Dynamic Causal Model for Steady State Responses Rosalyn
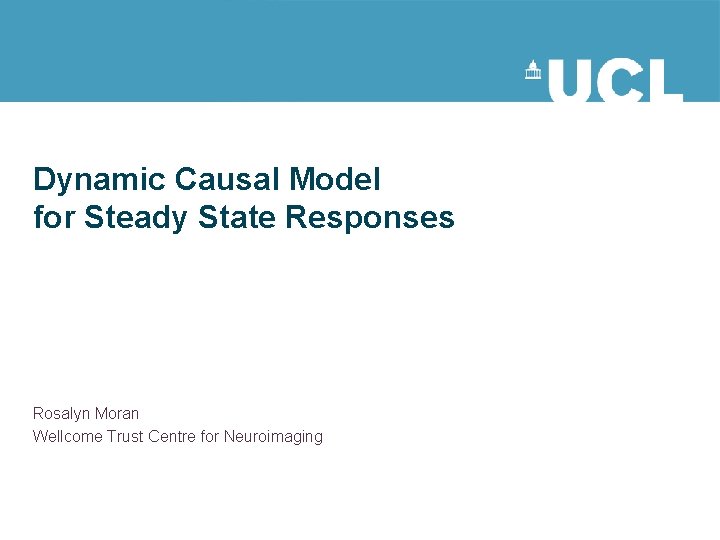
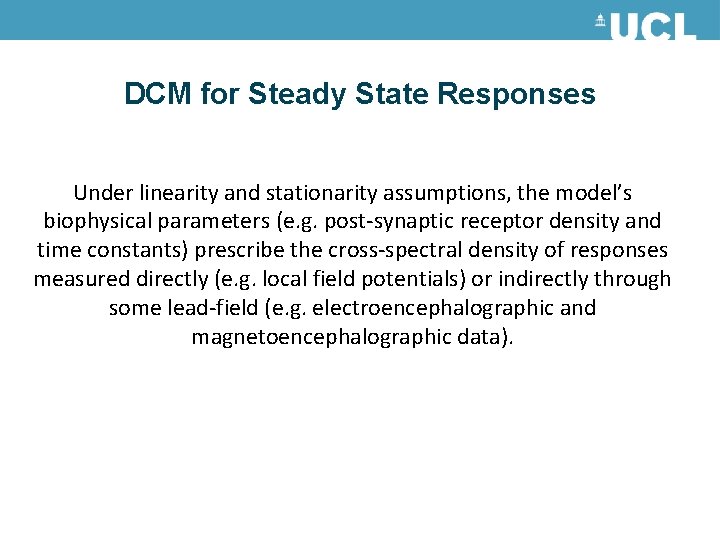
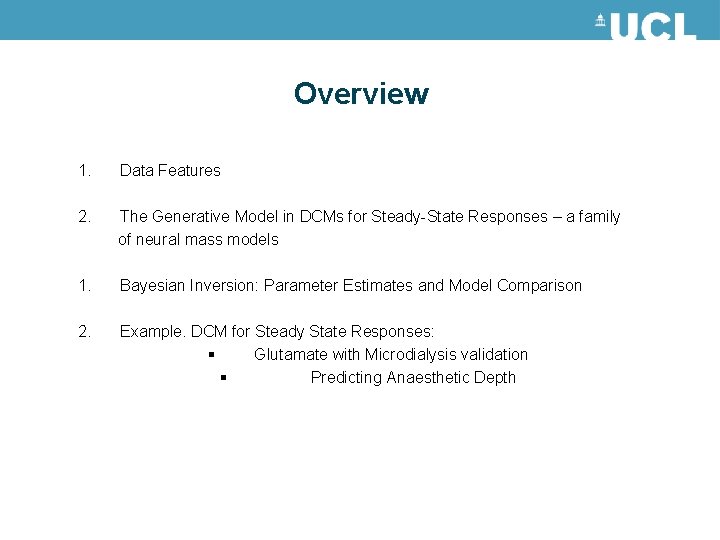
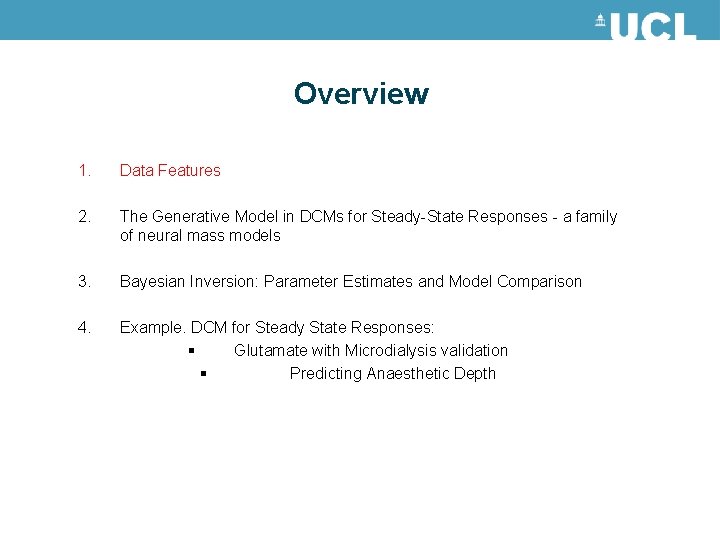
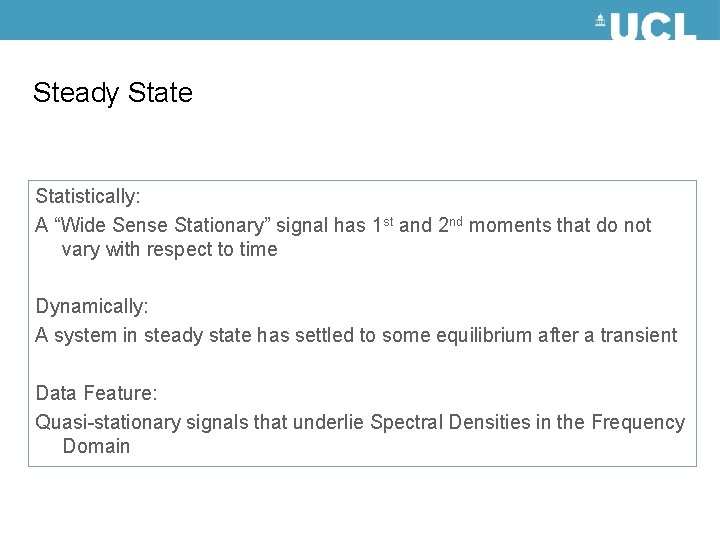
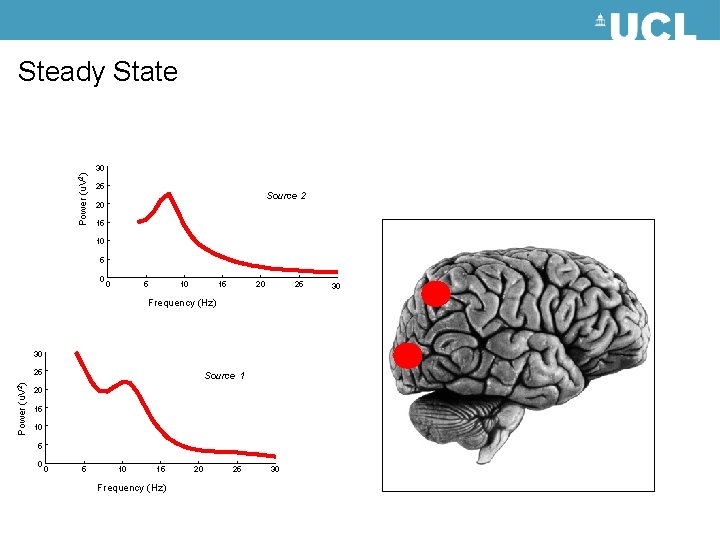
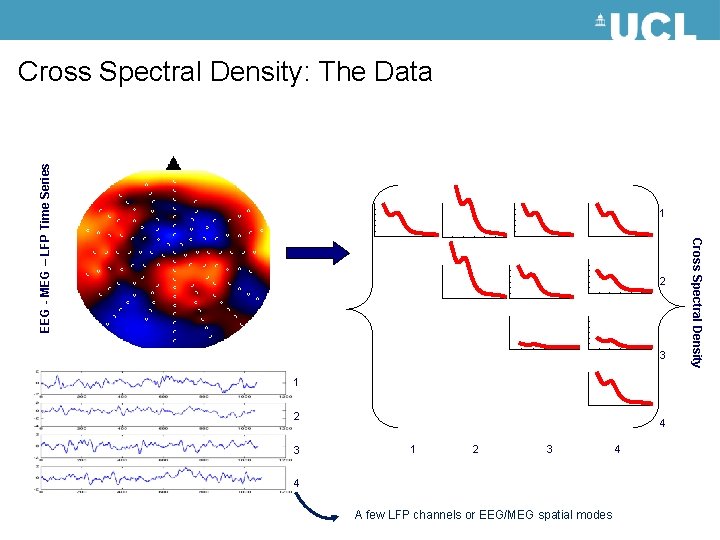
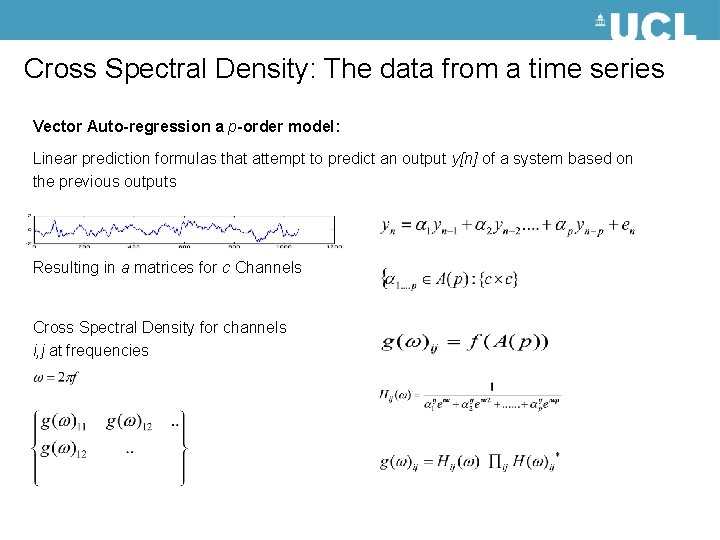
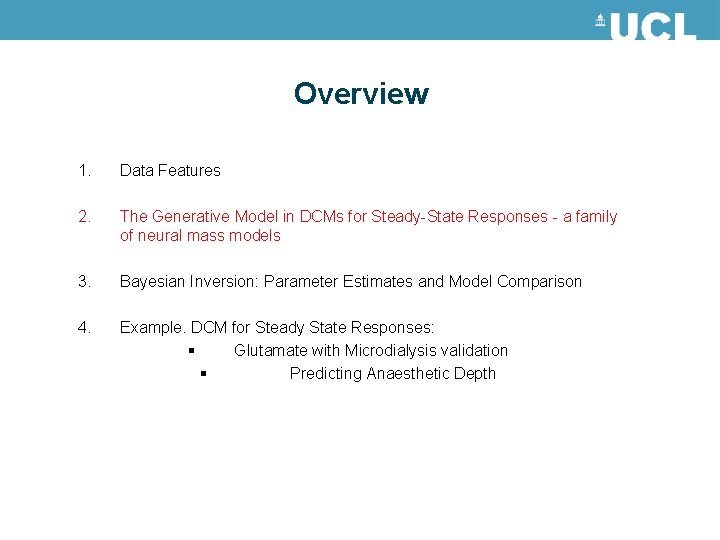
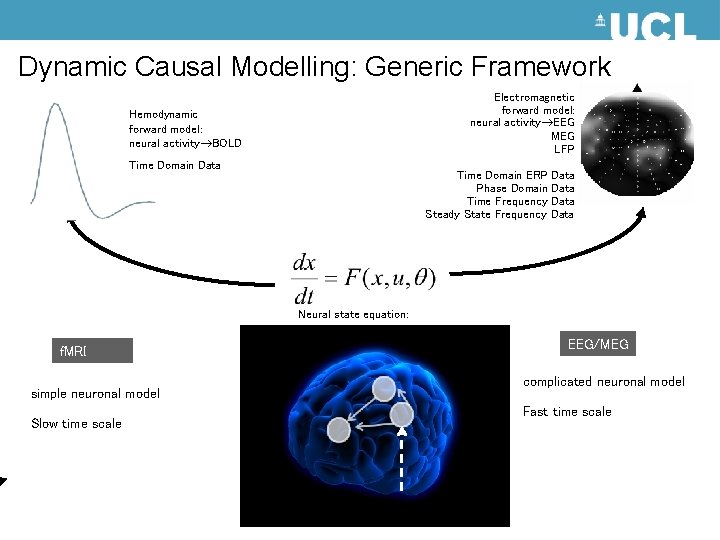
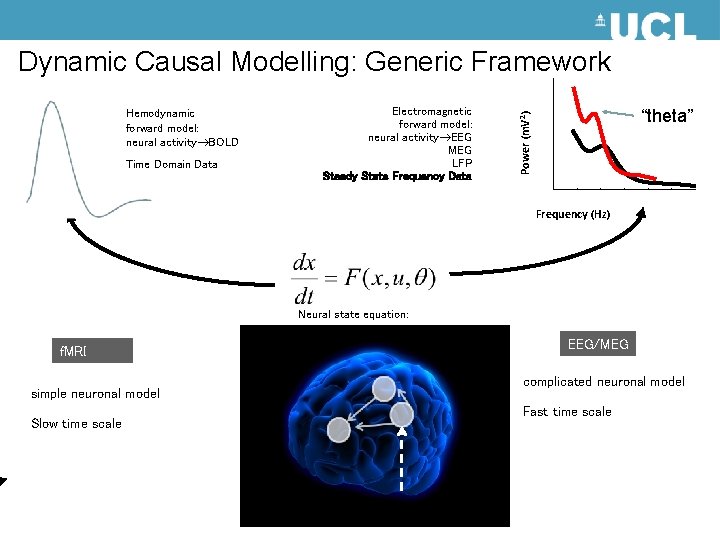
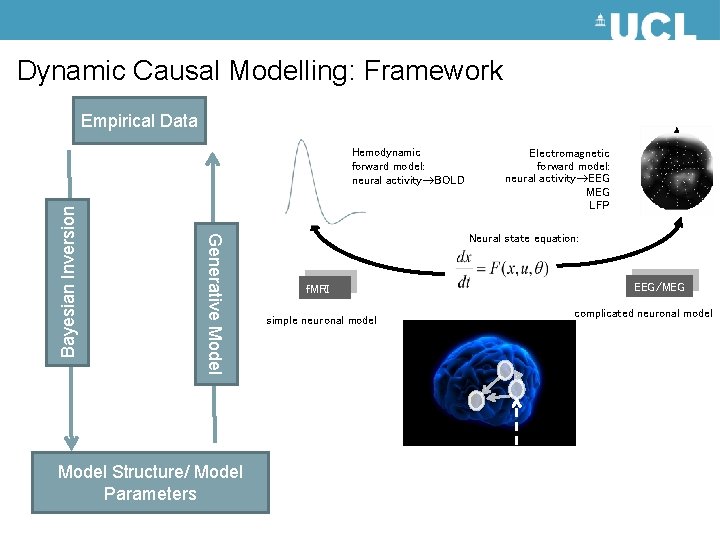
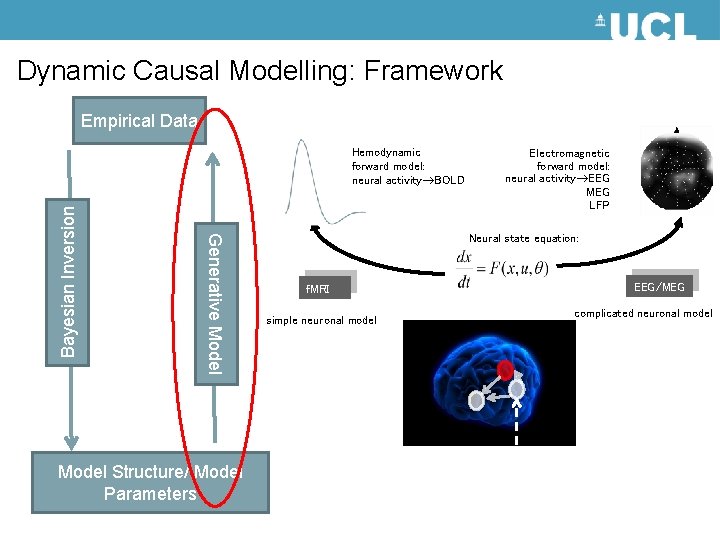
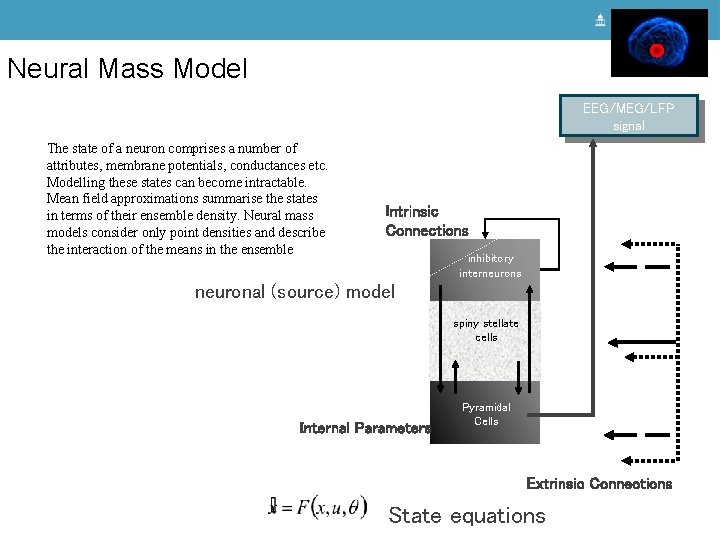
