Statistical Tests Karen H Hagglund M S Karen
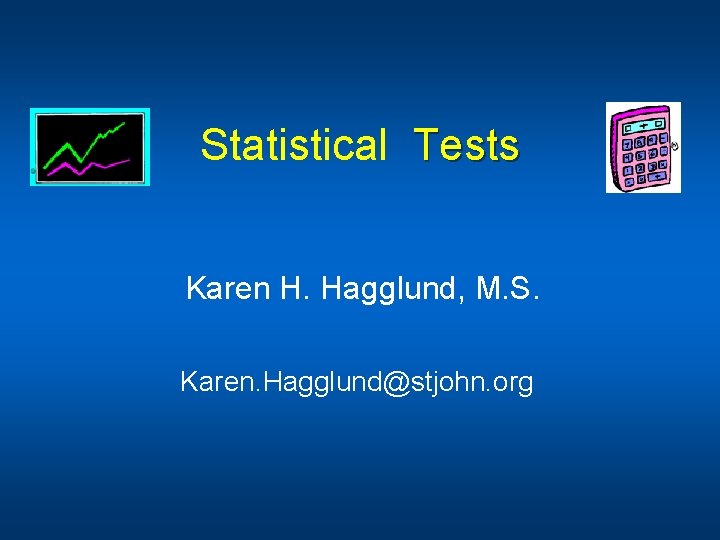
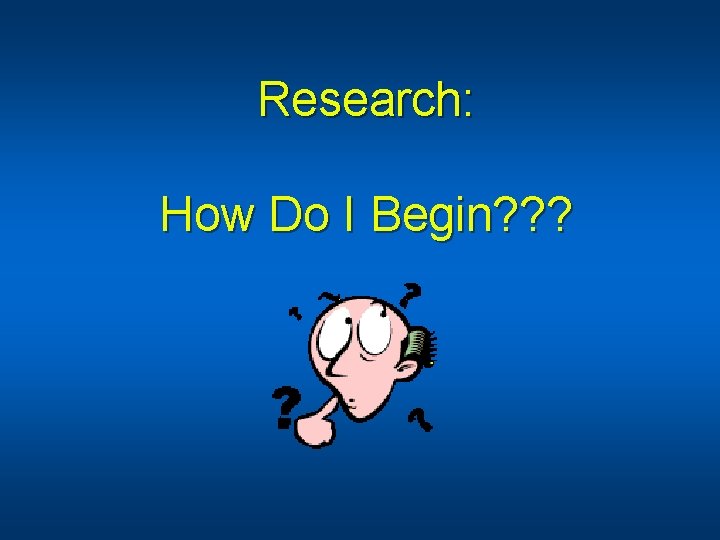
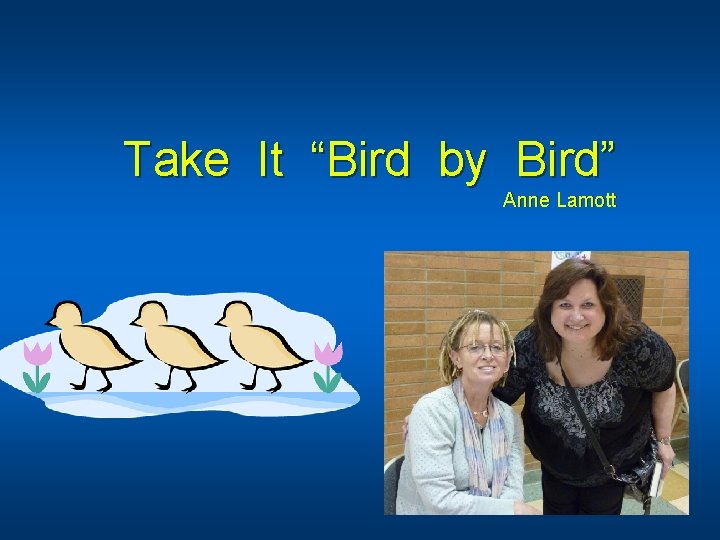
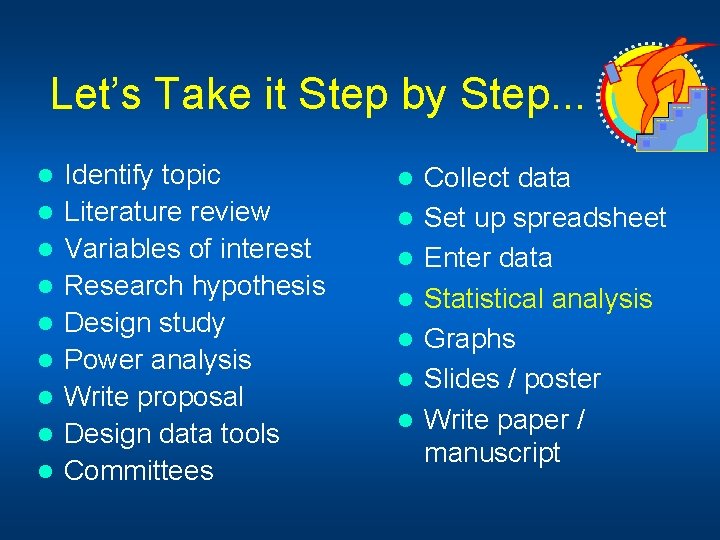
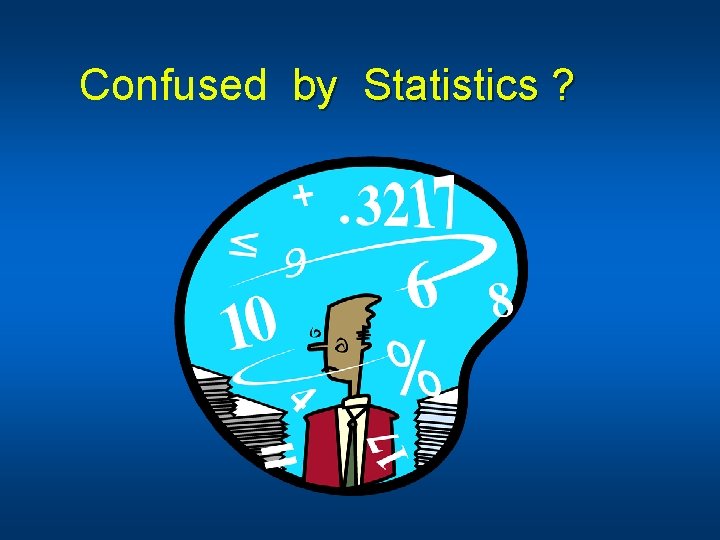
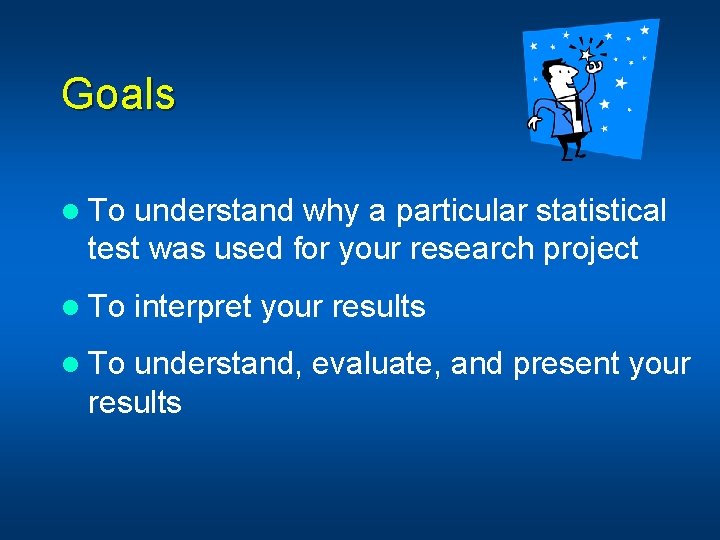
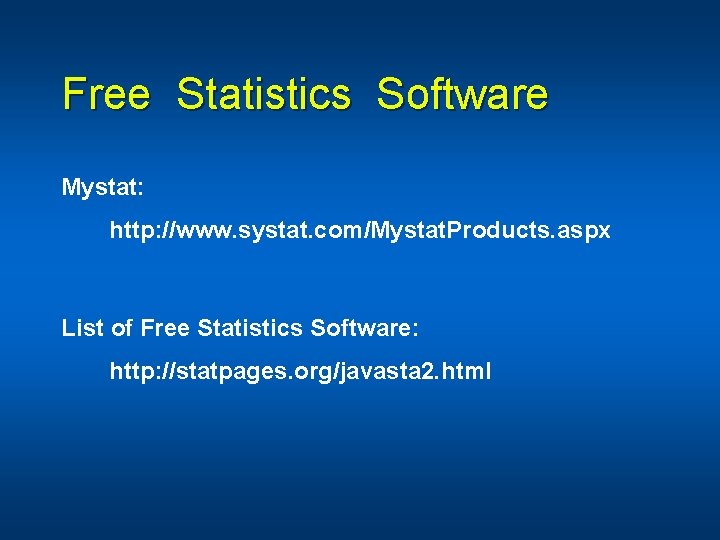
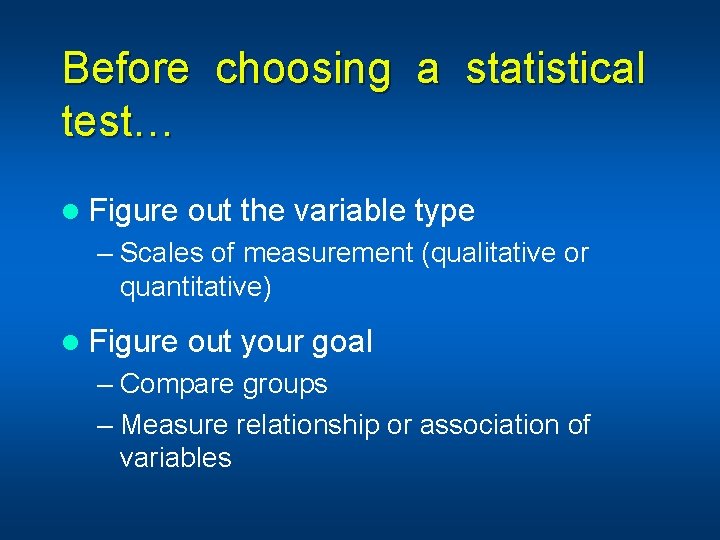
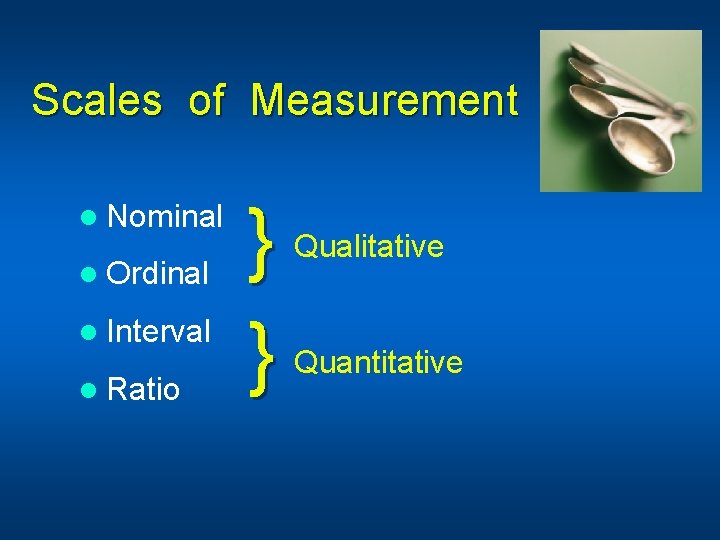
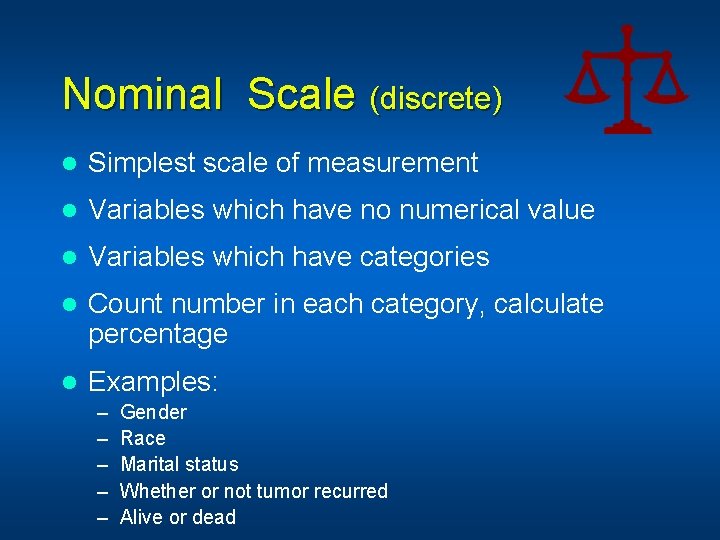
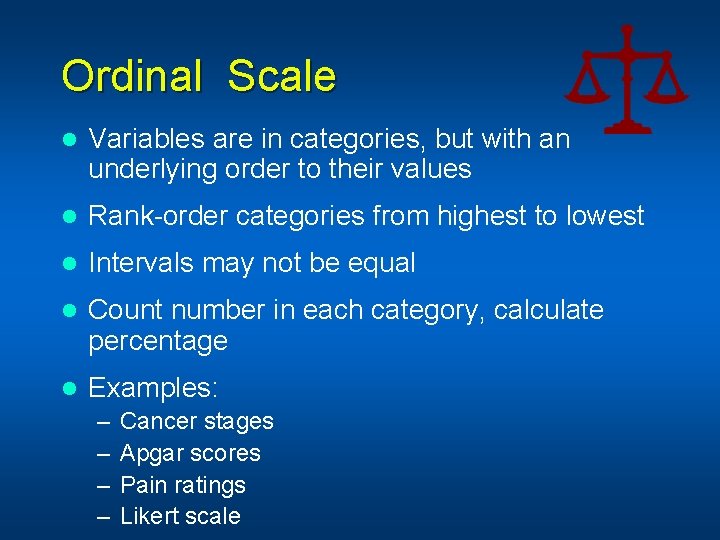
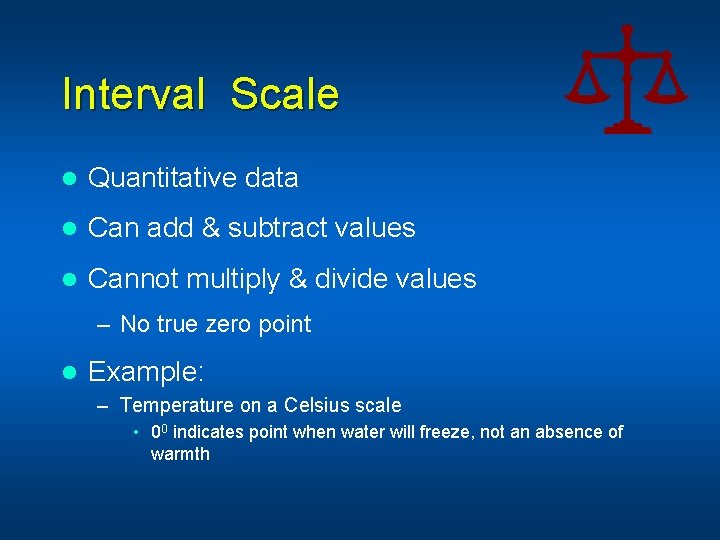
