Statistics and Data Analysis Professor William Greene Stern
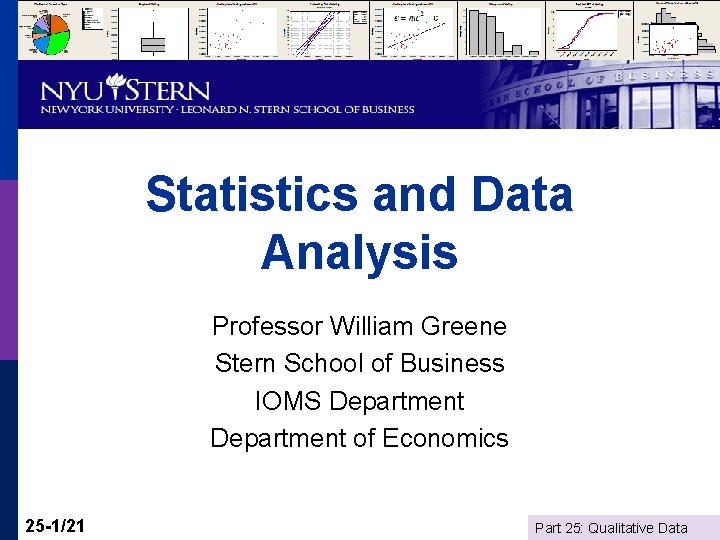
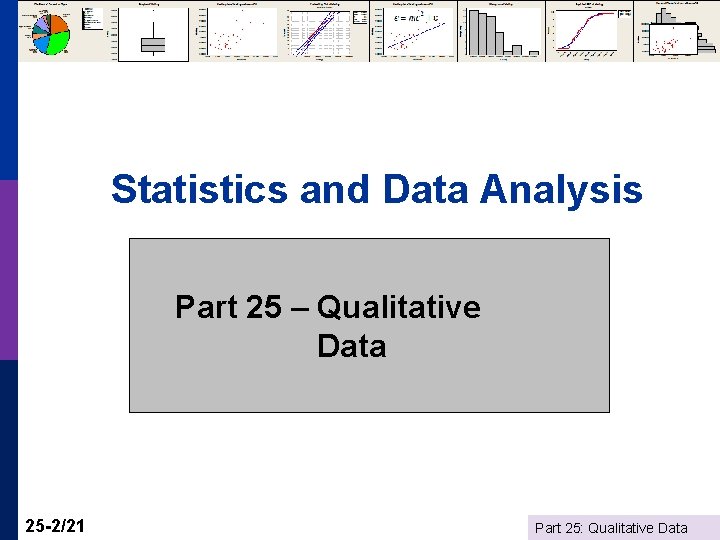
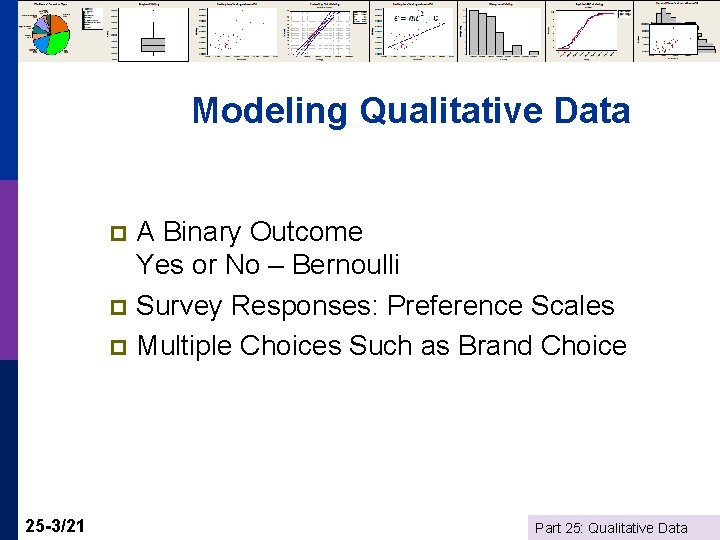
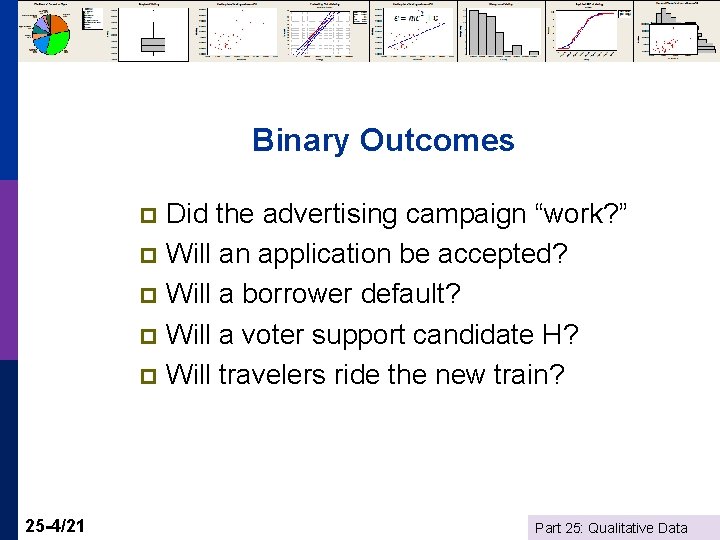
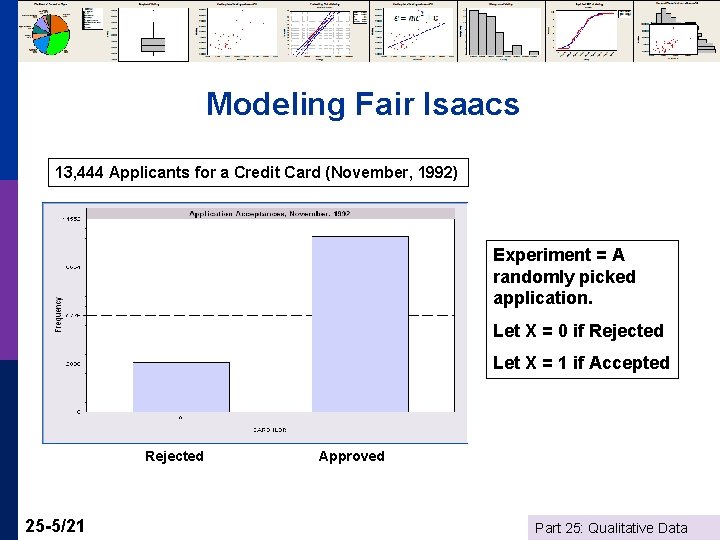
![Modelling The Probability p p 25 -6/21 Prob[Accept Application] = θ Prob[Reject Application ] Modelling The Probability p p 25 -6/21 Prob[Accept Application] = θ Prob[Reject Application ]](https://slidetodoc.com/presentation_image_h2/a92c07032cf4d6df6638fffeac9dd388/image-6.jpg)
![Bernoulli Regression p Prob[Accept] = θ = a function of n n n p Bernoulli Regression p Prob[Accept] = θ = a function of n n n p](https://slidetodoc.com/presentation_image_h2/a92c07032cf4d6df6638fffeac9dd388/image-7.jpg)
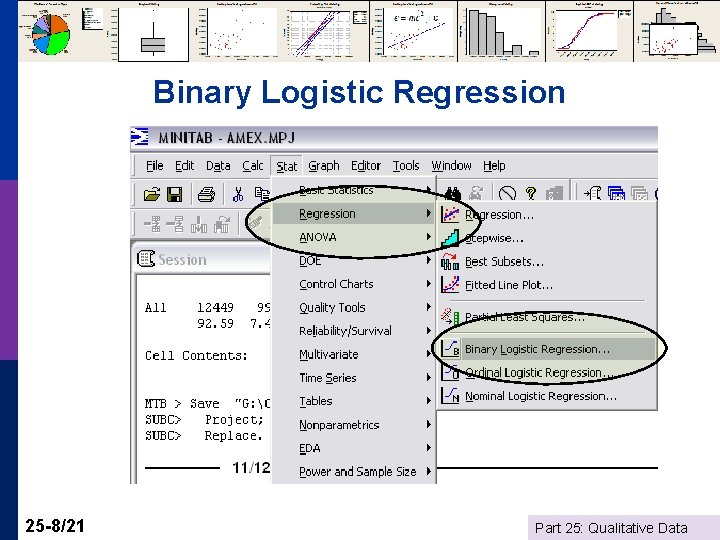
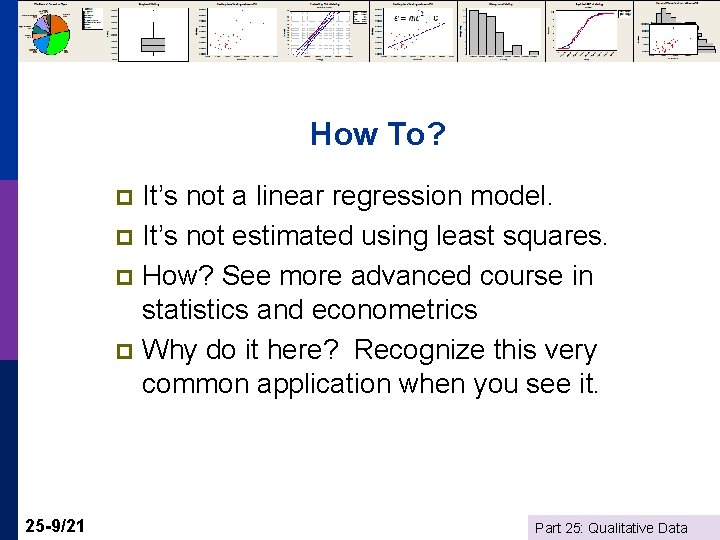
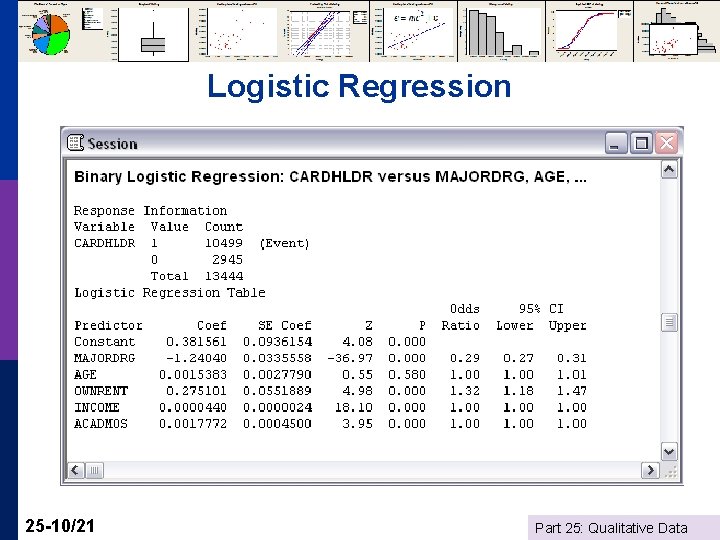
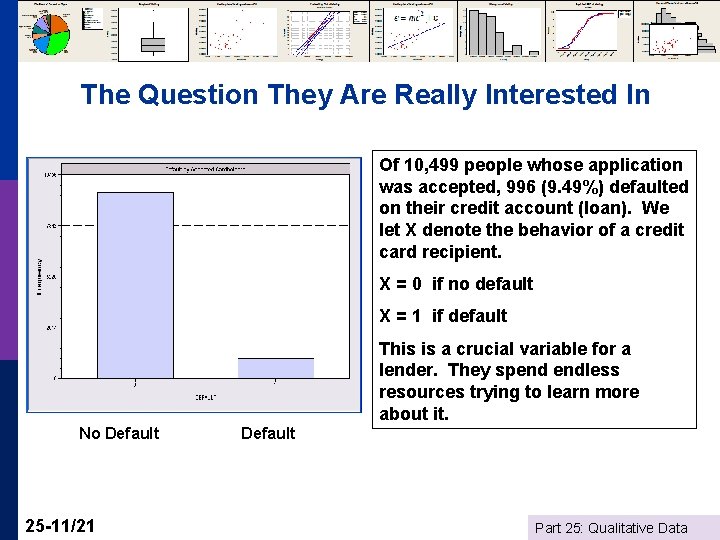
![A Statistical Model for Credit Scoring E[Profit per customer] = PD*E[Loss] + (1 -PD)*E[spending]*Merchant A Statistical Model for Credit Scoring E[Profit per customer] = PD*E[Loss] + (1 -PD)*E[spending]*Merchant](https://slidetodoc.com/presentation_image_h2/a92c07032cf4d6df6638fffeac9dd388/image-12.jpg)
