MISO Overview and Generation and Demand Management Improvement
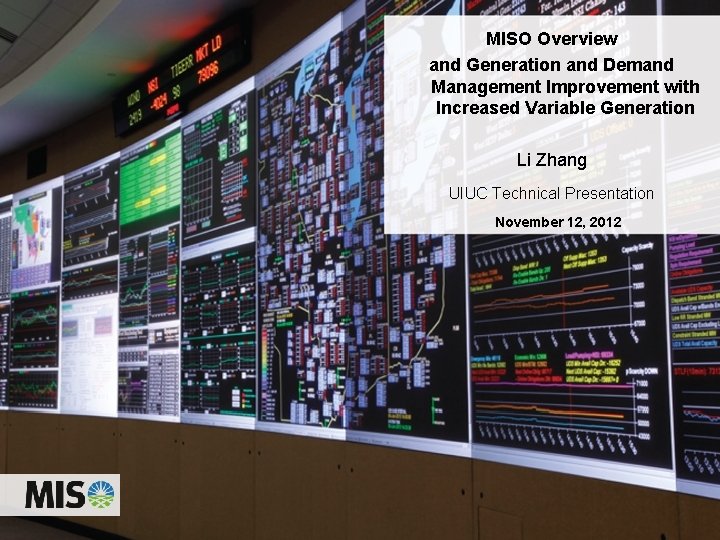
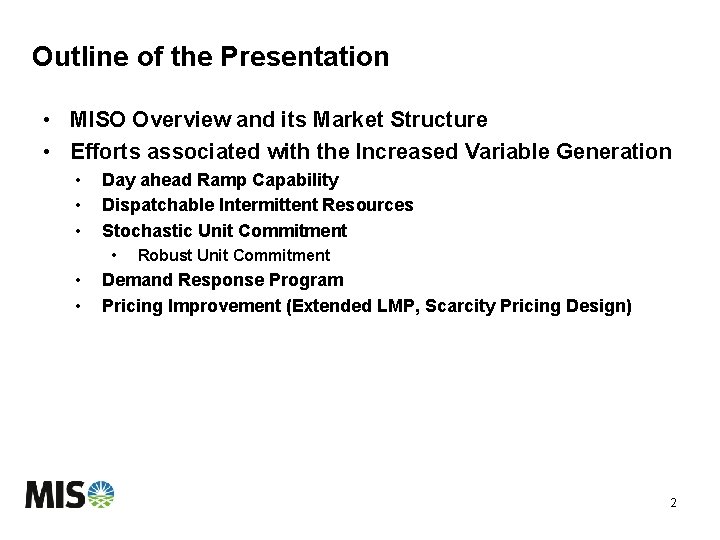
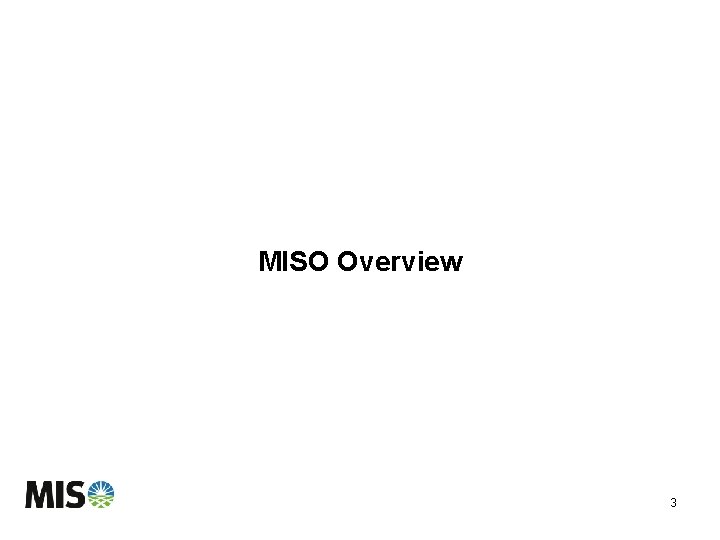
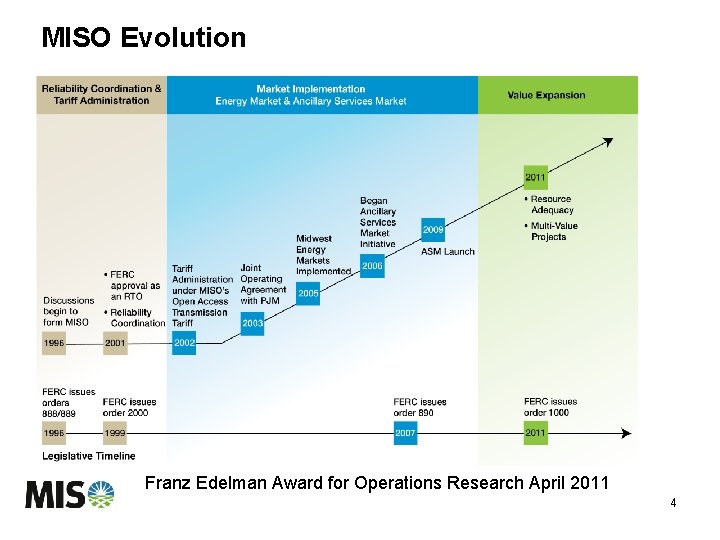
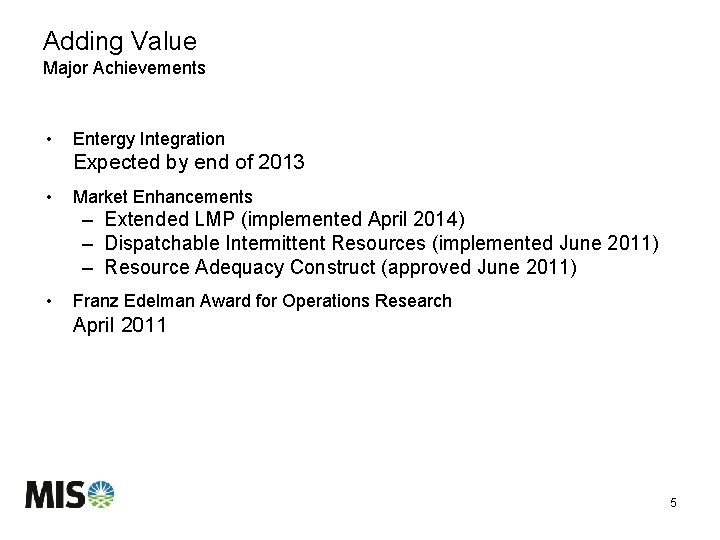
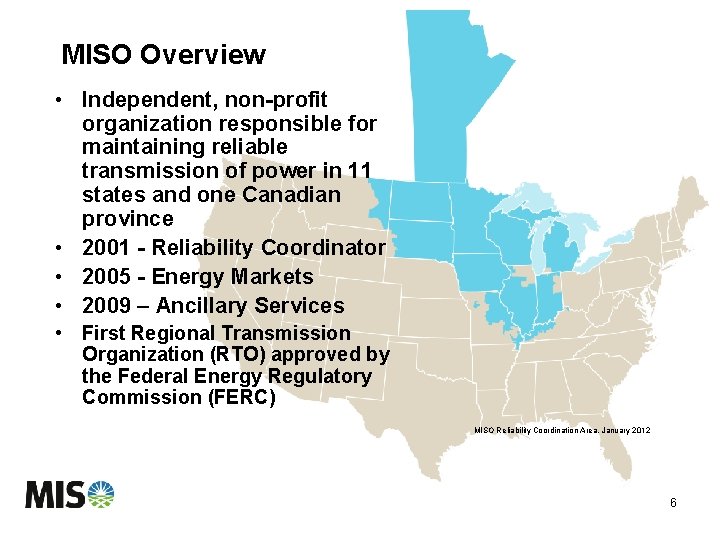
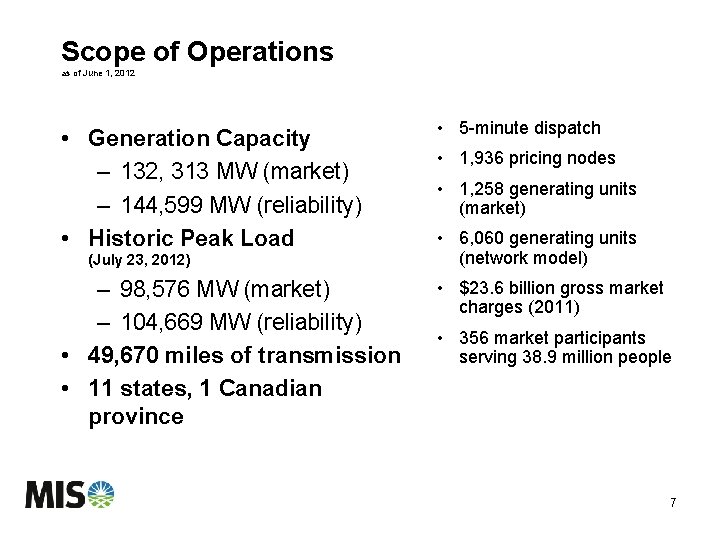
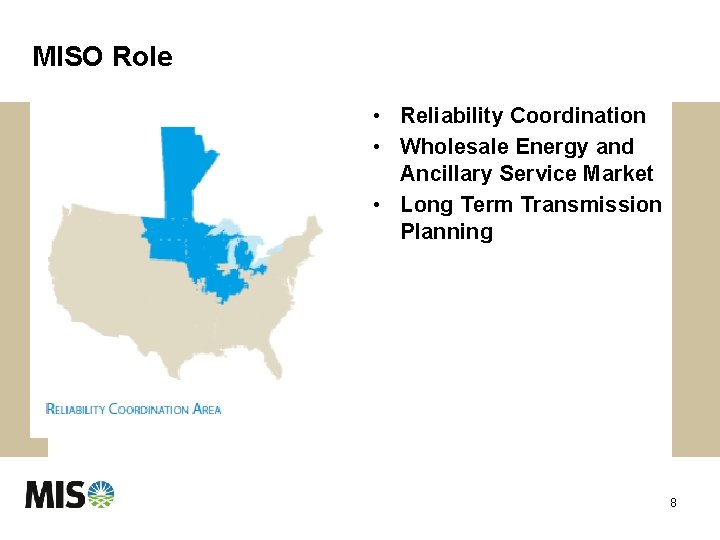
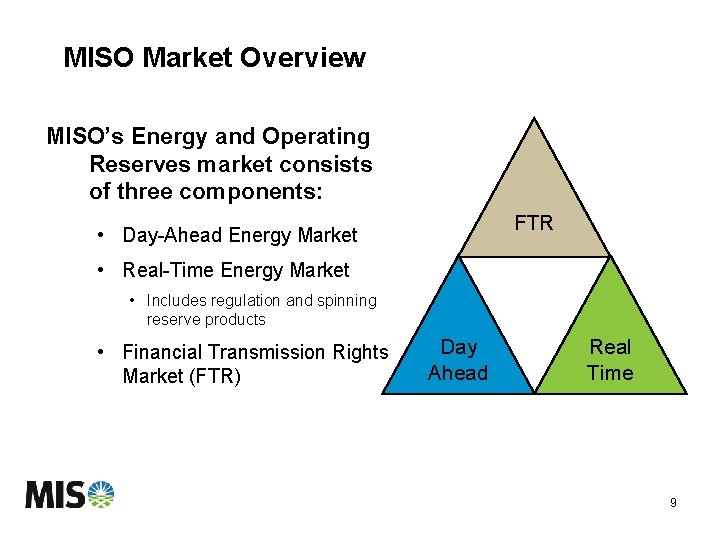
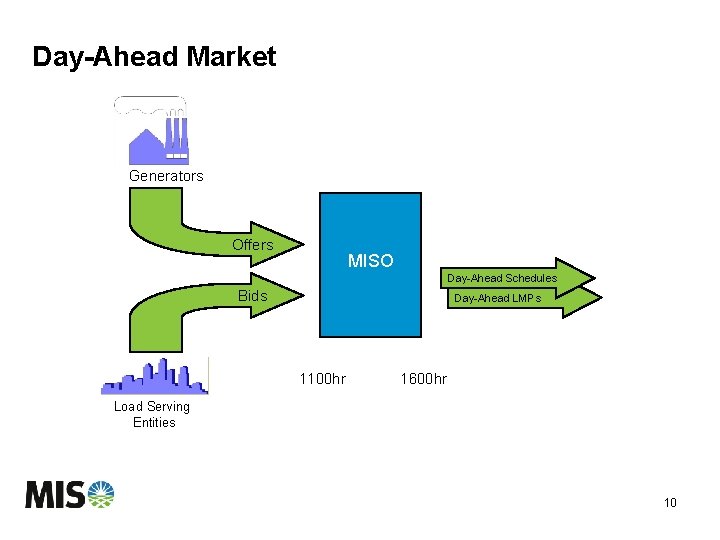
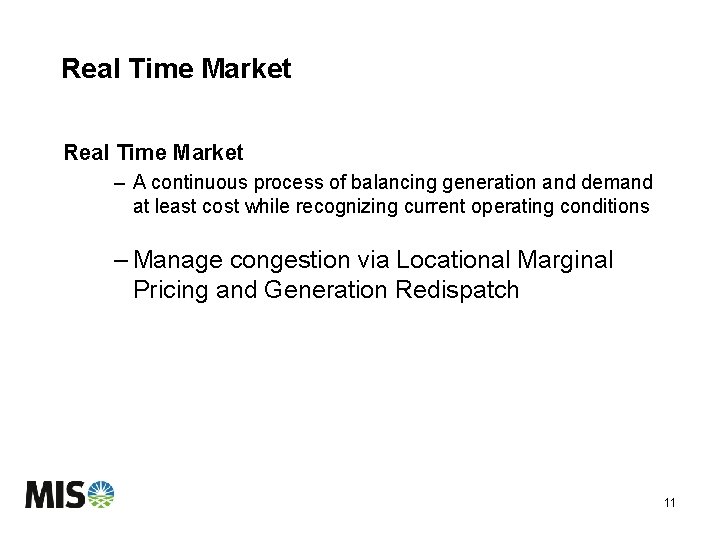
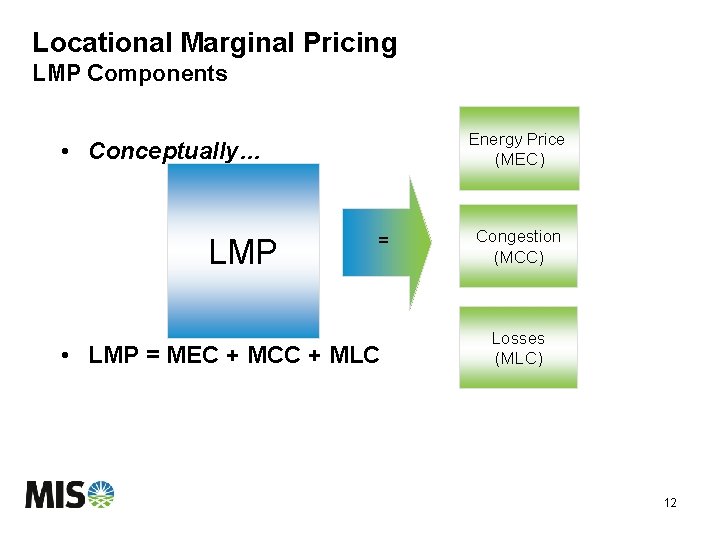
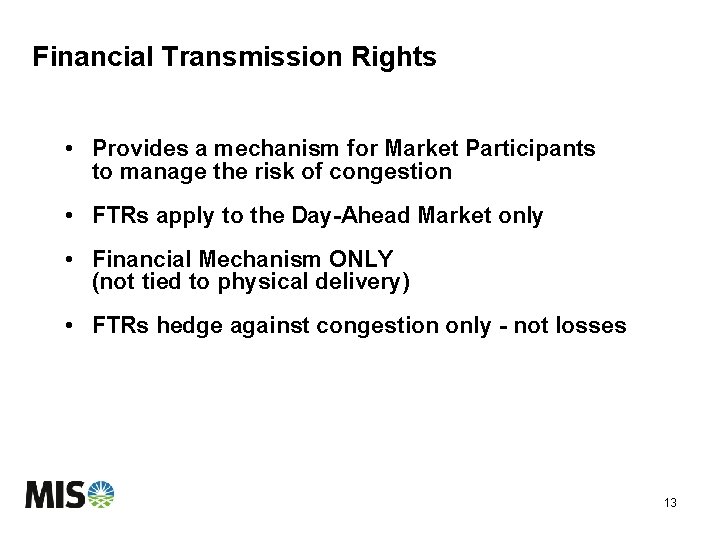
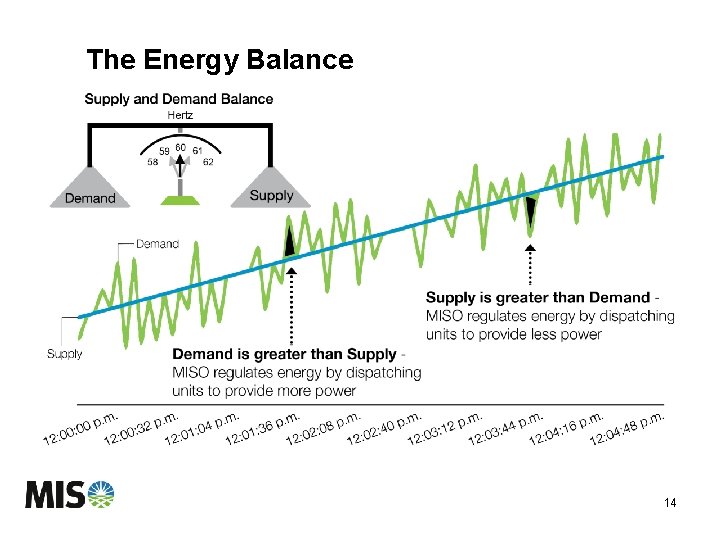
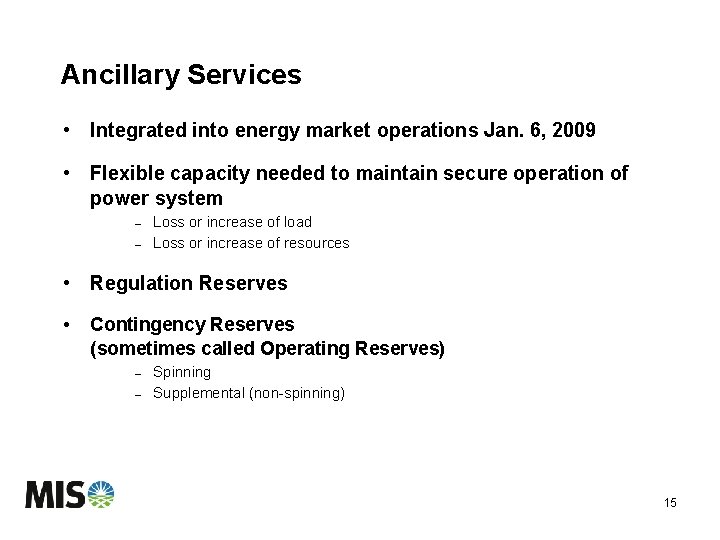
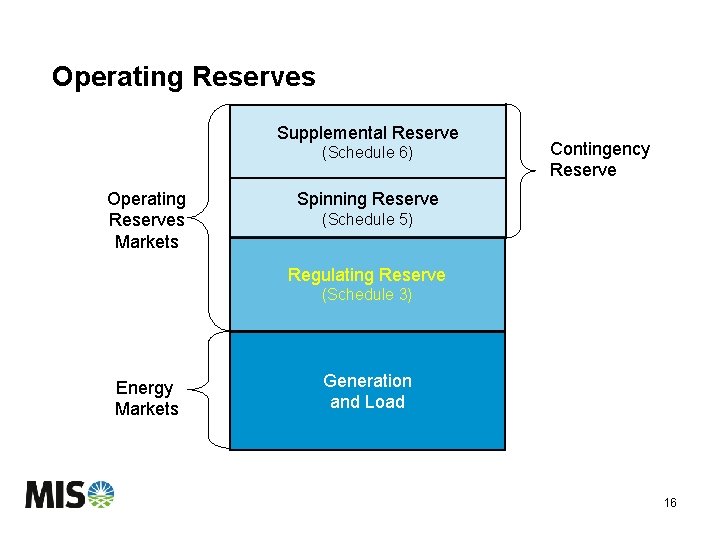
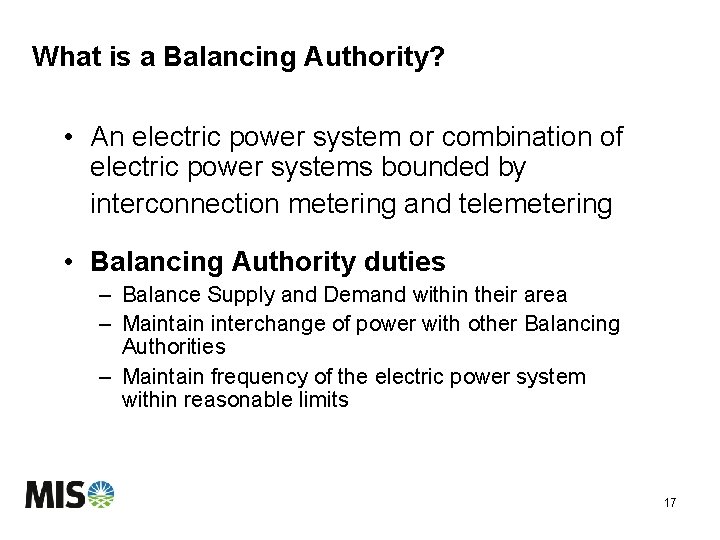
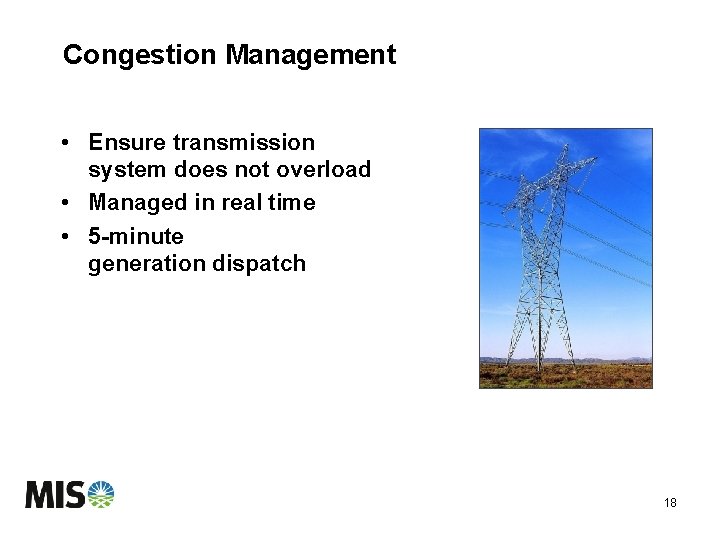
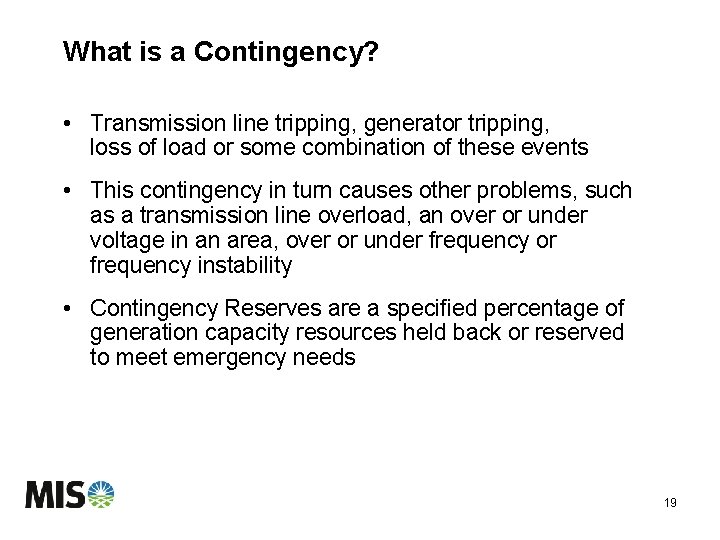
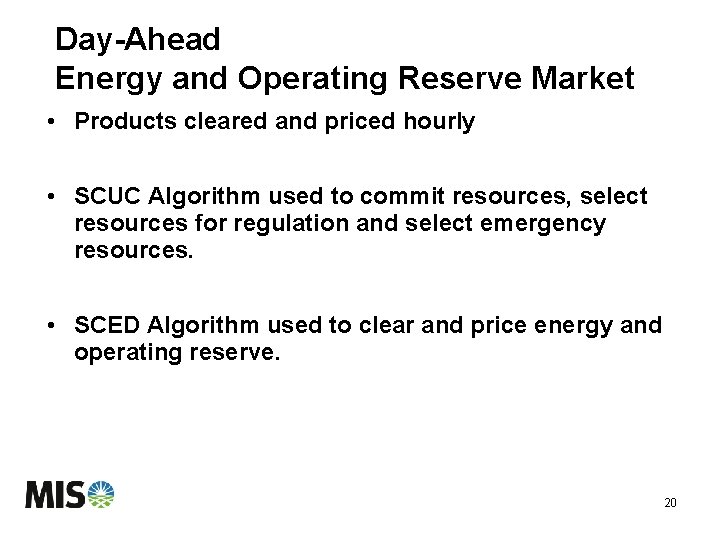
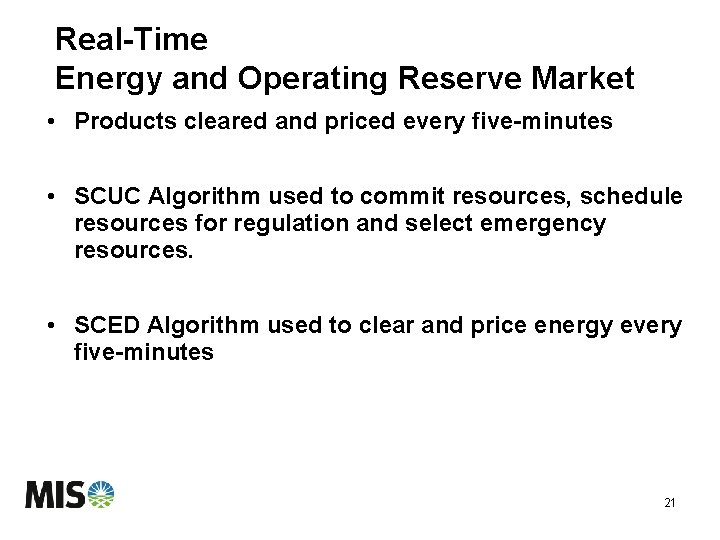
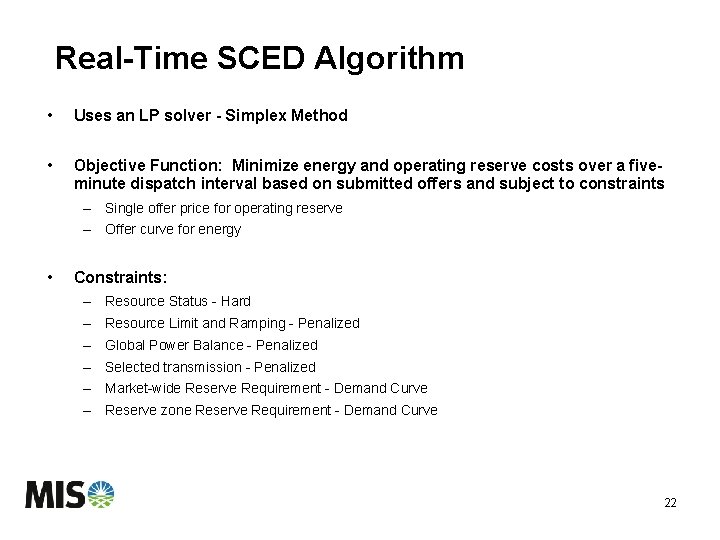
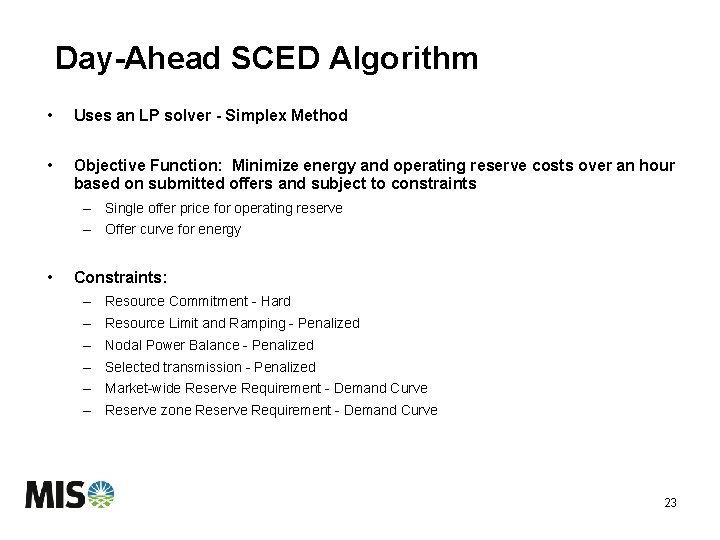
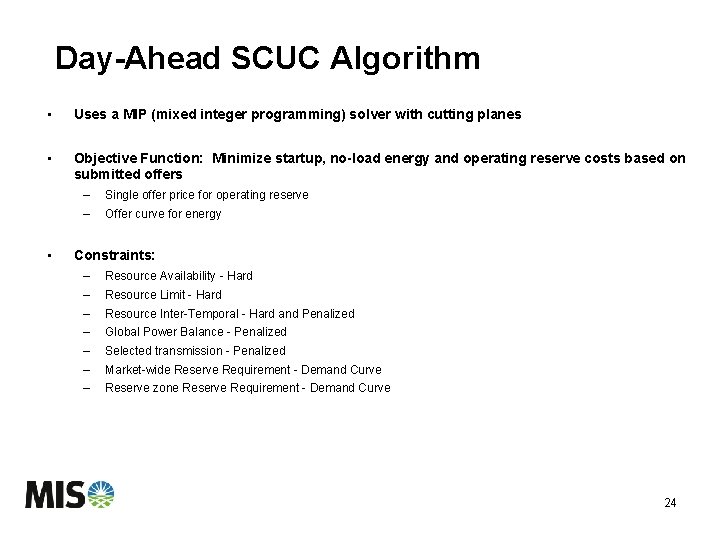
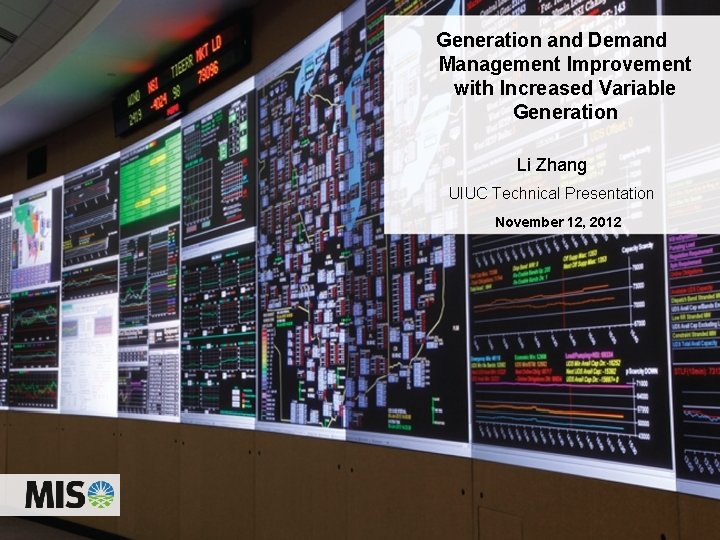
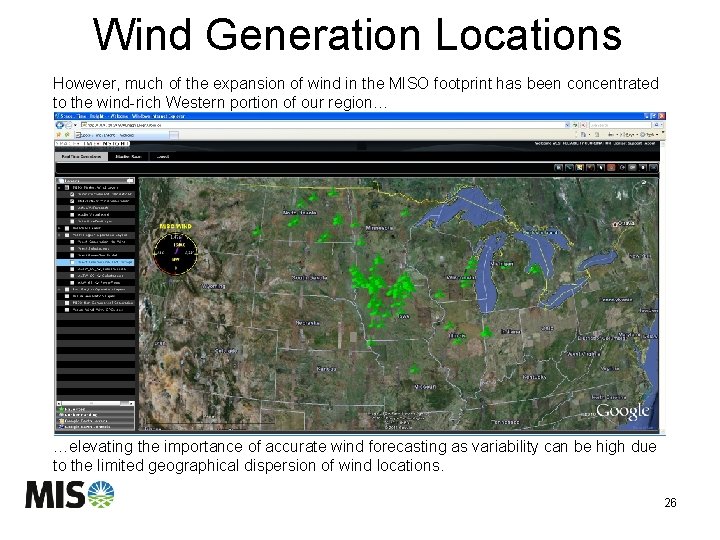
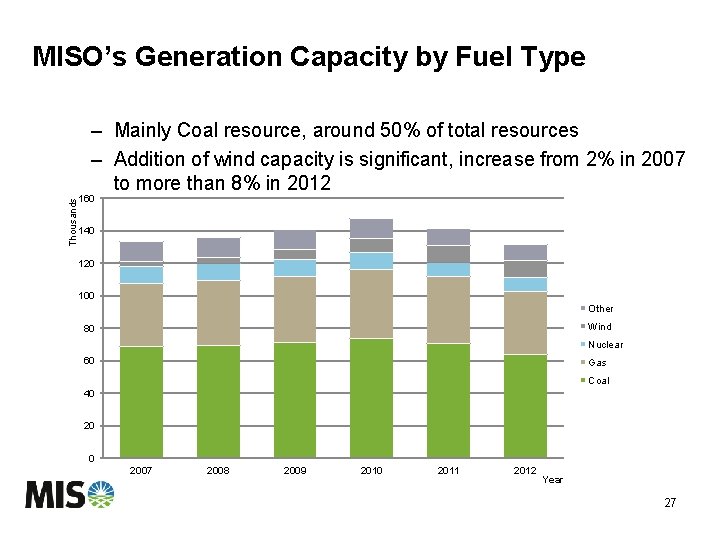
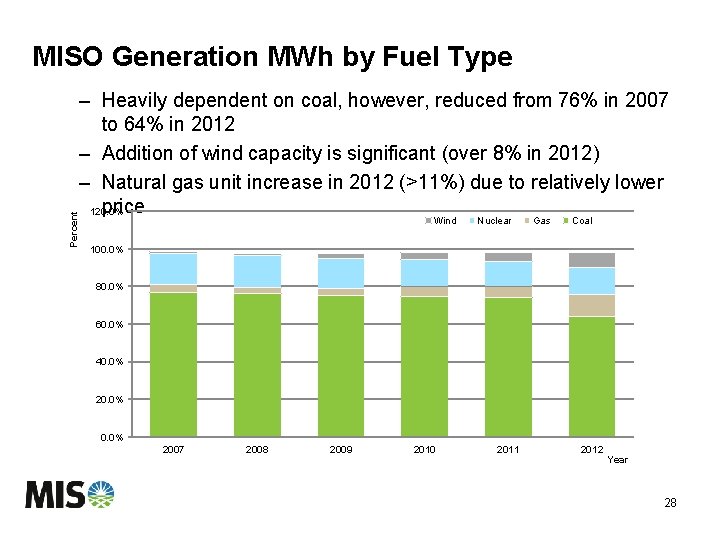
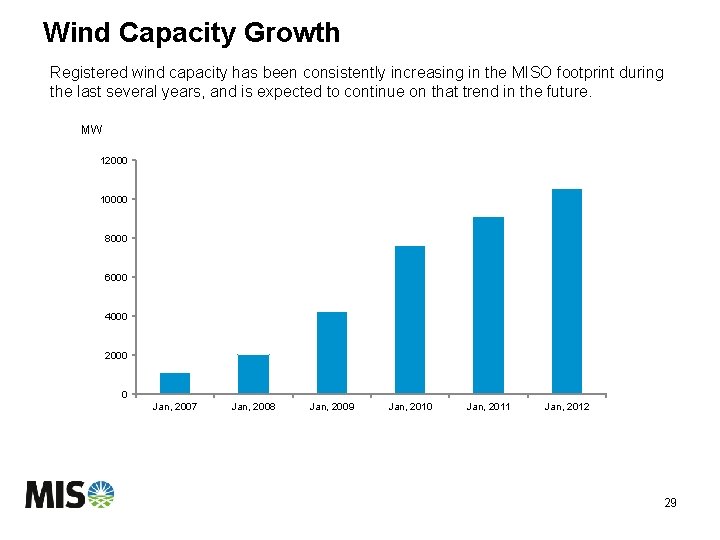
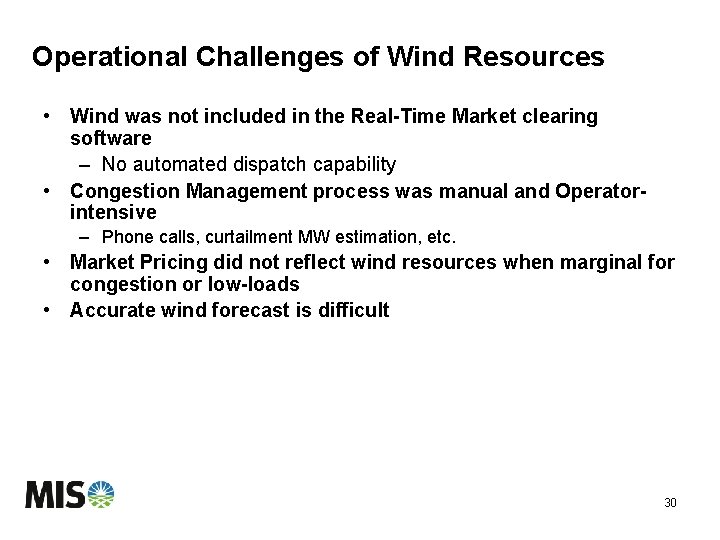
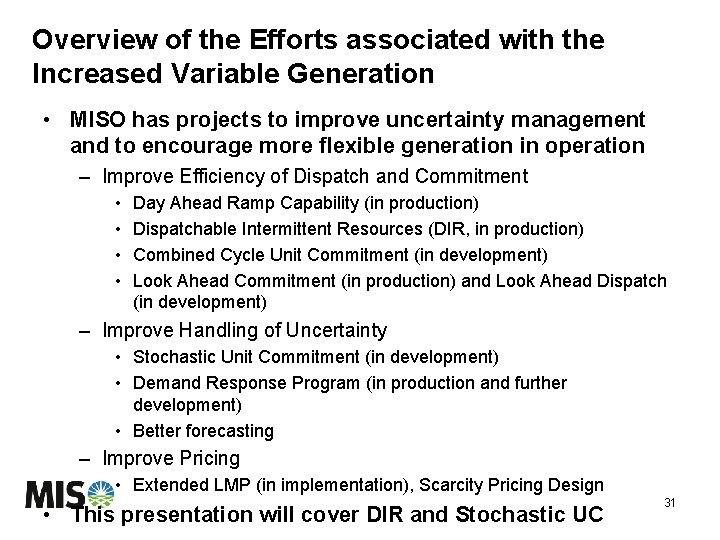
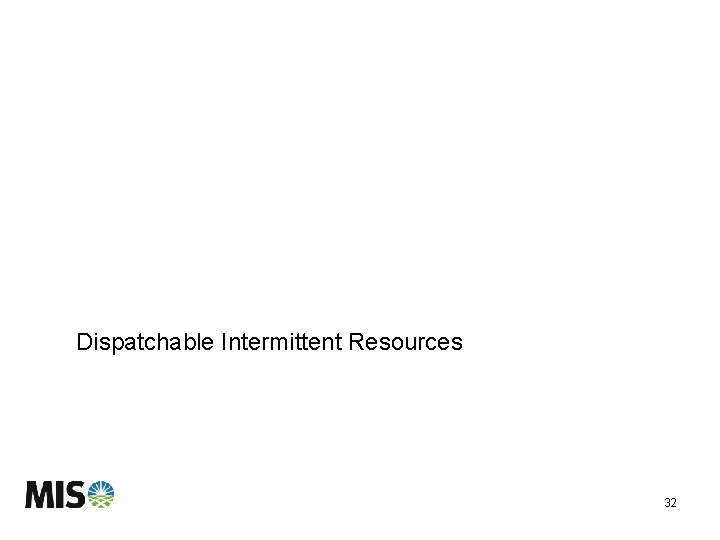
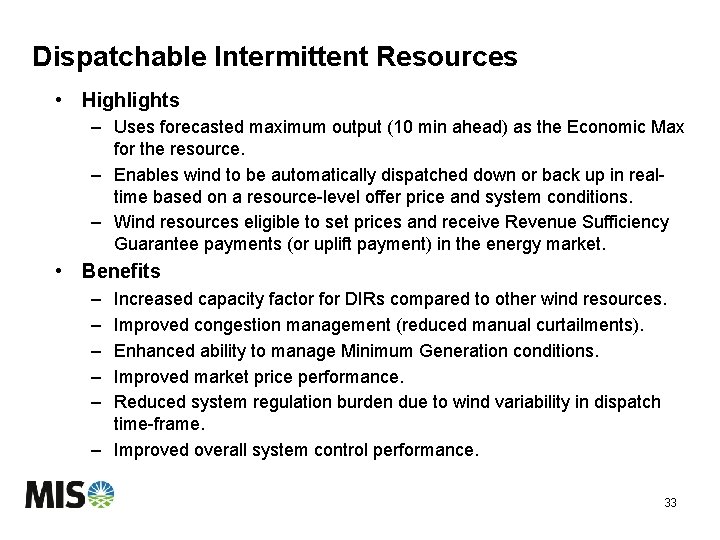
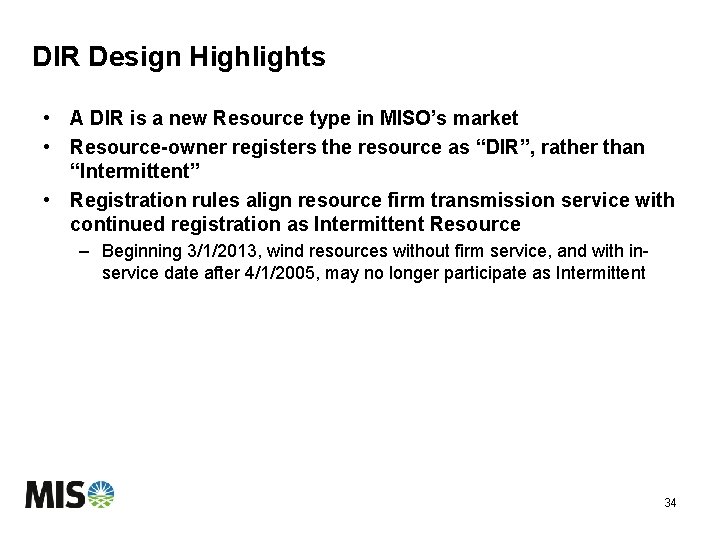
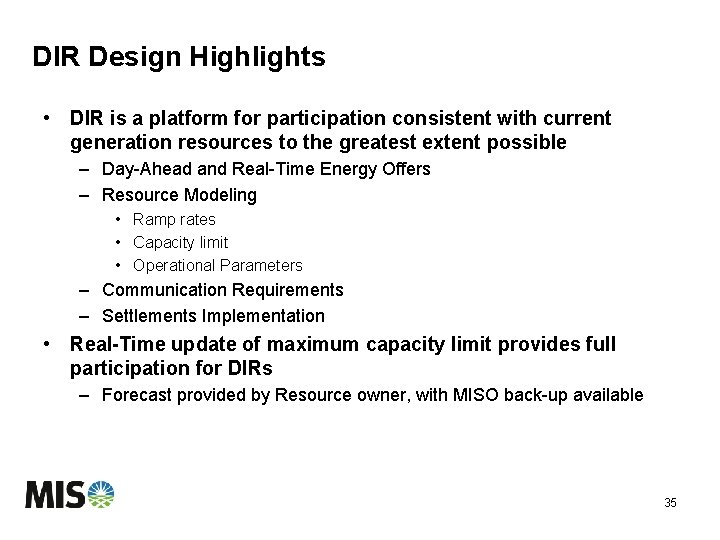
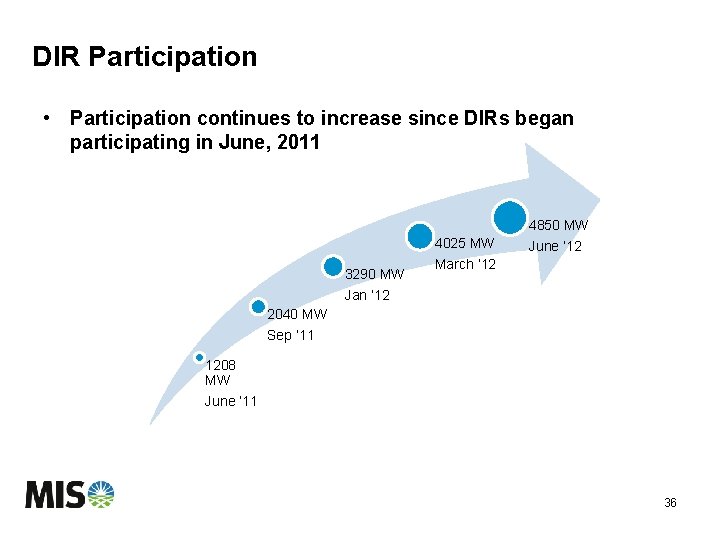
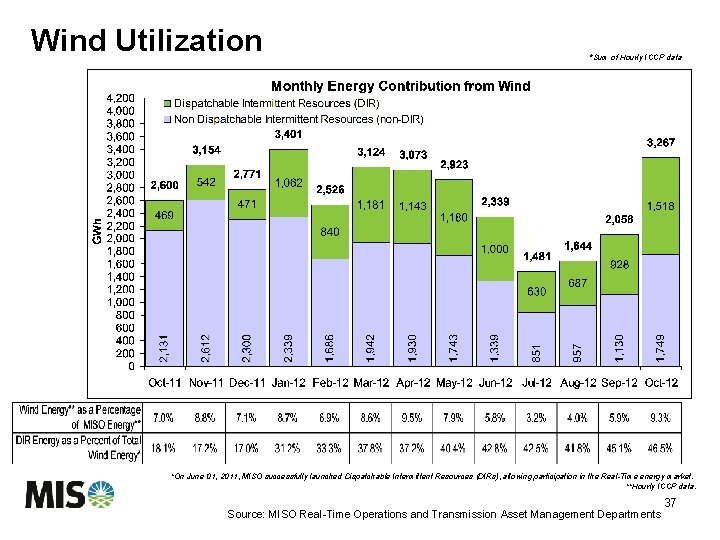
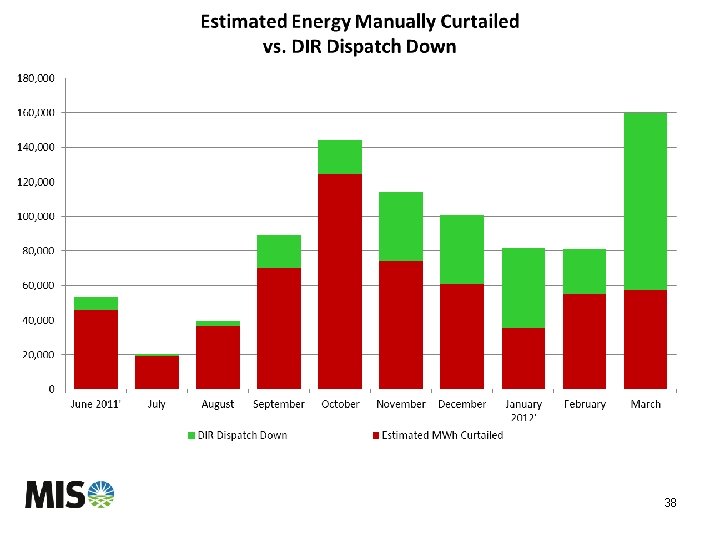
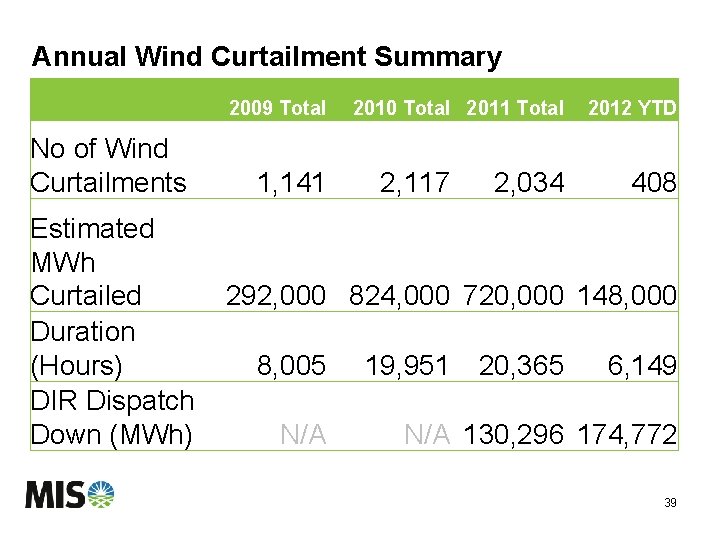
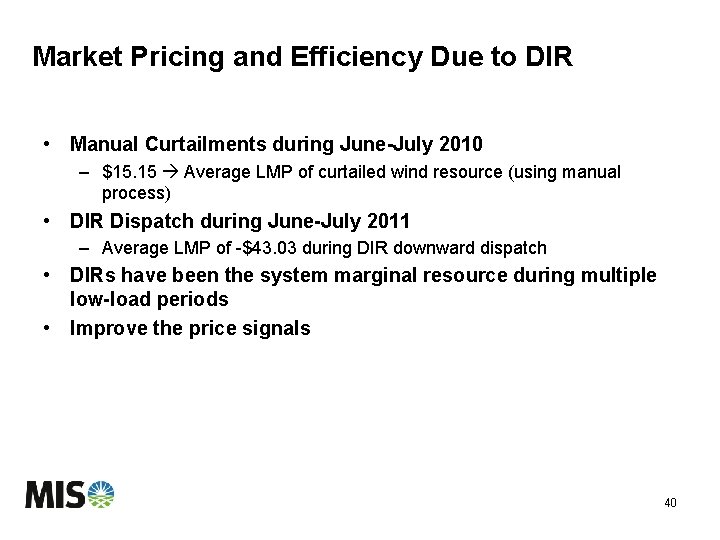
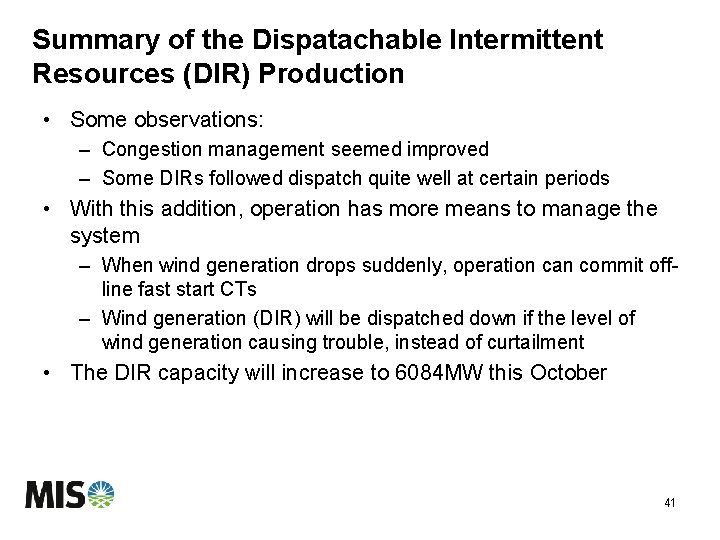
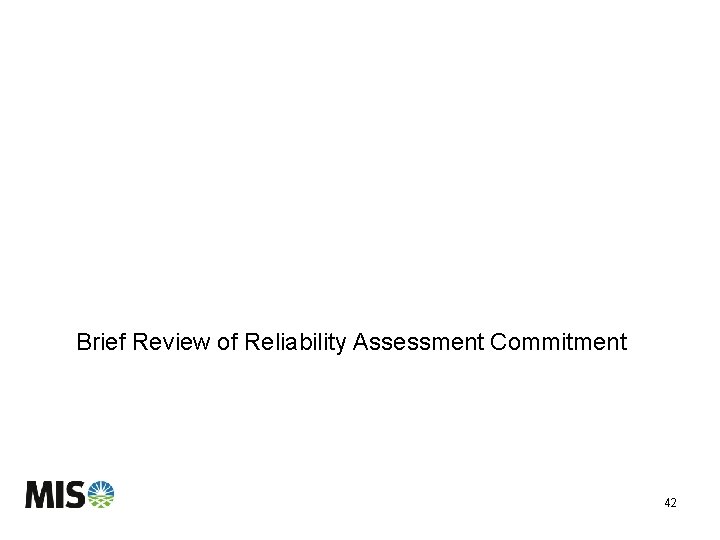
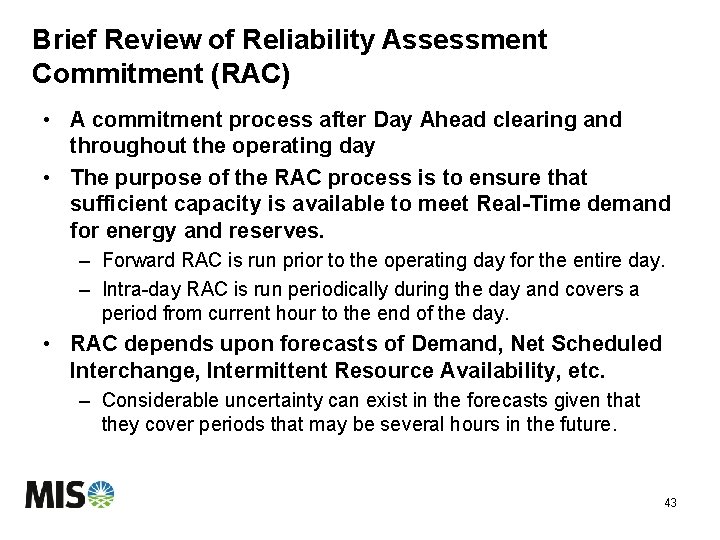
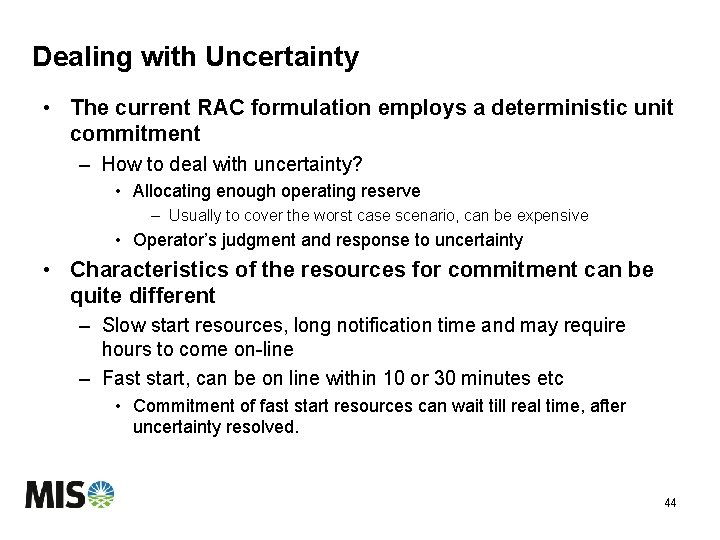
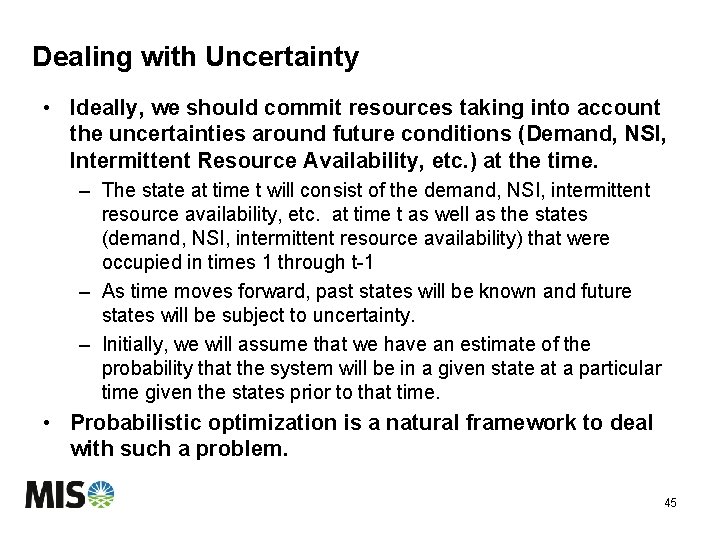
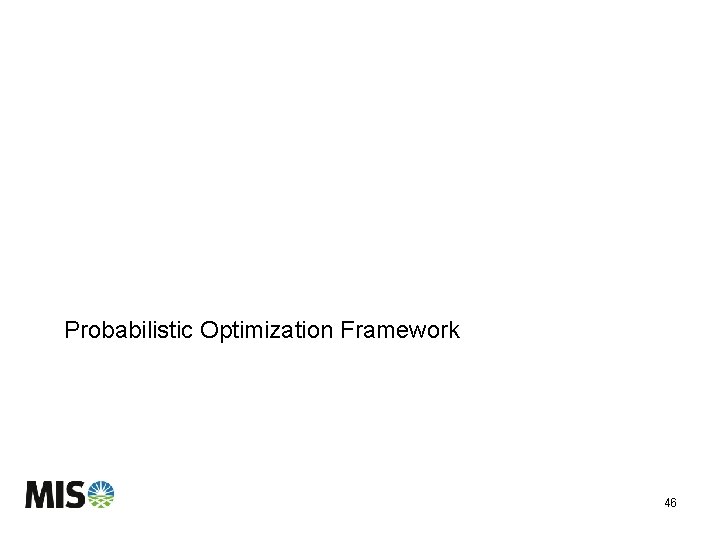
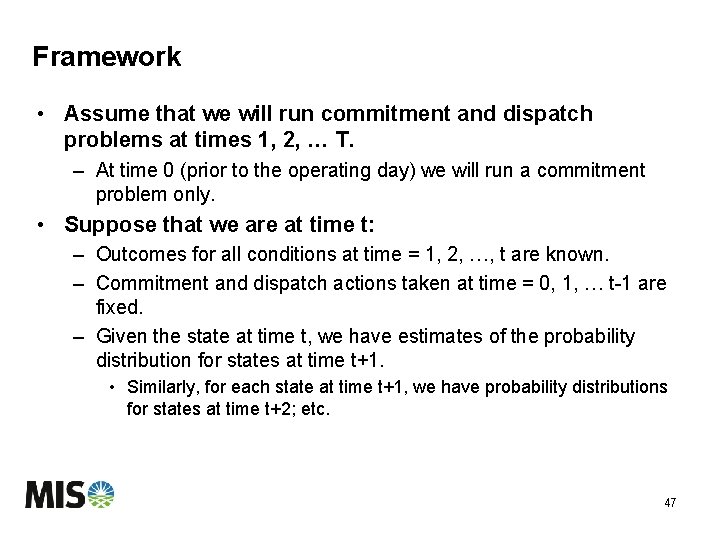
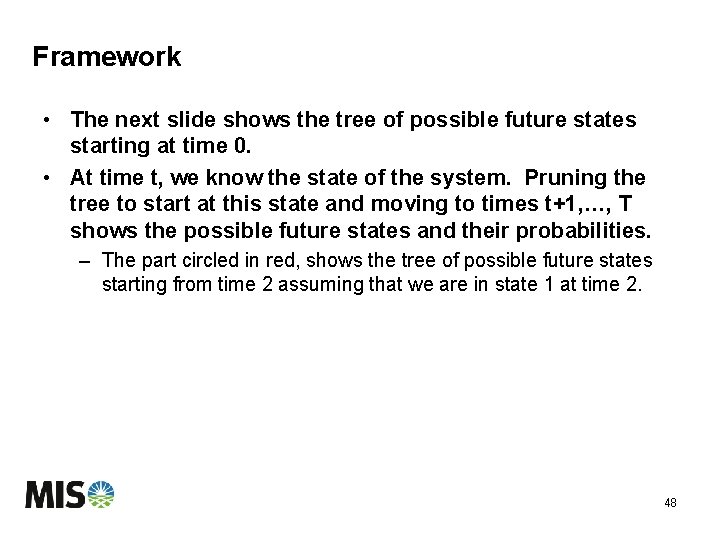
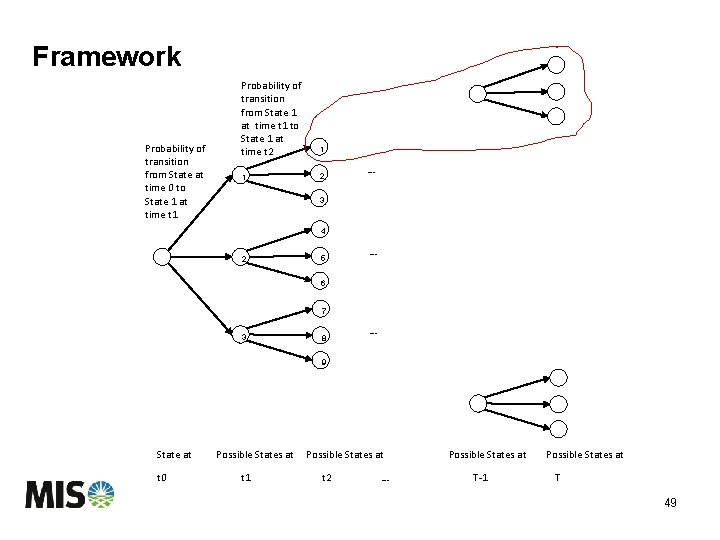
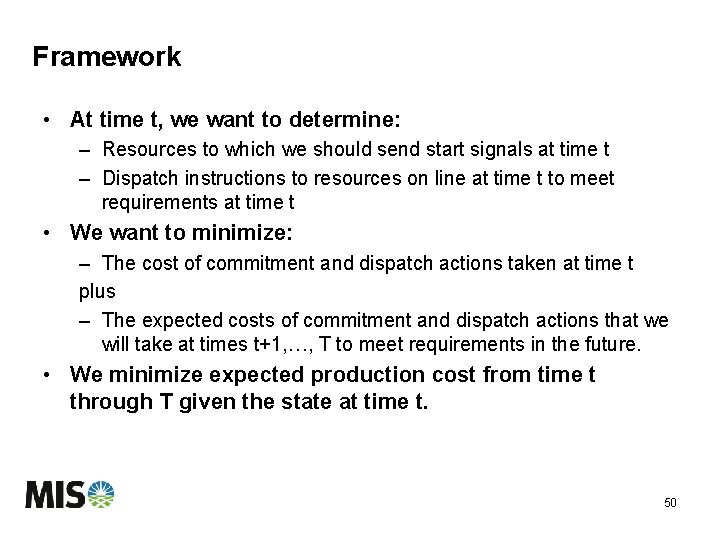
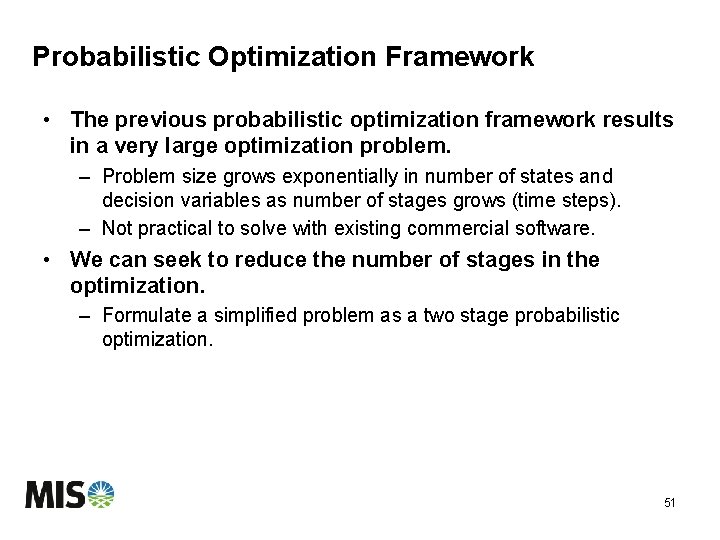
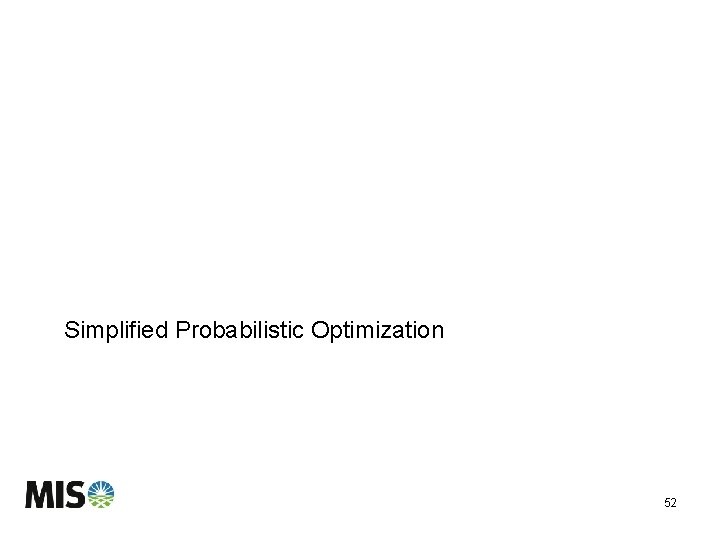
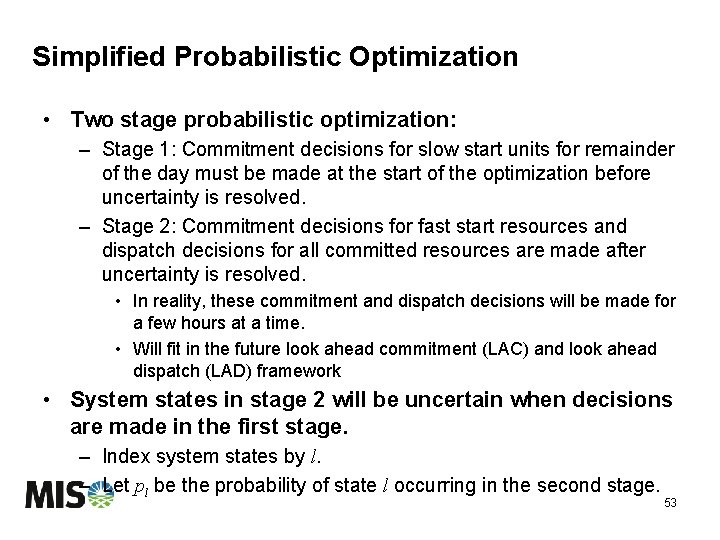
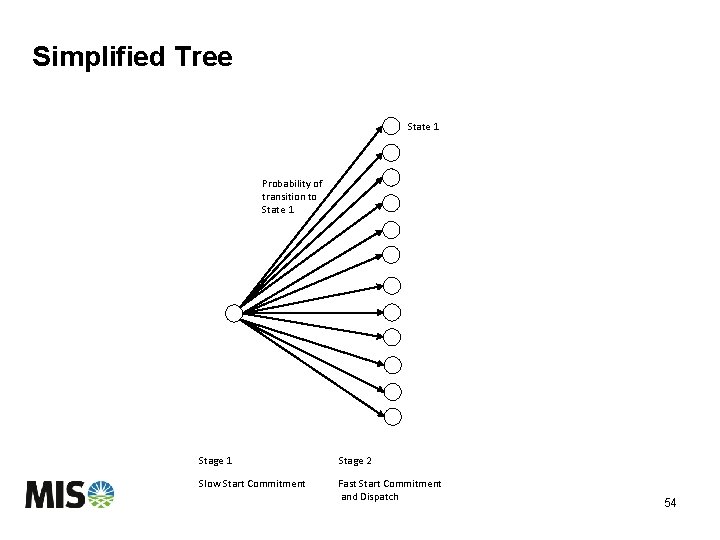
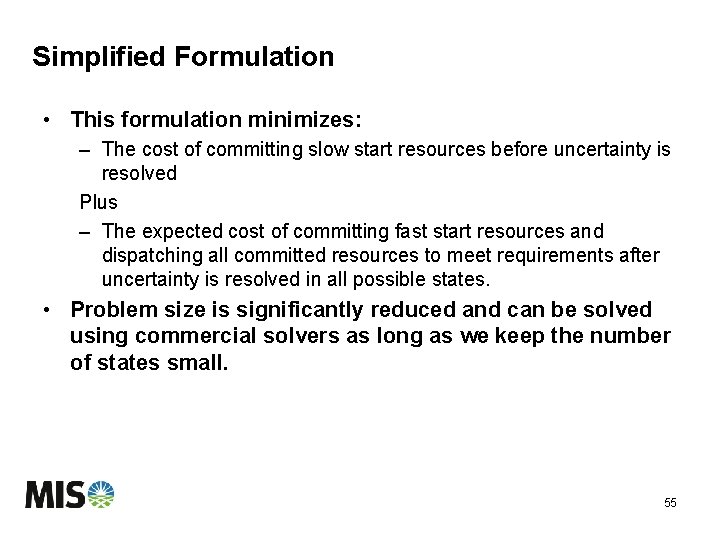
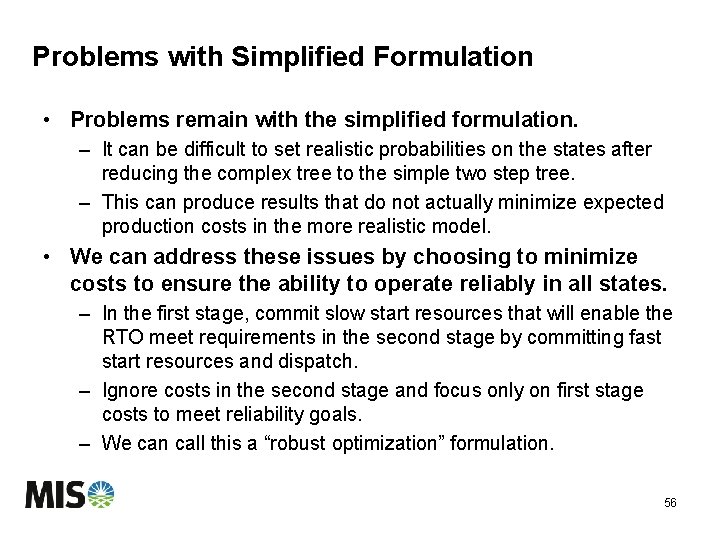
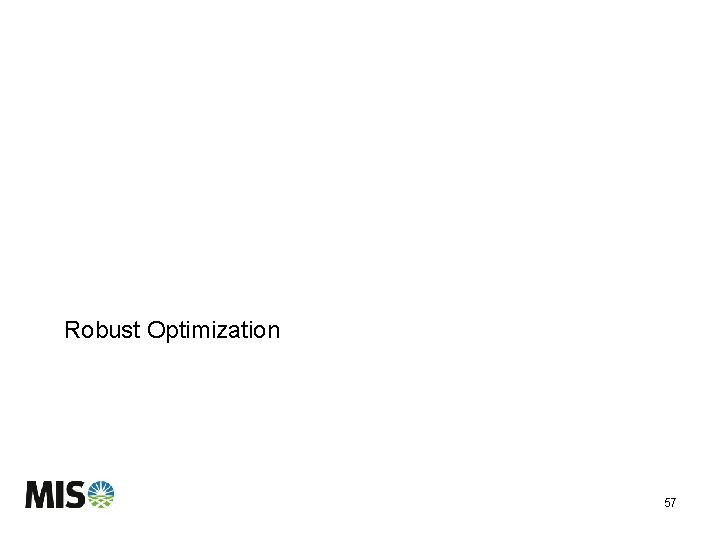
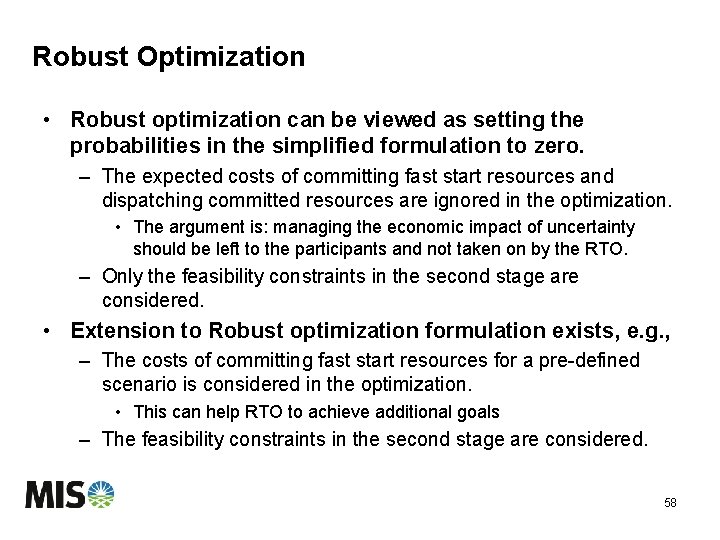
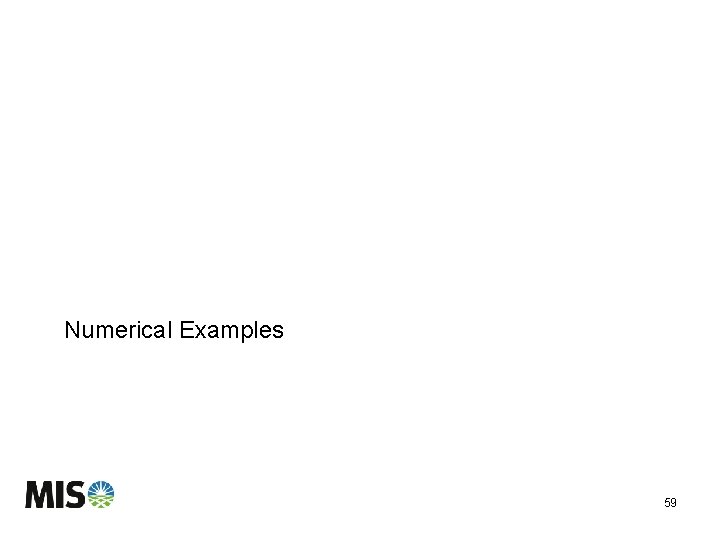
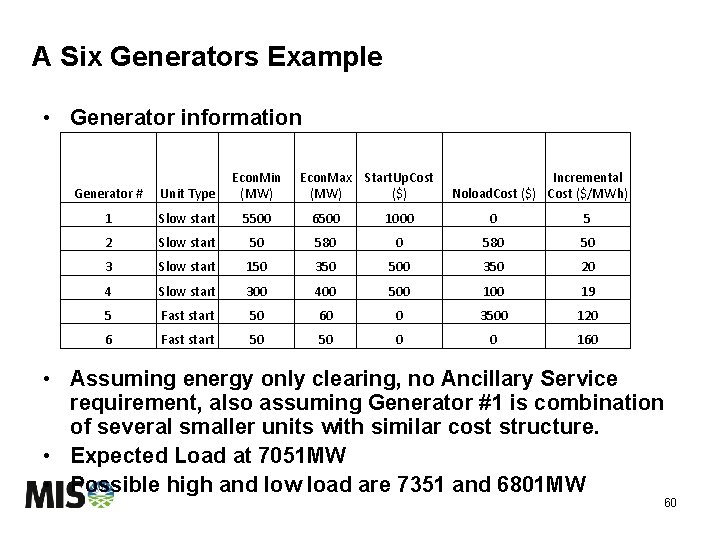
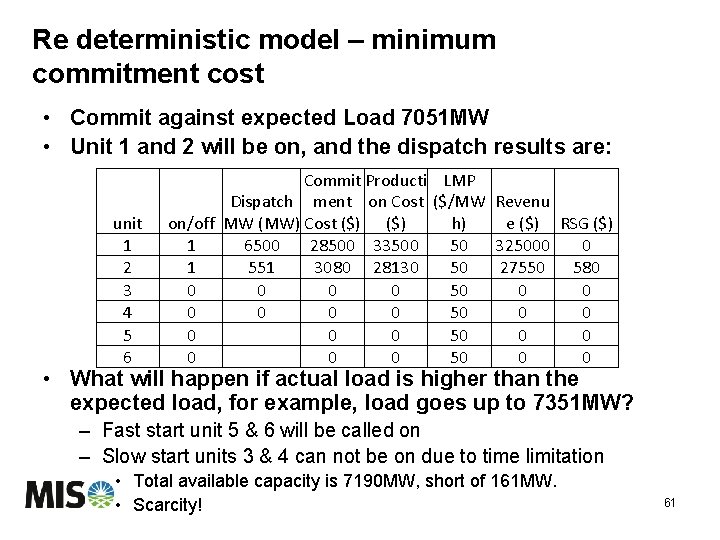
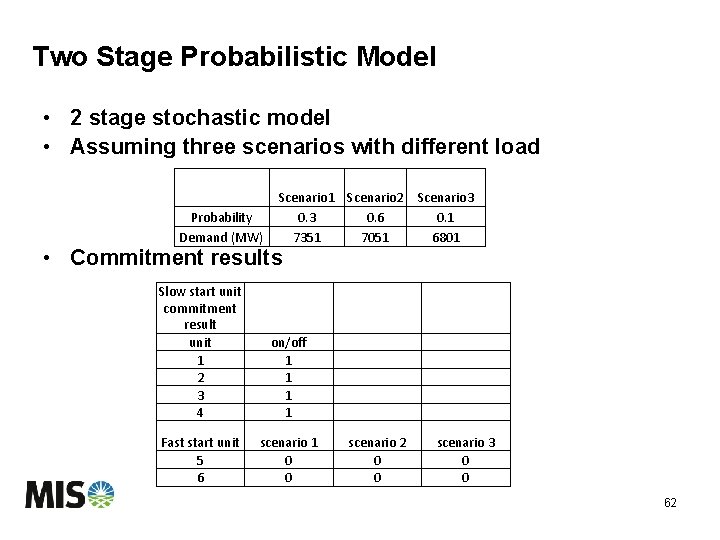
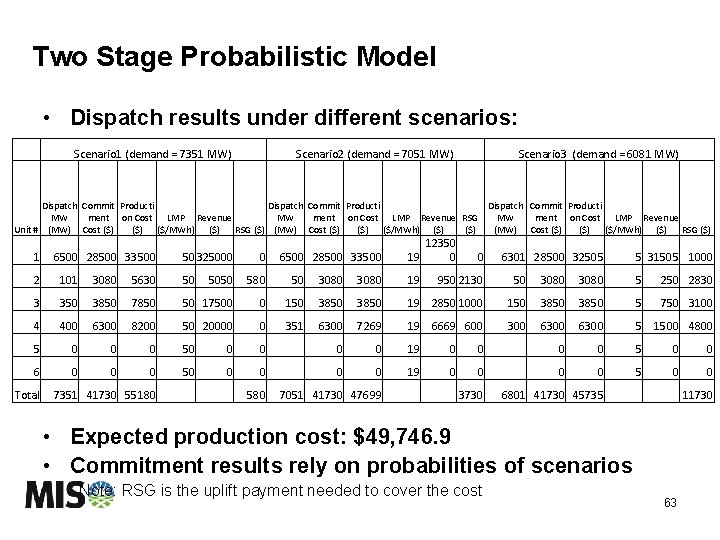
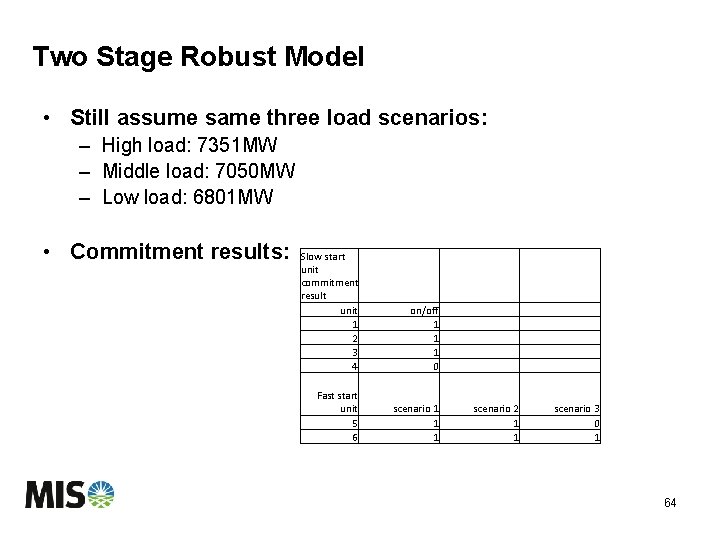
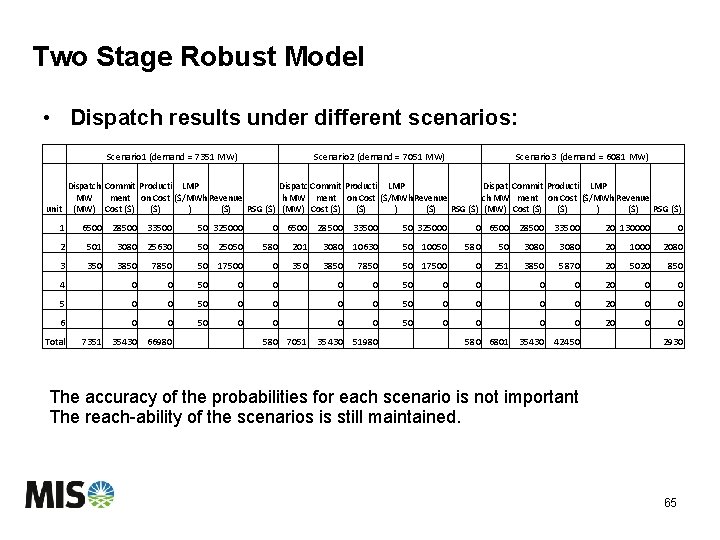
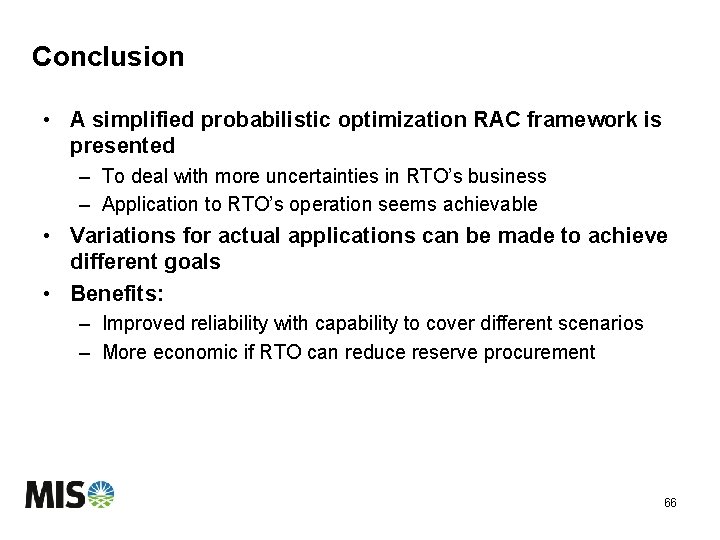
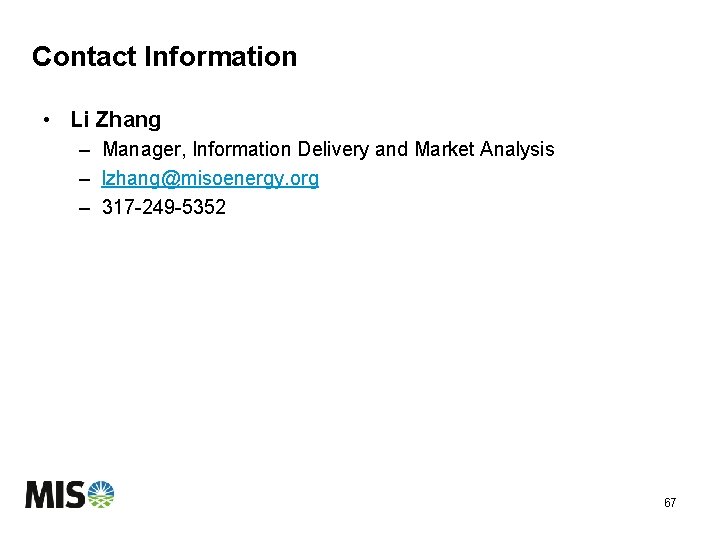
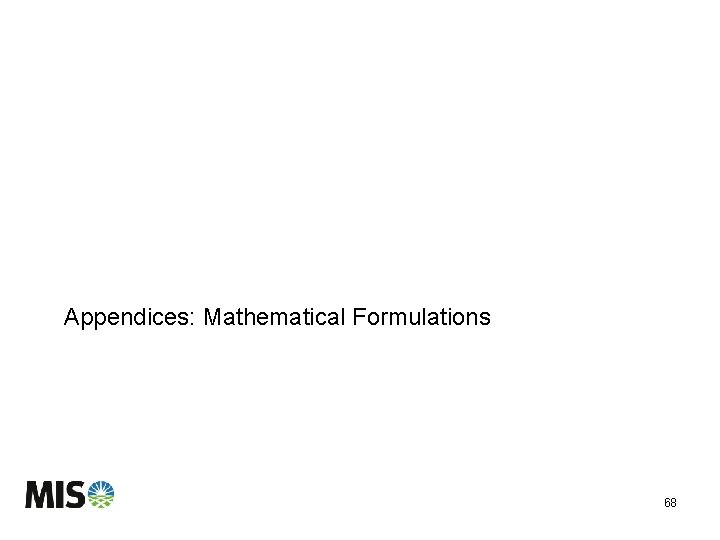
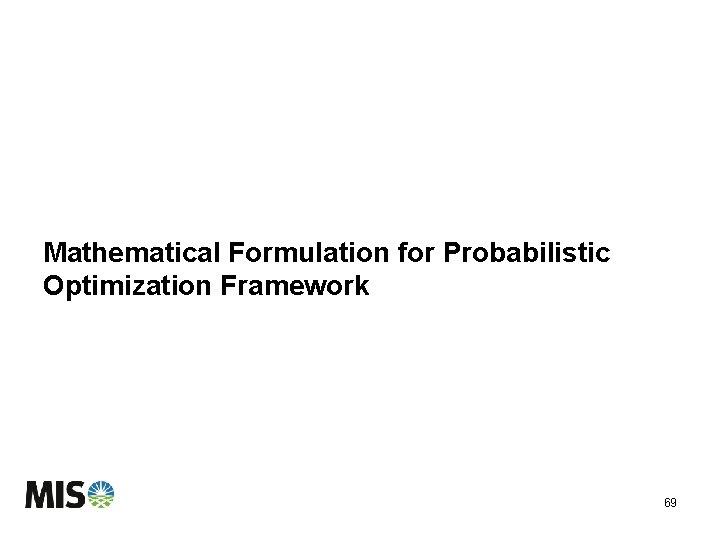
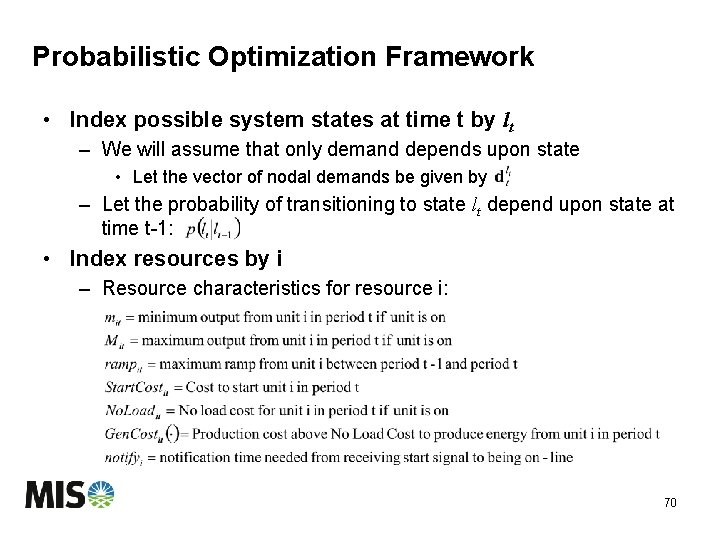
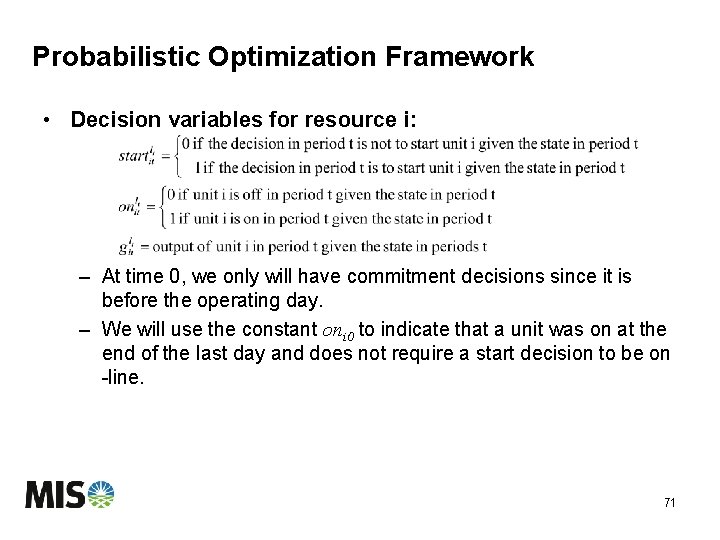
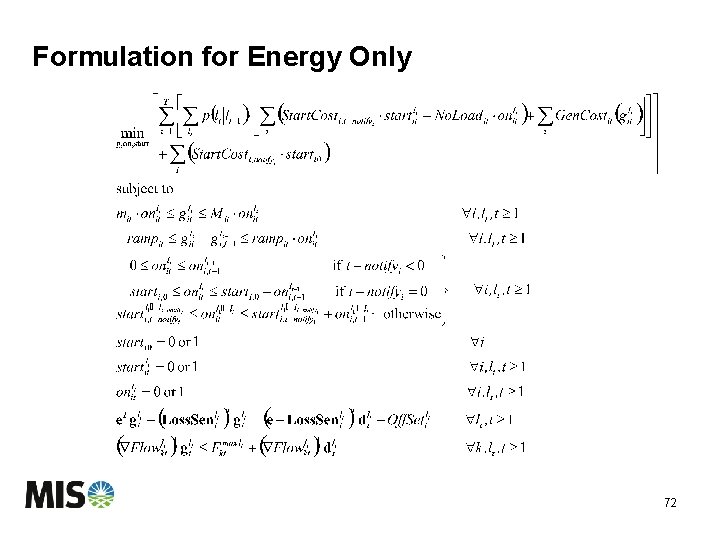
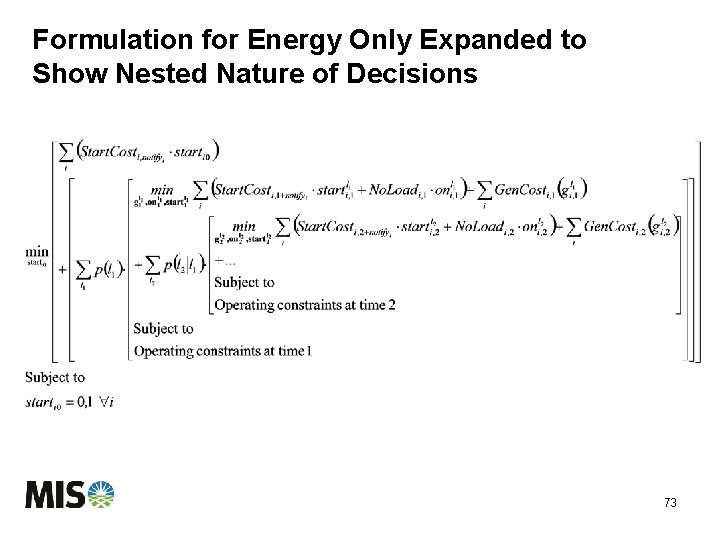
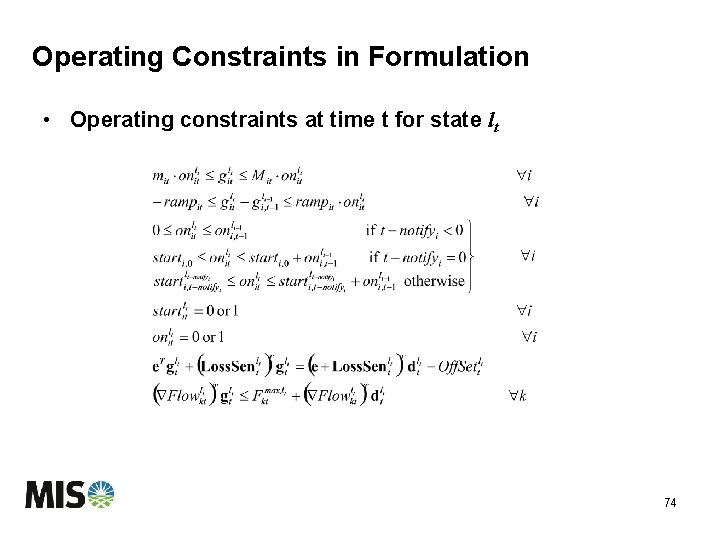
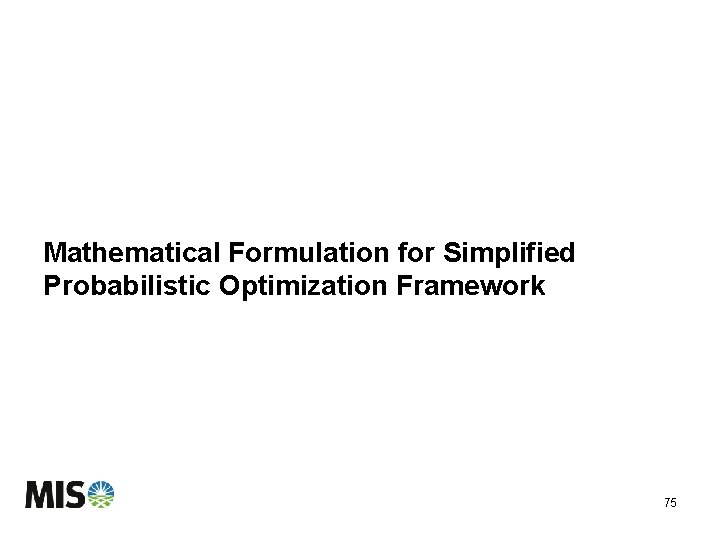
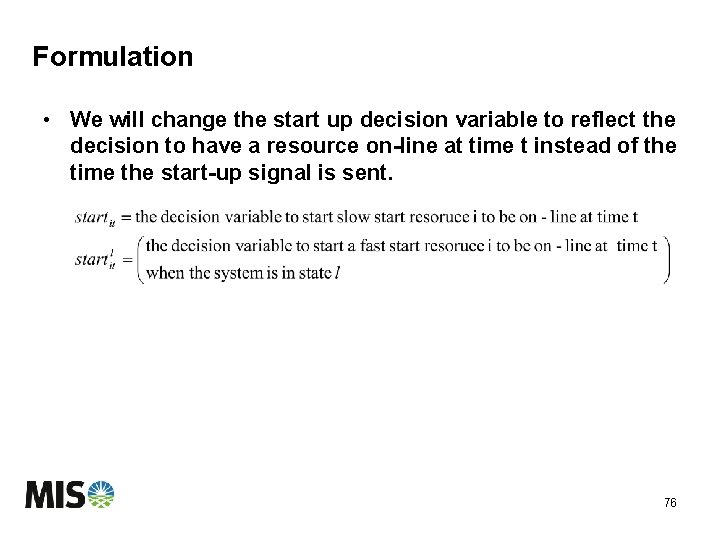
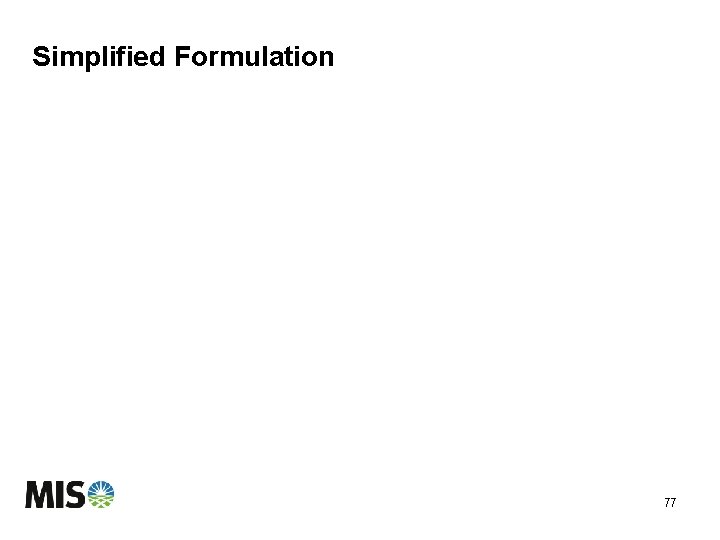
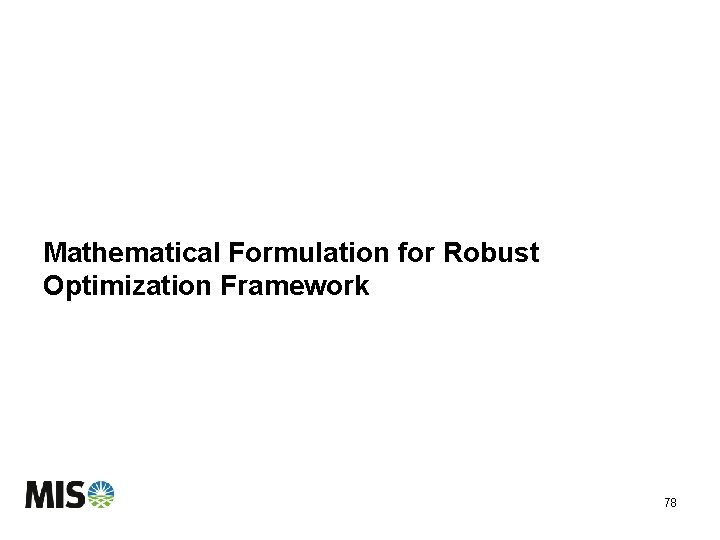
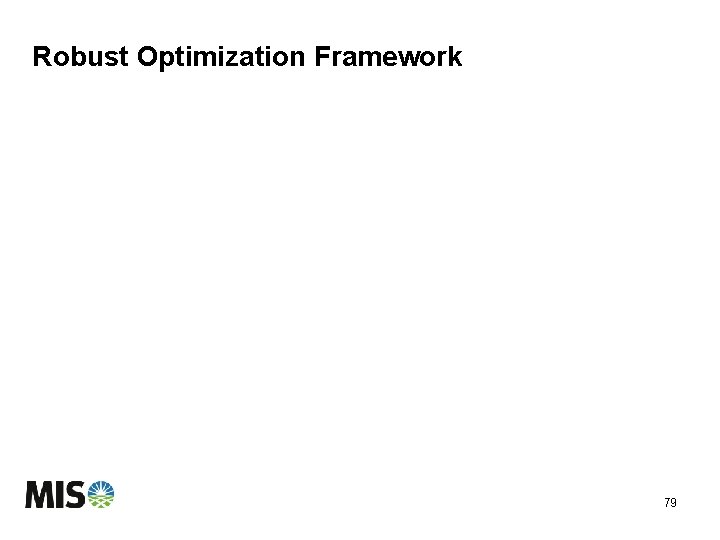
- Slides: 79
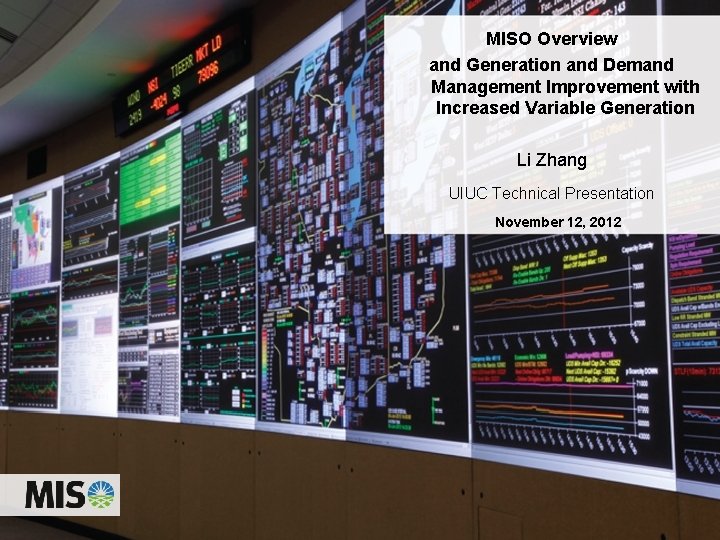
MISO Overview and Generation and Demand Management Improvement with Increased Variable Generation Li Zhang UIUC Technical Presentation November 12, 2012
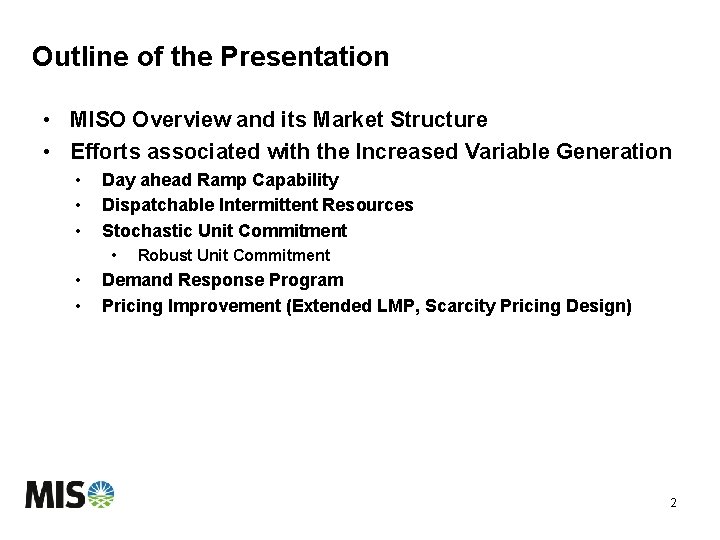
Outline of the Presentation • MISO Overview and its Market Structure • Efforts associated with the Increased Variable Generation • • • Day ahead Ramp Capability Dispatchable Intermittent Resources Stochastic Unit Commitment • • • Robust Unit Commitment Demand Response Program Pricing Improvement (Extended LMP, Scarcity Pricing Design) 2
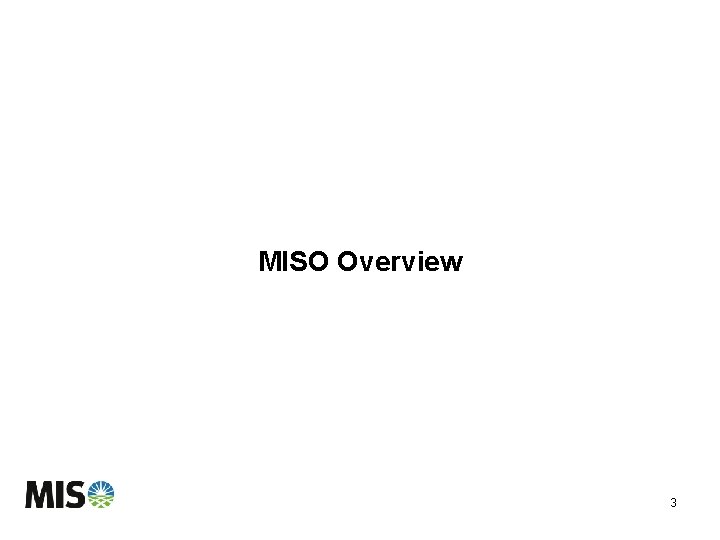
MISO Overview 3
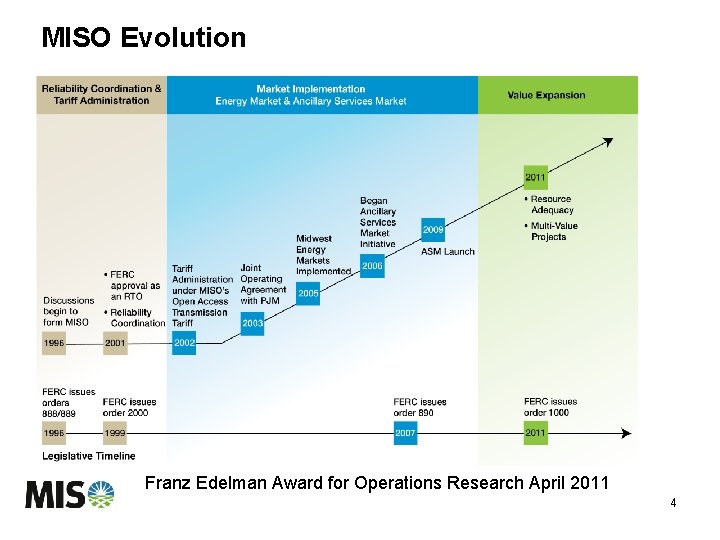
MISO Evolution Franz Edelman Award for Operations Research April 2011 4
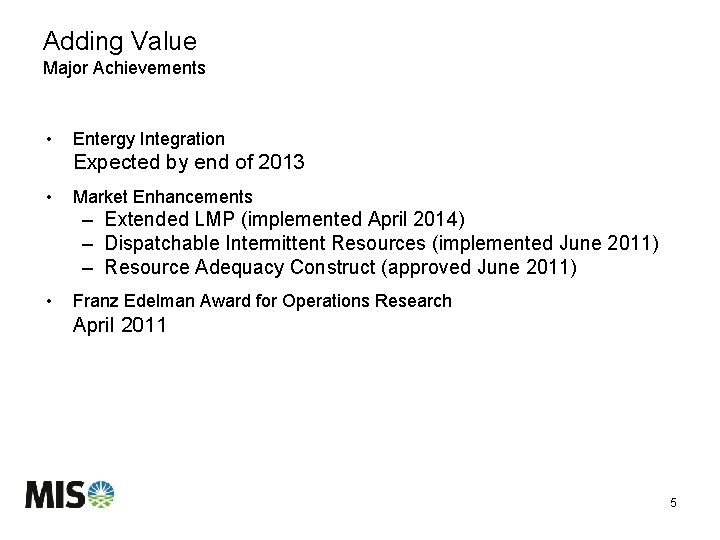