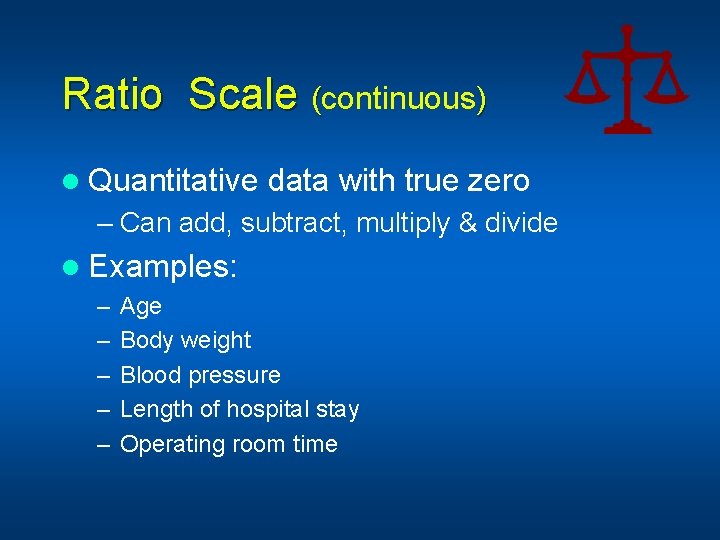
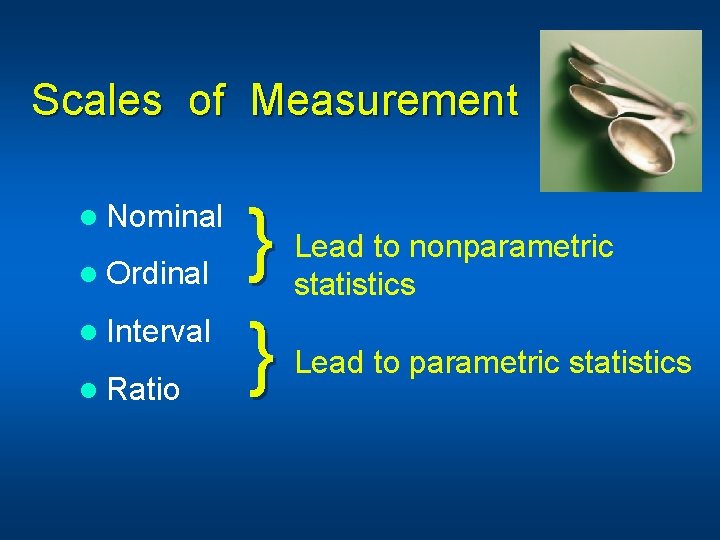
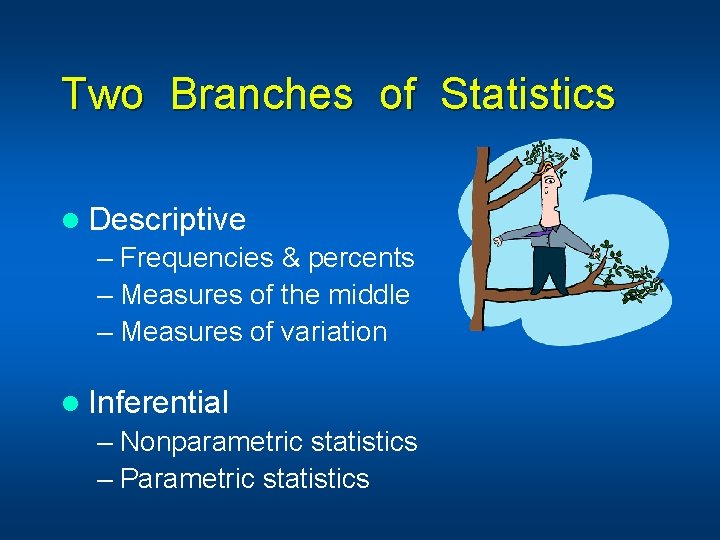
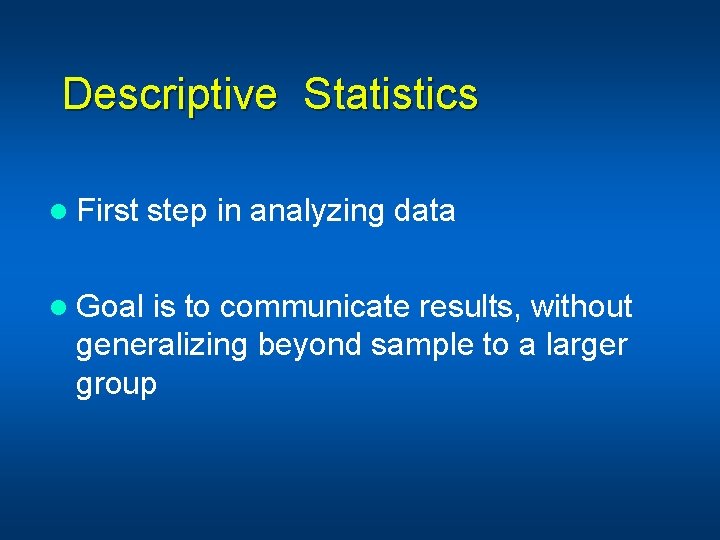
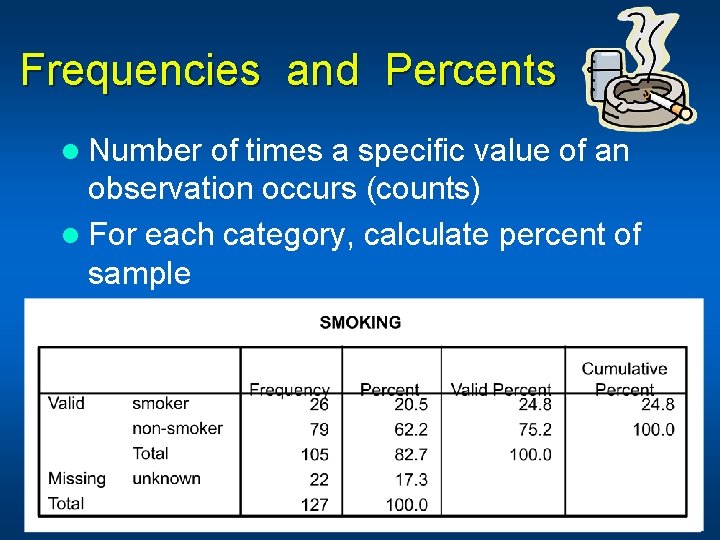
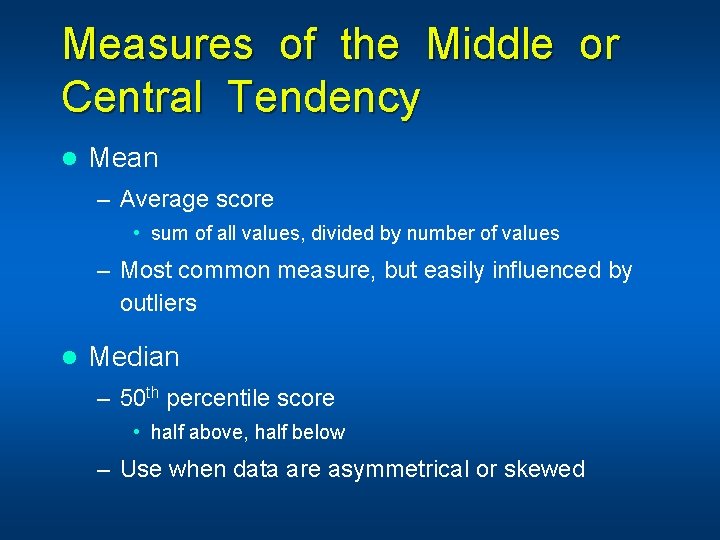
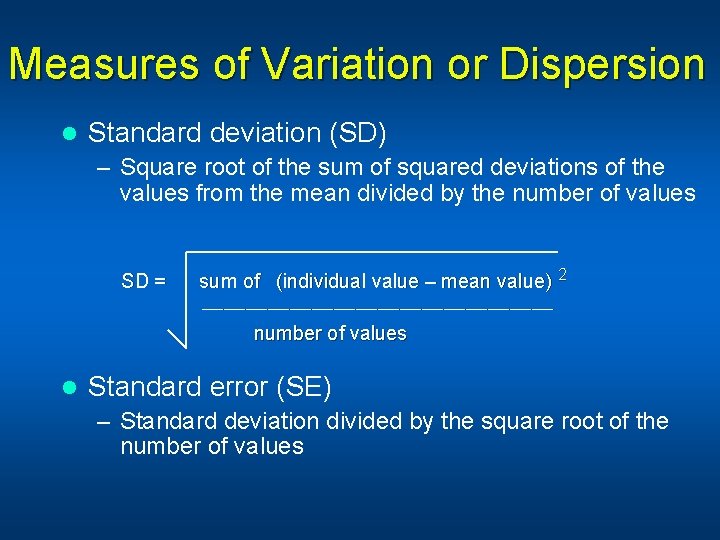
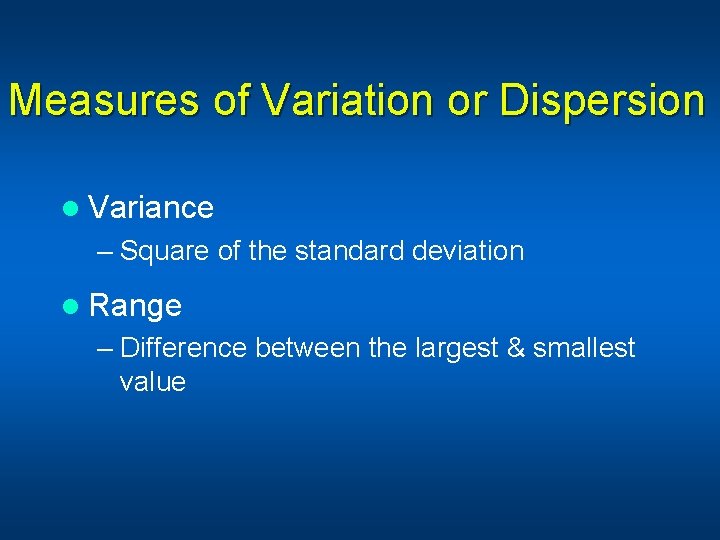
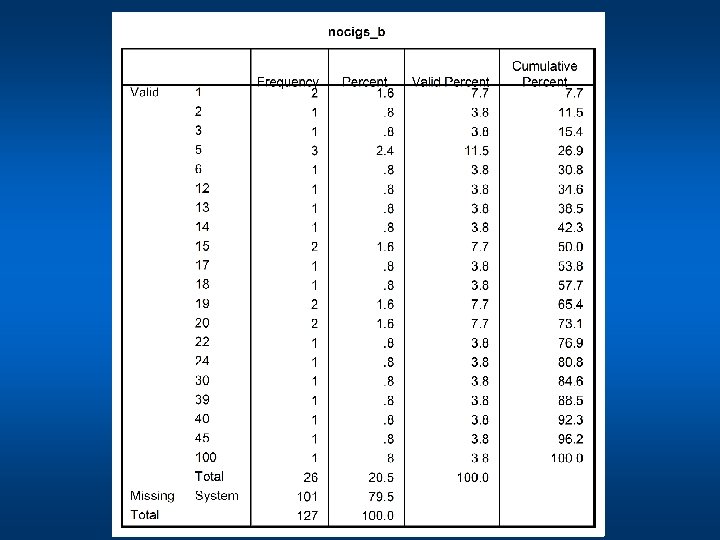
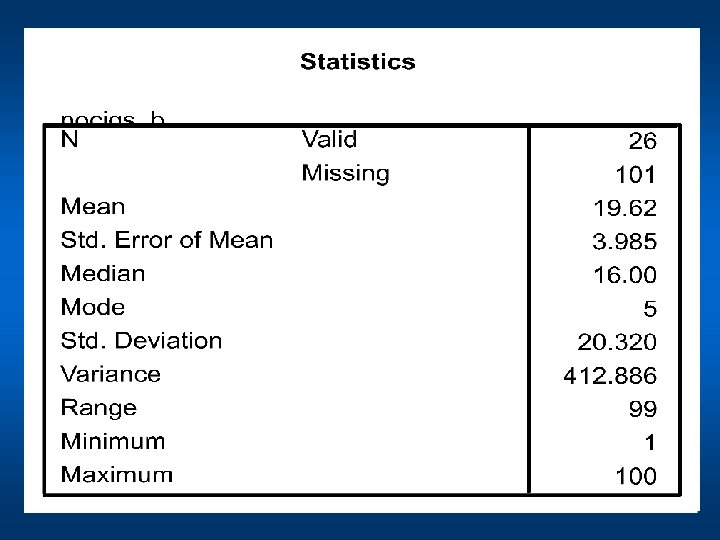
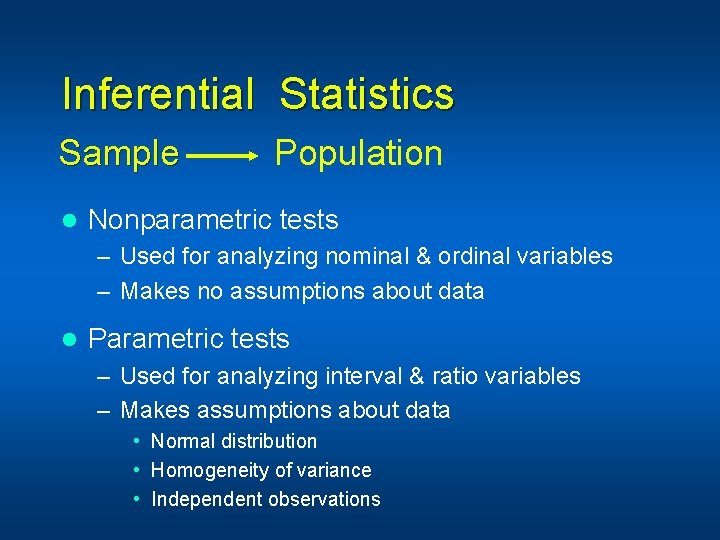
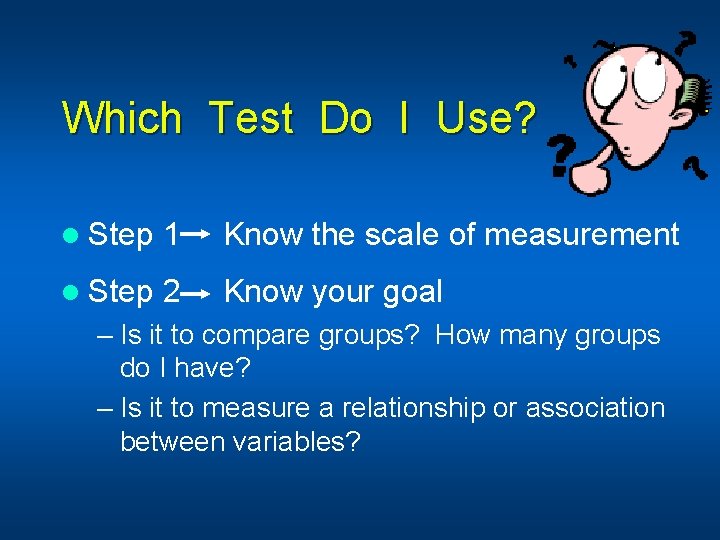
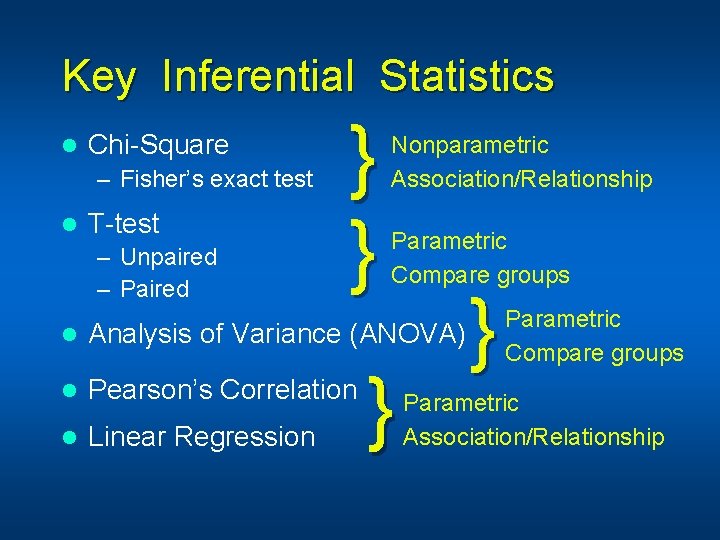
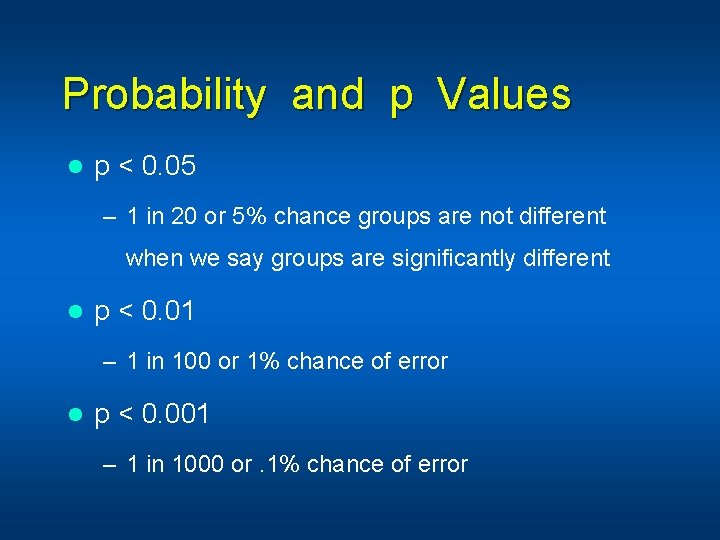
