Lecture 14 Convolutional Neural Networks on Surfaces via
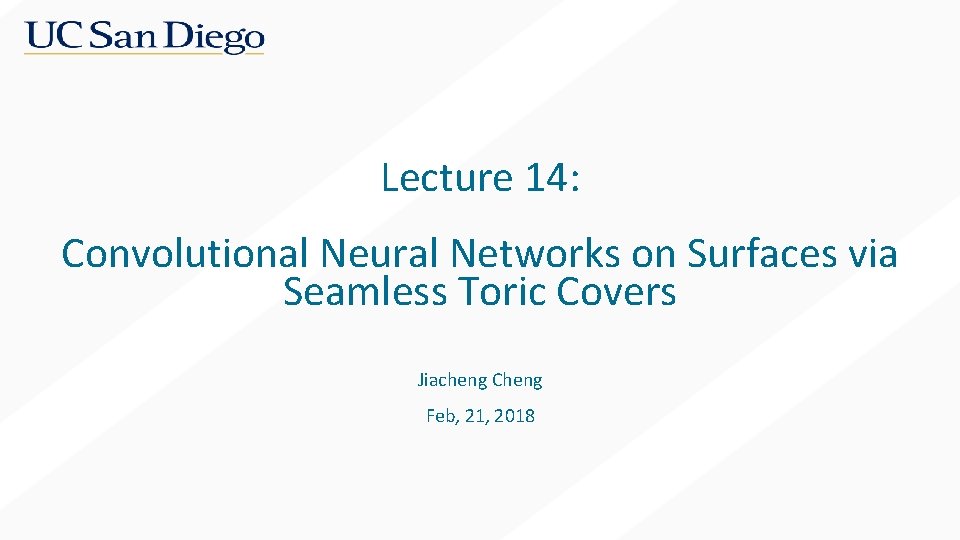
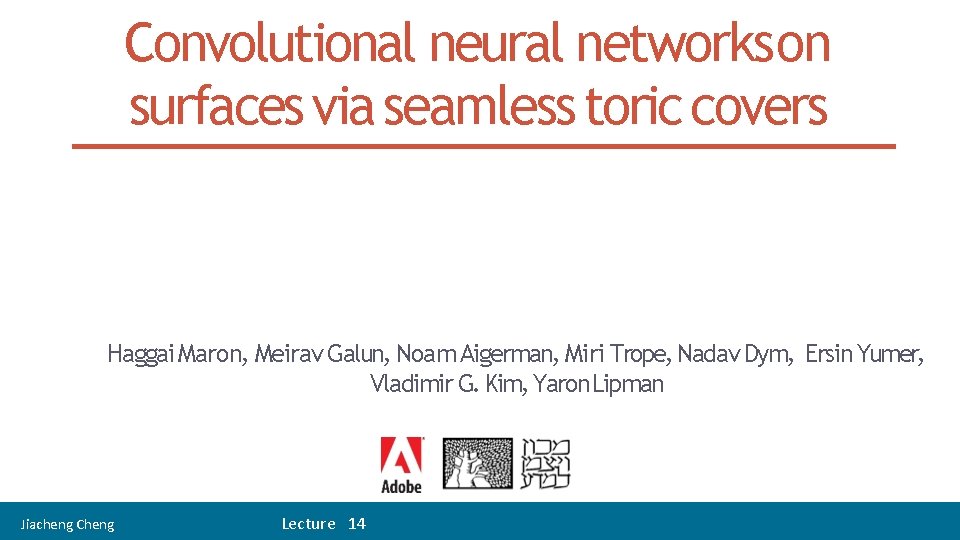
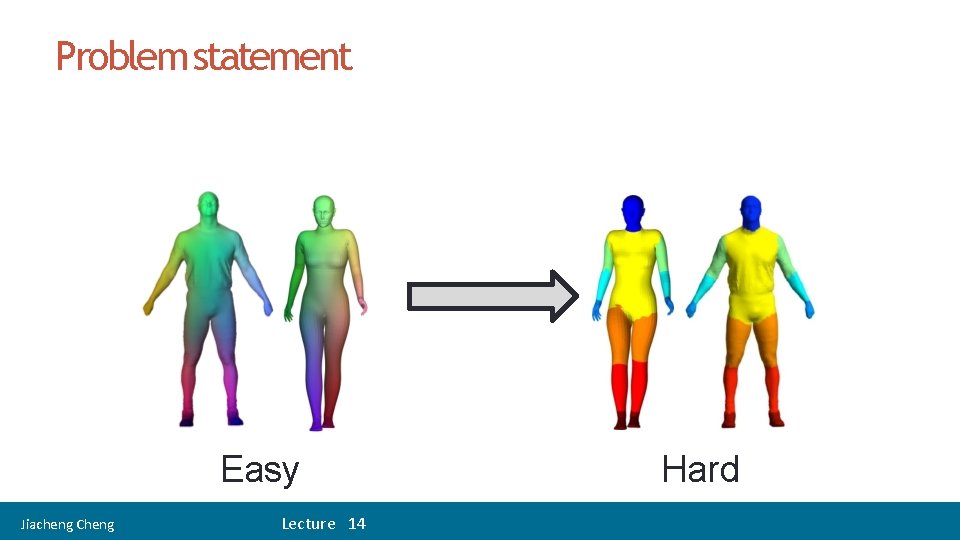
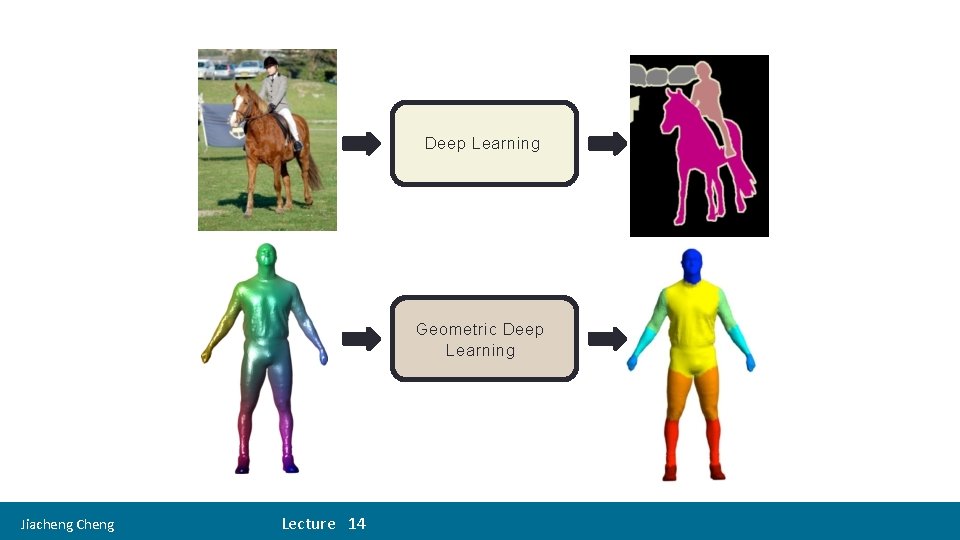
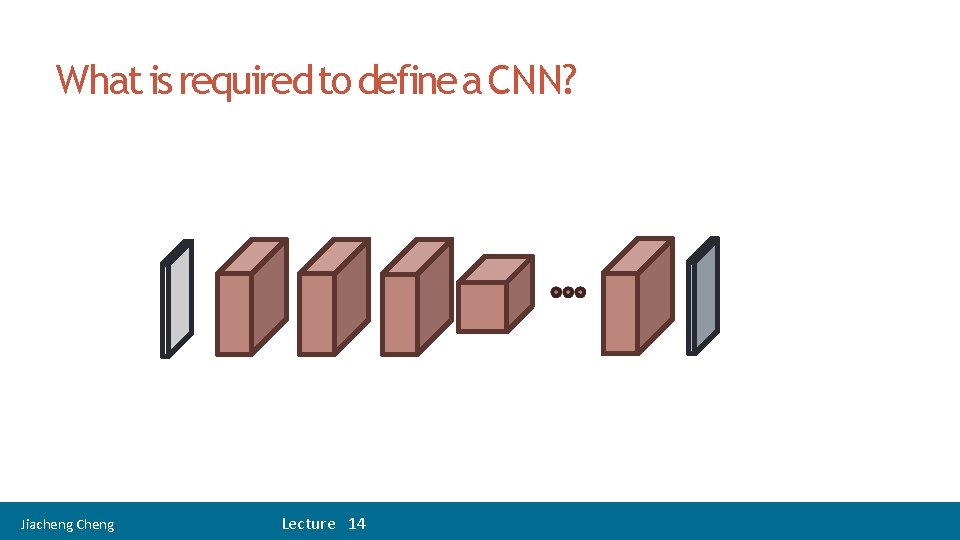
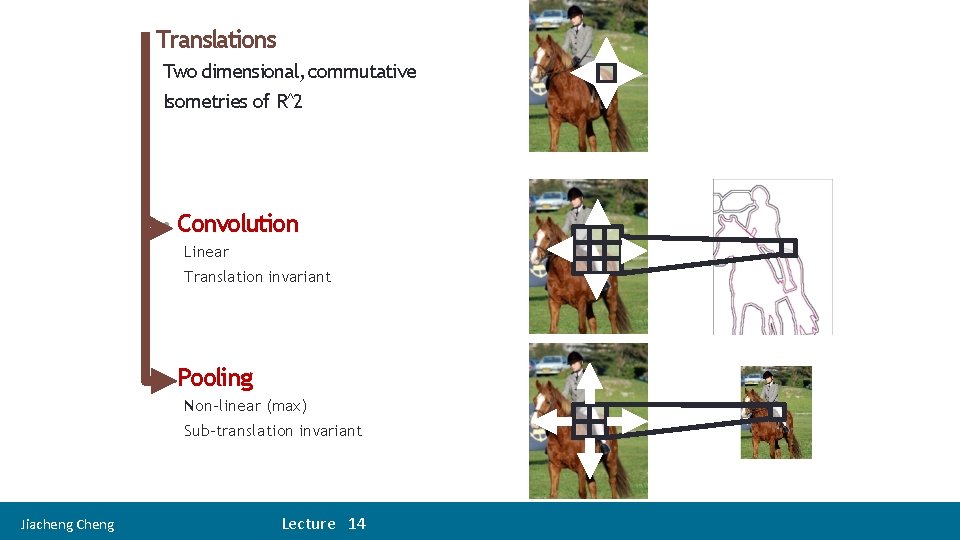
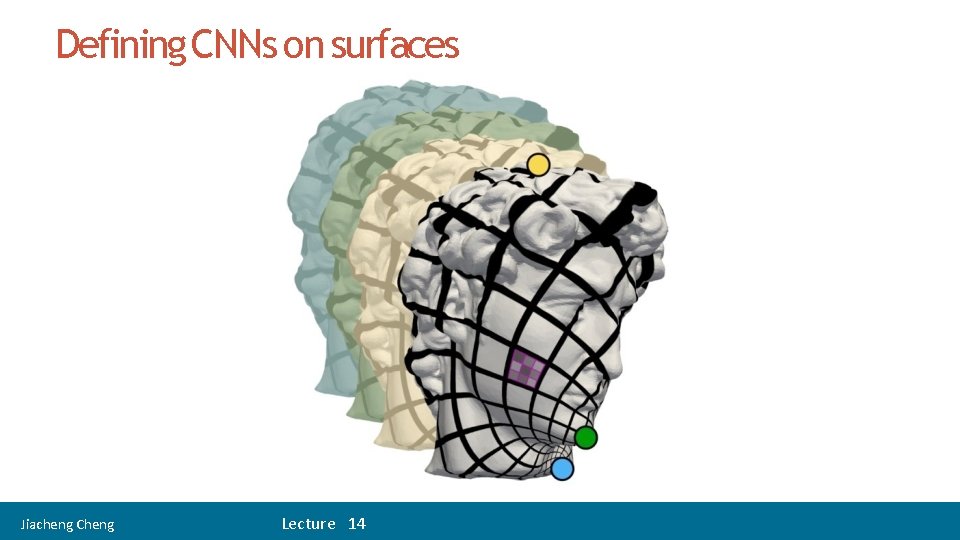
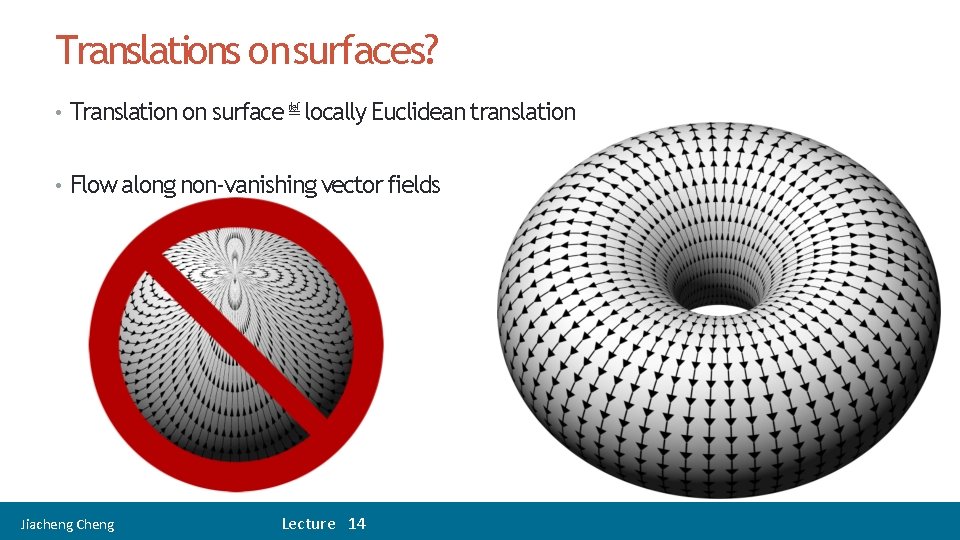
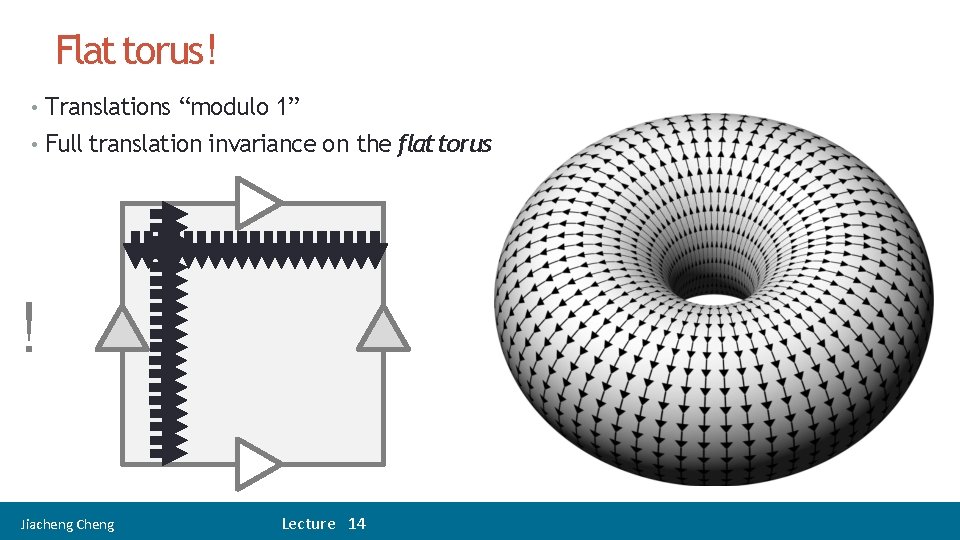
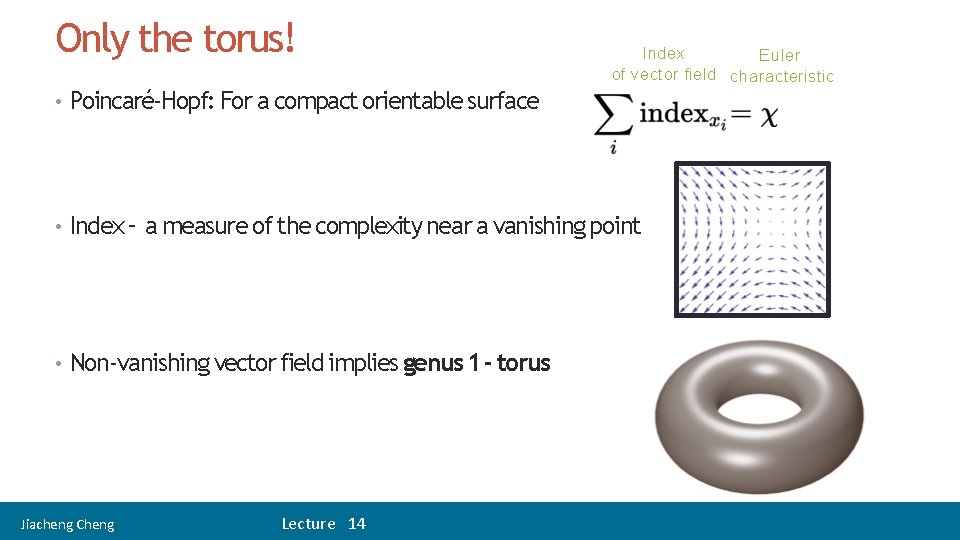
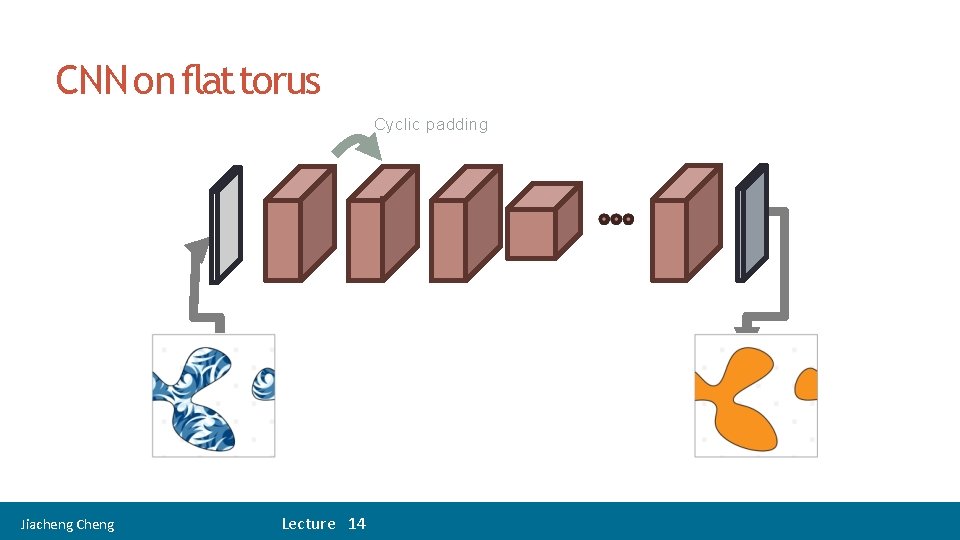
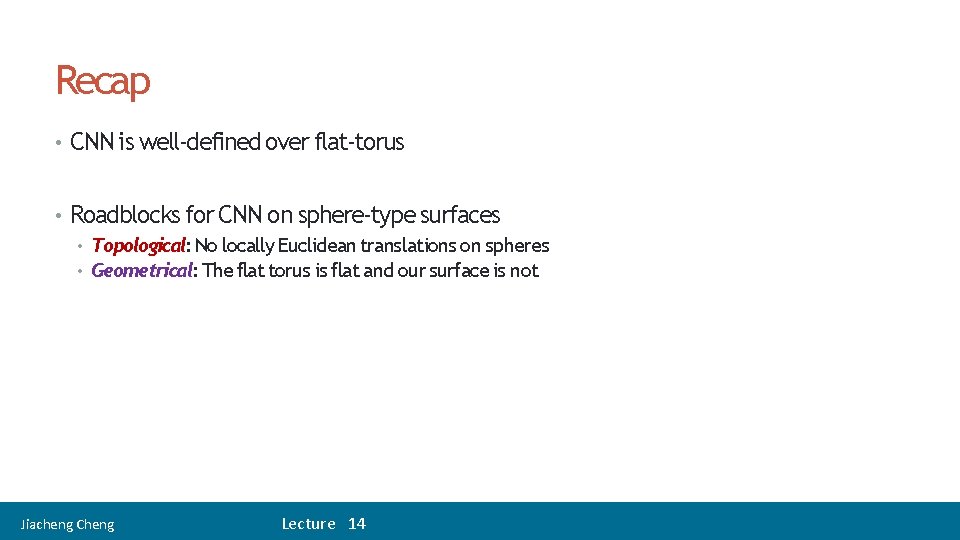
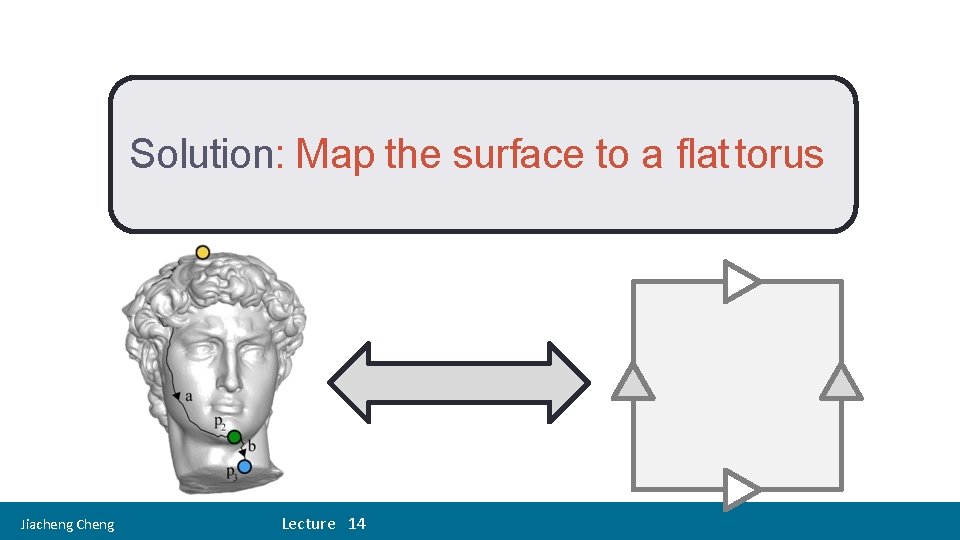
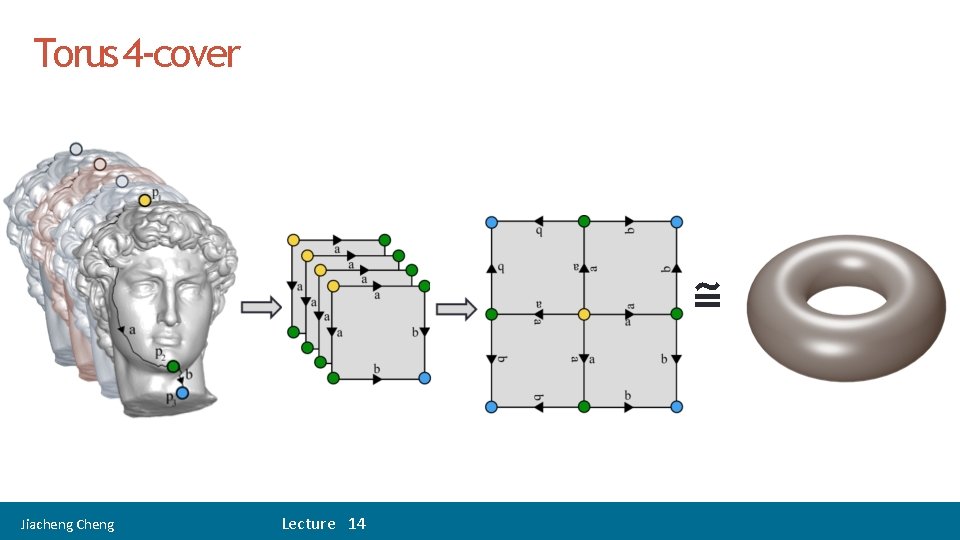
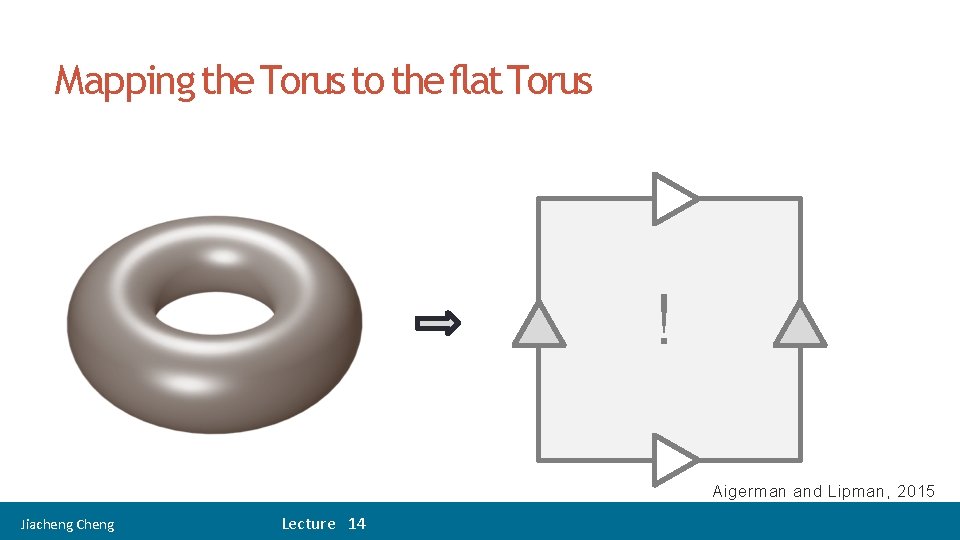
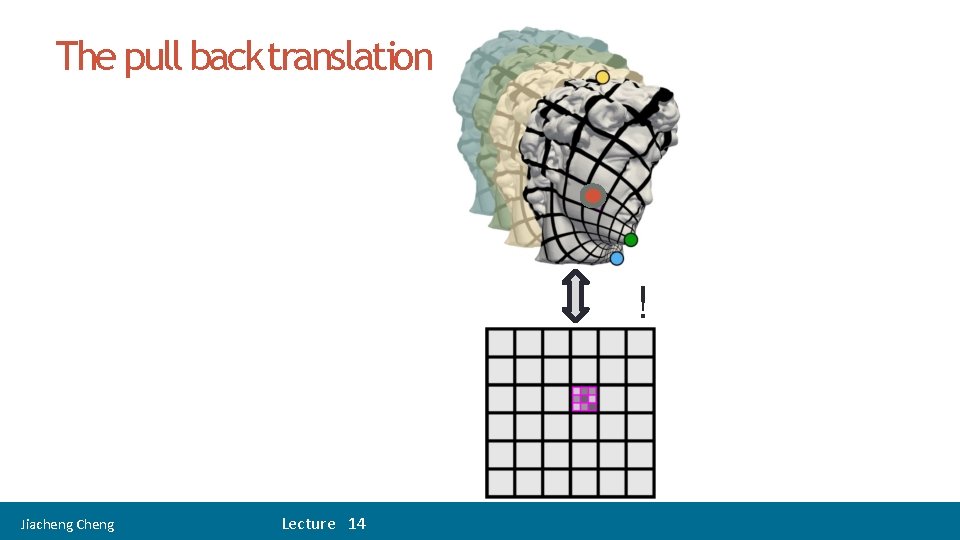
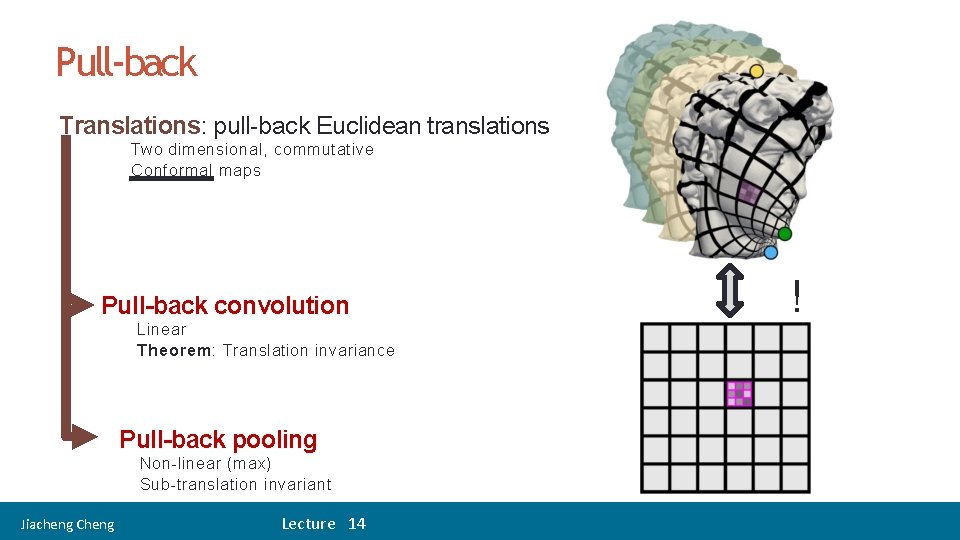
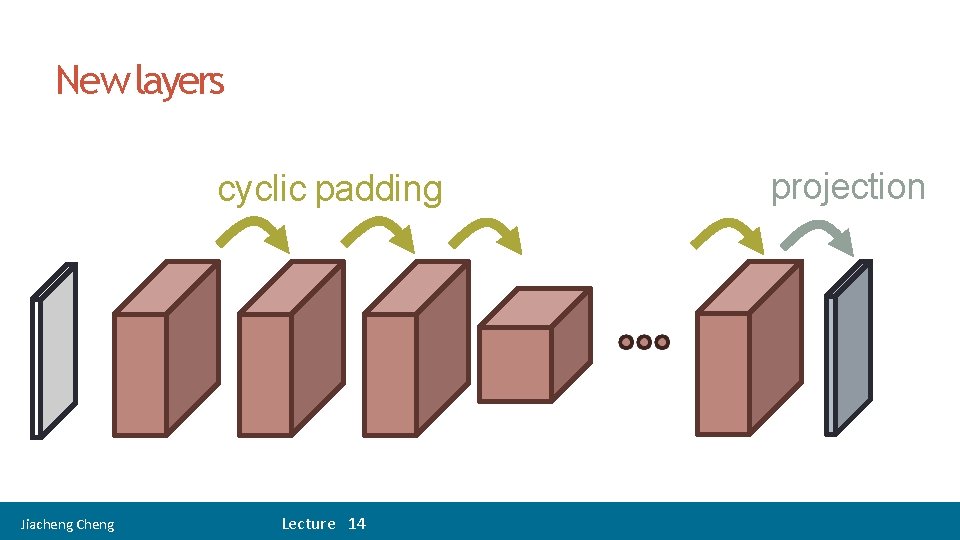
