NRCSE Where is environmental statistics going Peter Guttorp
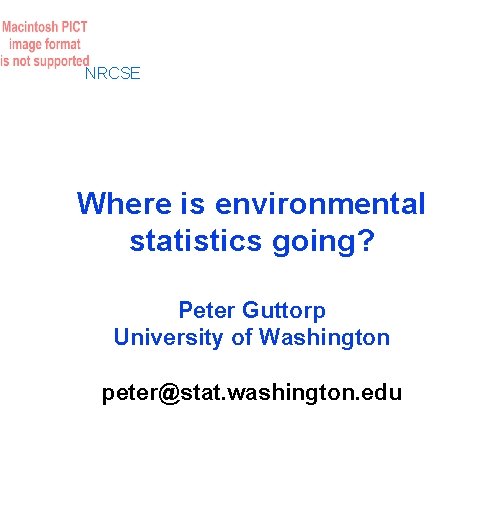
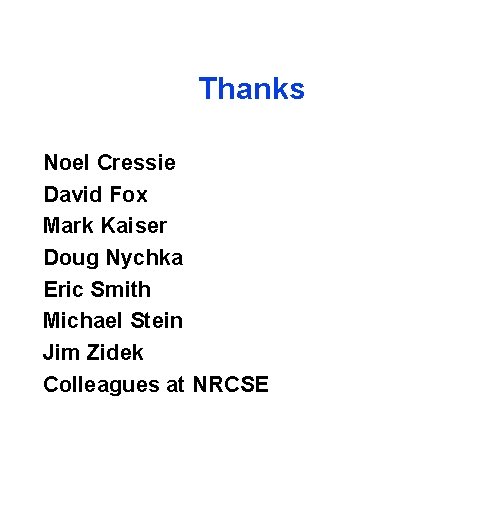
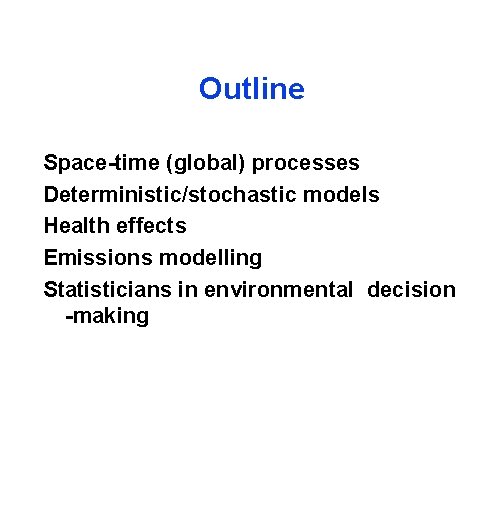
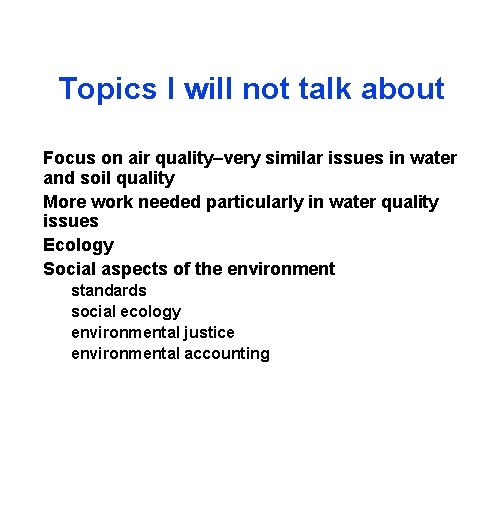
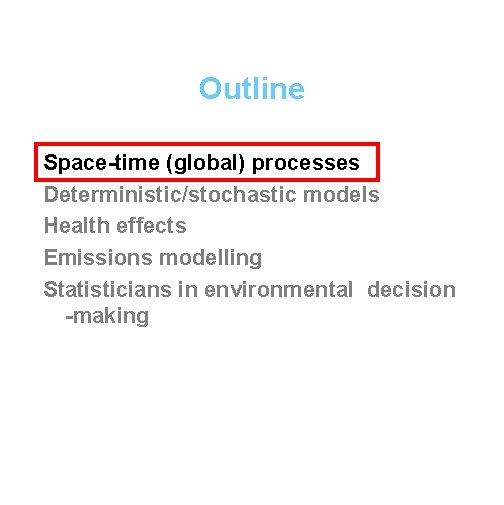
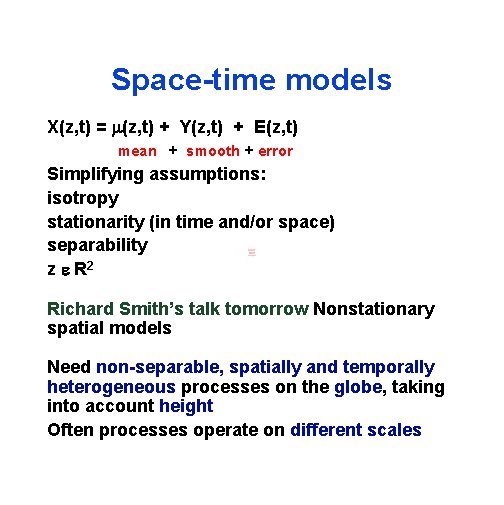
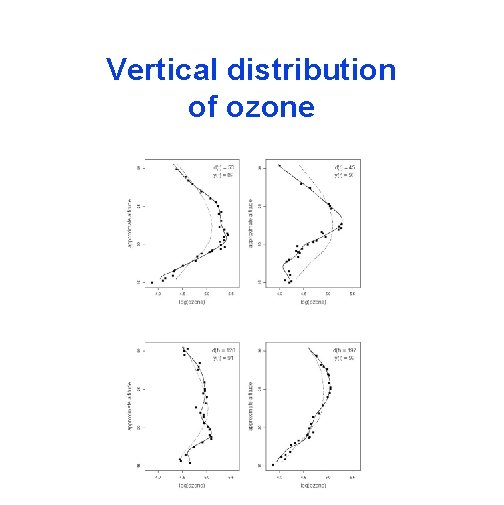
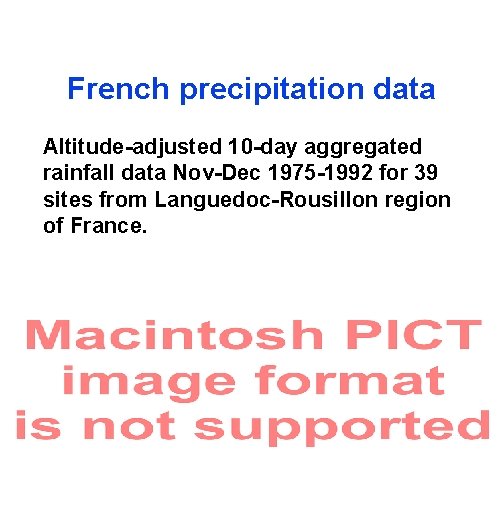
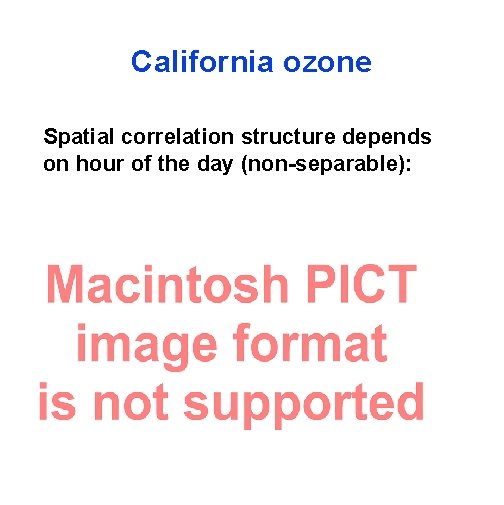
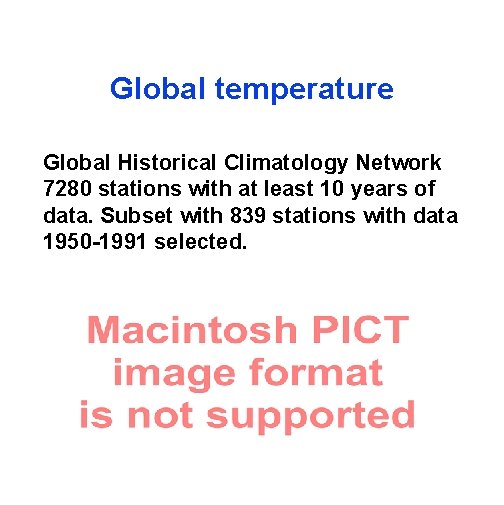
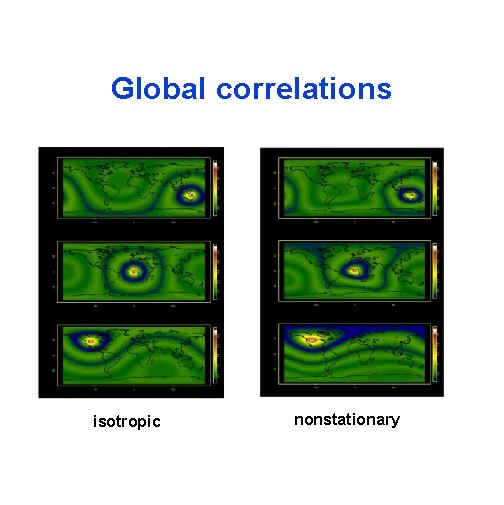
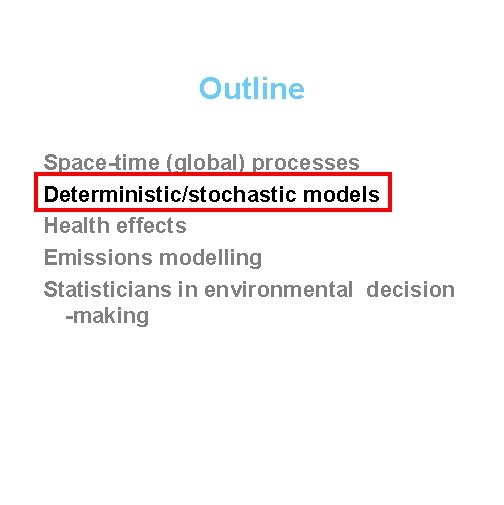
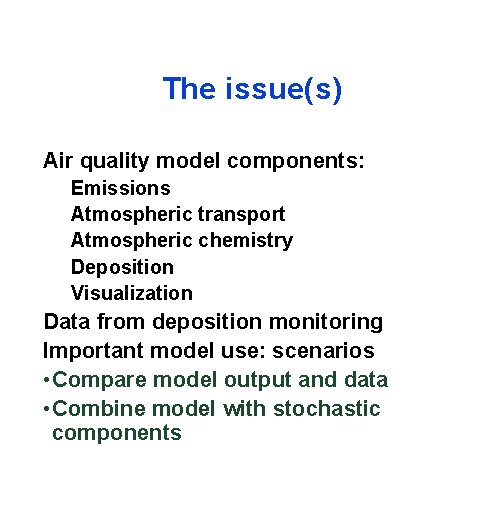
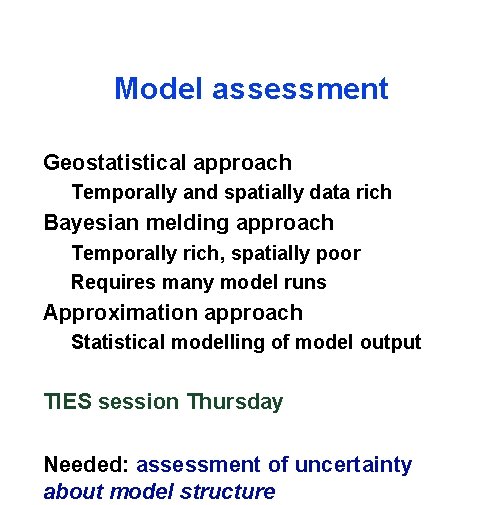
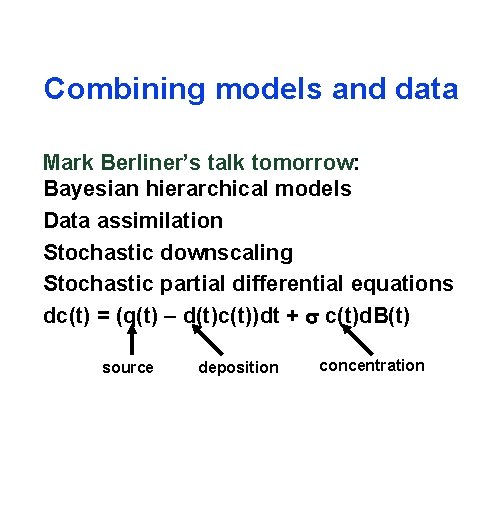
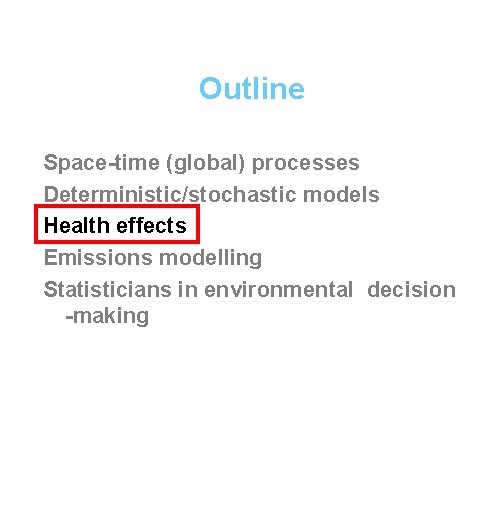
