Imperial College London Universit La Sapienza Roma Sydney
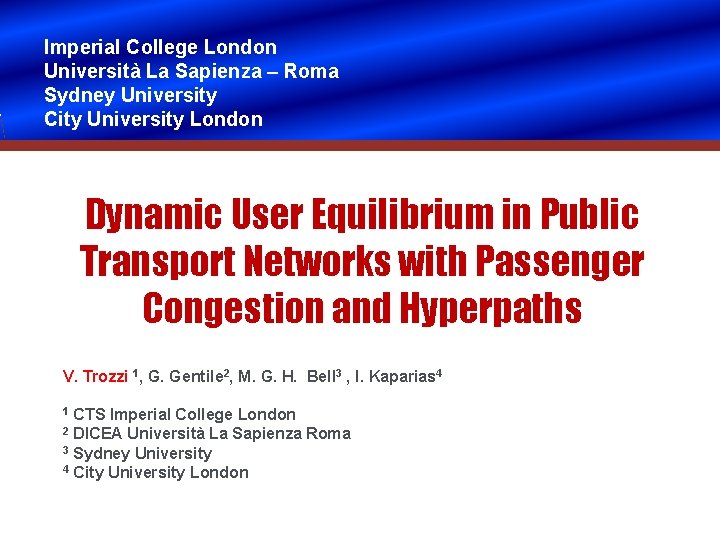
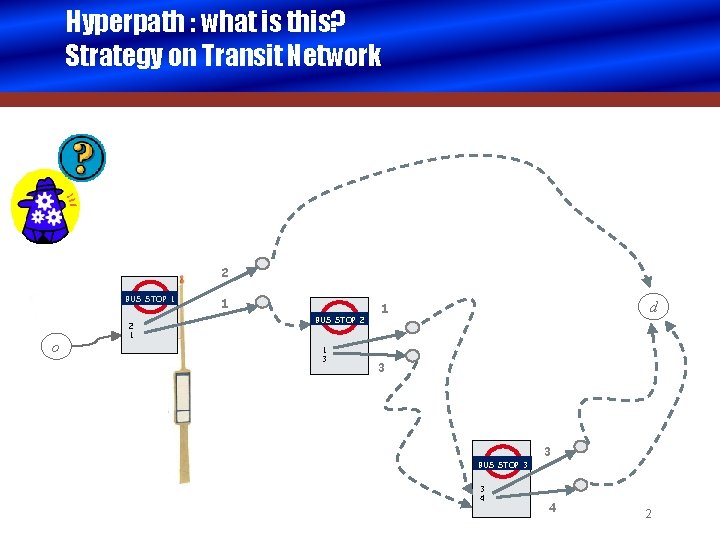
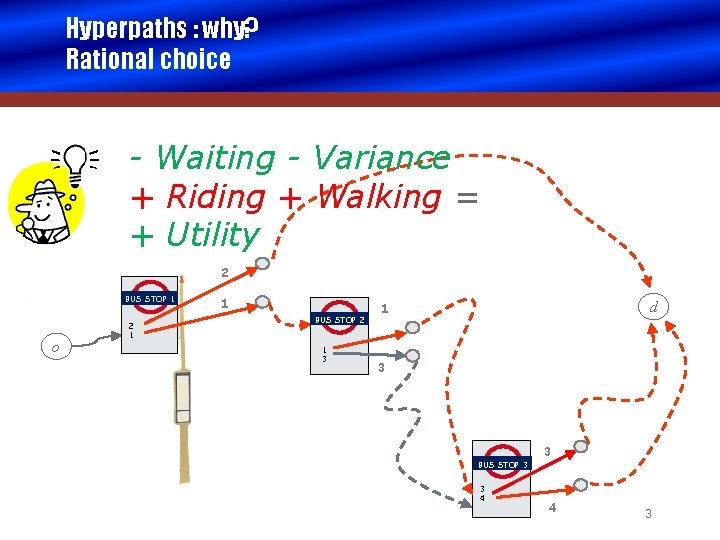
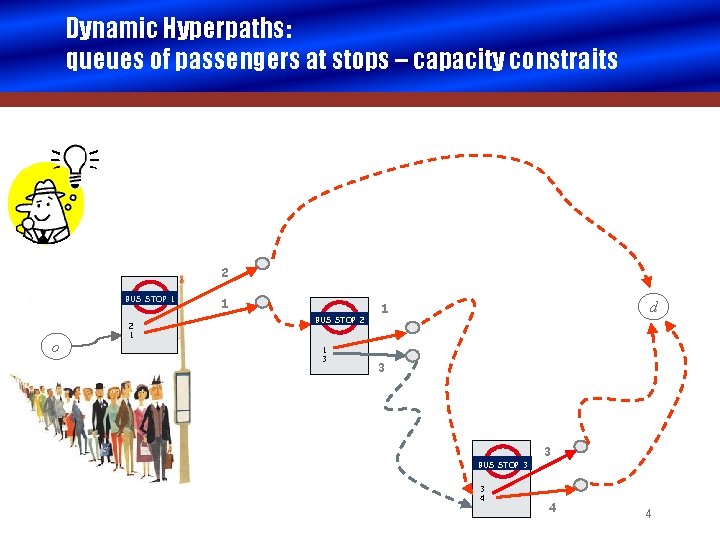
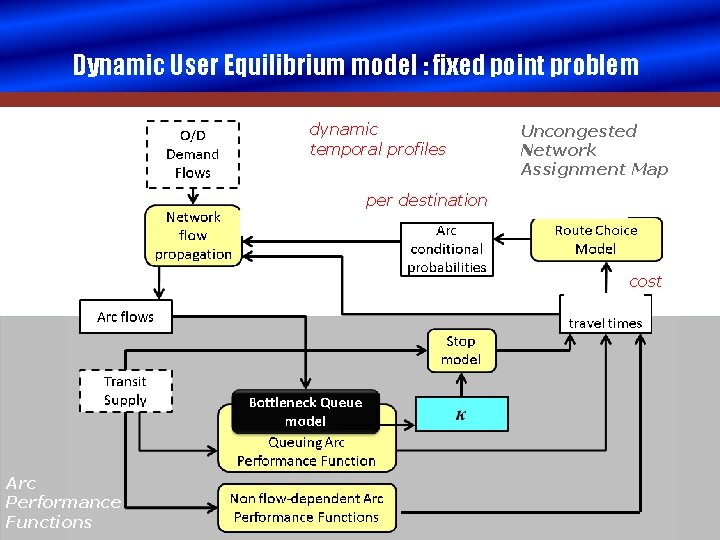
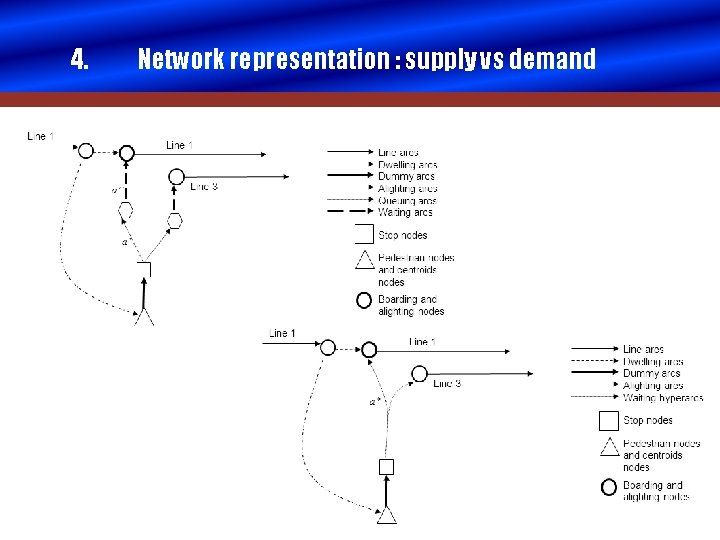
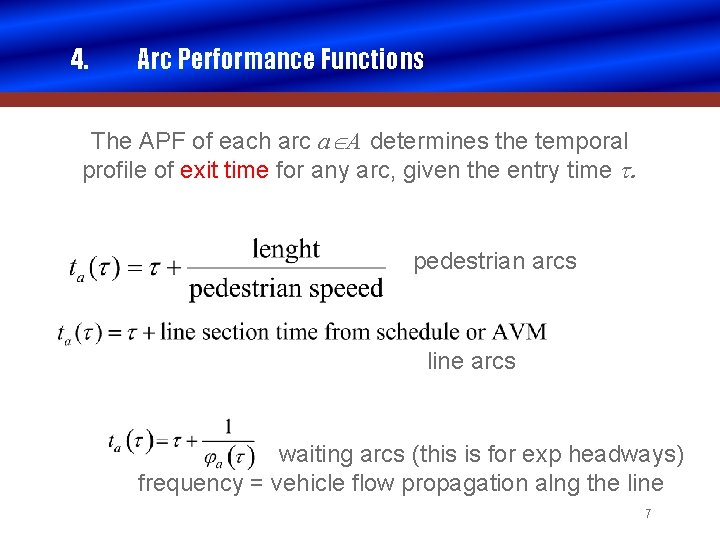
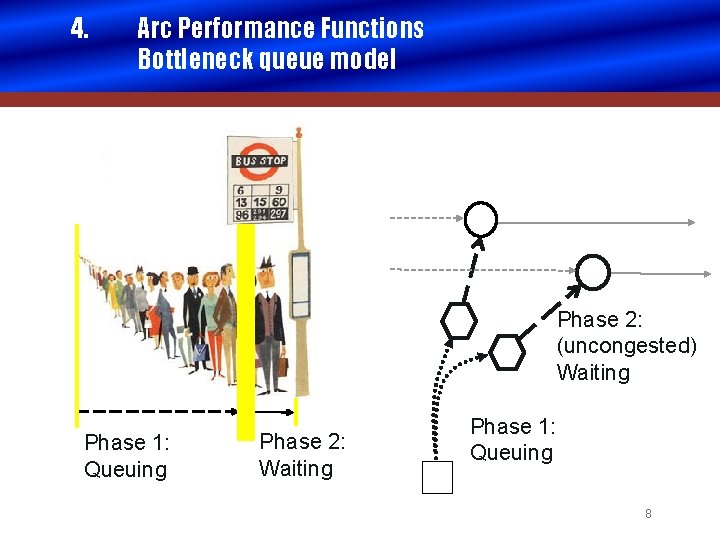
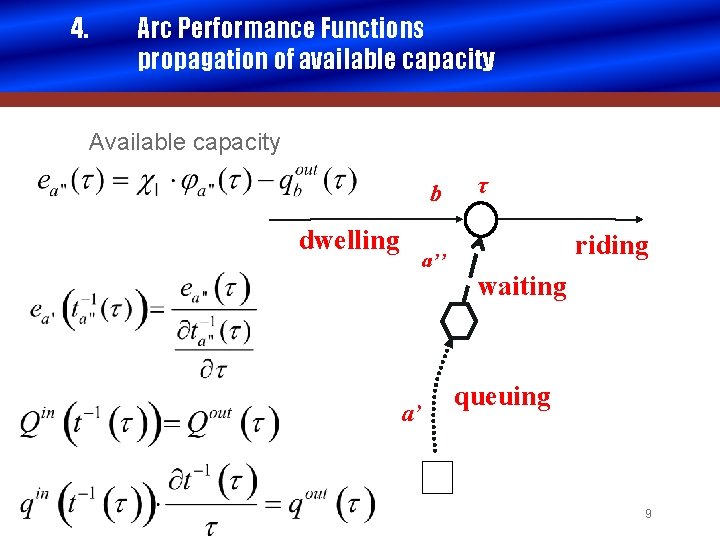
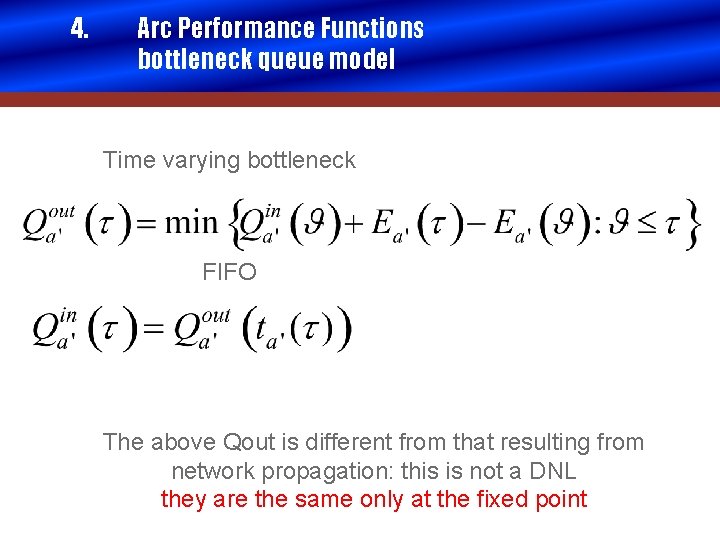
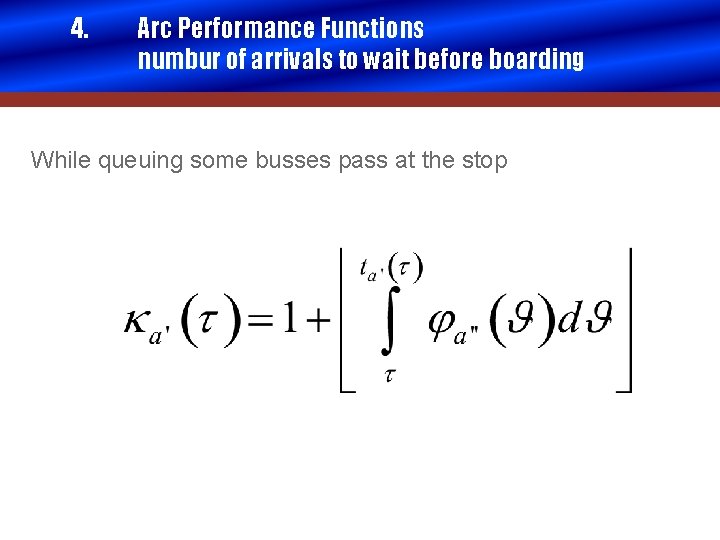
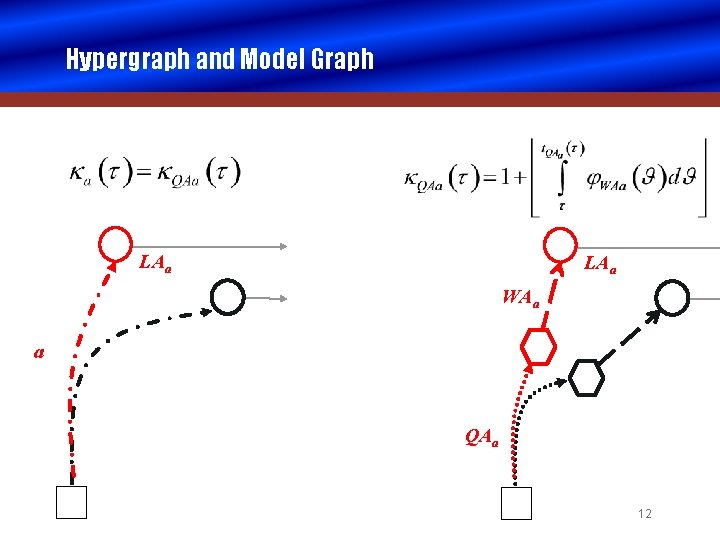
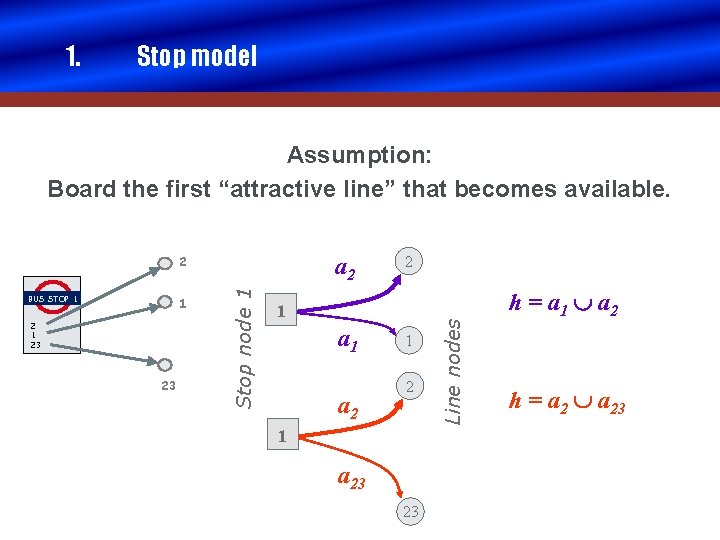
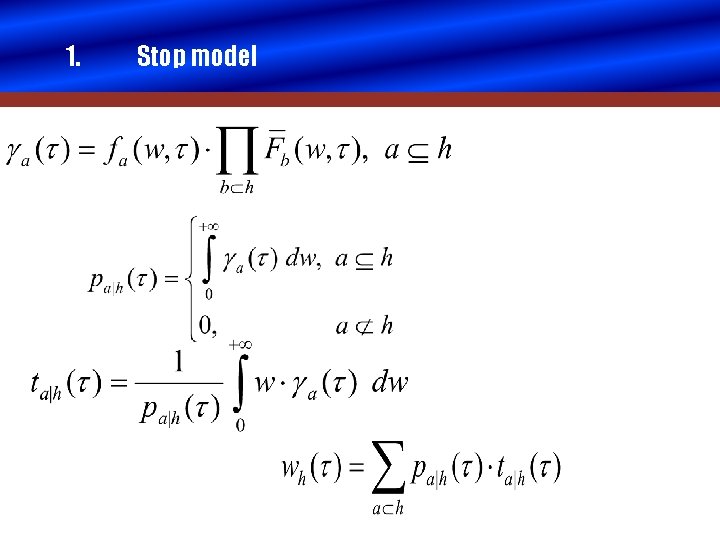
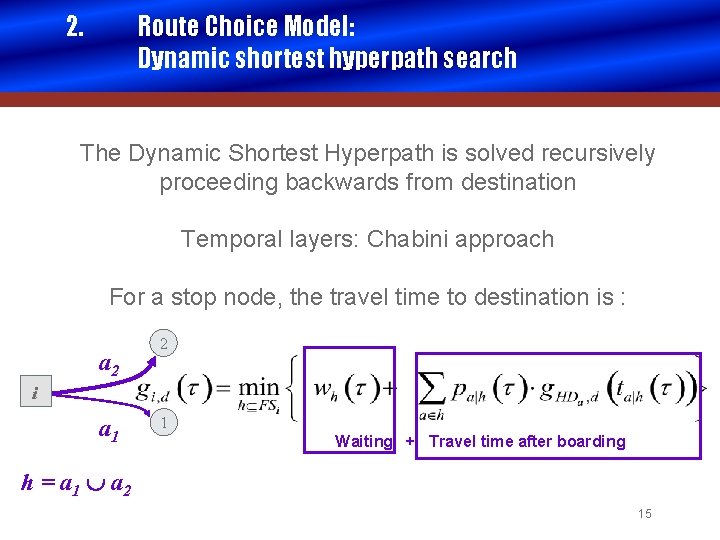
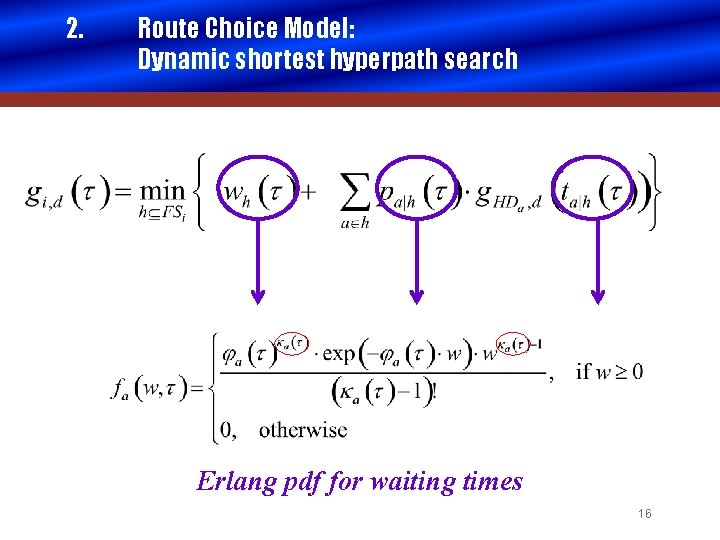
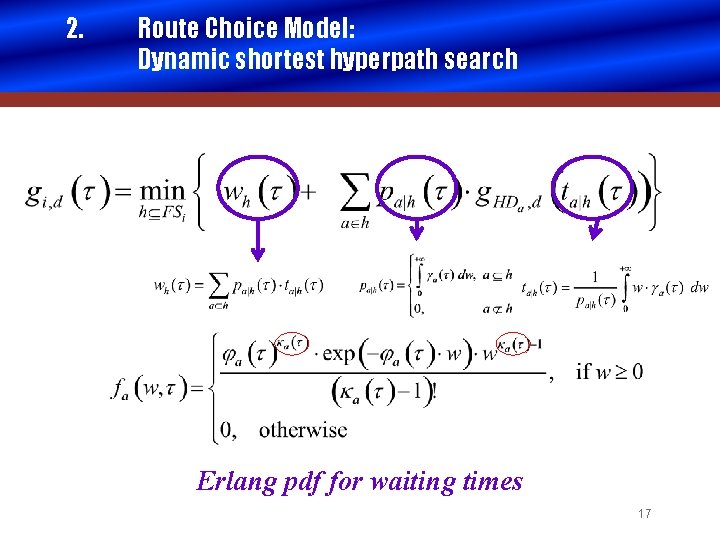
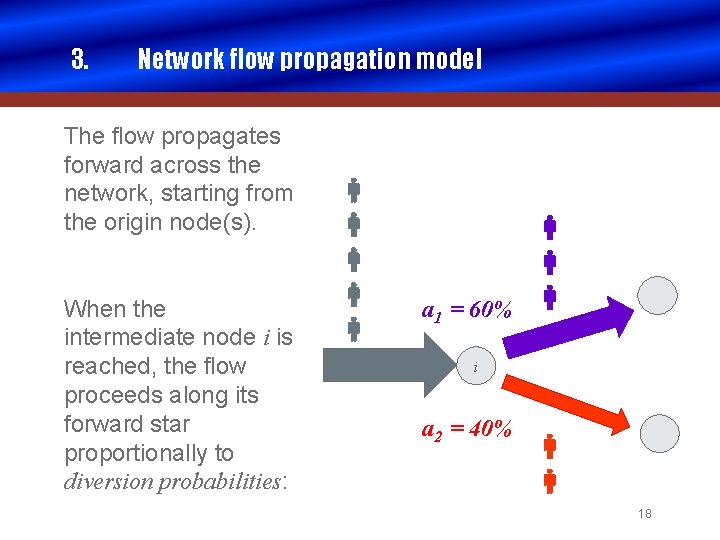
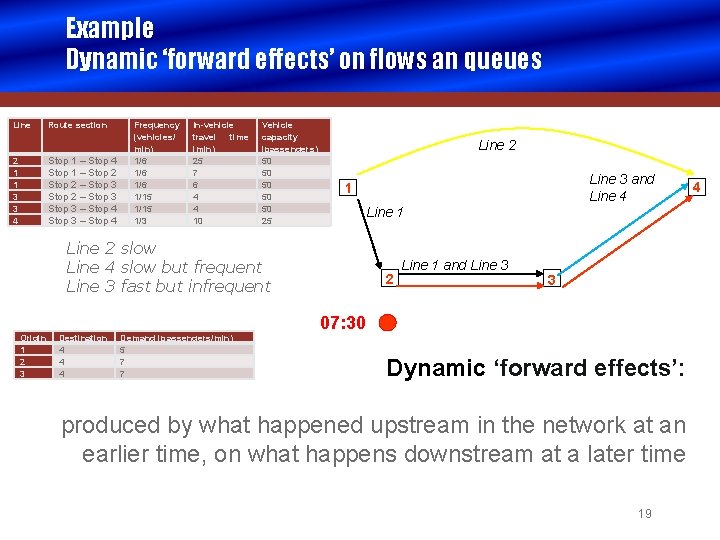
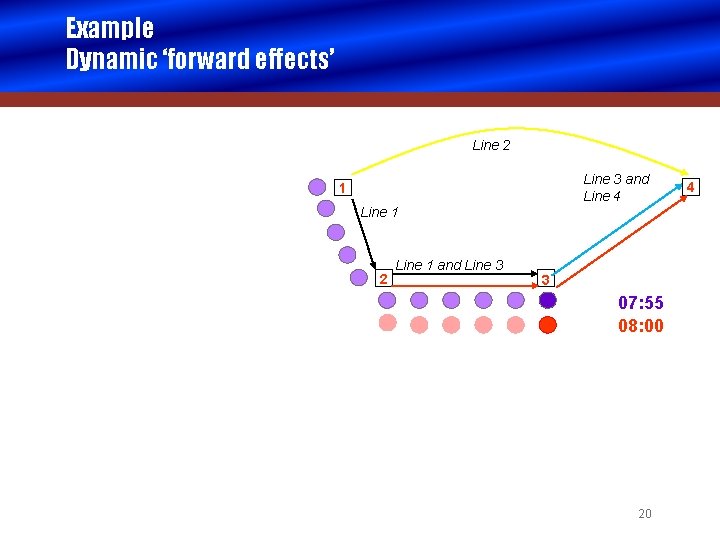
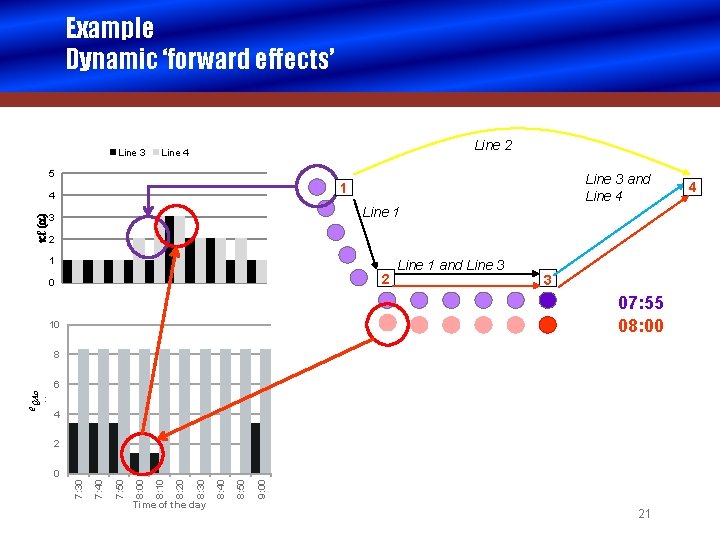
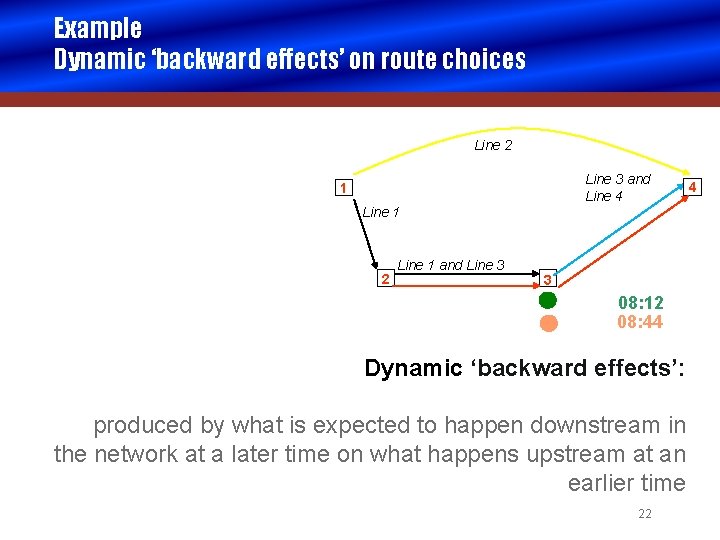
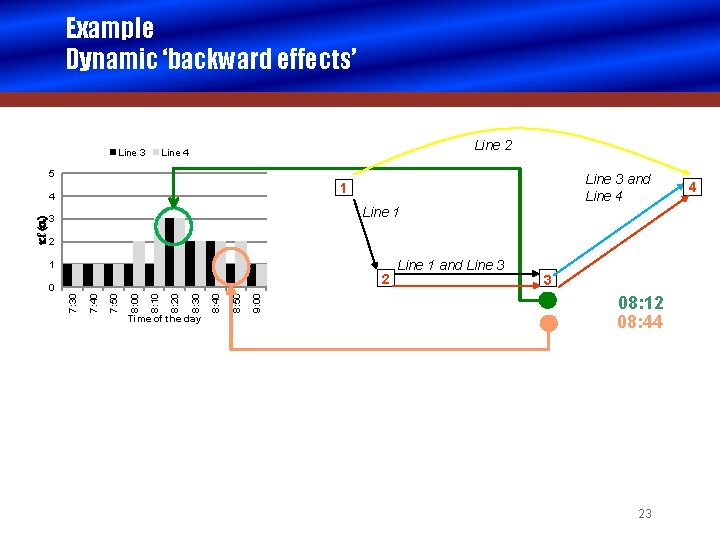
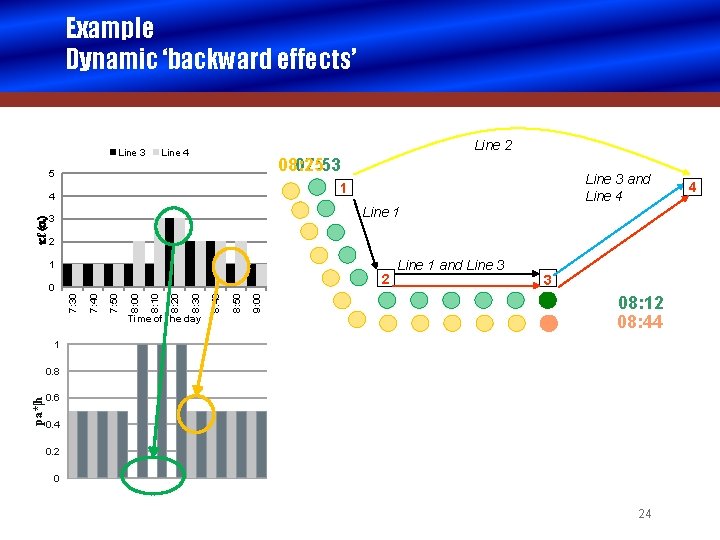