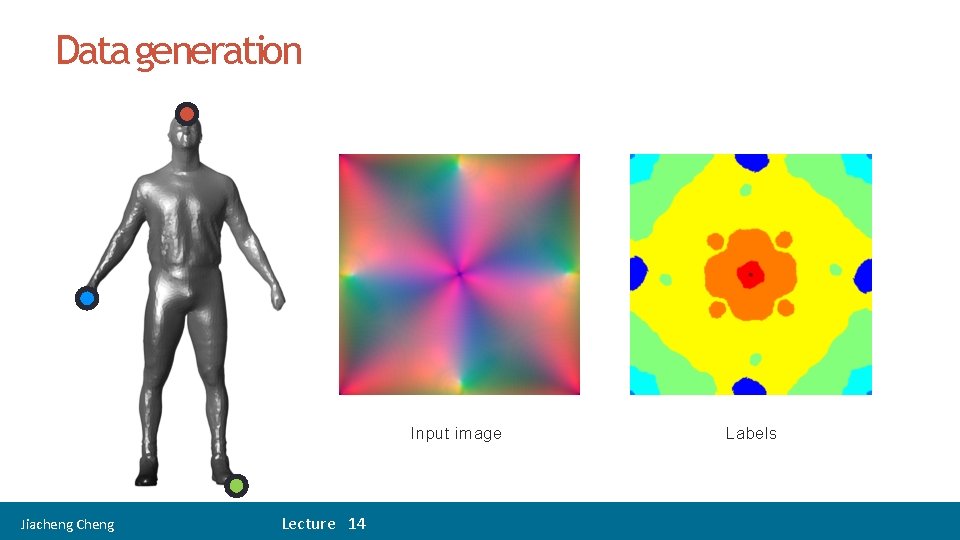
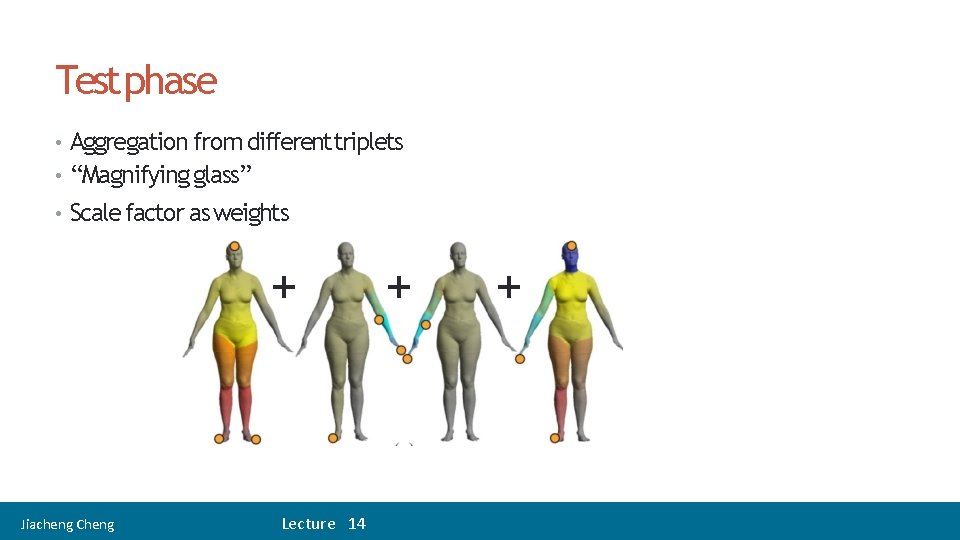
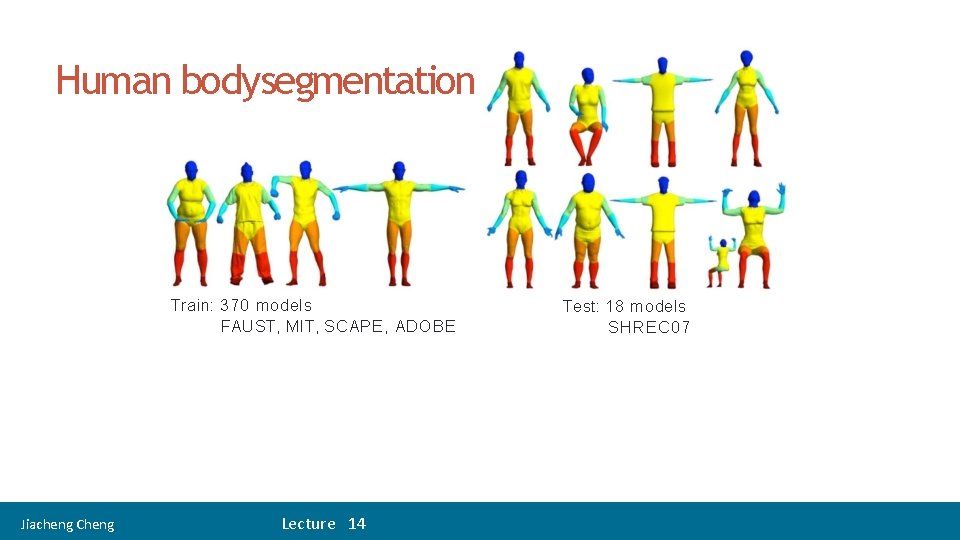
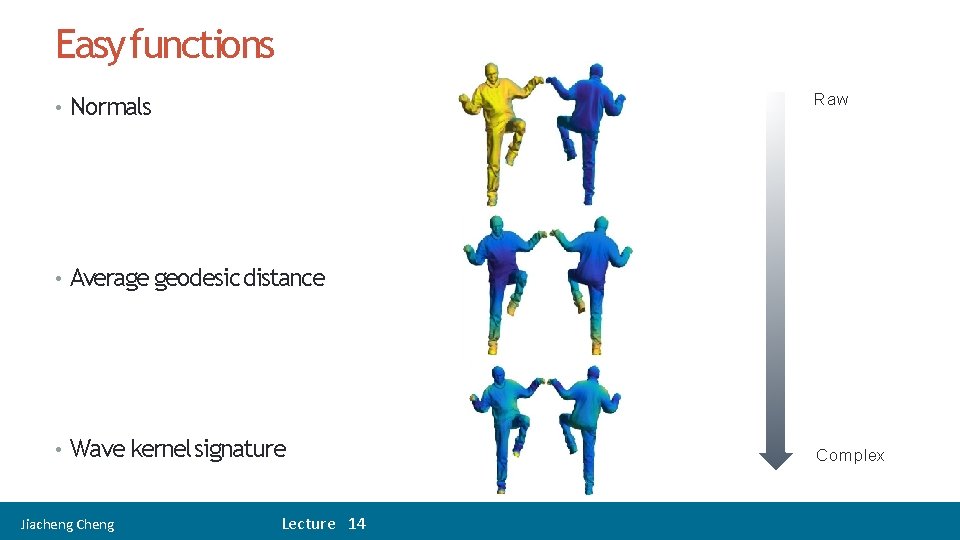
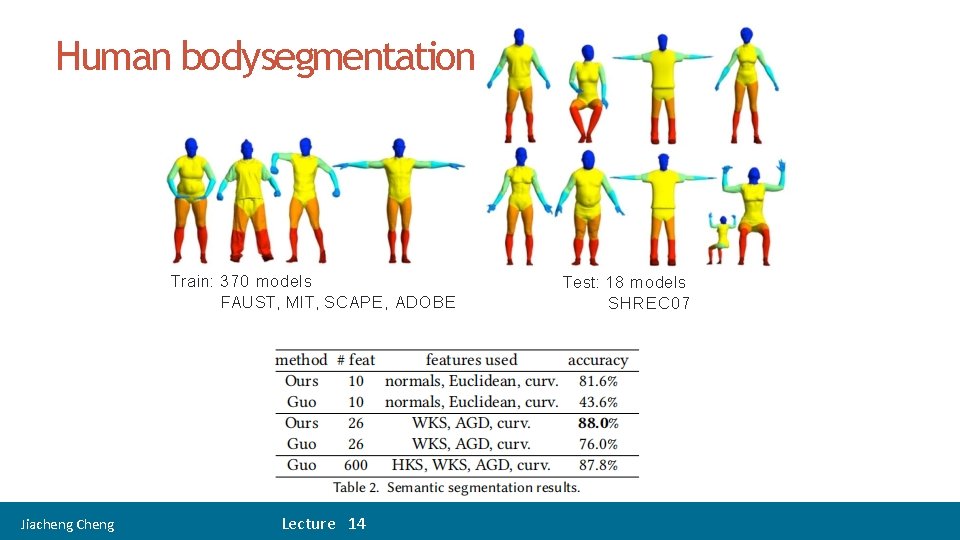
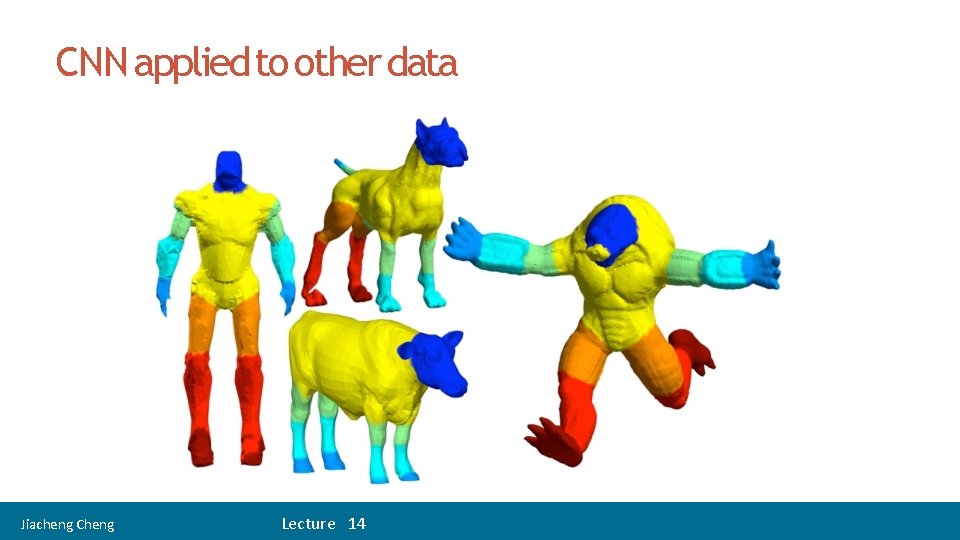
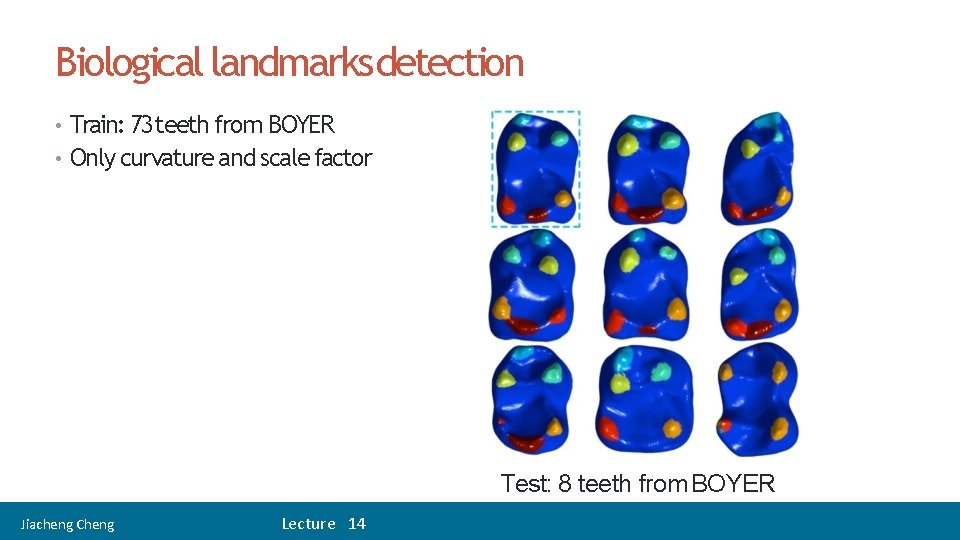
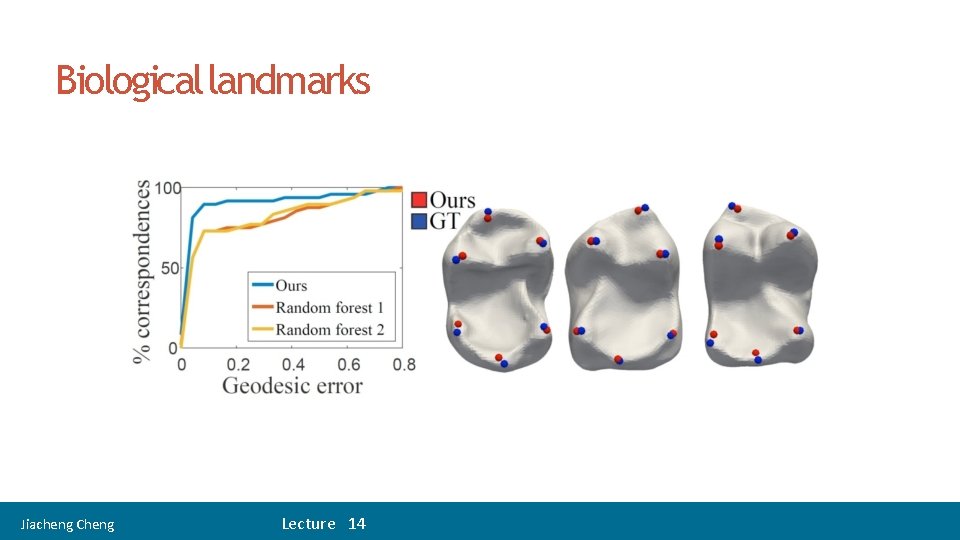
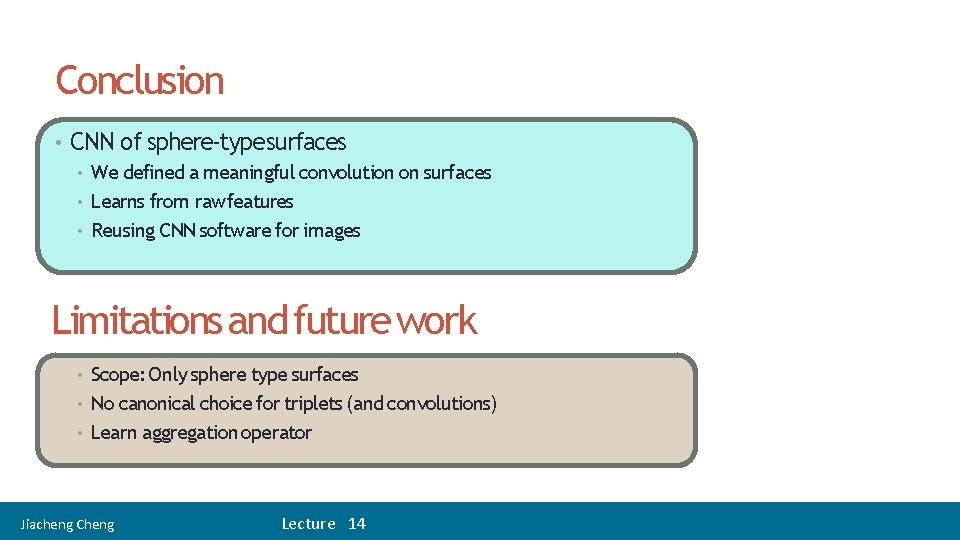
- Slides: 27
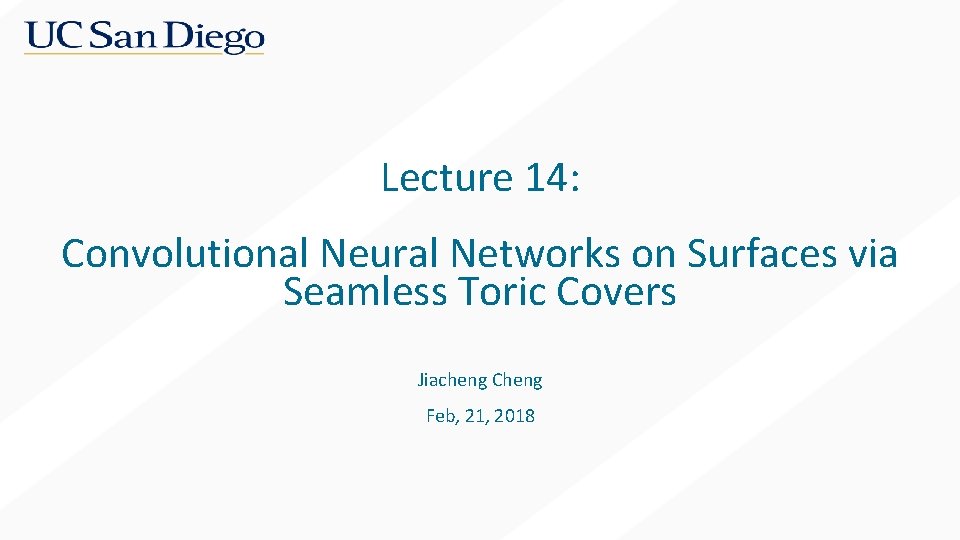
Lecture 14: Convolutional Neural Networks on Surfaces via Seamless Toric Covers Jiacheng Cheng Feb, 21, 2018
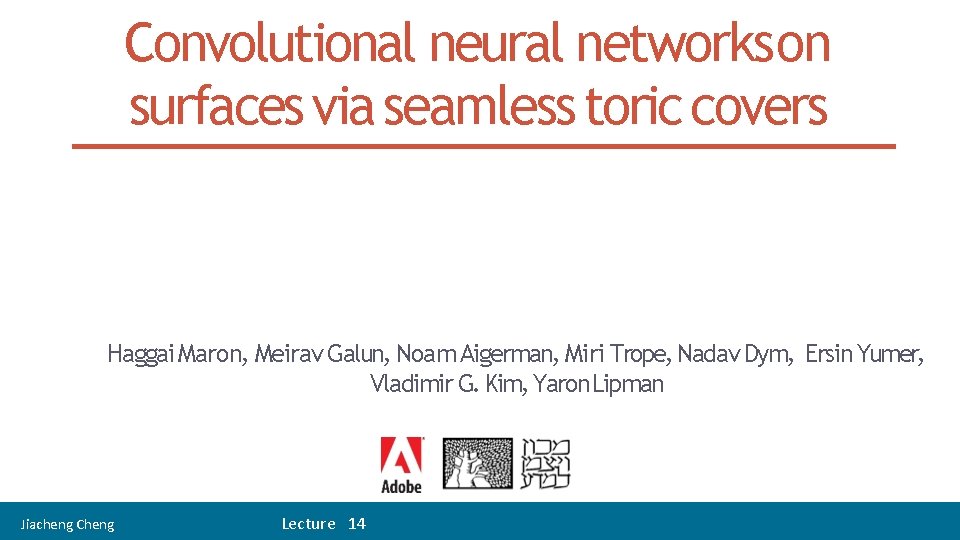
Convolutional neural networkson surfaces via seamless toric covers Haggai Maron, Meirav Galun, Noam Aigerman, Miri Trope, Nadav Dym, Ersin Yumer, Vladimir G. Kim, Yaron Lipman Jiacheng Cheng Lecture 14
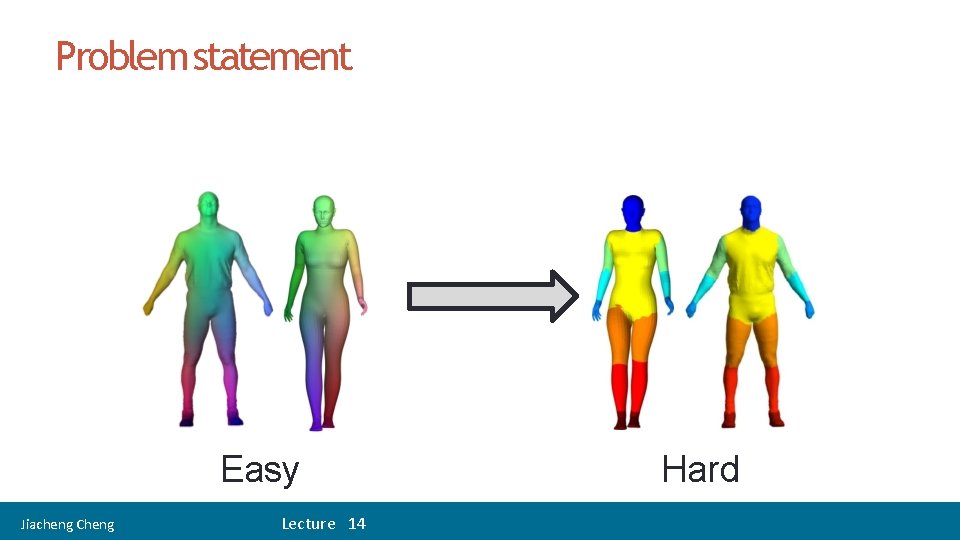
