The US 2010 Elections on Twitter Avishay Livne
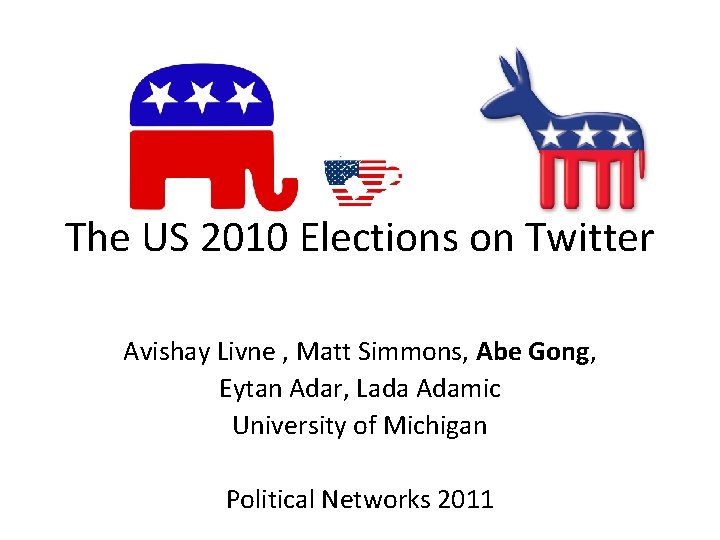
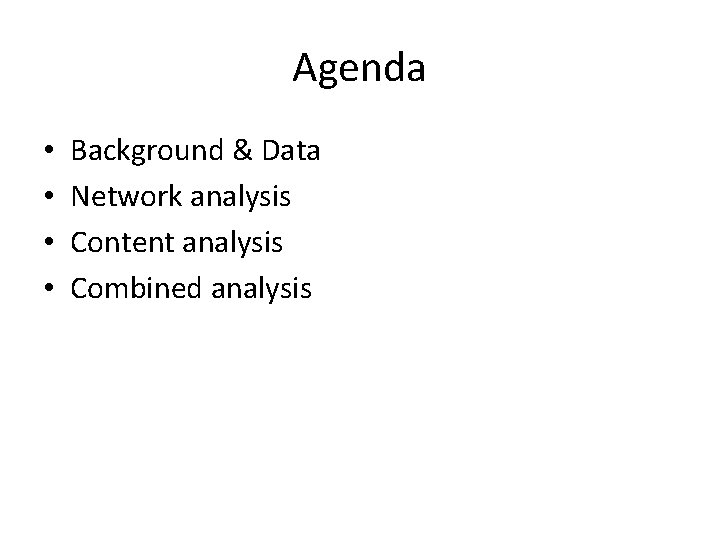
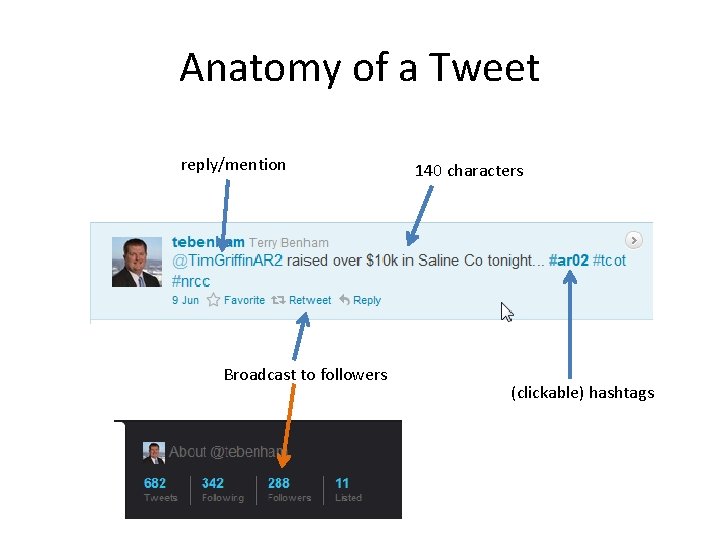
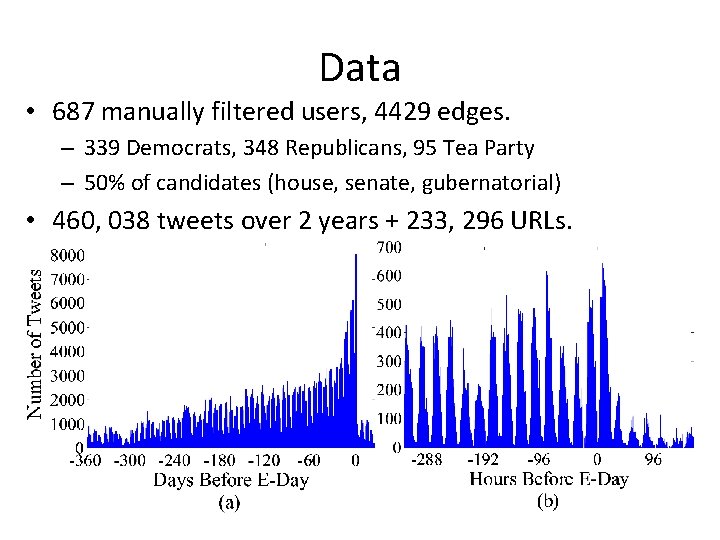
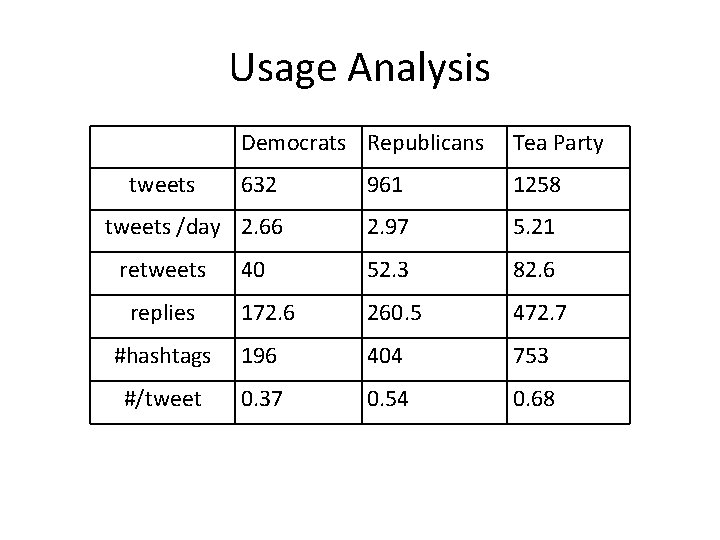
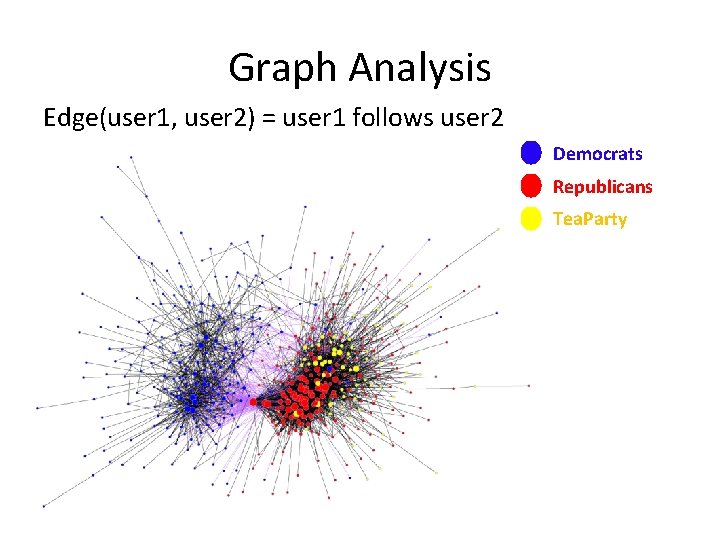
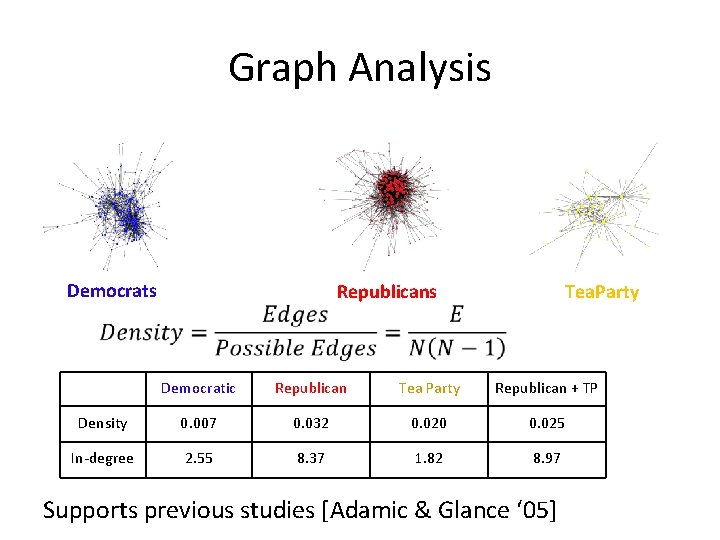
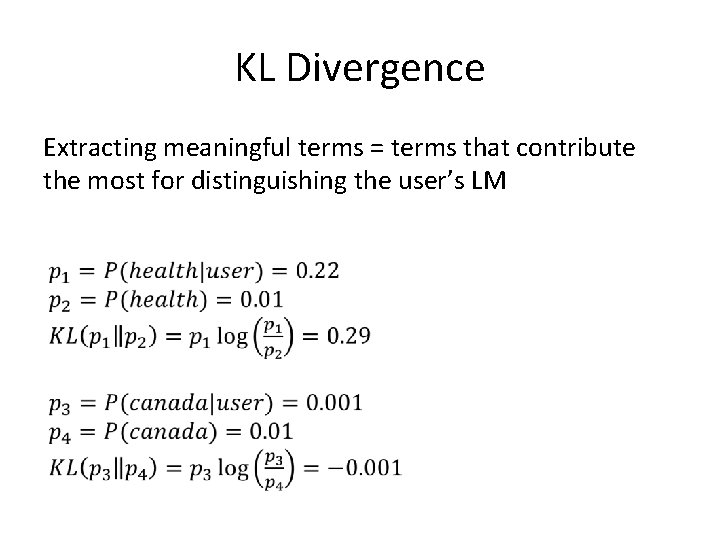
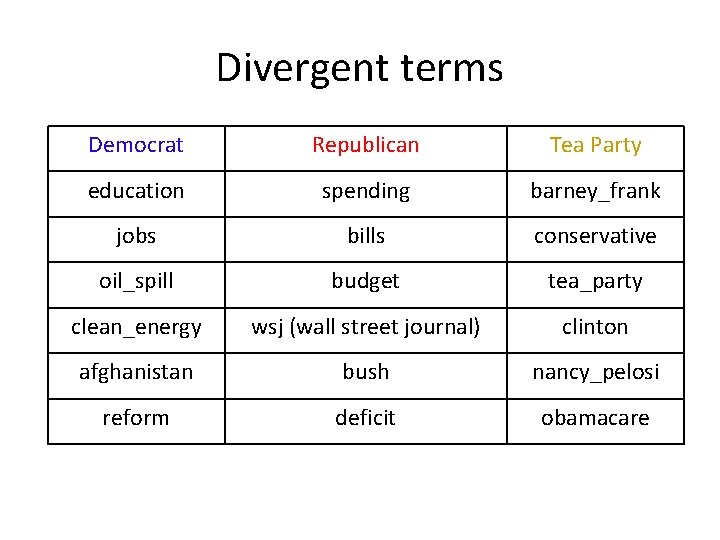
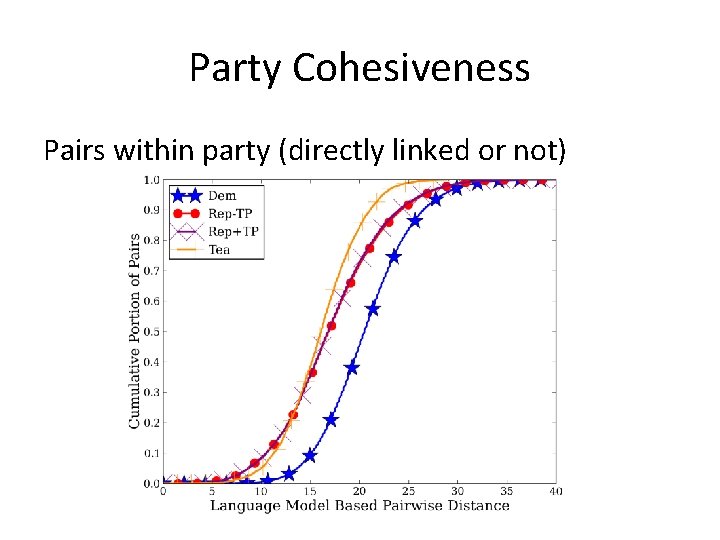
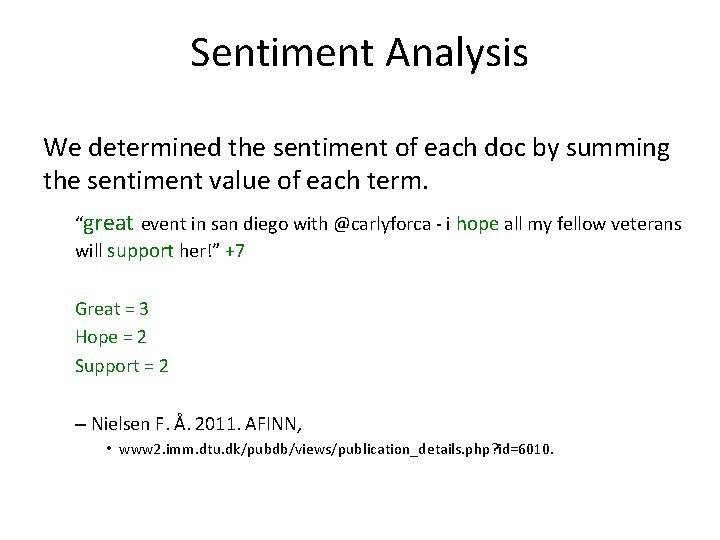
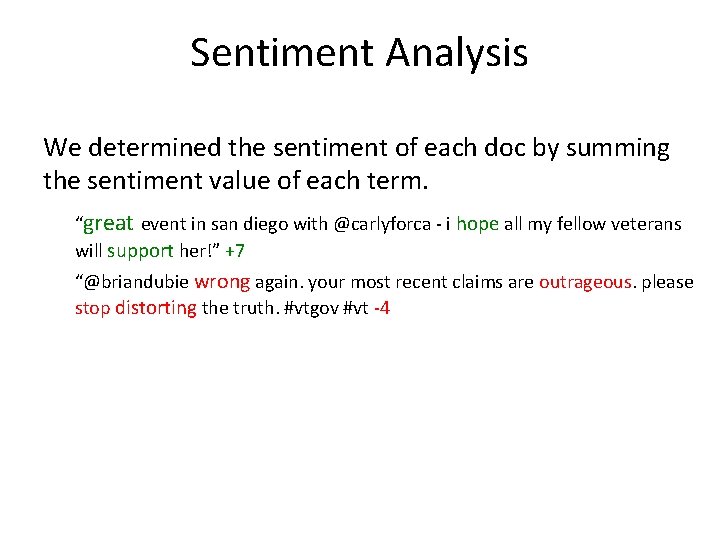
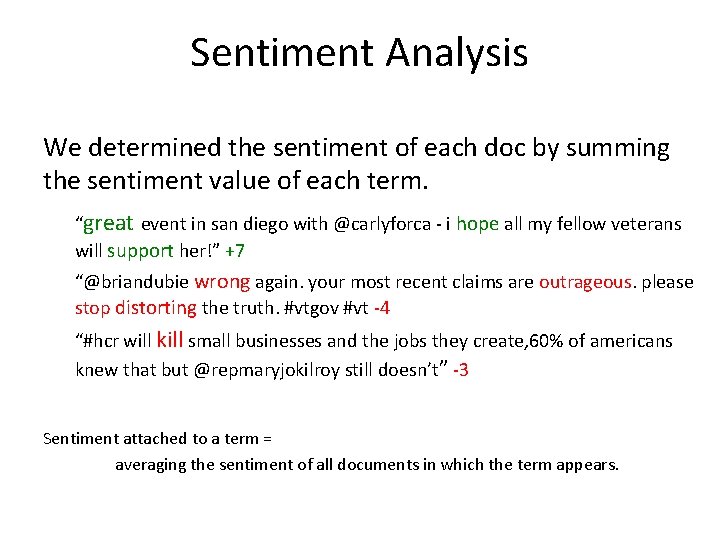
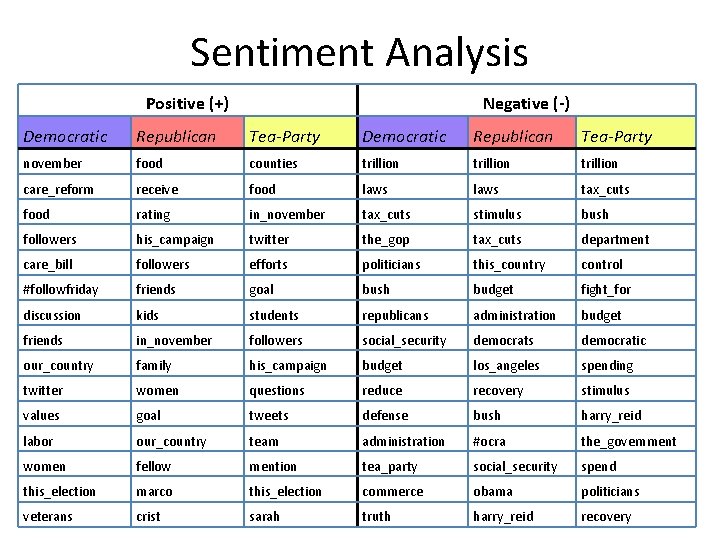
