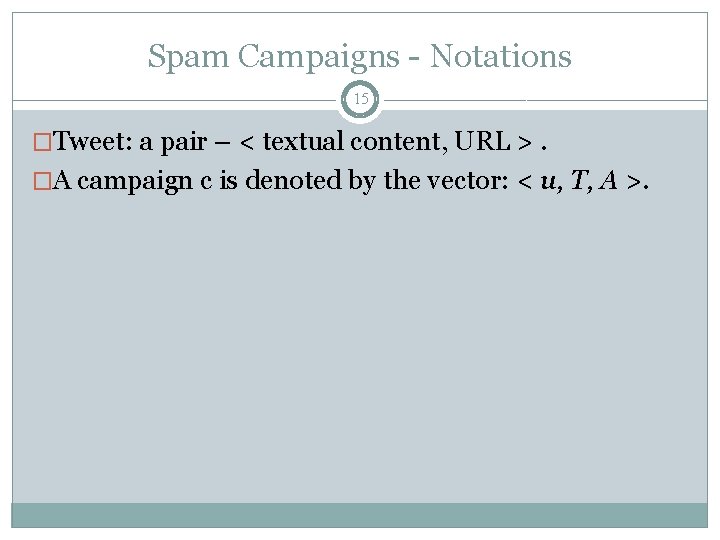
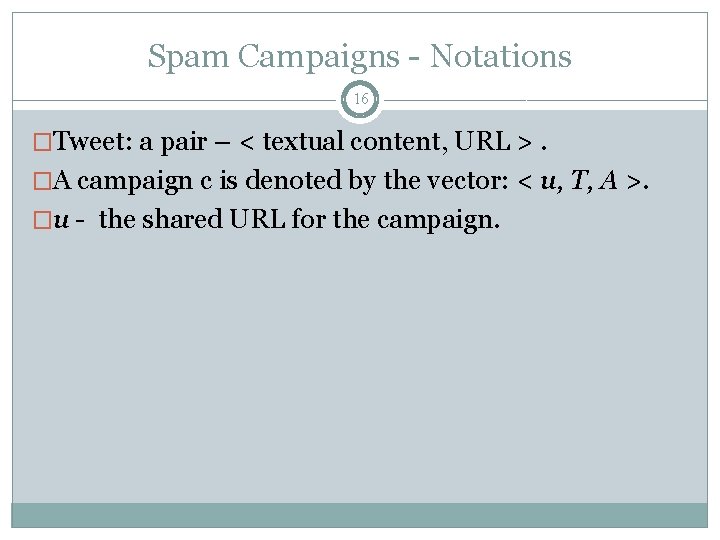
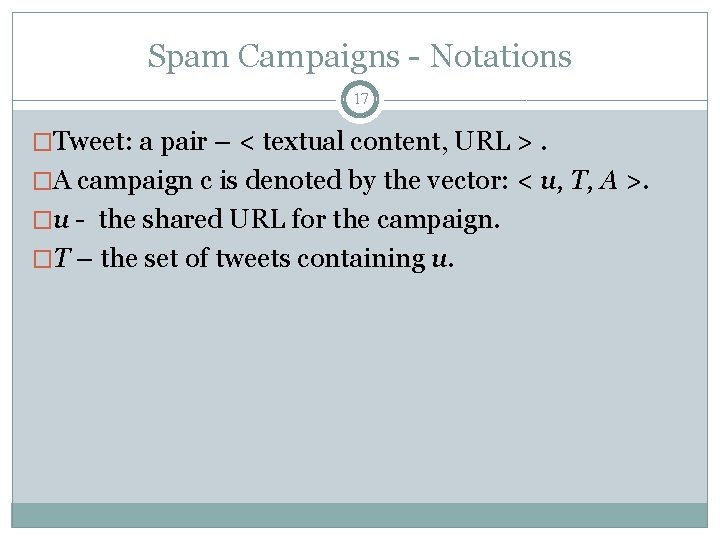
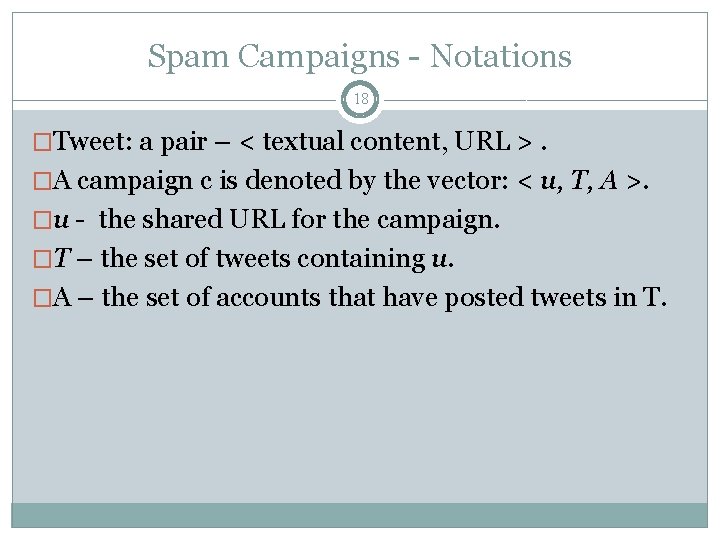
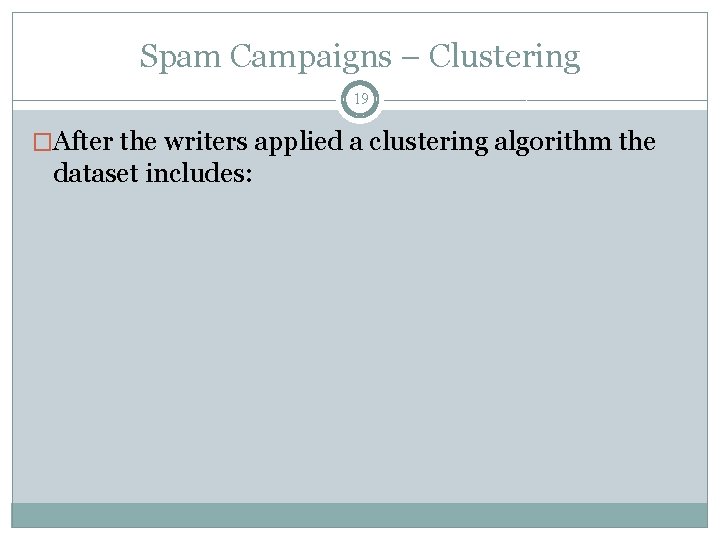
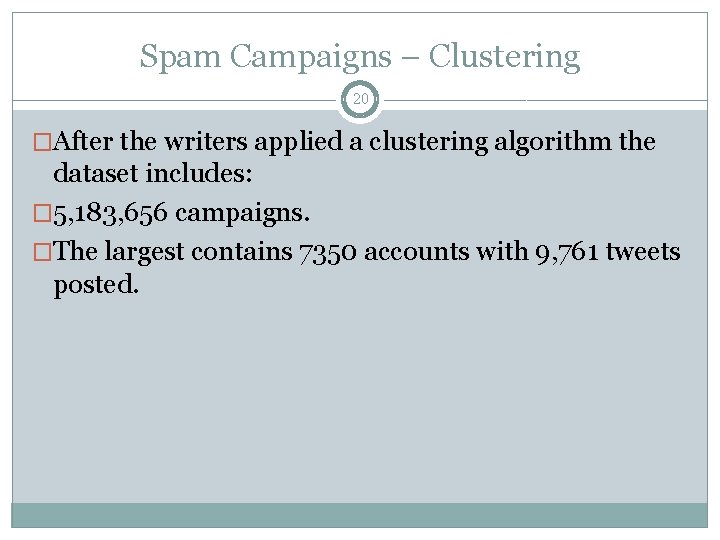
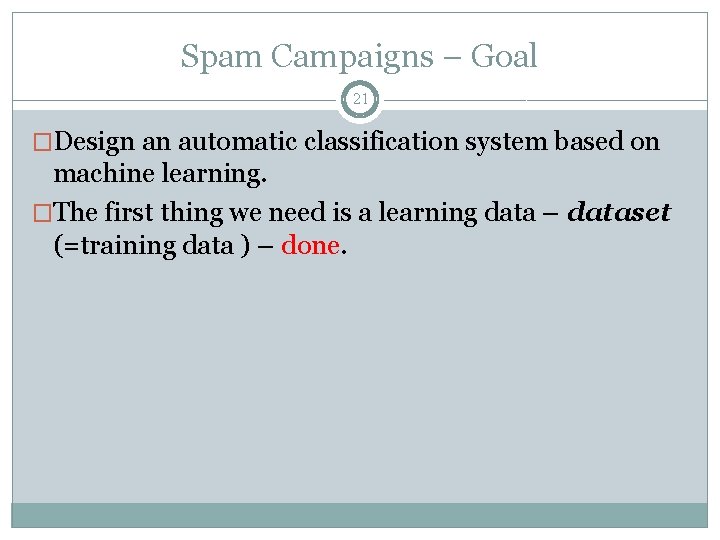
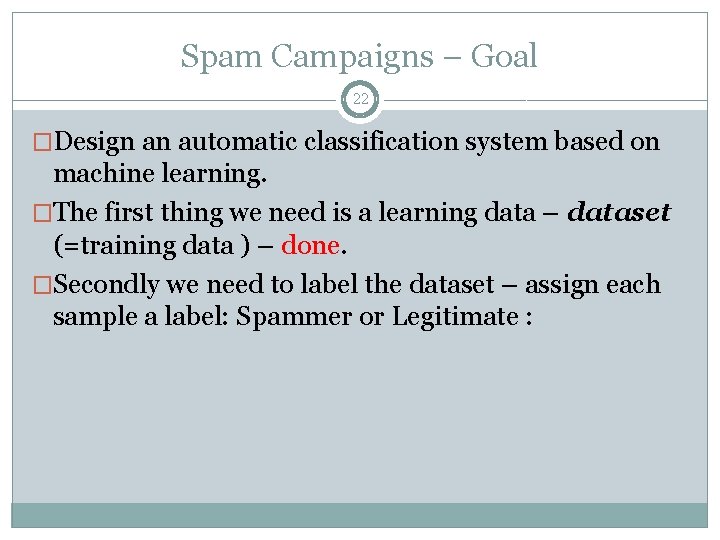
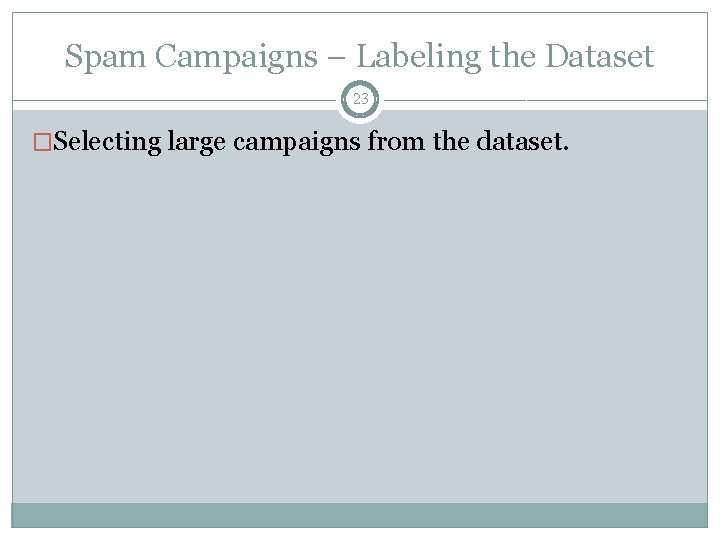
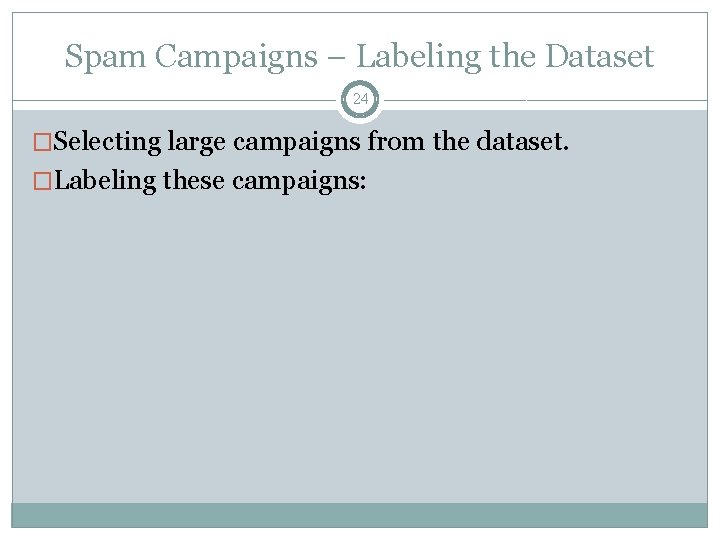
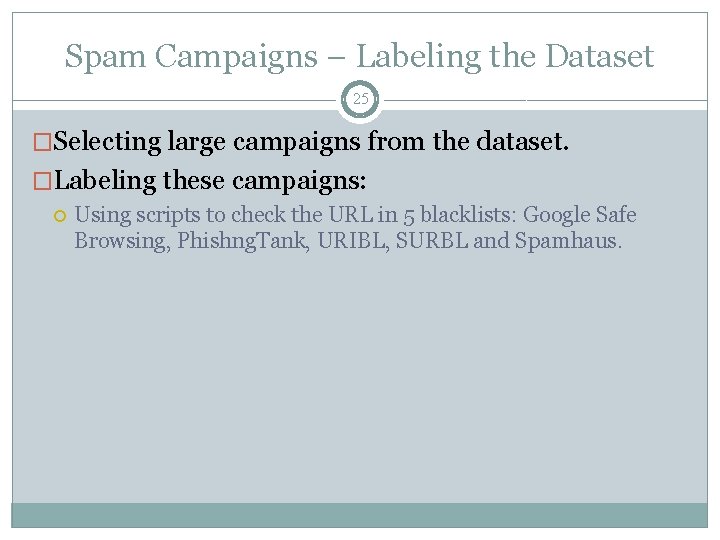
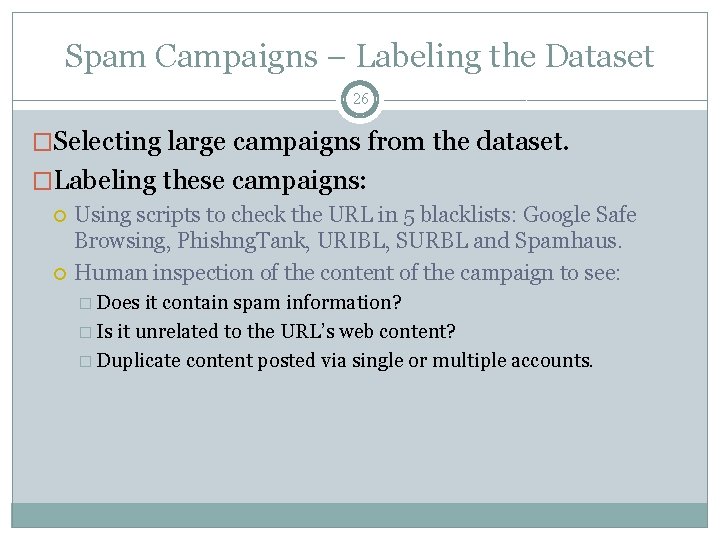
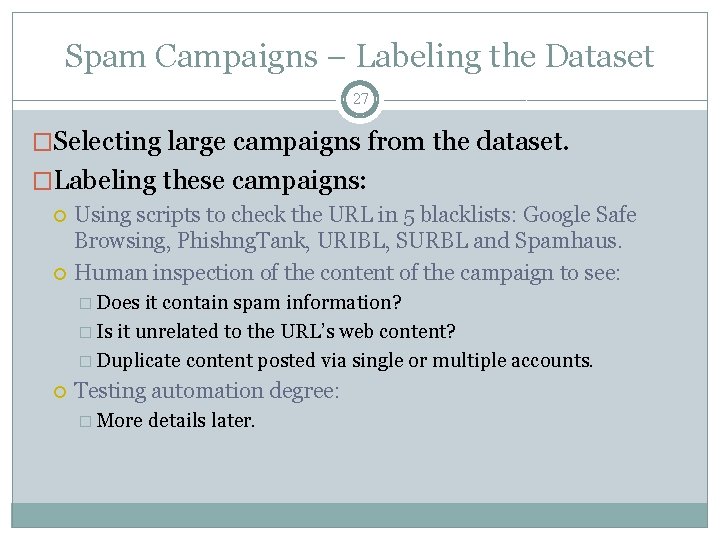
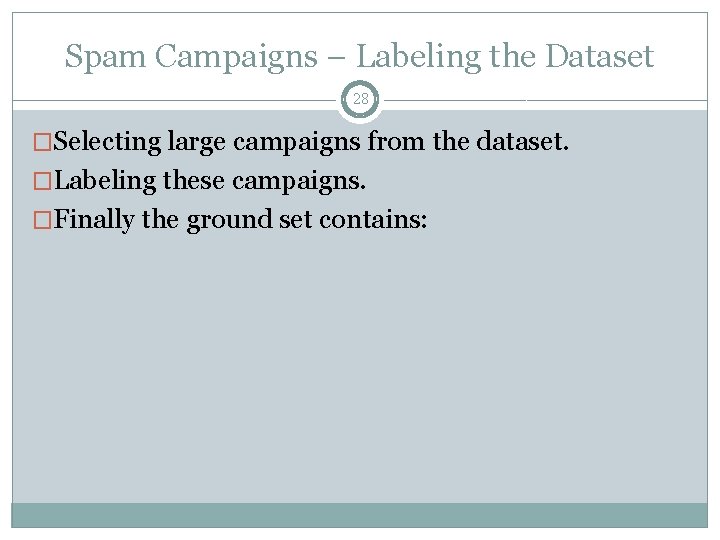
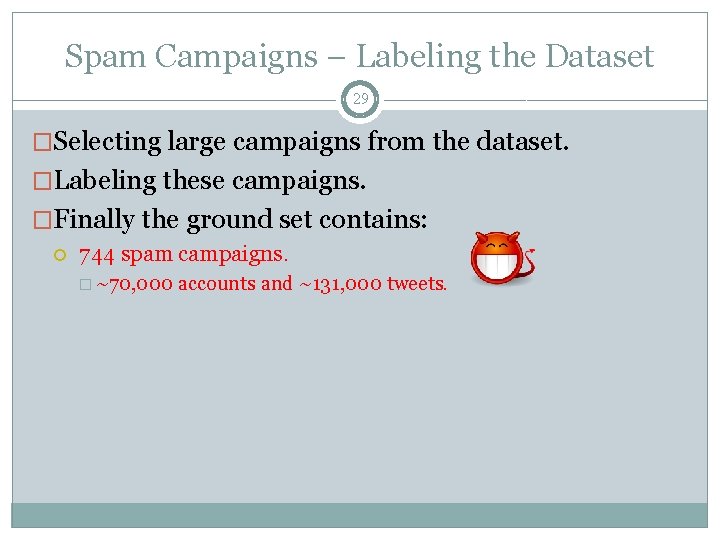
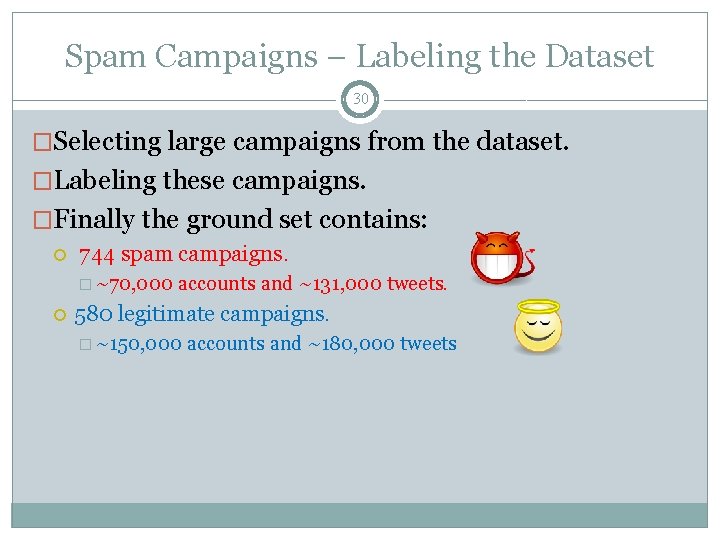
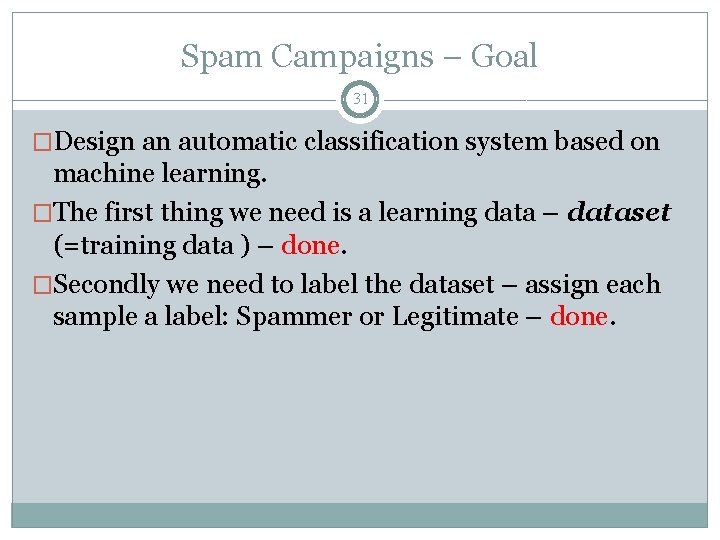
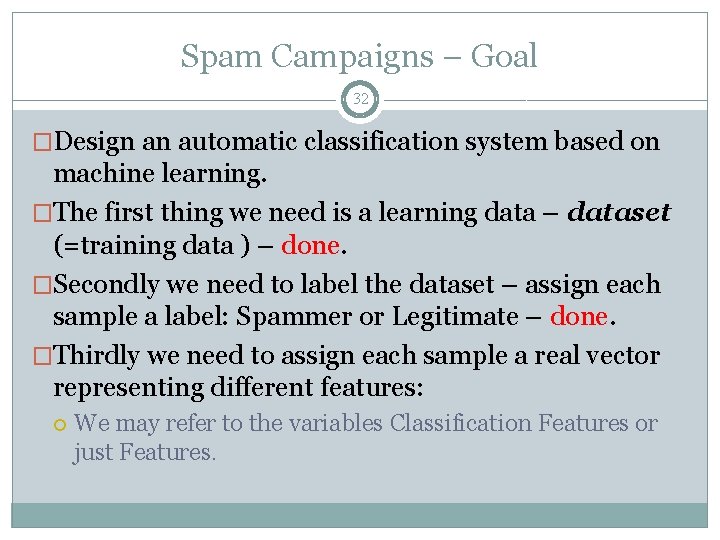
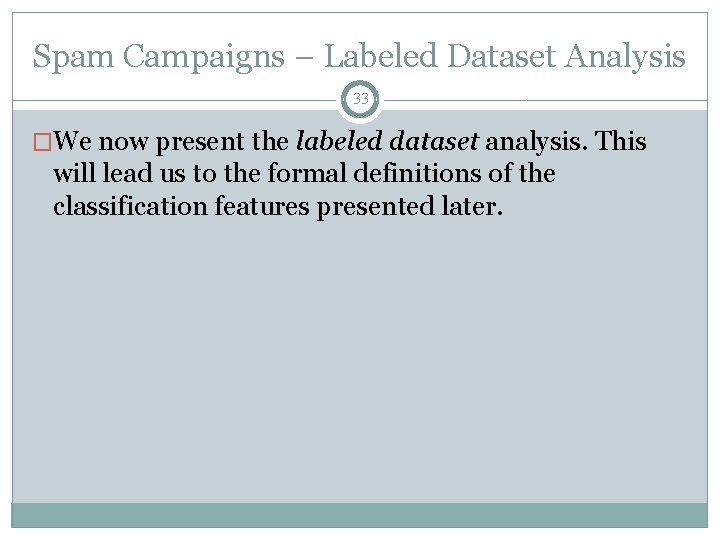
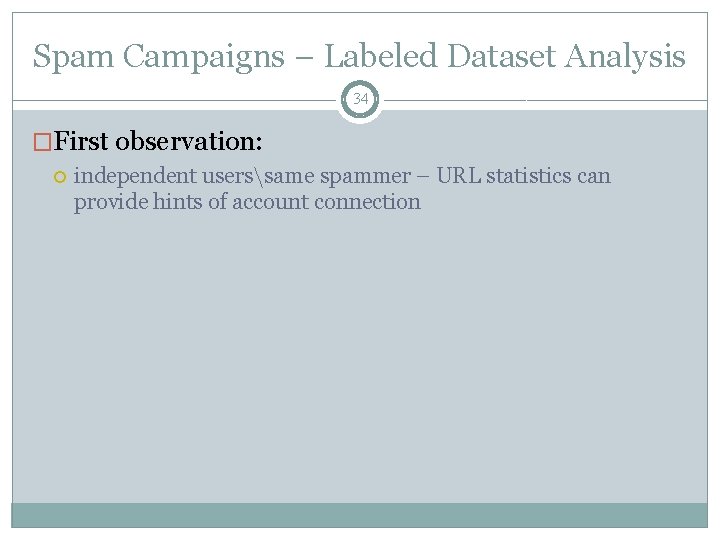
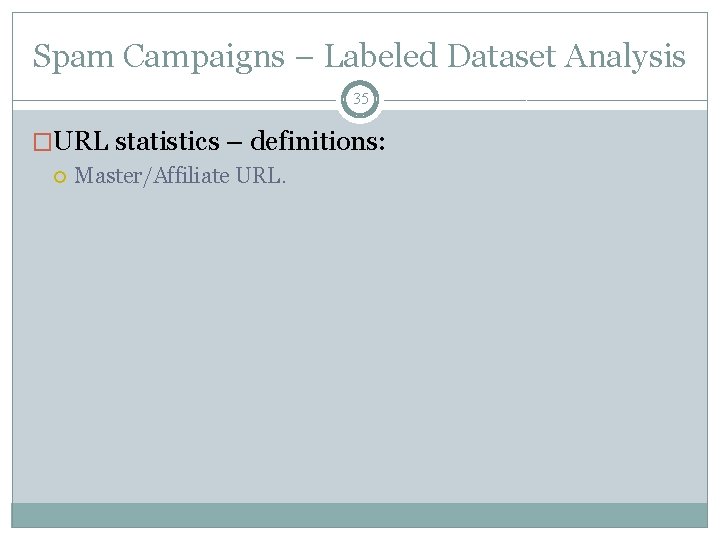
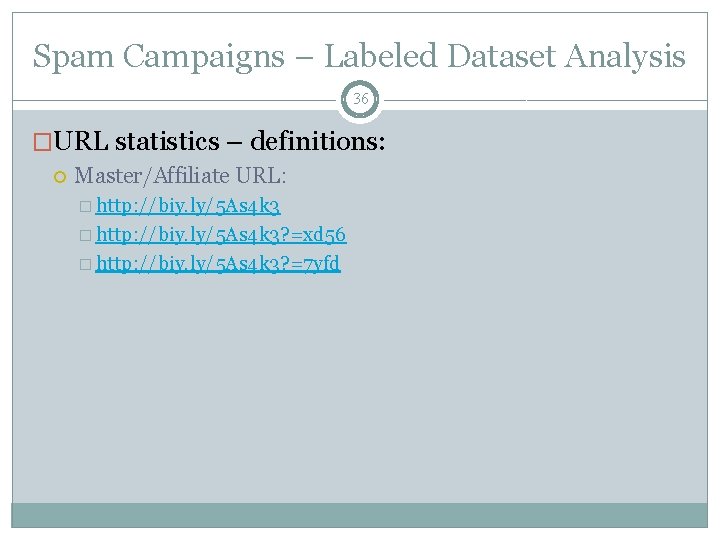
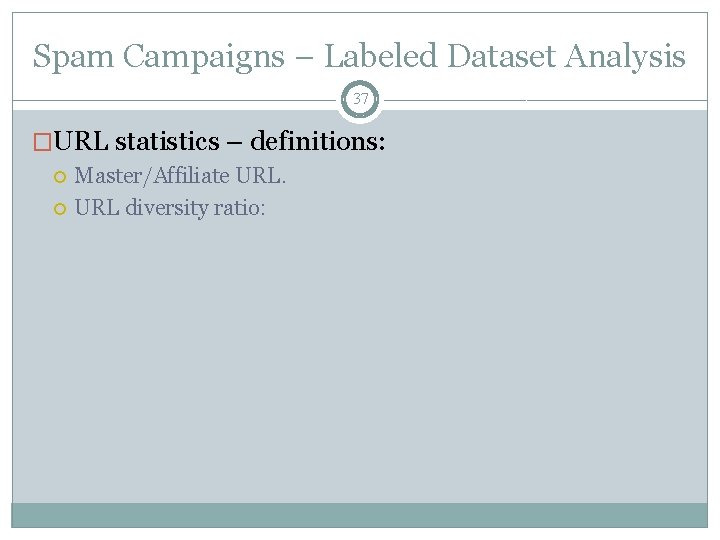
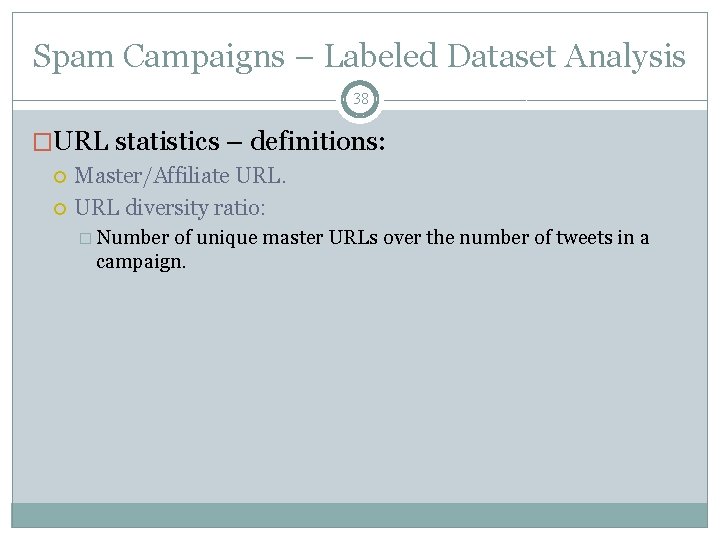
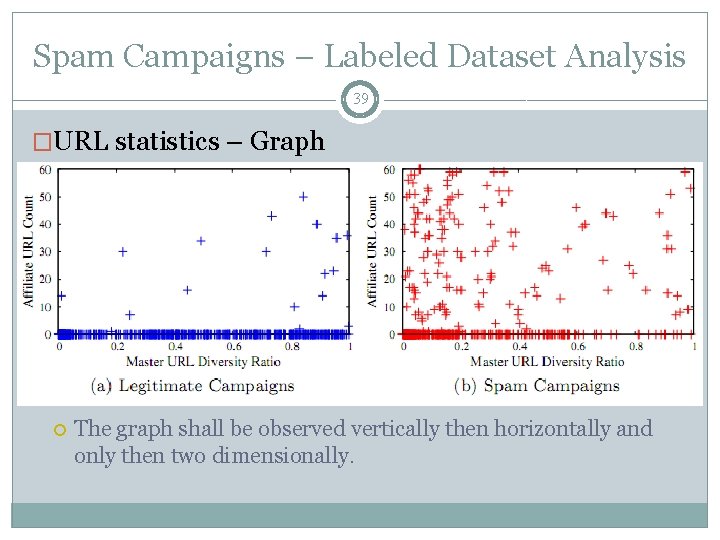
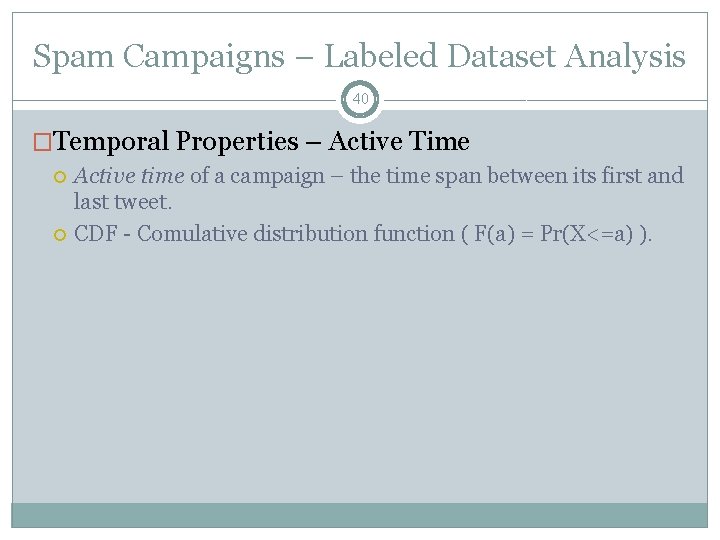
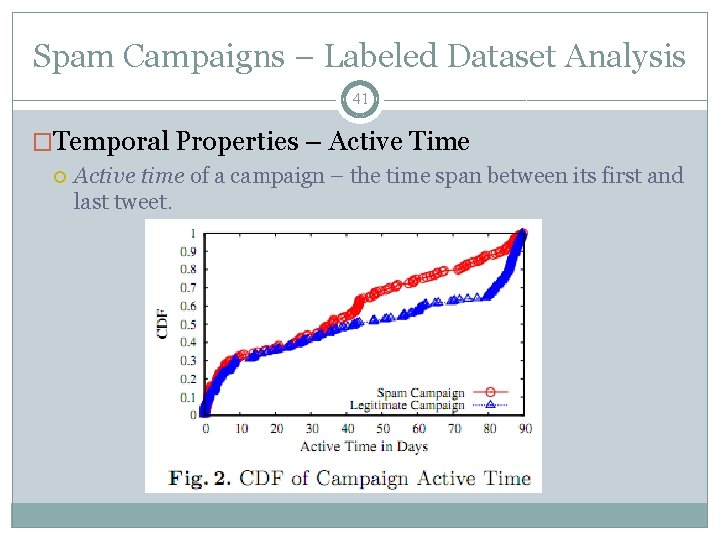
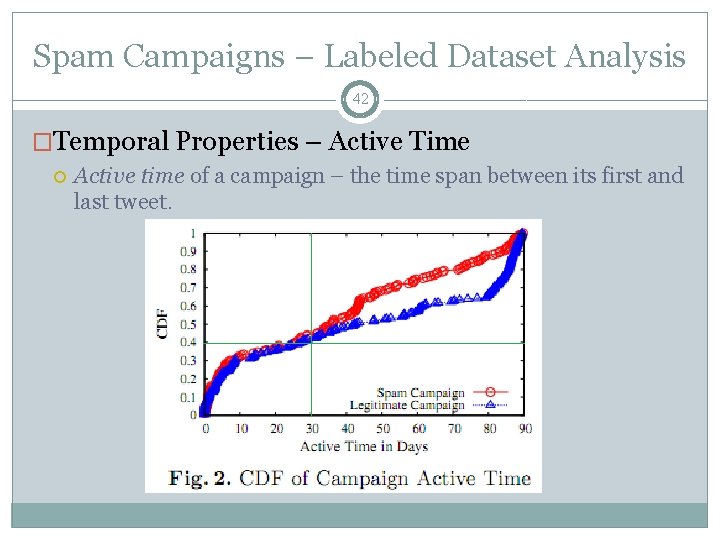
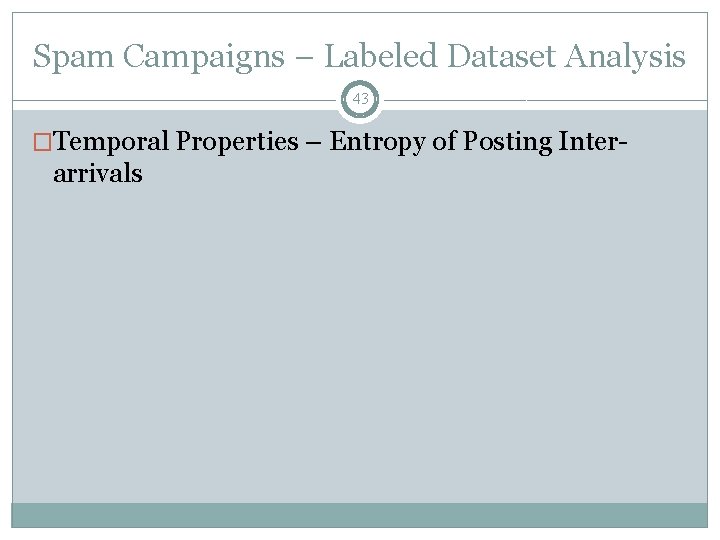
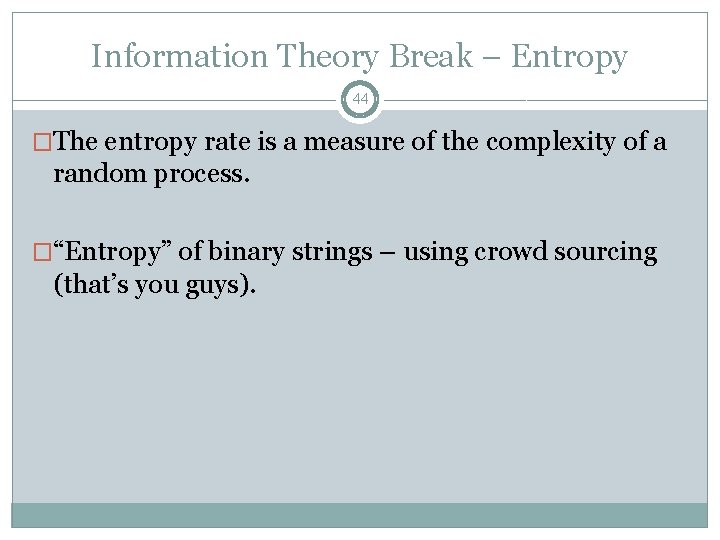
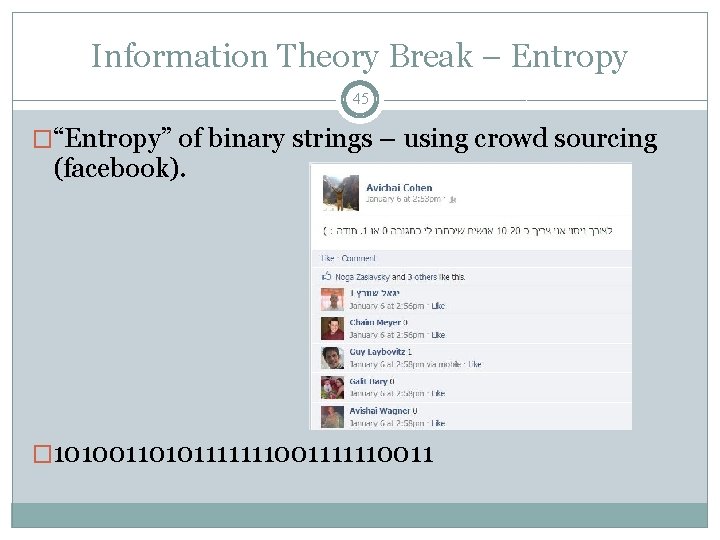
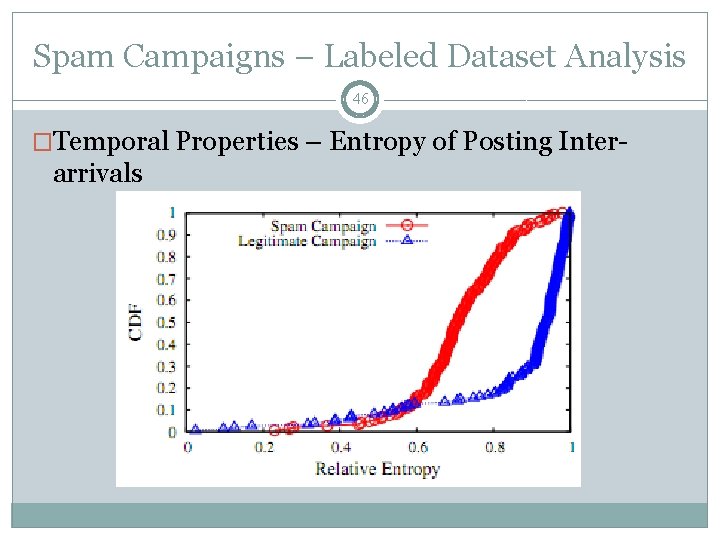
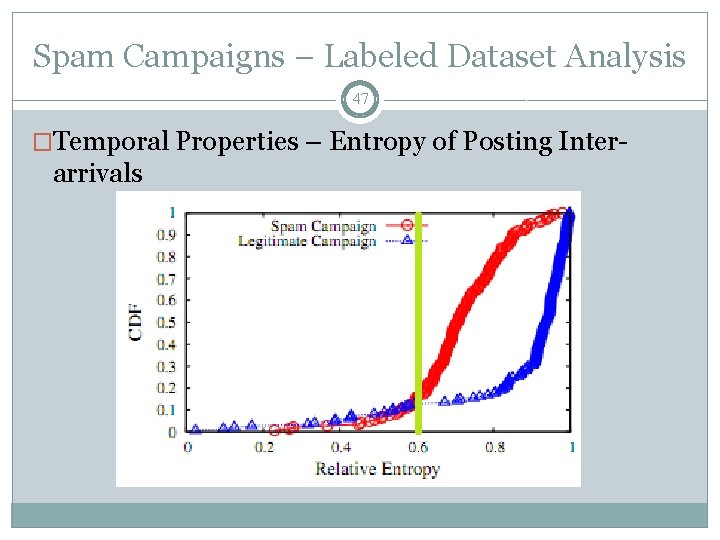
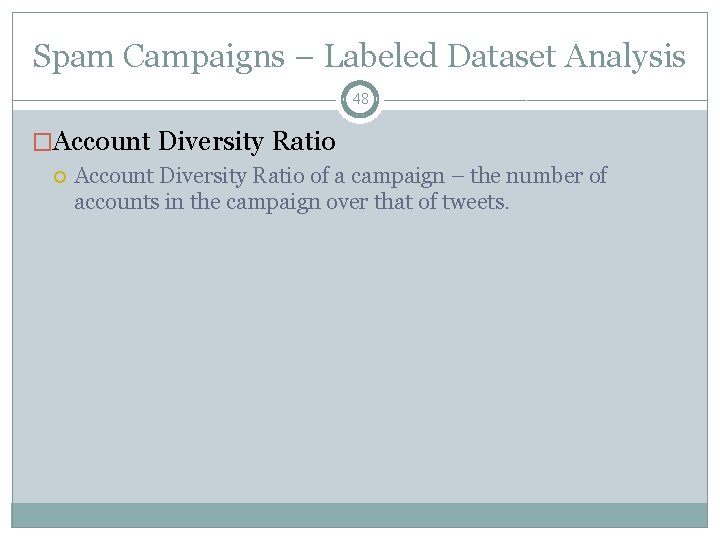
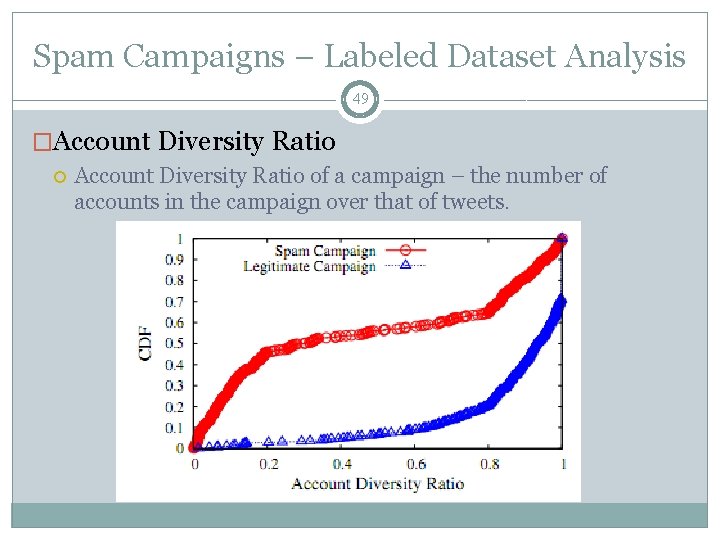
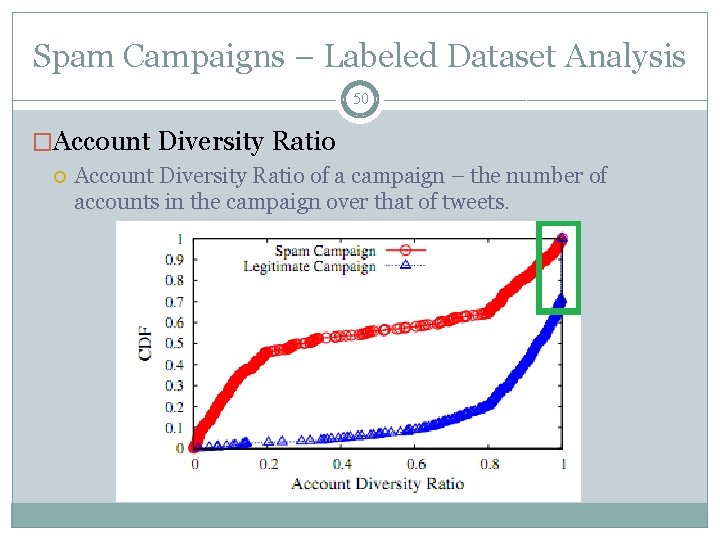
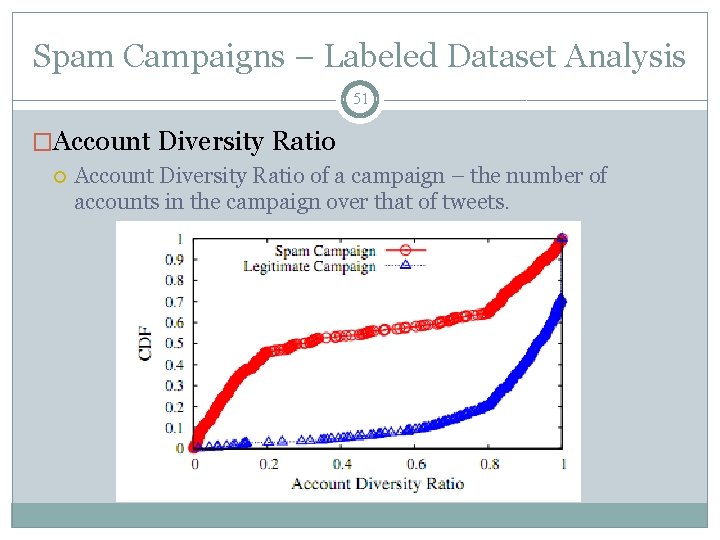
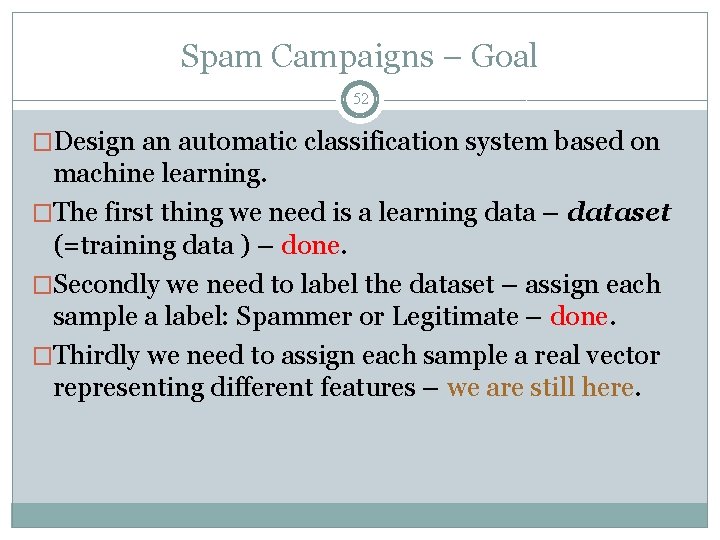
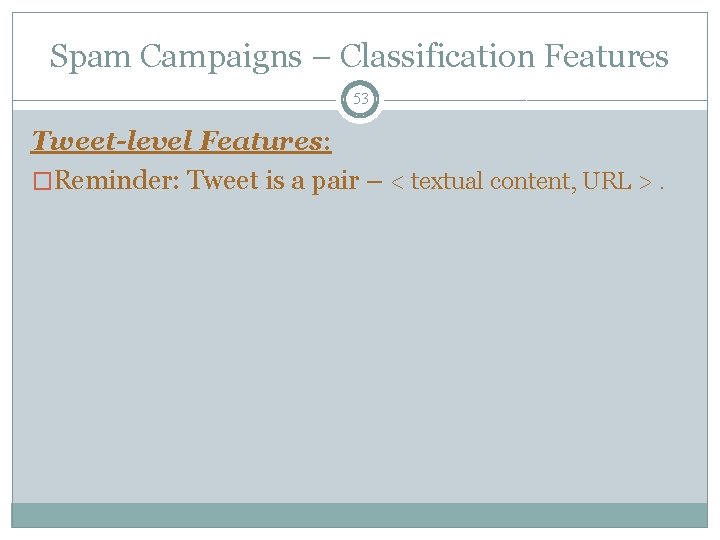
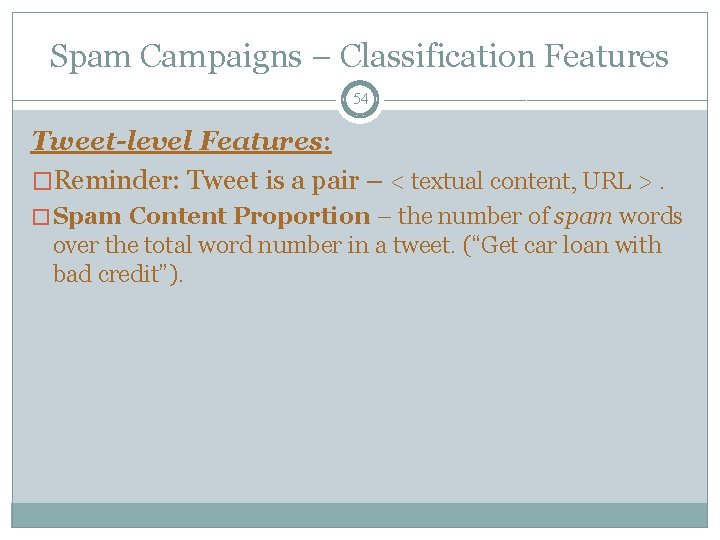