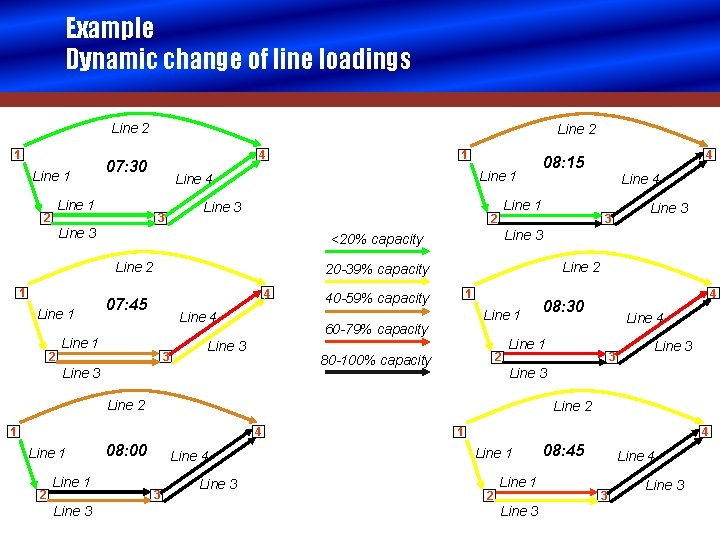
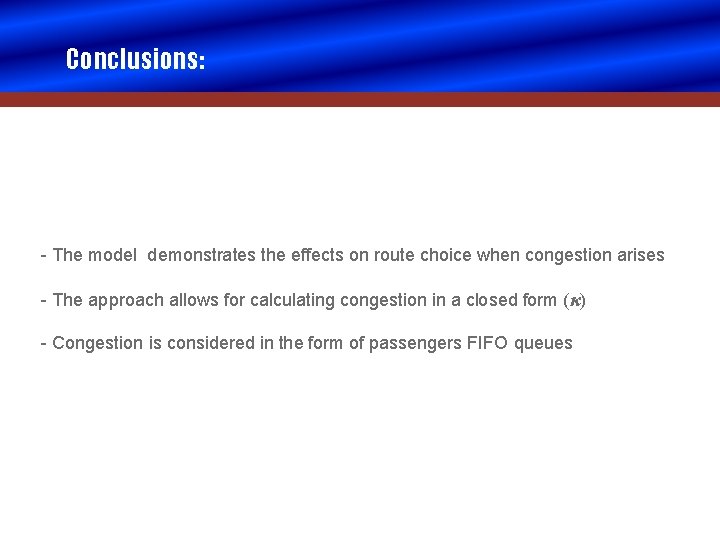
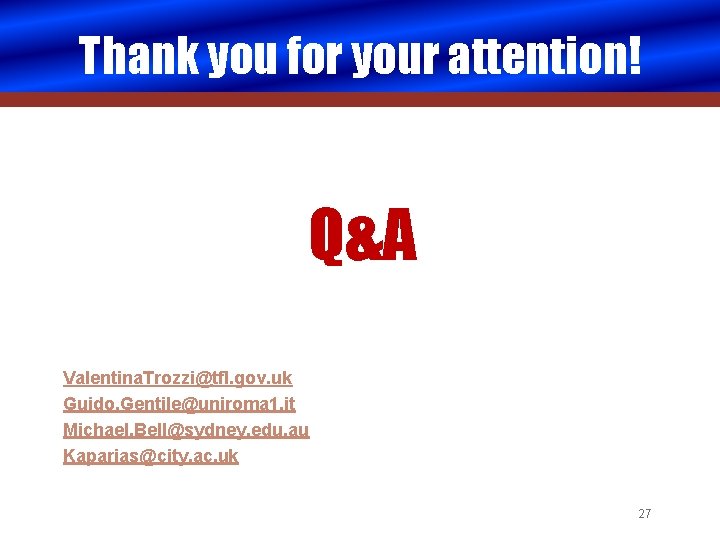
- Slides: 27
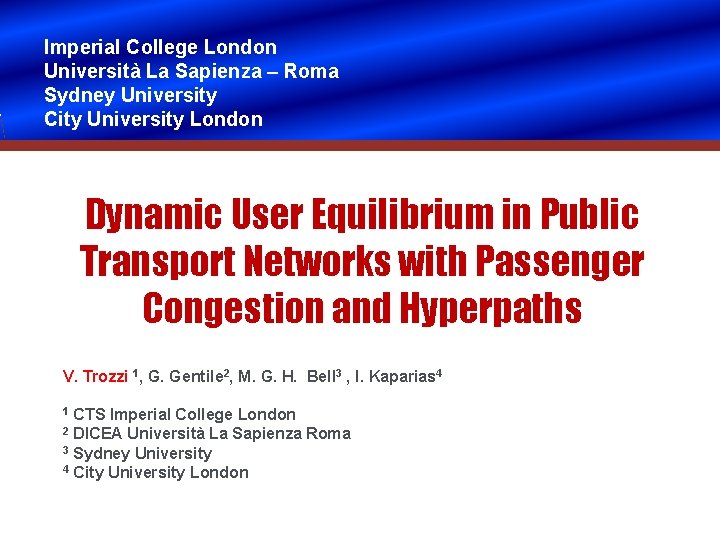
Imperial College London Università La Sapienza – Roma Sydney University City University London Dynamic User Equilibrium in Public Transport Networks with Passenger Congestion and Hyperpaths V. Trozzi 1, G. Gentile 2, M. G. H. Bell 3 , I. Kaparias 4 CTS Imperial College London DICEA Università La Sapienza Roma 3 Sydney University 4 City University London 1 2
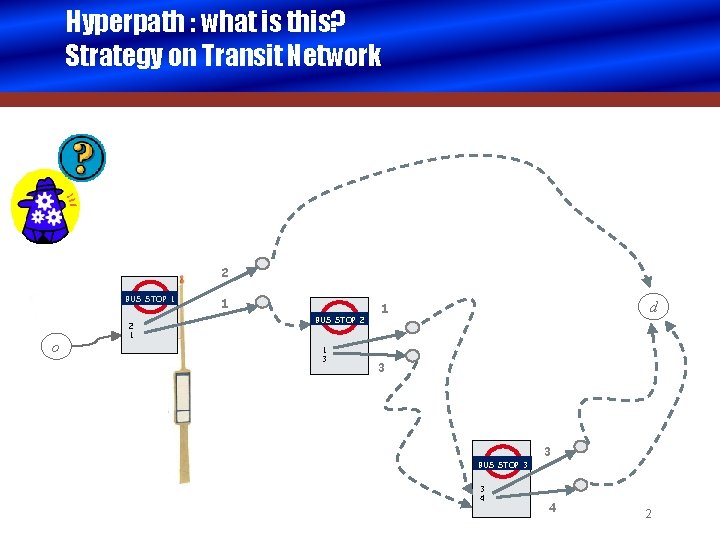
Hyperpath : what is this? Strategy on Transit Network 2 BUS STOP 1 o 2 1 1 BUS STOP 2 1 3 d 1 3 BUS STOP 3 3 4 2
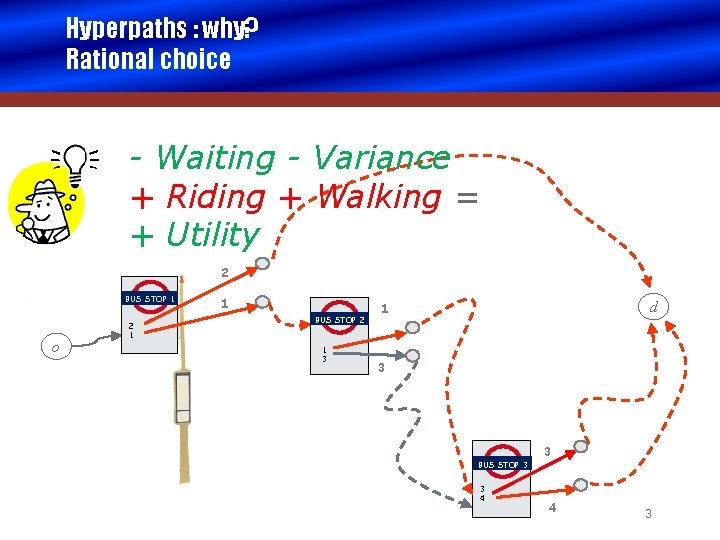
Hyperpaths : why? Rational choice - Waiting - Variance + Riding + Walking = + Utility 2 BUS STOP 1 o 2 1 1 BUS STOP 2 1 3 d 1 3 BUS STOP 3 3 4 3
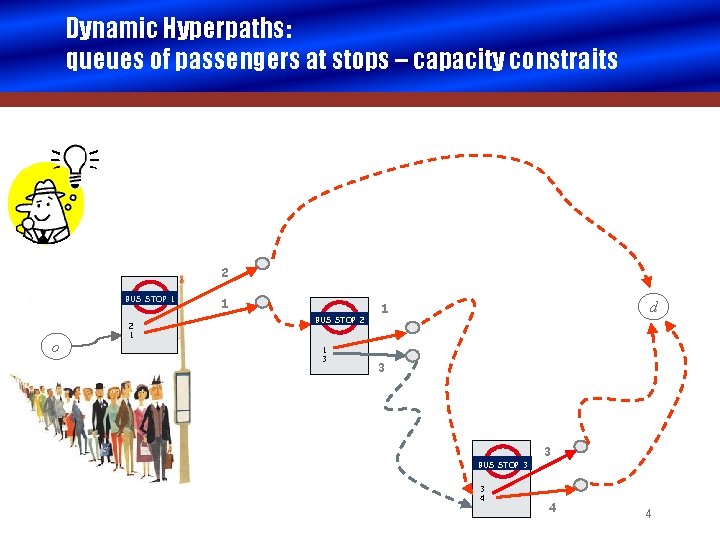
Dynamic Hyperpaths: queues of passengers at stops – capacity constraits 2 BUS STOP 1 o 2 1 1 BUS STOP 2 1 3 d 1 3 BUS STOP 3 3 4 4