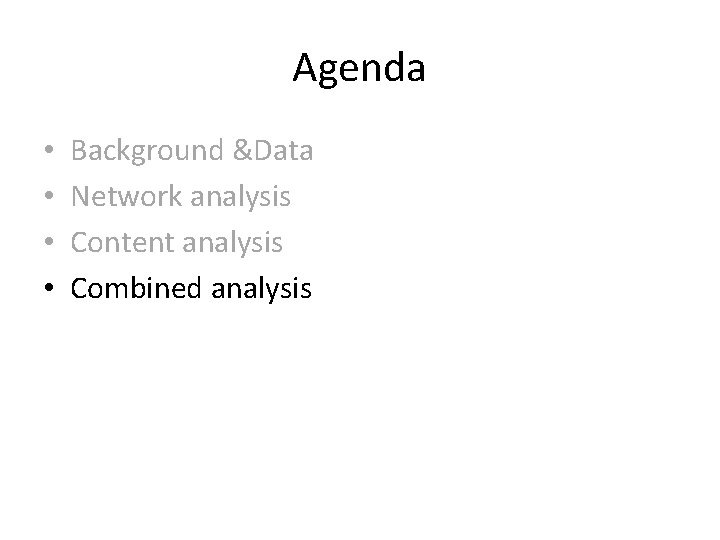
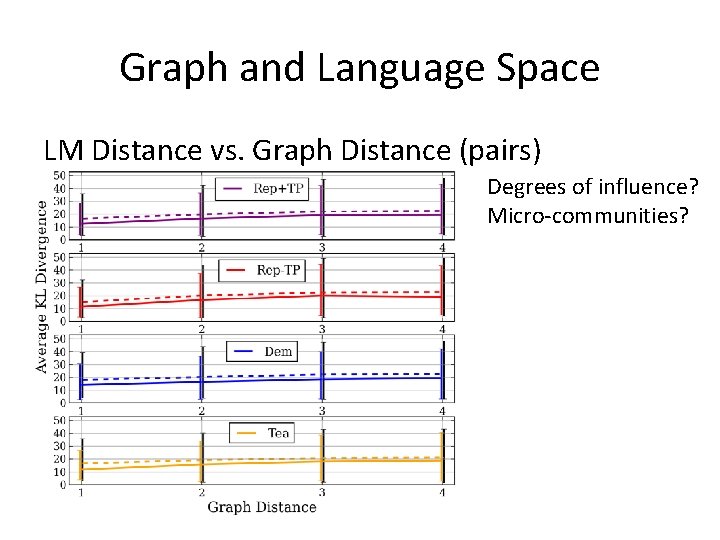
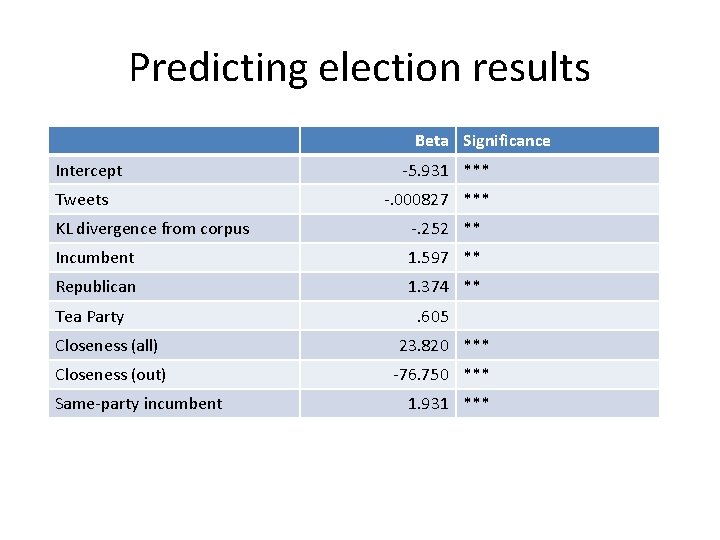
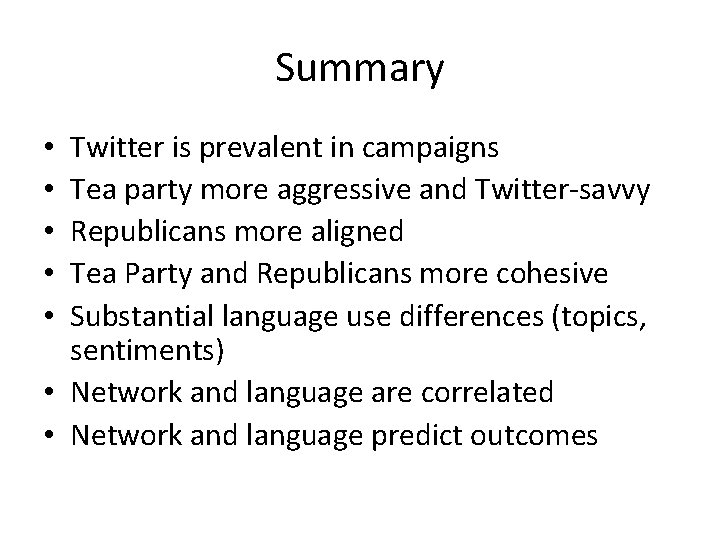
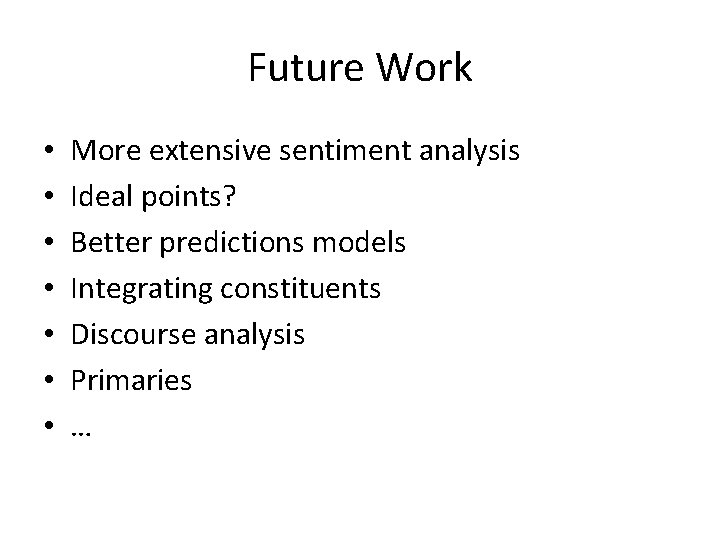
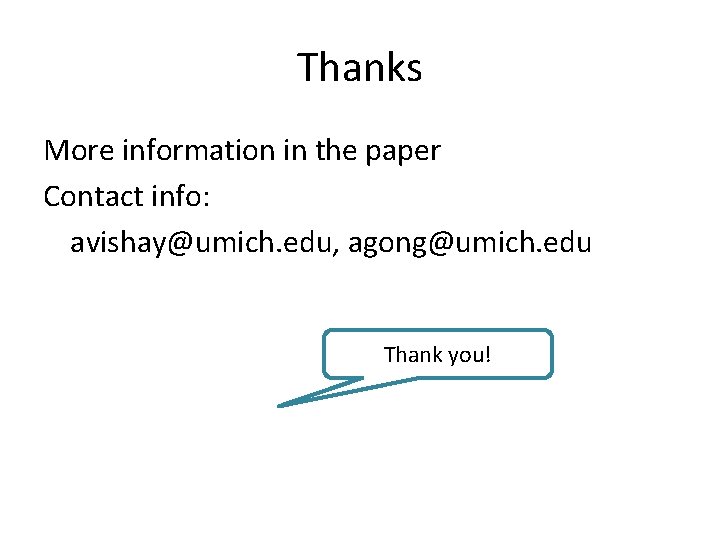
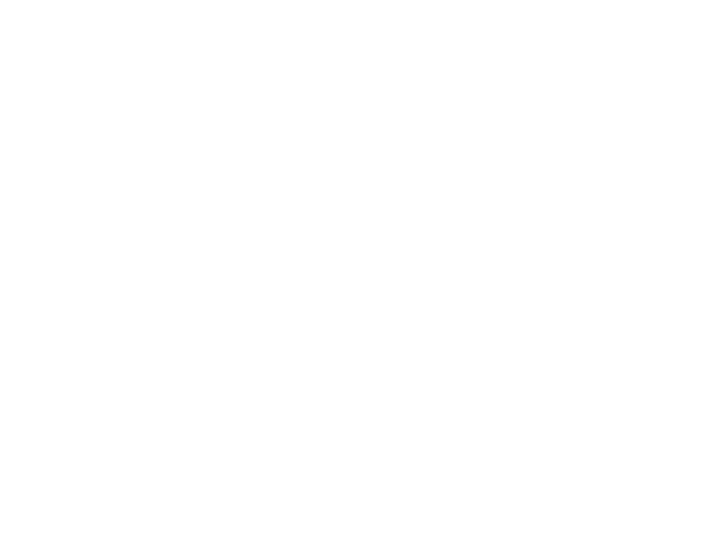
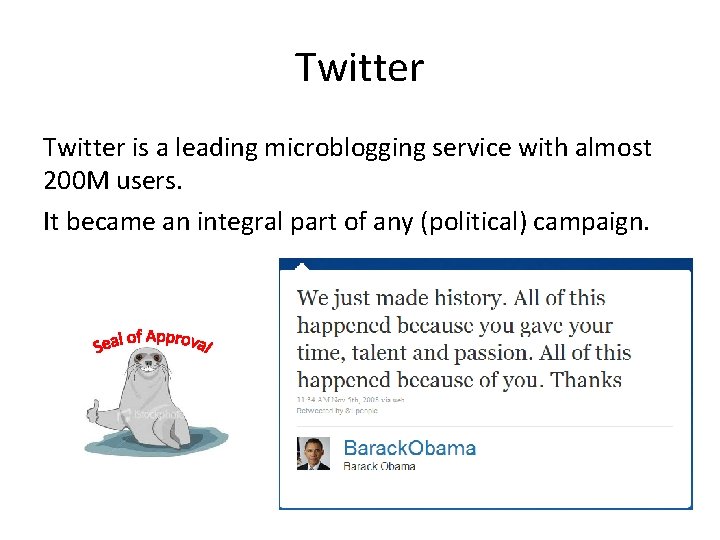
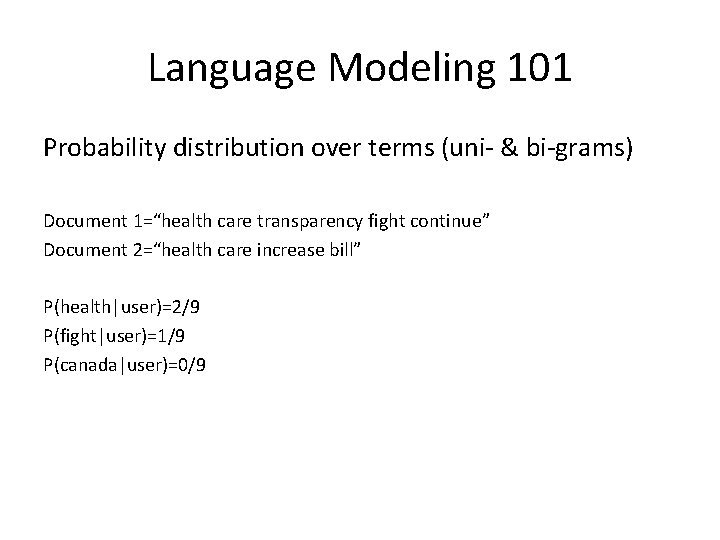
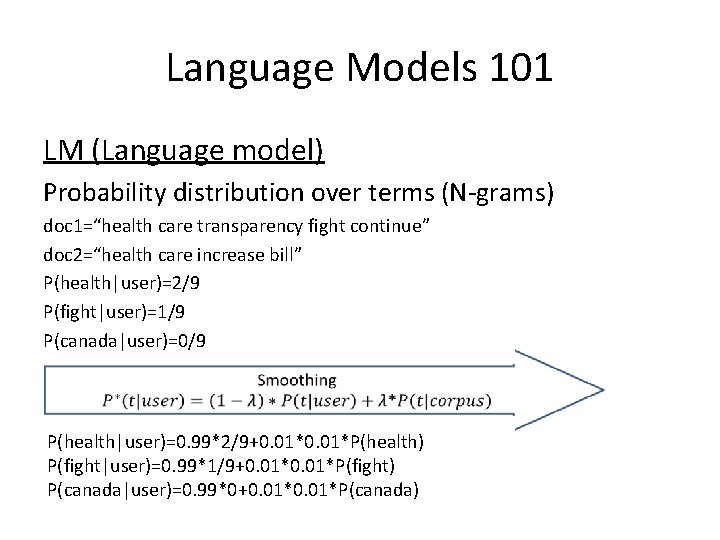
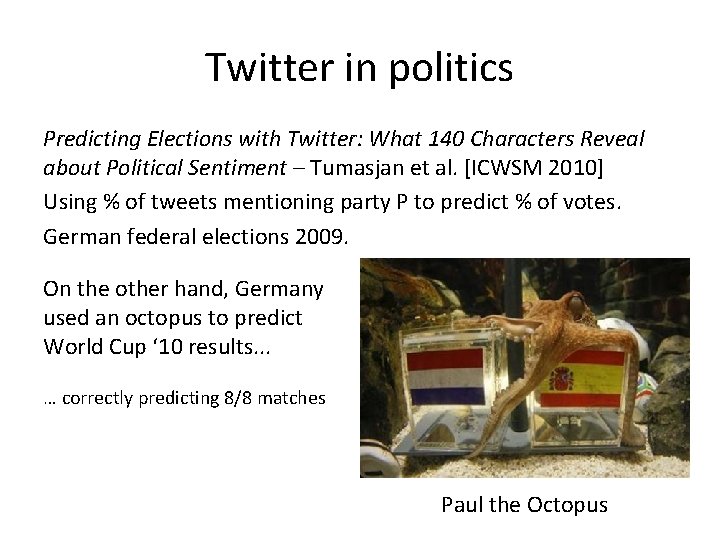
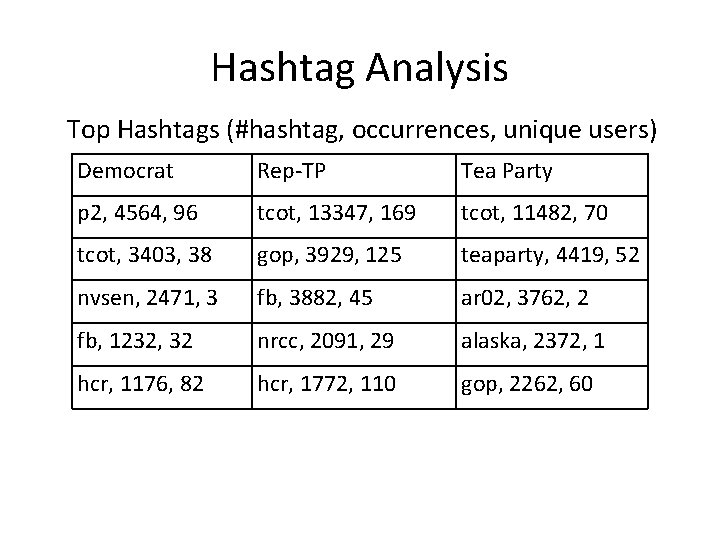
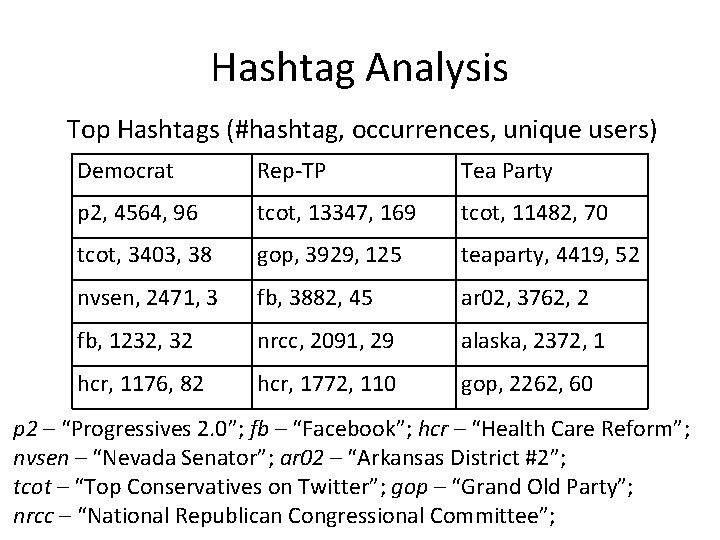