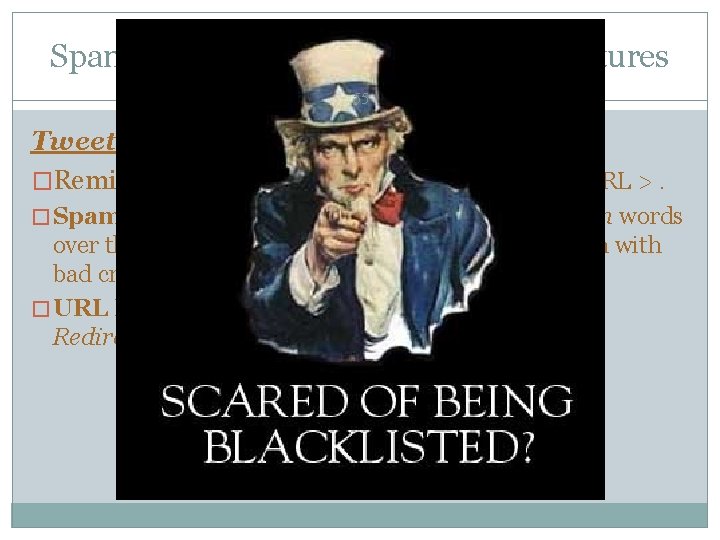
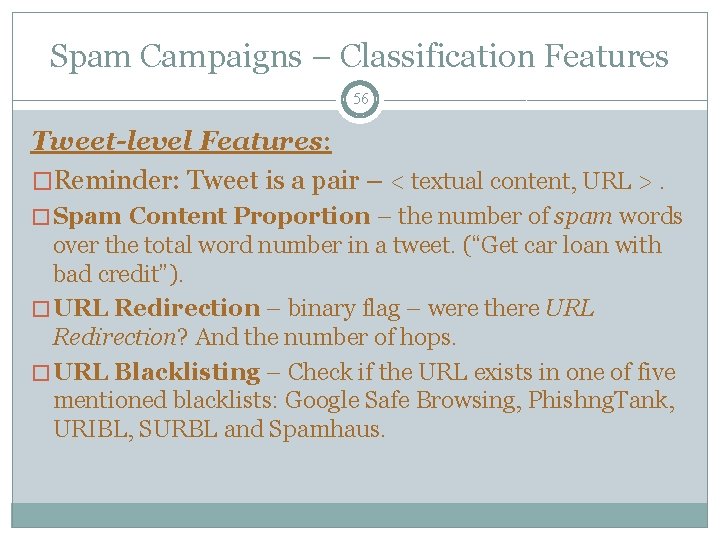
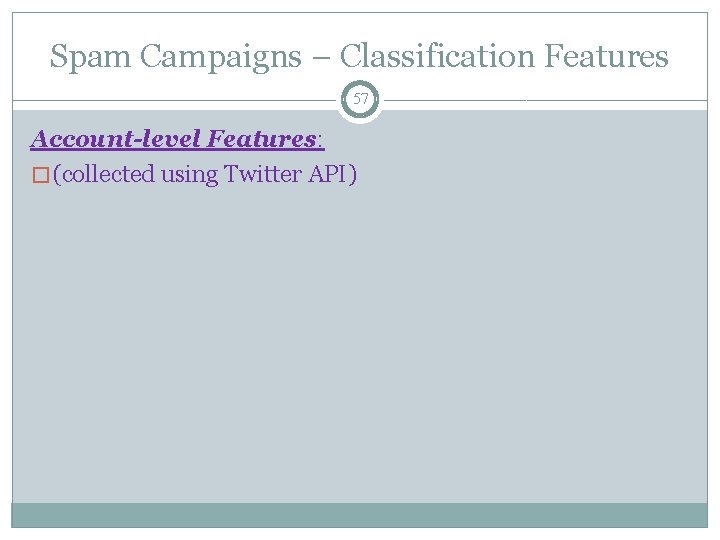
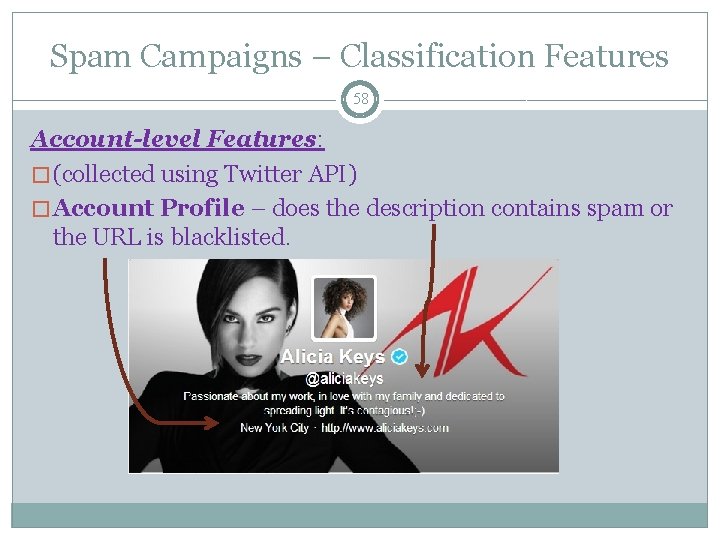
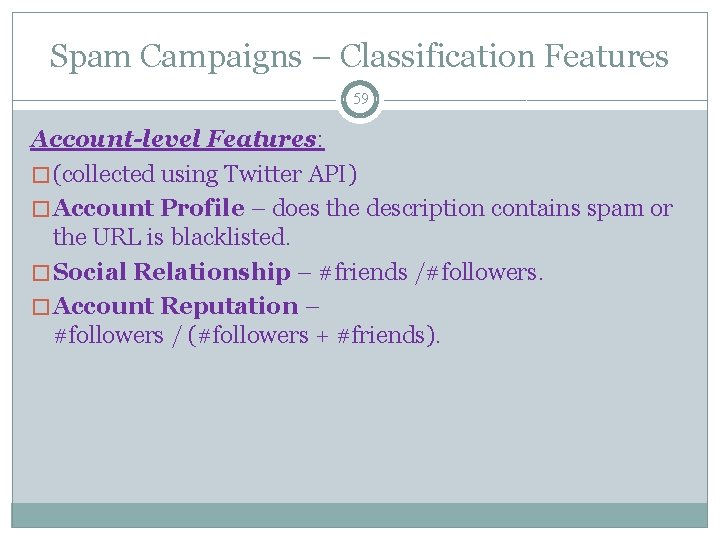
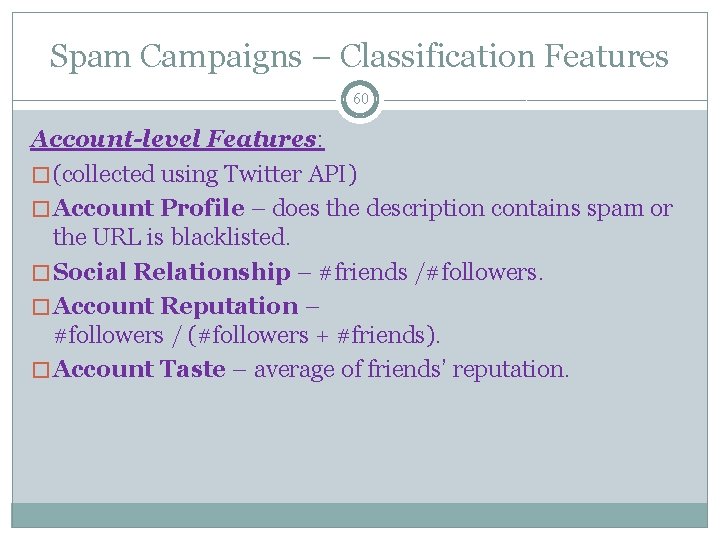
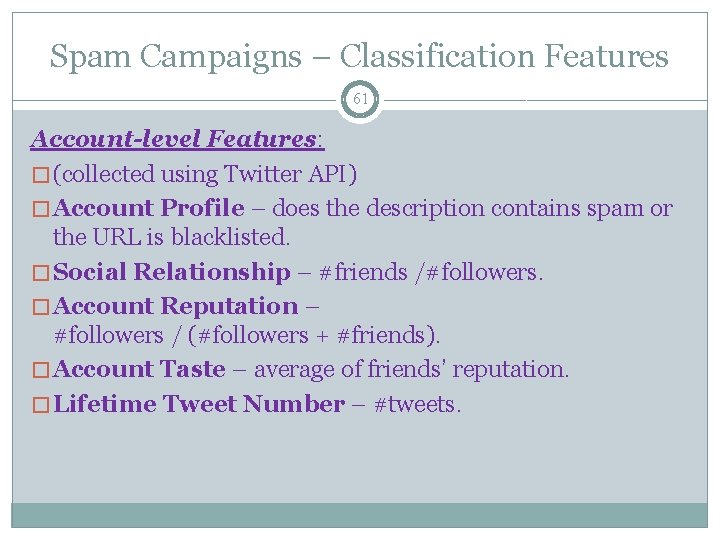
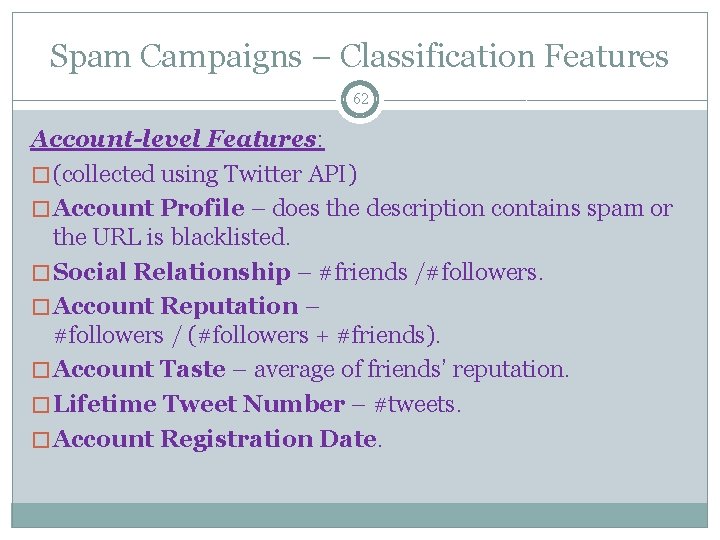
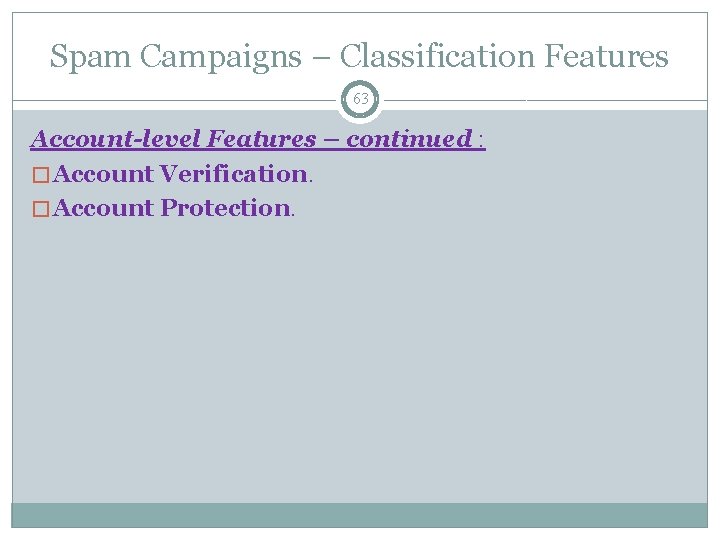
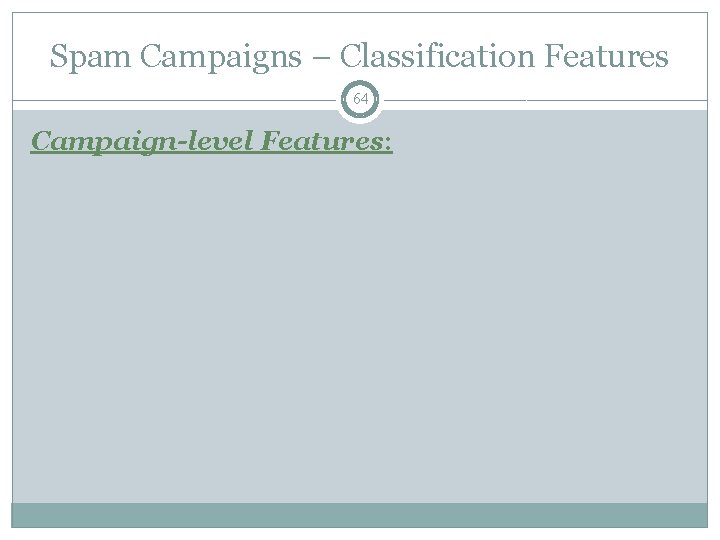
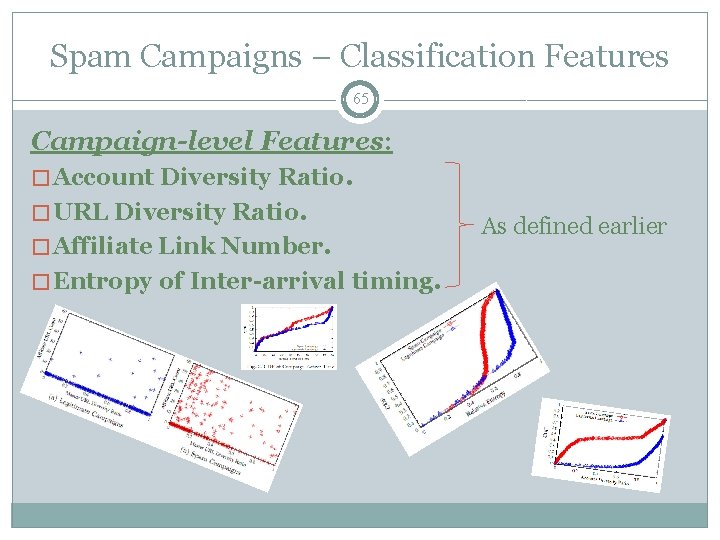
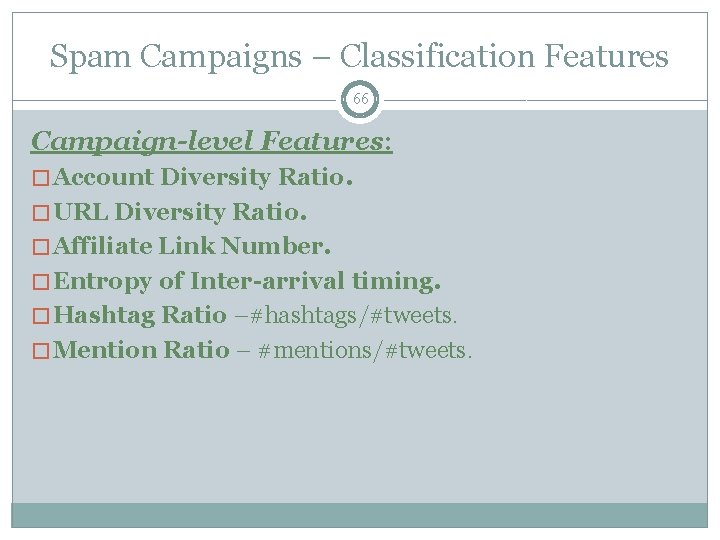
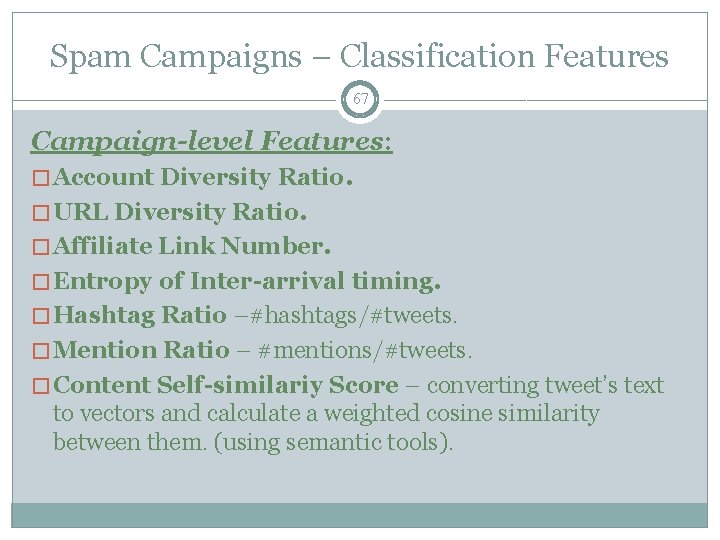
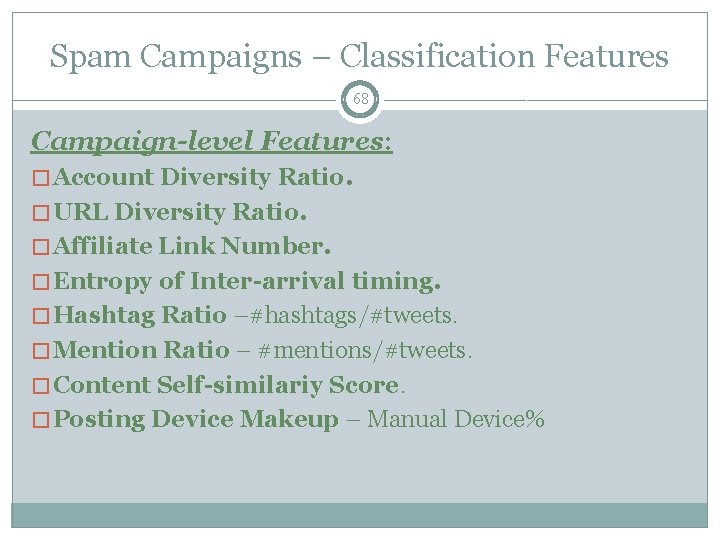
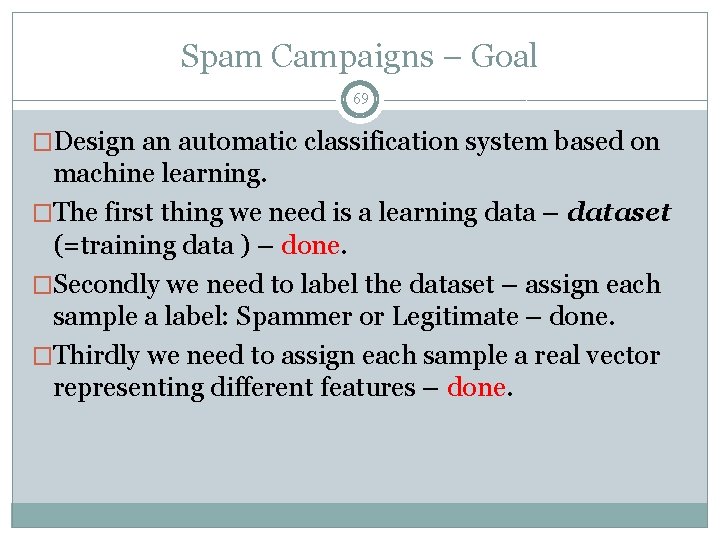
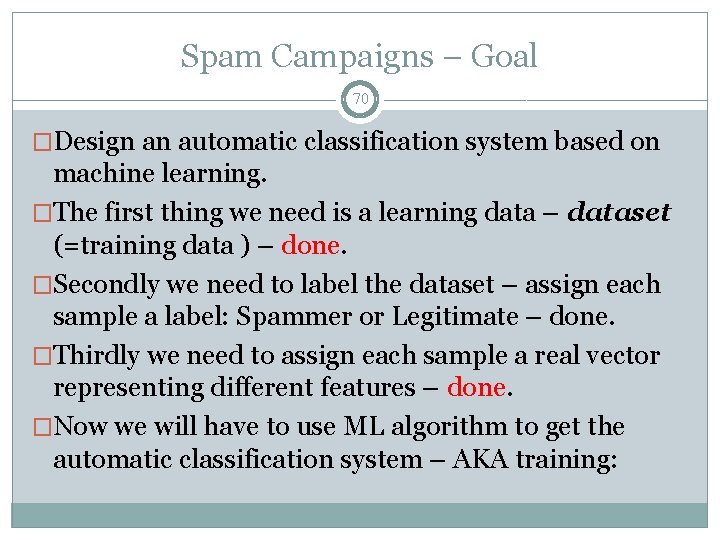
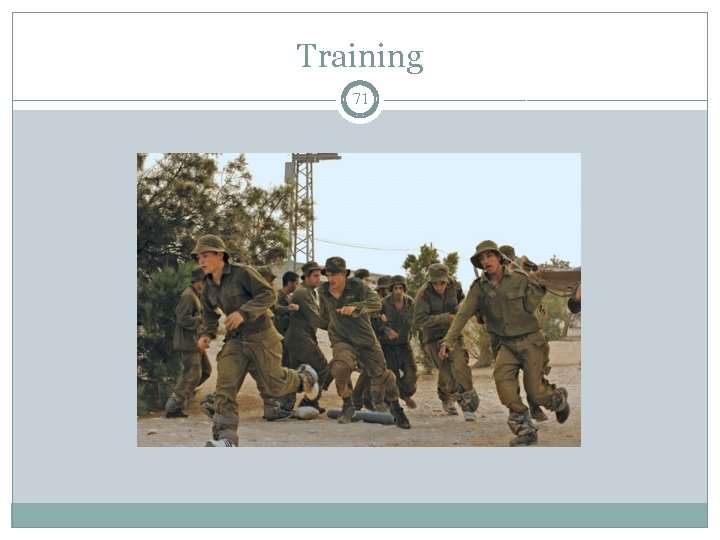
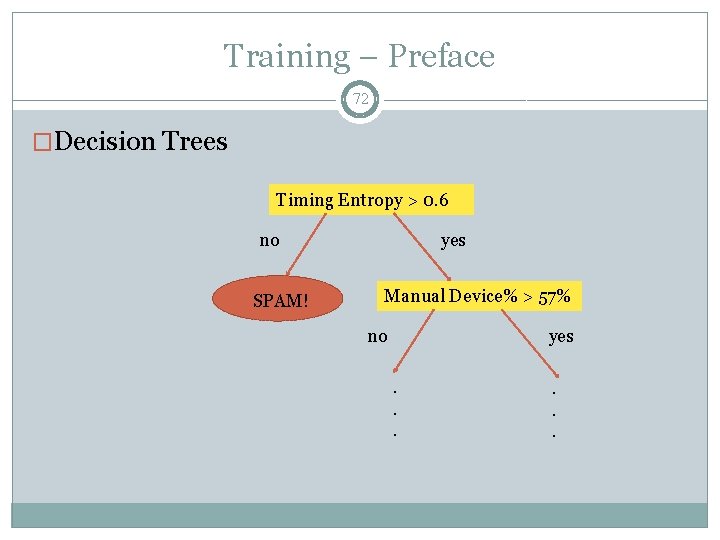
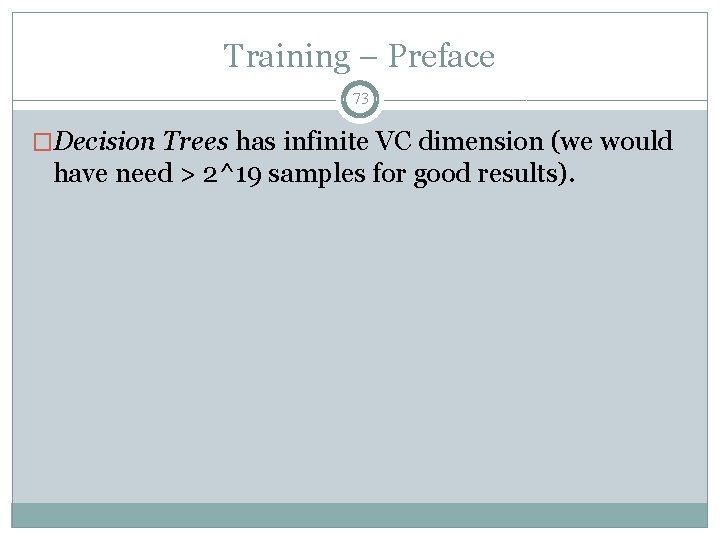
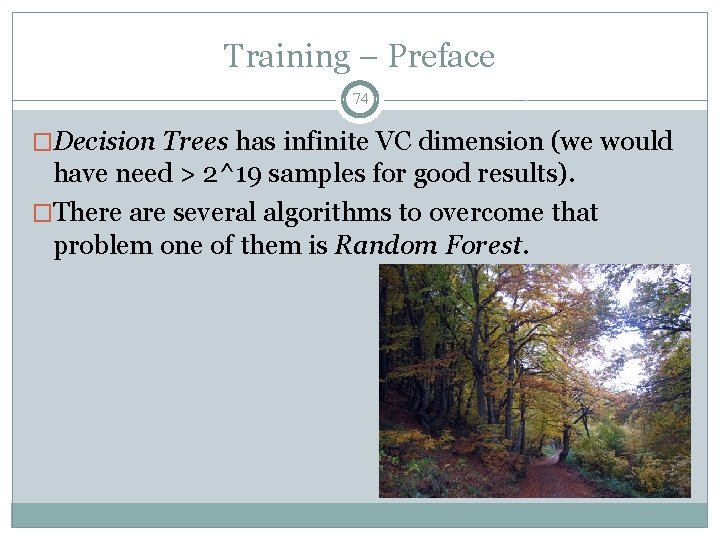
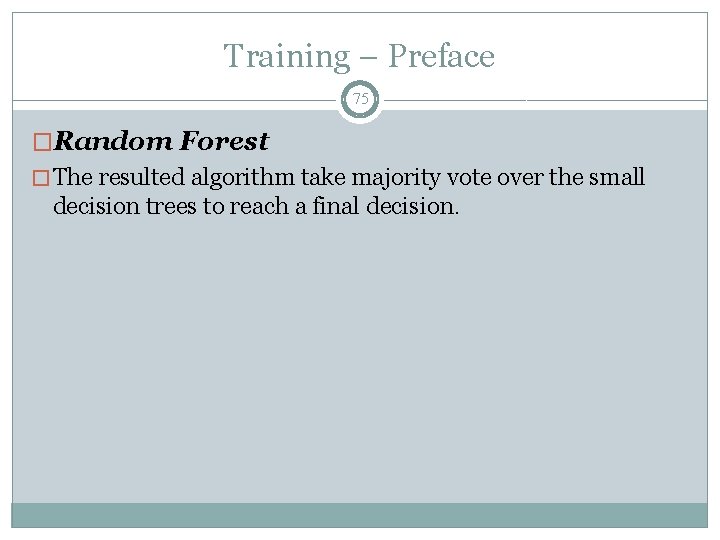
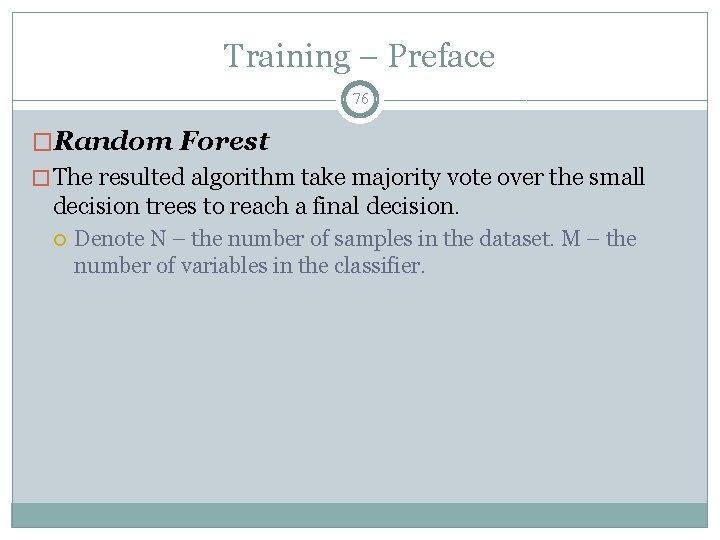
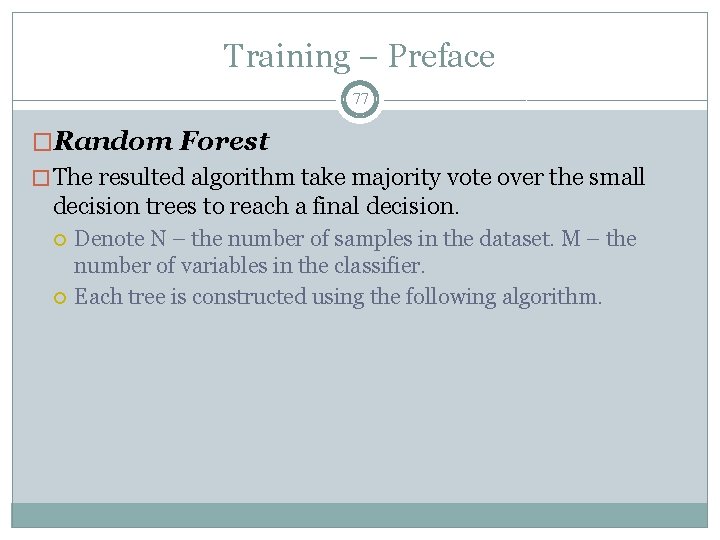
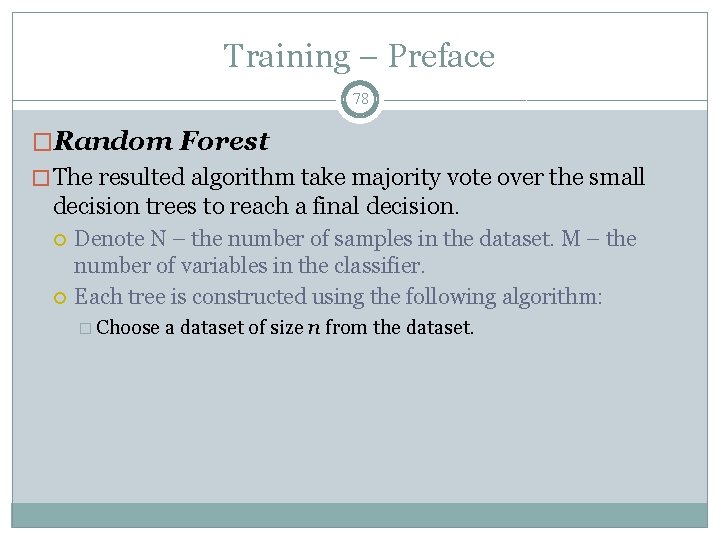
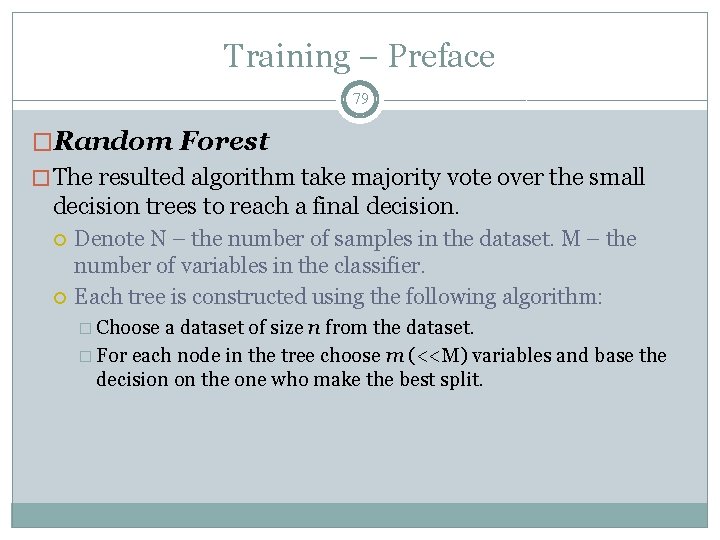
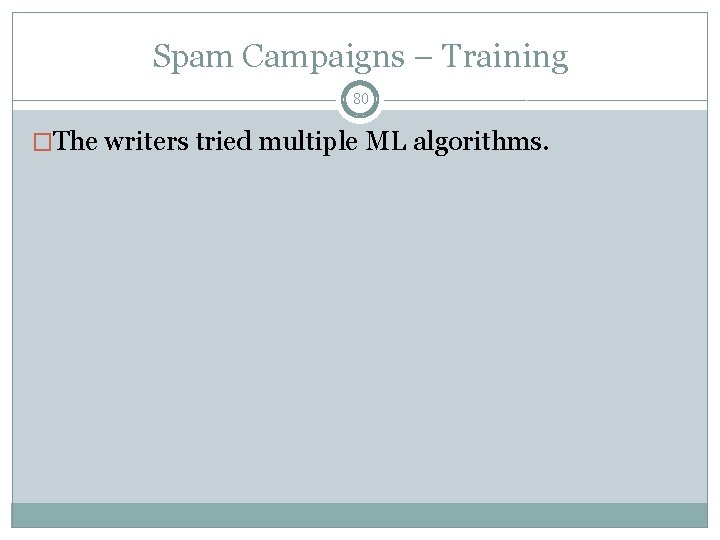
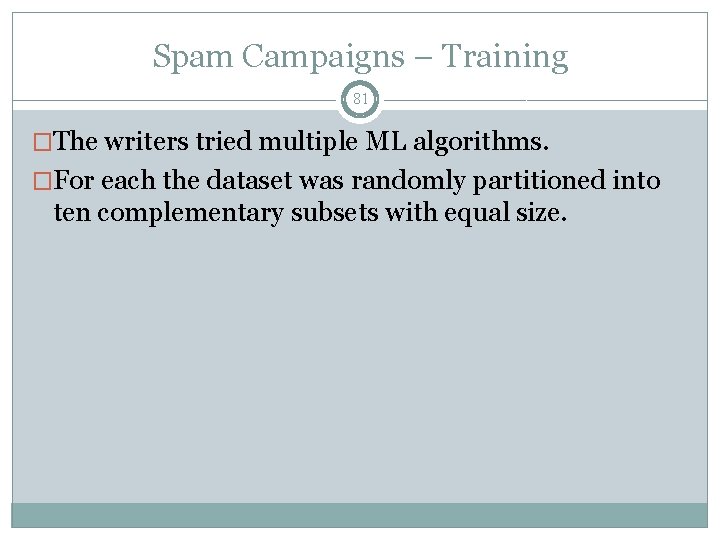
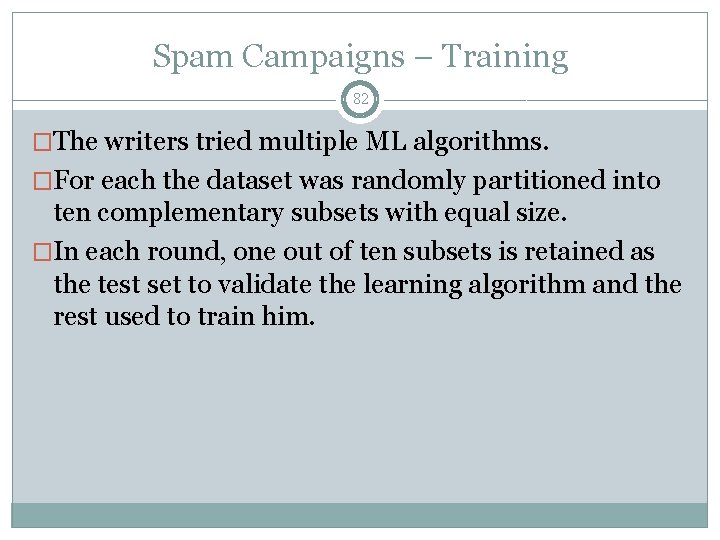
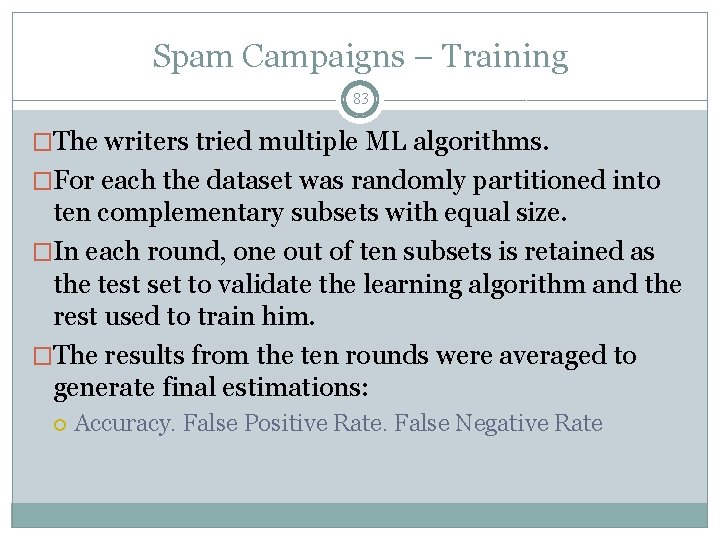
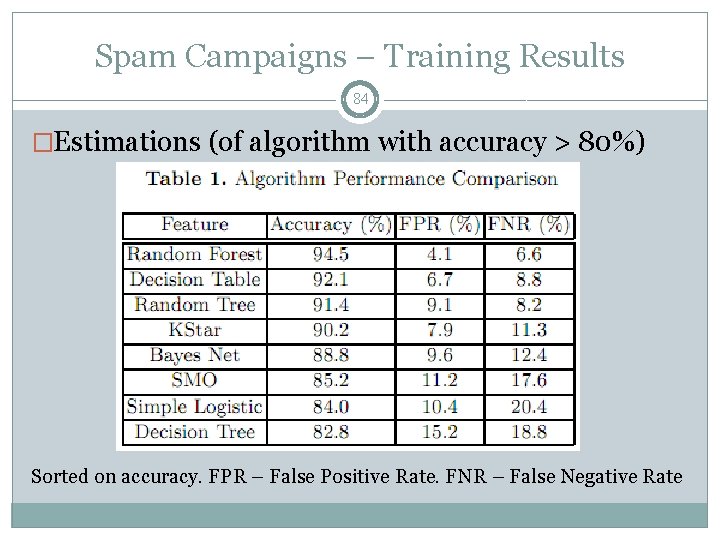
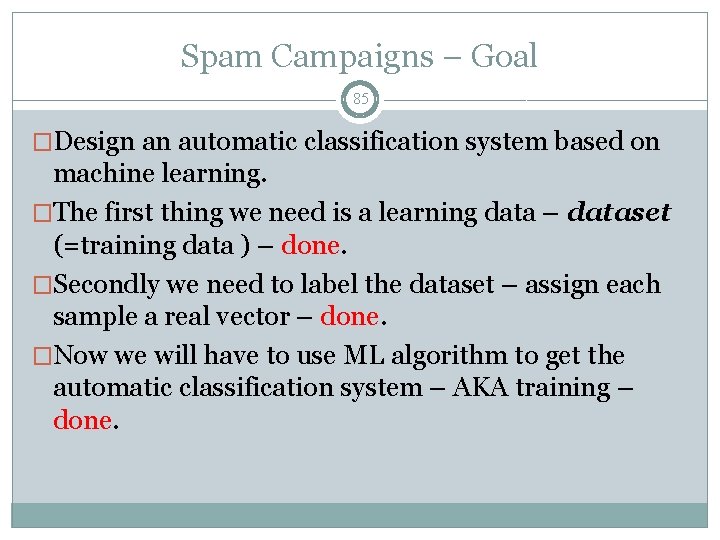
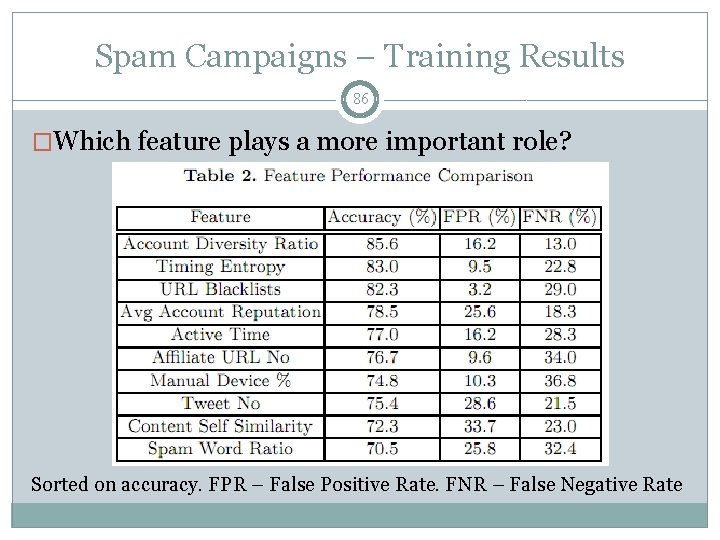
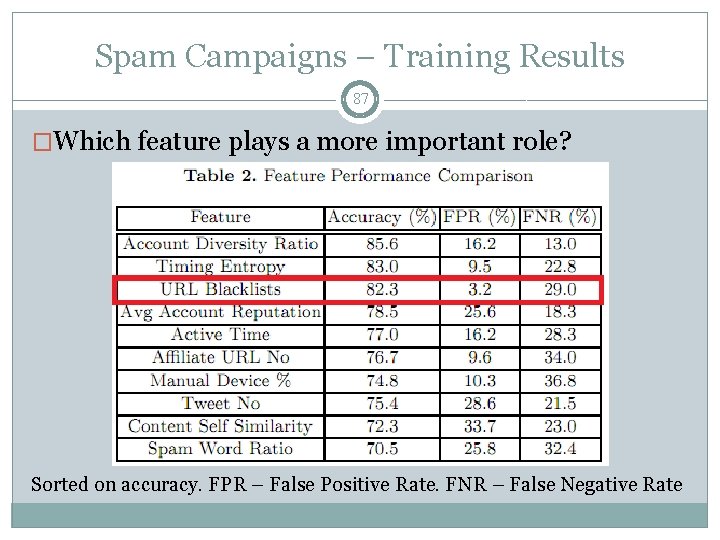
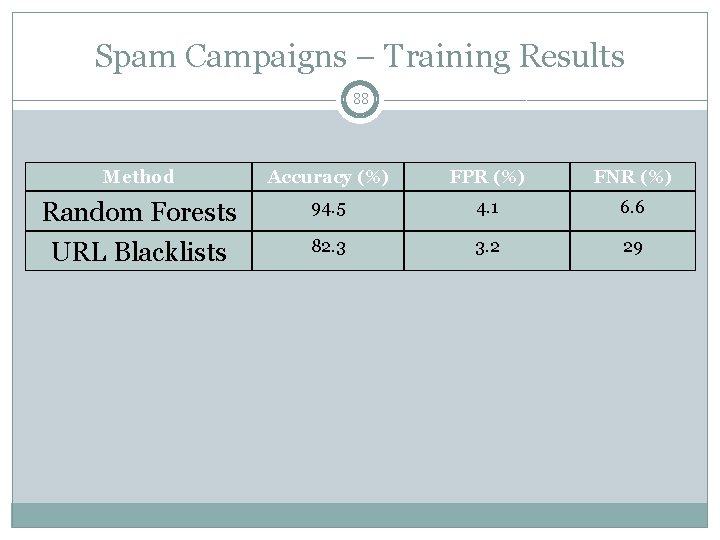
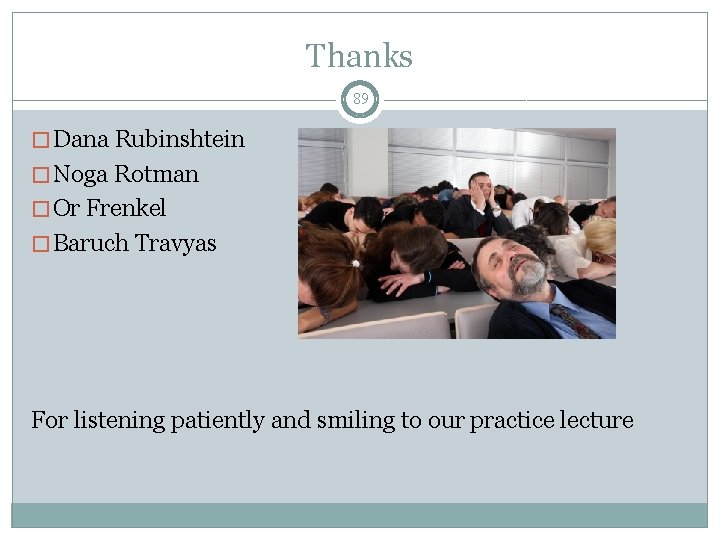
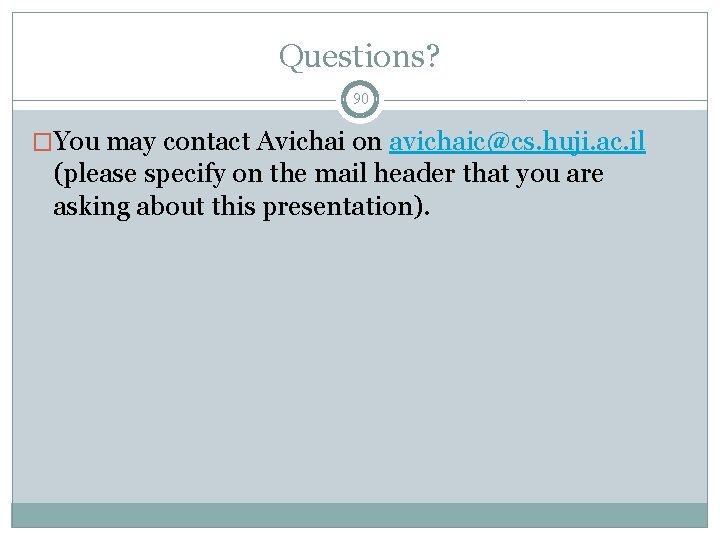
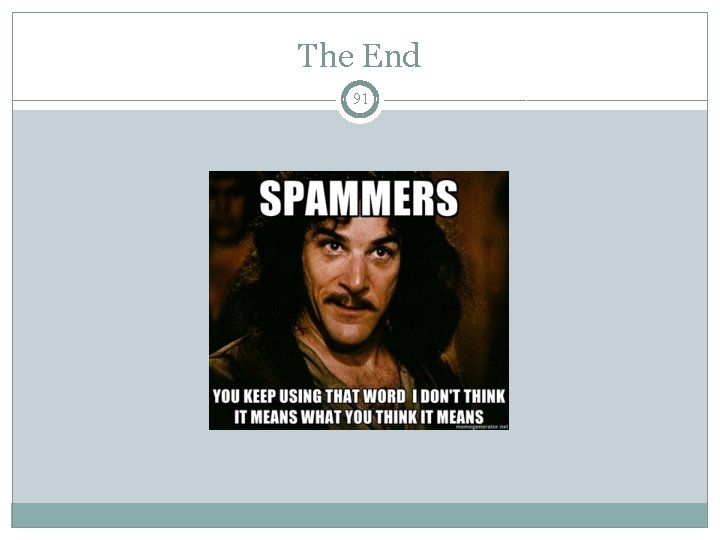
- Slides: 91
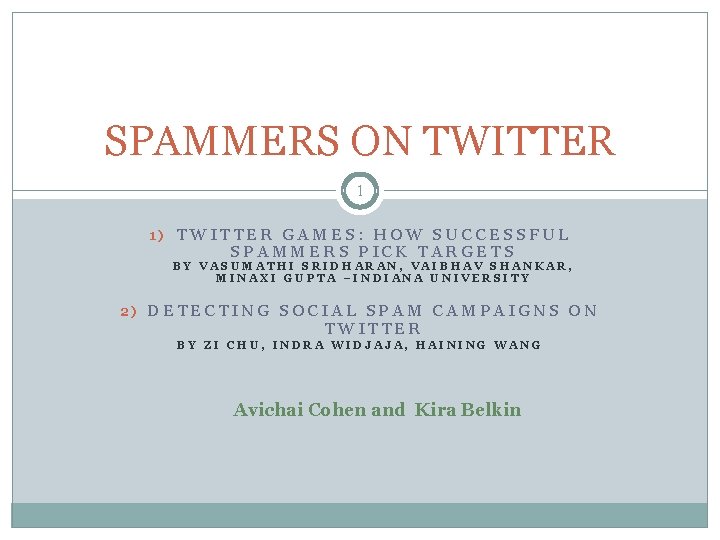