Problem statement Easy Jiacheng Cheng Lecture 14 Hard
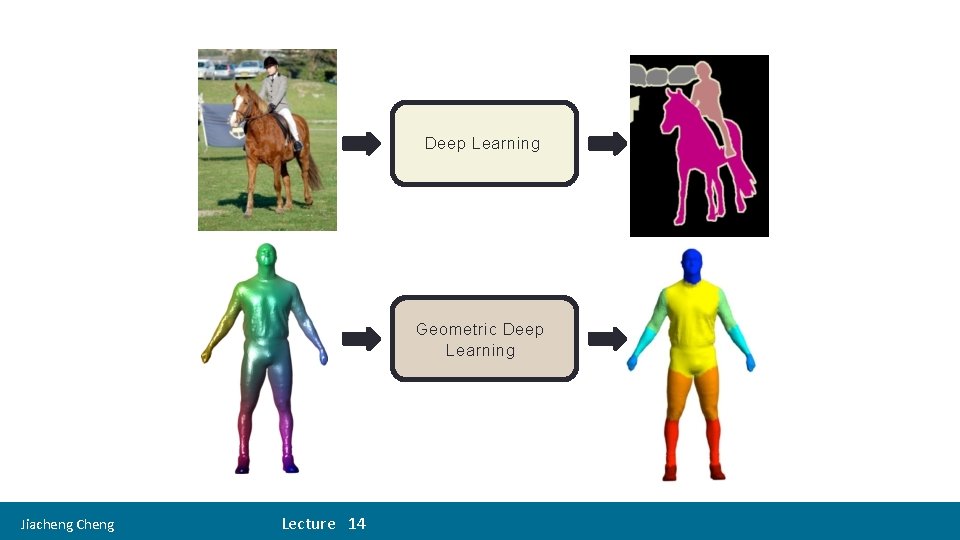
Deep Learning Geometric Deep Learning Jiacheng Cheng Lecture 14
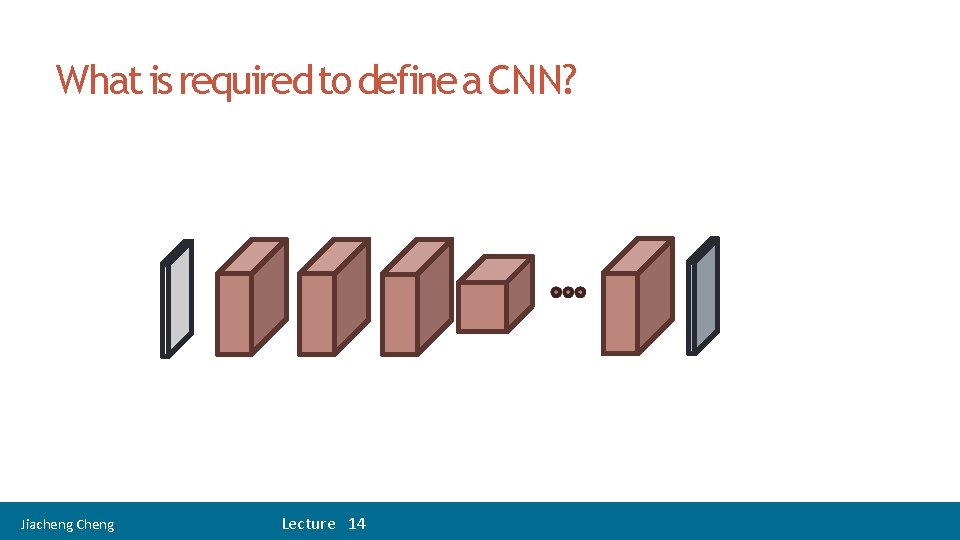
What is required to define a CNN? Jiacheng Cheng Lecture 14
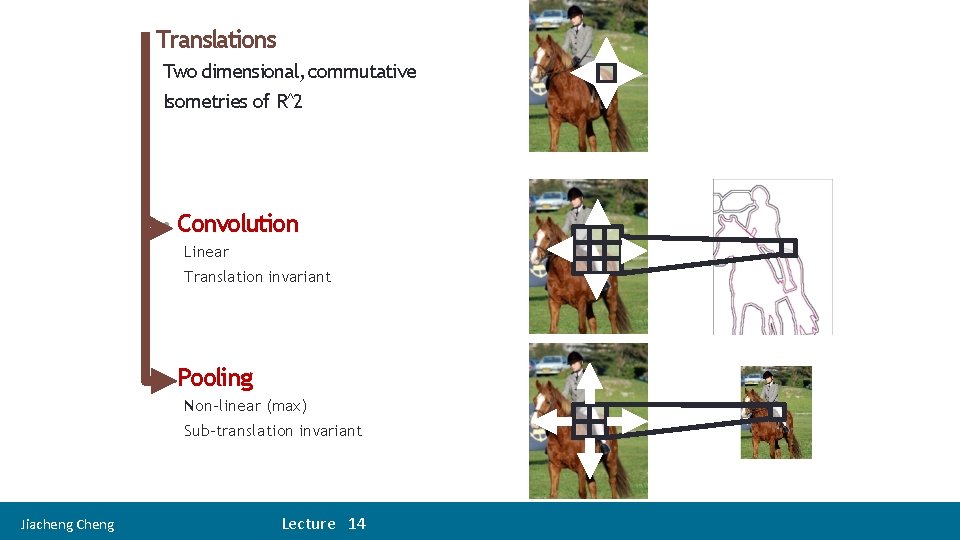
• Translations Two dimensional, commutative Isometries of R^2 • Convolution Linear Translation invariant • Pooling Non-linear (max) Sub-translation invariant Jiacheng Cheng Lecture 14
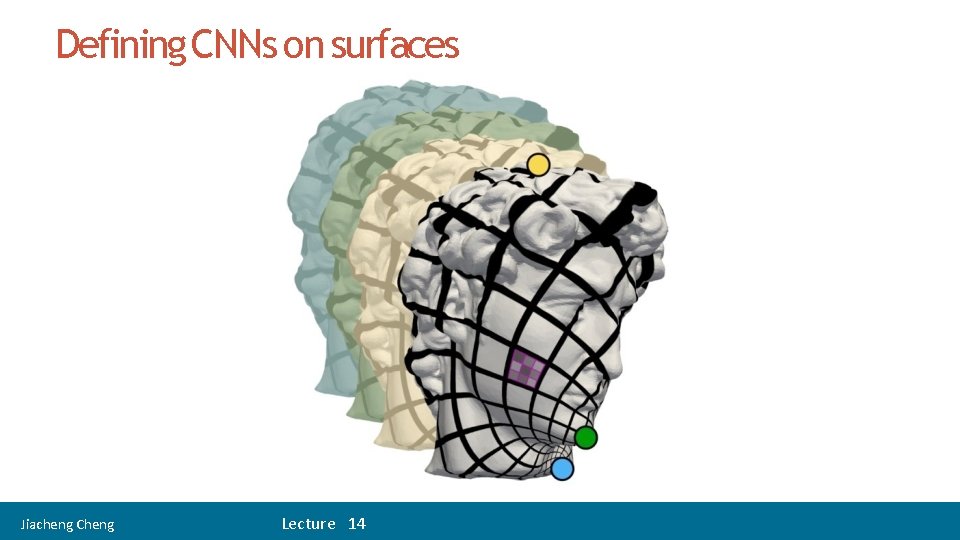
Defining CNNs on surfaces Jiacheng Cheng Lecture 14
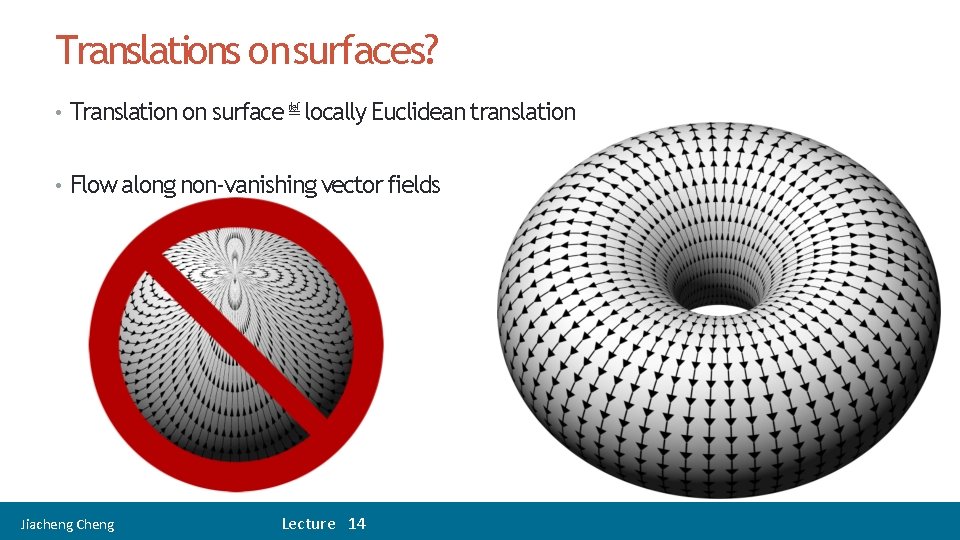
Translations on surfaces? • Translation on surface ≝ locally Euclidean translation • Flow along non-vanishing vector fields Jiacheng Cheng Lecture 14
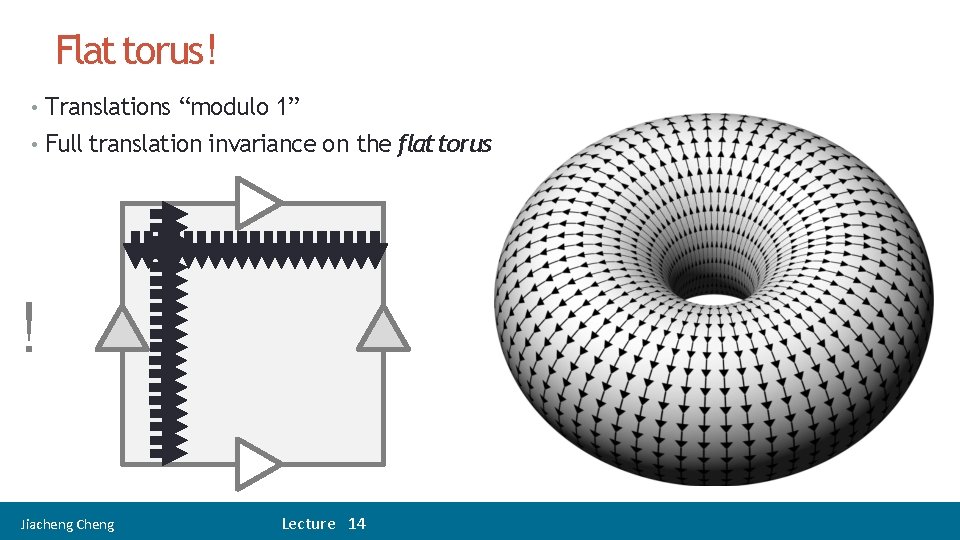
