Participant Presentations Please Sign Up Name Email Onyen
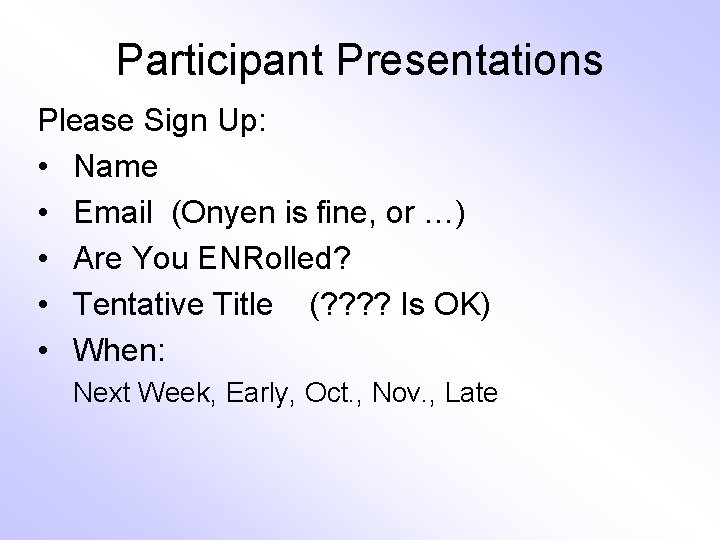
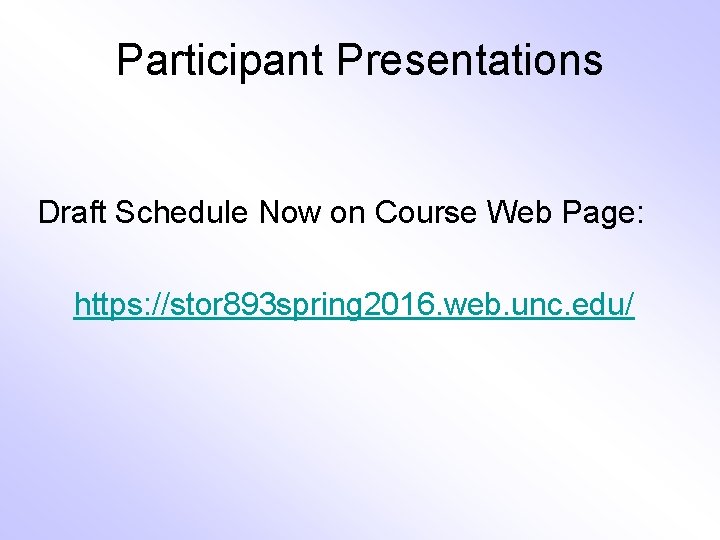
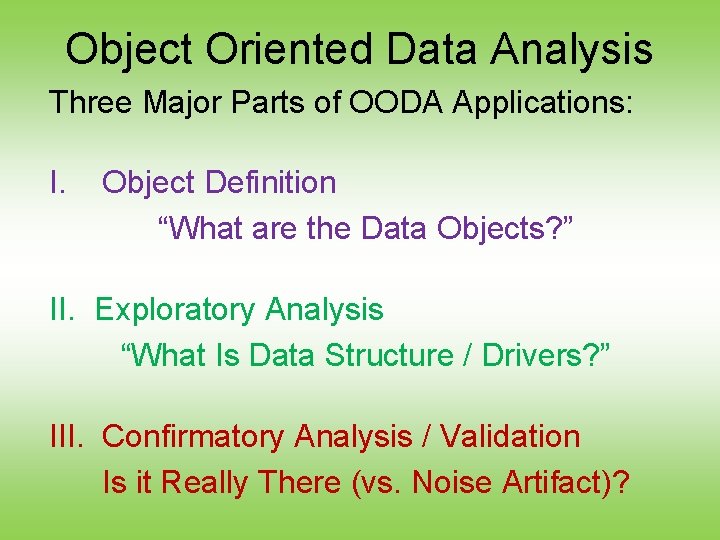
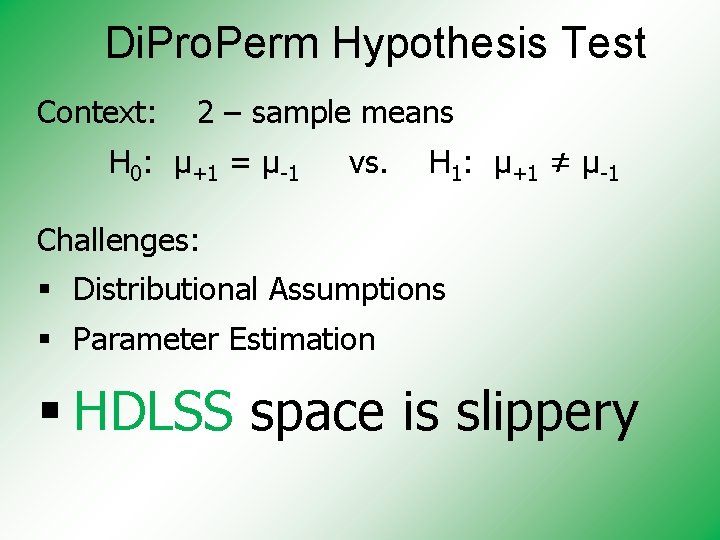
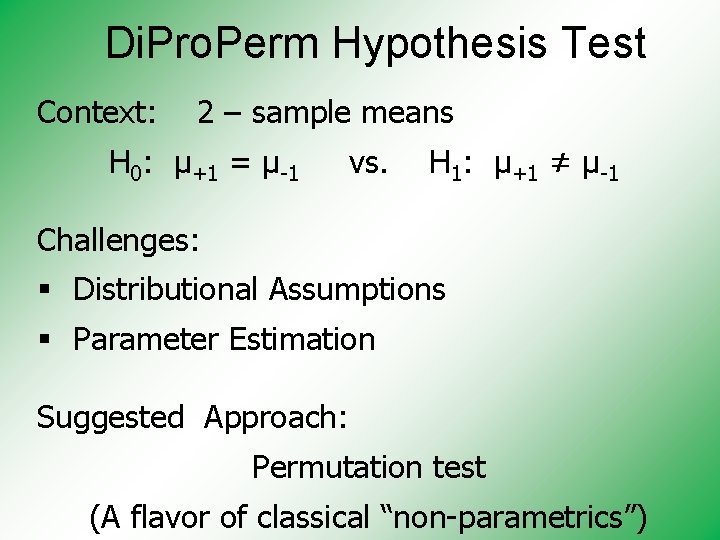
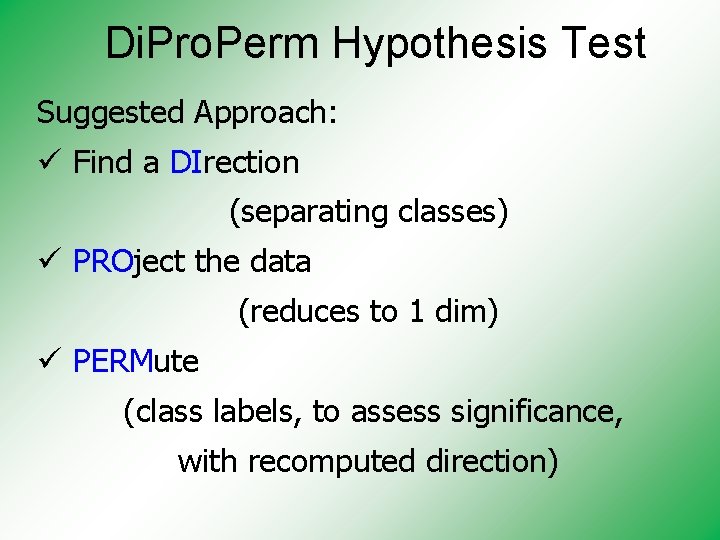
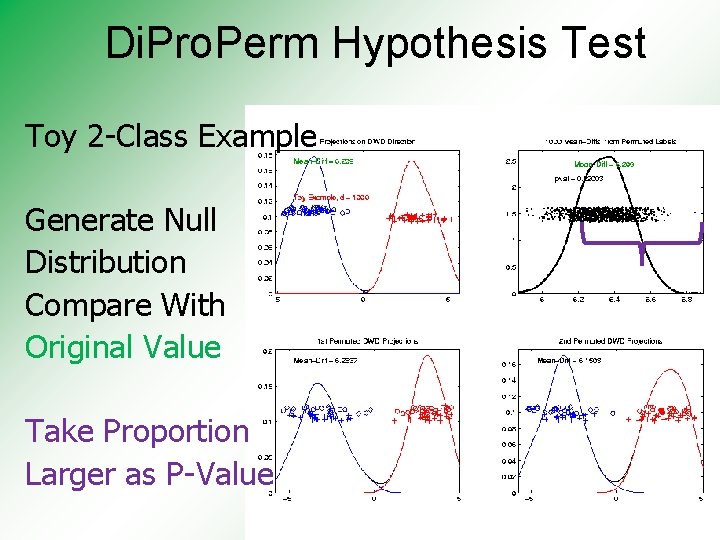
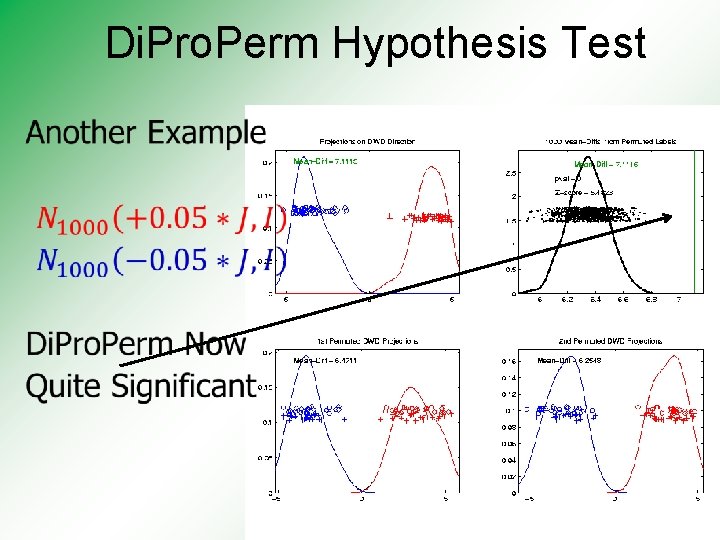
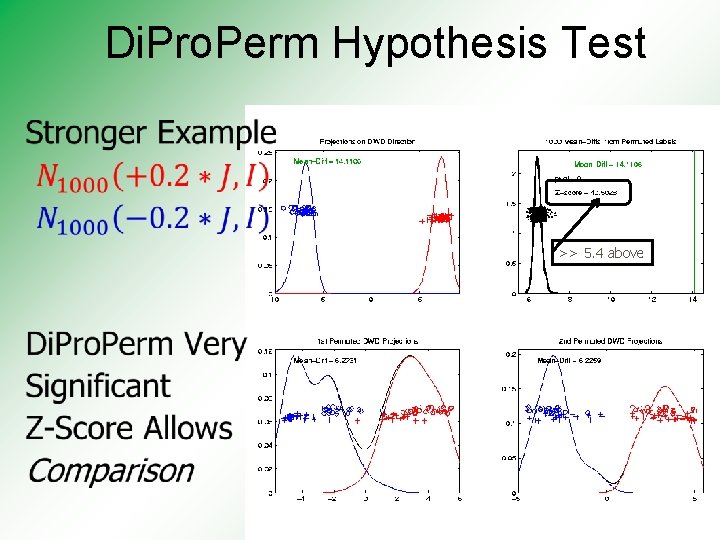
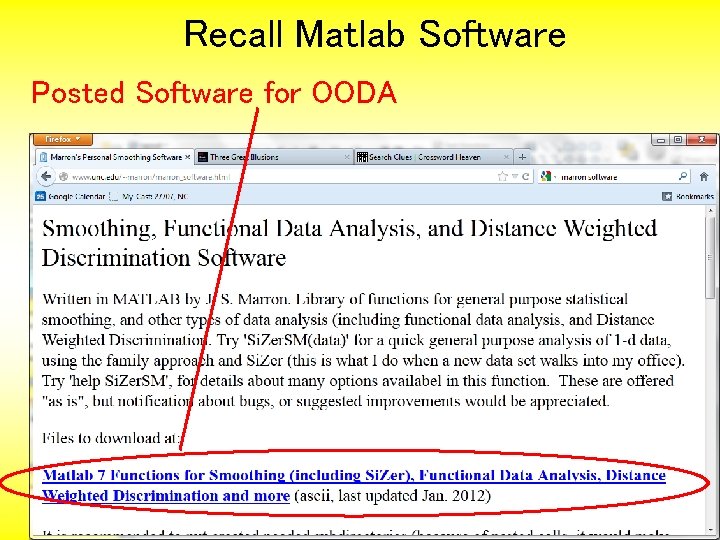
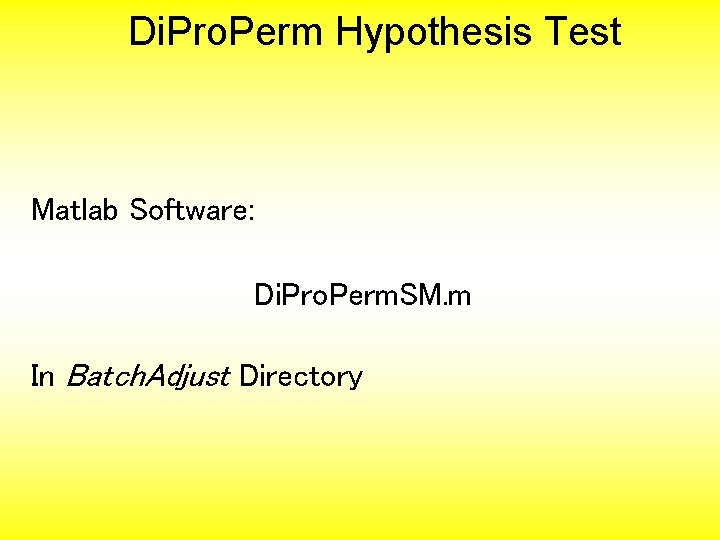
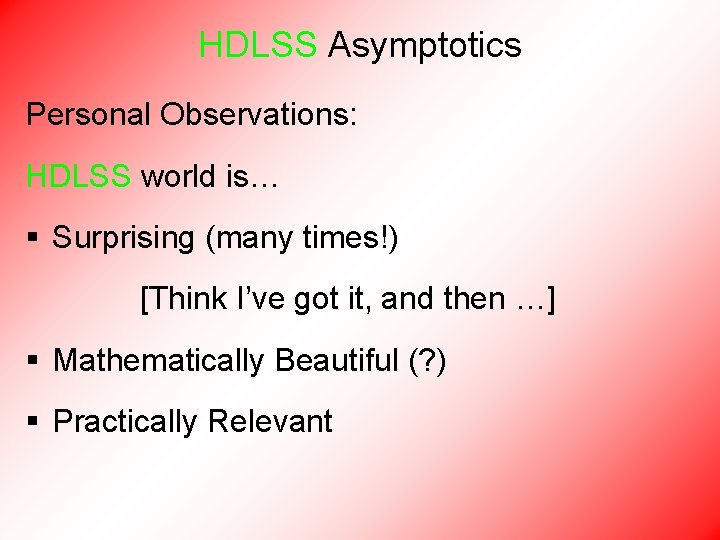
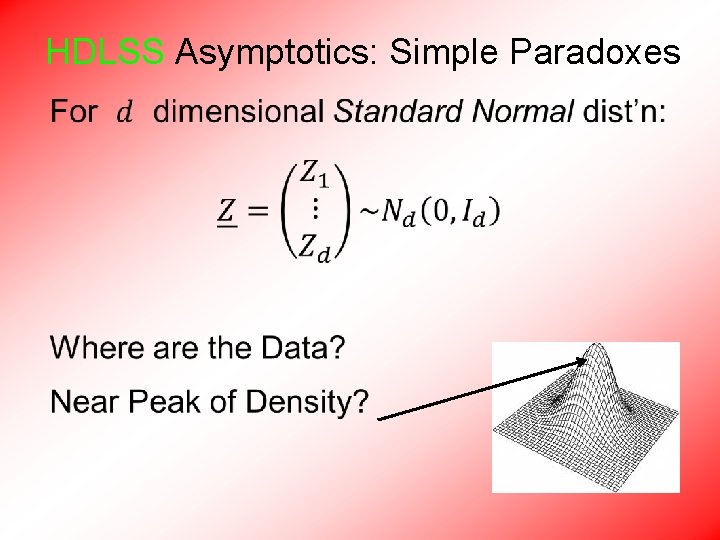
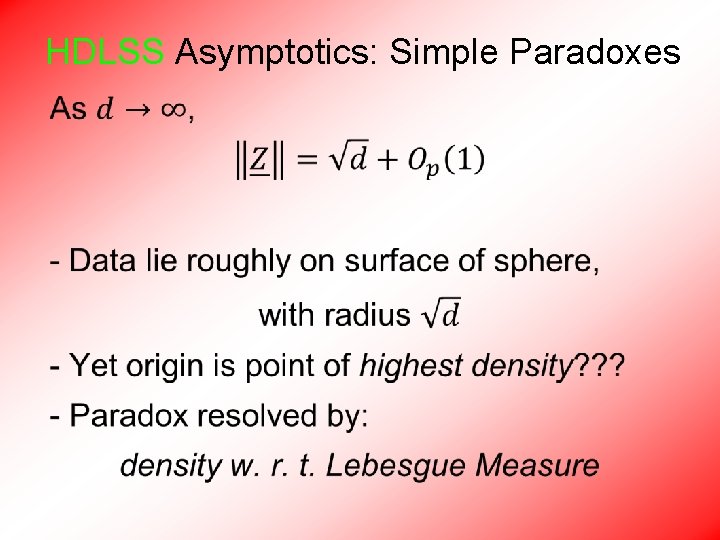
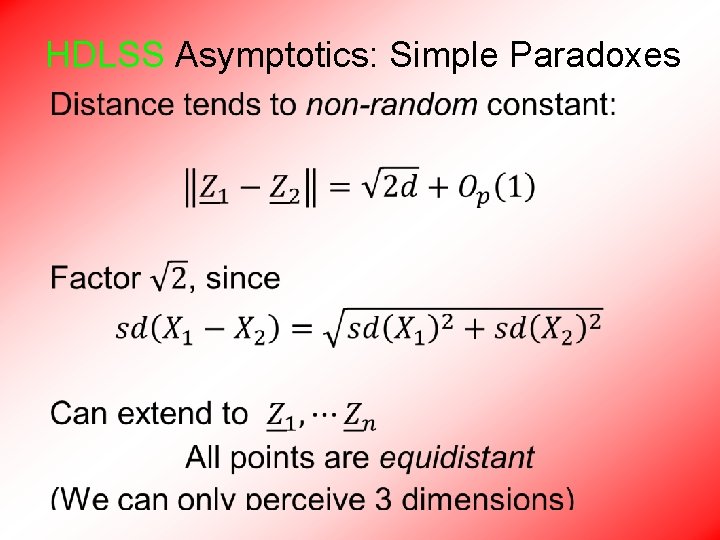
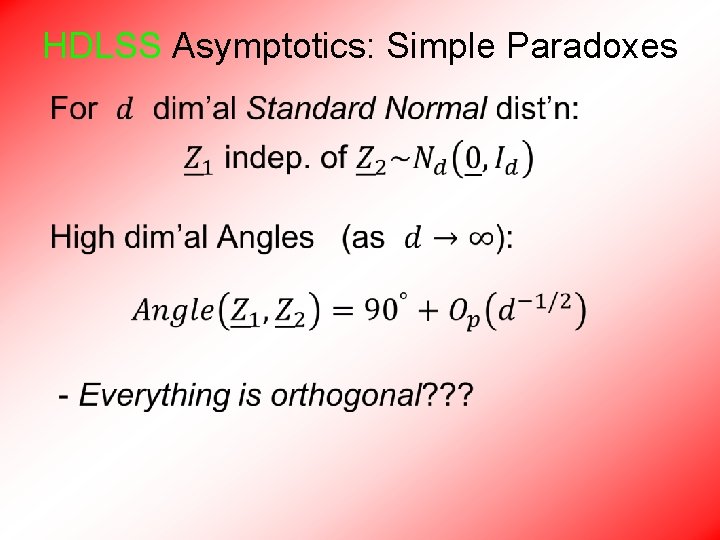
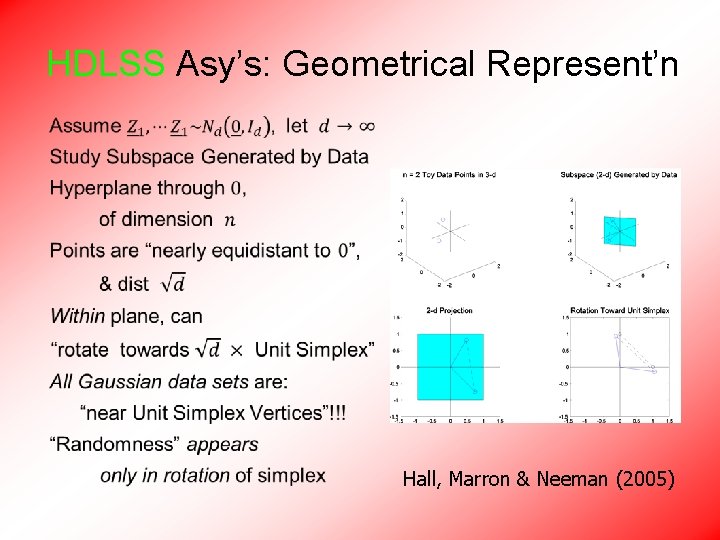
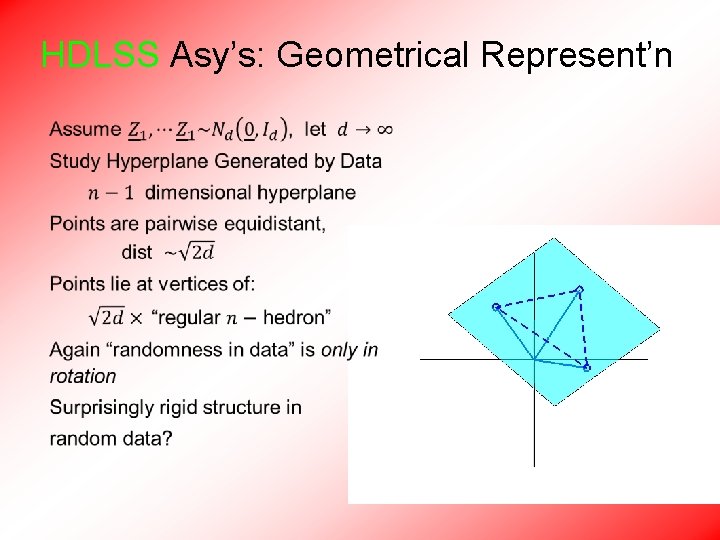
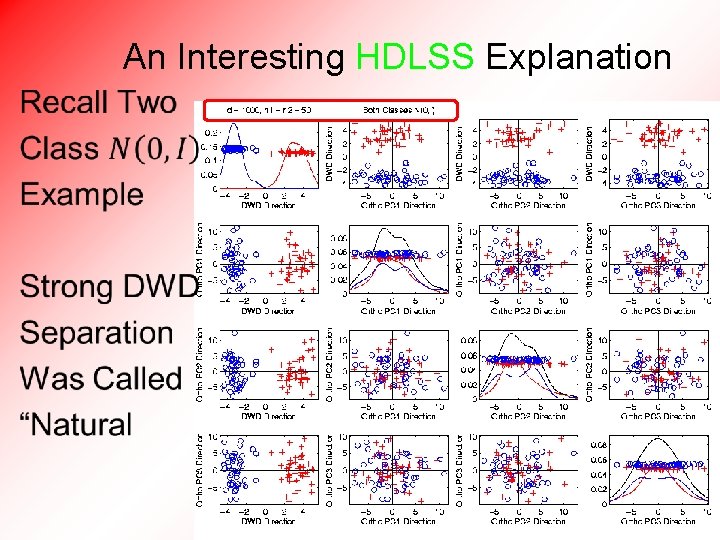
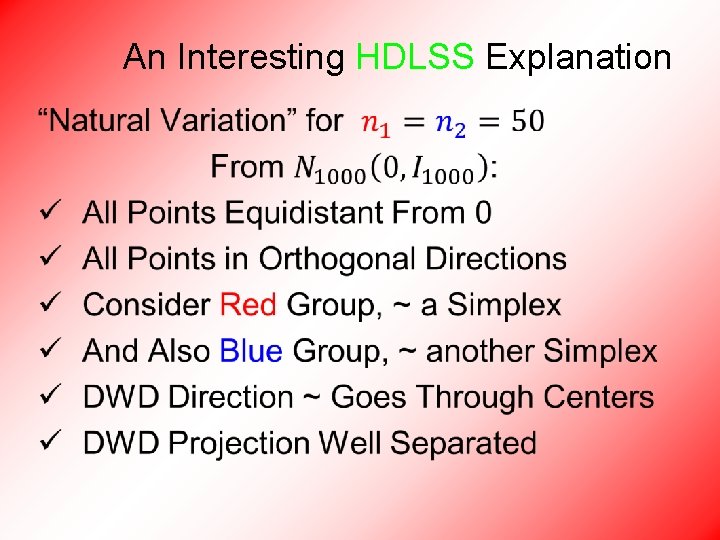
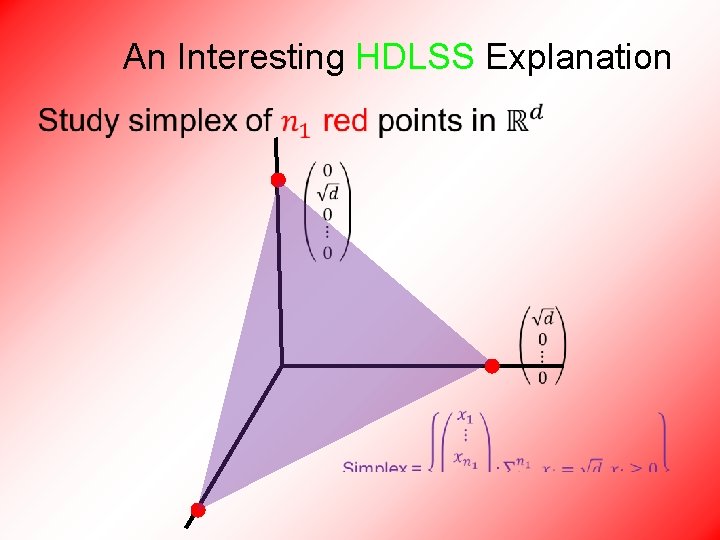
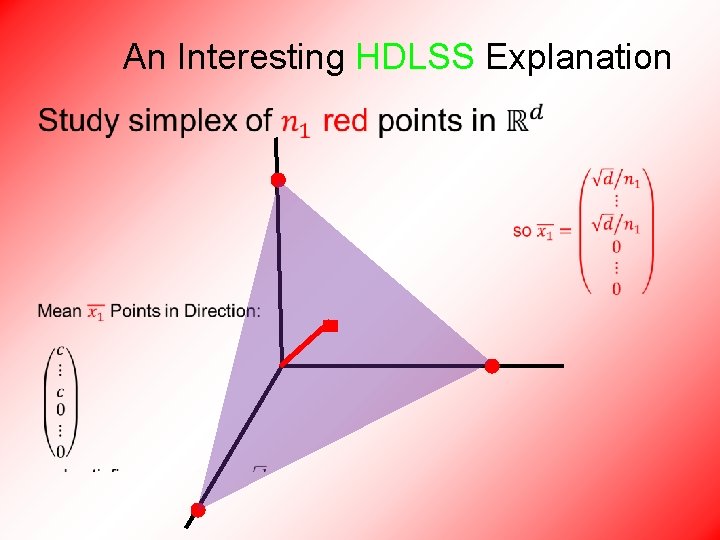
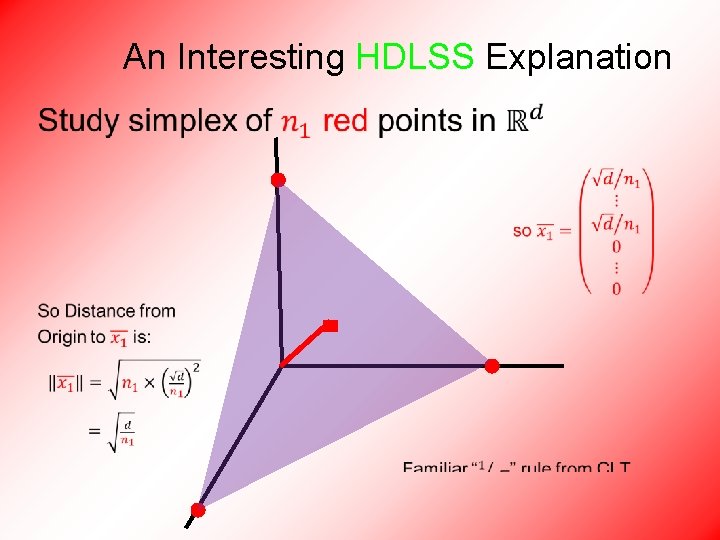
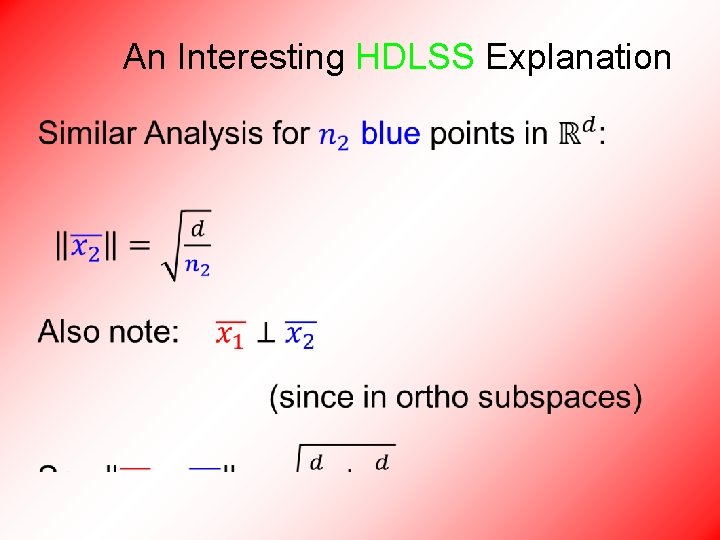
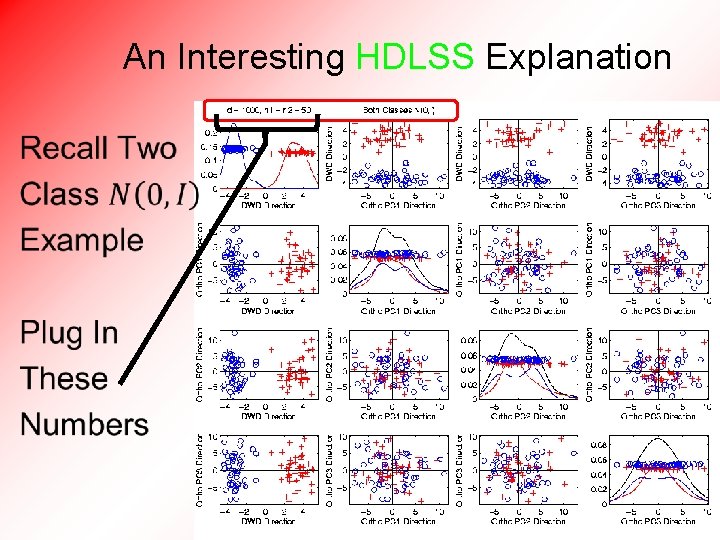
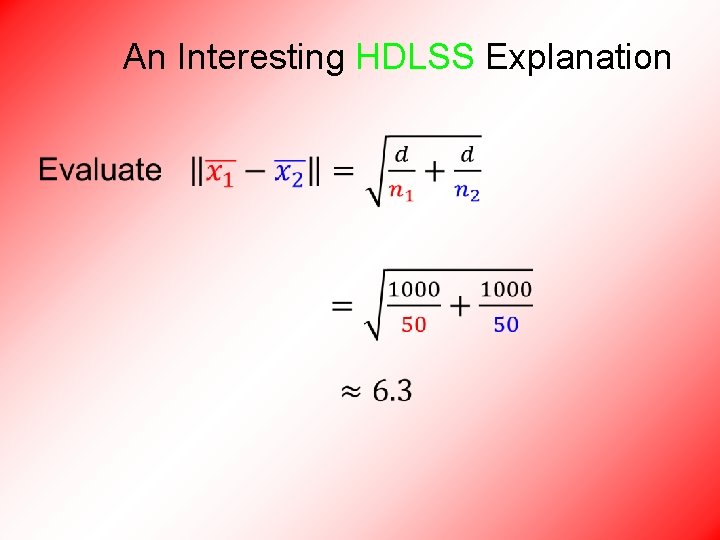