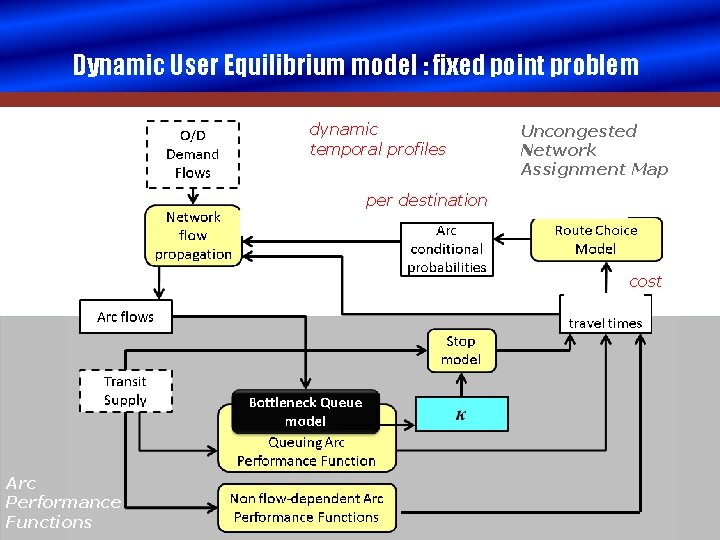
Dynamic User Equilibrium model : fixed point problem dynamic temporal profiles Uncongested Network Assignment Map per destination cost Arc Performance Functions
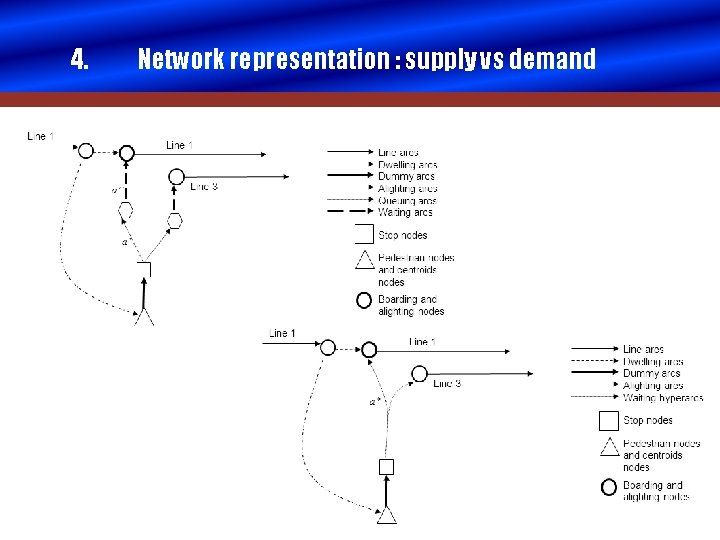
4. Network representation : supply vs demand 6
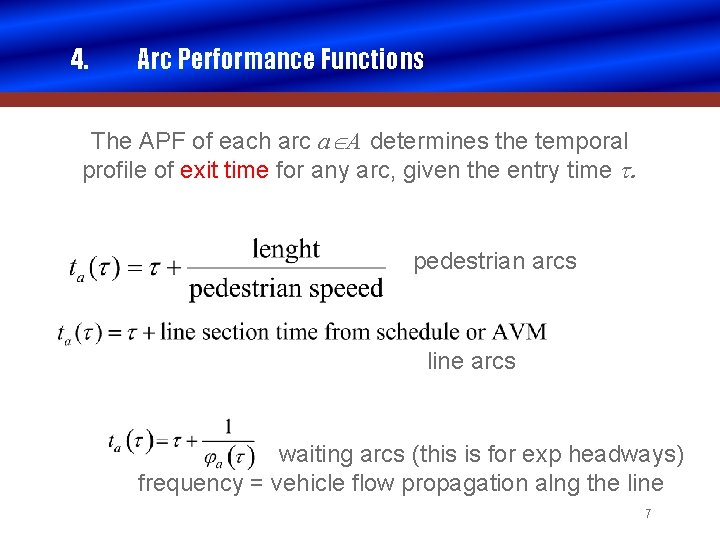
4. Arc Performance Functions The APF of each arc a A determines the temporal profile of exit time for any arc, given the entry time . pedestrian arcs line arcs waiting arcs (this is for exp headways) frequency = vehicle flow propagation alng the line 7
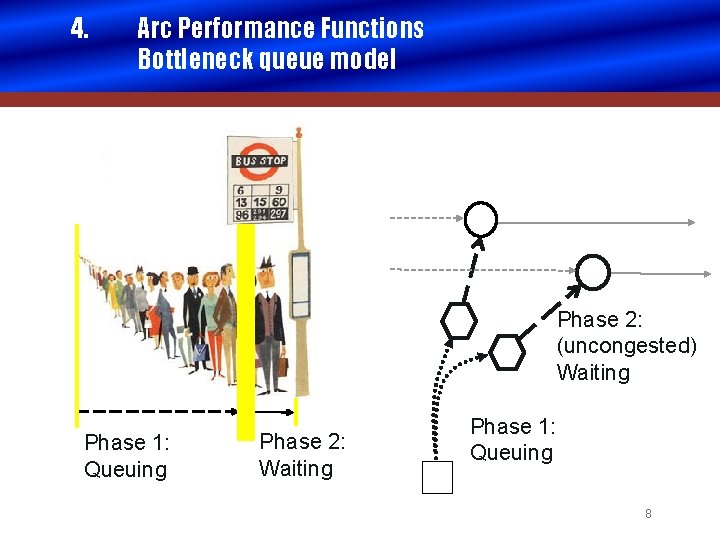
4. Arc Performance Functions Bottleneck queue model Phase 2: (uncongested) Waiting Phase 1: Queuing Phase 2: Waiting Phase 1: Queuing 8
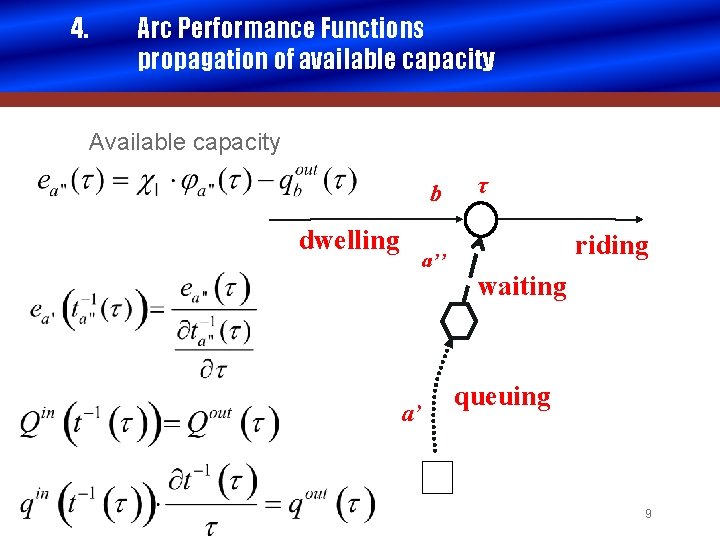
4. Arc Performance Functions propagation of available capacity Available capacity b dwelling a’’ a’ τ riding waiting queuing 9
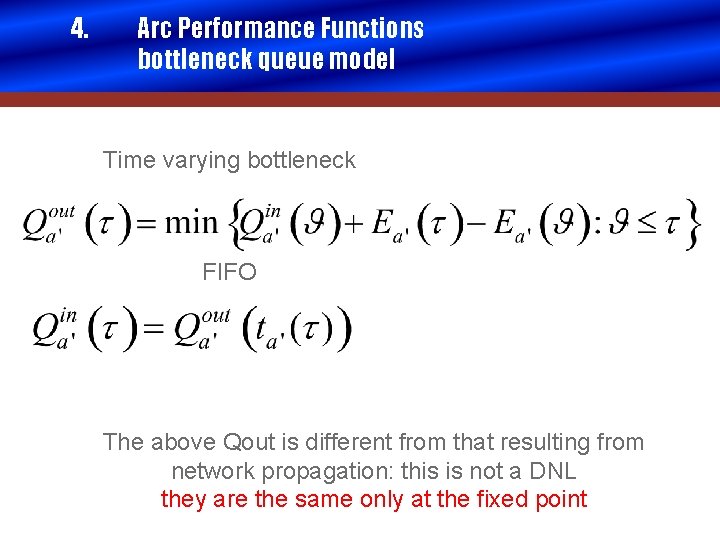
