Reasoning Under Uncertainty Kostas Kontogiannis ECE 457 Terminology
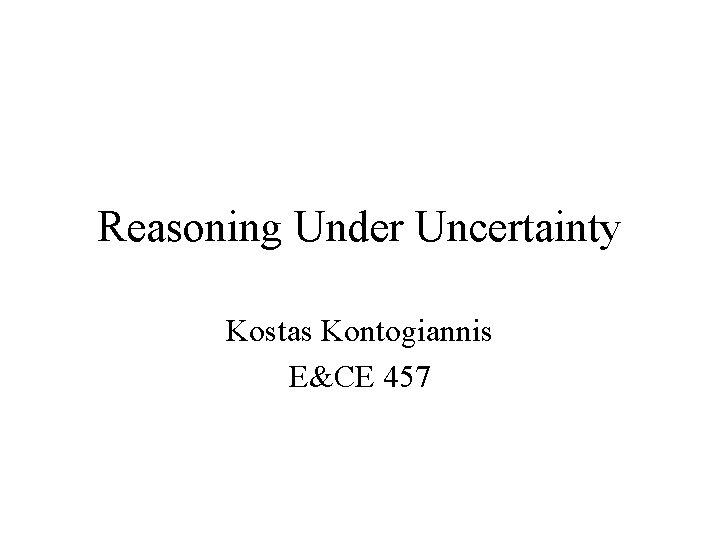
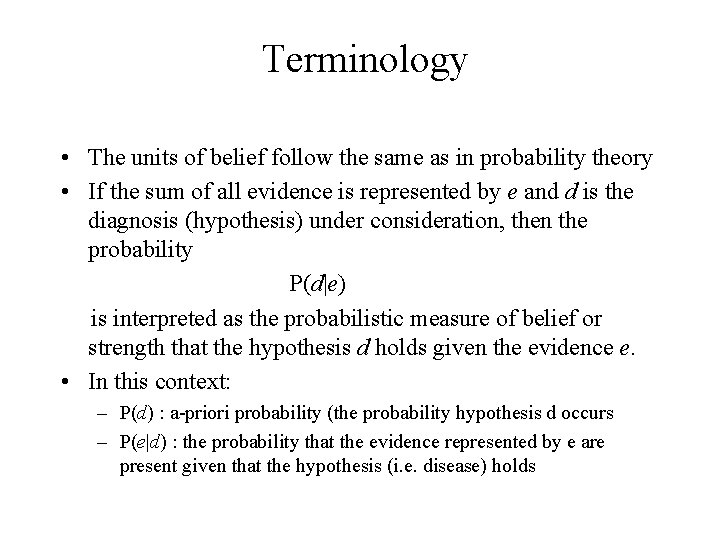
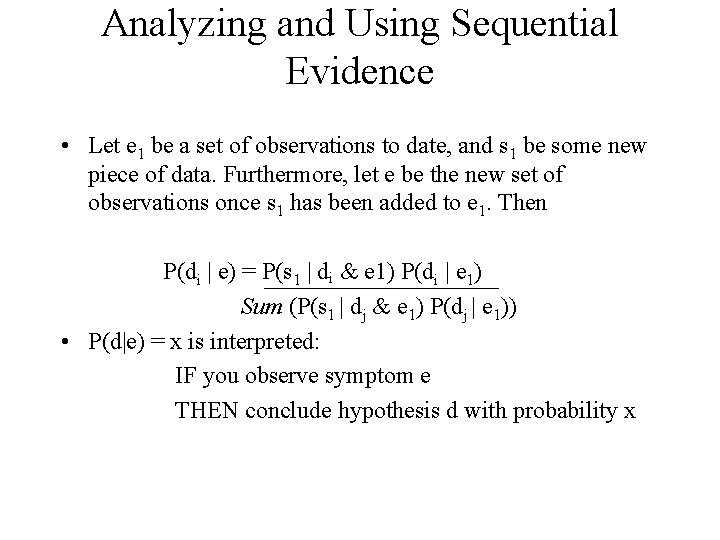
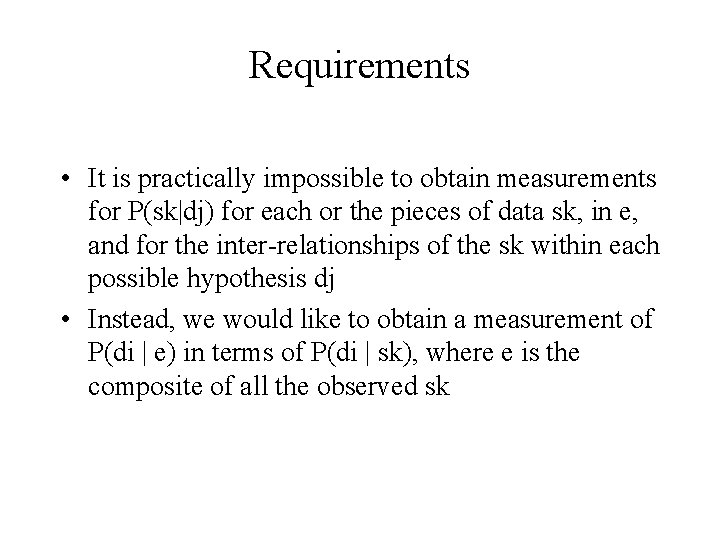
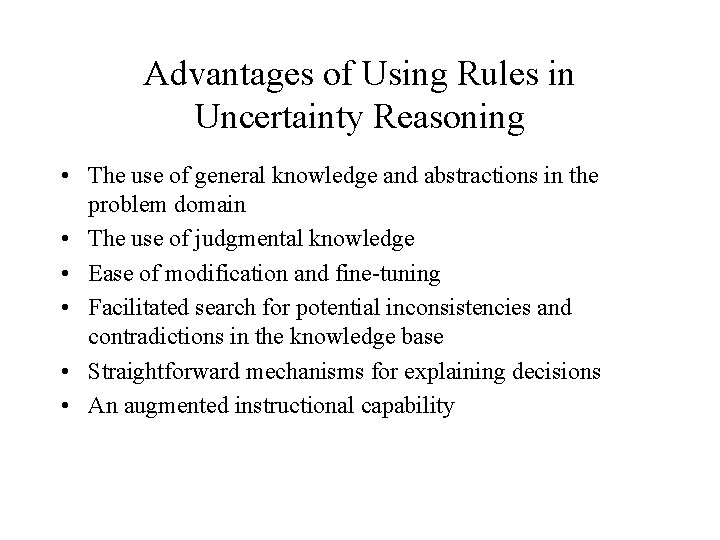
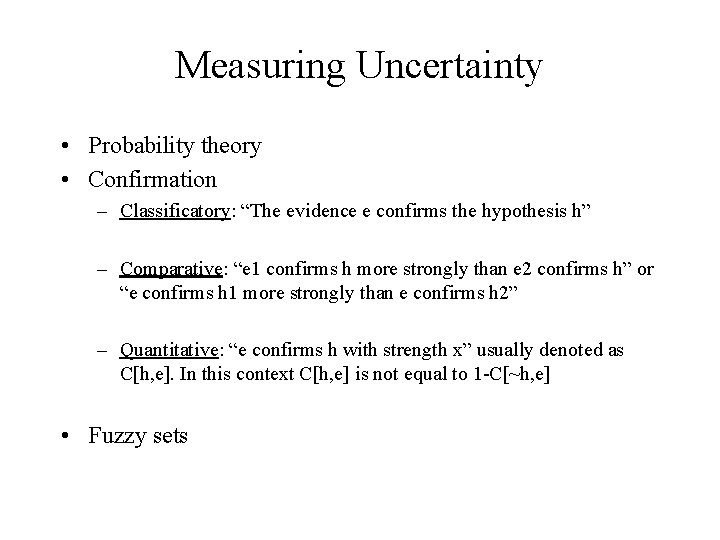
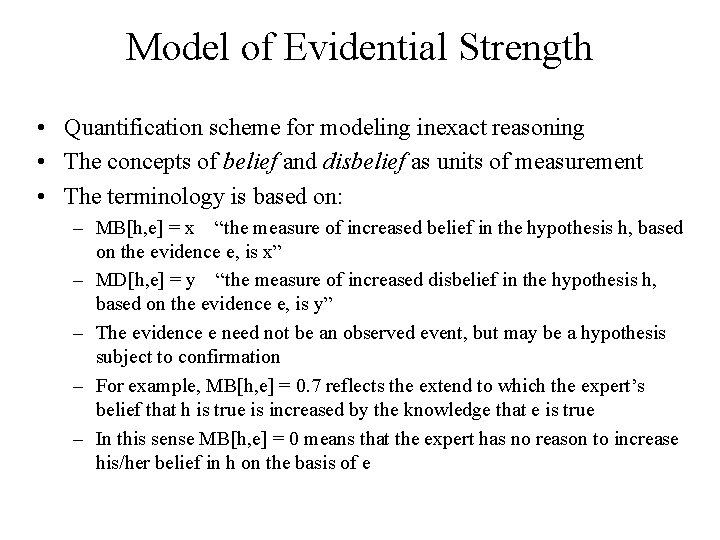
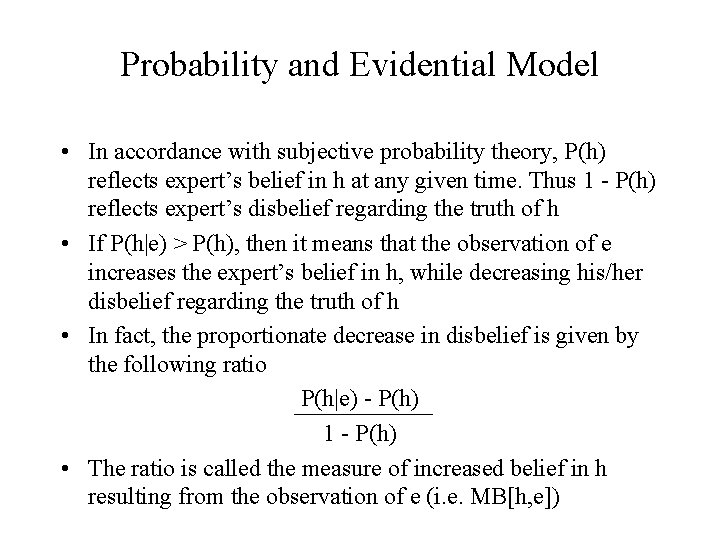
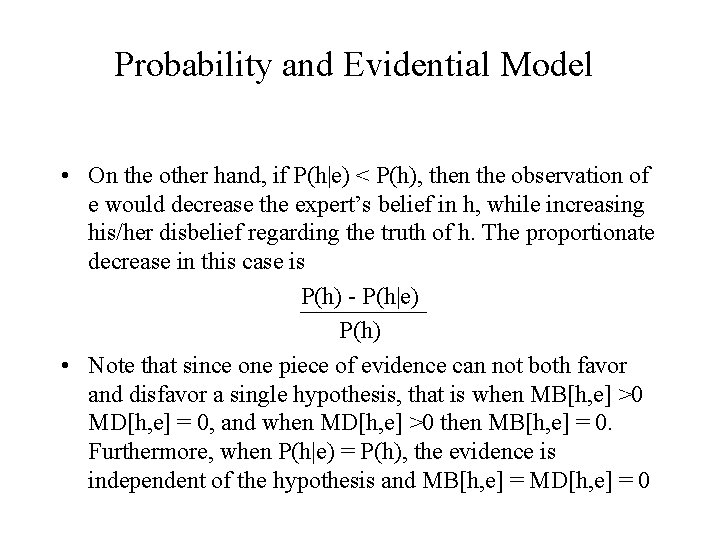
![Definitions of Evidential Model MB[h, e] = { 1 if P(h) = 1 max[P(h|e), Definitions of Evidential Model MB[h, e] = { 1 if P(h) = 1 max[P(h|e),](https://slidetodoc.com/presentation_image_h/dbf5a6adc40aeb4abaa854c074553c46/image-10.jpg)
![Characteristics of Belief Measures • Range of degrees: 0 <= MB[h, e] <= 1 Characteristics of Belief Measures • Range of degrees: 0 <= MB[h, e] <= 1](https://slidetodoc.com/presentation_image_h/dbf5a6adc40aeb4abaa854c074553c46/image-11.jpg)
![Characteristics of Belief Measures • Suggested limits and ranges: -1 = CF[h, ~h] <= Characteristics of Belief Measures • Suggested limits and ranges: -1 = CF[h, ~h] <=](https://slidetodoc.com/presentation_image_h/dbf5a6adc40aeb4abaa854c074553c46/image-12.jpg)
![More Characteristics of Belief Measures • CF[h, e] + CF[~h, e] =/= 1 • More Characteristics of Belief Measures • CF[h, e] + CF[~h, e] =/= 1 •](https://slidetodoc.com/presentation_image_h/dbf5a6adc40aeb4abaa854c074553c46/image-13.jpg)
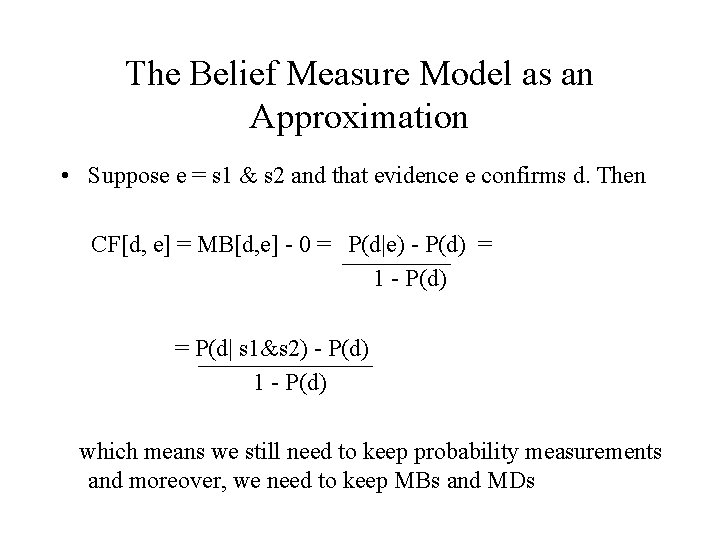
![Defining Criteria for Approximation • MB[h, e+] increases toward 1 as confirming evidence is Defining Criteria for Approximation • MB[h, e+] increases toward 1 as confirming evidence is](https://slidetodoc.com/presentation_image_h/dbf5a6adc40aeb4abaa854c074553c46/image-15.jpg)
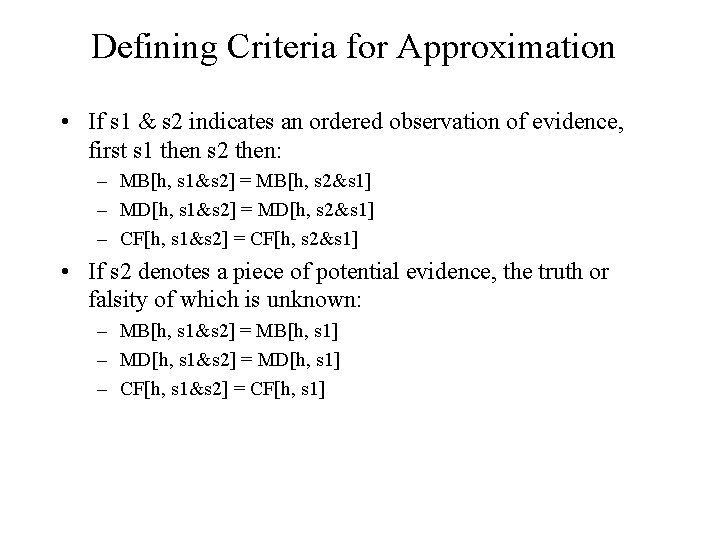
