Opportunities and Challenges for Statisticians in Advanced Pharmaceutical
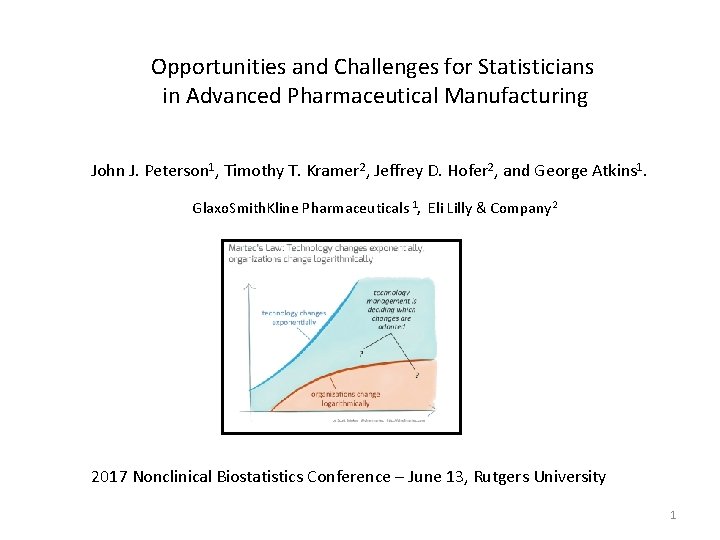
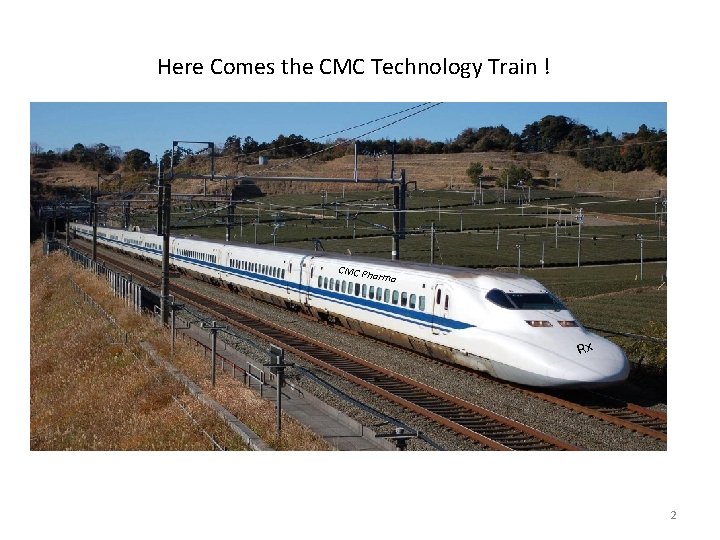
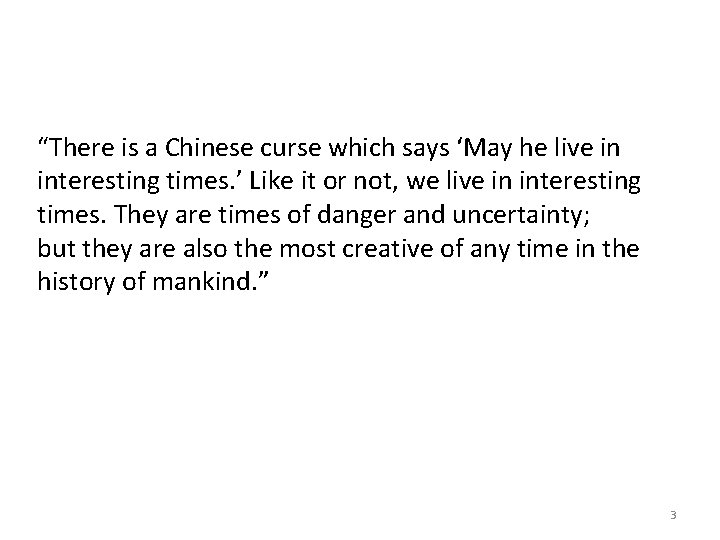
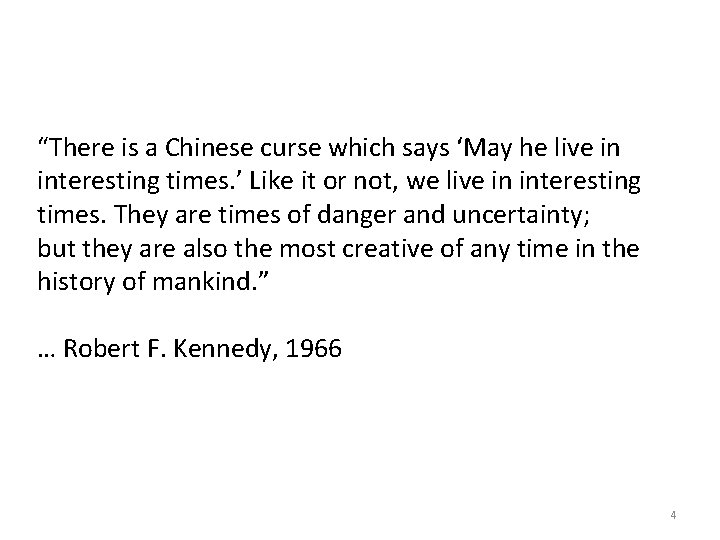
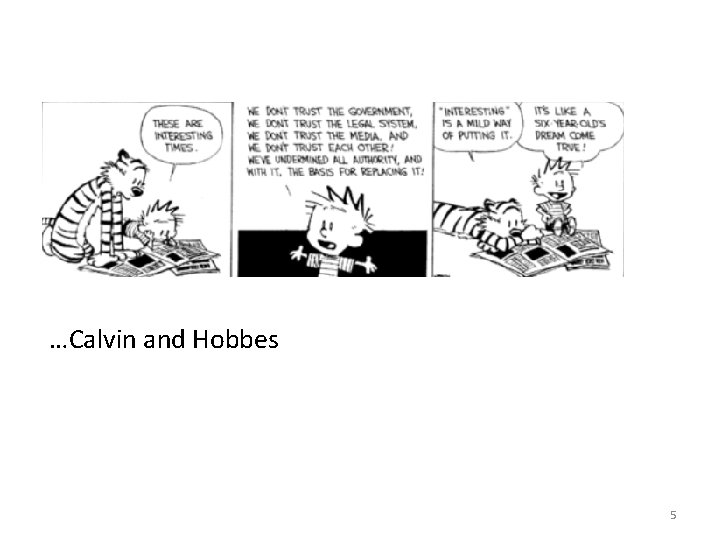
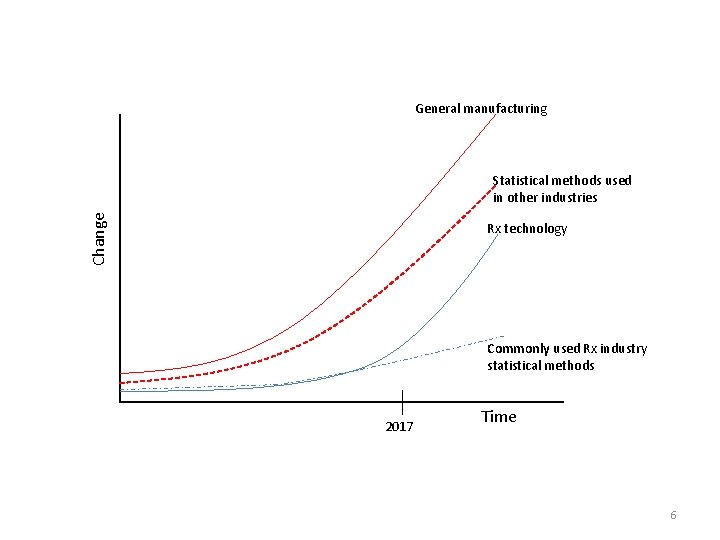
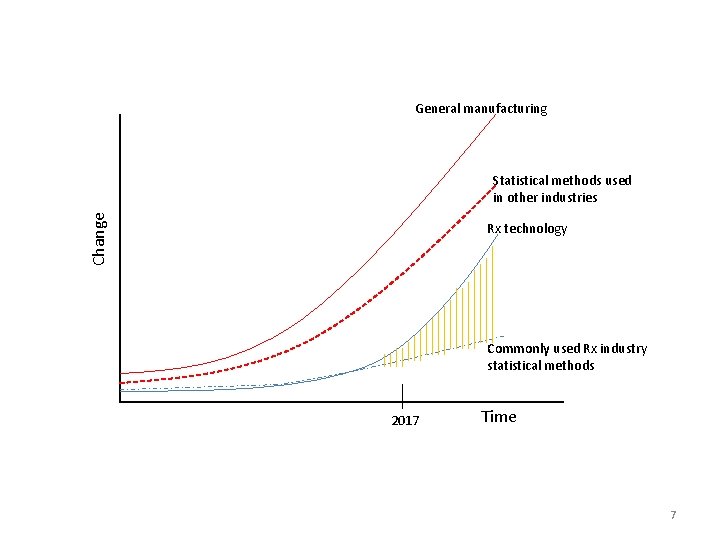
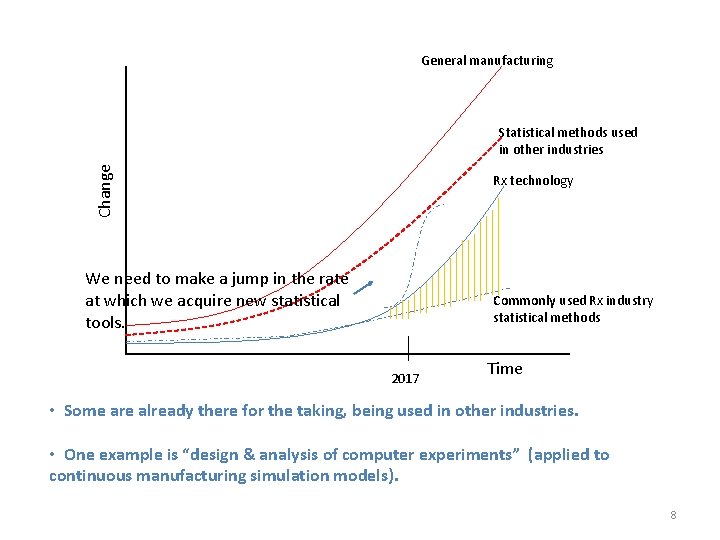
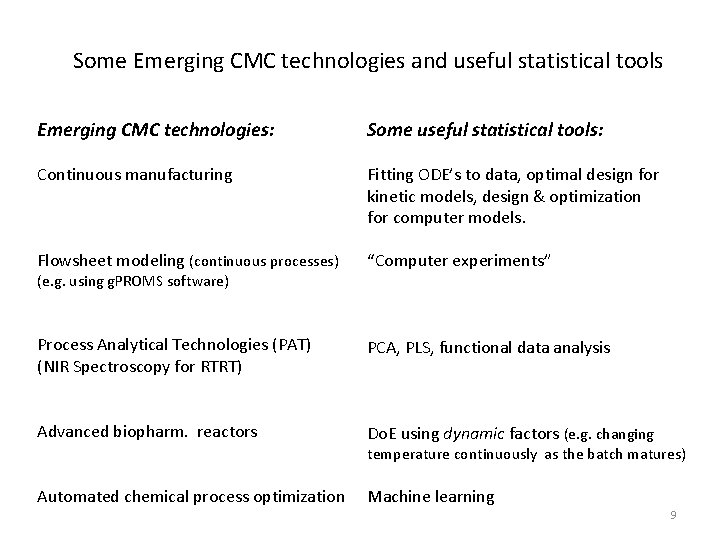
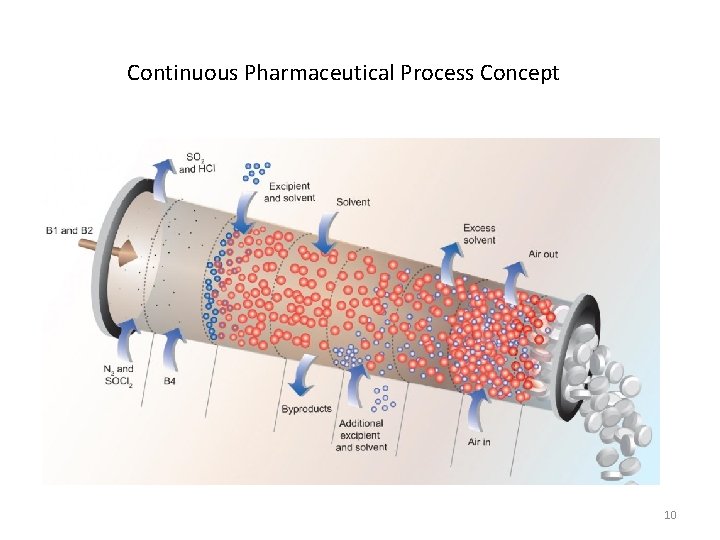
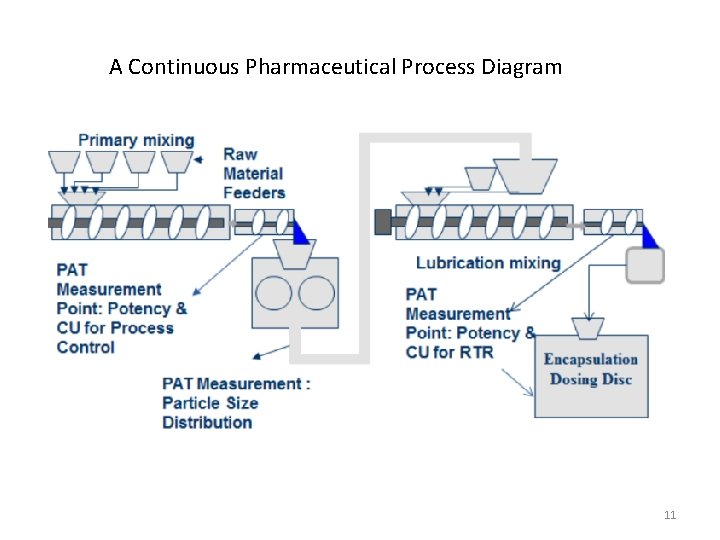
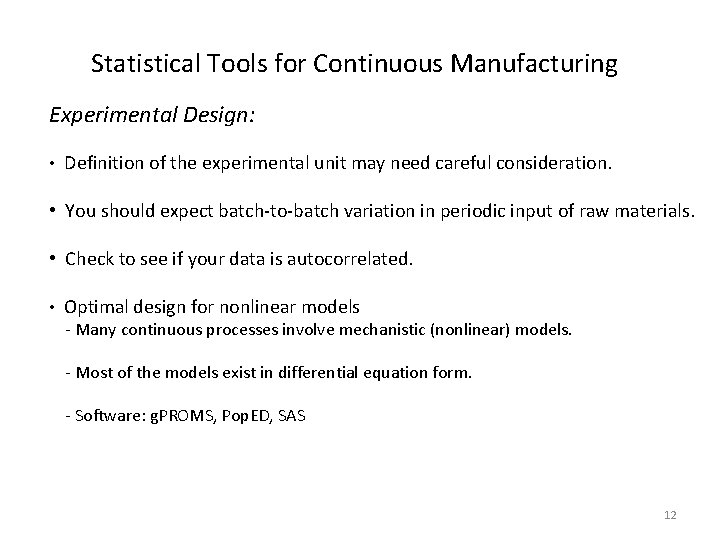
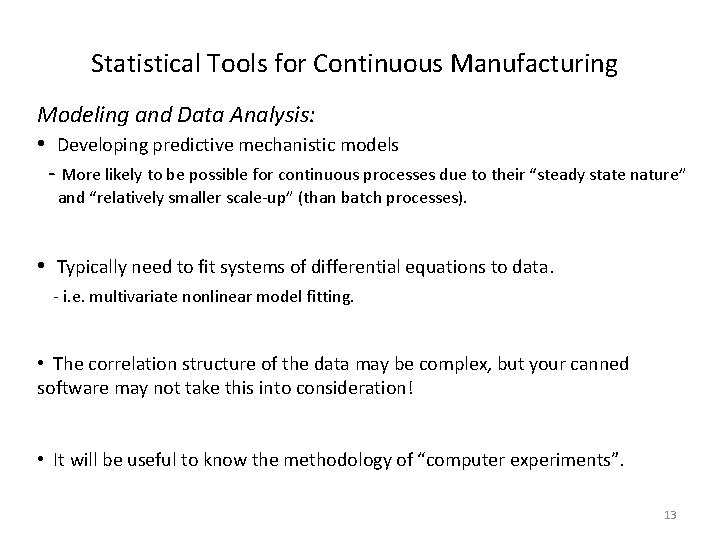
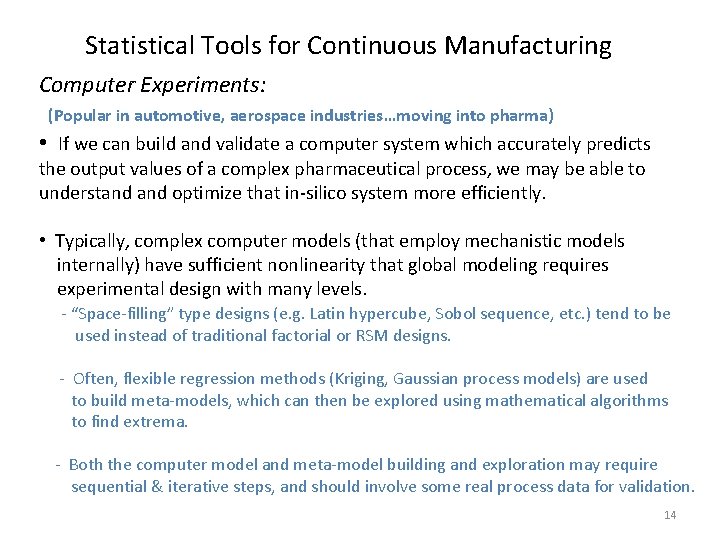
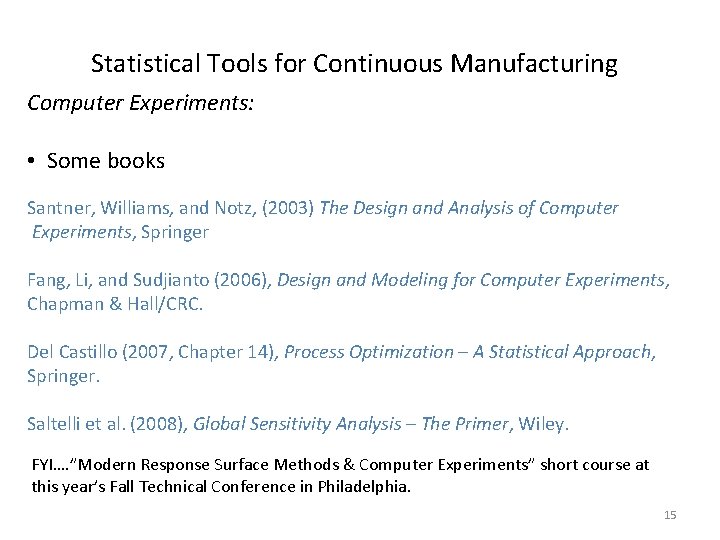
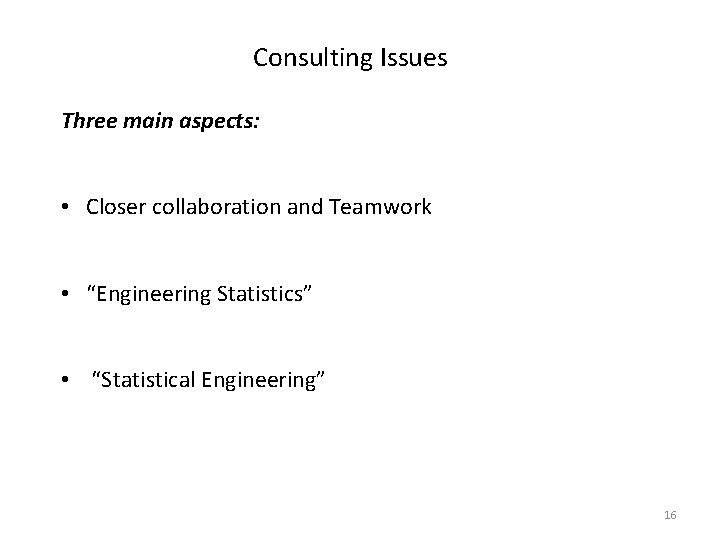
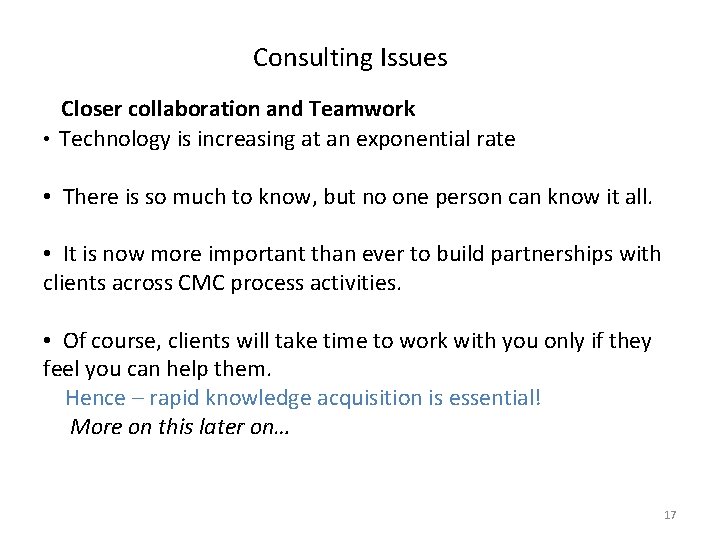
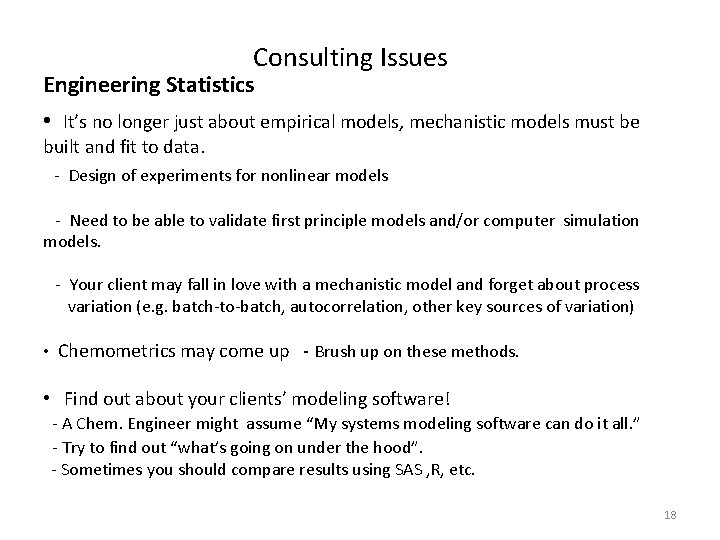
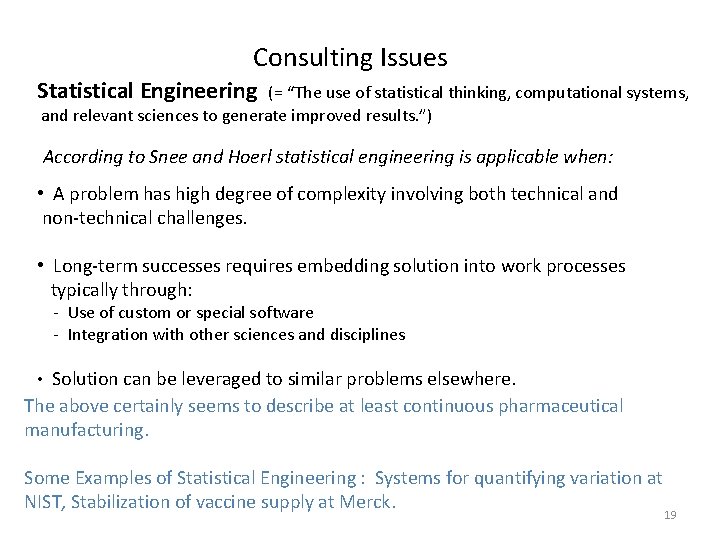
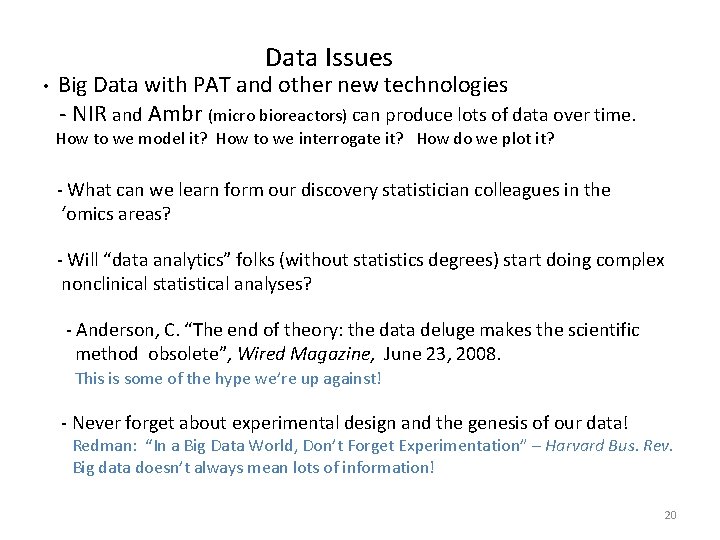
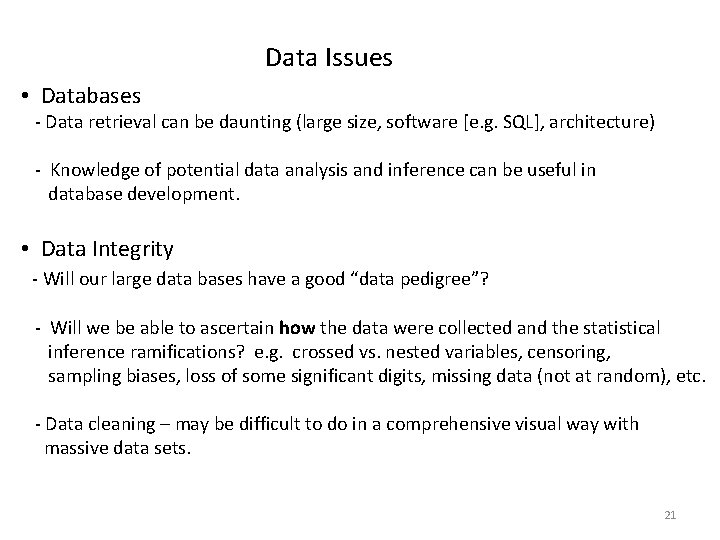
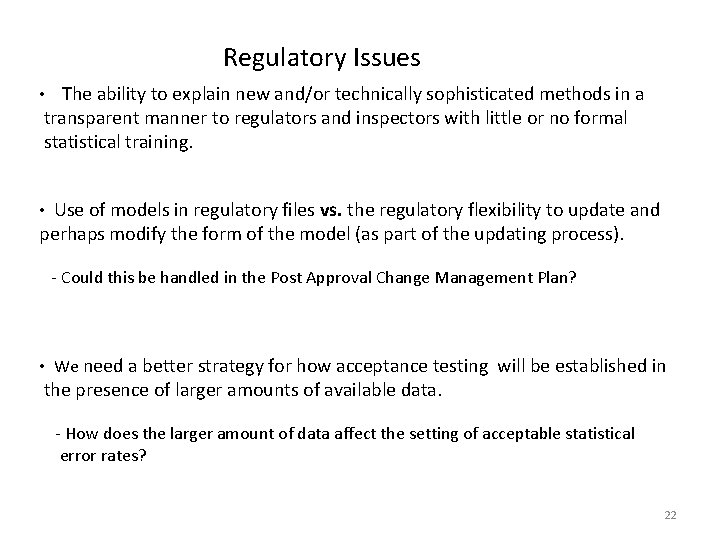
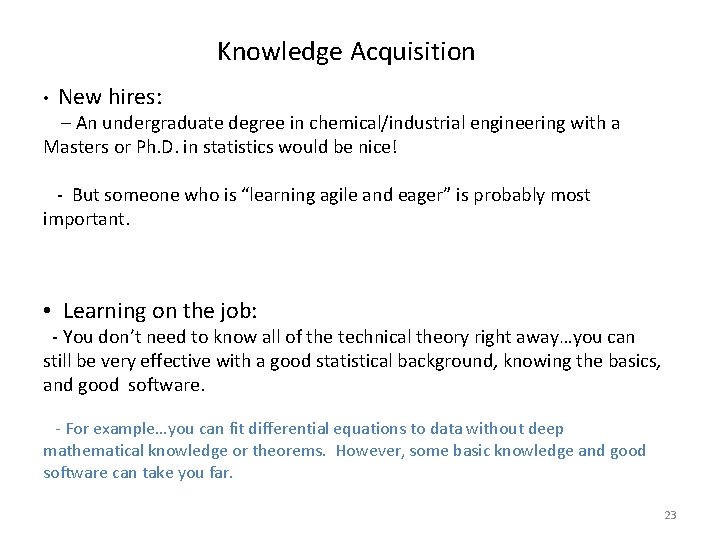
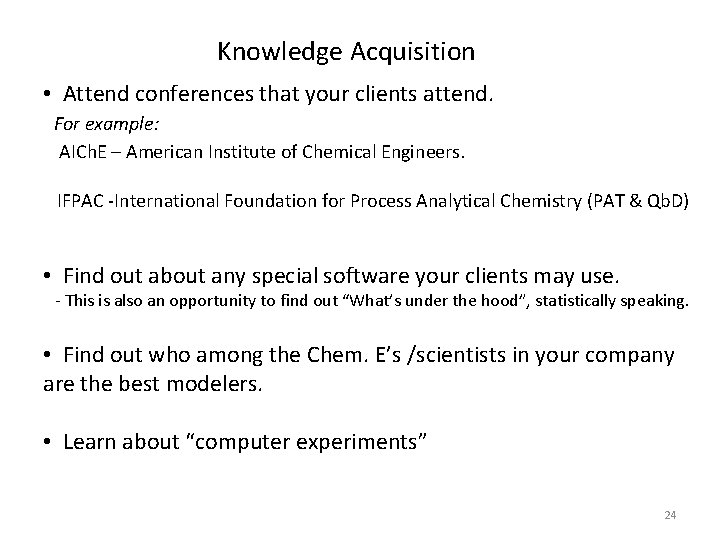
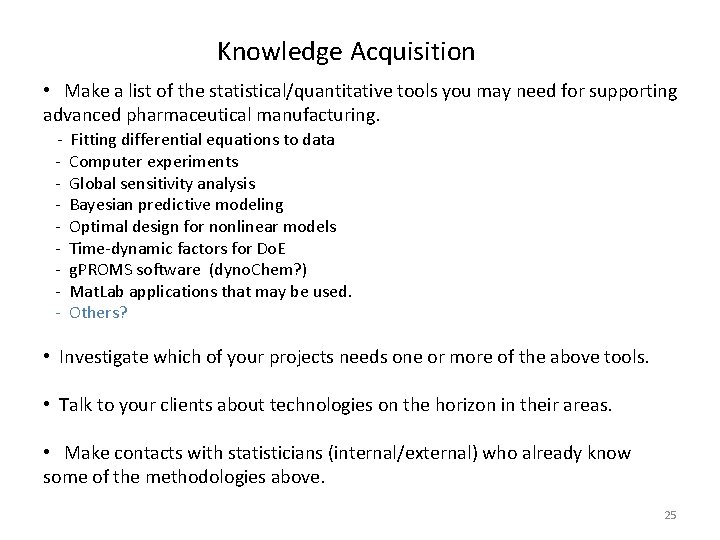
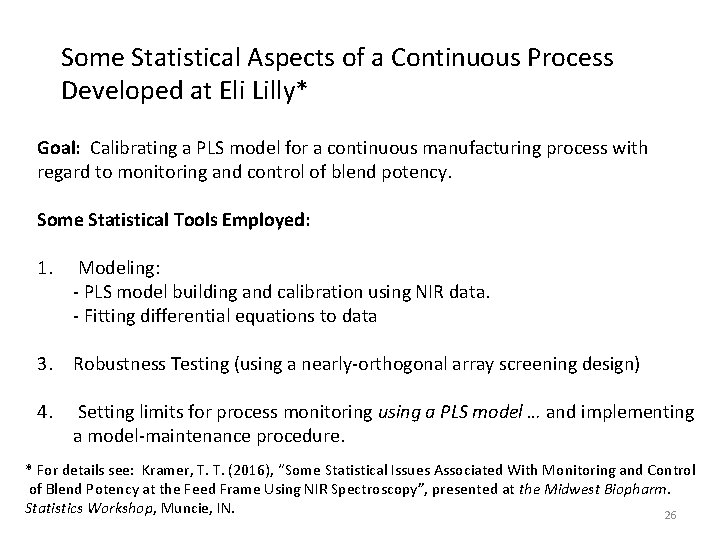
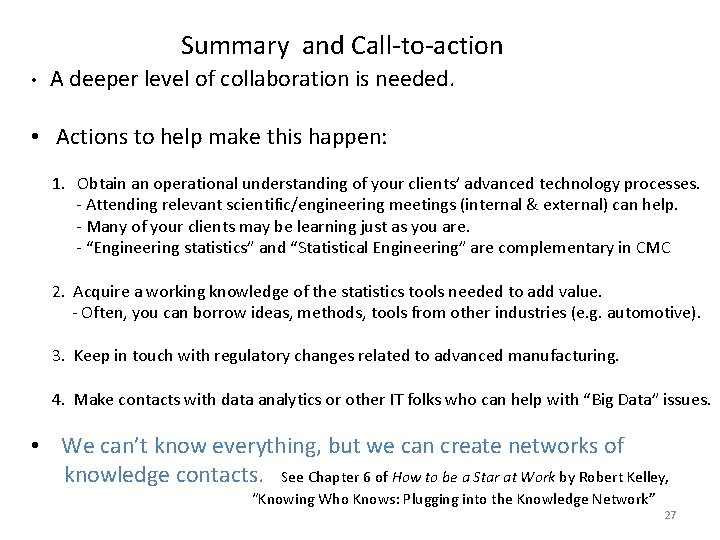
- Slides: 27
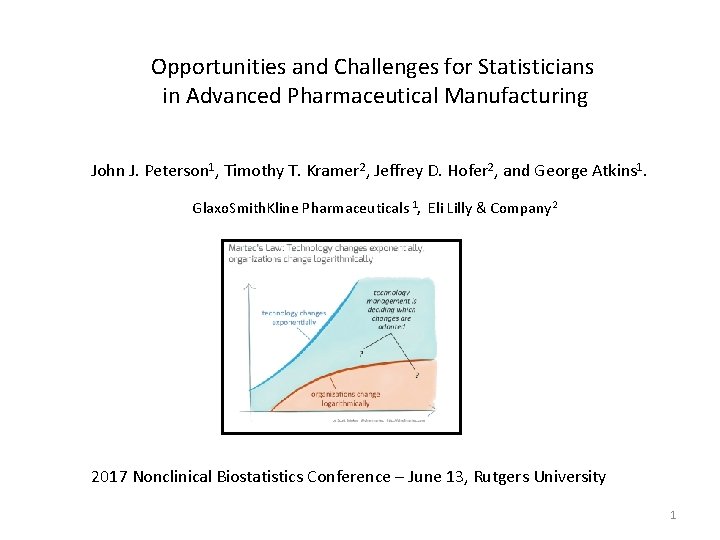
Opportunities and Challenges for Statisticians in Advanced Pharmaceutical Manufacturing John J. Peterson 1, Timothy T. Kramer 2, Jeffrey D. Hofer 2, and George Atkins 1. Glaxo. Smith. Kline Pharmaceuticals 1, Eli Lilly & Company 2 2017 Nonclinical Biostatistics Conference – June 13, Rutgers University 1
