Least Squares ANOVA ANCOV Least Squares ANOVA Do
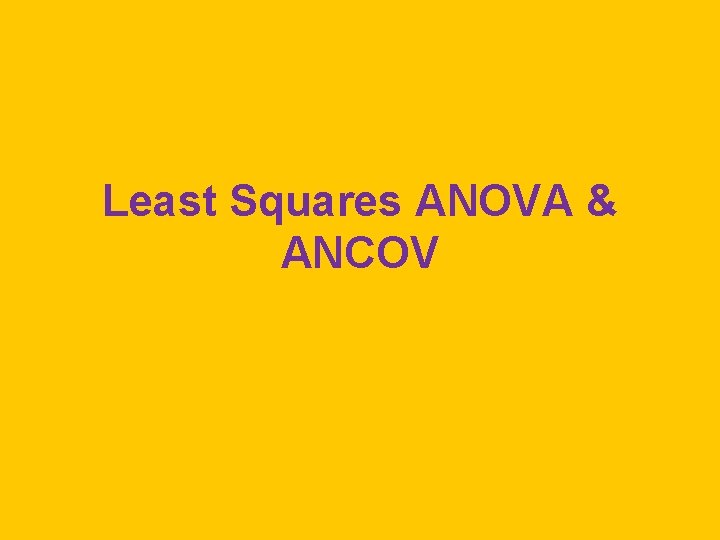
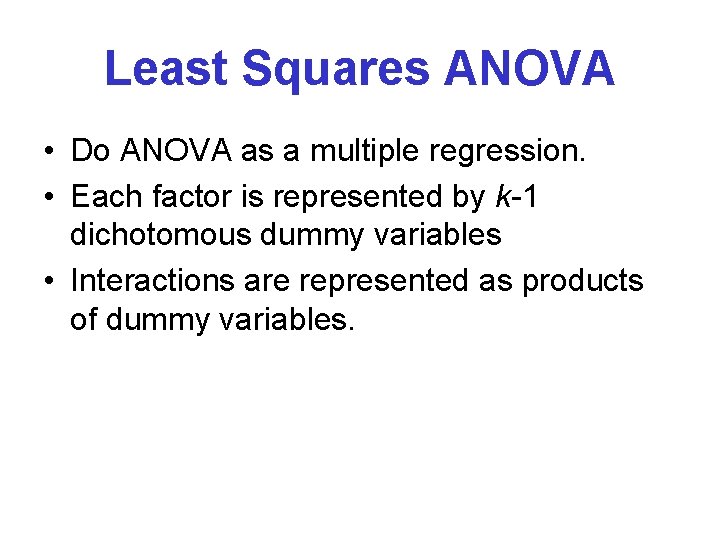
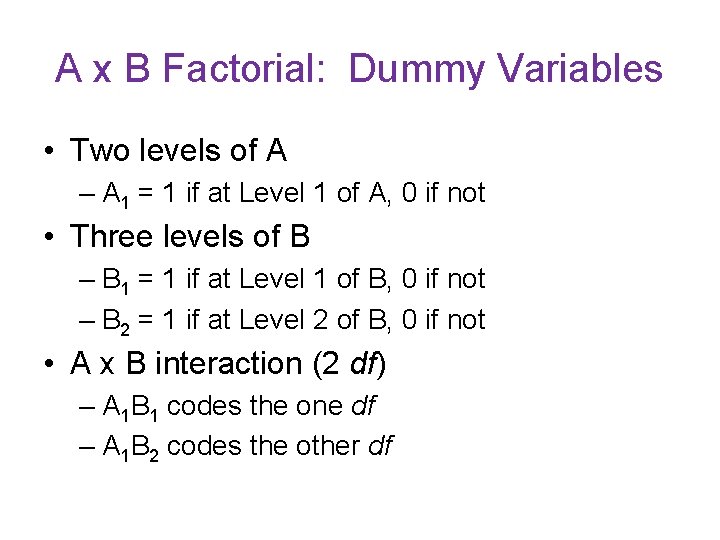
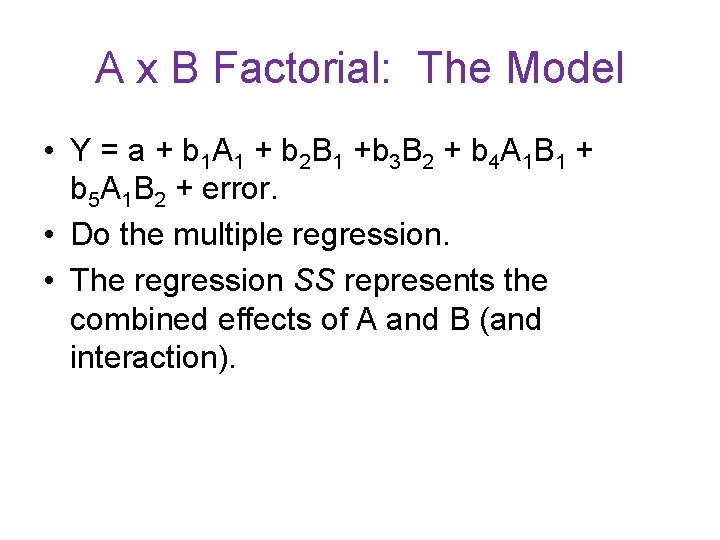
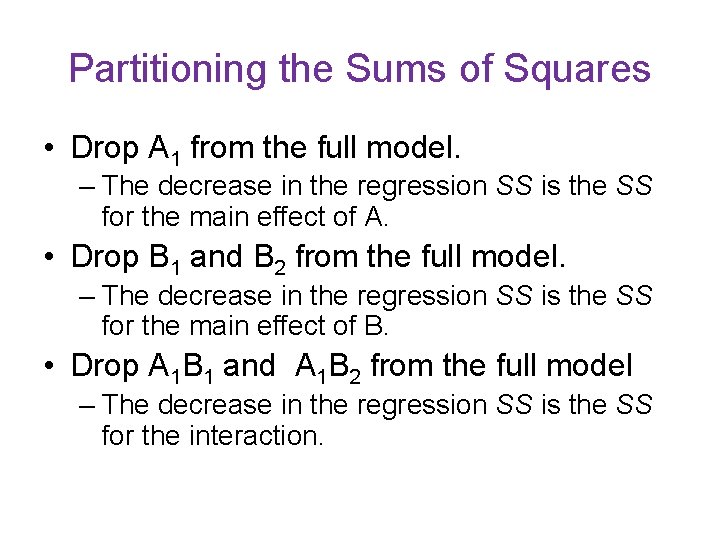