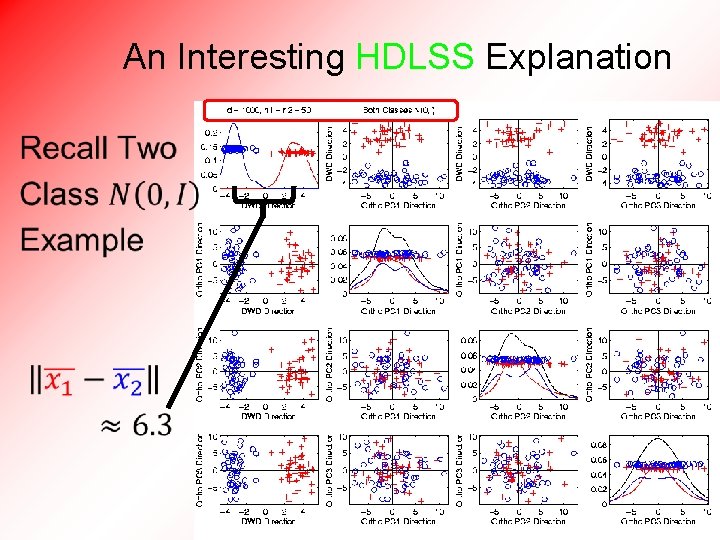
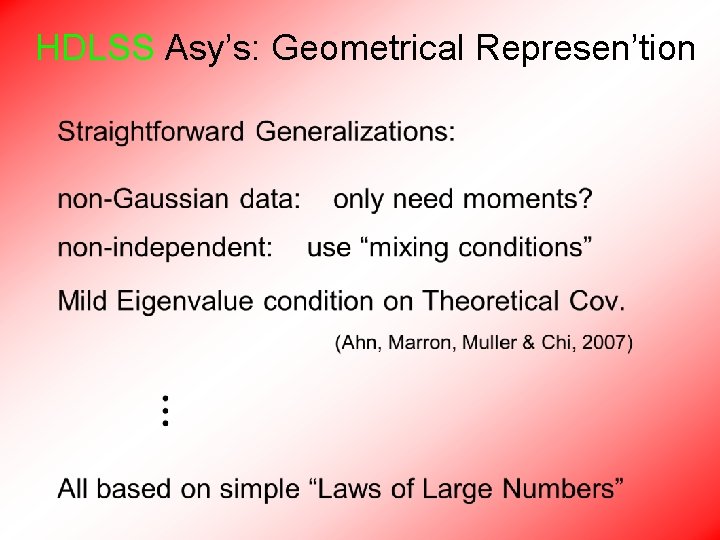
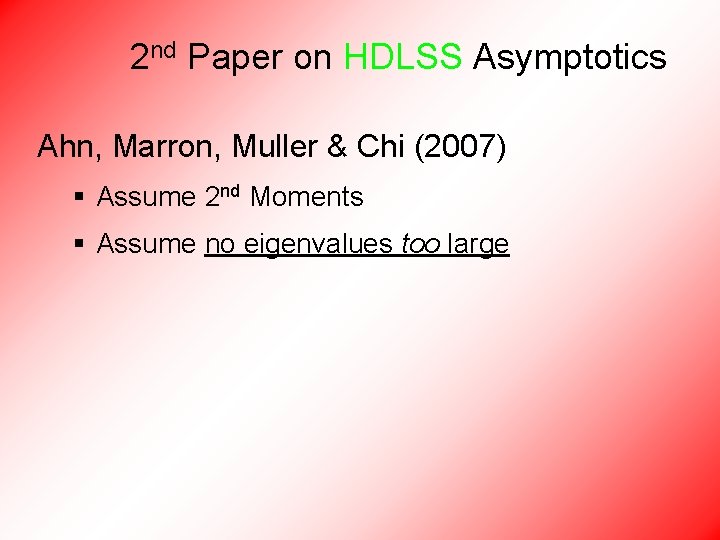
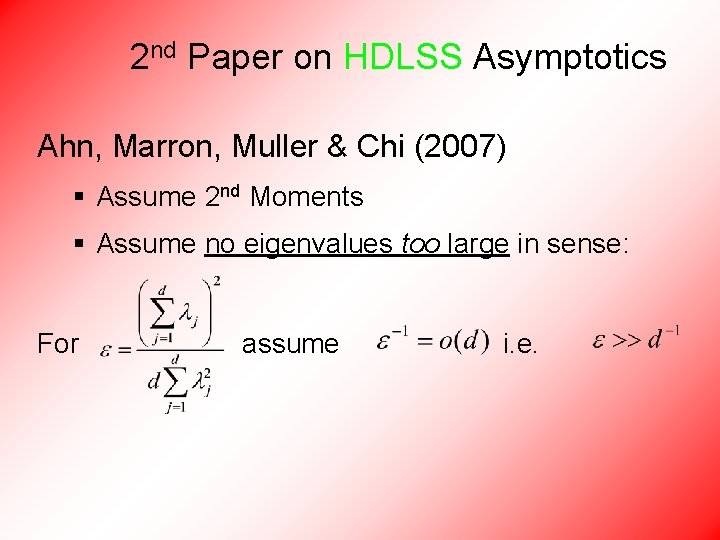
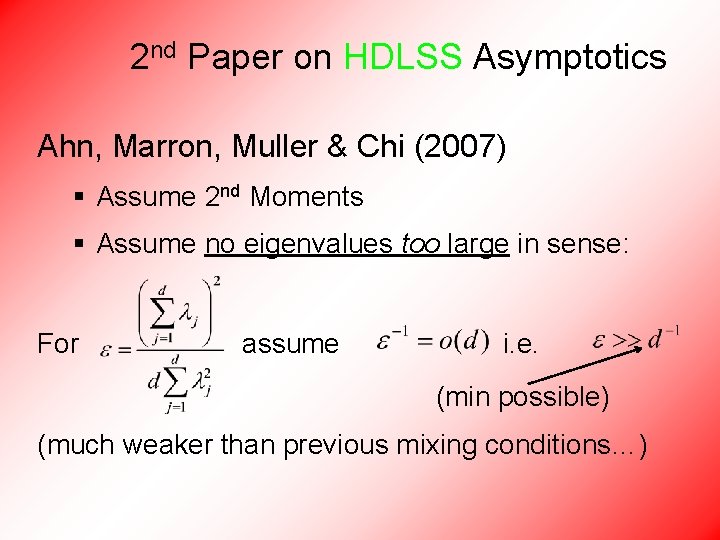
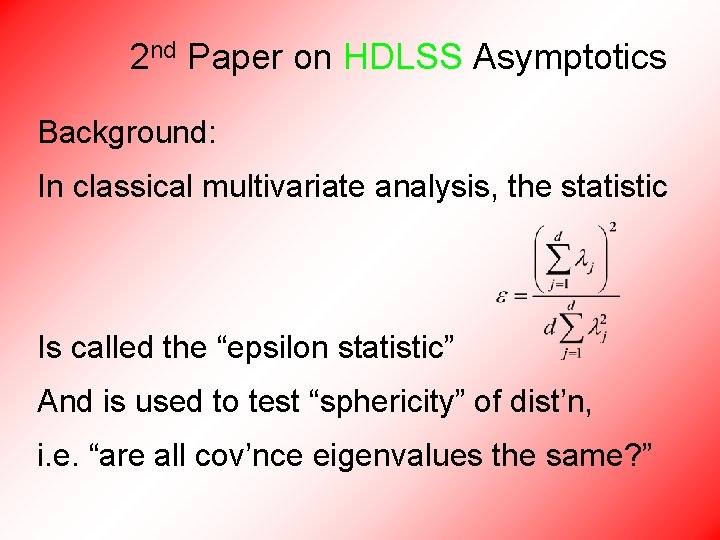
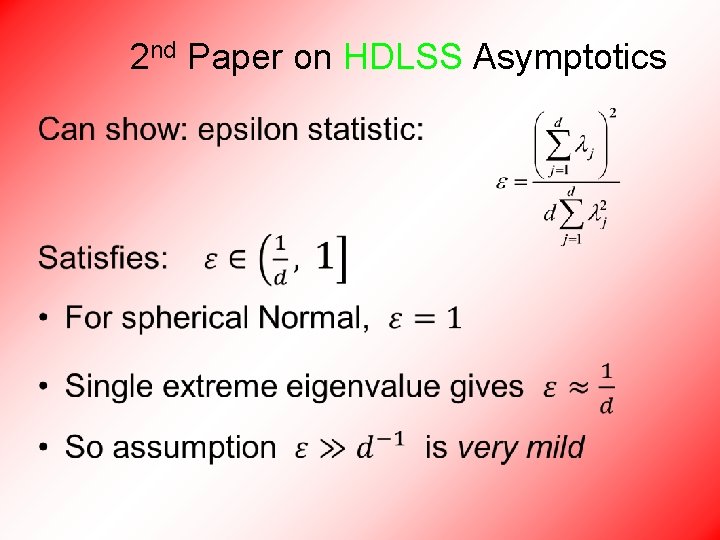
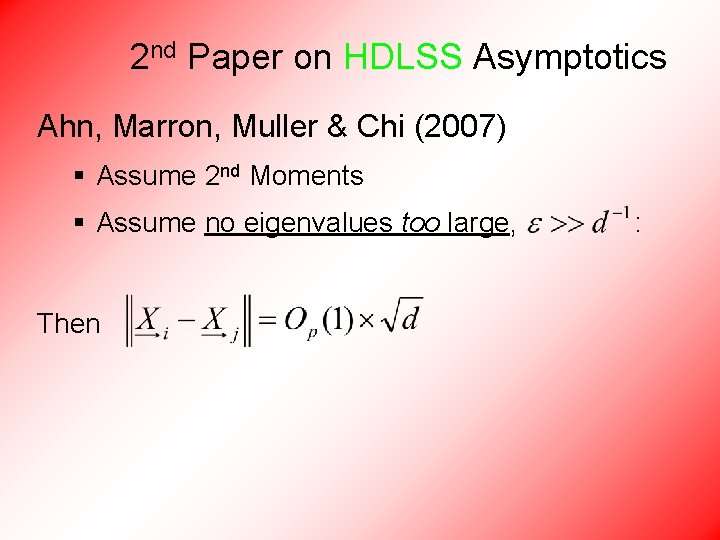
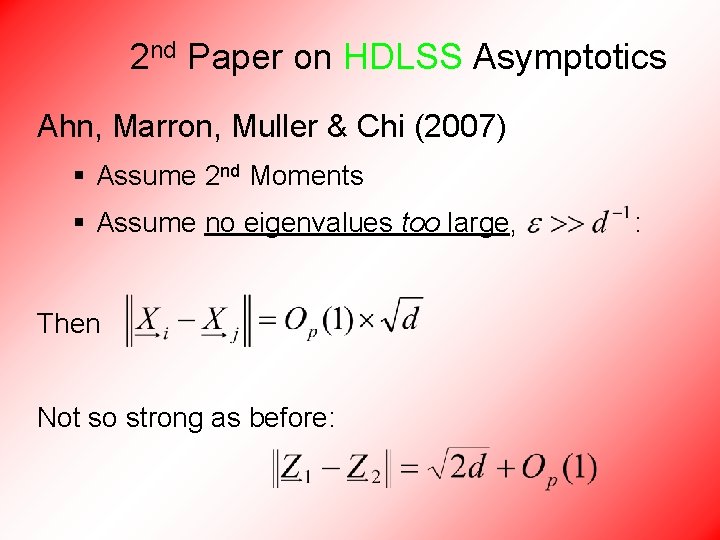
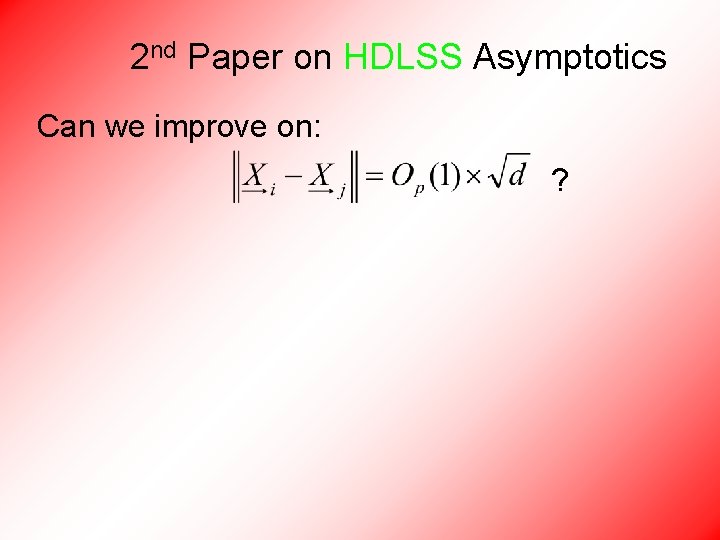
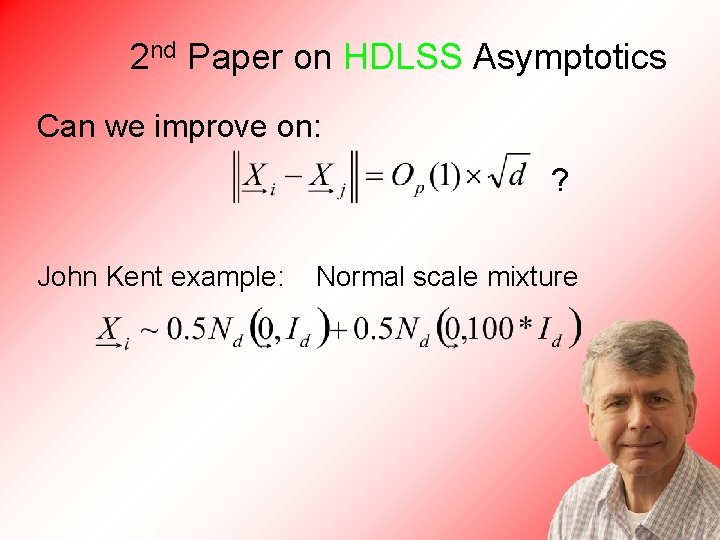
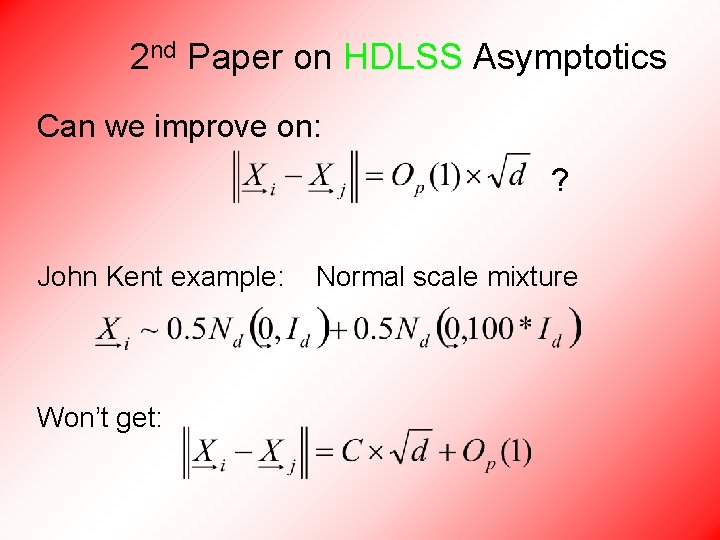
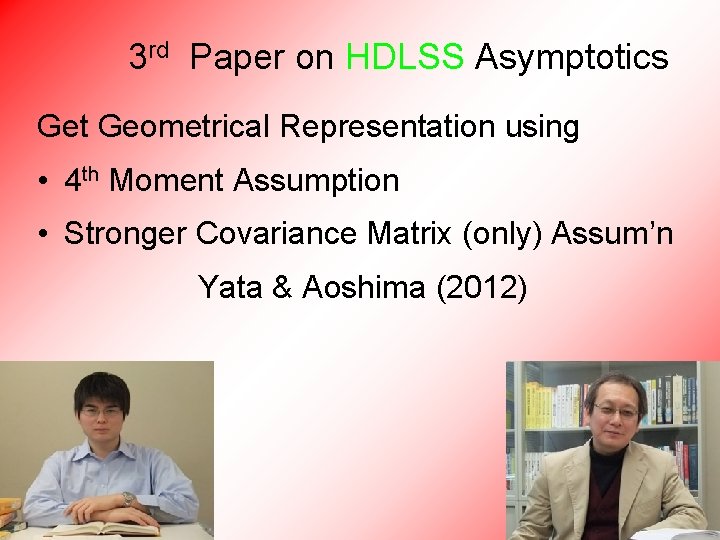
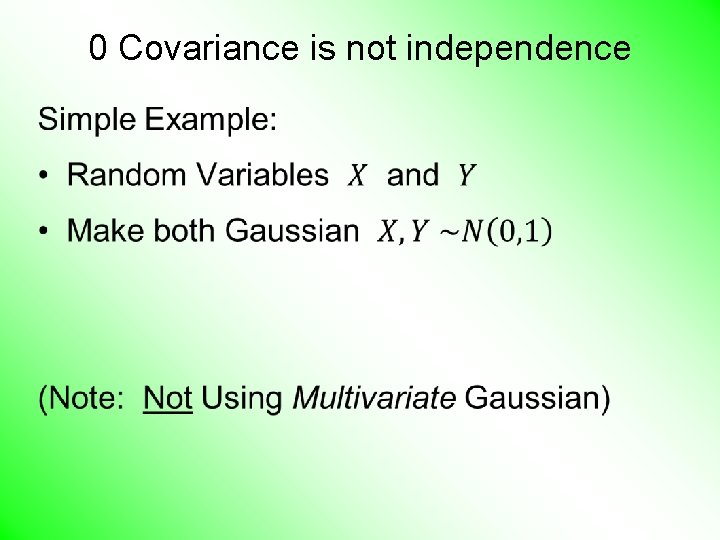
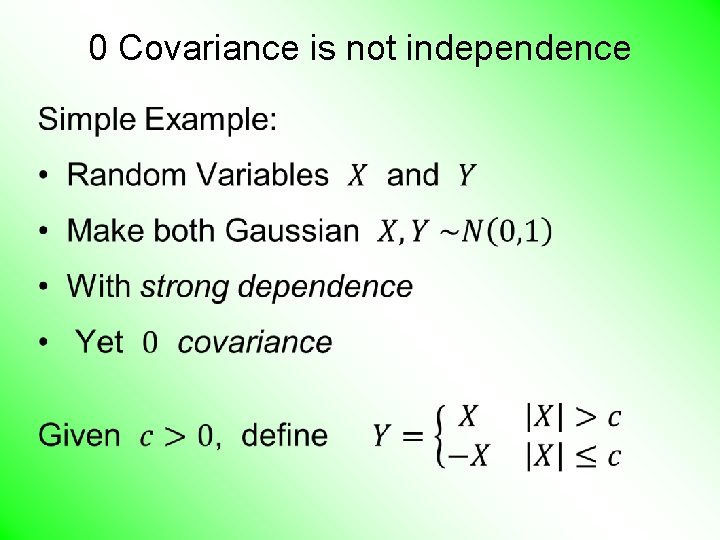
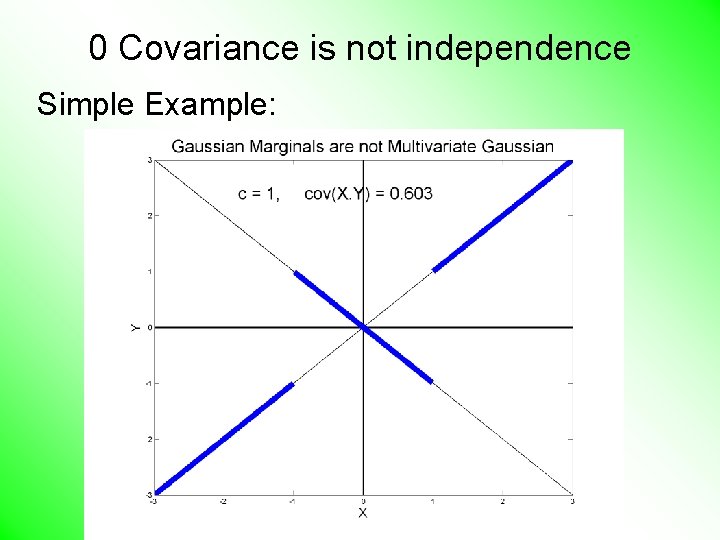
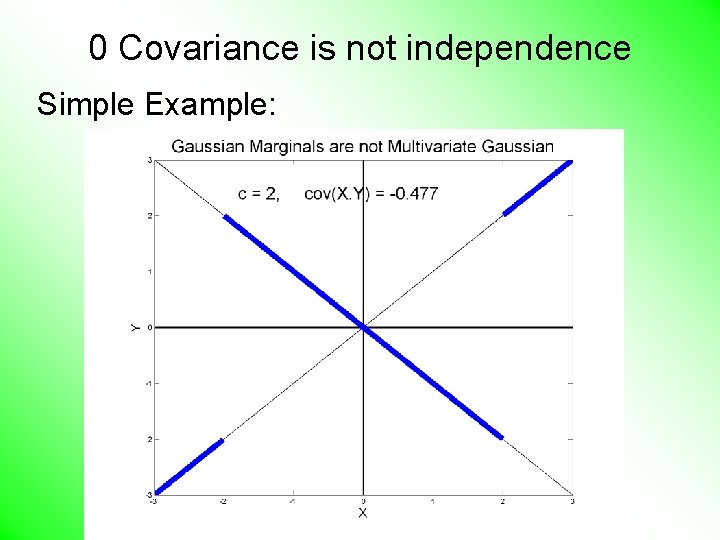
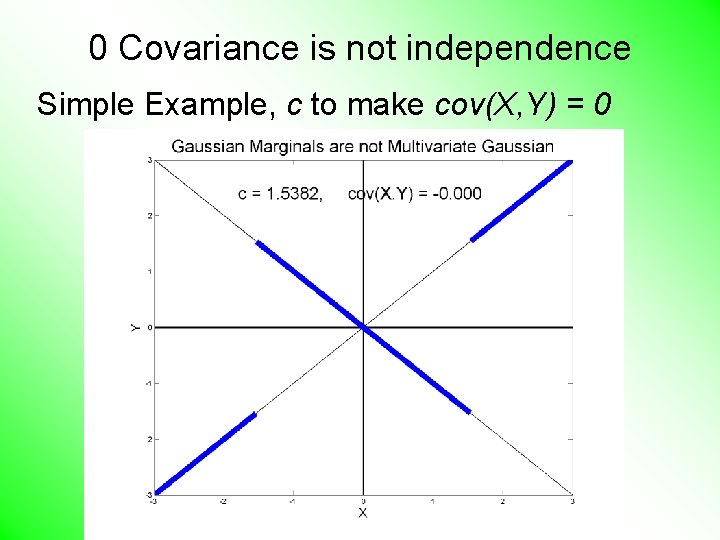
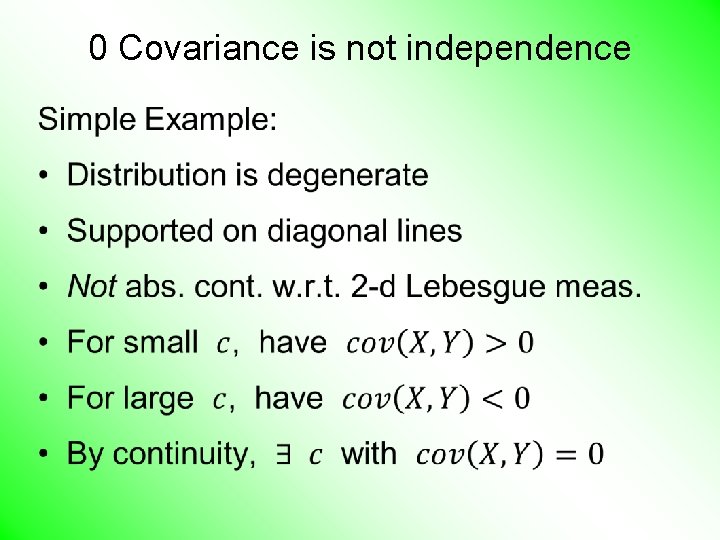
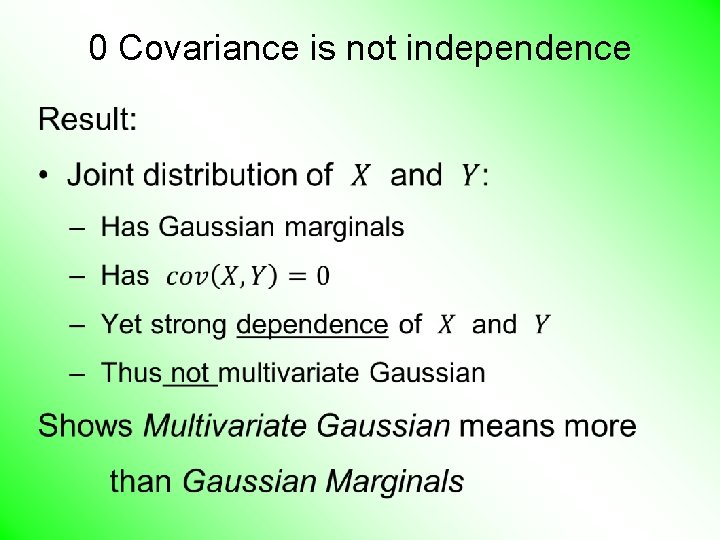
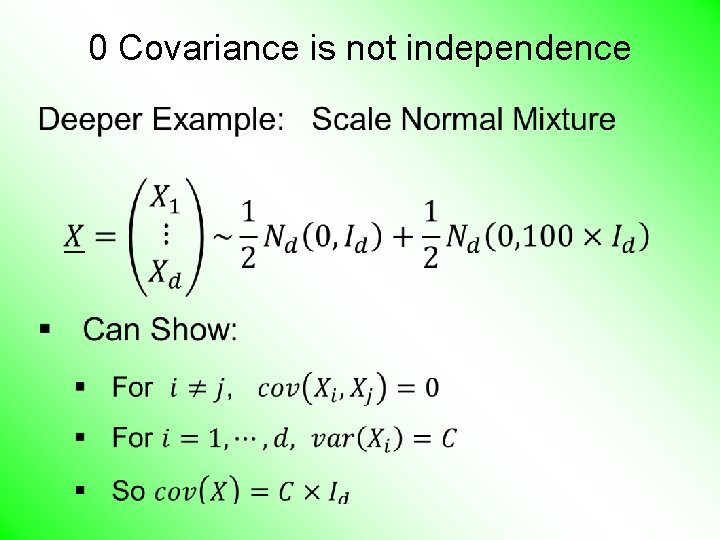
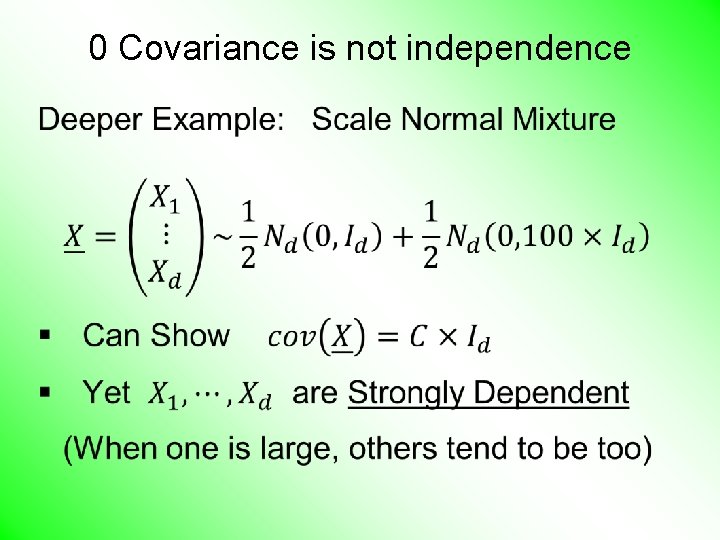
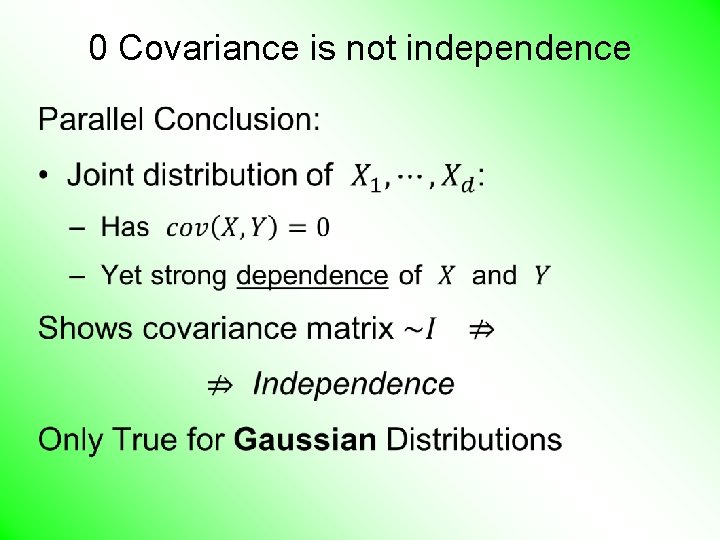
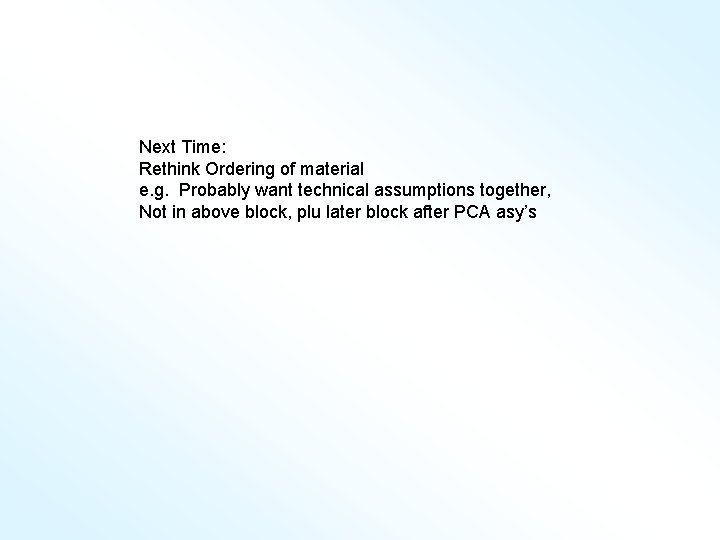
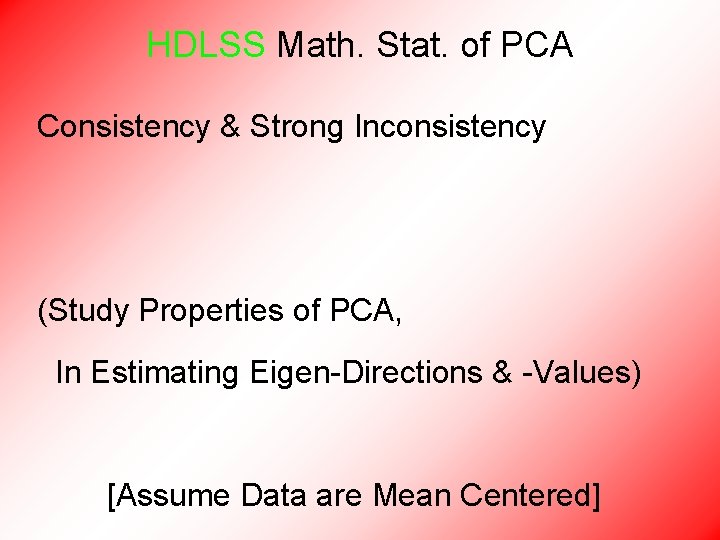
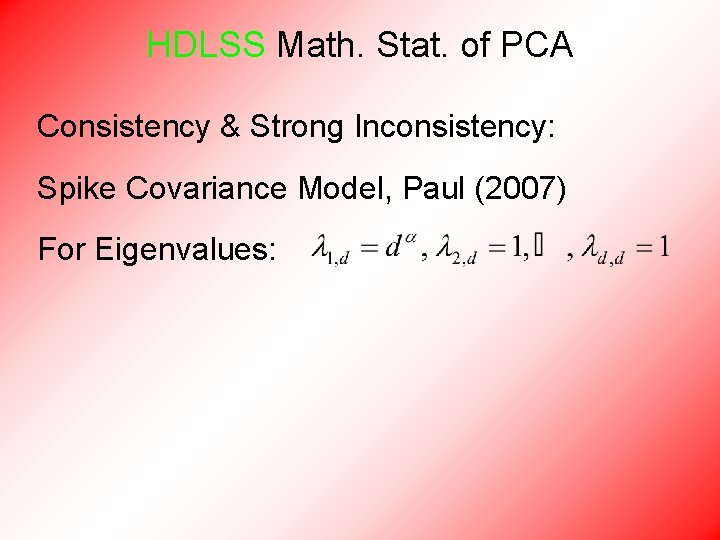
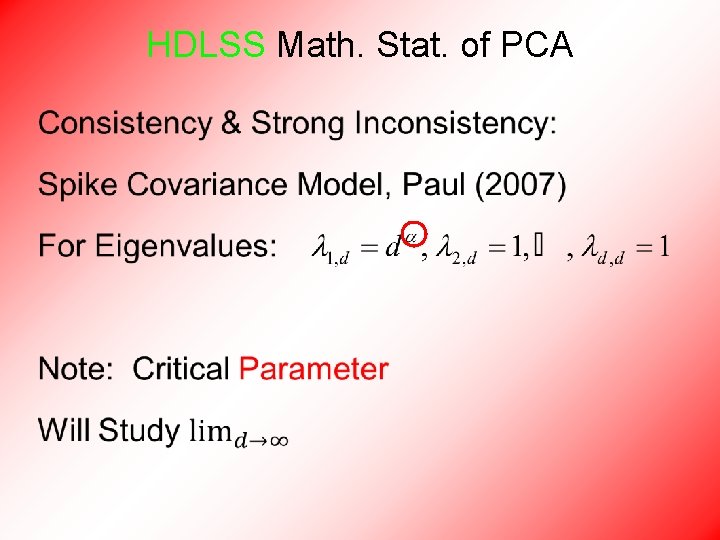