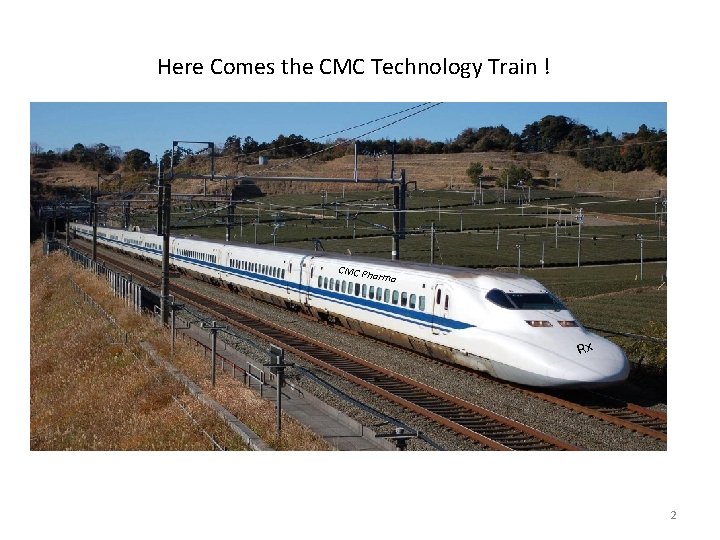
Here Comes the CMC Technology Train ! CMC Ph arma Rx 2
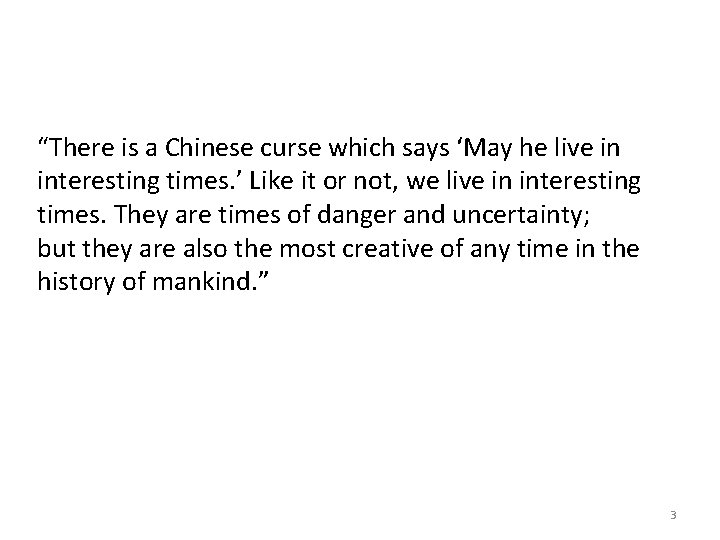
“There is a Chinese curse which says ‘May he live in interesting times. ’ Like it or not, we live in interesting times. They are times of danger and uncertainty; but they are also the most creative of any time in the history of mankind. ” 3
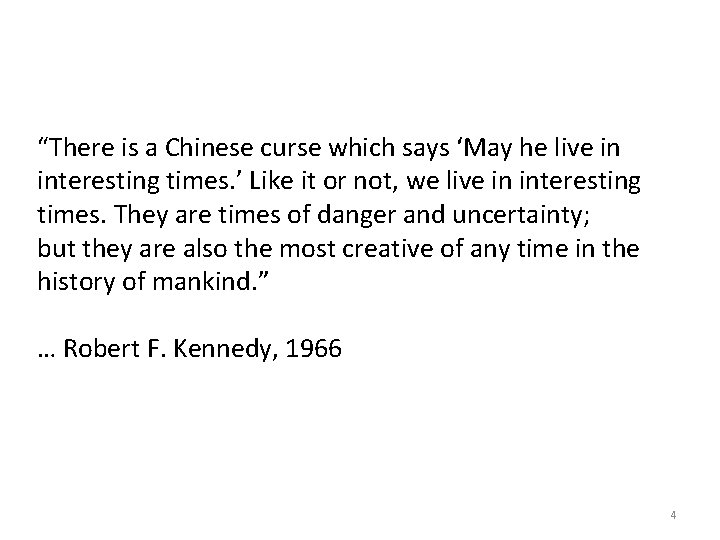
“There is a Chinese curse which says ‘May he live in interesting times. ’ Like it or not, we live in interesting times. They are times of danger and uncertainty; but they are also the most creative of any time in the history of mankind. ” … Robert F. Kennedy, 1966 4
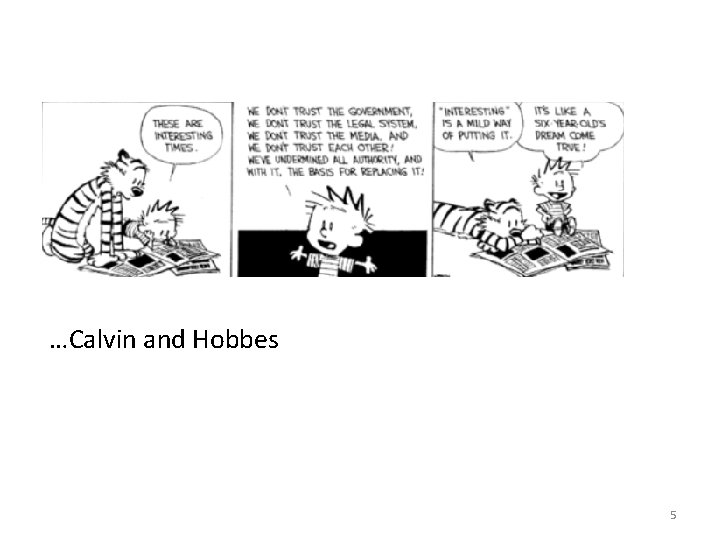
…Calvin and Hobbes 5
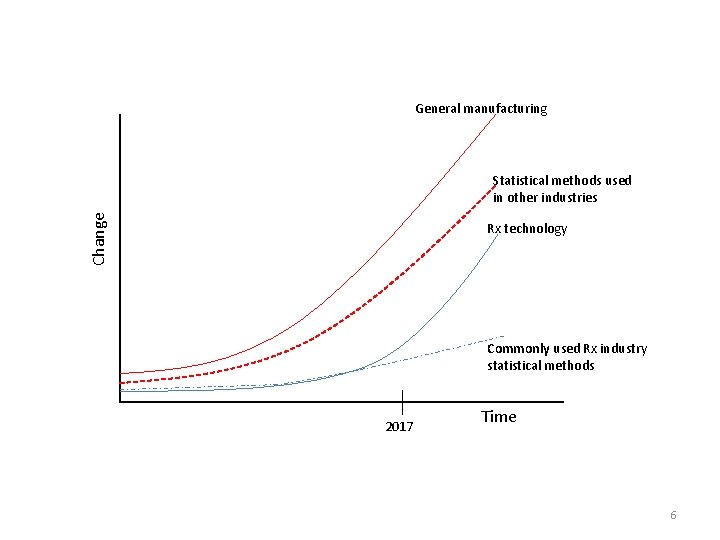
General manufacturing Change Statistical methods used in other industries Rx technology Commonly used Rx industry statistical methods 2017 Time 6
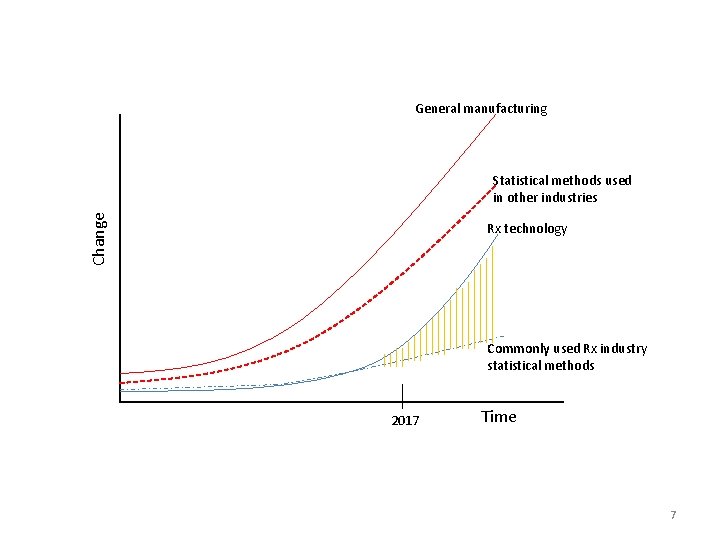
General manufacturing Change Statistical methods used in other industries Rx technology Commonly used Rx industry statistical methods 2017 Time 7
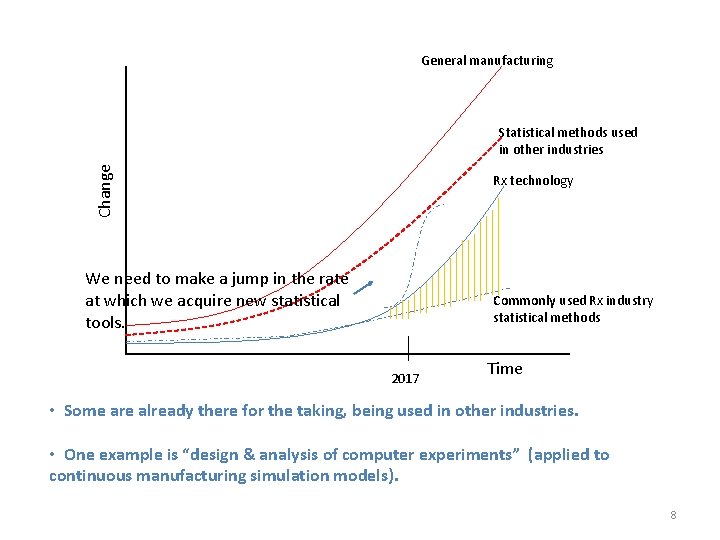