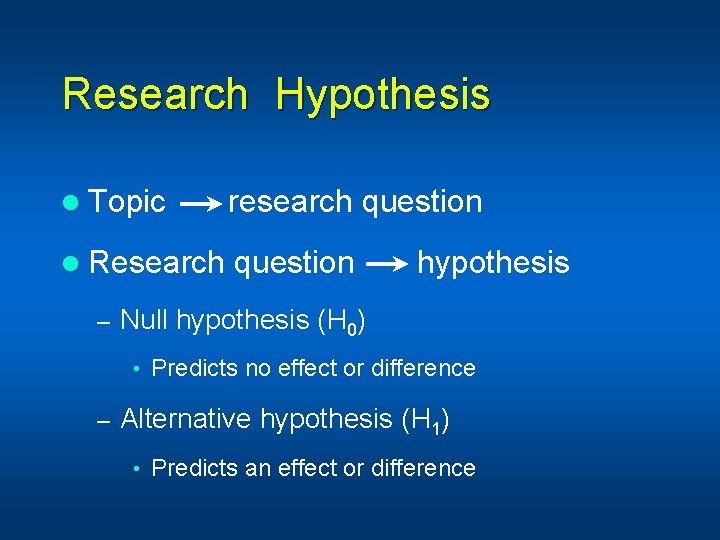
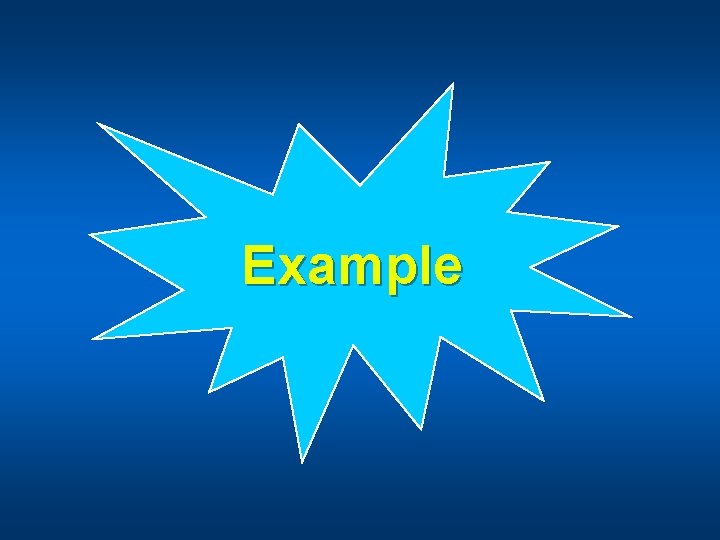
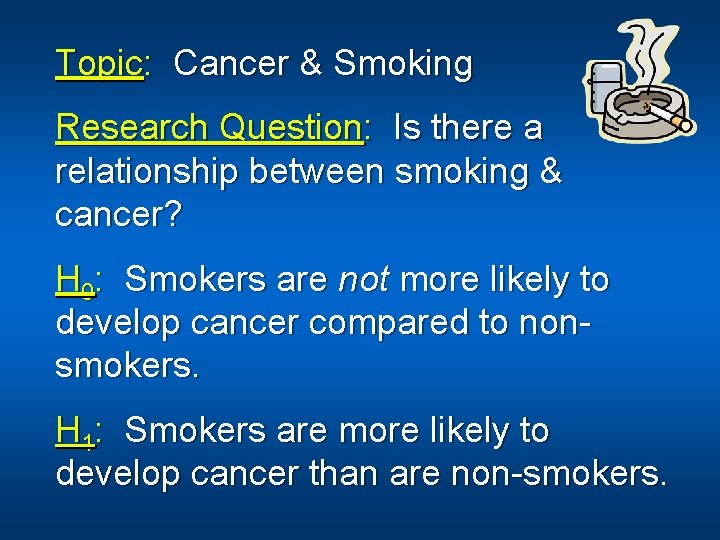
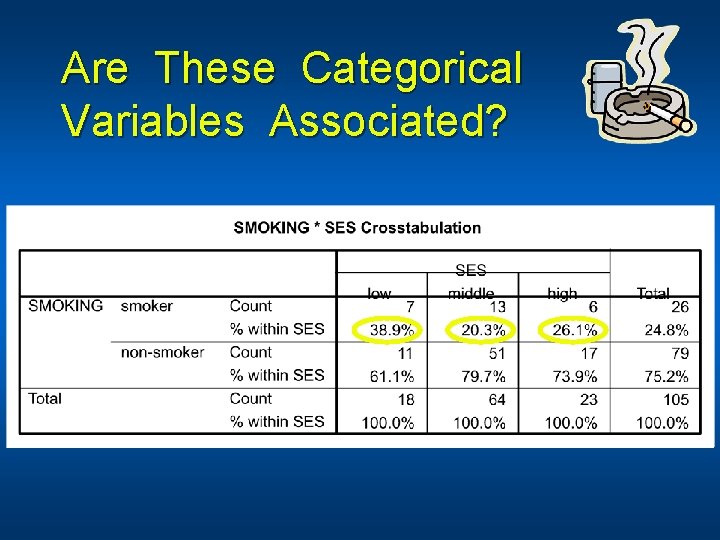
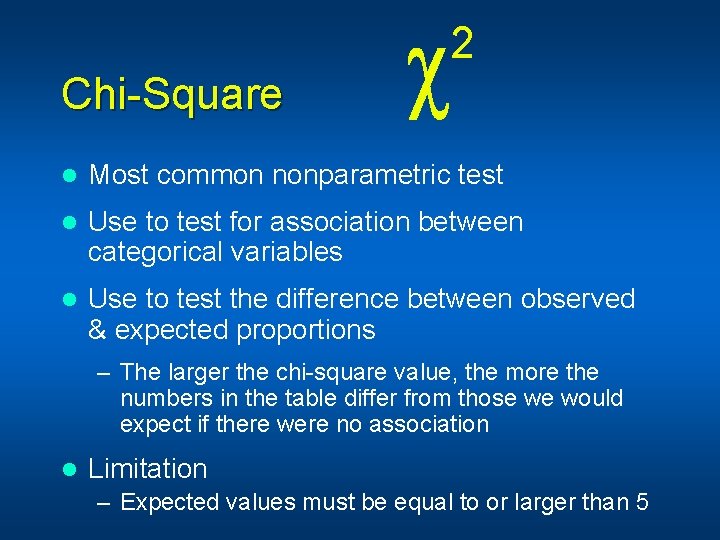
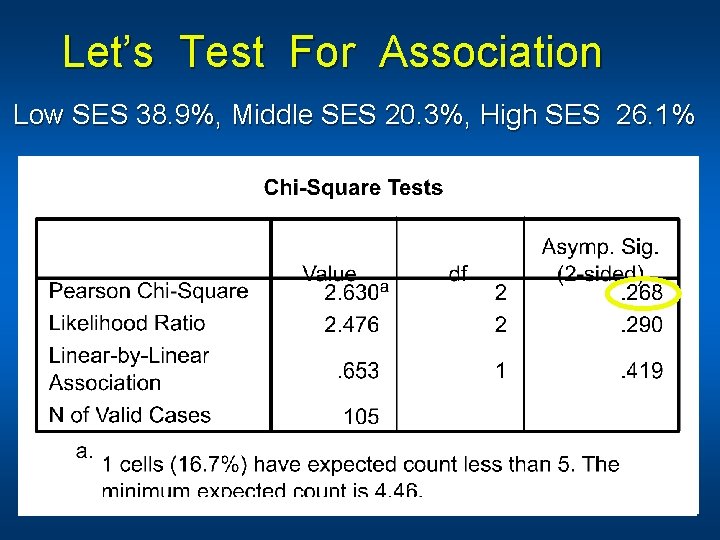
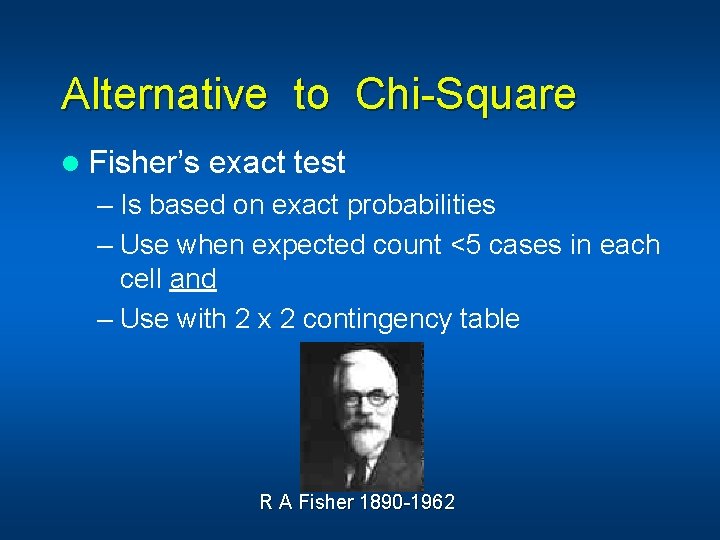
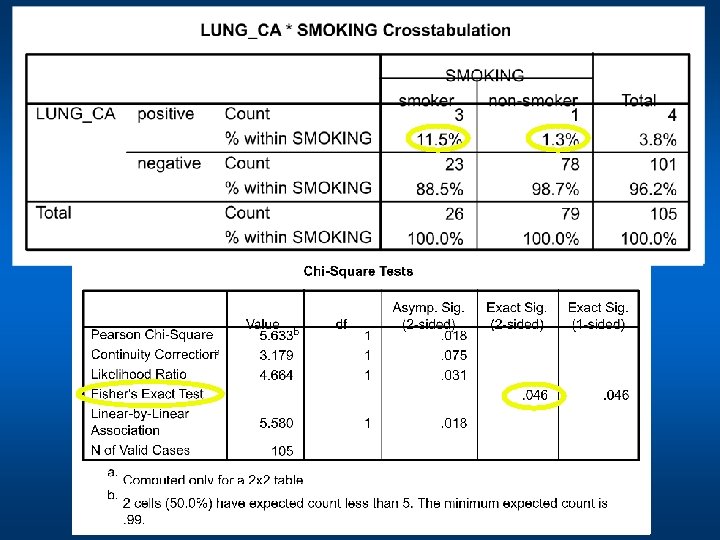
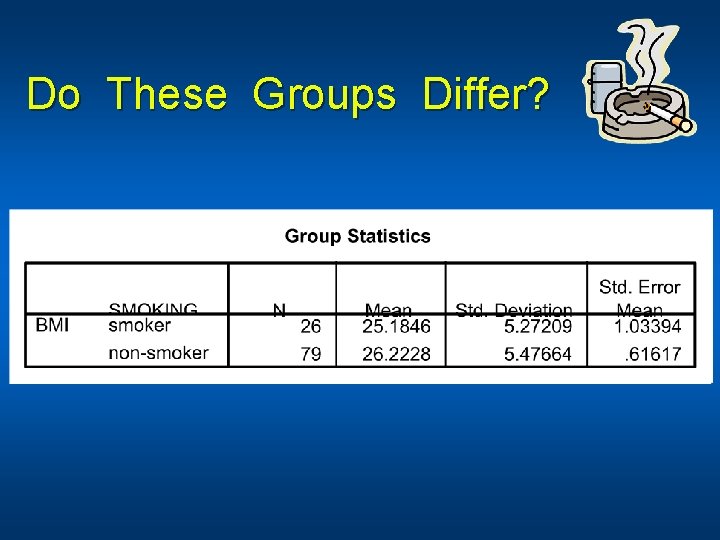
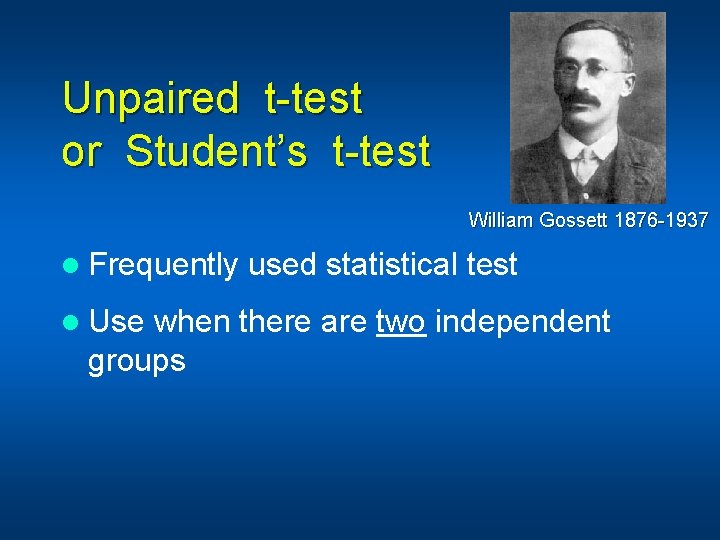
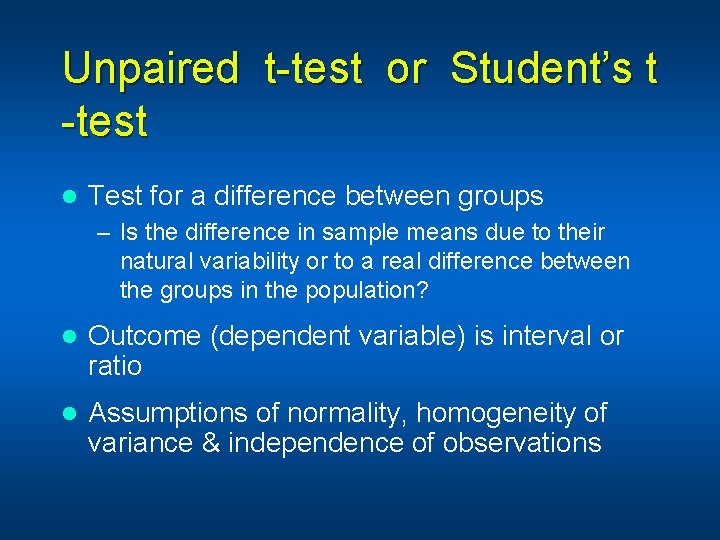
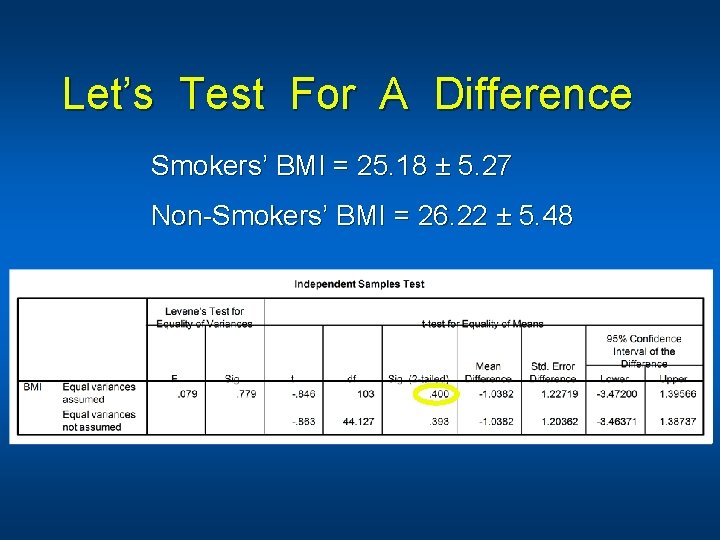
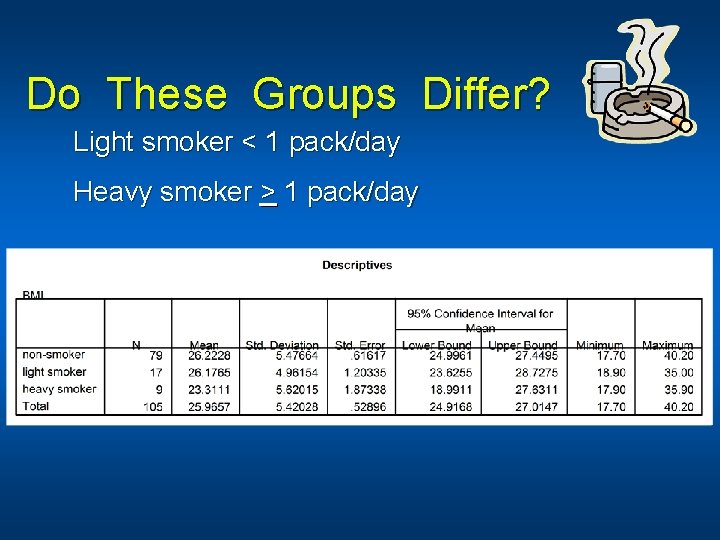