4. Arc Performance Functions bottleneck queue model Time varying bottleneck FIFO The above Qout is different from that resulting from network propagation: this is not a DNL they are the same only at the fixed point
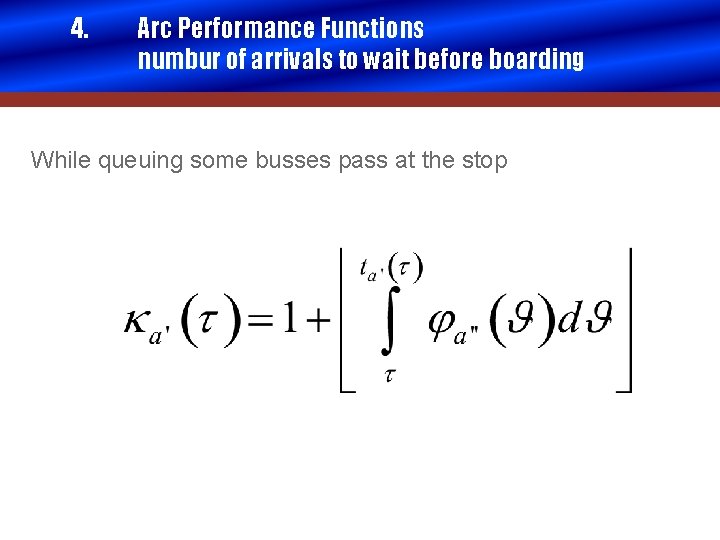
4. Arc Performance Functions numbur of arrivals to wait before boarding While queuing some busses pass at the stop
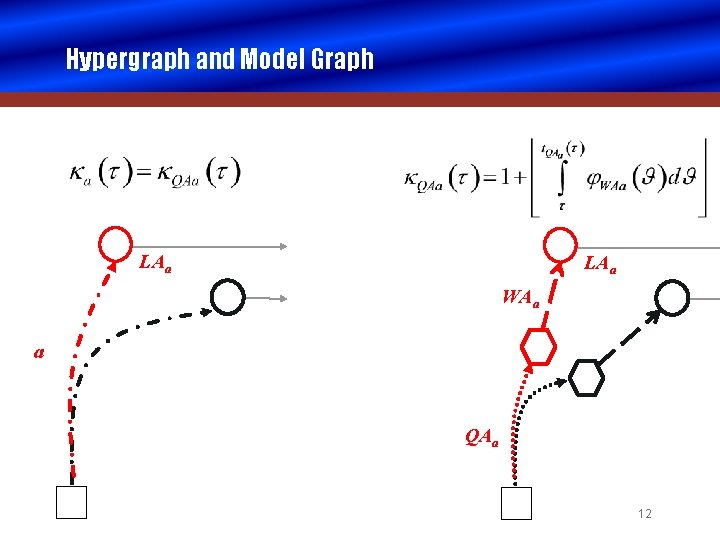
Hypergraph and Model Graph LAa WAa a QAa 12
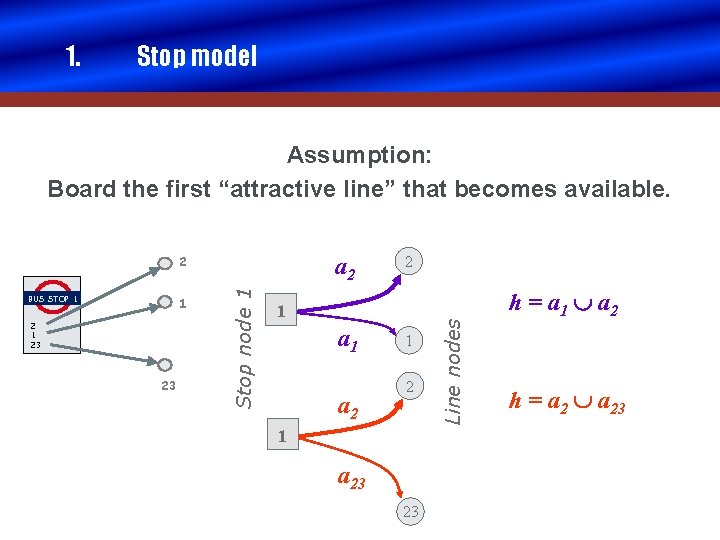
1. Stop model Assumption: Board the first “attractive line” that becomes available. 1 23 23 Stop node 1 BUS STOP 1 2 1 a 23 23 Line nodes a 2 2 h = a 1 a 2 h = a 23
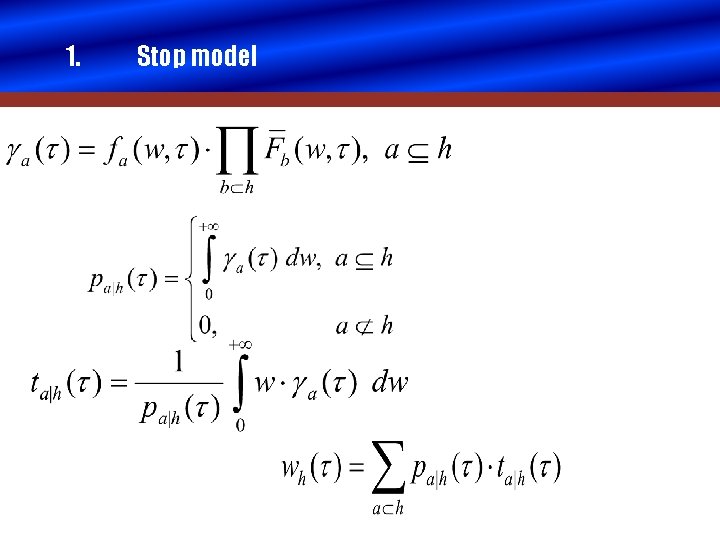
1. Stop model
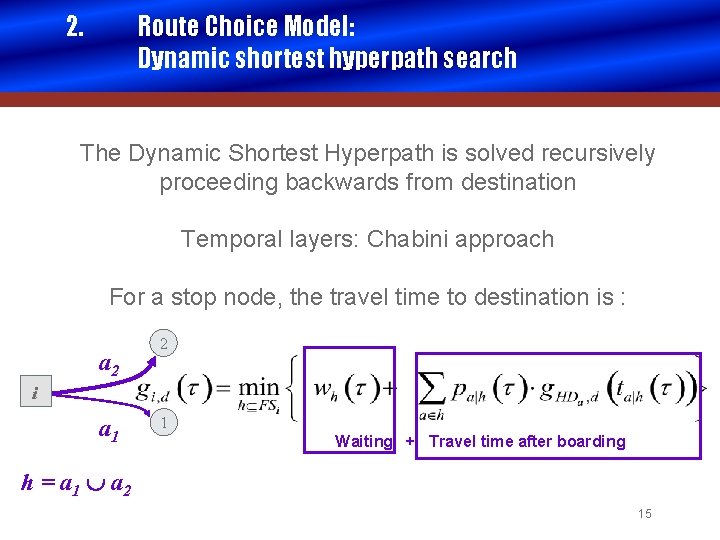
2. Route Choice Model: Dynamic shortest hyperpath search The Dynamic Shortest Hyperpath is solved recursively proceeding backwards from destination Temporal layers: Chabini approach For a stop node, the travel time to destination is : a 2 2 i a 1 1 Waiting + Travel time after boarding h = a 1 a 2 15
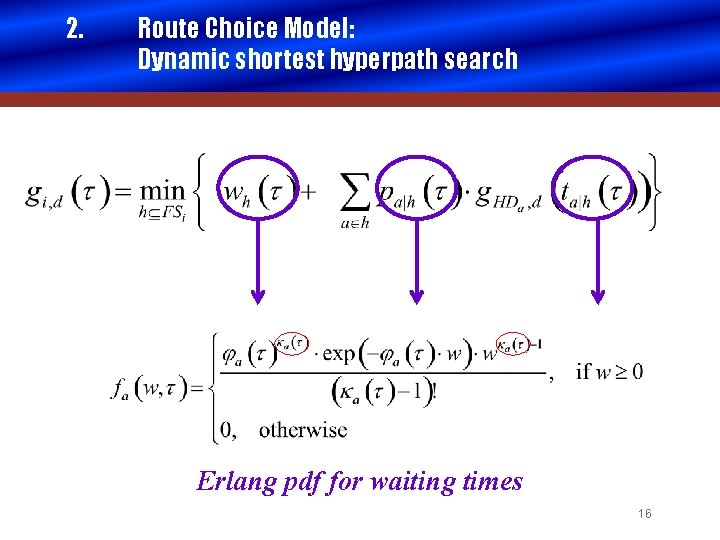