![Combining Functions MB[h, s 1&s 2] MD[h, s 1&s 2] = = { { Combining Functions MB[h, s 1&s 2] MD[h, s 1&s 2] = = { {](https://slidetodoc.com/presentation_image_h/dbf5a6adc40aeb4abaa854c074553c46/image-17.jpg)
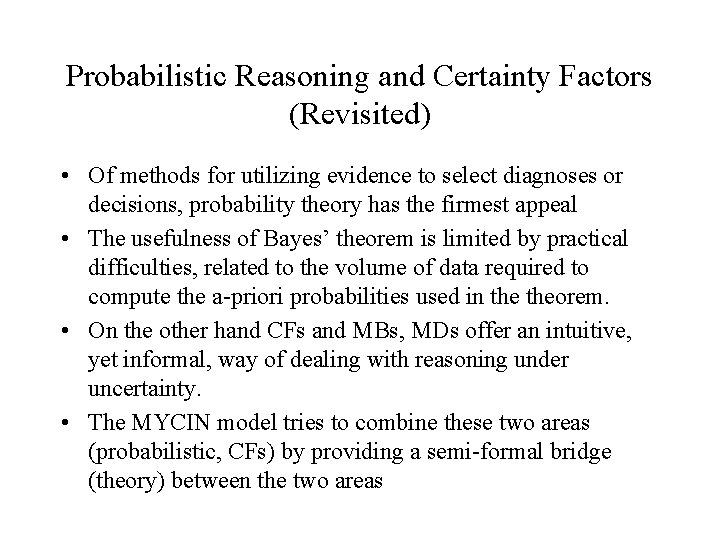
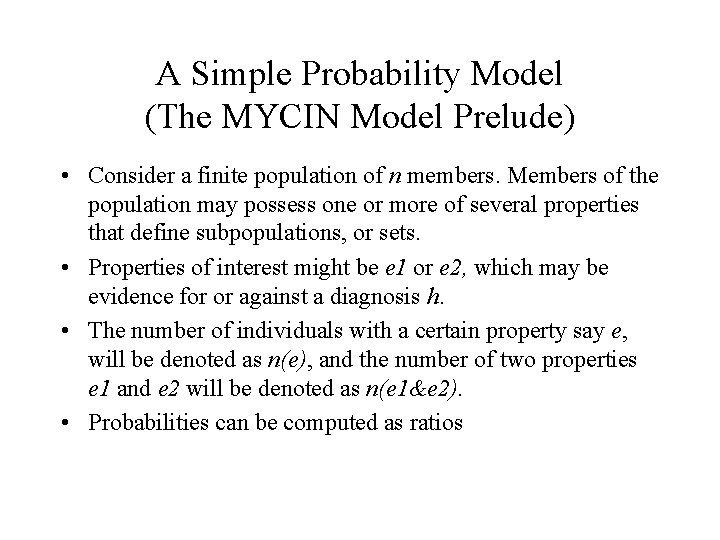
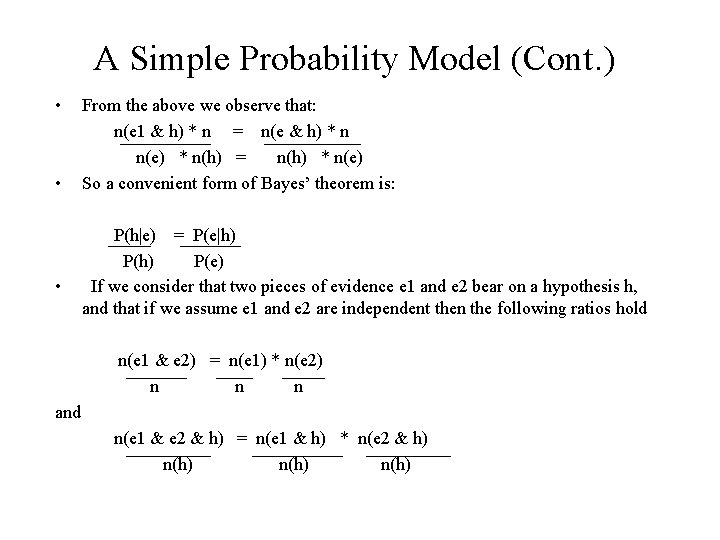
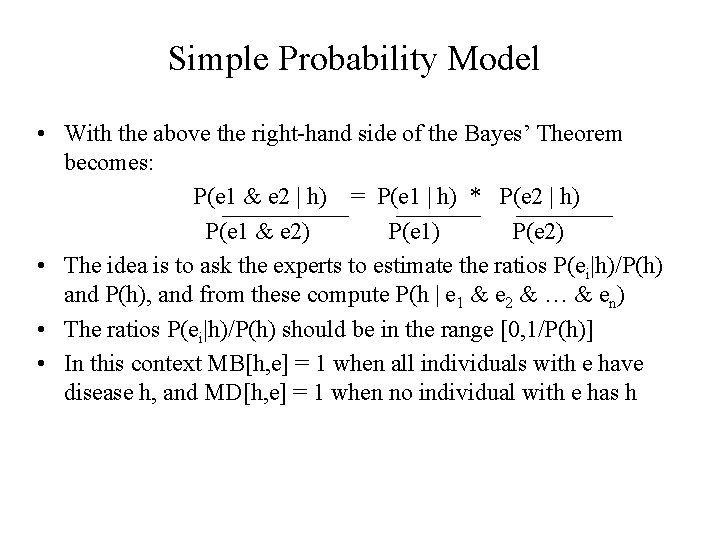
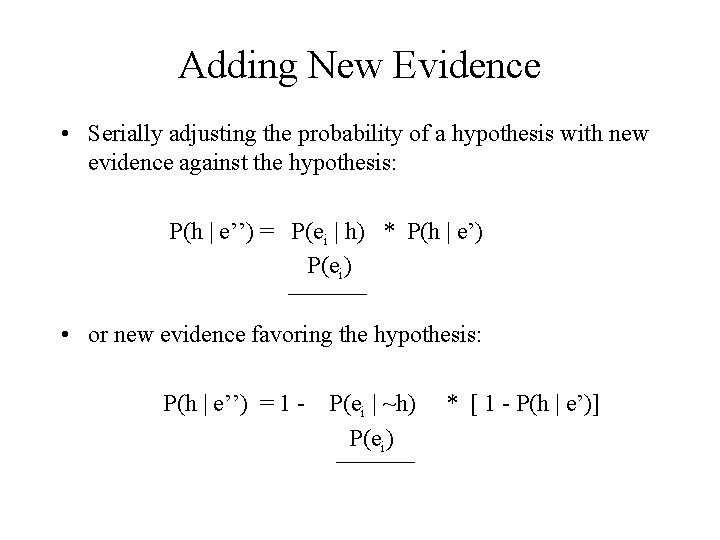
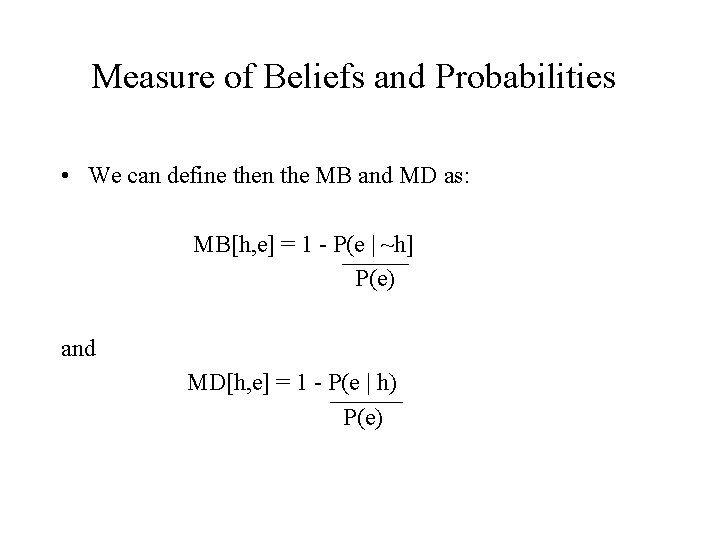
![The MYCIN Model • MB[h 1 & h 2, e] = min(MB[h 1, e], The MYCIN Model • MB[h 1 & h 2, e] = min(MB[h 1, e],](https://slidetodoc.com/presentation_image_h/dbf5a6adc40aeb4abaa854c074553c46/image-24.jpg)
- Slides: 24
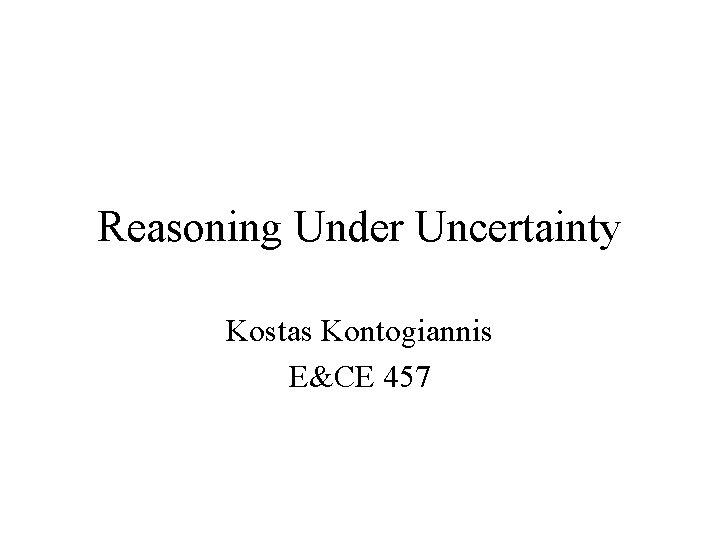
Reasoning Under Uncertainty Kostas Kontogiannis E&CE 457
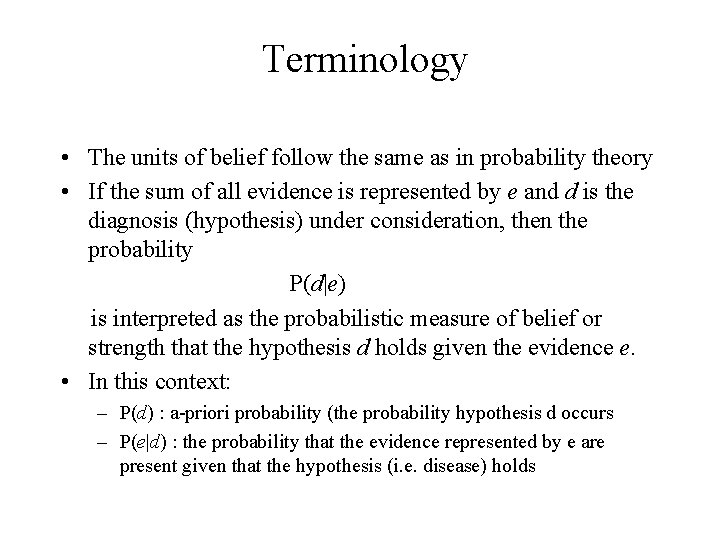
Terminology • The units of belief follow the same as in probability theory • If the sum of all evidence is represented by e and d is the diagnosis (hypothesis) under consideration, then the probability P(d|e) is interpreted as the probabilistic measure of belief or strength that the hypothesis d holds given the evidence e. • In this context: – P(d) : a-priori probability (the probability hypothesis d occurs – P(e|d) : the probability that the evidence represented by e are present given that the hypothesis (i. e. disease) holds
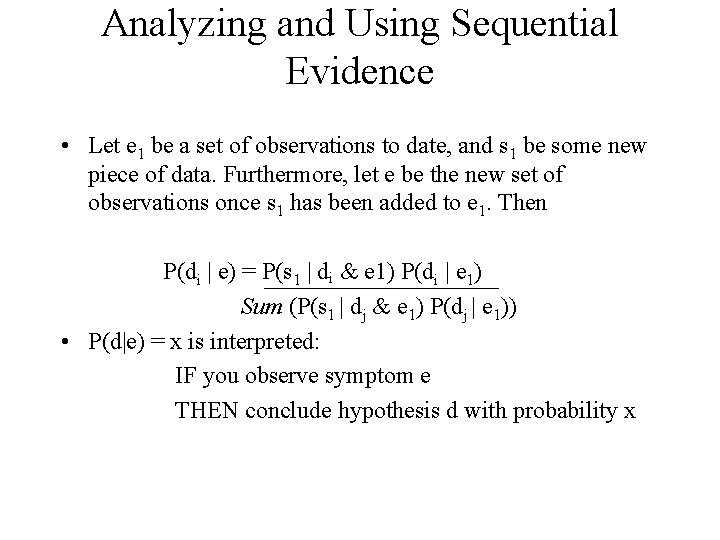
Analyzing and Using Sequential Evidence • Let e 1 be a set of observations to date, and s 1 be some new piece of data. Furthermore, let e be the new set of observations once s 1 has been added to e 1. Then P(di | e) = P(s 1 | di & e 1) P(di | e 1) Sum (P(s 1 | dj & e 1) P(dj | e 1)) • P(d|e) = x is interpreted: IF you observe symptom e THEN conclude hypothesis d with probability x
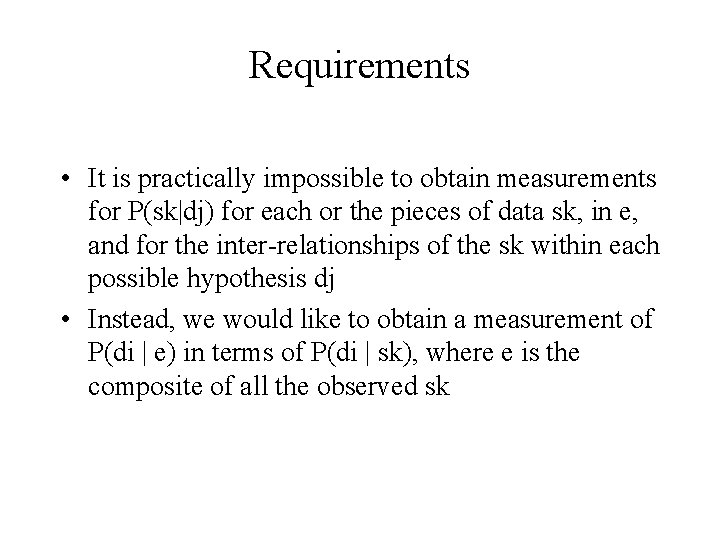