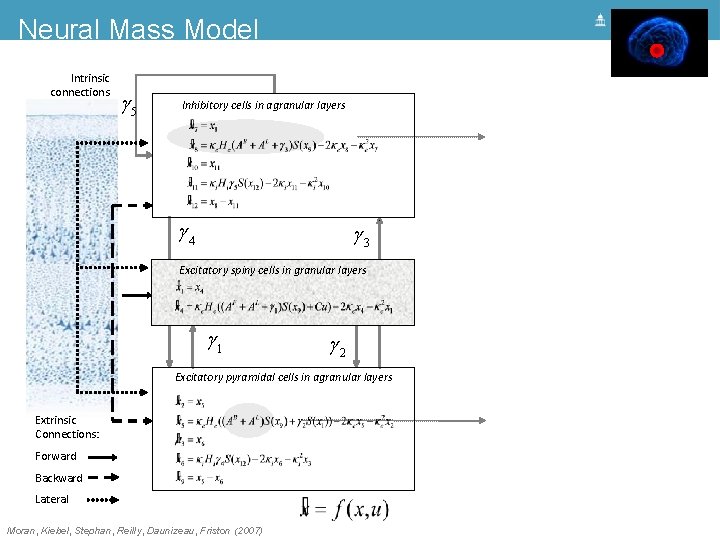
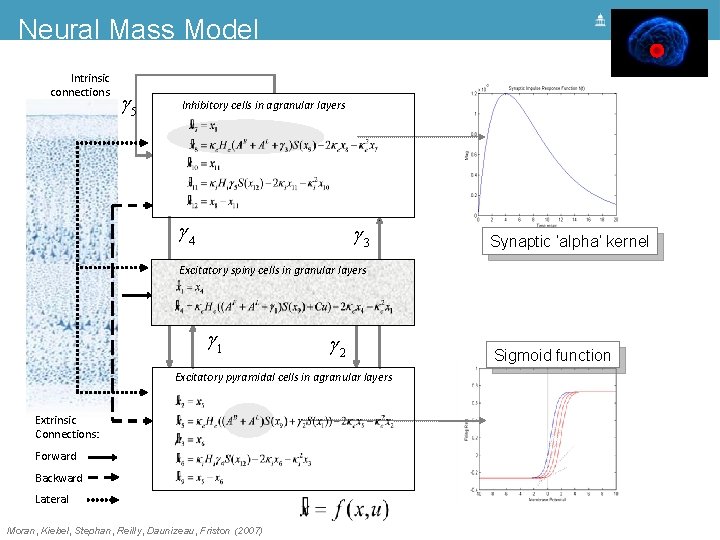
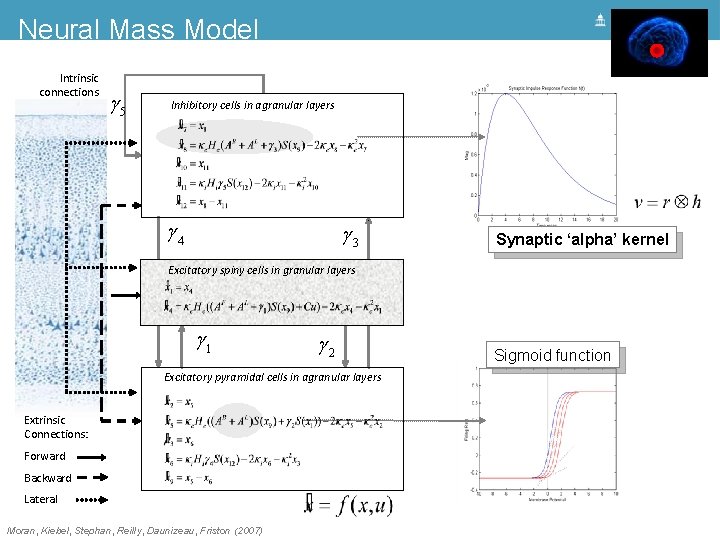
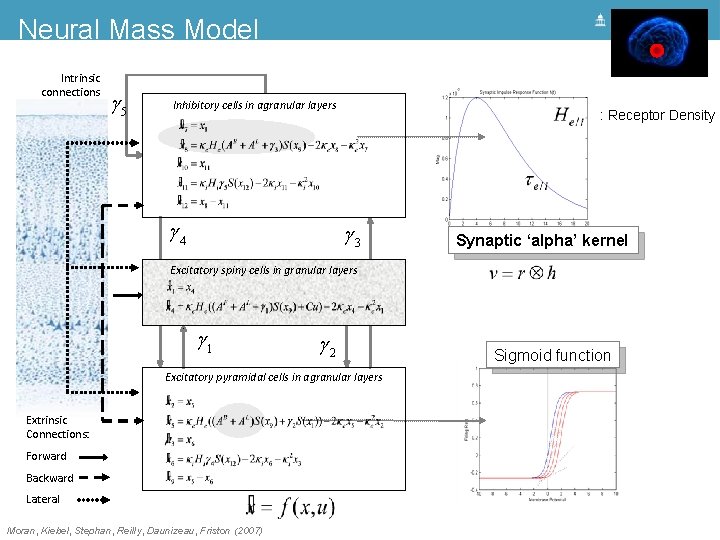
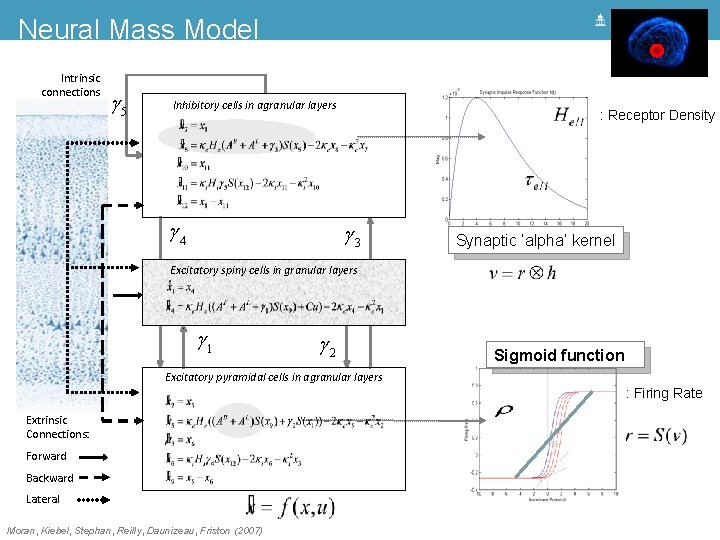
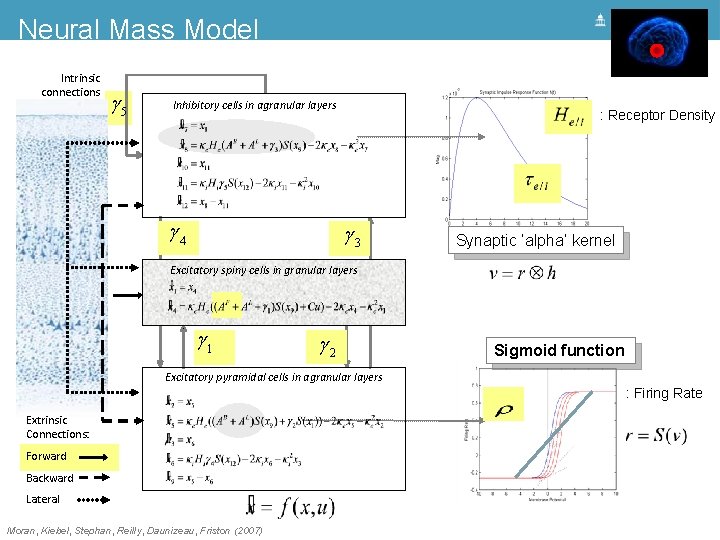
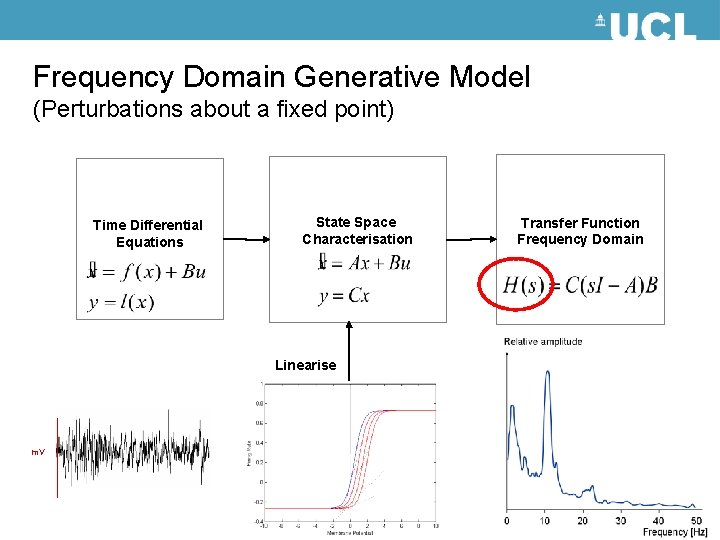
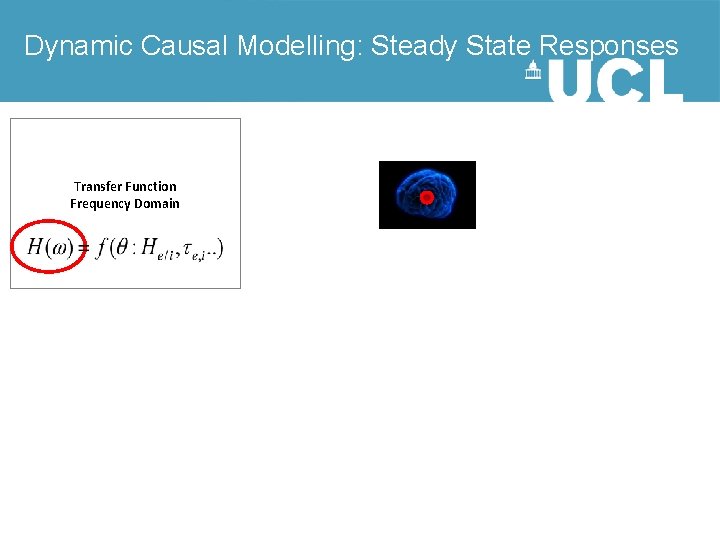
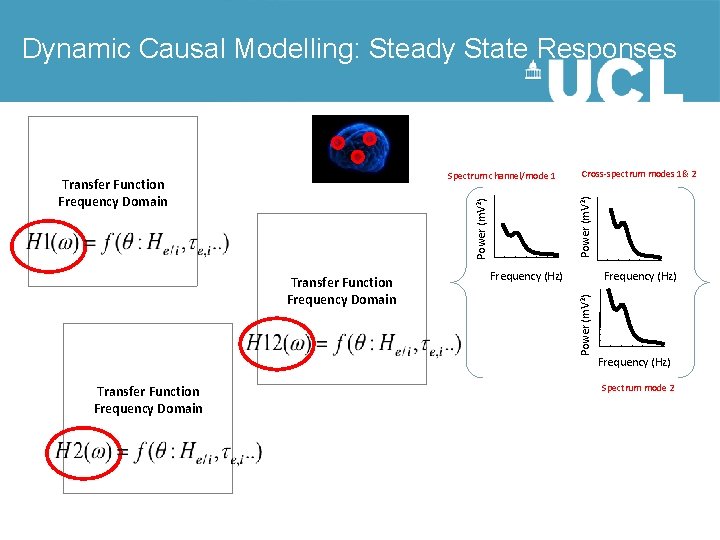
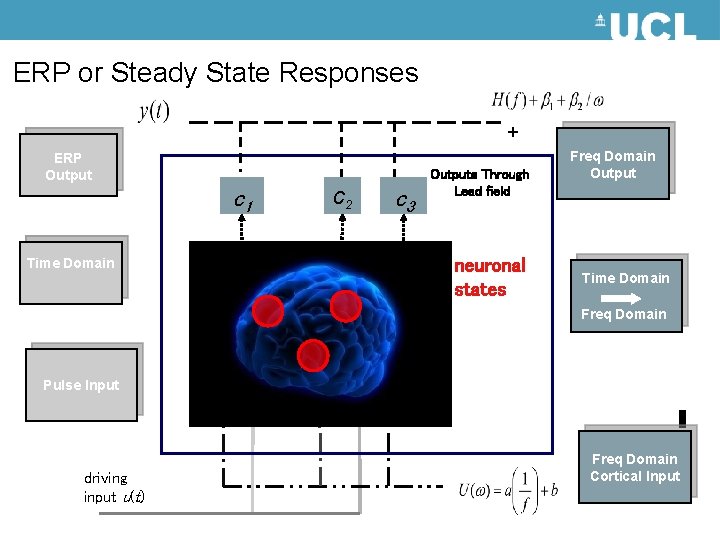
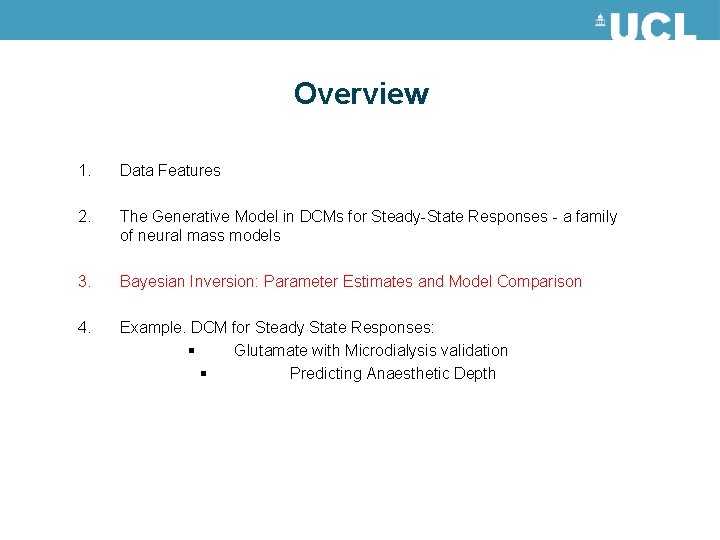
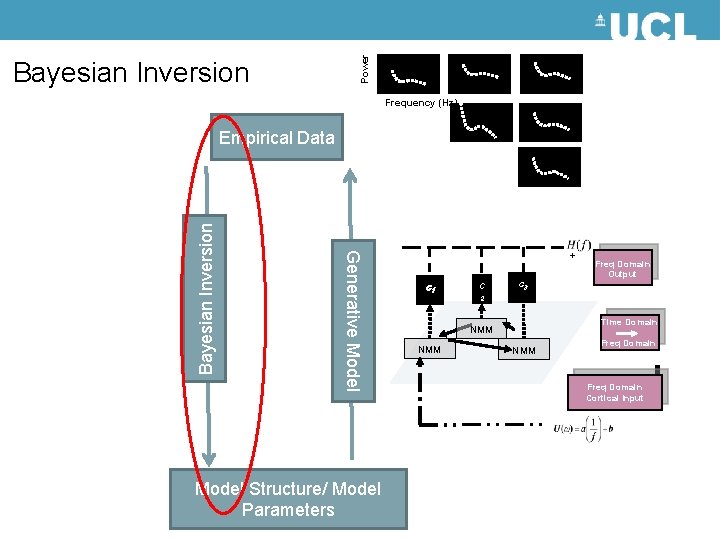
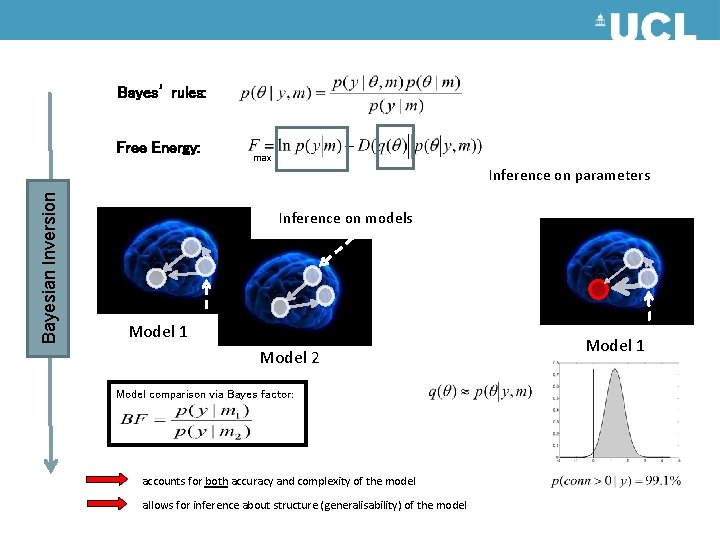
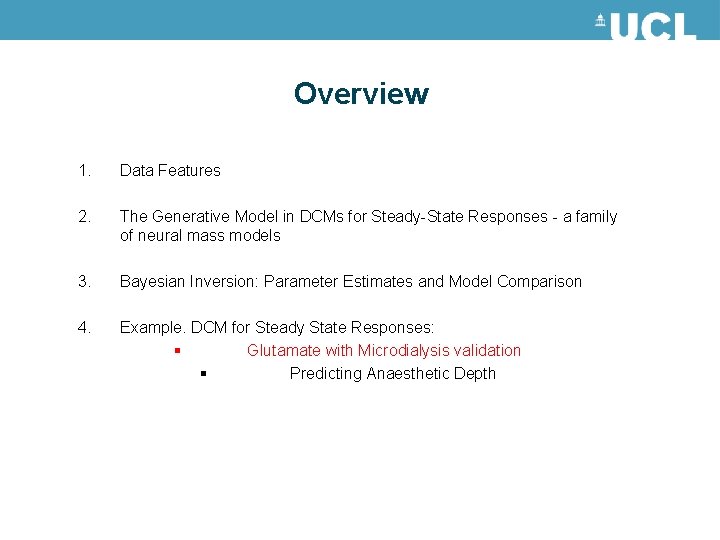
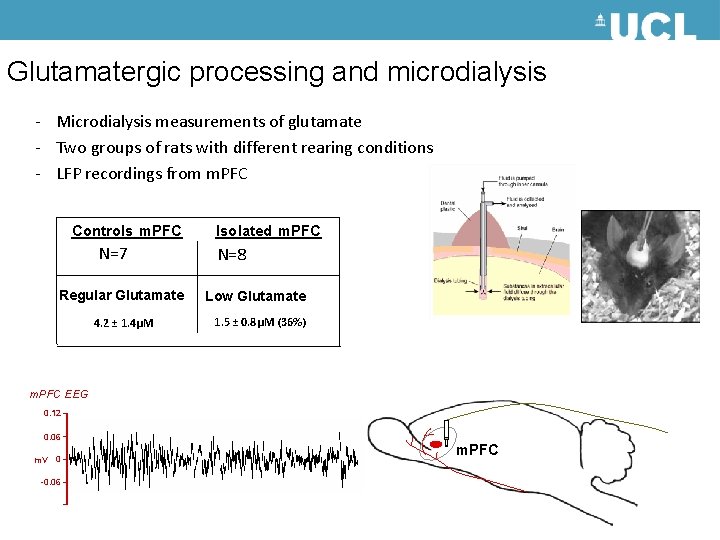
