Access information Anywhere Anytime and Anyway make a
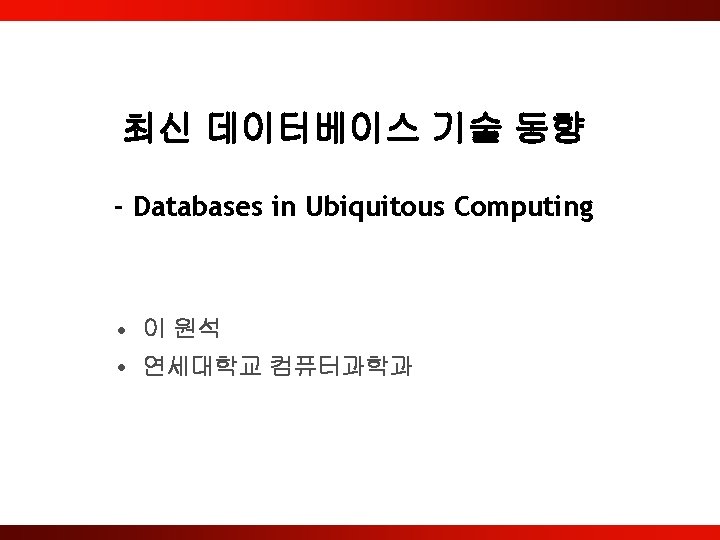
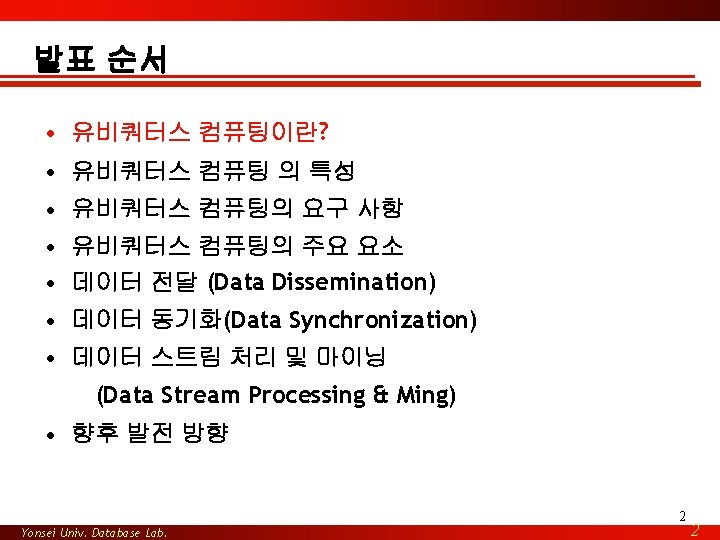
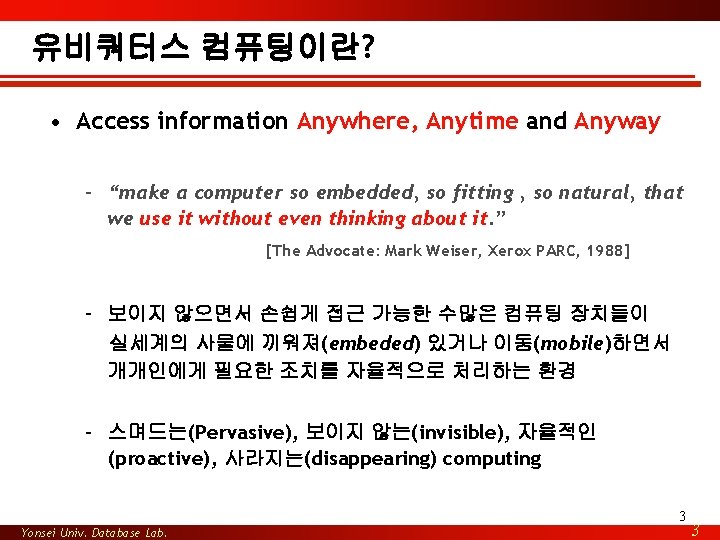
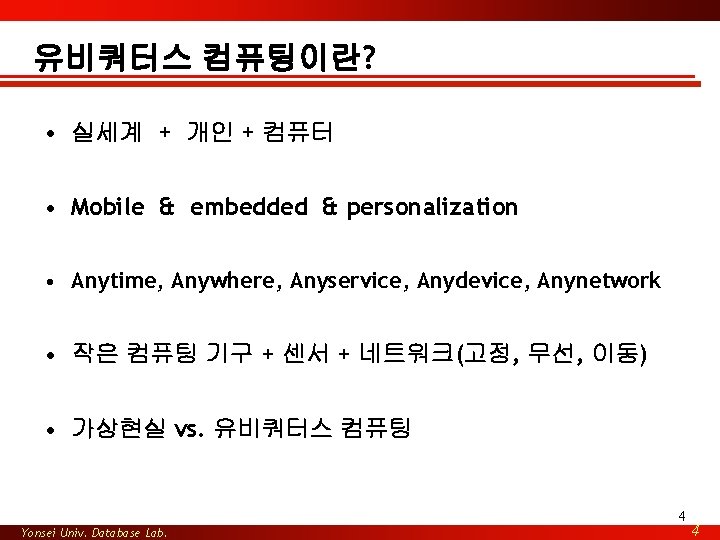
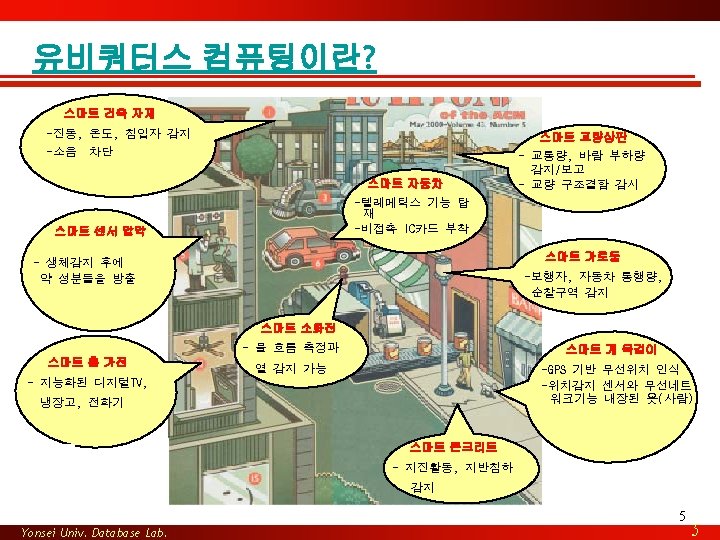
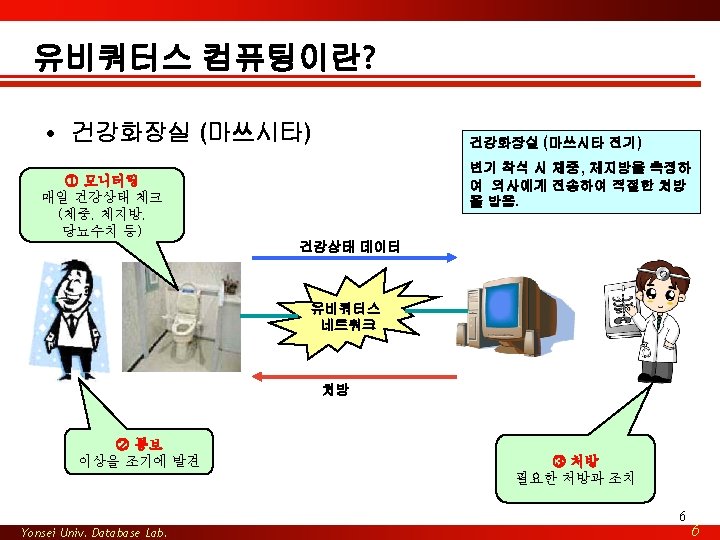
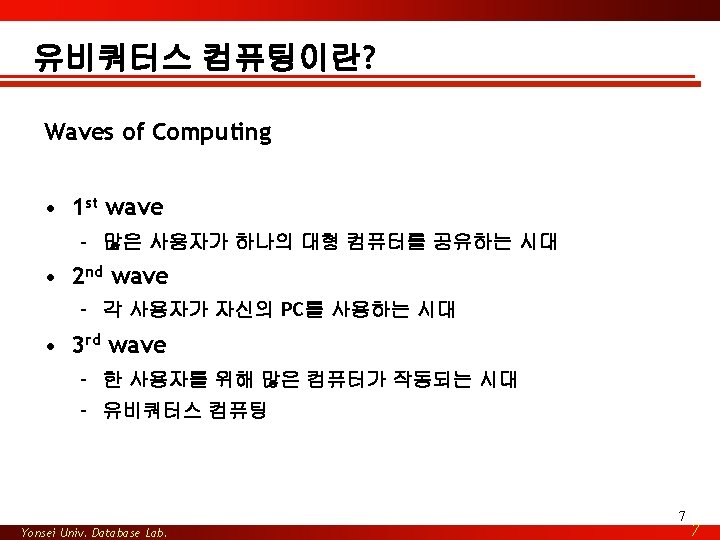
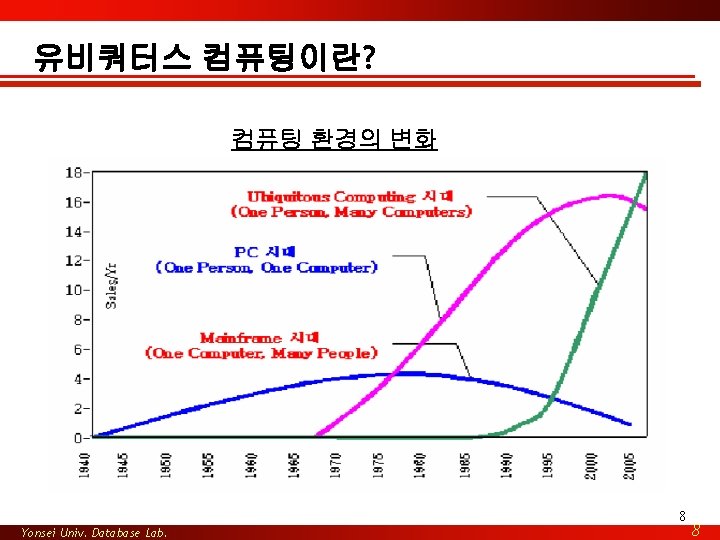
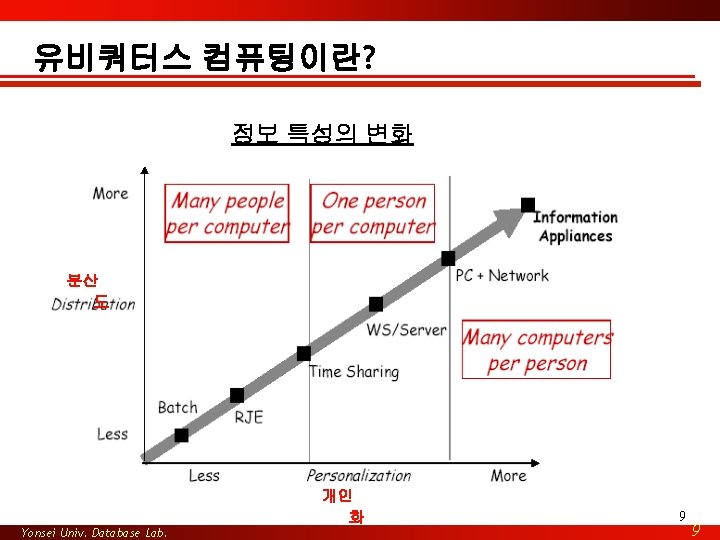
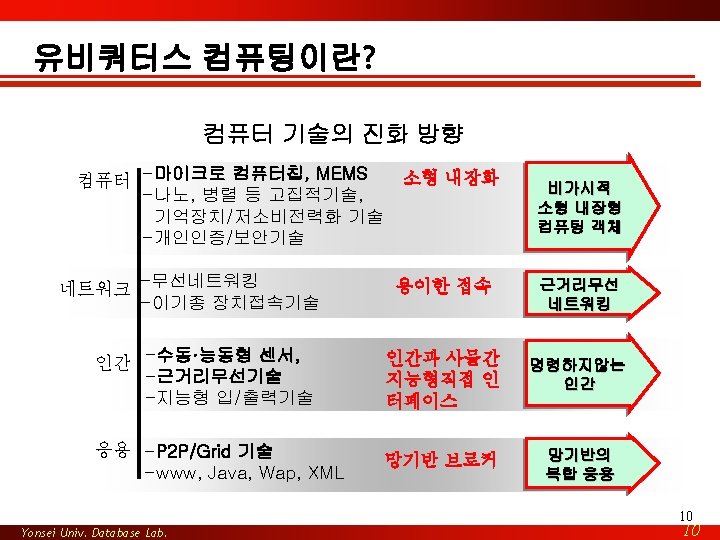
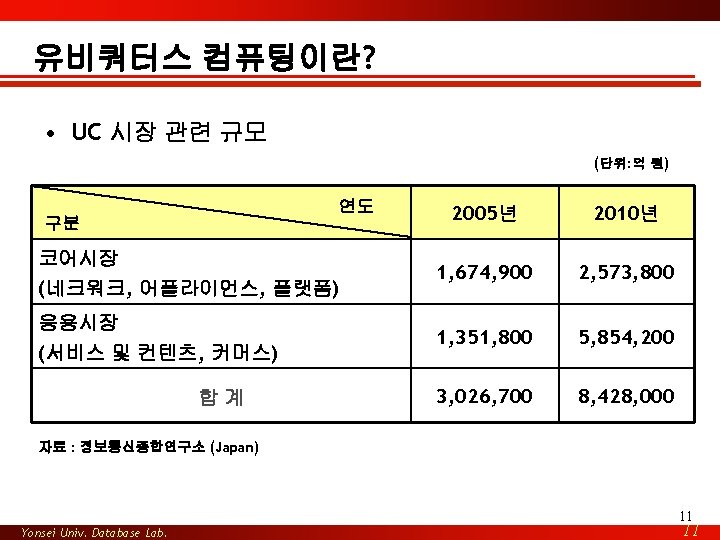
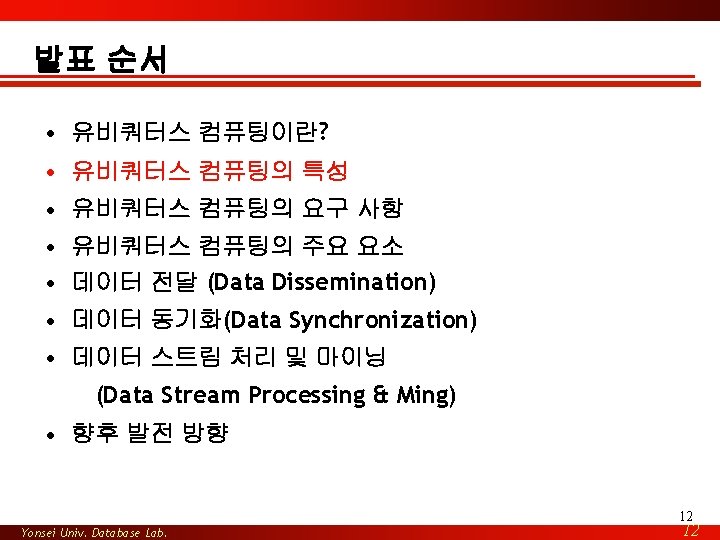
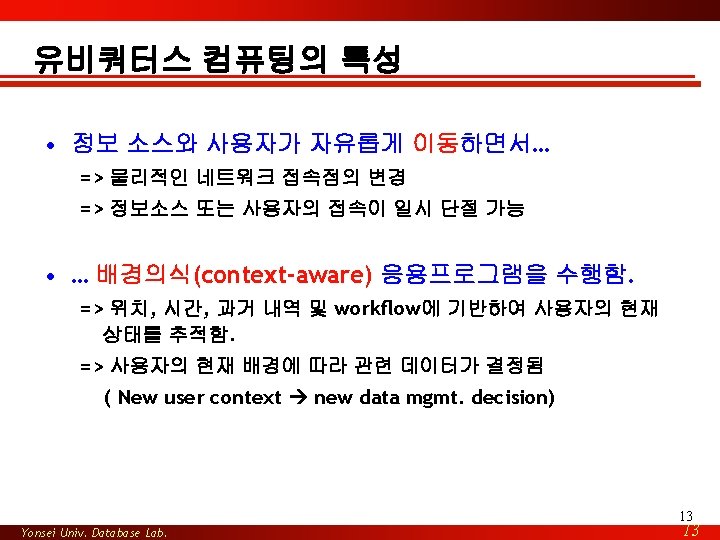
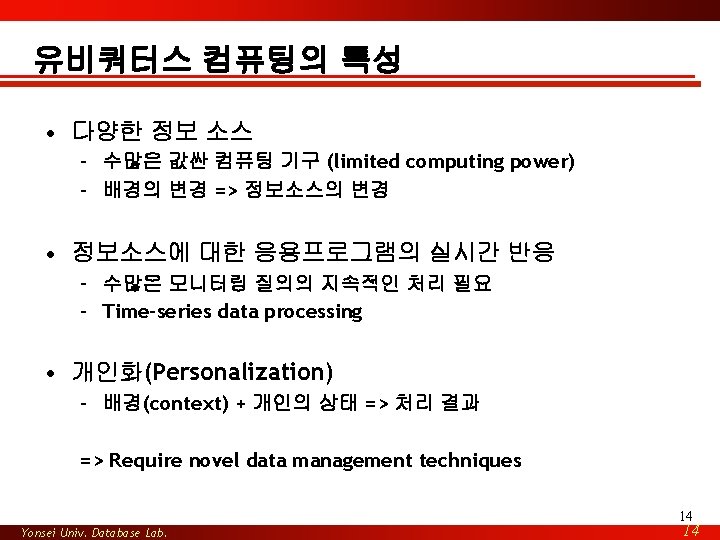
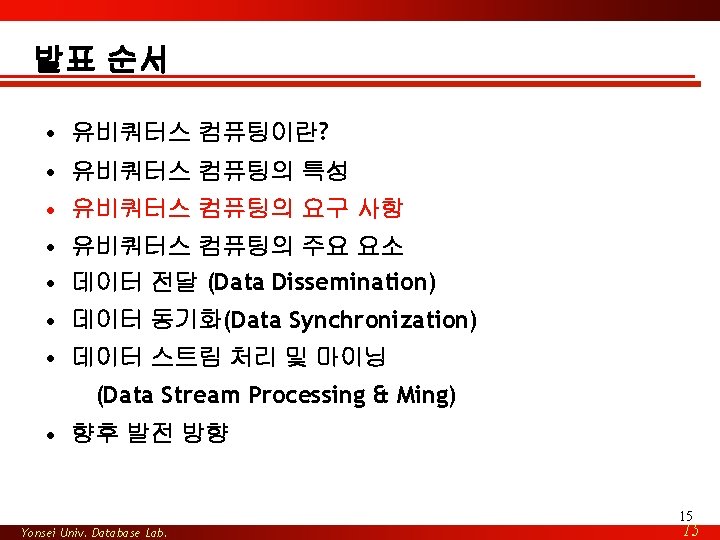
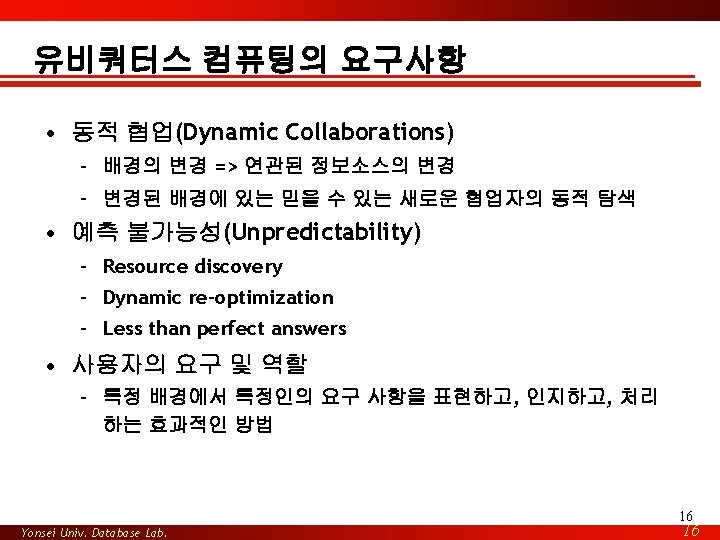
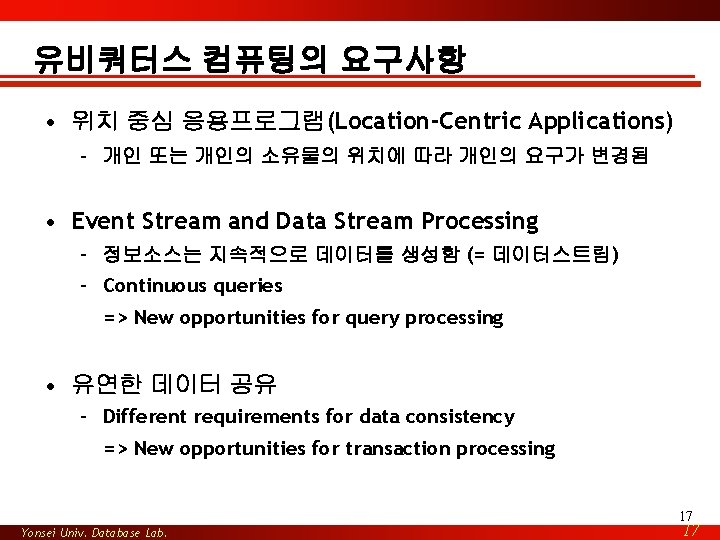
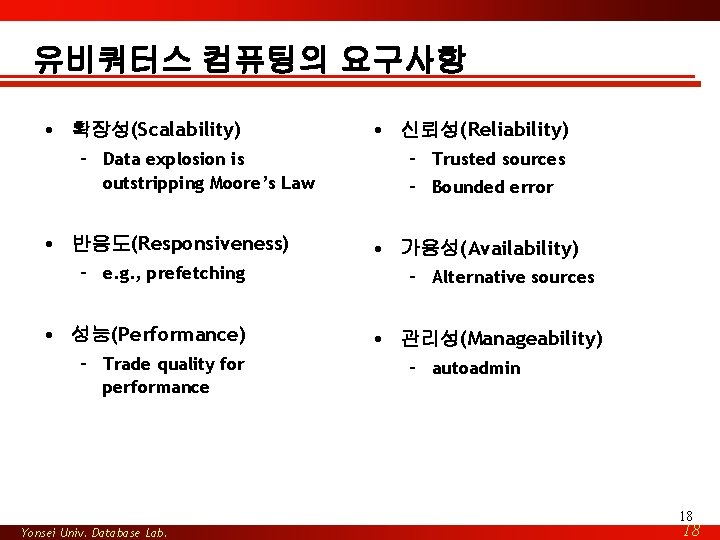
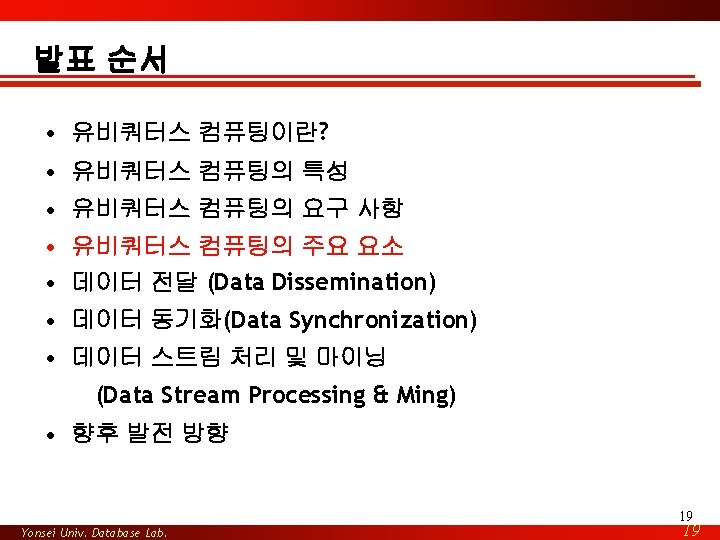
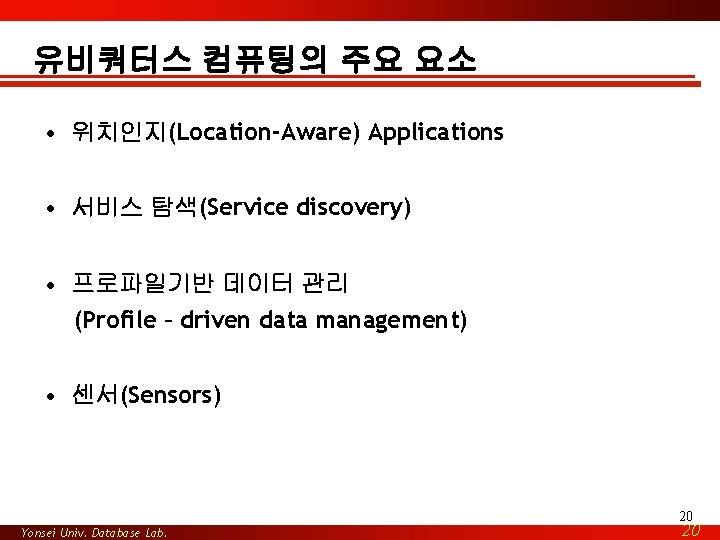
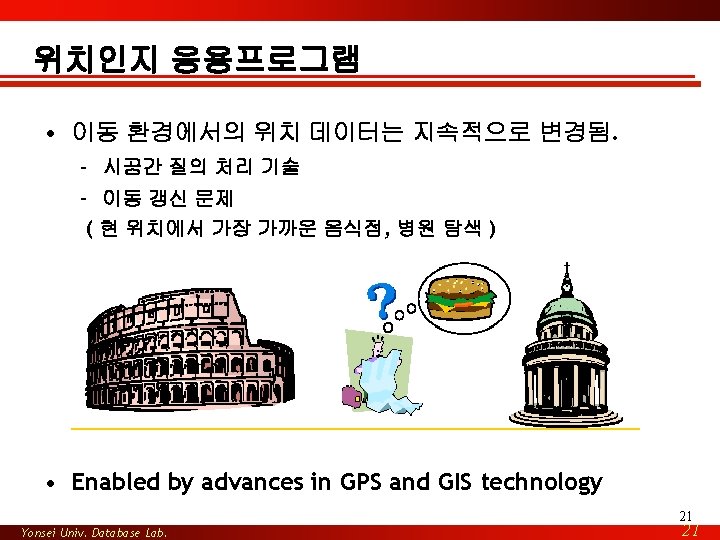
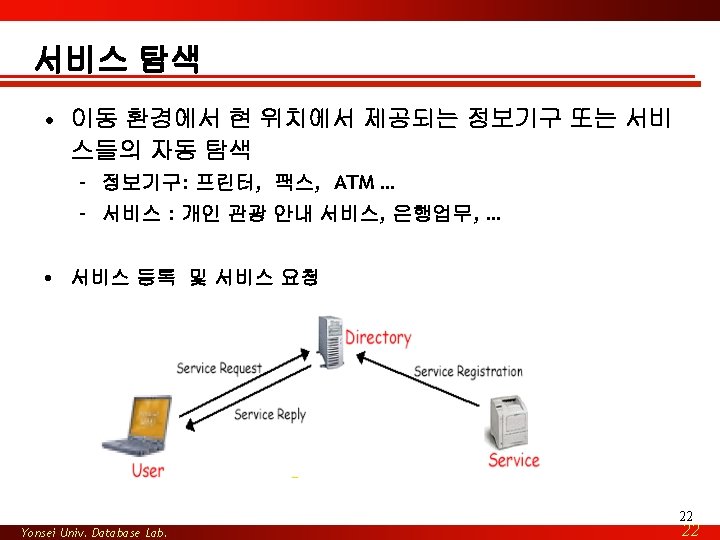
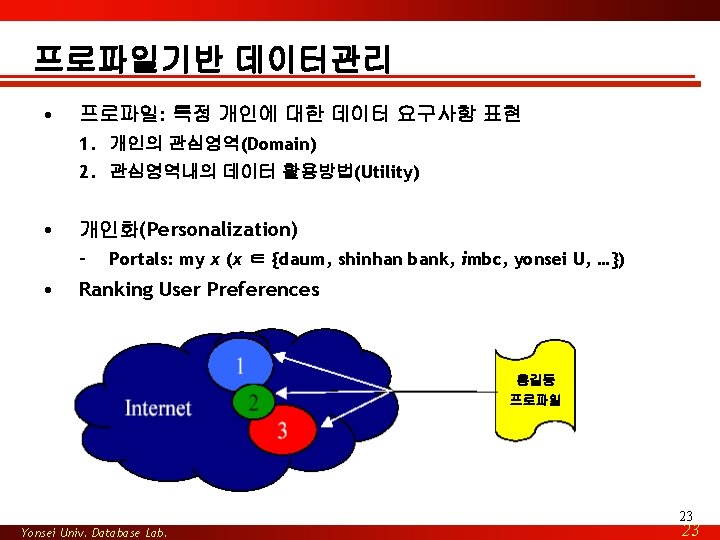
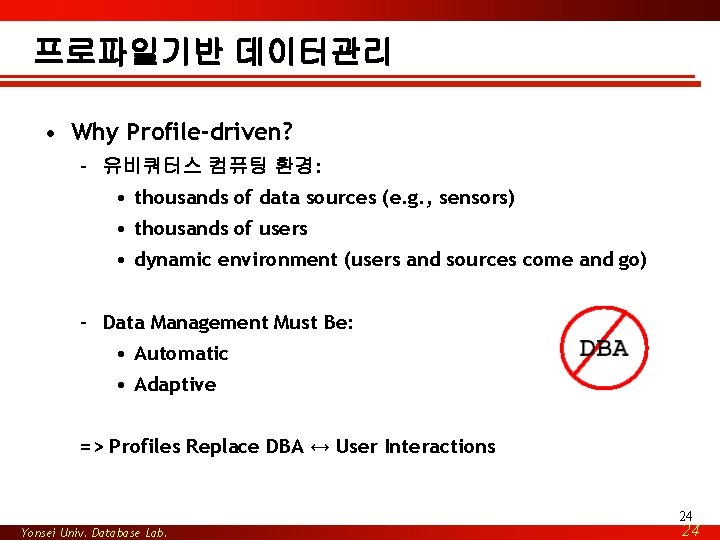
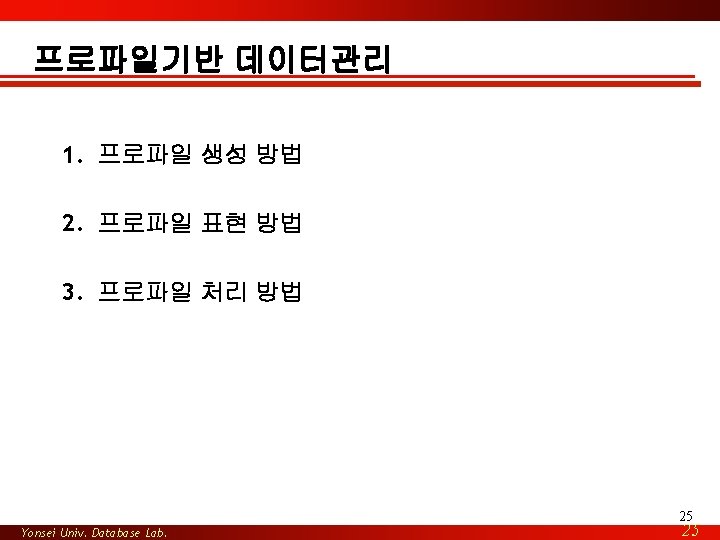
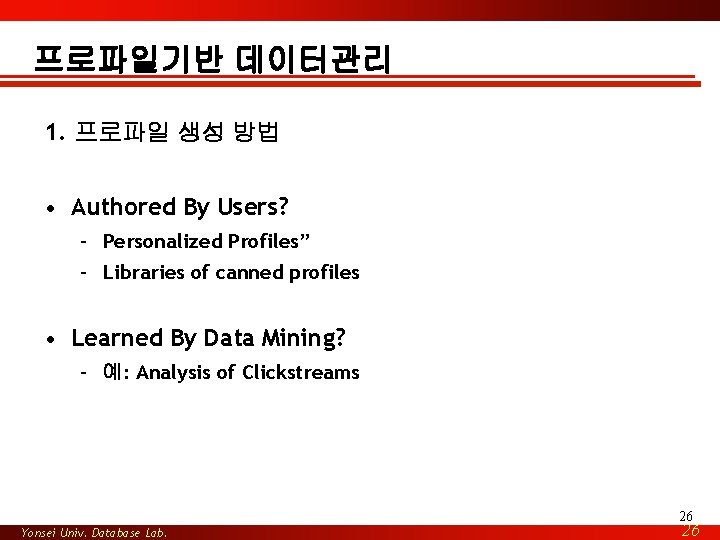
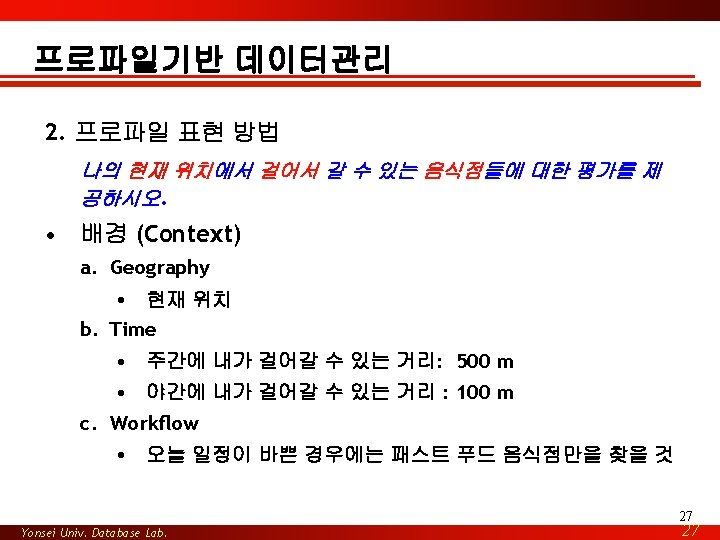
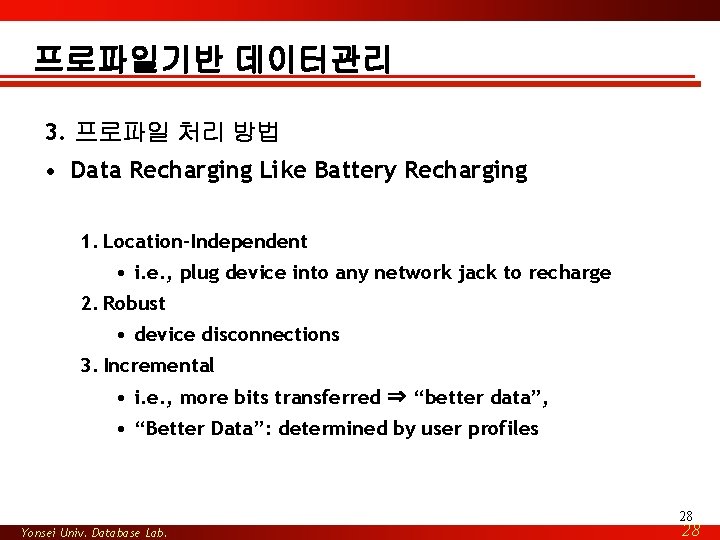
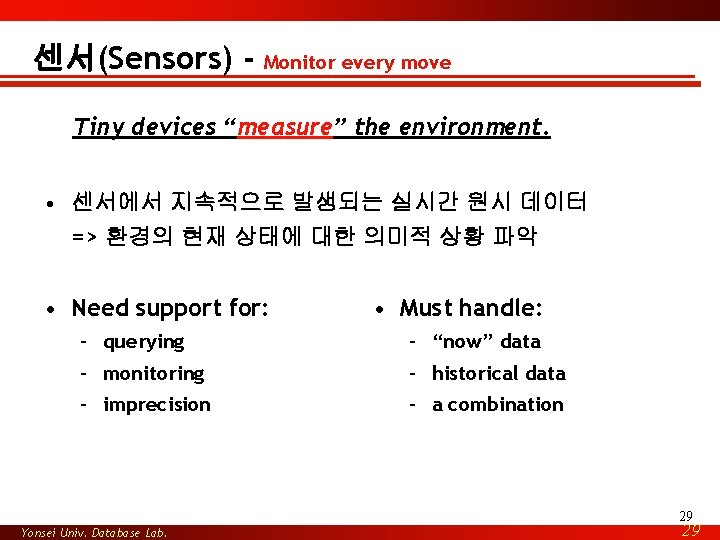
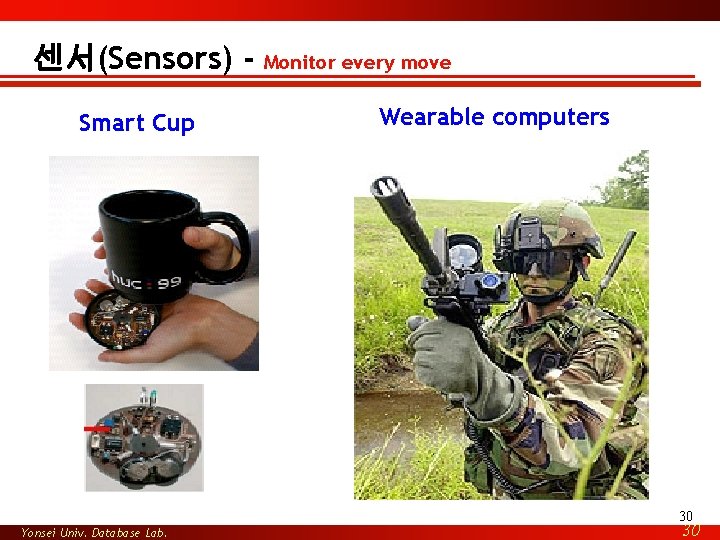
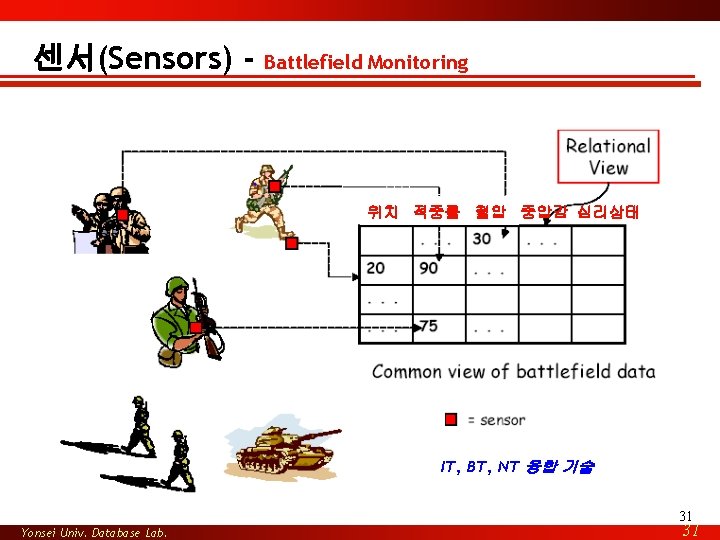
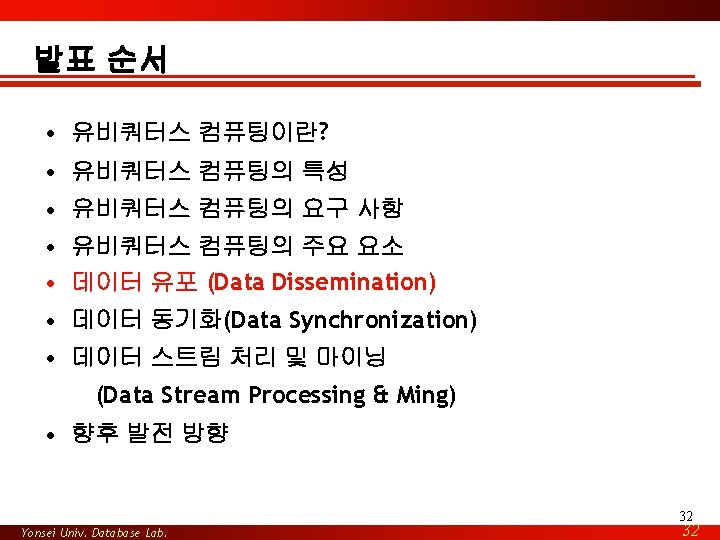
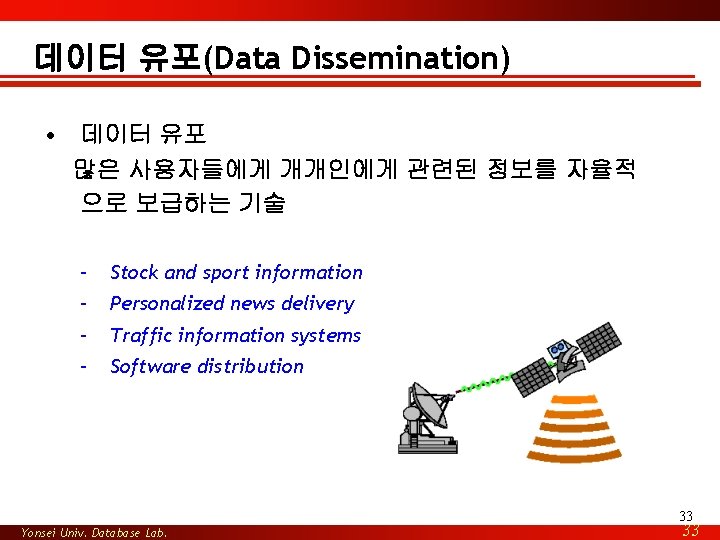
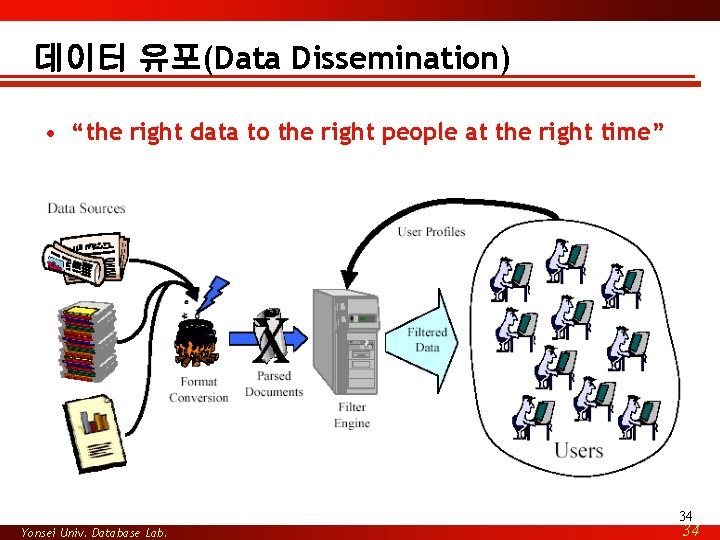
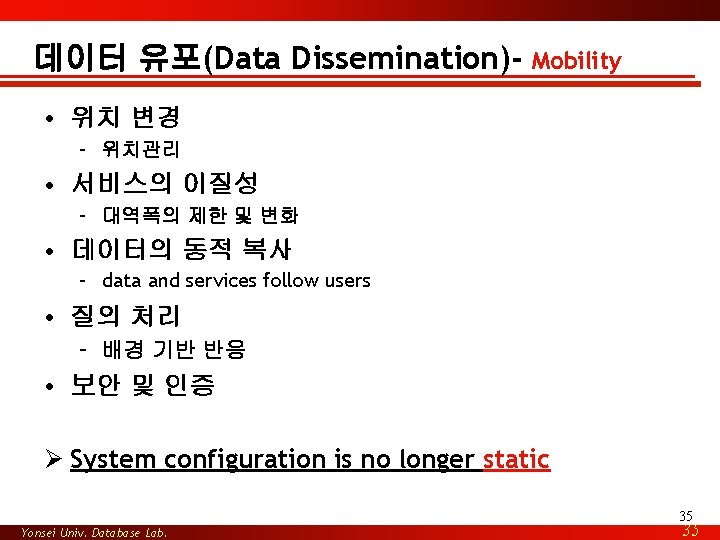
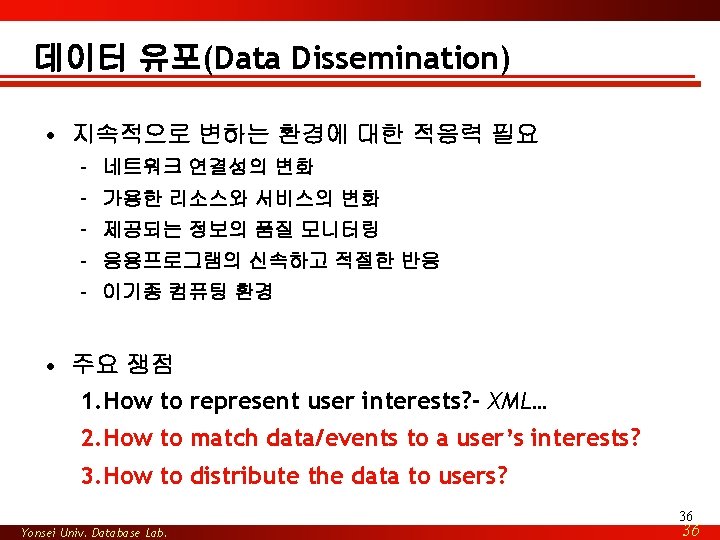
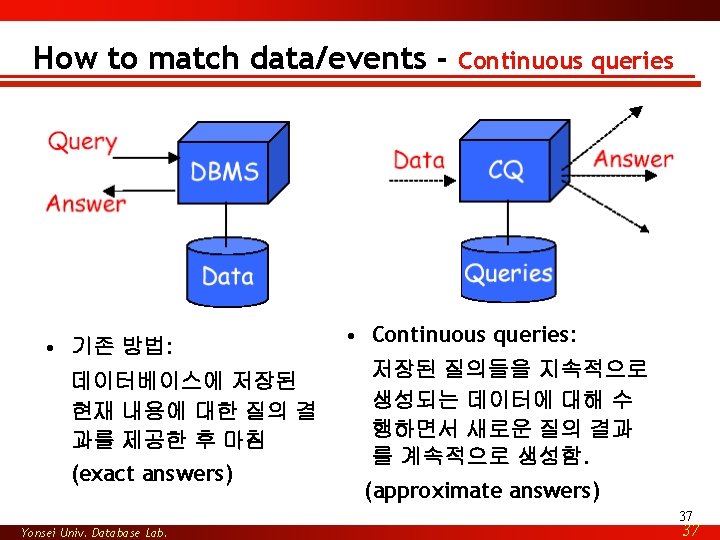
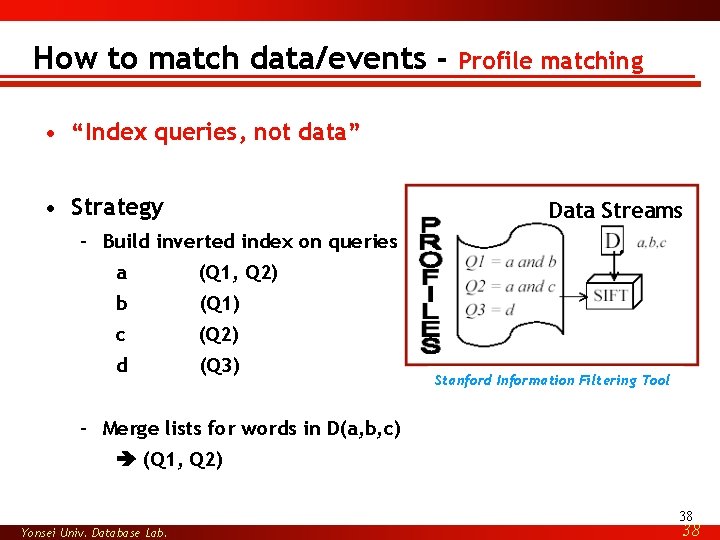
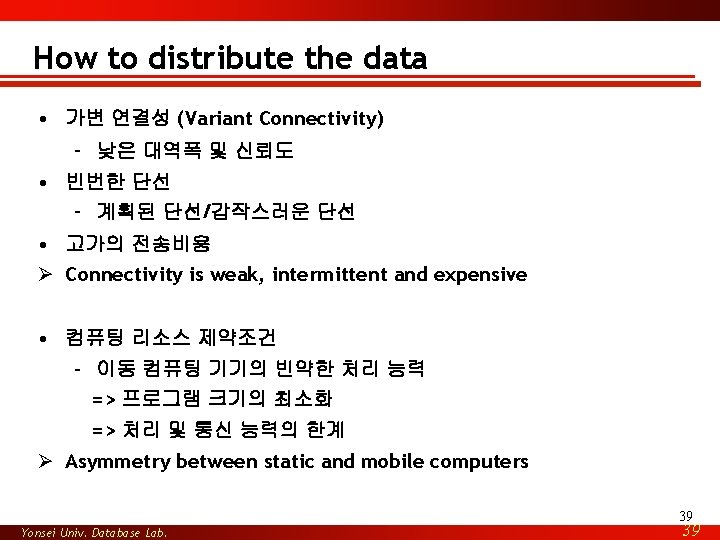
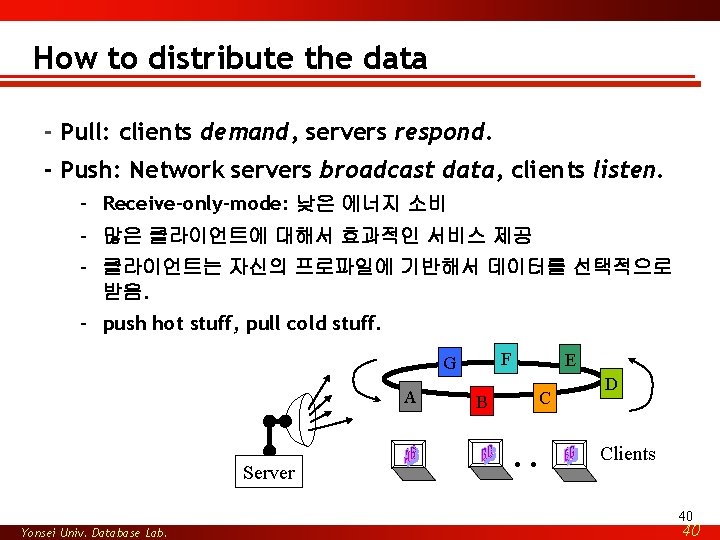
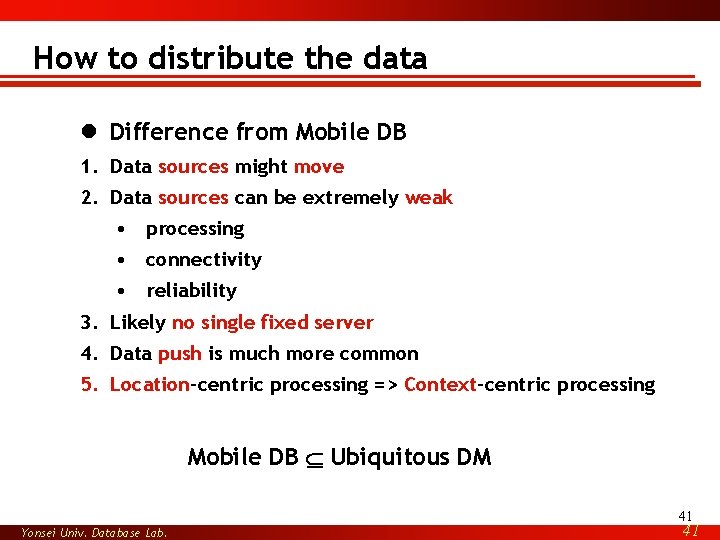
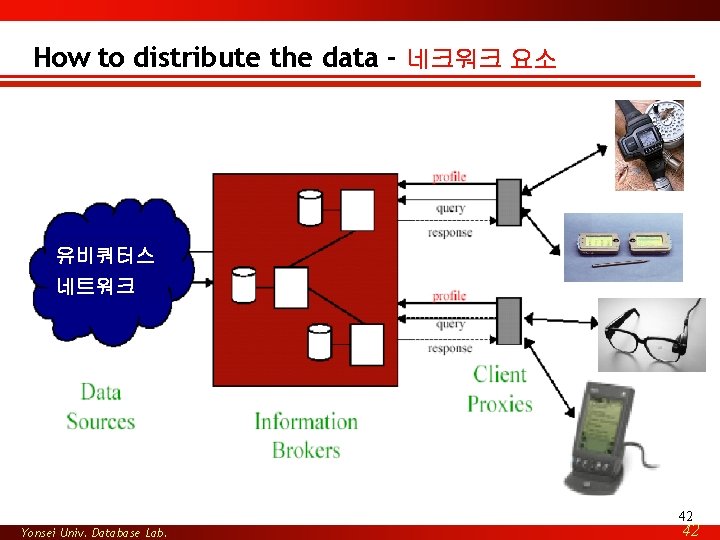
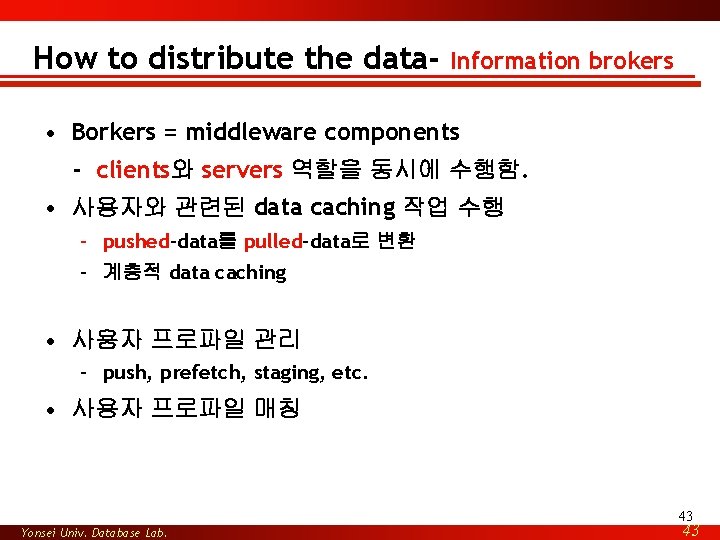
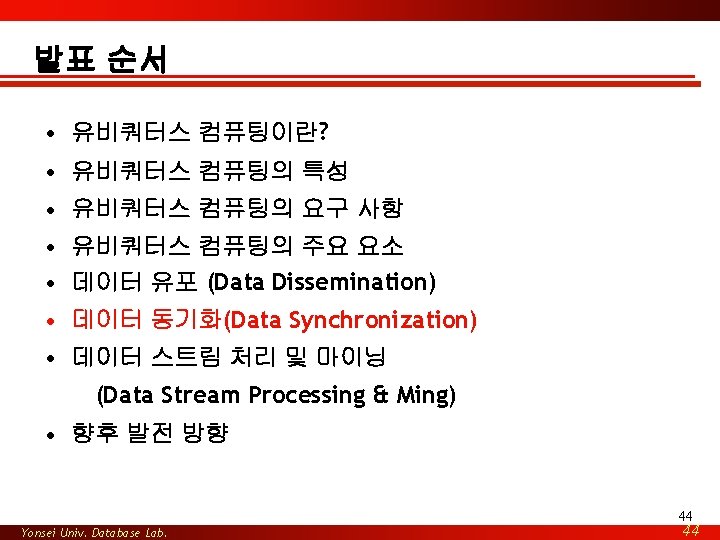
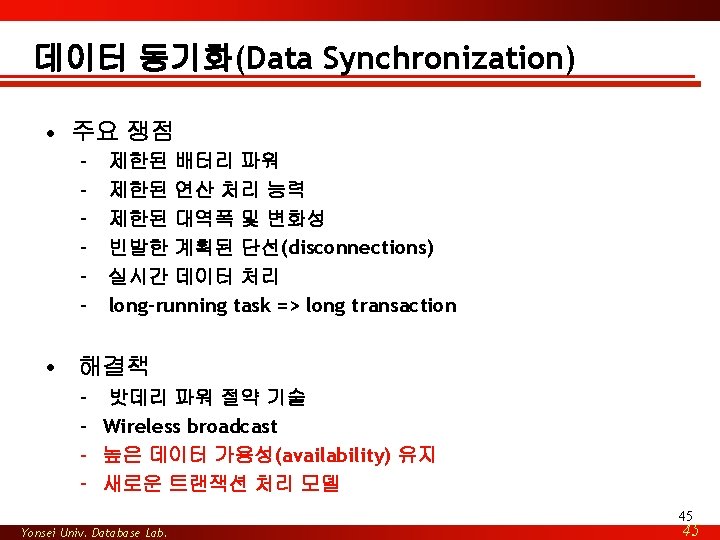
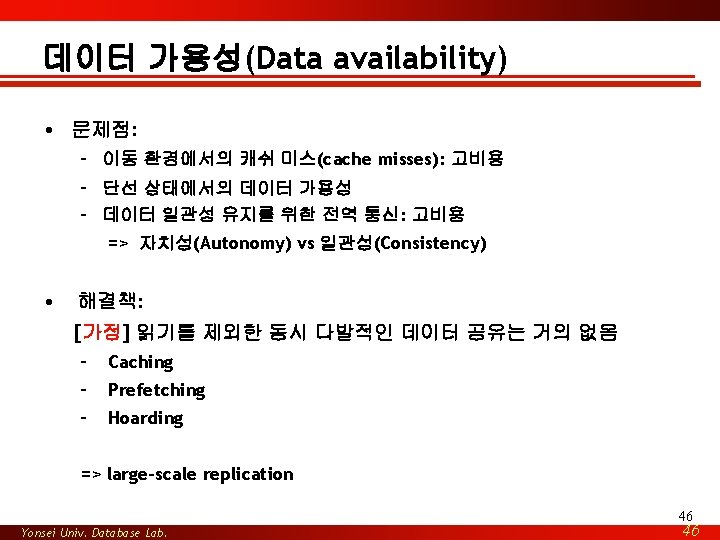
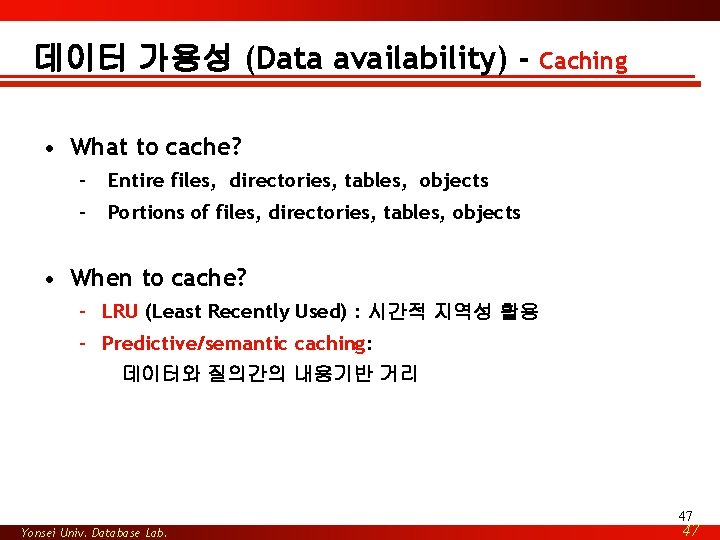
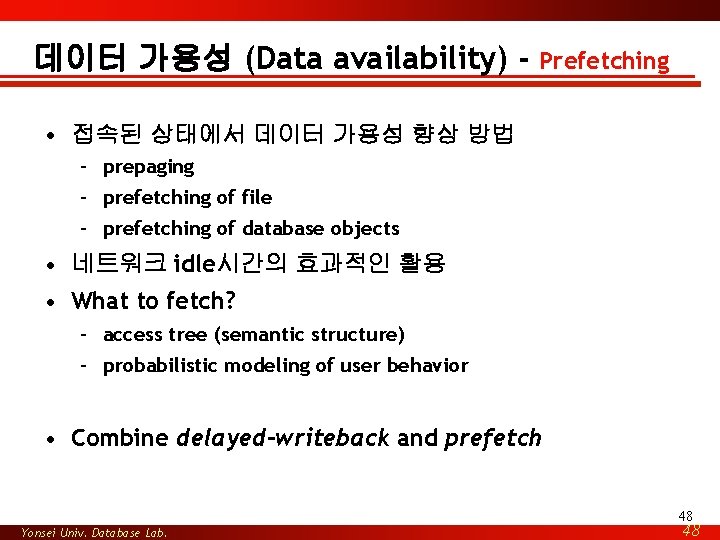
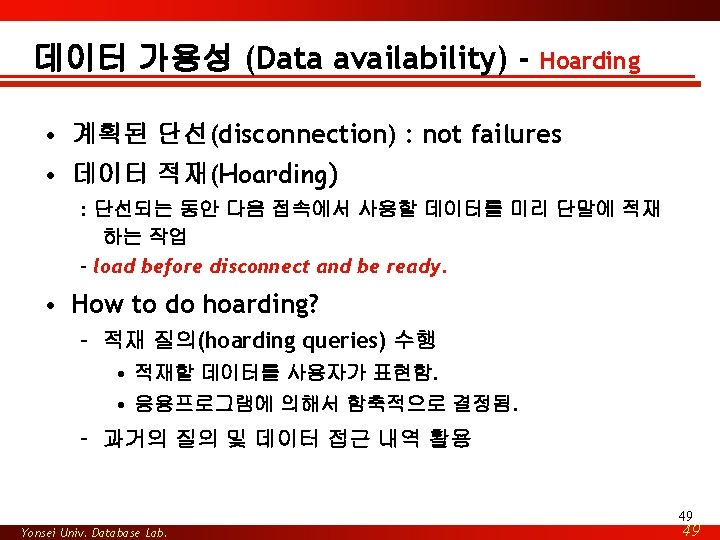
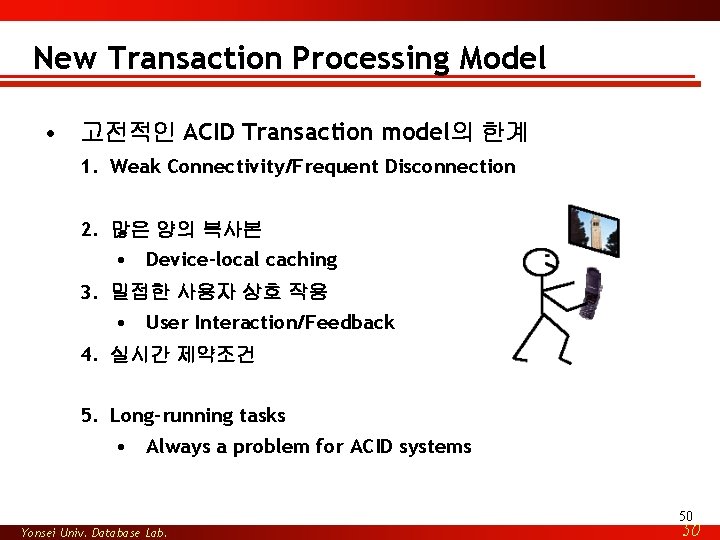
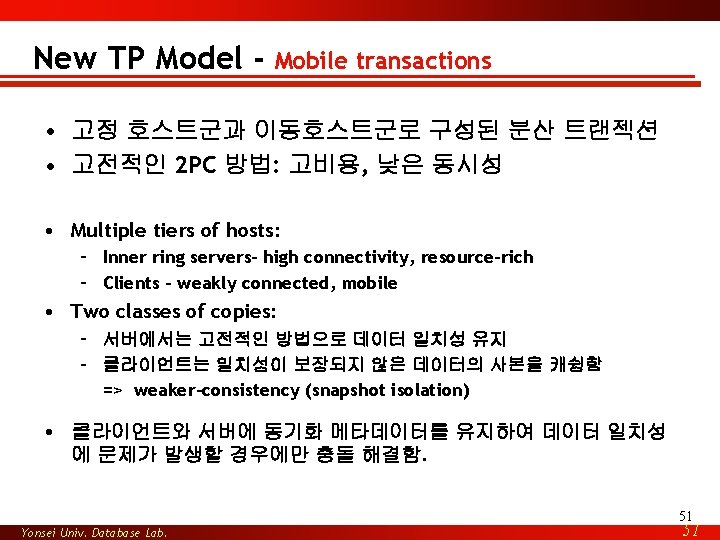
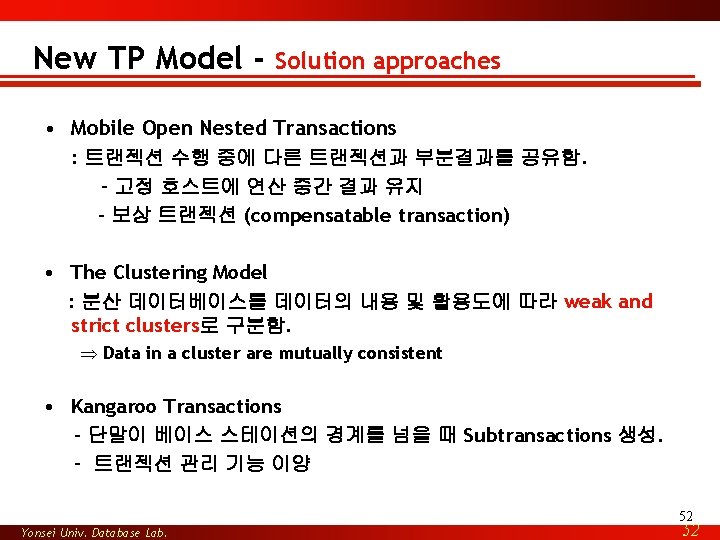