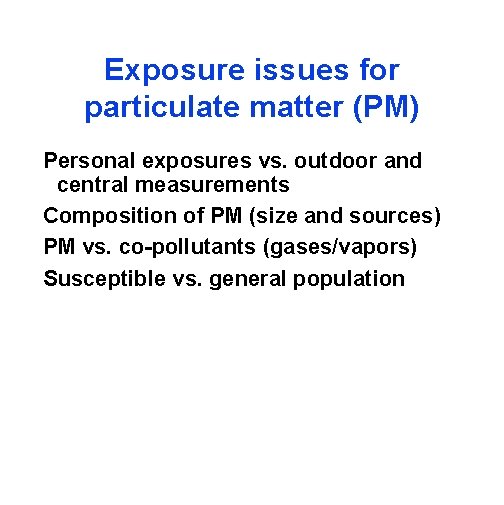
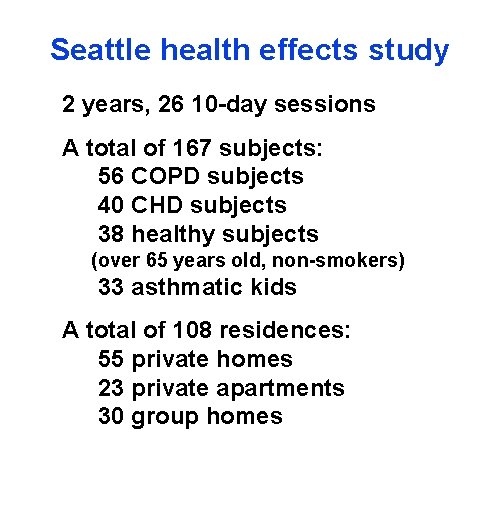
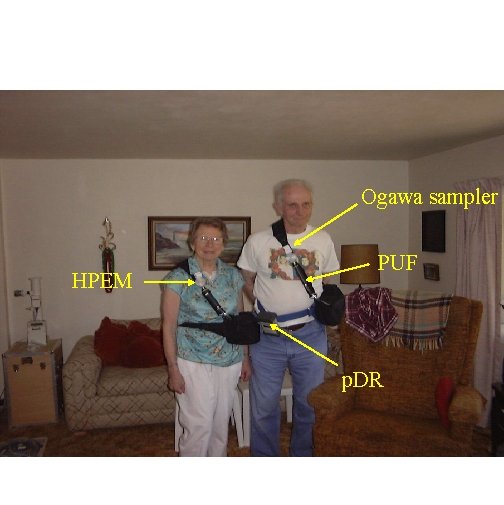
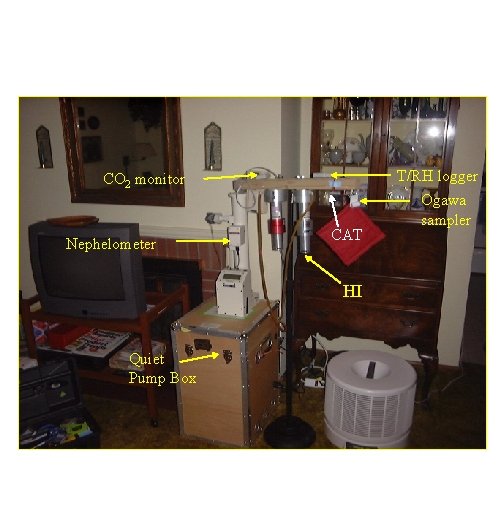
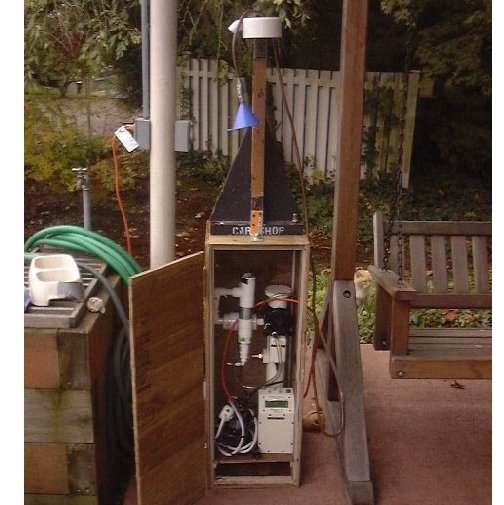
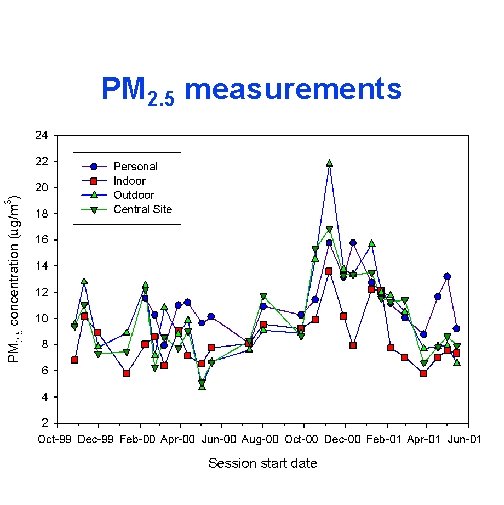
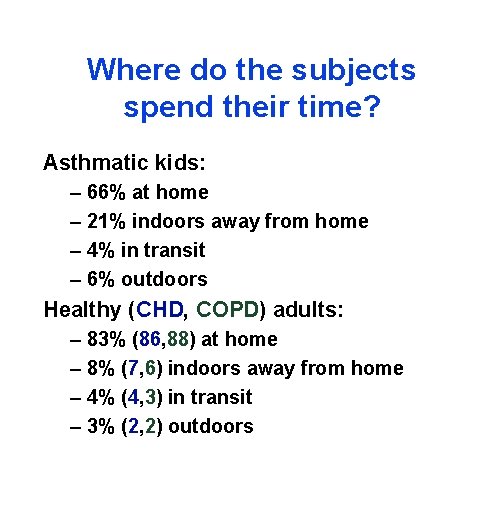
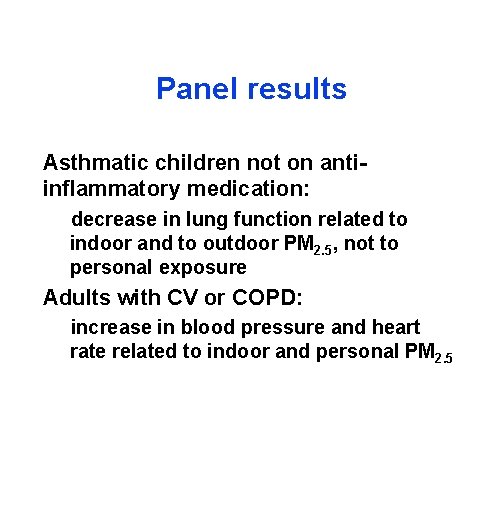
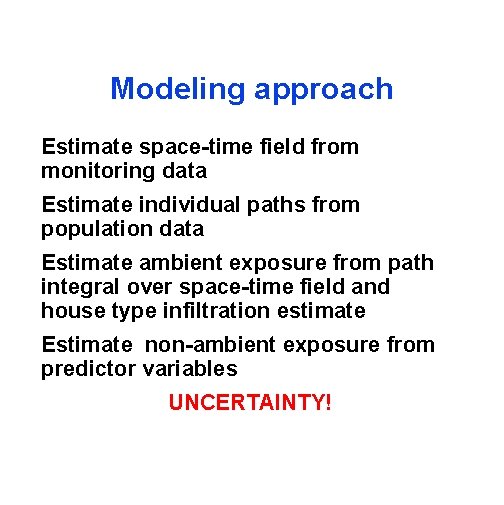
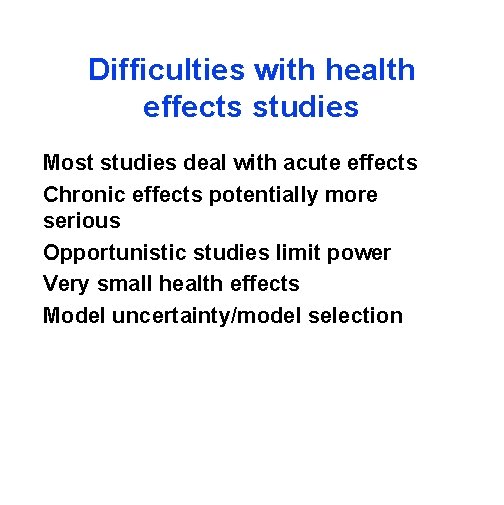
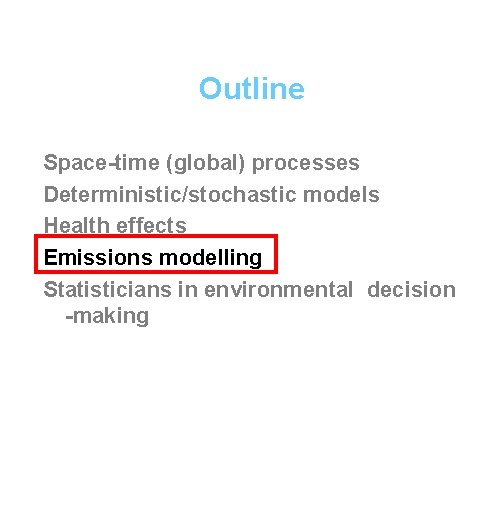
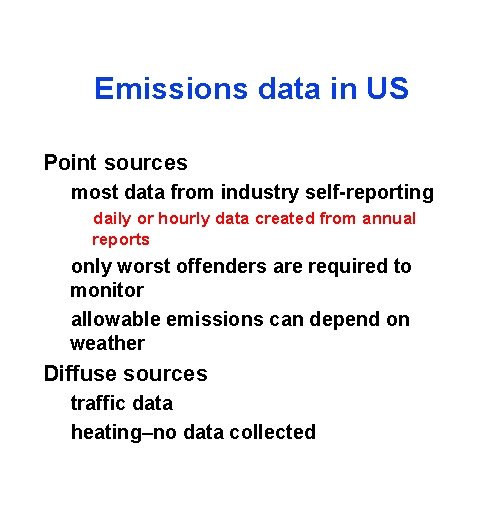
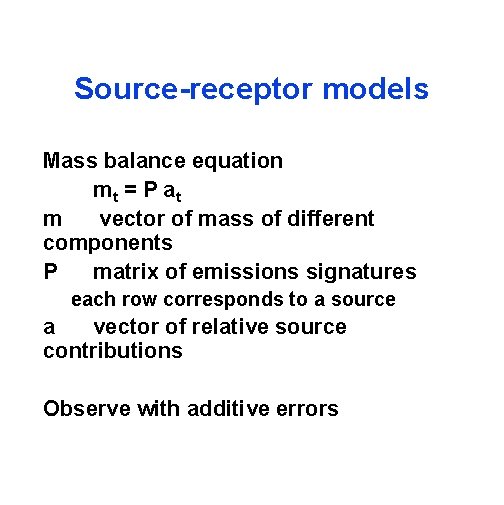
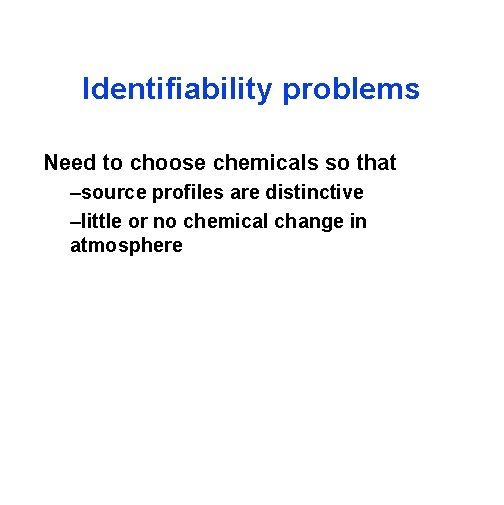
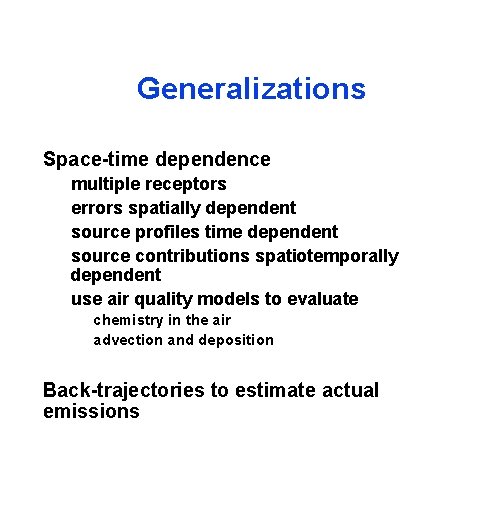
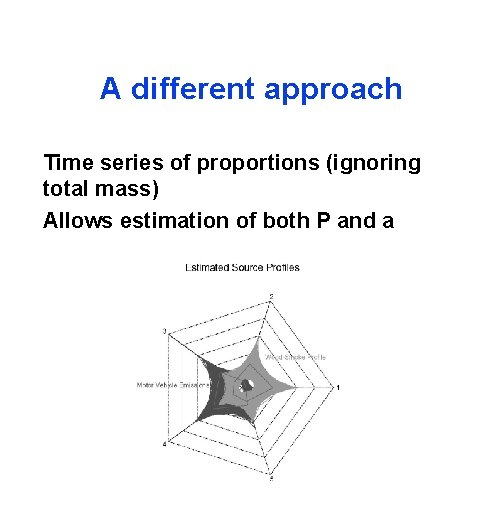
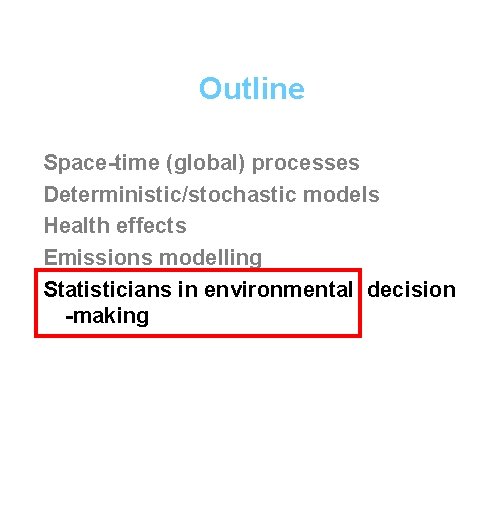