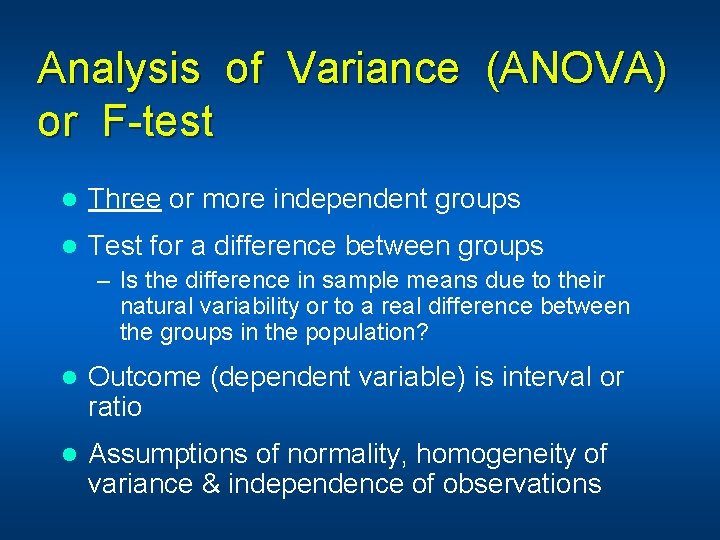
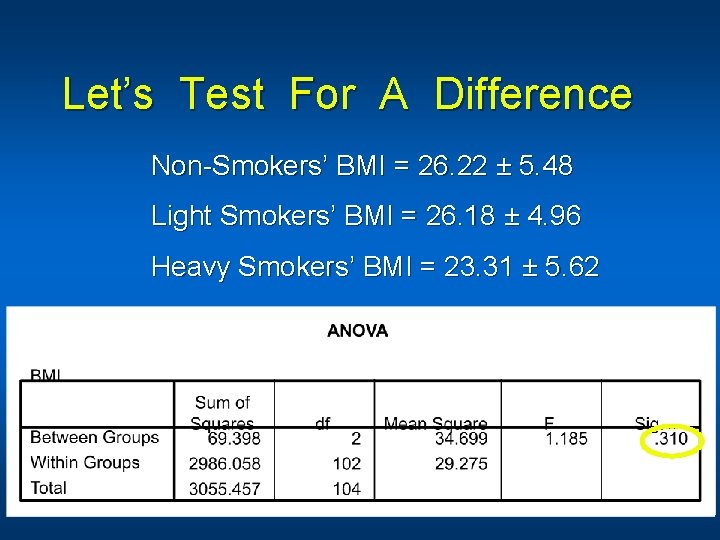
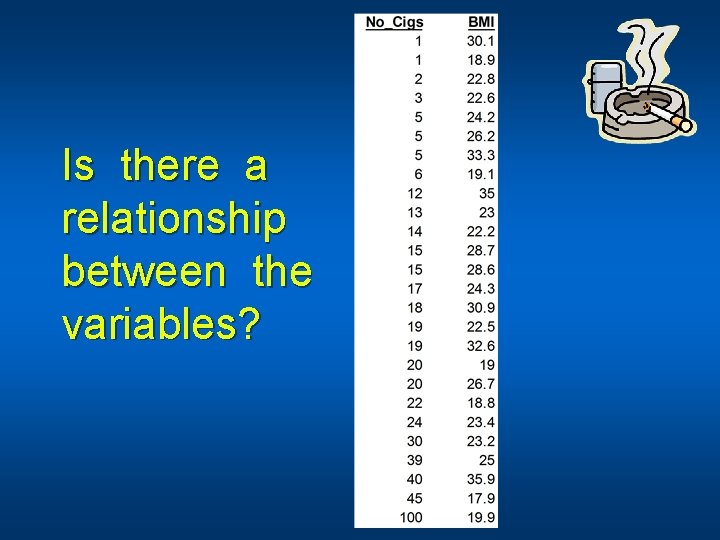
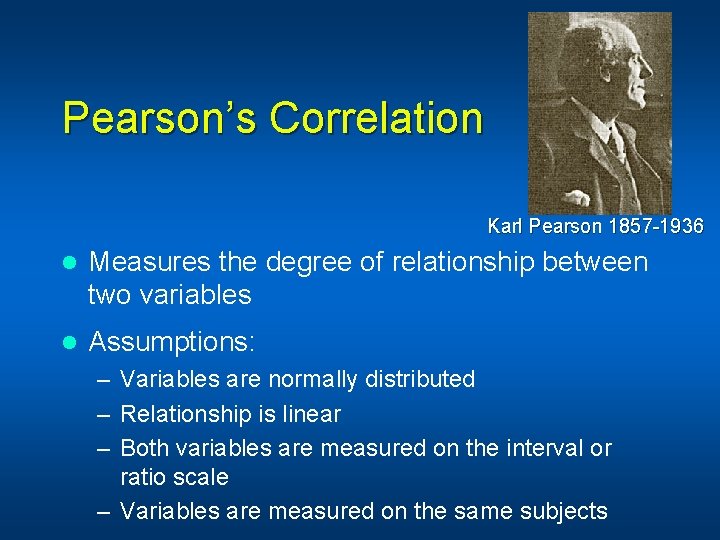
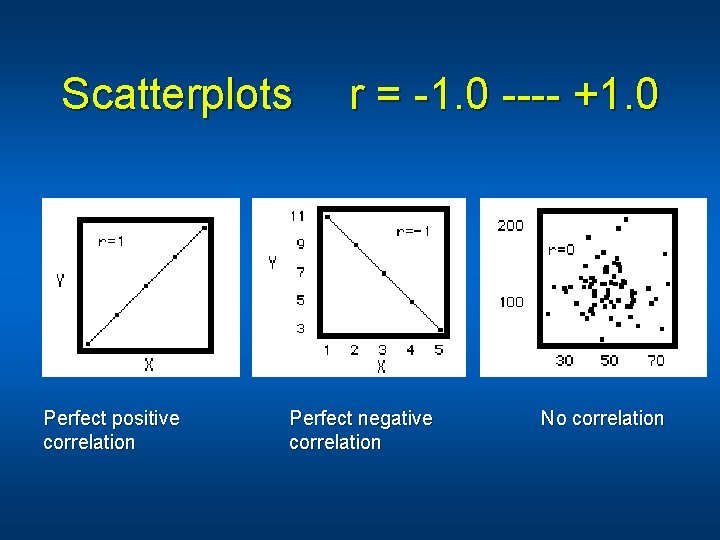
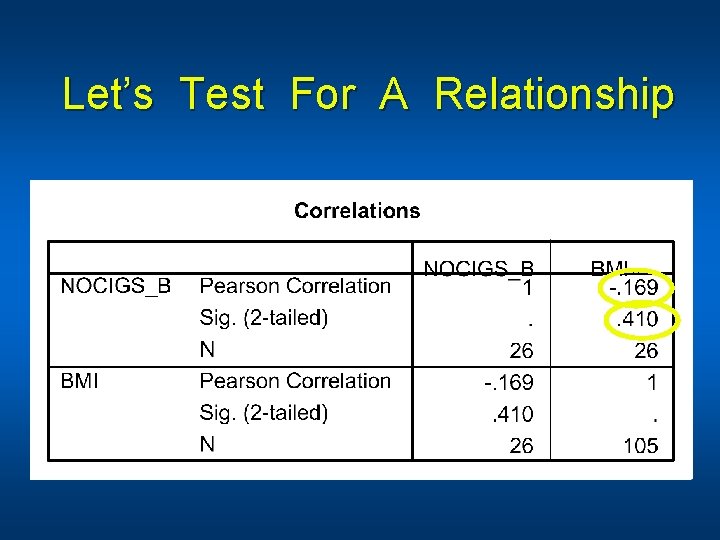
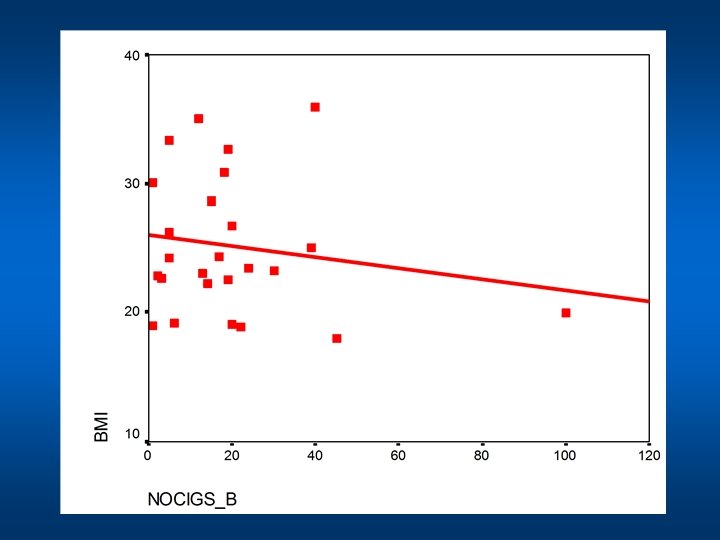
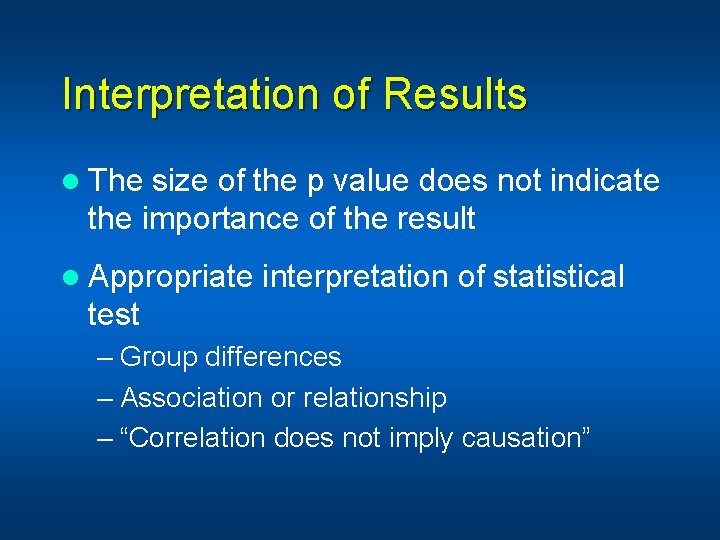
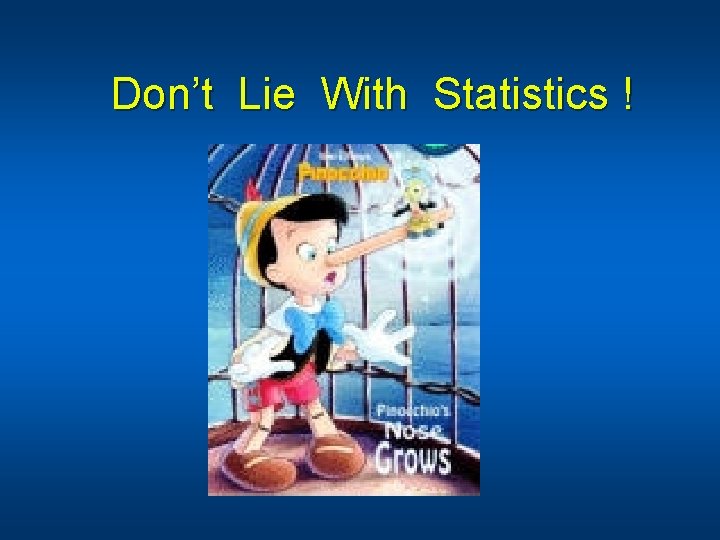
- Slides: 48
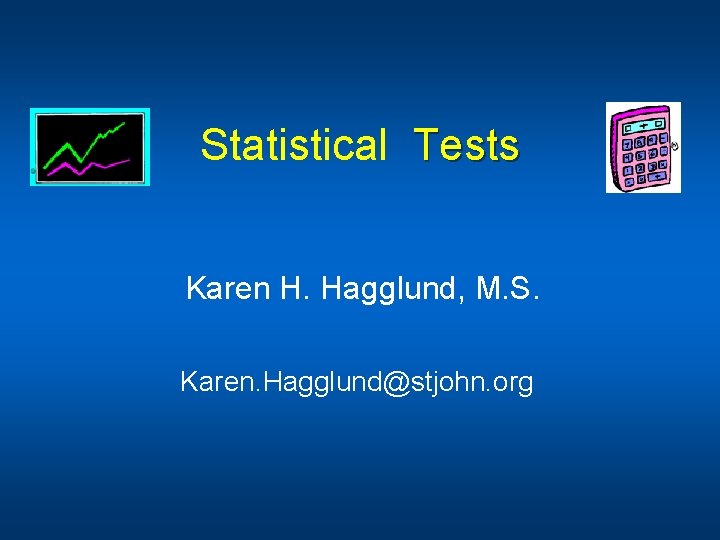
Statistical Tests Karen H. Hagglund, M. S. Karen. Hagglund@stjohn. org
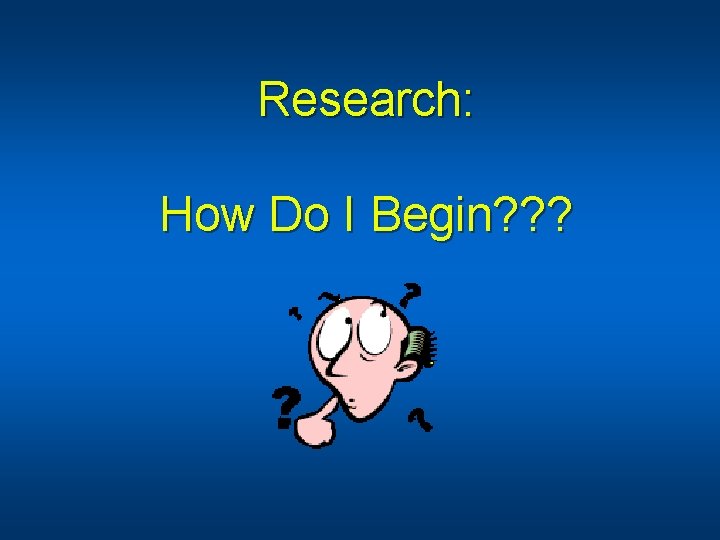
Research: How Do I Begin? ? ?