General manufacturing Change Statistical methods used in other industries Rx technology We need to make a jump in the rate at which we acquire new statistical tools. Commonly used Rx industry statistical methods 2017 Time • Some are already there for the taking, being used in other industries. • One example is “design & analysis of computer experiments” (applied to continuous manufacturing simulation models). 8
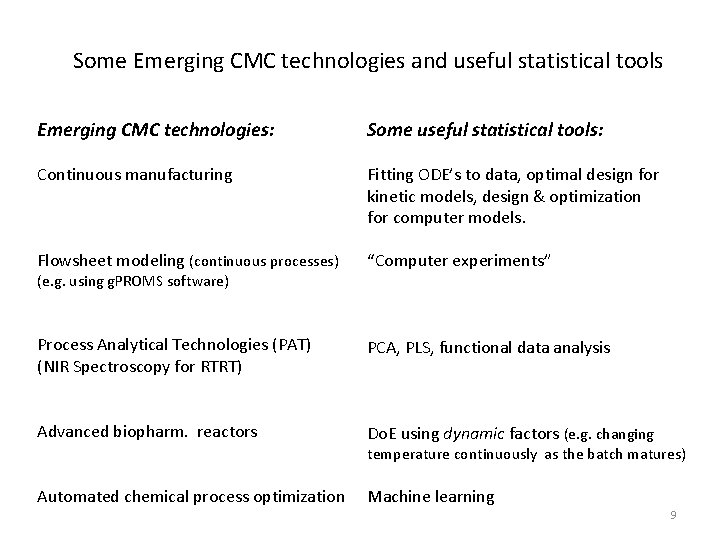
Some Emerging CMC technologies and useful statistical tools Emerging CMC technologies: Some useful statistical tools: Continuous manufacturing Fitting ODE’s to data, optimal design for kinetic models, design & optimization for computer models. Flowsheet modeling (continuous processes) “Computer experiments” Process Analytical Technologies (PAT) (NIR Spectroscopy for RTRT) PCA, PLS, functional data analysis Advanced biopharm. reactors Do. E using dynamic factors (e. g. changing (e. g. using g. PROMS software) temperature continuously as the batch matures) Automated chemical process optimization Machine learning 9
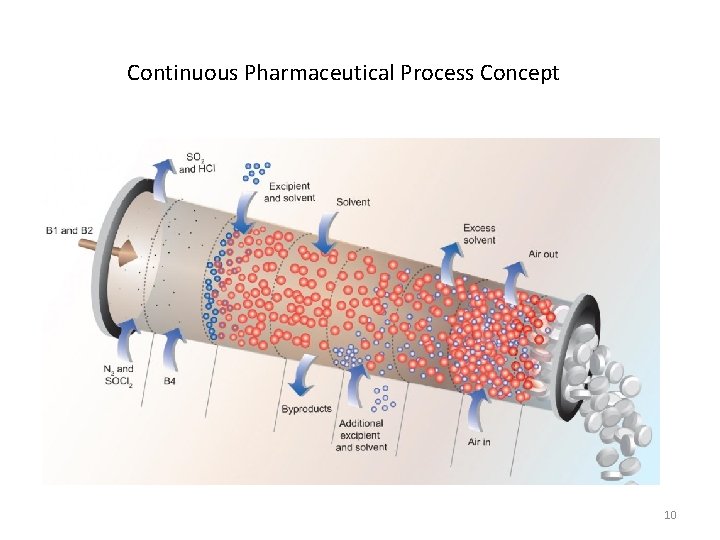
Continuous Pharmaceutical Process Concept 10
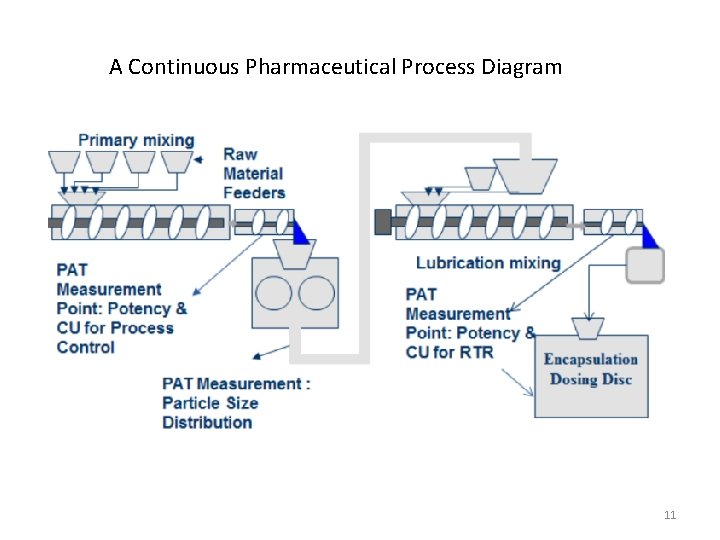
A Continuous Pharmaceutical Process Diagram 11
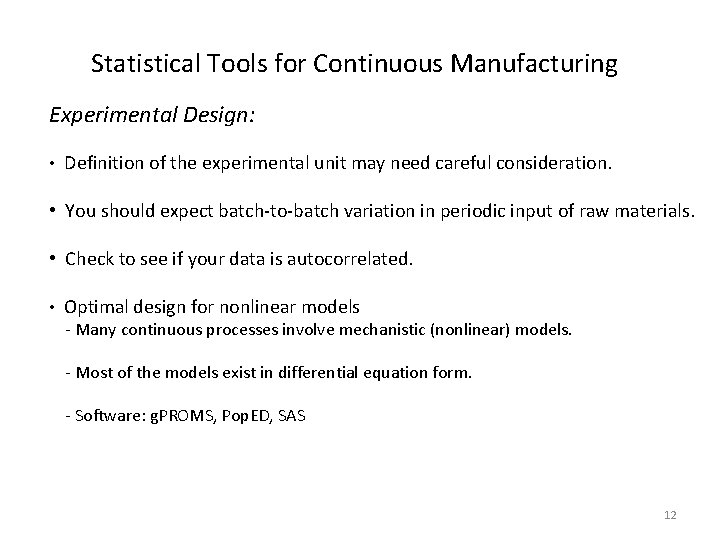