Flat torus! • Translations “modulo 1” • Full translation invariance on the flat torus ! Jiacheng Cheng Lecture 14
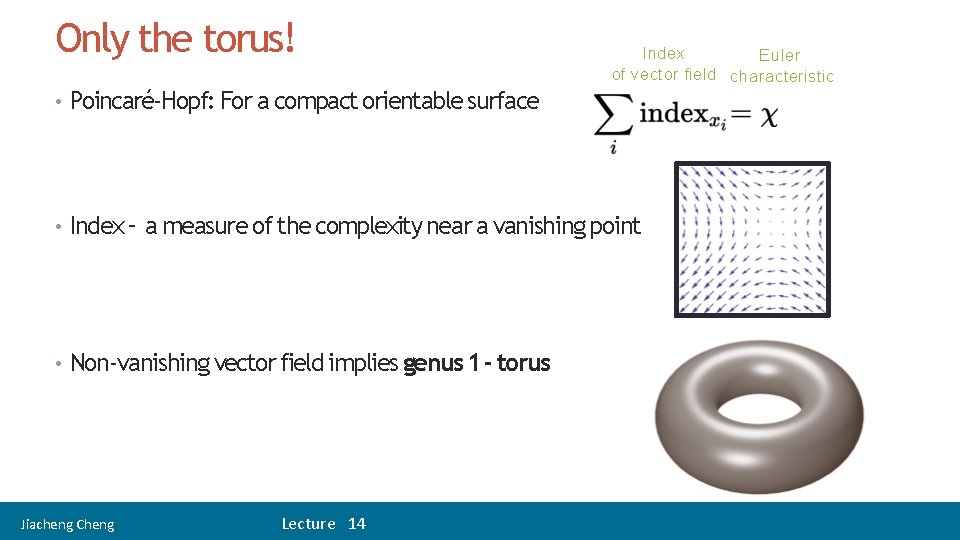
Only the torus! Index Euler of vector field characteristic • Poincaré-Hopf: For a compact orientable surface • Index – a measure of the complexity near a vanishing point • Non-vanishing vector field implies genus 1 - torus Jiacheng Cheng Lecture 14
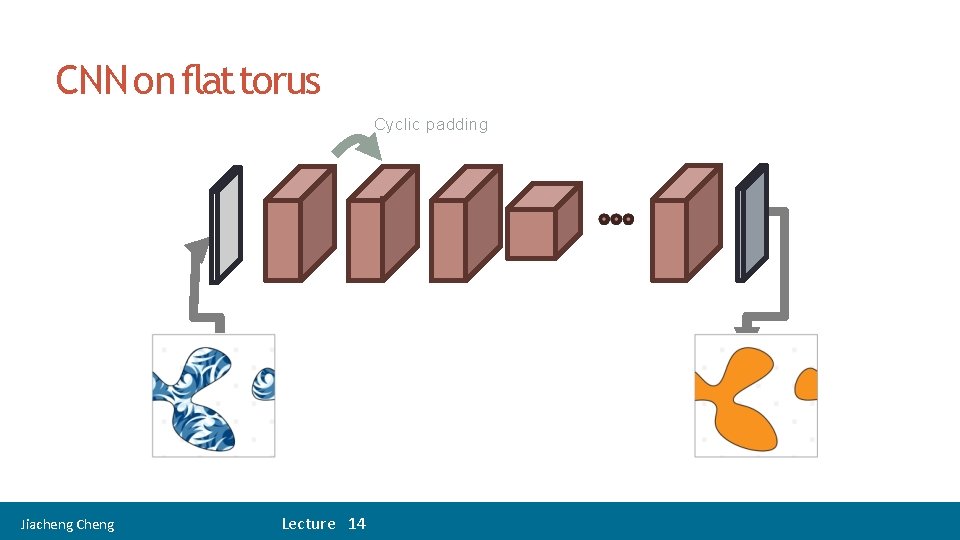
15 CNN on flat torus Cyclic padding Jiacheng Cheng Lecture 14
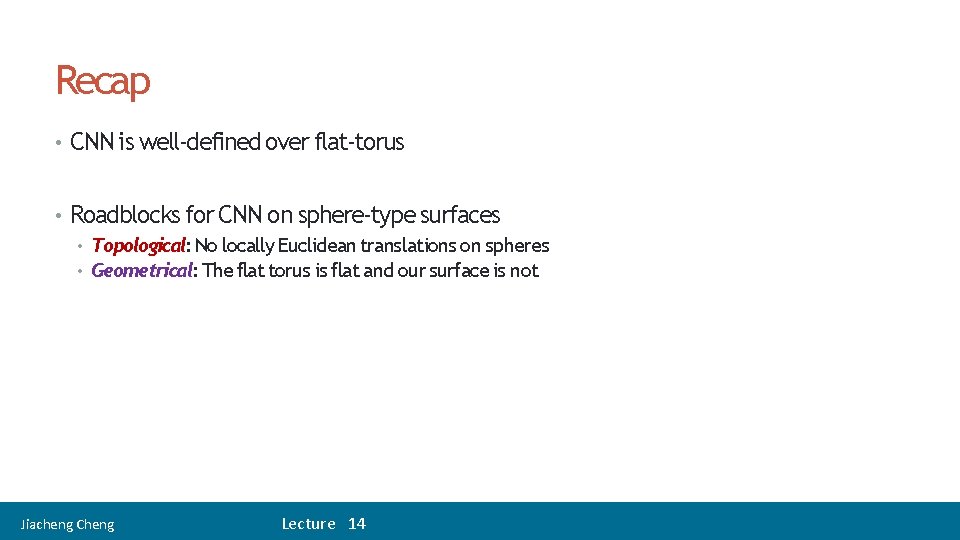
16 Recap • CNN is well-defined over flat-torus • Roadblocks for CNN on sphere-type surfaces • Topological: No locally Euclidean translations on spheres • Geometrical: The flat torus is flat and our surface is not Jiacheng Cheng Lecture 14
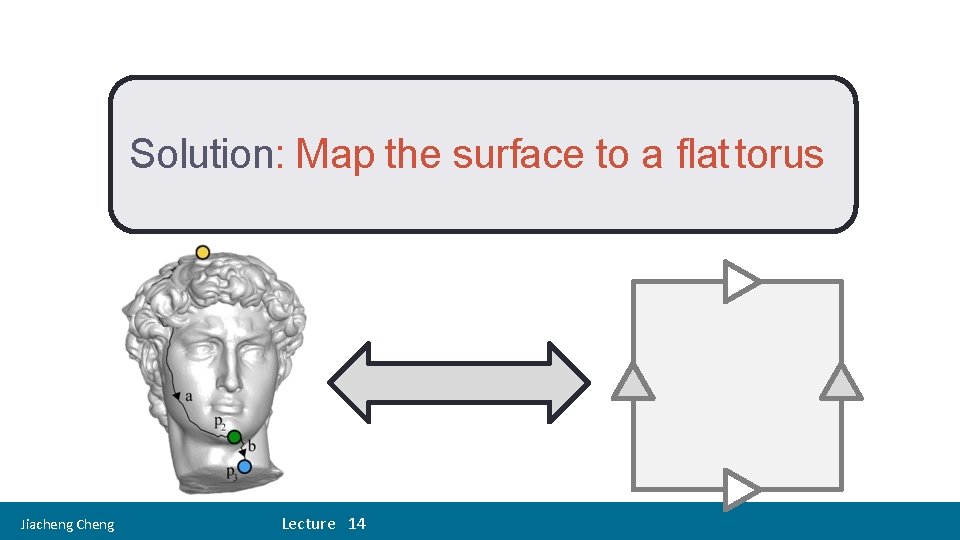