Requirements • It is practically impossible to obtain measurements for P(sk|dj) for each or the pieces of data sk, in e, and for the inter-relationships of the sk within each possible hypothesis dj • Instead, we would like to obtain a measurement of P(di | e) in terms of P(di | sk), where e is the composite of all the observed sk
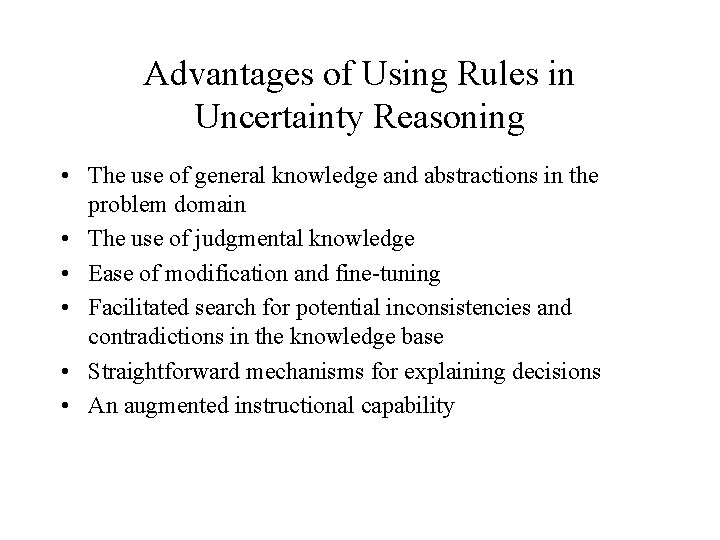
Advantages of Using Rules in Uncertainty Reasoning • The use of general knowledge and abstractions in the problem domain • The use of judgmental knowledge • Ease of modification and fine-tuning • Facilitated search for potential inconsistencies and contradictions in the knowledge base • Straightforward mechanisms for explaining decisions • An augmented instructional capability
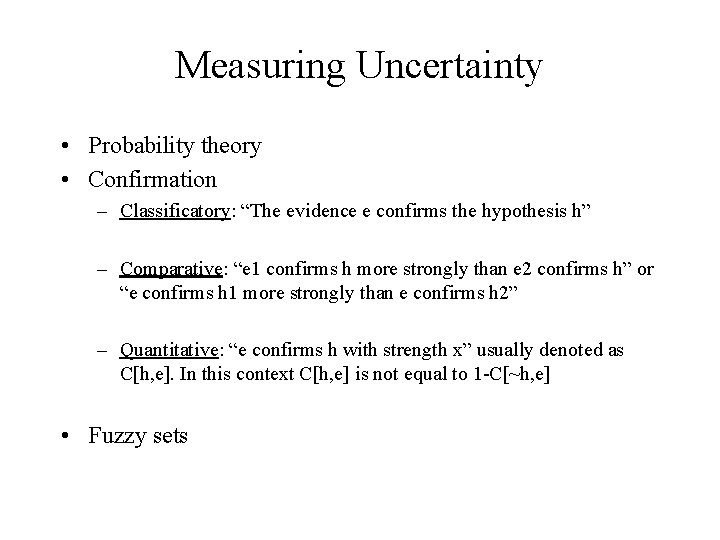
Measuring Uncertainty • Probability theory • Confirmation – Classificatory: “The evidence e confirms the hypothesis h” – Comparative: “e 1 confirms h more strongly than e 2 confirms h” or “e confirms h 1 more strongly than e confirms h 2” – Quantitative: “e confirms h with strength x” usually denoted as C[h, e]. In this context C[h, e] is not equal to 1 -C[~h, e] • Fuzzy sets
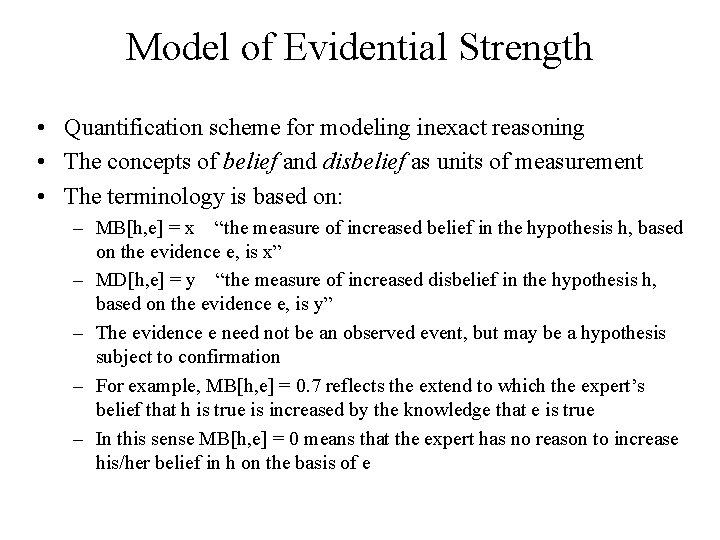
Model of Evidential Strength • Quantification scheme for modeling inexact reasoning • The concepts of belief and disbelief as units of measurement • The terminology is based on: – MB[h, e] = x “the measure of increased belief in the hypothesis h, based on the evidence e, is x” – MD[h, e] = y “the measure of increased disbelief in the hypothesis h, based on the evidence e, is y” – The evidence e need not be an observed event, but may be a hypothesis subject to confirmation – For example, MB[h, e] = 0. 7 reflects the extend to which the expert’s belief that h is true is increased by the knowledge that e is true – In this sense MB[h, e] = 0 means that the expert has no reason to increase his/her belief in h on the basis of e