![Topic Modeling (LDA) • We extracted latent topics using LDA [Blei, Ng, Jordan 2002] Topic Modeling (LDA) • We extracted latent topics using LDA [Blei, Ng, Jordan 2002]](https://slidetodoc.com/presentation_image_h2/96baff93cdcd885948fa47d2264a4391/image-28.jpg)
![Topics Topic terms Affinity Difference tax, jobs, spending, [O]bama, stimulus -0. 047618 health, care, Topics Topic terms Affinity Difference tax, jobs, spending, [O]bama, stimulus -0. 047618 health, care,](https://slidetodoc.com/presentation_image_h2/96baff93cdcd885948fa47d2264a4391/image-29.jpg)
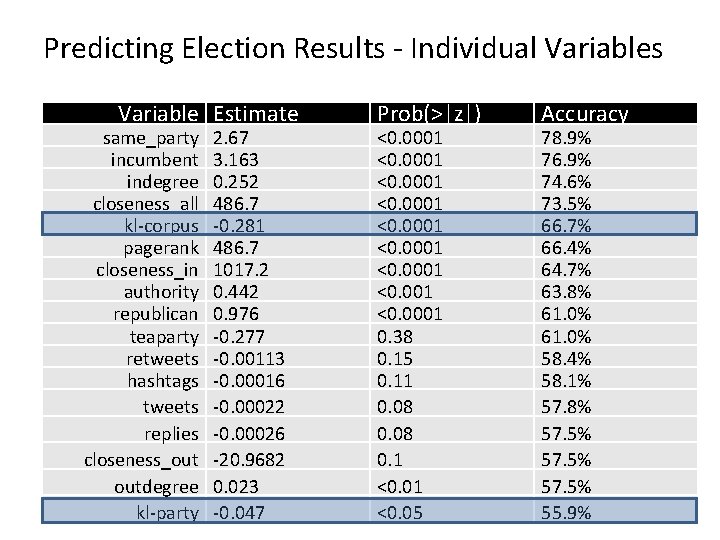
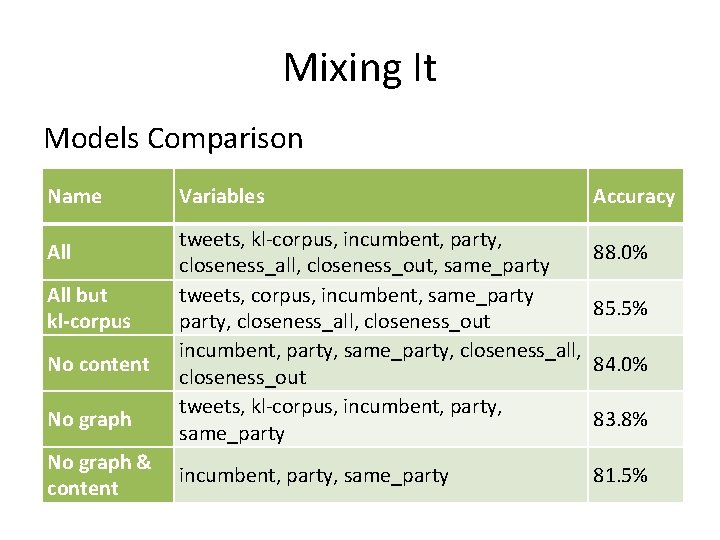
- Slides: 31
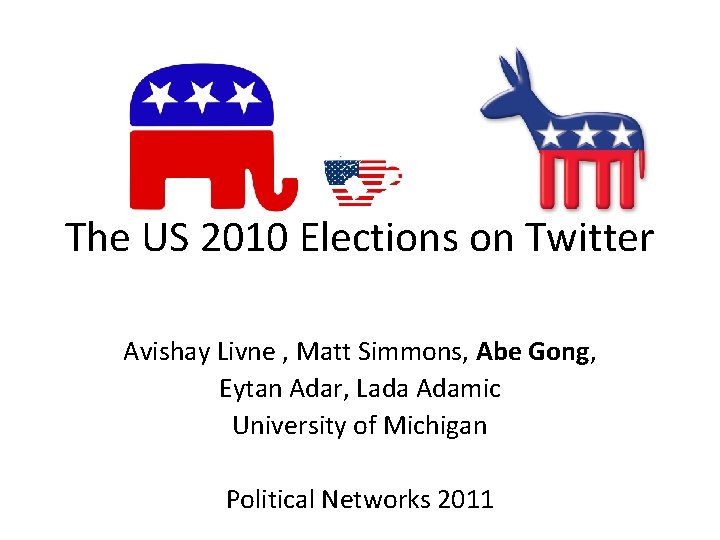