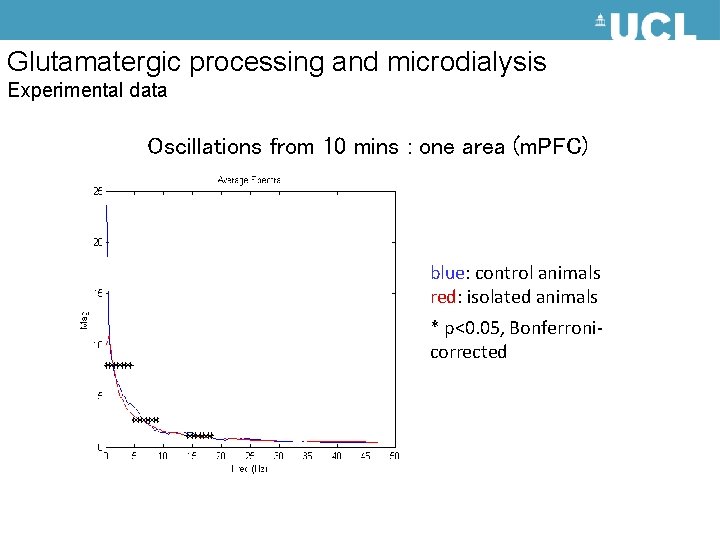
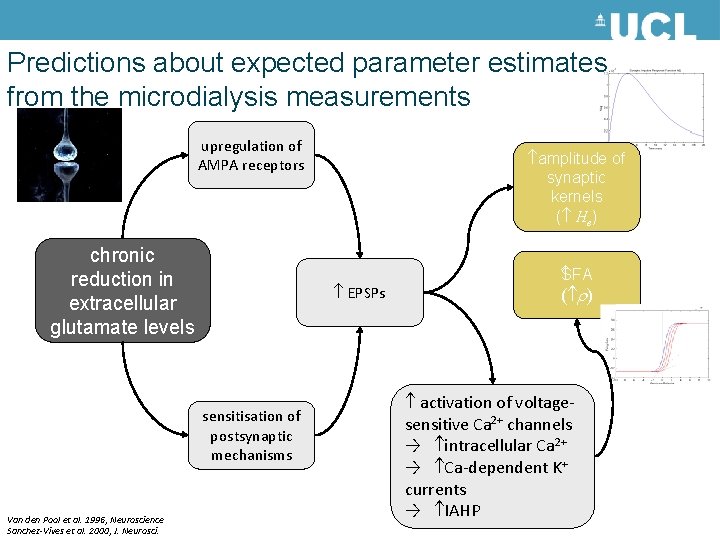
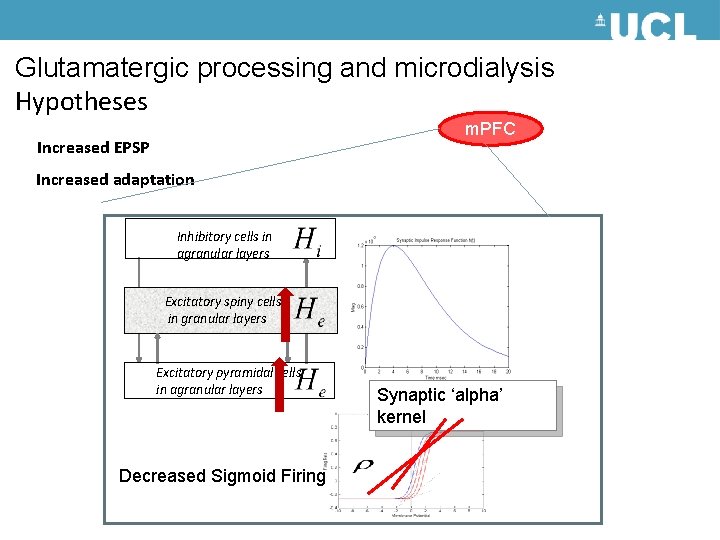
![Glutamatergic processing and microdialysis Results g 5 [3. 8 6. 3] Inhibitory cells in Glutamatergic processing and microdialysis Results g 5 [3. 8 6. 3] Inhibitory cells in](https://slidetodoc.com/presentation_image_h2/6d855fa7306b96ec4c3a09aeaedae772/image-33.jpg)
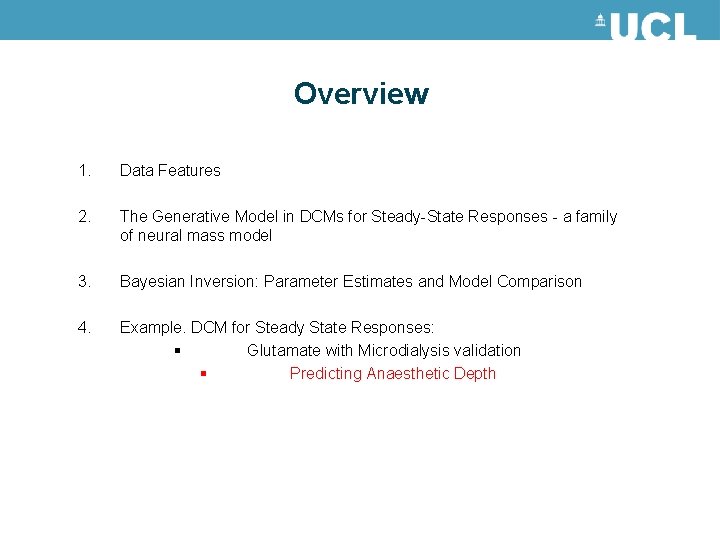
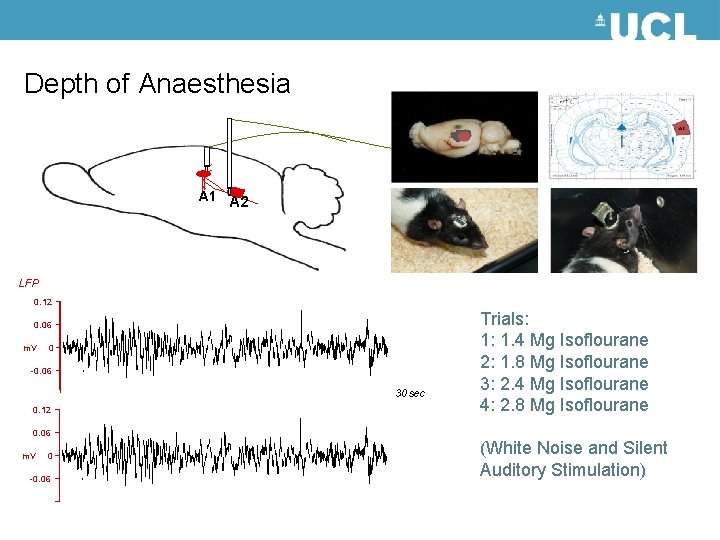
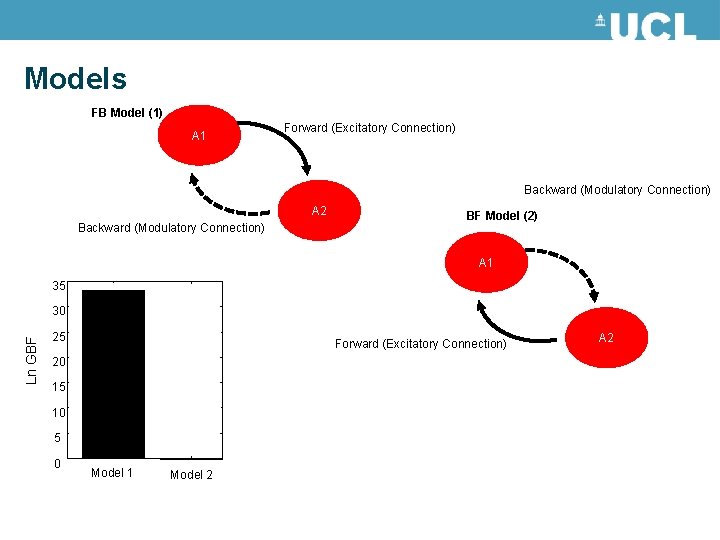
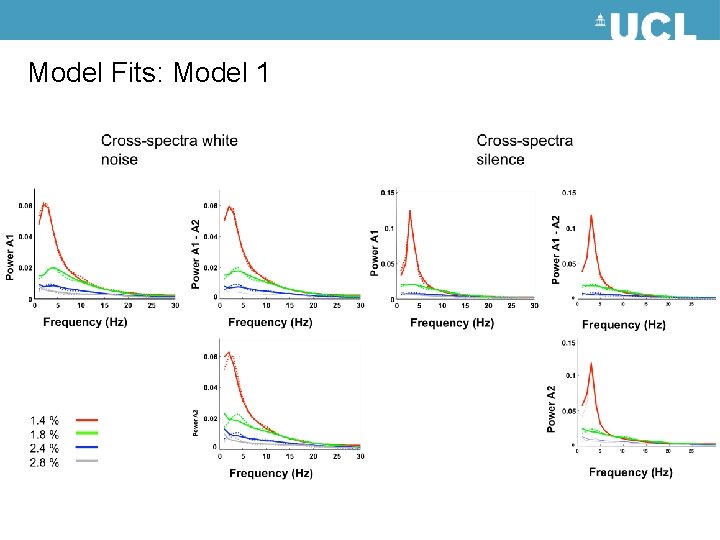
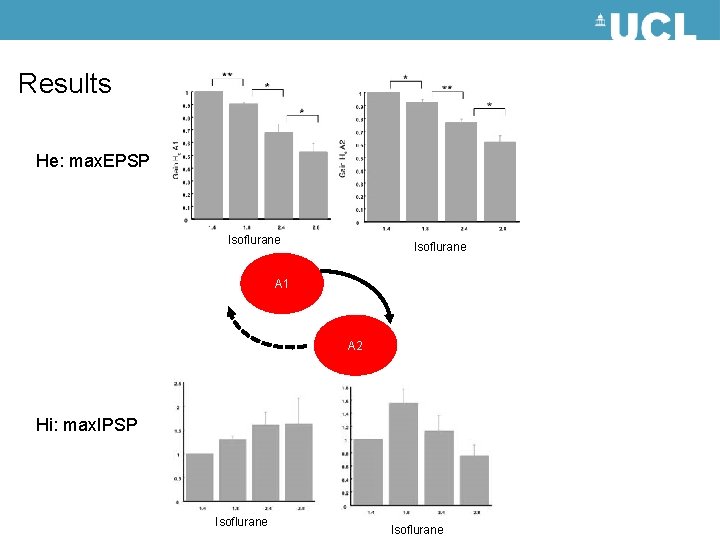
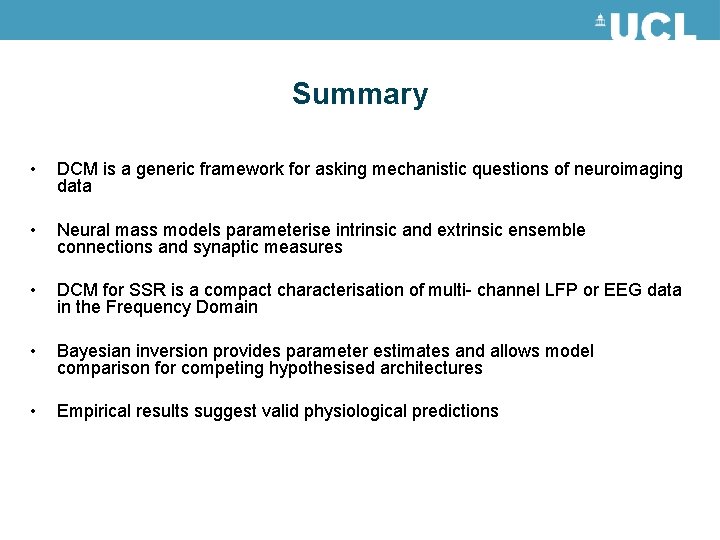