Adding Value Major Achievements • Entergy Integration Expected by end of 2013 • Market Enhancements – Extended LMP (implemented April 2014) – Dispatchable Intermittent Resources (implemented June 2011) – Resource Adequacy Construct (approved June 2011) • Franz Edelman Award for Operations Research April 2011 5
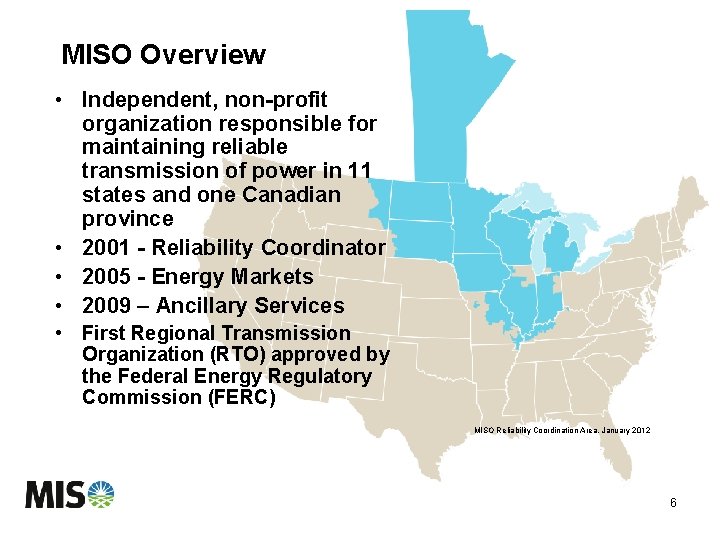
MISO Overview • Independent, non-profit organization responsible for maintaining reliable transmission of power in 11 states and one Canadian province • 2001 - Reliability Coordinator • 2005 - Energy Markets • 2009 – Ancillary Services • First Regional Transmission Organization (RTO) approved by the Federal Energy Regulatory Commission (FERC) MISO Reliability Coordination Area, January 2012 6
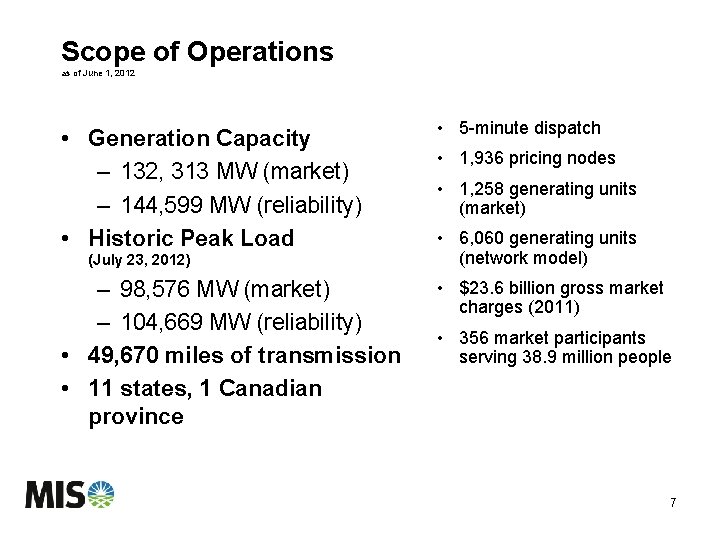
Scope of Operations as of June 1, 2012 • Generation Capacity – 132, 313 MW (market) – 144, 599 MW (reliability) • Historic Peak Load (July 23, 2012) – 98, 576 MW (market) – 104, 669 MW (reliability) • 49, 670 miles of transmission • 11 states, 1 Canadian province • 5 -minute dispatch • 1, 936 pricing nodes • 1, 258 generating units (market) • 6, 060 generating units (network model) • $23. 6 billion gross market charges (2011) • 356 market participants serving 38. 9 million people 7
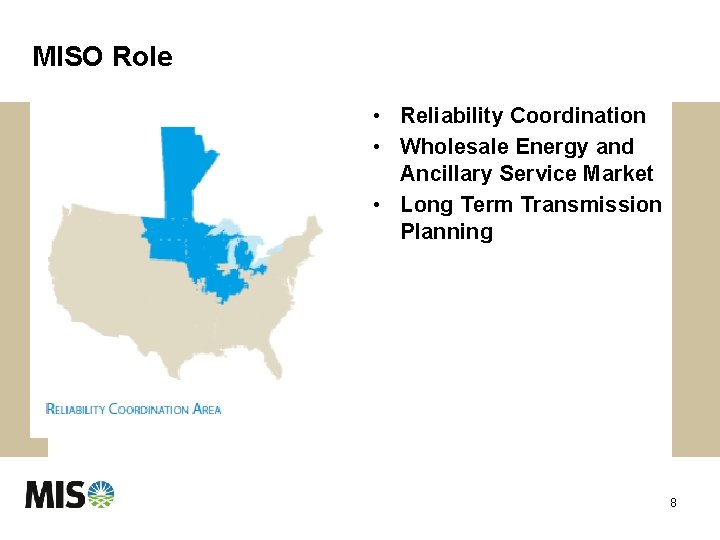
MISO Role • Reliability Coordination • Wholesale Energy and Ancillary Service Market • Long Term Transmission Planning 8
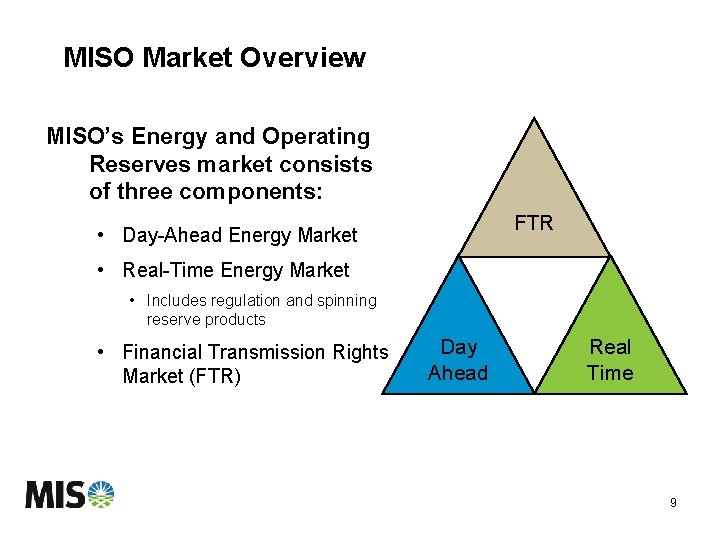
MISO Market Overview MISO’s Energy and Operating Reserves market consists of three components: FTR • Day-Ahead Energy Market • Real-Time Energy Market • Includes regulation and spinning reserve products • Financial Transmission Rights Market (FTR) Day Ahead Real Time 9
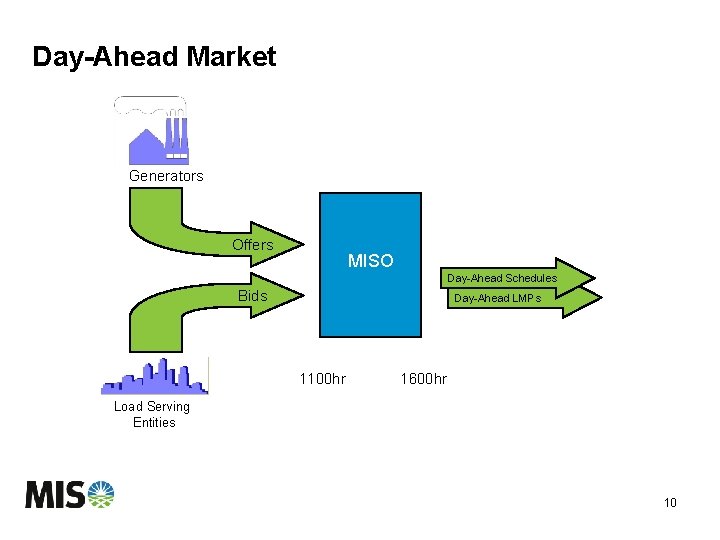
Day-Ahead Market Generators Offers MISO Day-Ahead Schedules Bids Day-Ahead LMPs 1100 hr 1600 hr Load Serving Entities 10
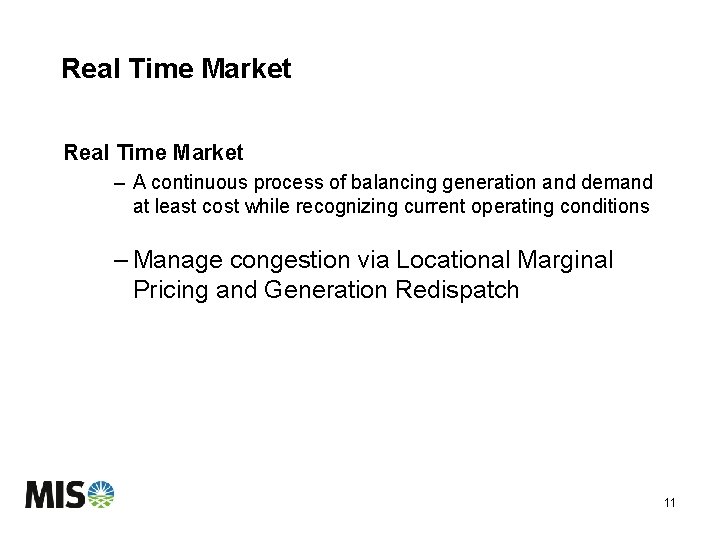
Real Time Market – A continuous process of balancing generation and demand at least cost while recognizing current operating conditions – Manage congestion via Locational Marginal Pricing and Generation Redispatch 11
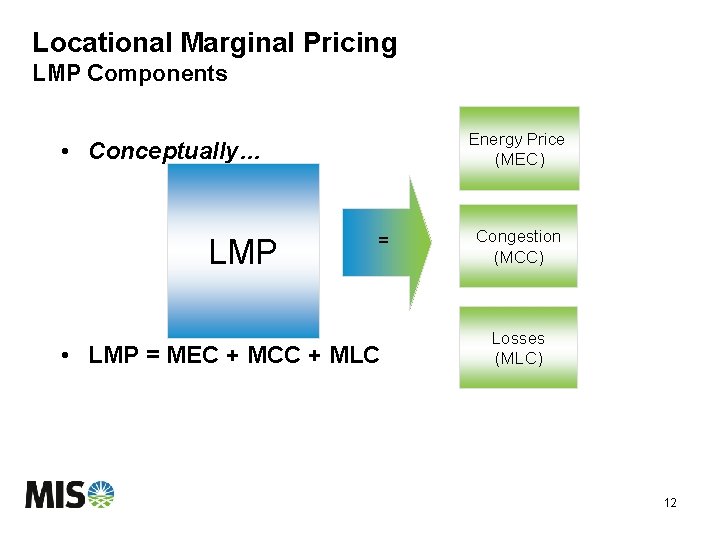
Locational Marginal Pricing LMP Components Energy Price (MEC) • Conceptually… LMP = • LMP = MEC + MCC + MLC Congestion (MCC) Losses (MLC) 12
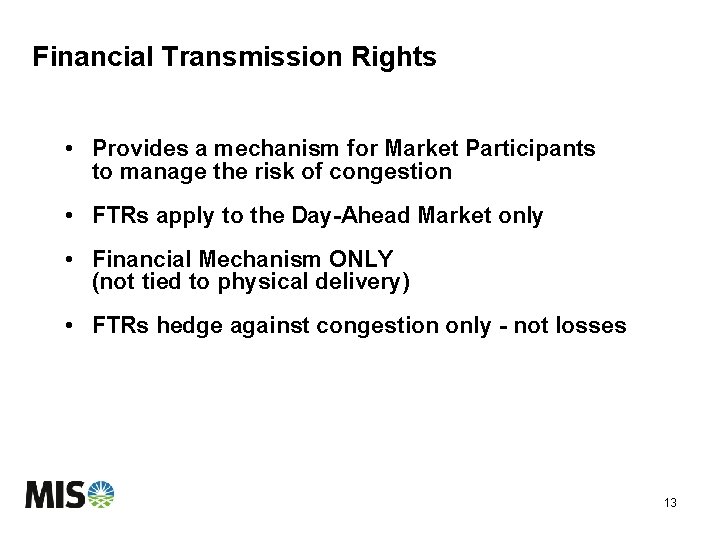
Financial Transmission Rights • Provides a mechanism for Market Participants to manage the risk of congestion • FTRs apply to the Day-Ahead Market only • Financial Mechanism ONLY (not tied to physical delivery) • FTRs hedge against congestion only - not losses 13
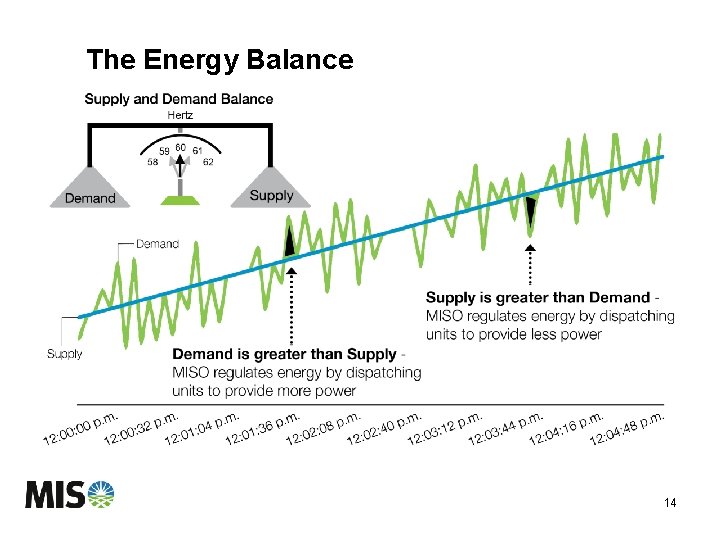
The Energy Balance 14
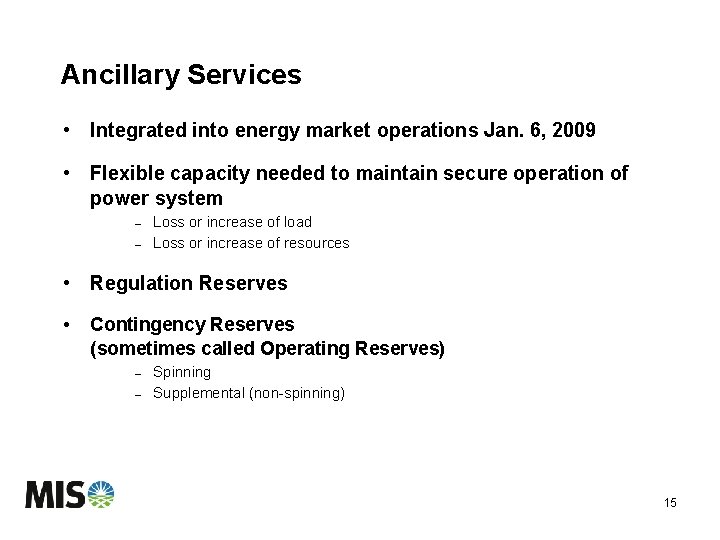
Ancillary Services • Integrated into energy market operations Jan. 6, 2009 • Flexible capacity needed to maintain secure operation of power system – – Loss or increase of load Loss or increase of resources • Regulation Reserves • Contingency Reserves (sometimes called Operating Reserves) – – Spinning Supplemental (non-spinning) 15
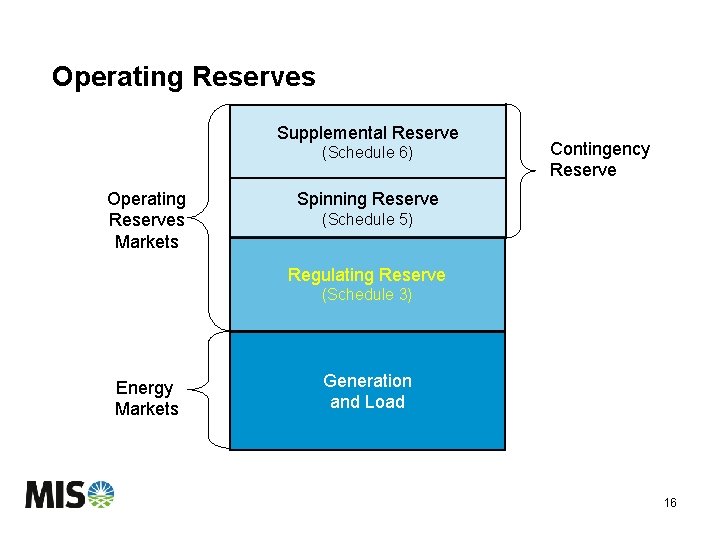
Operating Reserves Supplemental Reserve (Schedule 6) Operating Reserves Markets Contingency Reserve Spinning Reserve (Schedule 5) Regulating Reserve (Schedule 3) Energy Markets Generation and Load 16
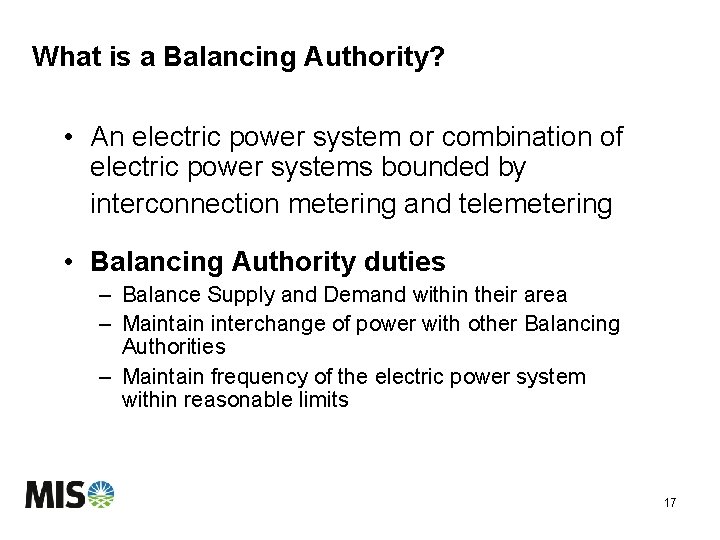
What is a Balancing Authority? • An electric power system or combination of electric power systems bounded by interconnection metering and telemetering • Balancing Authority duties – Balance Supply and Demand within their area – Maintain interchange of power with other Balancing Authorities – Maintain frequency of the electric power system within reasonable limits 17
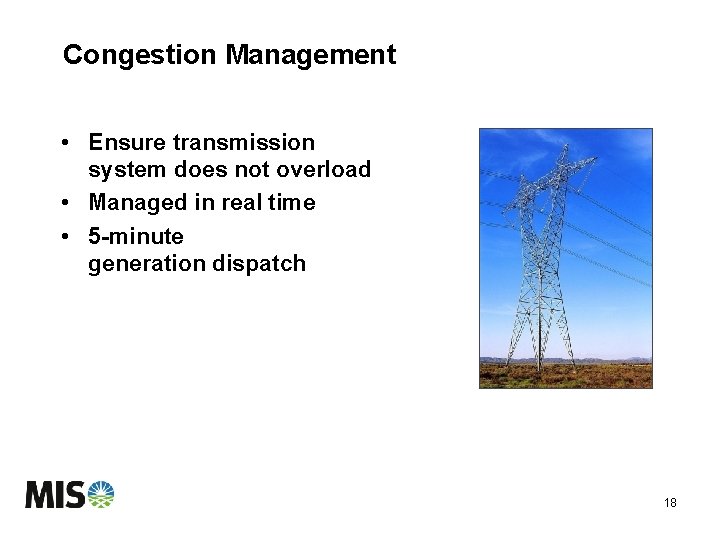
Congestion Management • Ensure transmission system does not overload • Managed in real time • 5 -minute generation dispatch 18
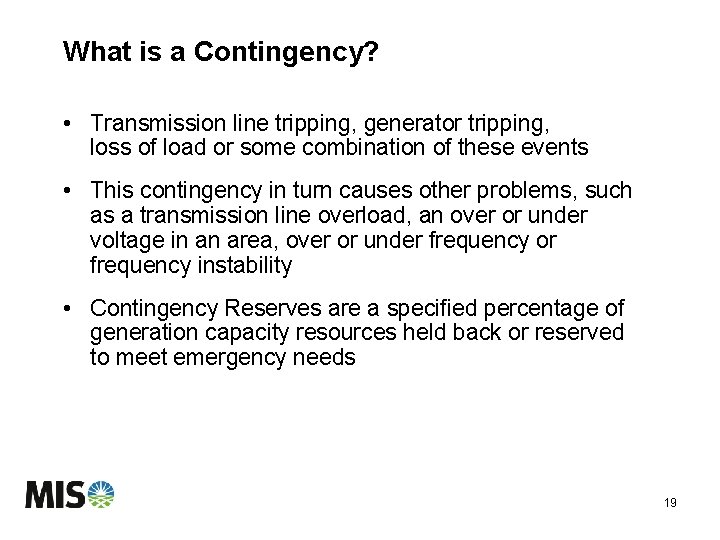
What is a Contingency? • Transmission line tripping, generator tripping, loss of load or some combination of these events • This contingency in turn causes other problems, such as a transmission line overload, an over or under voltage in an area, over or under frequency or frequency instability • Contingency Reserves are a specified percentage of generation capacity resources held back or reserved to meet emergency needs 19
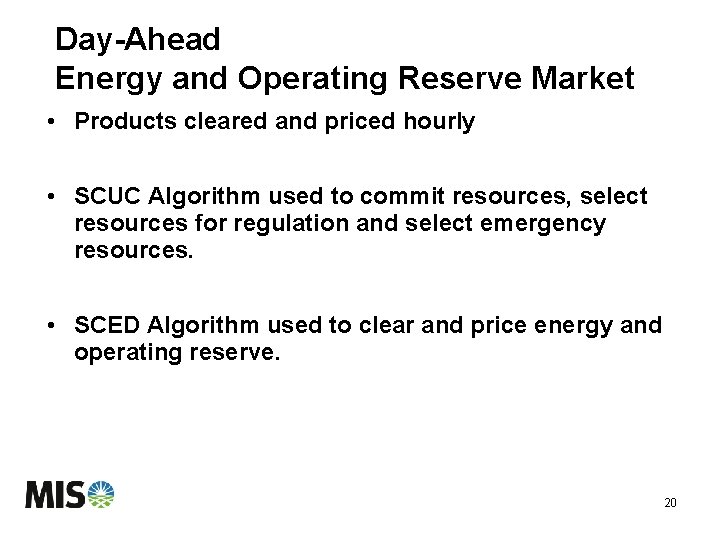