The US 2010 Elections on Twitter Avishay Livne , Matt Simmons, Abe Gong, Eytan Adar, Lada Adamic University of Michigan Political Networks 2011
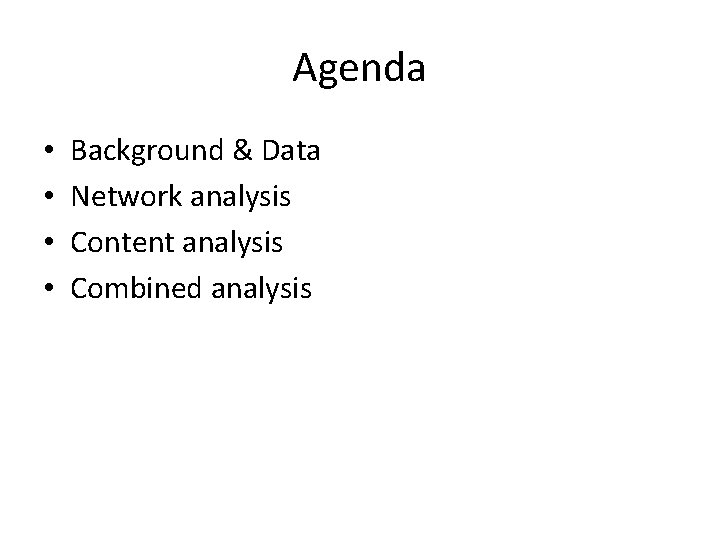
Agenda • • Background & Data Network analysis Content analysis Combined analysis
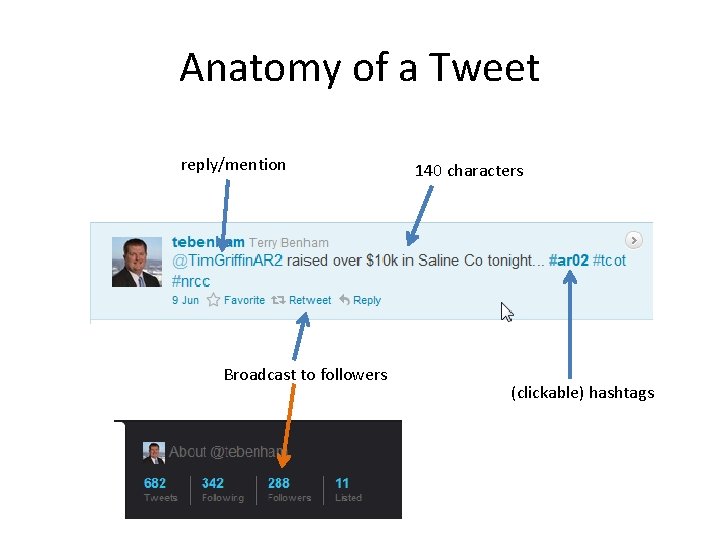
Anatomy of a Tweet reply/mention Broadcast to followers 140 characters (clickable) hashtags
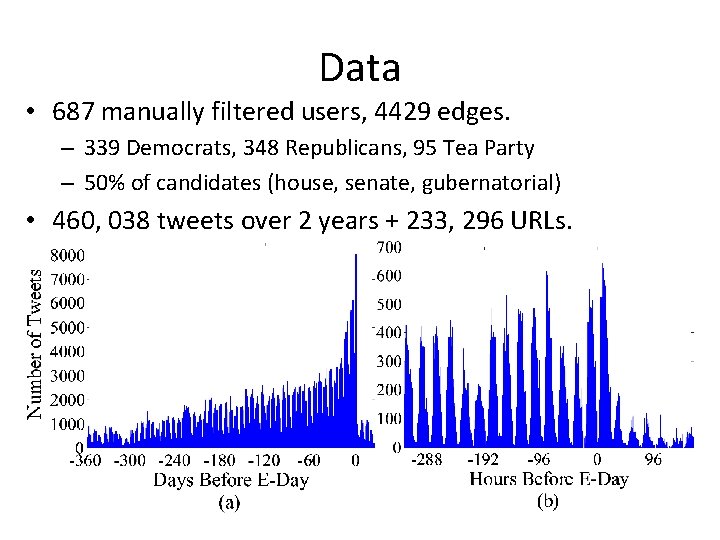
Data • 687 manually filtered users, 4429 edges. – 339 Democrats, 348 Republicans, 95 Tea Party – 50% of candidates (house, senate, gubernatorial) • 460, 038 tweets over 2 years + 233, 296 URLs.