SPAMMERS ON TWITTER 1 1) TWITTER GAMES: HOW SUCCESSFUL SPAMMERS PICK TARGETS BY VASUMATHI SRIDHARAN, VAIBHAV SHANKAR, MINAXI GUPTA –INDIANA UNIVERSITY 2) DETECTING SOCIAL SPAM CAMPAIGNS ON TWITTER BY ZI CHU, INDRA WIDJAJA, HAINING WANG Avichai Cohen and Kira Belkin
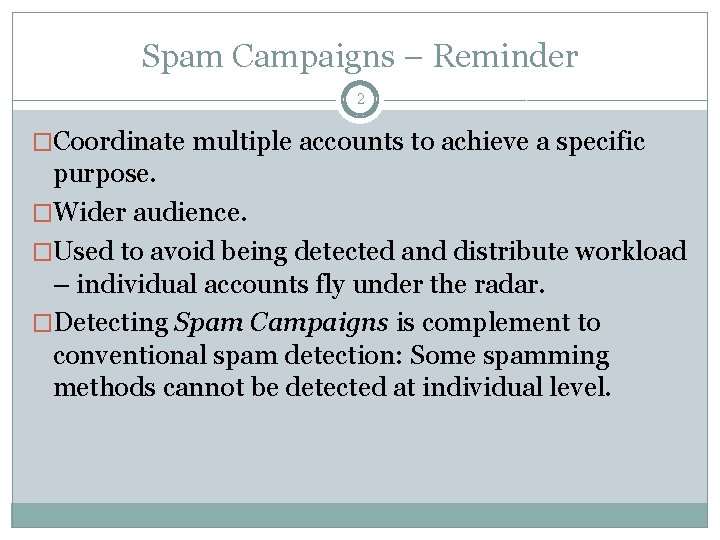
Spam Campaigns – Reminder 2 �Coordinate multiple accounts to achieve a specific purpose. �Wider audience. �Used to avoid being detected and distribute workload – individual accounts fly under the radar. �Detecting Spam Campaigns is complement to conventional spam detection: Some spamming methods cannot be detected at individual level.
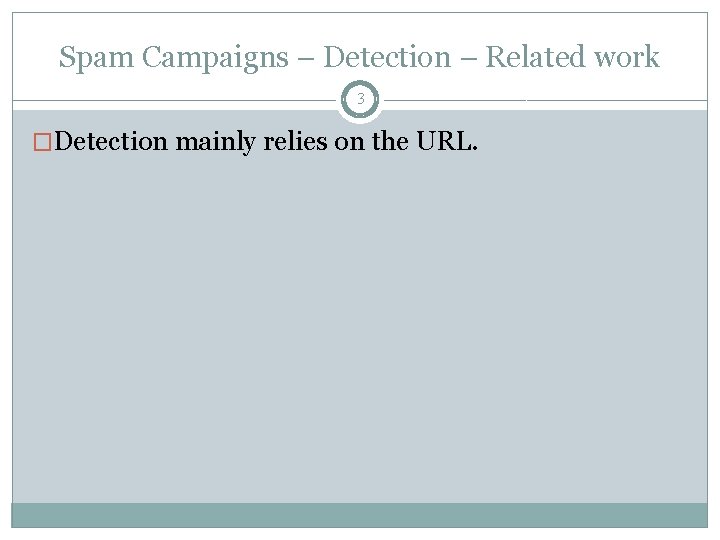
Spam Campaigns – Detection – Related work 3 �Detection mainly relies on the URL.
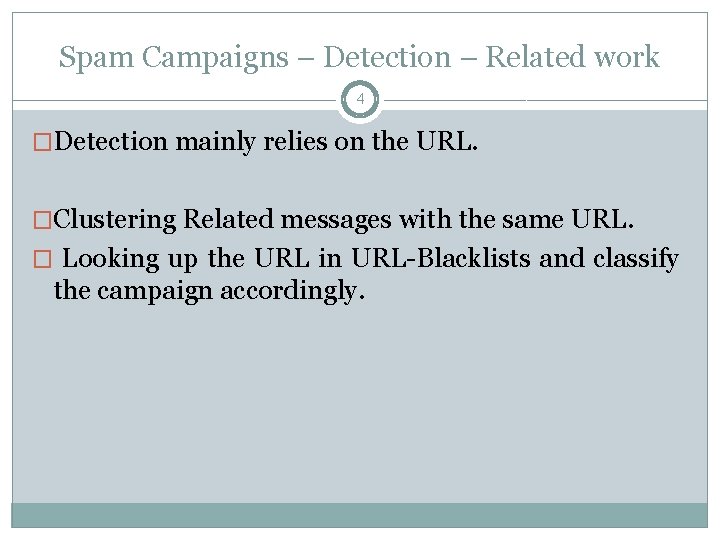
Spam Campaigns – Detection – Related work 4 �Detection mainly relies on the URL. �Clustering Related messages with the same URL. � Looking up the URL in URL-Blacklists and classify the campaign accordingly.
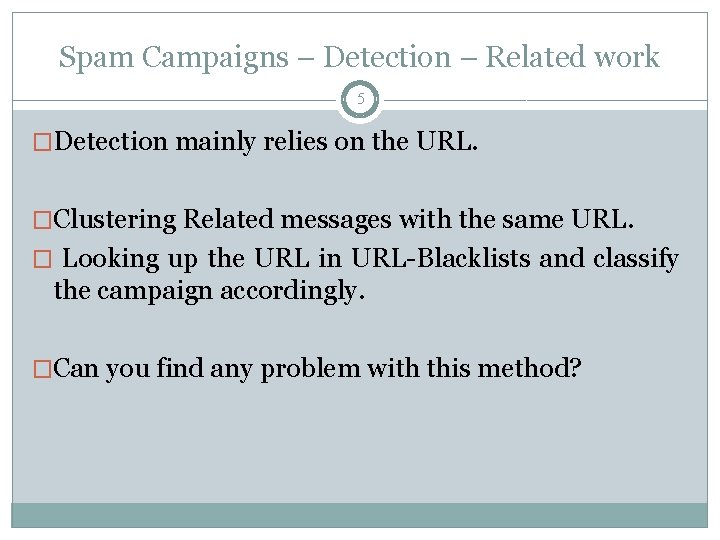
Spam Campaigns – Detection – Related work 5 �Detection mainly relies on the URL. �Clustering Related messages with the same URL. � Looking up the URL in URL-Blacklists and classify the campaign accordingly. �Can you find any problem with this method?
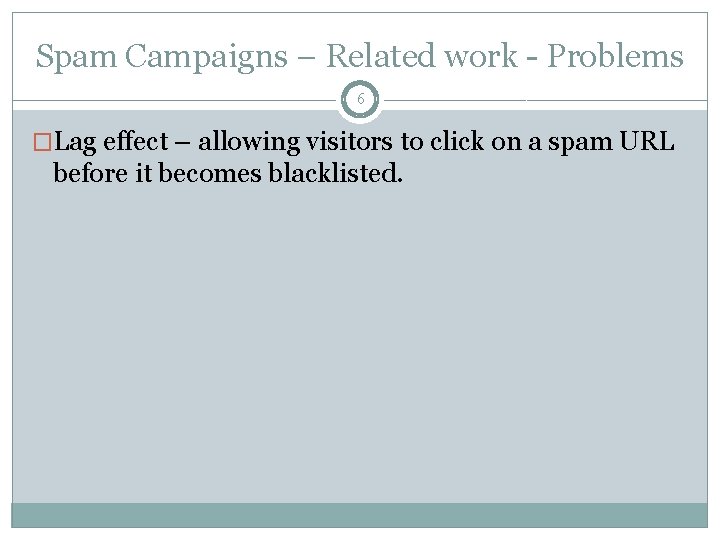
Spam Campaigns – Related work - Problems 6 �Lag effect – allowing visitors to click on a spam URL before it becomes blacklisted.
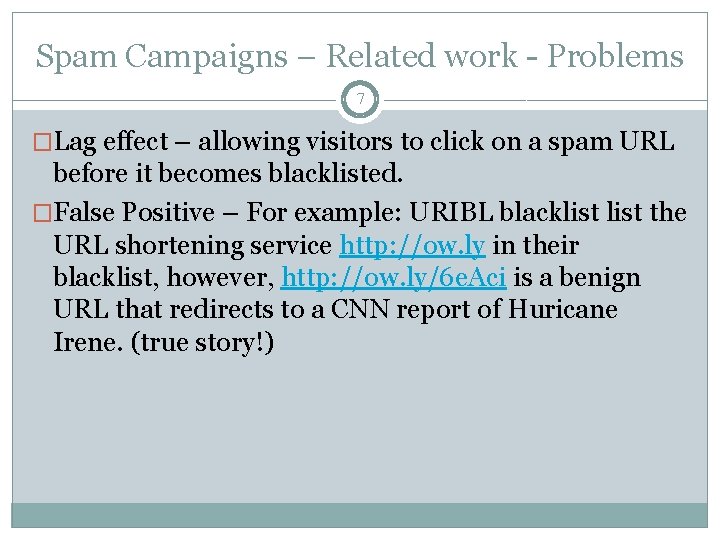