Statistical Tools for Continuous Manufacturing Experimental Design: • Definition of the experimental unit may need careful consideration. • You should expect batch-to-batch variation in periodic input of raw materials. • Check to see if your data is autocorrelated. • Optimal design for nonlinear models - Many continuous processes involve mechanistic (nonlinear) models. - Most of the models exist in differential equation form. - Software: g. PROMS, Pop. ED, SAS 12
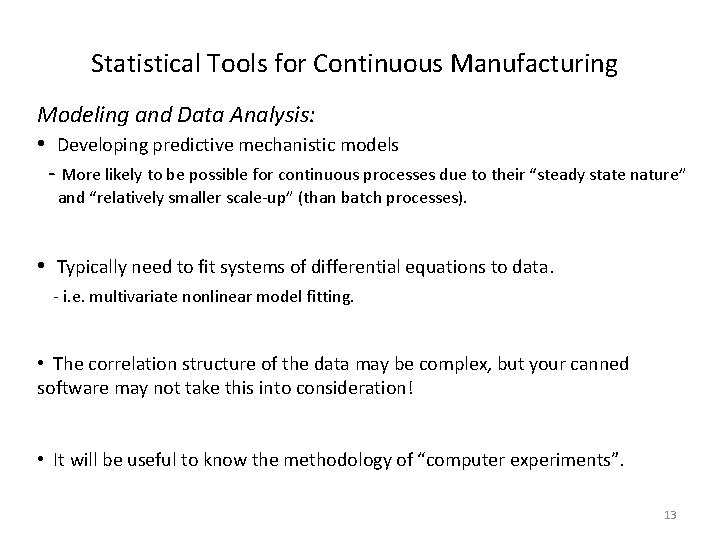
Statistical Tools for Continuous Manufacturing Modeling and Data Analysis: • Developing predictive mechanistic models - More likely to be possible for continuous processes due to their “steady state nature” and “relatively smaller scale-up” (than batch processes). • Typically need to fit systems of differential equations to data. - i. e. multivariate nonlinear model fitting. • The correlation structure of the data may be complex, but your canned software may not take this into consideration! • It will be useful to know the methodology of “computer experiments”. 13
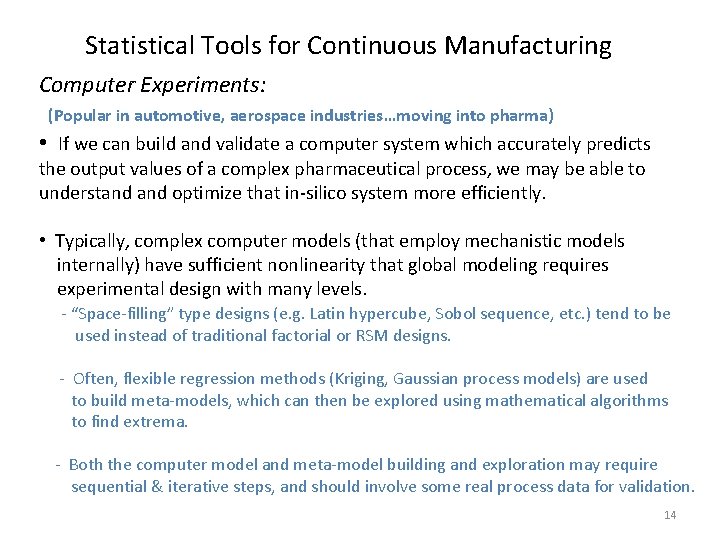
Statistical Tools for Continuous Manufacturing Computer Experiments: (Popular in automotive, aerospace industries…moving into pharma) • If we can build and validate a computer system which accurately predicts the output values of a complex pharmaceutical process, we may be able to understand optimize that in-silico system more efficiently. • Typically, complex computer models (that employ mechanistic models internally) have sufficient