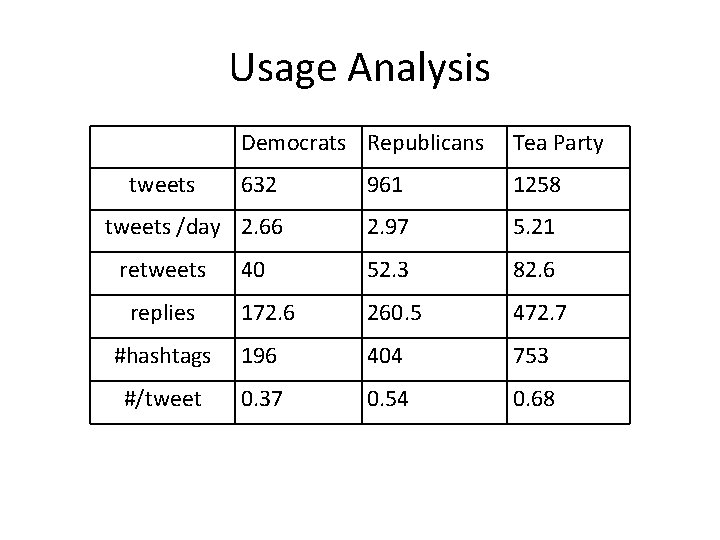
Usage Analysis Democrats Republicans Tea Party 632 961 1258 tweets /day 2. 66 2. 97 5. 21 40 52. 3 82. 6 172. 6 260. 5 472. 7 #hashtags 196 404 753 #/tweet 0. 37 0. 54 0. 68 tweets replies
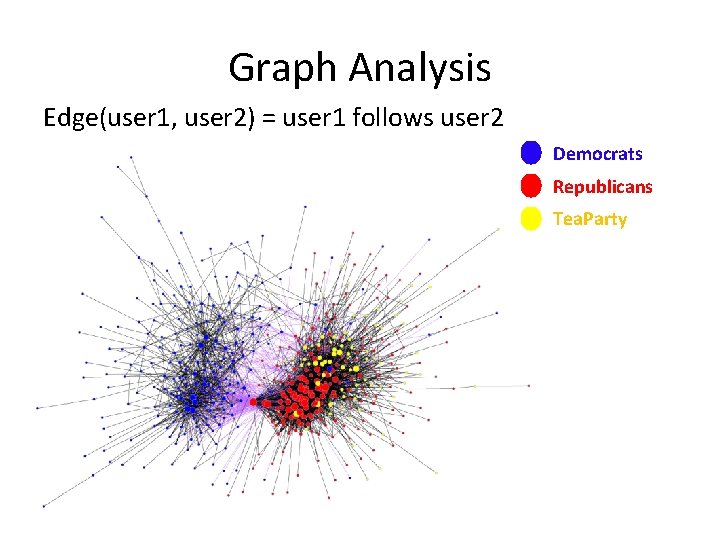
Graph Analysis Edge(user 1, user 2) = user 1 follows user 2 Democrats Republicans Tea. Party
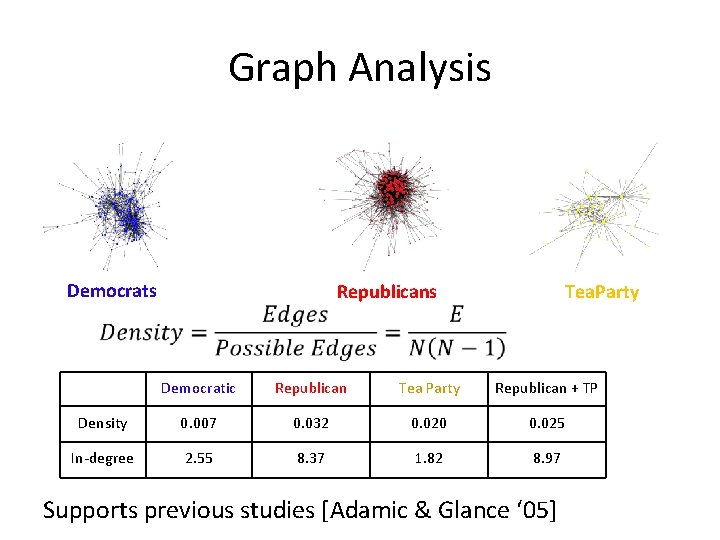
Graph Analysis Democrats Republicans Tea. Party Democratic Republican Tea Party Republican + TP Density 0. 007 0. 032 0. 020 0. 025 In-degree 2. 55 8. 37 1. 82 8. 97 Supports previous studies [Adamic & Glance ‘ 05]
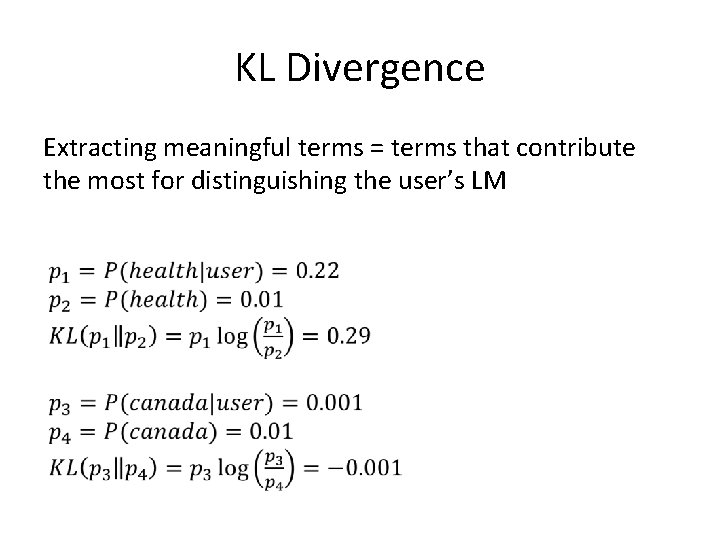
KL Divergence Extracting meaningful terms = terms that contribute the most for distinguishing the user’s LM
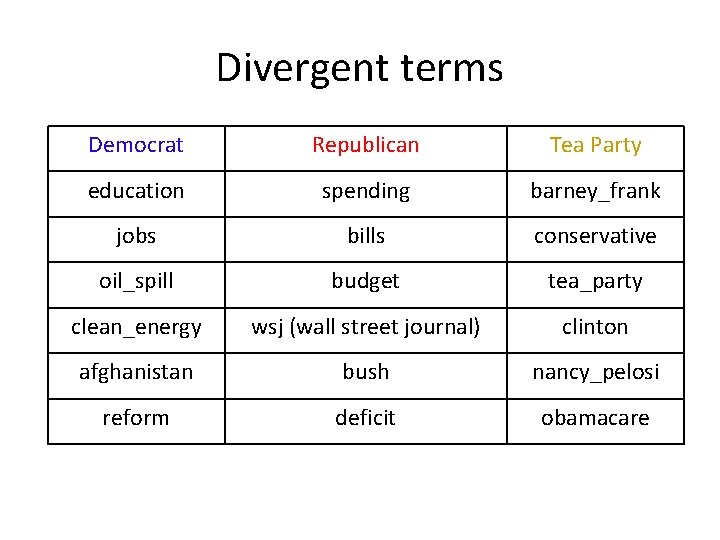
Divergent terms Democrat Republican Tea Party education spending barney_frank jobs bills conservative oil_spill budget tea_party clean_energy wsj (wall street journal) clinton afghanistan bush nancy_pelosi reform deficit obamacare
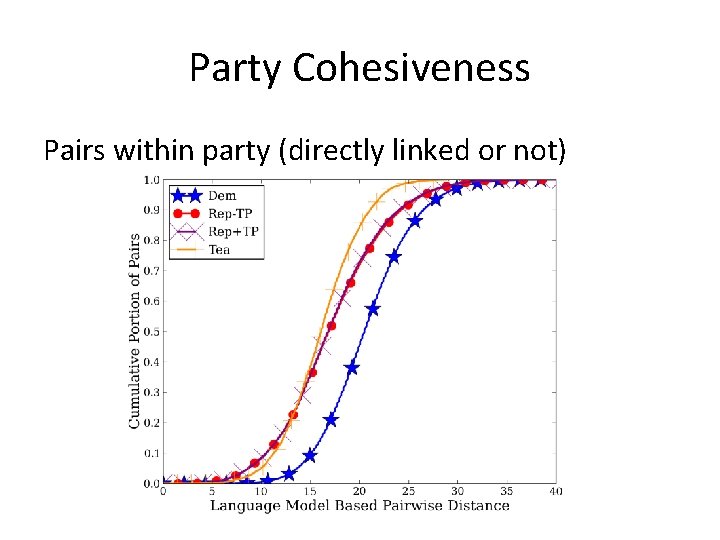
Party Cohesiveness Pairs within party (directly linked or not)
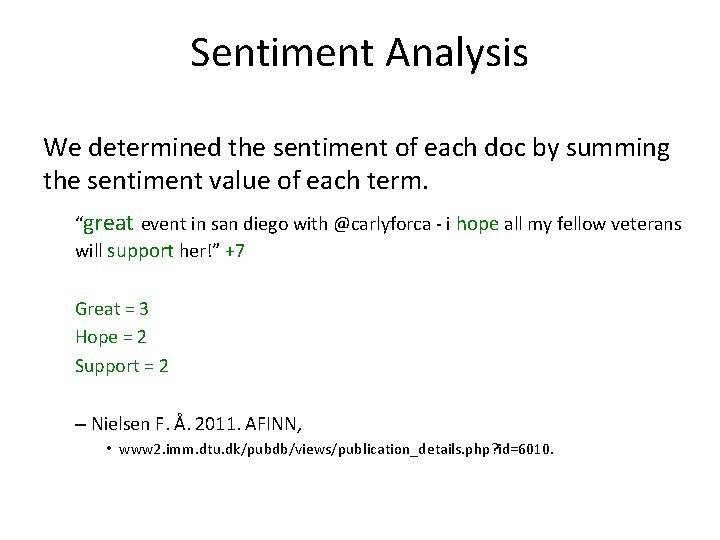
Sentiment Analysis We determined the sentiment of each doc by summing the sentiment value of each term. “great event in san diego with @carlyforca - i hope all my fellow veterans will support her!” +7 Great = 3 Hope = 2 Support = 2 – Nielsen F. Å. 2011. AFINN, • www 2. imm. dtu. dk/pubdb/views/publication_details. php? id=6010.