Day-Ahead Energy and Operating Reserve Market • Products cleared and priced hourly • SCUC Algorithm used to commit resources, select resources for regulation and select emergency resources. • SCED Algorithm used to clear and price energy and operating reserve. 20
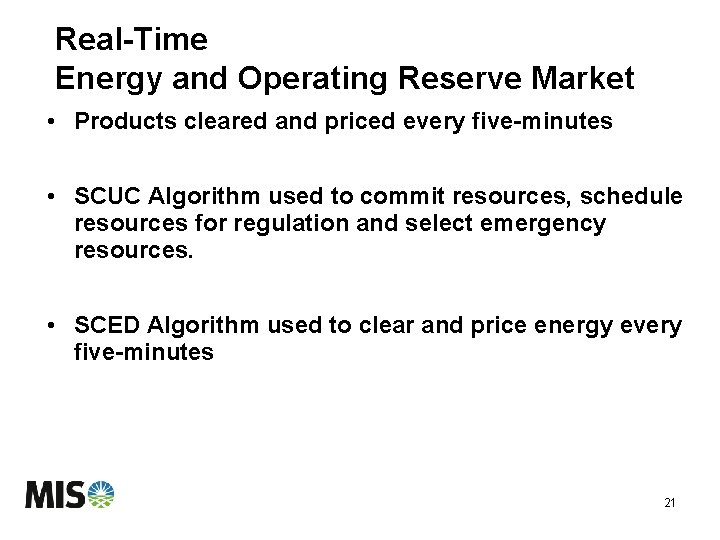
Real-Time Energy and Operating Reserve Market • Products cleared and priced every five-minutes • SCUC Algorithm used to commit resources, schedule resources for regulation and select emergency resources. • SCED Algorithm used to clear and price energy every five-minutes 21
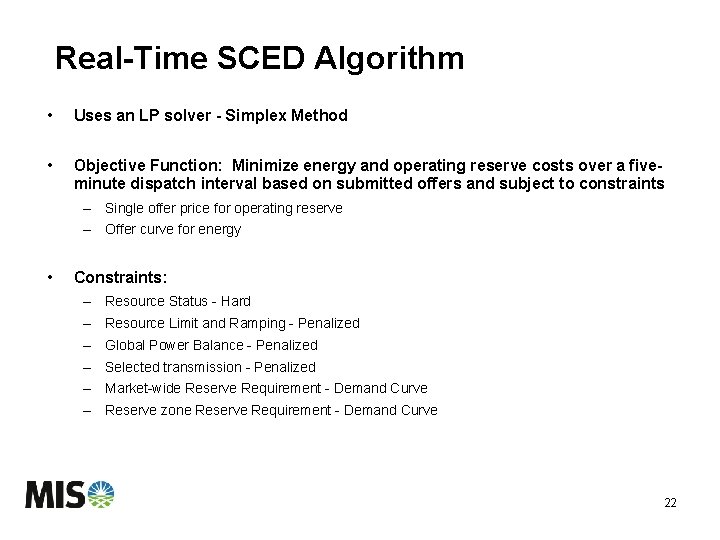
Real-Time SCED Algorithm • Uses an LP solver - Simplex Method • Objective Function: Minimize energy and operating reserve costs over a fiveminute dispatch interval based on submitted offers and subject to constraints – Single offer price for operating reserve – Offer curve for energy • Constraints: – Resource Status - Hard – Resource Limit and Ramping - Penalized – Global Power Balance - Penalized – Selected transmission - Penalized – Market-wide Reserve Requirement - Demand Curve – Reserve zone Reserve Requirement - Demand Curve 22
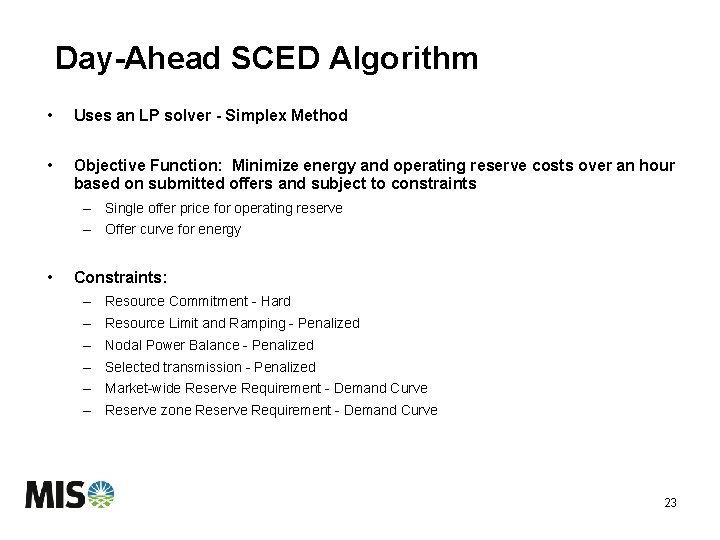
Day-Ahead SCED Algorithm • Uses an LP solver - Simplex Method • Objective Function: Minimize energy and operating reserve costs over an hour based on submitted offers and subject to constraints – Single offer price for operating reserve – Offer curve for energy • Constraints: – Resource Commitment - Hard – Resource Limit and Ramping - Penalized – Nodal Power Balance - Penalized – Selected transmission - Penalized – Market-wide Reserve Requirement - Demand Curve – Reserve zone Reserve Requirement - Demand Curve 23
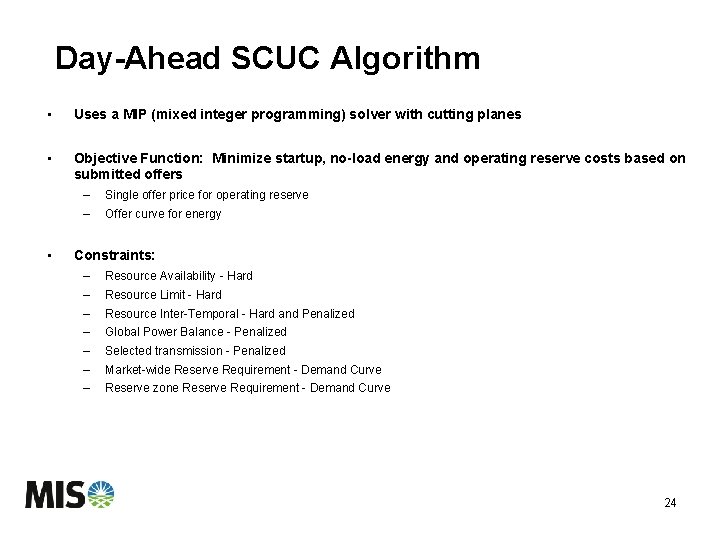
Day-Ahead SCUC Algorithm • Uses a MIP (mixed integer programming) solver with cutting planes • Objective Function: Minimize startup, no-load energy and operating reserve costs based on submitted offers – – • Single offer price for operating reserve Offer curve for energy Constraints: – – – – Resource Availability - Hard Resource Limit - Hard Resource Inter-Temporal - Hard and Penalized Global Power Balance - Penalized Selected transmission - Penalized Market-wide Reserve Requirement - Demand Curve Reserve zone Reserve Requirement - Demand Curve 24
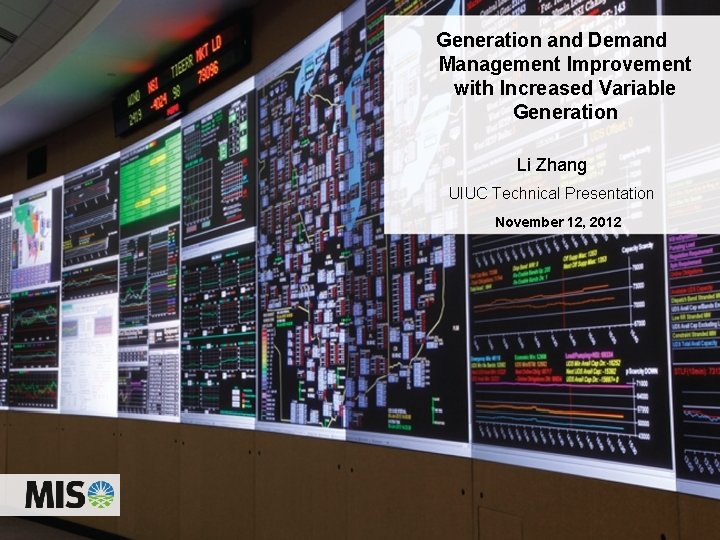
Generation and Demand Management Improvement with Increased Variable Generation Li Zhang UIUC Technical Presentation November 12, 2012
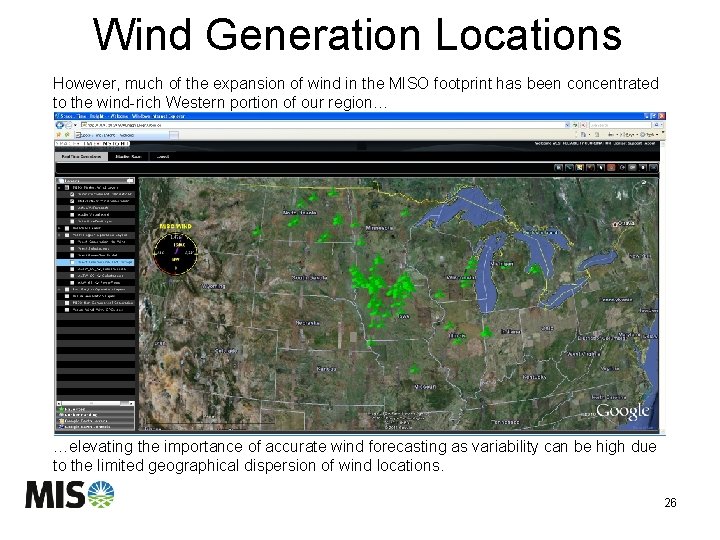
Wind Generation Locations However, much of the expansion of wind in the MISO footprint has been concentrated to the wind-rich Western portion of our region… …elevating the importance of accurate wind forecasting as variability can be high due to the limited geographical dispersion of wind locations. 26
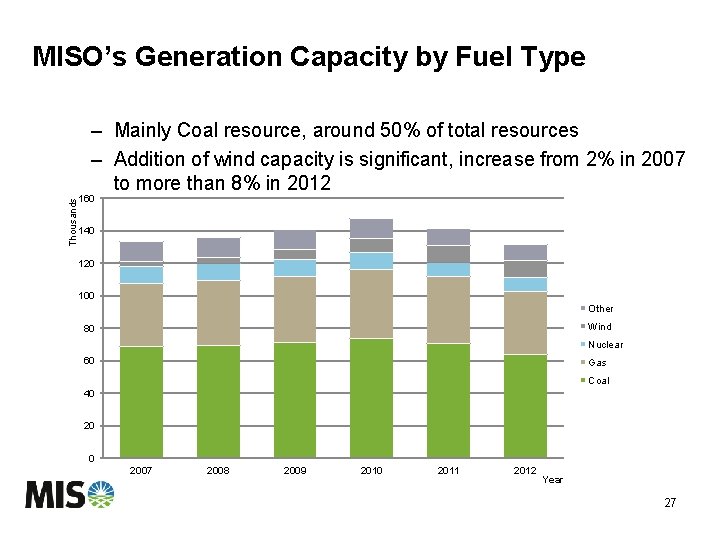
MISO’s Generation Capacity by Fuel Type Thousands – Mainly Coal resource, around 50% of total resources – Addition of wind capacity is significant, increase from 2% in 2007 to more than 8% in 2012 160 140 120 100 Other Wind 80 Nuclear 60 Gas Coal 40 2007 2008 2009 2010 2011 2012 Year 27
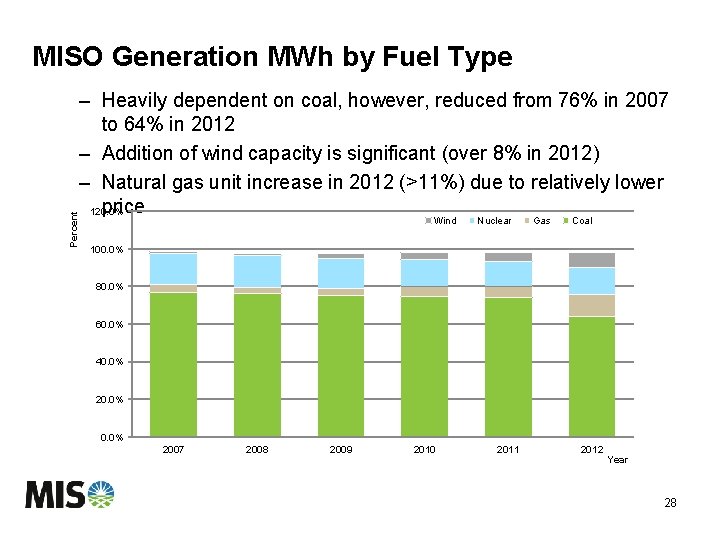
MISO Generation MWh by Fuel Type Percent – Heavily dependent on coal, however, reduced from 76% in 2007 to 64% in 2012 – Addition of wind capacity is significant (over 8% in 2012) – Natural gas unit increase in 2012 (>11%) due to relatively lower price 120. 0% Wind Nuclear Gas Coal 100. 0% 80. 0% 60. 0% 40. 0% 2007 2008 2009 2010 2011 2012 Year 28
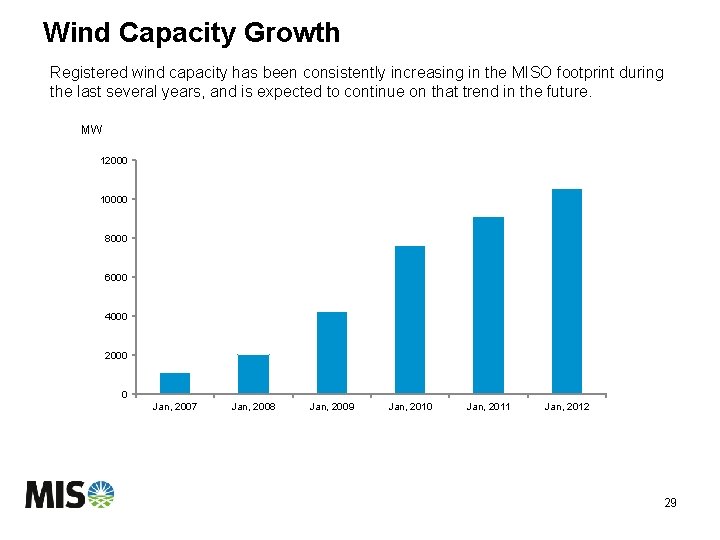
Wind Capacity Growth Registered wind capacity has been consistently increasing in the MISO footprint during the last several years, and is expected to continue on that trend in the future. MW 12000 10000 8000 6000 4000 2000 0 Jan, 2007 Jan, 2008 Jan, 2009 Jan, 2010 Jan, 2011 Jan, 2012 29
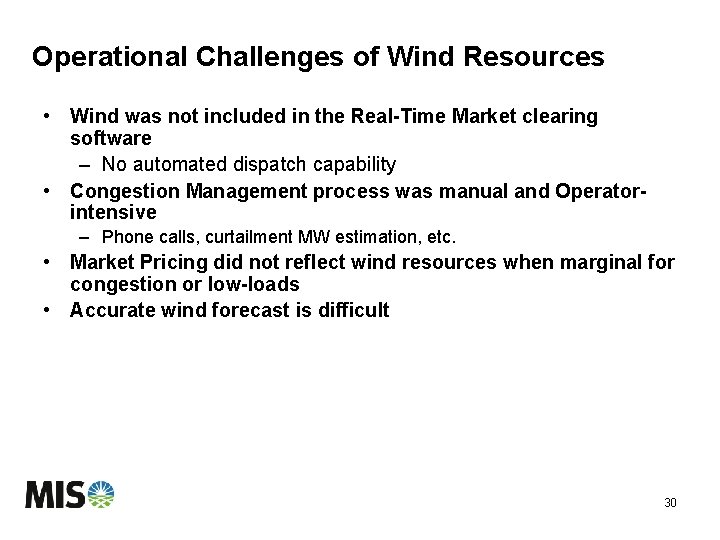
Operational Challenges of Wind Resources • Wind was not included in the Real-Time Market clearing software – No automated dispatch capability • Congestion Management process was manual and Operatorintensive – Phone calls, curtailment MW estimation, etc. • Market Pricing did not reflect wind resources when marginal for congestion or low-loads • Accurate wind forecast is difficult 30