17 Solution: Map the surface to a flat torus Jiacheng Cheng Lecture 14
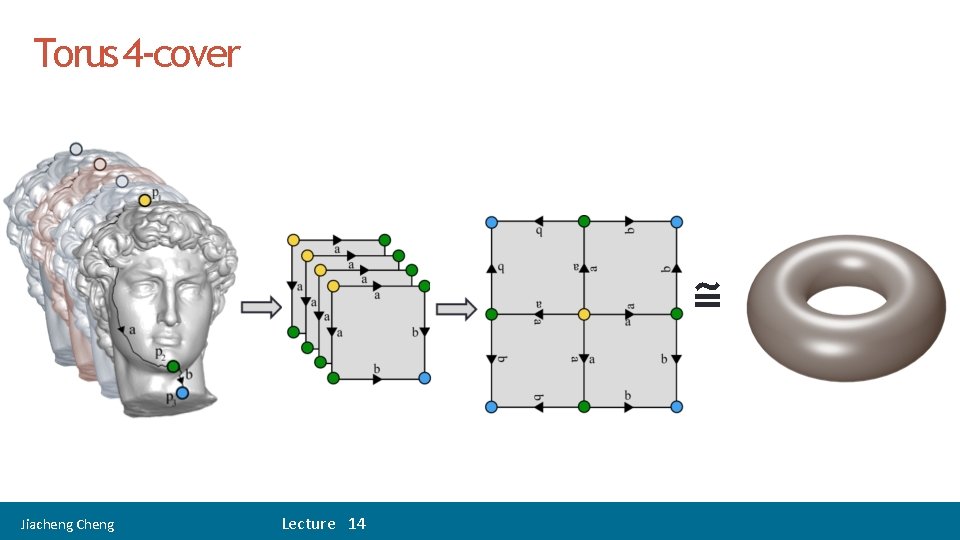
Torus 4 -cover ≅ Jiacheng Cheng Lecture 14
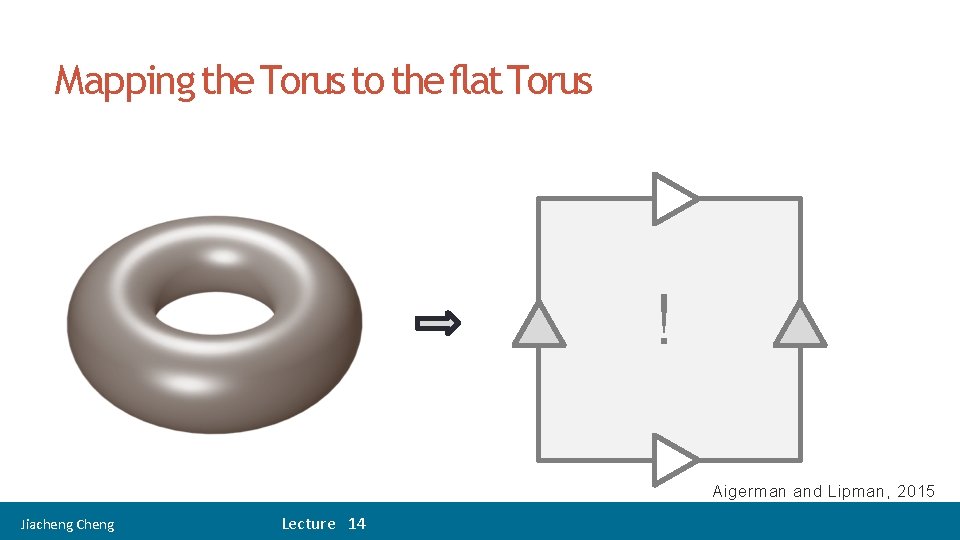
19 Mapping the Torus to the flat Torus ! Aigerman and Lipman, 2015 Jiacheng Cheng Lecture 14
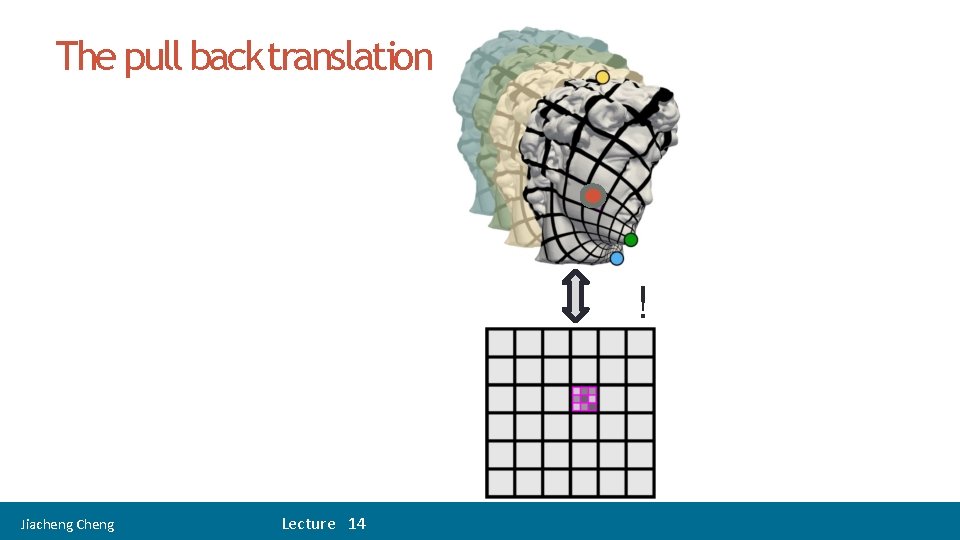
The pull back translation ! Jiacheng Cheng Lecture 14
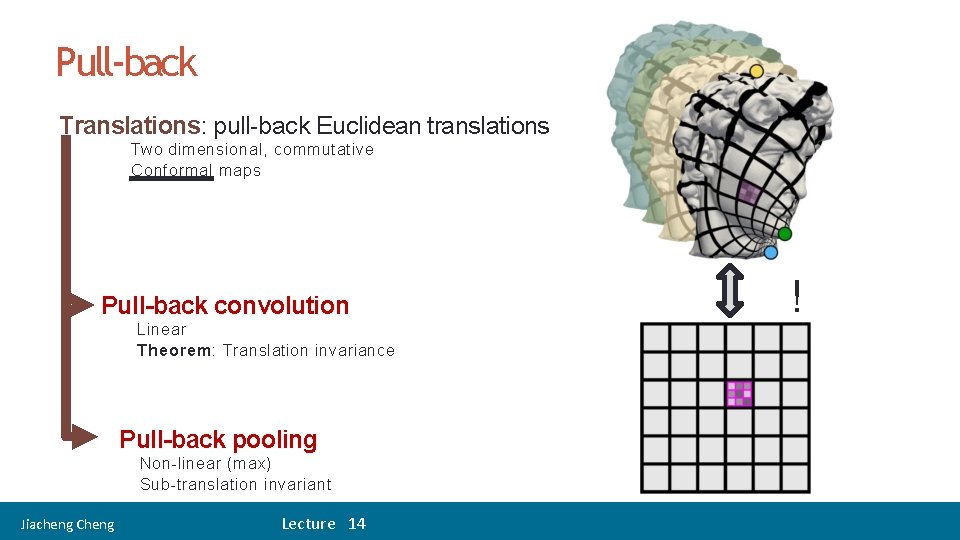
22 Pull-back Translations: pull-back Euclidean translations Two dimensional, commutative Conformal maps Pull-back convolution Linear Theorem: Translation invariance Pull-back pooling Non-linear (max) Sub-translation invariant Jiacheng Cheng Lecture 14 !
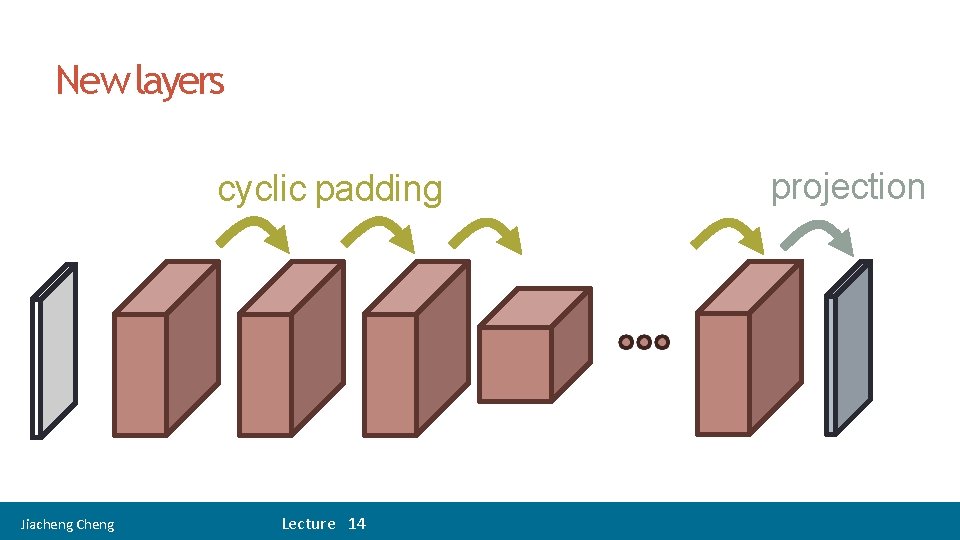
24 New layers cyclic padding Jiacheng Cheng Lecture 14 projection
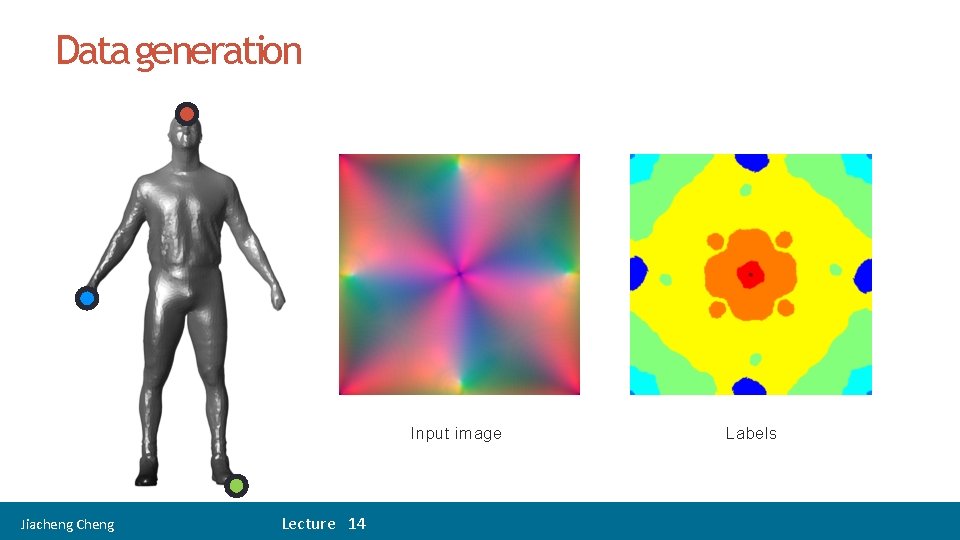