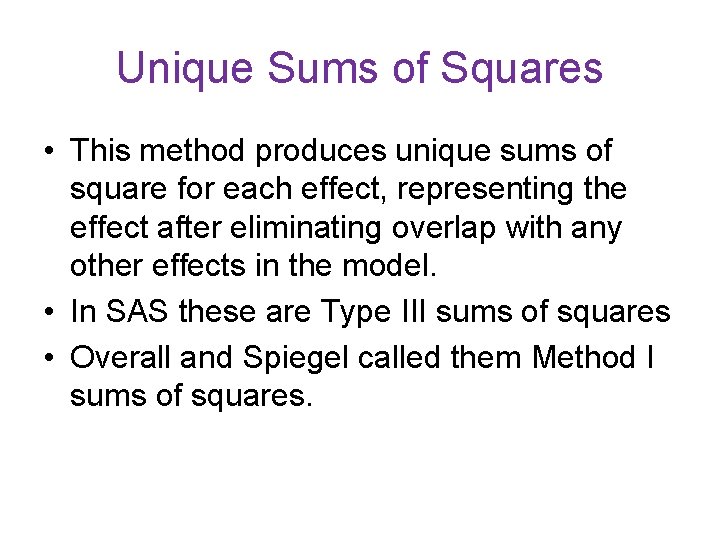
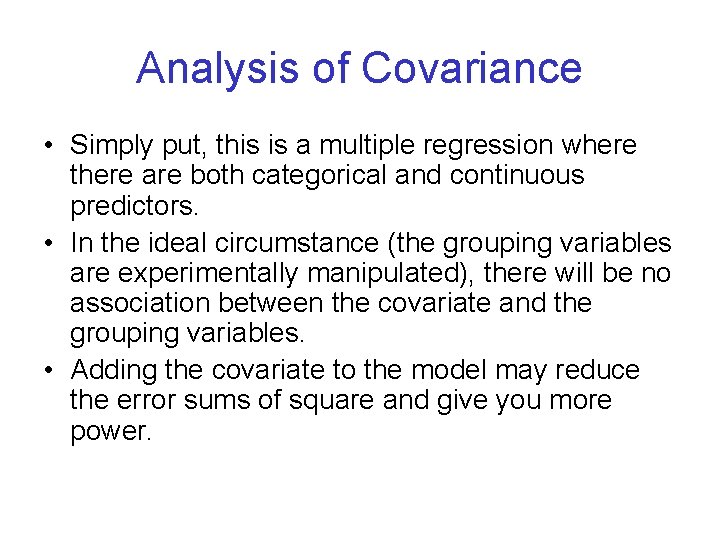
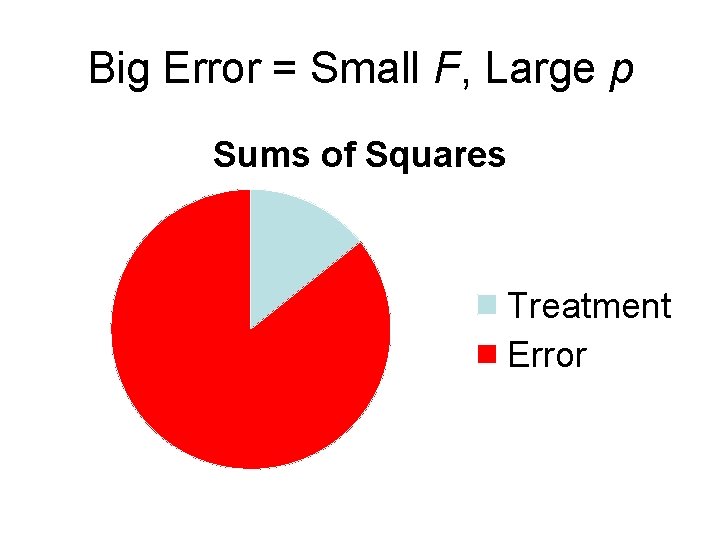
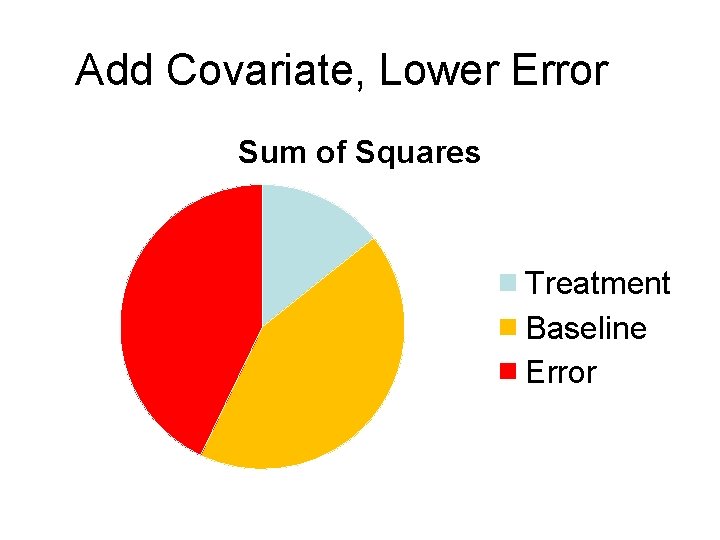
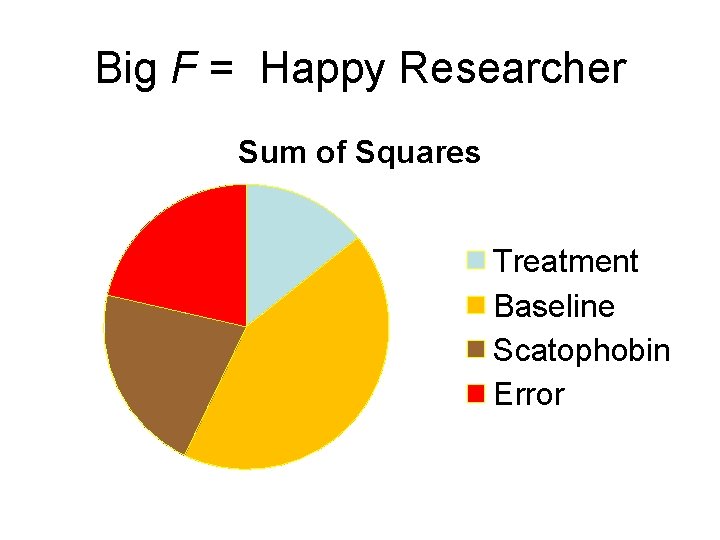
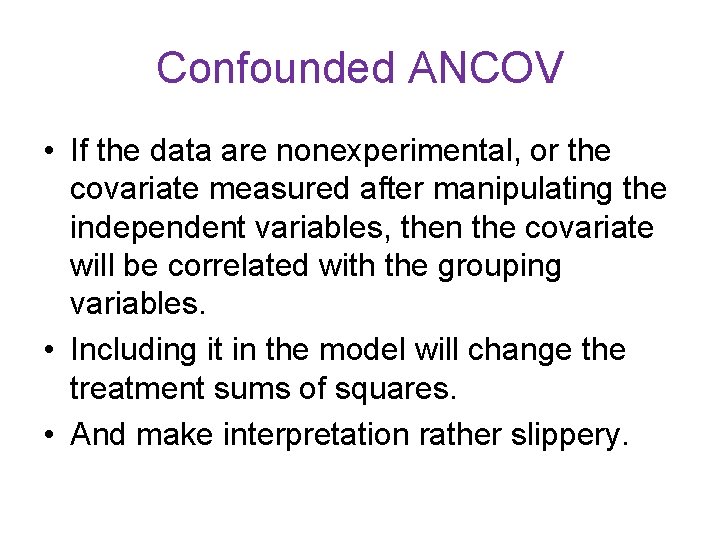
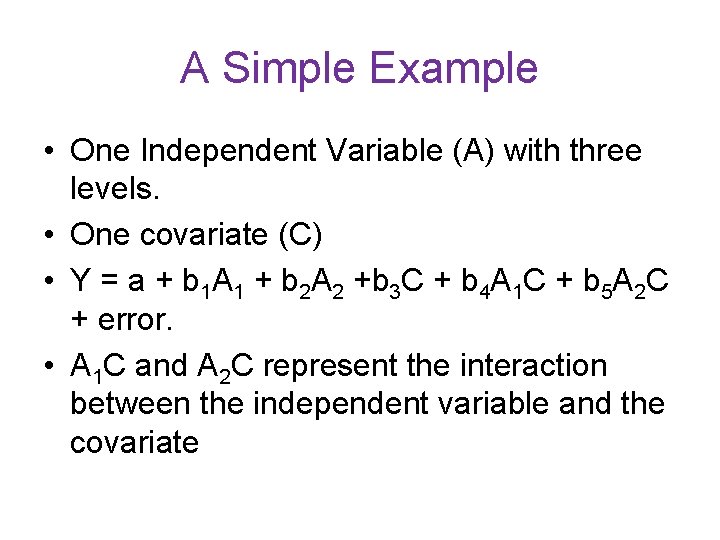