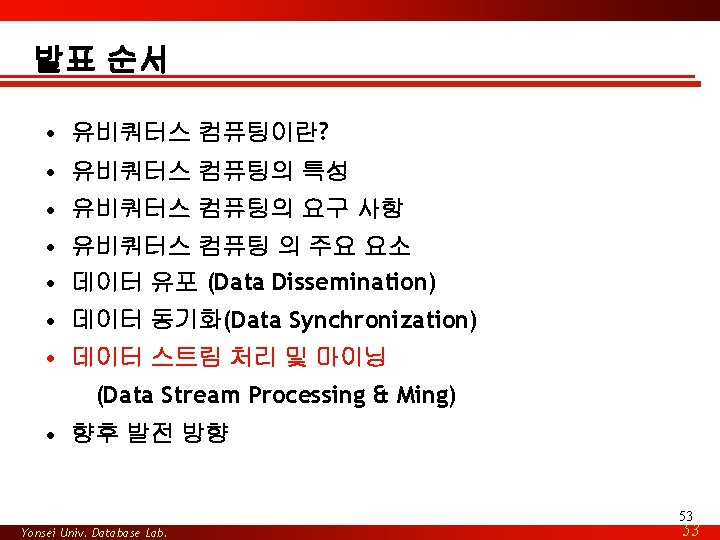
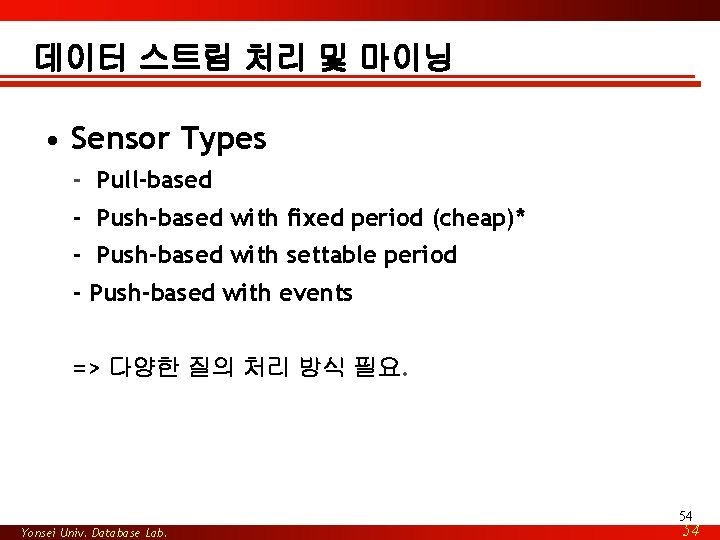
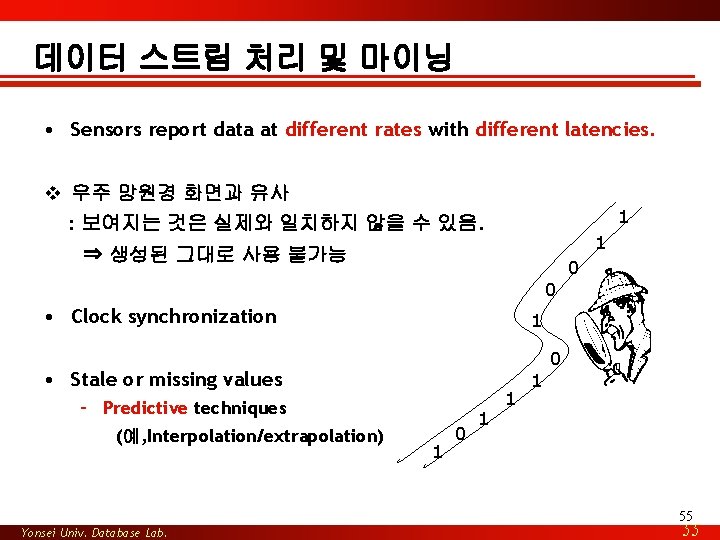
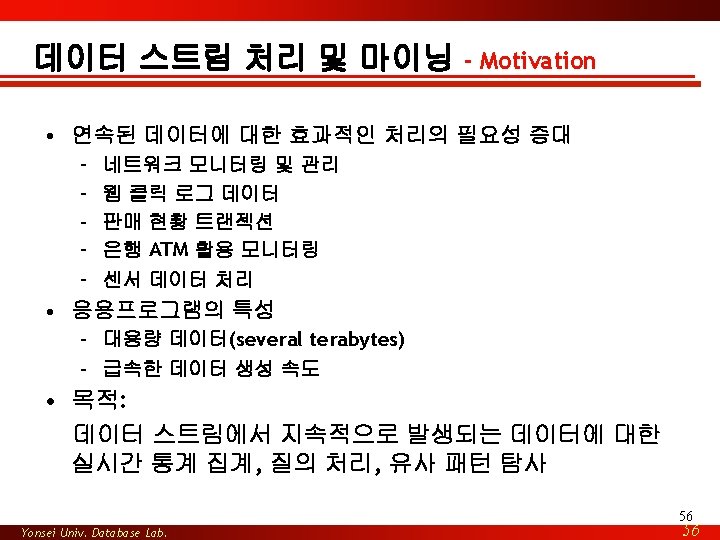
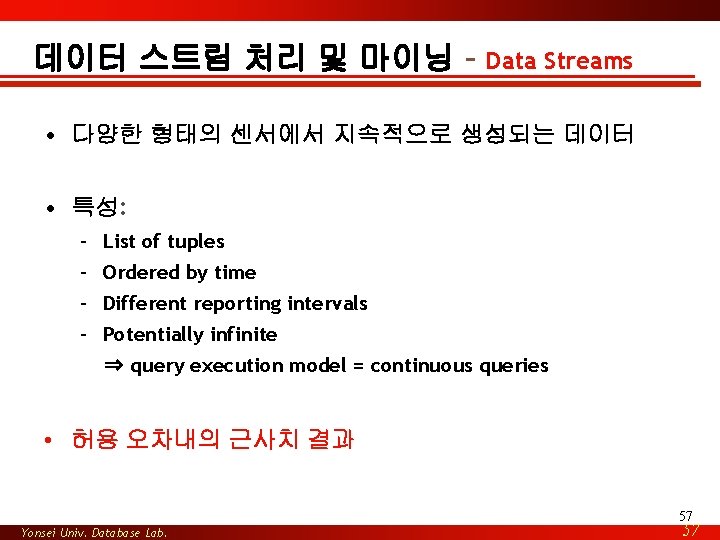
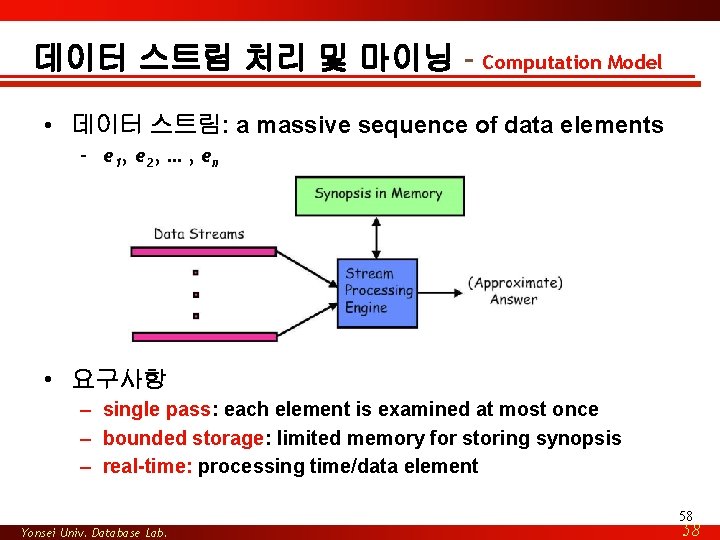
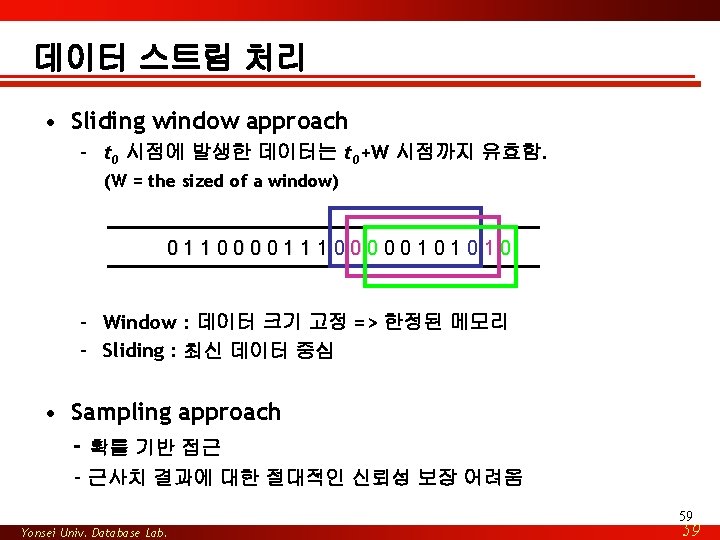
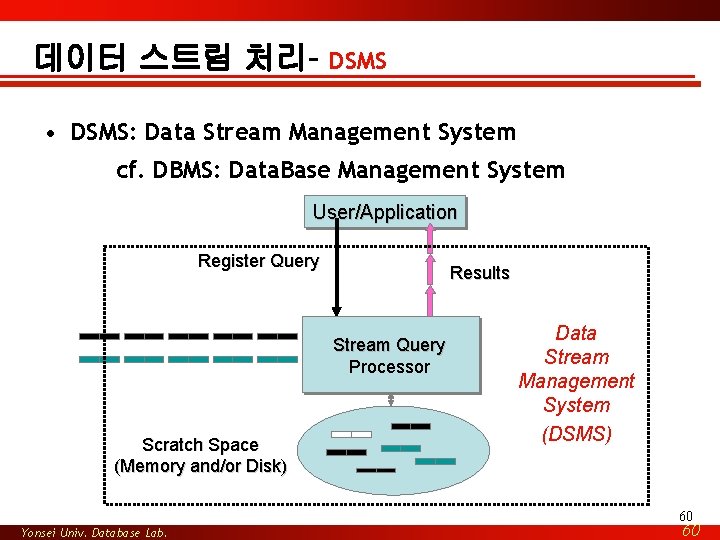
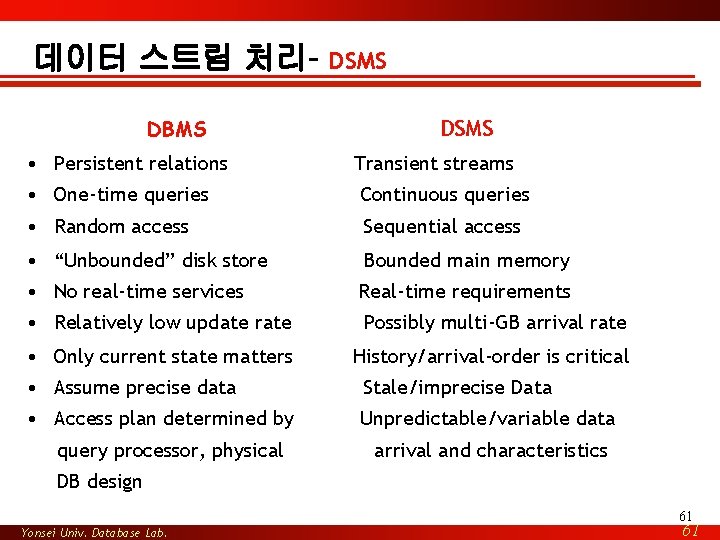
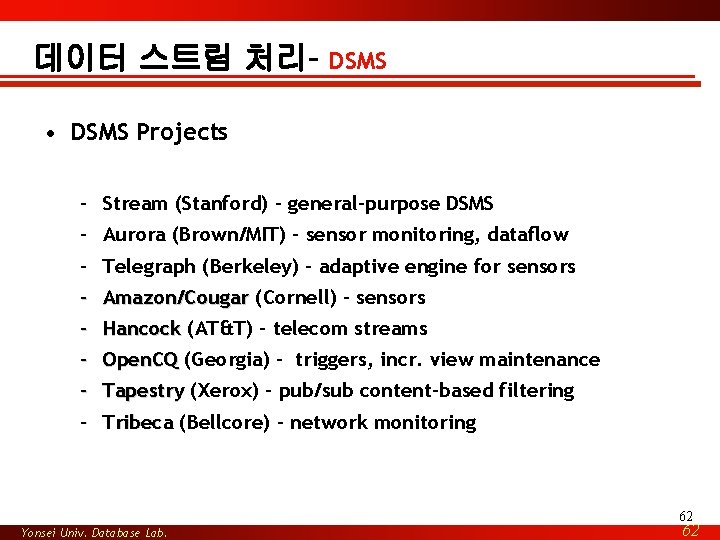
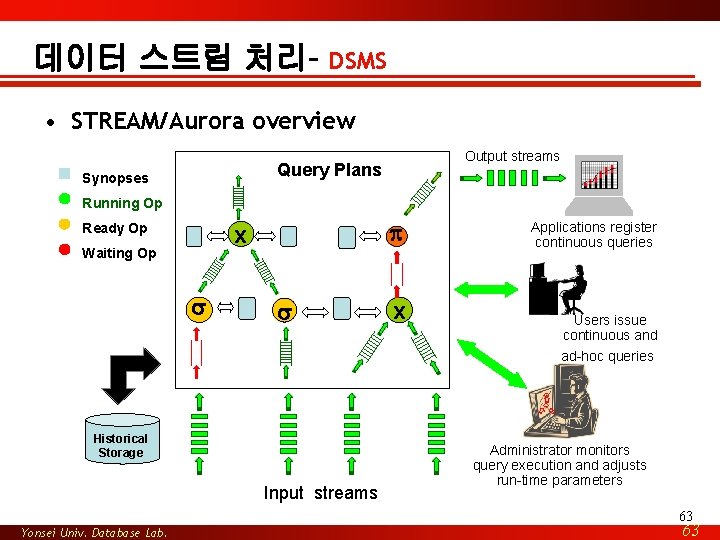
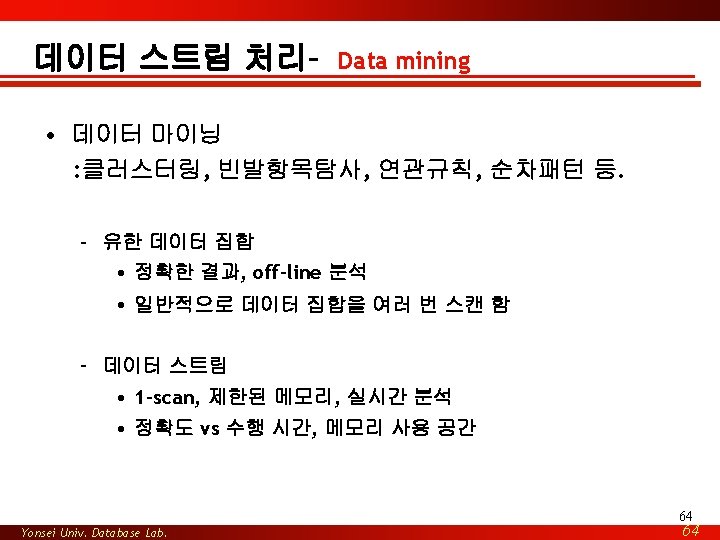
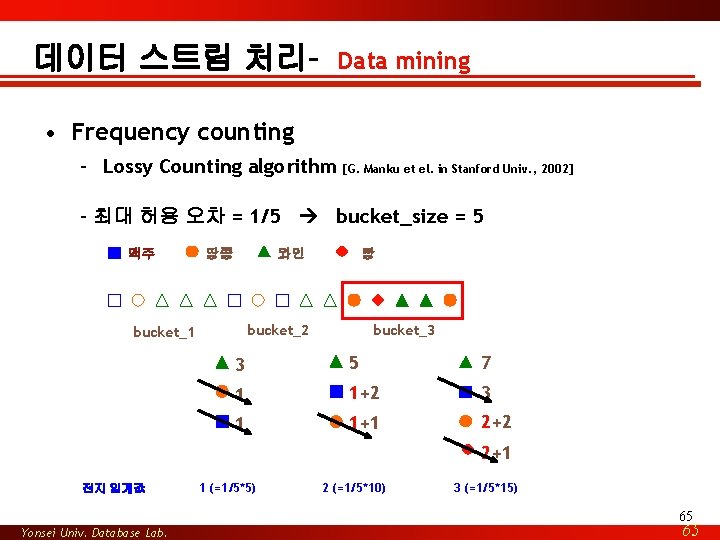
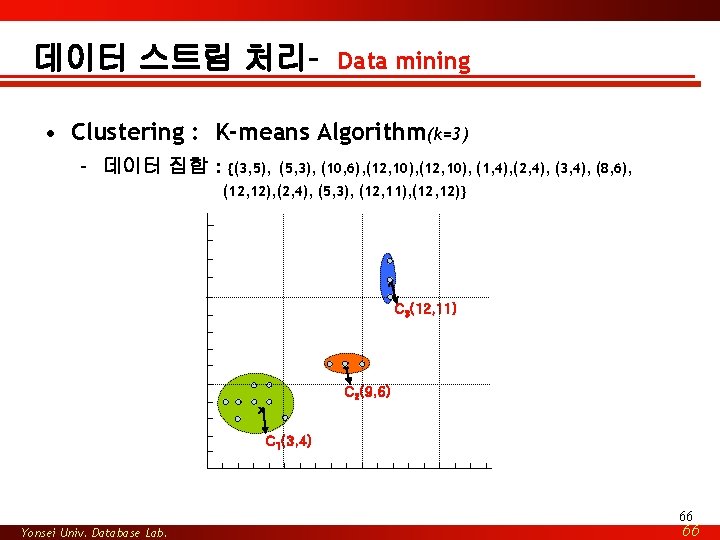
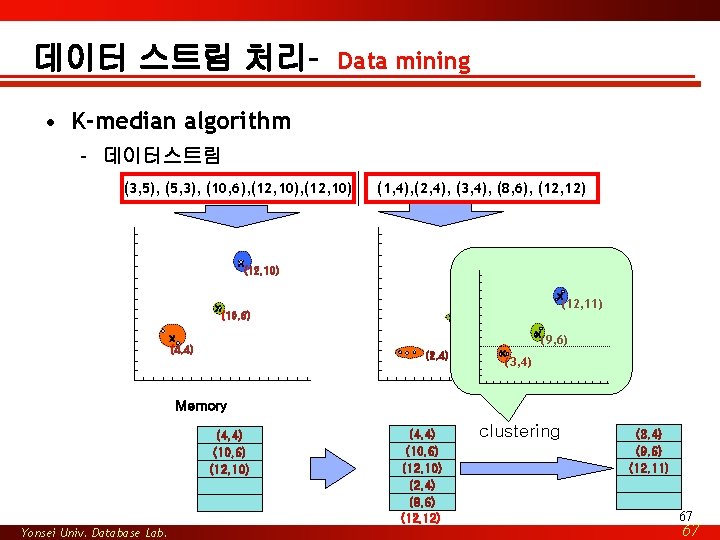
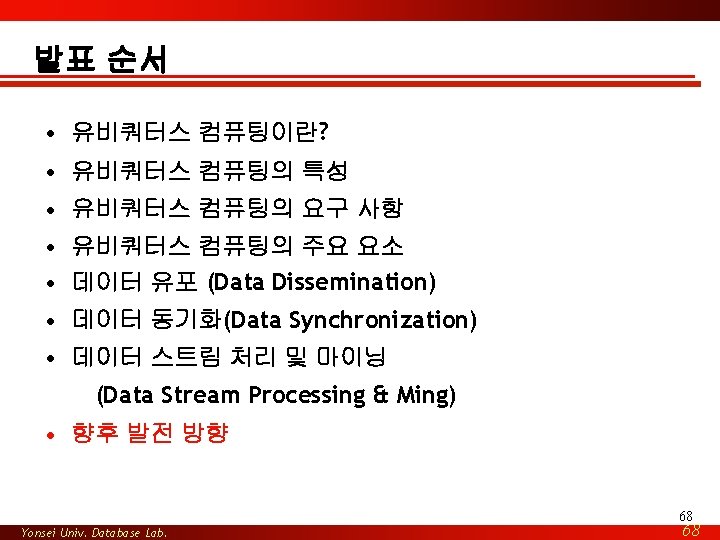
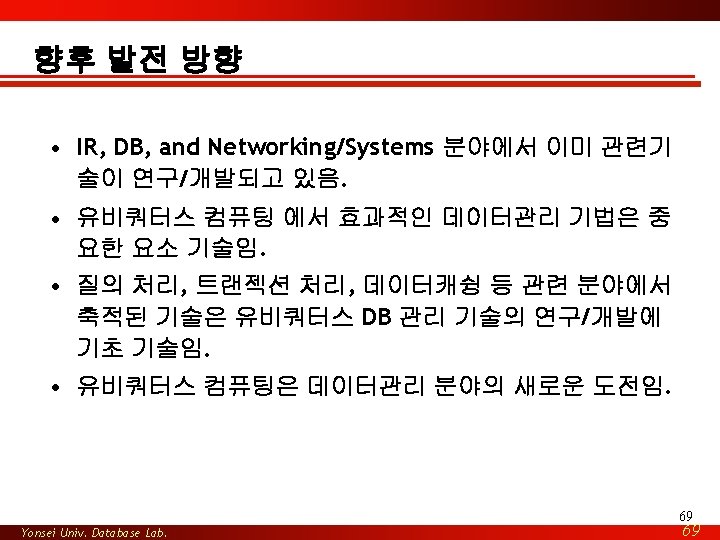
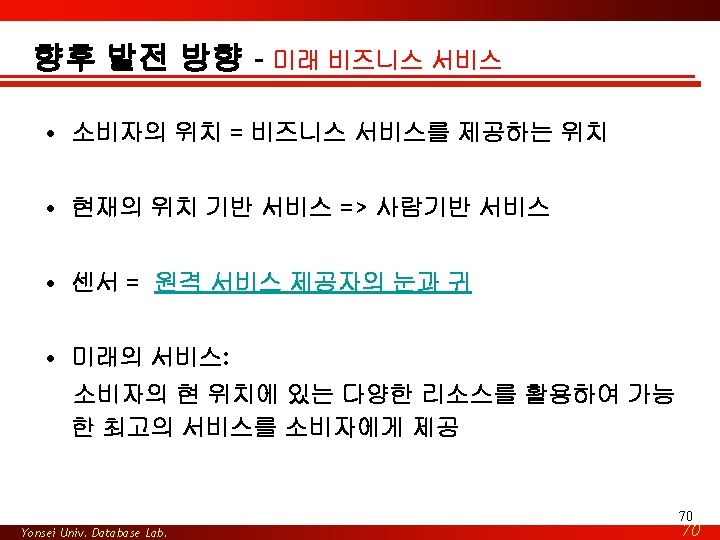
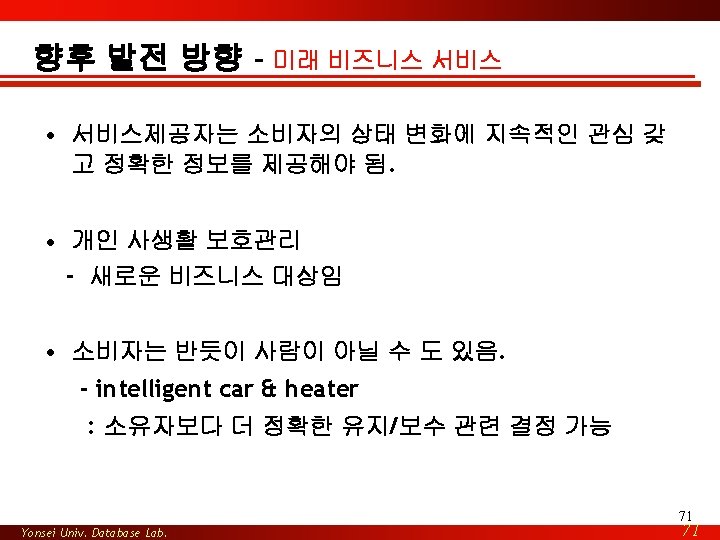
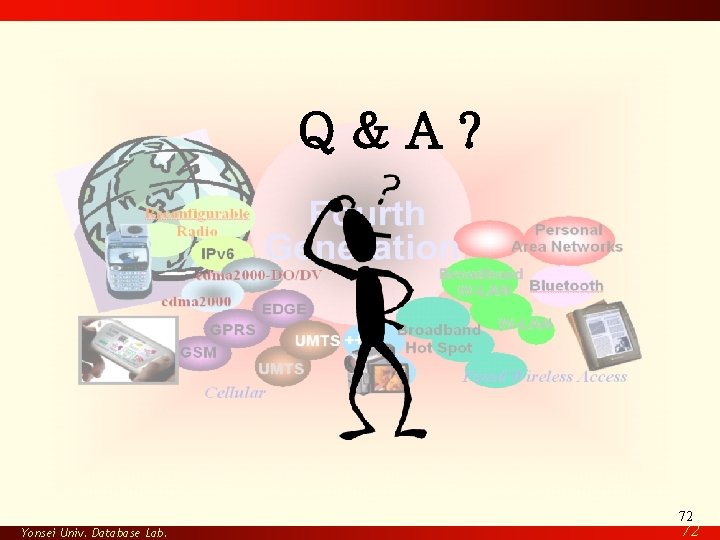
- Slides: 72
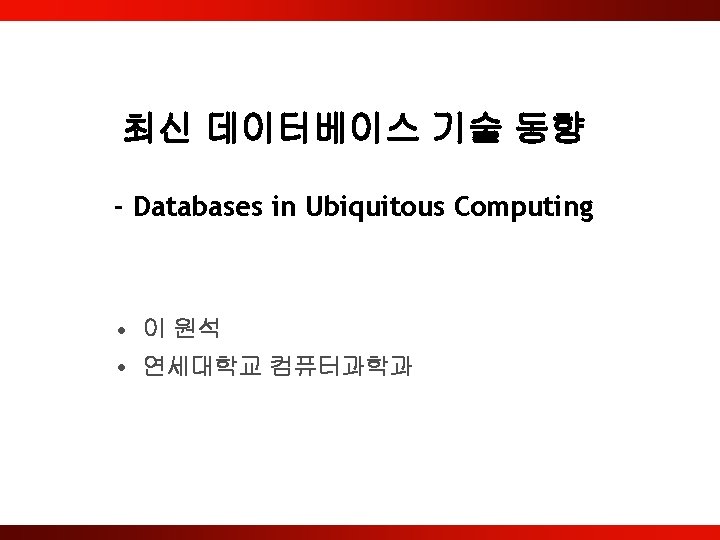
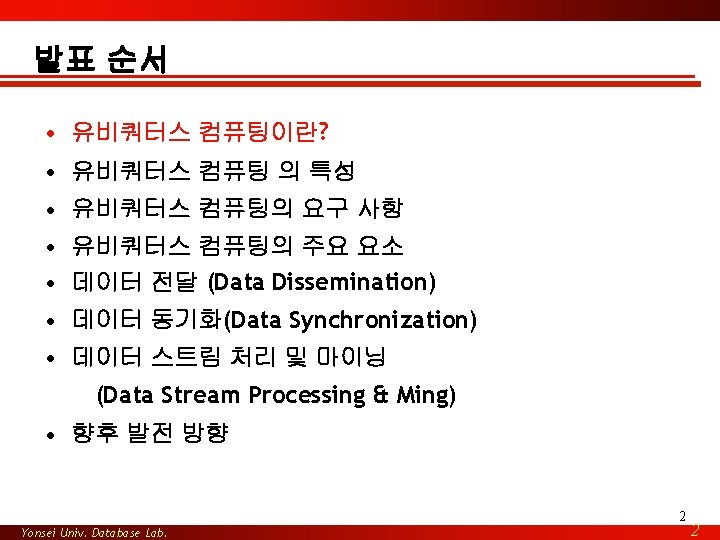
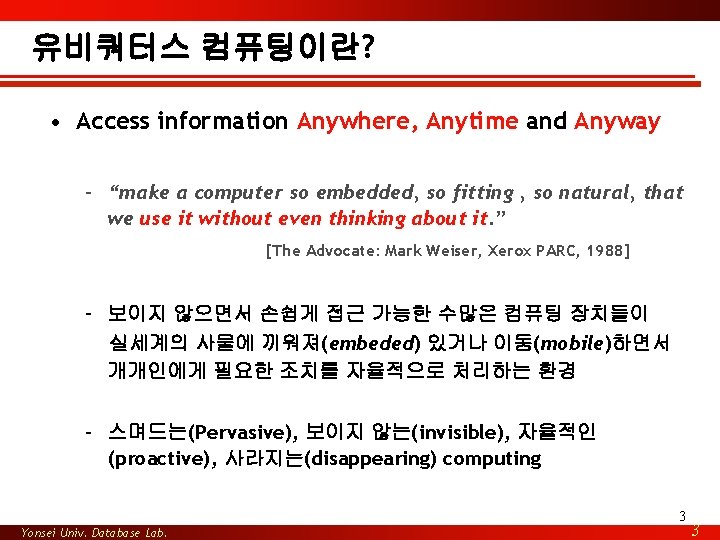
유비쿼터스 컴퓨팅이란? • Access information Anywhere, Anytime and Anyway – “make a computer so embedded, so fitting , so natural, that we use it without even thinking about it. ” [The Advocate: Mark Weiser, Xerox PARC, 1988] – 보이지 않으면서 손쉽게 접근 가능한 수많은 컴퓨팅 장치들이 실세계의 사물에 끼워져(embeded) 있거나 이동(mobile)하면서 개개인에게 필요한 조치를 자율적으로 처리하는 환경 – 스며드는(Pervasive), 보이지 않는(invisible), 자율적인 (proactive), 사라지는(disappearing) computing 3 Yonsei Univ. Database Lab. 3
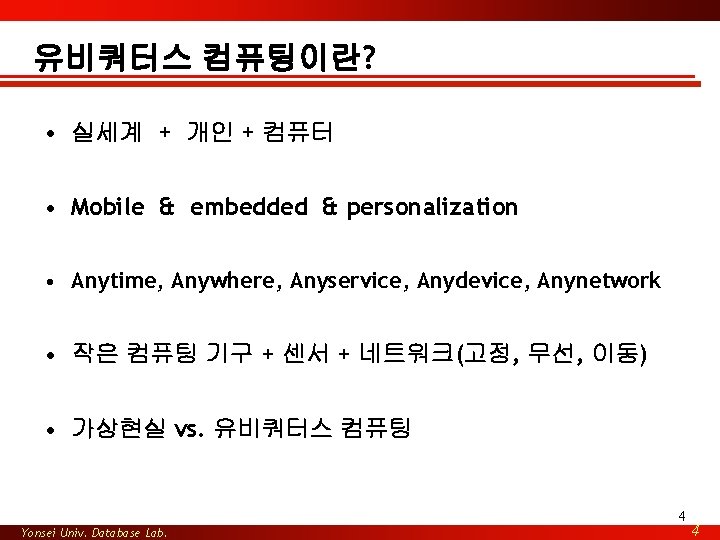
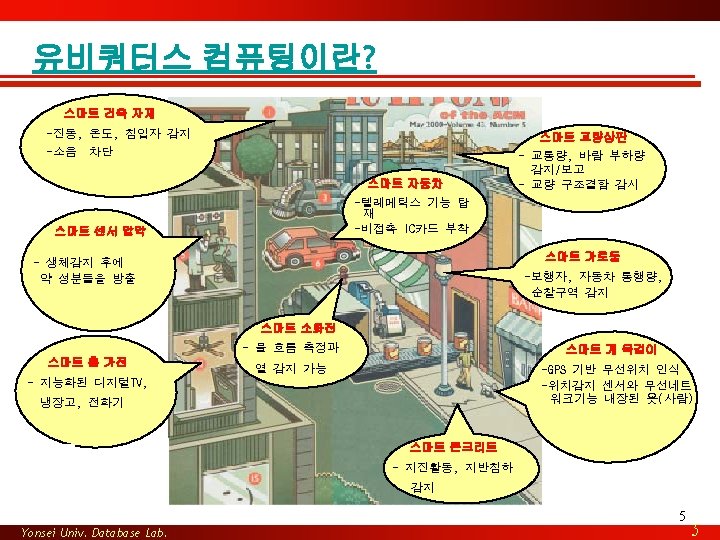
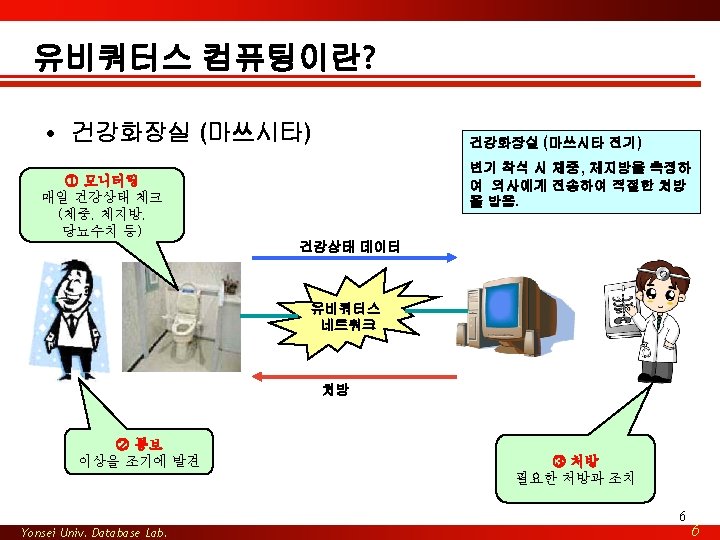
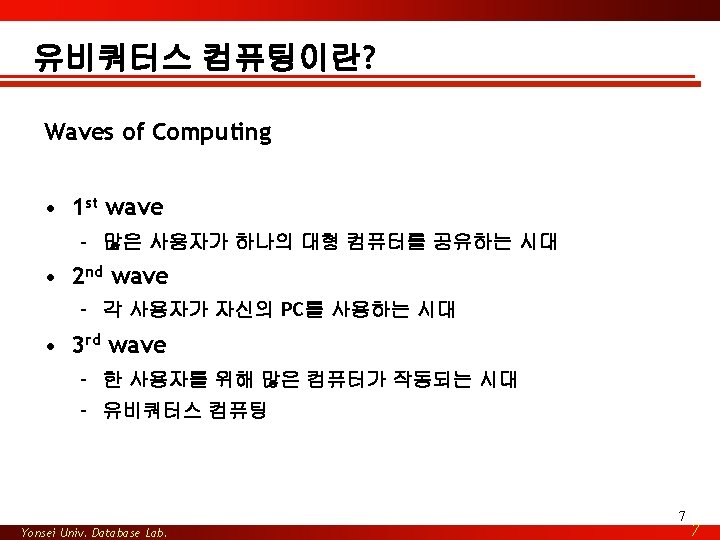
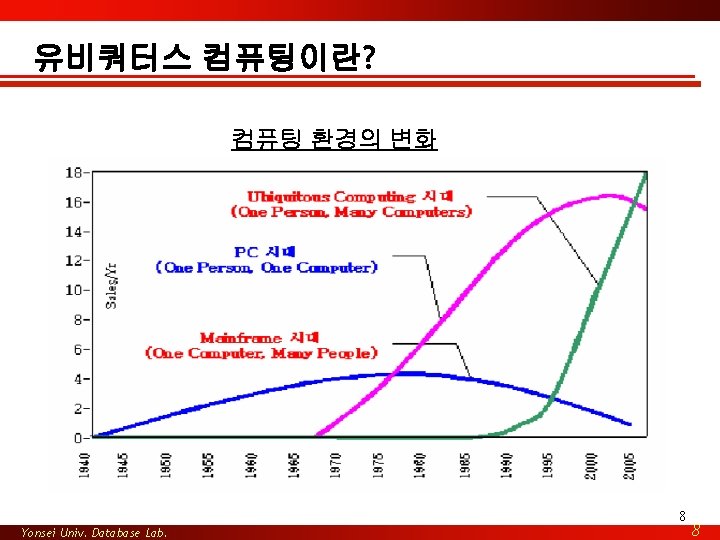
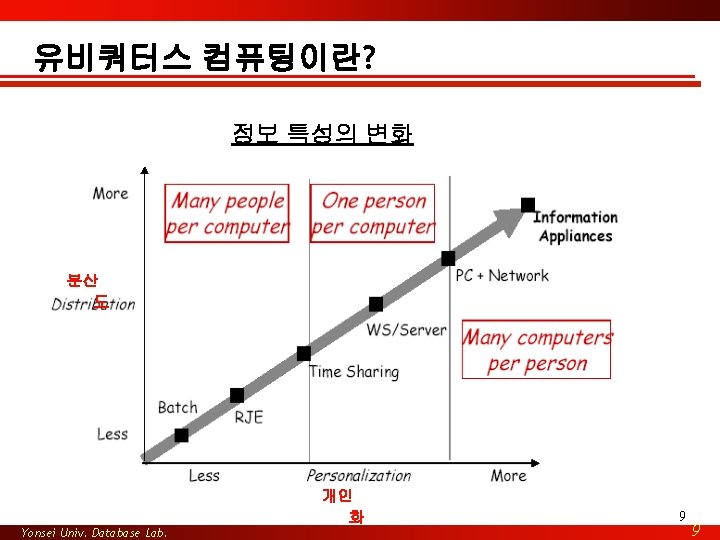
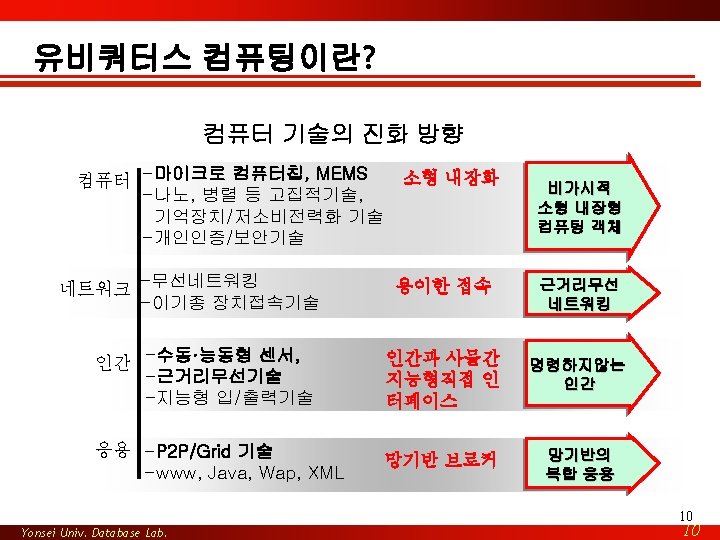
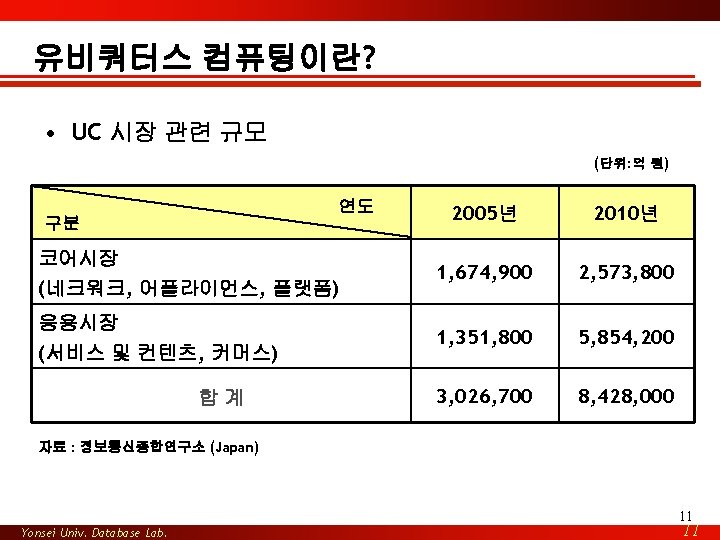
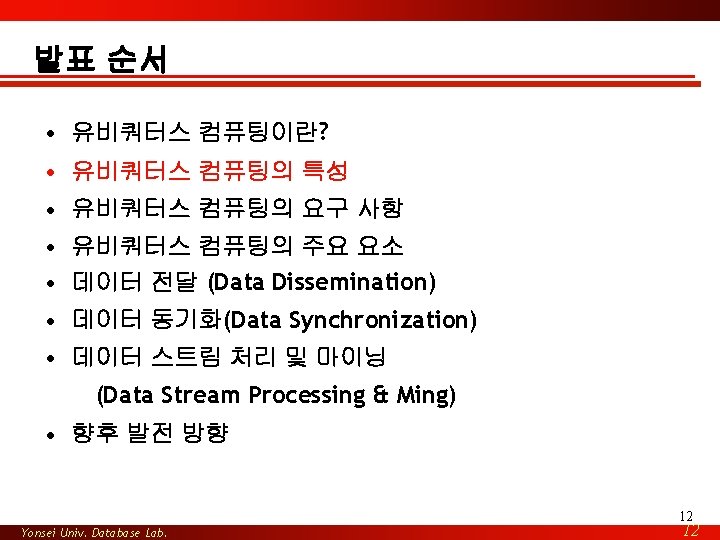
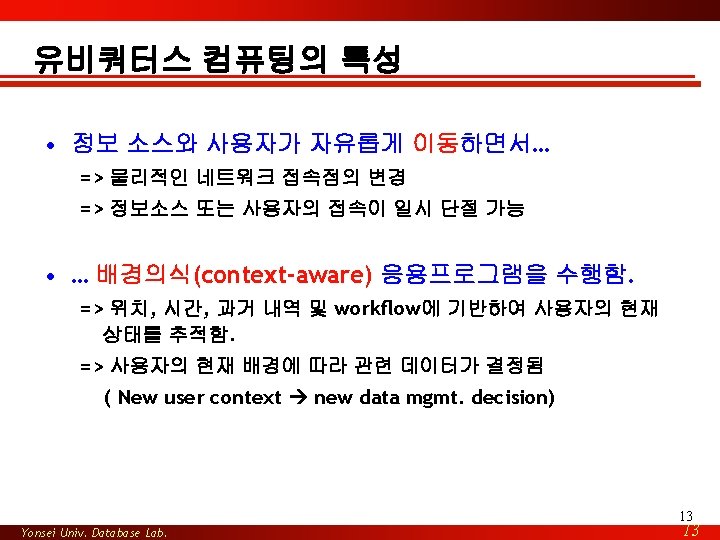
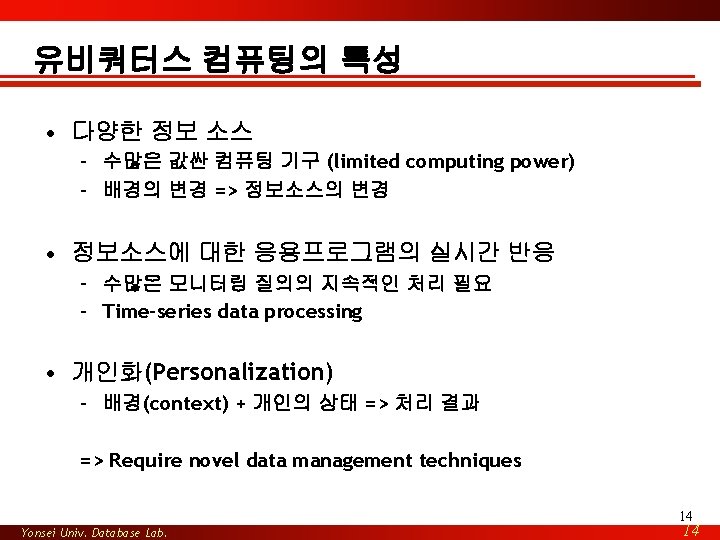
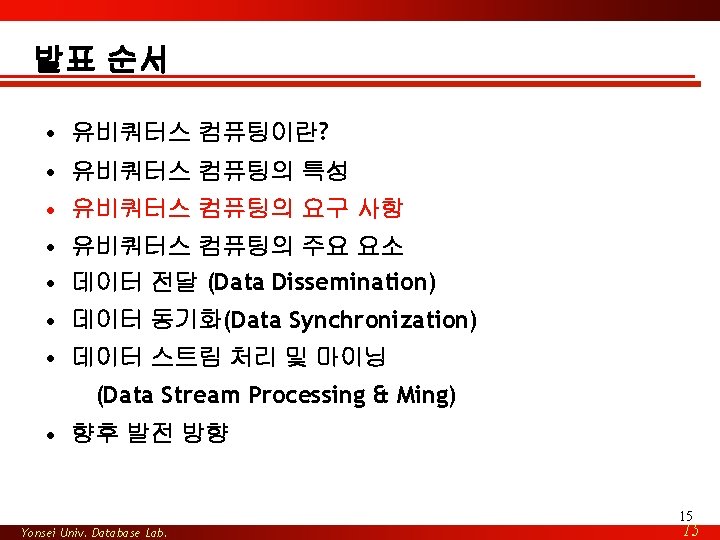
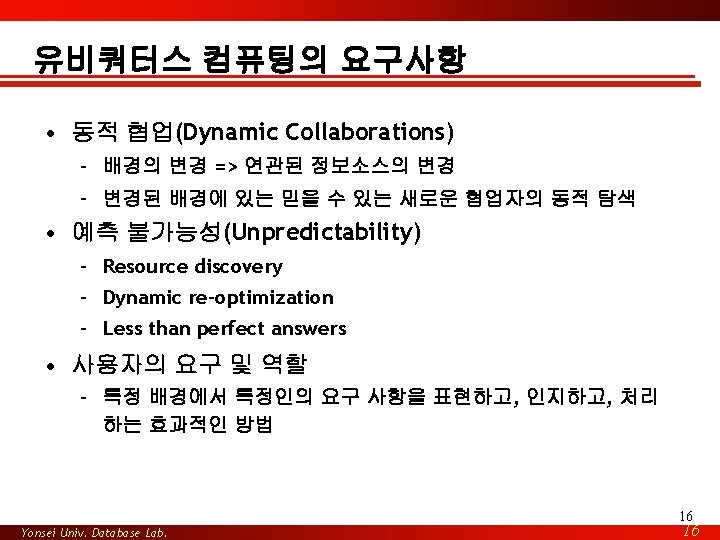
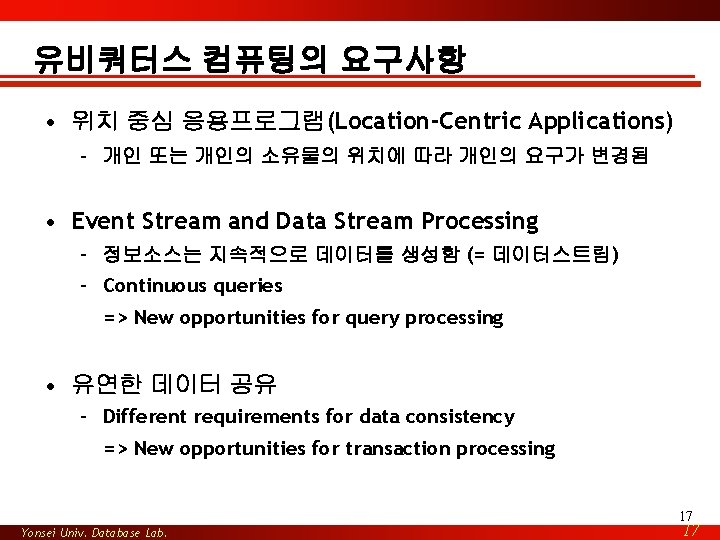