Data generation Input image Jiacheng Cheng Lecture 14 Labels
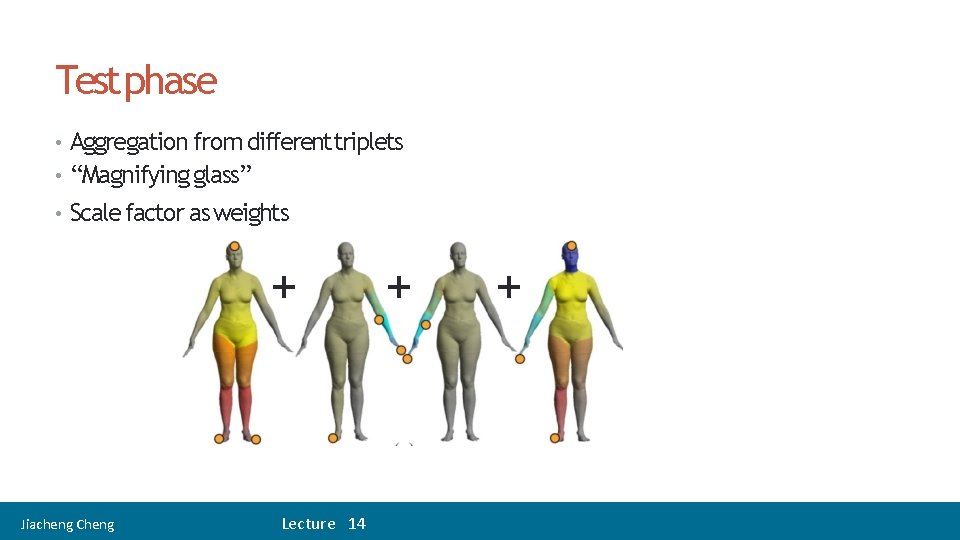
26 Testphase • Aggregation from differenttriplets • “Magnifying glass” • Scale factor as weights + Jiacheng Cheng Lecture 14 + + =
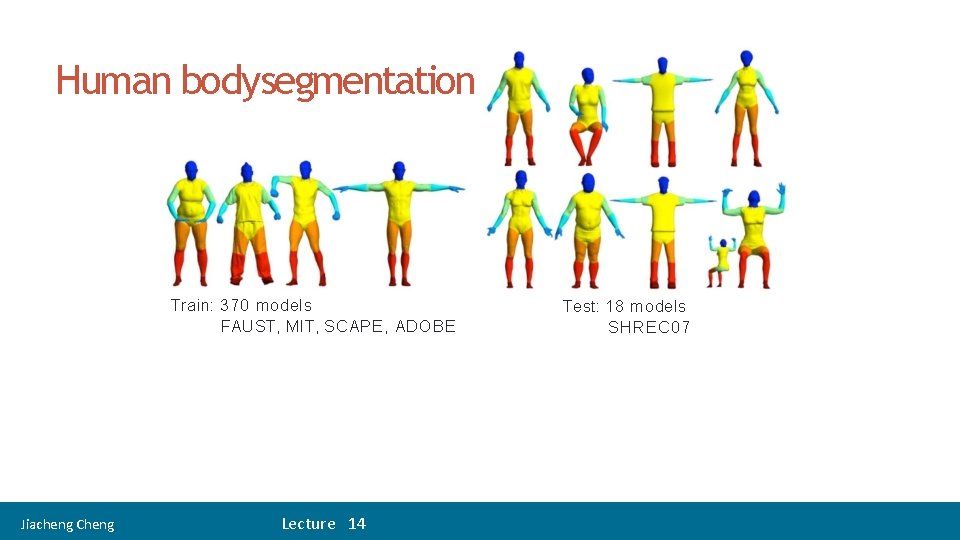
27 Human bodysegmentation Train: 370 models FAUST, MIT, SCAPE, ADOBE Jiacheng Cheng Lecture 14 Test: 18 models SHREC 07
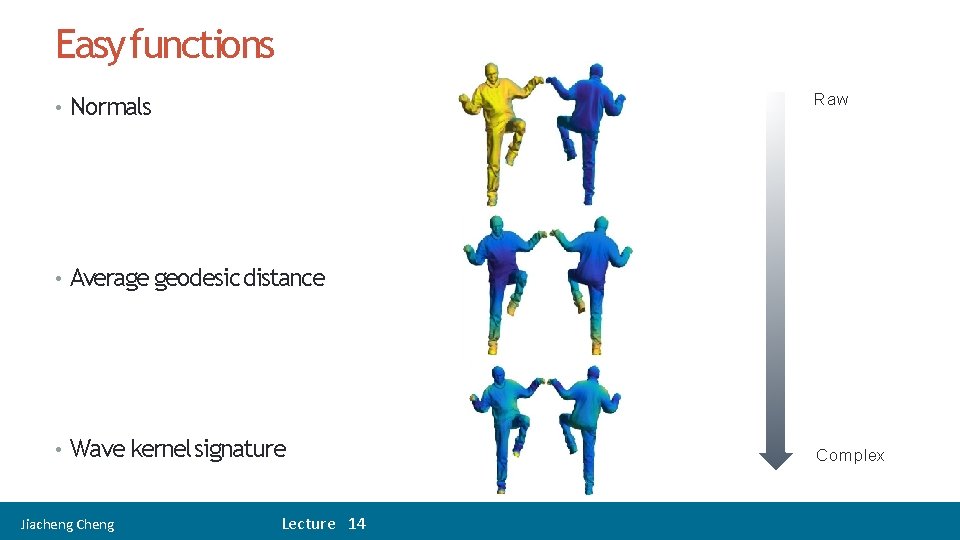
Easy functions Raw • Normals • Average geodesic distance • Wave kernel signature Jiacheng Cheng Lecture 14 Complex
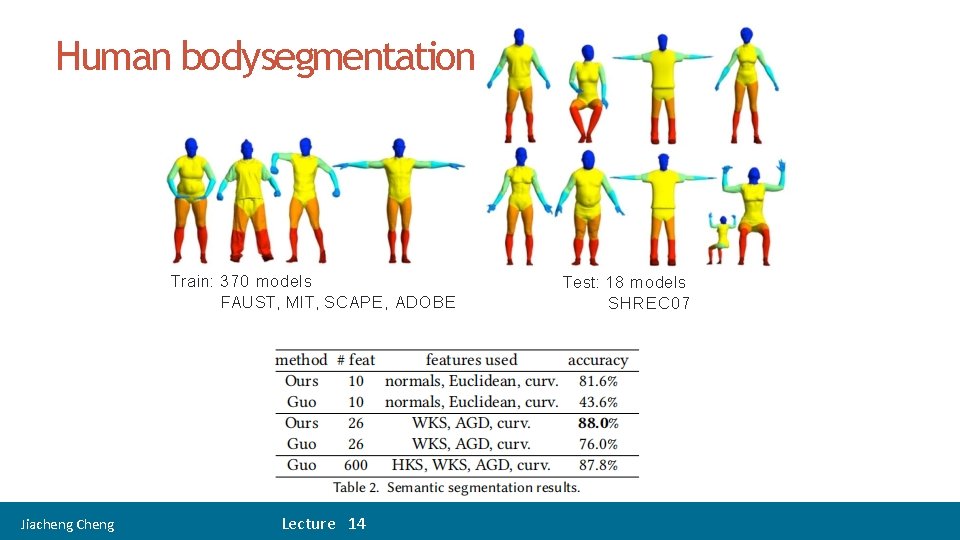
Human bodysegmentation Train: 370 models FAUST, MIT, SCAPE, ADOBE Jiacheng Cheng Lecture 14 Test: 18 models SHREC 07
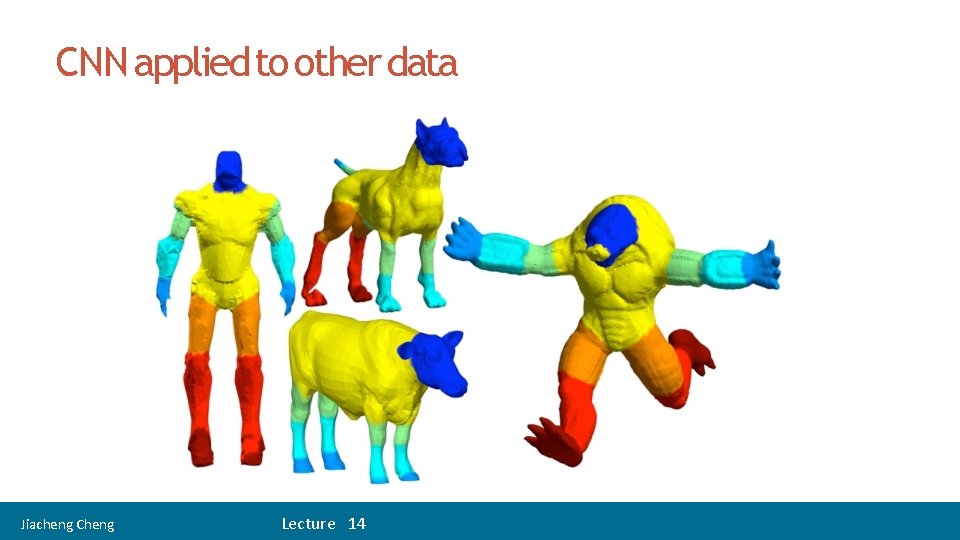
CNN applied to other data Jiacheng Cheng Lecture 14