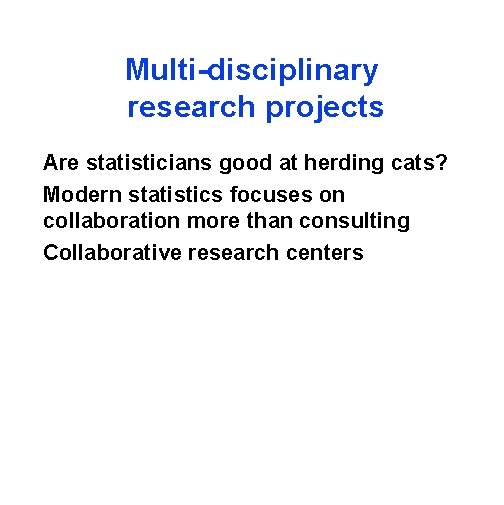
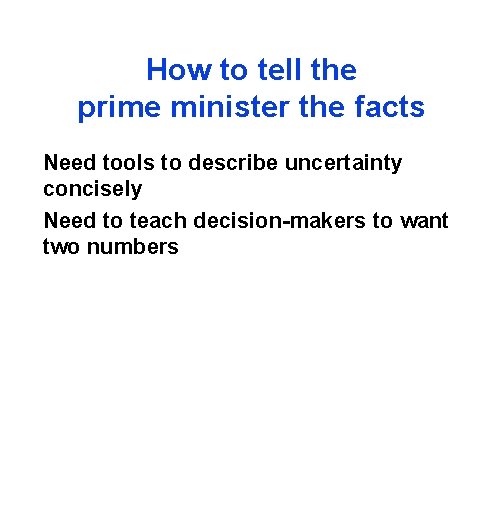
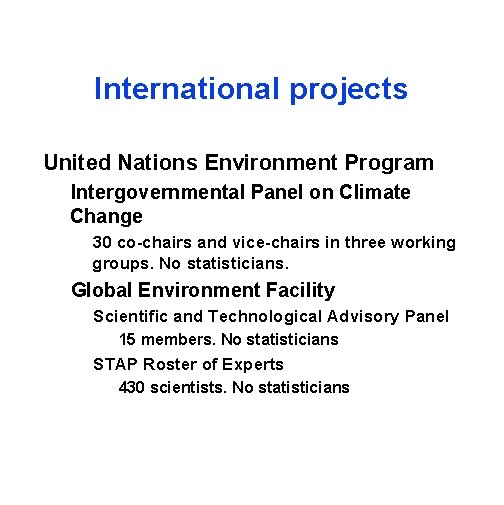
- Slides: 36
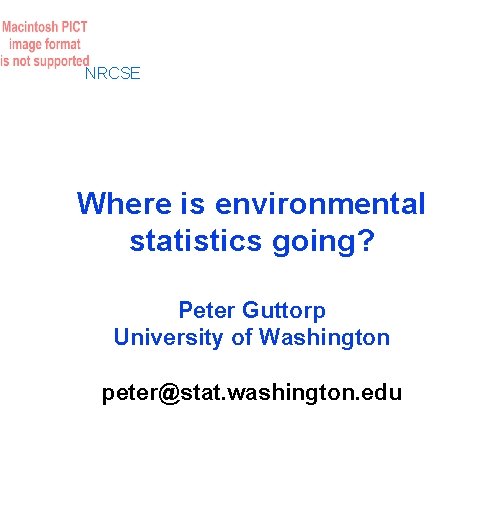
NRCSE Where is environmental statistics going? Peter Guttorp University of Washington peter@stat. washington. edu
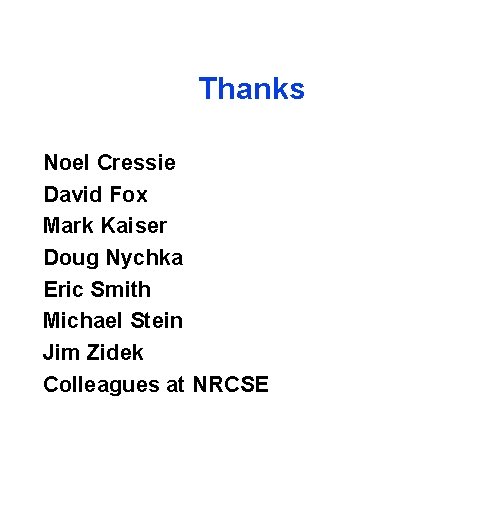
Thanks Noel Cressie David Fox Mark Kaiser Doug Nychka Eric Smith Michael Stein Jim Zidek Colleagues at NRCSE
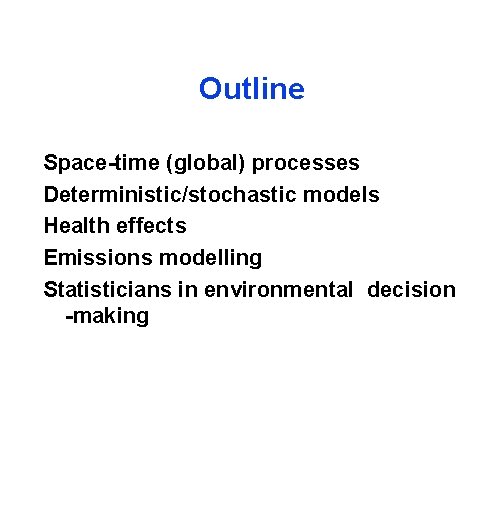
Outline Space-time (global) processes Deterministic/stochastic models Health effects Emissions modelling Statisticians in environmental decision -making
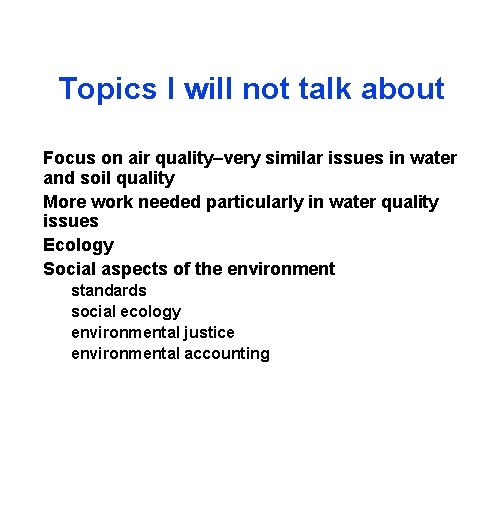