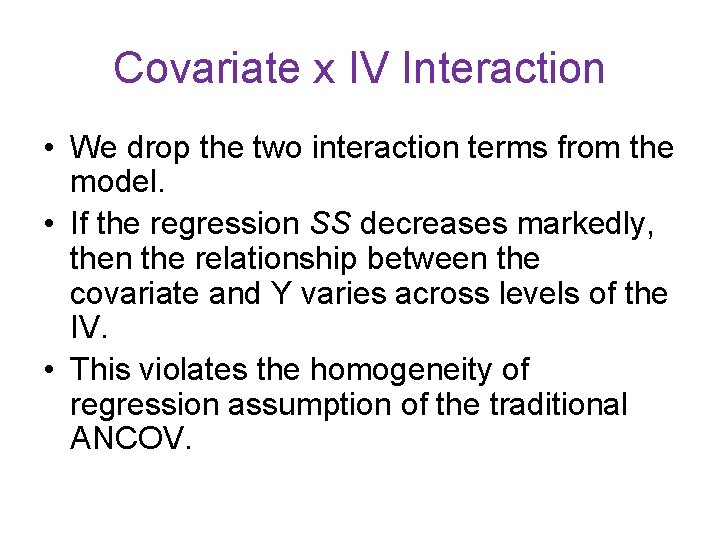
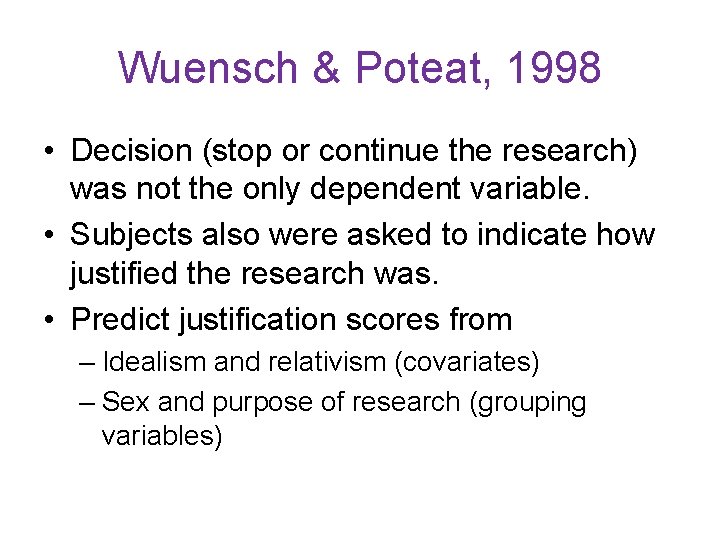
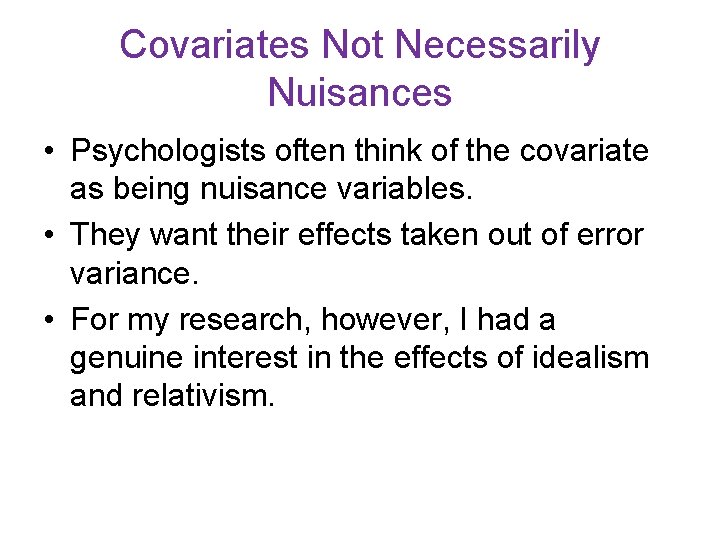
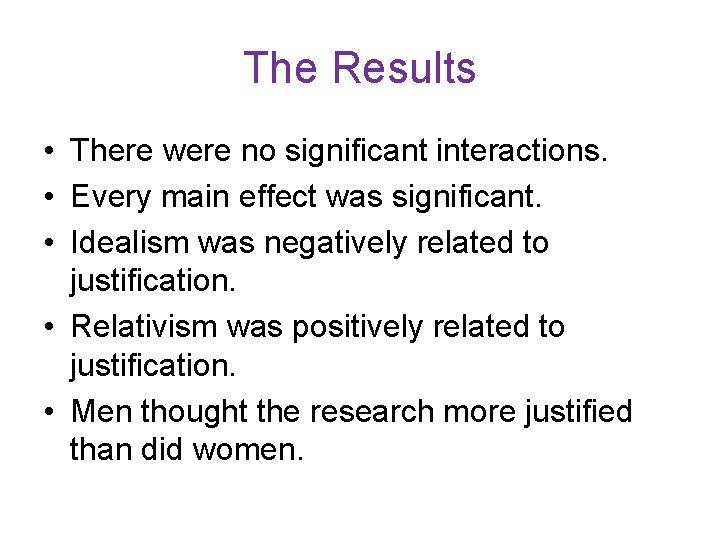
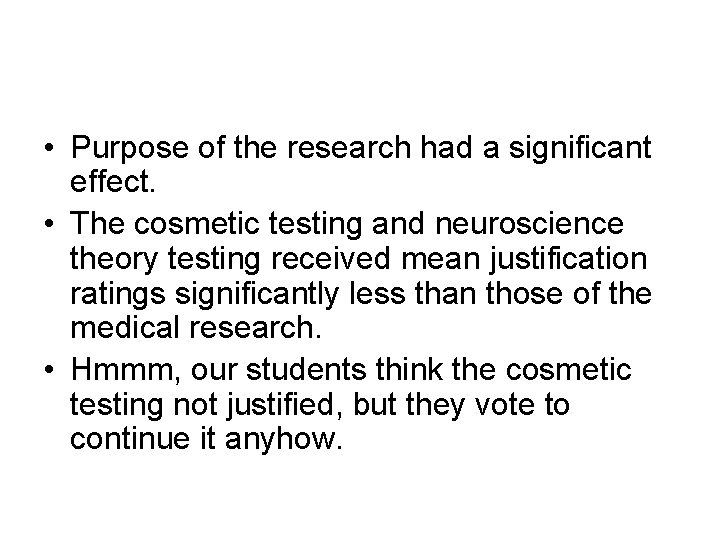
- Slides: 17
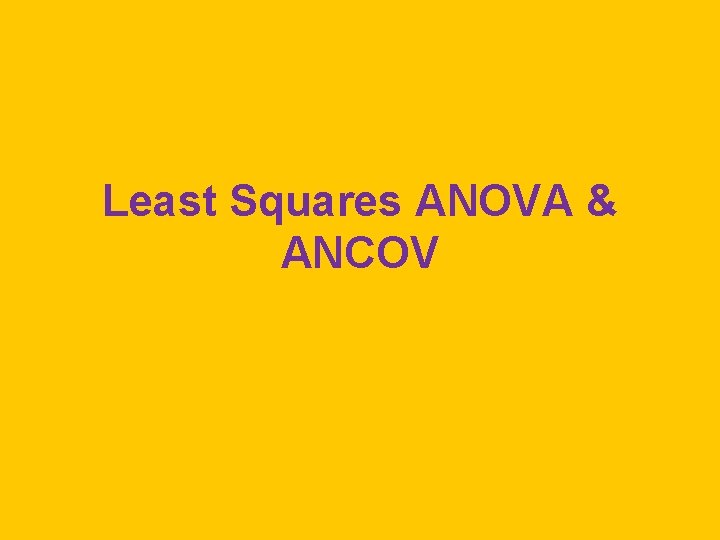