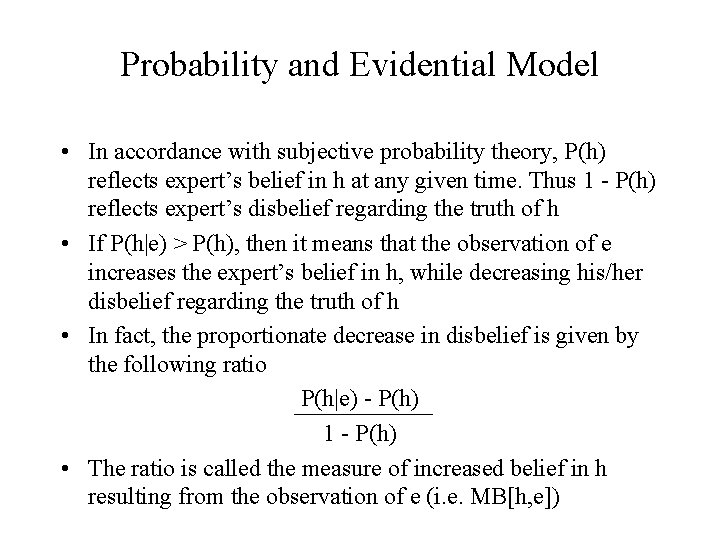
Probability and Evidential Model • In accordance with subjective probability theory, P(h) reflects expert’s belief in h at any given time. Thus 1 - P(h) reflects expert’s disbelief regarding the truth of h • If P(h|e) > P(h), then it means that the observation of e increases the expert’s belief in h, while decreasing his/her disbelief regarding the truth of h • In fact, the proportionate decrease in disbelief is given by the following ratio P(h|e) - P(h) 1 - P(h) • The ratio is called the measure of increased belief in h resulting from the observation of e (i. e. MB[h, e])
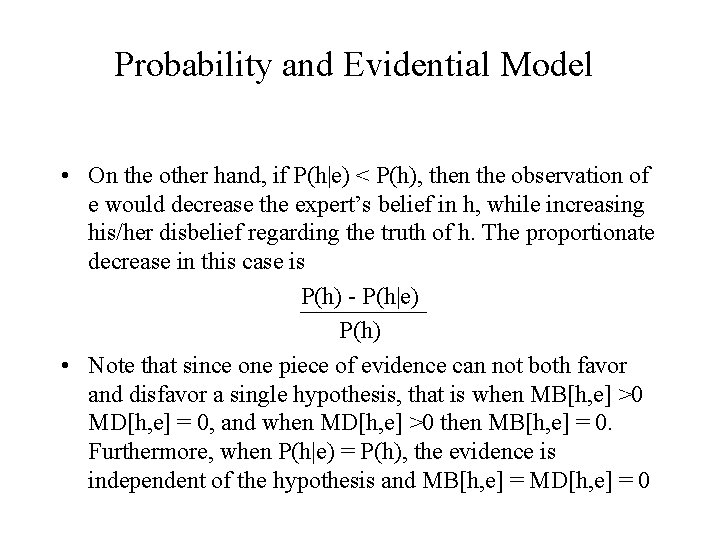
Probability and Evidential Model • On the other hand, if P(h|e) < P(h), then the observation of e would decrease the expert’s belief in h, while increasing his/her disbelief regarding the truth of h. The proportionate decrease in this case is P(h) - P(h|e) P(h) • Note that since one piece of evidence can not both favor and disfavor a single hypothesis, that is when MB[h, e] >0 MD[h, e] = 0, and when MD[h, e] >0 then MB[h, e] = 0. Furthermore, when P(h|e) = P(h), the evidence is independent of the hypothesis and MB[h, e] = MD[h, e] = 0
![Definitions of Evidential Model MBh e 1 if Ph 1 maxPhe Definitions of Evidential Model MB[h, e] = { 1 if P(h) = 1 max[P(h|e),](https://slidetodoc.com/presentation_image_h/dbf5a6adc40aeb4abaa854c074553c46/image-10.jpg)
Definitions of Evidential Model MB[h, e] = { 1 if P(h) = 1 max[P(h|e), P(h)] - P(h) otherwise 1 - P(h) MD[h, e] = { 1 if P(h) = 0 min[P(h|e), P(h)] - P(h) otherwise - P(h) CF[h, e] = MB[h, e] - MD[h, e]
![Characteristics of Belief Measures Range of degrees 0 MBh e 1 Characteristics of Belief Measures • Range of degrees: 0 <= MB[h, e] <= 1](https://slidetodoc.com/presentation_image_h/dbf5a6adc40aeb4abaa854c074553c46/image-11.jpg)