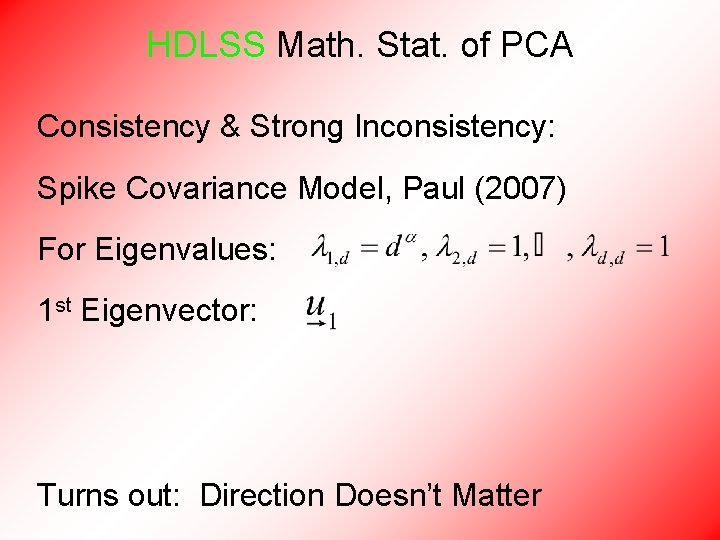
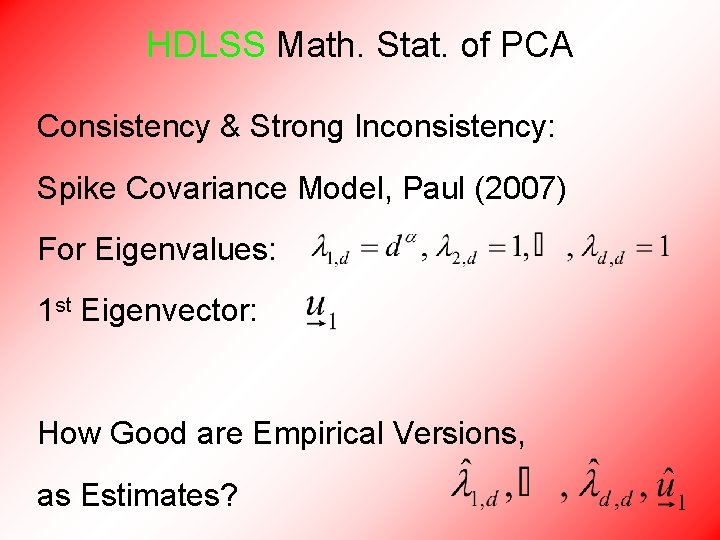
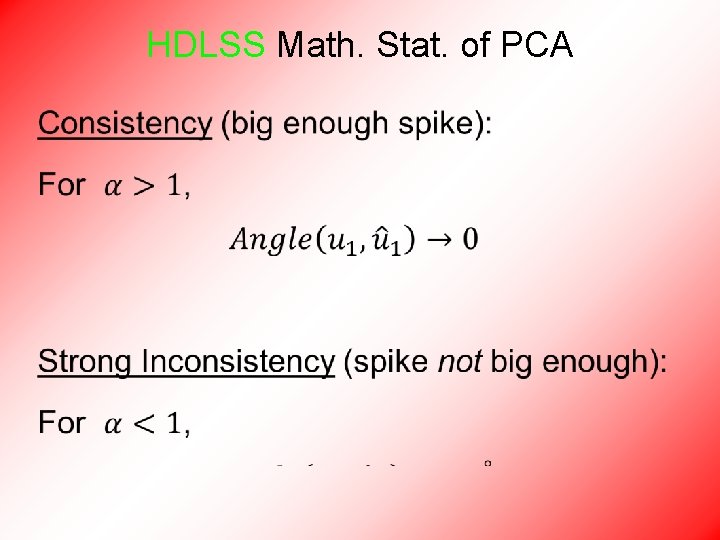
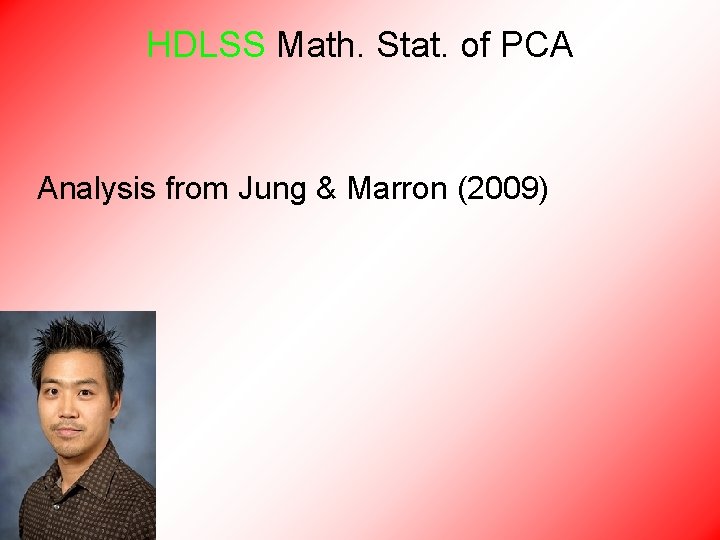
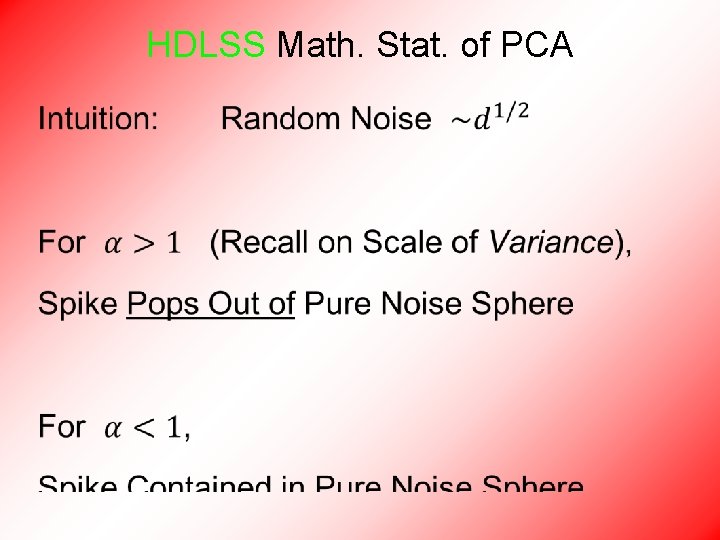
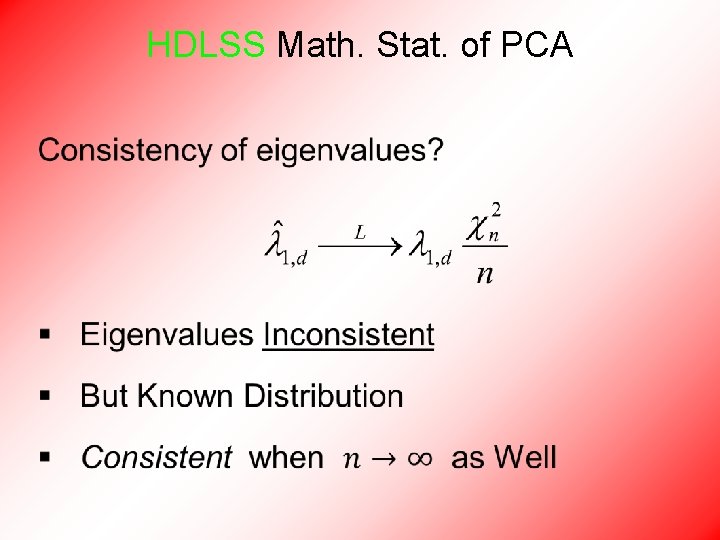
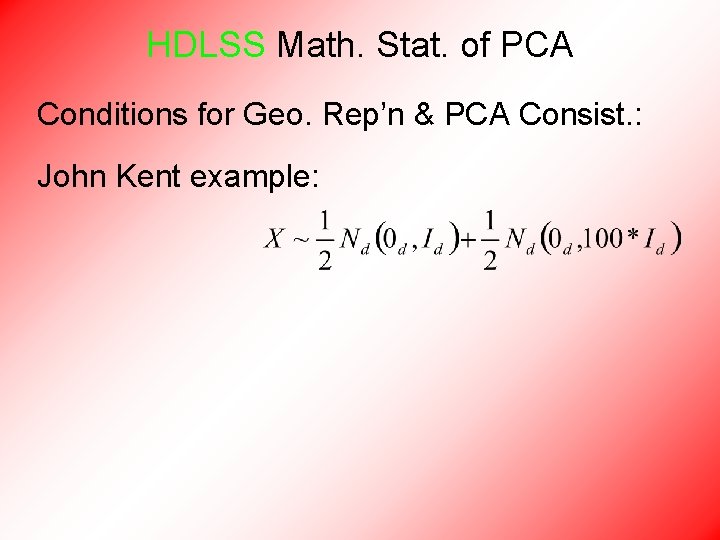
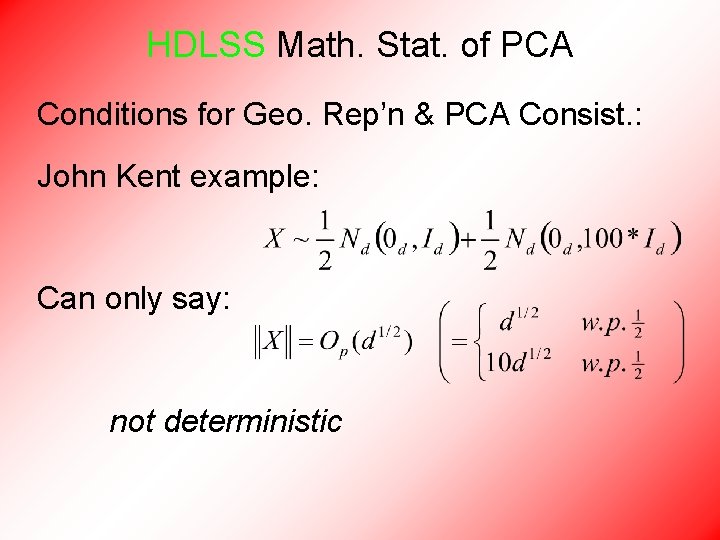
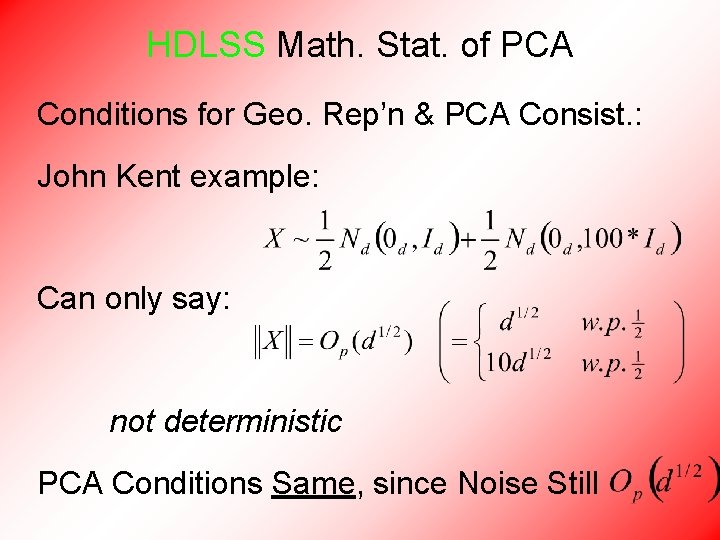
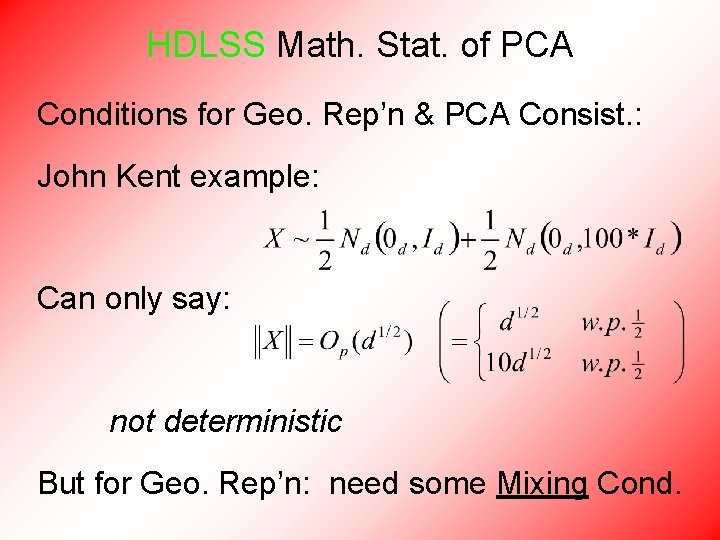
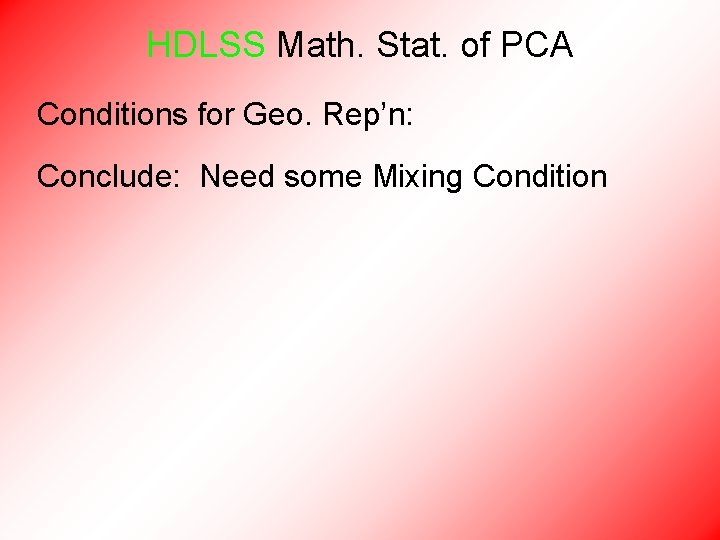
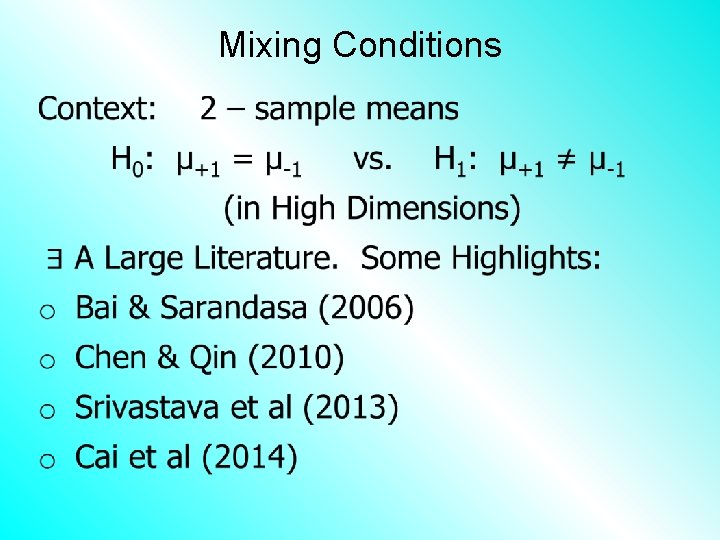
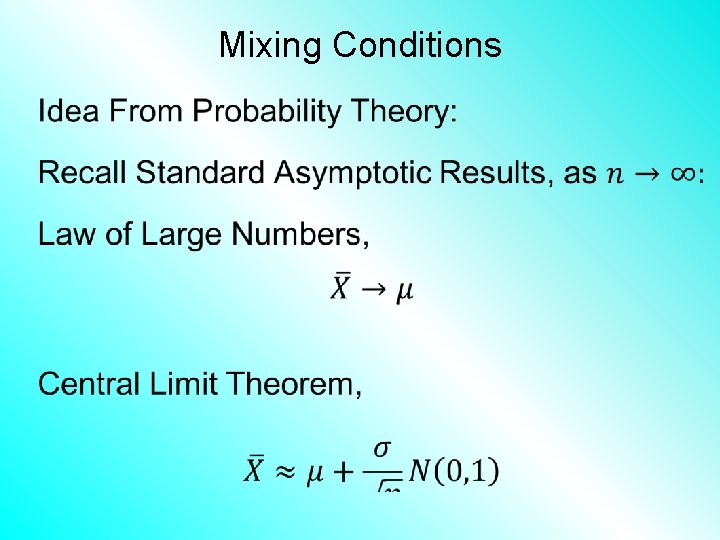
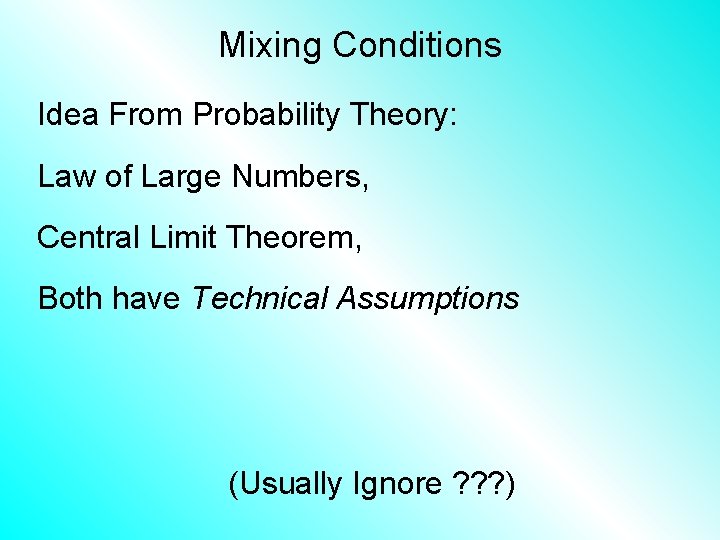
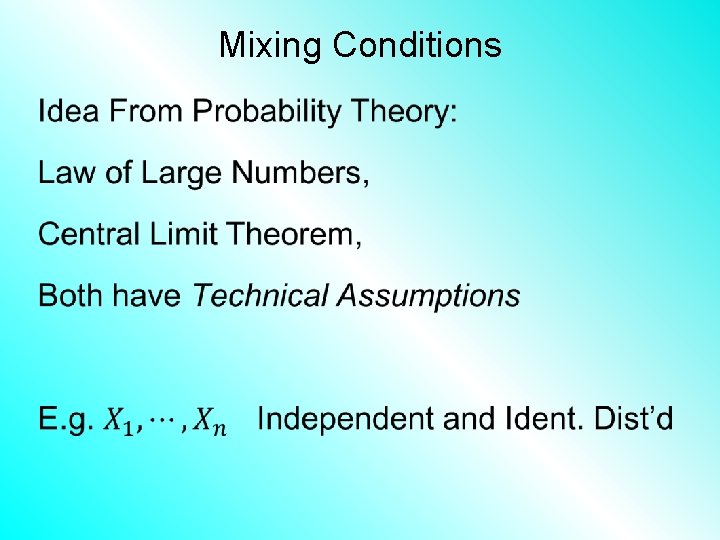
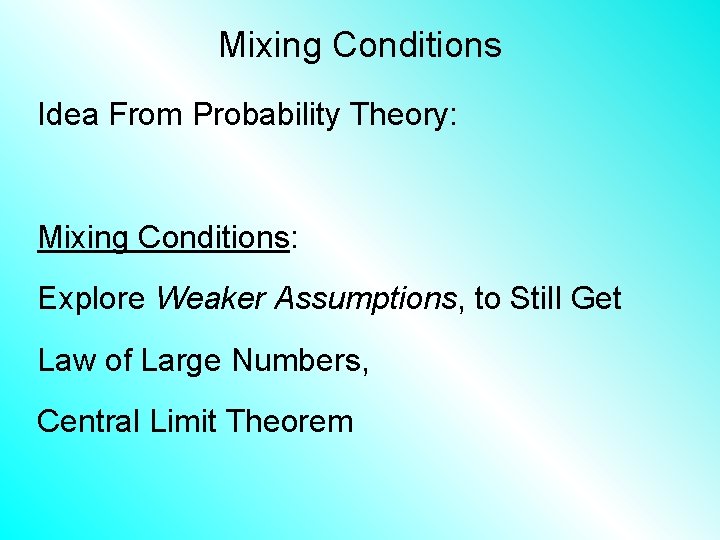
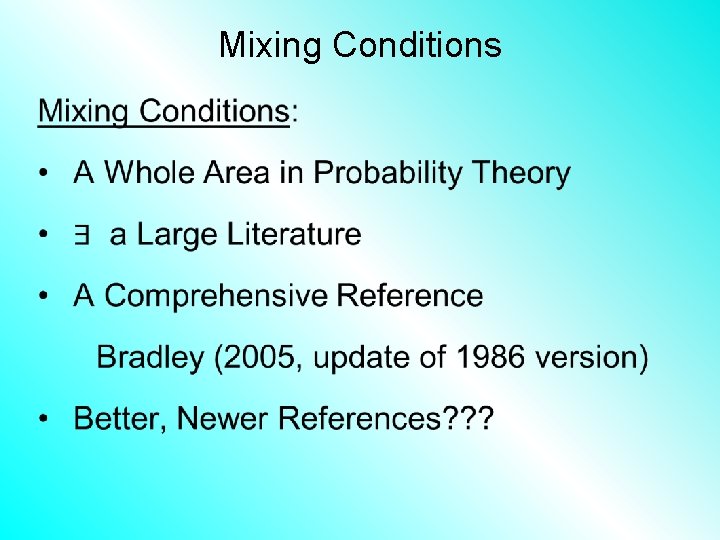
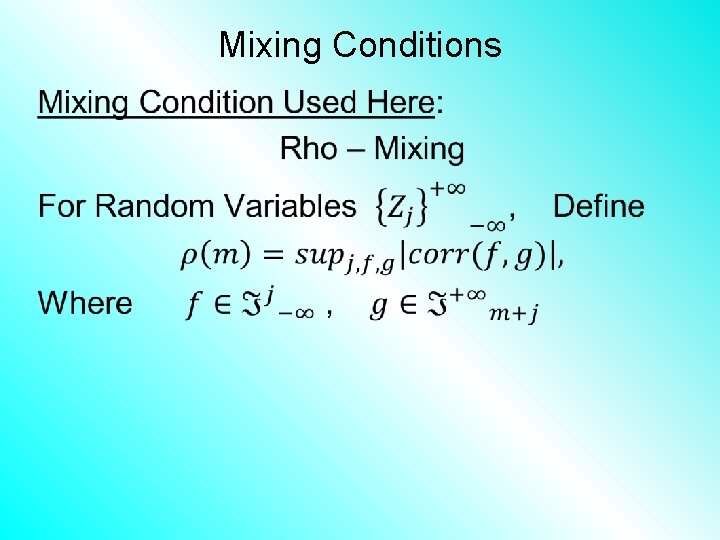
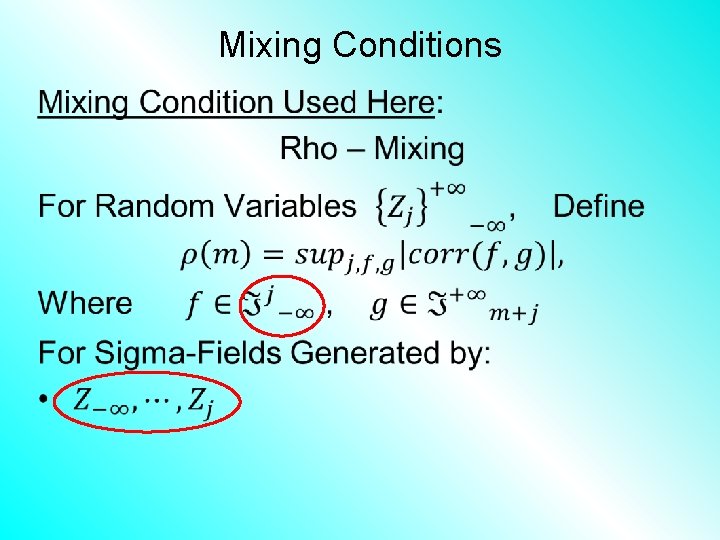
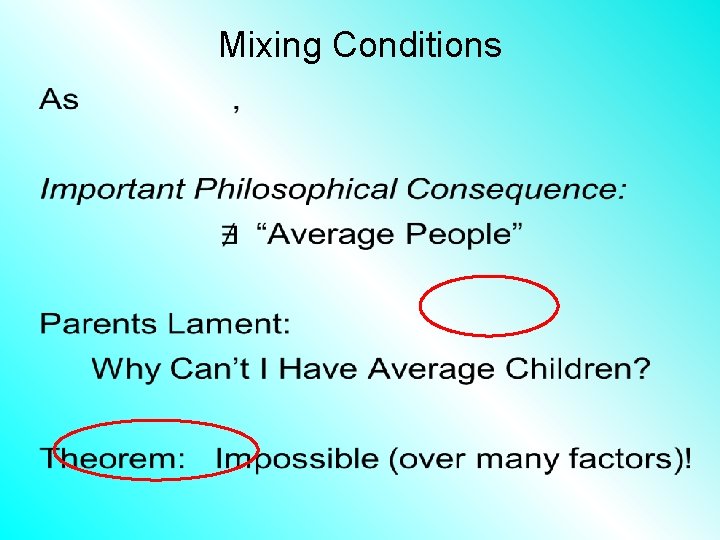
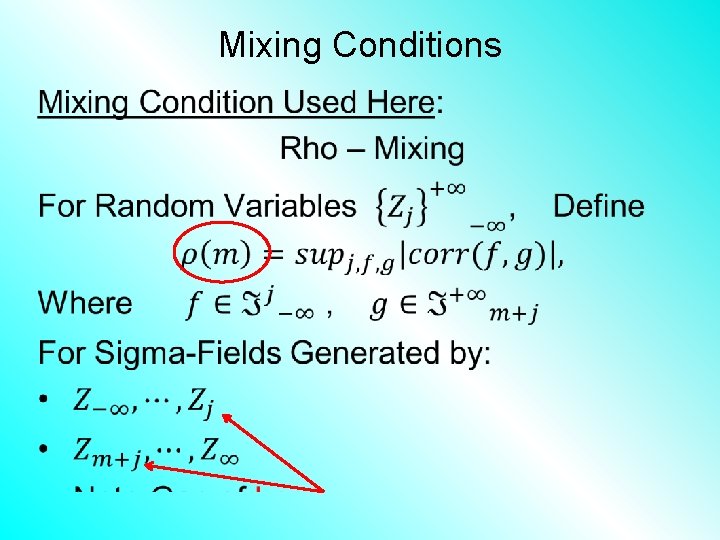
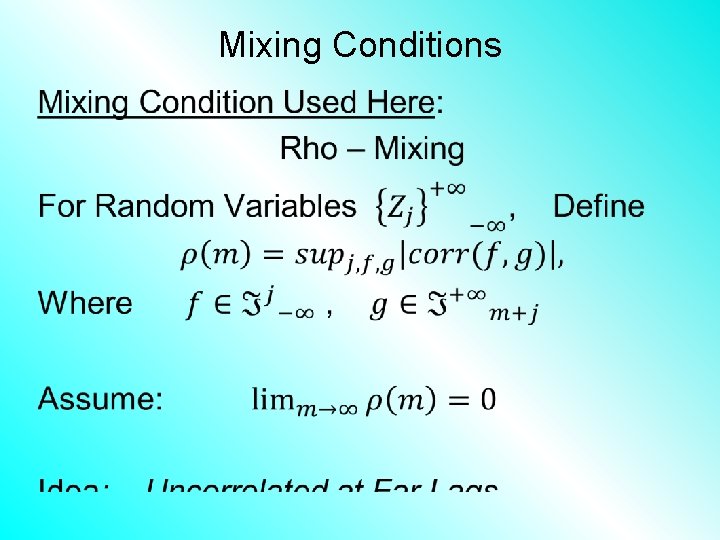
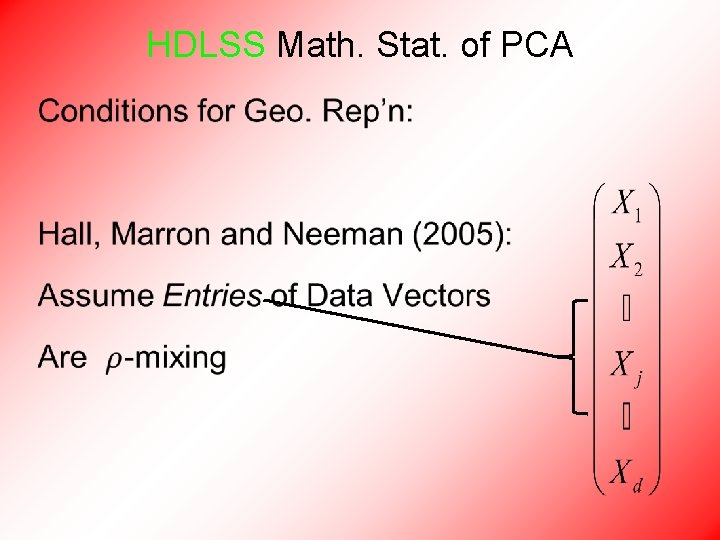
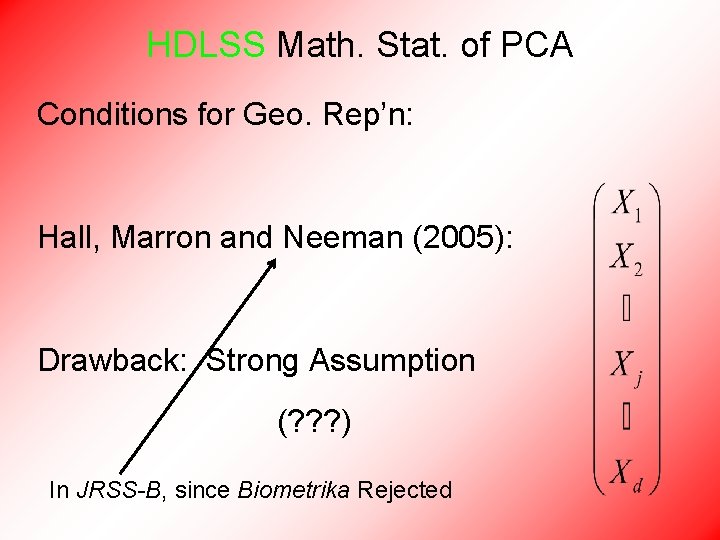
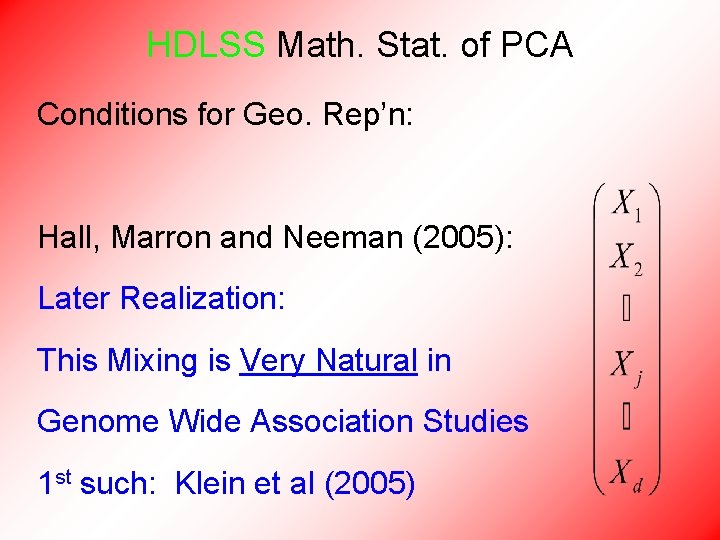
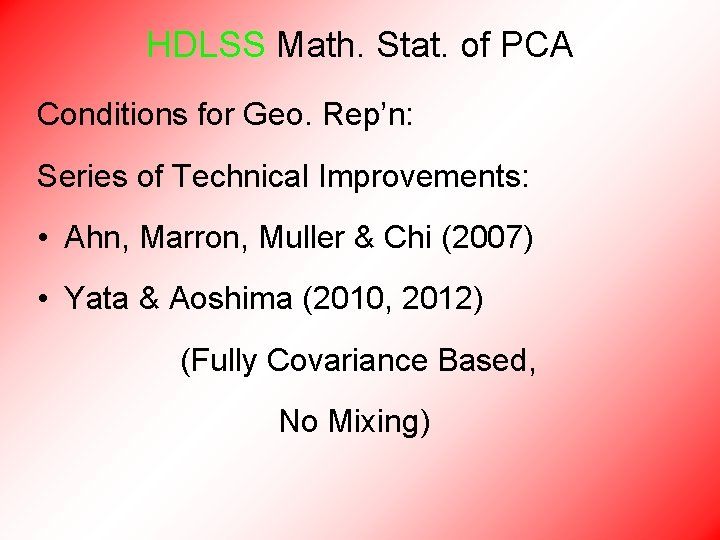
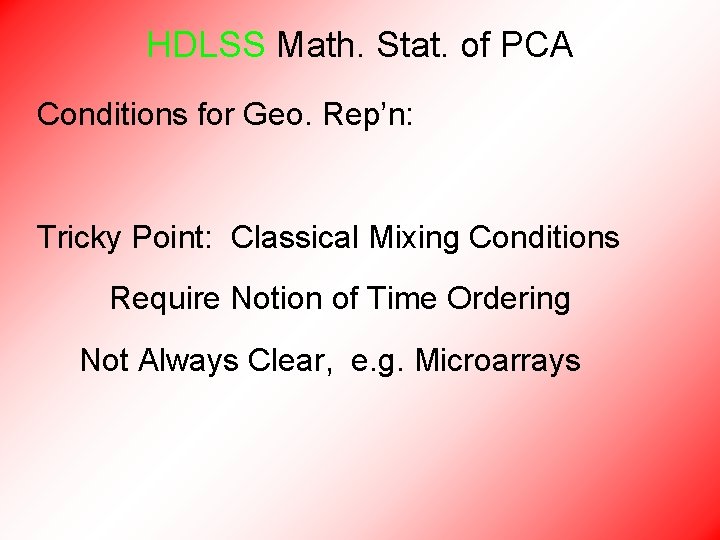