Least Squares ANOVA & ANCOV
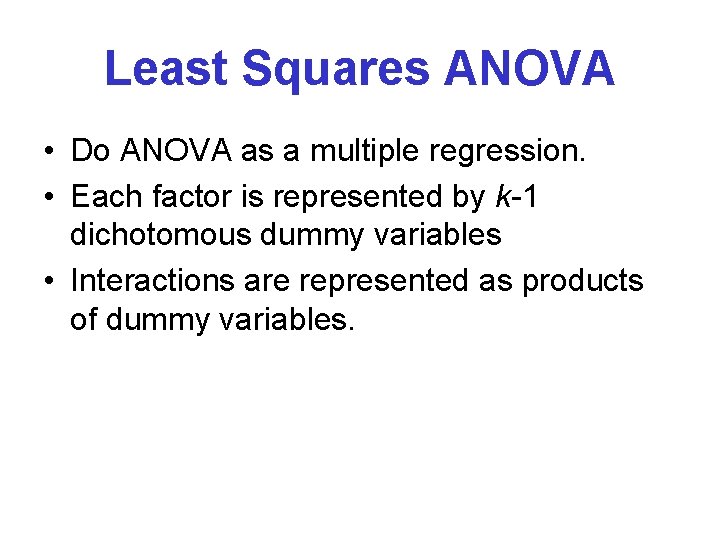
Least Squares ANOVA • Do ANOVA as a multiple regression. • Each factor is represented by k-1 dichotomous dummy variables • Interactions are represented as products of dummy variables.