유비쿼터스 컴퓨팅의 요구사항 • 위치 중심 응용프로그램(Location-Centric Applications) – 개인 또는 개인의 소유물의 위치에 따라 개인의 요구가 변경됨 • Event Stream and Data Stream Processing – 정보소스는 지속적으로 데이터를 생성함 (= 데이터스트림) – Continuous queries => New opportunities for query processing • 유연한 데이터 공유 – Different requirements for data consistency => New opportunities for transaction processing 17 Yonsei Univ. Database Lab. 17
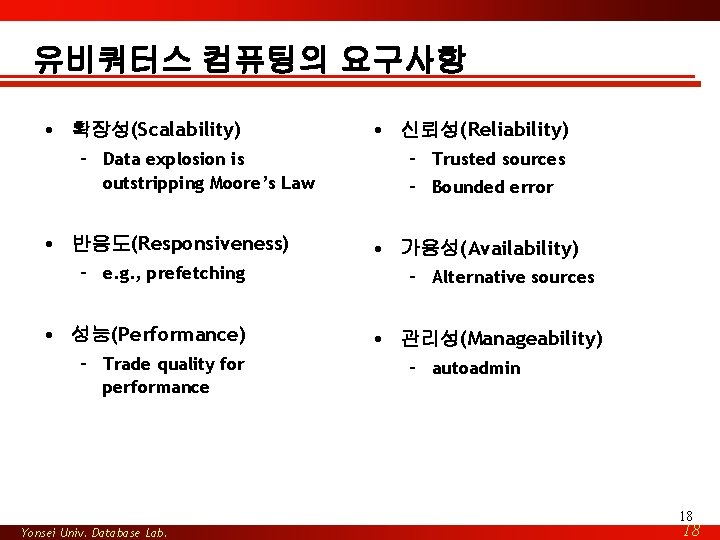
유비쿼터스 컴퓨팅의 요구사항 • 확장성(Scalability) – Data explosion is outstripping Moore’s Law • 반응도(Responsiveness) – e. g. , prefetching • 성능(Performance) – Trade quality for performance • 신뢰성(Reliability) – Trusted sources – Bounded error • 가용성(Availability) – Alternative sources • 관리성(Manageability) – autoadmin 18 Yonsei Univ. Database Lab. 18
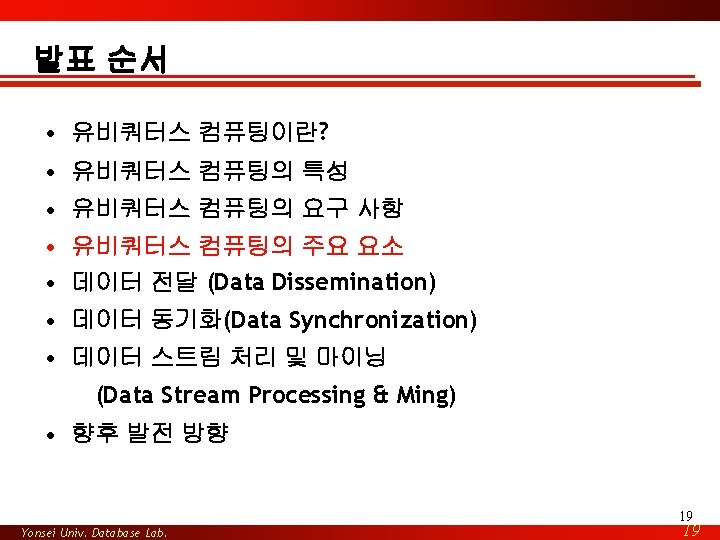
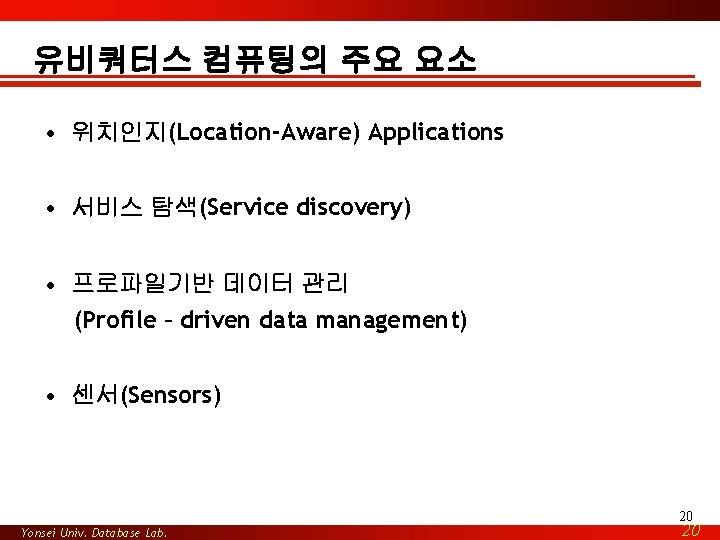
유비쿼터스 컴퓨팅의 주요 요소 • 위치인지(Location-Aware) Applications • 서비스 탐색(Service discovery) • 프로파일기반 데이터 관리 (Profile – driven data management) • 센서(Sensors) 20 Yonsei Univ. Database Lab. 20
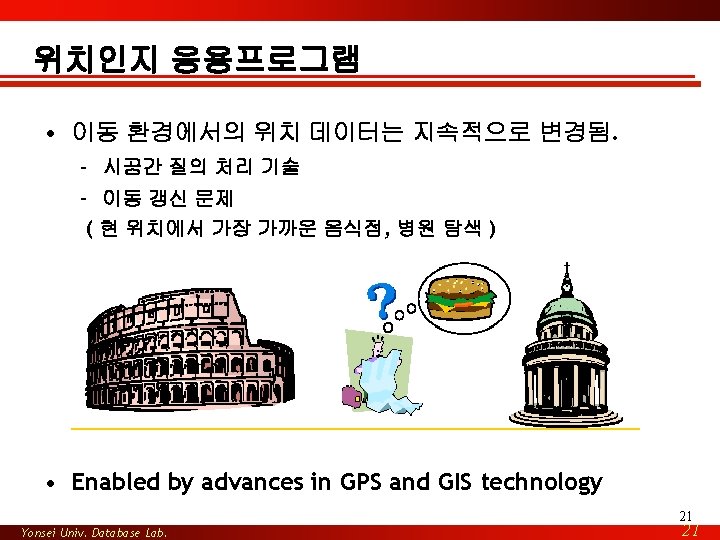
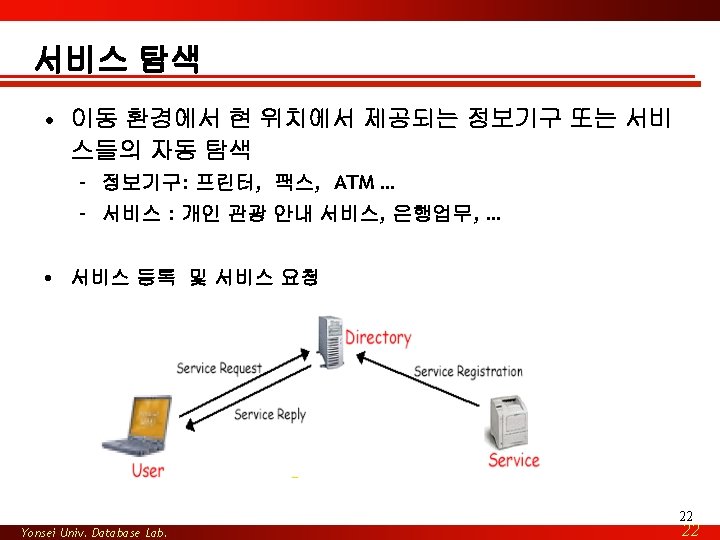
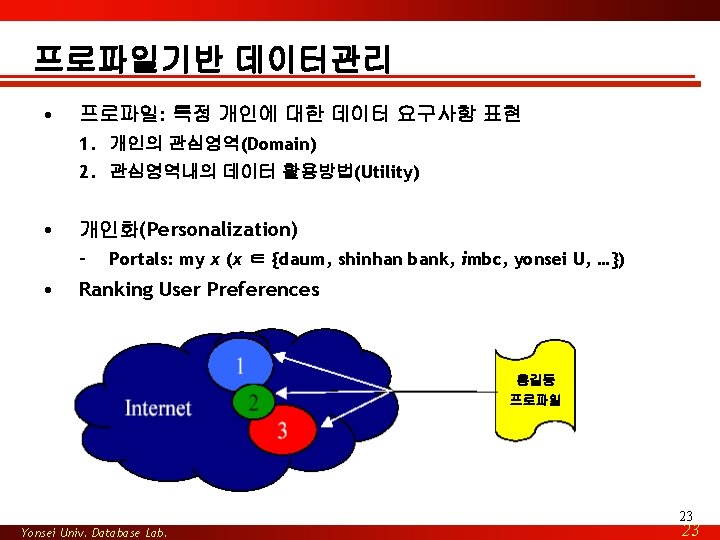
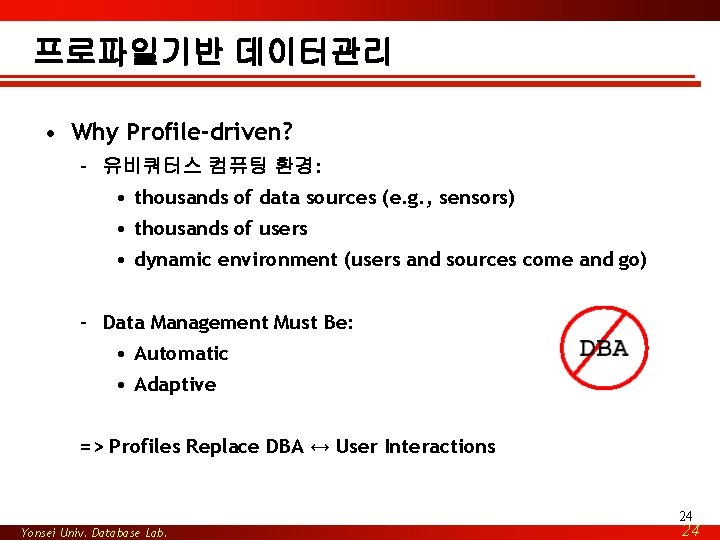
프로파일기반 데이터관리 • Why Profile-driven? – 유비쿼터스 컴퓨팅 환경: • thousands of data sources (e. g. , sensors) • thousands of users • dynamic environment (users and sources come and go) – Data Management Must Be: • Automatic • Adaptive => Profiles Replace DBA ↔ User Interactions 24 Yonsei Univ. Database Lab. 24
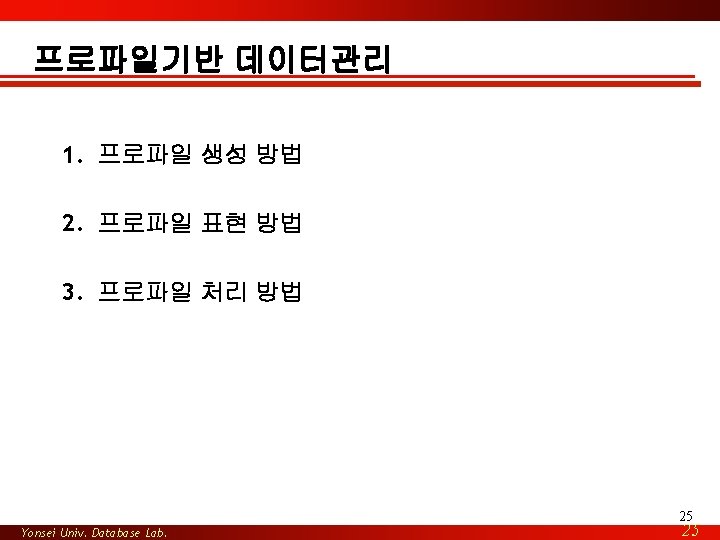
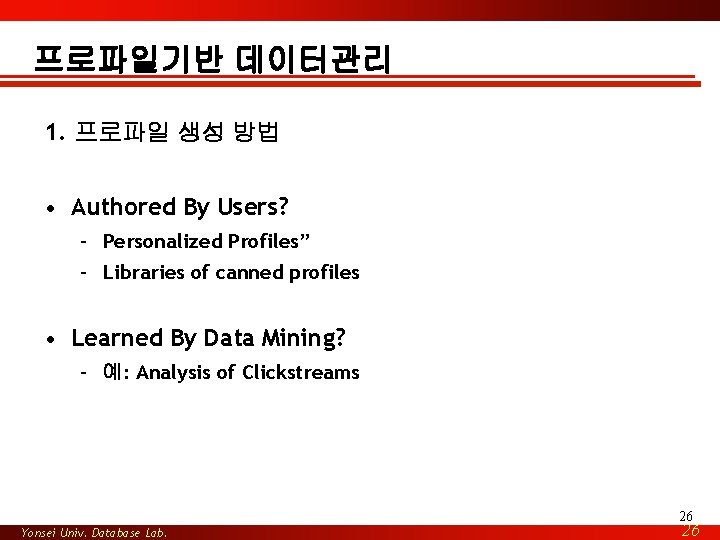
프로파일기반 데이터관리 1. 프로파일 생성 방법 • Authored By Users? – Personalized Profiles” – Libraries of canned profiles • Learned By Data Mining? – 예: Analysis of Clickstreams 26 Yonsei Univ. Database Lab. 26
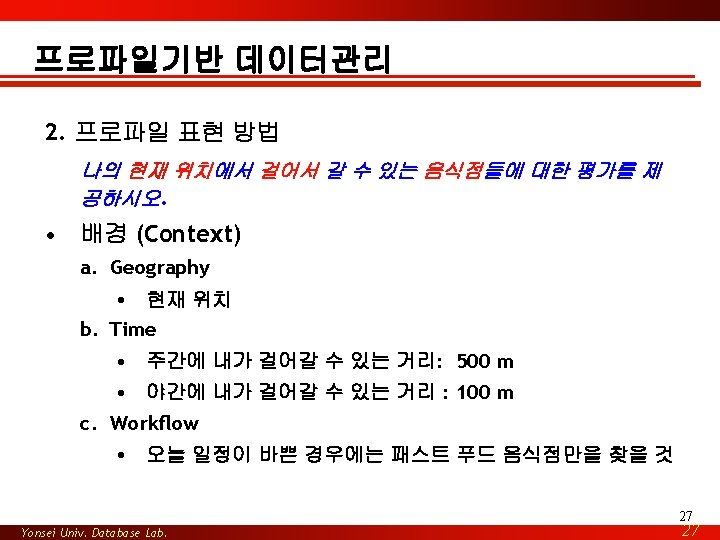
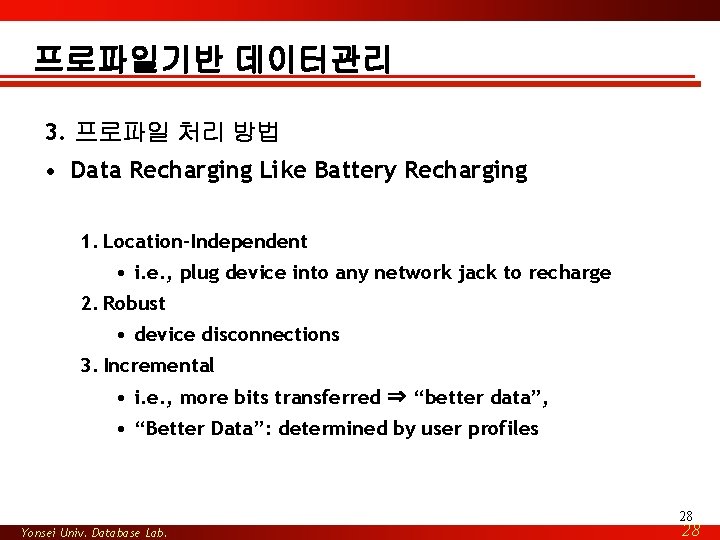
프로파일기반 데이터관리 3. 프로파일 처리 방법 • Data Recharging Like Battery Recharging 1. Location-Independent • i. e. , plug device into any network jack to recharge 2. Robust • device disconnections 3. Incremental • i. e. , more bits transferred ⇒ “better data”, • “Better Data”: determined by user profiles 28 Yonsei Univ. Database Lab. 28
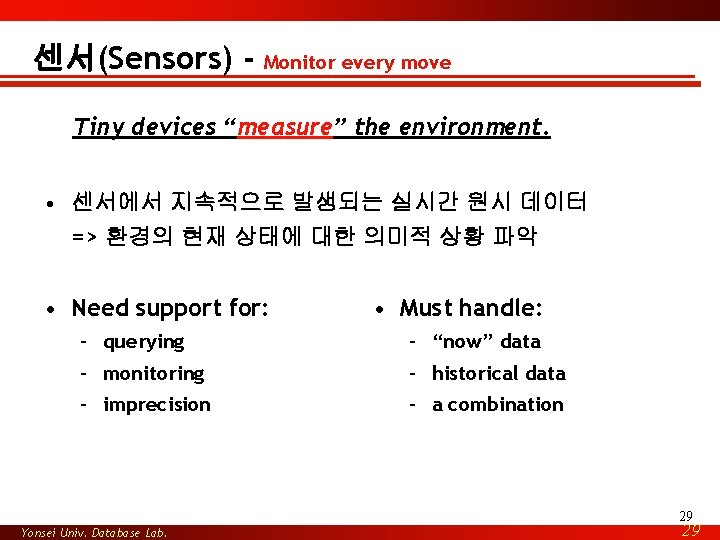
센서(Sensors) - Monitor every move Tiny devices “measure” the environment. • 센서에서 지속적으로 발생되는 실시간 원시 데이터 => 환경의 현재 상태에 대한 의미적 상황 파악 • Need support for: • Must handle: – querying – “now” data – monitoring – historical data – imprecision – a combination 29 Yonsei Univ. Database Lab. 29