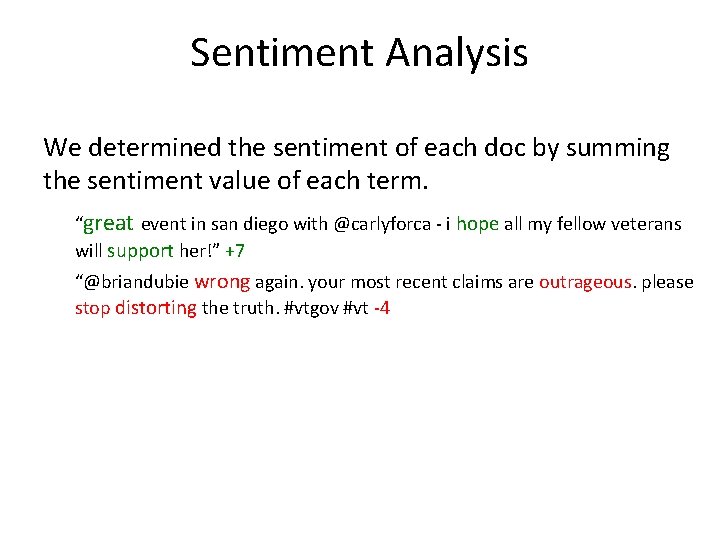
Sentiment Analysis We determined the sentiment of each doc by summing the sentiment value of each term. “great event in san diego with @carlyforca - i hope all my fellow veterans will support her!” +7 “@briandubie wrong again. your most recent claims are outrageous. please stop distorting the truth. #vtgov #vt -4
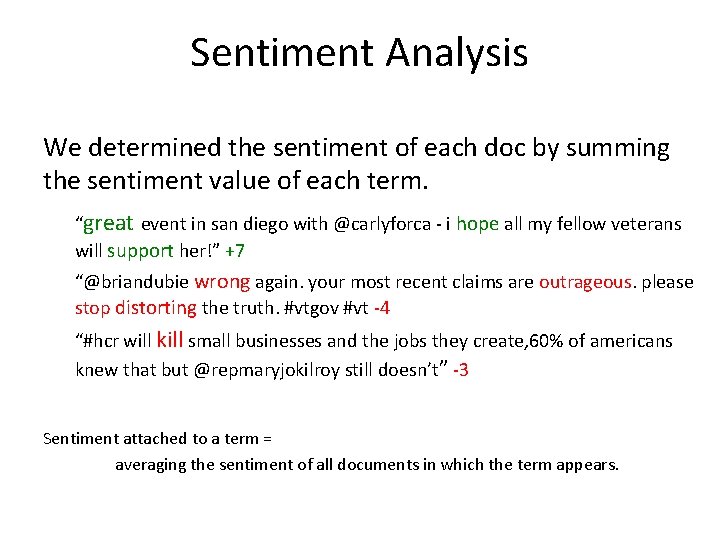
Sentiment Analysis We determined the sentiment of each doc by summing the sentiment value of each term. “great event in san diego with @carlyforca - i hope all my fellow veterans will support her!” +7 “@briandubie wrong again. your most recent claims are outrageous. please stop distorting the truth. #vtgov #vt -4 “#hcr will kill small businesses and the jobs they create, 60% of americans knew that but @repmaryjokilroy still doesn’t” -3 Sentiment attached to a term = averaging the sentiment of all documents in which the term appears.
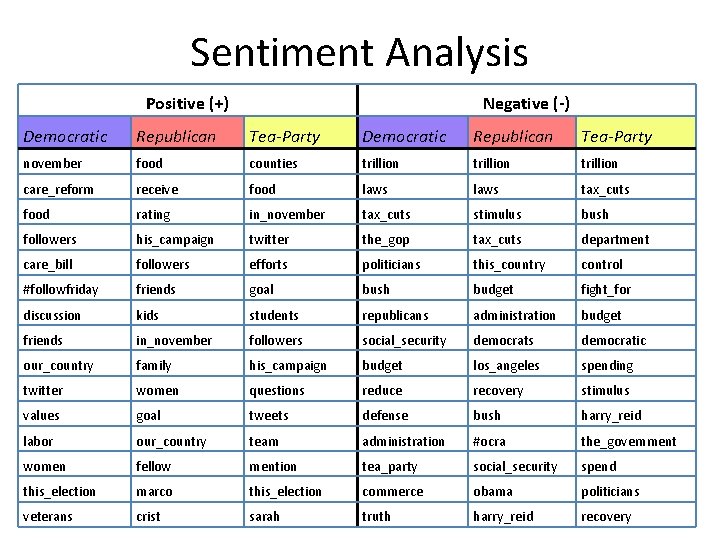
Sentiment Analysis Positive (+) Negative (-) Democratic Republican Tea-Party november food counties trillion care_reform receive food laws tax_cuts food rating in_november tax_cuts stimulus bush followers his_campaign twitter the_gop tax_cuts department care_bill followers efforts politicians this_country control #followfriday friends goal bush budget fight_for discussion kids students republicans administration budget friends in_november followers social_security democrats democratic our_country family his_campaign budget los_angeles spending twitter women questions reduce recovery stimulus values goal tweets defense bush harry_reid labor our_country team administration #ocra the_government women fellow mention tea_party social_security spend this_election marco this_election commerce obama politicians veterans crist sarah truth harry_reid recovery
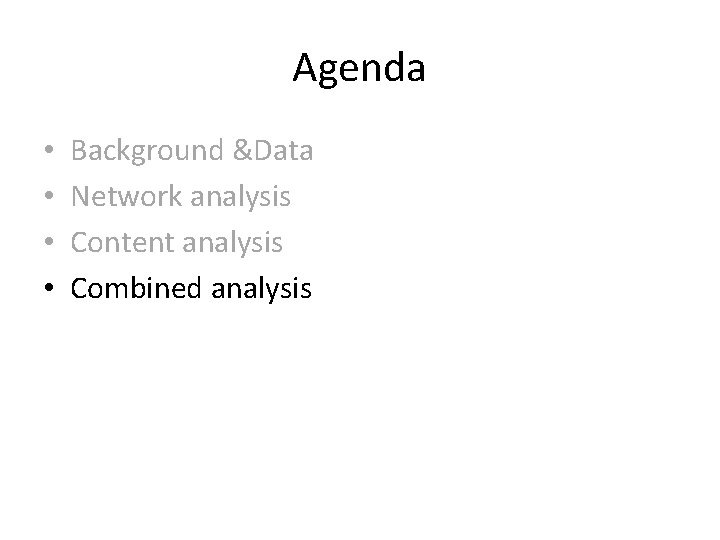
Agenda • • Background &Data Network analysis Content analysis Combined analysis
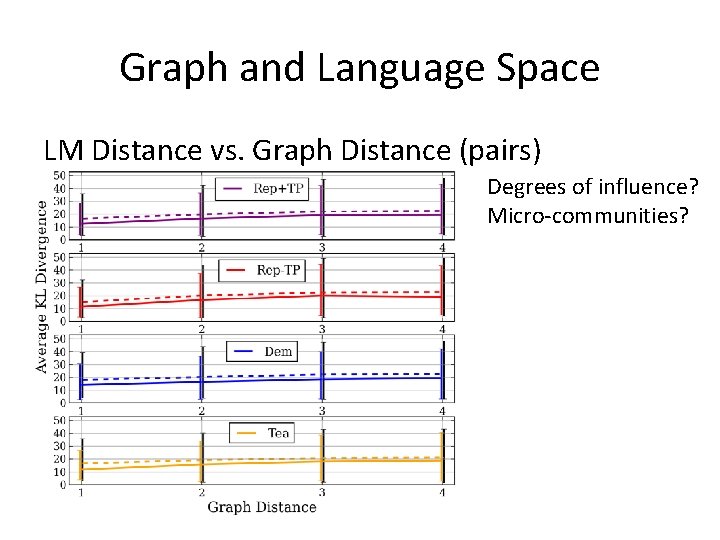
Graph and Language Space LM Distance vs. Graph Distance (pairs) Degrees of influence? Micro-communities?