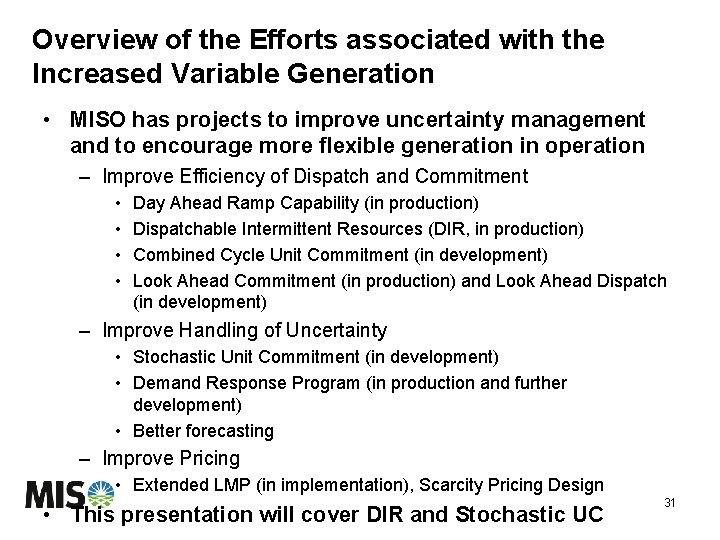
Overview of the Efforts associated with the Increased Variable Generation • MISO has projects to improve uncertainty management and to encourage more flexible generation in operation – Improve Efficiency of Dispatch and Commitment • • Day Ahead Ramp Capability (in production) Dispatchable Intermittent Resources (DIR, in production) Combined Cycle Unit Commitment (in development) Look Ahead Commitment (in production) and Look Ahead Dispatch (in development) – Improve Handling of Uncertainty • Stochastic Unit Commitment (in development) • Demand Response Program (in production and further development) • Better forecasting – Improve Pricing • Extended LMP (in implementation), Scarcity Pricing Design • This presentation will cover DIR and Stochastic UC 31
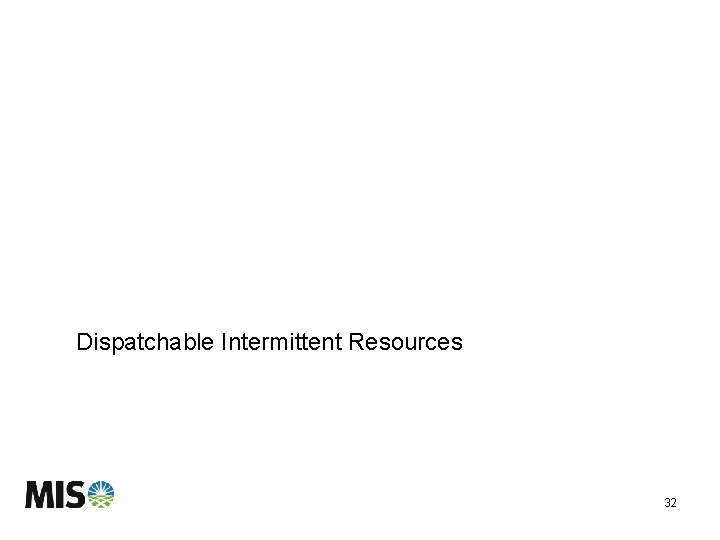
Dispatchable Intermittent Resources 32
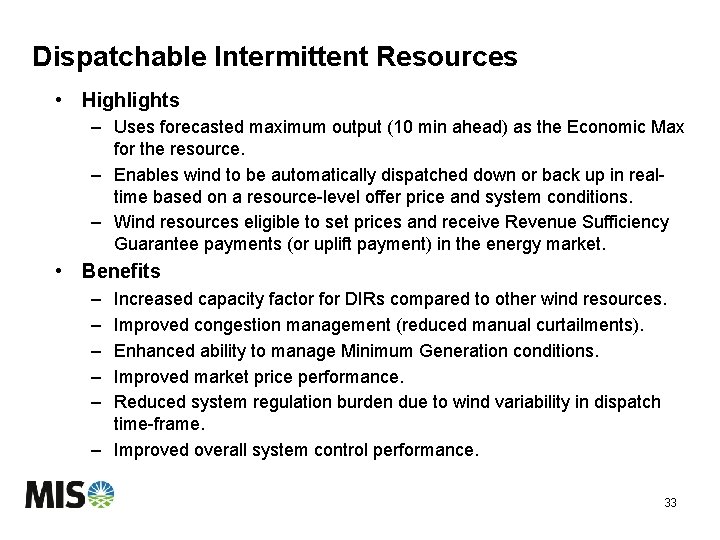
Dispatchable Intermittent Resources • Highlights – Uses forecasted maximum output (10 min ahead) as the Economic Max for the resource. – Enables wind to be automatically dispatched down or back up in realtime based on a resource-level offer price and system conditions. – Wind resources eligible to set prices and receive Revenue Sufficiency Guarantee payments (or uplift payment) in the energy market. • Benefits – – – Increased capacity factor for DIRs compared to other wind resources. Improved congestion management (reduced manual curtailments). Enhanced ability to manage Minimum Generation conditions. Improved market price performance. Reduced system regulation burden due to wind variability in dispatch time-frame. – Improved overall system control performance. 33
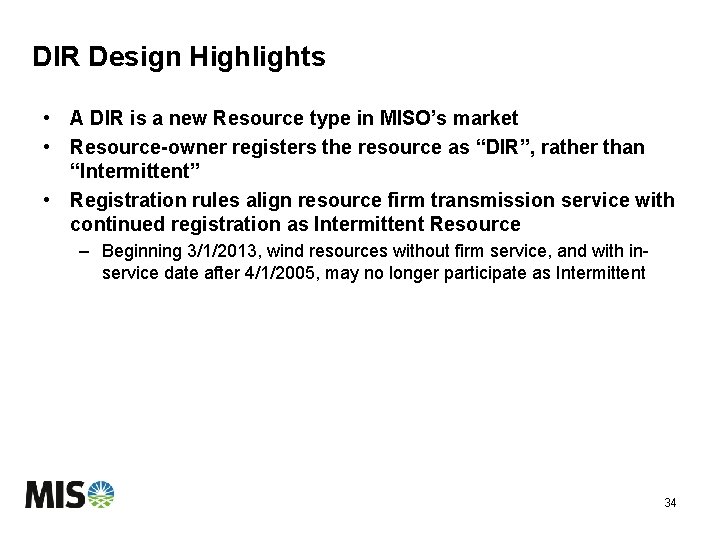
DIR Design Highlights • A DIR is a new Resource type in MISO’s market • Resource-owner registers the resource as “DIR”, rather than “Intermittent” • Registration rules align resource firm transmission service with continued registration as Intermittent Resource – Beginning 3/1/2013, wind resources without firm service, and with inservice date after 4/1/2005, may no longer participate as Intermittent 34
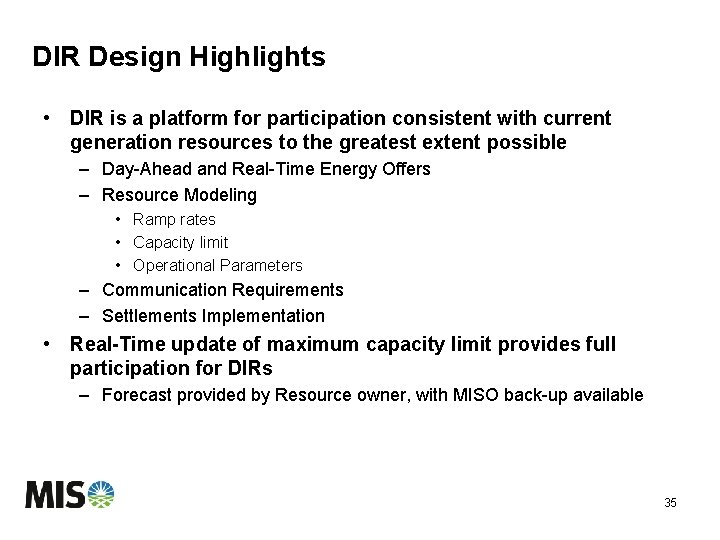
DIR Design Highlights • DIR is a platform for participation consistent with current generation resources to the greatest extent possible – Day-Ahead and Real-Time Energy Offers – Resource Modeling • Ramp rates • Capacity limit • Operational Parameters – Communication Requirements – Settlements Implementation • Real-Time update of maximum capacity limit provides full participation for DIRs – Forecast provided by Resource owner, with MISO back-up available 35
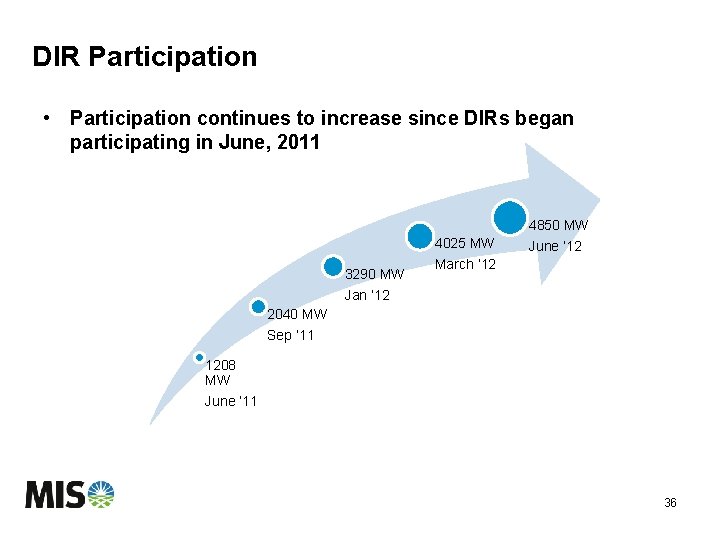
DIR Participation • Participation continues to increase since DIRs began participating in June, 2011 4850 MW 4025 MW 3290 MW June ‘ 12 March ‘ 12 Jan ‘ 12 2040 MW Sep ‘ 11 1208 MW June ‘ 11 36
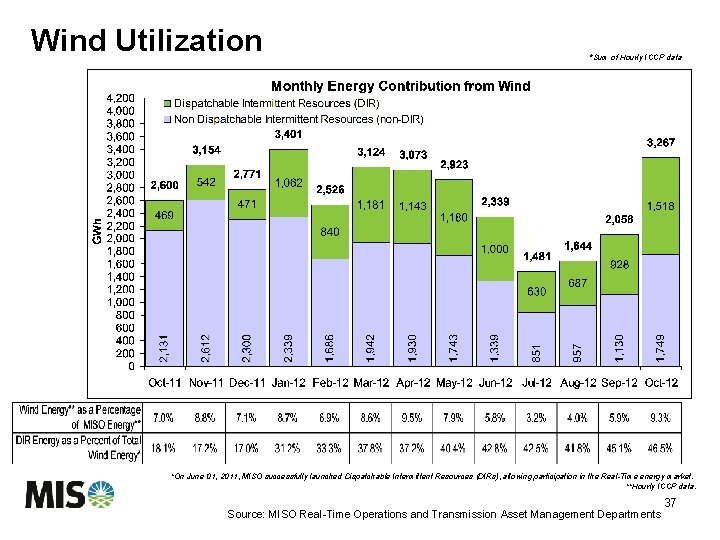
Wind Utilization *Sum of Hourly ICCP data *On June 01, 2011, MISO successfully launched Dispatchable Intermittent Resources (DIRs), allowing participation in the Real-Time energy market. **Hourly ICCP data. Source: MISO Real-Time Operations and Transmission Asset Management Departments 37
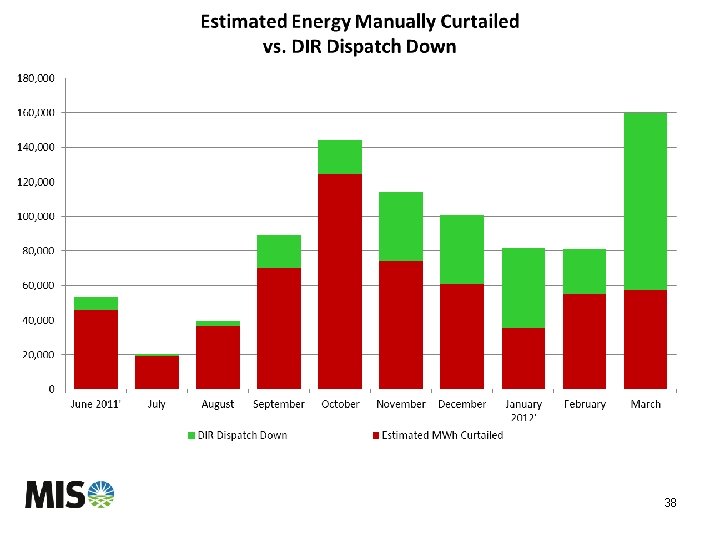
38
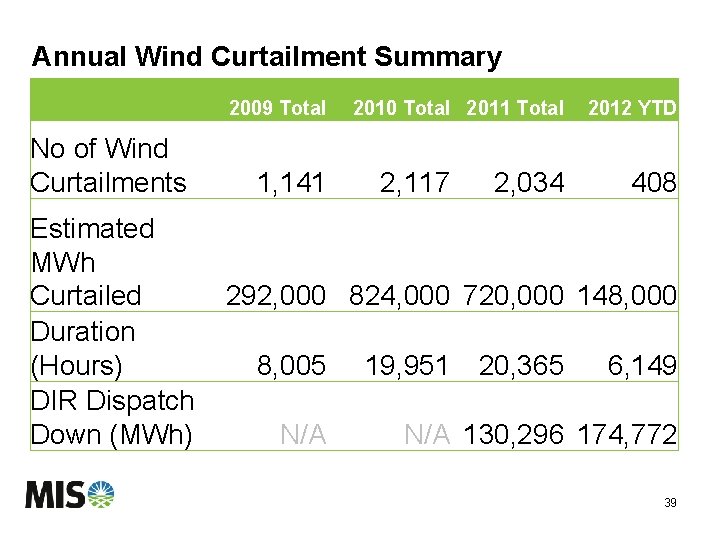
Annual Wind Curtailment Summary 2009 Total No of Wind Curtailments Estimated MWh Curtailed Duration (Hours) DIR Dispatch Down (MWh) 1, 141 2010 Total 2011 Total 2, 117 2, 034 2012 YTD 408 292, 000 824, 000 720, 000 148, 000 8, 005 N/A 19, 951 20, 365 6, 149 N/A 130, 296 174, 772 39
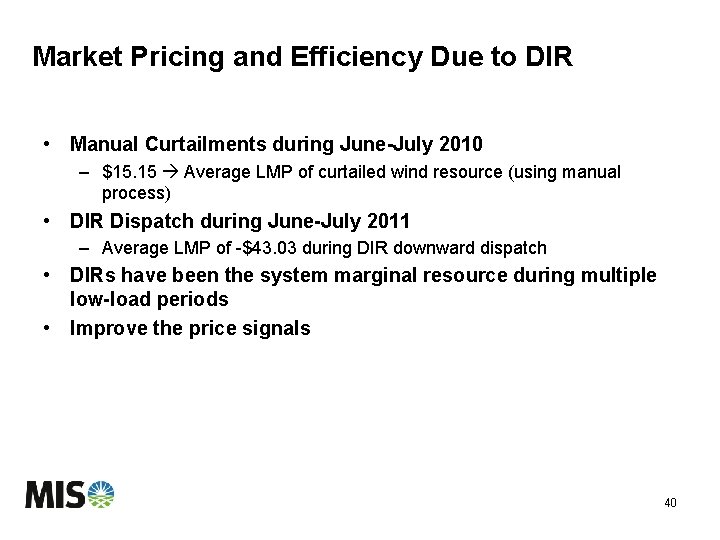
Market Pricing and Efficiency Due to DIR • Manual Curtailments during June-July 2010 – $15. 15 Average LMP of curtailed wind resource (using manual process) • DIR Dispatch during June-July 2011 – Average LMP of -$43. 03 during DIR downward dispatch • DIRs have been the system marginal resource during multiple low-load periods • Improve the price signals 40
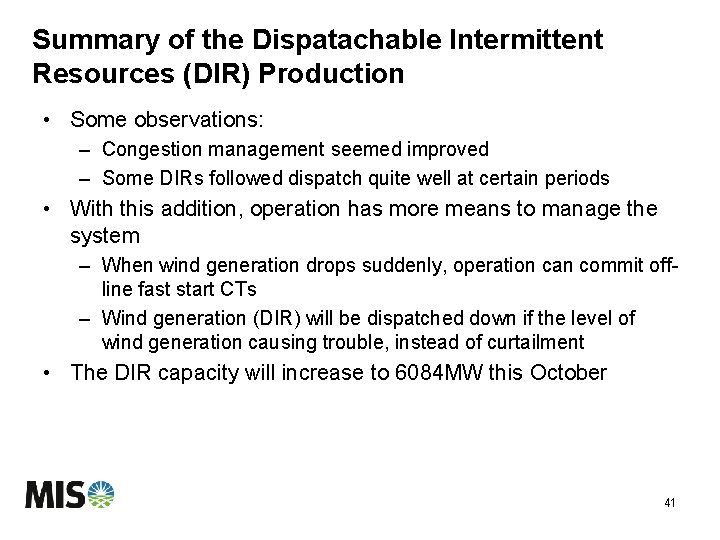