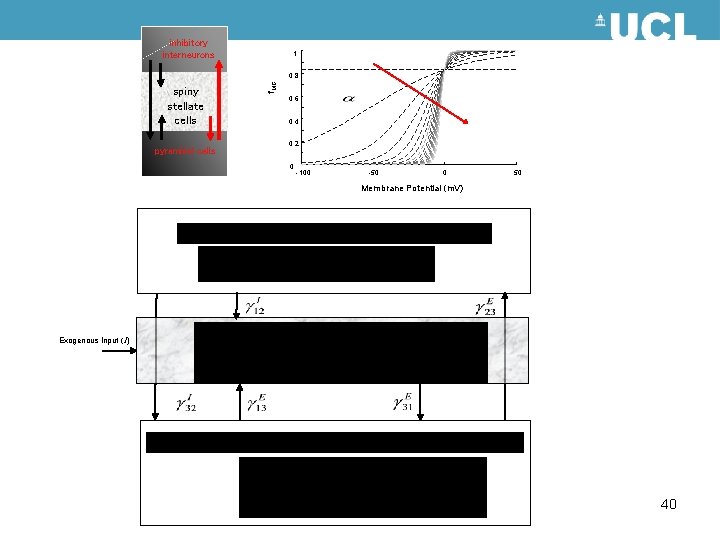
- Slides: 40
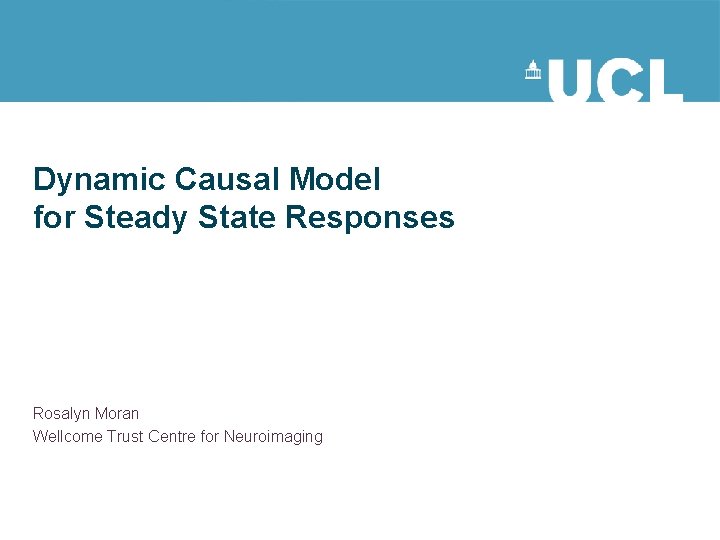
Dynamic Causal Model for Steady State Responses Rosalyn Moran Wellcome Trust Centre for Neuroimaging
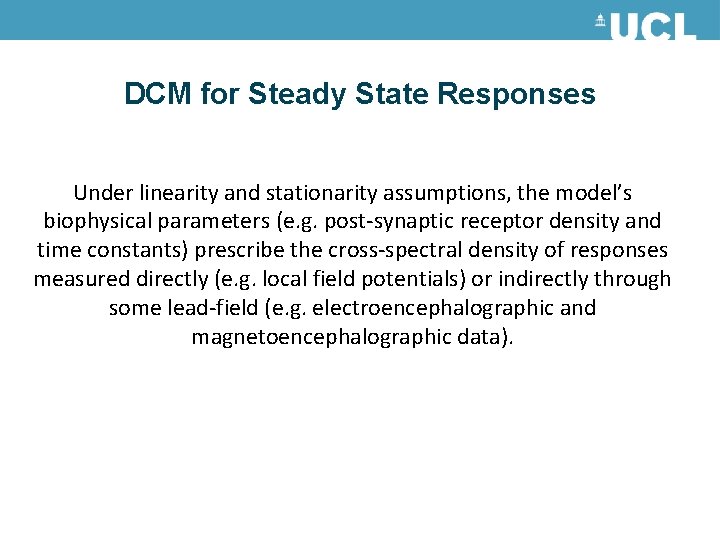
DCM for Steady State Responses Under linearity and stationarity assumptions, the model’s biophysical parameters (e. g. post-synaptic receptor density and time constants) prescribe the cross-spectral density of responses measured directly (e. g. local field potentials) or indirectly through some lead-field (e. g. electroencephalographic and magnetoencephalographic data).