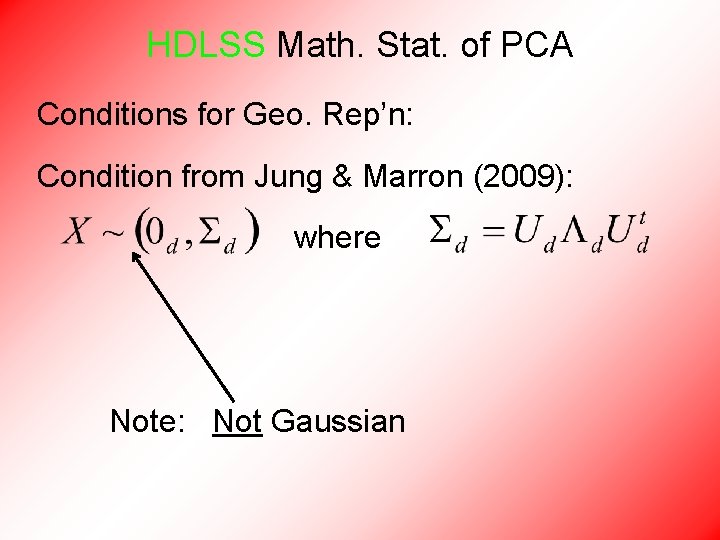
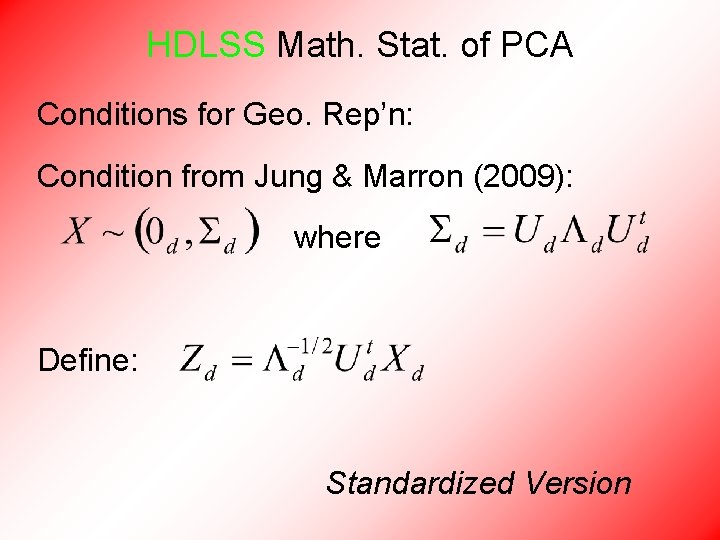
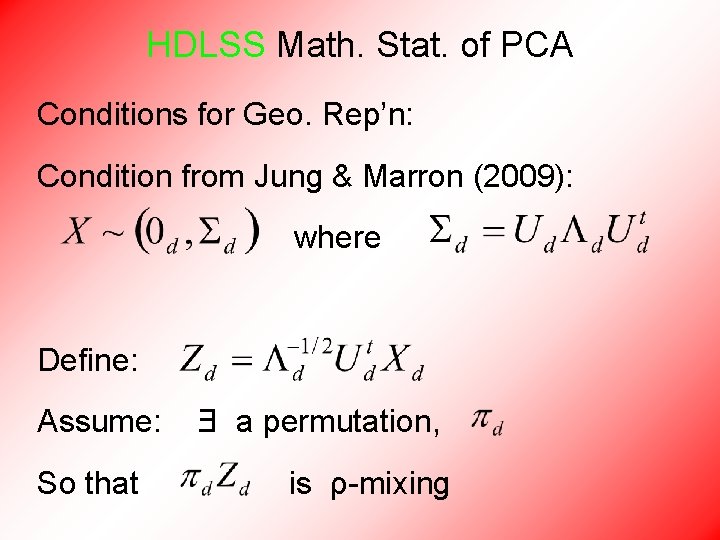
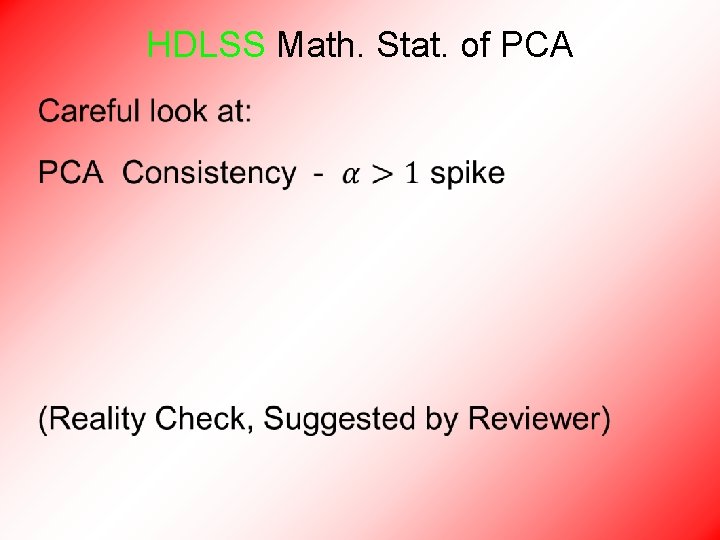
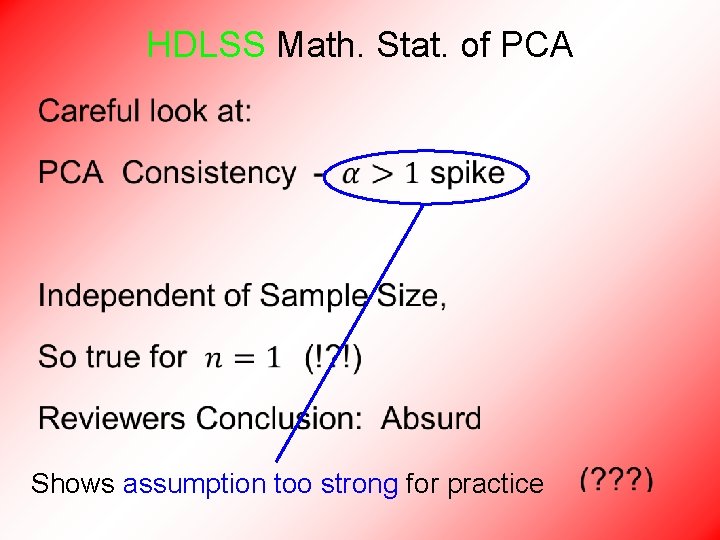
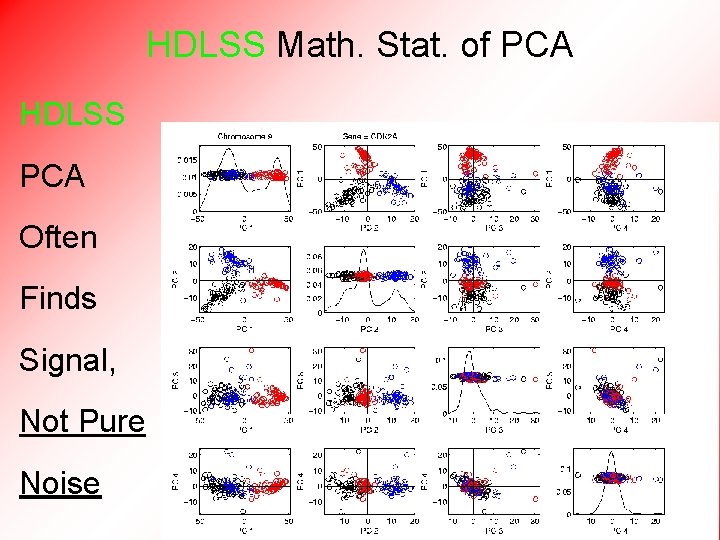
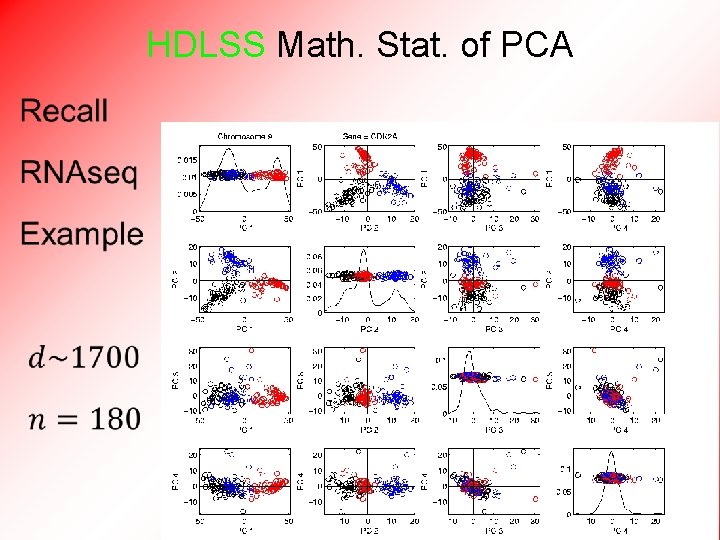
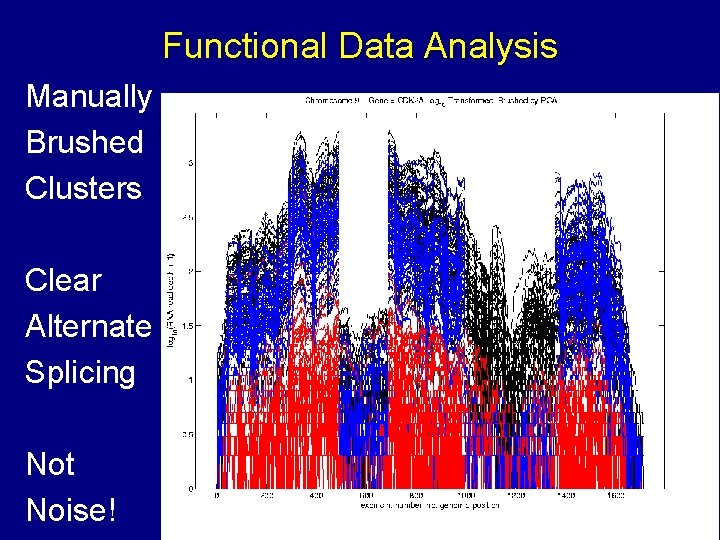
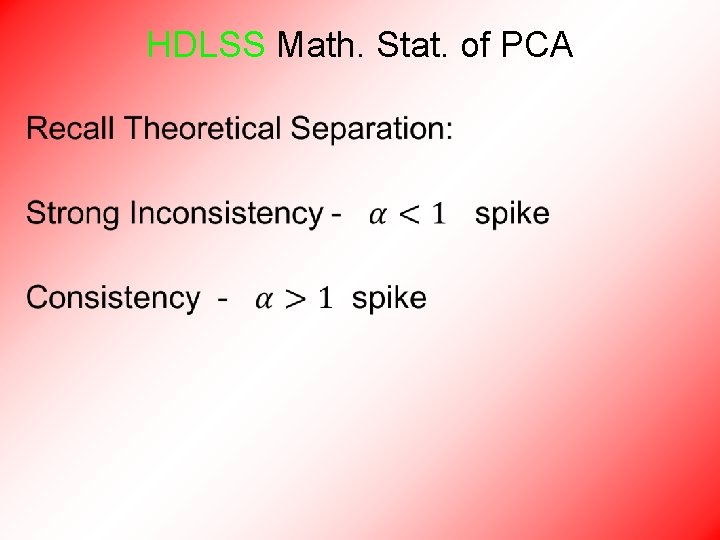
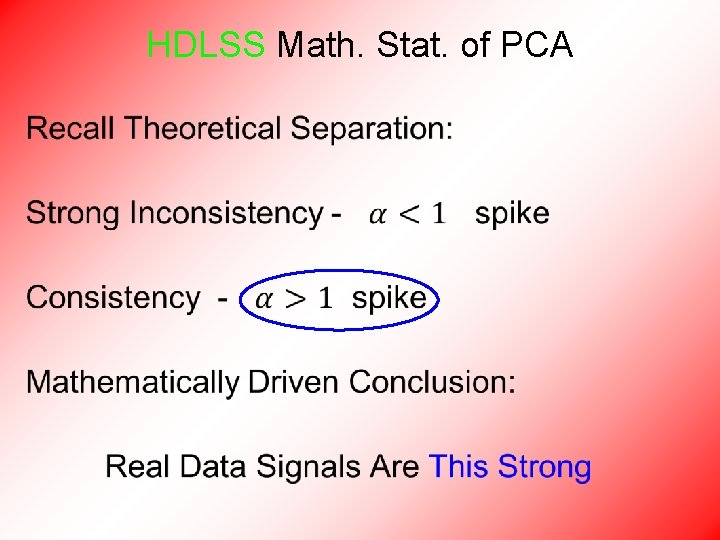
- Slides: 90
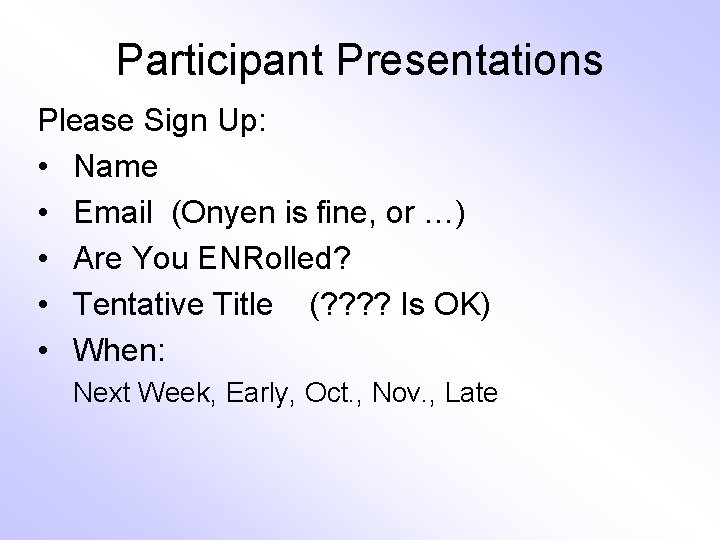
Participant Presentations Please Sign Up: • Name • Email (Onyen is fine, or …) • Are You ENRolled? • Tentative Title (? ? Is OK) • When: Next Week, Early, Oct. , Nov. , Late
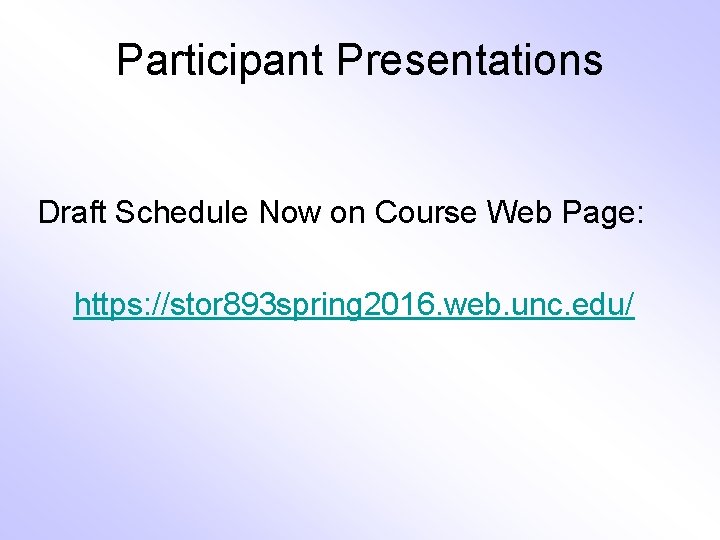
Participant Presentations Draft Schedule Now on Course Web Page: https: //stor 893 spring 2016. web. unc. edu/
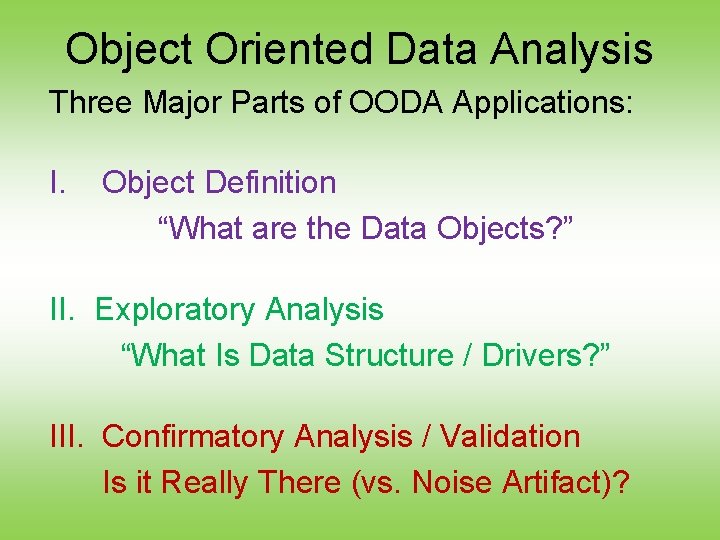
Object Oriented Data Analysis Three Major Parts of OODA Applications: I. Object Definition “What are the Data Objects? ” II. Exploratory Analysis “What Is Data Structure / Drivers? ” III. Confirmatory Analysis / Validation Is it Really There (vs. Noise Artifact)?