Summary of the Dispatachable Intermittent Resources (DIR) Production • Some observations: – Congestion management seemed improved – Some DIRs followed dispatch quite well at certain periods • With this addition, operation has more means to manage the system – When wind generation drops suddenly, operation can commit offline fast start CTs – Wind generation (DIR) will be dispatched down if the level of wind generation causing trouble, instead of curtailment • The DIR capacity will increase to 6084 MW this October 41
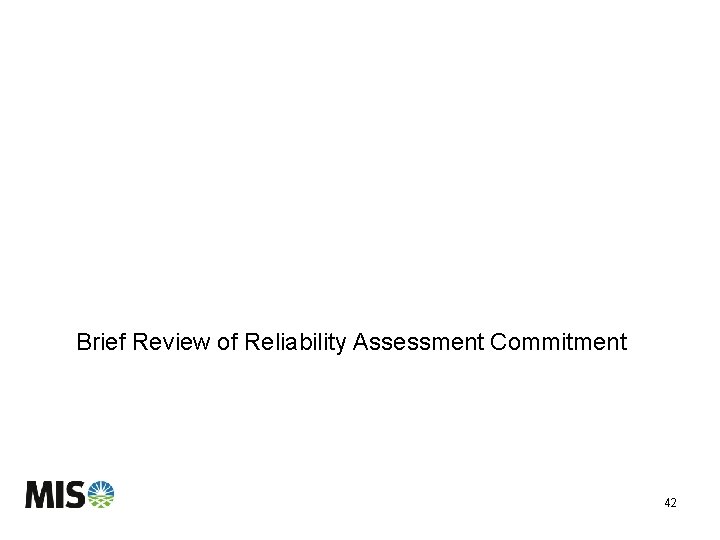
Brief Review of Reliability Assessment Commitment 42
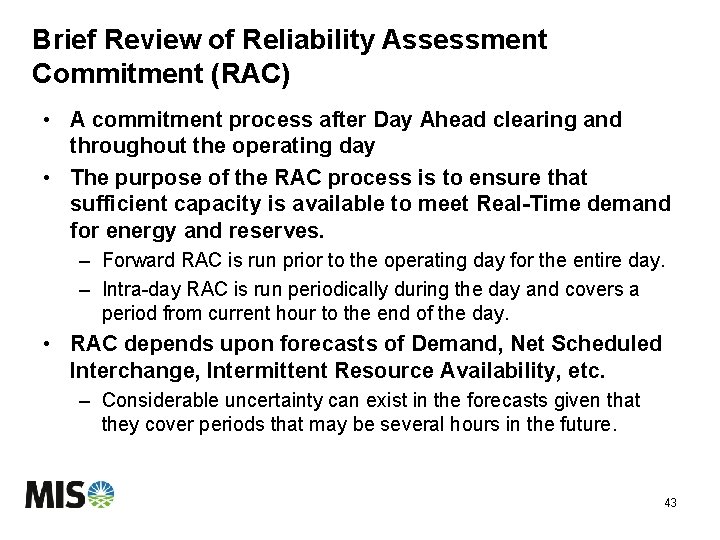
Brief Review of Reliability Assessment Commitment (RAC) • A commitment process after Day Ahead clearing and throughout the operating day • The purpose of the RAC process is to ensure that sufficient capacity is available to meet Real-Time demand for energy and reserves. – Forward RAC is run prior to the operating day for the entire day. – Intra-day RAC is run periodically during the day and covers a period from current hour to the end of the day. • RAC depends upon forecasts of Demand, Net Scheduled Interchange, Intermittent Resource Availability, etc. – Considerable uncertainty can exist in the forecasts given that they cover periods that may be several hours in the future. 43
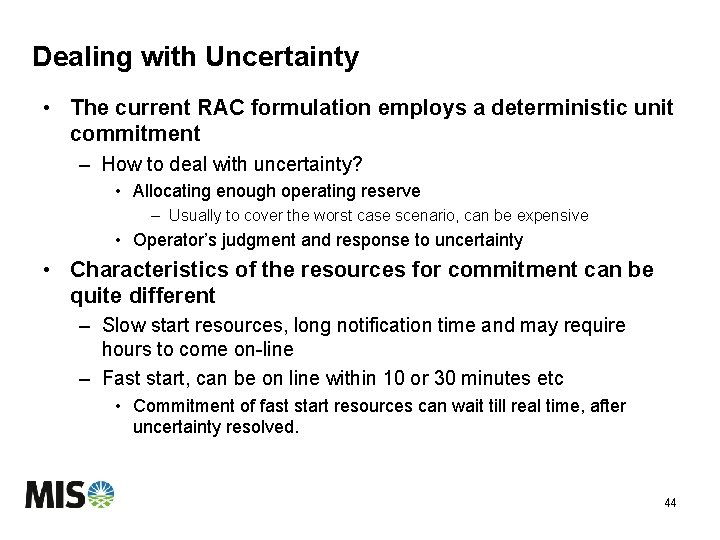
Dealing with Uncertainty • The current RAC formulation employs a deterministic unit commitment – How to deal with uncertainty? • Allocating enough operating reserve – Usually to cover the worst case scenario, can be expensive • Operator’s judgment and response to uncertainty • Characteristics of the resources for commitment can be quite different – Slow start resources, long notification time and may require hours to come on-line – Fast start, can be on line within 10 or 30 minutes etc • Commitment of fast start resources can wait till real time, after uncertainty resolved. 44
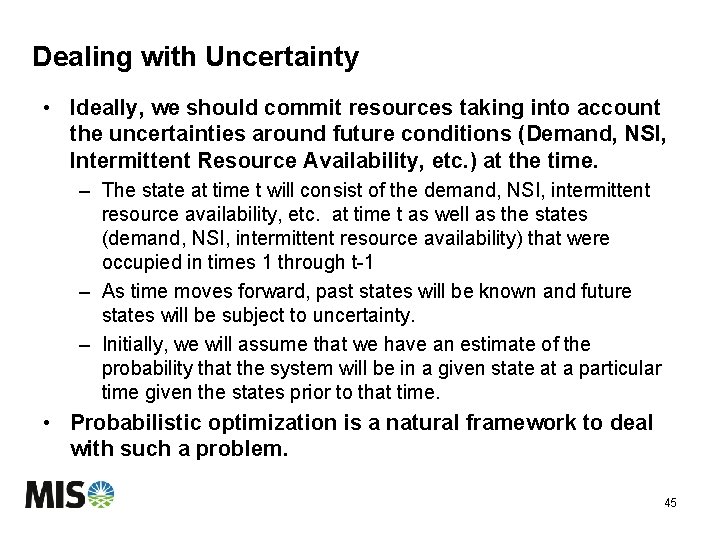
Dealing with Uncertainty • Ideally, we should commit resources taking into account the uncertainties around future conditions (Demand, NSI, Intermittent Resource Availability, etc. ) at the time. – The state at time t will consist of the demand, NSI, intermittent resource availability, etc. at time t as well as the states (demand, NSI, intermittent resource availability) that were occupied in times 1 through t-1 – As time moves forward, past states will be known and future states will be subject to uncertainty. – Initially, we will assume that we have an estimate of the probability that the system will be in a given state at a particular time given the states prior to that time. • Probabilistic optimization is a natural framework to deal with such a problem. 45
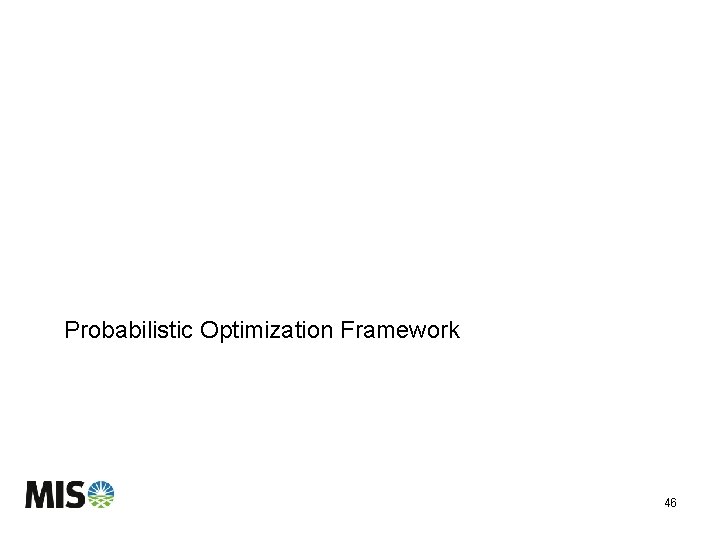
Probabilistic Optimization Framework 46
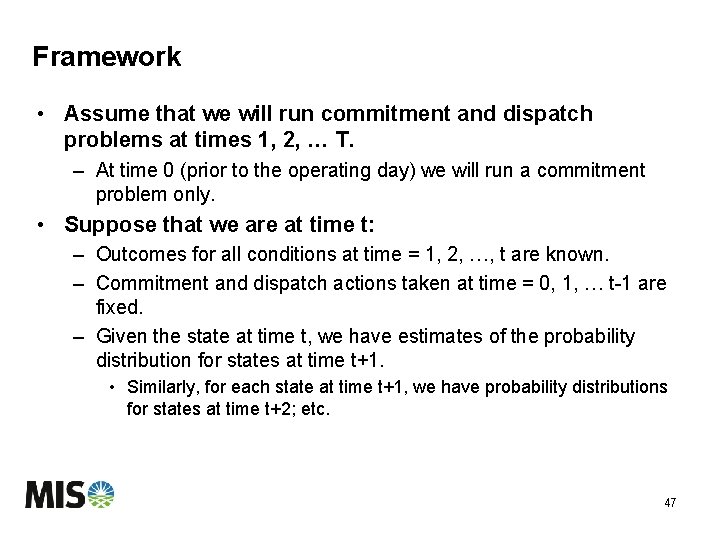
Framework • Assume that we will run commitment and dispatch problems at times 1, 2, … T. – At time 0 (prior to the operating day) we will run a commitment problem only. • Suppose that we are at time t: – Outcomes for all conditions at time = 1, 2, …, t are known. – Commitment and dispatch actions taken at time = 0, 1, … t-1 are fixed. – Given the state at time t, we have estimates of the probability distribution for states at time t+1. • Similarly, for each state at time t+1, we have probability distributions for states at time t+2; etc. 47
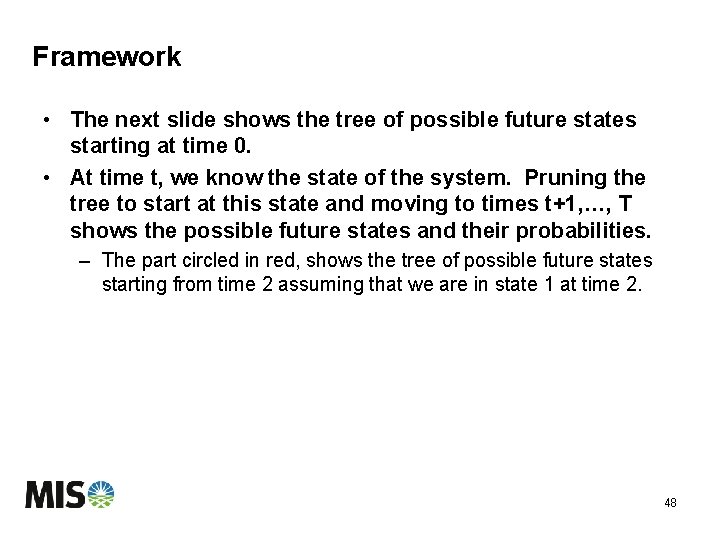
Framework • The next slide shows the tree of possible future states starting at time 0. • At time t, we know the state of the system. Pruning the tree to start at this state and moving to times t+1, …, T shows the possible future states and their probabilities. – The part circled in red, shows the tree of possible future states starting from time 2 assuming that we are in state 1 at time 2. 48
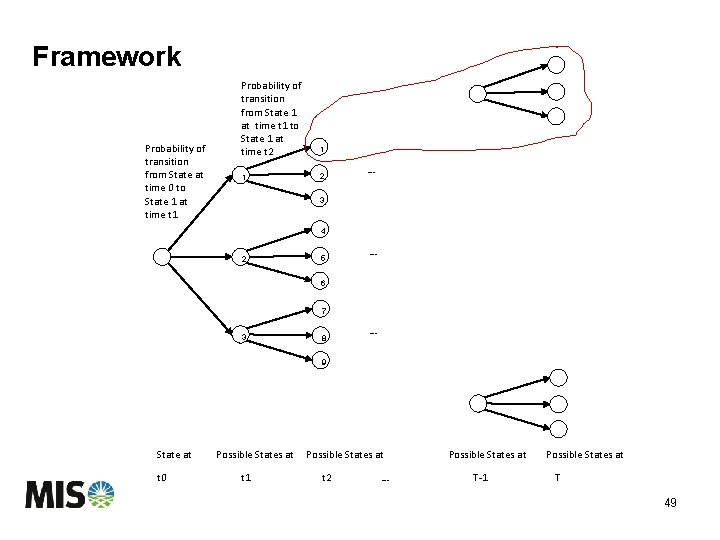
Framework Probability of transition from State at time 0 to State 1 at time t 1 Probability of transition from State 1 at time t 1 to State 1 at time t 2 1 1 2 … 3 4 2 5 … 6 7 3 8 … 9 State at Possible States at t 0 t 1 t 2 … T-1 T 49
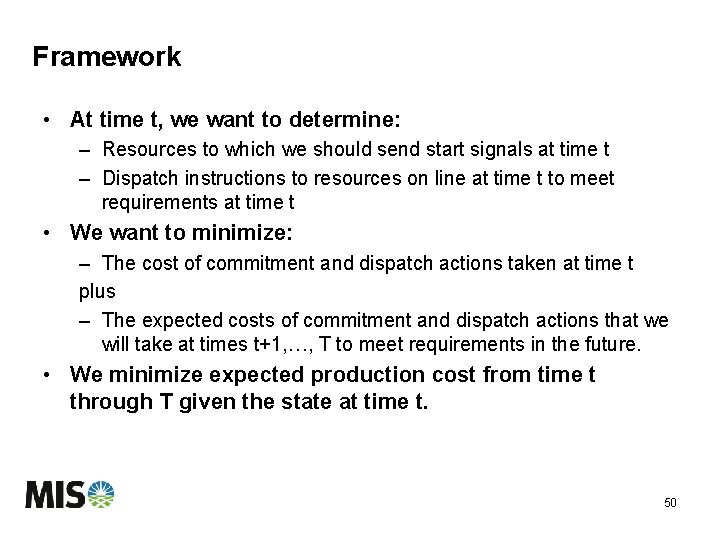