Spam Campaigns – Related work - Problems 7 �Lag effect – allowing visitors to click on a spam URL before it becomes blacklisted. �False Positive – For example: URIBL blacklist the URL shortening service http: //ow. ly in their blacklist, however, http: //ow. ly/6 e. Aci is a benign URL that redirects to a CNN report of Huricane Irene. (true story!)
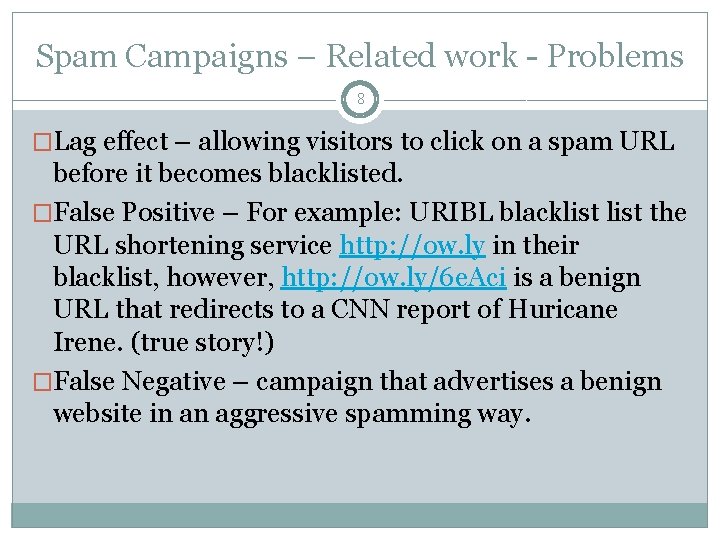
Spam Campaigns – Related work - Problems 8 �Lag effect – allowing visitors to click on a spam URL before it becomes blacklisted. �False Positive – For example: URIBL blacklist the URL shortening service http: //ow. ly in their blacklist, however, http: //ow. ly/6 e. Aci is a benign URL that redirects to a CNN report of Huricane Irene. (true story!) �False Negative – campaign that advertises a benign website in an aggressive spamming way.
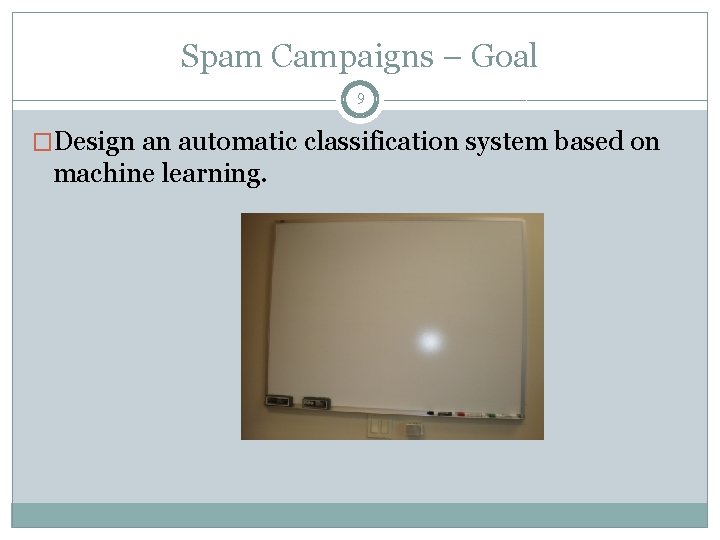
Spam Campaigns – Goal 9 �Design an automatic classification system based on machine learning.
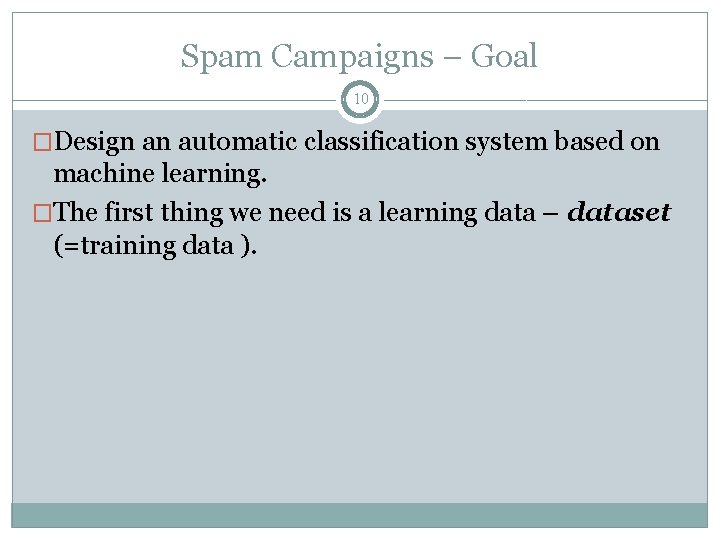
Spam Campaigns – Goal 10 �Design an automatic classification system based on machine learning. �The first thing we need is a learning data – dataset (=training data ).
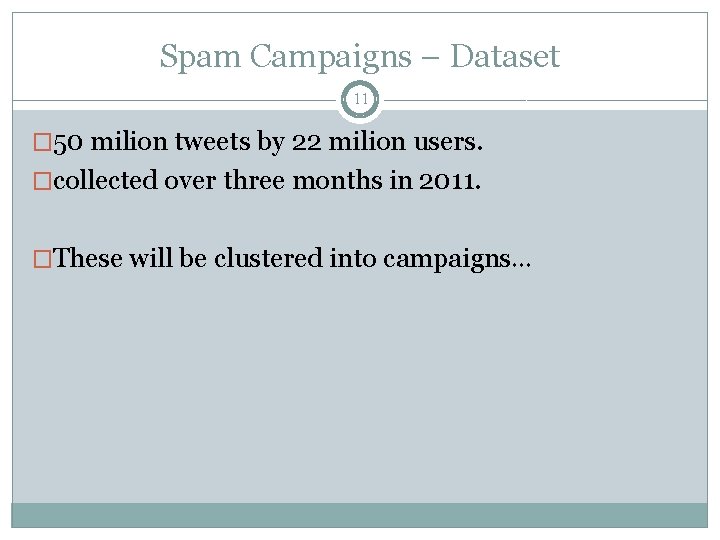
Spam Campaigns – Dataset 11 � 50 milion tweets by 22 milion users. �collected over three months in 2011. �These will be clustered into campaigns…
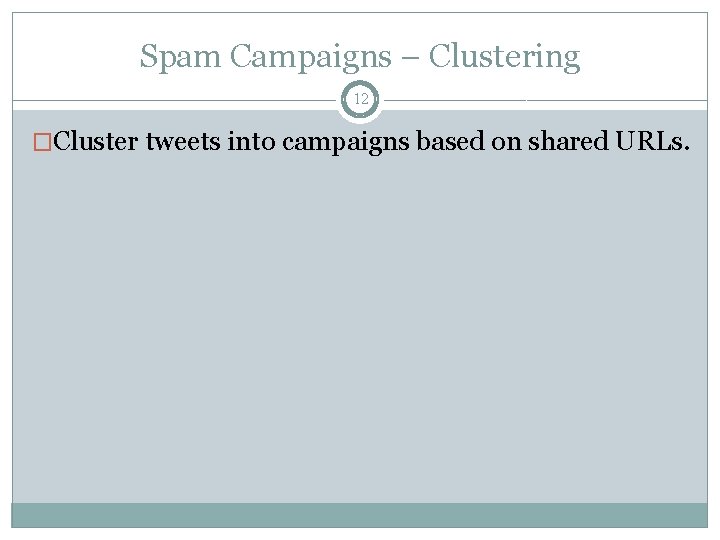
Spam Campaigns – Clustering 12 �Cluster tweets into campaigns based on shared URLs.
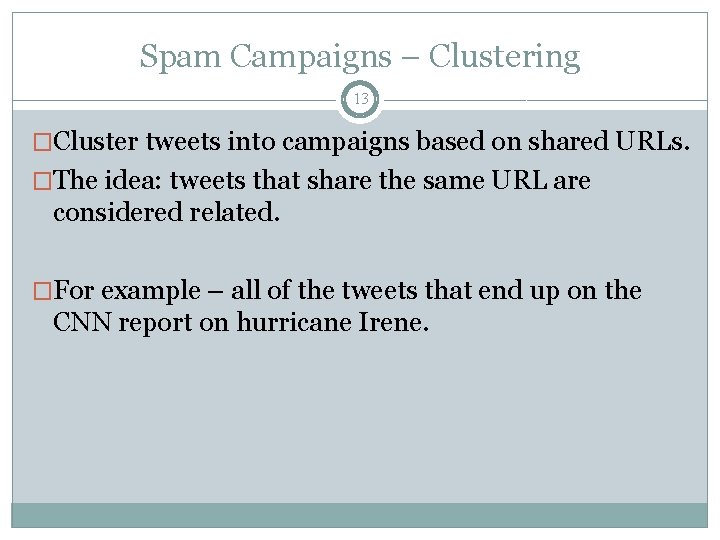
Spam Campaigns – Clustering 13 �Cluster tweets into campaigns based on shared URLs. �The idea: tweets that share the same URL are considered related. �For example – all of the tweets that end up on the CNN report on hurricane Irene.