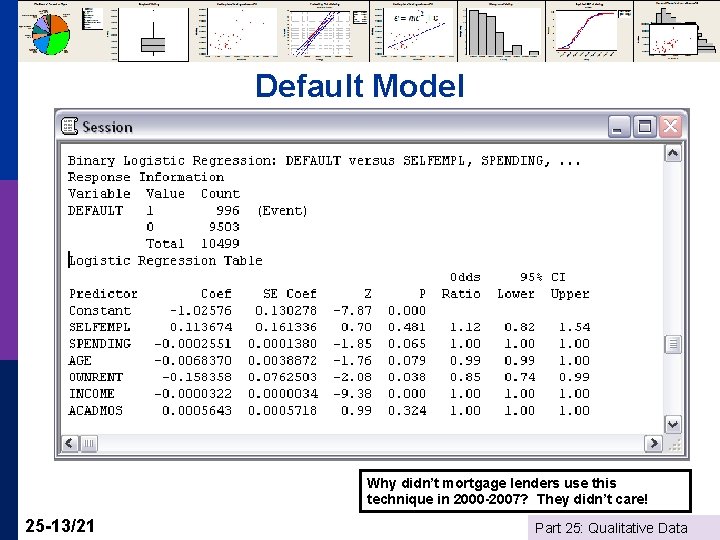
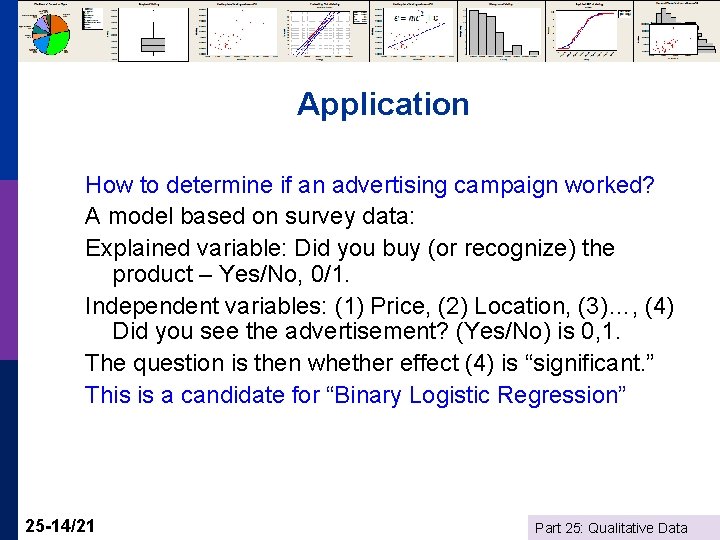
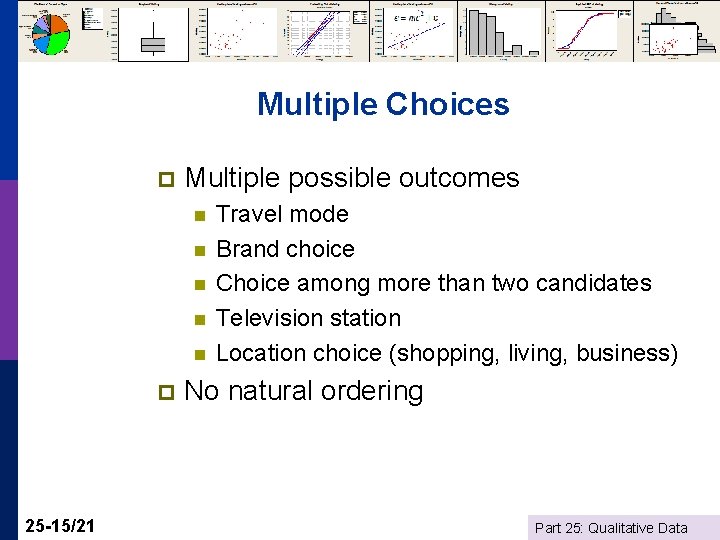
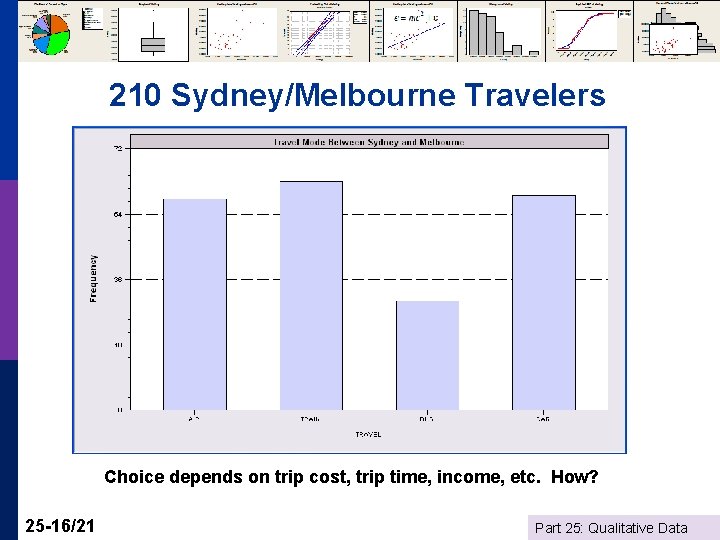
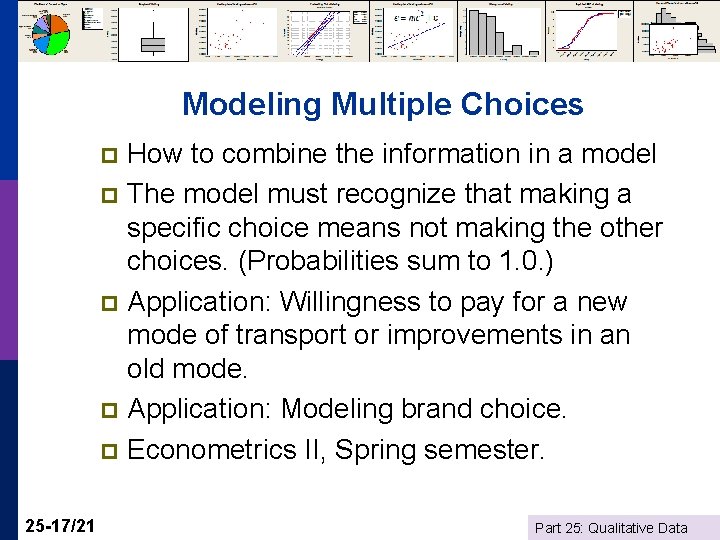
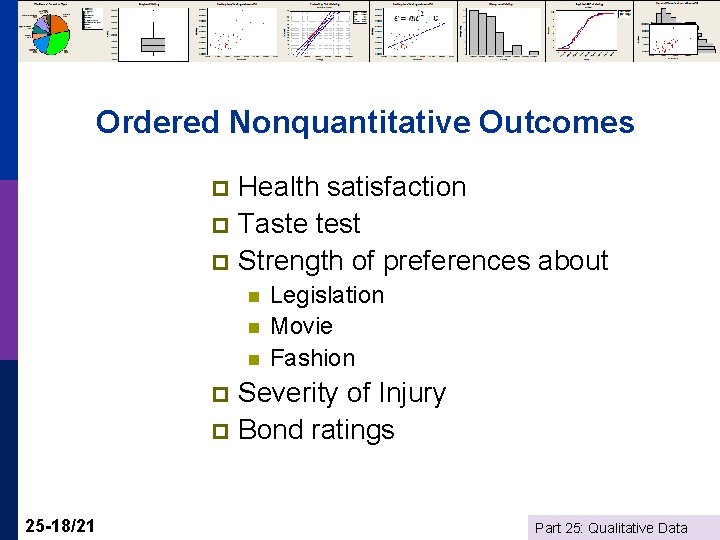
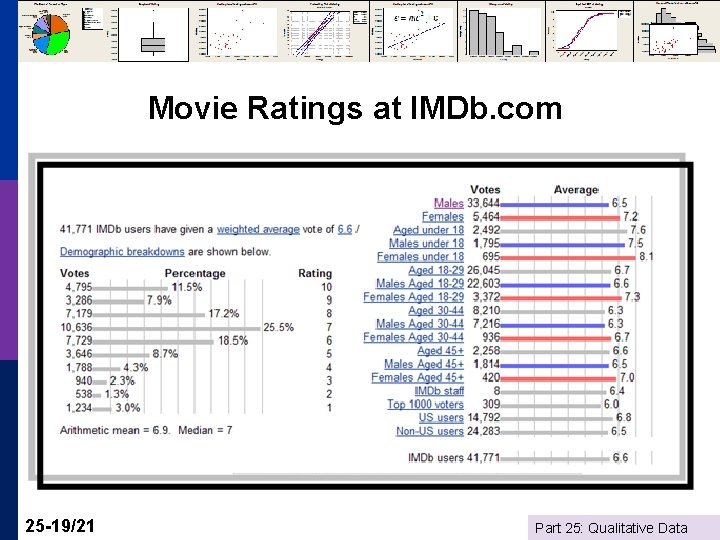
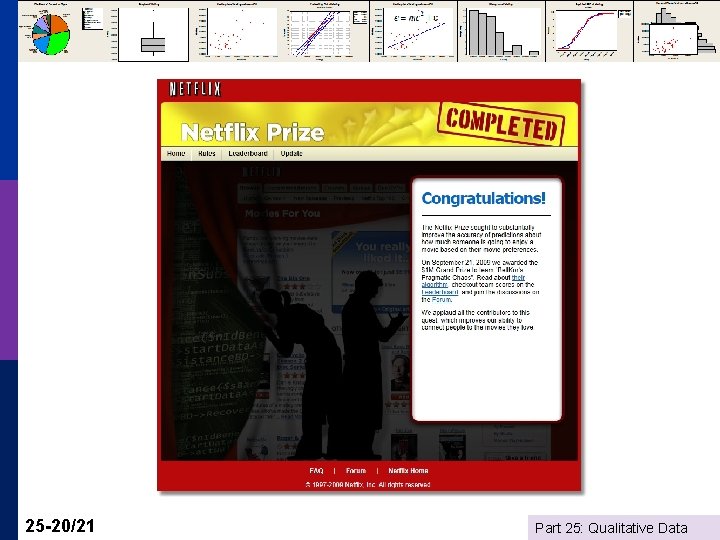
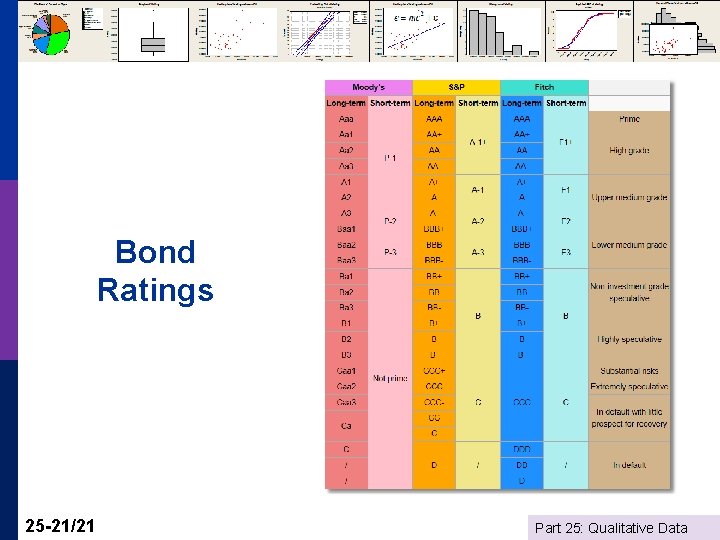
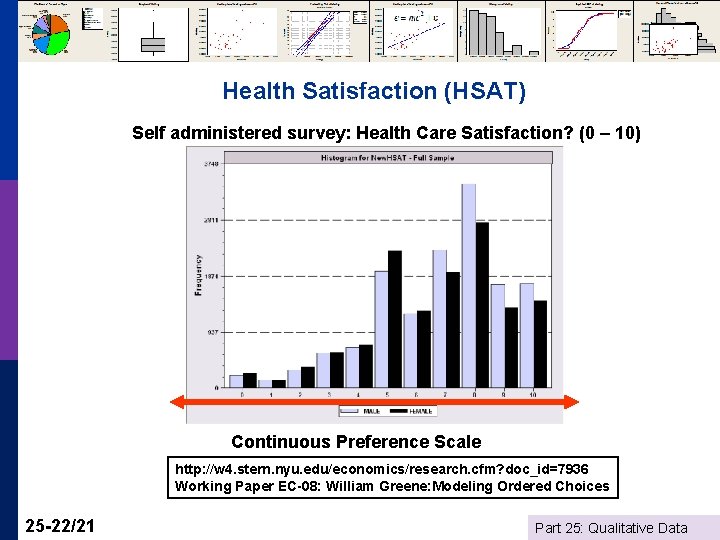
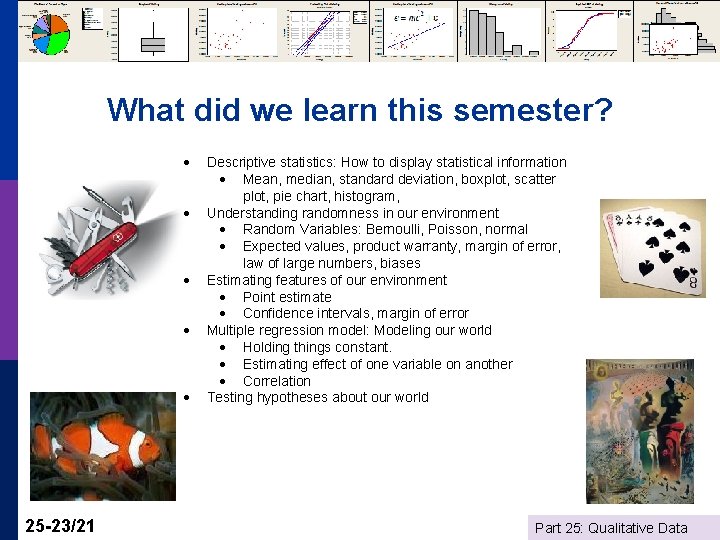
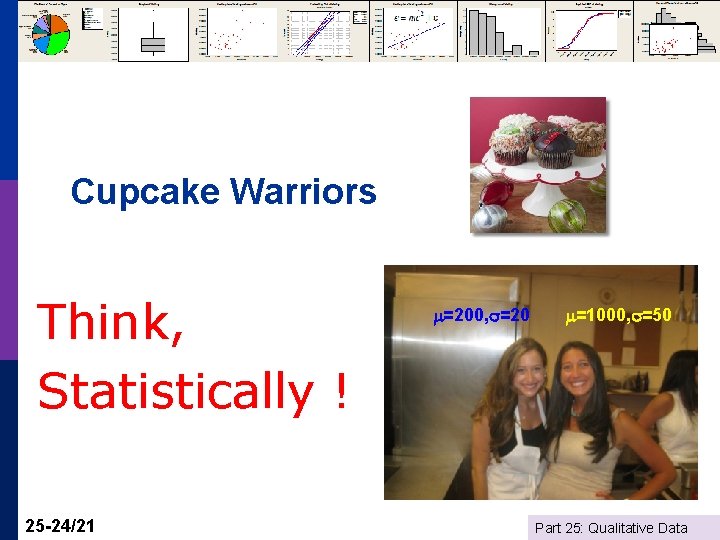
- Slides: 24
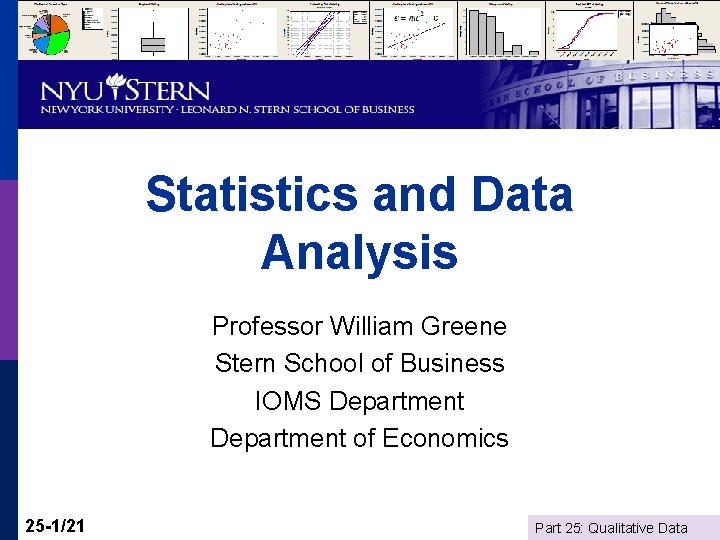
Statistics and Data Analysis Professor William Greene Stern School of Business IOMS Department of Economics 25 -1/21 Part 25: Qualitative Data
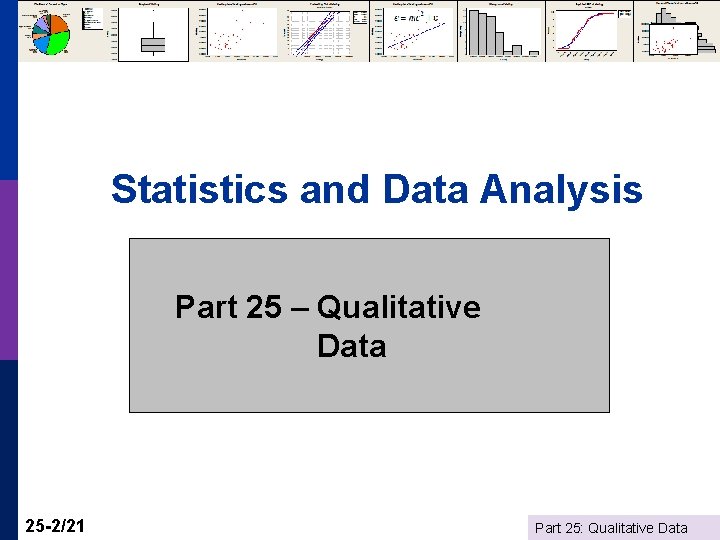
Statistics and Data Analysis Part 25 – Qualitative Data 25 -2/21 Part 25: Qualitative Data
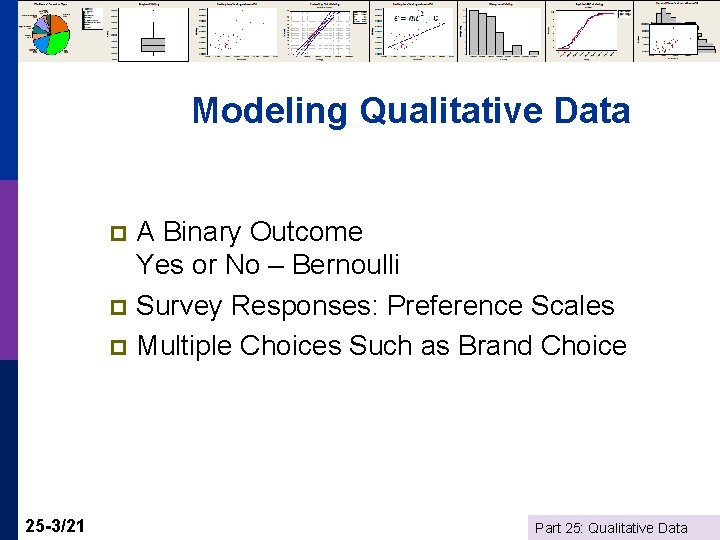
Modeling Qualitative Data A Binary Outcome Yes or No – Bernoulli p Survey Responses: Preference Scales p Multiple Choices Such as Brand Choice p 25 -3/21 Part 25: Qualitative Data
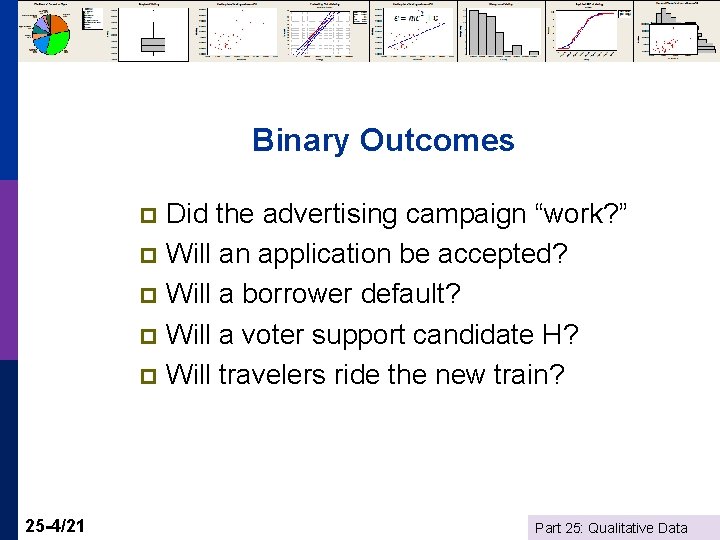