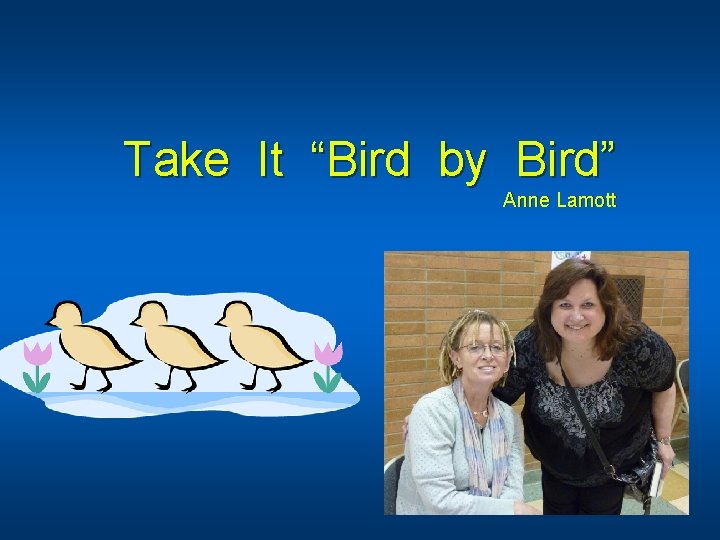
Take It “Bird by Bird” Anne Lamott
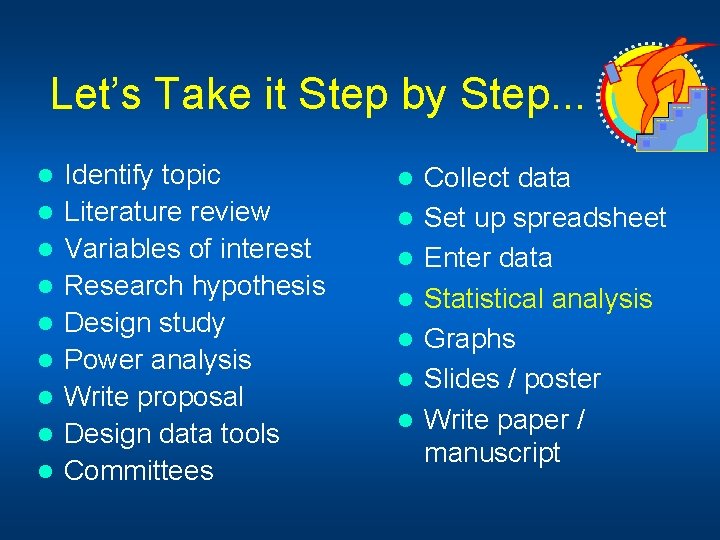
Let’s Take it Step by Step. . . l l l l l Identify topic Literature review Variables of interest Research hypothesis Design study Power analysis Write proposal Design data tools Committees l l l l Collect data Set up spreadsheet Enter data Statistical analysis Graphs Slides / poster Write paper / manuscript
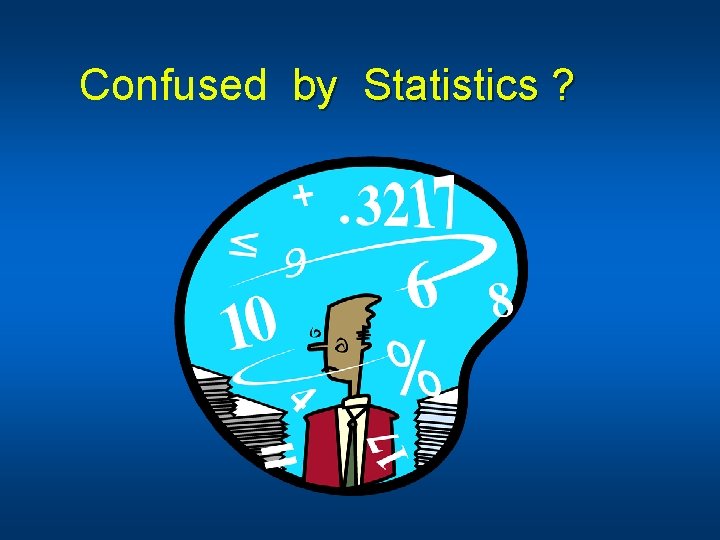
Confused by Statistics ?
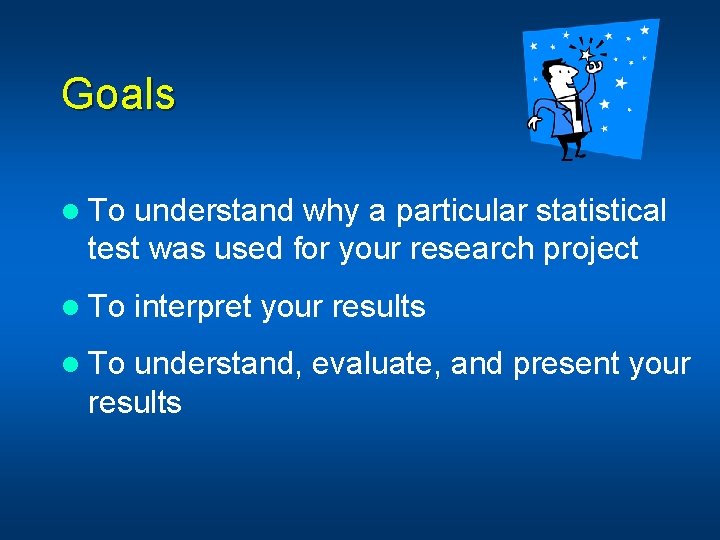
Goals l To understand why a particular statistical test was used for your research project l To interpret your results l To understand, evaluate, and present your results
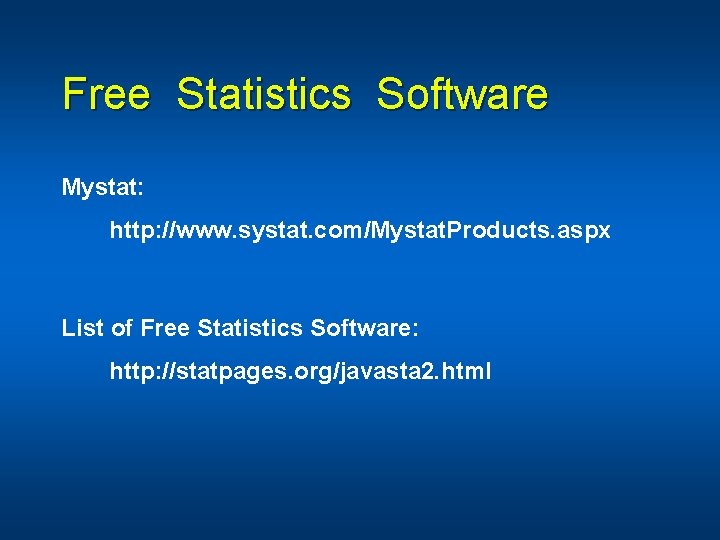
Free Statistics Software Mystat: http: //www. systat. com/Mystat. Products. aspx List of Free Statistics Software: http: //statpages. org/javasta 2. html
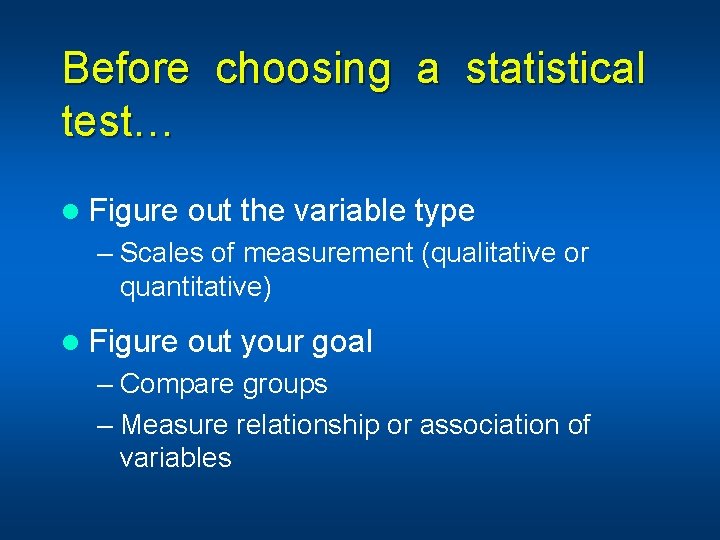
Before choosing a statistical test… l Figure out the variable type – Scales of measurement (qualitative or quantitative) l Figure out your goal – Compare groups – Measure relationship or association of variables
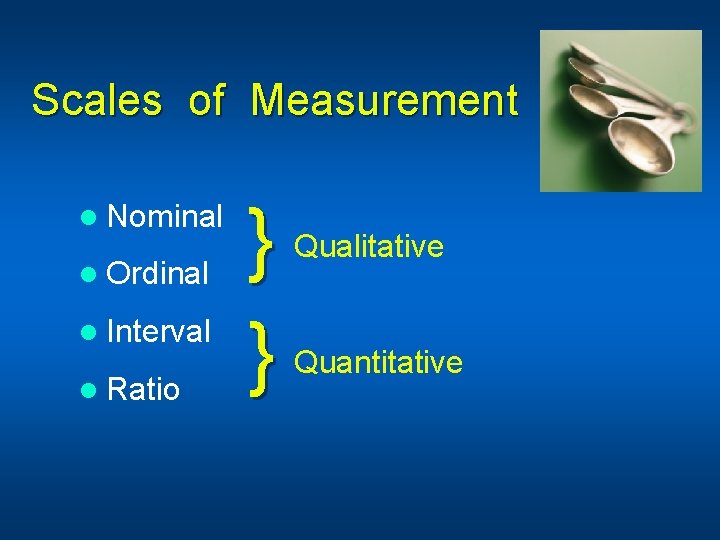
Scales of Measurement l Nominal l Ordinal l Interval l Ratio } } Qualitative Quantitative
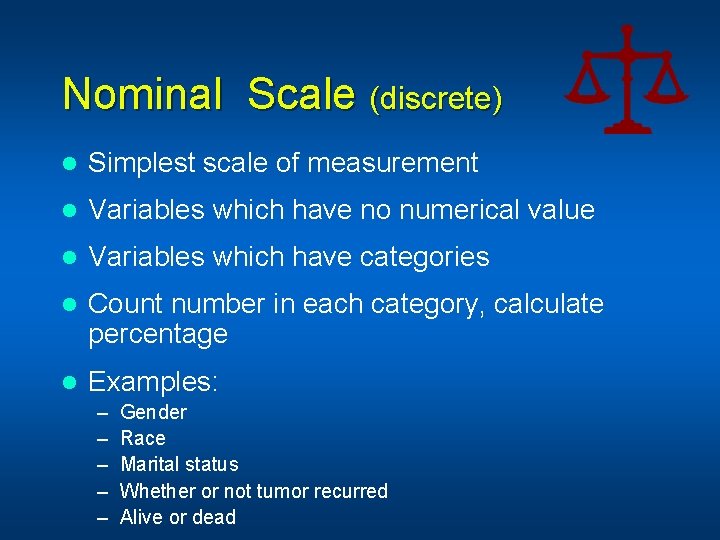
Nominal Scale (discrete) l Simplest scale of measurement l Variables which have no numerical value l Variables which have categories l Count number in each category, calculate percentage l Examples: – – – Gender Race Marital status Whether or not tumor recurred Alive or dead
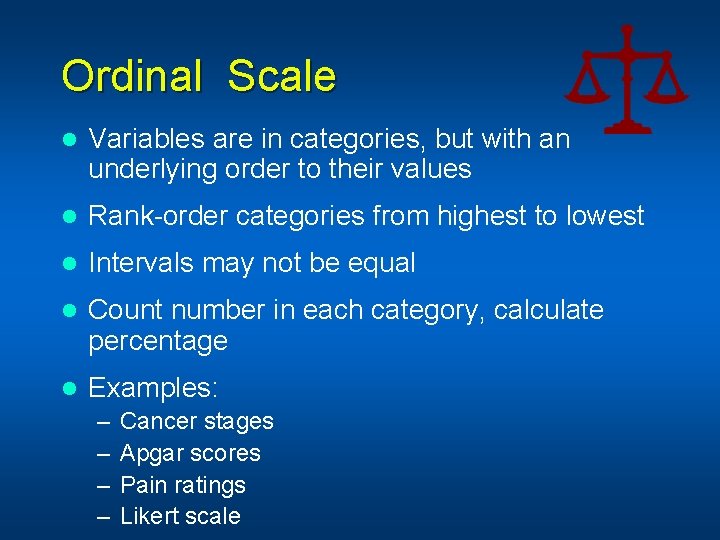
Ordinal Scale l Variables are in categories, but with an underlying order to their values l Rank-order categories from highest to lowest l Intervals may not be equal l Count number in each category, calculate percentage l Examples: – – Cancer stages Apgar scores Pain ratings Likert scale
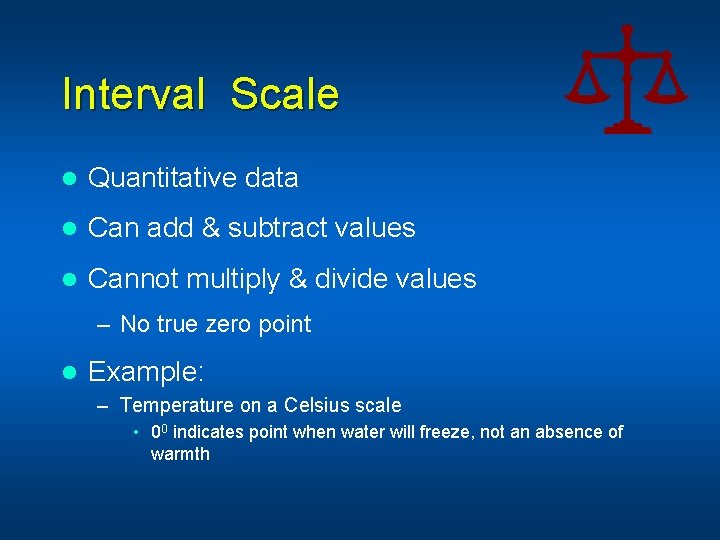
Interval Scale l Quantitative data l Can add & subtract values l Cannot multiply & divide values – No true zero point l Example: – Temperature on a Celsius scale • 00 indicates point when water will freeze, not an absence of warmth
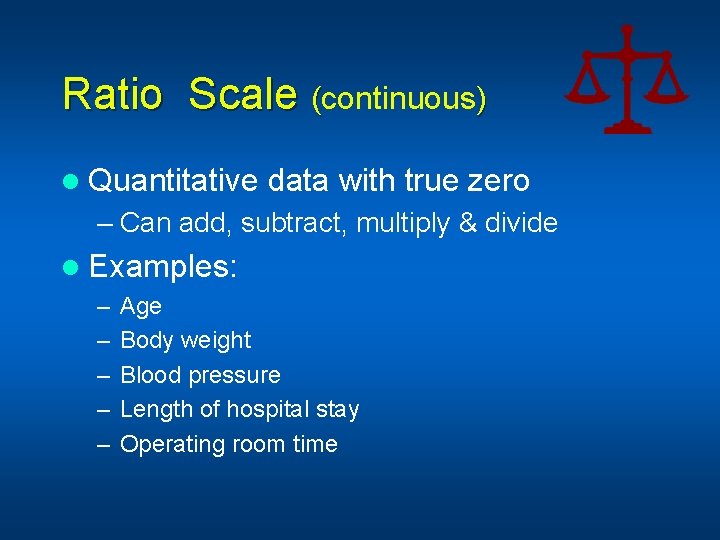
Ratio Scale (continuous) l Quantitative data with true zero – Can add, subtract, multiply & divide l Examples: – – – Age Body weight Blood pressure Length of hospital stay Operating room time
