Multiple flavors of mass analyzers Single MS peptide
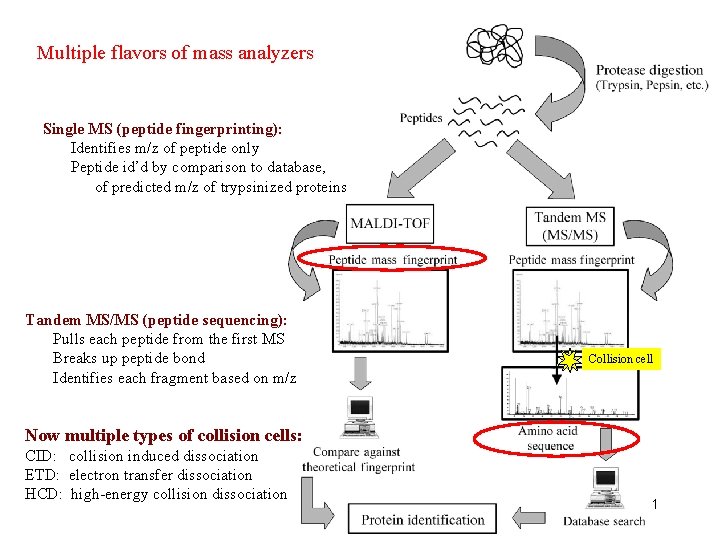
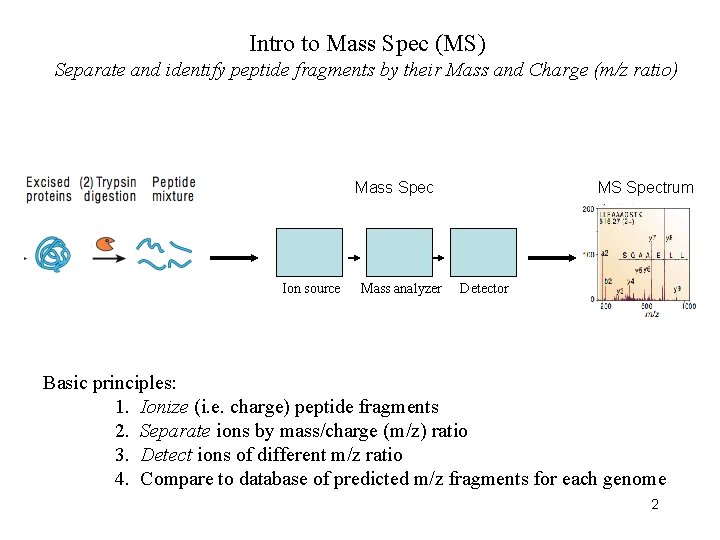
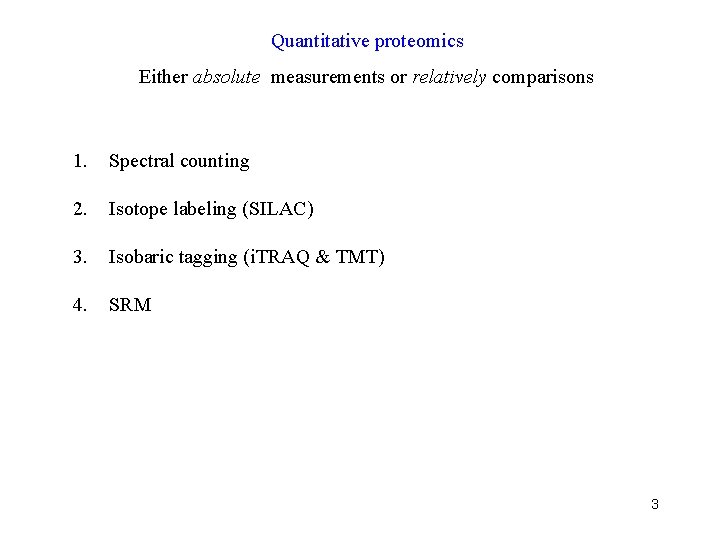
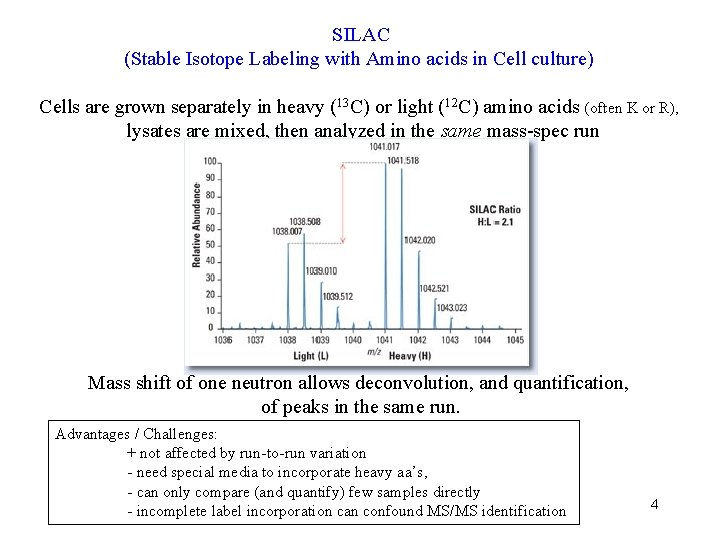
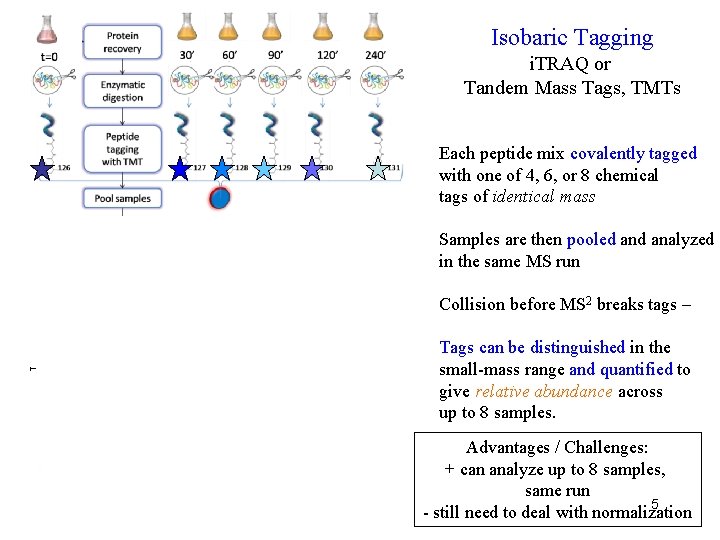
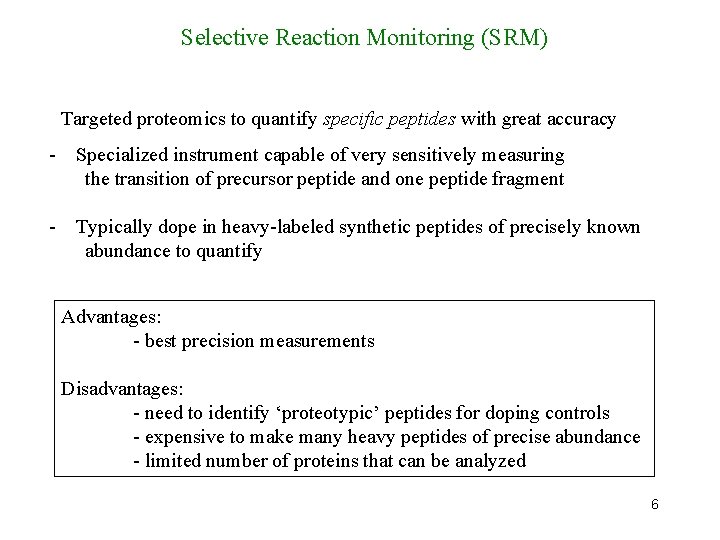
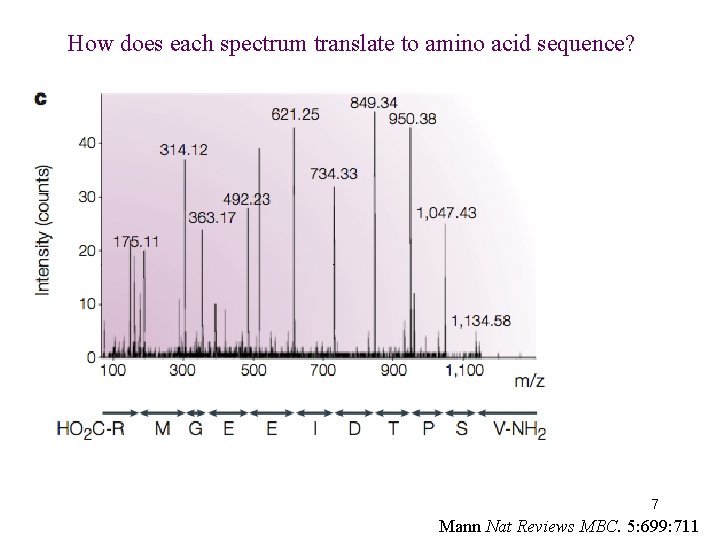
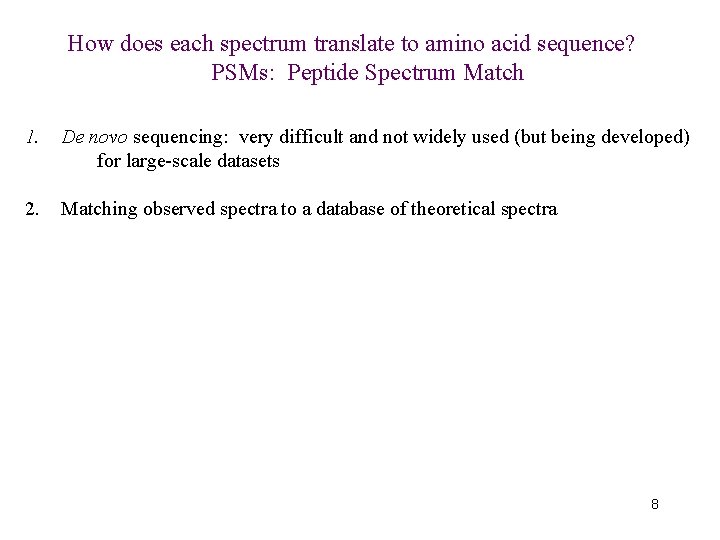
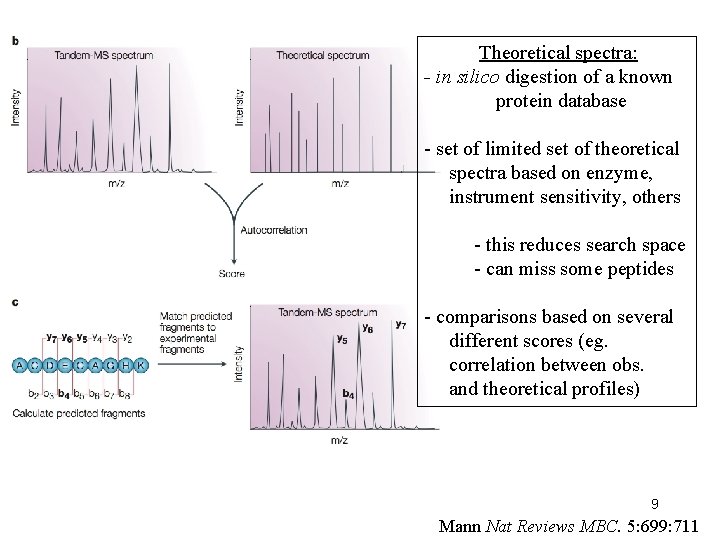
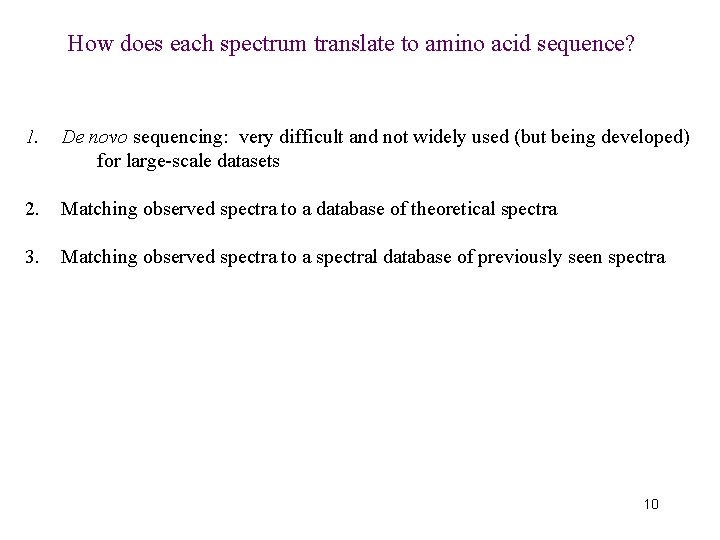
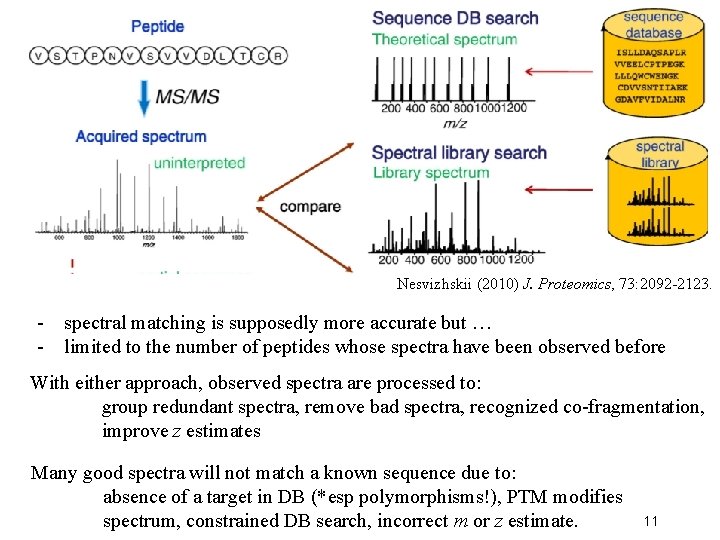
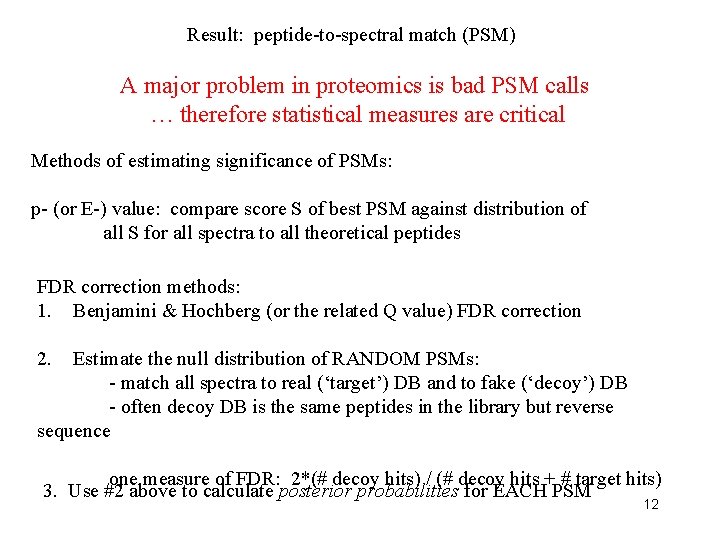
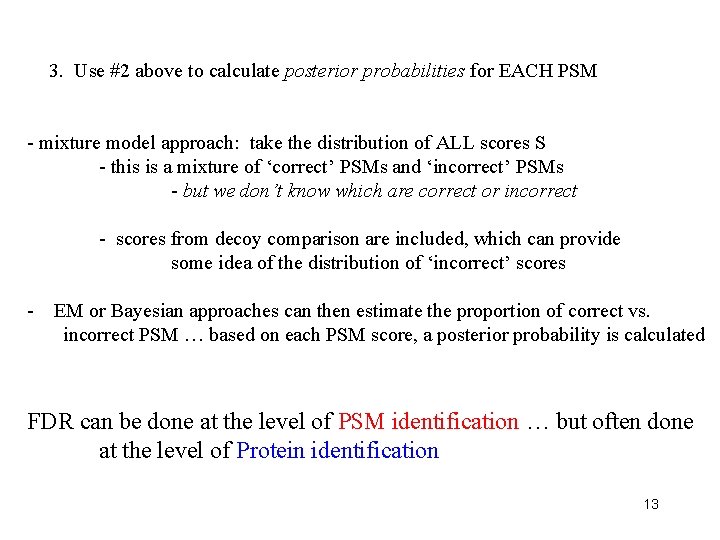
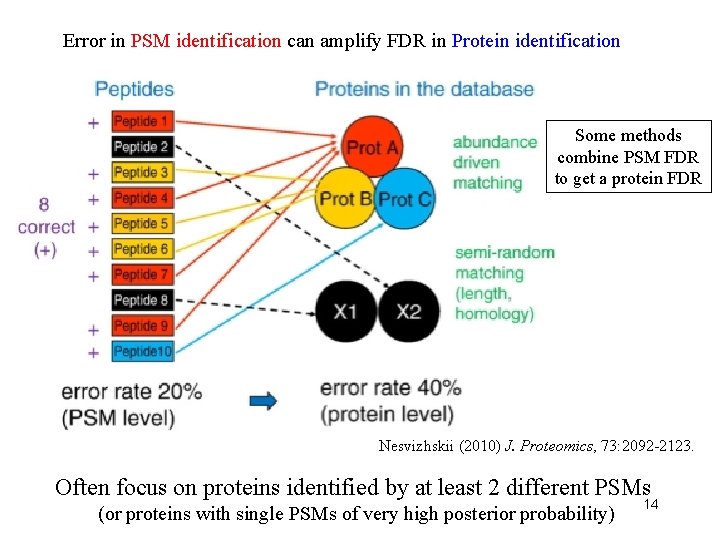
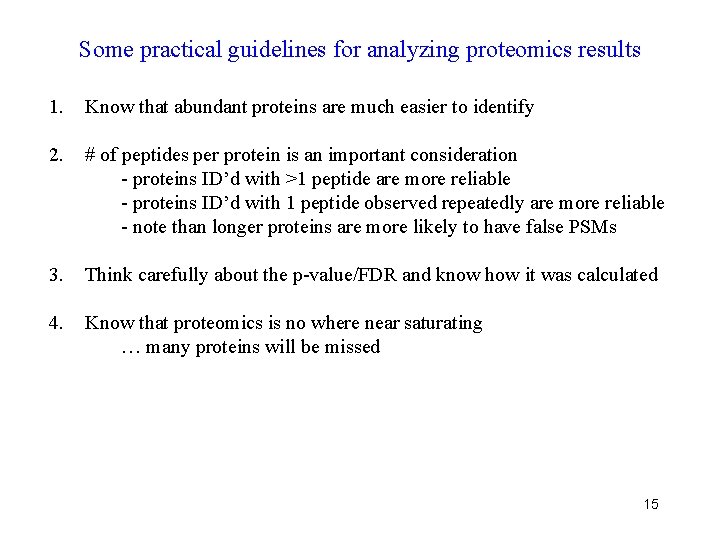
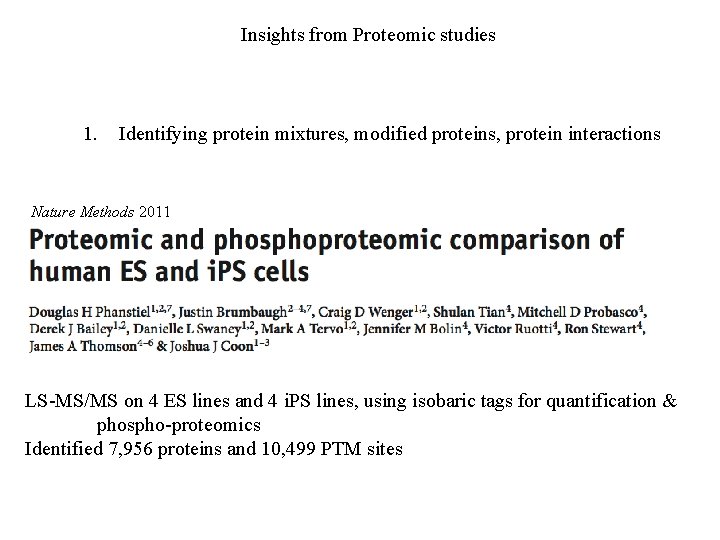
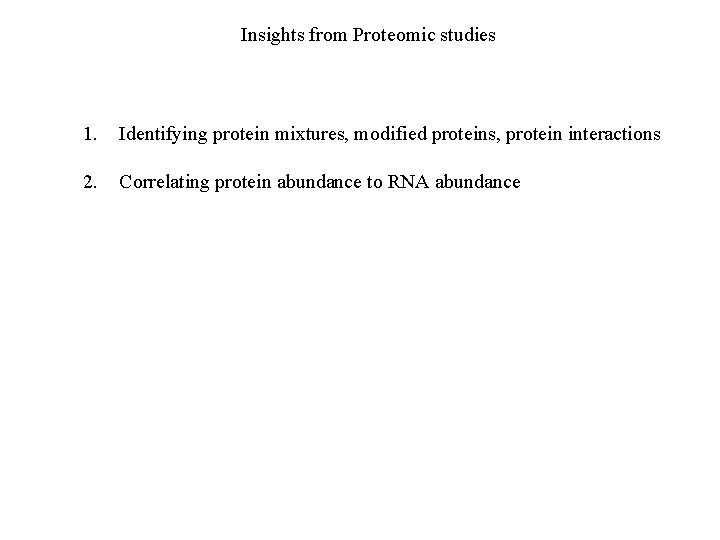
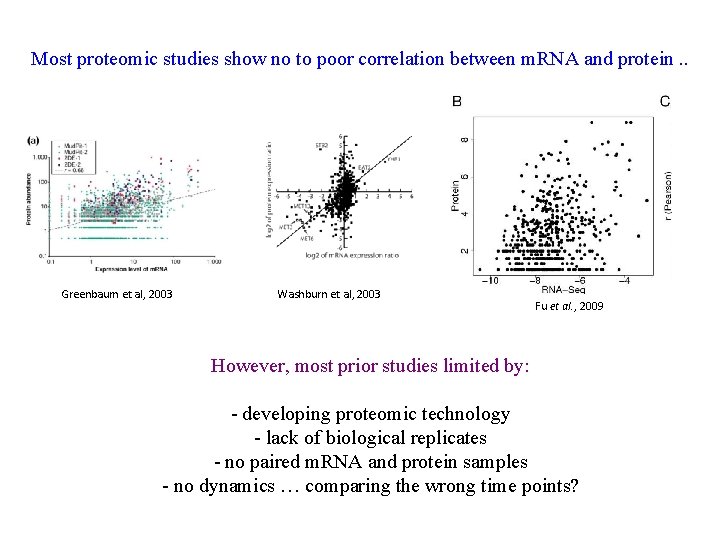
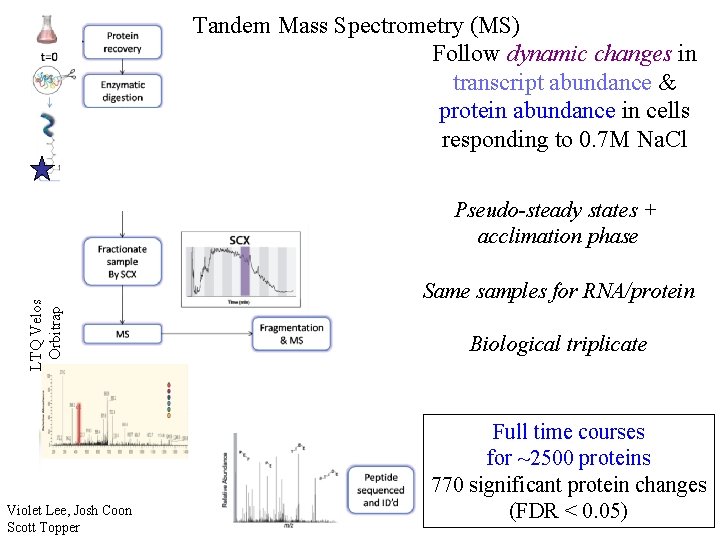
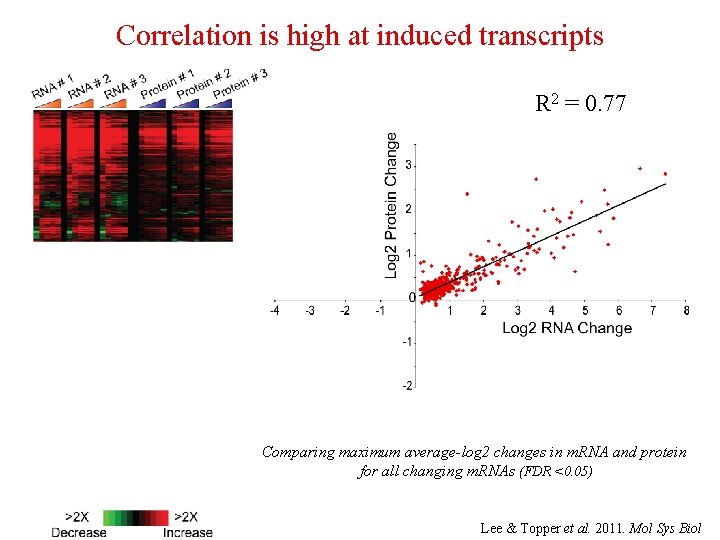
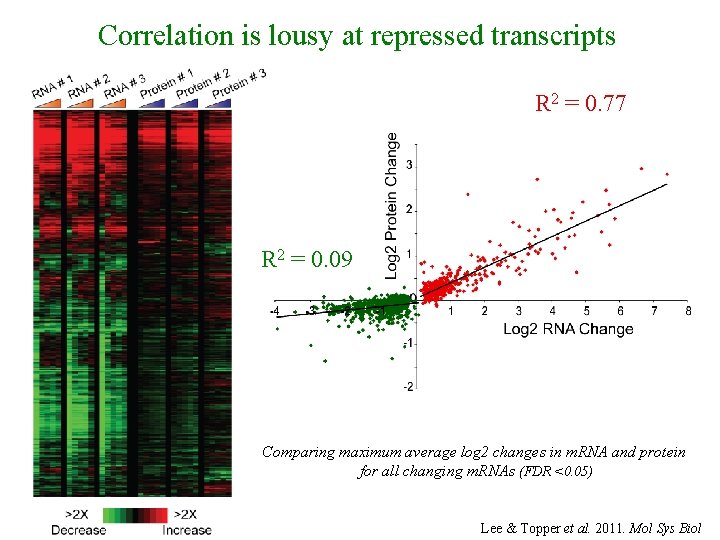
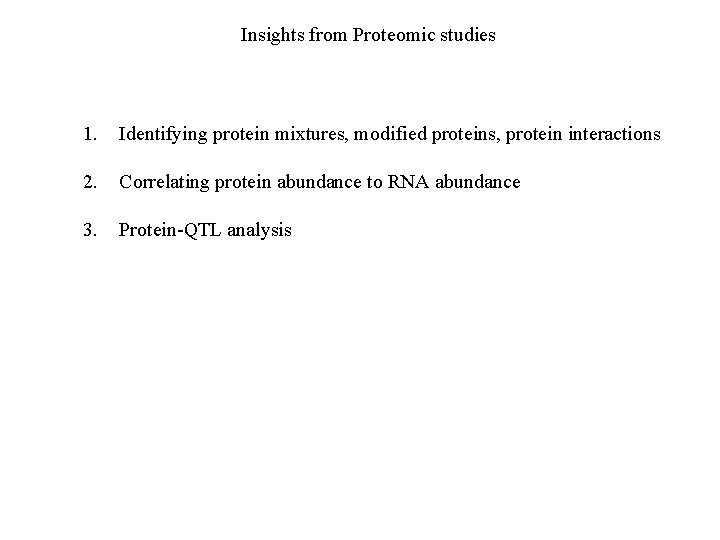
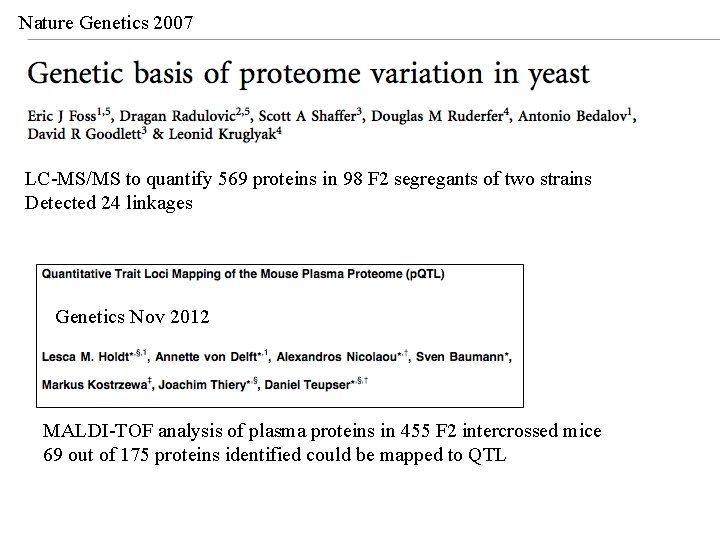
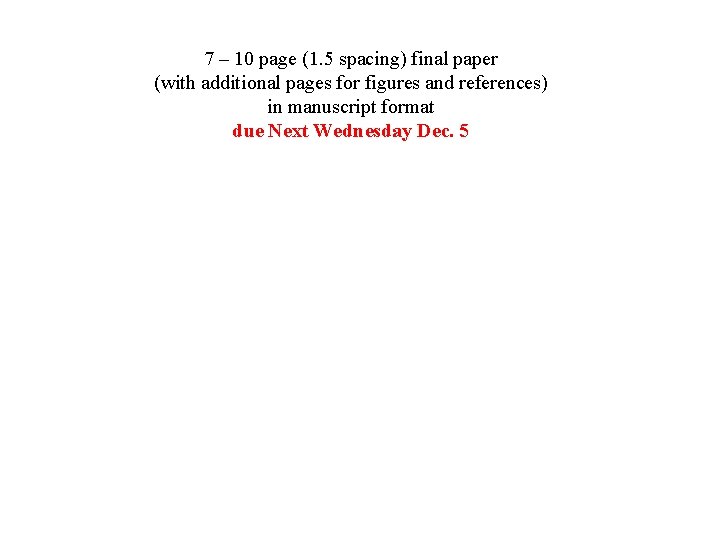
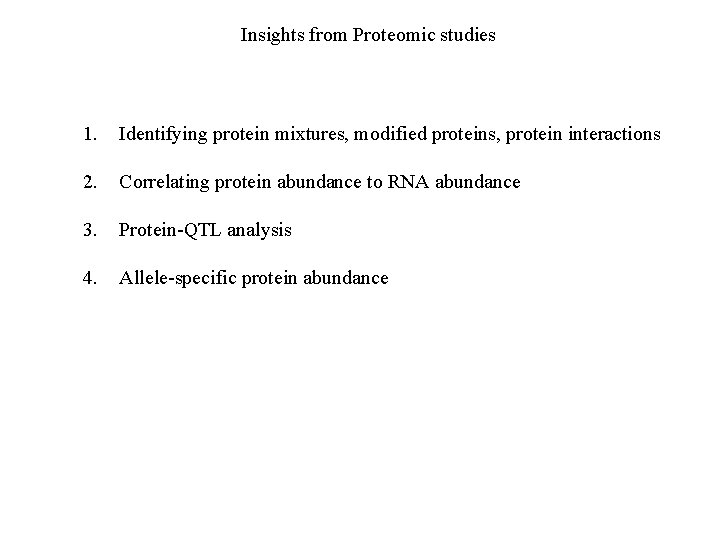
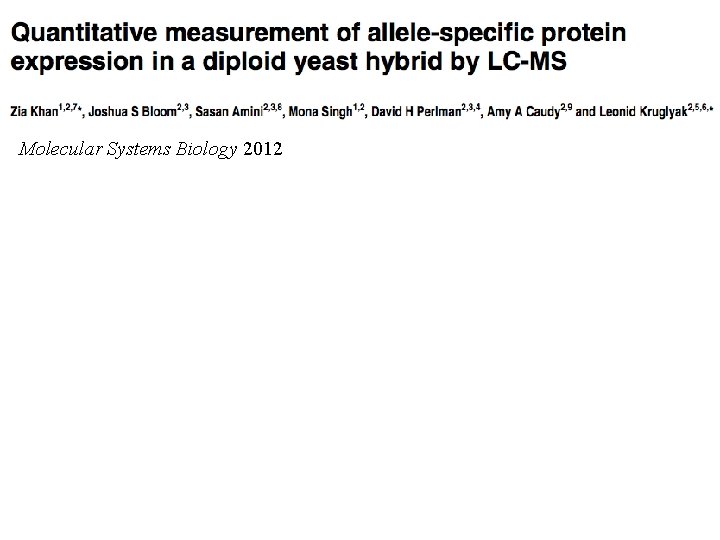
- Slides: 26
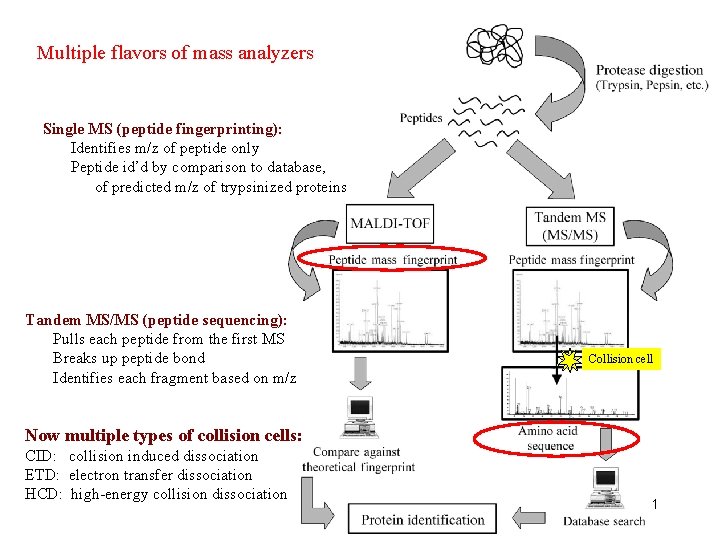
Multiple flavors of mass analyzers Single MS (peptide fingerprinting): Identifies m/z of peptide only Peptide id’d by comparison to database, of predicted m/z of trypsinized proteins Tandem MS/MS (peptide sequencing): Pulls each peptide from the first MS Breaks up