Topics I will not talk about Focus on air quality–very similar issues in water and soil quality More work needed particularly in water quality issues Ecology Social aspects of the environment standards social ecology environmental justice environmental accounting
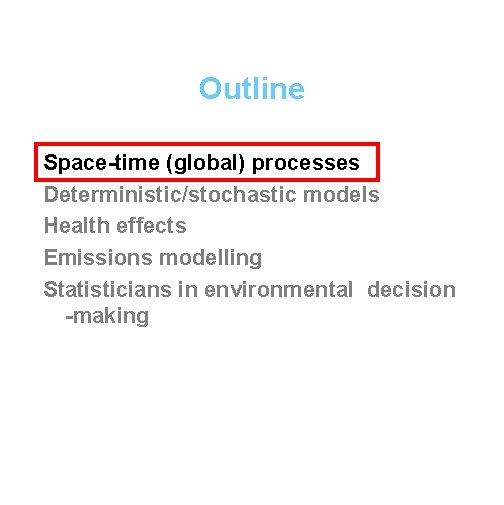
Outline Space-time (global) processes Deterministic/stochastic models Health effects Emissions modelling Statisticians in environmental decision -making
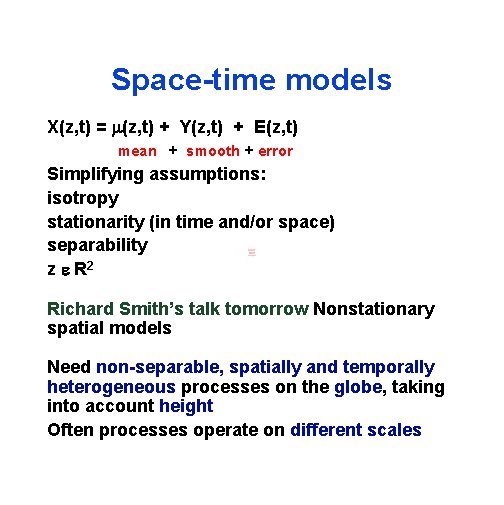
Space-time models X(z, t) = m(z, t) + Y(z, t) + E(z, t) mean + smooth + error Simplifying assumptions: isotropy stationarity (in time and/or space) separability z e R 2 Richard Smith’s talk tomorrow Nonstationary spatial models Need non-separable, spatially and