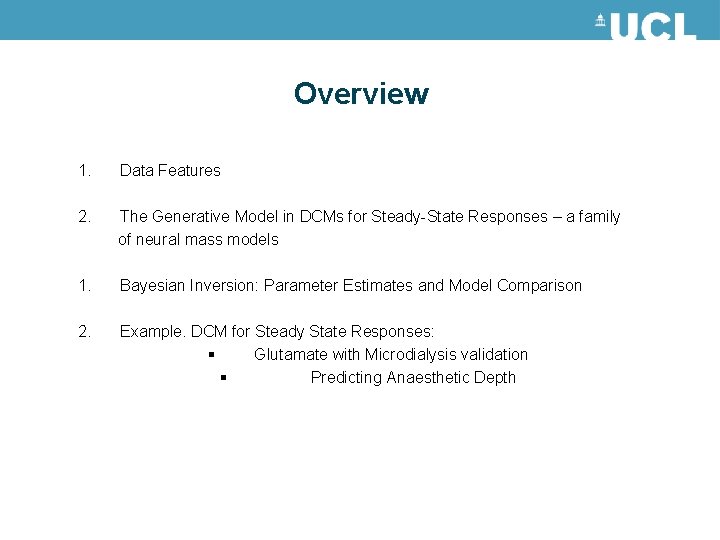
Overview 1. Data Features 2. The Generative Model in DCMs for Steady-State Responses – a family of neural mass models 1. Bayesian Inversion: Parameter Estimates and Model Comparison 2. Example. DCM for Steady State Responses: § Glutamate with Microdialysis validation § Predicting Anaesthetic Depth
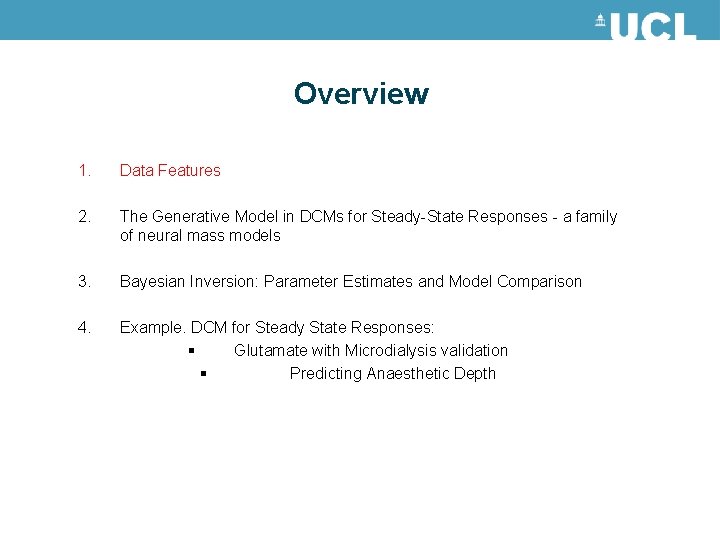
Overview 1. Data Features 2. The Generative Model in DCMs for Steady-State Responses - a family of neural mass models 3. Bayesian Inversion: Parameter Estimates and Model Comparison 4. Example. DCM for Steady State Responses: § Glutamate with Microdialysis validation § Predicting Anaesthetic Depth
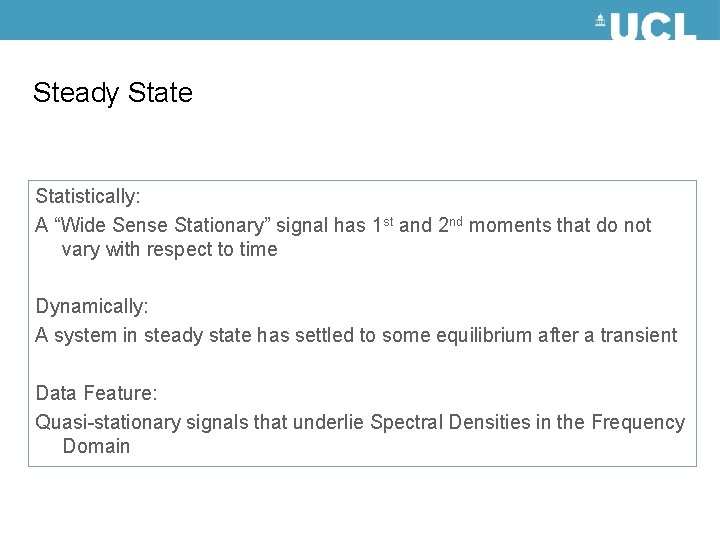
Steady State Statistically: A “Wide Sense Stationary” signal has 1 st and 2 nd moments that do not vary with respect to time Dynamically: A system in steady state has settled to some equilibrium after a transient Data Feature: Quasi-stationary signals that underlie Spectral Densities in the Frequency Domain
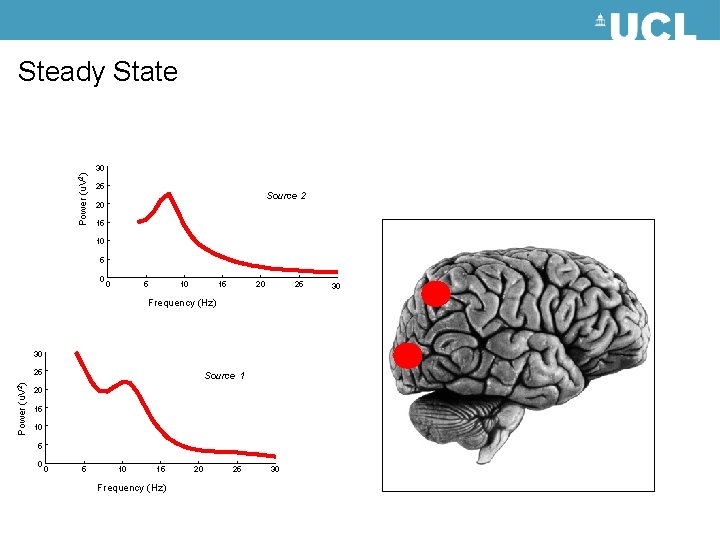
Steady State Power (u. V 2) 30 25 Source 2 20 15 10 5 0 0 5 10 15 20 25 Frequency (Hz) 30 Power (u. V 2) 25 Source 1 20 15 10 5 0 0 5 10 15 Frequency (Hz) 20 25 30 30
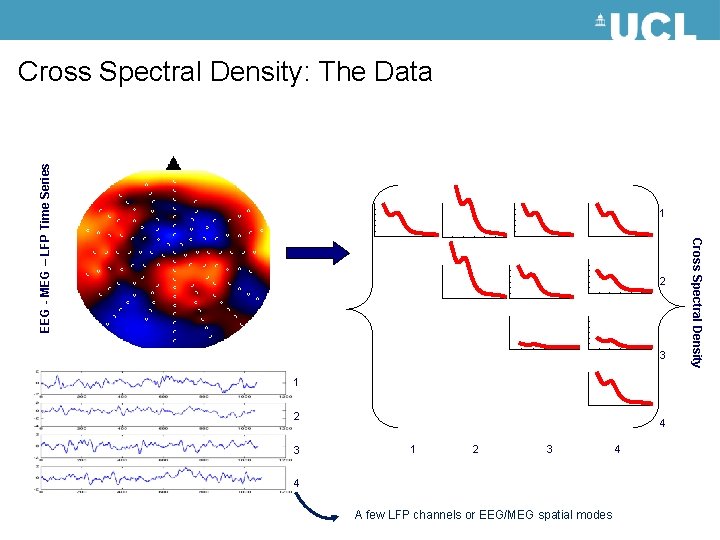
EEG - MEG – LFP Time Series Cross Spectral Density: The Data 1 3 1 2 3 4 A few LFP channels or EEG/MEG spatial modes 4 Cross Spectral Density 2
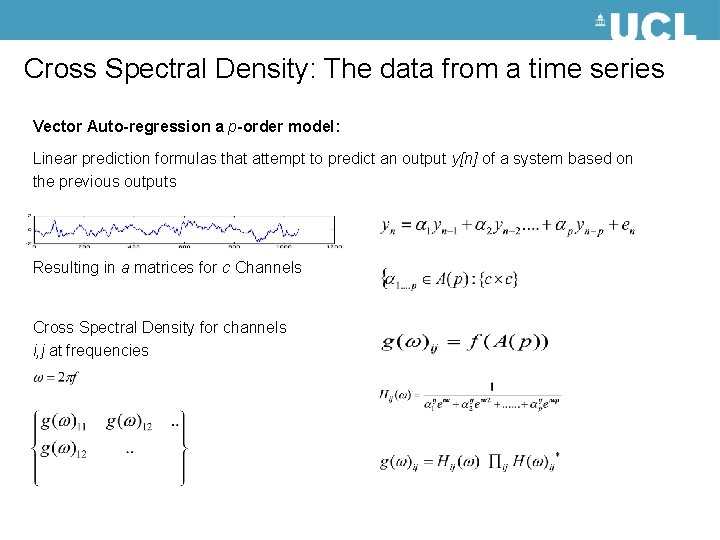
Cross Spectral Density: The data from a time series Vector Auto-regression a p-order model: Linear prediction formulas that attempt to predict an output y[n] of a system based on the previous outputs Resulting in a matrices for c Channels Cross Spectral Density for channels i, j at frequencies
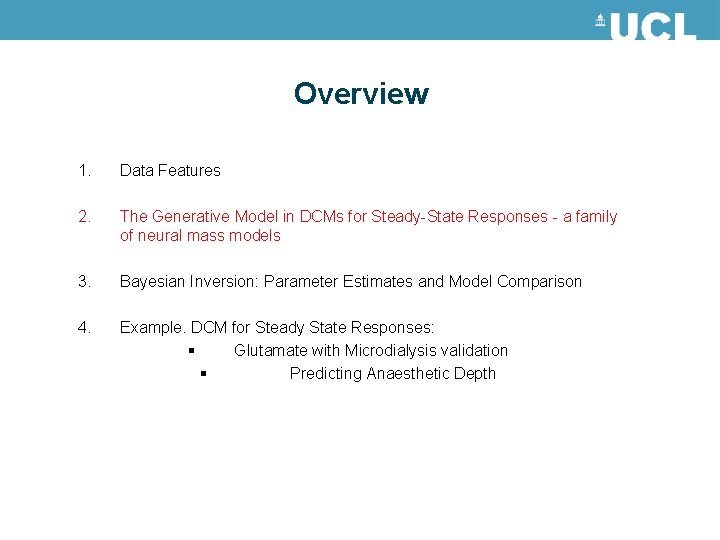
Overview 1. Data Features 2. The Generative Model in DCMs for Steady-State Responses - a family of neural mass models 3. Bayesian Inversion: Parameter Estimates and Model Comparison 4. Example. DCM for Steady State Responses: § Glutamate with Microdialysis validation § Predicting Anaesthetic Depth
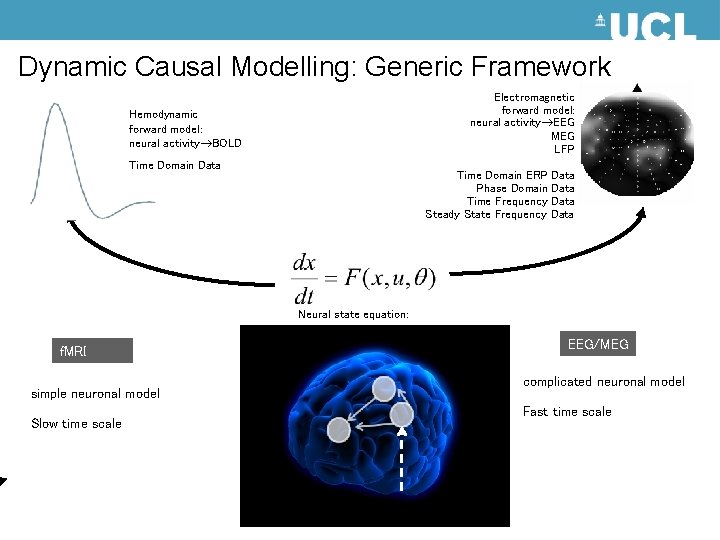
Dynamic Causal Modelling: Generic Framework Electromagnetic forward model: neural activity EEG MEG LFP Hemodynamic forward model: neural activity BOLD Time Domain Data Time Domain ERP Data Phase Domain Data Time Frequency Data Steady State Frequency Data Neural state equation: f. MRI simple neuronal model Slow time scale EEG/MEG complicated neuronal model Fast time scale
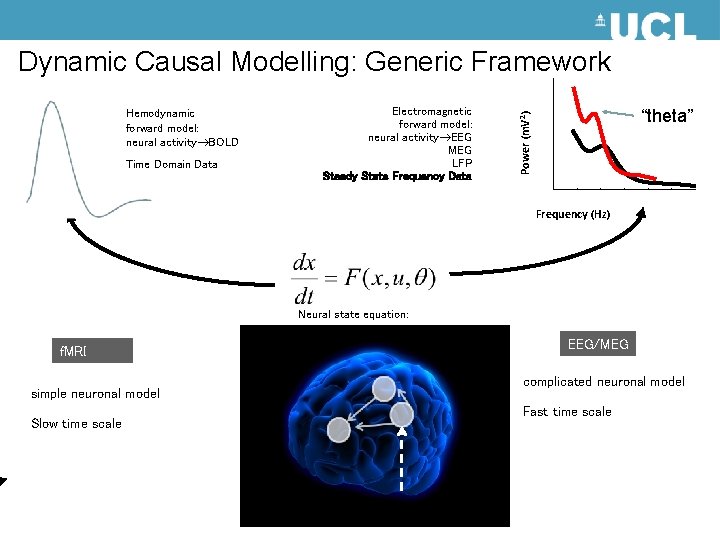
Dynamic Causal Modelling: Generic Framework Time Domain Data Electromagnetic forward model: neural activity EEG MEG LFP Steady State Frequency Data “theta” Power (m. V 2) Hemodynamic forward model: neural activity BOLD Frequency (Hz) Neural state equation: f. MRI simple neuronal model Slow time scale EEG/MEG complicated neuronal model Fast time scale
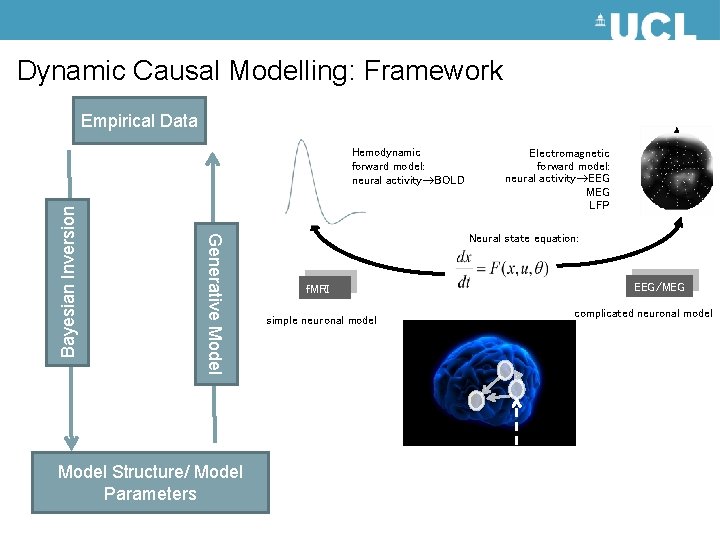
Dynamic Causal Modelling: Framework Empirical Data Generative Model Bayesian Inversion Hemodynamic forward model: neural activity BOLD Model Structure/ Model Parameters Electromagnetic forward model: neural activity EEG MEG LFP Neural state equation: f. MRI simple neuronal model EEG/MEG complicated neuronal model
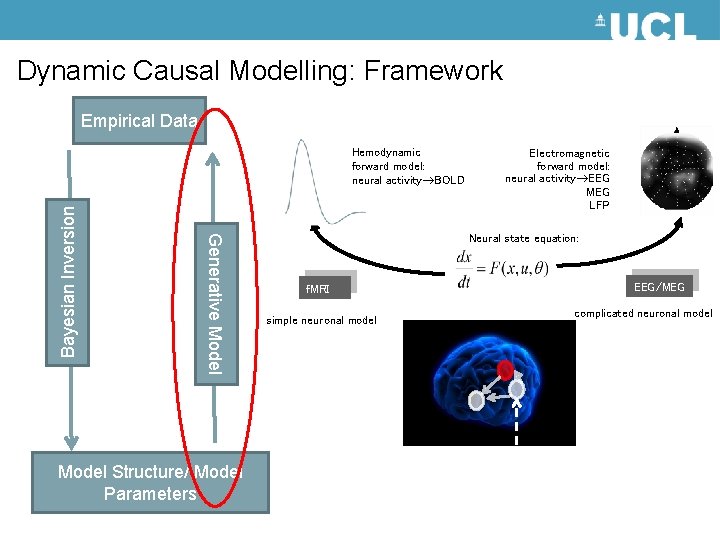
Dynamic Causal Modelling: Framework Empirical Data Generative Model Bayesian Inversion Hemodynamic forward model: neural activity BOLD Model Structure/ Model Parameters Electromagnetic forward model: neural activity EEG MEG LFP Neural state equation: f. MRI simple neuronal model EEG/MEG complicated neuronal model