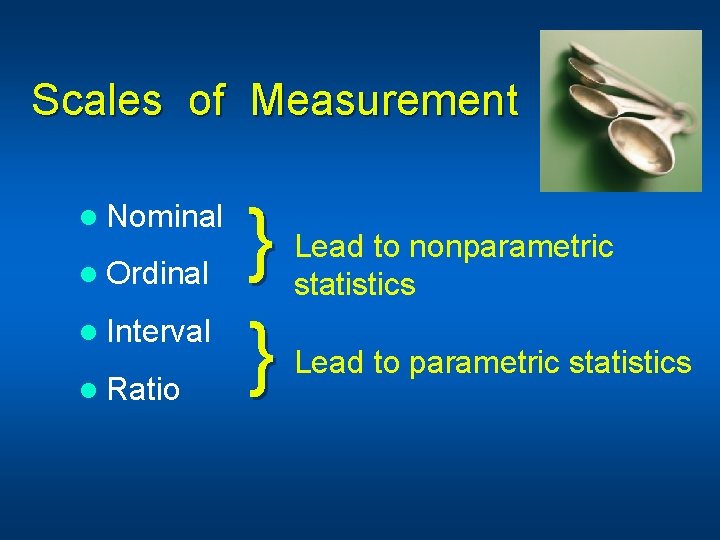
Scales of Measurement l Nominal l Ordinal l Interval l Ratio } } Lead to nonparametric statistics Lead to parametric statistics
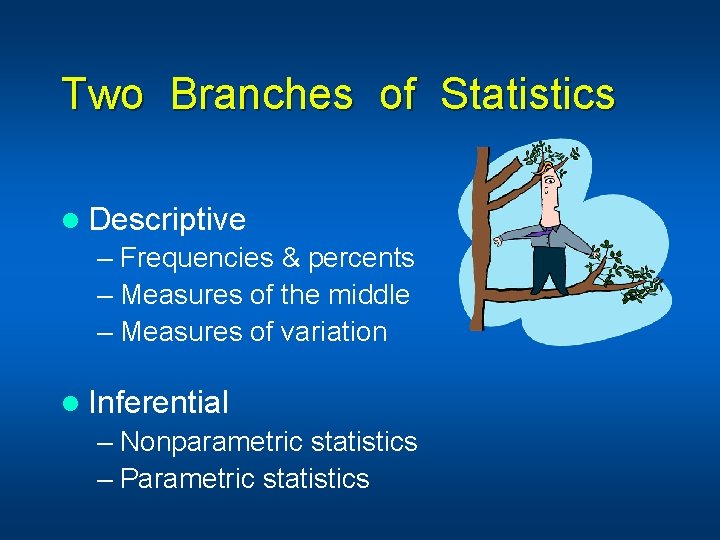
Two Branches of Statistics l Descriptive – Frequencies & percents – Measures of the middle – Measures of variation l Inferential – Nonparametric statistics – Parametric statistics
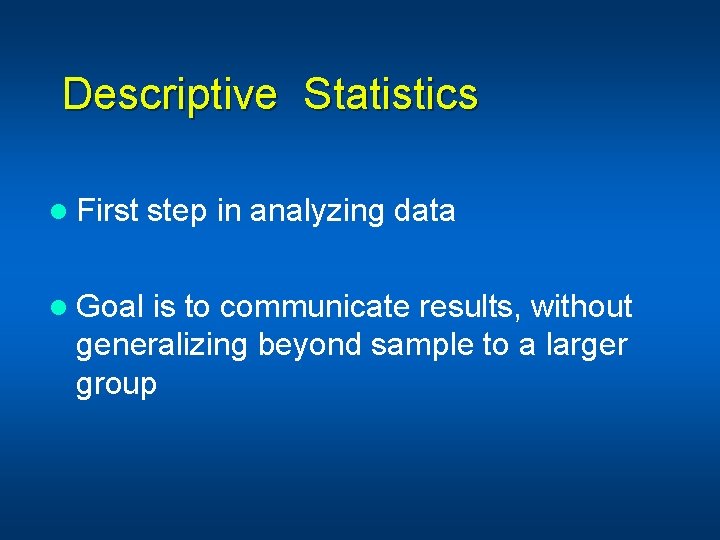
Descriptive Statistics l First step in analyzing data l Goal is to communicate results, without generalizing beyond sample to a larger group
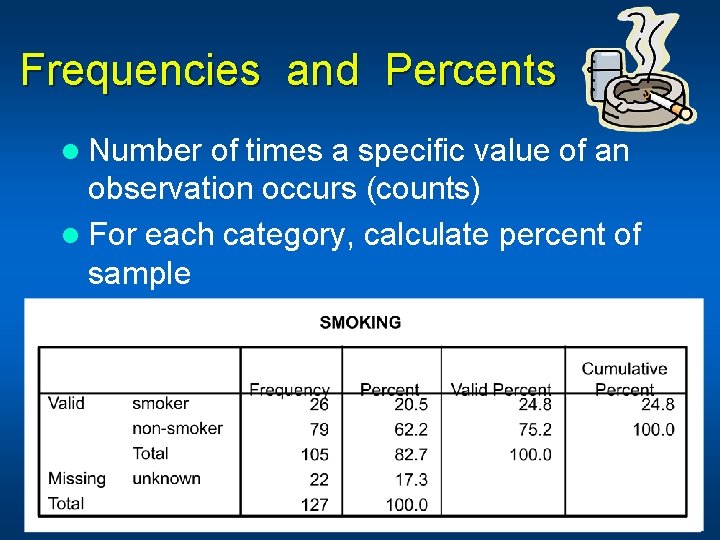
Frequencies and Percents Number of times a specific value of an observation occurs (counts) l For each category, calculate percent of sample l
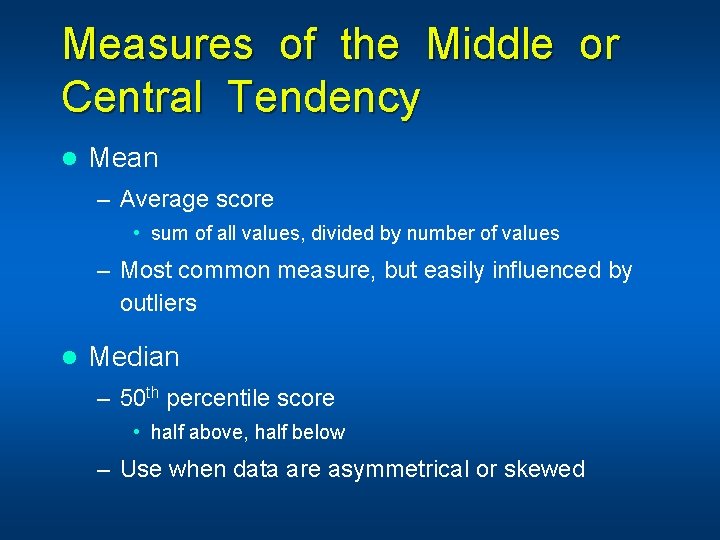
Measures of the Middle or Central Tendency l Mean – Average score • sum of all values, divided by number of values – Most common measure, but easily influenced by outliers l Median – 50 th percentile score • half above, half below – Use when data are asymmetrical or skewed
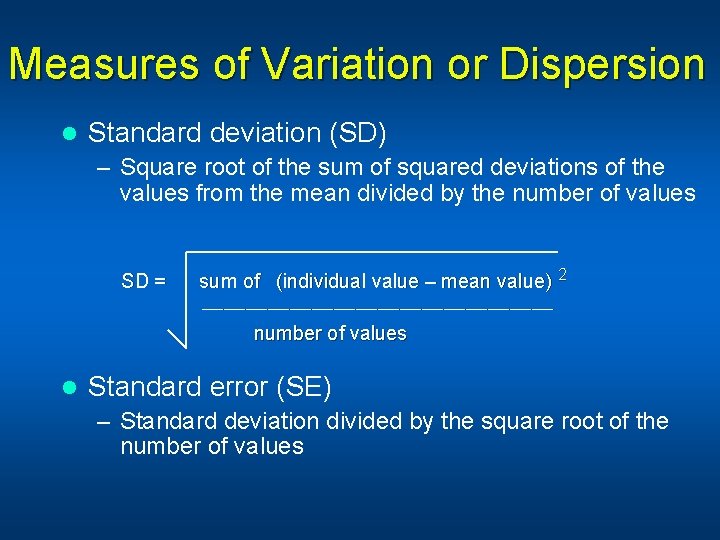
Measures of Variation or Dispersion l Standard deviation (SD) – Square root of the sum of squared deviations of the values from the mean divided by the number of values SD = sum of (individual value – mean value) 2 ________________________ number of values l Standard error (SE) – Standard deviation divided by the square root of the number of values
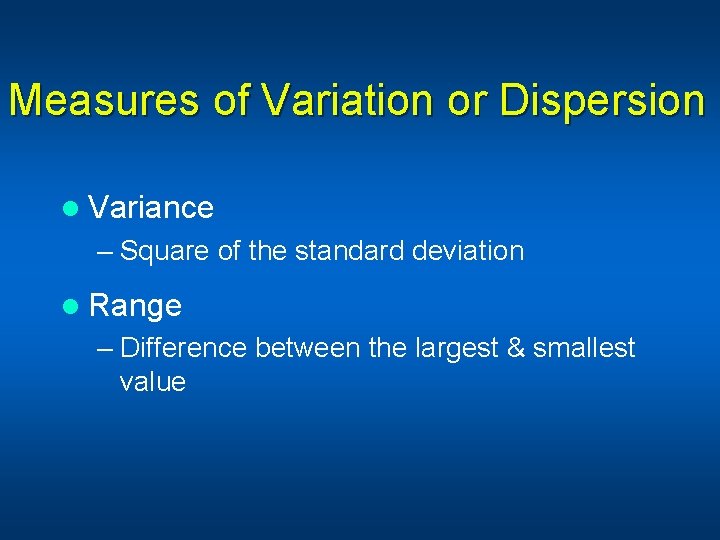
Measures of Variation or Dispersion l Variance – Square of the standard deviation l Range – Difference between the largest & smallest value
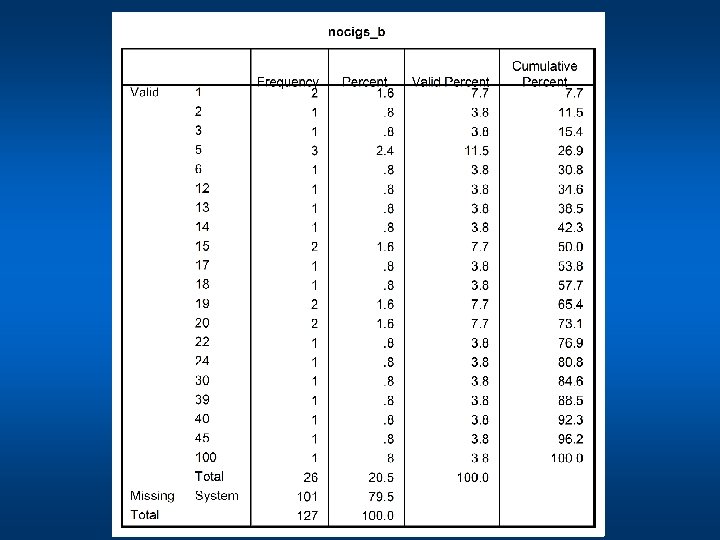
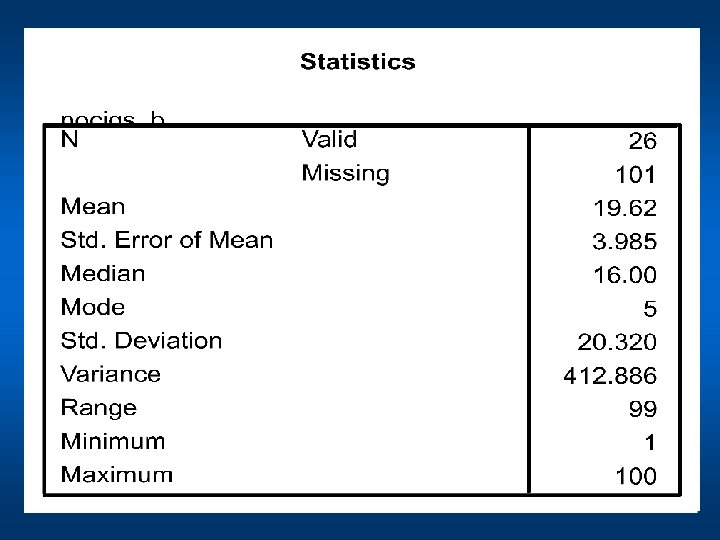
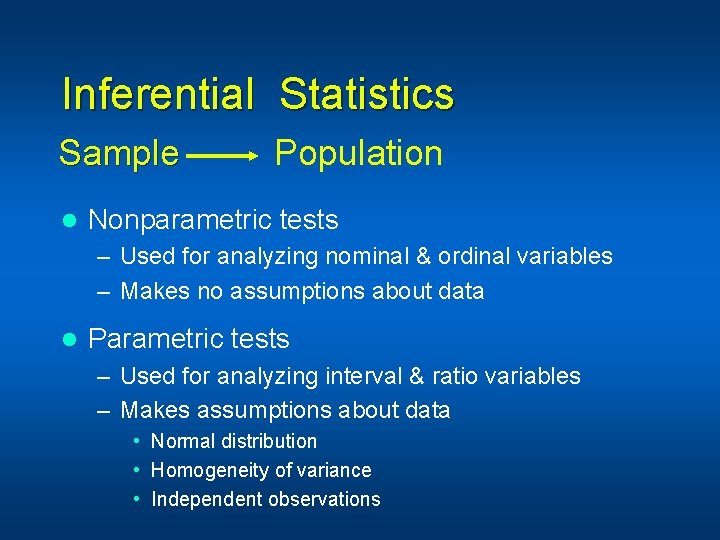
Inferential Statistics Sample l Population Nonparametric tests – Used for analyzing nominal & ordinal variables – Makes no assumptions about data l Parametric tests – Used for analyzing interval & ratio variables – Makes assumptions about data • Normal distribution • Homogeneity of variance • Independent observations
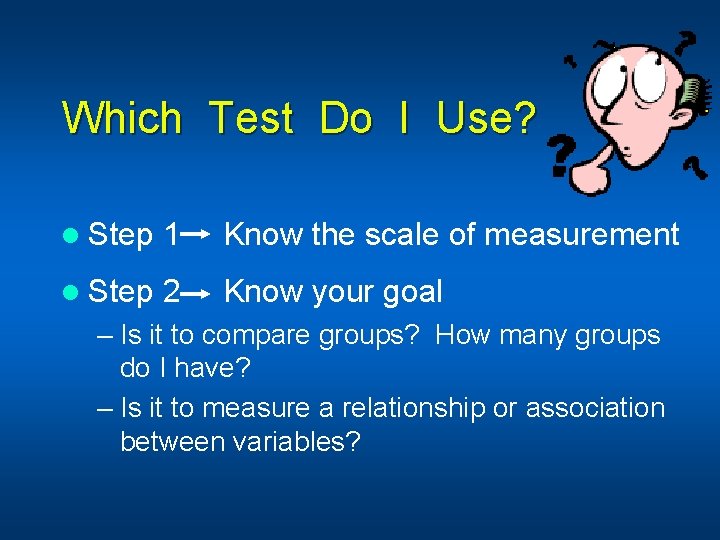
Which Test Do I Use? l Step 1 Know the scale of measurement l Step 2 Know your goal – Is it to compare groups? How many groups do I have? – Is it to measure a relationship or association between variables?