temporally heterogeneous processes on the globe, taking into account height Often processes operate on different scales
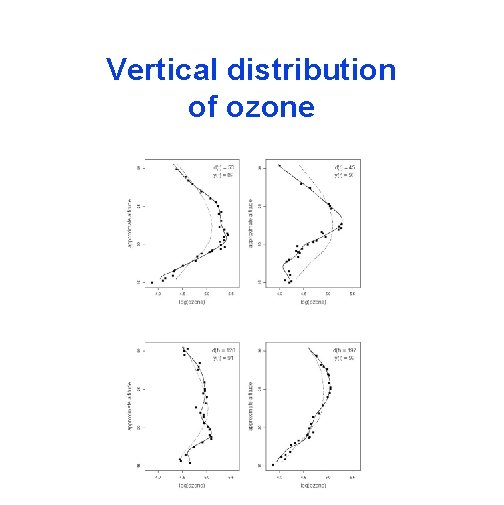
Vertical distribution of ozone
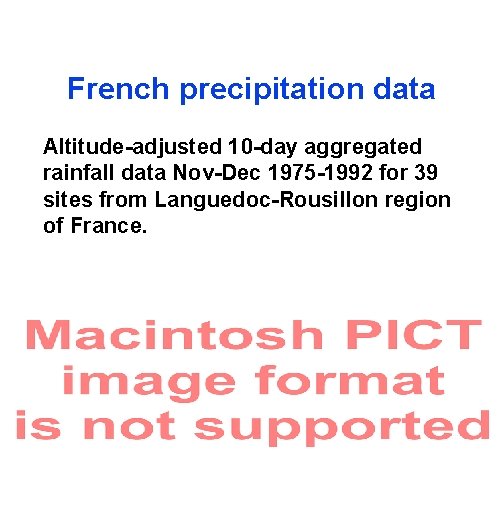
French precipitation data Altitude-adjusted 10 -day aggregated rainfall data Nov-Dec 1975 -1992 for 39 sites from Languedoc-Rousillon region of France.
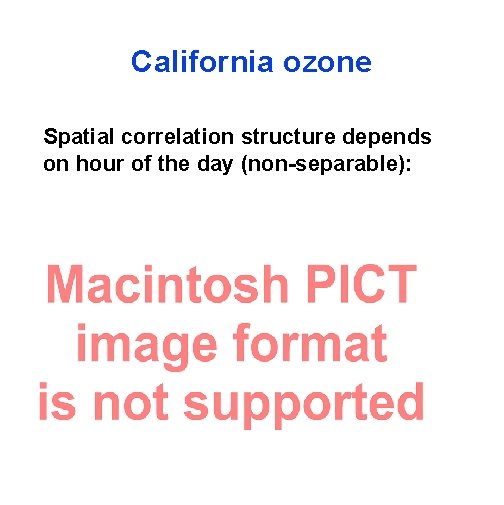
California ozone Spatial correlation structure depends on hour of the day (non-separable):
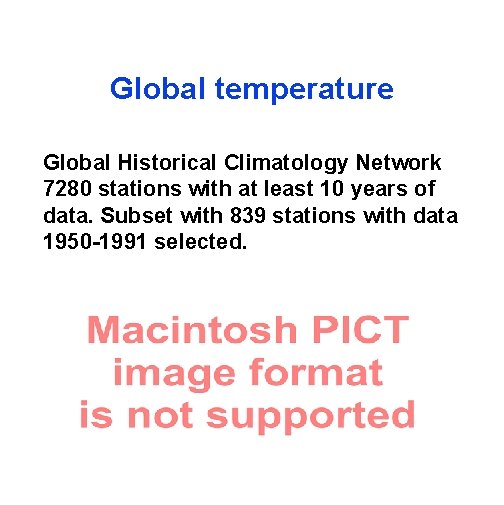
Global temperature Global Historical Climatology Network 7280 stations with at least 10 years of data. Subset with 839 stations with data 1950 -1991 selected.