Characteristics of Belief Measures • Range of degrees: 0 <= MB[h, e] <= 1 0 <= MD[h, e] <= 1 -1 <= CF[h, e] <= +1 • Evidential strength of mutually exclusive hypotheses – If h is shown to be certain P(h|e) = 1 MB[[h, e] = 1 MD[h, e] = 0 CF[h, e] = 1 – If the negation of h is shown to be certain P(~h|e) = 1 MB[h, e] = 0 MD[h, e] = 1 CF[h, e] = -1
![Characteristics of Belief Measures Suggested limits and ranges 1 CFh h Characteristics of Belief Measures • Suggested limits and ranges: -1 = CF[h, ~h] <=](https://slidetodoc.com/presentation_image_h/dbf5a6adc40aeb4abaa854c074553c46/image-12.jpg)
Characteristics of Belief Measures • Suggested limits and ranges: -1 = CF[h, ~h] <= CF[h, e] <= C[h, h] = +1 • Note: MB[~h, e] = 1 if and only if MD[h, e] = 1 • For mutually exclusive hypotheses h 1 and h 2, if MB[h 1, e] = 1, then MD[h 2, e] = 1 • Lack of evidence: – MB[h, e] = 0 if h is not confirmed by e (i. e. e and h are independent or e disconfirms h) – MD[h, e] = 0 if h is not disconfirmed by e (i. e. e and h are independent or e confirms h) – CF[h, e] = 0 if e neither confirms nor disconfirms h (i. e. e and h are independent)
![More Characteristics of Belief Measures CFh e CFh e 1 More Characteristics of Belief Measures • CF[h, e] + CF[~h, e] =/= 1 •](https://slidetodoc.com/presentation_image_h/dbf5a6adc40aeb4abaa854c074553c46/image-13.jpg)
More Characteristics of Belief Measures • CF[h, e] + CF[~h, e] =/= 1 • MB[h, e] = MD[~h, e]
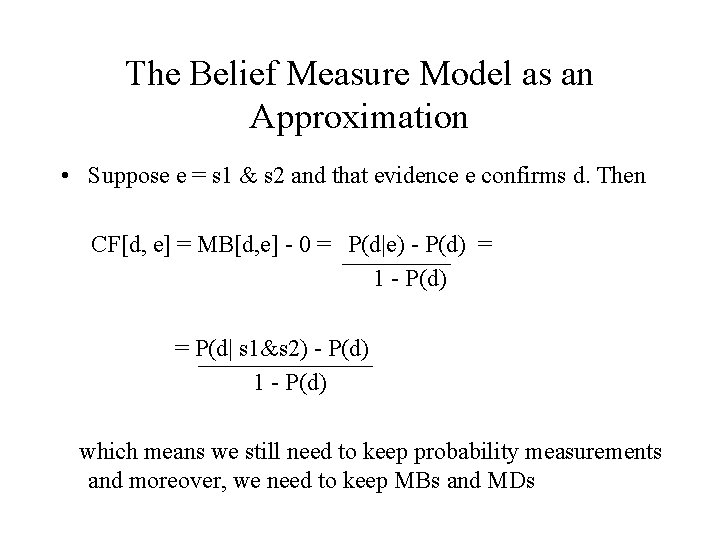
The Belief Measure Model as an Approximation • Suppose e = s 1 & s 2 and that evidence e confirms d. Then CF[d, e] = MB[d, e] - 0 = P(d|e) - P(d) = 1 - P(d) = P(d| s 1&s 2) - P(d) 1 - P(d) which means we still need to keep probability measurements and moreover, we need to keep MBs and MDs