Binary Outcomes Did the advertising campaign “work? ” p Will an application be accepted? p Will a borrower default? p Will a voter support candidate H? p Will travelers ride the new train? p 25 -4/21 Part 25: Qualitative Data
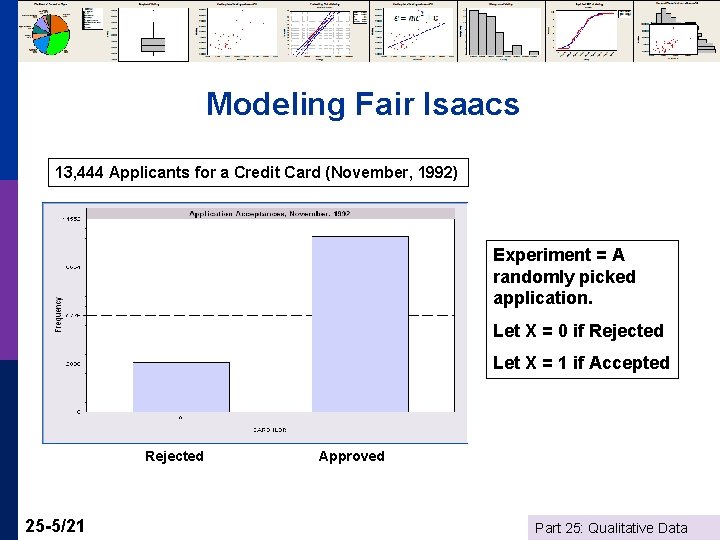
Modeling Fair Isaacs 13, 444 Applicants for a Credit Card (November, 1992) Experiment = A randomly picked application. Let X = 0 if Rejected Let X = 1 if Accepted Rejected 25 -5/21 Approved Part 25: Qualitative Data
![Modelling The Probability p p 25 621 ProbAccept Application θ ProbReject Application Modelling The Probability p p 25 -6/21 Prob[Accept Application] = θ Prob[Reject Application ]](https://slidetodoc.com/presentation_image_h2/a92c07032cf4d6df6638fffeac9dd388/image-6.jpg)
Modelling The Probability p p 25 -6/21 Prob[Accept Application] = θ Prob[Reject Application ] = 1 – θ Is that all there is? n Individual 1: Income = $100, 000, lived at the same address for 10 years, owns the home, no derogatory reports, age 35. n Individual 2: Income = $15, 000, just moved to the rental apartment, 10 major derogatory reports, age 22. n Same value of θ? ? Not likely. Part 25: Qualitative Data