nonlinearity that global modeling requires experimental design with many levels. - “Space-filling” type designs (e. g. Latin hypercube, Sobol sequence, etc. ) tend to be used instead of traditional factorial or RSM designs. - Often, flexible regression methods (Kriging, Gaussian process models) are used to build meta-models, which can then be explored using mathematical algorithms to find extrema. - Both the computer model and meta-model building and exploration may require sequential & iterative steps, and should involve some real process data for validation. 14
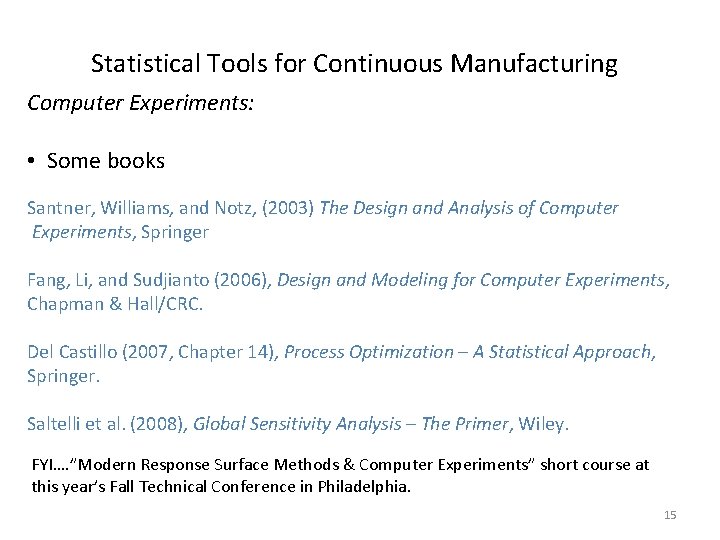
Statistical Tools for Continuous Manufacturing Computer Experiments: • Some books Santner, Williams, and Notz, (2003) The Design and Analysis of Computer Experiments, Springer Fang, Li, and Sudjianto (2006), Design and Modeling for Computer Experiments, Chapman & Hall/CRC. Del Castillo (2007, Chapter 14), Process Optimization – A Statistical Approach, Springer. Saltelli et al. (2008), Global Sensitivity Analysis – The Primer, Wiley. FYI…. ”Modern Response Surface Methods & Computer Experiments” short course at this year’s Fall Technical Conference in Philadelphia. 15
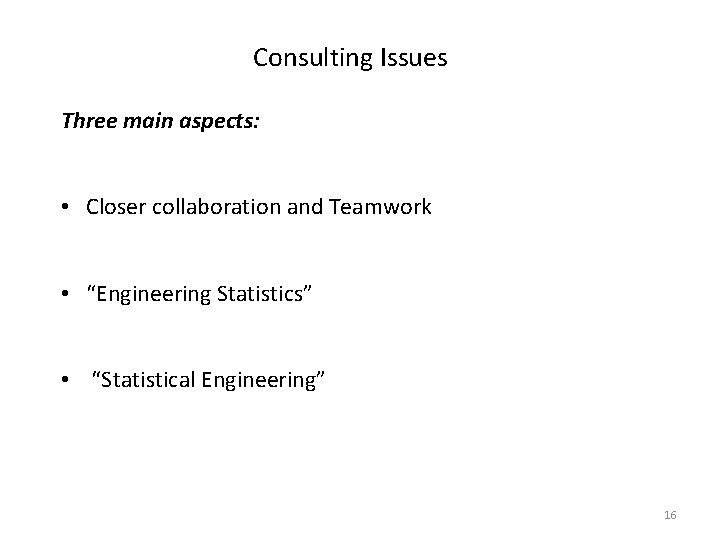
Consulting Issues Three main aspects: • Closer collaboration and Teamwork • “Engineering Statistics” • “Statistical Engineering” 16
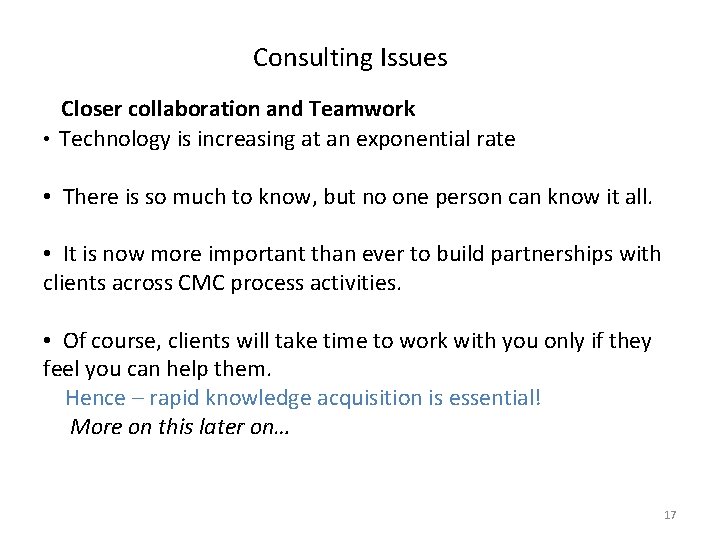