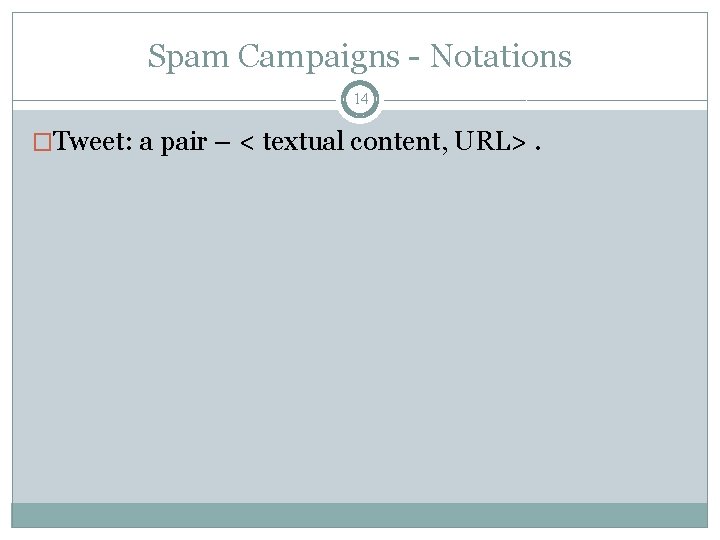
Spam Campaigns - Notations 14 �Tweet: a pair – < textual content, URL>.
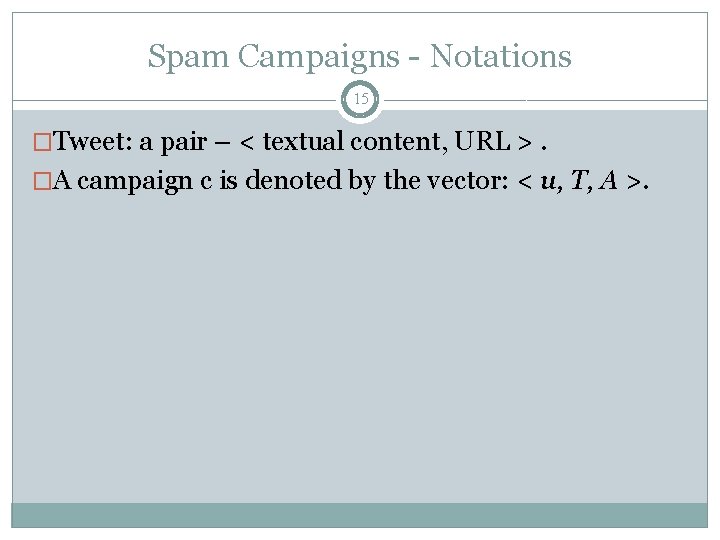
Spam Campaigns - Notations 15 �Tweet: a pair – < textual content, URL >. �A campaign c is denoted by the vector: < u, T, A >.
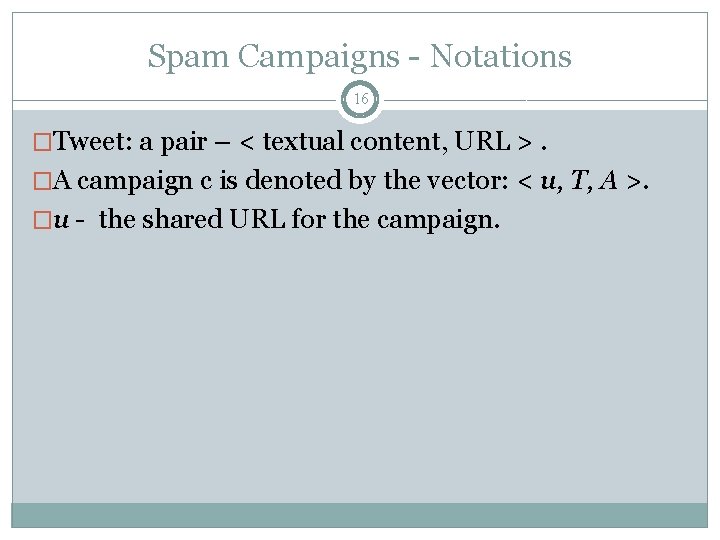
Spam Campaigns - Notations 16 �Tweet: a pair – < textual content, URL >. �A campaign c is denoted by the vector: < u, T, A >. �u - the shared URL for the campaign.
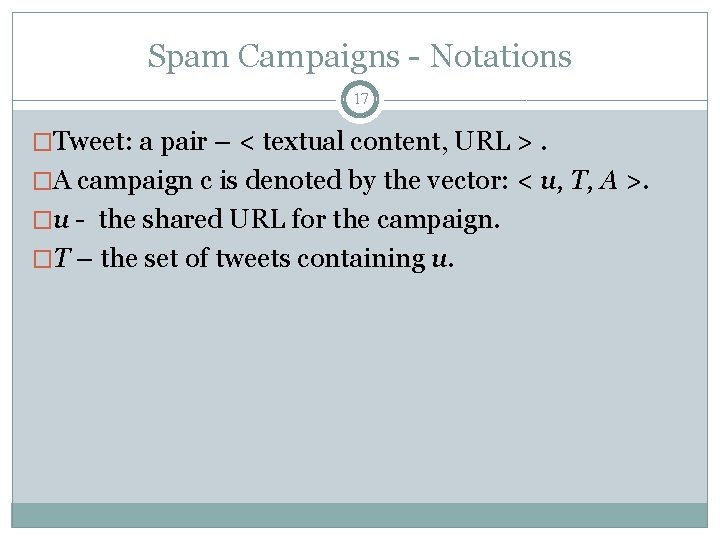
Spam Campaigns - Notations 17 �Tweet: a pair – < textual content, URL >. �A campaign c is denoted by the vector: < u, T, A >. �u - the shared URL for the campaign. �T – the set of tweets containing u.
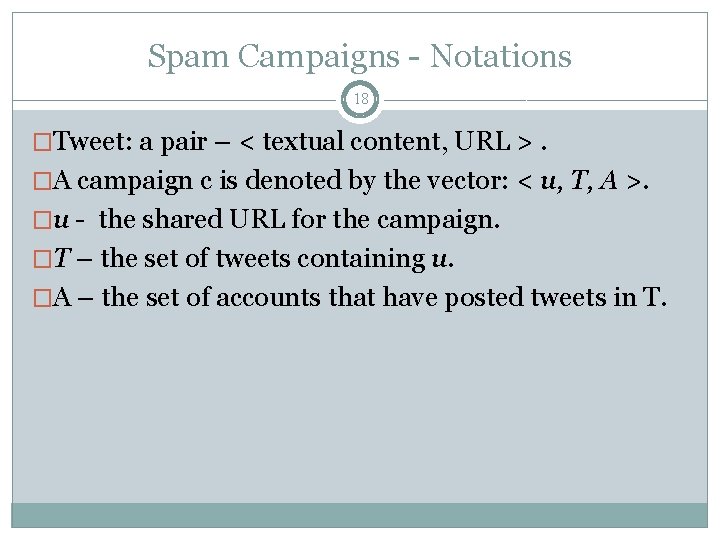
Spam Campaigns - Notations 18 �Tweet: a pair – < textual content, URL >. �A campaign c is denoted by the vector: < u, T, A >. �u - the shared URL for the campaign. �T – the set of tweets containing u. �A – the set of accounts that have posted tweets in T.
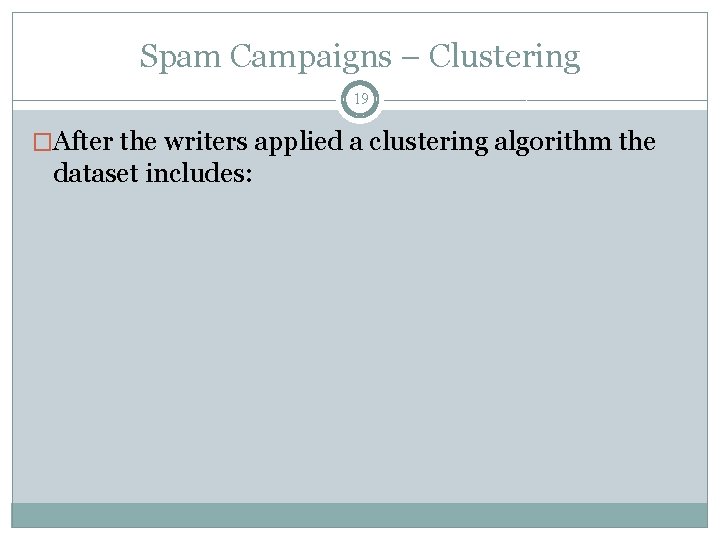
Spam Campaigns – Clustering 19 �After the writers applied a clustering algorithm the dataset includes:
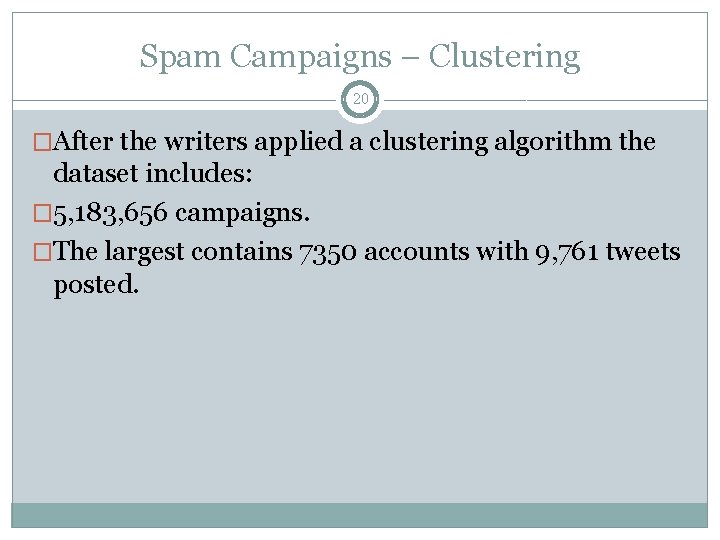
Spam Campaigns – Clustering 20 �After the writers applied a clustering algorithm the dataset includes: � 5, 183, 656 campaigns. �The largest contains 7350 accounts with 9, 761 tweets posted.
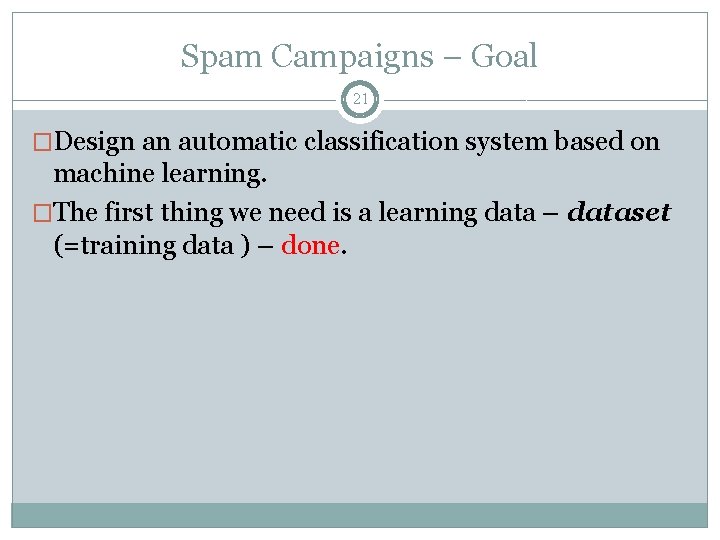
Spam Campaigns – Goal 21 �Design an automatic classification system based on machine learning. �The first thing we need is a learning data – dataset (=training data ) – done.
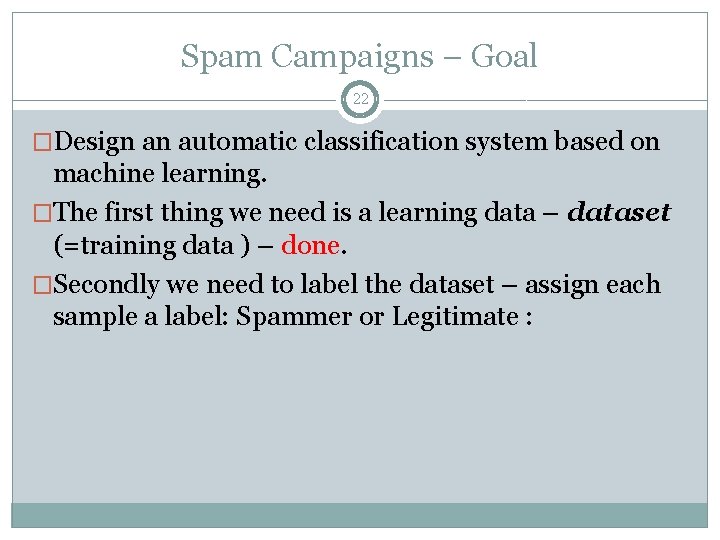
Spam Campaigns – Goal 22 �Design an automatic classification system based on machine learning. �The first thing we need is a learning data – dataset (=training data ) – done. �Secondly we need to label the dataset – assign each sample a label: Spammer or Legitimate :
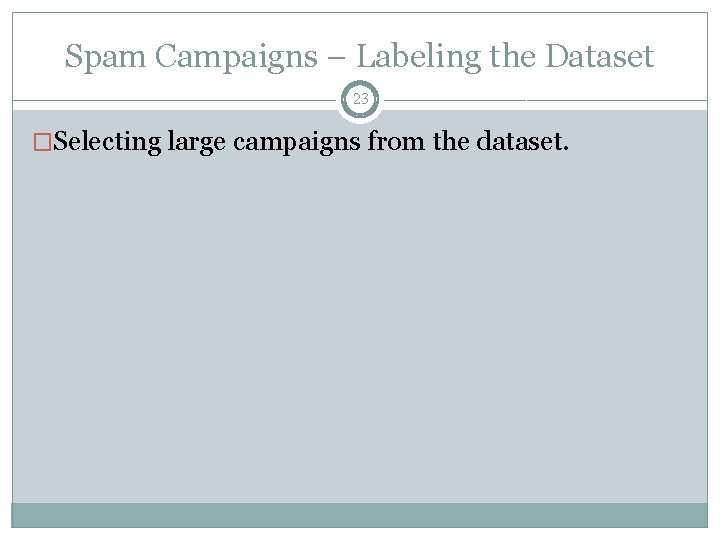
Spam Campaigns – Labeling the Dataset 23 �Selecting large campaigns from the dataset.
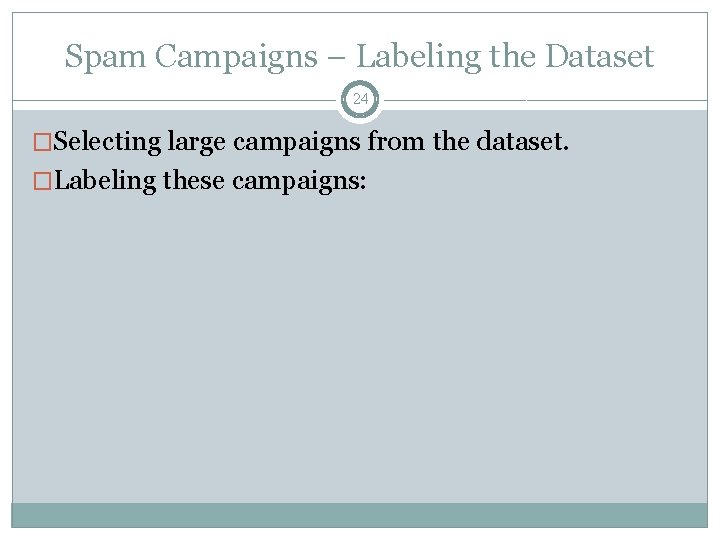
Spam Campaigns – Labeling the Dataset 24 �Selecting large campaigns from the dataset. �Labeling these campaigns:
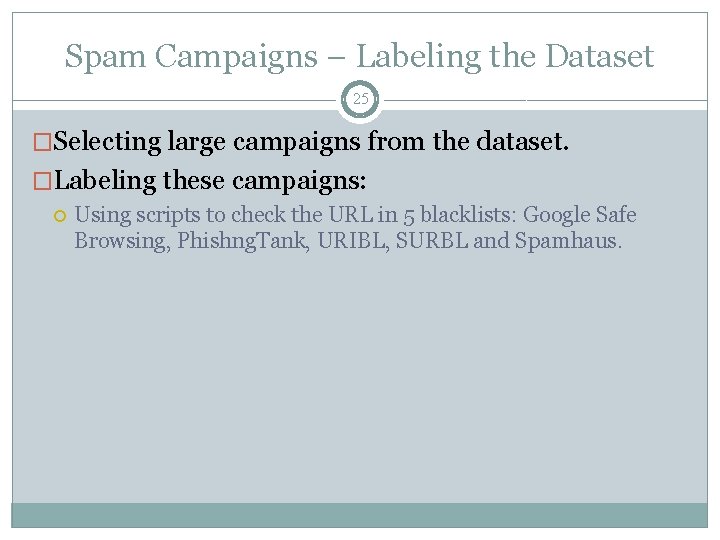
Spam Campaigns – Labeling the Dataset 25 �Selecting large campaigns from the dataset. �Labeling these campaigns: Using scripts to check the URL in 5 blacklists: Google Safe Browsing, Phishng. Tank, URIBL, SURBL and Spamhaus.
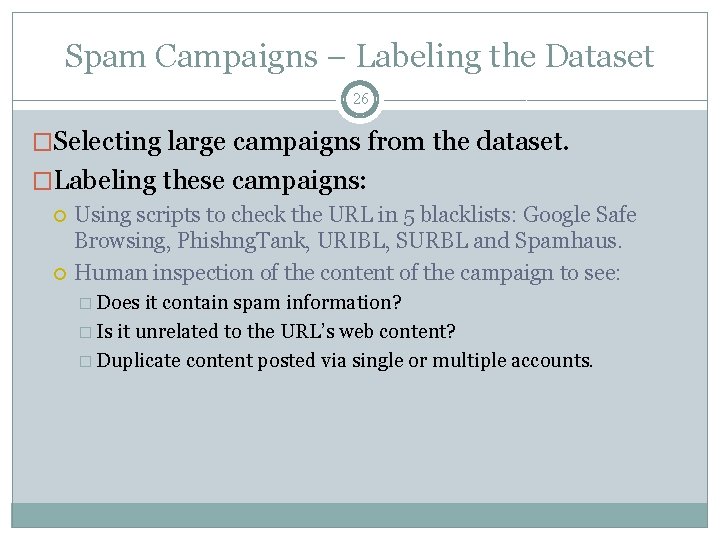
Spam Campaigns – Labeling the Dataset 26 �Selecting large campaigns from the dataset. �Labeling these campaigns: Using scripts to check the URL in 5 blacklists: Google Safe Browsing, Phishng. Tank, URIBL, SURBL and Spamhaus. Human inspection of the content of the campaign to see: � Does it contain spam information? � Is it unrelated to the URL’s web content? � Duplicate content posted via single or multiple accounts.
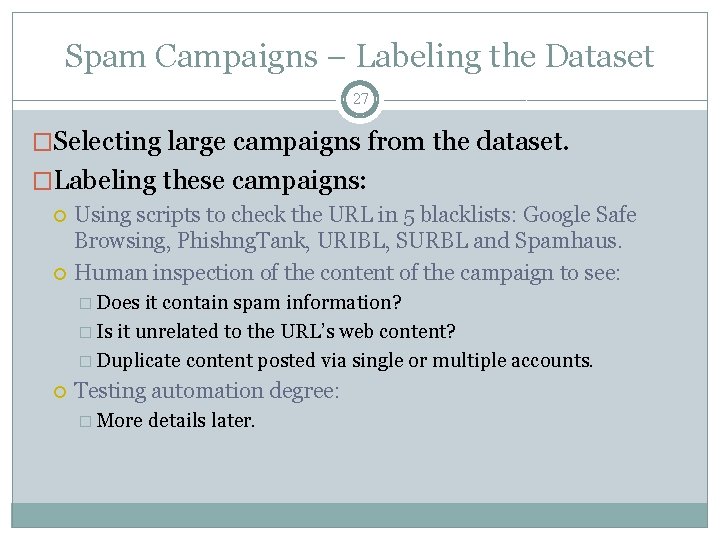
Spam Campaigns – Labeling the Dataset 27 �Selecting large campaigns from the dataset. �Labeling these campaigns: Using scripts to check the URL in 5 blacklists: Google Safe Browsing, Phishng. Tank, URIBL, SURBL and Spamhaus. Human inspection of the content of the campaign to see: � Does it contain spam information? � Is it unrelated to the URL’s web content? � Duplicate content posted via single or multiple accounts. Testing automation degree: � More details later.
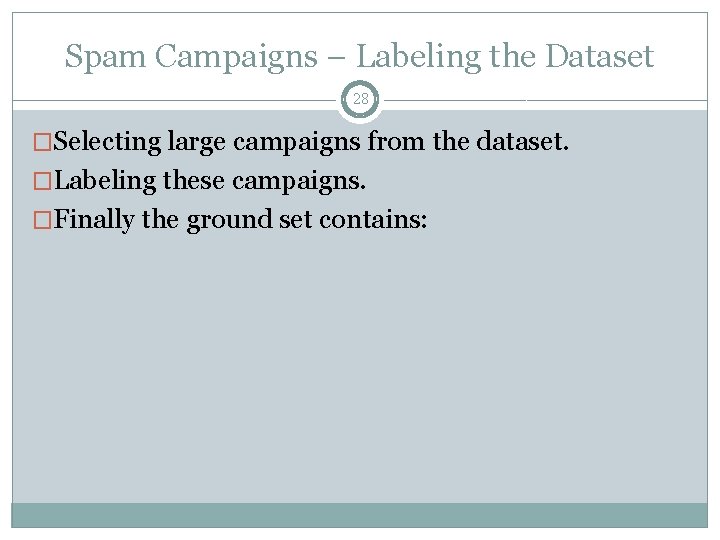
Spam Campaigns – Labeling the Dataset 28 �Selecting large campaigns from the dataset. �Labeling these campaigns. �Finally the ground set contains:
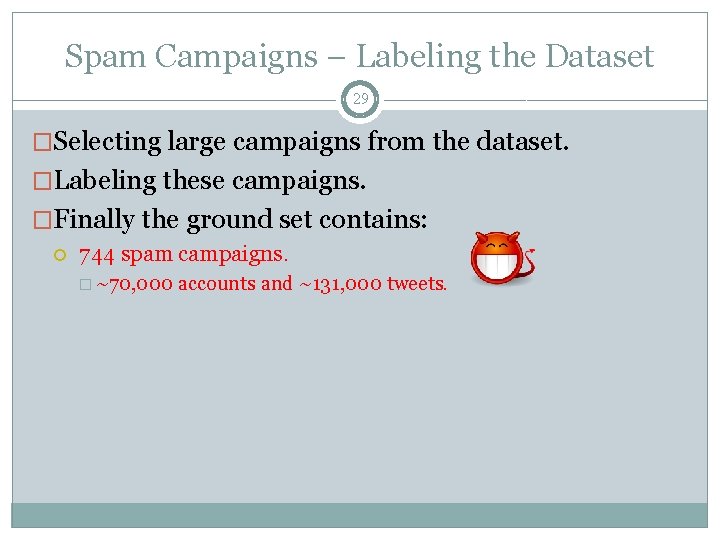
Spam Campaigns – Labeling the Dataset 29 �Selecting large campaigns from the dataset. �Labeling these campaigns. �Finally the ground set contains: 744 spam campaigns. � ~70, 000 accounts and ~131, 000 tweets.
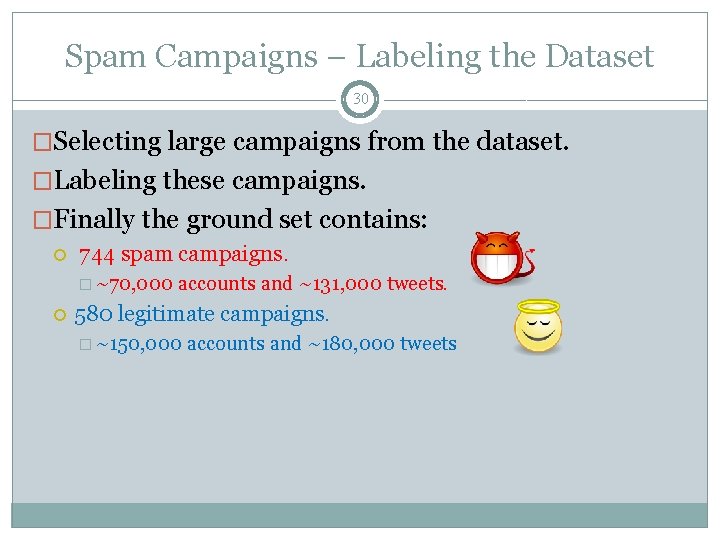
Spam Campaigns – Labeling the Dataset 30 �Selecting large campaigns from the dataset. �Labeling these campaigns. �Finally the ground set contains: 744 spam campaigns. � ~70, 000 accounts and ~131, 000 tweets. 580 legitimate campaigns. � ~150, 000 accounts and ~180, 000 tweets
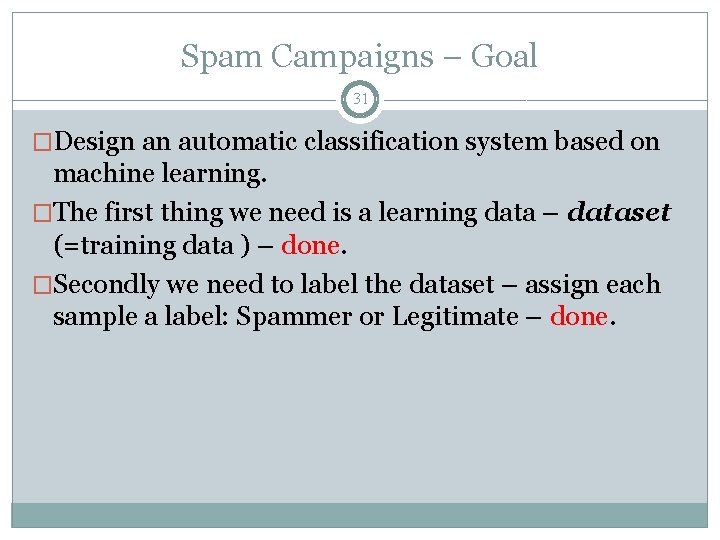
Spam Campaigns – Goal 31 �Design an automatic classification system based on machine learning. �The first thing we need is a learning data – dataset (=training data ) – done. �Secondly we need to label the dataset – assign each sample a label: Spammer or Legitimate – done.
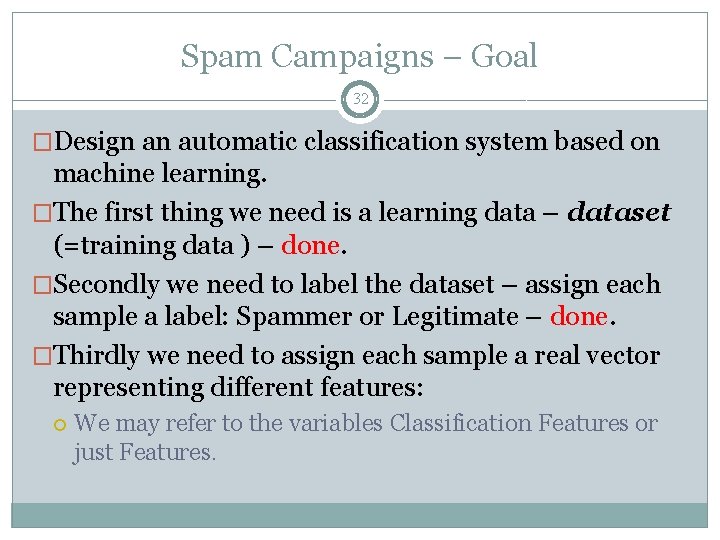
Spam Campaigns – Goal 32 �Design an automatic classification system based on machine learning. �The first thing we need is a learning data – dataset (=training data ) – done. �Secondly we need to label the dataset – assign each sample a label: Spammer or Legitimate – done. �Thirdly we need to assign each sample a real vector representing different features: We may refer to the variables Classification Features or just Features.