Framework • At time t, we want to determine: – Resources to which we should send start signals at time t – Dispatch instructions to resources on line at time t to meet requirements at time t • We want to minimize: – The cost of commitment and dispatch actions taken at time t plus – The expected costs of commitment and dispatch actions that we will take at times t+1, …, T to meet requirements in the future. • We minimize expected production cost from time t through T given the state at time t. 50
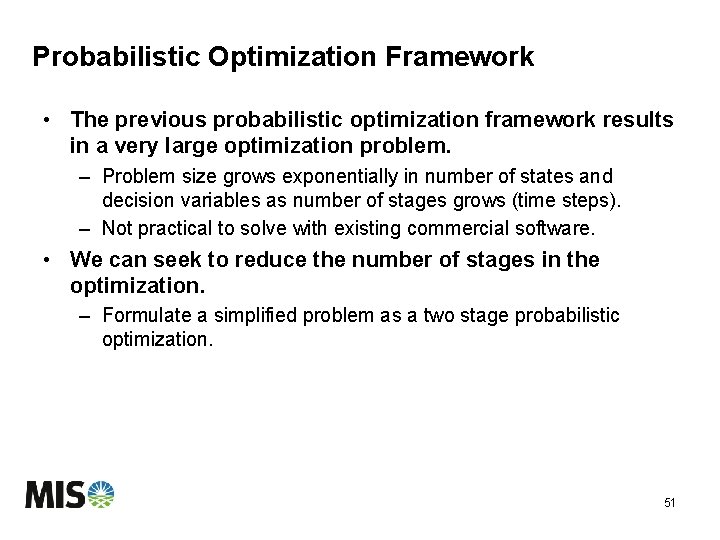
Probabilistic Optimization Framework • The previous probabilistic optimization framework results in a very large optimization problem. – Problem size grows exponentially in number of states and decision variables as number of stages grows (time steps). – Not practical to solve with existing commercial software. • We can seek to reduce the number of stages in the optimization. – Formulate a simplified problem as a two stage probabilistic optimization. 51
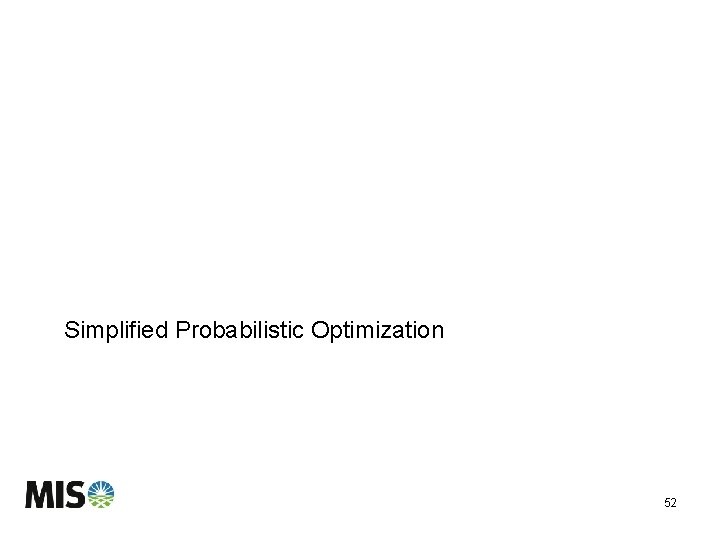
Simplified Probabilistic Optimization 52
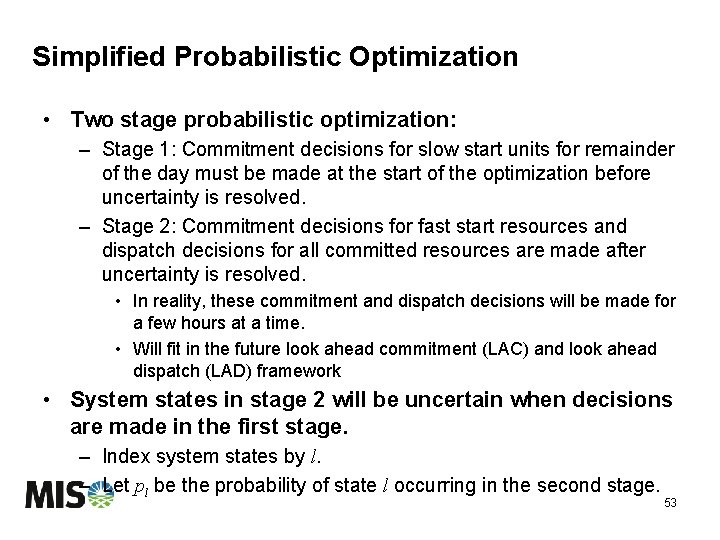
Simplified Probabilistic Optimization • Two stage probabilistic optimization: – Stage 1: Commitment decisions for slow start units for remainder of the day must be made at the start of the optimization before uncertainty is resolved. – Stage 2: Commitment decisions for fast start resources and dispatch decisions for all committed resources are made after uncertainty is resolved. • In reality, these commitment and dispatch decisions will be made for a few hours at a time. • Will fit in the future look ahead commitment (LAC) and look ahead dispatch (LAD) framework • System states in stage 2 will be uncertain when decisions are made in the first stage. – Index system states by l. – Let pl be the probability of state l occurring in the second stage. 53
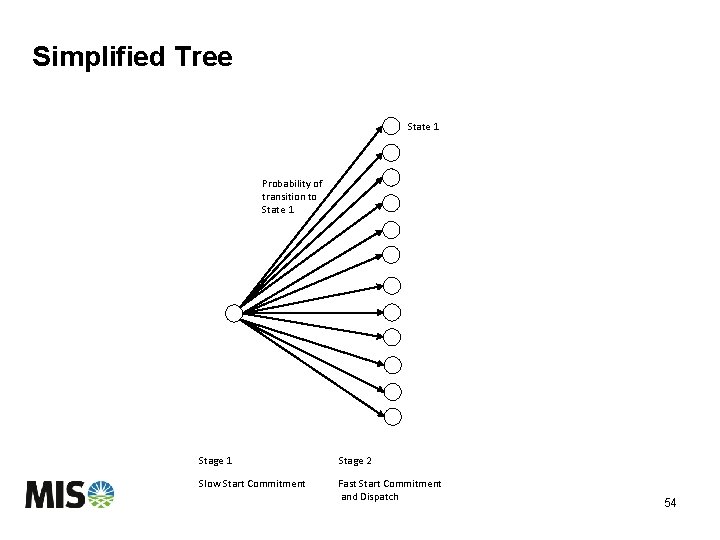
Simplified Tree State 1 Probability of transition to State 1 Stage 1 Stage 2 Slow Start Commitment Fast Start Commitment and Dispatch 54
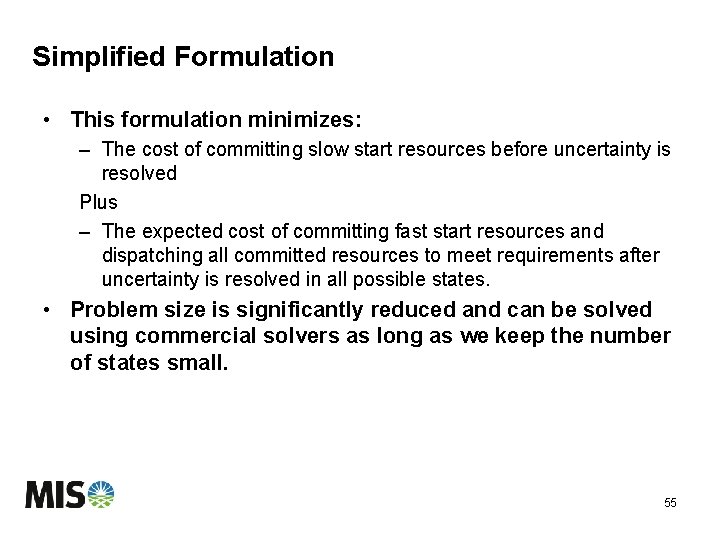
Simplified Formulation • This formulation minimizes: – The cost of committing slow start resources before uncertainty is resolved Plus – The expected cost of committing fast start resources and dispatching all committed resources to meet requirements after uncertainty is resolved in all possible states. • Problem size is significantly reduced and can be solved using commercial solvers as long as we keep the number of states small. 55
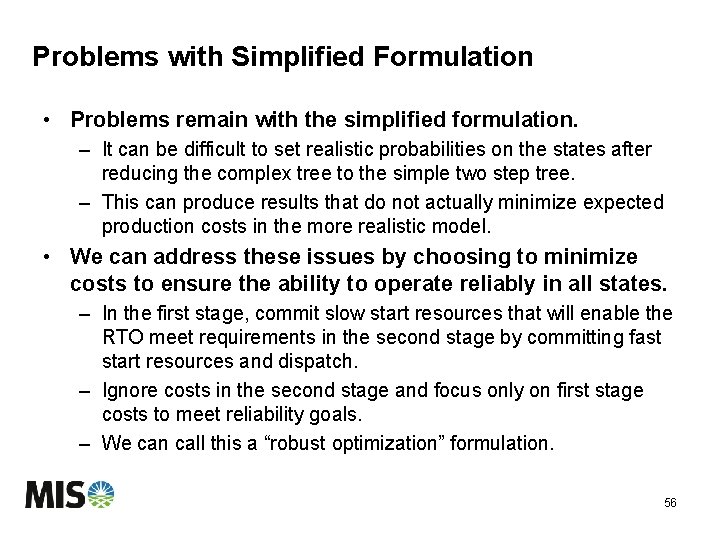
Problems with Simplified Formulation • Problems remain with the simplified formulation. – It can be difficult to set realistic probabilities on the states after reducing the complex tree to the simple two step tree. – This can produce results that do not actually minimize expected production costs in the more realistic model. • We can address these issues by choosing to minimize costs to ensure the ability to operate reliably in all states. – In the first stage, commit slow start resources that will enable the RTO meet requirements in the second stage by committing fast start resources and dispatch. – Ignore costs in the second stage and focus only on first stage costs to meet reliability goals. – We can call this a “robust optimization” formulation. 56
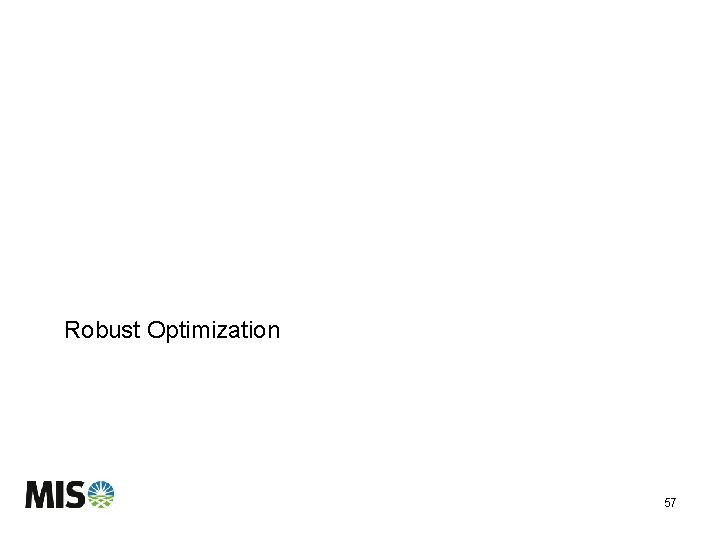
Robust Optimization 57
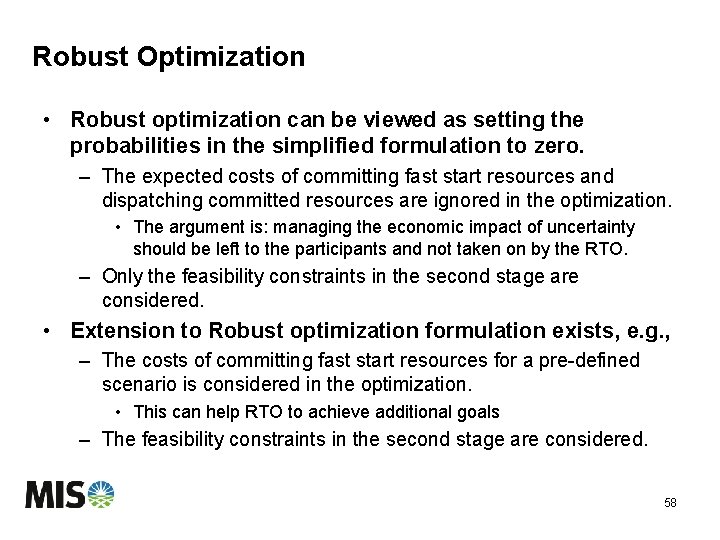
Robust Optimization • Robust optimization can be viewed as setting the probabilities in the simplified formulation to zero. – The expected costs of committing fast start resources and dispatching committed resources are ignored in the optimization. • The argument is: managing the economic impact of uncertainty should be left to the participants and not taken on by the RTO. – Only the feasibility constraints in the second stage are considered. • Extension to Robust optimization formulation exists, e. g. , – The costs of committing fast start resources for a pre-defined scenario is considered in the optimization. • This can help RTO to achieve additional goals – The feasibility constraints in the second stage are considered. 58
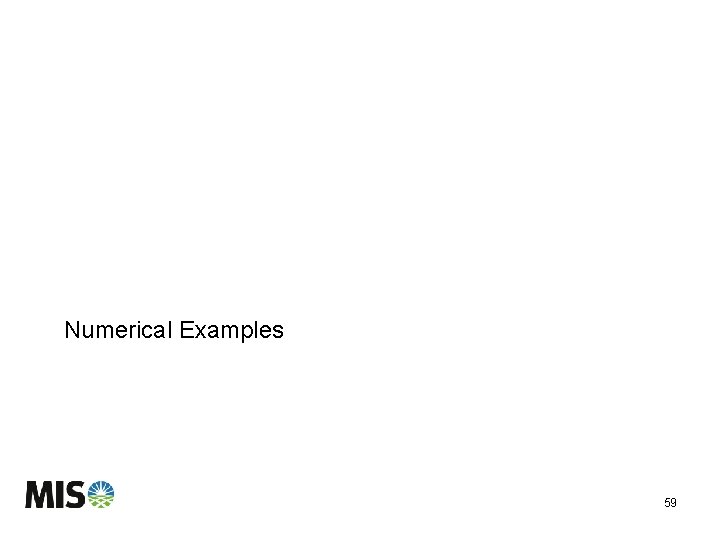
Numerical Examples 59
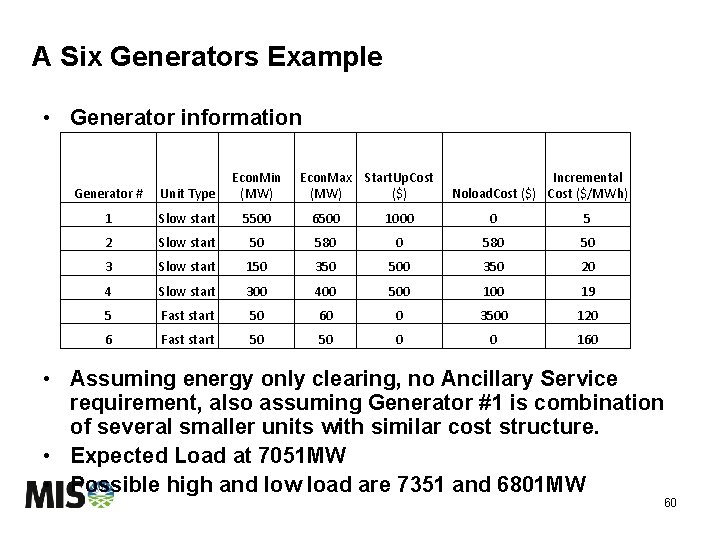