Consulting Issues Closer collaboration and Teamwork • Technology is increasing at an exponential rate • There is so much to know, but no one person can know it all. • It is now more important than ever to build partnerships with clients across CMC process activities. • Of course, clients will take time to work with you only if they feel you can help them. Hence – rapid knowledge acquisition is essential! More on this later on… 17
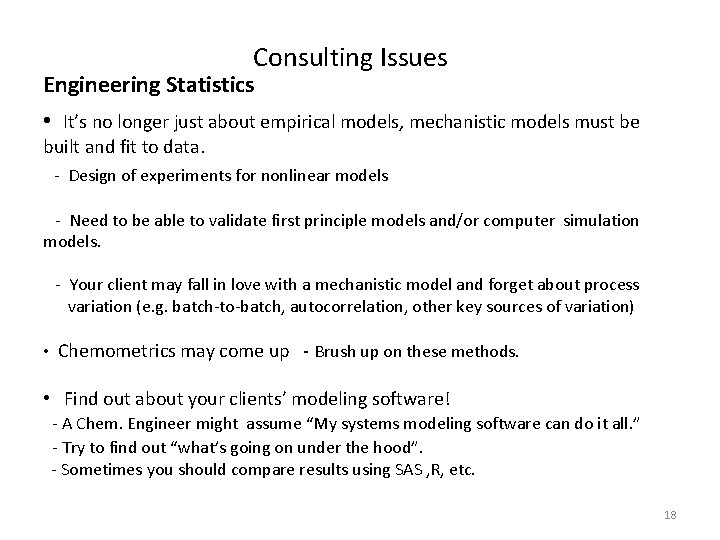
Consulting Issues Engineering Statistics • It’s no longer just about empirical models, mechanistic models must be built and fit to data. - Design of experiments for nonlinear models - Need to be able to validate first principle models and/or computer simulation models. - Your client may fall in love with a mechanistic model and forget about process variation (e. g. batch-to-batch, autocorrelation, other key sources of variation) • Chemometrics may come up - Brush up on these methods. • Find out about your clients’ modeling software! - A Chem. Engineer might assume “My systems modeling software can do it all. ” - Try to find out “what’s going on under the hood”. - Sometimes you should compare results using SAS , R, etc. 18
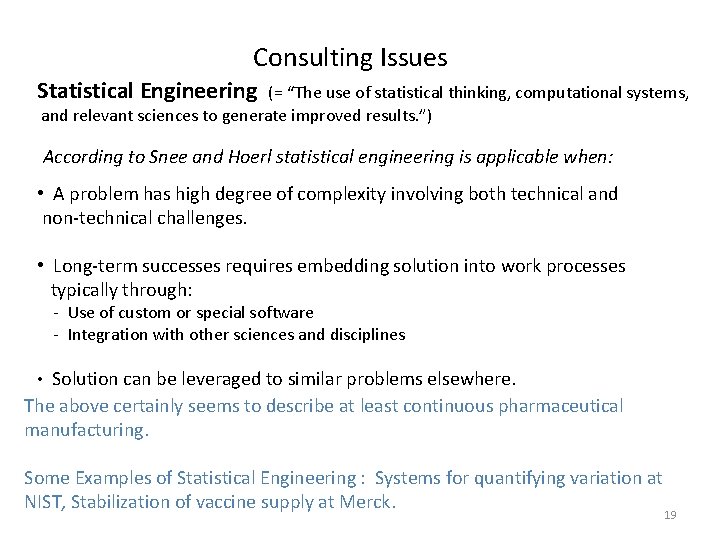