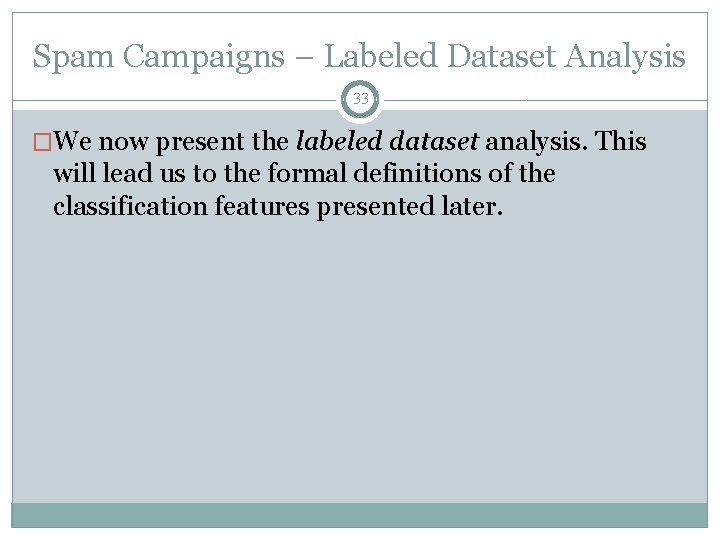
Spam Campaigns – Labeled Dataset Analysis 33 �We now present the labeled dataset analysis. This will lead us to the formal definitions of the classification features presented later.
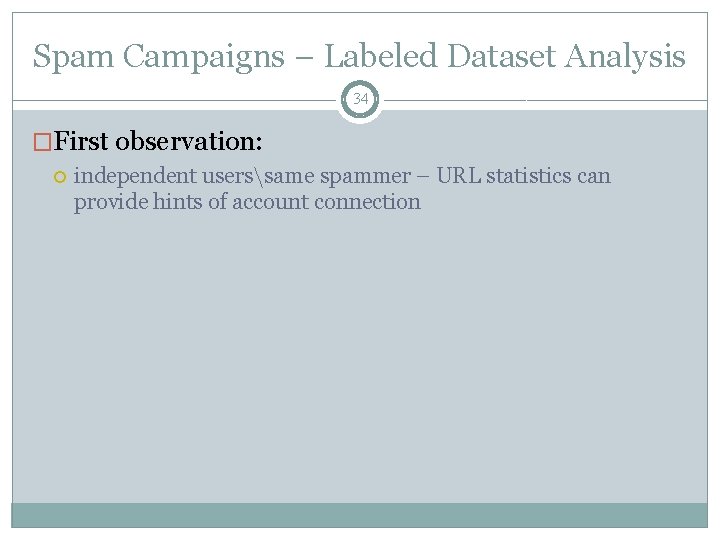
Spam Campaigns – Labeled Dataset Analysis 34 �First observation: independent userssame spammer – URL statistics can provide hints of account connection
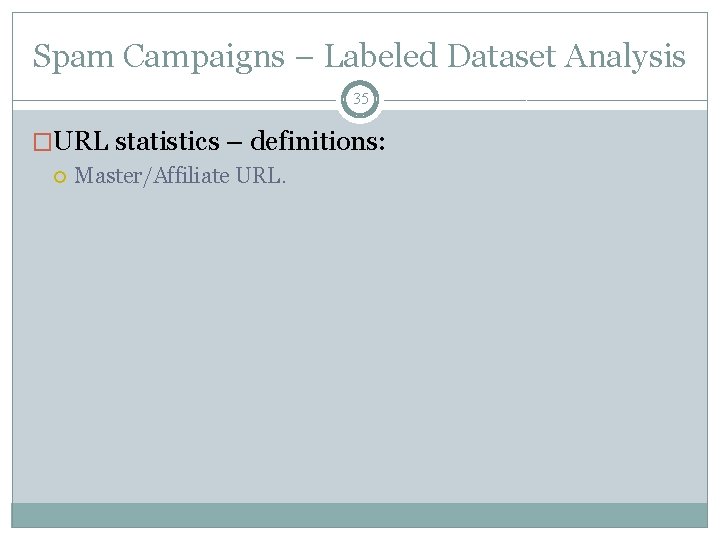
Spam Campaigns – Labeled Dataset Analysis 35 �URL statistics – definitions: Master/Affiliate URL.
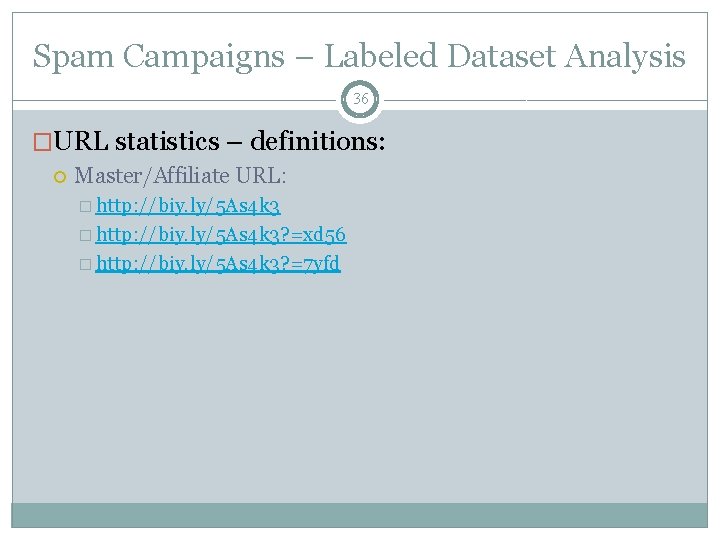
Spam Campaigns – Labeled Dataset Analysis 36 �URL statistics – definitions: Master/Affiliate URL: � http: //biy. ly/5 As 4 k 3? =xd 56 � http: //biy. ly/5 As 4 k 3? =7 yfd
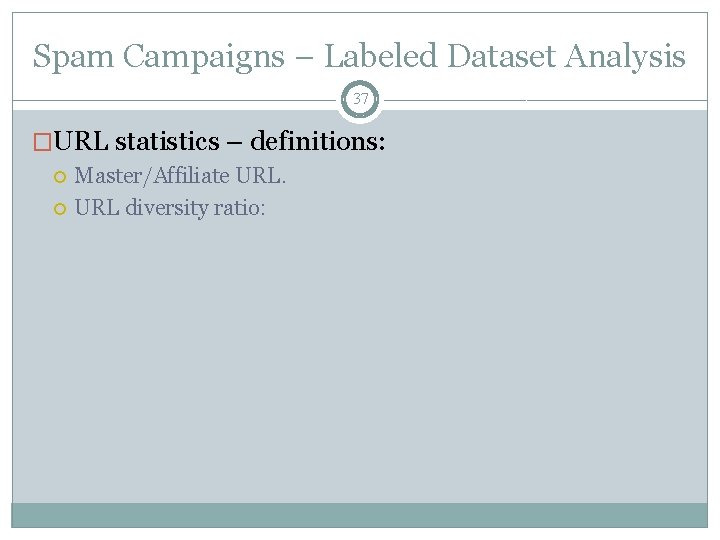
Spam Campaigns – Labeled Dataset Analysis 37 �URL statistics – definitions: Master/Affiliate URL diversity ratio:
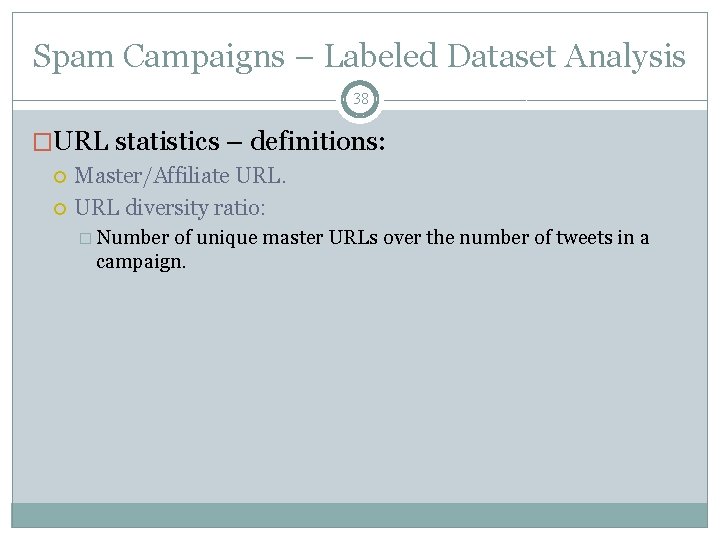
Spam Campaigns – Labeled Dataset Analysis 38 �URL statistics – definitions: Master/Affiliate URL diversity ratio: � Number of unique master URLs over the number of tweets in a campaign.
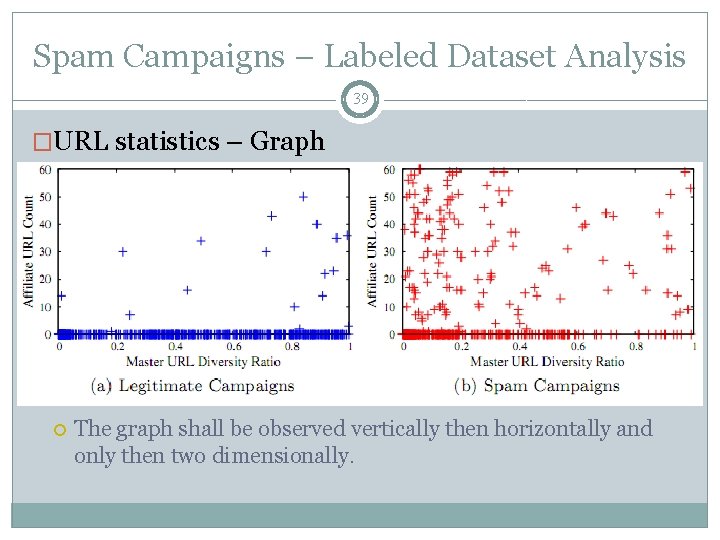
Spam Campaigns – Labeled Dataset Analysis 39 �URL statistics – Graph The graph shall be observed vertically then horizontally and only then two dimensionally.
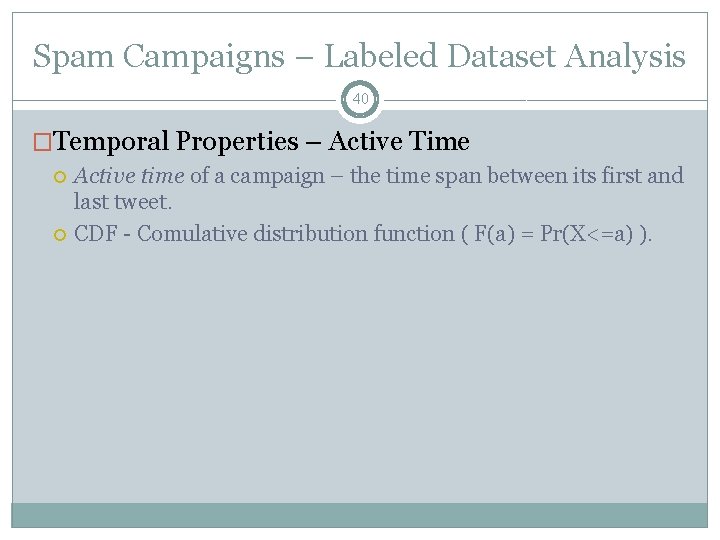
Spam Campaigns – Labeled Dataset Analysis 40 �Temporal Properties – Active Time Active time of a campaign – the time span between its first and last tweet. CDF - Comulative distribution function ( F(a) = Pr(X<=a) ).
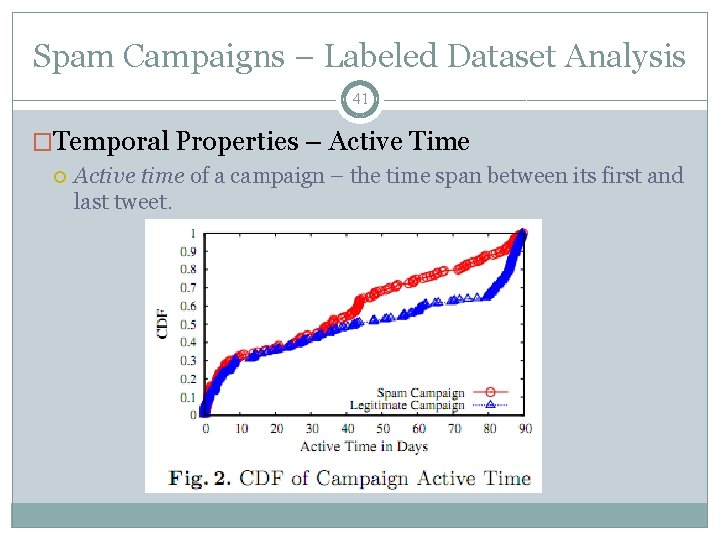
Spam Campaigns – Labeled Dataset Analysis 41 �Temporal Properties – Active Time Active time of a campaign – the time span between its first and last tweet.
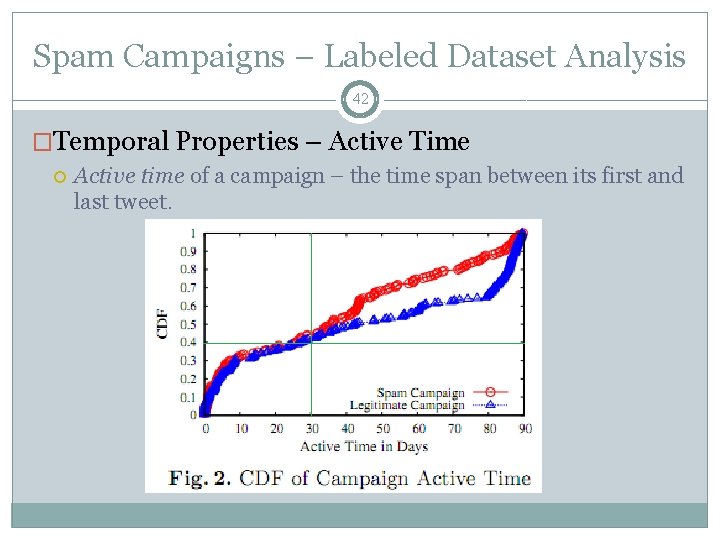
Spam Campaigns – Labeled Dataset Analysis 42 �Temporal Properties – Active Time Active time of a campaign – the time span between its first and last tweet.
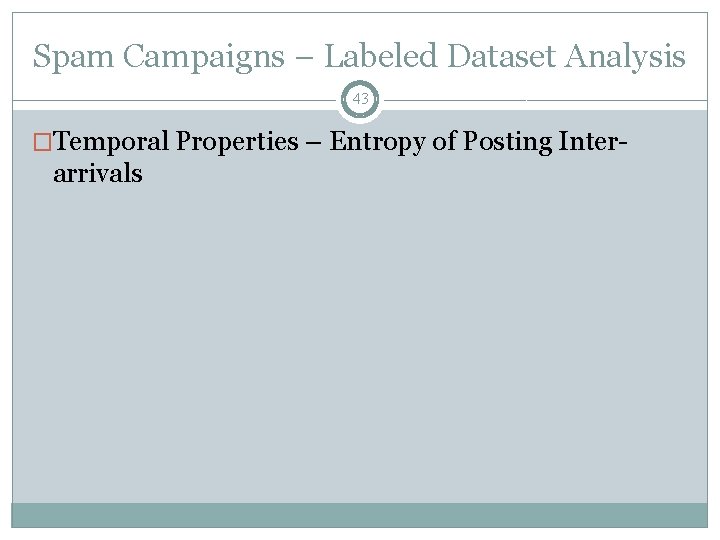
Spam Campaigns – Labeled Dataset Analysis 43 �Temporal Properties – Entropy of Posting Inter- arrivals
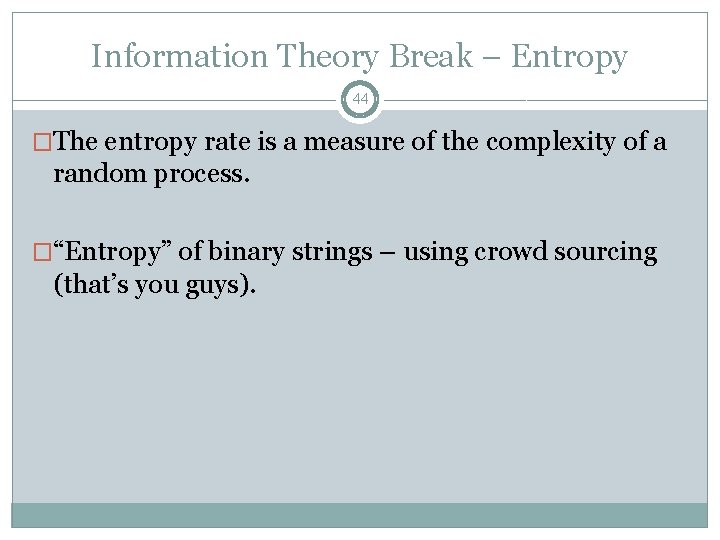
Information Theory Break – Entropy 44 �The entropy rate is a measure of the complexity of a random process. �“Entropy” of binary strings – using crowd sourcing (that’s you guys).
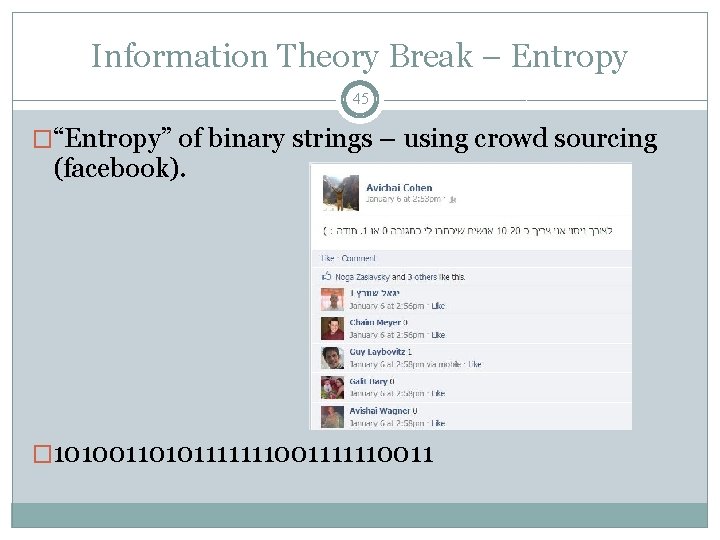
Information Theory Break – Entropy 45 �“Entropy” of binary strings – using crowd sourcing (facebook). � 101001101011111110011
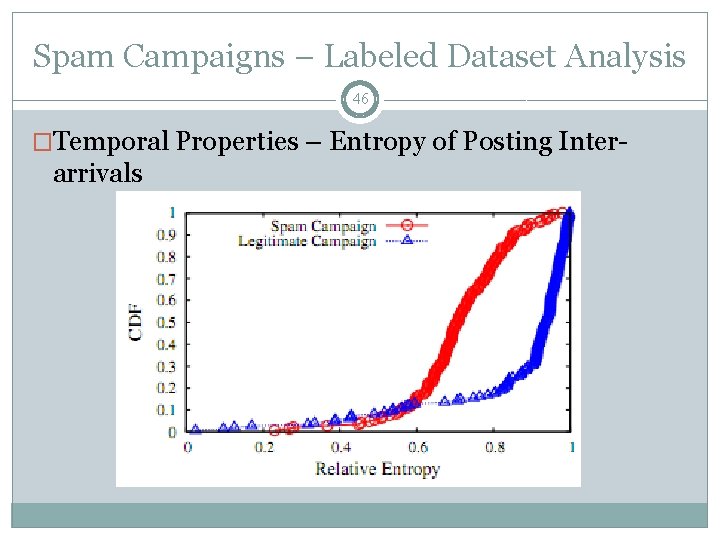
Spam Campaigns – Labeled Dataset Analysis 46 �Temporal Properties – Entropy of Posting Inter- arrivals
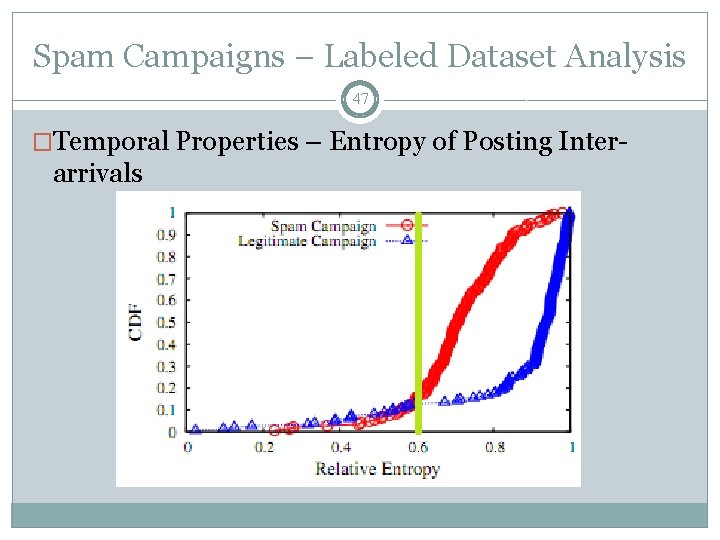
Spam Campaigns – Labeled Dataset Analysis 47 �Temporal Properties – Entropy of Posting Inter- arrivals
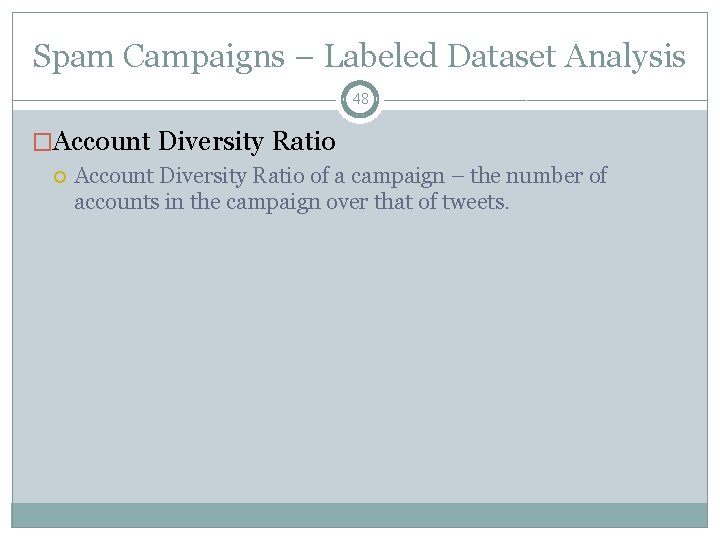
Spam Campaigns – Labeled Dataset Analysis 48 �Account Diversity Ratio of a campaign – the number of accounts in the campaign over that of tweets.
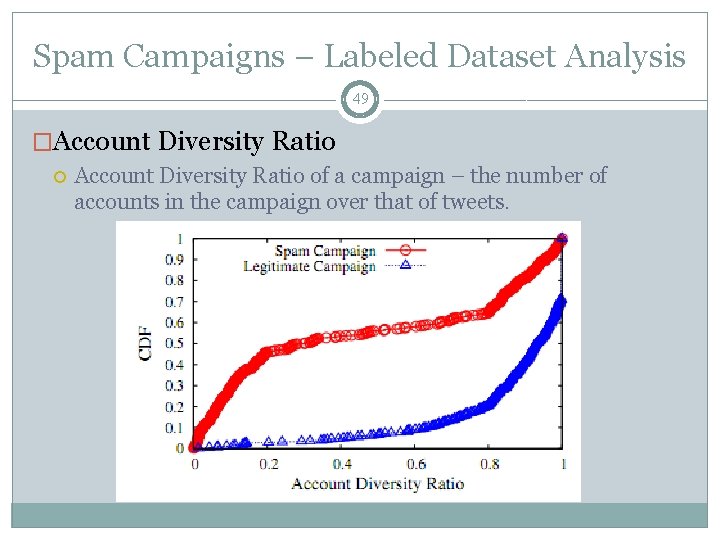
Spam Campaigns – Labeled Dataset Analysis 49 �Account Diversity Ratio of a campaign – the number of accounts in the campaign over that of tweets.
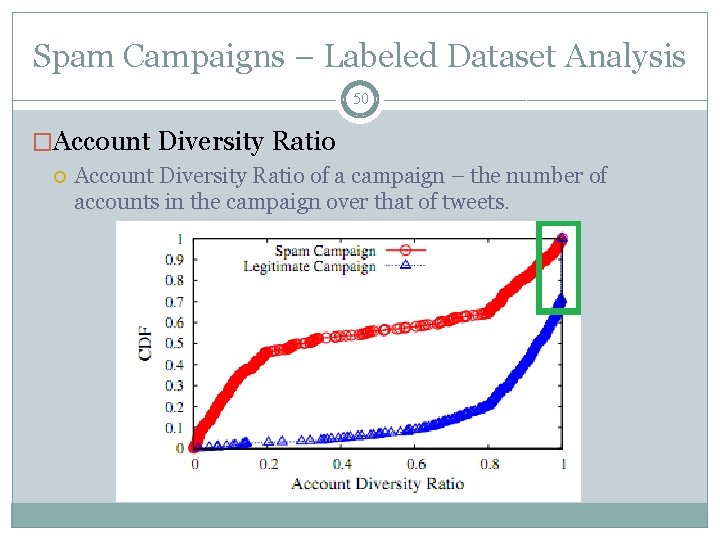
Spam Campaigns – Labeled Dataset Analysis 50 �Account Diversity Ratio of a campaign – the number of accounts in the campaign over that of tweets.
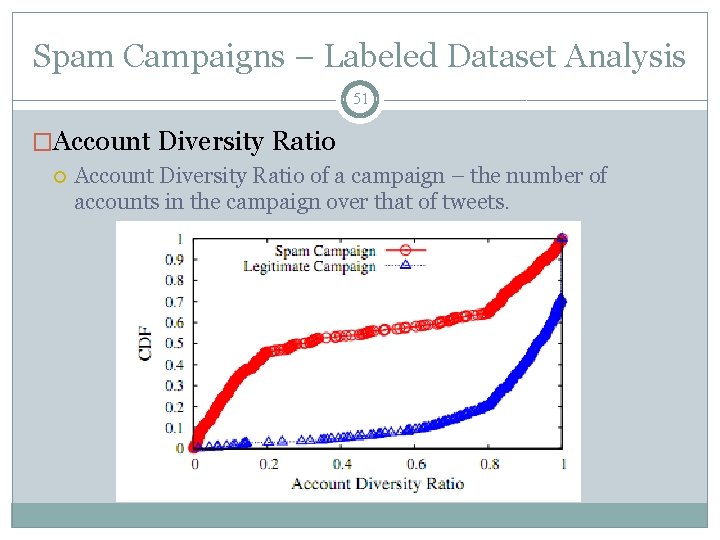
Spam Campaigns – Labeled Dataset Analysis 51 �Account Diversity Ratio of a campaign – the number of accounts in the campaign over that of tweets.
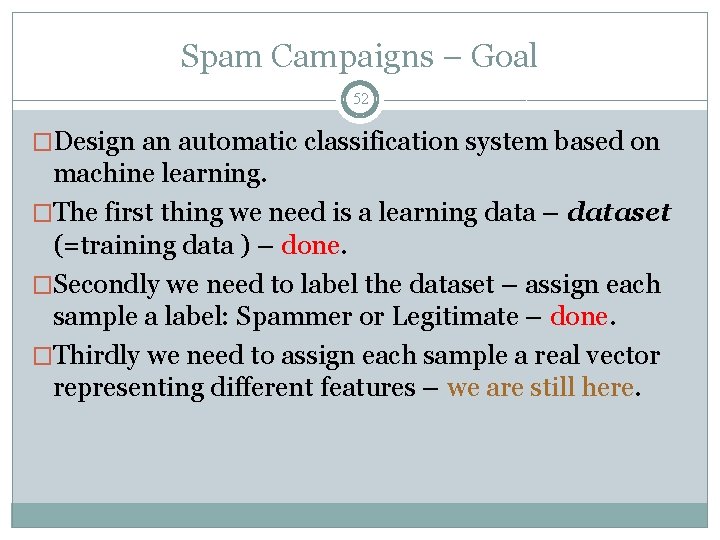
Spam Campaigns – Goal 52 �Design an automatic classification system based on machine learning. �The first thing we need is a learning data – dataset (=training data ) – done. �Secondly we need to label the dataset – assign each sample a label: Spammer or Legitimate – done. �Thirdly we need to assign each sample a real vector representing different features – we are still here.
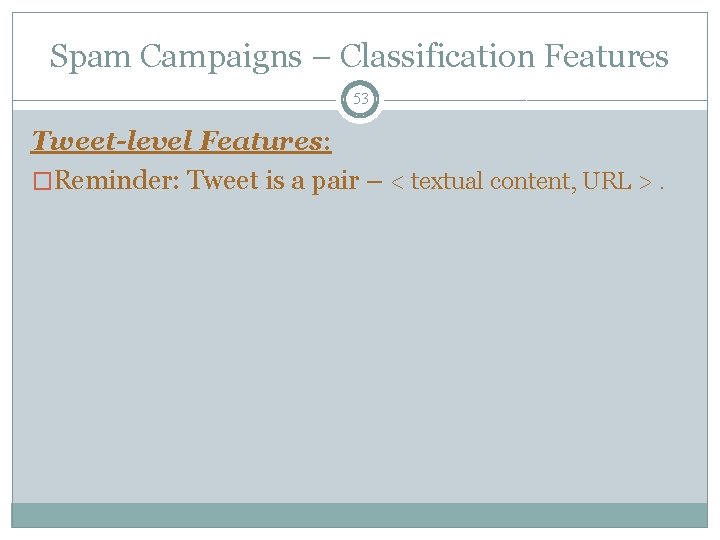
Spam Campaigns – Classification Features 53 Tweet-level Features: �Reminder: Tweet is a pair – < textual content, URL >.