peptide bond Identifies each fragment based on m/z Collision cell Now multiple types of collision cells: CID: collision induced dissociation ETD: electron transfer dissociation HCD: high-energy collision dissociation 1
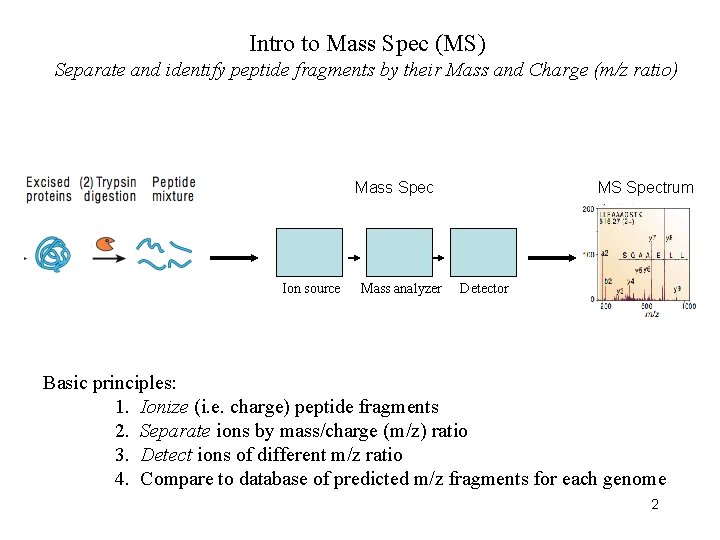
Intro to Mass Spec (MS) Separate and identify peptide fragments by their Mass and Charge (m/z ratio) Mass Spec Ion source Mass analyzer MS Spectrum Detector Basic principles: 1. Ionize (i. e. charge) peptide fragments 2. Separate ions by mass/charge (m/z) ratio 3. Detect ions of different m/z ratio 4. Compare to database of predicted m/z fragments for each genome 2
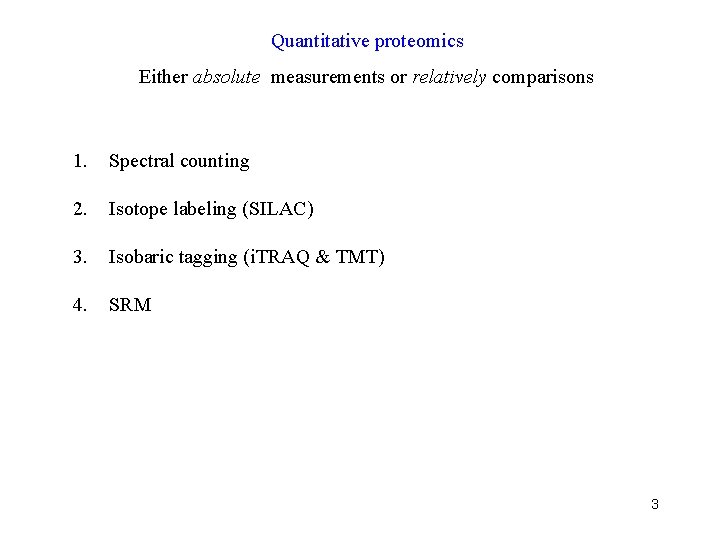
Quantitative proteomics Either absolute measurements or relatively comparisons 1. Spectral counting 2. Isotope labeling (SILAC) 3. Isobaric tagging (i. TRAQ & TMT) 4. SRM 3
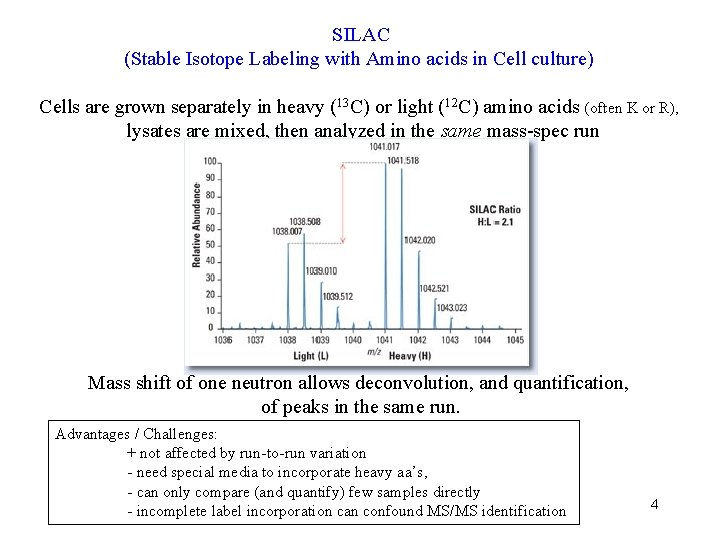
SILAC (Stable Isotope Labeling with Amino acids in Cell culture) Cells are grown separately in heavy (13 C) or light (12 C) amino acids (often K or R), lysates are mixed, then analyzed in the same mass-spec run Mass shift of one neutron allows deconvolution, and quantification, of peaks in the same run. Advantages / Challenges: + not affected by run-to-run variation - need special media to incorporate heavy aa’s, - can only compare (and quantify) few samples directly - incomplete label incorporation can confound MS/MS identification 4