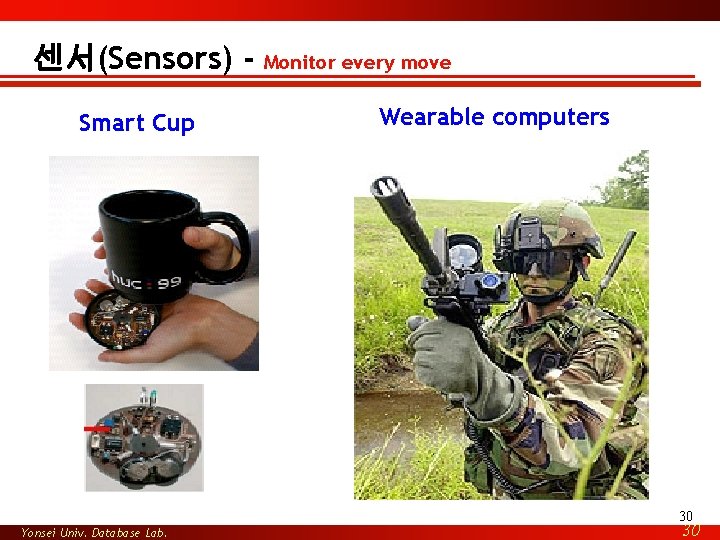
센서(Sensors) Smart Cup Monitor every move Wearable computers 30 Yonsei Univ. Database Lab. 30
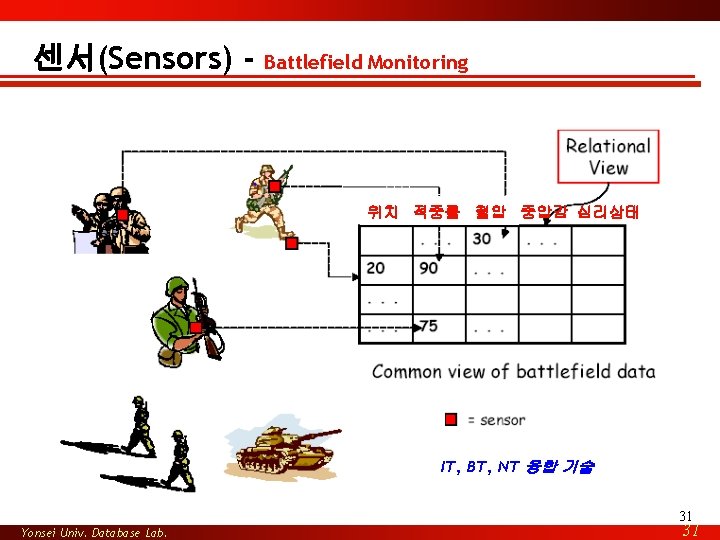
센서(Sensors) - Battlefield Monitoring 위치 적중률 혈압 중압감 심리상태 IT, BT, NT 융합 기술 31 Yonsei Univ. Database Lab. 31
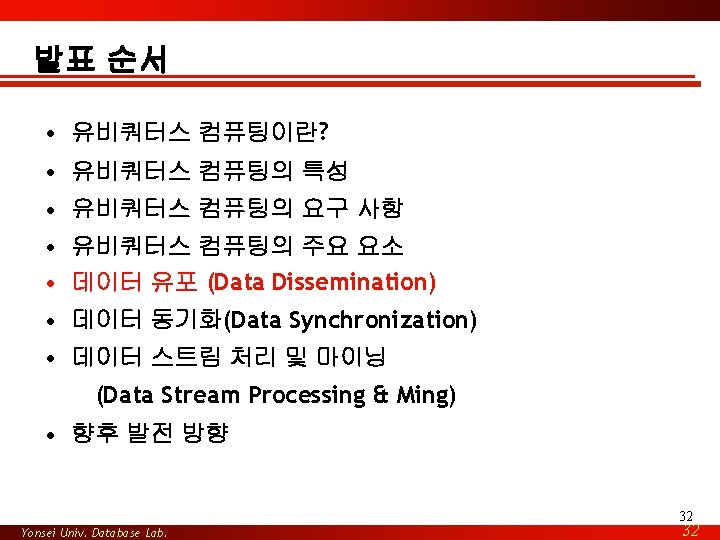
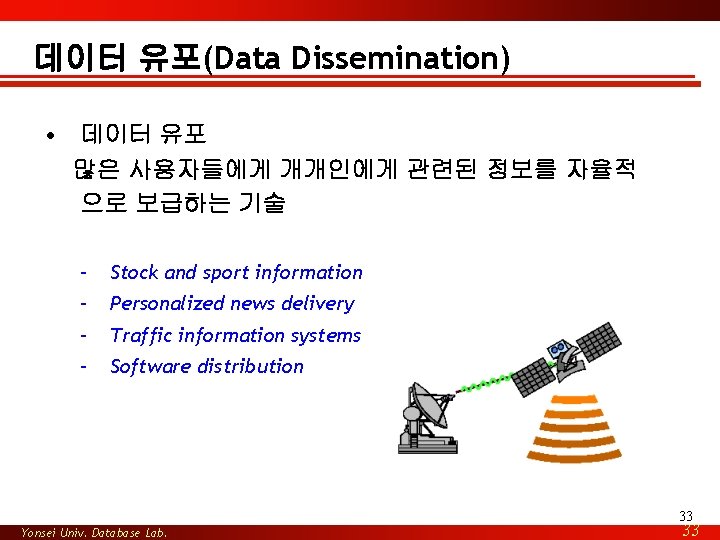
데이터 유포(Data Dissemination) • 데이터 유포 많은 사용자들에게 개개인에게 관련된 정보를 자율적 으로 보급하는 기술 – Stock and sport information – Personalized news delivery – Traffic information systems – Software distribution 33 Yonsei Univ. Database Lab. 33
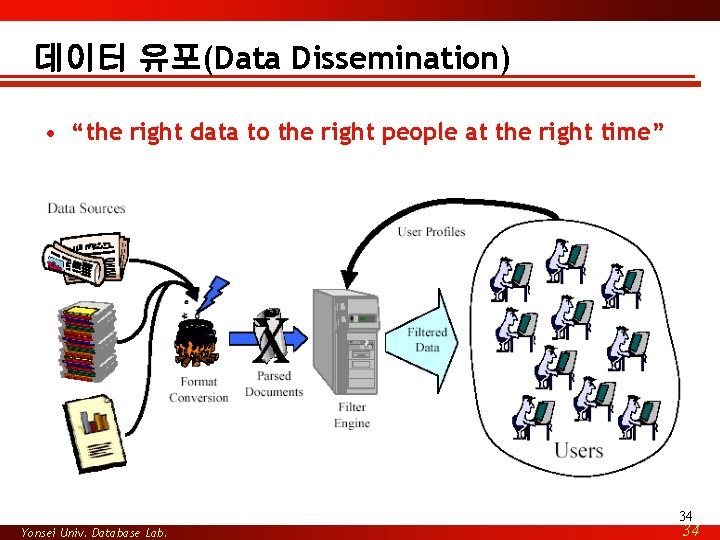
데이터 유포(Data Dissemination) • “the right data to the right people at the right time” 34 Yonsei Univ. Database Lab. 34
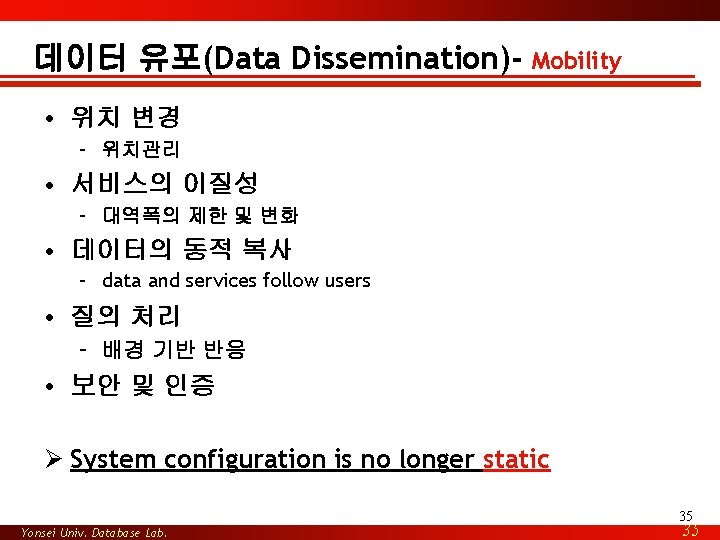
데이터 유포(Data Dissemination)- Mobility • 위치 변경 – 위치관리 • 서비스의 이질성 – 대역폭의 제한 및 변화 • 데이터의 동적 복사 – data and services follow users • 질의 처리 – 배경 기반 반응 • 보안 및 인증 Ø System configuration is no longer static 35 Yonsei Univ. Database Lab. 35
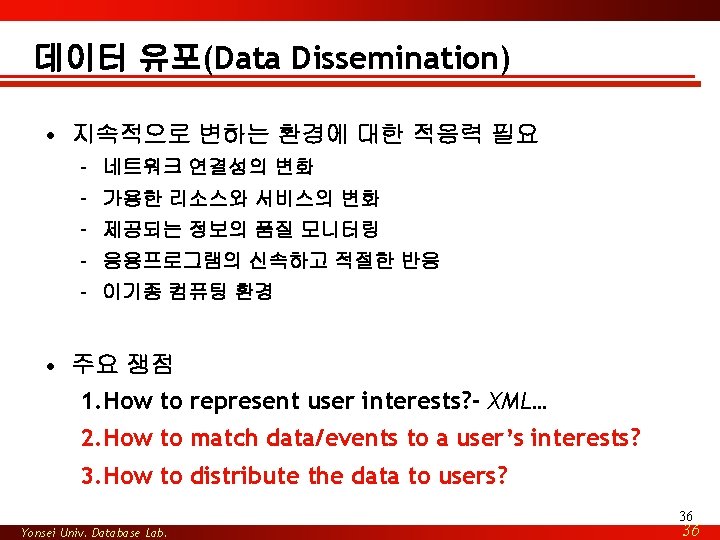
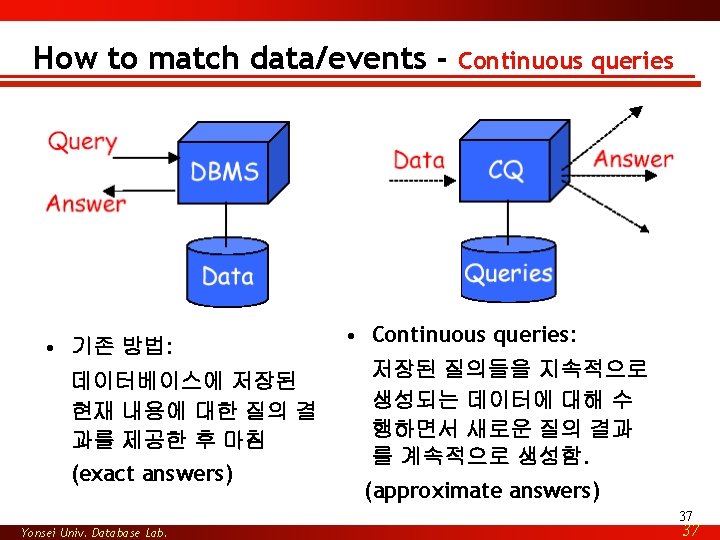
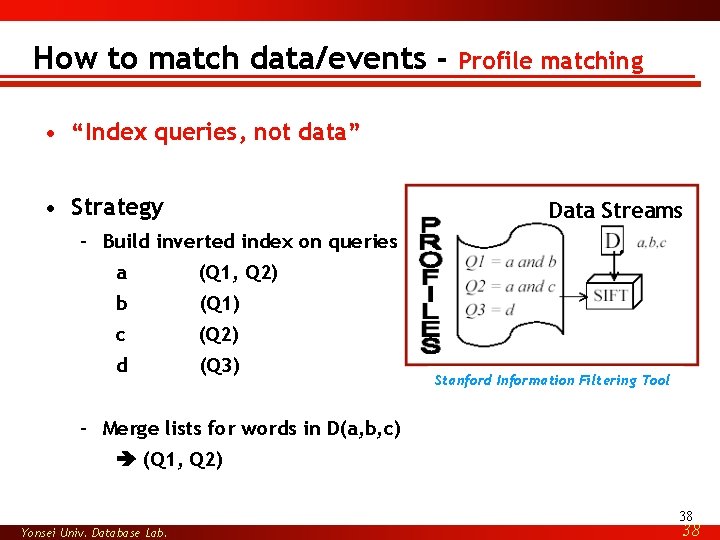
How to match data/events - Profile matching • “Index queries, not data” • Strategy Data Streams – Build inverted index on queries a (Q 1, Q 2) b (Q 1) c (Q 2) d (Q 3) Stanford Information Filtering Tool – Merge lists for words in D(a, b, c) (Q 1, Q 2) 38 Yonsei Univ. Database Lab. 38
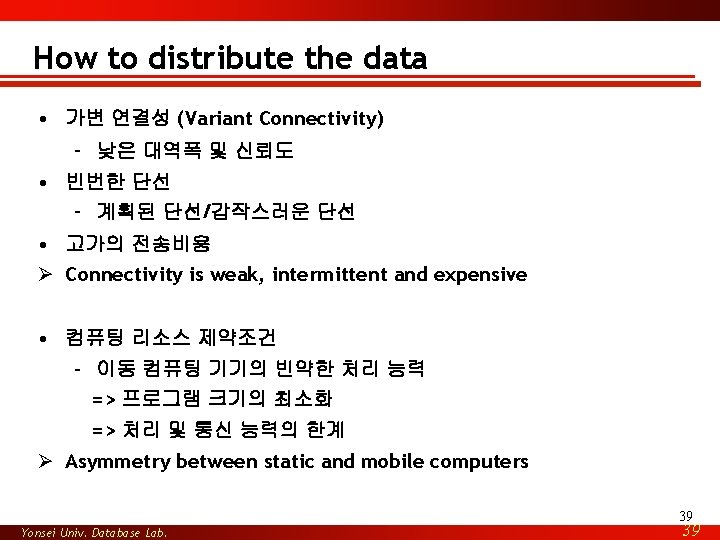
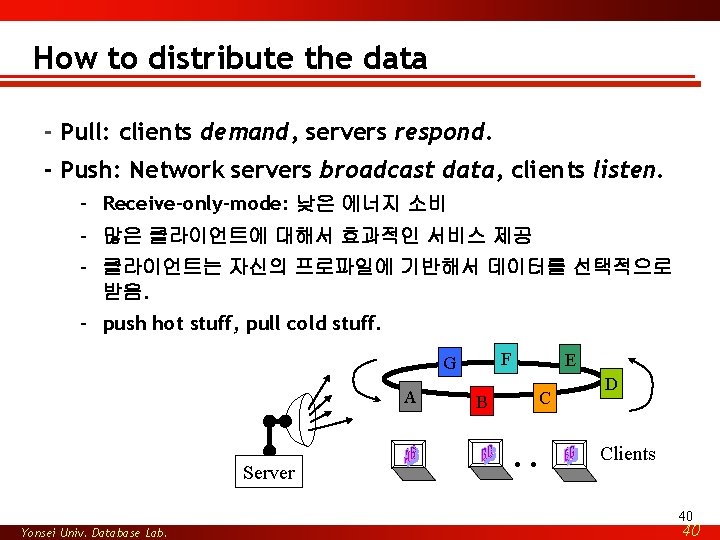
How to distribute the data - Pull: clients demand, servers respond. - Push: Network servers broadcast data, clients listen. – Receive-only-mode: 낮은 에너지 소비 – 많은 클라이언트에 대해서 효과적인 서비스 제공 – 클라이언트는 자신의 프로파일에 기반해서 데이터를 선택적으로 받음. – push hot stuff, pull cold stuff. F G A Server E C B . . D Clients 40 Yonsei Univ. Database Lab. 40
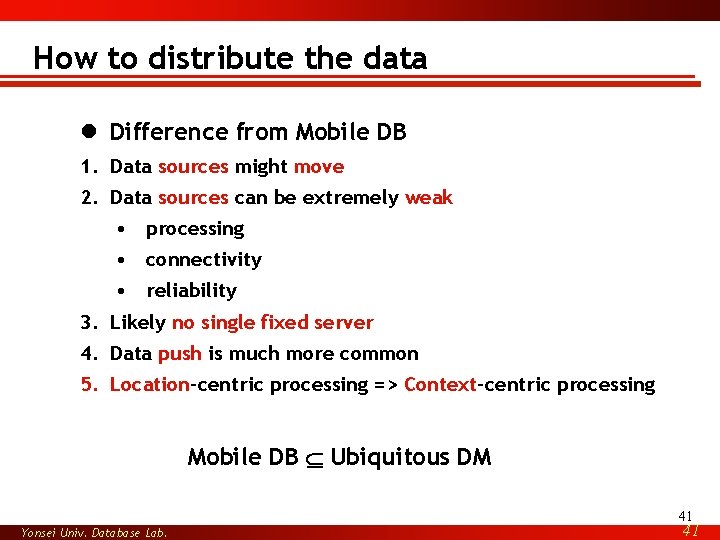
How to distribute the data l Difference from Mobile DB 1. Data sources might move 2. Data sources can be extremely weak • processing • connectivity • reliability 3. Likely no single fixed server 4. Data push is much more common 5. Location-centric processing => Context-centric processing Mobile DB Ubiquitous DM 41 Yonsei Univ. Database Lab. 41