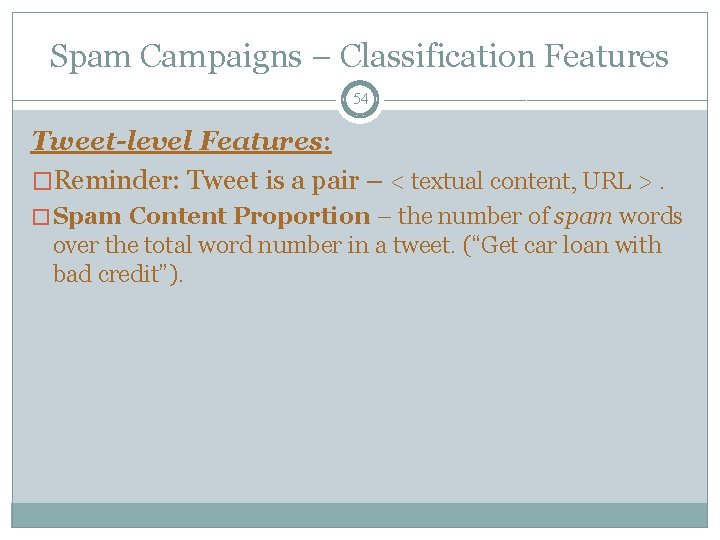
Spam Campaigns – Classification Features 54 Tweet-level Features: �Reminder: Tweet is a pair – < textual content, URL >. � Spam Content Proportion – the number of spam words over the total word number in a tweet. (“Get car loan with bad credit”).
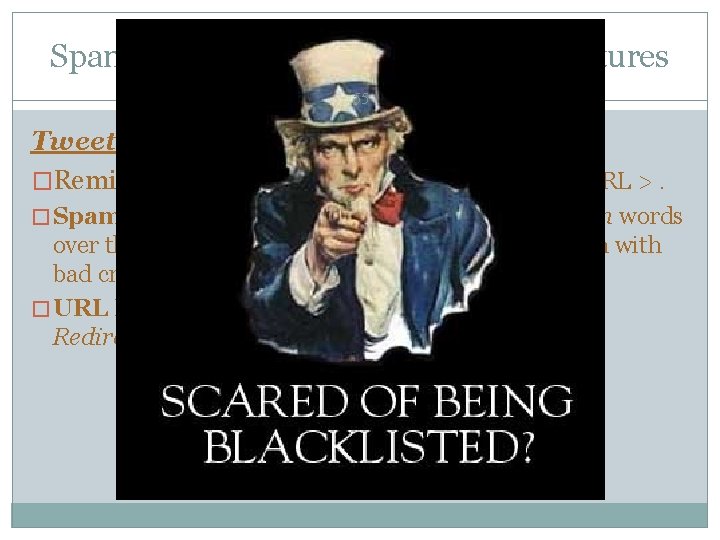
Spam Campaigns – Classification Features 55 Tweet-level Features: �Reminder: Tweet is a pair – < textual content, URL >. � Spam Content Proportion – the number of spam words over the total word number in a tweet. (“Get car loan with bad credit”). � URL Redirection – binary flag – were there URL Redirection? And the number of hops.
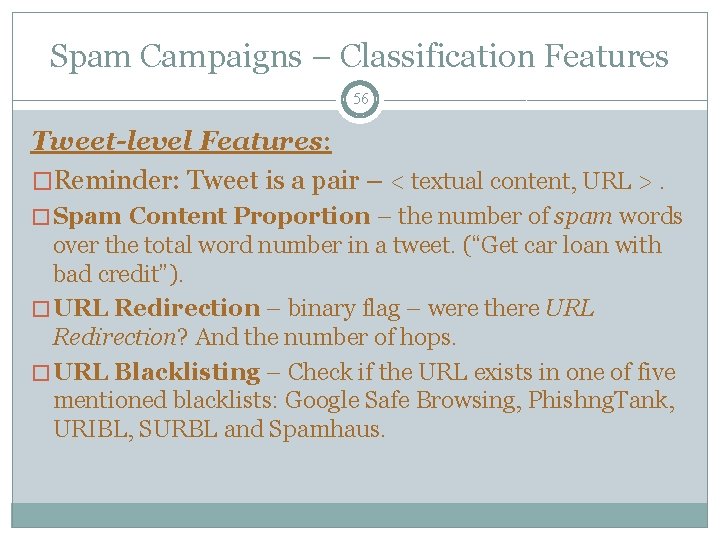
Spam Campaigns – Classification Features 56 Tweet-level Features: �Reminder: Tweet is a pair – < textual content, URL >. � Spam Content Proportion – the number of spam words over the total word number in a tweet. (“Get car loan with bad credit”). � URL Redirection – binary flag – were there URL Redirection? And the number of hops. � URL Blacklisting – Check if the URL exists in one of five mentioned blacklists: Google Safe Browsing, Phishng. Tank, URIBL, SURBL and Spamhaus.
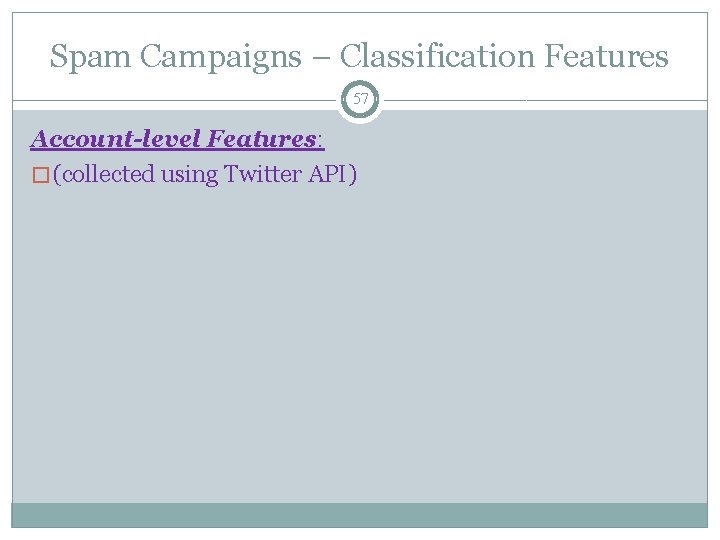
Spam Campaigns – Classification Features 57 Account-level Features: � (collected using Twitter API)
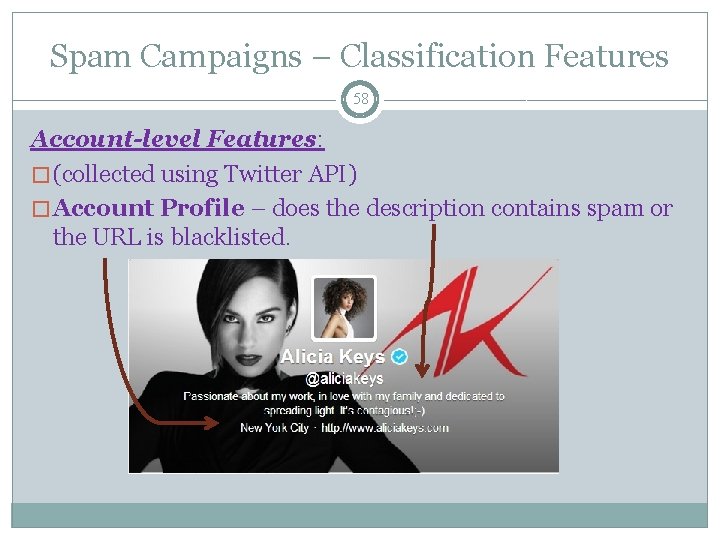
Spam Campaigns – Classification Features 58 Account-level Features: � (collected using Twitter API) � Account Profile – does the description contains spam or the URL is blacklisted.
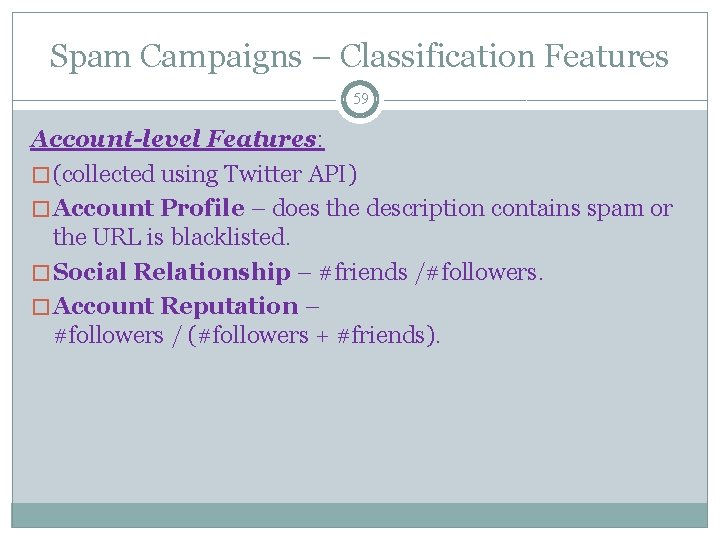
Spam Campaigns – Classification Features 59 Account-level Features: � (collected using Twitter API) � Account Profile – does the description contains spam or the URL is blacklisted. � Social Relationship – #friends /#followers. � Account Reputation – #followers / (#followers + #friends).
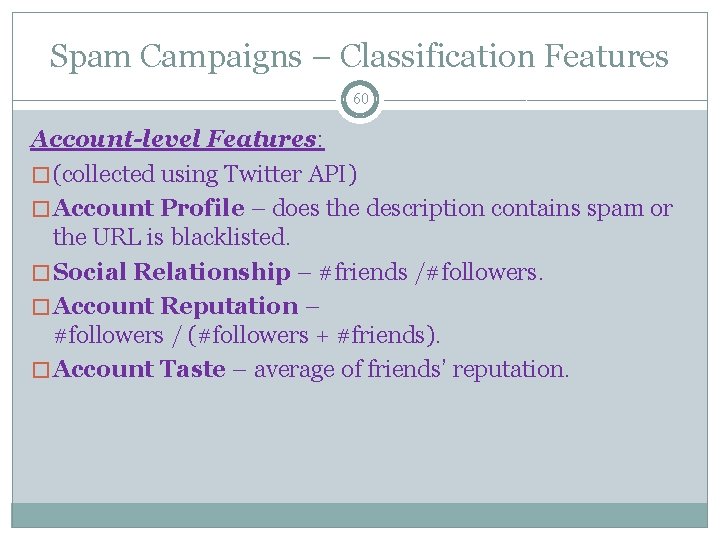
Spam Campaigns – Classification Features 60 Account-level Features: � (collected using Twitter API) � Account Profile – does the description contains spam or the URL is blacklisted. � Social Relationship – #friends /#followers. � Account Reputation – #followers / (#followers + #friends). � Account Taste – average of friends’ reputation.
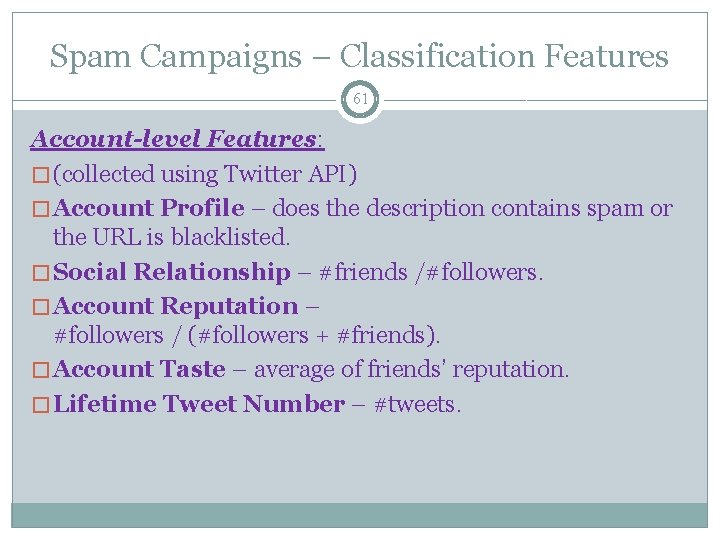
Spam Campaigns – Classification Features 61 Account-level Features: � (collected using Twitter API) � Account Profile – does the description contains spam or the URL is blacklisted. � Social Relationship – #friends /#followers. � Account Reputation – #followers / (#followers + #friends). � Account Taste – average of friends’ reputation. � Lifetime Tweet Number – #tweets.
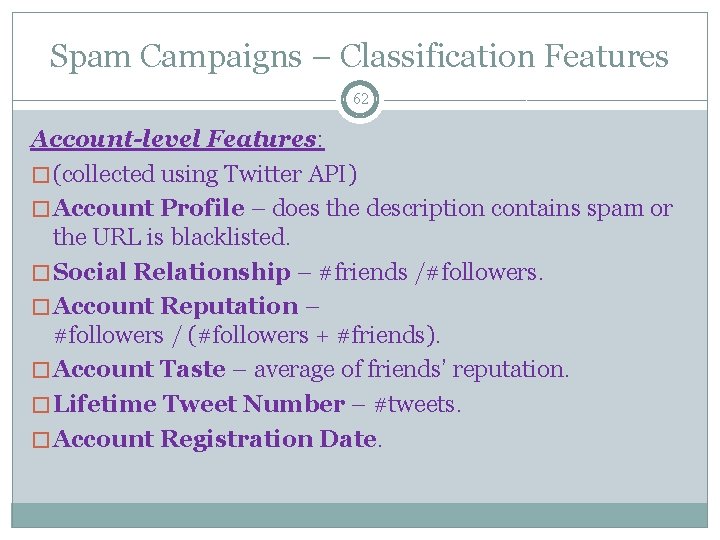
Spam Campaigns – Classification Features 62 Account-level Features: � (collected using Twitter API) � Account Profile – does the description contains spam or the URL is blacklisted. � Social Relationship – #friends /#followers. � Account Reputation – #followers / (#followers + #friends). � Account Taste – average of friends’ reputation. � Lifetime Tweet Number – #tweets. � Account Registration Date.
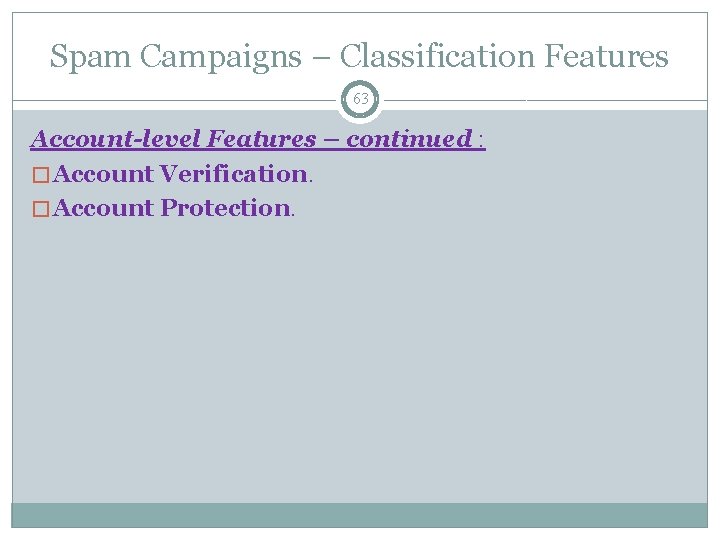
Spam Campaigns – Classification Features 63 Account-level Features – continued : � Account Verification. � Account Protection.
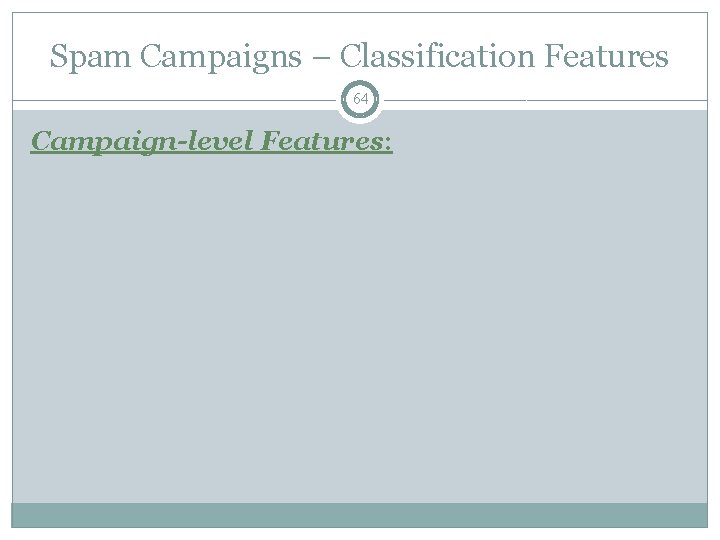
Spam Campaigns – Classification Features 64 Campaign-level Features:
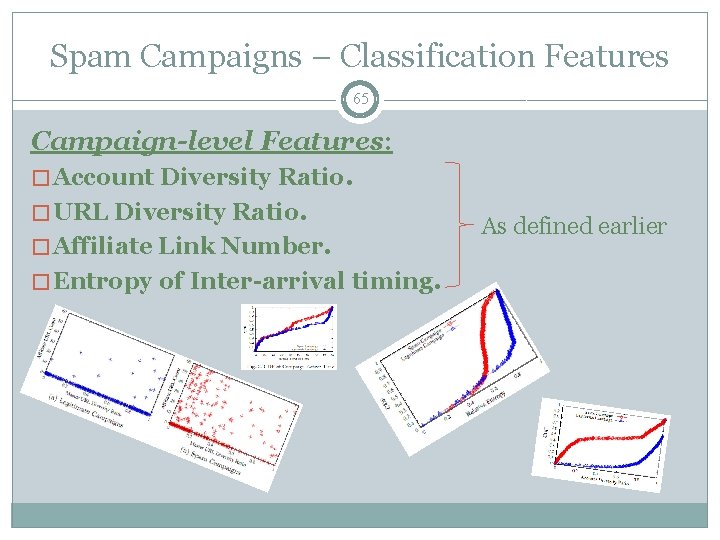
Spam Campaigns – Classification Features 65 Campaign-level Features: � Account Diversity Ratio. � URL Diversity Ratio. � Affiliate Link Number. � Entropy of Inter-arrival timing. As defined earlier
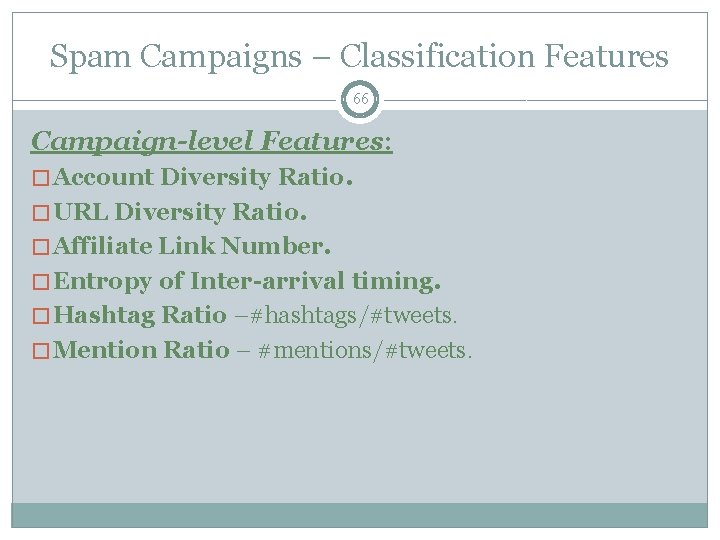
Spam Campaigns – Classification Features 66 Campaign-level Features: � Account Diversity Ratio. � URL Diversity Ratio. � Affiliate Link Number. � Entropy of Inter-arrival timing. � Hashtag Ratio –#hashtags/#tweets. � Mention Ratio – #mentions/#tweets.
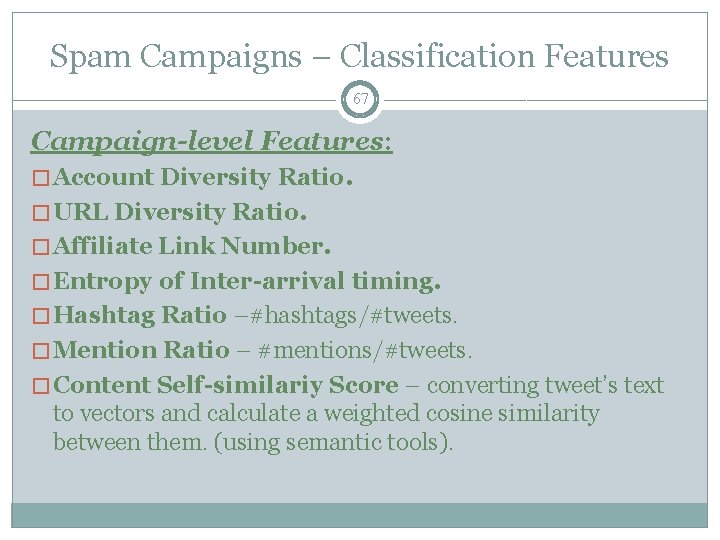
Spam Campaigns – Classification Features 67 Campaign-level Features: � Account Diversity Ratio. � URL Diversity Ratio. � Affiliate Link Number. � Entropy of Inter-arrival timing. � Hashtag Ratio –#hashtags/#tweets. � Mention Ratio – #mentions/#tweets. � Content Self-similariy Score – converting tweet’s text to vectors and calculate a weighted cosine similarity between them. (using semantic tools).
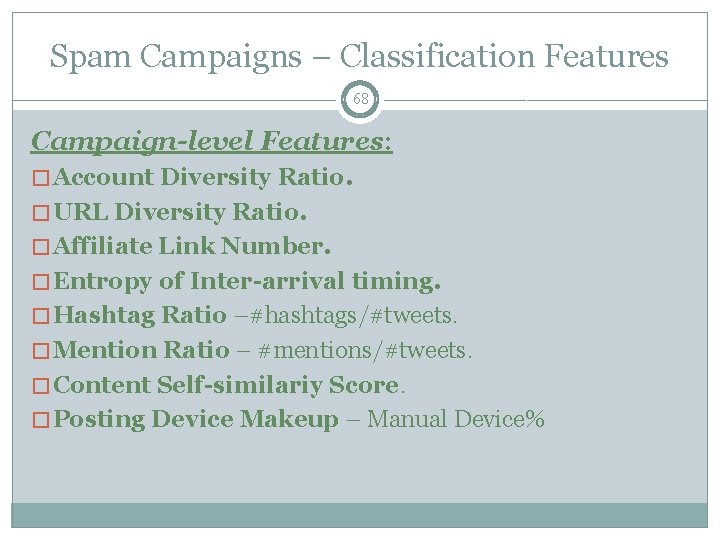
Spam Campaigns – Classification Features 68 Campaign-level Features: � Account Diversity Ratio. � URL Diversity Ratio. � Affiliate Link Number. � Entropy of Inter-arrival timing. � Hashtag Ratio –#hashtags/#tweets. � Mention Ratio – #mentions/#tweets. � Content Self-similariy Score. � Posting Device Makeup – Manual Device%
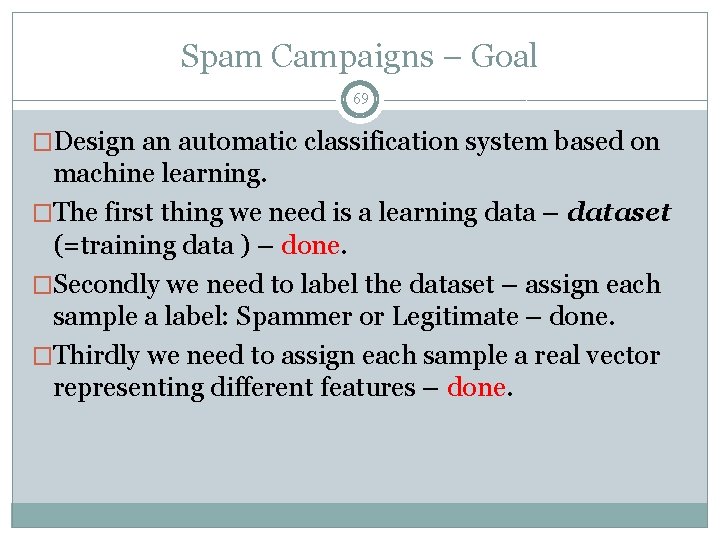
Spam Campaigns – Goal 69 �Design an automatic classification system based on machine learning. �The first thing we need is a learning data – dataset (=training data ) – done. �Secondly we need to label the dataset – assign each sample a label: Spammer or Legitimate – done. �Thirdly we need to assign each sample a real vector representing different features – done.
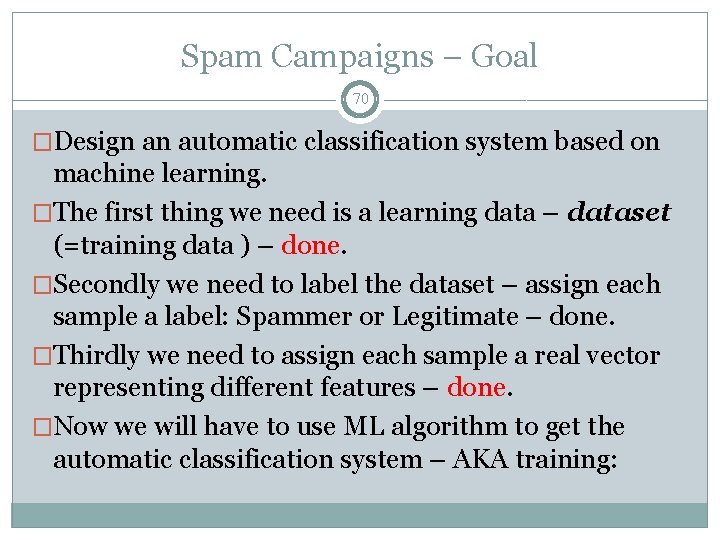
Spam Campaigns – Goal 70 �Design an automatic classification system based on machine learning. �The first thing we need is a learning data – dataset (=training data ) – done. �Secondly we need to label the dataset – assign each sample a label: Spammer or Legitimate – done. �Thirdly we need to assign each sample a real vector representing different features – done. �Now we will have to use ML algorithm to get the automatic classification system – AKA training:
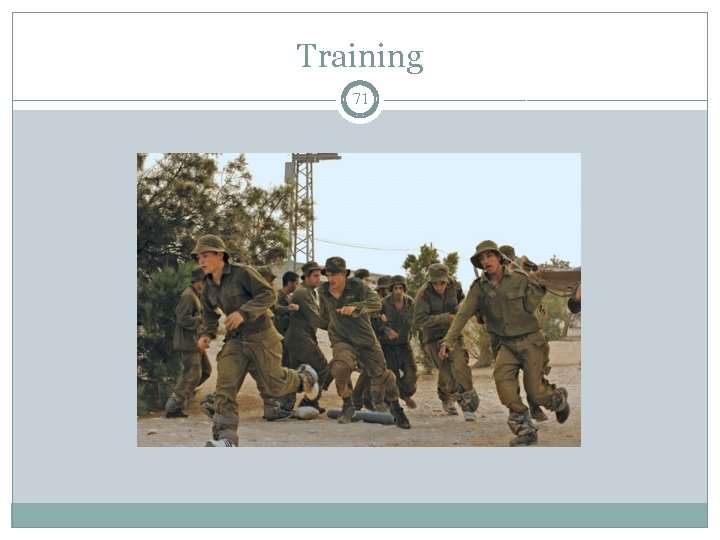
Training 71
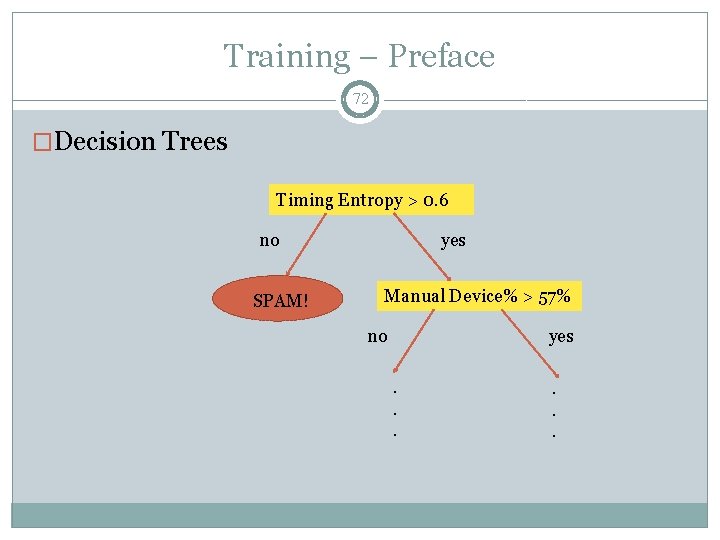
Training – Preface 72 �Decision Trees Timing Entropy > 0. 6 no SPAM! yes Manual Device% > 57% no yes. . .