2. Route Choice Model: Dynamic shortest hyperpath search Erlang pdf for waiting times 16
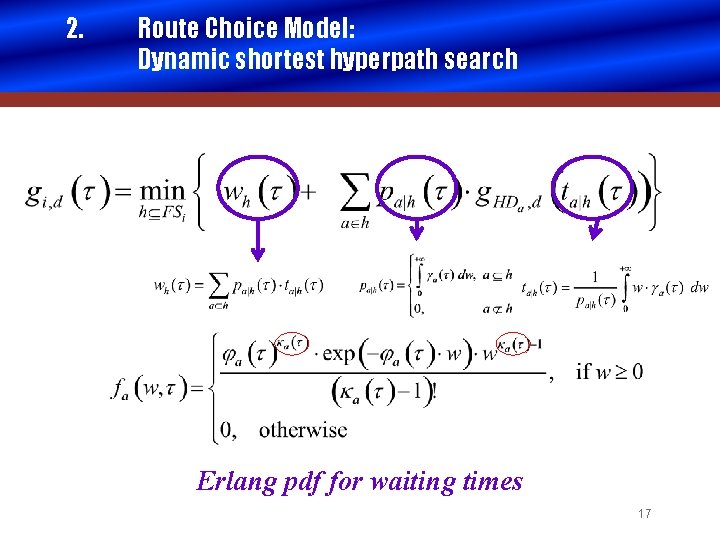
2. Route Choice Model: Dynamic shortest hyperpath search Erlang pdf for waiting times 17
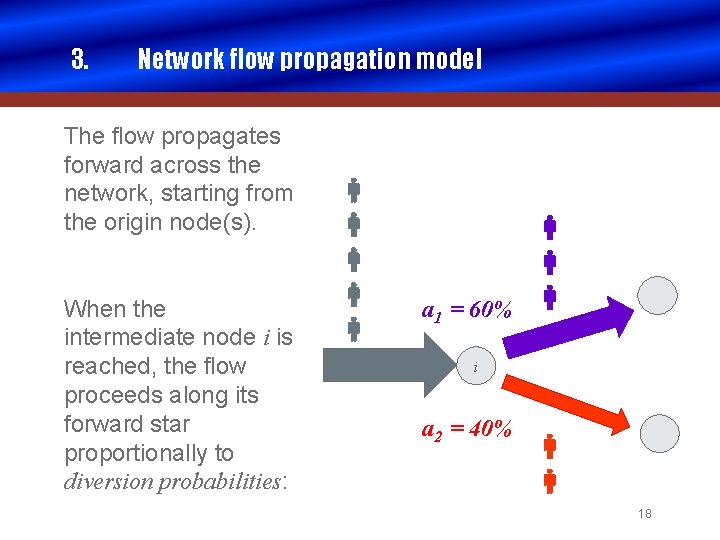
3. Network flow propagation model The flow propagates forward across the network, starting from the origin node(s). When the intermediate node i is reached, the flow proceeds along its forward star proportionally to diversion probabilities: a 1 = 60% i a 2 = 40% 18
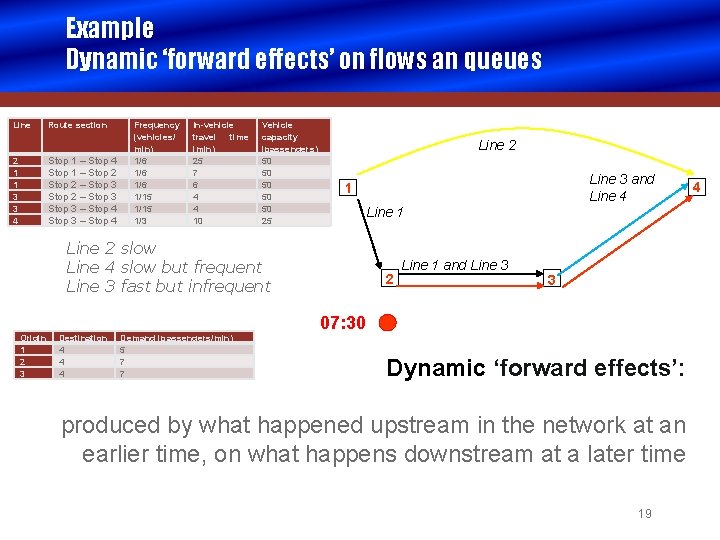
Example Dynamic ‘forward effects’ on flows an queues Route section 2 1 1 3 3 4 Stop 1 – Stop 2 – Stop 3 – Stop 4 Frequency (vehicles/ min) 1/6 1/6 1/15 1/3 In-vehicle travel time (min) 25 7 6 4 4 10 Vehicle capacity (passengers) 50 50 50 25 07: 30 Line 3 and Line 4 1 Line 2 slow Line 4 slow but frequent Line 3 fast but infrequent Origin 1 2 3 Destination 4 4 4 Demand (passengers/min) 5 7 7 Line 2 Line 1 and Line 3 3 07: 30 Dynamic ‘forward effects’: produced by what happened upstream in the network at an earlier time, on what happens downstream at a later time 19 4