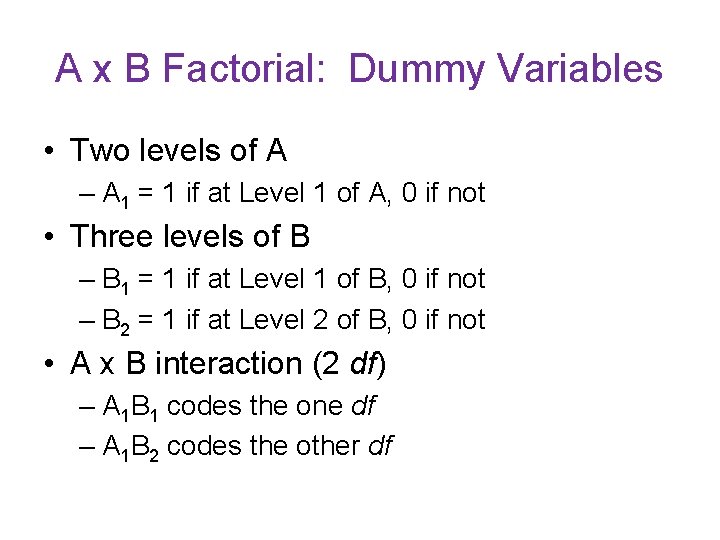
A x B Factorial: Dummy Variables • Two levels of A – A 1 = 1 if at Level 1 of A, 0 if not • Three levels of B – B 1 = 1 if at Level 1 of B, 0 if not – B 2 = 1 if at Level 2 of B, 0 if not • A x B interaction (2 df) – A 1 B 1 codes the one df – A 1 B 2 codes the other df
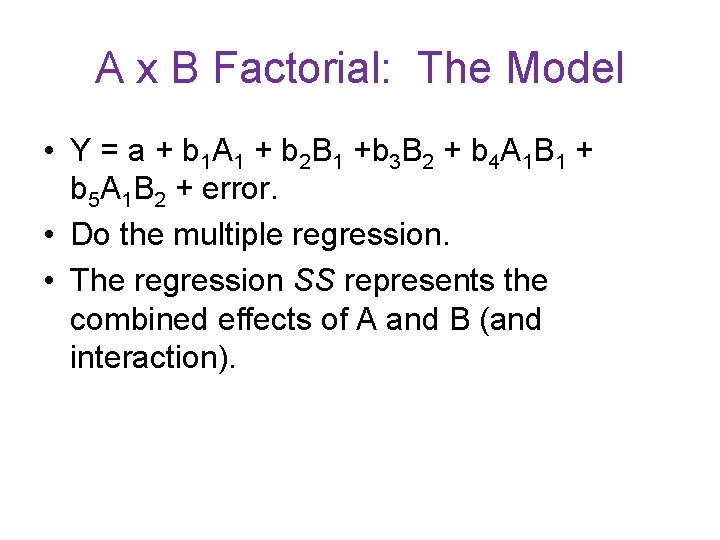
A x B Factorial: The Model • Y = a + b 1 A 1 + b 2 B 1 +b 3 B 2 + b 4 A 1 B 1 + b 5 A 1 B 2 + error. • Do the multiple regression. • The regression SS represents the combined effects of A and B (and interaction).
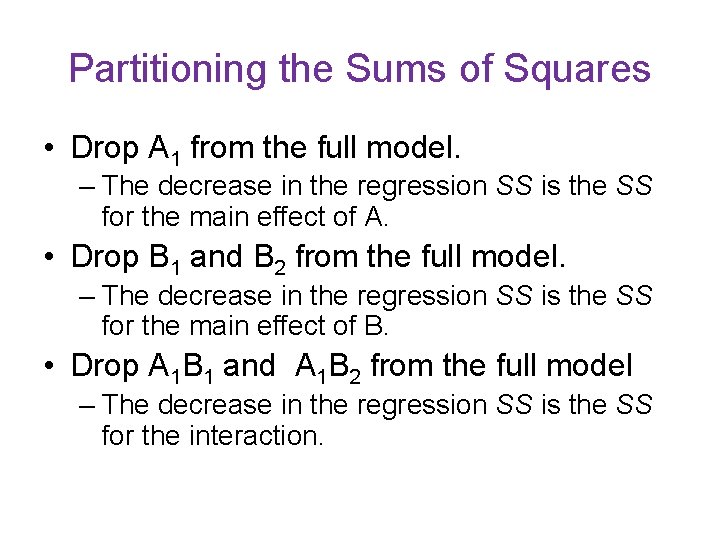
Partitioning the Sums of Squares • Drop A 1 from the full model. – The decrease in the regression SS is the SS for the main effect of A. • Drop B 1 and B 2 from the full model. – The decrease in the regression SS is the SS for the main effect of B. • Drop A 1 B 1 and A 1 B 2 from the full model – The decrease in the regression SS is the SS for the interaction.