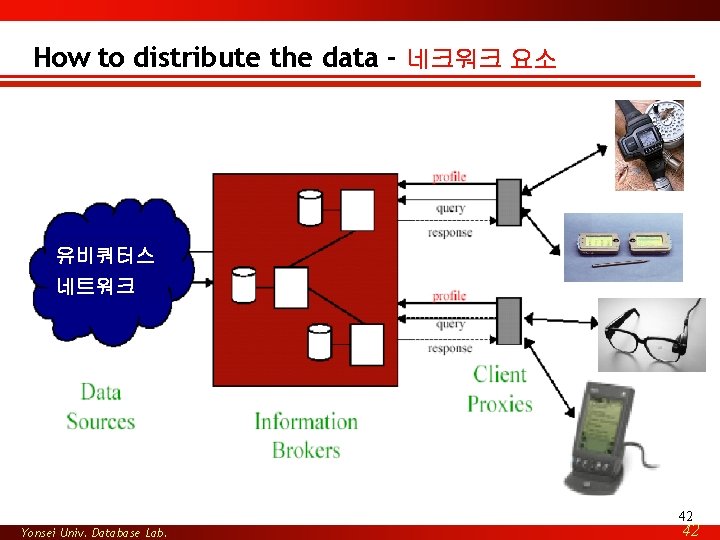
How to distribute the data - 네크워크 요소 유비쿼터스 네트워크 42 Yonsei Univ. Database Lab. 42
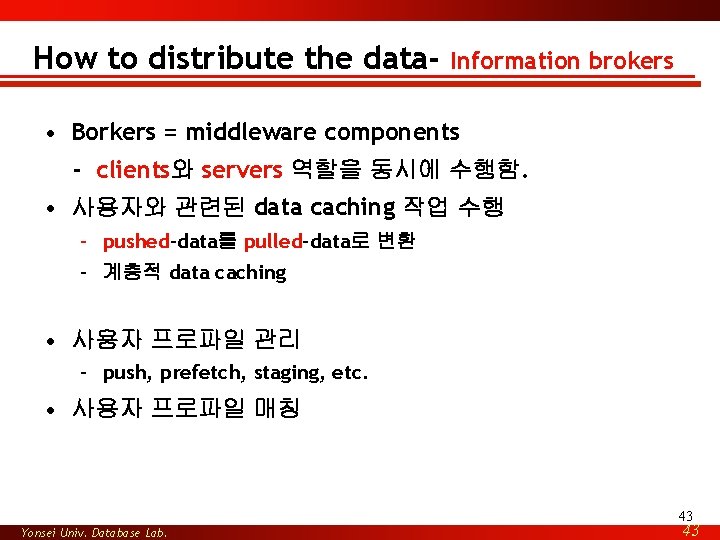
How to distribute the data- Information brokers • Borkers = middleware components - clients와 servers 역할을 동시에 수행함. • 사용자와 관련된 data caching 작업 수행 – pushed-data를 pulled-data로 변환 – 계층적 data caching • 사용자 프로파일 관리 – push, prefetch, staging, etc. • 사용자 프로파일 매칭 43 Yonsei Univ. Database Lab. 43
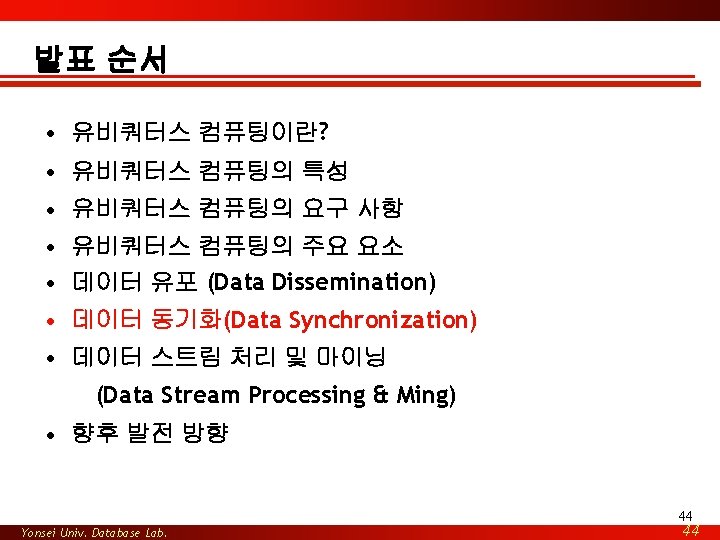
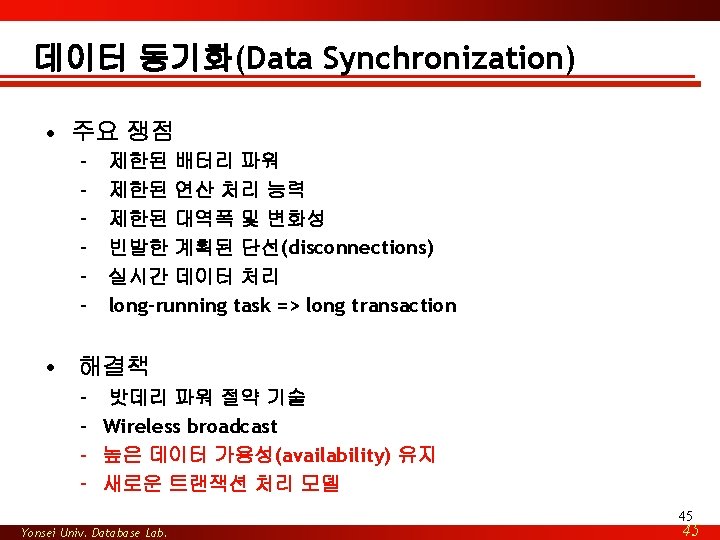
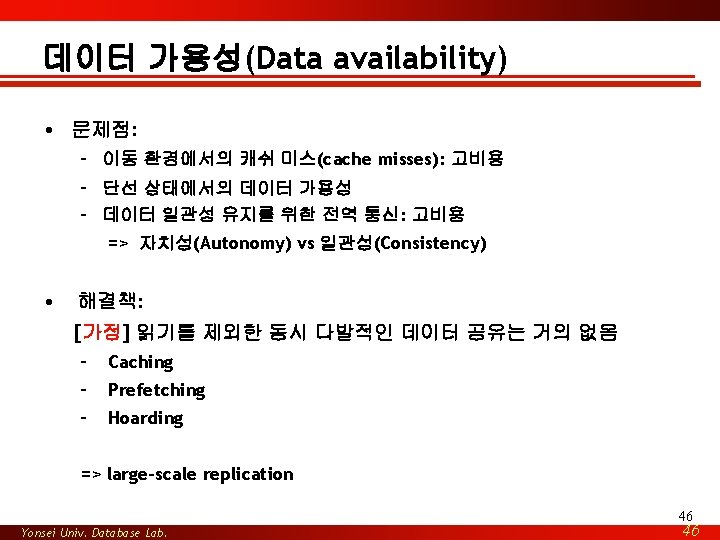
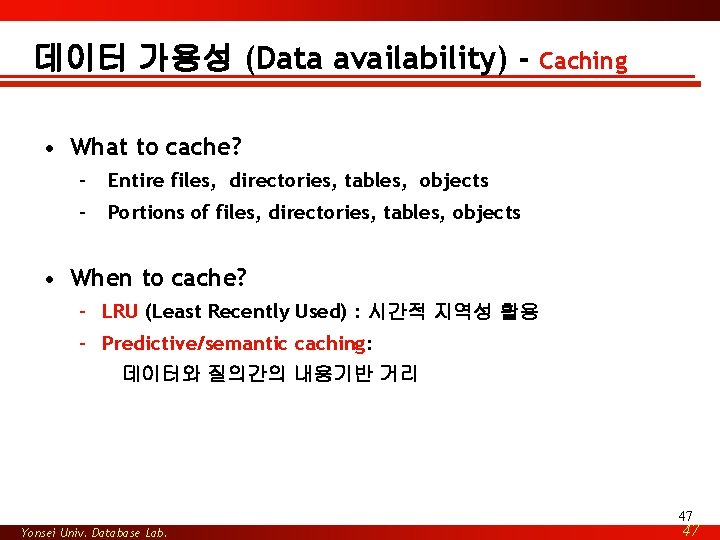
데이터 가용성 (Data availability) - Caching • What to cache? – Entire files, directories, tables, objects – Portions of files, directories, tables, objects • When to cache? – LRU (Least Recently Used) : 시간적 지역성 활용 – Predictive/semantic caching: 데이터와 질의간의 내용기반 거리 47 Yonsei Univ. Database Lab. 47
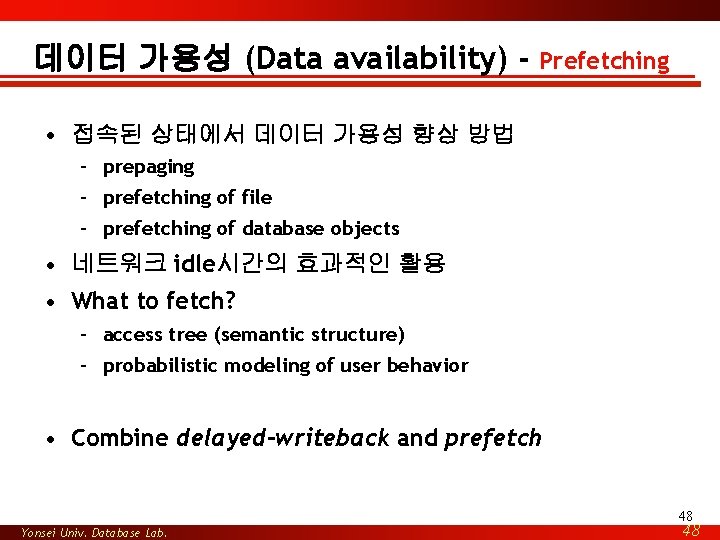
데이터 가용성 (Data availability) - Prefetching • 접속된 상태에서 데이터 가용성 향상 방법 – prepaging – prefetching of file – prefetching of database objects • 네트워크 idle시간의 효과적인 활용 • What to fetch? – access tree (semantic structure) – probabilistic modeling of user behavior • Combine delayed-writeback and prefetch 48 Yonsei Univ. Database Lab. 48
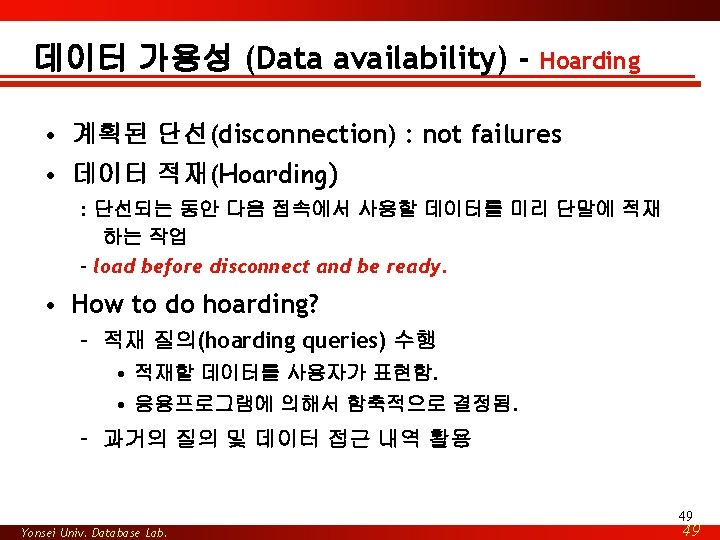
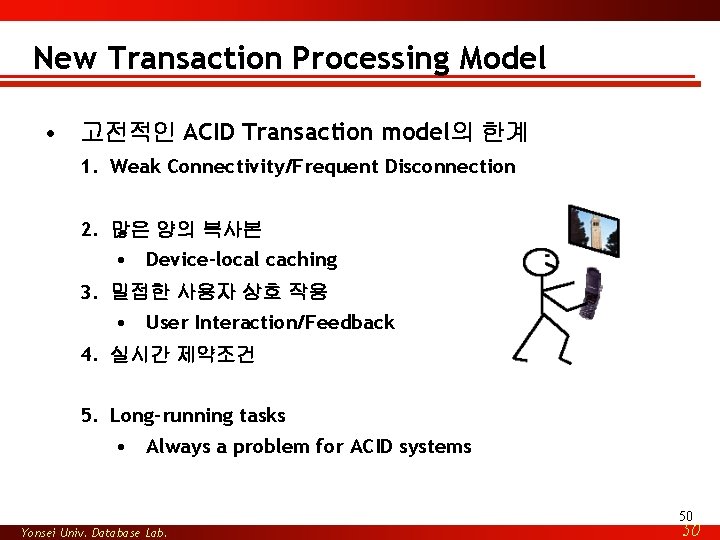
New Transaction Processing Model • 고전적인 ACID Transaction model의 한계 1. Weak Connectivity/Frequent Disconnection 2. 많은 양의 복사본 • Device-local caching 3. 밀접한 사용자 상호 작용 • User Interaction/Feedback 4. 실시간 제약조건 5. Long-running tasks • Always a problem for ACID systems 50 Yonsei Univ. Database Lab. 50
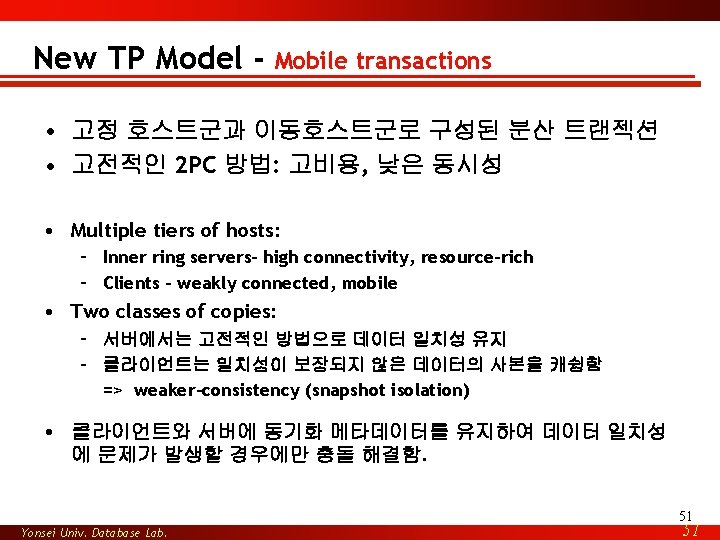
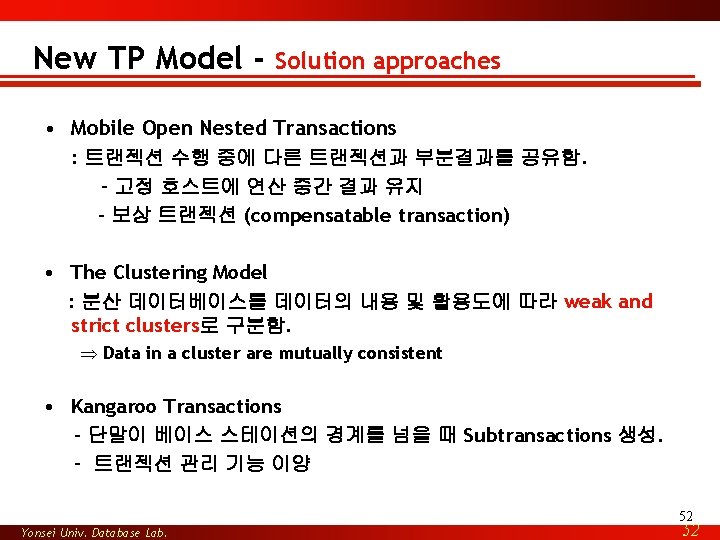
New TP Model - Solution approaches • Mobile Open Nested Transactions : 트랜젝션 수행 중에 다른 트랜젝션과 부분결과를 공유함. - 고정 호스트에 연산 중간 결과 유지 - 보상 트랜젝션 (compensatable transaction) • The Clustering Model : 분산 데이터베이스를 데이터의 내용 및 활용도에 따라 weak and strict clusters로 구분함. Þ Data in a cluster are mutually consistent • Kangaroo Transactions - 단말이 베이스 스테이션의 경계를 넘을 때 Subtransactions 생성. - 트랜젝션 관리 기능 이양 52 Yonsei Univ. Database Lab. 52