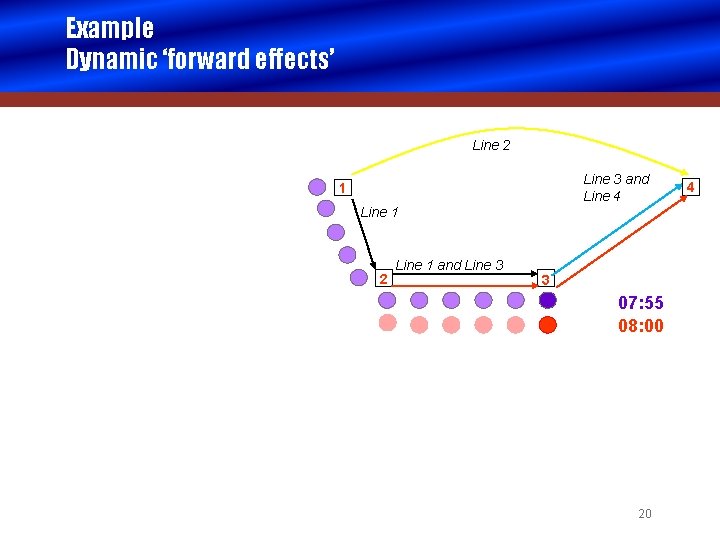
Example Dynamic ‘forward effects’ Line 2 Line 3 and Line 4 1 Line 1 2 Line 1 and Line 3 3 07: 55 08: 00 20 4
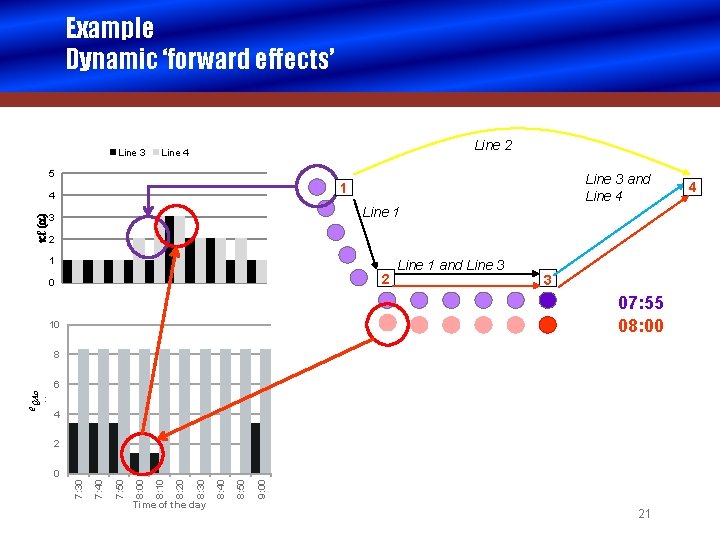
Example Dynamic ‘forward effects’ Line 3 Line 2 Line 4 5 kℓ (a) 4 Line 3 and Line 4 1 Line 1 3 2 1 2 0 Line 1 and Line 3 3 07: 55 08: 00 10 8 x e. QAa 6 4 Time of the day 9: 00 8: 50 8: 40 8: 30 8: 20 8: 10 8: 00 7: 50 7: 40 0 7: 30 2 21 4
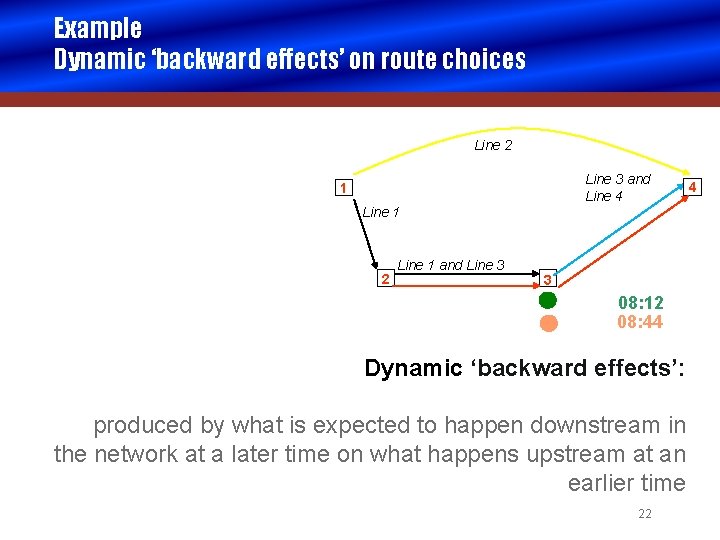
Example Dynamic ‘backward effects’ on route choices Line 2 Line 3 and Line 4 1 Line 1 2 Line 1 and Line 3 3 08: 12 08: 44 Dynamic ‘backward effects’: produced by what is expected to happen downstream in the network at a later time on what happens upstream at an earlier time 22 4
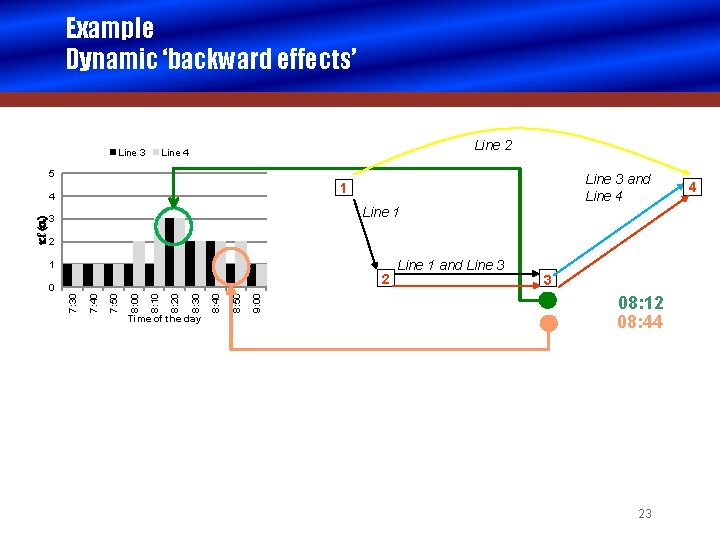
Example Dynamic ‘backward effects’ Line 3 Line 2 Line 4 5 Line 1 3 2 1 Time of the day 9: 00 8: 50 8: 40 8: 30 8: 20 8: 10 8: 00 7: 50 7: 40 0 2 7: 30 kℓ (a) 4 Line 3 and Line 4 1 Line 1 and Line 3 3 08: 12 08: 44 23 4