Consulting Issues Statistical Engineering (= “The use of statistical thinking, computational systems, and relevant sciences to generate improved results. ”) According to Snee and Hoerl statistical engineering is applicable when: • A problem has high degree of complexity involving both technical and non-technical challenges. • Long-term successes requires embedding solution into work processes typically through: - Use of custom or special software - Integration with other sciences and disciplines • Solution can be leveraged to similar problems elsewhere. The above certainly seems to describe at least continuous pharmaceutical manufacturing. Some Examples of Statistical Engineering : Systems for quantifying variation at NIST, Stabilization of vaccine supply at Merck. 19
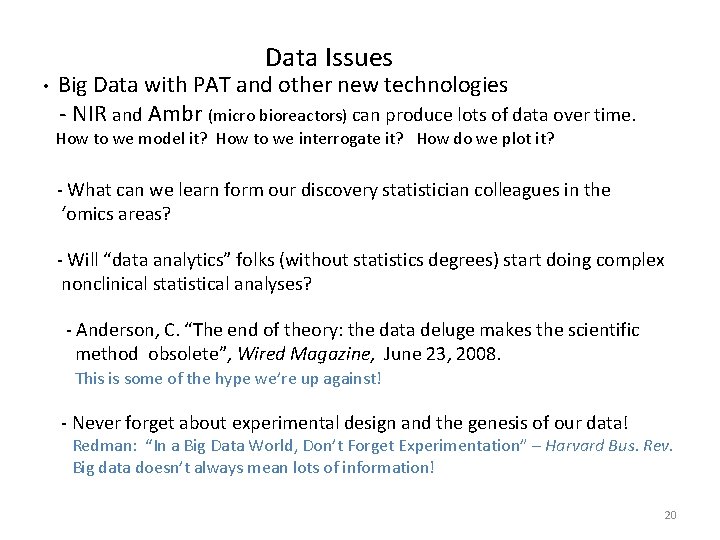
Data Issues • Big Data with PAT and other new technologies - NIR and Ambr (micro bioreactors) can produce lots of data over time. How to we model it? How to we interrogate it? How do we plot it? - What can we learn form our discovery statistician colleagues in the ‘omics areas? - Will “data analytics” folks (without statistics degrees) start doing complex nonclinical statistical analyses? - Anderson, C. “The end of theory: the data deluge makes the scientific method obsolete”, Wired Magazine, June 23, 2008. This is some of the hype we’re up against! - Never forget about experimental design and the genesis of our data! Redman: “In a Big Data World, Don’t Forget Experimentation” – Harvard Bus. Rev. Big data doesn’t always mean lots of information! 20