![Defining Criteria for Approximation MBh e increases toward 1 as confirming evidence is Defining Criteria for Approximation • MB[h, e+] increases toward 1 as confirming evidence is](https://slidetodoc.com/presentation_image_h/dbf5a6adc40aeb4abaa854c074553c46/image-15.jpg)
Defining Criteria for Approximation • MB[h, e+] increases toward 1 as confirming evidence is found, equaling 1 if and only f a piece of evidence logically implies h with certainty • MD[h, e-] increases toward 1 as disconfirming evidence is found, equaling 1 if and only if a piece of evidence logically implies ~h with certainty • CF[h, e-] <= CF[h, e- & e+] <= CF[h, e+] • MB[h, e+] = 1 then MD[h, e-] = 0 and CF[h, e+] = 1 • MD[h, e-] = 1 then MB[h, e+] = 0 and CF[h, e-] = -1 • The case where MB[h, e+] = MD[h, e-] = 1 is contradictory and hence CF is undefined
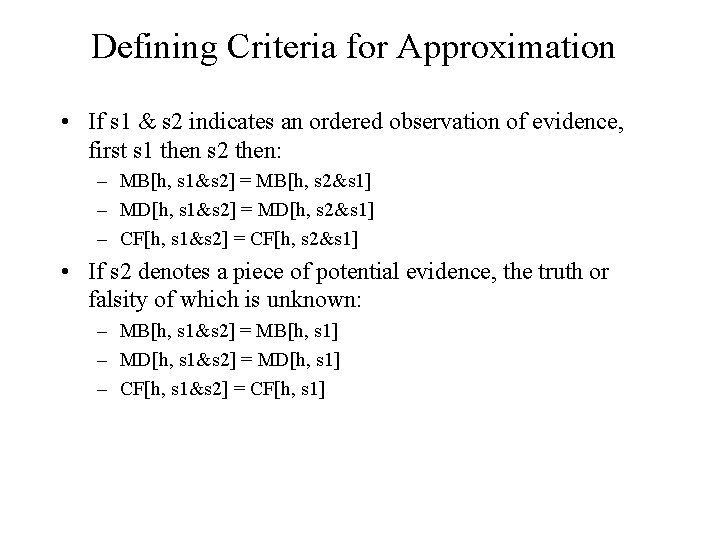
Defining Criteria for Approximation • If s 1 & s 2 indicates an ordered observation of evidence, first s 1 then s 2 then: – MB[h, s 1&s 2] = MB[h, s 2&s 1] – MD[h, s 1&s 2] = MD[h, s 2&s 1] – CF[h, s 1&s 2] = CF[h, s 2&s 1] • If s 2 denotes a piece of potential evidence, the truth or falsity of which is unknown: – MB[h, s 1&s 2] = MB[h, s 1] – MD[h, s 1&s 2] = MD[h, s 1] – CF[h, s 1&s 2] = CF[h, s 1]
![Combining Functions MBh s 1s 2 MDh s 1s 2 Combining Functions MB[h, s 1&s 2] MD[h, s 1&s 2] = = { {](https://slidetodoc.com/presentation_image_h/dbf5a6adc40aeb4abaa854c074553c46/image-17.jpg)
Combining Functions MB[h, s 1&s 2] MD[h, s 1&s 2] = = { { 0 If MD[h, s 1&s 2] = 1 MB[h, s 1] + MB[h, s 2](1 - MB[h, s 1]) 0 otherwise If MB[h, s 1&s 2] = 1 MD[h, s 1] + MD[h, s 2](1 - MD[h, s 1]) MB[h 1 or h 2, e] = max(MB[h 1, e], MB[h 2, e]) MD[h 1 or h 2, e] = min(MD[h 1, e], MD[h 2, e]) MB[h, s 1] = MB’[h, s 1] * max(0, CF[s 1, e]) MD[h, s 1] = MD’[h, s 1] * max(0, CF[s 1, e]) otherwise
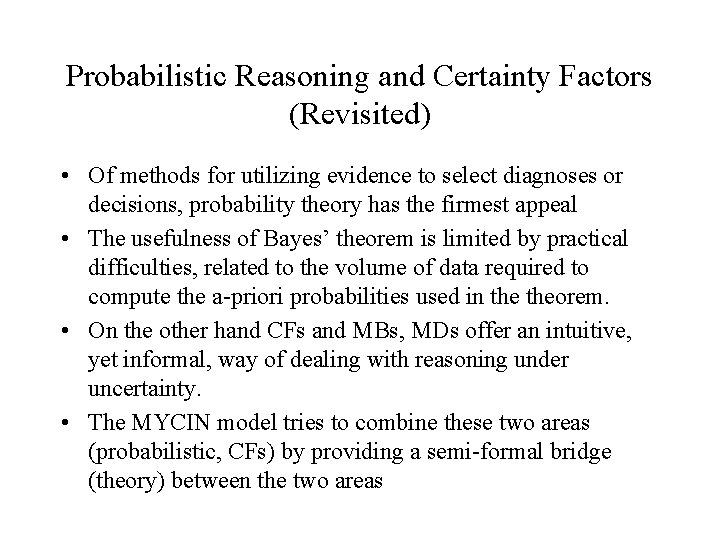