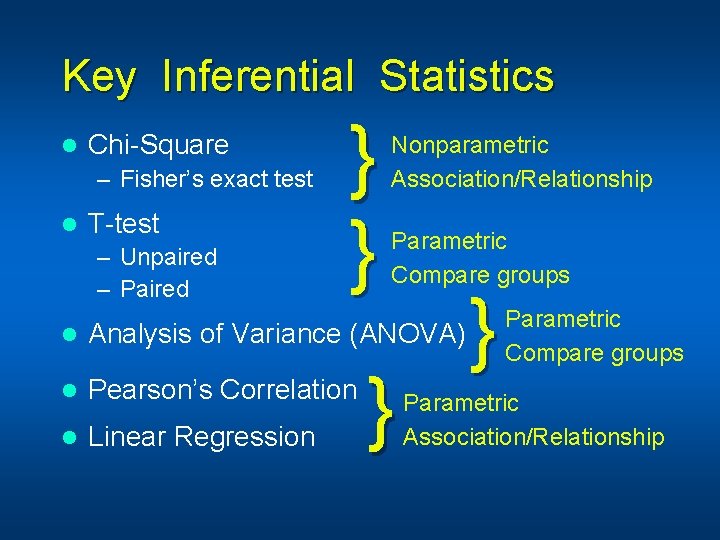
Key Inferential Statistics l Chi-Square – Fisher’s exact test l T-test – Unpaired – Paired } } Nonparametric Association/Relationship Parametric Compare groups l Analysis of Variance (ANOVA) l Pearson’s Correlation l Linear Regression } } Parametric Compare groups Parametric Association/Relationship
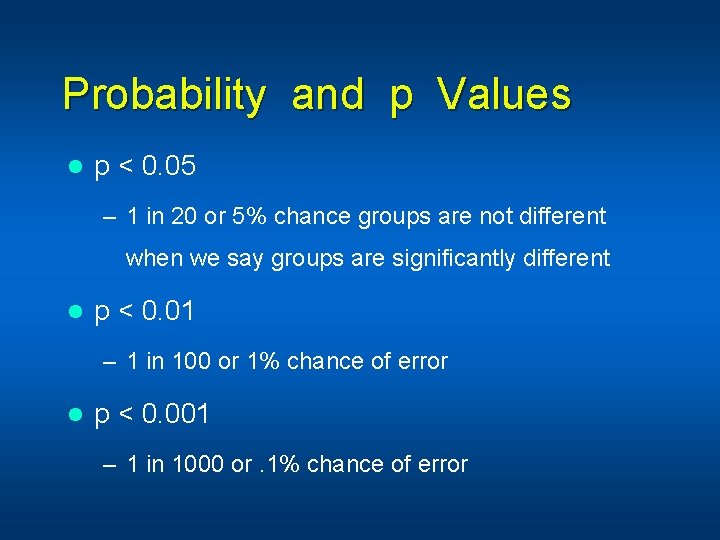
Probability and p Values l p < 0. 05 – 1 in 20 or 5% chance groups are not different when we say groups are significantly different l p < 0. 01 – 1 in 100 or 1% chance of error l p < 0. 001 – 1 in 1000 or. 1% chance of error
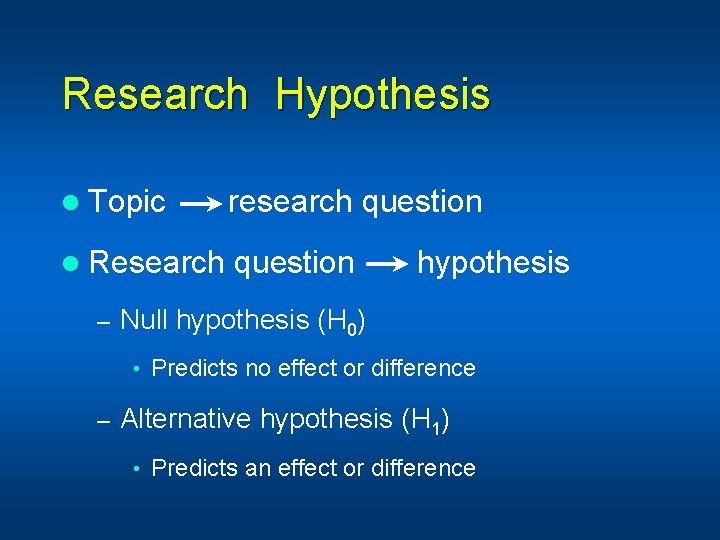
Research Hypothesis l Topic research question l Research question – Null hypothesis (H 0) • – hypothesis Predicts no effect or difference Alternative hypothesis (H 1) • Predicts an effect or difference
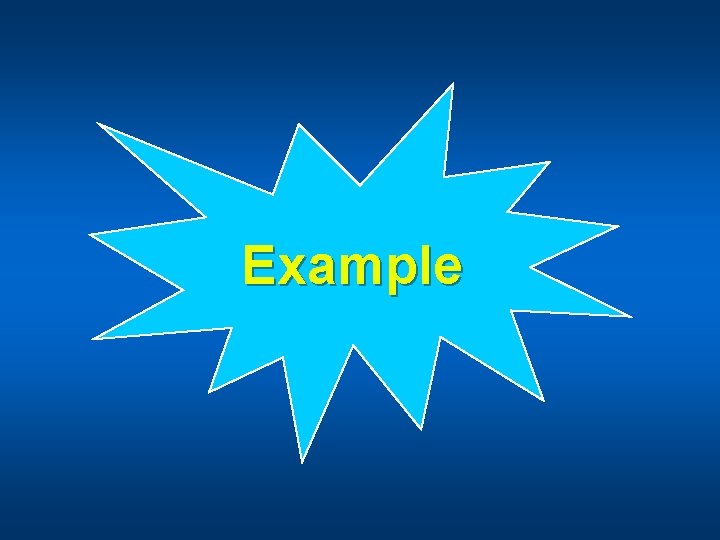
Example
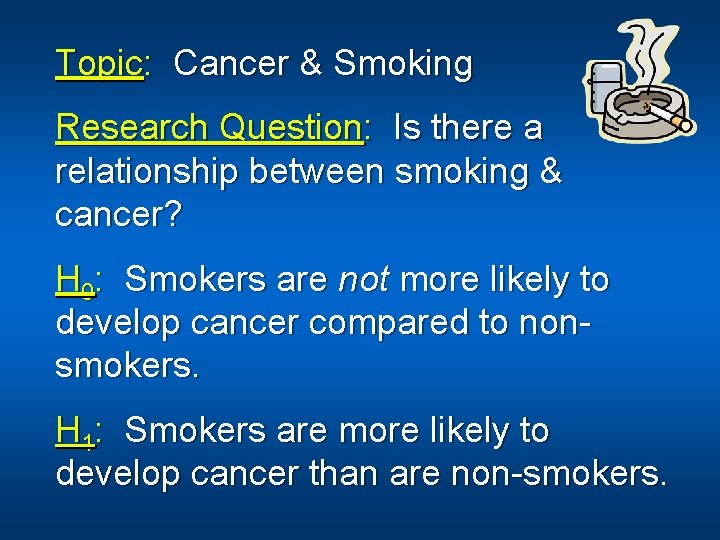
Topic: Cancer & Smoking Research Question: Is there a relationship between smoking & cancer? H 0: Smokers are not more likely to develop cancer compared to nonsmokers. H 1: Smokers are more likely to develop cancer than are non-smokers.
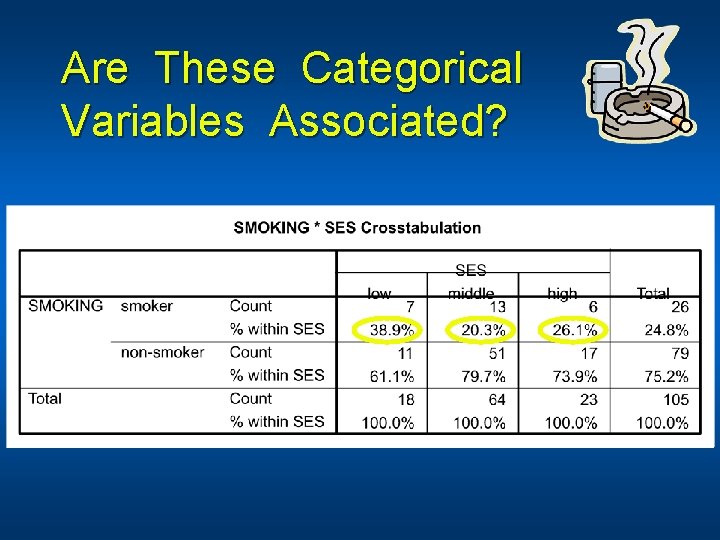
Are These Categorical Variables Associated?
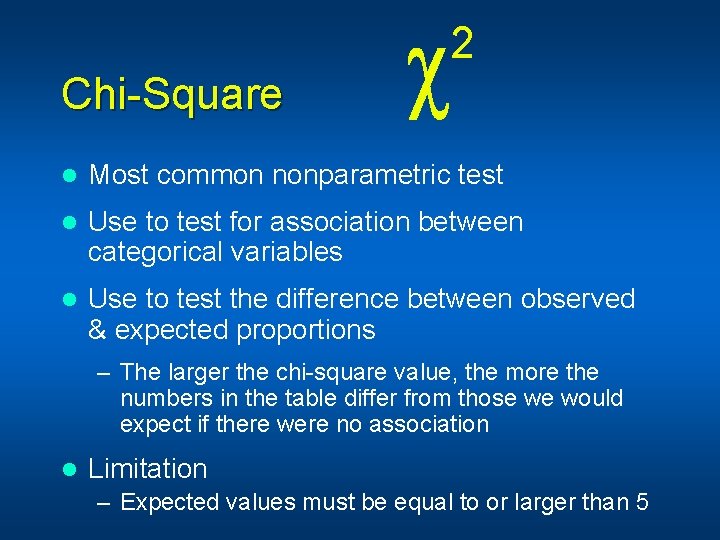
2 Chi-Square l Most common nonparametric test l Use to test for association between categorical variables l Use to test the difference between observed & expected proportions – The larger the chi-square value, the more the numbers in the table differ from those we would expect if there were no association l Limitation – Expected values must be equal to or larger than 5
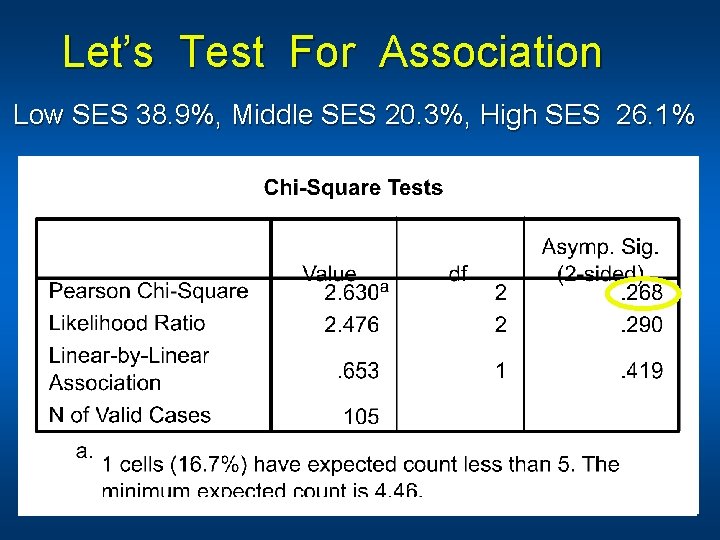
Let’s Test For Association Low SES 38. 9%, Middle SES 20. 3%, High SES 26. 1%
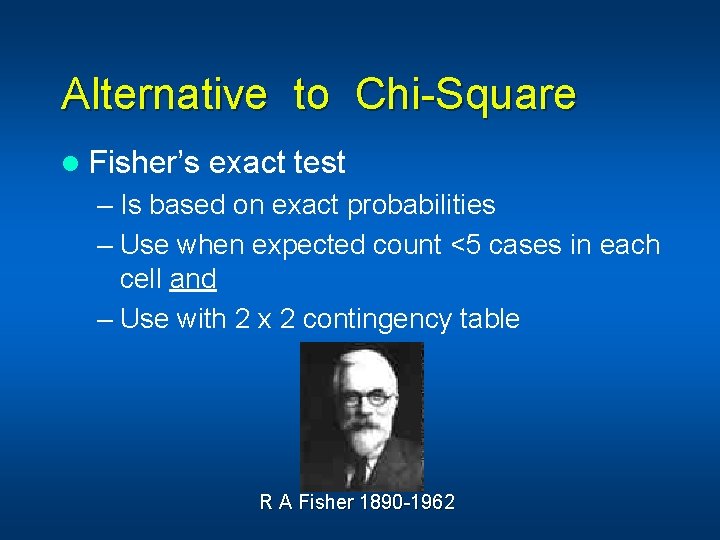
Alternative to Chi-Square l Fisher’s exact test – Is based on exact probabilities – Use when expected count <5 cases in each cell and – Use with 2 x 2 contingency table R A Fisher 1890 -1962
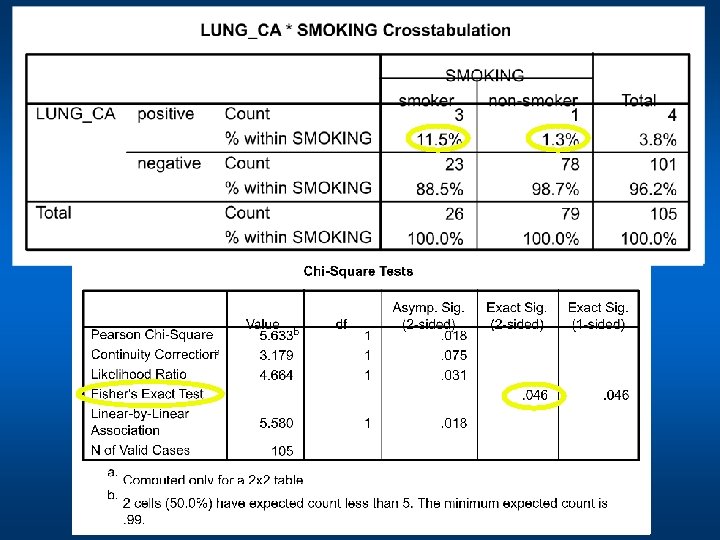
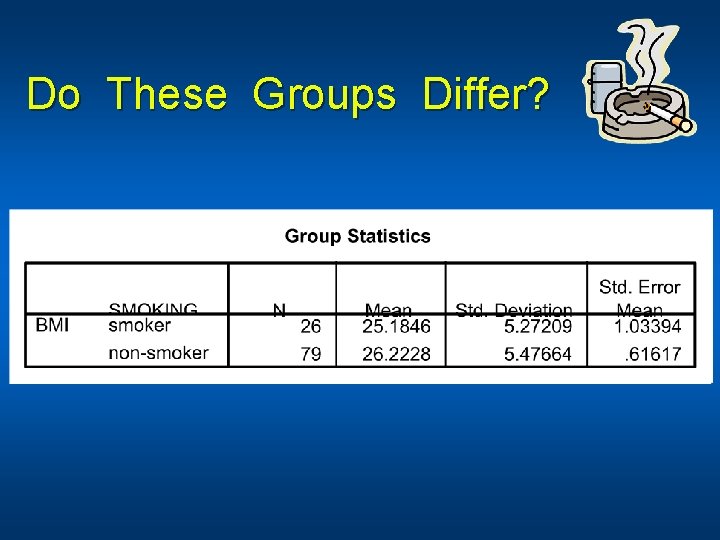
Do These Groups Differ?