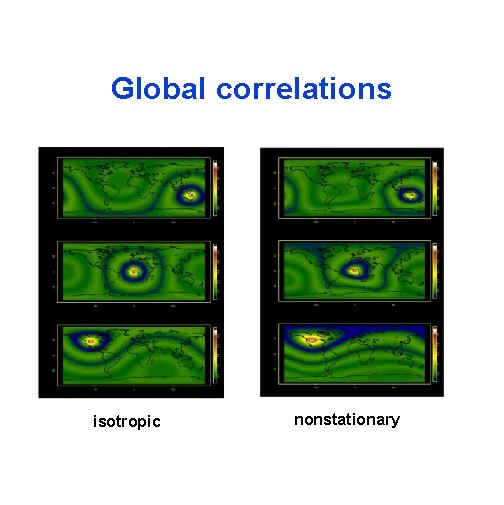
Global correlations isotropic nonstationary
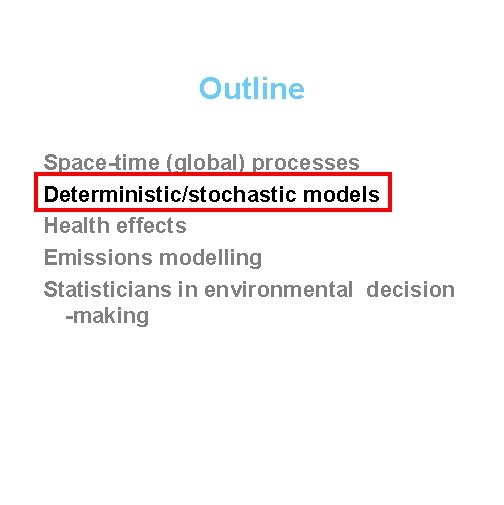
Outline Space-time (global) processes Deterministic/stochastic models Health effects Emissions modelling Statisticians in environmental decision -making
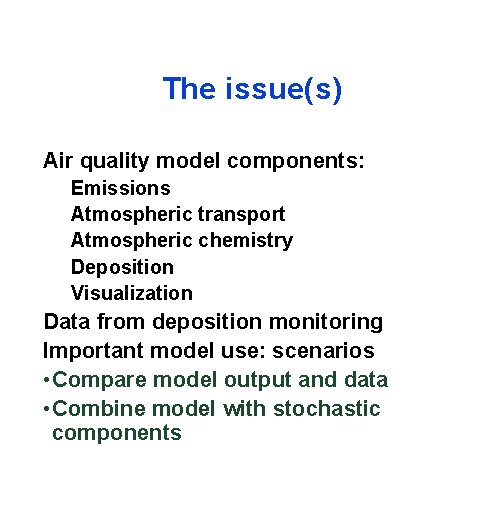
The issue(s) Air quality model components: Emissions Atmospheric transport Atmospheric chemistry Deposition Visualization Data from deposition monitoring Important model use: scenarios • Compare model output and data • Combine model with stochastic components
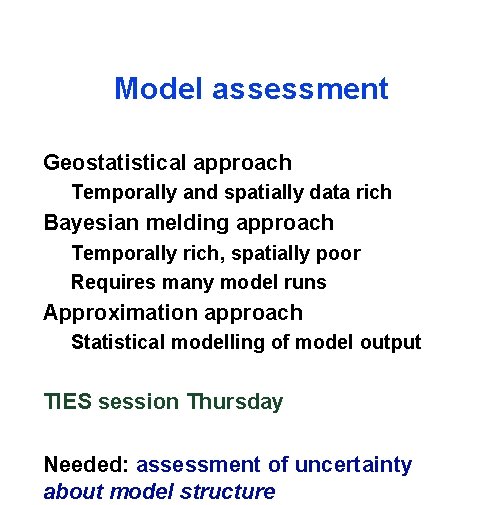
Model assessment Geostatistical approach Temporally and spatially data rich Bayesian melding approach Temporally rich, spatially poor Requires many model runs Approximation approach Statistical modelling of model output TIES session Thursday Needed: assessment of uncertainty about model structure
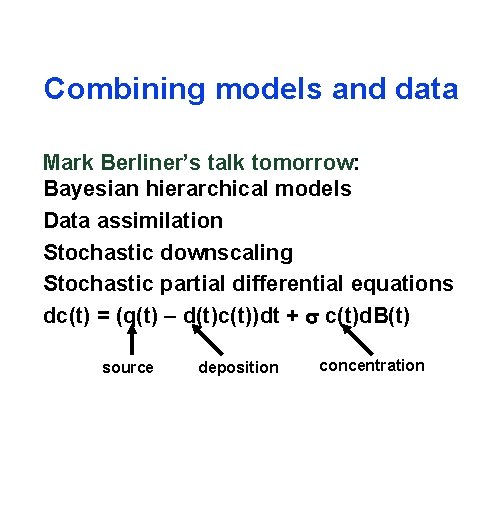
Combining models and data Mark Berliner’s talk tomorrow: Bayesian hierarchical models Data assimilation Stochastic downscaling Stochastic partial differential equations dc(t) = (q(t) – d(t)c(t))dt + s c(t)d. B(t) source deposition concentration
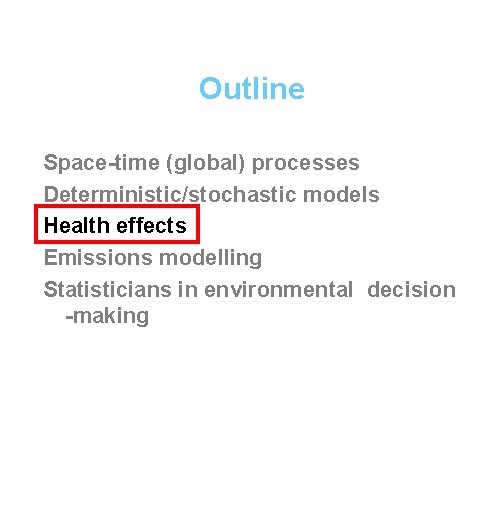
Outline Space-time (global) processes Deterministic/stochastic models Health effects Emissions modelling Statisticians in environmental decision -making
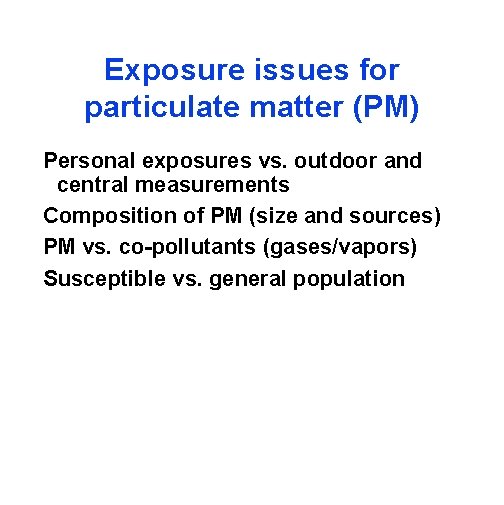
Exposure issues for particulate matter (PM) Personal exposures vs. outdoor and central measurements Composition of PM (size and sources) PM vs. co-pollutants (gases/vapors) Susceptible vs. general population
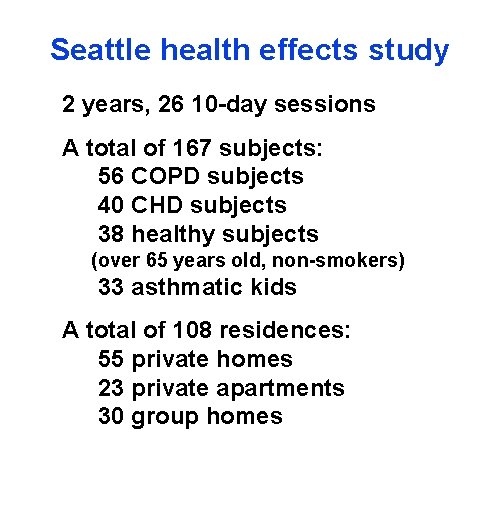
Seattle health effects study 2 years, 26 10 -day sessions A total of 167 subjects: 56 COPD subjects 40 CHD subjects 38 healthy subjects (over 65 years old, non-smokers) 33 asthmatic kids A total of 108 residences: 55 private homes 23 private apartments 30 group homes
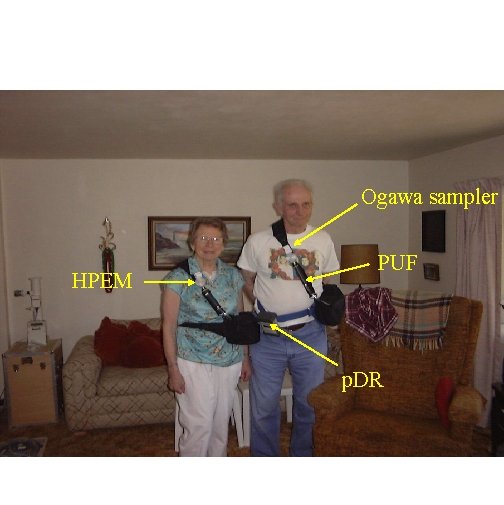
Ogawa sampler HPEM PUF p. DR
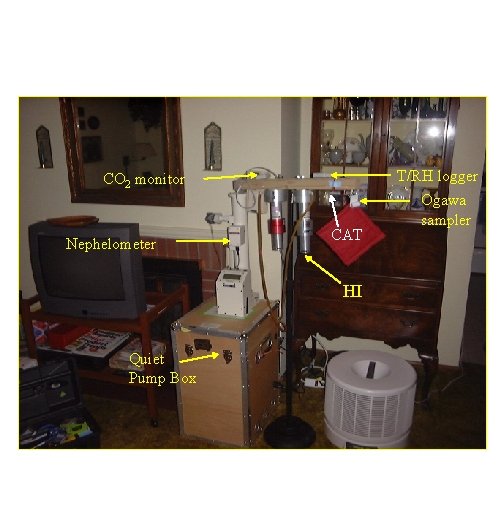
CO 2 monitor Nephelometer CAT HI Quiet Pump Box T/RH logger Ogawa sampler
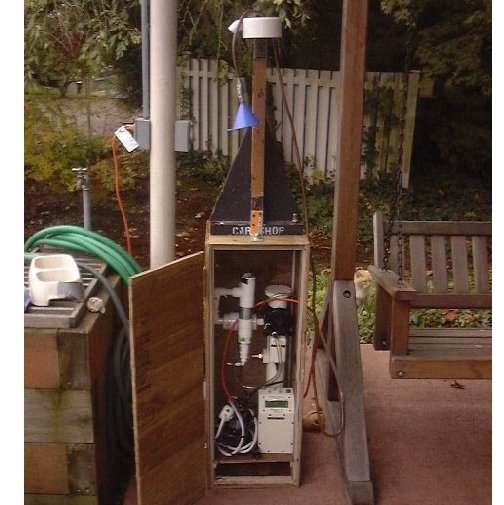
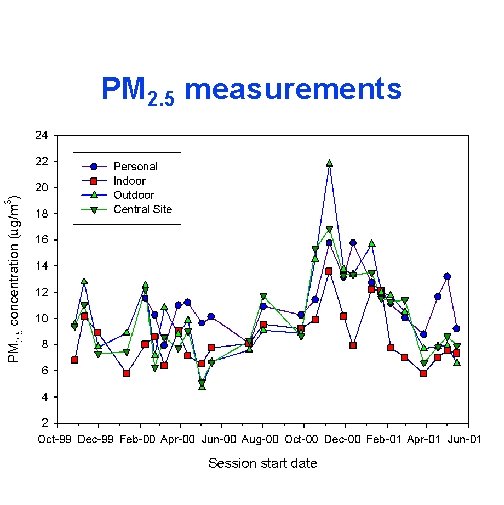
PM 2. 5 measurements
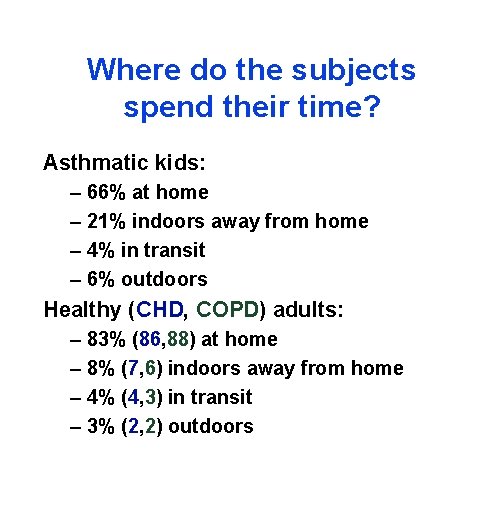