![Bernoulli Regression p ProbAccept θ a function of n n n p Bernoulli Regression p Prob[Accept] = θ = a function of n n n p](https://slidetodoc.com/presentation_image_h2/a92c07032cf4d6df6638fffeac9dd388/image-7.jpg)
Bernoulli Regression p Prob[Accept] = θ = a function of n n n p p p 25 -7/21 Age Income Derogatory reports Length at address Own their home Looks like regression Is closely related to regression A way of handling outcomes (dependent variables) that are Yes/No, 0/1, etc. Part 25: Qualitative Data
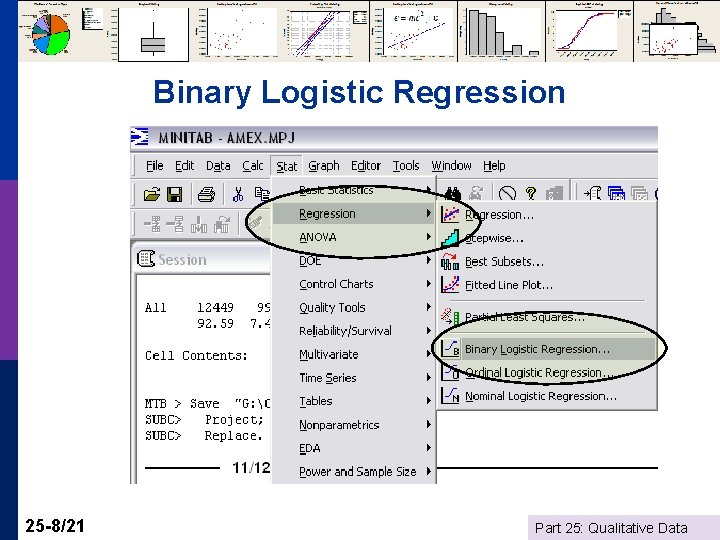
Binary Logistic Regression 25 -8/21 Part 25: Qualitative Data
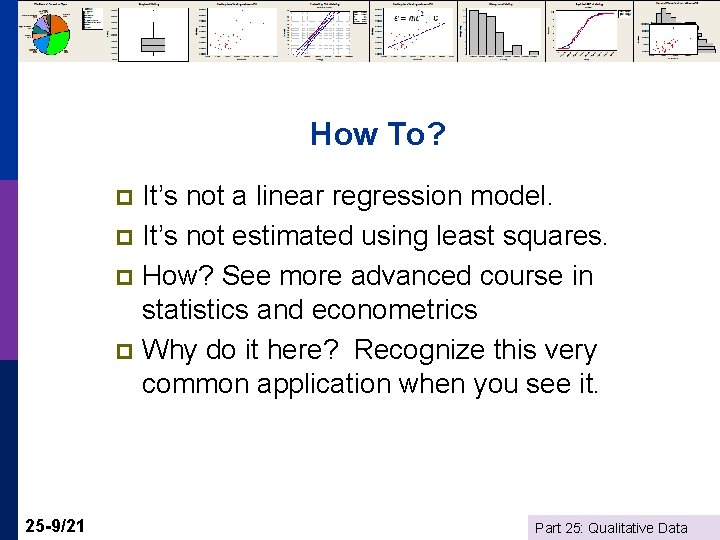
How To? It’s not a linear regression model. p It’s not estimated using least squares. p How? See more advanced course in statistics and econometrics p Why do it here? Recognize this very common application when you see it. p 25 -9/21 Part 25: Qualitative Data
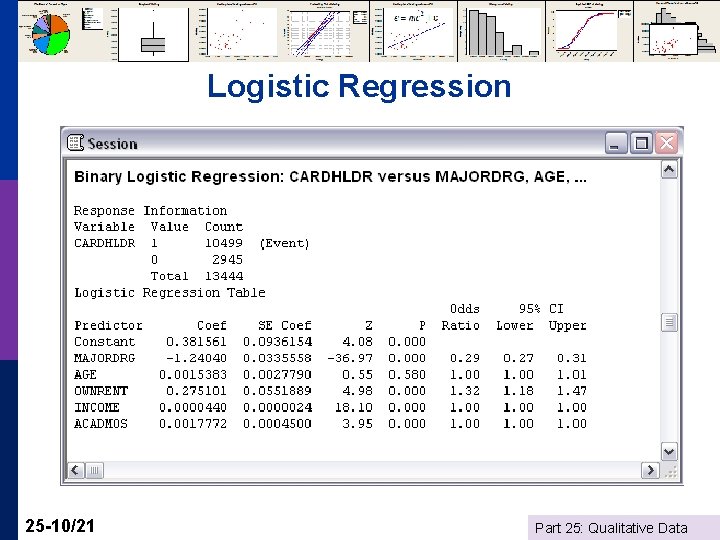
Logistic Regression 25 -10/21 Part 25: Qualitative Data
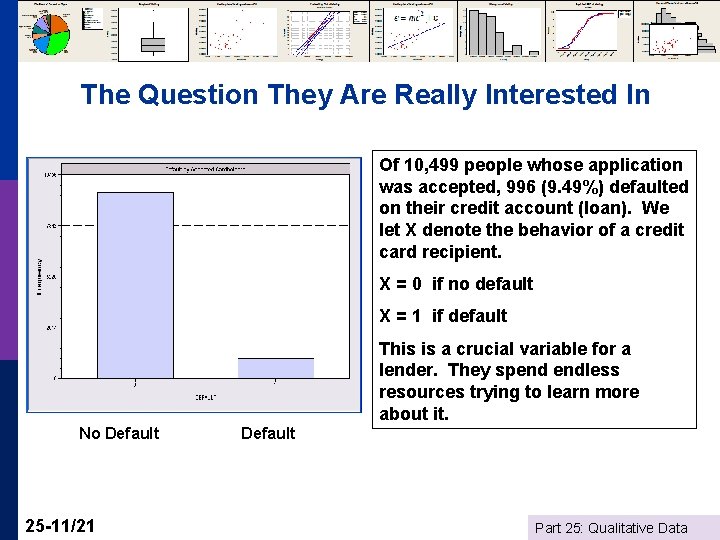