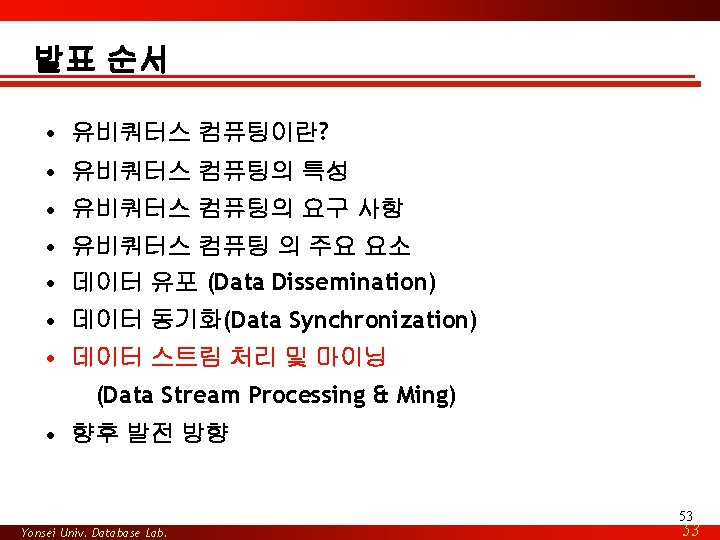
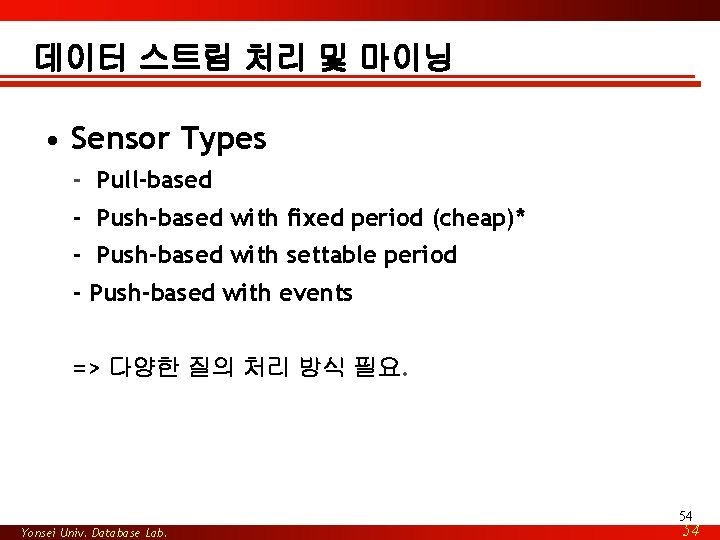
데이터 스트림 처리 및 마이닝 • Sensor Types - Pull-based - Push-based with fixed period (cheap)* - Push-based with settable period - Push-based with events => 다양한 질의 처리 방식 필요. 54 Yonsei Univ. Database Lab. 54
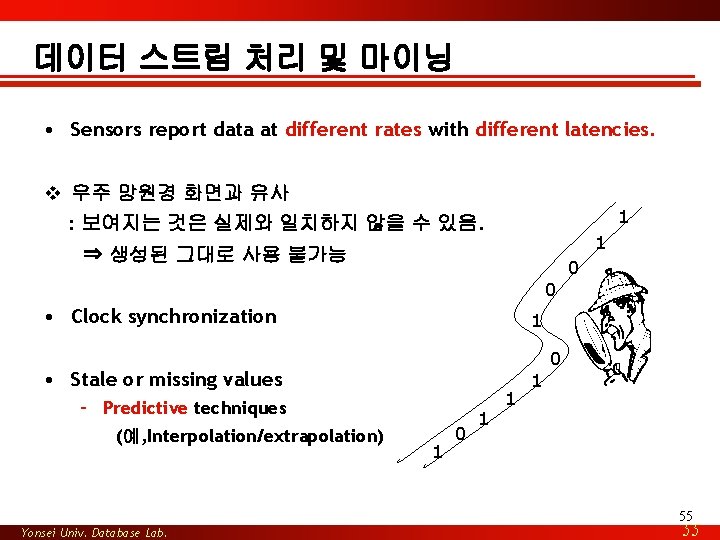
데이터 스트림 처리 및 마이닝 • Sensors report data at different rates with different latencies. v 우주 망원경 화면과 유사 : 보여지는 것은 실제와 일치하지 않을 수 있음. 1 1 ⇒ 생성된 그대로 사용 불가능 0 0 • Clock synchronization 1 0 • Stale or missing values – Predictive techniques (예, Interpolation/extrapolation) 1 0 1 1 1 55 Yonsei Univ. Database Lab. 55
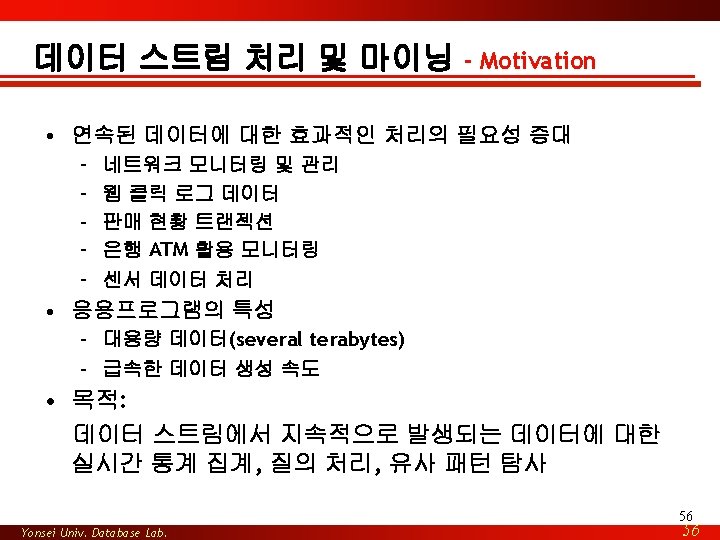
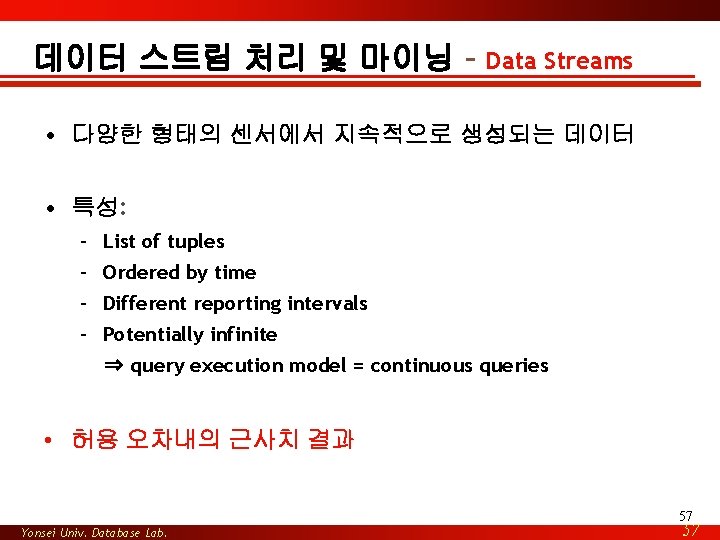
데이터 스트림 처리 및 마이닝 – Data Streams • 다양한 형태의 센서에서 지속적으로 생성되는 데이터 • 특성: – List of tuples – Ordered by time – Different reporting intervals – Potentially infinite ⇒ query execution model = continuous queries • 허용 오차내의 근사치 결과 57 Yonsei Univ. Database Lab. 57
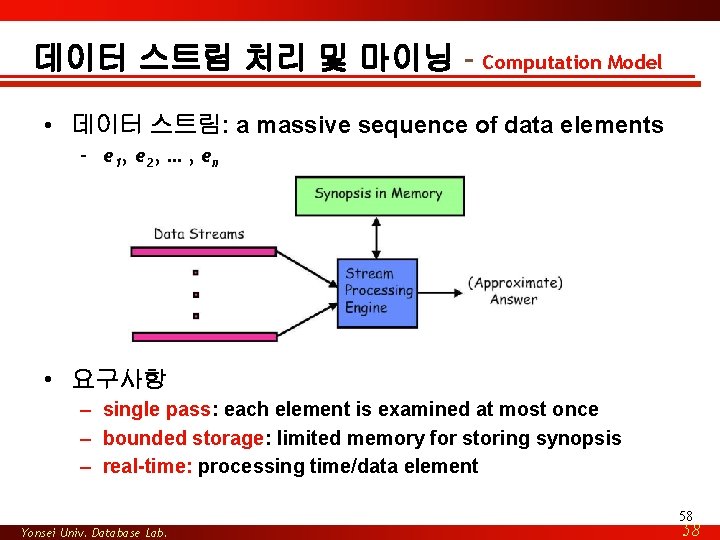
데이터 스트림 처리 및 마이닝 – Computation Model • 데이터 스트림: a massive sequence of data elements – e 1 , e 2 , … , e n • 요구사항 – single pass: each element is examined at most once – bounded storage: limited memory for storing synopsis – real-time: processing time/data element 58 Yonsei Univ. Database Lab. 58
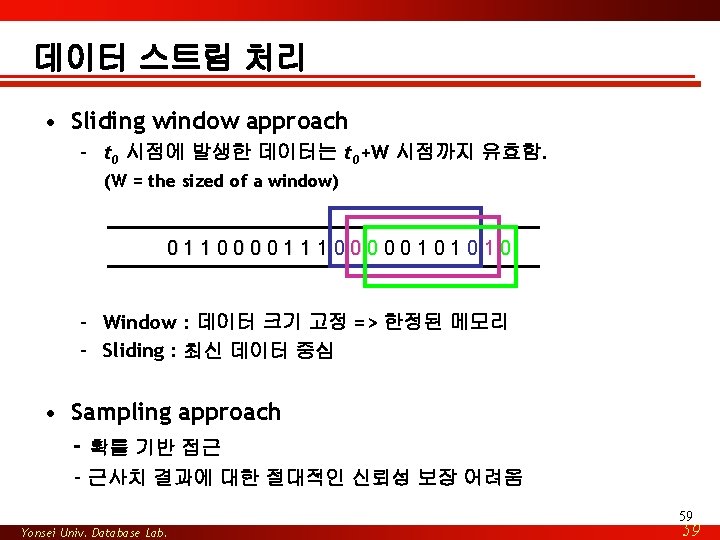
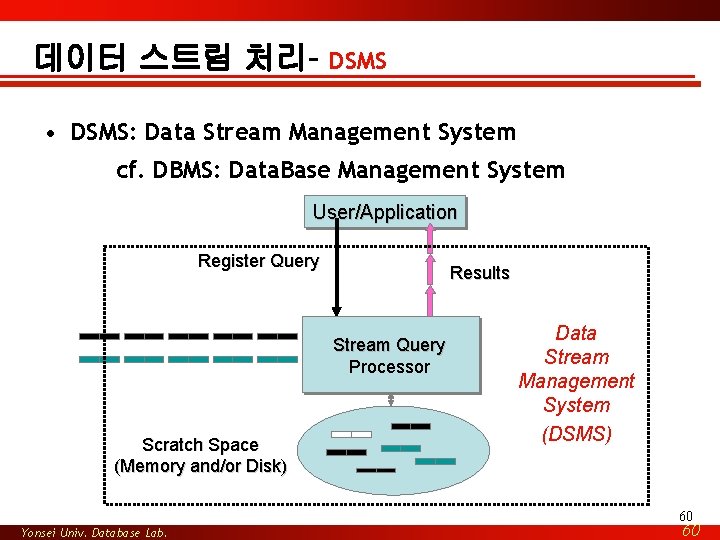
데이터 스트림 처리– DSMS • DSMS: Data Stream Management System cf. DBMS: Data. Base Management System User/Application Register Query Results Stream Query Processor Scratch Space (Memory and/or Disk) Data Stream Management System (DSMS) 60 Yonsei Univ. Database Lab. 60
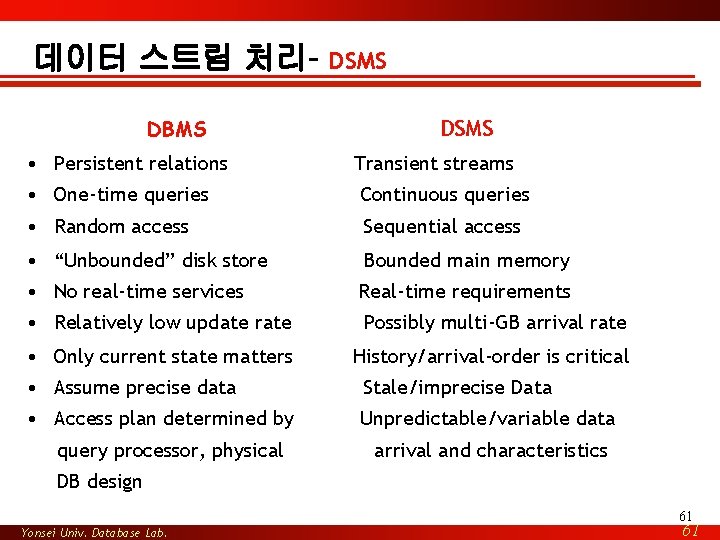
데이터 스트림 처리– DBMS DSMS • Persistent relations Transient streams • One-time queries Continuous queries • Random access Sequential access • “Unbounded” disk store Bounded main memory • No real-time services Real-time requirements • Relatively low update rate Possibly multi-GB arrival rate • Only current state matters History/arrival-order is critical • Assume precise data Stale/imprecise Data • Access plan determined by Unpredictable/variable data query processor, physical arrival and characteristics DB design 61 Yonsei Univ. Database Lab. 61