Probabilistic Reasoning and Certainty Factors (Revisited) • Of methods for utilizing evidence to select diagnoses or decisions, probability theory has the firmest appeal • The usefulness of Bayes’ theorem is limited by practical difficulties, related to the volume of data required to compute the a-priori probabilities used in theorem. • On the other hand CFs and MBs, MDs offer an intuitive, yet informal, way of dealing with reasoning under uncertainty. • The MYCIN model tries to combine these two areas (probabilistic, CFs) by providing a semi-formal bridge (theory) between the two areas
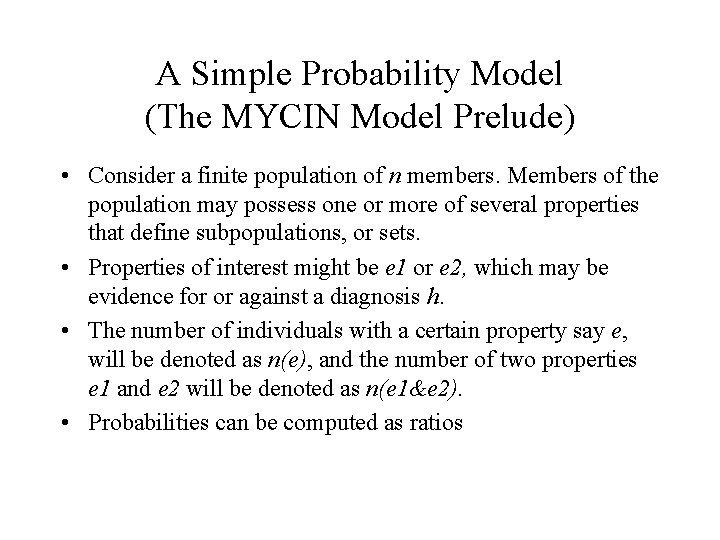
A Simple Probability Model (The MYCIN Model Prelude) • Consider a finite population of n members. Members of the population may possess one or more of several properties that define subpopulations, or sets. • Properties of interest might be e 1 or e 2, which may be evidence for or against a diagnosis h. • The number of individuals with a certain property say e, will be denoted as n(e), and the number of two properties e 1 and e 2 will be denoted as n(e 1&e 2). • Probabilities can be computed as ratios
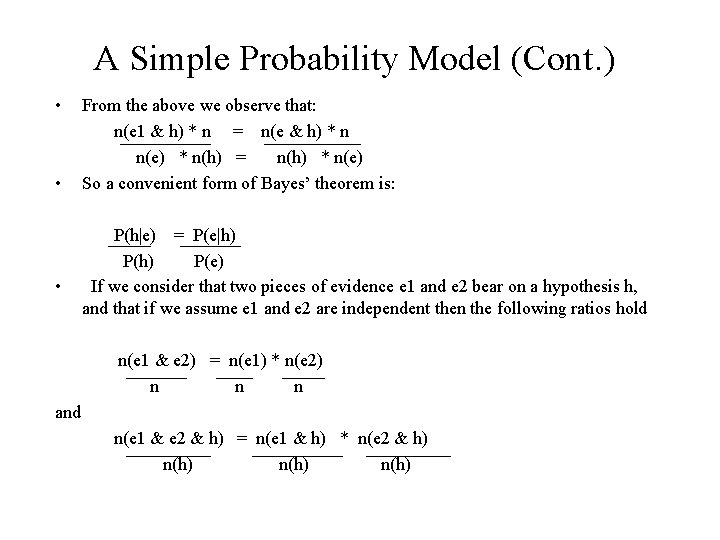
A Simple Probability Model (Cont. ) • • • From the above we observe that: n(e 1 & h) * n = n(e & h) * n n(e) * n(h) = n(h) * n(e) So a convenient form of Bayes’ theorem is: P(h|e) = P(e|h) P(e) If we consider that two pieces of evidence e 1 and e 2 bear on a hypothesis h, and that if we assume e 1 and e 2 are independent then the following ratios hold n(e 1 & e 2) = n(e 1) * n(e 2) n n n and n(e 1 & e 2 & h) = n(e 1 & h) * n(e 2 & h) n(h)
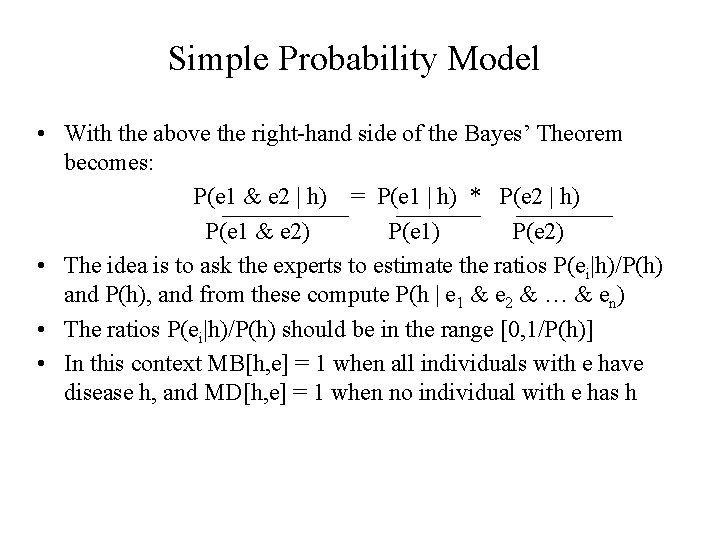