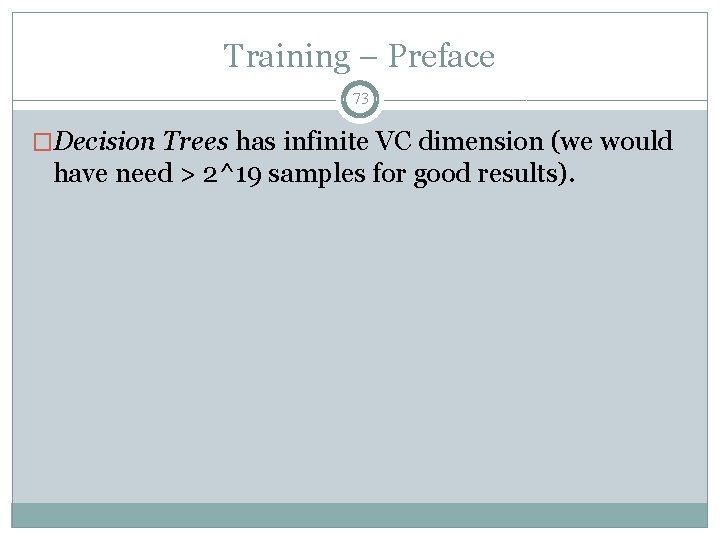
Training – Preface 73 �Decision Trees has infinite VC dimension (we would have need > 2^19 samples for good results).
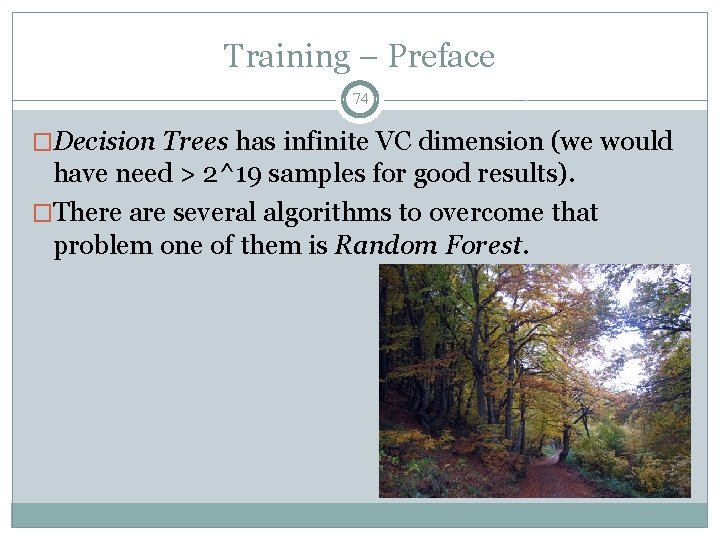
Training – Preface 74 �Decision Trees has infinite VC dimension (we would have need > 2^19 samples for good results). �There are several algorithms to overcome that problem one of them is Random Forest.
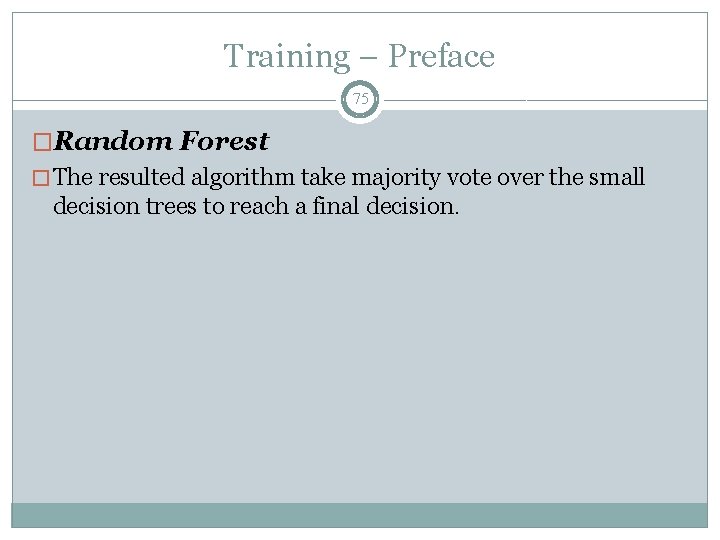
Training – Preface 75 �Random Forest � The resulted algorithm take majority vote over the small decision trees to reach a final decision.
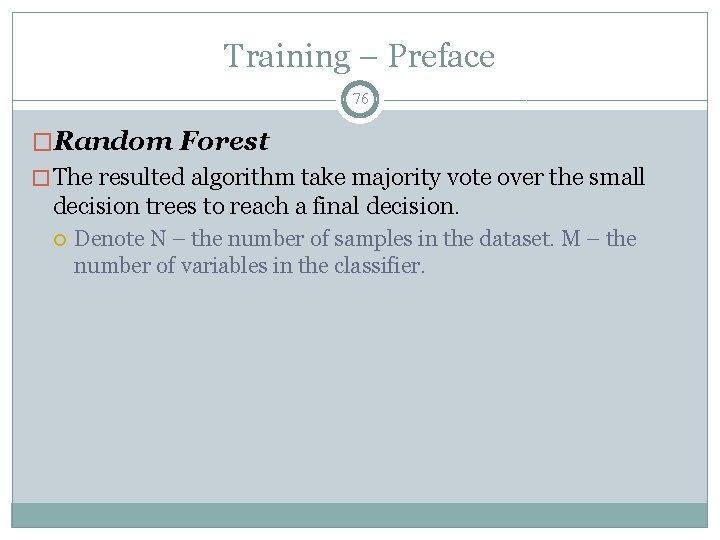
Training – Preface 76 �Random Forest � The resulted algorithm take majority vote over the small decision trees to reach a final decision. Denote N – the number of samples in the dataset. M – the number of variables in the classifier.
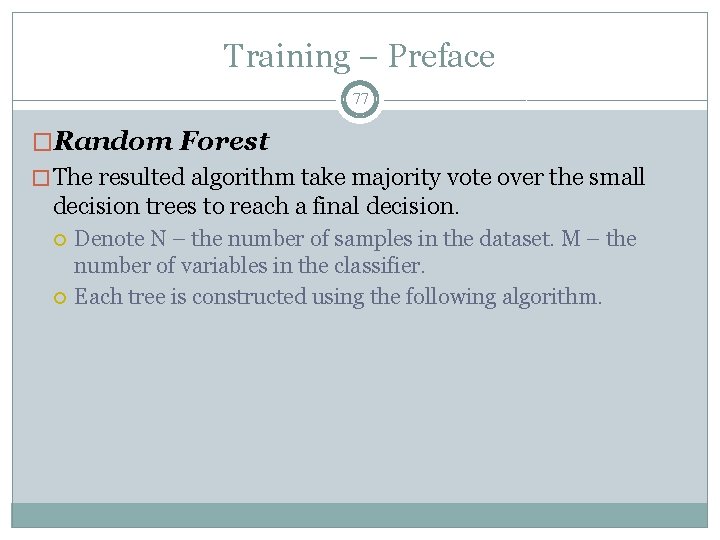
Training – Preface 77 �Random Forest � The resulted algorithm take majority vote over the small decision trees to reach a final decision. Denote N – the number of samples in the dataset. M – the number of variables in the classifier. Each tree is constructed using the following algorithm.
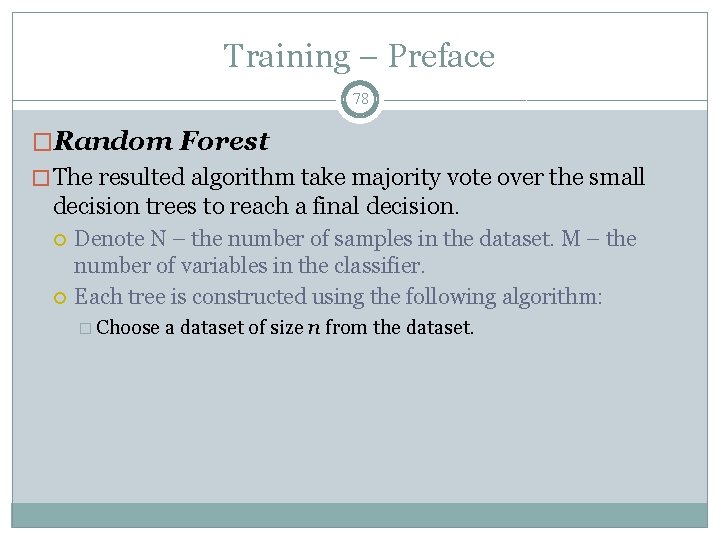
Training – Preface 78 �Random Forest � The resulted algorithm take majority vote over the small decision trees to reach a final decision. Denote N – the number of samples in the dataset. M – the number of variables in the classifier. Each tree is constructed using the following algorithm: � Choose a dataset of size n from the dataset.
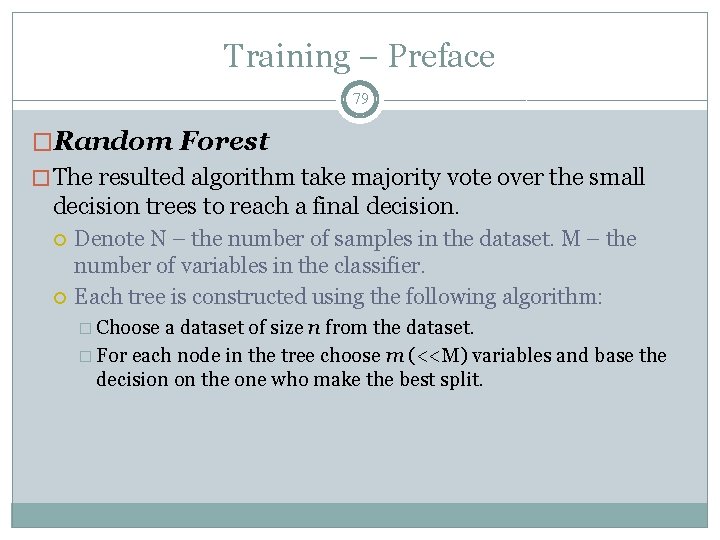
Training – Preface 79 �Random Forest � The resulted algorithm take majority vote over the small decision trees to reach a final decision. Denote N – the number of samples in the dataset. M – the number of variables in the classifier. Each tree is constructed using the following algorithm: � Choose a dataset of size n from the dataset. � For each node in the tree choose m (<<M) variables and base the decision on the one who make the best split.
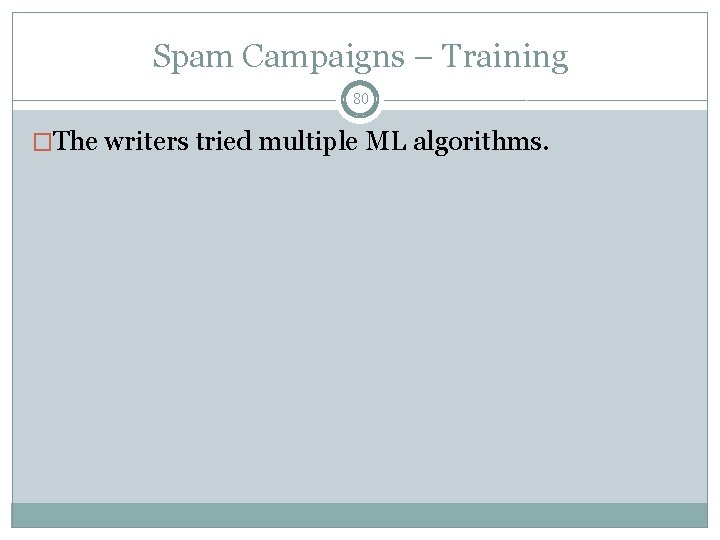
Spam Campaigns – Training 80 �The writers tried multiple ML algorithms.
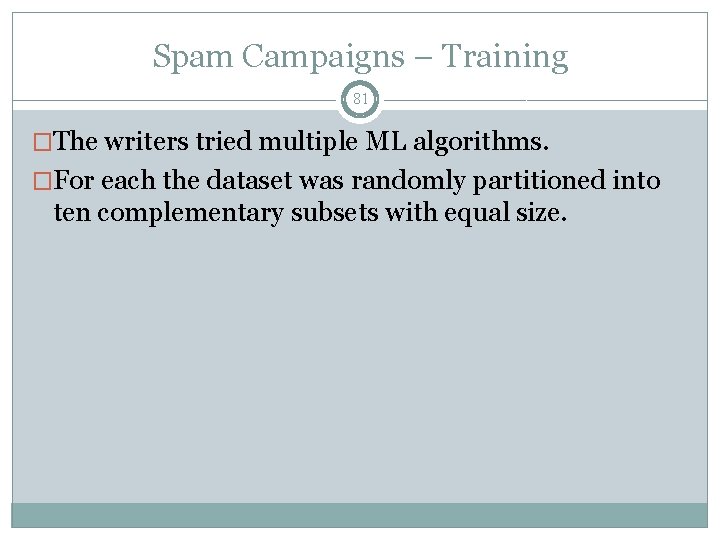
Spam Campaigns – Training 81 �The writers tried multiple ML algorithms. �For each the dataset was randomly partitioned into ten complementary subsets with equal size.
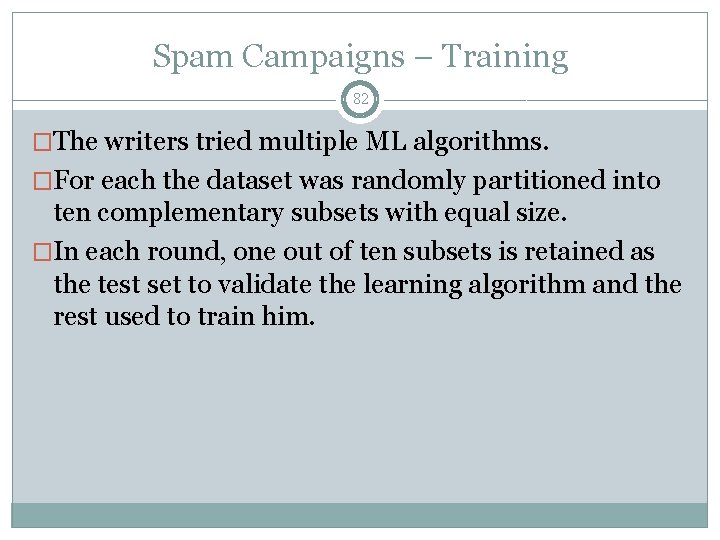
Spam Campaigns – Training 82 �The writers tried multiple ML algorithms. �For each the dataset was randomly partitioned into ten complementary subsets with equal size. �In each round, one out of ten subsets is retained as the test set to validate the learning algorithm and the rest used to train him.
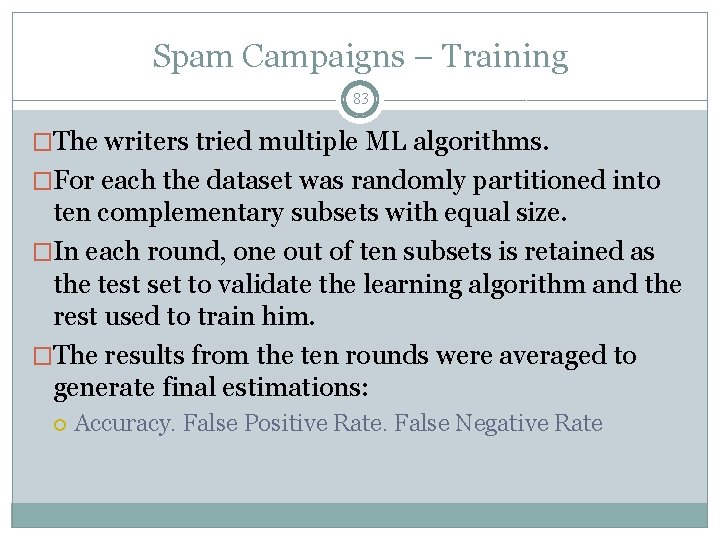
Spam Campaigns – Training 83 �The writers tried multiple ML algorithms. �For each the dataset was randomly partitioned into ten complementary subsets with equal size. �In each round, one out of ten subsets is retained as the test set to validate the learning algorithm and the rest used to train him. �The results from the ten rounds were averaged to generate final estimations: Accuracy. False Positive Rate. False Negative Rate
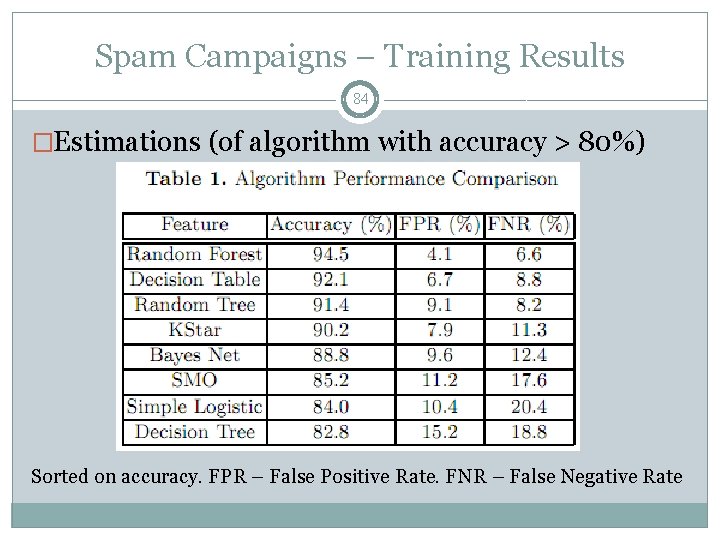
Spam Campaigns – Training Results 84 �Estimations (of algorithm with accuracy > 80%) Sorted on accuracy. FPR – False Positive Rate. FNR – False Negative Rate
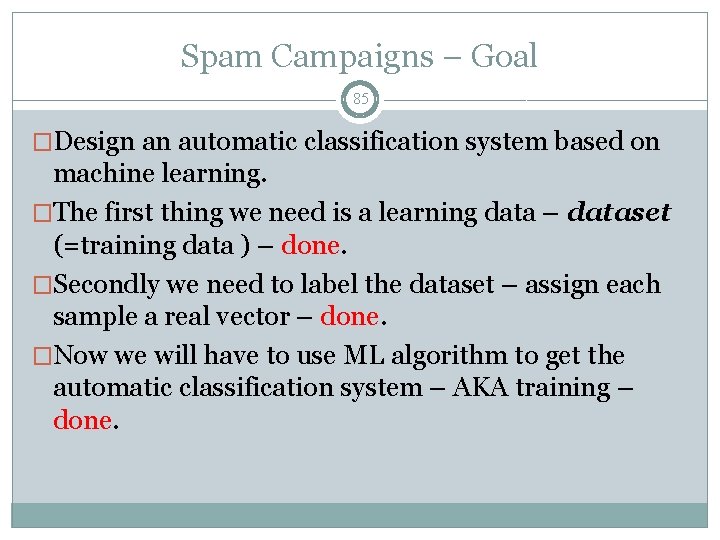
Spam Campaigns – Goal 85 �Design an automatic classification system based on machine learning. �The first thing we need is a learning data – dataset (=training data ) – done. �Secondly we need to label the dataset – assign each sample a real vector – done. �Now we will have to use ML algorithm to get the automatic classification system – AKA training – done.
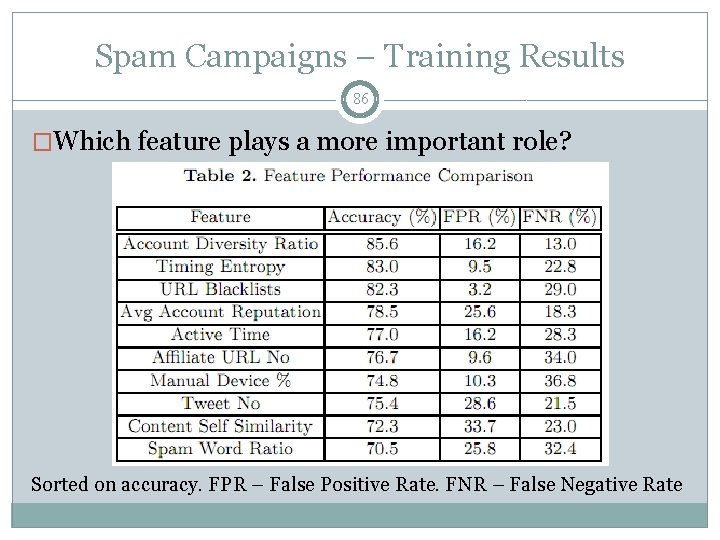
Spam Campaigns – Training Results 86 �Which feature plays a more important role? Sorted on accuracy. FPR – False Positive Rate. FNR – False Negative Rate
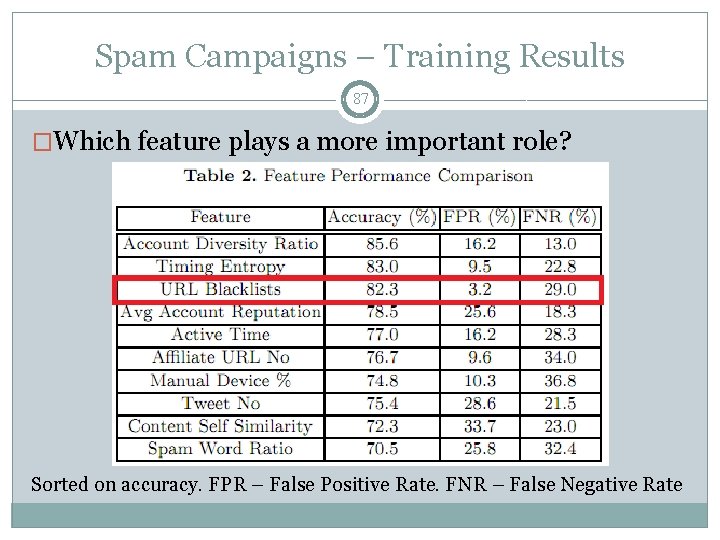
Spam Campaigns – Training Results 87 �Which feature plays a more important role? Sorted on accuracy. FPR – False Positive Rate. FNR – False Negative Rate
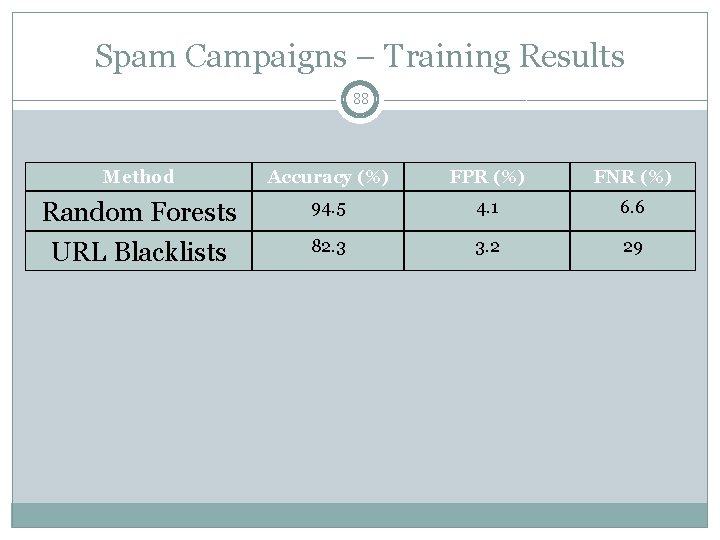
Spam Campaigns – Training Results 88 Method Accuracy (%) FPR (%) FNR (%) Random Forests URL Blacklists 94. 5 4. 1 6. 6 82. 3 3. 2 29
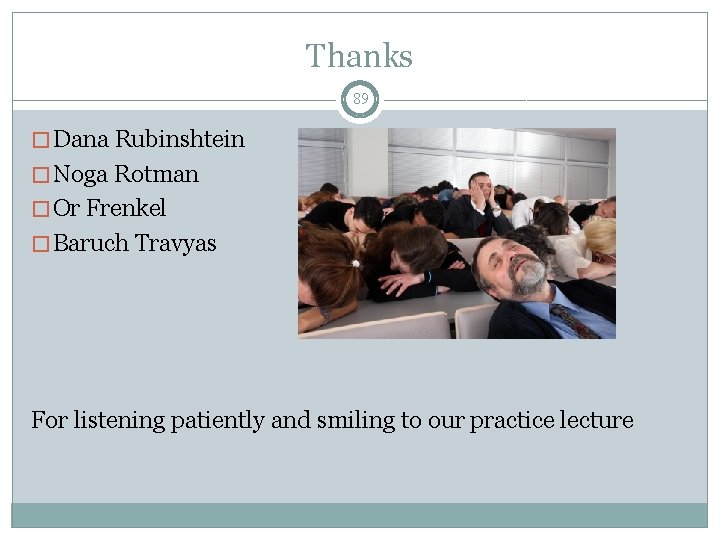
Thanks 89 � Dana Rubinshtein � Noga Rotman � Or Frenkel � Baruch Travyas For listening patiently and smiling to our practice lecture
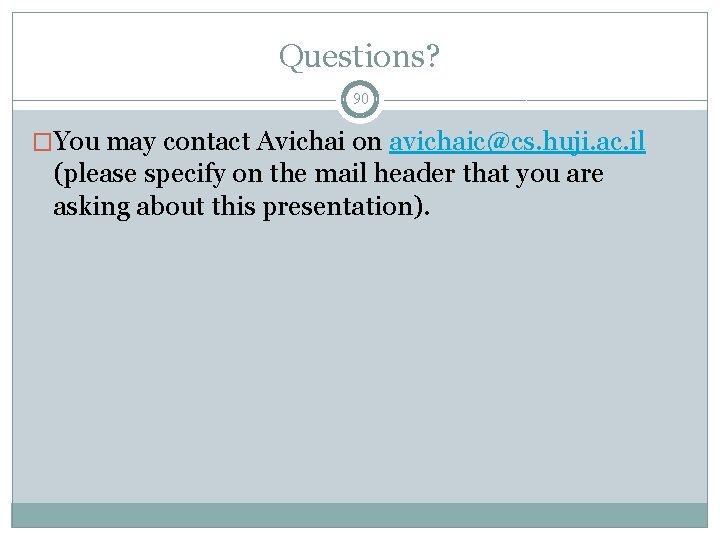
Questions? 90 �You may contact Avichai on avichaic@cs. huji. ac. il (please specify on the mail header that you are asking about this presentation).
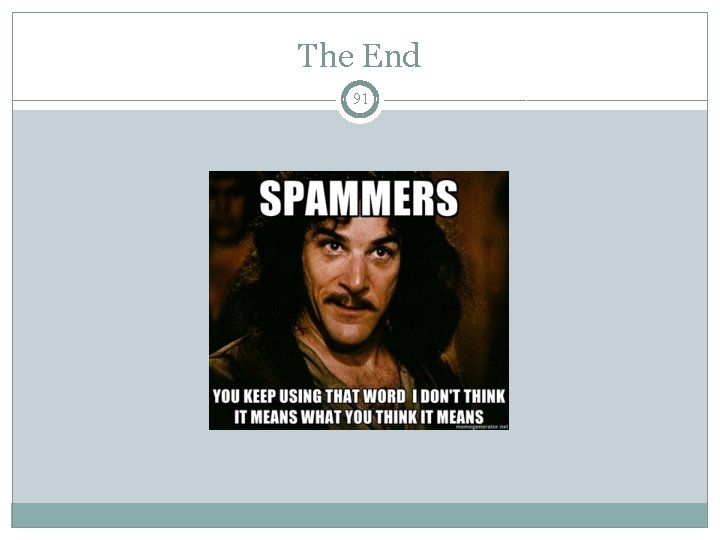
The End 91
What happens in chapter 8 of the hunger games
Types of games indoor and outdoor
Jason lee twitter
Seamless mpls architecture
Hashtags twitter statistics
Pntrack
Goes twitter
Twitter api language
On twitter
Cari cesarotti
Twitter ref 2021
Http_twitter
Ref 2021 twitter
Twitter como herramienta educativa
A recipe for meaningful gamification
Twitter
Características de twitter
Twitter
Concept of human resources management
Meteorolojibey twitter
Karim emile bitar
Zimta website
Forticlin
Twitter sentiment
Sarah jacobson williams
Twitter syria
Nocebo effect
Angel garcia banchs twitter
How to use twitter effectively for business
Tamp twitter
Twitter
Twitter
Twitter
Twitter, inc. peakontor
Julie bayley twitter
Ukfp twitter
Www.twitter.com home
Twitter
Twitter
Twitter gossi the dog
Twitter
Uses of job analysis
Tweepy.cursor(api.search
Twitter@yuehying
Twitter como fonte de notícias
Poulton lancelyn twitter
Https://mywi
Ssis twitter
Twitter.com
Pubs twitter
Pesisvalmennus
Twitter
Twitter
Inflectional and derivational morphemes exercises
Creare cont twitter
Manfred touron about
Twitter doc box
Markz live youtube
Wordpress twitter
What is armitage
Teacher twitter
Twitter most used words
Linq2 アプリ
Trend
Twitter hashtag talleres
John mandrola twitter
Twitter angel garcia banchs
How to use twitter for beginners
Twitter erhan erkut
Inurl:twitter.com
Social media osint
Stata twitter
Jelly bean twitter
Pyspark sentiment analysis
Biblioteca de mundet
Biblioteca mundet ub
Osintdefense
Rawwcf twitter
Twitter shorten url
Nick linford twitter
Jack harrison family barrister twitter
Klaus dodds
Econ ra twitter
Twitter preparatic
@blind twitter
How to use twitter in the classroom
Jo14 gaming twitter
Halit keskin twitter
Twitterment
Chewing lice
Html
Katy huberty twitter