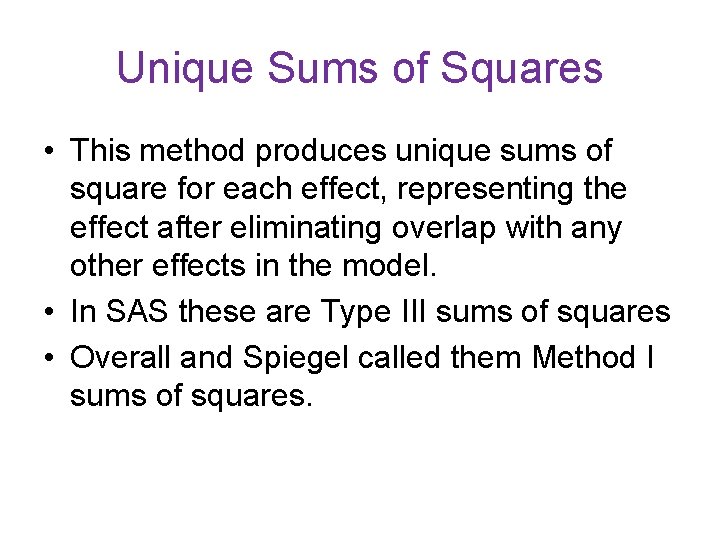
Unique Sums of Squares • This method produces unique sums of square for each effect, representing the effect after eliminating overlap with any other effects in the model. • In SAS these are Type III sums of squares • Overall and Spiegel called them Method I sums of squares.
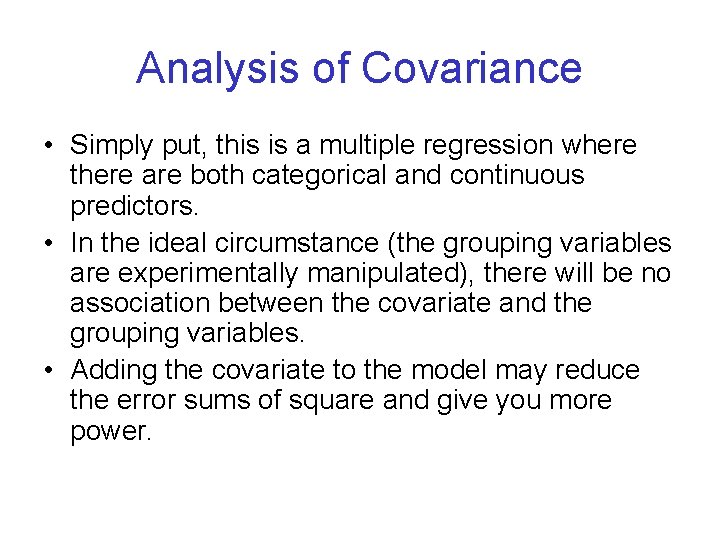
Analysis of Covariance • Simply put, this is a multiple regression where there are both categorical and continuous predictors. • In the ideal circumstance (the grouping variables are experimentally manipulated), there will be no association between the covariate and the grouping variables. • Adding the covariate to the model may reduce the error sums of square and give you more power.
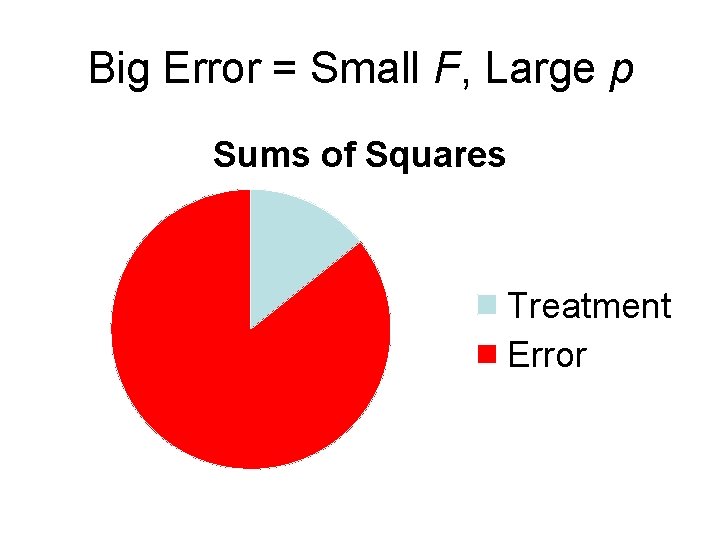
Big Error = Small F, Large p Sums of Squares Treatment Error
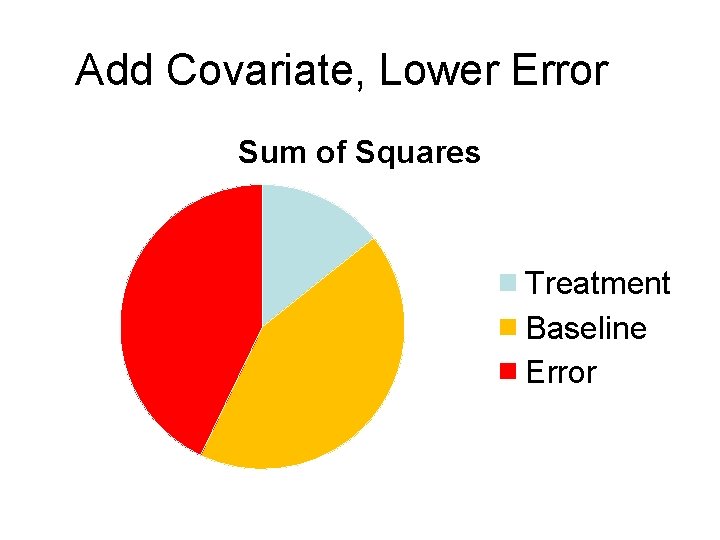
Add Covariate, Lower Error Sum of Squares Treatment Baseline Error
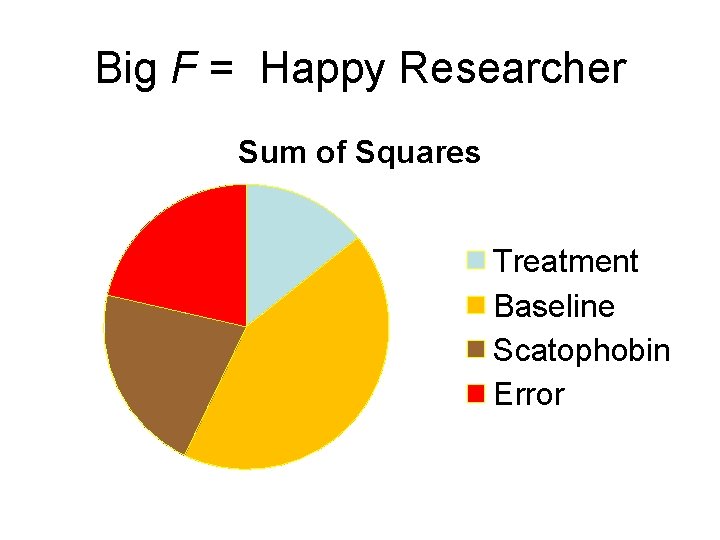