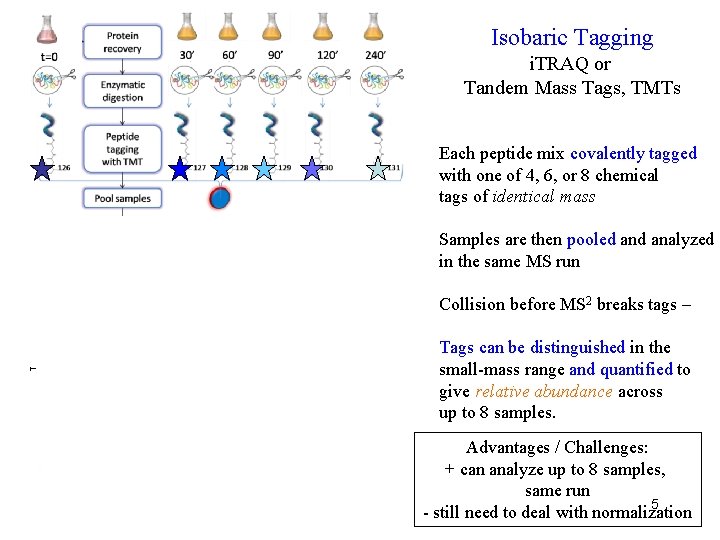
Isobaric Tagging i. TRAQ or Tandem Mass Tags, TMTs Each peptide mix covalently tagged with one of 4, 6, or 8 chemical tags of identical mass LTQ Velos Orbitrap Samples are then pooled analyzed in the same MS run Collision before MS 2 breaks tags – Tags can be distinguished in the small-mass range and quantified to give relative abundance across up to 8 samples. Advantages / Challenges: + can analyze up to 8 samples, same run 5 - still need to deal with normalization
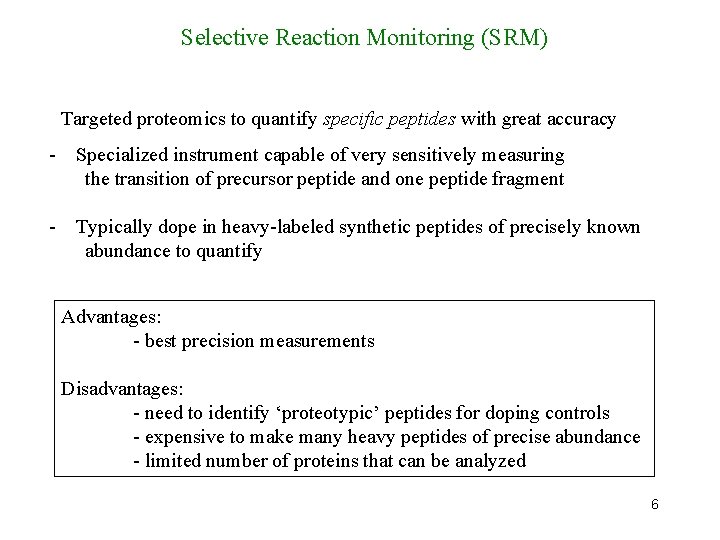
Selective Reaction Monitoring (SRM) Targeted proteomics to quantify specific peptides with great accuracy - Specialized instrument capable of very sensitively measuring the transition of precursor peptide and one peptide fragment - Typically dope in heavy-labeled synthetic peptides of precisely known abundance to quantify Advantages: - best precision measurements Disadvantages: - need to identify ‘proteotypic’ peptides for doping controls - expensive to make many heavy peptides of precise abundance - limited number of proteins that can be analyzed 6
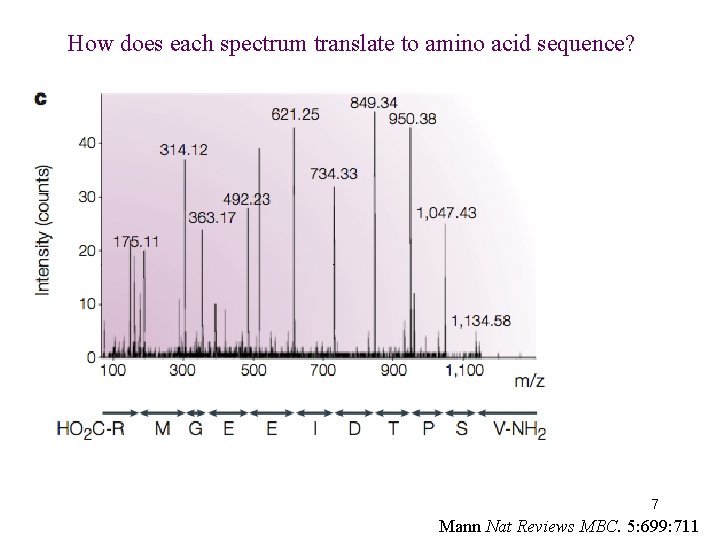
How does each spectrum translate to amino acid sequence? 7 Mann Nat Reviews MBC. 5: 699: 711
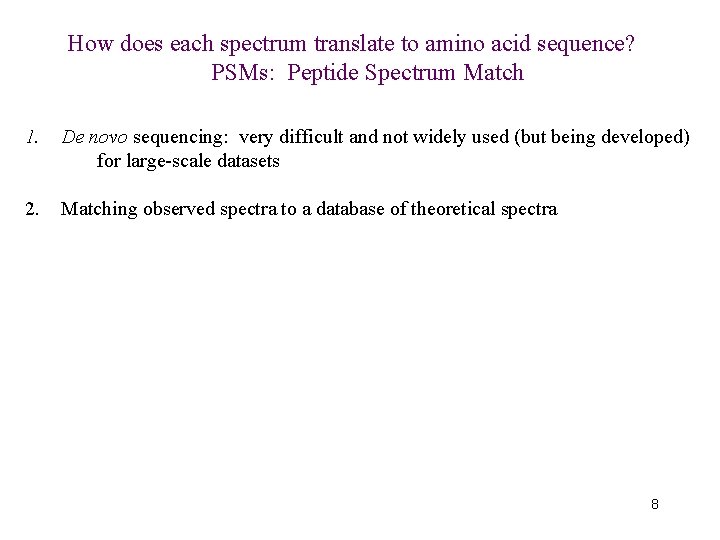
How does each spectrum translate to amino acid sequence? PSMs: Peptide Spectrum Match 1. De novo sequencing: very difficult and not widely used (but being developed) for large-scale datasets 2. Matching observed spectra to a database of theoretical spectra 8