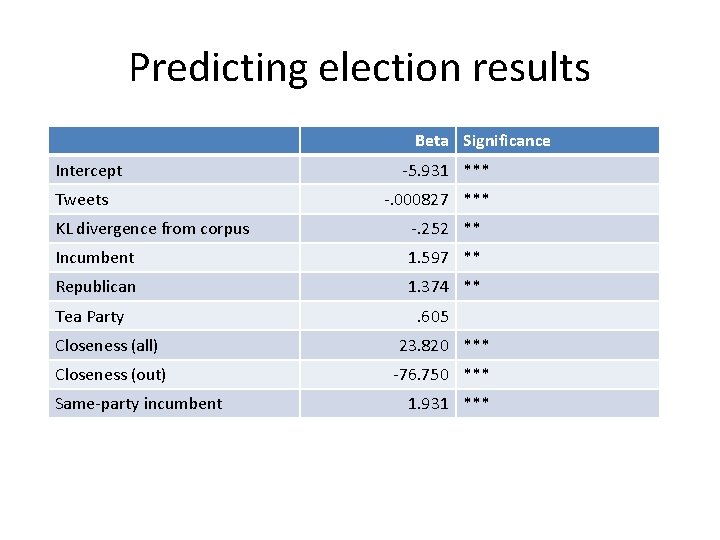
Predicting election results Beta Significance Intercept Tweets -5. 931 *** -. 000827 *** KL divergence from corpus -. 252 ** Incumbent 1. 597 ** Republican 1. 374 ** Tea Party . 605 Closeness (all) 23. 820 *** Closeness (out) -76. 750 *** Same-party incumbent 1. 931 ***
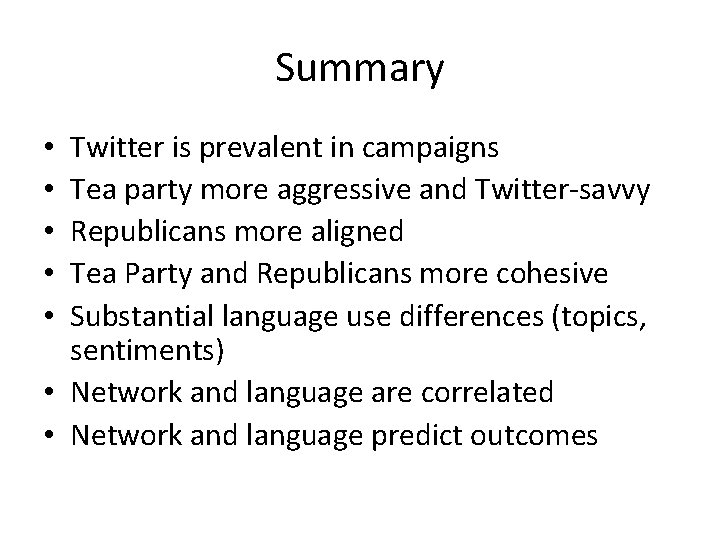
Summary Twitter is prevalent in campaigns Tea party more aggressive and Twitter-savvy Republicans more aligned Tea Party and Republicans more cohesive Substantial language use differences (topics, sentiments) • Network and language are correlated • Network and language predict outcomes • • •
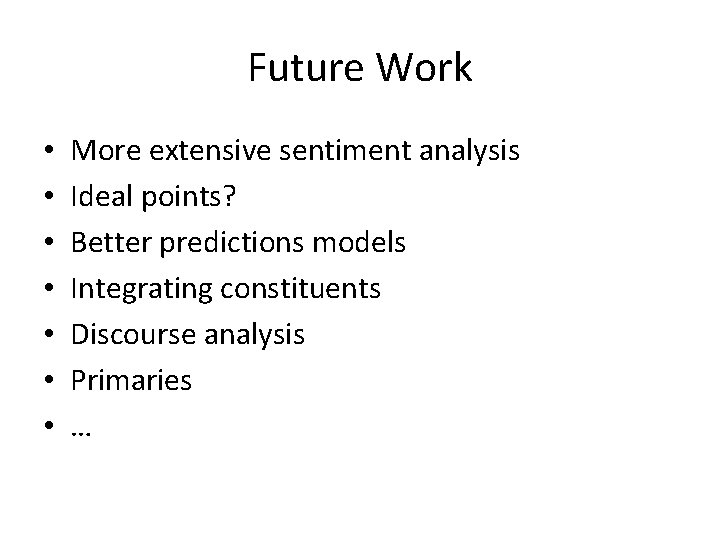
Future Work • • More extensive sentiment analysis Ideal points? Better predictions models Integrating constituents Discourse analysis Primaries …
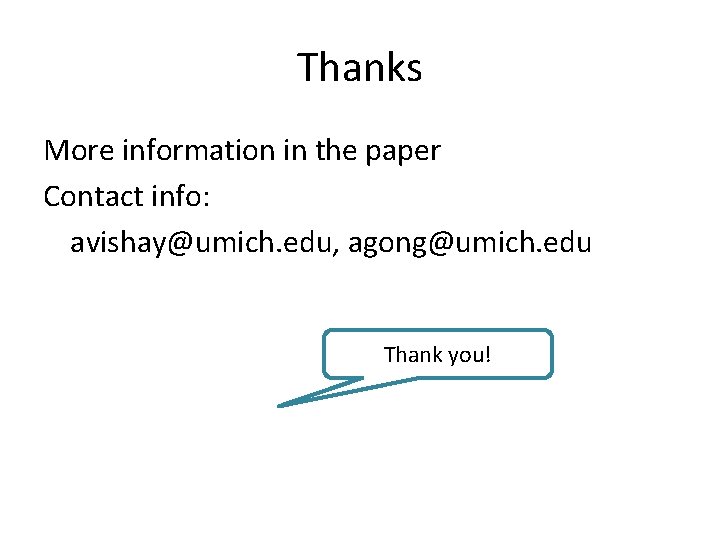
Thanks More information in the paper Contact info: avishay@umich. edu, agong@umich. edu Thank you!
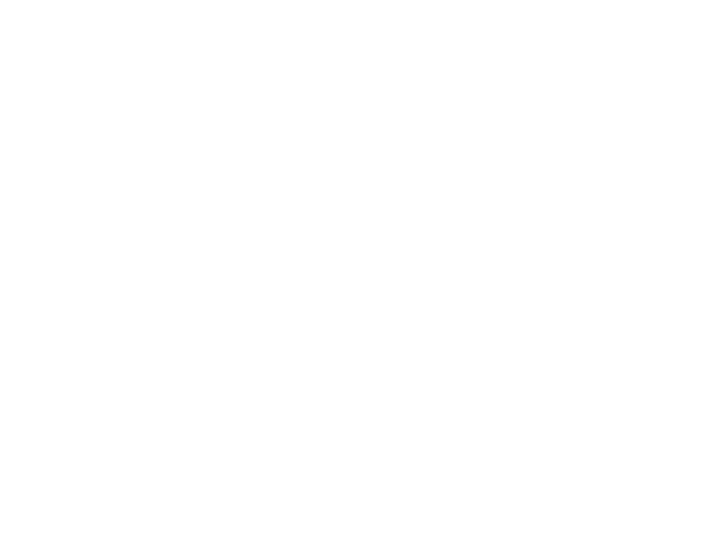
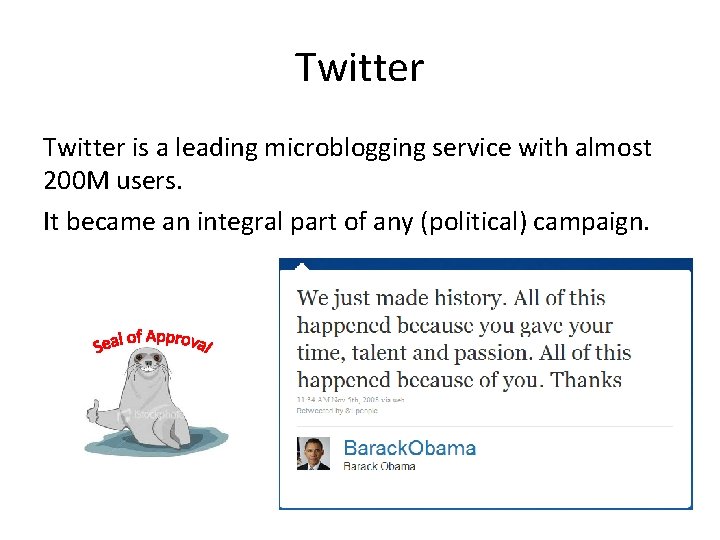
Twitter is a leading microblogging service with almost 200 M users. It became an integral part of any (political) campaign.
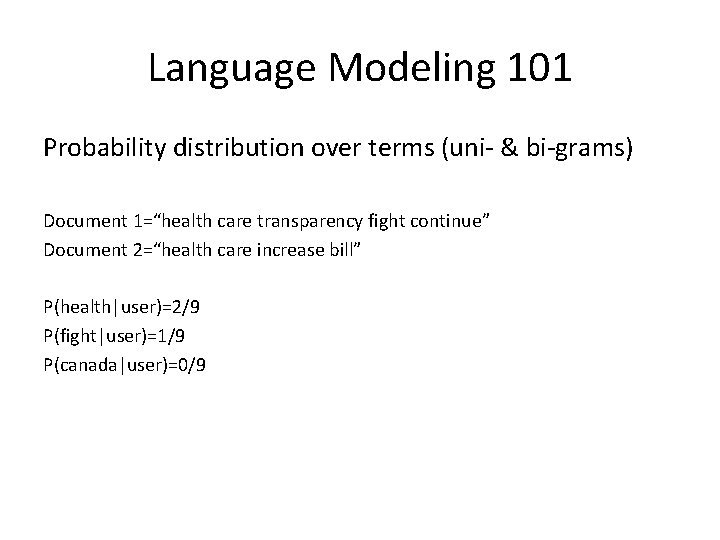
Language Modeling 101 Probability distribution over terms (uni- & bi-grams) Document 1=“health care transparency fight continue” Document 2=“health care increase bill” P(health|user)=2/9 P(fight|user)=1/9 P(canada|user)=0/9
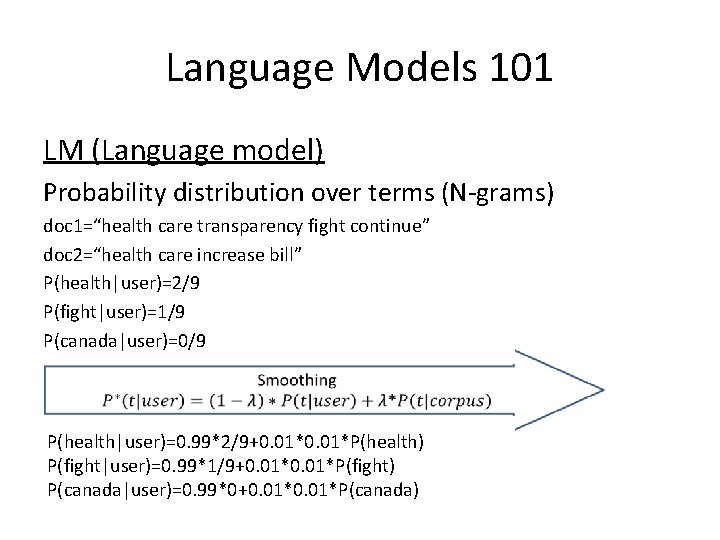
Language Models 101 LM (Language model) Probability distribution over terms (N-grams) doc 1=“health care transparency fight continue” doc 2=“health care increase bill” P(health|user)=2/9 P(fight|user)=1/9 P(canada|user)=0/9 P(health|user)=0. 99*2/9+0. 01*P(health) P(fight|user)=0. 99*1/9+0. 01*P(fight) P(canada|user)=0. 99*0+0. 01*P(canada)
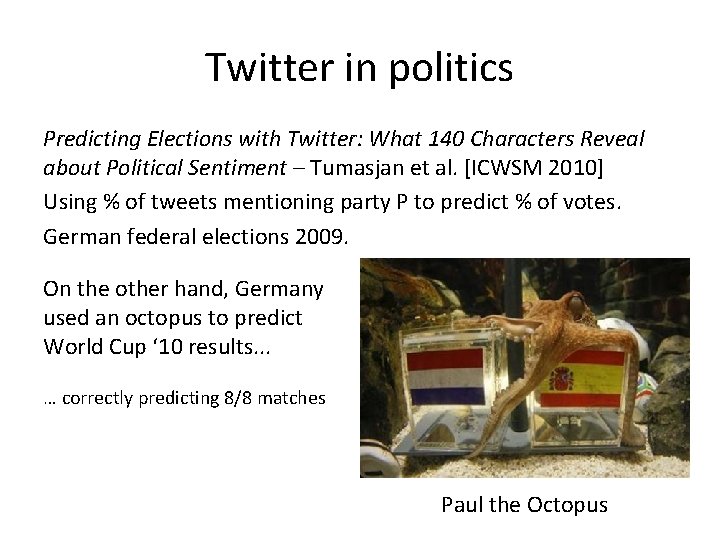
Twitter in politics Predicting Elections with Twitter: What 140 Characters Reveal about Political Sentiment – Tumasjan et al. [ICWSM 2010] Using % of tweets mentioning party P to predict % of votes. German federal elections 2009. On the other hand, Germany used an octopus to predict World Cup ‘ 10 results. . . … correctly predicting 8/8 matches Paul the Octopus
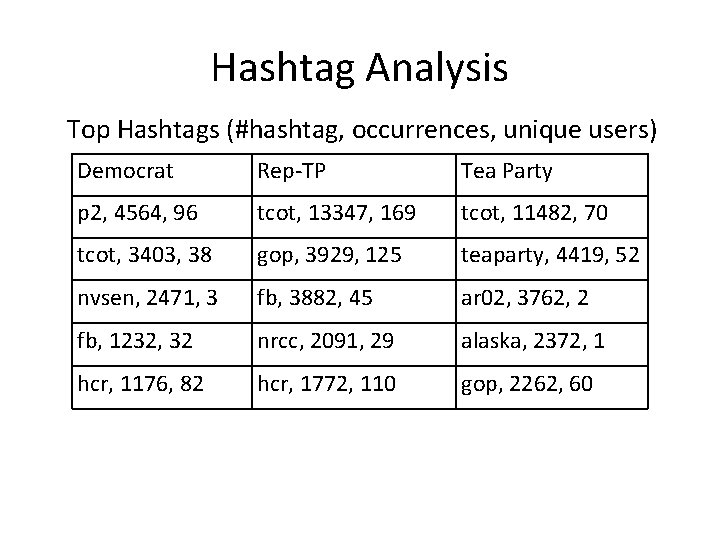
Hashtag Analysis Top Hashtags (#hashtag, occurrences, unique users) Democrat Rep-TP Tea Party p 2, 4564, 96 tcot, 13347, 169 tcot, 11482, 70 tcot, 3403, 38 gop, 3929, 125 teaparty, 4419, 52 nvsen, 2471, 3 fb, 3882, 45 ar 02, 3762, 2 fb, 1232, 32 nrcc, 2091, 29 alaska, 2372, 1 hcr, 1176, 82 hcr, 1772, 110 gop, 2262, 60
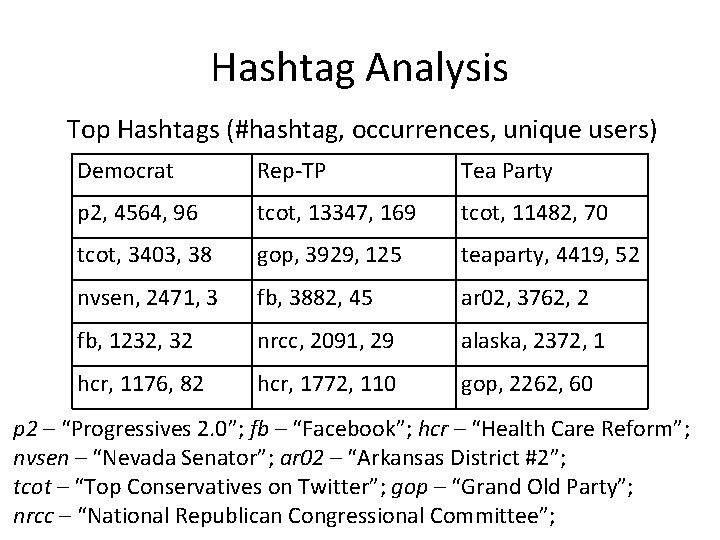
Hashtag Analysis Top Hashtags (#hashtag, occurrences, unique users) Democrat Rep-TP Tea Party p 2, 4564, 96 tcot, 13347, 169 tcot, 11482, 70 tcot, 3403, 38 gop, 3929, 125 teaparty, 4419, 52 nvsen, 2471, 3 fb, 3882, 45 ar 02, 3762, 2 fb, 1232, 32 nrcc, 2091, 29 alaska, 2372, 1 hcr, 1176, 82 hcr, 1772, 110 gop, 2262, 60 p 2 – “Progressives 2. 0”; fb – “Facebook”; hcr – “Health Care Reform”; nvsen – “Nevada Senator”; ar 02 – “Arkansas District #2”; tcot – “Top Conservatives on Twitter”; gop – “Grand Old Party”; nrcc – “National Republican Congressional Committee”;
![Topic Modeling LDA We extracted latent topics using LDA Blei Ng Jordan 2002 Topic Modeling (LDA) • We extracted latent topics using LDA [Blei, Ng, Jordan 2002]](https://slidetodoc.com/presentation_image_h2/96baff93cdcd885948fa47d2264a4391/image-28.jpg)