Big F = Happy Researcher Sum of Squares Treatment Baseline Scatophobin Error
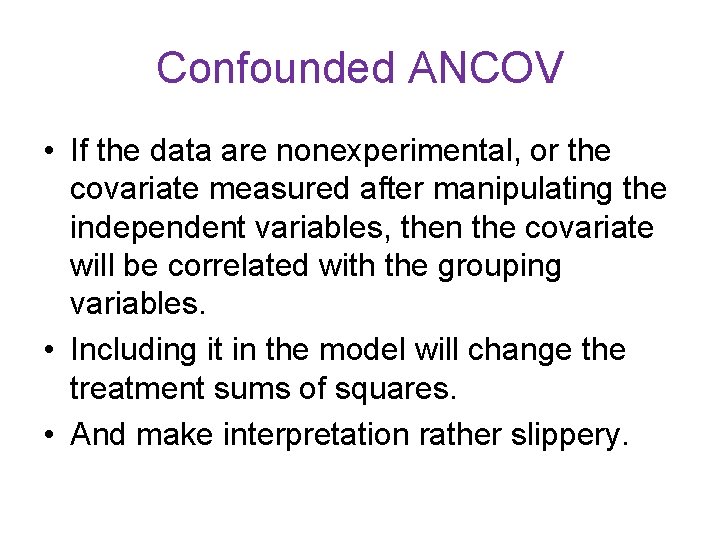
Confounded ANCOV • If the data are nonexperimental, or the covariate measured after manipulating the independent variables, then the covariate will be correlated with the grouping variables. • Including it in the model will change the treatment sums of squares. • And make interpretation rather slippery.
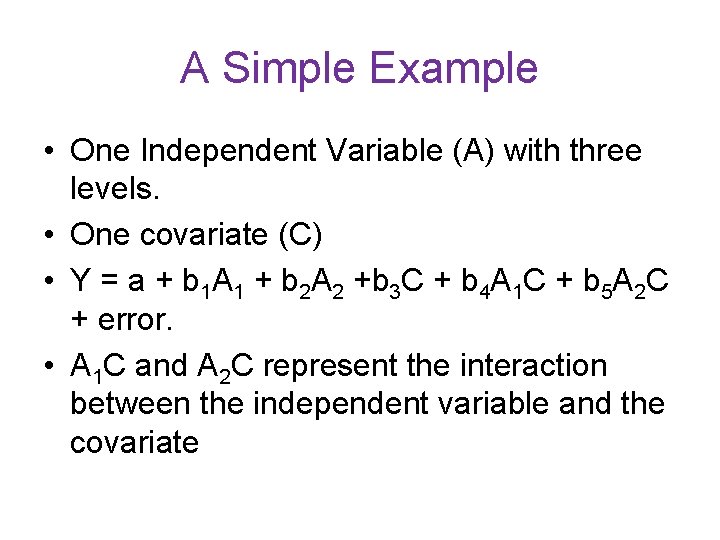
A Simple Example • One Independent Variable (A) with three levels. • One covariate (C) • Y = a + b 1 A 1 + b 2 A 2 +b 3 C + b 4 A 1 C + b 5 A 2 C + error. • A 1 C and A 2 C represent the interaction between the independent variable and the covariate
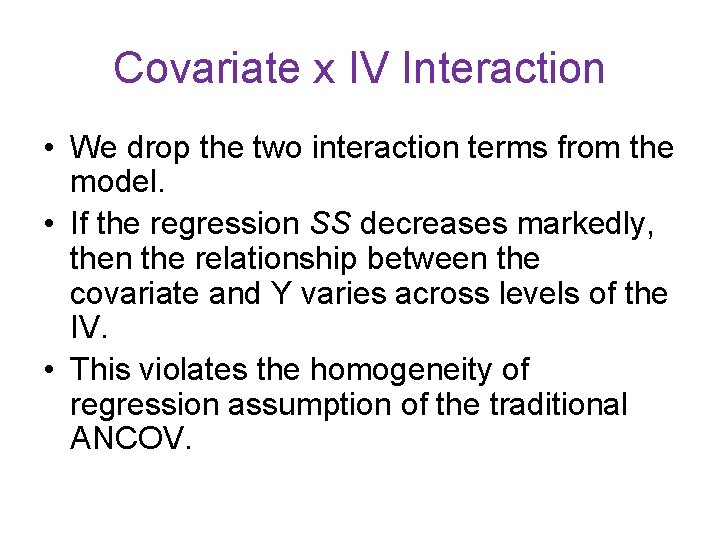
Covariate x IV Interaction • We drop the two interaction terms from the model. • If the regression SS decreases markedly, then the relationship between the covariate and Y varies across levels of the IV. • This violates the homogeneity of regression assumption of the traditional ANCOV.