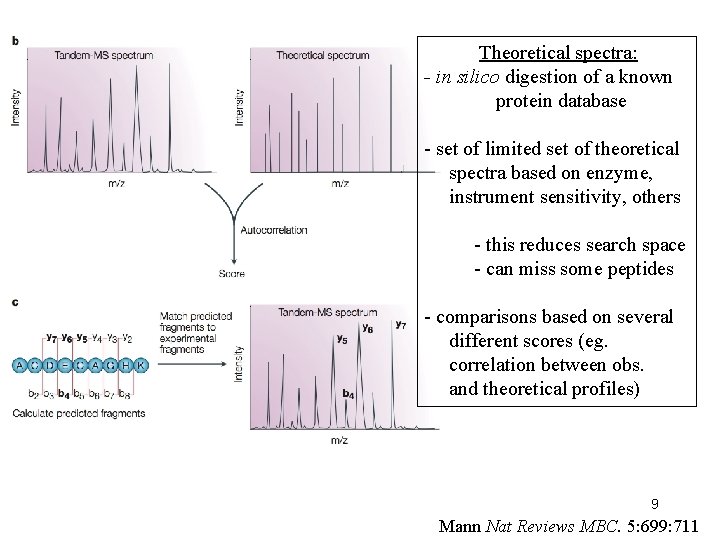
Theoretical spectra: - in silico digestion of a known protein database - set of limited set of theoretical spectra based on enzyme, instrument sensitivity, others - this reduces search space - can miss some peptides - comparisons based on several different scores (eg. correlation between obs. and theoretical profiles) 9 Mann Nat Reviews MBC. 5: 699: 711
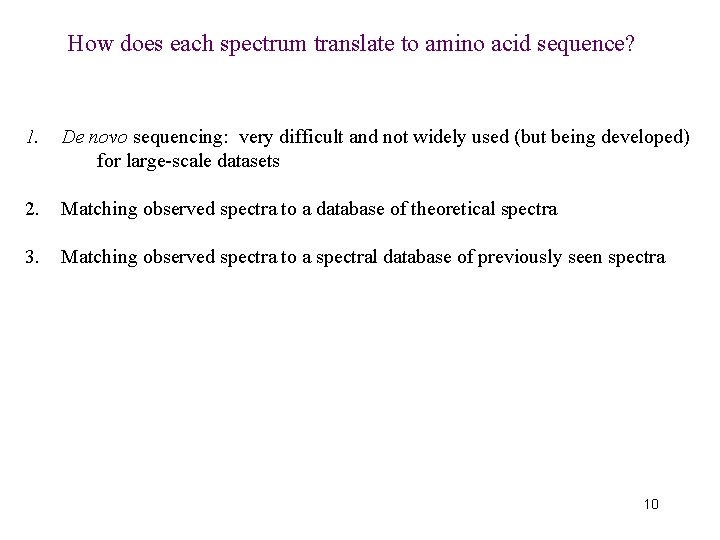
How does each spectrum translate to amino acid sequence? 1. De novo sequencing: very difficult and not widely used (but being developed) for large-scale datasets 2. Matching observed spectra to a database of theoretical spectra 3. Matching observed spectra to a spectral database of previously seen spectra 10
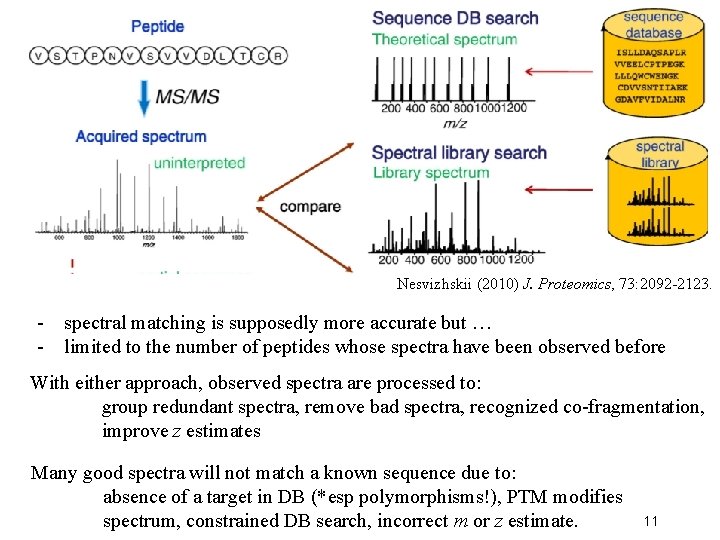
Nesvizhskii (2010) J. Proteomics, 73: 2092 -2123. - spectral matching is supposedly more accurate but … - limited to the number of peptides whose spectra have been observed before With either approach, observed spectra are processed to: group redundant spectra, remove bad spectra, recognized co-fragmentation, improve z estimates Many good spectra will not match a known sequence due to: absence of a target in DB (*esp polymorphisms!), PTM modifies spectrum, constrained DB search, incorrect m or z estimate. 11
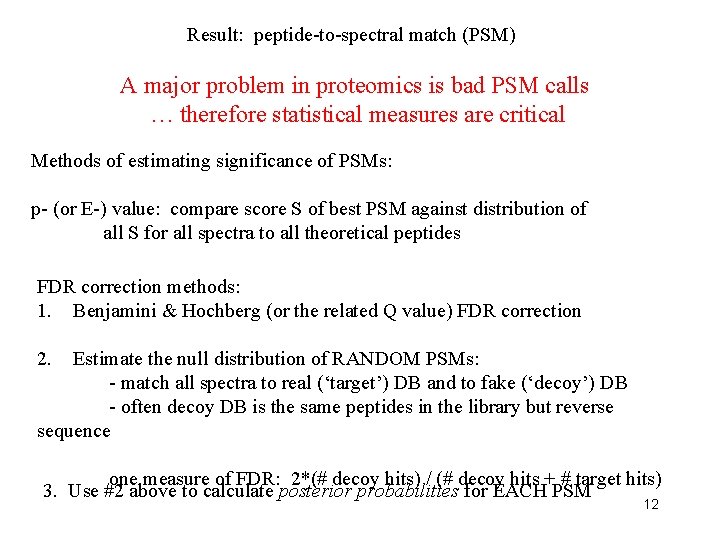