Topic Modeling (LDA) • We extracted latent topics using LDA [Blei, Ng, Jordan 2002] • Which outputs the affinity of documents with each topic. • By averaging over candidate’s document we determined the affinity of a candidate with each topic. • By averaging over candidates we determined the affinity of a party with each topic. • “Topic” is defined as a distribution over the terms in the corpus (how related is each term to the topic).
![Topics Topic terms Affinity Difference tax jobs spending Obama stimulus 0 047618 health care Topics Topic terms Affinity Difference tax, jobs, spending, [O]bama, stimulus -0. 047618 health, care,](https://slidetodoc.com/presentation_image_h2/96baff93cdcd885948fa47d2264a4391/image-29.jpg)
Topics Topic terms Affinity Difference tax, jobs, spending, [O]bama, stimulus -0. 047618 health, care, bill, house, reform -0. 032136 tcot, barney, teaparty, [Sean Bielat], twisters -0. 020878 live, show, interview, radio, fox -0. 018375 posted, facebook, photos, video, check -0. 014608 ff, great, followfriday, twitter, followers -0. 012113 obama, people, dont, good, government -0. 010277 great, county, meeting, day, tonight -0. 007769 campaign, tcot, twitter, facebook, support -0. 007624 john, david, ad, [P]elosi, [Sharron A]ngle -0. 002737 vote, endorsement, [H]armer, ca 10, candidate -0. 001998 change, view, changed, committee, energy 0. 002625 great, day, parade, good, time 0. 002746 ar 02, ar 2, [T]im [Griffin], vote, join 0. 003104 [O]bama, oil, president, hearing, bp 0. 007417 day, happy, great, women, honor 0. 018366 vote, day, early, election, voting 0. 022653 bill, house, voted, senate, reform 0. 028481
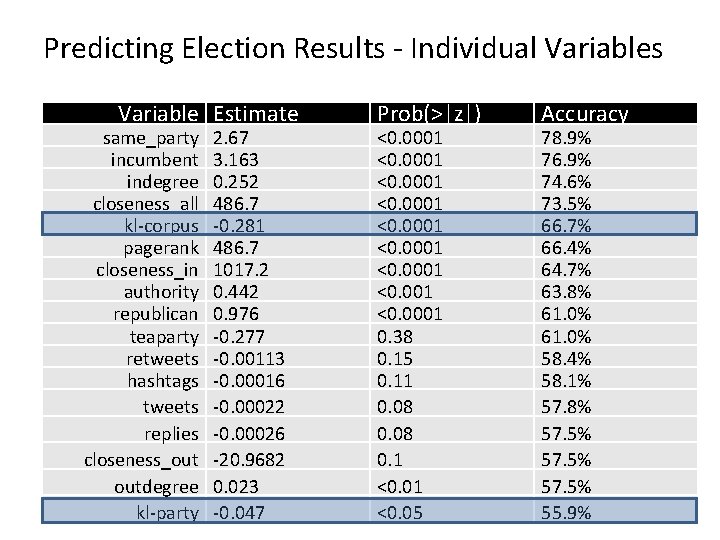
Predicting Election Results - Individual Variables Variable Estimate same_party incumbent indegree closeness_all kl-corpus pagerank closeness_in authority republican teaparty retweets hashtags tweets replies closeness_out outdegree kl-party 2. 67 3. 163 0. 252 486. 7 -0. 281 486. 7 1017. 2 0. 442 0. 976 -0. 277 -0. 00113 -0. 00016 -0. 00022 -0. 00026 -20. 9682 0. 023 -0. 047 Prob(>|z|) <0. 0001 <0. 0001 0. 38 0. 15 0. 11 0. 08 0. 1 <0. 05 Accuracy 78. 9% 76. 9% 74. 6% 73. 5% 66. 7% 66. 4% 64. 7% 63. 8% 61. 0% 58. 4% 58. 1% 57. 8% 57. 5% 55. 9%
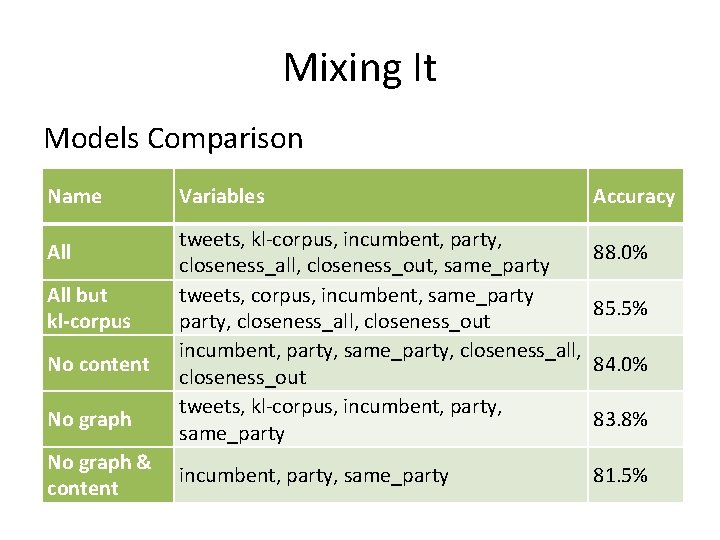
Mixing It Models Comparison Name All but kl-corpus No content No graph & content Variables tweets, kl-corpus, incumbent, party, closeness_all, closeness_out, same_party tweets, corpus, incumbent, same_party, closeness_all, closeness_out incumbent, party, same_party, closeness_all, closeness_out tweets, kl-corpus, incumbent, party, same_party Accuracy 88. 0% 85. 5% 84. 0% 83. 8% 81. 5%
Stalin promise free elections
African charter on democracy, elections and governance
Presidential elections exploration and announcement
Conclusion on elections
Governing marriage laws and conducting elections
Ap lang synthesis essay television presidential elections
David becker elections
“elections are key to democracy”
Trời xanh đây là của chúng ta thể thơ
Các số nguyên tố
Tỉ lệ cơ thể trẻ em
Fecboak
Các châu lục và đại dương trên thế giới
Thế nào là hệ số cao nhất
ưu thế lai là gì
Hệ hô hấp
Tư thế ngồi viết
đặc điểm cơ thể của người tối cổ
Bàn tay mà dây bẩn
Hình ảnh bộ gõ cơ thể búng tay
Mật thư tọa độ 5x5
Tư thế ngồi viết
Gấu đi như thế nào
Thẻ vin
Thể thơ truyền thống
Các châu lục và đại dương trên thế giới
Từ ngữ thể hiện lòng nhân hậu
Diễn thế sinh thái là
Slidetodoc
Ví dụ về giọng cùng tên
Vẽ hình chiếu vuông góc của vật thể sau
101012 bằng