Simple Probability Model • With the above the right-hand side of the Bayes’ Theorem becomes: P(e 1 & e 2 | h) = P(e 1 | h) * P(e 2 | h) P(e 1 & e 2) P(e 1) P(e 2) • The idea is to ask the experts to estimate the ratios P(ei|h)/P(h) and P(h), and from these compute P(h | e 1 & e 2 & … & en) • The ratios P(ei|h)/P(h) should be in the range [0, 1/P(h)] • In this context MB[h, e] = 1 when all individuals with e have disease h, and MD[h, e] = 1 when no individual with e has h
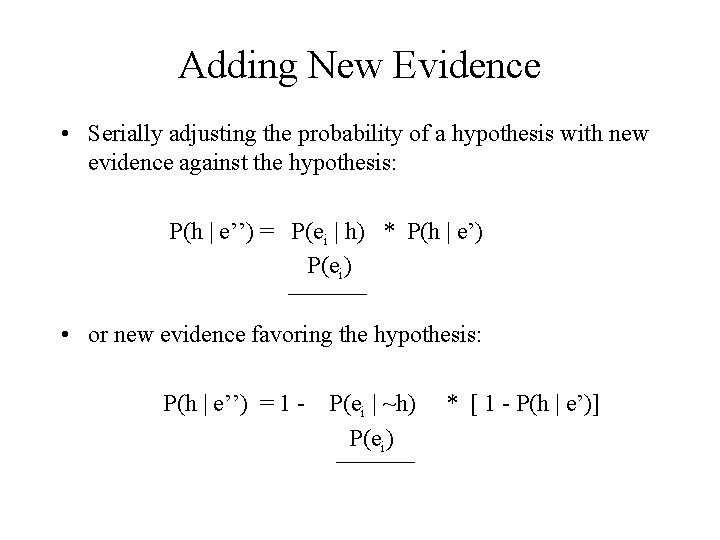
Adding New Evidence • Serially adjusting the probability of a hypothesis with new evidence against the hypothesis: P(h | e’’) = P(ei | h) * P(h | e’) P(ei) • or new evidence favoring the hypothesis: P(h | e’’) = 1 - P(ei | ~h) P(ei) * [ 1 - P(h | e’)]
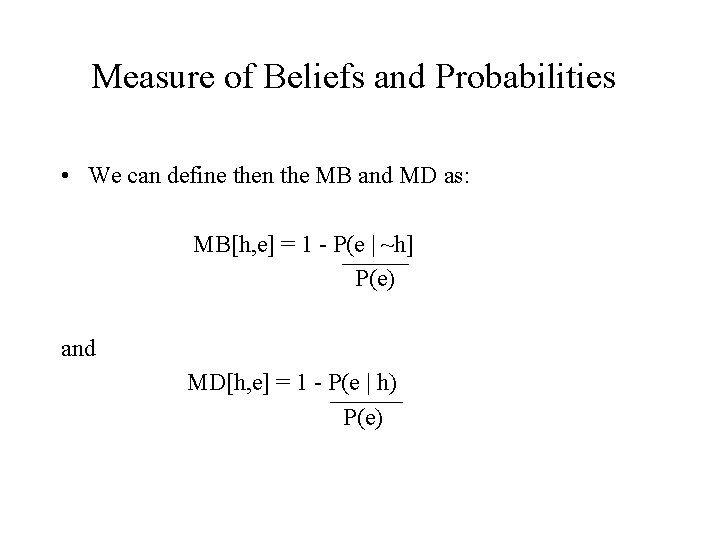
Measure of Beliefs and Probabilities • We can define then the MB and MD as: MB[h, e] = 1 - P(e | ~h] P(e) and MD[h, e] = 1 - P(e | h) P(e)
![The MYCIN Model MBh 1 h 2 e minMBh 1 e The MYCIN Model • MB[h 1 & h 2, e] = min(MB[h 1, e],](https://slidetodoc.com/presentation_image_h/dbf5a6adc40aeb4abaa854c074553c46/image-24.jpg)
The MYCIN Model • MB[h 1 & h 2, e] = min(MB[h 1, e], MB[h 2, e]) • MD[h 1 & h 2, e] = max(MD[h 1, e], MD[h 2, e]) • MB[h 1 or h 2, e) = max(MB[h 1, e], MB[h 2, e]) • MD[h 1 or h 2, e) = min(MD[h 1, e], MDh 2, e]) • 1 - MD[h, e 1 & e 2] = (1 - MD[h, e 1])*(1 -MD[h, e 2]) • 1 - MB[h, e 1 & e 2] = (1 - MB[h, e 1])*(1 -MB[h, e 2]) • CF(h, ef & ea) = MB[h, ef] - MD[h, ea]
Reiters
Selin aviyente
Kostas andreadis
Liūnė sutema
Kostas glampedakis
Kostas ostrauskas
Capital budgeting under risk and uncertainty
Expected profit under uncertainty
Uncertainity in decision making
Decision-making under uncertainty
What is deductive reasoning
No mayten tree is deciduous
Inductive reasoning vs deductive reasoning
Patterns and inductive reasoning
Inductive vs deductive reasoning
Inductive argument
Valid deductive argument
Cpsc 457
Cpsc 457
888-457-5851
Tcg retirement
Kj457
Enee457
Cs 457
Artaxerxes decree 445