Result: peptide-to-spectral match (PSM) A major problem in proteomics is bad PSM calls … therefore statistical measures are critical Methods of estimating significance of PSMs: p- (or E-) value: compare score S of best PSM against distribution of all S for all spectra to all theoretical peptides FDR correction methods: 1. Benjamini & Hochberg (or the related Q value) FDR correction 2. Estimate the null distribution of RANDOM PSMs: - match all spectra to real (‘target’) DB and to fake (‘decoy’) DB - often decoy DB is the same peptides in the library but reverse sequence one measure of FDR: 2*(# decoy hits) / (# decoy hits + # target hits) 3. Use #2 above to calculate posterior probabilities for EACH PSM 12
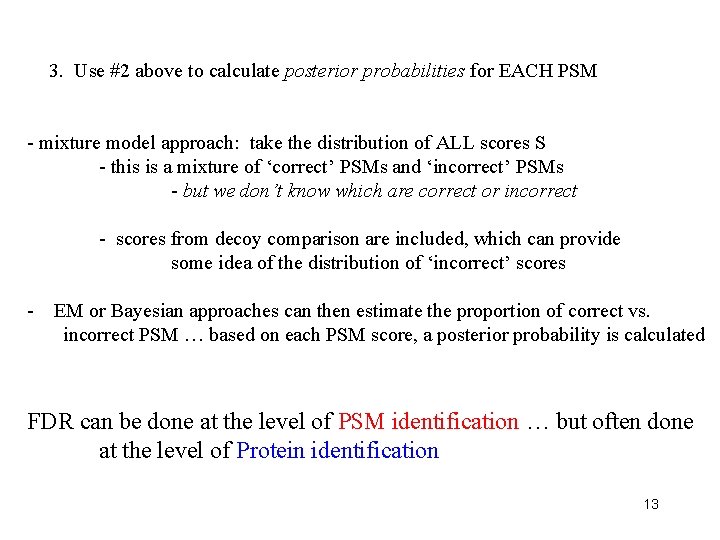
3. Use #2 above to calculate posterior probabilities for EACH PSM - mixture model approach: take the distribution of ALL scores S - this is a mixture of ‘correct’ PSMs and ‘incorrect’ PSMs - but we don’t know which are correct or incorrect - scores from decoy comparison are included, which can provide some idea of the distribution of ‘incorrect’ scores - EM or Bayesian approaches can then estimate the proportion of correct vs. incorrect PSM … based on each PSM score, a posterior probability is calculated FDR can be done at the level of PSM identification … but often done at the level of Protein identification 13
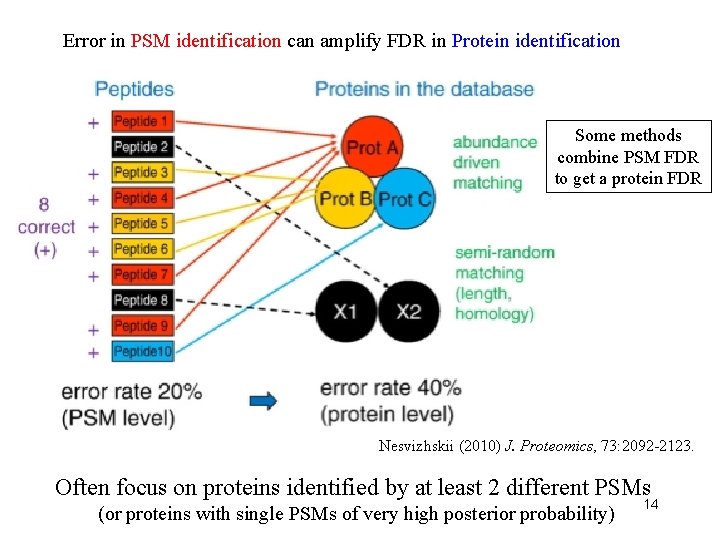