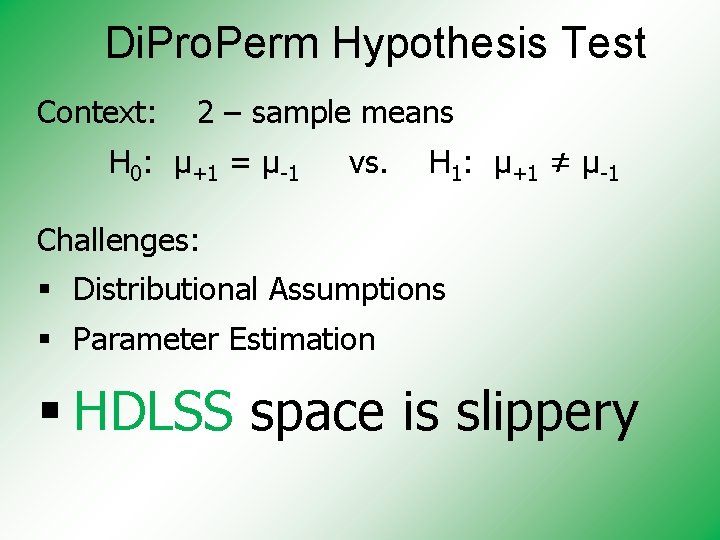
Di. Pro. Perm Hypothesis Test Context: 2 – sample means H 0: μ+1 = μ-1 vs. H 1: μ+1 ≠ μ-1 Challenges: § Distributional Assumptions § Parameter Estimation § HDLSS space is slippery
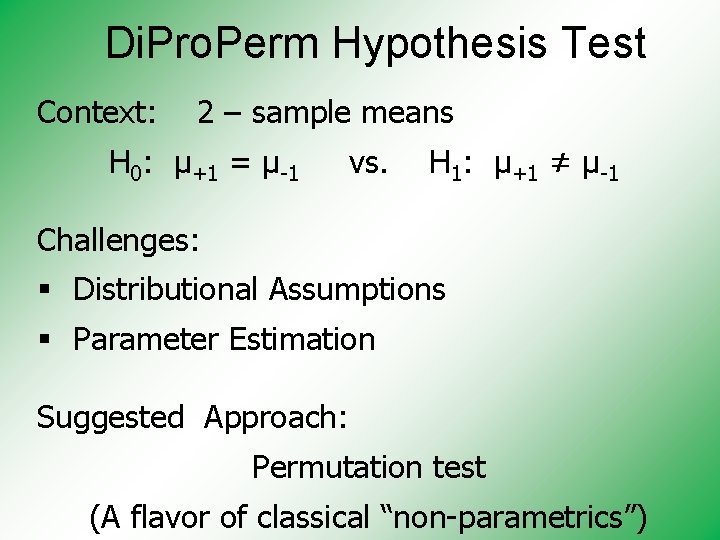
Di. Pro. Perm Hypothesis Test Context: 2 – sample means H 0: μ+1 = μ-1 vs. H 1: μ+1 ≠ μ-1 Challenges: § Distributional Assumptions § Parameter Estimation Suggested Approach: Permutation test (A flavor of classical “non-parametrics”)
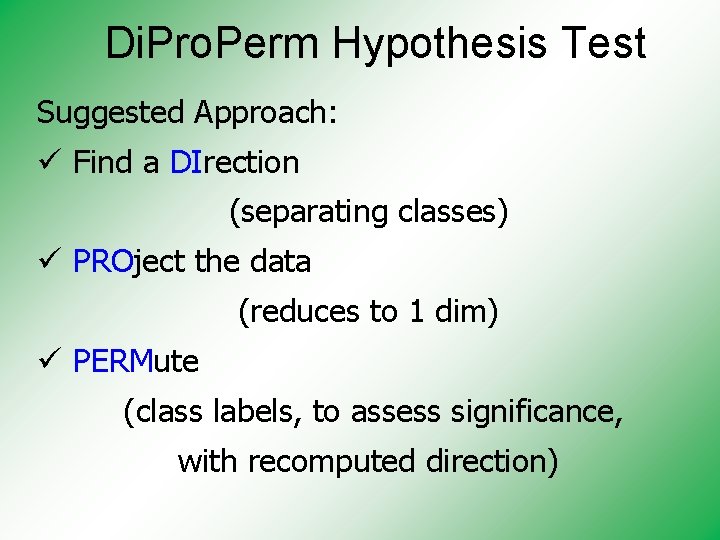
Di. Pro. Perm Hypothesis Test Suggested Approach: ü Find a DIrection (separating classes) ü PROject the data (reduces to 1 dim) ü PERMute (class labels, to assess significance, with recomputed direction)
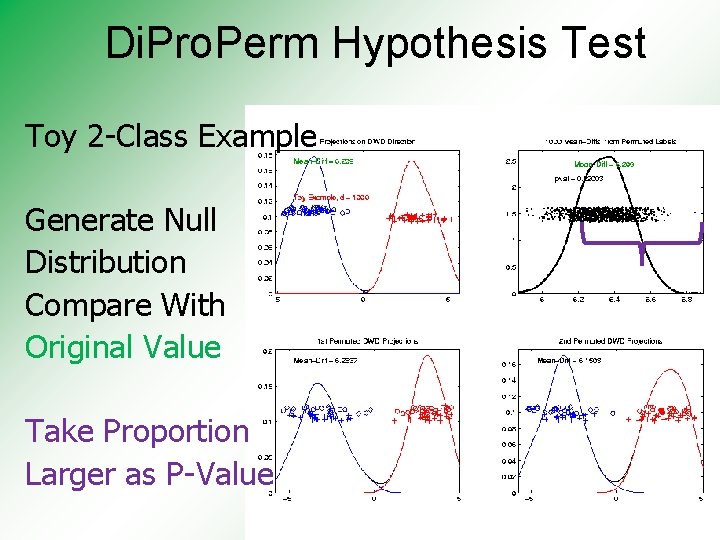
Di. Pro. Perm Hypothesis Test Toy 2 -Class Example Generate Null Distribution Compare With Original Value Take Proportion Larger as P-Value
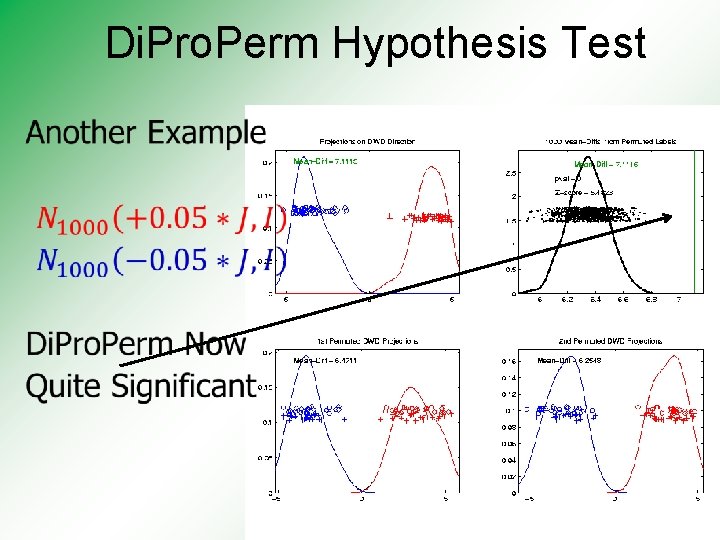
Di. Pro. Perm Hypothesis Test
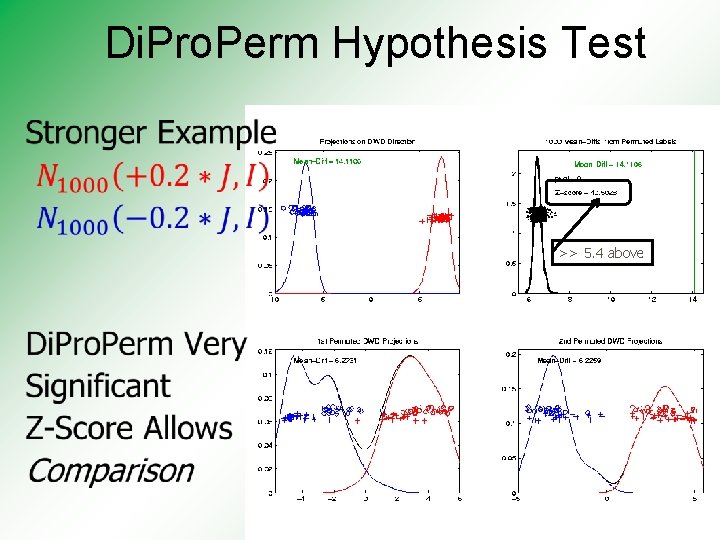
Di. Pro. Perm Hypothesis Test >> 5. 4 above
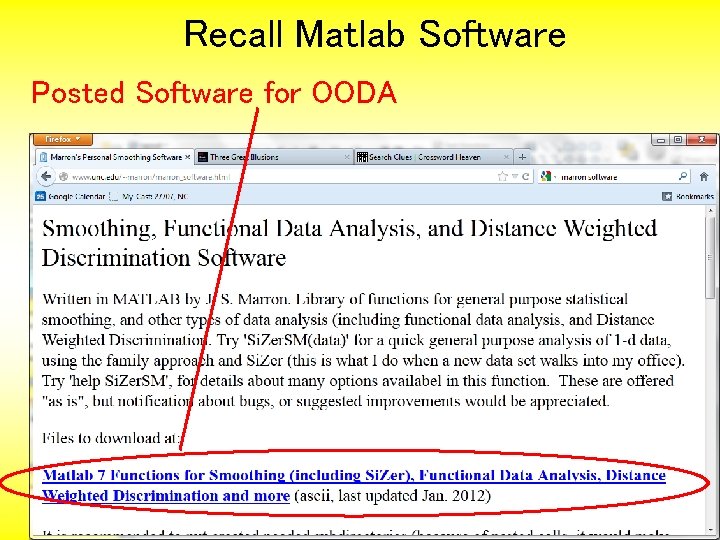
Recall Matlab Software Posted Software for OODA
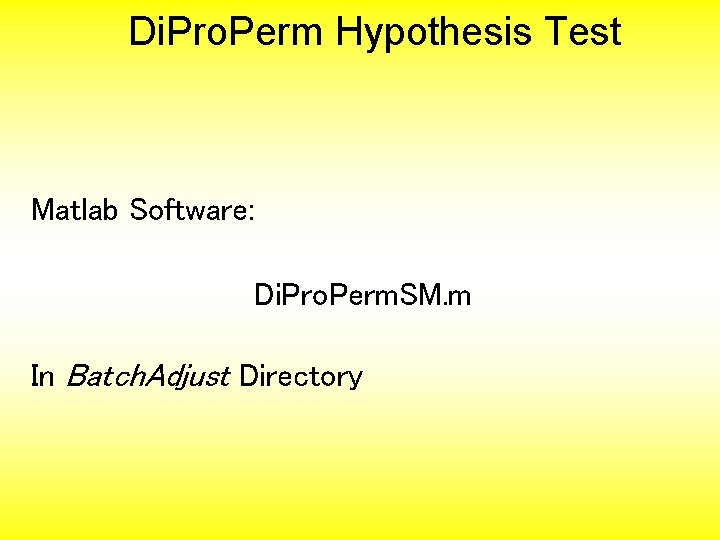
Di. Pro. Perm Hypothesis Test Matlab Software: Di. Pro. Perm. SM. m In Batch. Adjust Directory
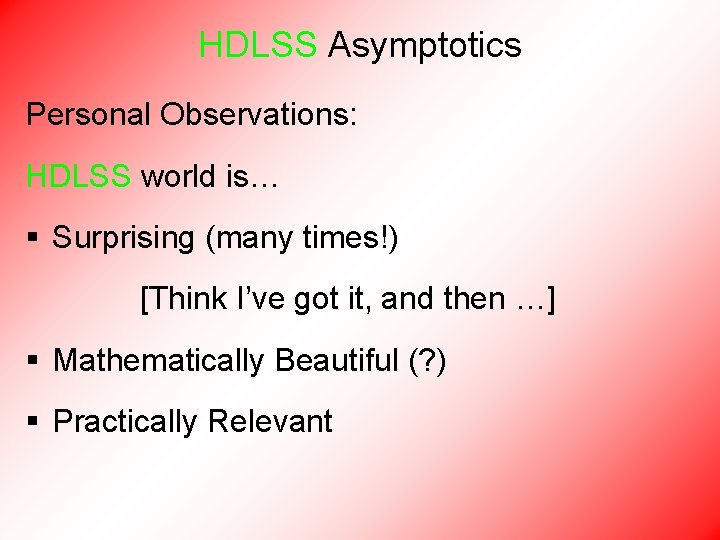
HDLSS Asymptotics Personal Observations: HDLSS world is… § Surprising (many times!) [Think I’ve got it, and then …] § Mathematically Beautiful (? ) § Practically Relevant
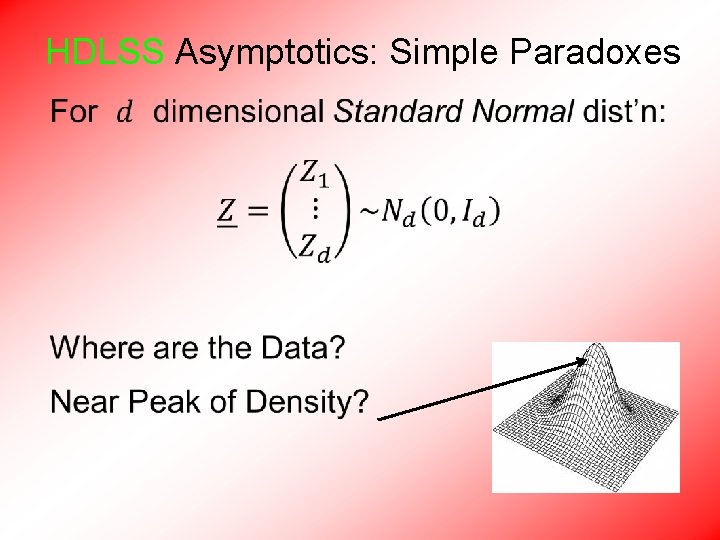
HDLSS Asymptotics: Simple Paradoxes •
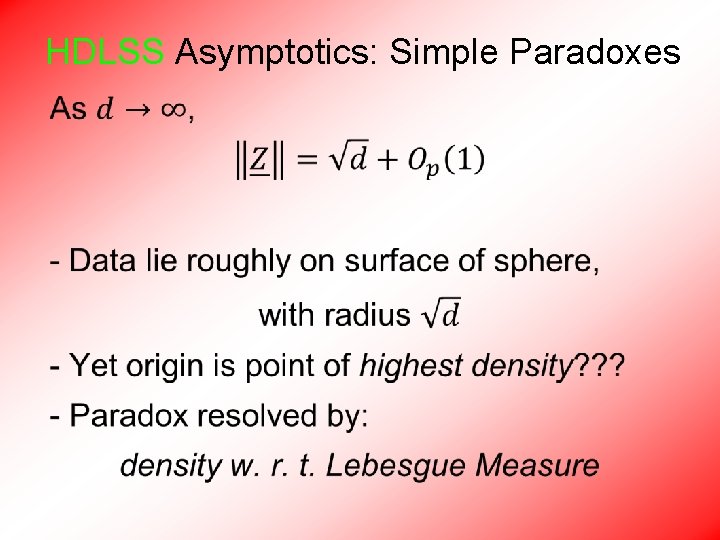
HDLSS Asymptotics: Simple Paradoxes •
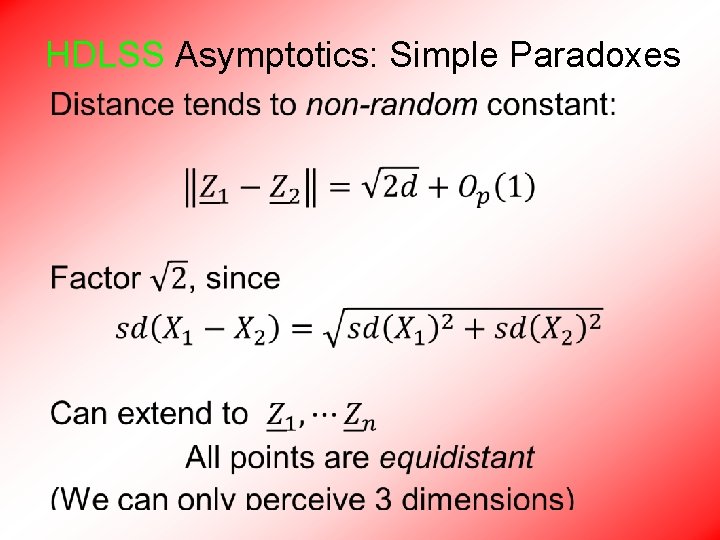
HDLSS Asymptotics: Simple Paradoxes •
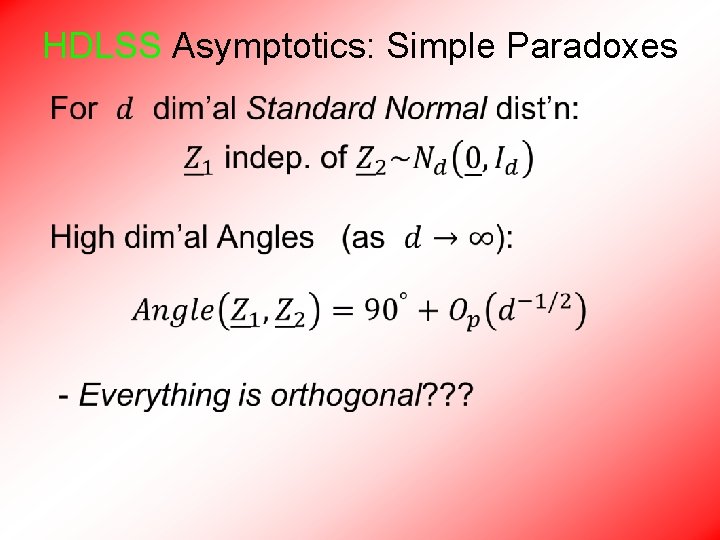
HDLSS Asymptotics: Simple Paradoxes •
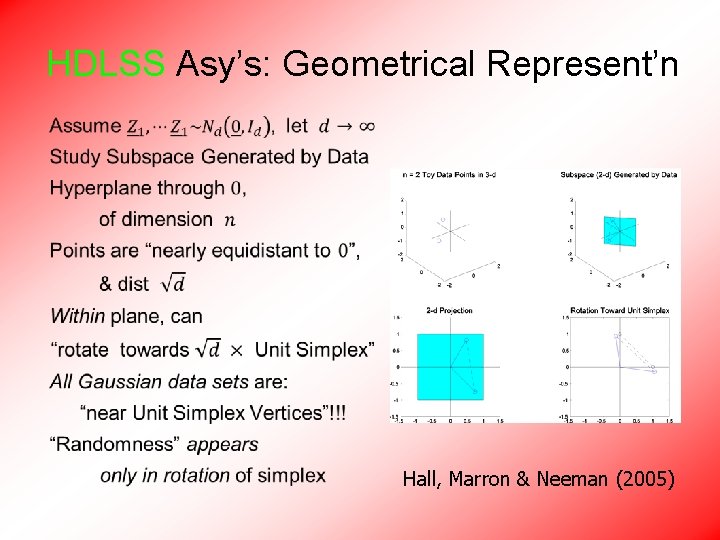
HDLSS Asy’s: Geometrical Represent’n • Hall, Marron & Neeman (2005)
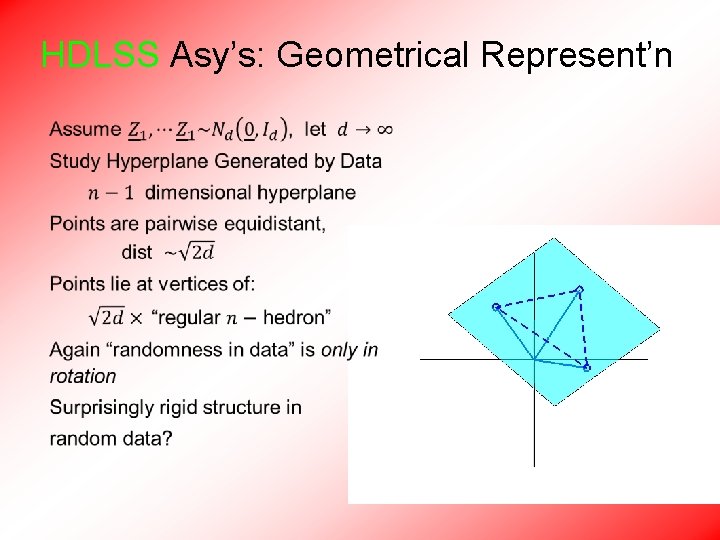
HDLSS Asy’s: Geometrical Represent’n •
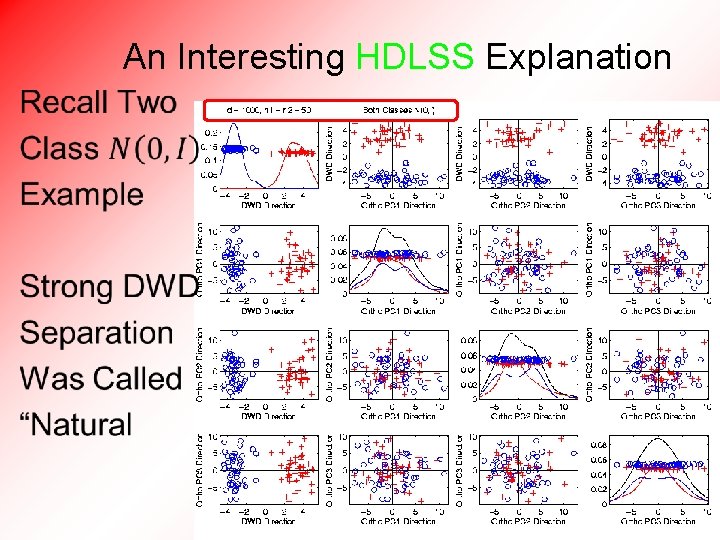
An Interesting HDLSS Explanation •
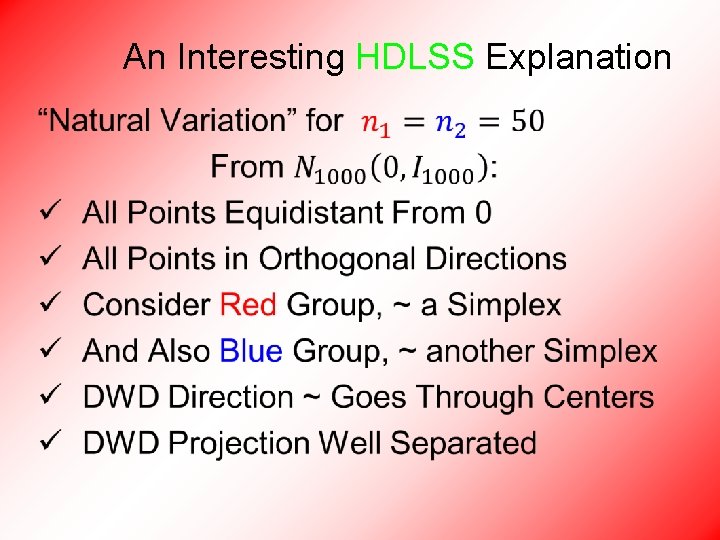
An Interesting HDLSS Explanation •
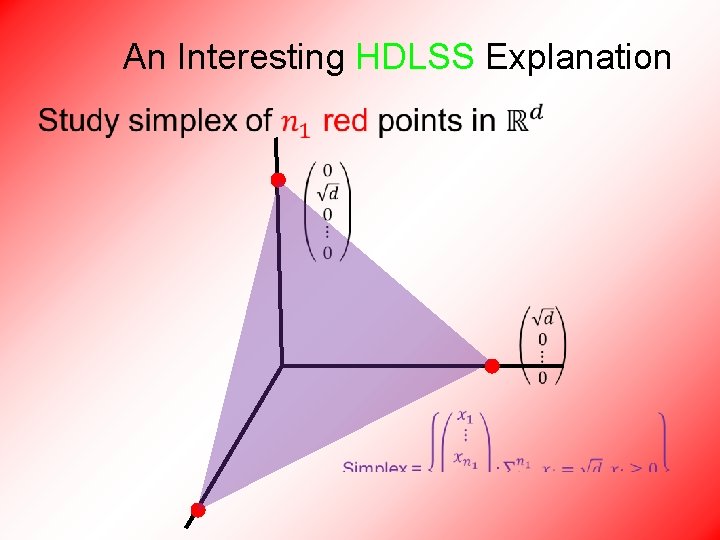
An Interesting HDLSS Explanation •
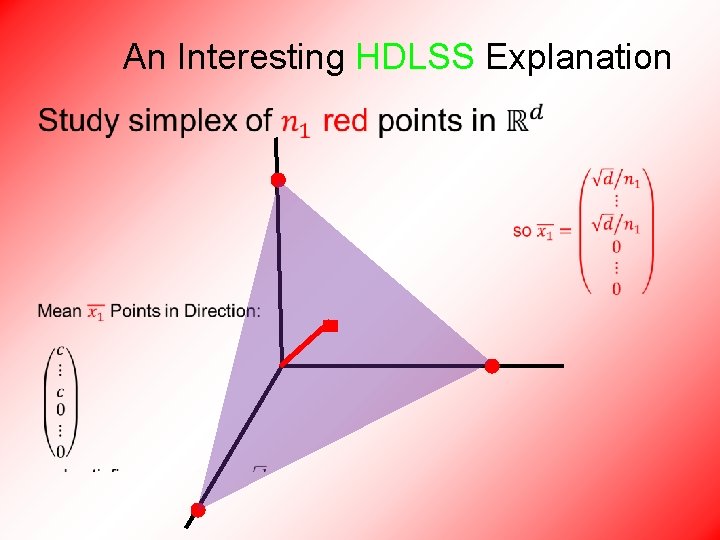
An Interesting HDLSS Explanation •
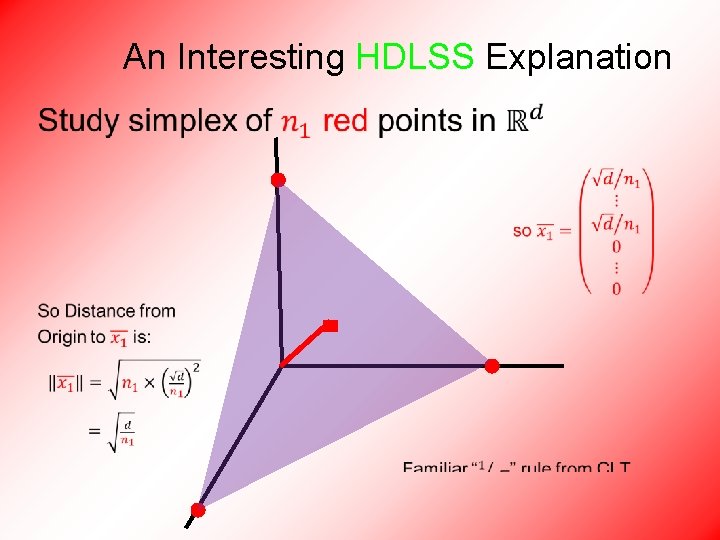
An Interesting HDLSS Explanation •
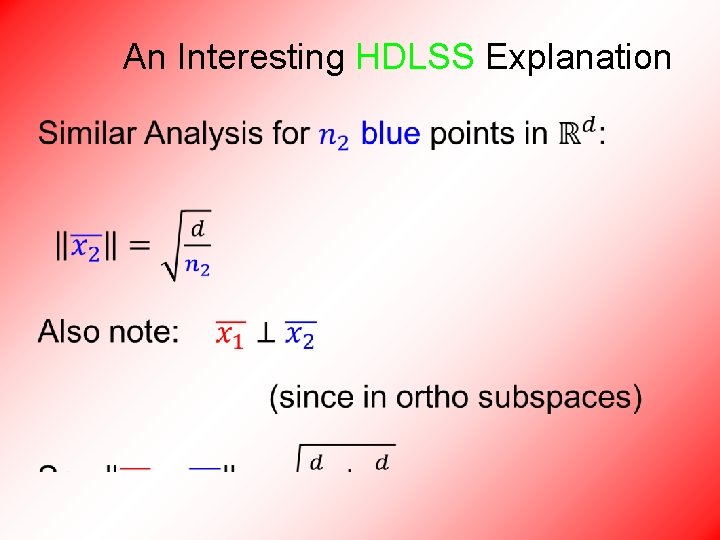
An Interesting HDLSS Explanation •
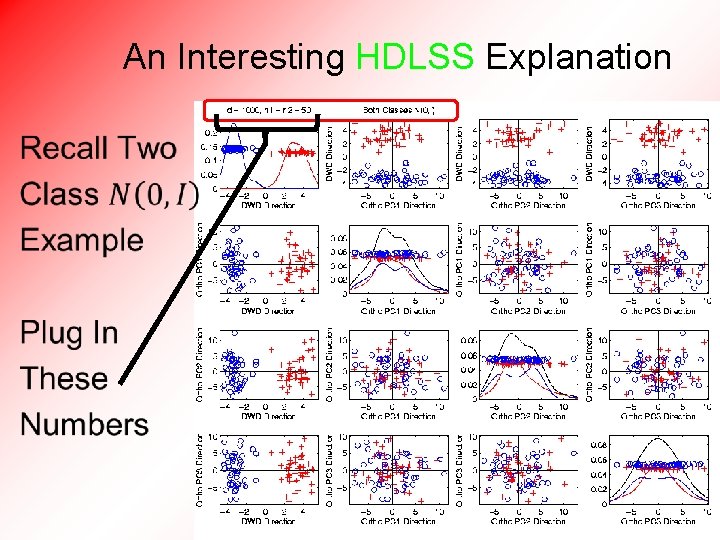
An Interesting HDLSS Explanation •
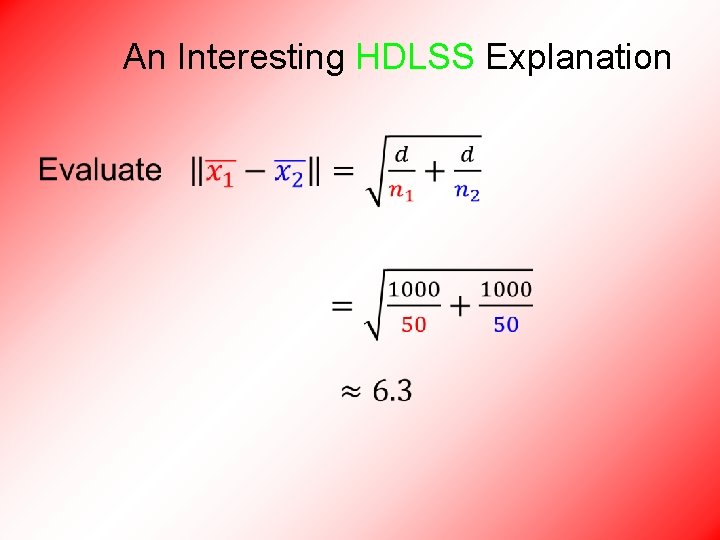
An Interesting HDLSS Explanation •
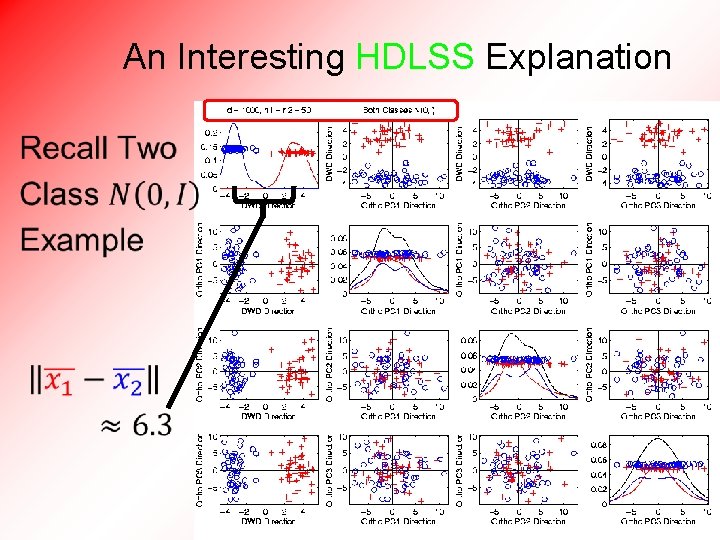
An Interesting HDLSS Explanation •
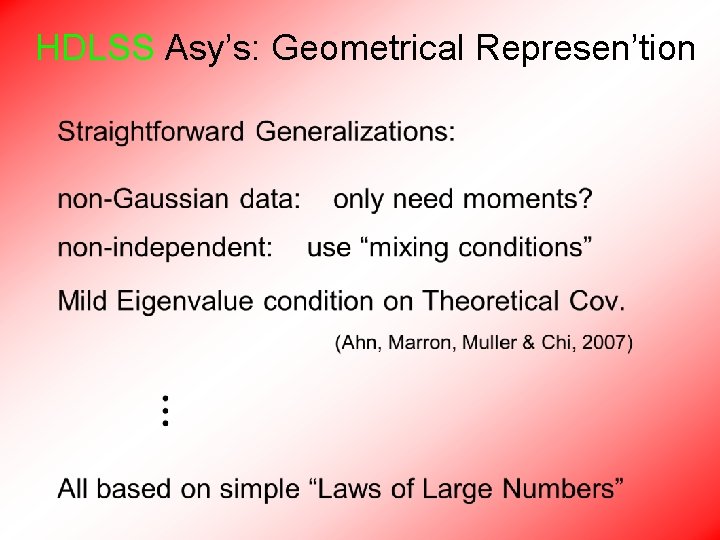
HDLSS Asy’s: Geometrical Represen’tion •
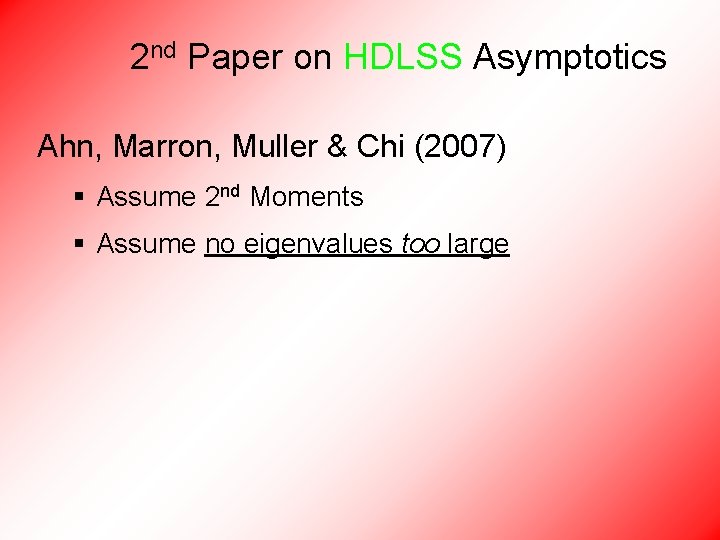
2 nd Paper on HDLSS Asymptotics Ahn, Marron, Muller & Chi (2007) § Assume 2 nd Moments § Assume no eigenvalues too large
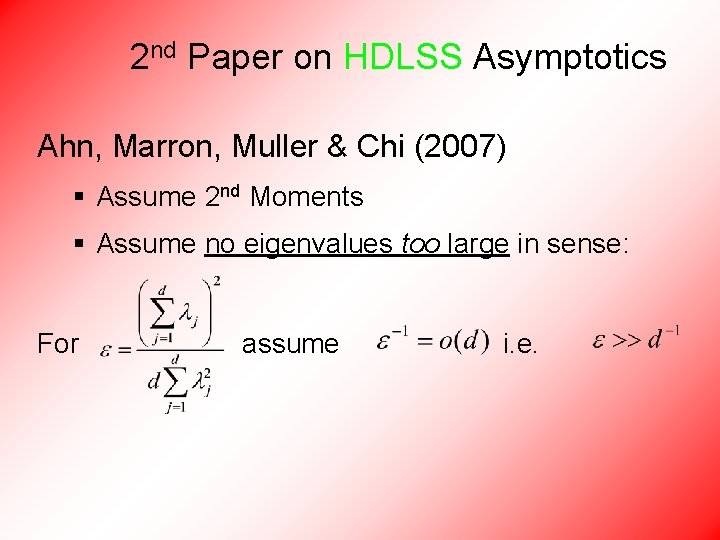
2 nd Paper on HDLSS Asymptotics Ahn, Marron, Muller & Chi (2007) § Assume 2 nd Moments § Assume no eigenvalues too large in sense: For assume i. e.