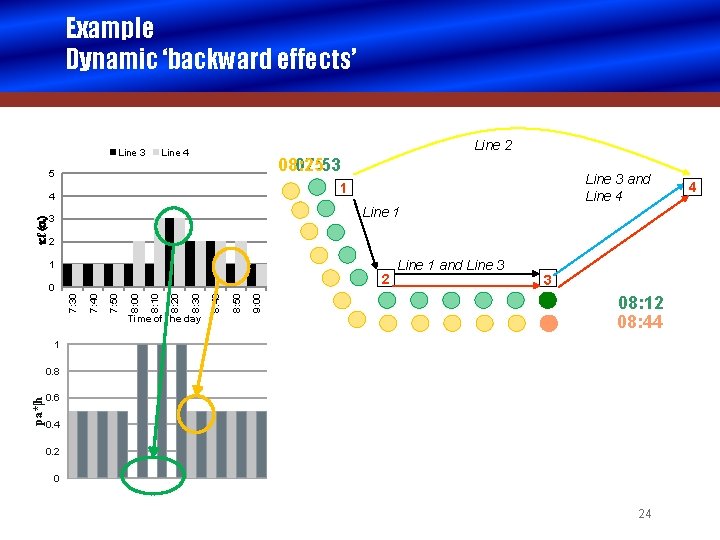
Example Dynamic ‘backward effects’ Line 3 Line 4 07: 53 08: 25 5 kℓ (a) 4 Line 2 Line 3 and Line 4 1 Line 1 3 2 1 Time of the day 9: 00 8: 50 8: 40 8: 30 8: 20 8: 10 8: 00 7: 50 7: 40 7: 30 0 2 Line 1 and Line 3 3 08: 12 08: 44 1 pa*|h 0. 8 0. 6 0. 4 0. 2 0 24 4
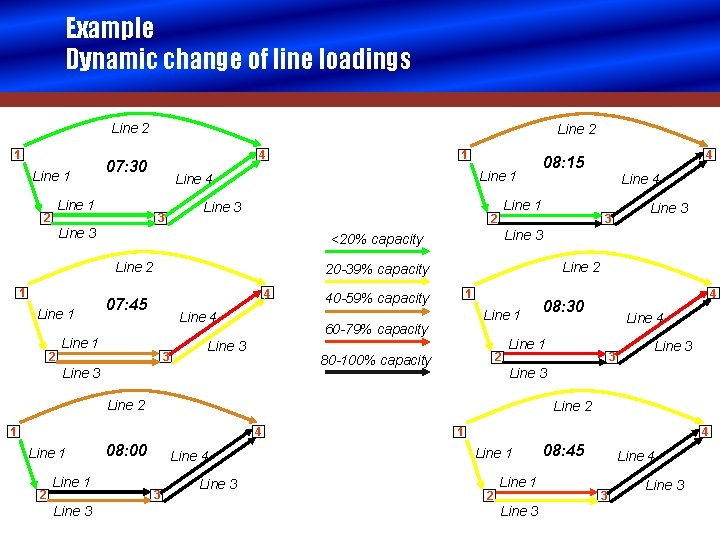
Example Dynamic change of line loadings Line 2 Line 1 07: 30 Line 1 2 4 Line 1 Line 3 Line 1 2 20 -39% capacity 4 07: 45 Line 4 Line 1 3 40 -59% capacity Line 1 2 80 -100% capacity Line 3 Line 1 2 Line 1 Line 3 08: 00 Line 4 3 Line 3 4 08: 30 Line 4 Line 1 Line 3 3 Line 2 1 4 Line 3 Line 2 1 60 -79% capacity Line 3 3 1 Line 4 Line 3 <20% capacity Line 2 4 08: 15 Line 1 2 Line 3 1 Line 4 3 1 Line 2 1 4 Line 1 2 Line 1 Line 3 08: 45 Line 4 3 Line 3 25
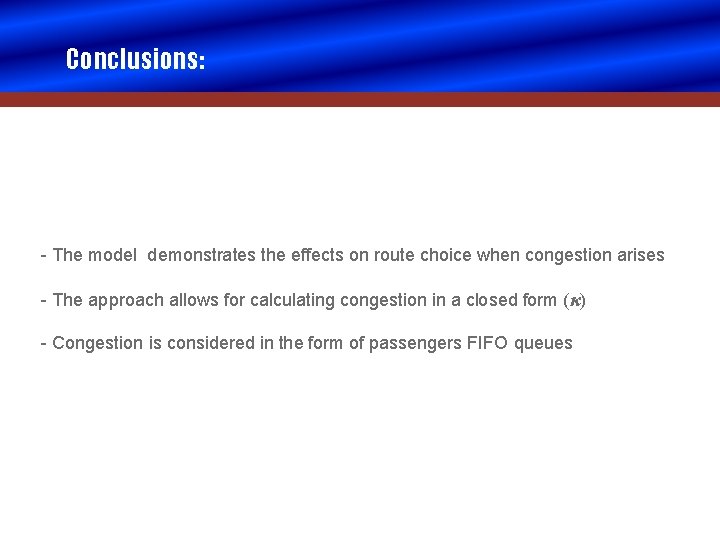
Conclusions: - The model demonstrates the effects on route choice when congestion arises - The approach allows for calculating congestion in a closed form (κ) - Congestion is considered in the form of passengers FIFO queues