Where do the subjects spend their time? Asthmatic kids: – 66% at home – 21% indoors away from home – 4% in transit – 6% outdoors Healthy (CHD, COPD) adults: – 83% (86, 88) at home – 8% (7, 6) indoors away from home – 4% (4, 3) in transit – 3% (2, 2) outdoors
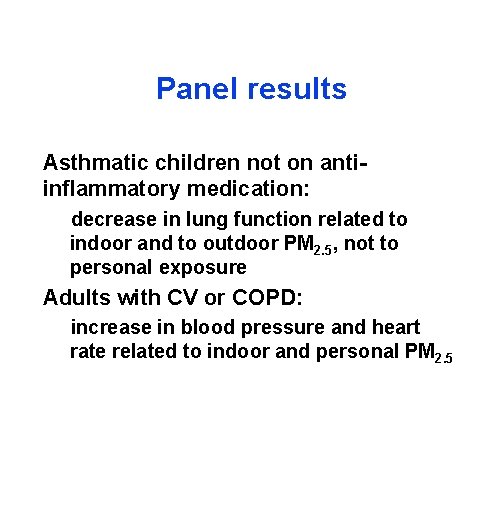
Panel results Asthmatic children not on antiinflammatory medication: decrease in lung function related to indoor and to outdoor PM 2. 5, not to personal exposure Adults with CV or COPD: increase in blood pressure and heart rate related to indoor and personal PM 2. 5
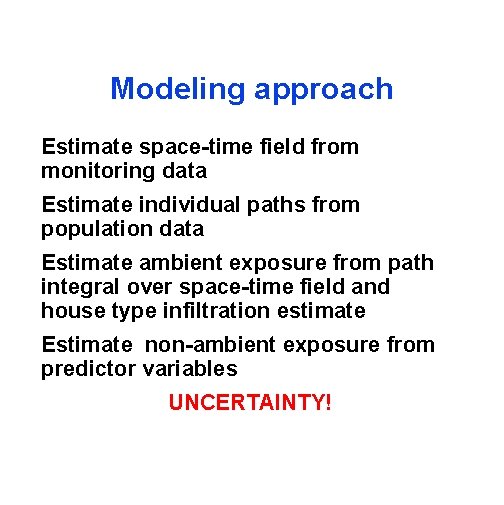
Modeling approach Estimate space-time field from monitoring data Estimate individual paths from population data Estimate ambient exposure from path integral over space-time field and house type infiltration estimate Estimate non-ambient exposure from predictor variables UNCERTAINTY!
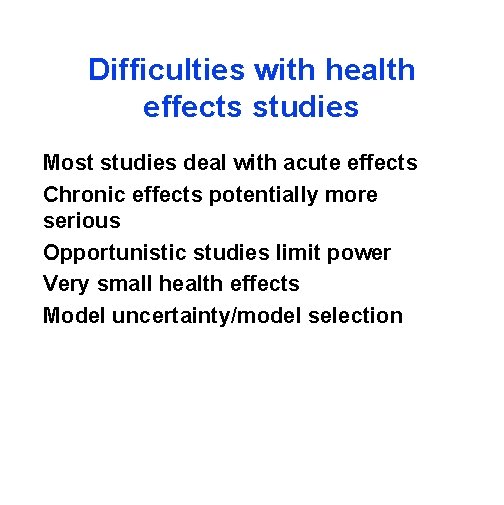
Difficulties with health effects studies Most studies deal with acute effects Chronic effects potentially more serious Opportunistic studies limit power Very small health effects Model uncertainty/model selection
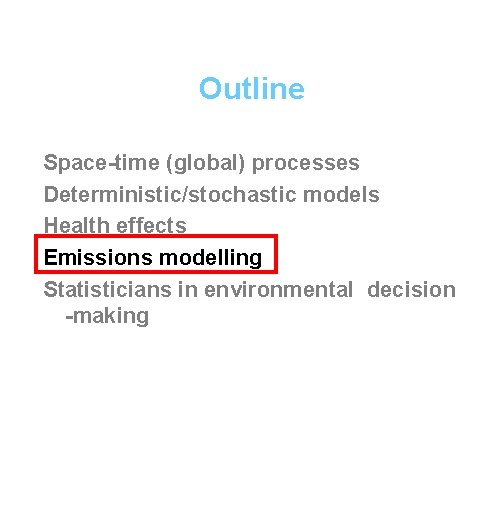
Outline Space-time (global) processes Deterministic/stochastic models Health effects Emissions modelling Statisticians in environmental decision -making
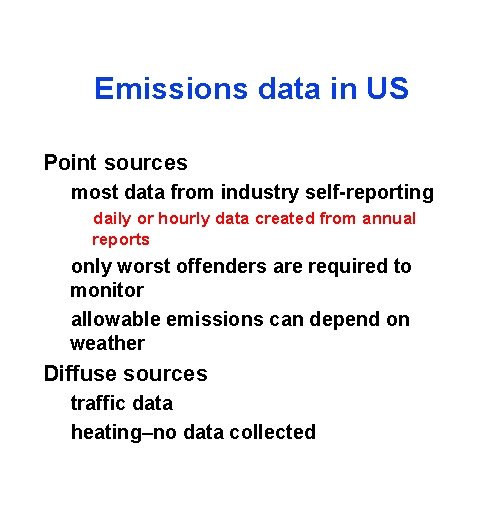
Emissions data in US Point sources most data from industry self-reporting daily or hourly data created from annual reports only worst offenders are required to monitor allowable emissions can depend on weather Diffuse sources traffic data heating–no data collected
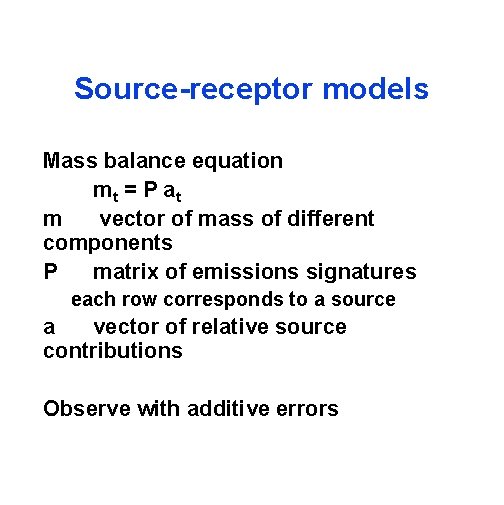
Source-receptor models Mass balance equation mt = P a t m vector of mass of different components P matrix of emissions signatures each row corresponds to a source a vector of relative source contributions Observe with additive errors
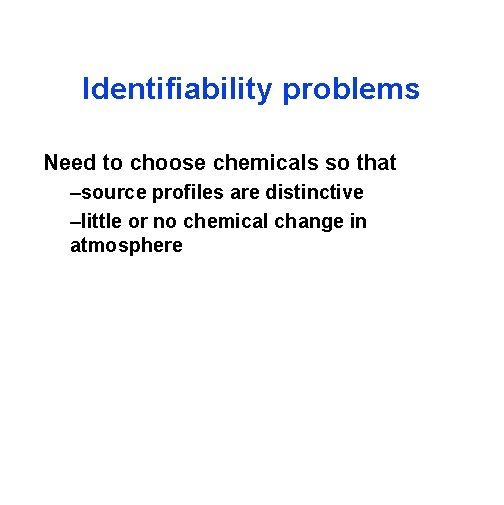
Identifiability problems Need to choose chemicals so that –source profiles are distinctive –little or no chemical change in atmosphere
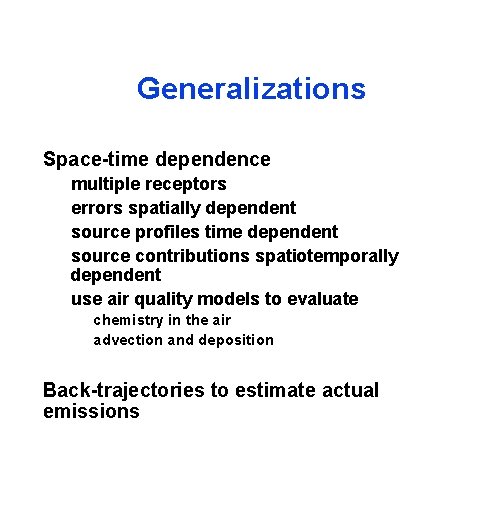
Generalizations Space-time dependence multiple receptors errors spatially dependent source profiles time dependent source contributions spatiotemporally dependent use air quality models to evaluate chemistry in the air advection and deposition Back-trajectories to estimate actual emissions