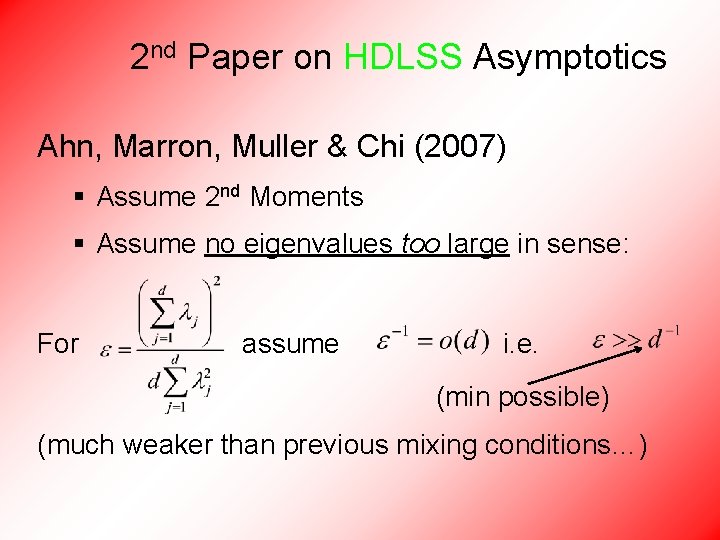
2 nd Paper on HDLSS Asymptotics Ahn, Marron, Muller & Chi (2007) § Assume 2 nd Moments § Assume no eigenvalues too large in sense: For assume i. e. (min possible) (much weaker than previous mixing conditions…)
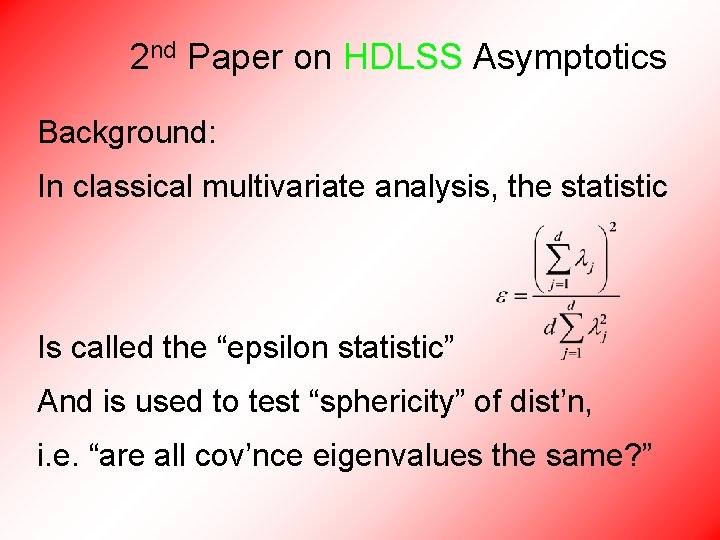
2 nd Paper on HDLSS Asymptotics Background: In classical multivariate analysis, the statistic Is called the “epsilon statistic” And is used to test “sphericity” of dist’n, i. e. “are all cov’nce eigenvalues the same? ”
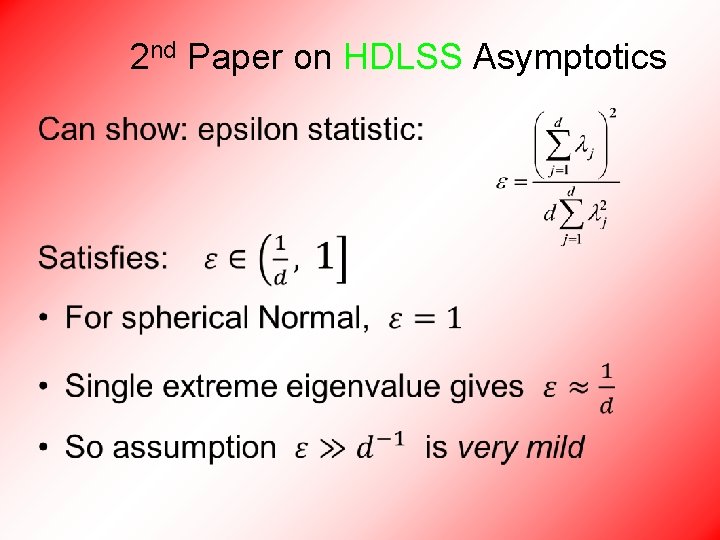
2 nd Paper on HDLSS Asymptotics •
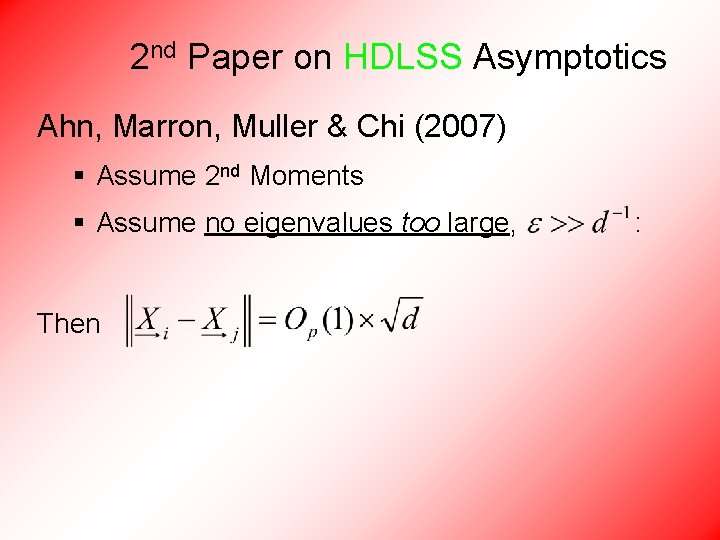
2 nd Paper on HDLSS Asymptotics Ahn, Marron, Muller & Chi (2007) § Assume 2 nd Moments § Assume no eigenvalues too large, Then :
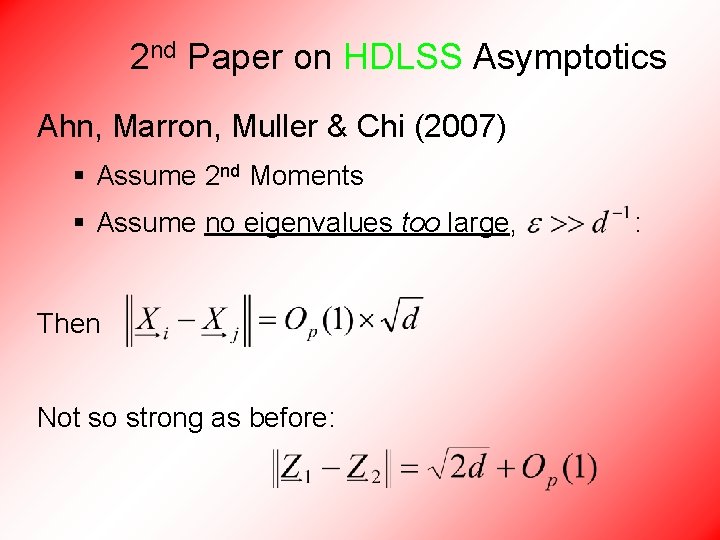
2 nd Paper on HDLSS Asymptotics Ahn, Marron, Muller & Chi (2007) § Assume 2 nd Moments § Assume no eigenvalues too large, Then Not so strong as before: :
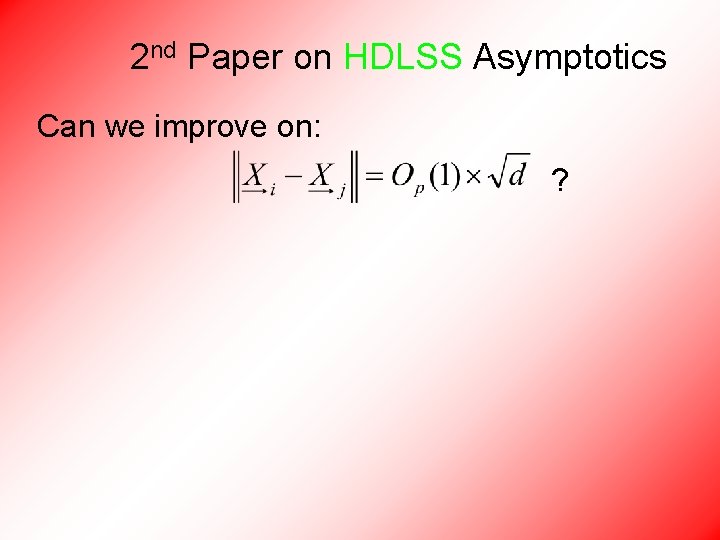
2 nd Paper on HDLSS Asymptotics Can we improve on: ?
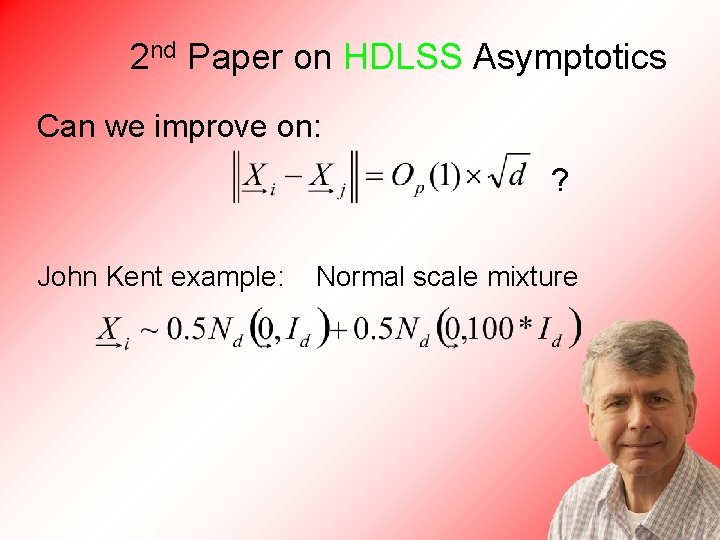
2 nd Paper on HDLSS Asymptotics Can we improve on: ? John Kent example: Normal scale mixture
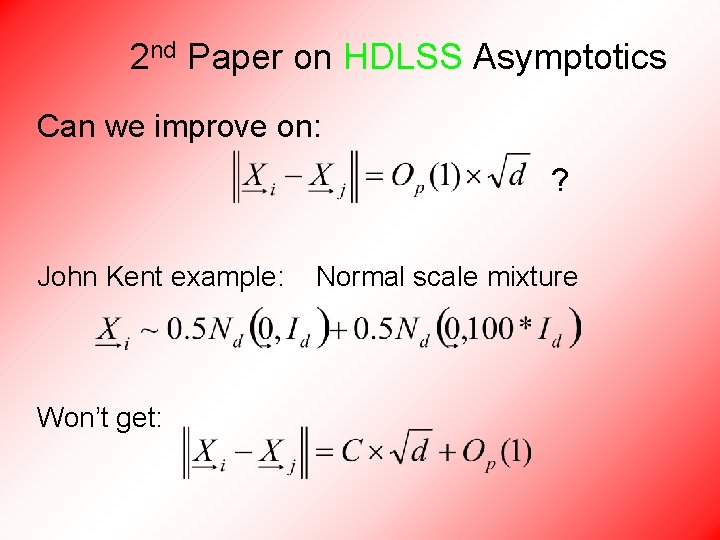
2 nd Paper on HDLSS Asymptotics Can we improve on: ? John Kent example: Won’t get: Normal scale mixture
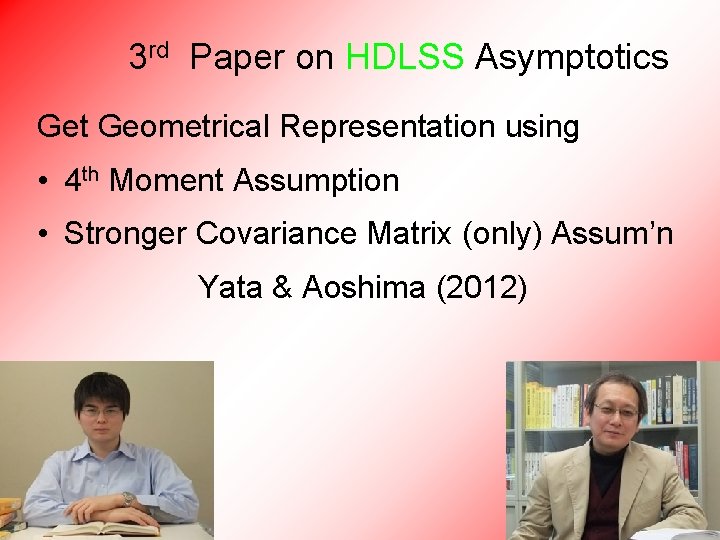
3 rd Paper on HDLSS Asymptotics Get Geometrical Representation using • 4 th Moment Assumption • Stronger Covariance Matrix (only) Assum’n Yata & Aoshima (2012)
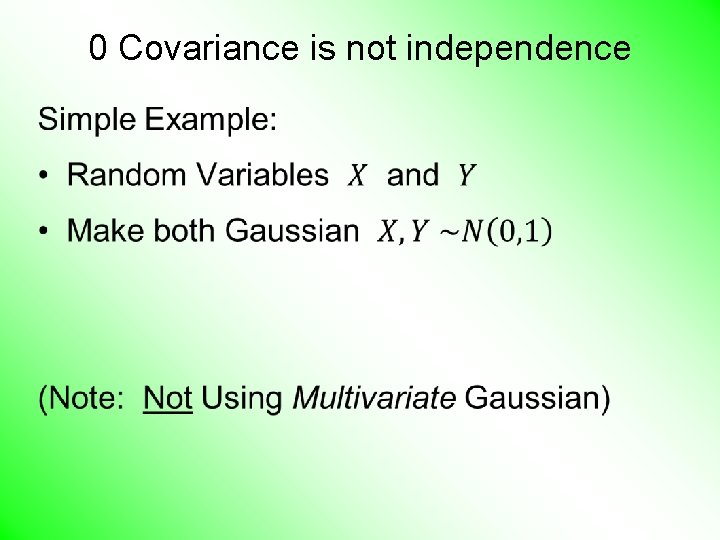
0 Covariance is not independence •
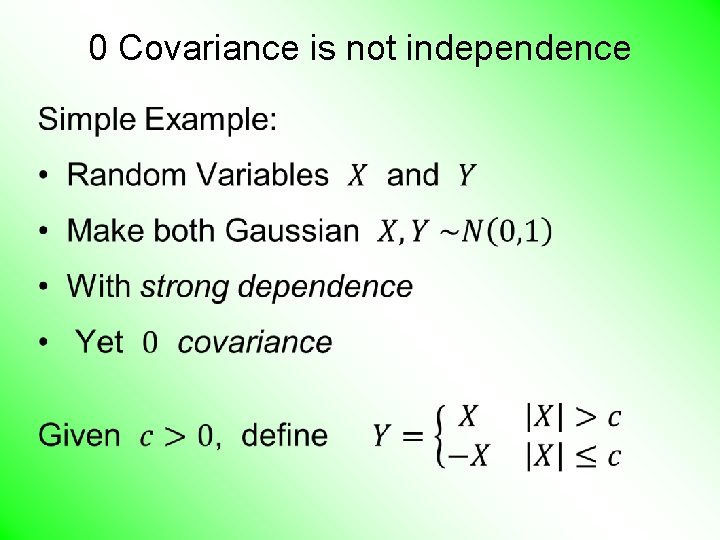
0 Covariance is not independence •
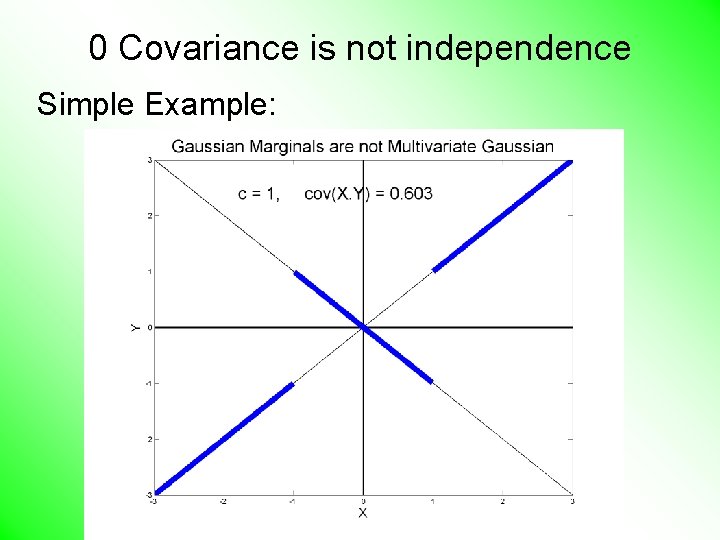
0 Covariance is not independence Simple Example:
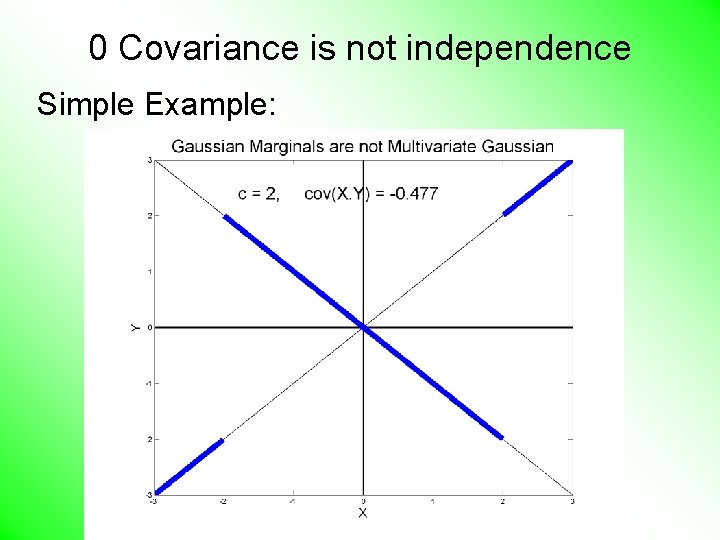
0 Covariance is not independence Simple Example:
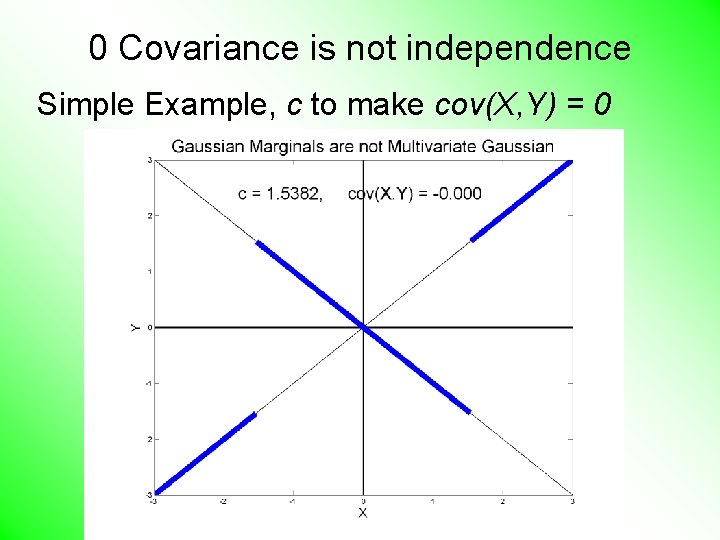
0 Covariance is not independence Simple Example, c to make cov(X, Y) = 0
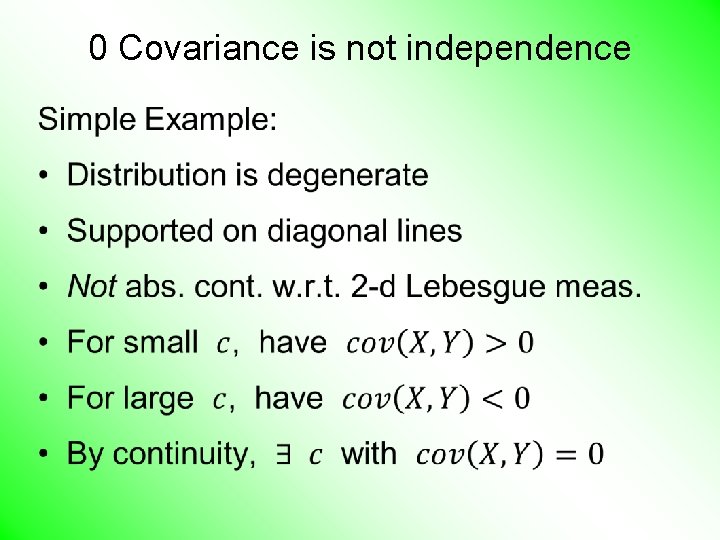
0 Covariance is not independence •
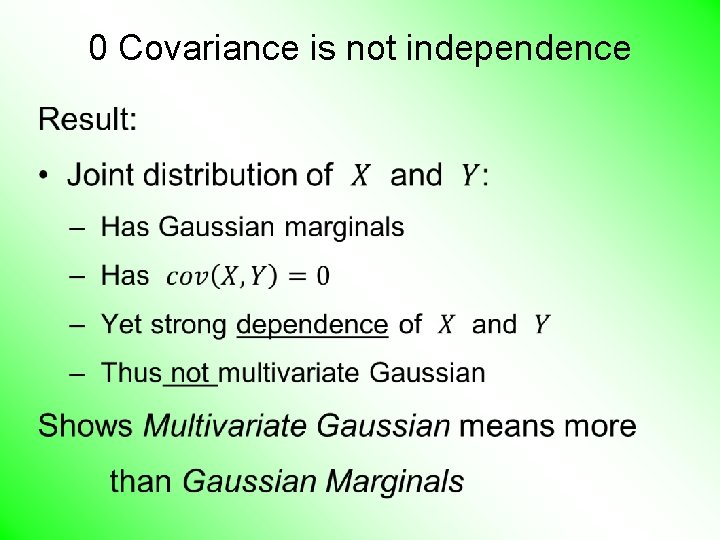
0 Covariance is not independence •
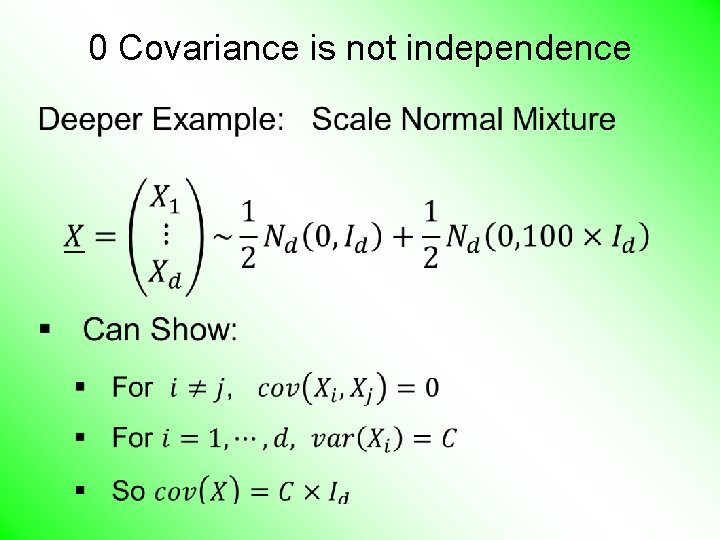
0 Covariance is not independence •