Error in PSM identification can amplify FDR in Protein identification Some methods combine PSM FDR to get a protein FDR Nesvizhskii (2010) J. Proteomics, 73: 2092 -2123. Often focus on proteins identified by at least 2 different PSMs (or proteins with single PSMs of very high posterior probability) 14
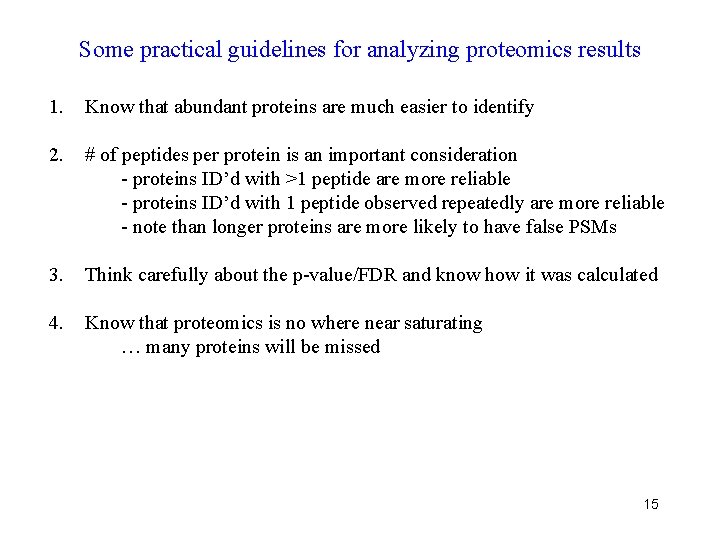
Some practical guidelines for analyzing proteomics results 1. Know that abundant proteins are much easier to identify 2. # of peptides per protein is an important consideration - proteins ID’d with >1 peptide are more reliable - proteins ID’d with 1 peptide observed repeatedly are more reliable - note than longer proteins are more likely to have false PSMs 3. Think carefully about the p-value/FDR and know how it was calculated 4. Know that proteomics is no where near saturating … many proteins will be missed 15
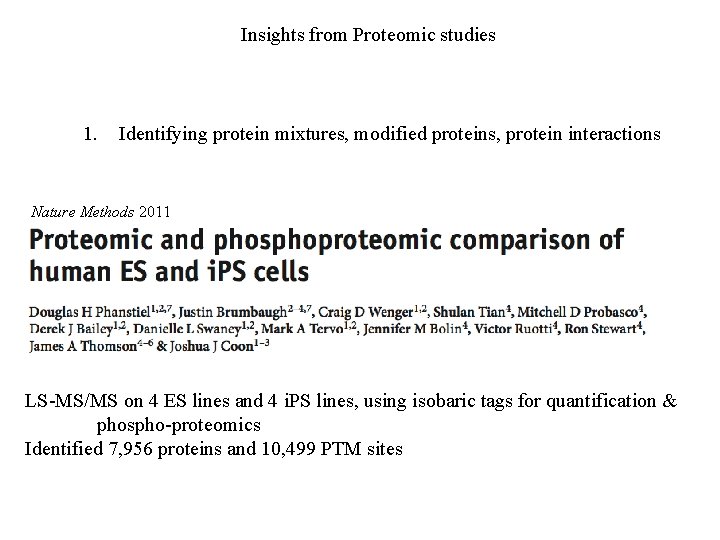
Insights from Proteomic studies 1. Identifying protein mixtures, modified proteins, protein interactions Nature Methods 2011 LS-MS/MS on 4 ES lines and 4 i. PS lines, using isobaric tags for quantification & phospho-proteomics Identified 7, 956 proteins and 10, 499 PTM sites
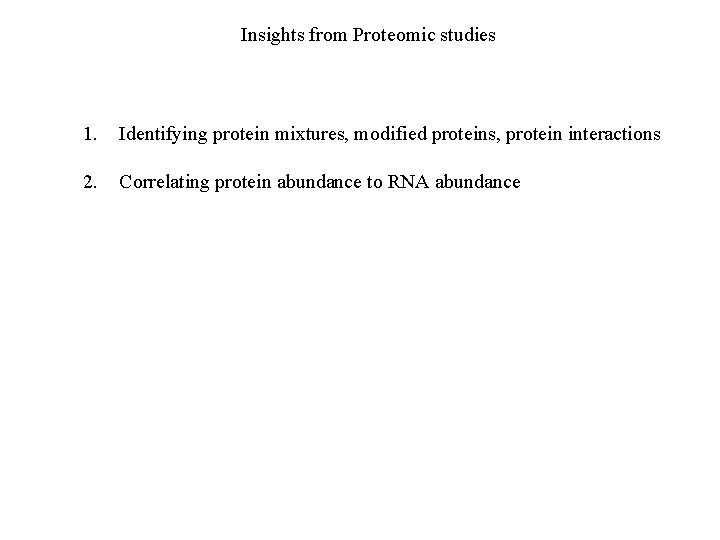
Insights from Proteomic studies 1. Identifying protein mixtures, modified proteins, protein interactions 2. Correlating protein abundance to RNA abundance
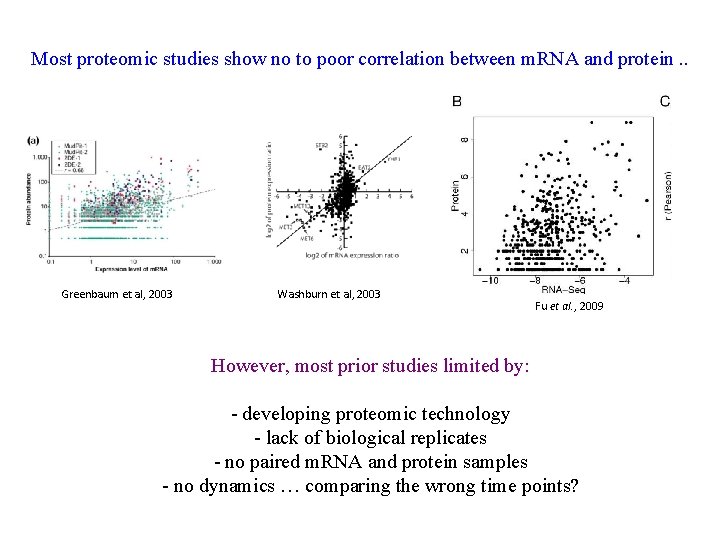