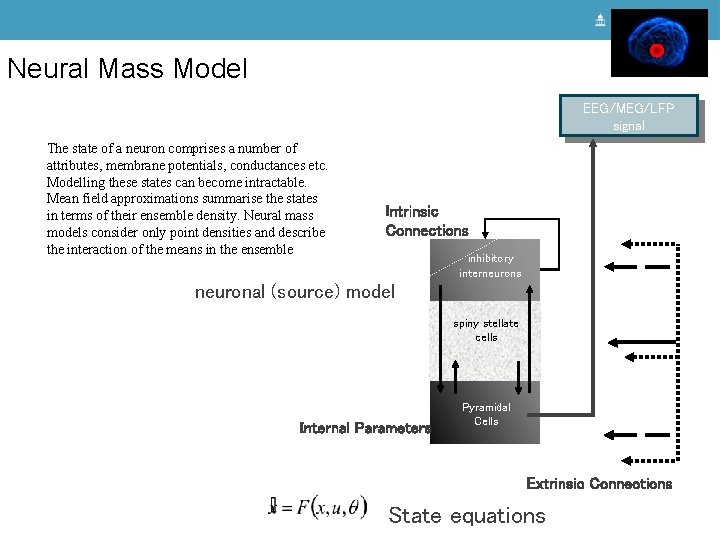
Neural Mass Model EEG/MEG/LFP signal The state of a neuron comprises a number of attributes, membrane potentials, conductances etc. Modelling these states can become intractable. Mean field approximations summarise the states in terms of their ensemble density. Neural mass models consider only point densities and describe the interaction of the means in the ensemble Intrinsic Connections inhibitory interneurons neuronal (source) model spiny stellate cells Internal Parameters Pyramidal Cells Extrinsic Connections State equations
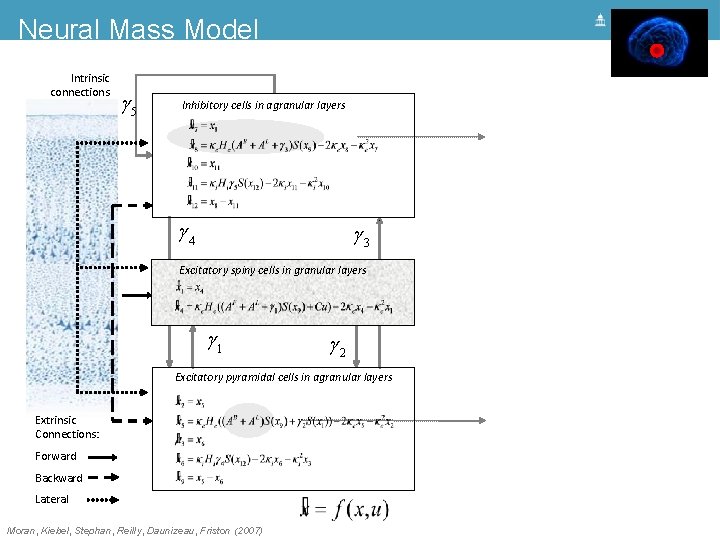
Neural Mass Model Intrinsic connections g 5 Inhibitory cells in agranular layers g 4 g 3 Excitatory spiny cells in granular layers = x 4 x& 1 = ke He (g 1 s(x 9 - a) + u) - 2 ke x 4 - ke 2 x 1 x& 4 g 1 g 2 Excitatory pyramidal cells in agranular layers Extrinsic Connections: Forward Backward Lateral Moran, Kiebel, Stephan, Reilly, Daunizeau, Friston (2007)
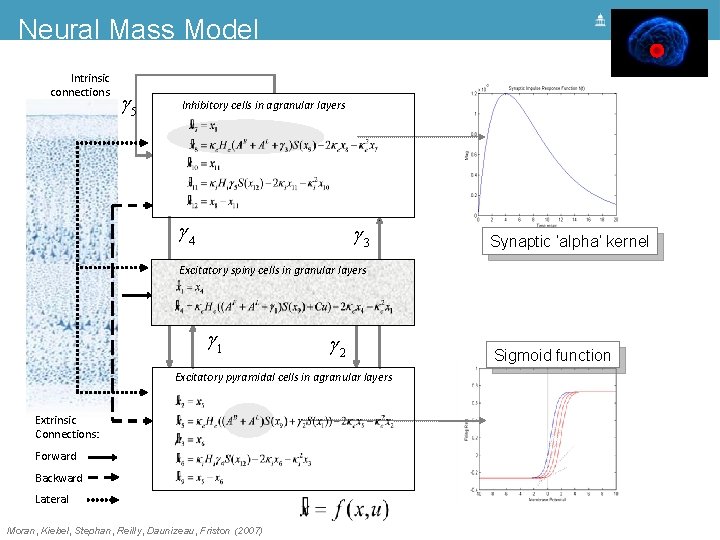
Neural Mass Model Intrinsic connections g 5 Inhibitory cells in agranular layers g 4 g 3 Synaptic ‘alpha’ kernel Excitatory spiny cells in granular layers = x 4 x& 1 = ke He (g 1 s(x 9 - a) + u) - 2 ke x 4 - ke 2 x 1 x& 4 g 1 g 2 Excitatory pyramidal cells in agranular layers Extrinsic Connections: Forward Backward Lateral Moran, Kiebel, Stephan, Reilly, Daunizeau, Friston (2007) Sigmoid function
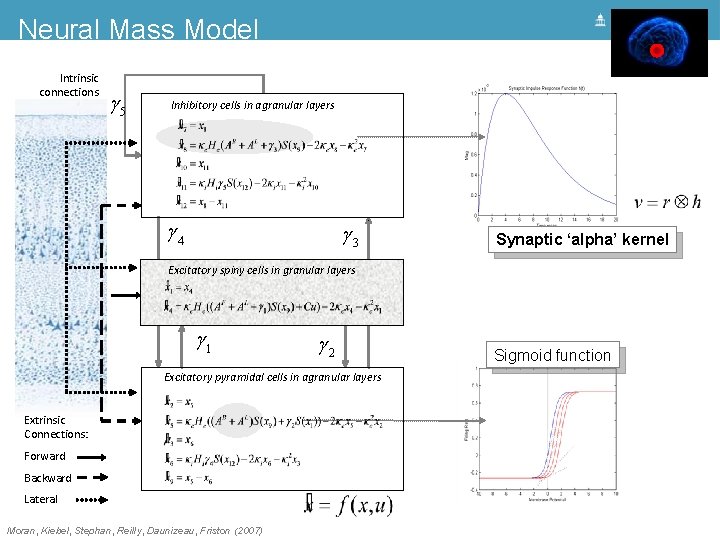
Neural Mass Model Intrinsic connections g 5 Inhibitory cells in agranular layers g 4 g 3 Synaptic ‘alpha’ kernel Excitatory spiny cells in granular layers = x 4 x& 1 = ke He (g 1 s(x 9 - a) + u) - 2 ke x 4 - ke 2 x 1 x& 4 g 1 g 2 Excitatory pyramidal cells in agranular layers Extrinsic Connections: Forward Backward Lateral Moran, Kiebel, Stephan, Reilly, Daunizeau, Friston (2007) Sigmoid function
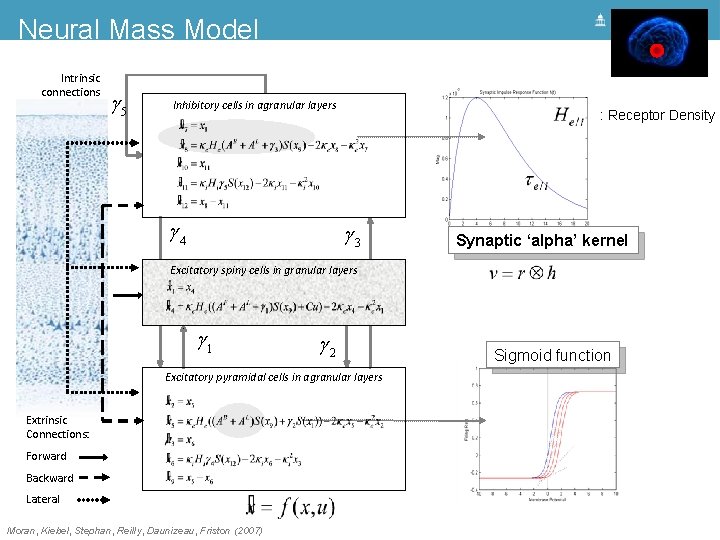
Neural Mass Model Intrinsic connections g 5 Inhibitory cells in agranular layers g 4 : Receptor Density g 3 Synaptic ‘alpha’ kernel Excitatory spiny cells in granular layers = x 4 x& 1 = ke He (g 1 s(x 9 - a) + u) - 2 ke x 4 - ke 2 x 1 x& 4 g 1 g 2 Excitatory pyramidal cells in agranular layers Extrinsic Connections: Forward Backward Lateral Moran, Kiebel, Stephan, Reilly, Daunizeau, Friston (2007) Sigmoid function
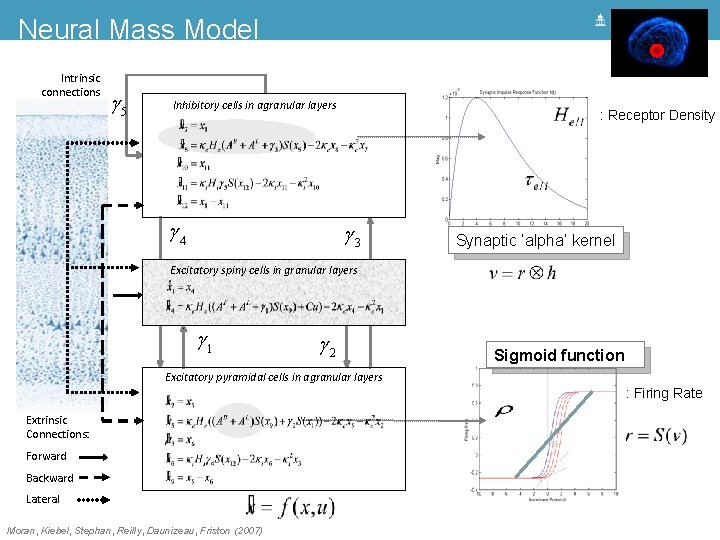
Neural Mass Model Intrinsic connections g 5 Inhibitory cells in agranular layers g 4 : Receptor Density g 3 Synaptic ‘alpha’ kernel Excitatory spiny cells in granular layers = x 4 x& 1 = ke He (g 1 s(x 9 - a) + u) - 2 ke x 4 - ke 2 x 1 x& 4 g 1 g 2 Sigmoid function Excitatory pyramidal cells in agranular layers : Firing Rate Extrinsic Connections: Forward Backward Lateral Moran, Kiebel, Stephan, Reilly, Daunizeau, Friston (2007)
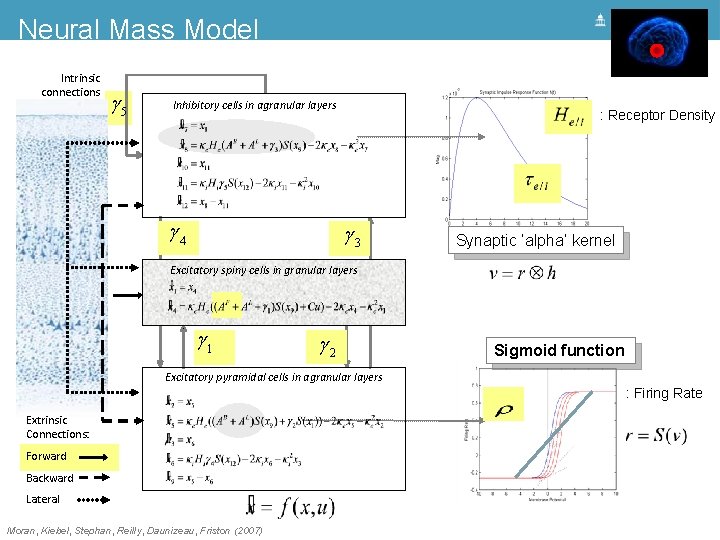
Neural Mass Model Intrinsic connections g 5 Inhibitory cells in agranular layers g 4 : Receptor Density g 3 Synaptic ‘alpha’ kernel Excitatory spiny cells in granular layers = x 4 x& 1 = ke He (g 1 s(x 9 - a) + u) - 2 ke x 4 - ke 2 x 1 x& 4 g 1 g 2 Sigmoid function Excitatory pyramidal cells in agranular layers : Firing Rate Extrinsic Connections: Forward Backward Lateral Moran, Kiebel, Stephan, Reilly, Daunizeau, Friston (2007)
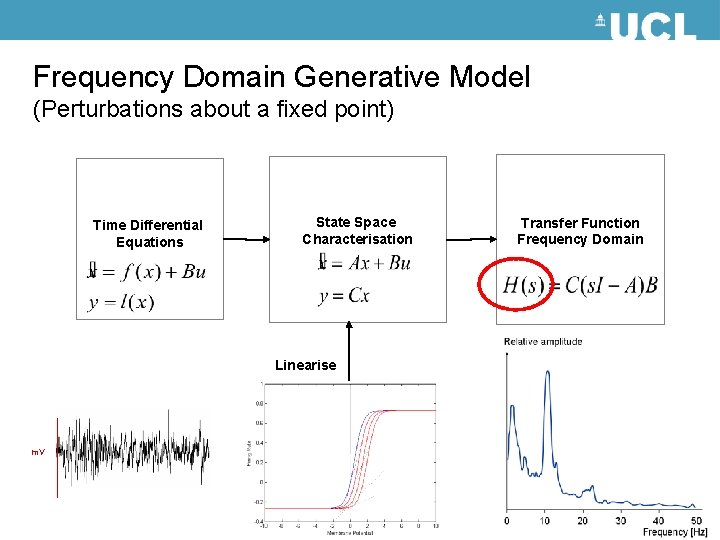
Frequency Domain Generative Model (Perturbations about a fixed point) Time Differential Equations State Space Characterisation Linearise m. V Transfer Function Frequency Domain
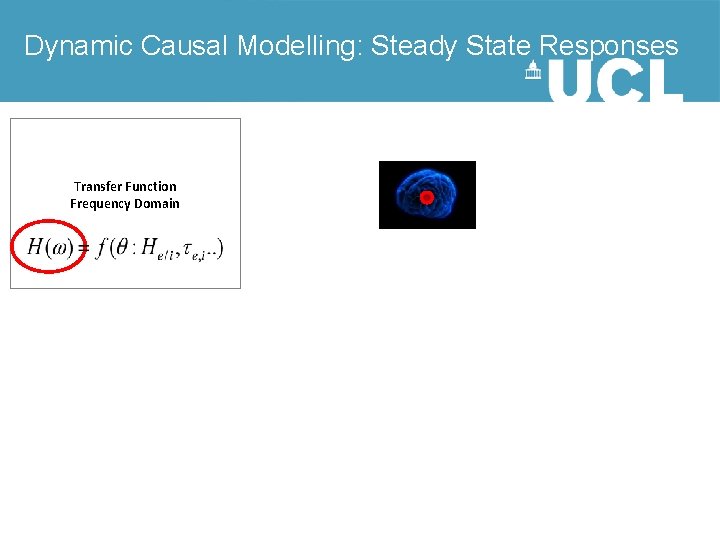