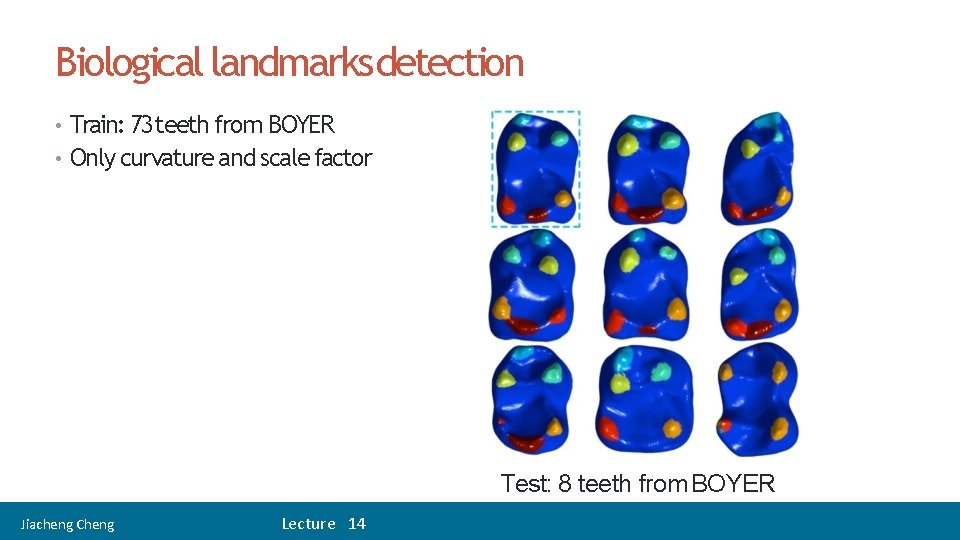
Biological landmarksdetection • Train: 73 teeth from BOYER • Only curvature and scale factor Test: 8 teeth from BOYER Jiacheng Cheng Lecture 14
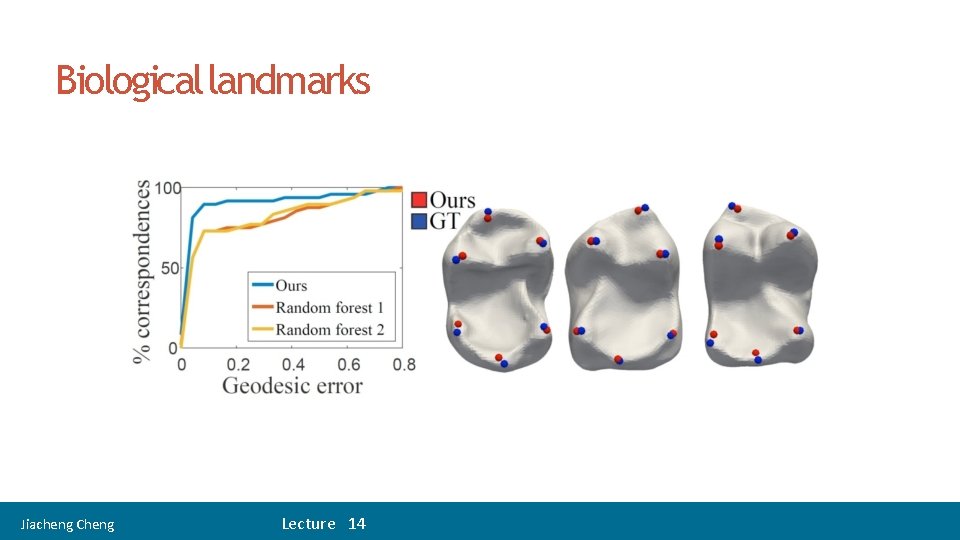
32 Biological landmarks Jiacheng Cheng Lecture 14
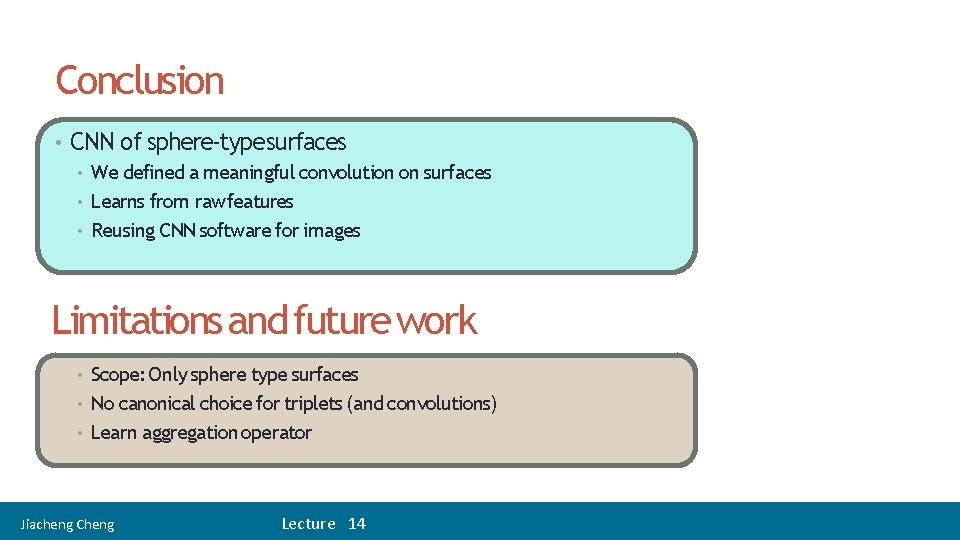
35 Conclusion • CNN of sphere-typesurfaces • We defined a meaningful convolution on surfaces • Learns from rawfeatures • Reusing CNN software for images Limitations and future work Scope: Only sphere type surfaces • No canonical choice for triplets (and convolutions) • Learn aggregation operator • Jiacheng Cheng Lecture 14
Convolutional neural networks
Visualizing and understanding convolutional networks
Csrmm
Convolutional neural network alternatives
Convolutional neural networks for visual recognition
Introduction to convolutional neural networks
Leon gatys
Convolutional neural networks
Cnn ppt
Convolutional neural network
Convolutional deep belief networks
Image super resolution using deep convolutional networks
Superpixel segmentation with fully convolutional networks
Deep convolutional networks
Modeling relational data with graph convolutional networks
Graph neural network lecture
The wake-sleep algorithm for unsupervised neural networks
Neural networks simon haykin
Nvdla
Andrew ng rnn
Newff matlab
Vc dimension neural network
Bharath subramanyam
On the computational efficiency of training neural networks
Few shot learning with graph neural networks
Deep neural networks and mixed integer linear optimization
Predicting nba games using neural networks
Neural networks for rf and microwave design