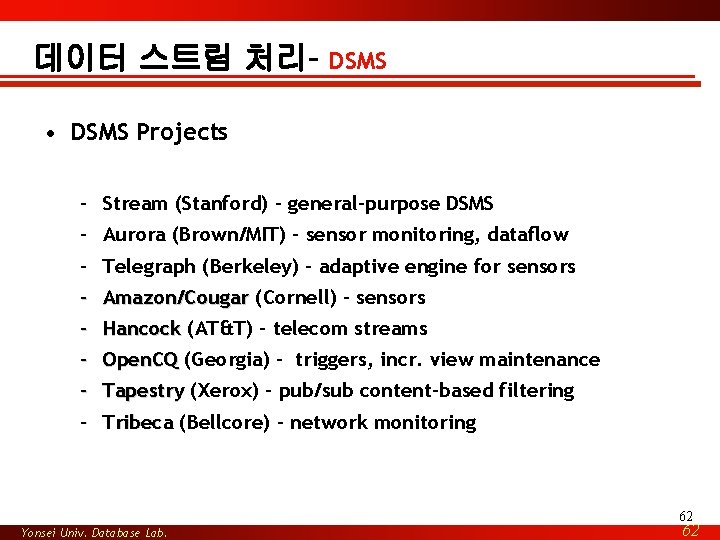
데이터 스트림 처리– DSMS • DSMS Projects – Stream (Stanford) – general-purpose DSMS – Aurora (Brown/MIT) – sensor monitoring, dataflow – Telegraph (Berkeley) – adaptive engine for sensors – Amazon/Cougar (Cornell) – sensors – Hancock (AT&T) – telecom streams – Open. CQ (Georgia) – triggers, incr. view maintenance – Tapestry (Xerox) – pub/sub content-based filtering – Tribeca (Bellcore) – network monitoring 62 Yonsei Univ. Database Lab. 62
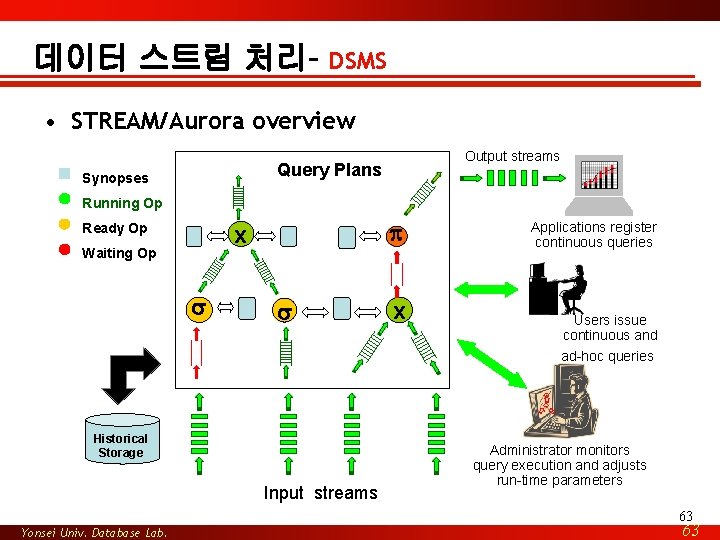
데이터 스트림 처리– DSMS • STREAM/Aurora overview Output streams Query Plans Synopses Running Op Ready Op p x Waiting Op s s Historical Storage Input streams x Applications register continuous queries Users issue continuous and ad-hoc queries Administrator monitors query execution and adjusts run-time parameters 63 Yonsei Univ. Database Lab. 63
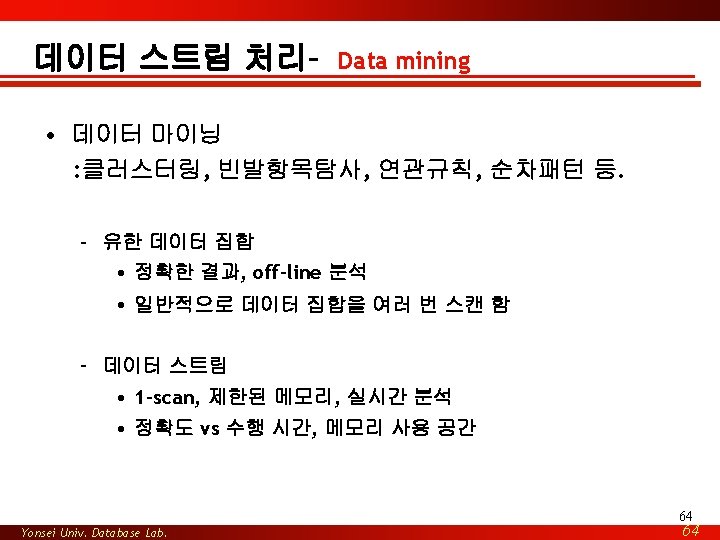
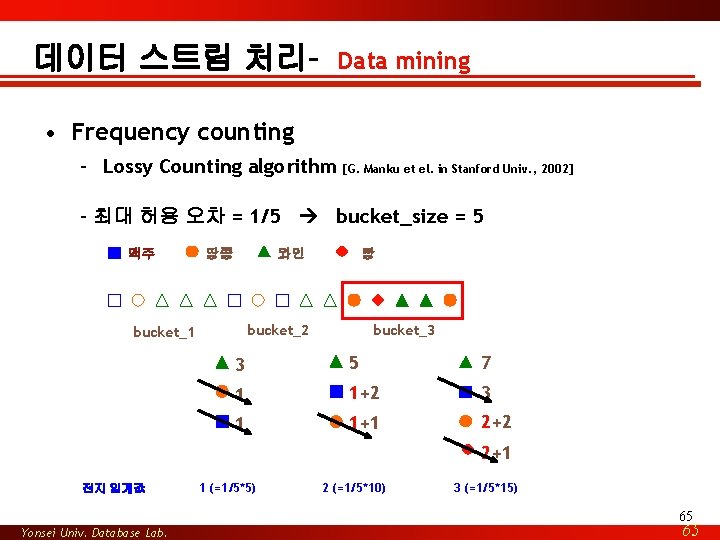
데이터 스트림 처리– Data mining • Frequency counting – Lossy Counting algorithm [G. Manku et el. in Stanford Univ. , 2002] - 최대 허용 오차 = 1/5 bucket_size = 5 맥주 땅콩 와인 빵 bucket_2 bucket_1 bucket_3 3 5 7 1 1+2 3 1 1+1 2+2 2+1 전지 임계값 1 (=1/5*5) 2 (=1/5*10) 3 (=1/5*15) 65 Yonsei Univ. Database Lab. 65
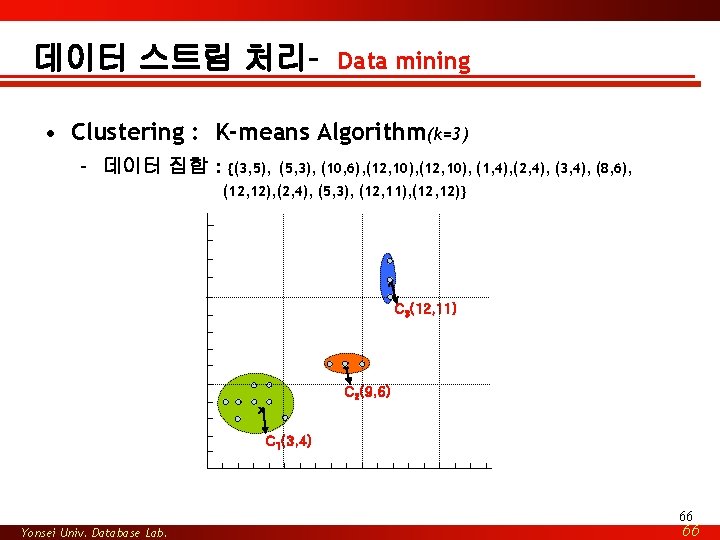
데이터 스트림 처리– Data mining • Clustering : K-means Algorithm(k=3) – 데이터 집합 : {(3, 5), (5, 3), (10, 6), (12, 10), (1, 4), (2, 4), (3, 4), (8, 6), (12, 12), (2, 4), (5, 3), (12, 11), (12, 12)} x C 3(12, 11) x C 2(9, 6) x C 1(3, 4) 66 Yonsei Univ. Database Lab. 66
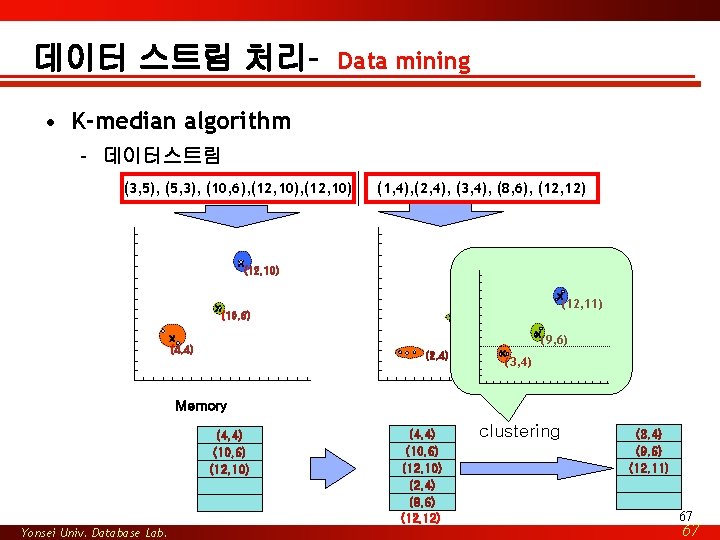
데이터 스트림 처리– Data mining • K-median algorithm – 데이터스트림 (3, 5), (5, 3), (10, 6), (12, 10) (1, 4), (2, 4), (3, 4), (8, 6), (12, 12) x x (12, 10) (12, 12) x (12, 11) x (10, 6) x (8, 6) x (4, 4) x (2, 4) x (3, 4) x (9, 6) Memory (4, 4) (10, 6) (12, 10) Yonsei Univ. Database Lab. (4, 4) (10, 6) (12, 10) (2, 4) (8, 6) (12, 12) clustering (3, 4) (9, 6) (12, 11) 67 67
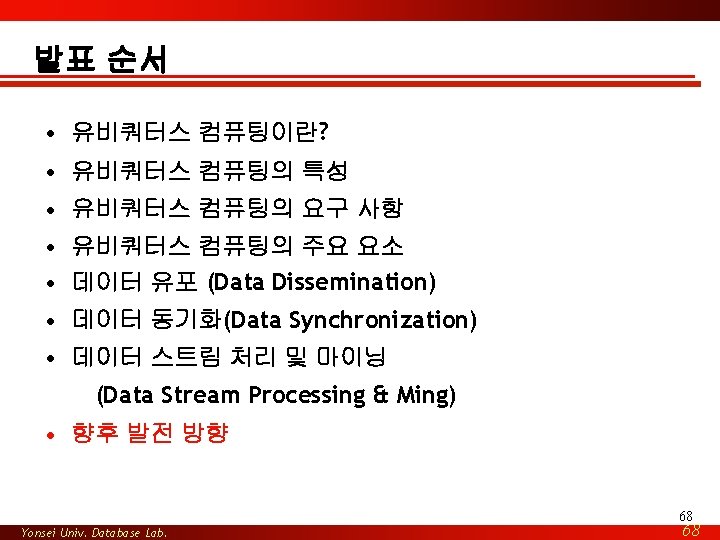