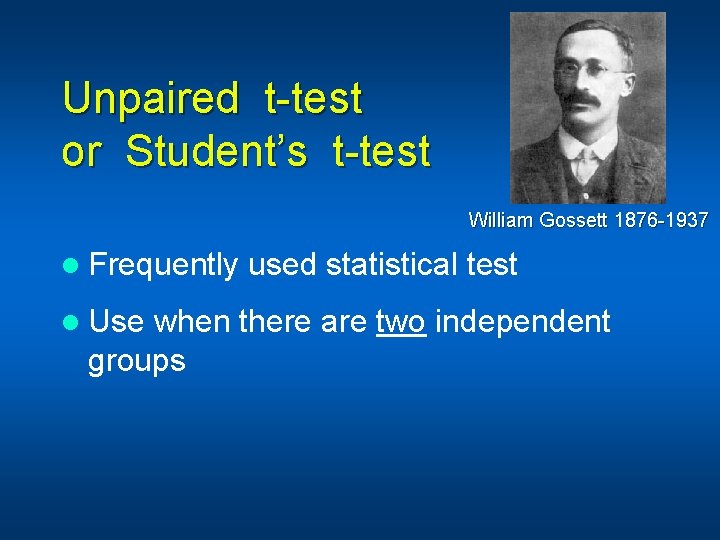
Unpaired t-test or Student’s t-test William Gossett 1876 -1937 l Frequently used statistical test l Use when there are two independent groups
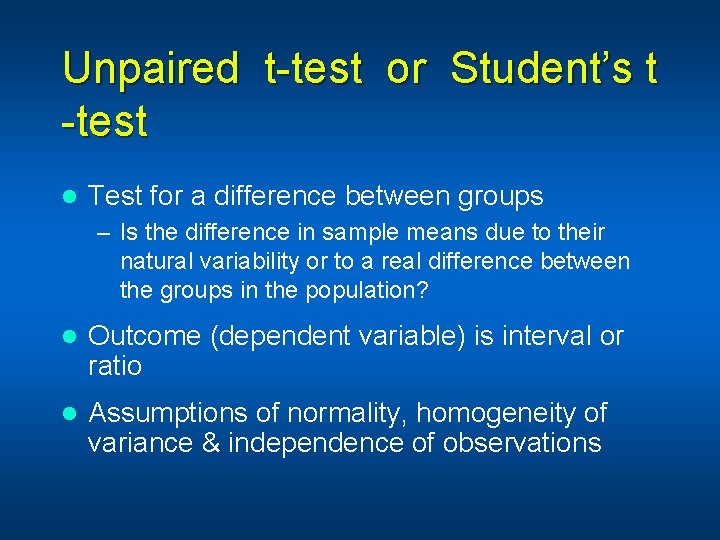
Unpaired t-test or Student’s t -test l Test for a difference between groups – Is the difference in sample means due to their natural variability or to a real difference between the groups in the population? l Outcome (dependent variable) is interval or ratio l Assumptions of normality, homogeneity of variance & independence of observations
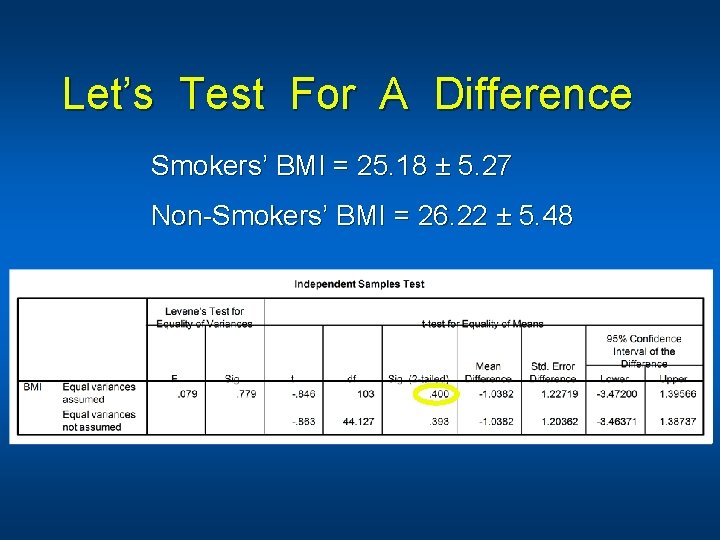
Let’s Test For A Difference Smokers’ BMI = 25. 18 ± 5. 27 Non-Smokers’ BMI = 26. 22 ± 5. 48
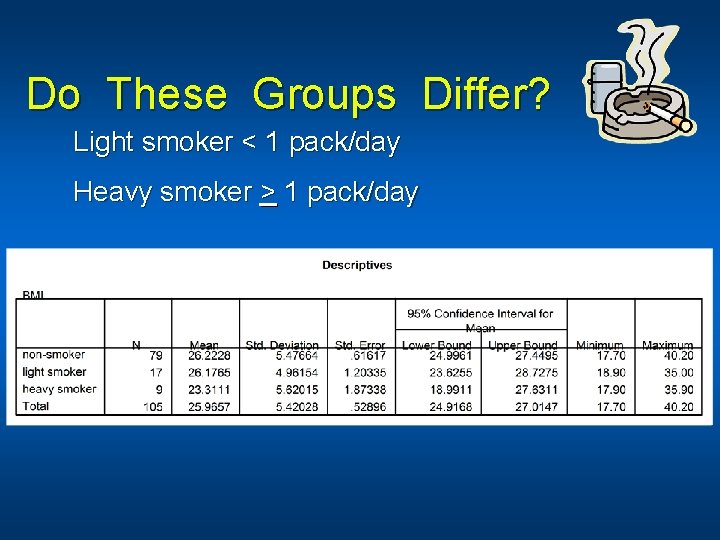
Do These Groups Differ? Light smoker < 1 pack/day Heavy smoker > 1 pack/day
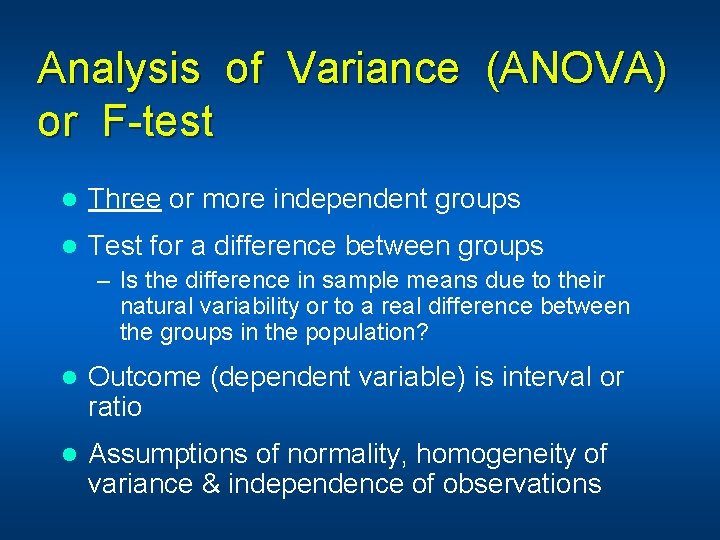
Analysis of Variance (ANOVA) or F-test l Three or more independent groups l Test for a difference between groups – Is the difference in sample means due to their natural variability or to a real difference between the groups in the population? l Outcome (dependent variable) is interval or ratio l Assumptions of normality, homogeneity of variance & independence of observations
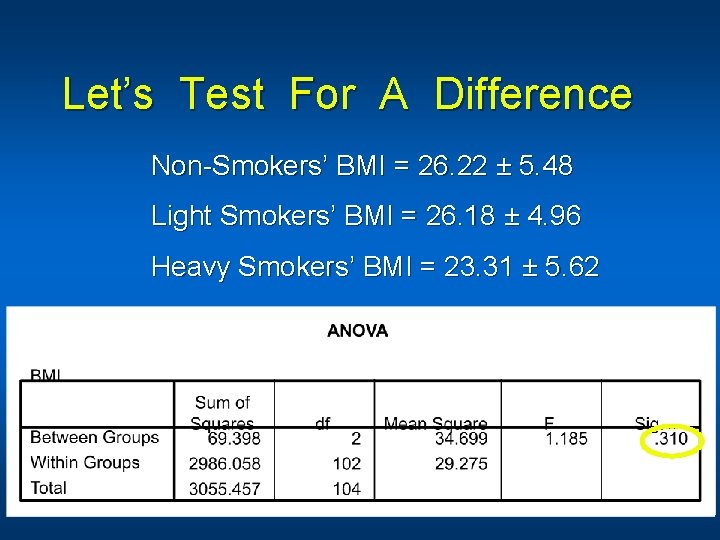
Let’s Test For A Difference Non-Smokers’ BMI = 26. 22 ± 5. 48 Light Smokers’ BMI = 26. 18 ± 4. 96 Heavy Smokers’ BMI = 23. 31 ± 5. 62
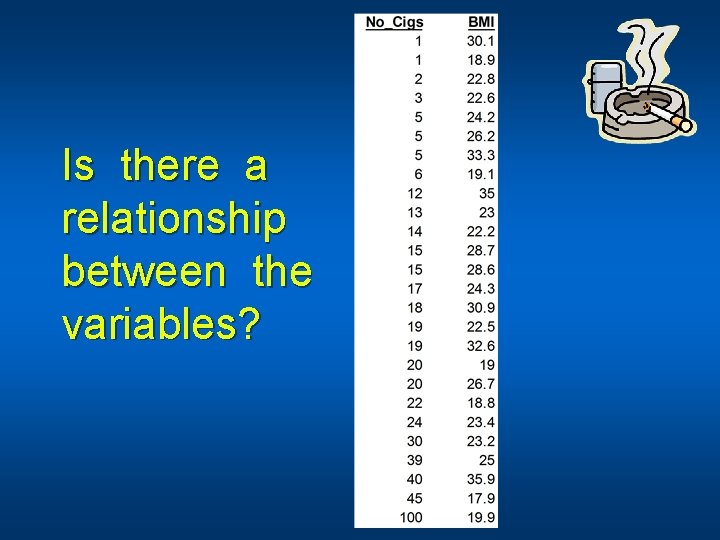
Is there a relationship between the variables?
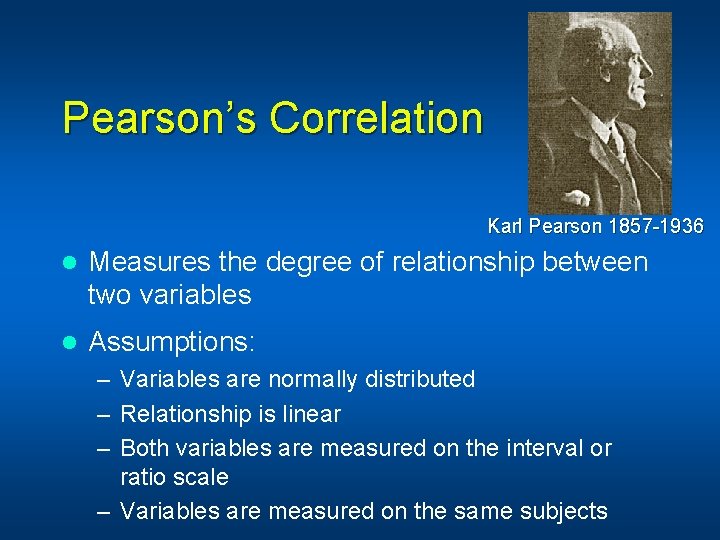
Pearson’s Correlation Karl Pearson 1857 -1936 l Measures the degree of relationship between two variables l Assumptions: – Variables are normally distributed – Relationship is linear – Both variables are measured on the interval or ratio scale – Variables are measured on the same subjects
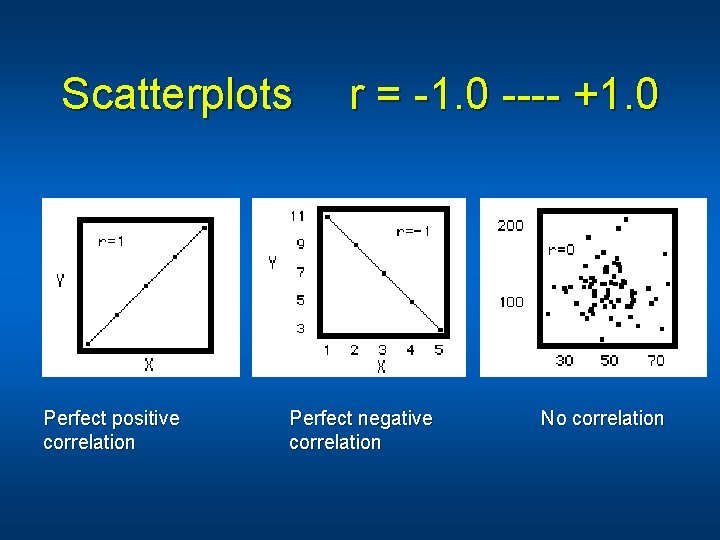
Scatterplots Perfect positive correlation r = -1. 0 ---- +1. 0 Perfect negative correlation No correlation
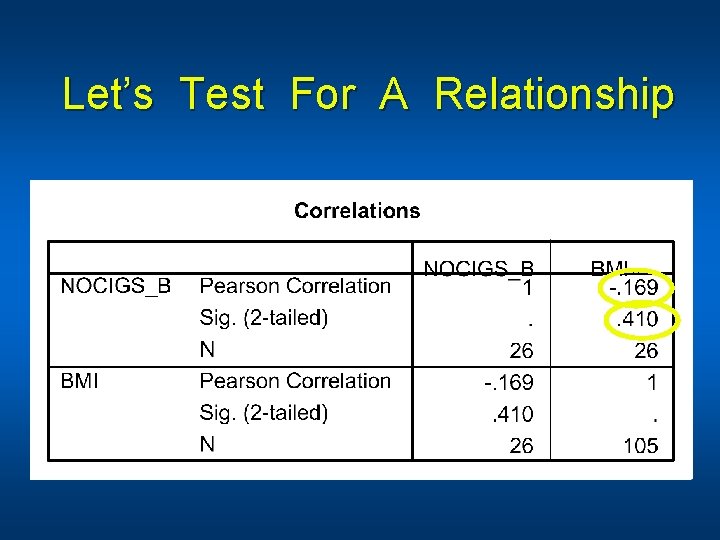
Let’s Test For A Relationship
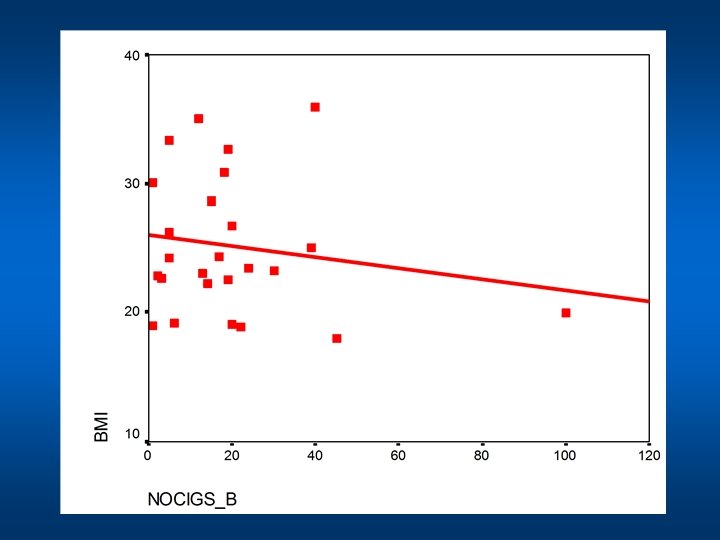
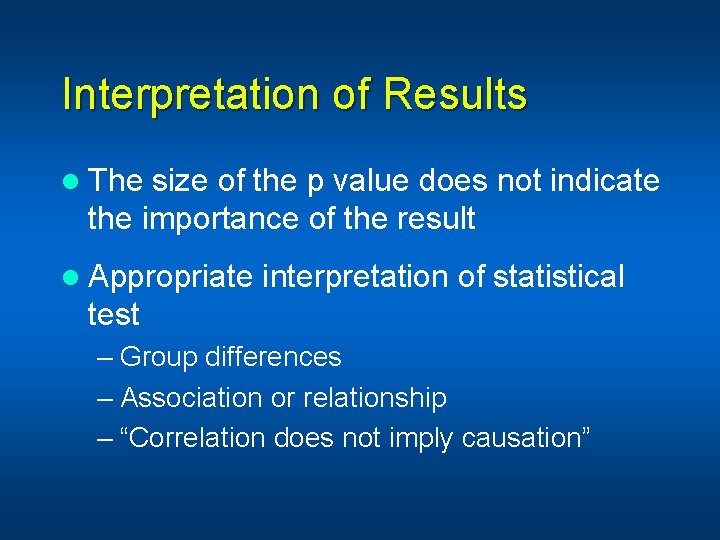
Interpretation of Results l The size of the p value does not indicate the importance of the result l Appropriate interpretation of statistical test – Group differences – Association or relationship – “Correlation does not imply causation”
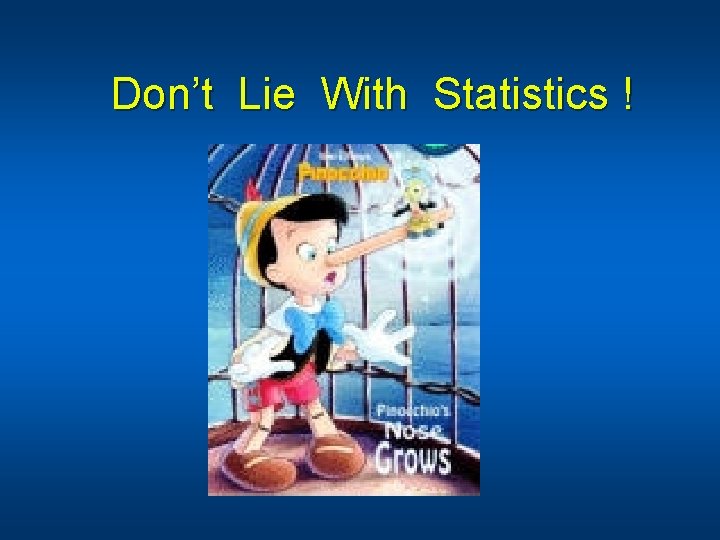
Don’t Lie With Statistics !
Ace different tests iq tests but
Stats table psychology
Yyxyxx
Univariate statistical tests
Psychology statistical test table
T test ordinal data
Constituency tests syntax exercises
Year 3 mental maths tests
Oaks portal test administrators
List and describe 3 tests of cerebellar function
Wigan lea mental maths
Rhomberg test
Normal lft
Unit 10, unit 10 review tests, unit 10 general test
Parallelogram notes
Managers can use overt integrity tests to
Copper acetate test for lipids
Denatured protein
Content uniformity definition
Loughborough uni testing
Stanford binet test
Family and friends 2 tests
Chapter 24 diagnostic tests and specimen collection
City and guilds secure assess
Nonparametric tests
Hops history questions
Uil number sense tricks - elementary
Meap practice tests
Intelligence tests are “biased” in the sense that
Hippoevent
Worry
One of the most carefully produced assessment tests is the
Hemastix blood test
Qualitative tests for amino acids
Positive nachlas test
Physical fitness test grade 9
Tea dccr
Summary of tests for series
Psychometric tests
Types of significance tests
Anemia classification
Chapter 20 more about tests and intervals
Dugas test shoulder
Family and friends tests
Special tests for ankle
Ethylene oxide pcr tests
Uil number sense scoring
Year 4 mental maths tests
Stanag 6001 speaking examples