The Question They Are Really Interested In Of 10, 499 people whose application was accepted, 996 (9. 49%) defaulted on their credit account (loan). We let X denote the behavior of a credit card recipient. X = 0 if no default X = 1 if default This is a crucial variable for a lender. They spend endless resources trying to learn more about it. No Default 25 -11/21 Default Part 25: Qualitative Data
![A Statistical Model for Credit Scoring EProfit per customer PDELoss 1 PDEspendingMerchant A Statistical Model for Credit Scoring E[Profit per customer] = PD*E[Loss] + (1 -PD)*E[spending]*Merchant](https://slidetodoc.com/presentation_image_h2/a92c07032cf4d6df6638fffeac9dd388/image-12.jpg)
A Statistical Model for Credit Scoring E[Profit per customer] = PD*E[Loss] + (1 -PD)*E[spending]*Merchant Fees etc p E[Spending] = f(Income, Age, …, PD) Riskier customers spend more on average p E[Loss|Default] = Spending - Recovery (about half) p PD = F(Income, Age, Ownrent, …, Acceptance) p 25 -12/21 Part 25: Qualitative Data
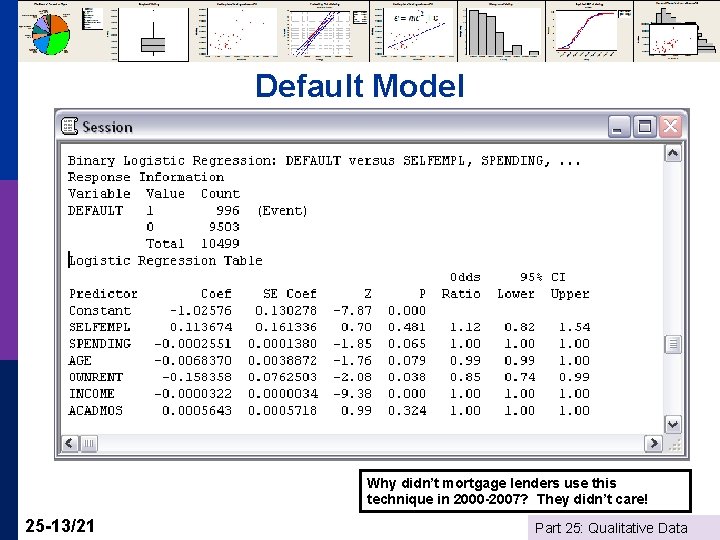
Default Model Why didn’t mortgage lenders use this technique in 2000 -2007? They didn’t care! 25 -13/21 Part 25: Qualitative Data
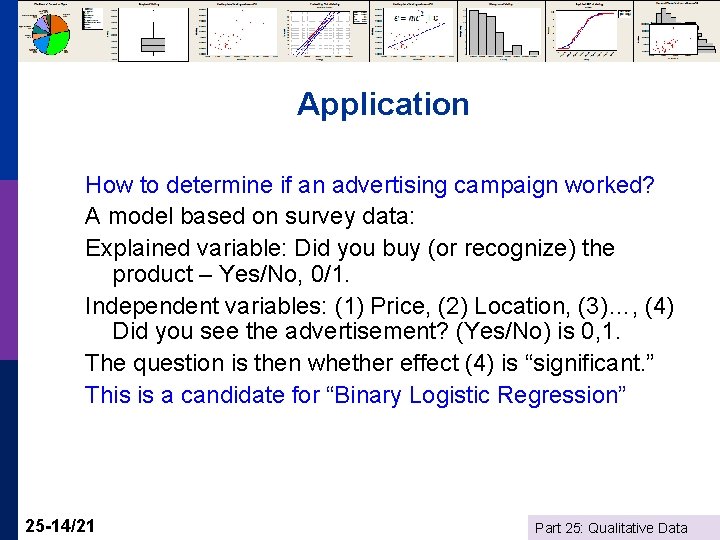