A Six Generators Example • Generator information Generator # Unit Type Econ. Min (MW) Econ. Max Start. Up. Cost (MW) ($) Incremental Noload. Cost ($) Cost ($/MWh) 1 Slow start 5500 6500 1000 0 5 2 Slow start 50 580 50 3 Slow start 150 350 500 350 20 4 Slow start 300 400 500 19 5 Fast start 50 60 0 3500 120 6 Fast start 50 50 0 0 160 • Assuming energy only clearing, no Ancillary Service requirement, also assuming Generator #1 is combination of several smaller units with similar cost structure. • Expected Load at 7051 MW • Possible high and low load are 7351 and 6801 MW 60
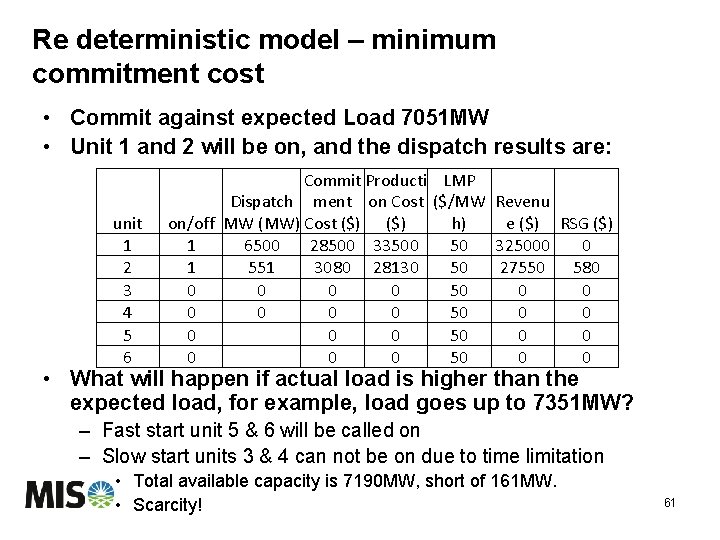
Re deterministic model – minimum commitment cost • Commit against expected Load 7051 MW • Unit 1 and 2 will be on, and the dispatch results are: unit 1 2 3 4 5 6 Commit Producti LMP Dispatch ment on Cost ($/MW Revenu on/off MW (MW) Cost ($) h) e ($) RSG ($) 1 6500 28500 33500 50 325000 0 1 551 3080 28130 50 27550 580 0 0 0 0 50 0 0 0 50 0 0 • What will happen if actual load is higher than the expected load, for example, load goes up to 7351 MW? – Fast start unit 5 & 6 will be called on – Slow start units 3 & 4 can not be on due to time limitation • Total available capacity is 7190 MW, short of 161 MW. • Scarcity! 61
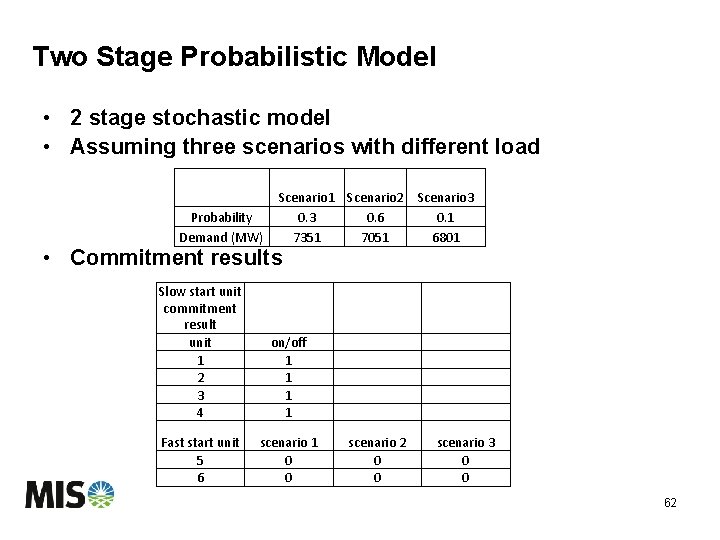
Two Stage Probabilistic Model • 2 stage stochastic model • Assuming three scenarios with different load Probability Demand (MW) Scenario 1 Scenario 2 0. 3 0. 6 7351 7051 • Commitment results Scenario 3 0. 1 6801 Slow start unit commitment result unit 1 2 3 4 on/off 1 1 Fast start unit 5 6 scenario 1 0 0 scenario 2 0 0 scenario 3 0 0 62
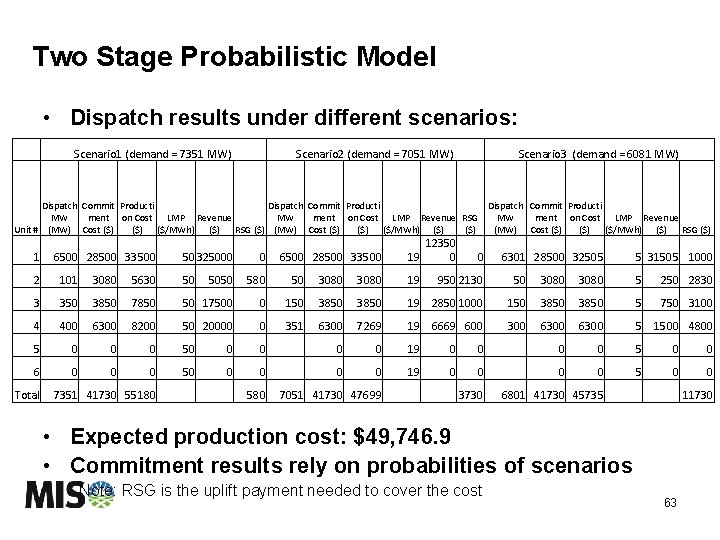
Two Stage Probabilistic Model • Dispatch results under different scenarios: Scenario 1 (demand = 7351 MW) Scenario 2 (demand = 7051 MW) Scenario 3 (demand = 6081 MW) Dispatch Commit Producti MW ment on Cost LMP Revenue RSG MW ment on Cost LMP Revenue Unit # (MW) Cost ($) ($/MWh) ($) RSG ($) (MW) Cost ($) ($) ($/MWh) ($) RSG ($) 1 6500 28500 33500 50 325000 0 6500 28500 33500 12350 19 0 0 6301 28500 32505 5 31505 1000 2 101 3080 5630 50 5050 580 50 3080 19 950 2130 50 3080 5 250 2830 3 350 3850 7850 50 17500 0 150 3850 19 2850 1000 150 3850 5 750 3100 4 400 6300 8200 50 20000 0 351 6300 7269 19 6669 600 300 6300 5 1500 4800 5 0 0 0 50 0 0 19 0 0 5 0 0 6 0 0 0 50 0 0 19 0 0 5 0 0 Total 7351 41730 55180 580 7051 41730 47699 3730 6801 41730 45735 11730 • Expected production cost: $49, 746. 9 • Commitment results rely on probabilities of scenarios Note: RSG is the uplift payment needed to cover the cost 63
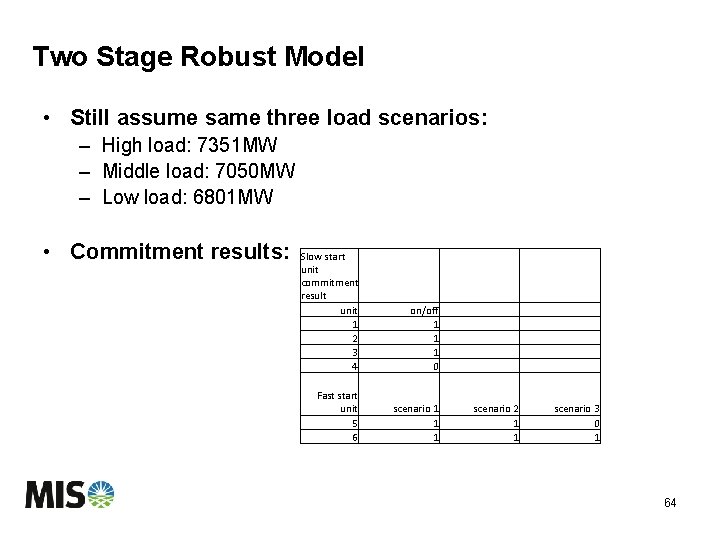
Two Stage Robust Model • Still assume same three load scenarios: – High load: 7351 MW – Middle load: 7050 MW – Low load: 6801 MW • Commitment results: Slow start unit commitment result unit 1 2 3 4 on/off 1 1 1 0 Fast start unit 5 6 scenario 1 1 1 scenario 2 1 1 scenario 3 0 1 64
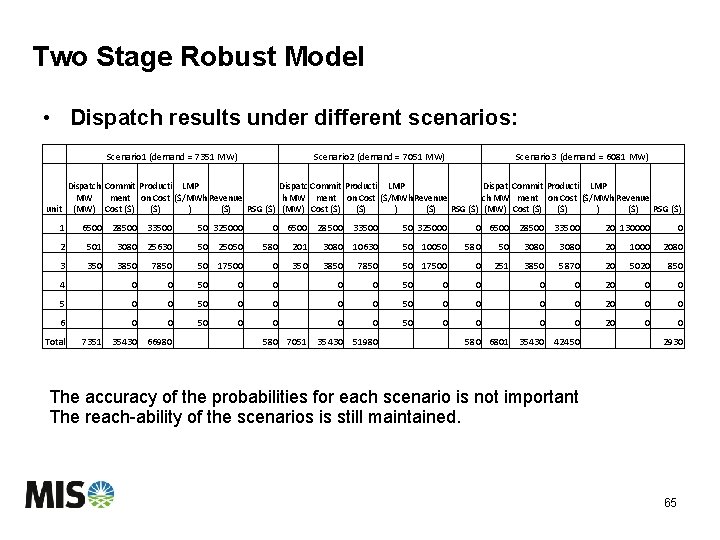
Two Stage Robust Model • Dispatch results under different scenarios: Scenario 1 (demand = 7351 MW) Scenario 2 (demand = 7051 MW) Scenario 3 (demand = 6081 MW) Dispatch Commit Producti LMP Dispatc Commit Producti LMP Dispat Commit Producti LMP MW ment on Cost ($/MWh Revenue h MW ment on Cost ($/MWh Revenue ch MW ment on Cost ($/MWh Revenue unit (MW) Cost ($) ($) RSG ($) (MW) Cost ($) ) ($) RSG ($) 1 6500 28500 33500 50 325000 0 6500 28500 33500 20 130000 0 2 501 3080 25630 50 25050 580 201 3080 10630 50 10050 580 50 3080 20 1000 2080 3 350 3850 7850 50 17500 0 251 3850 5870 20 5020 850 4 0 0 50 0 0 20 0 0 50 0 0 20 0 0 6 0 0 50 0 0 20 0 0 Total 7351 35430 66980 580 7051 35430 51980 580 6801 35430 42450 2930 The accuracy of the probabilities for each scenario is not important The reach-ability of the scenarios is still maintained. 65
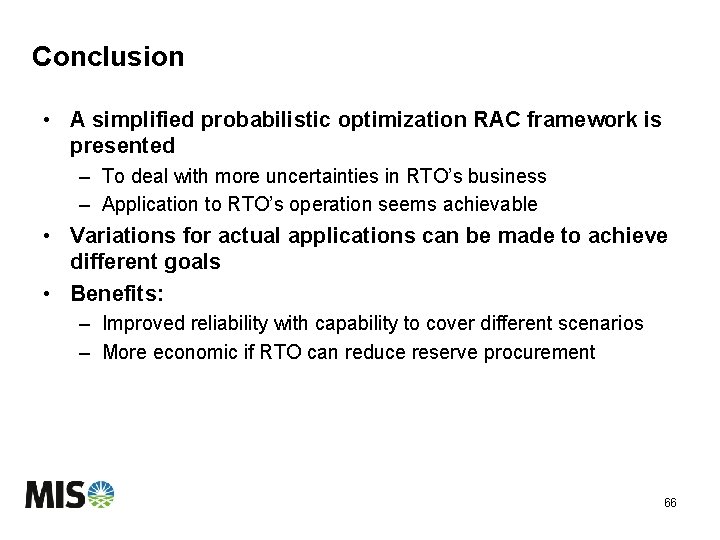
Conclusion • A simplified probabilistic optimization RAC framework is presented – To deal with more uncertainties in RTO’s business – Application to RTO’s operation seems achievable • Variations for actual applications can be made to achieve different goals • Benefits: – Improved reliability with capability to cover different scenarios – More economic if RTO can reduce reserve procurement 66
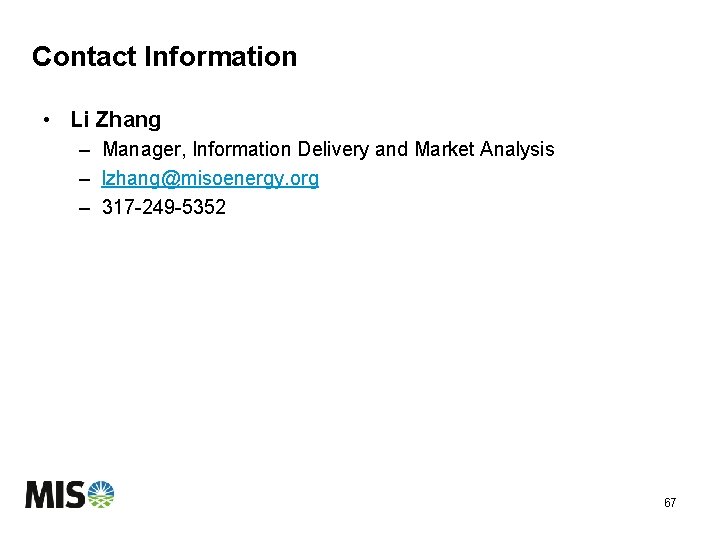
Contact Information • Li Zhang – Manager, Information Delivery and Market Analysis – lzhang@misoenergy. org – 317 -249 -5352 67
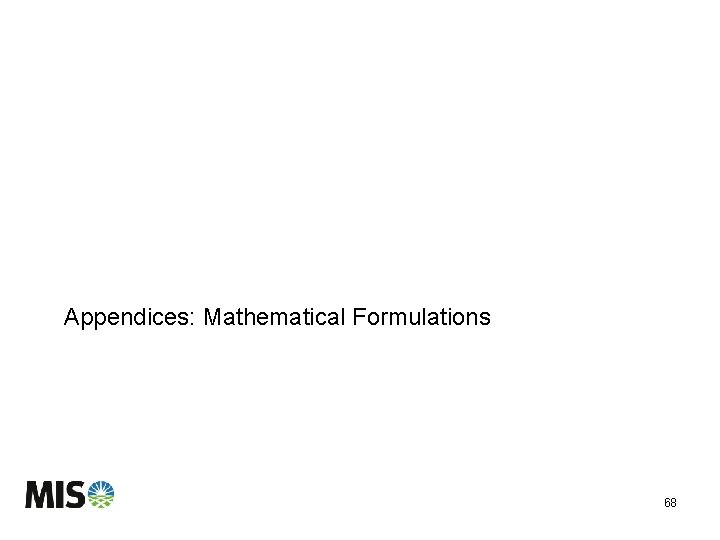