Most proteomic studies show no to poor correlation between m. RNA and protein. . Greenbaum et al, 2003 Washburn et al, 2003 Fu et al. , 2009 However, most prior studies limited by: - developing proteomic technology - lack of biological replicates - no paired m. RNA and protein samples - no dynamics … comparing the wrong time points?
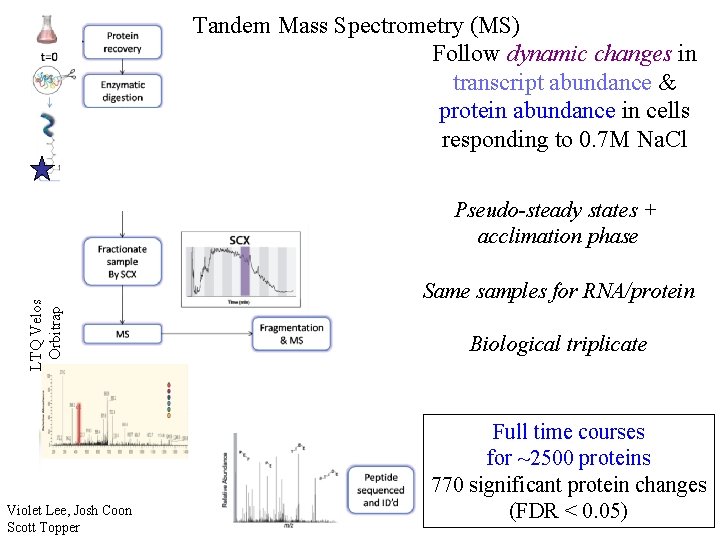
Tandem Mass Spectrometry (MS) Follow dynamic changes in transcript abundance & protein abundance in cells responding to 0. 7 M Na. Cl LTQ Velos Orbitrap Pseudo-steady states + acclimation phase Violet Lee, Josh Coon Scott Topper Same samples for RNA/protein Biological triplicate Full time courses for ~2500 proteins 770 significant protein changes (FDR < 0. 05)
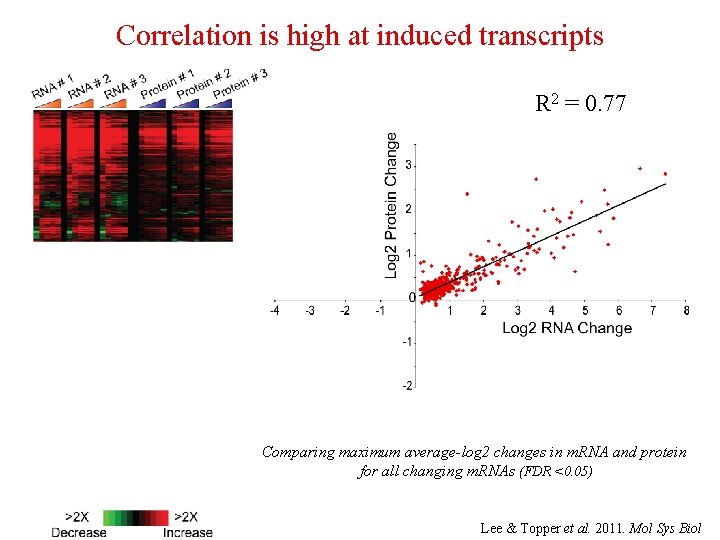
Correlation is high at induced transcripts R 2 = 0. 77 Comparing maximum average-log 2 changes in m. RNA and protein for all changing m. RNAs (FDR <0. 05) Lee & Topper et al. 2011. Mol Sys Biol
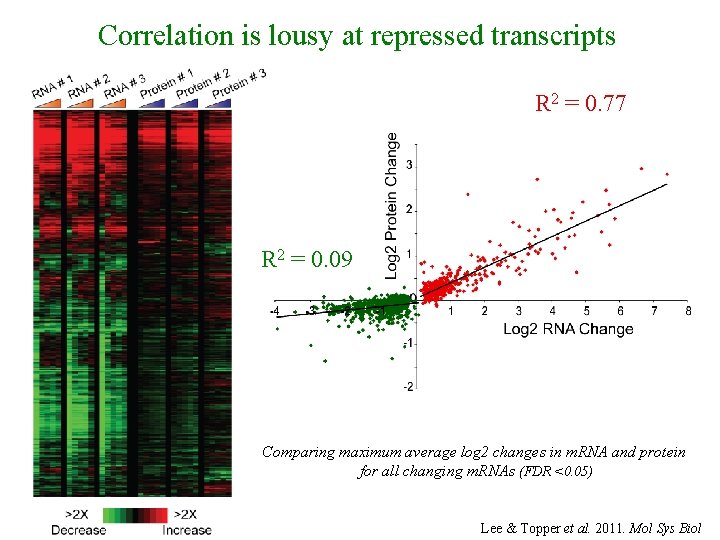
Correlation is lousy at repressed transcripts R 2 = 0. 77 R 2 = 0. 09 Comparing maximum average log 2 changes in m. RNA and protein for all changing m. RNAs (FDR <0. 05) Lee & Topper et al. 2011. Mol Sys Biol
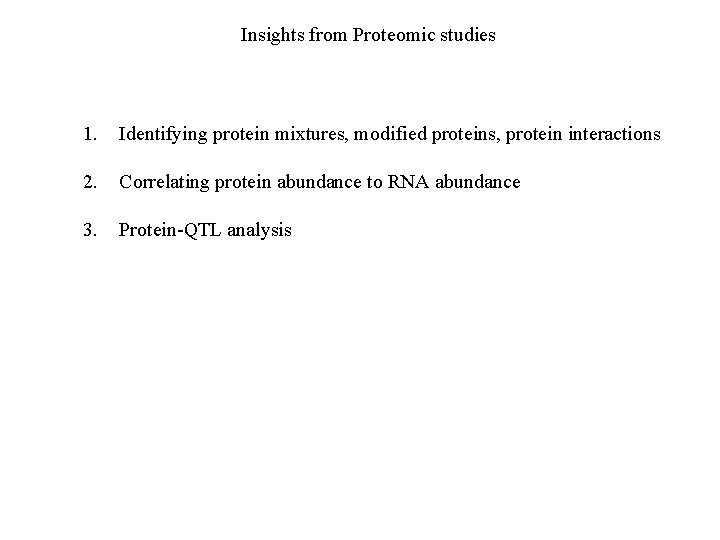
Insights from Proteomic studies 1. Identifying protein mixtures, modified proteins, protein interactions 2. Correlating protein abundance to RNA abundance 3. Protein-QTL analysis
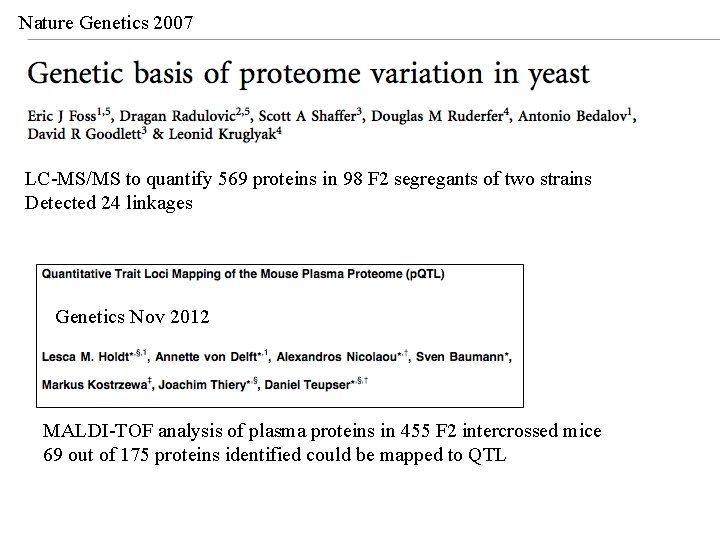