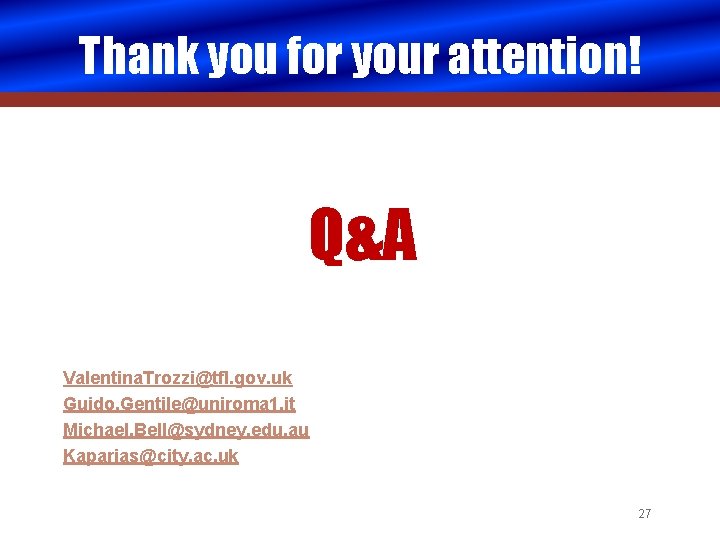
Thank you for your attention! Q&A Dynamic User Equilibrium in Public Transport Networks with Passenger Congestion and Hyperpaths Thank you for your attention Valentina. Trozzi@tfl. gov. uk Guido. Gentile@uniroma 1. it Michael. Bell@sydney. edu. au Kaparias@city. ac. uk 27
Universit of london
Imperial college london parking
Imperial college london
Nanterre universit
Universit sherbrooke
Universit
Rotterdam school of economics
Imperial college blackboard
Imperial college
University college london
Kaufmann international london
"union place"
West london recovery college
"kings college london"
"nordic innovation"
Kings college maths school
Claudio luci sapienza
Interactive graphics sapienza
Vincenzo patera sapienza
Edificio fermi sapienza
Bixy sapienza
Emma galli sapienza
Fiorenzo parziale
Stefano lupi sapienza
Uweb sapienza
Paolo palange sapienza
Economia del turismo sapienza
Sapienza dono dello spirito santo