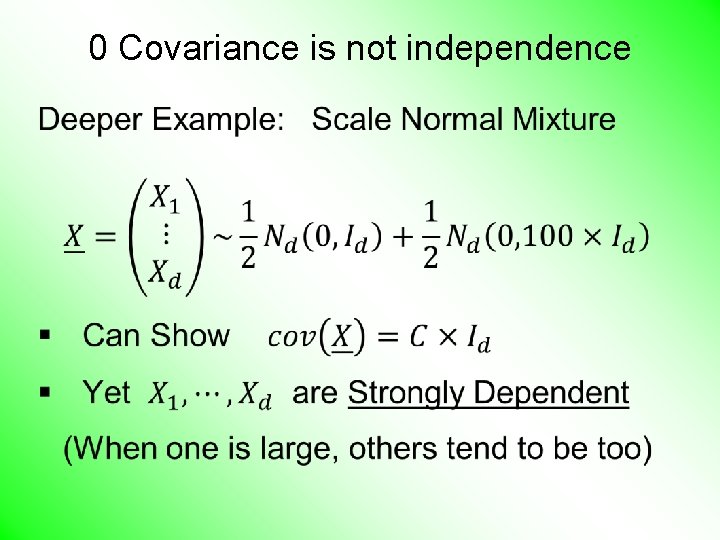
0 Covariance is not independence •
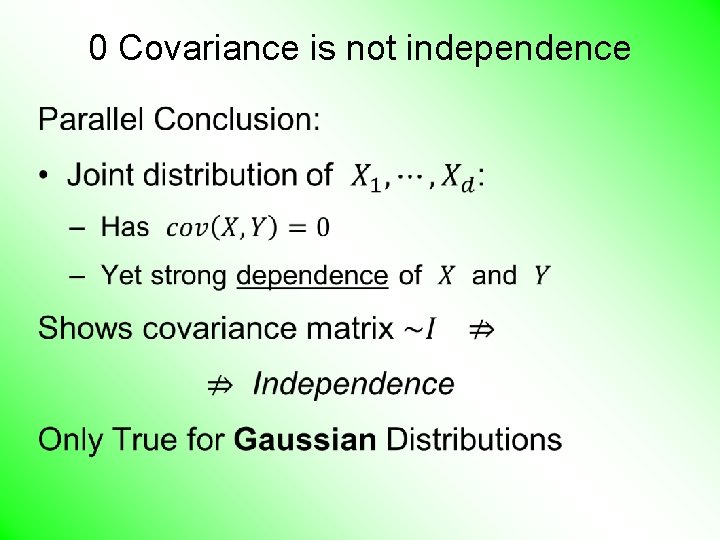
0 Covariance is not independence •
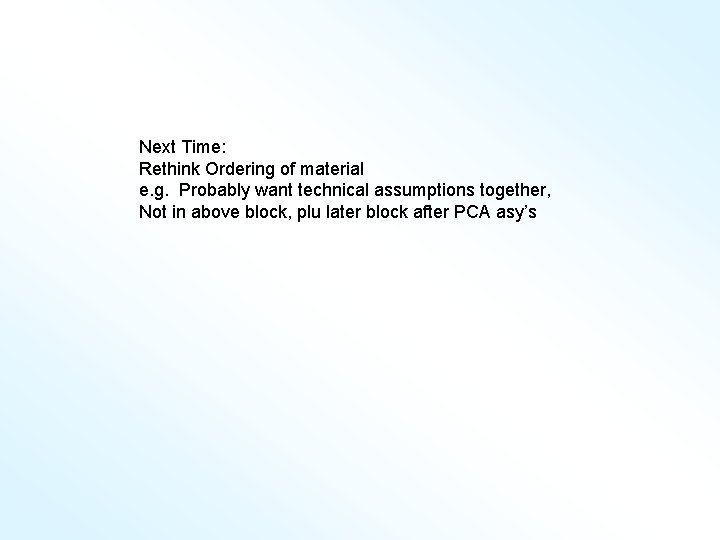
Next Time: Rethink Ordering of material e. g. Probably want technical assumptions together, Not in above block, plu later block after PCA asy’s
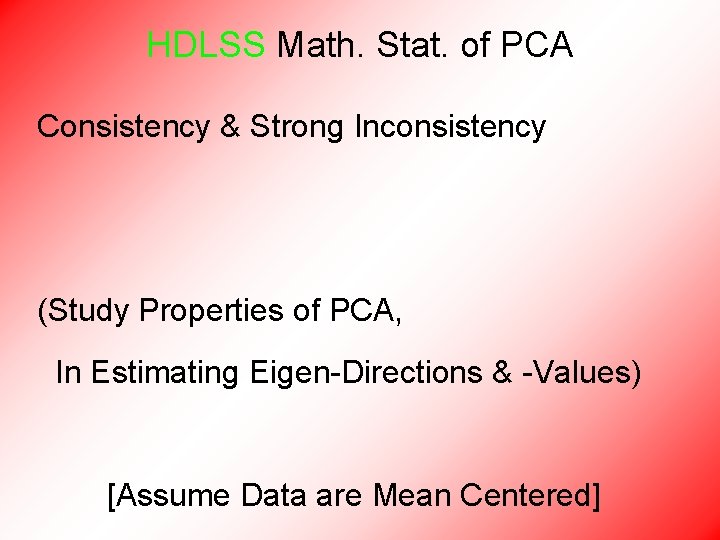
HDLSS Math. Stat. of PCA Consistency & Strong Inconsistency (Study Properties of PCA, In Estimating Eigen-Directions & -Values) [Assume Data are Mean Centered]
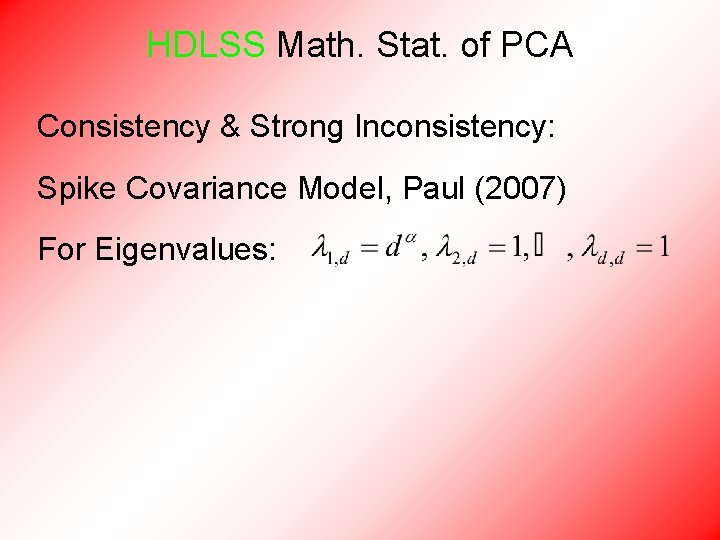
HDLSS Math. Stat. of PCA Consistency & Strong Inconsistency: Spike Covariance Model, Paul (2007) For Eigenvalues:
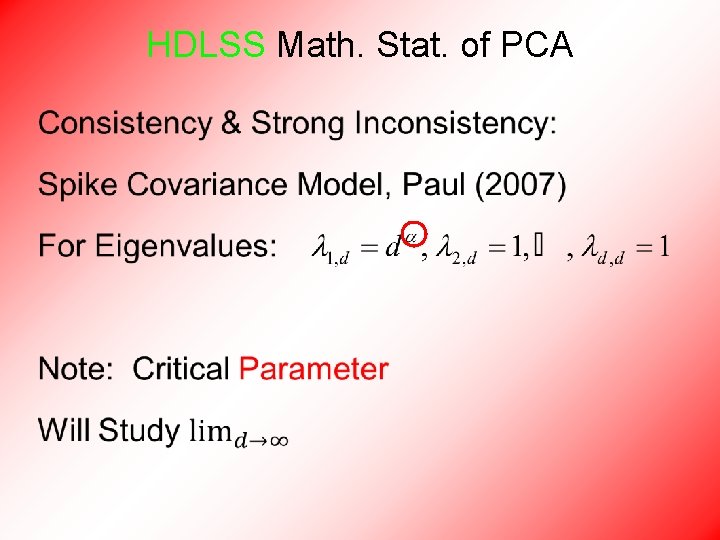
HDLSS Math. Stat. of PCA •
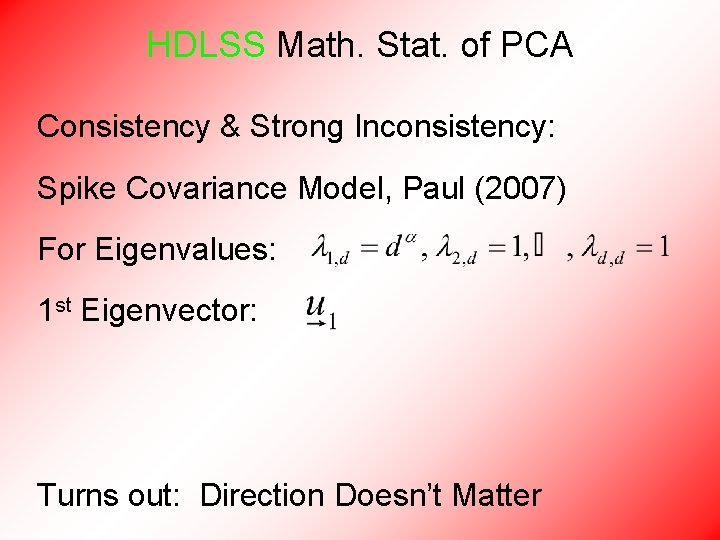
HDLSS Math. Stat. of PCA Consistency & Strong Inconsistency: Spike Covariance Model, Paul (2007) For Eigenvalues: 1 st Eigenvector: Turns out: Direction Doesn’t Matter
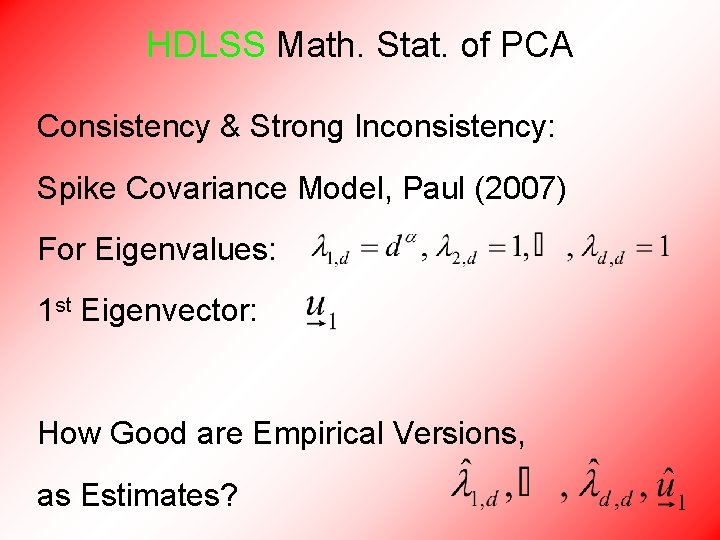
HDLSS Math. Stat. of PCA Consistency & Strong Inconsistency: Spike Covariance Model, Paul (2007) For Eigenvalues: 1 st Eigenvector: How Good are Empirical Versions, as Estimates?
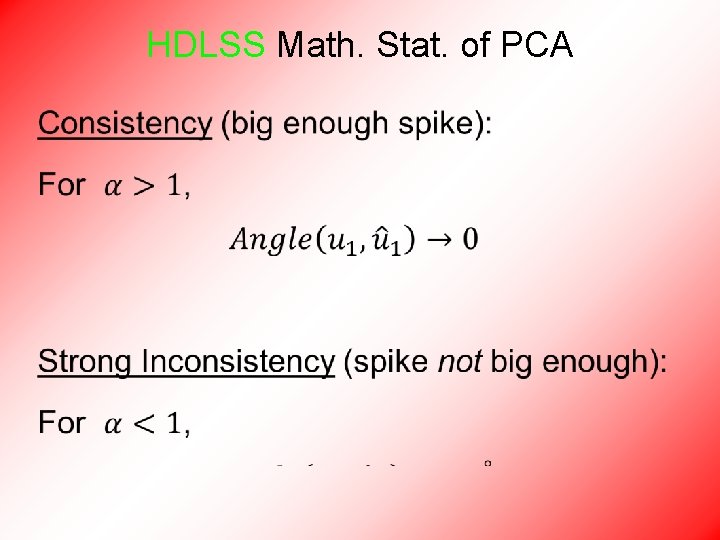
HDLSS Math. Stat. of PCA •
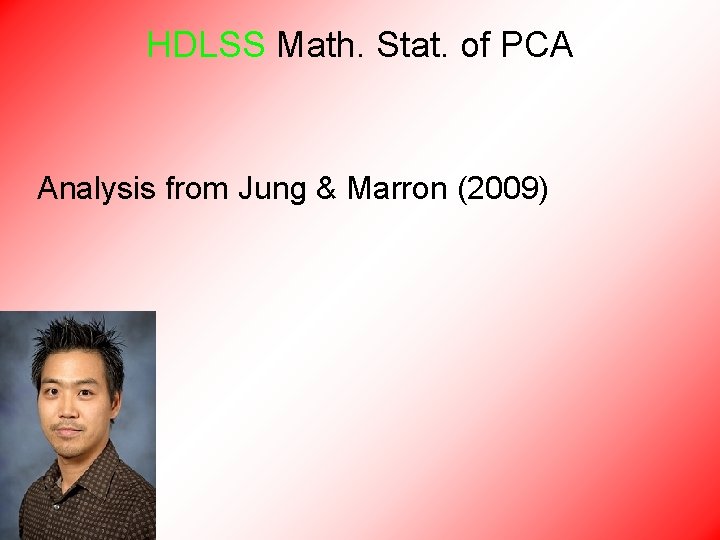
HDLSS Math. Stat. of PCA Analysis from Jung & Marron (2009)
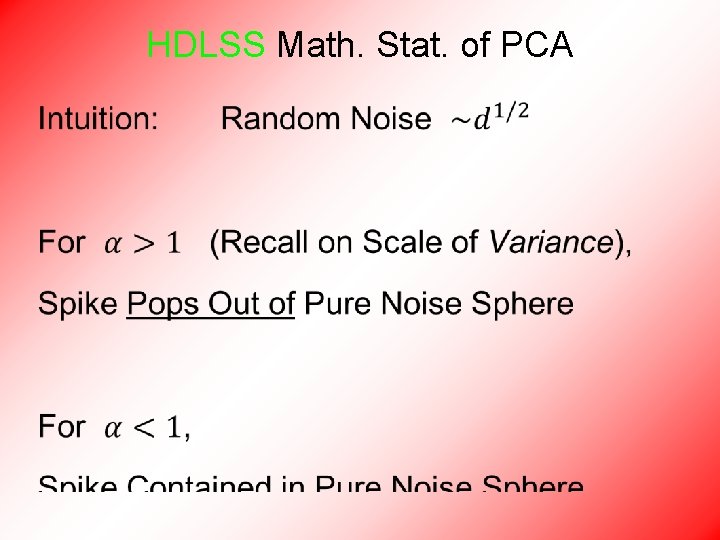
HDLSS Math. Stat. of PCA •
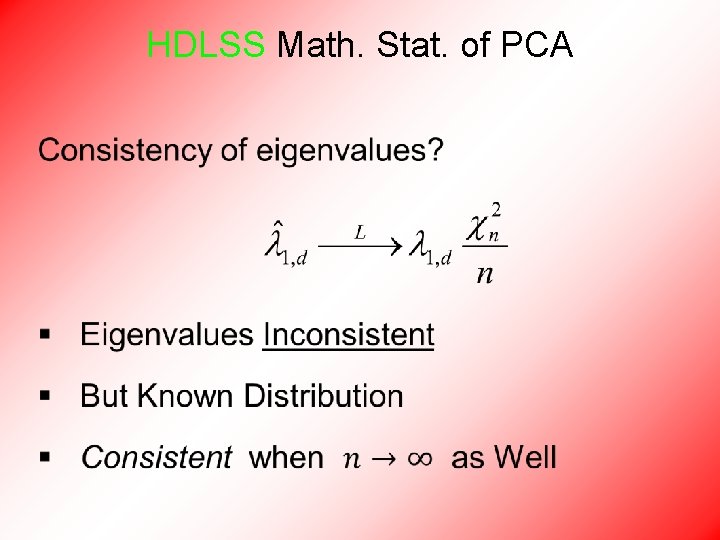
HDLSS Math. Stat. of PCA •
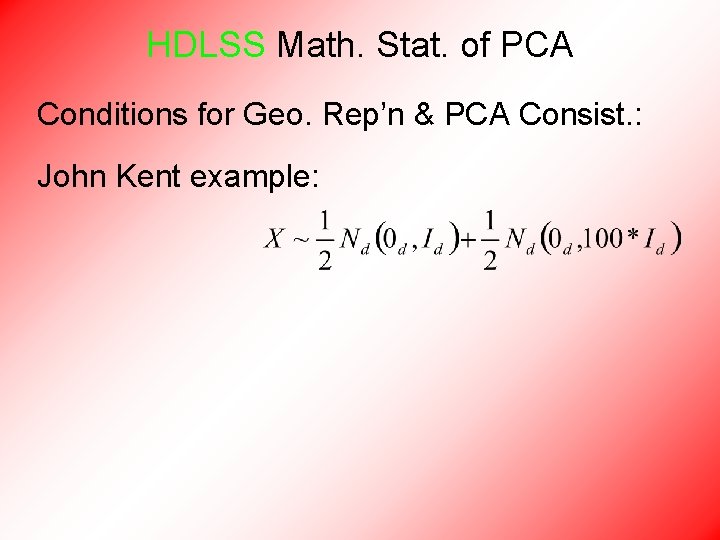
HDLSS Math. Stat. of PCA Conditions for Geo. Rep’n & PCA Consist. : John Kent example:
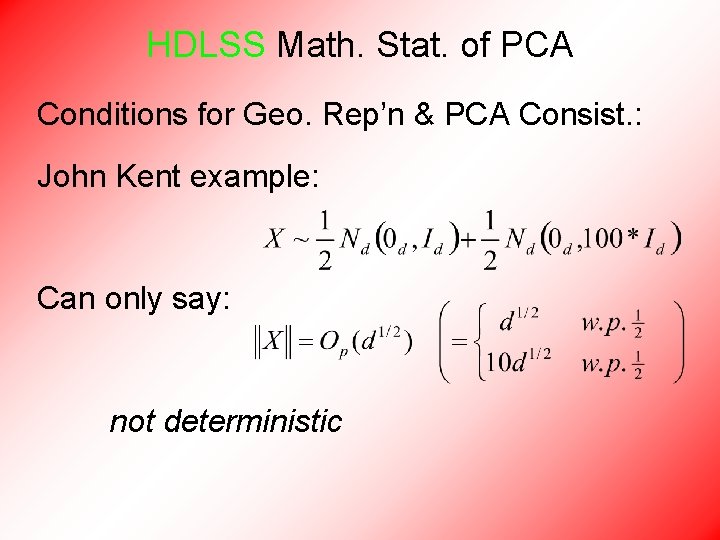
HDLSS Math. Stat. of PCA Conditions for Geo. Rep’n & PCA Consist. : John Kent example: Can only say: not deterministic
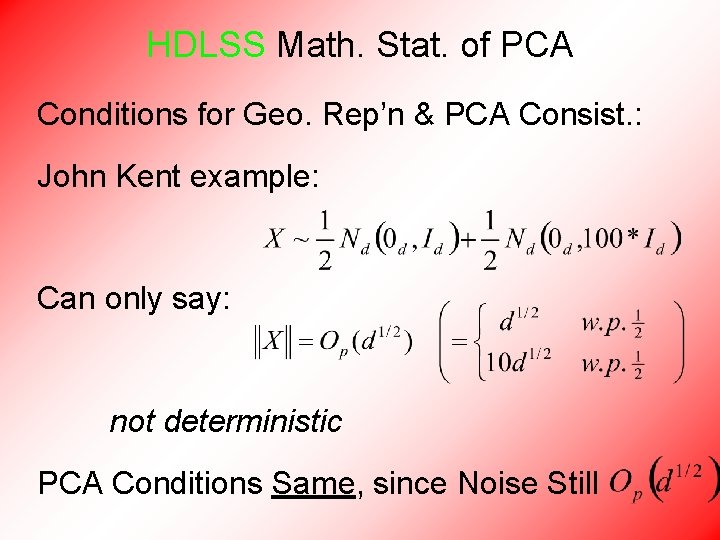
HDLSS Math. Stat. of PCA Conditions for Geo. Rep’n & PCA Consist. : John Kent example: Can only say: not deterministic PCA Conditions Same, since Noise Still
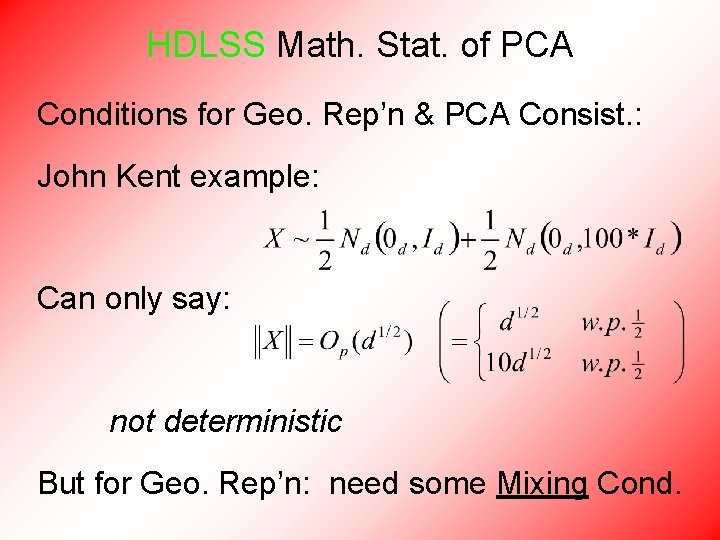
HDLSS Math. Stat. of PCA Conditions for Geo. Rep’n & PCA Consist. : John Kent example: Can only say: not deterministic But for Geo. Rep’n: need some Mixing Cond.