Dynamic Causal Modelling: Steady State Responses Transfer Function Frequency Domain
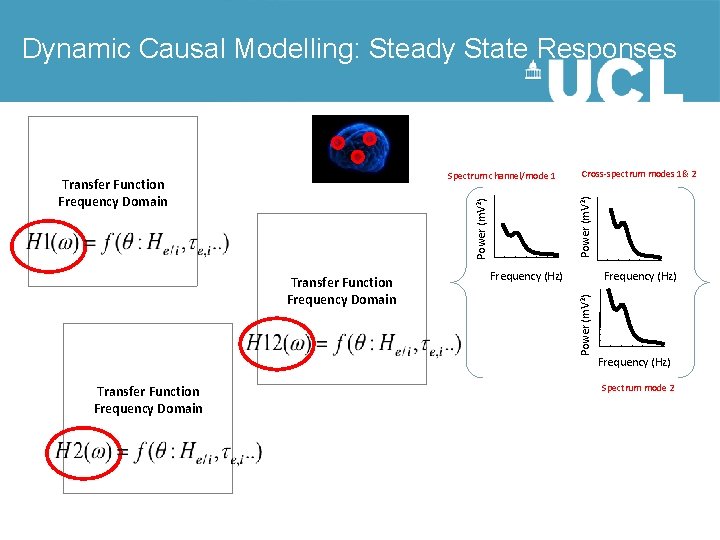
Dynamic Causal Modelling: Steady State Responses Power (m. V 2) Transfer Function Frequency Domain Frequency (Hz) Power (m. V 2) Transfer Function Frequency Domain Cross-spectrum modes 1& 2 Power (m. V 2) Spectrum channel/mode 1 Transfer Function Frequency Domain Frequency (Hz) Spectrum mode 2
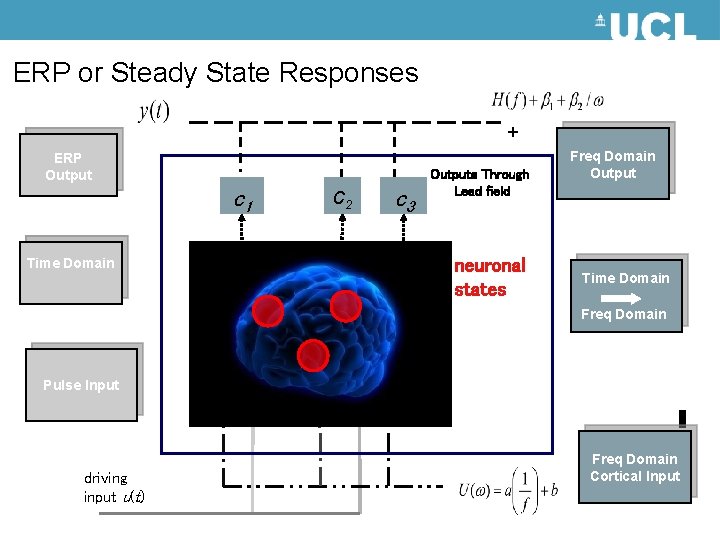
ERP or Steady State Responses + ERP Output c 1 Time Domain c 2 output s 2(t) output s 1(t) c 3 output s 3(t) Outputs Through Lead field neuronal states Freq Domain Output Time Domain Freq Domain Pulse Input driving input u(t) Freq Domain Cortical Input
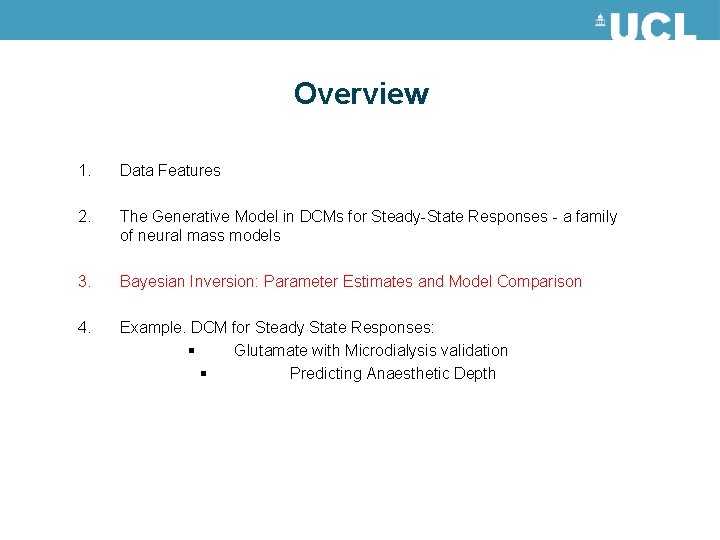
Overview 1. Data Features 2. The Generative Model in DCMs for Steady-State Responses - a family of neural mass models 3. Bayesian Inversion: Parameter Estimates and Model Comparison 4. Example. DCM for Steady State Responses: § Glutamate with Microdialysis validation § Predicting Anaesthetic Depth
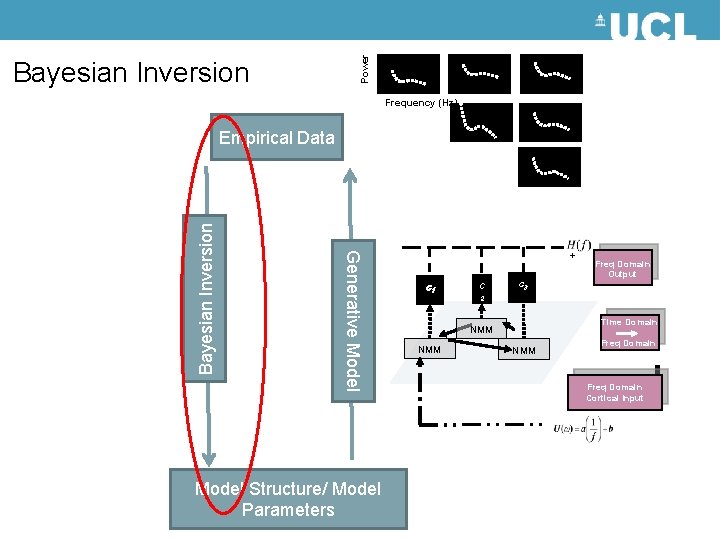
Power Bayesian Inversion Frequency (Hz) Generative Model Bayesian Inversion Empirical Data Model Structure/ Model Parameters + c 1 c c 3 Freq Domain Output 2 Time Domain NMM NMM Freq Domain Cortical Input
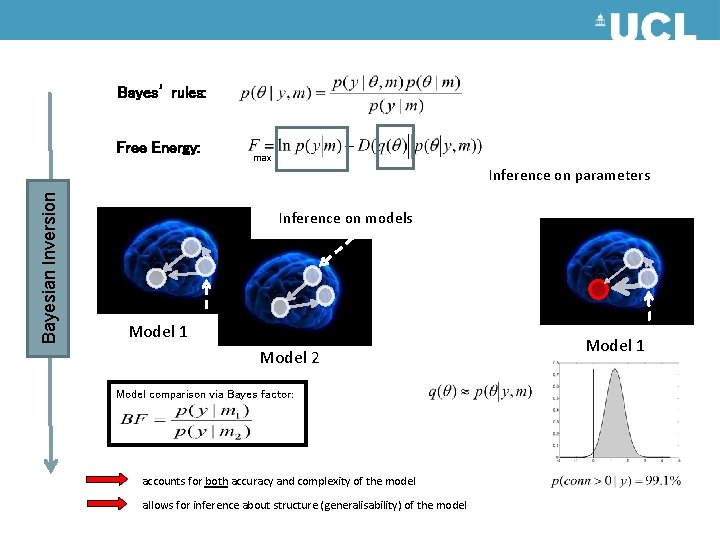
Bayes’ rules: Free Energy: max Bayesian Inversion Inference on parameters Inference on models Model 1 Model 2 Model comparison via Bayes factor: accounts for both accuracy and complexity of the model allows for inference about structure (generalisability) of the model Model 1
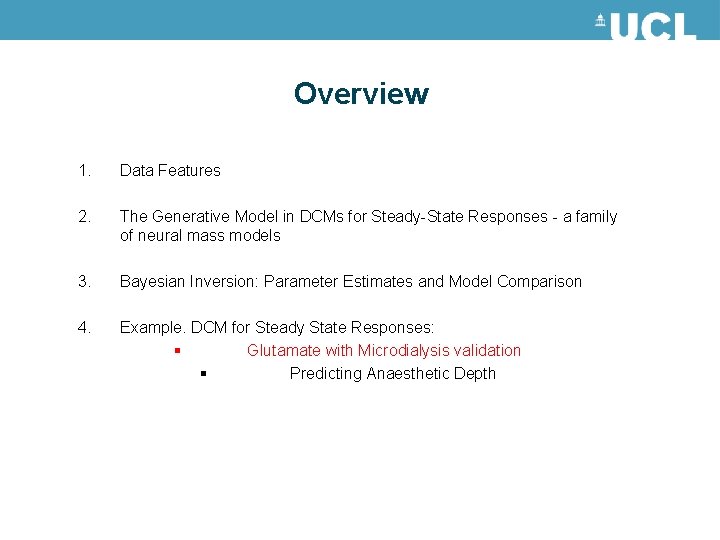
Overview 1. Data Features 2. The Generative Model in DCMs for Steady-State Responses - a family of neural mass models 3. Bayesian Inversion: Parameter Estimates and Model Comparison 4. Example. DCM for Steady State Responses: § Glutamate with Microdialysis validation § Predicting Anaesthetic Depth
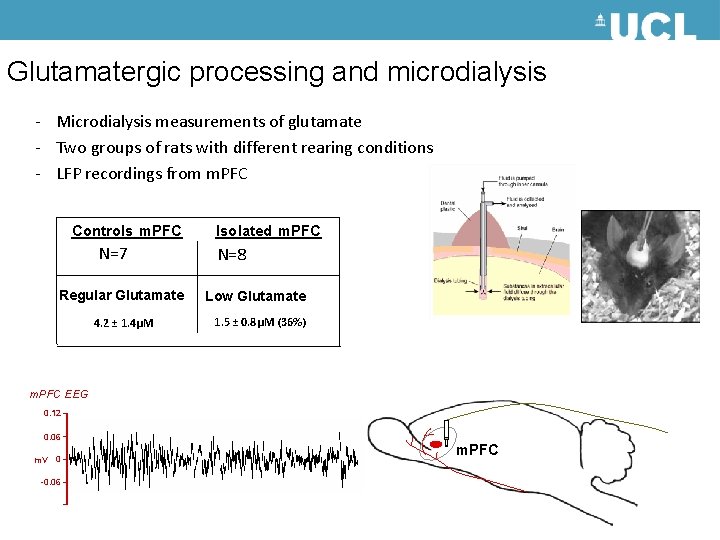
Glutamatergic processing and microdialysis - Microdialysis measurements of glutamate - Two groups of rats with different rearing conditions - LFP recordings from m. PFC Controls m. PFC N=7 Regular Glutamate 4. 2 ± 1. 4μM Isolated m. PFC N=8 Low Glutamate 1. 5 ± 0. 8μM (36%) m. PFC EEG 0. 12 0. 06 m. V 0 -0. 06 m. PFC
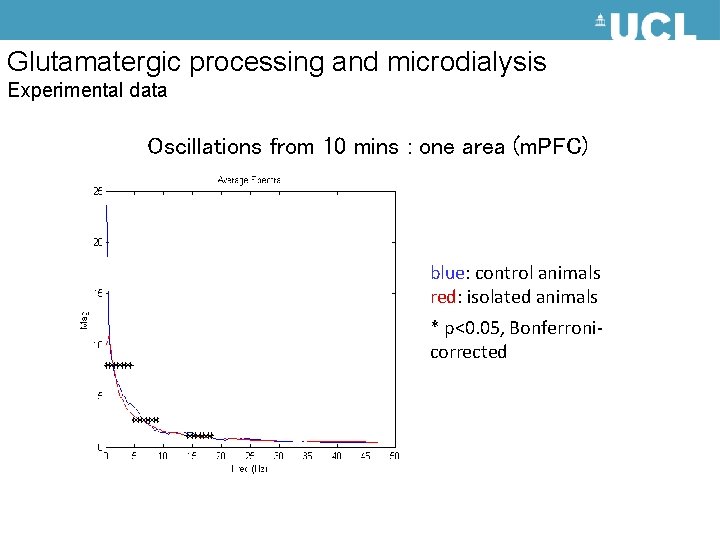
Glutamatergic processing and microdialysis Experimental data Oscillations from 10 mins : one area (m. PFC) blue: control animals red: isolated animals * p<0. 05, Bonferronicorrected
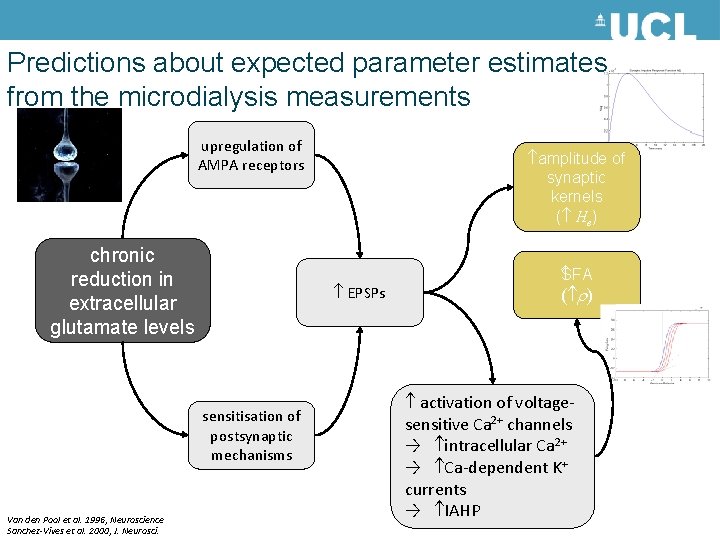
Predictions about expected parameter estimates from the microdialysis measurements upregulation of AMPA receptors chronic reduction in extracellular glutamate levels EPSPs sensitisation of postsynaptic mechanisms Van den Pool et al. 1996, Neuroscience Sanchez-Vives et al. 2000, J. Neurosci. amplitude of synaptic kernels ( He) SFA ( ) activation of voltagesensitive Ca 2+ channels → intracellular Ca 2+ → Ca-dependent K+ currents → IAHP
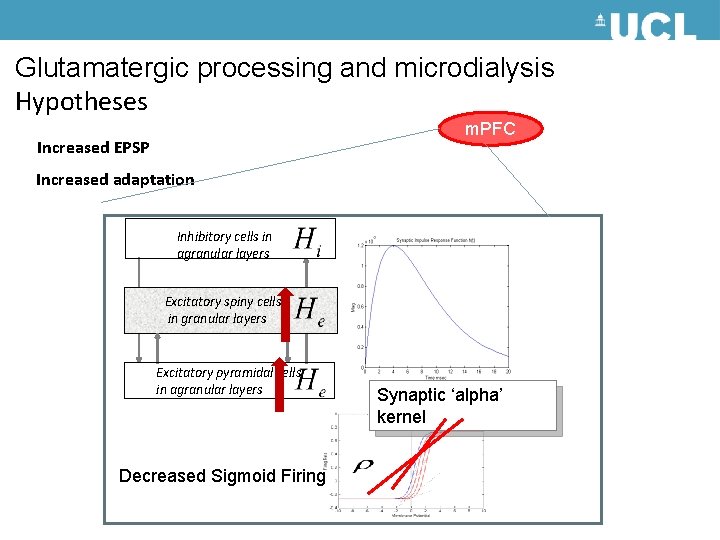
Glutamatergic processing and microdialysis Hypotheses m. PFC Increased EPSP Increased adaptation Inhibitory cells in agranular layers Excitatory spiny cells in granular layers Excitatory pyramidal cells in agranular layers Decreased Sigmoid Firing Synaptic ‘alpha’ kernel
![Glutamatergic processing and microdialysis Results g 5 3 8 6 3 Inhibitory cells in Glutamatergic processing and microdialysis Results g 5 [3. 8 6. 3] Inhibitory cells in](https://slidetodoc.com/presentation_image_h2/6d855fa7306b96ec4c3a09aeaedae772/image-33.jpg)