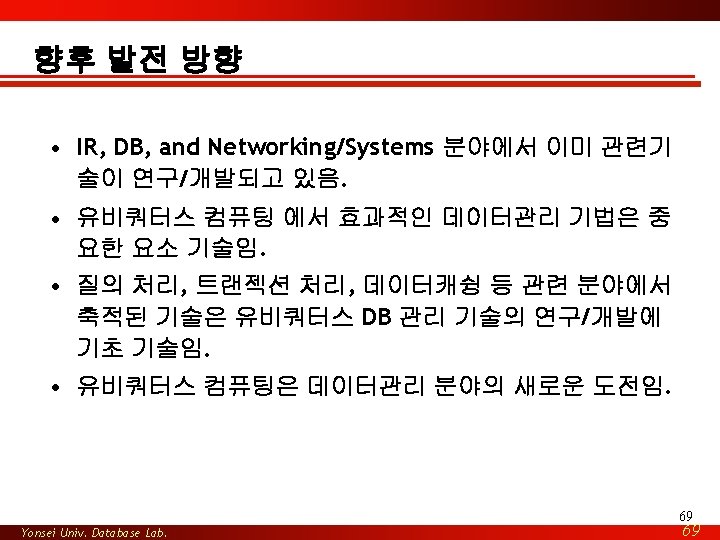
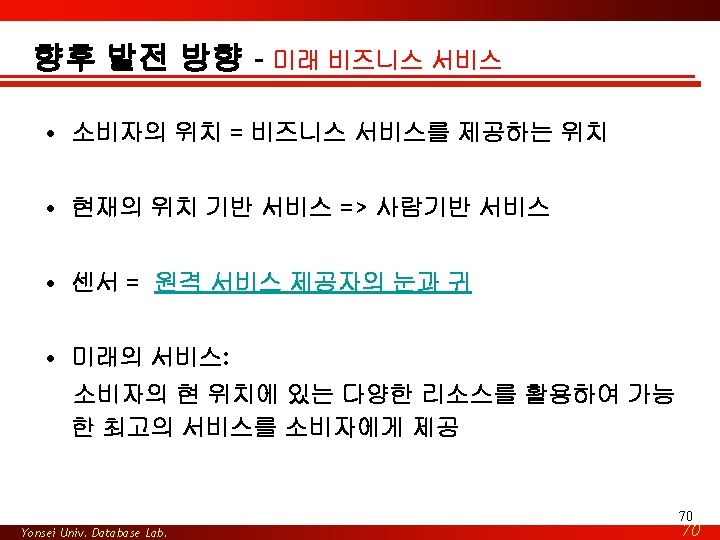
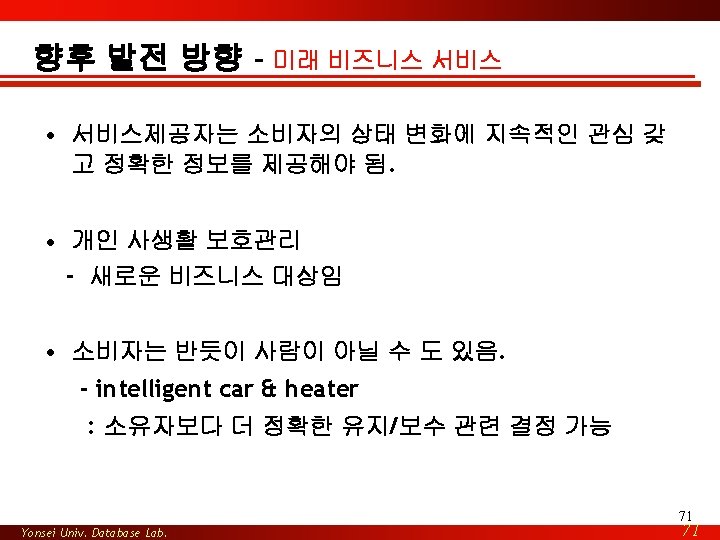
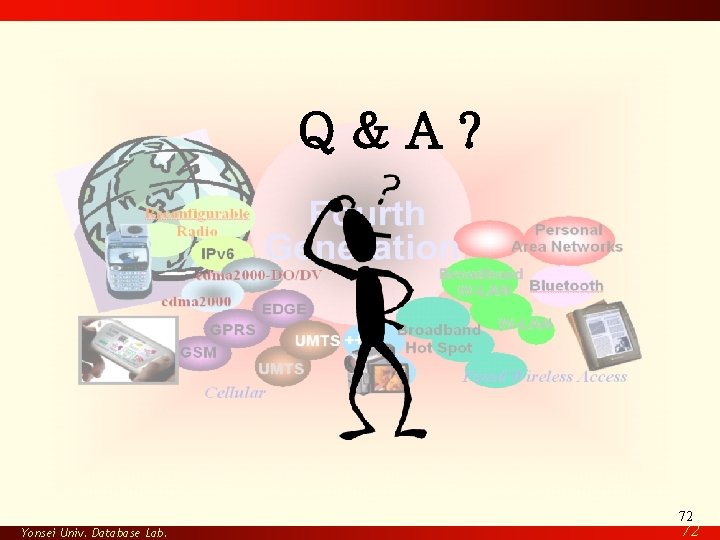
Q&A? 72 Yonsei Univ. Database Lab. 72
Anytime anywhere any device
Anytime anywhere any device
Mercy bears richer fruits than strict justice
Metaphase pdm
How to access tally remotely
I can quit anytime
Tv anytime
Anytime cosmetics
Q anytime
Find the song
What is intelligence anyway by isaac asimov
Whose life is it anyway analysis
Whos line is it anyway
What is a civilization anyway
So what are you anyway by lawrence hill
What is constitution? *
Whose water is it anyway
Sustainable living meaning
Terminal access controller access control system plus
Terminal access controller access-control system
Multimedia diagram mil
Cover letter ucl
Practice click
Goanywhere agent
Any or anything
Ucl dropbox
Click preposition
Sitting anywhere at work
Uva netreg
Ub print from anywhere
Maximo upgrade
Anywhere
Click anywhere to start
Autocard download
Train anywhere
Targit decision suite
Myriam sigrist
Ibm mro inventory optimization
Click anywhere to start
Dispatch anywhere mobile
Java write once run anywhere
Ibm maximo roadmap
Click anywhere to start
Calcweb
Click anywhere to start
Click anywhere to start
Make the lie big
Go make a difference
Dawes plan
Disadvantages of using lims in a laboratory
Sharing of diverse information through universal web access
Trends in ict assistive media
Deductive reasoning geometry
Imperfect vs incomplete information
Phil and cath make and sell boomerangs
Pare culinary definition
Security maturity model gartner
Saunders lewis & thornhill 2009
Law of access cavity preparation
Difference between multiplexing and multiple access
1/3 diagonal rule egress
Exit exit access and exit discharge
Telenursing and remote access telehealth
Asvab cep post-test interpretation
Access networks and physical media
Special entry access scheme monash
Identity management roadmap
Aws identity and access management
What lasting legacies did the aksum kingdom leave
Assvab occu find
Enterprise identity services suite
Internet access techniques
Seas impact statement example