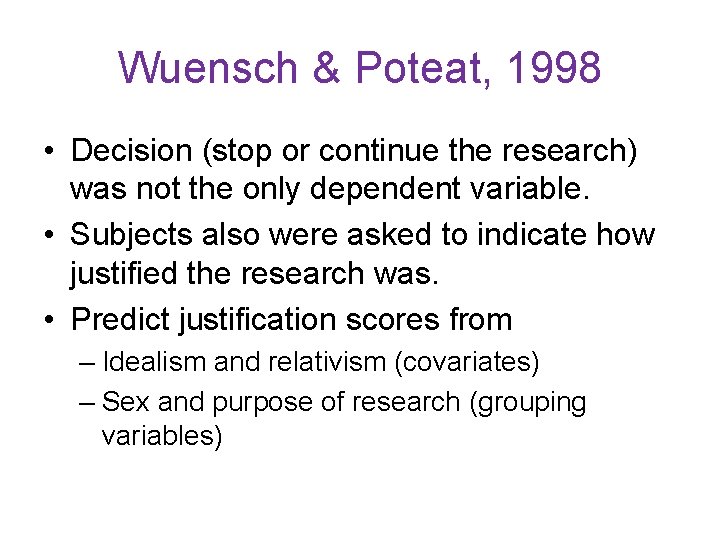
Wuensch & Poteat, 1998 • Decision (stop or continue the research) was not the only dependent variable. • Subjects also were asked to indicate how justified the research was. • Predict justification scores from – Idealism and relativism (covariates) – Sex and purpose of research (grouping variables)
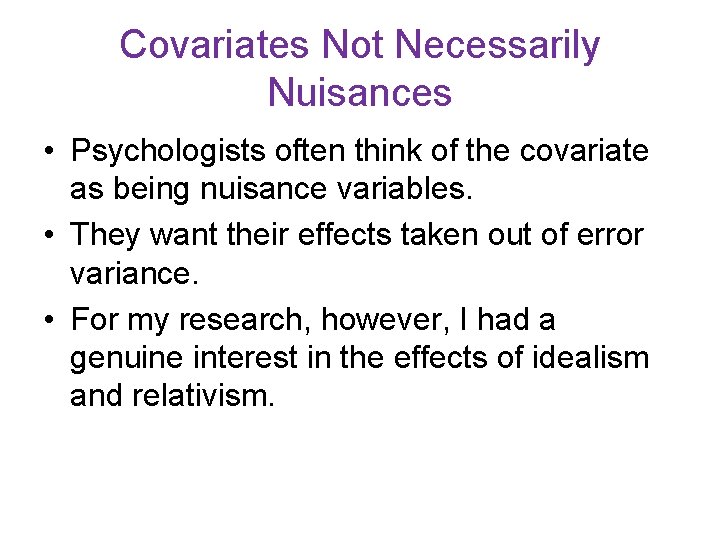
Covariates Not Necessarily Nuisances • Psychologists often think of the covariate as being nuisance variables. • They want their effects taken out of error variance. • For my research, however, I had a genuine interest in the effects of idealism and relativism.
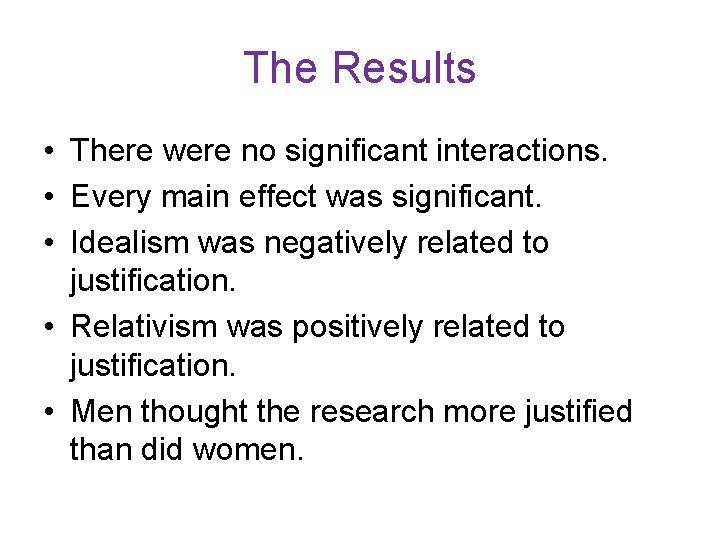
The Results • There were no significant interactions. • Every main effect was significant. • Idealism was negatively related to justification. • Relativism was positively related to justification. • Men thought the research more justified than did women.
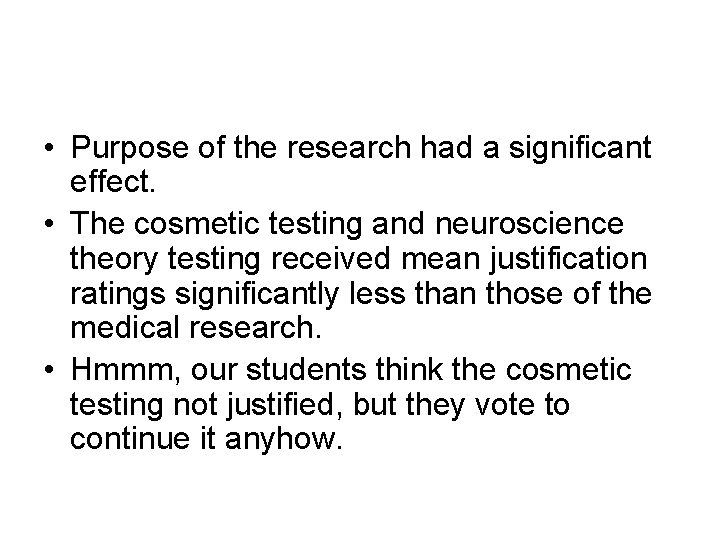
• Purpose of the research had a significant effect. • The cosmetic testing and neuroscience theory testing received mean justification ratings significantly less than those of the medical research. • Hmmm, our students think the cosmetic testing not justified, but they vote to continue it anyhow.
Ancov
One way anova vs two way anova
Contoh one way anova dan two way anova
Beda one way anova dan two way anova
Anova sum of squares formula
Damien thiesson
Youcubed squares and more squares answers
Least squares regression line statcrunch
Weighted least squares eviews
Regression berlin
Bivariate least squares regression
4d3d41669541f1bf19acde21e19e43d23ebbd23b
Dofs scatter plot
Polynomial regression least squares
Lms cis
Least squares regression line minitab
Continuous least squares approximation
Least square solution