Appendices: Mathematical Formulations 68
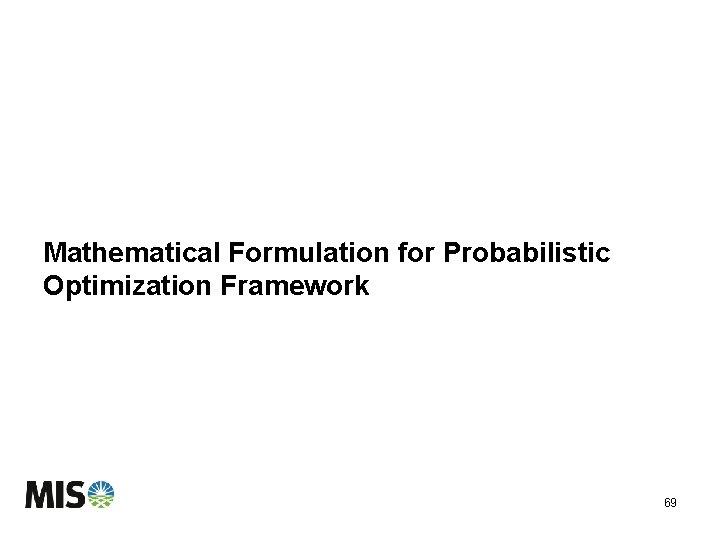
Mathematical Formulation for Probabilistic Optimization Framework 69
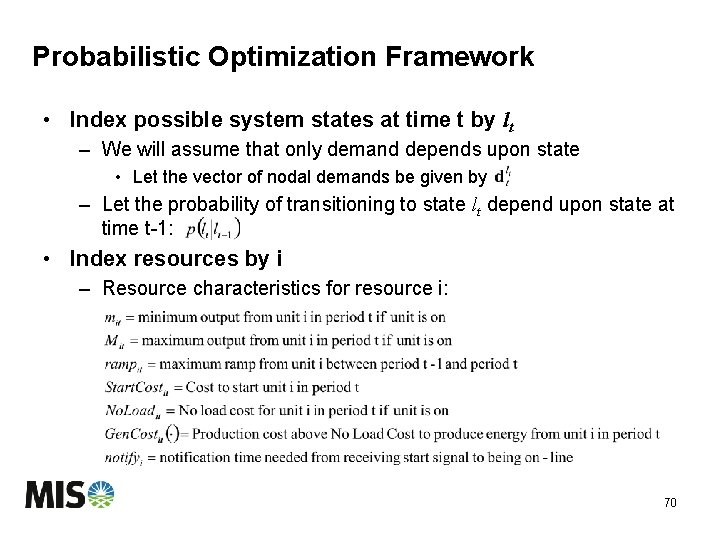
Probabilistic Optimization Framework • Index possible system states at time t by lt – We will assume that only demand depends upon state • Let the vector of nodal demands be given by – Let the probability of transitioning to state lt depend upon state at time t-1: • Index resources by i – Resource characteristics for resource i: 70
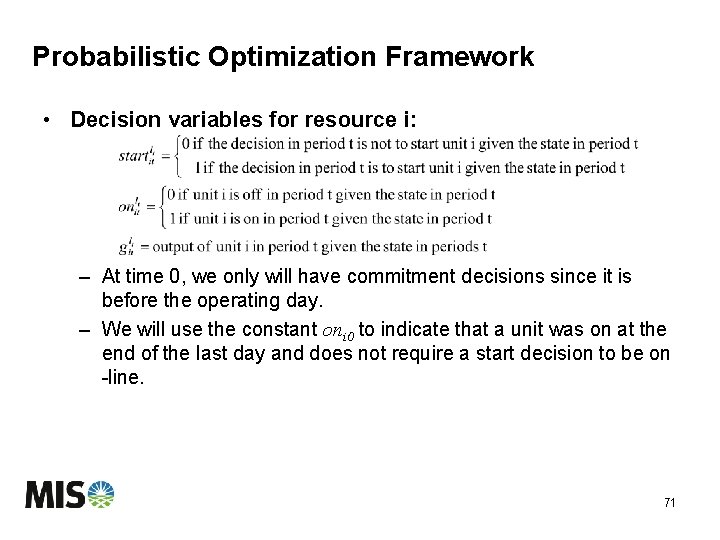
Probabilistic Optimization Framework • Decision variables for resource i: – At time 0, we only will have commitment decisions since it is before the operating day. – We will use the constant oni 0 to indicate that a unit was on at the end of the last day and does not require a start decision to be on -line. 71
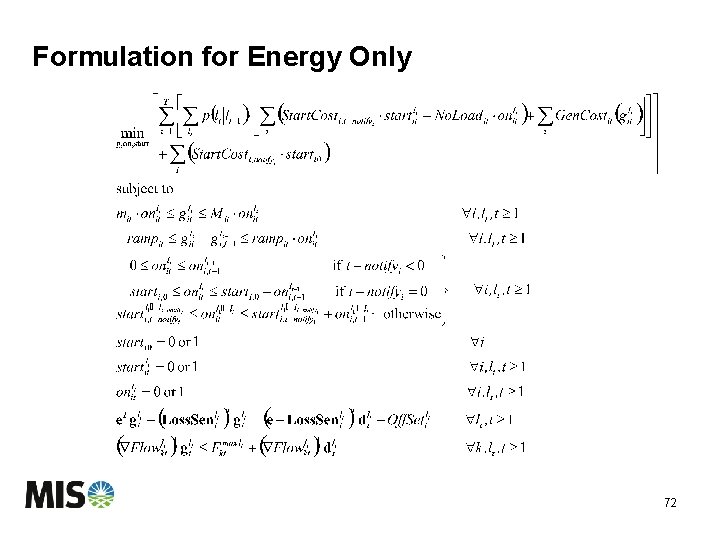
Formulation for Energy Only 72
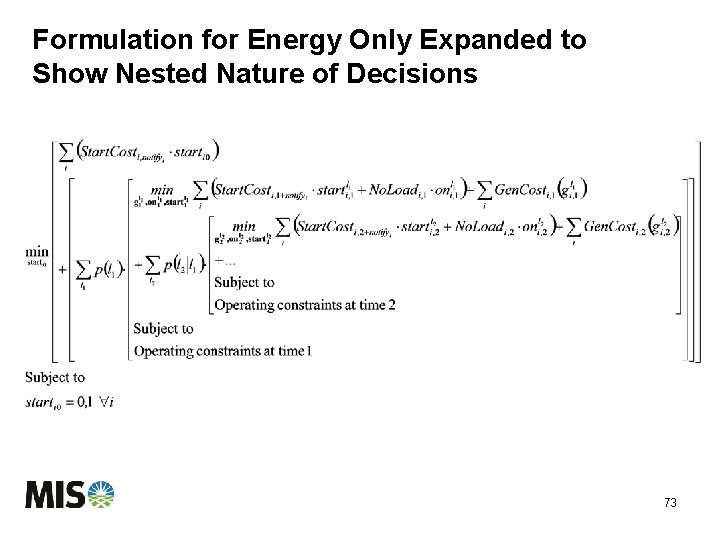
Formulation for Energy Only Expanded to Show Nested Nature of Decisions 73
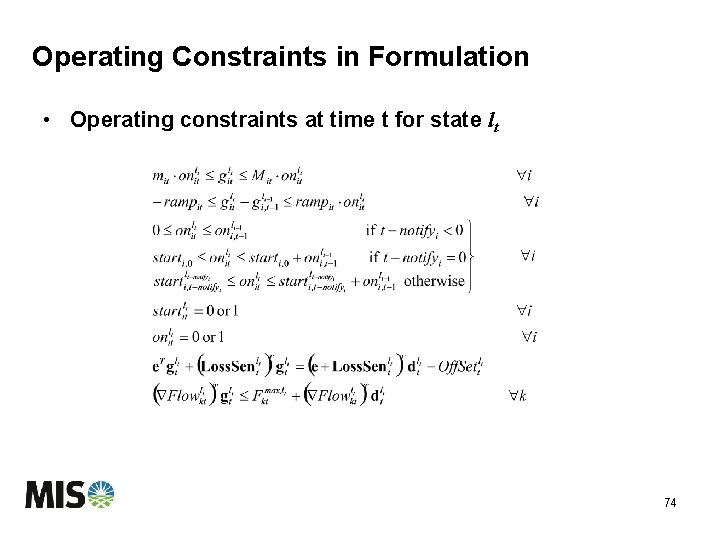
Operating Constraints in Formulation • Operating constraints at time t for state lt 74
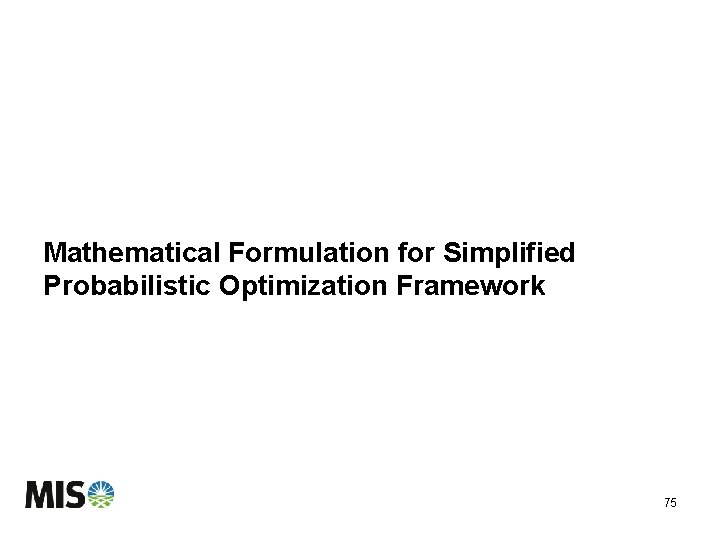
Mathematical Formulation for Simplified Probabilistic Optimization Framework 75
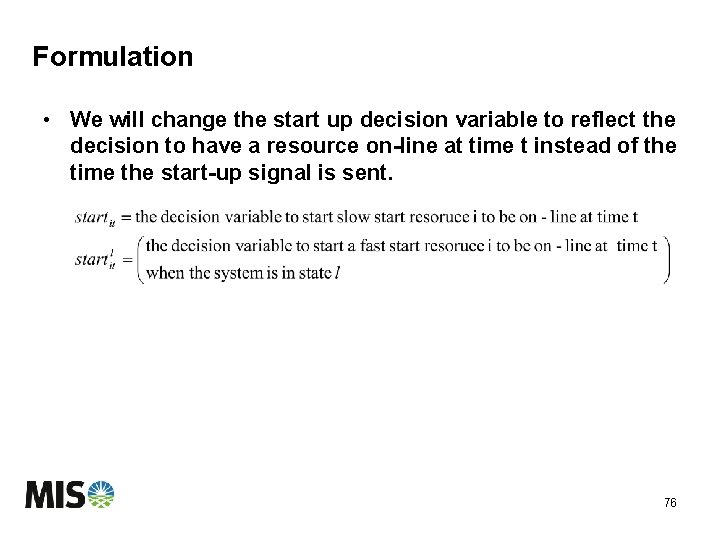
Formulation • We will change the start up decision variable to reflect the decision to have a resource on-line at time t instead of the time the start-up signal is sent. 76
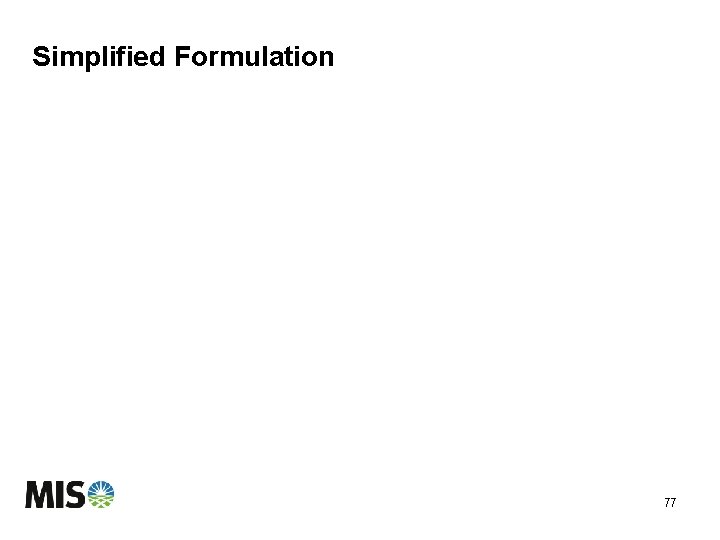
Simplified Formulation 77
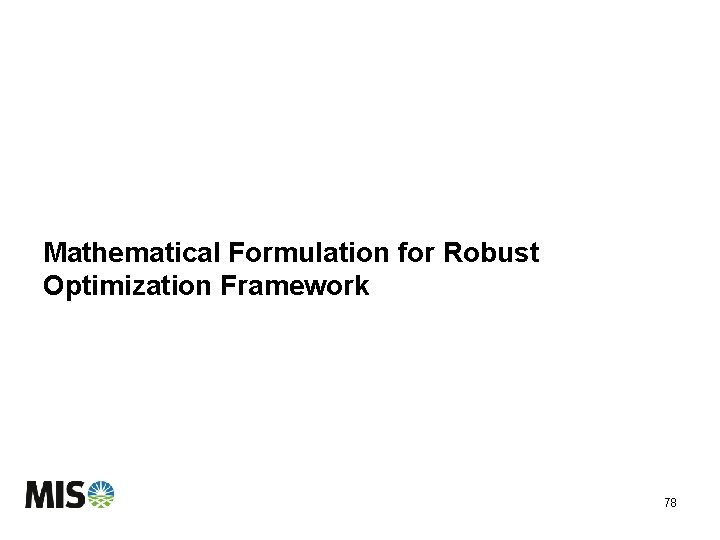
Mathematical Formulation for Robust Optimization Framework 78
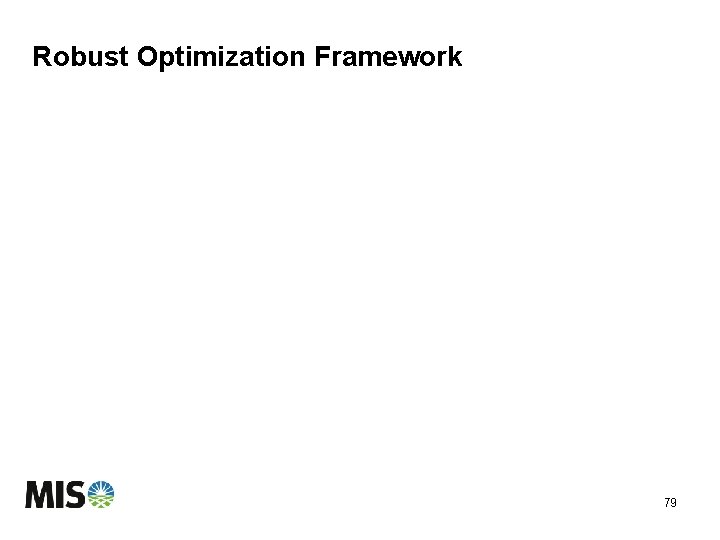
Robust Optimization Framework 79
Sat root words
Operating reserve
Mišo mudrić
Nuu miso
Communication spi
Miso rt displays
Singsi
Miso market portal
Miso lmp contour map
Miso ftr
First gen antipsychotics
God you are good and your mercy endureth forever
Module 5 supply and demand introduction and demand
On demand app generation qlik sense
Deficient demand and excess demand
What is dependent demand inventory
Ano ang halimbawa ng demand
Demand estimation and forecasting in managerial economics
Distinguish between individual demand and market demand
Independent demand inventory management
Demand management and capacity management
Deterministic and stochastic inventory models
Individual demand vs market demand
Dependent and independent demand in inventory management
Content management system database
Introduction of hrm
Management topics for project
Class diagram gym management system
Real-time interaction management vendors
Gambaran
Management overview
Overview of financial management
The commonly accepted goal of the mnc is to:
Chapter 1 an overview of financial management
Overview of financial management
Vendor selection matrix
Robson management corporation
Demand management sap
Demand management platform
Ipmi architecture
Demand management platform
Independent demand system
Independent demand examples
Contoh demand management
Demand forecasting operations management
Demand management incentive scheme
Implied demand uncertainty
Demand forecasting in operations management
What is independent demand
Independent
Trial and improvement
If you can't measure it you can't improve it
Improvements & betterments
Software process improvement and capability determination
Brushy creek water
Revised sbm assessment tool 2021
Sbar
Pyramid levels of management
Management pyramid
Top level management
Virusmax
Data quality and data cleaning an overview
Elements and their properties chapter 17
An overview of data warehousing and olap technology
What is bioinformatics an introduction and overview
An overview of data warehousing and olap technology
Data quality and data cleaning an overview
Data quality and data cleaning an overview
Overview of storage and indexing
Elements and their properties section 1 metals
Motivation work improvement team
Four improvement strategies in tqm
Together towards improvement
Promoting moral improvement
Fha title 1 loan
Performance improvement plan examples
Classes of supply army
Priority improvement areas
Ssis rating scale
Shaala siddhi dashboard