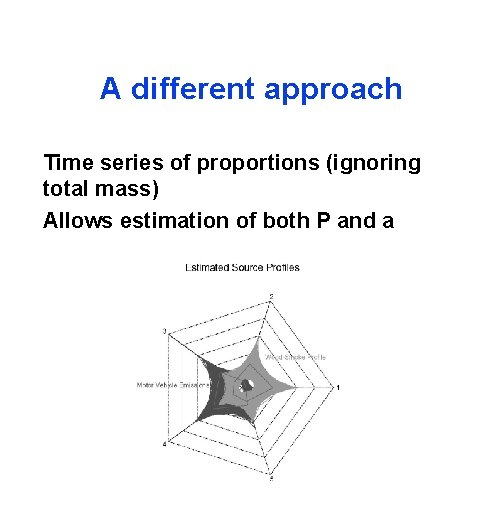
A different approach Time series of proportions (ignoring total mass) Allows estimation of both P and a
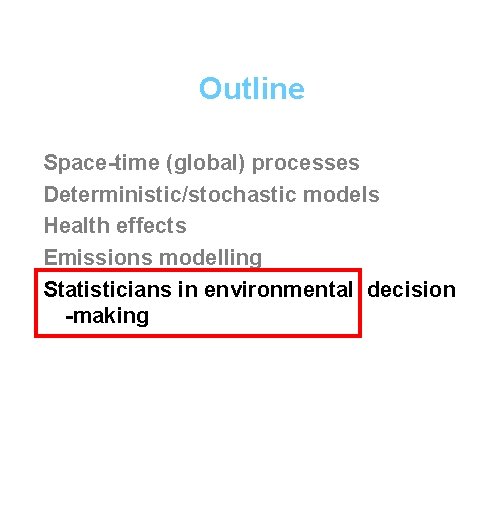
Outline Space-time (global) processes Deterministic/stochastic models Health effects Emissions modelling Statisticians in environmental decision -making
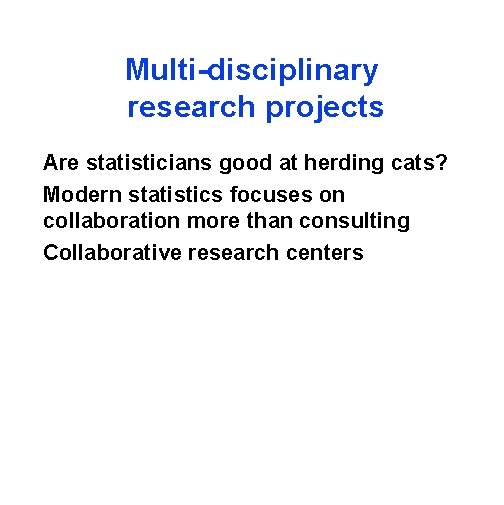
Multi-disciplinary research projects Are statisticians good at herding cats? Modern statistics focuses on collaboration more than consulting Collaborative research centers
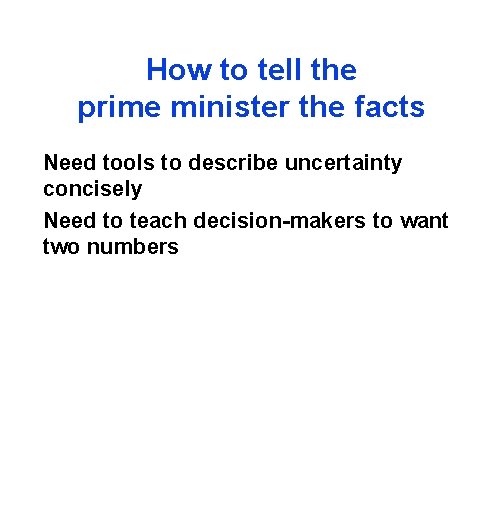
How to tell the prime minister the facts Need tools to describe uncertainty concisely Need to teach decision-makers to want two numbers
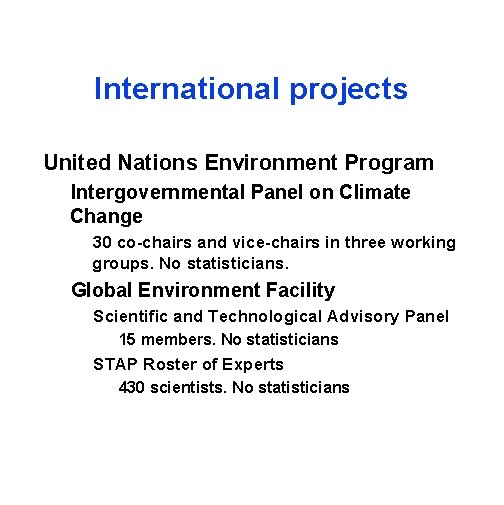
International projects United Nations Environment Program Intergovernmental Panel on Climate Change 30 co-chairs and vice-chairs in three working groups. No statisticians. Global Environment Facility Scientific and Technological Advisory Panel 15 members. No statisticians STAP Roster of Experts 430 scientists. No statisticians
Tic tac toe going high going low going criss cross lollipop
If you are going through hell, keep going
"environmental efficiency" "environmental monitoring"
Multivariate statistics for the environmental sciences
Introduction to statistics what is statistics
What's she going to do
Oraciones simples en futuro
To lucasta, going to the wars
Where is anne frank buried
Bear hunt by dr jean
Simple present tense pattern
Materi future tense
Penetration price
Going concern entity concept
Ionisation energy definition
A struggle between a character and an outside force
How did the vikings know where they were going
Tudor bowen-jones is going to spend his 90th birthday
Future with will
Be going to examples
Verb going to
Going back in order to go forward
He is going to travel
Jack is going skiing
Five friends decided on going to world destination
We aren't/we're not going to miss the train
Trend going down
Going to see king lear jackie kay
Draw a cell having 6 chromosomes that undergoes meiosis
Don manuel camus
International compensation approaches
Captive product pricing strategy
Trend going down
Going on concern principle
Can you guess who we are
2010
Accountin principles