Application How to determine if an advertising campaign worked? A model based on survey data: Explained variable: Did you buy (or recognize) the product – Yes/No, 0/1. Independent variables: (1) Price, (2) Location, (3)…, (4) Did you see the advertisement? (Yes/No) is 0, 1. The question is then whether effect (4) is “significant. ” This is a candidate for “Binary Logistic Regression” 25 -14/21 Part 25: Qualitative Data
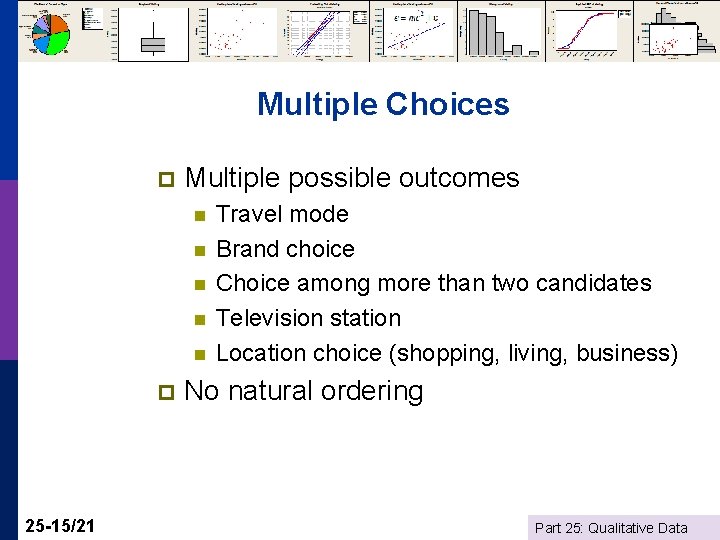
Multiple Choices p Multiple possible outcomes n n n p 25 -15/21 Travel mode Brand choice Choice among more than two candidates Television station Location choice (shopping, living, business) No natural ordering Part 25: Qualitative Data
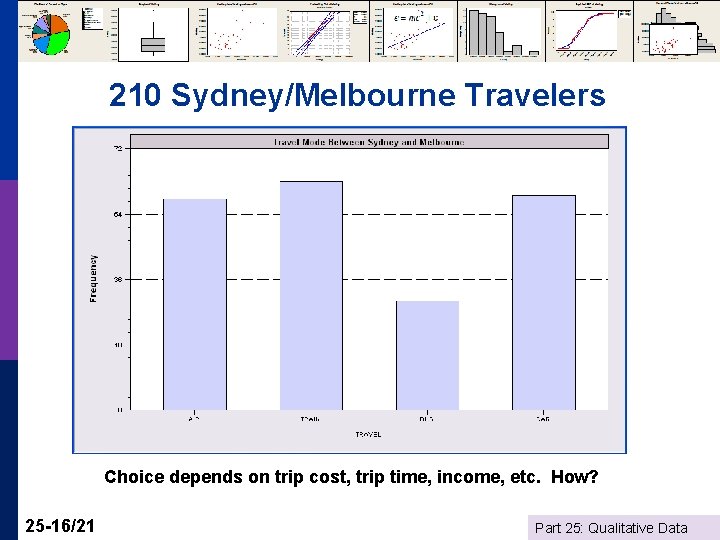
210 Sydney/Melbourne Travelers Choice depends on trip cost, trip time, income, etc. How? 25 -16/21 Part 25: Qualitative Data
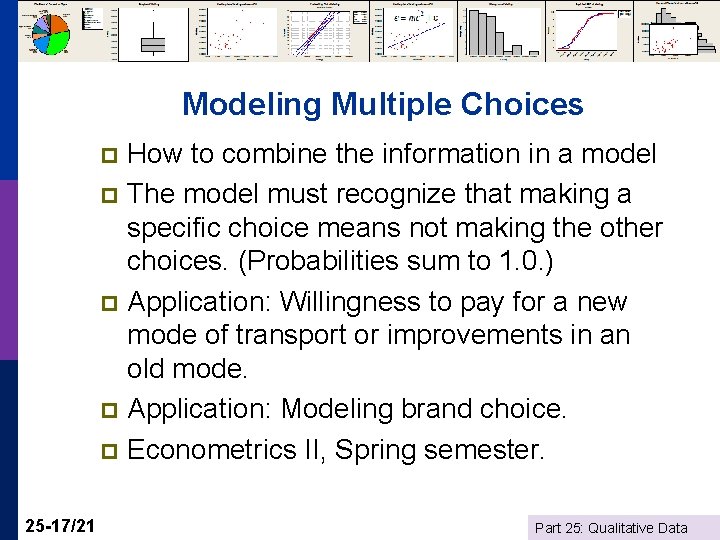
Modeling Multiple Choices How to combine the information in a model p The model must recognize that making a specific choice means not making the other choices. (Probabilities sum to 1. 0. ) p Application: Willingness to pay for a new mode of transport or improvements in an old mode. p Application: Modeling brand choice. p Econometrics II, Spring semester. p 25 -17/21 Part 25: Qualitative Data