Nature Genetics 2007 LC-MS/MS to quantify 569 proteins in 98 F 2 segregants of two strains Detected 24 linkages Genetics Nov 2012 MALDI-TOF analysis of plasma proteins in 455 F 2 intercrossed mice 69 out of 175 proteins identified could be mapped to QTL
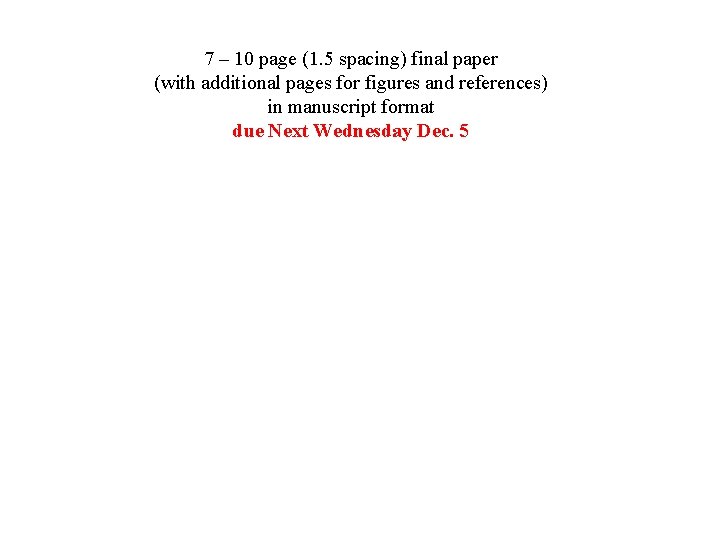
7 – 10 page (1. 5 spacing) final paper (with additional pages for figures and references) in manuscript format due Next Wednesday Dec. 5
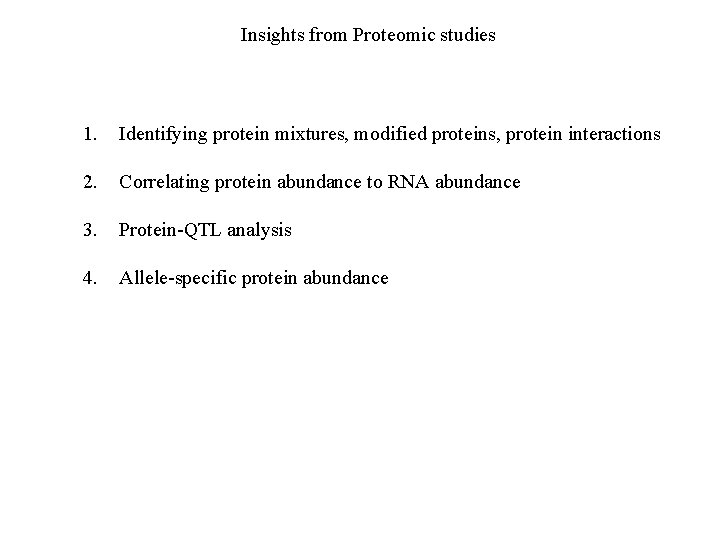
Insights from Proteomic studies 1. Identifying protein mixtures, modified proteins, protein interactions 2. Correlating protein abundance to RNA abundance 3. Protein-QTL analysis 4. Allele-specific protein abundance
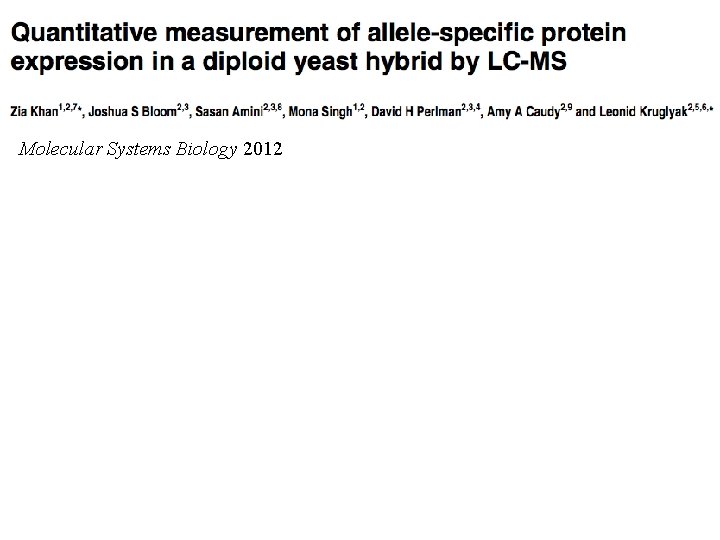
Molecular Systems Biology 2012
Mascot peptide mass fingerprint
Uwb protocol analyzers
Semi auto analyzer principle pdf
Urine analyzers
Harmonic distortion analyzer
Caracteristicas del alcohol
Principle of cooking
Lidl gourmet live yoghurt
Provides bulk and structure to baked products.
Ice cream flavors a to z
Flavors.tools
Flavors
Sku rationalization analytics
Fema
Frusen gladje ice cream
Fibroin secondary structure
How many amino acids are there
Safety precautions for permanent waving
Simple proteins
Elevated c peptide
Pulmonary hypertension differential diagnosis
C peptide interpretation
Pt141 tanning for sale
Importance of peptide bond
Lance wells
Conn syndrome
Atrial natriuretic peptide