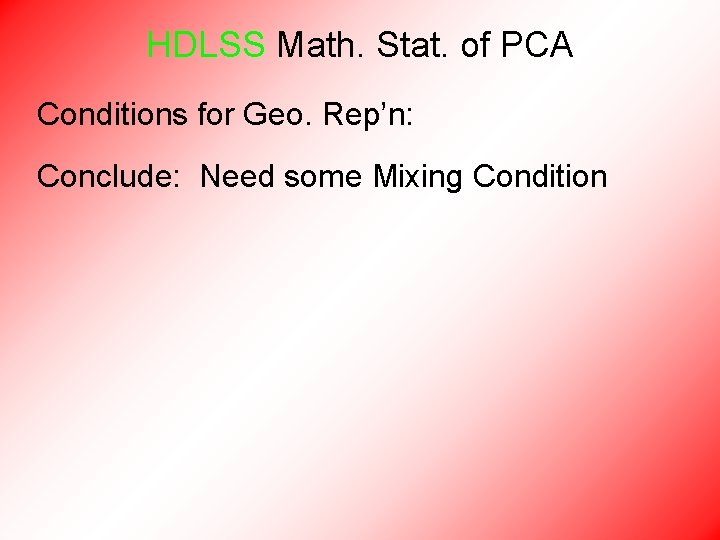
HDLSS Math. Stat. of PCA Conditions for Geo. Rep’n: Conclude: Need some Mixing Condition
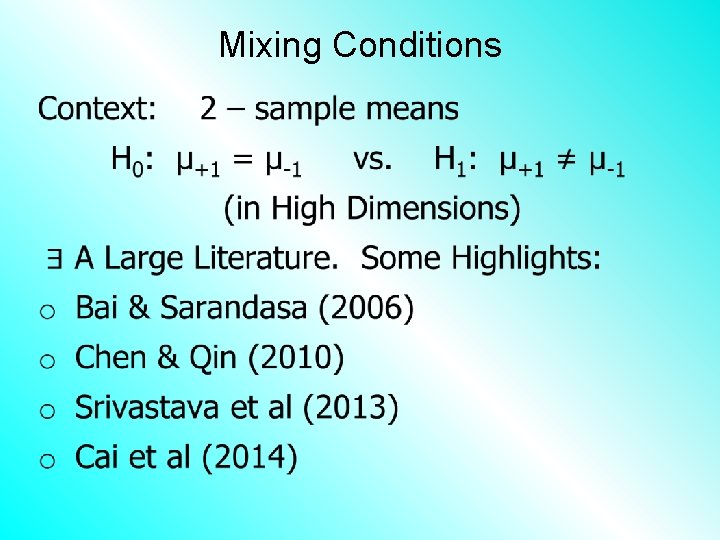
Mixing Conditions •
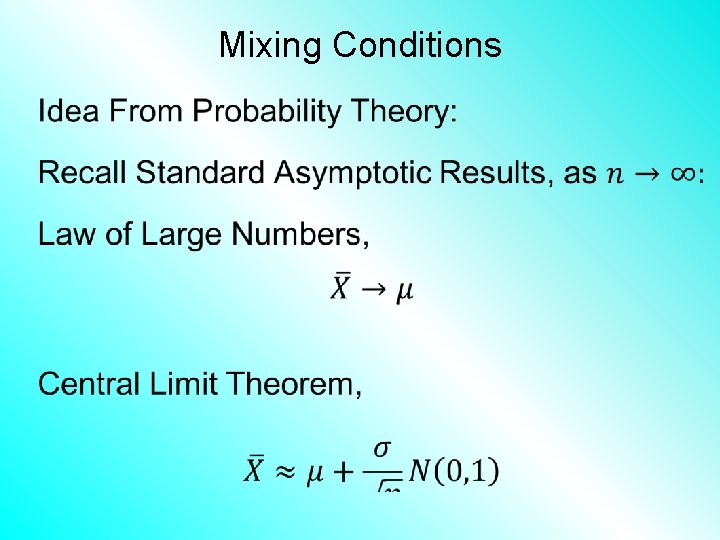
Mixing Conditions •
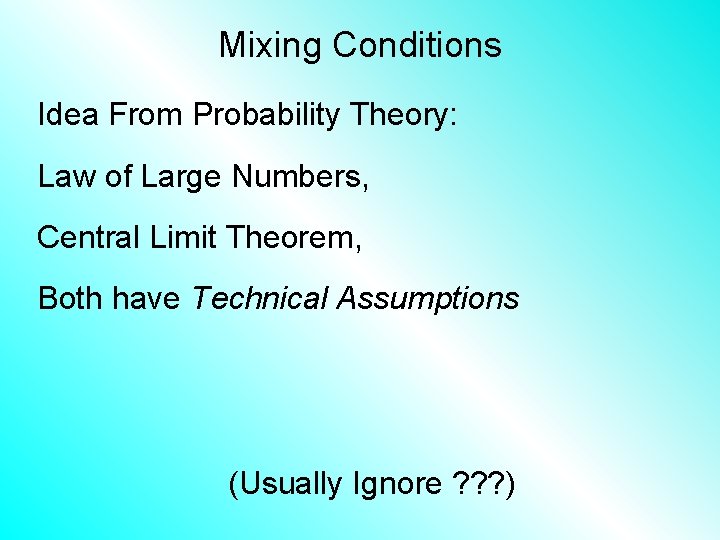
Mixing Conditions Idea From Probability Theory: Law of Large Numbers, Central Limit Theorem, Both have Technical Assumptions (Usually Ignore ? ? ? )
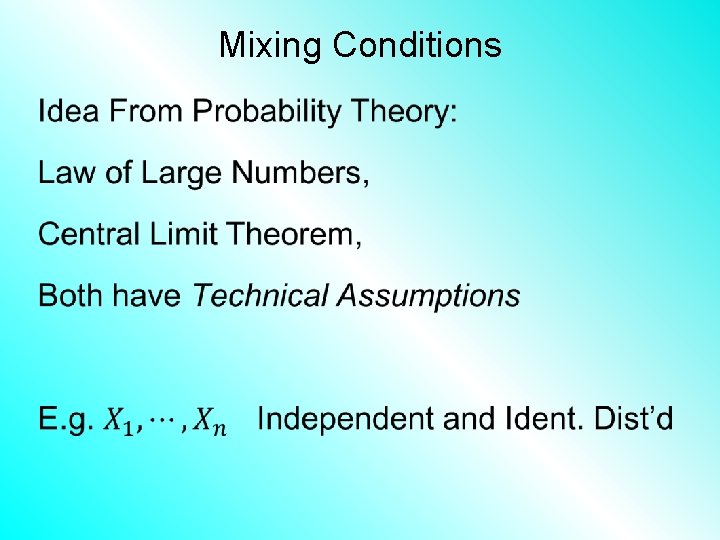
Mixing Conditions •
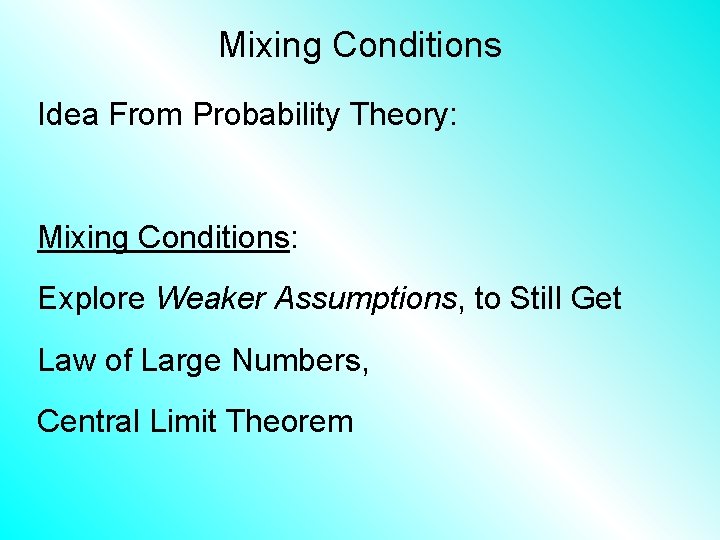
Mixing Conditions Idea From Probability Theory: Mixing Conditions: Explore Weaker Assumptions, to Still Get Law of Large Numbers, Central Limit Theorem
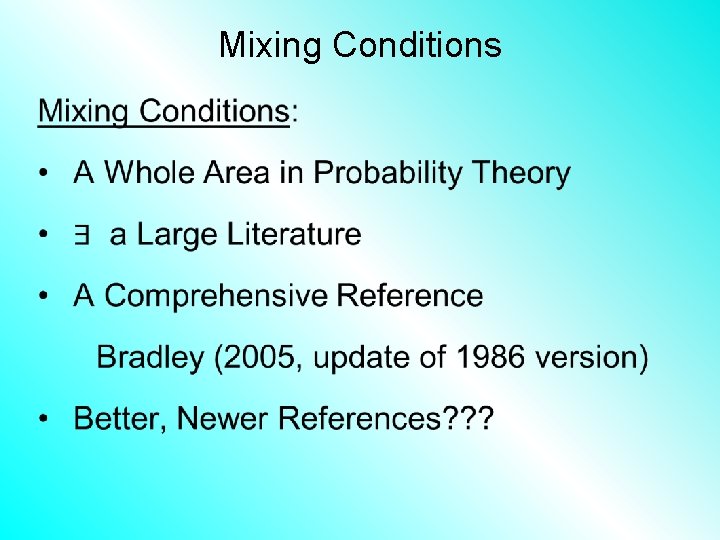
Mixing Conditions •
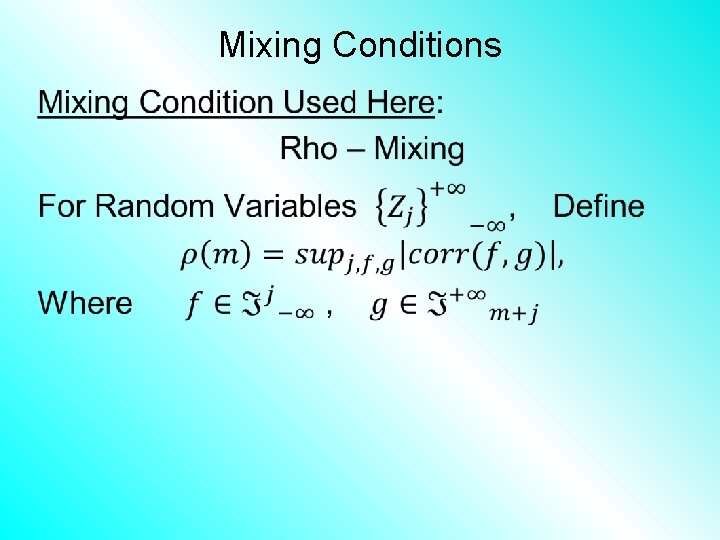
Mixing Conditions •
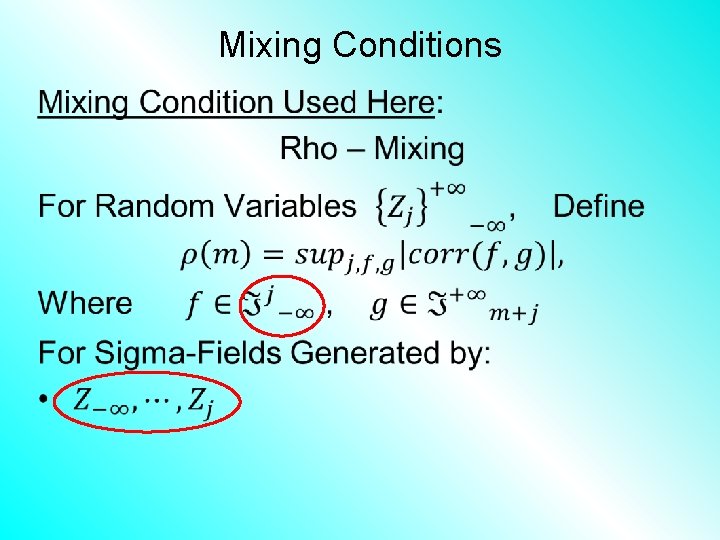
Mixing Conditions •
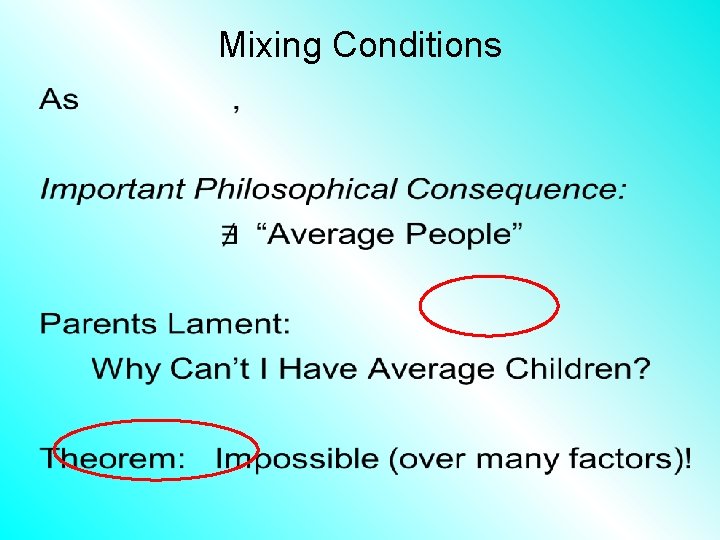
Mixing Conditions •
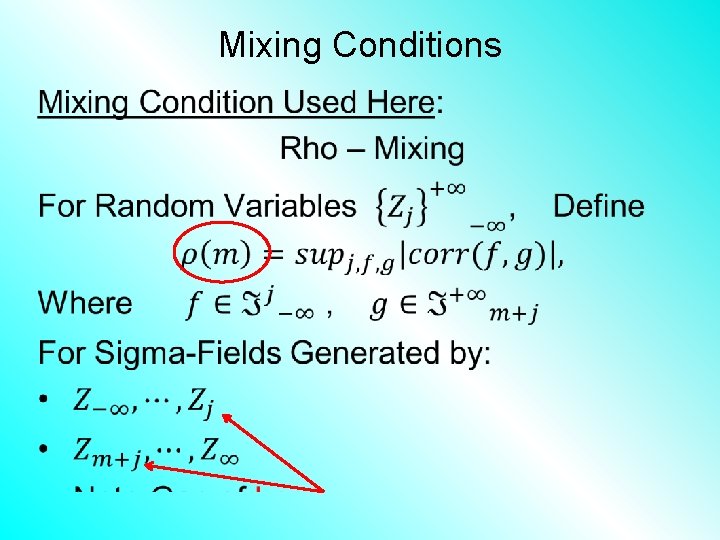
Mixing Conditions •
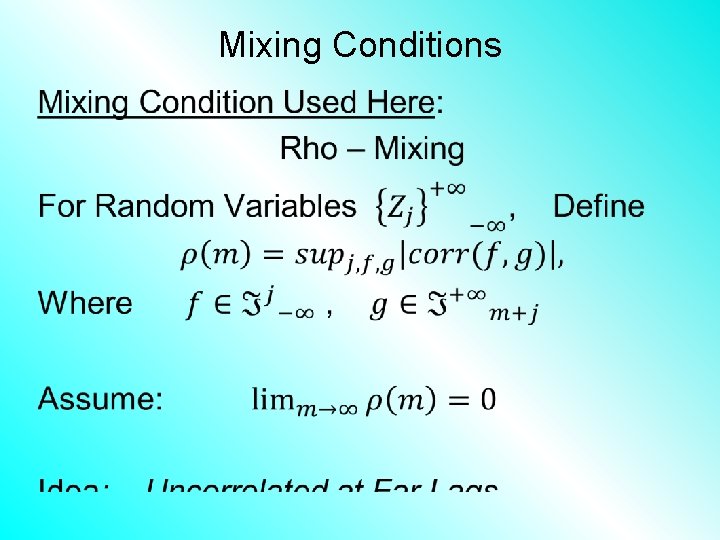
Mixing Conditions •
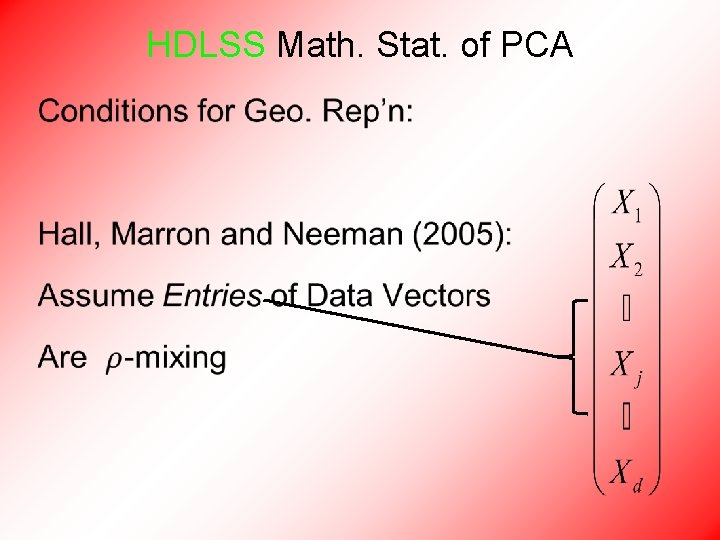
HDLSS Math. Stat. of PCA •
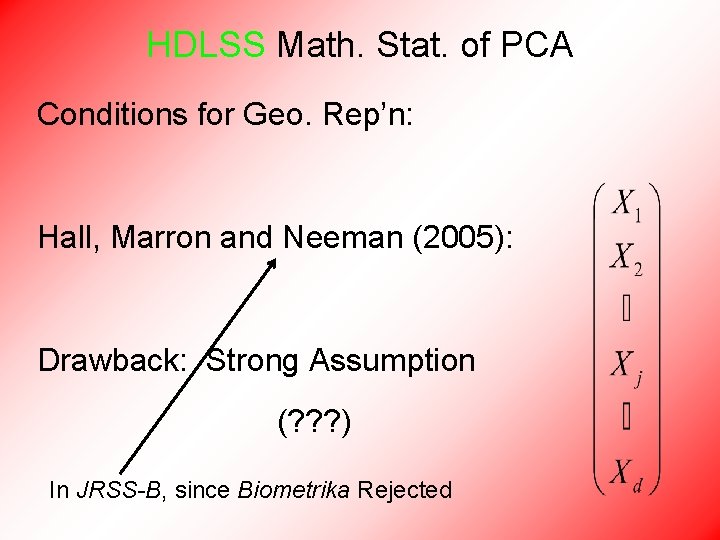
HDLSS Math. Stat. of PCA Conditions for Geo. Rep’n: Hall, Marron and Neeman (2005): Drawback: Strong Assumption (? ? ? ) In JRSS-B, since Biometrika Rejected
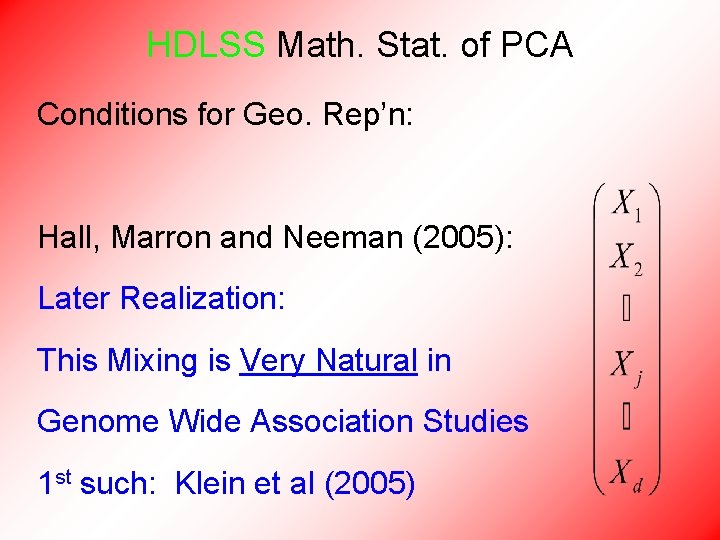
HDLSS Math. Stat. of PCA Conditions for Geo. Rep’n: Hall, Marron and Neeman (2005): Later Realization: This Mixing is Very Natural in Genome Wide Association Studies 1 st such: Klein et al (2005)
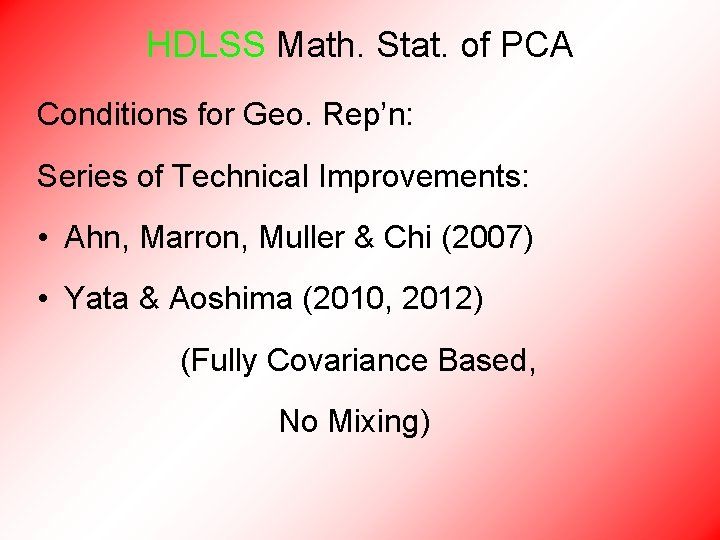
HDLSS Math. Stat. of PCA Conditions for Geo. Rep’n: Series of Technical Improvements: • Ahn, Marron, Muller & Chi (2007) • Yata & Aoshima (2010, 2012) (Fully Covariance Based, No Mixing)
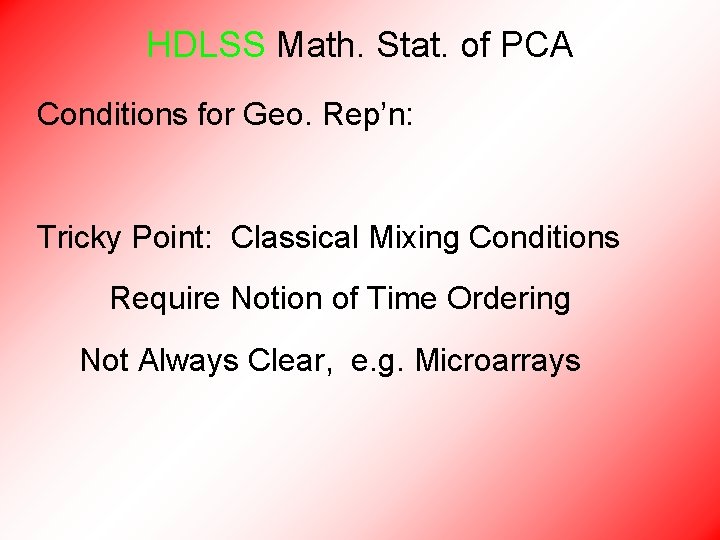
HDLSS Math. Stat. of PCA Conditions for Geo. Rep’n: Tricky Point: Classical Mixing Conditions Require Notion of Time Ordering Not Always Clear, e. g. Microarrays
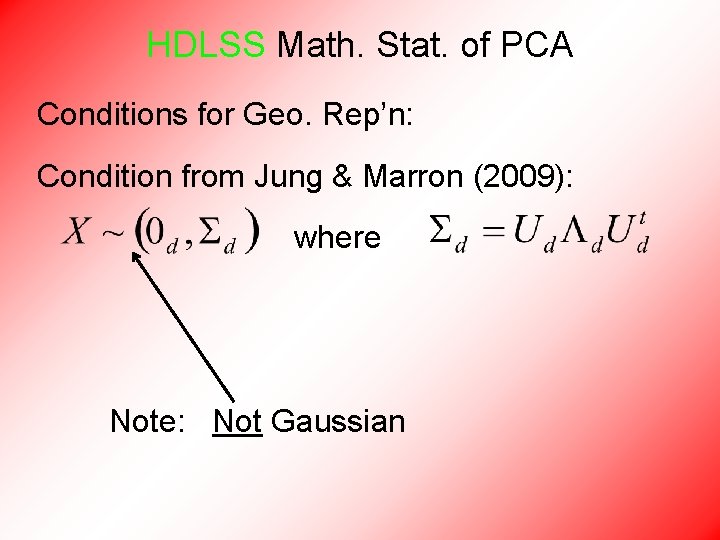
HDLSS Math. Stat. of PCA Conditions for Geo. Rep’n: Condition from Jung & Marron (2009): where Note: Not Gaussian
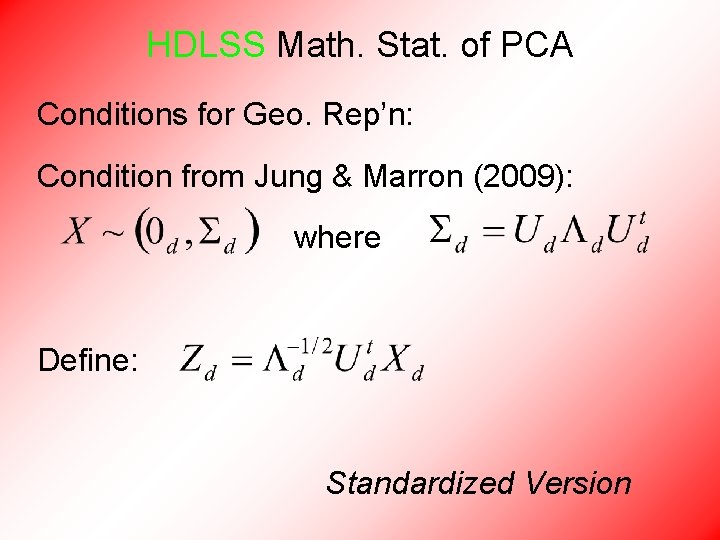
HDLSS Math. Stat. of PCA Conditions for Geo. Rep’n: Condition from Jung & Marron (2009): where Define: Standardized Version
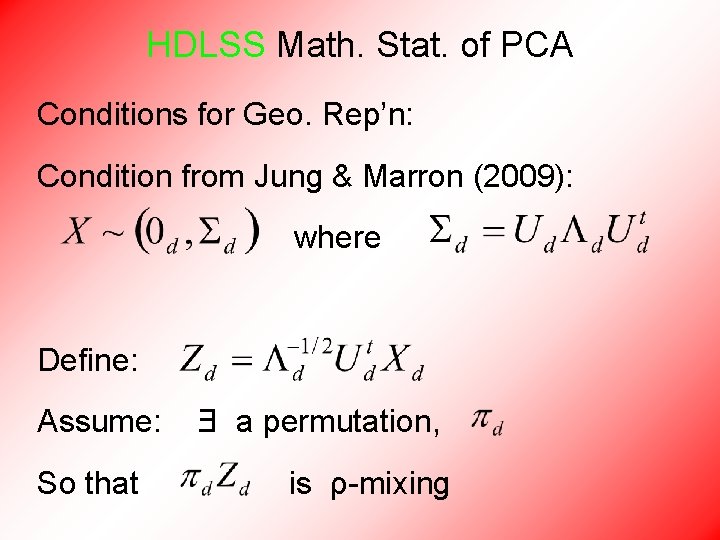
HDLSS Math. Stat. of PCA Conditions for Geo. Rep’n: Condition from Jung & Marron (2009): where Define: Assume: So that Ǝ a permutation, is ρ-mixing
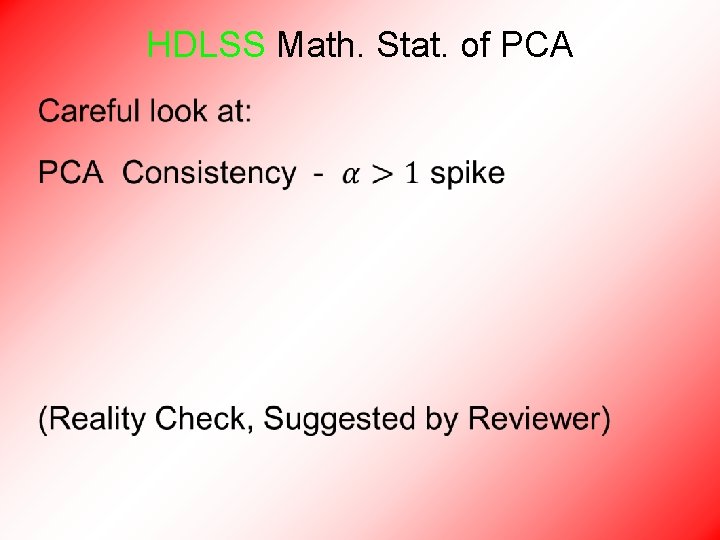
HDLSS Math. Stat. of PCA •
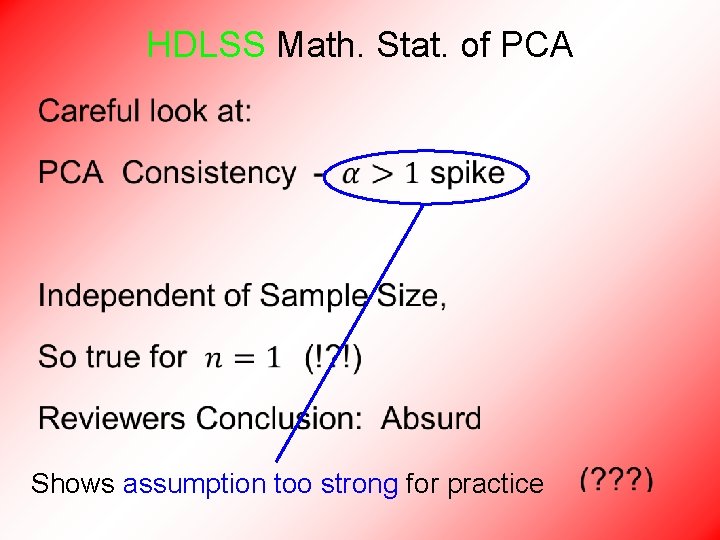
HDLSS Math. Stat. of PCA • Shows assumption too strong for practice
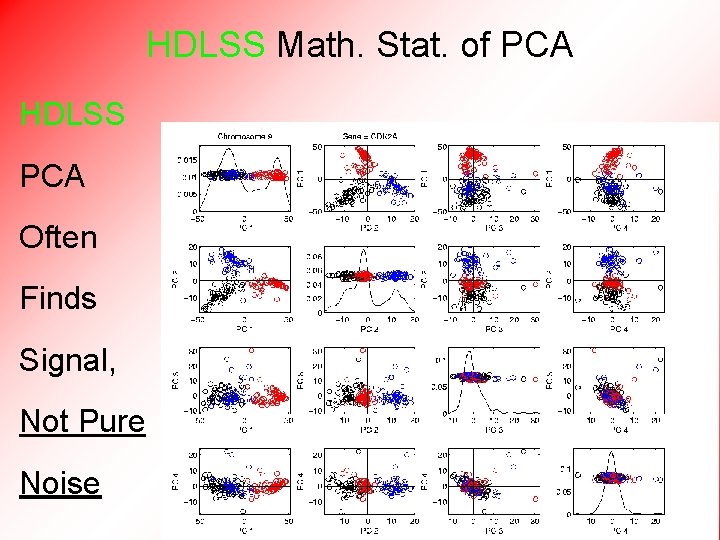
HDLSS Math. Stat. of PCA HDLSS PCA Often Finds Signal, Not Pure Noise
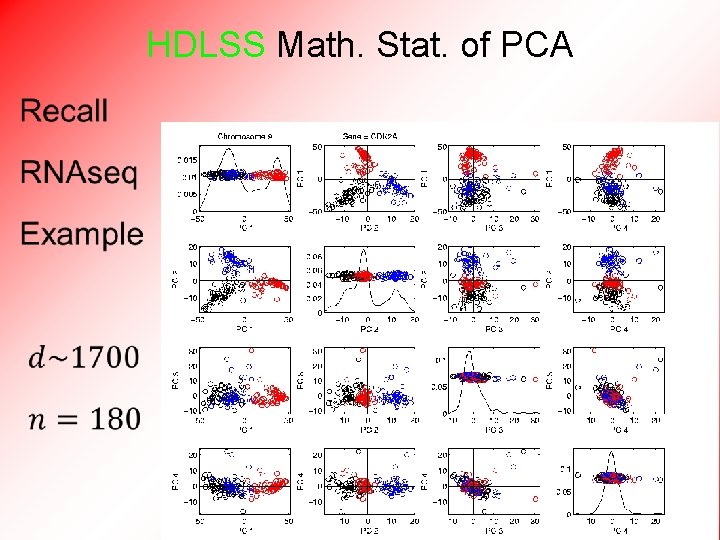
HDLSS Math. Stat. of PCA •
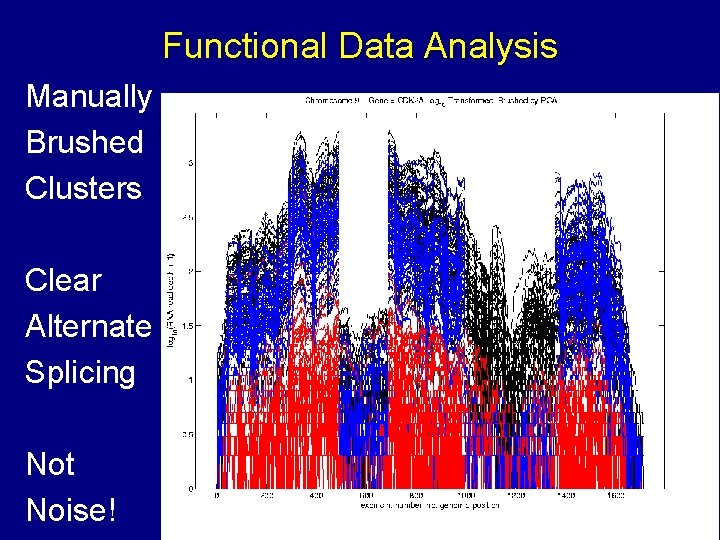
Functional Data Analysis Manually Brushed Clusters Clear Alternate Splicing Not Noise!
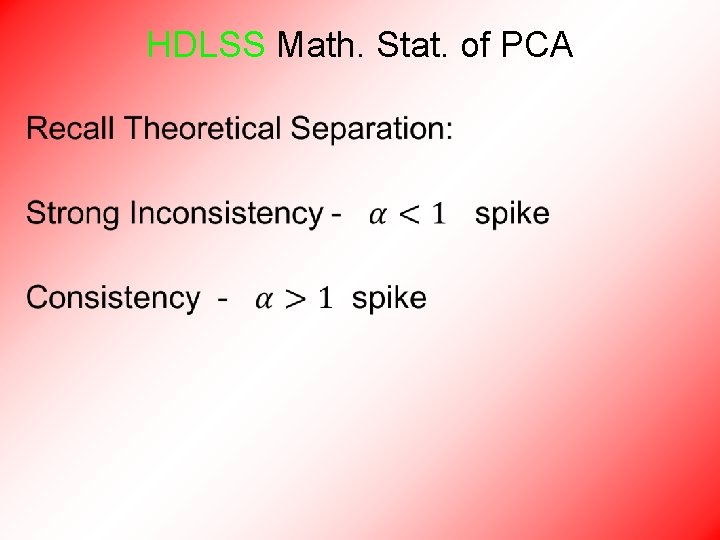
HDLSS Math. Stat. of PCA •
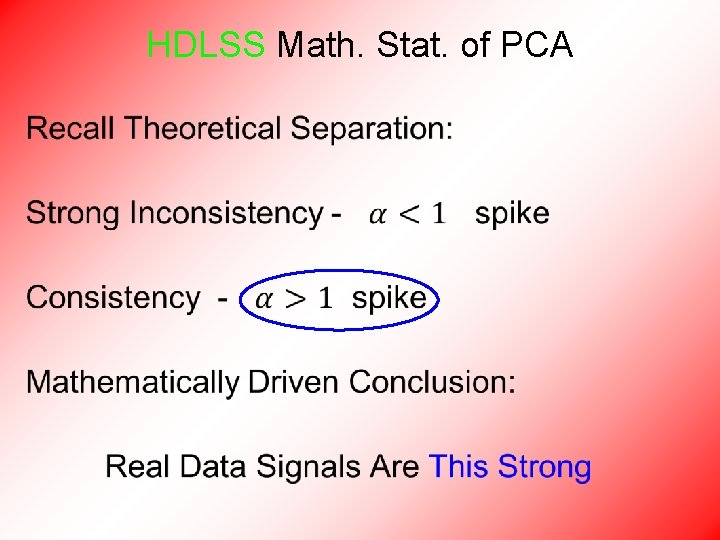
HDLSS Math. Stat. of PCA •
Onyen password change
All traffic signs and meanings
Will you please be quiet please themes
Please help yourself sign
Welcome please sign in
Name
Email informal format
Writing letters in french
Uat kick off email
Asystomatic
Trusso sign
Hypophosphatemia symptoms
Brudzinski's sign
When the signs are the same you add
Tlr participant worksheet answers
Ca tlr transfer
Participant sample
Weak entity contoh
Participant oriented evaluation
Participant tracking system
Normative theory of communication
Dsf.ca/participant
Participant-driven research
Covert observation
What is a participant observer
Participant media
Participant portal h2020
What is covert participant observation
Democratic participant media theory
Participant diary/log
John burton foundation
Participant diary/log
Carmen heijligers
Normative theories of mass communication
Narrative ethnography
Samhsa anger management workbook
Eacea participant portal
Safe at home participant
Complaint against depository participant
Complete observer
Non participant observation
Social function of narrative text
Ideolohikal ang pagpili ng wika at paksa sa pananaliksik
Participant expectations
Eacea participant portal
Proximodistal development
Please put here
Please write down your name
Please write your name
Craft of scientific presentations
Titles for mental health presentations
Best and worst powerpoint presentations
To maintain audience interest in a multimedia presentation
Slidetodoc.com
Desventajas de corel presentations
-is not one of the purposes for giving oral presentations.
Verbal support in presentations
How to make a tok presentation
World's worst presentation
Business presentations bristol
Setting up ria
Boardworks flash
Pyramid principle
Internet presentations
The most dangerous game powerpoint
Note card presentation
The end pictures for presentations
Useful phrases presentation
Efficient elements mac
You exec presentations
Catchy titles for presentations
Research project name
Camping merit badge powerpoint
Customer service presentations
Cisco stack 3750
Bad powerpoint presentations examples
Really bad powerpoint
Youexec
Roof ppt presentations
Https creativemarket.com templates presentations
Horse topics for presentations
Types of oral presentations
Hello my name is in sign language
Is a name term sign symbol
Chapter 31 branding packaging and labeling
Authors last name first name initial
Name above every other name
5 sided shape
Public string name
Whats her name
Name of presentation company name