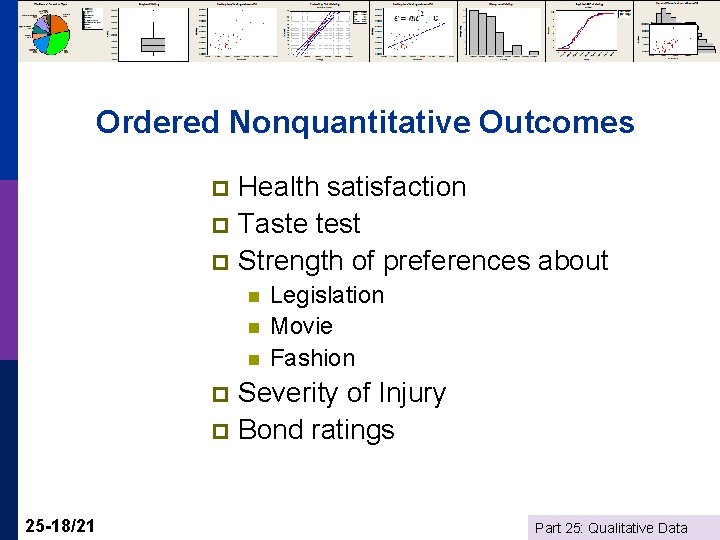
Ordered Nonquantitative Outcomes Health satisfaction p Taste test p Strength of preferences about p n n n Legislation Movie Fashion Severity of Injury p Bond ratings p 25 -18/21 Part 25: Qualitative Data
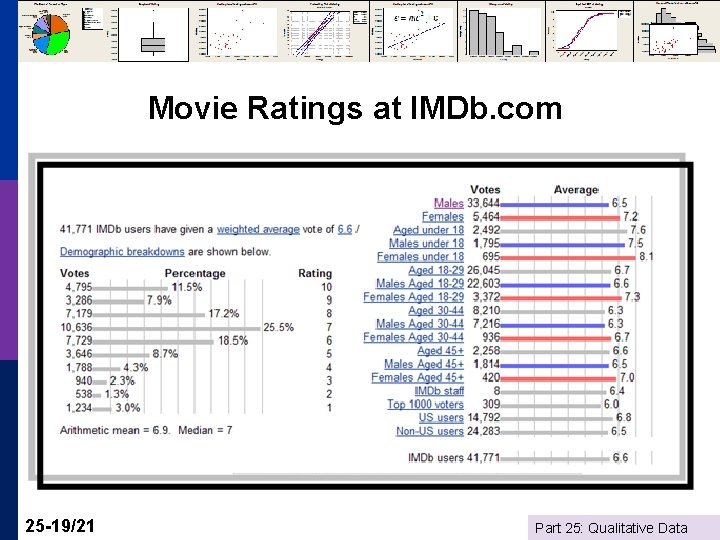
Movie Ratings at IMDb. com 25 -19/21 Part 25: Qualitative Data
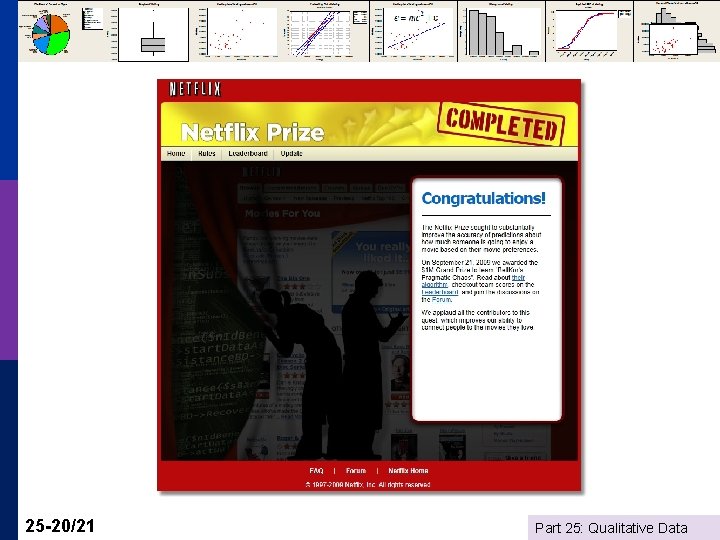
25 -20/21 Part 25: Qualitative Data
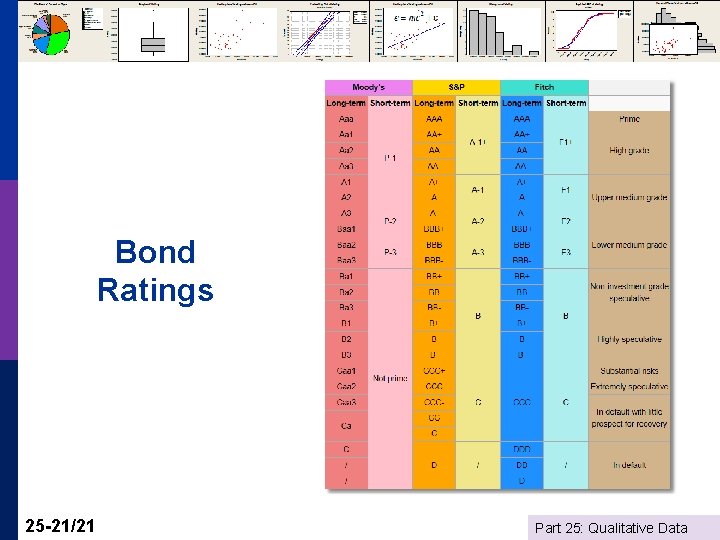
Bond Ratings 25 -21/21 Part 25: Qualitative Data
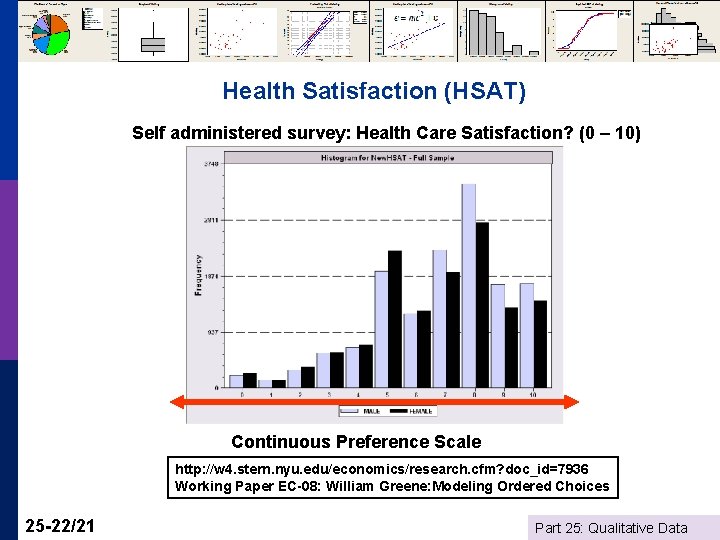
Health Satisfaction (HSAT) Self administered survey: Health Care Satisfaction? (0 – 10) Continuous Preference Scale http: //w 4. stern. nyu. edu/economics/research. cfm? doc_id=7936 Working Paper EC-08: William Greene: Modeling Ordered Choices 25 -22/21 Part 25: Qualitative Data
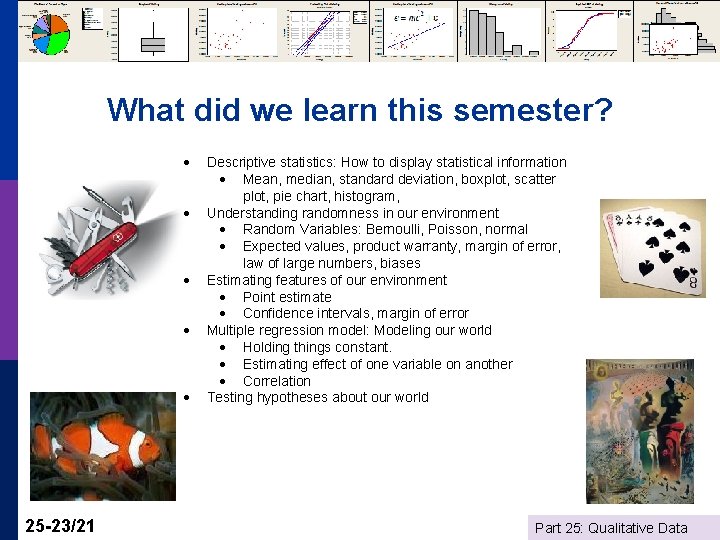
What did we learn this semester? · · · 25 -23/21 Descriptive statistics: How to display statistical information · Mean, median, standard deviation, boxplot, scatter plot, pie chart, histogram, Understanding randomness in our environment · Random Variables: Bernoulli, Poisson, normal · Expected values, product