Glutamatergic processing and microdialysis Results g 5 [3. 8 6. 3] Inhibitory cells in supragranular layers Extrinsic forward connections u g 4 g 3 [29, 37] (0. 04) (0. 4) Excitatory spiny cells in granular layers g 1 [161, 210] g 2 [195, 233] (0. 13) (0. 37) Excitatory pyramidal cells in infragranular layers Control group estimates in blue, isolated animals in red, p values in parentheses. Moran, Stephan, Kiebel, Rombach, O’Connor, Murphy, Reilly, Friston (2008) [0. 76, 1. 34] (0. 0003)
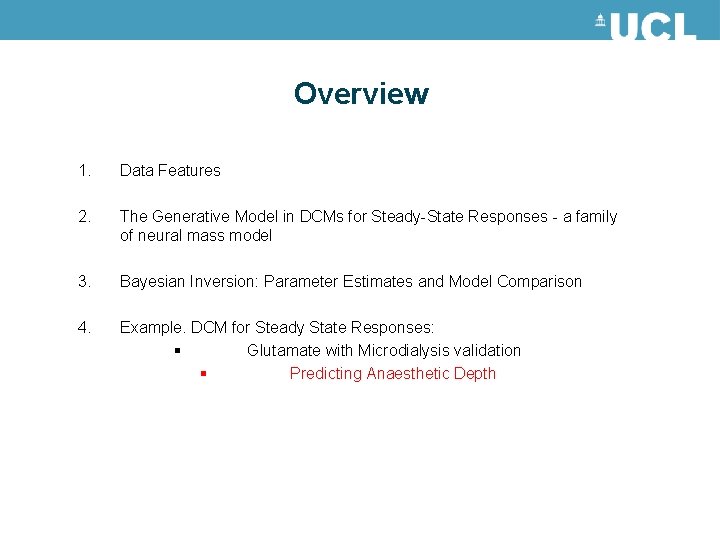
Overview 1. Data Features 2. The Generative Model in DCMs for Steady-State Responses - a family of neural mass model 3. Bayesian Inversion: Parameter Estimates and Model Comparison 4. Example. DCM for Steady State Responses: § Glutamate with Microdialysis validation § Predicting Anaesthetic Depth
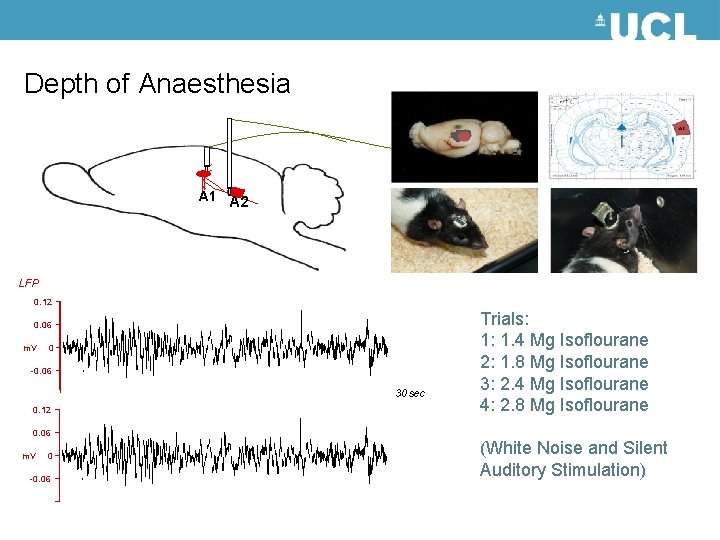
Depth of Anaesthesia A 1 A 2 LFP 0. 12 0. 06 m. V 0 -0. 06 30 sec 0. 12 Trials: 1: 1. 4 Mg Isoflourane 2: 1. 8 Mg Isoflourane 3: 2. 4 Mg Isoflourane 4: 2. 8 Mg Isoflourane 0. 06 m. V 0 -0. 06 (White Noise and Silent Auditory Stimulation)
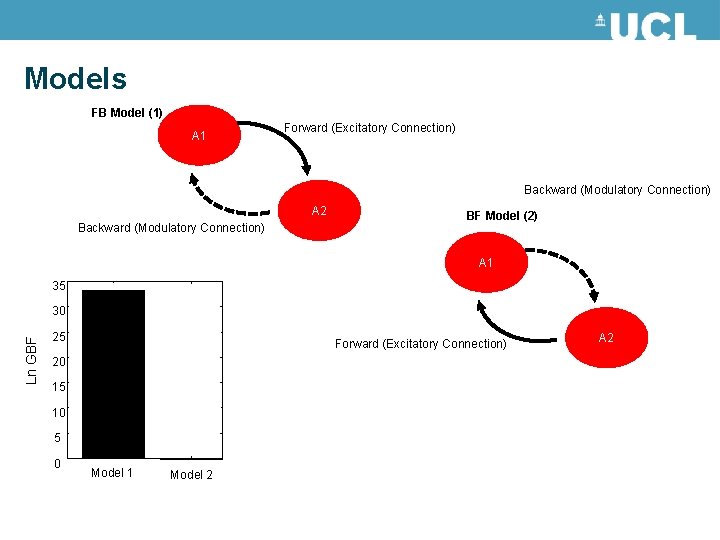
Models FB Model (1) A 1 Forward (Excitatory Connection) Backward (Modulatory Connection) A 2 Backward (Modulatory Connection) BF Model (2) A 1 35 Ln GBF 30 25 Forward (Excitatory Connection) 20 15 10 5 0 Model 1 Model 2 A 2
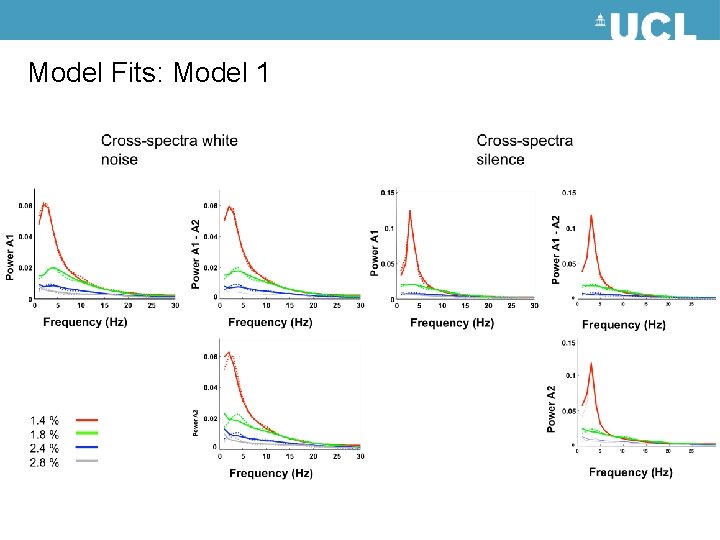
Model Fits: Model 1
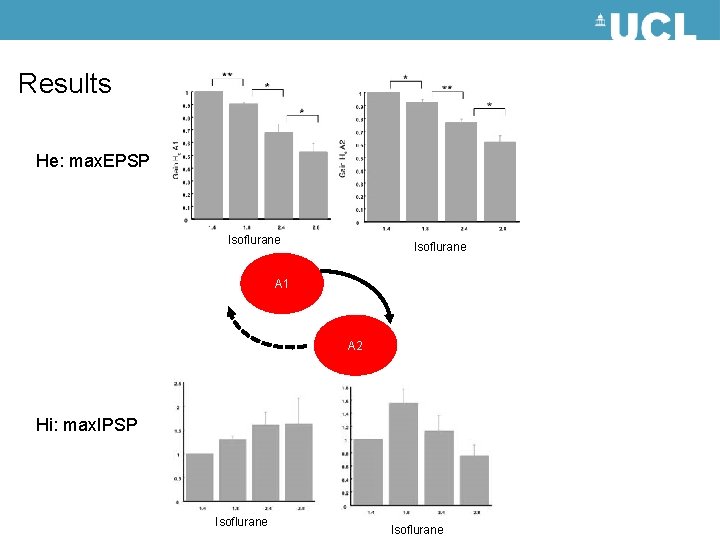
Results He: max. EPSP Isoflurane A 1 A 2 Hi: max. IPSP Isoflurane
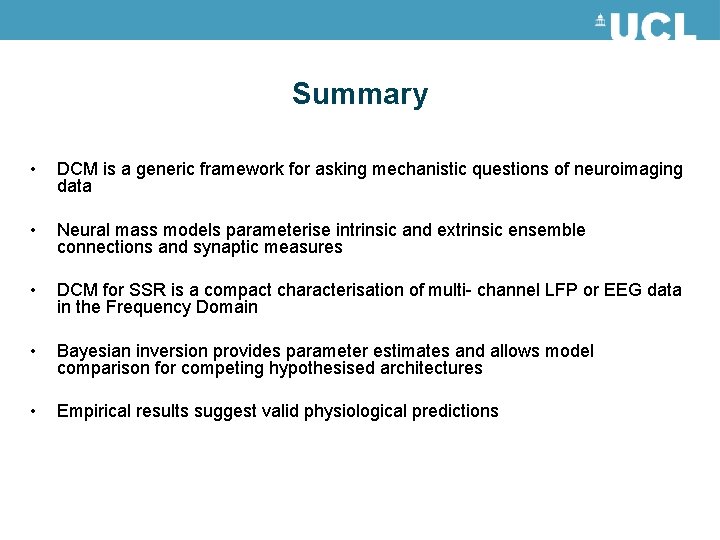
Summary • DCM is a generic framework for asking mechanistic questions of neuroimaging data • Neural mass models parameterise intrinsic and extrinsic ensemble connections and synaptic measures • DCM for SSR is a compact characterisation of multi- channel LFP or EEG data in the Frequency Domain • Bayesian inversion provides parameter estimates and allows model comparison for competing hypothesised architectures • Empirical results suggest valid physiological predictions
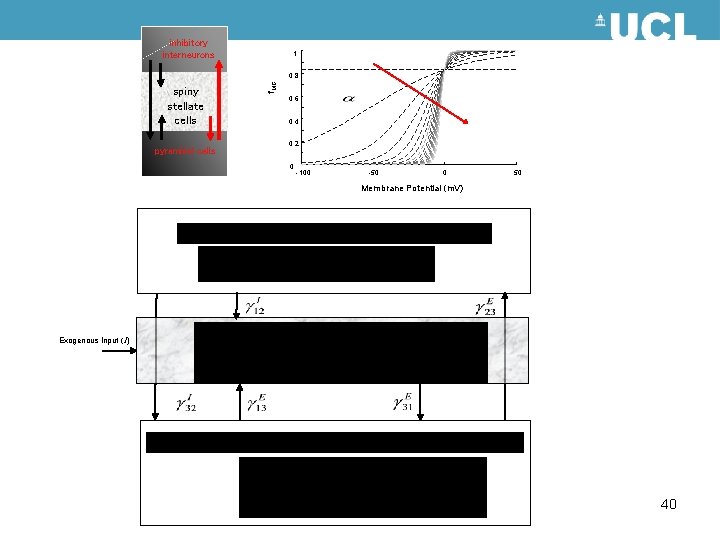
inhibitory interneurons pyramidal cells 0. 8 f. MG pyramidal cells spiny stellate cells pyramidal cells 1 0. 6 0. 4 0. 2 0 -100 -50 0 50 Membrane Potential (m. V) Exogenous Input (I) 40
Rosalyn moran
Rosalyn scaff
Marcela boni evangelista
Causalidad en la historia
Rosalyn moran
Rosalyn la liberte
Tim willet
Schilthuis steady state model
Steady state thermal analysis ansys
Steadystate error
Sinusoidal steady state
Secara matematis tingkat pengangguran steady state adalah
Steady state kondition
Michaelis menten steady state
What is steady flow process in thermodynamics
Standard test signals in control system are
In a normal operations/steady state eoc activation level,
Static fault
Sinusoidal steady state analysis of coupled circuits
Steady state vs equilibrium
What is steady-state thermal analysis?
Michaelis menten steady state
Steady-state error
Steady state error
Steady state error table
Hurtwitz
Transient and steady state response
Sinusoidal steady state power calculations
Sinusoidal steady state analysis solved problems
Steady state material balance
Steady state approximation examples
Pseudo 1st order reaction
What are 3 characteristics of enzymes
Steady state vs equilibrium
Pendekatan steady state
Error steady state adalah
Maintenance dose formula
Affirmation of the consequent aba
Sinusoidal steady state analysis
One dimensional steady state heat conduction
Error steady state adalah