warranty, margin of error, law of large numbers, biases Estimating features of our environment · Point estimate · Confidence intervals, margin of error Multiple regression model: Modeling our world · Holding things constant. · Estimating effect of one variable on another · Correlation Testing hypotheses about our world Part 25: Qualitative Data
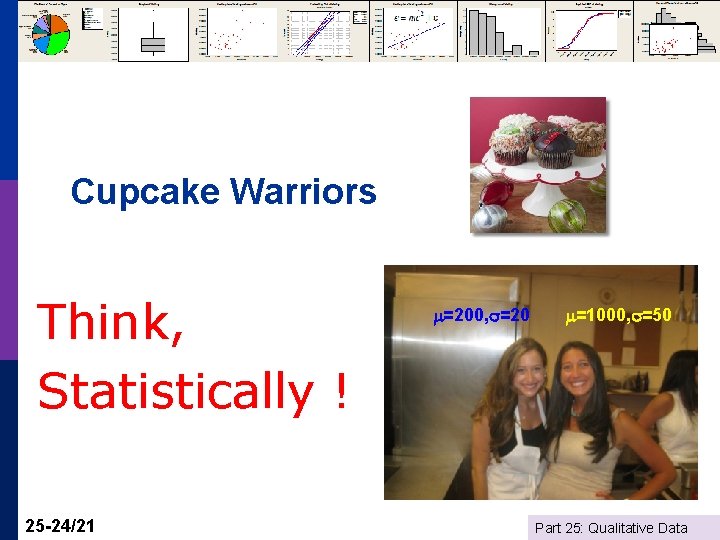
Cupcake Warriors Think, Statistically ! 25 -24/21 =200, =20 =1000, =50 Part 25: Qualitative Data
Aft end construction of ship
Promotion from associate professor to professor
Kylie greene
The tenth man graham greene summary
Stokes and green's theorem
Destructors graham greene
Linda r greene
Journey without maps graham greene
Robert greene shakespeare
Arin greene
Linda r greene
Livesinthebalance alsup
Adult expectations
Maxine greene releasing the imagination
Ericka greene md
Eric greene course
Charismatic conversation secrets
Uzuri pease-greene
Robin dunkin ucsc
Introduction to statistics what is statistics
Essential statistics william navidi pdf
William navidi elementary statistics pdf
William navidi essential statistics pdf
Essential statistics william navidi pdf
Barry navidi