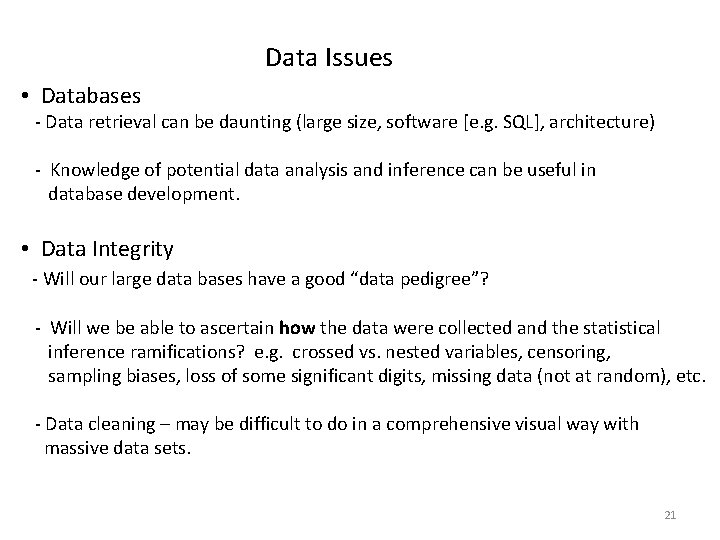
Data Issues • Databases - Data retrieval can be daunting (large size, software [e. g. SQL], architecture) - Knowledge of potential data analysis and inference can be useful in database development. • Data Integrity - Will our large data bases have a good “data pedigree”? - Will we be able to ascertain how the data were collected and the statistical inference ramifications? e. g. crossed vs. nested variables, censoring, sampling biases, loss of some significant digits, missing data (not at random), etc. - Data cleaning – may be difficult to do in a comprehensive visual way with massive data sets. 21
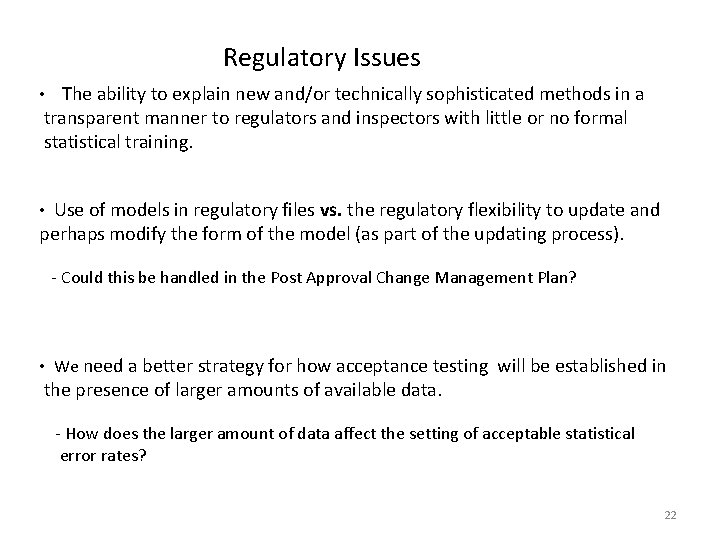
Regulatory Issues • The ability to explain new and/or technically sophisticated methods in a transparent manner to regulators and inspectors with little or no formal statistical training. • Use of models in regulatory files vs. the regulatory flexibility to update and perhaps modify the form of the model (as part of the updating process). - Could this be handled in the Post Approval Change Management Plan? • We need a better strategy for how acceptance testing will be established in the presence of larger amounts of available data. - How does the larger amount of data affect the setting of acceptable statistical error rates? 22
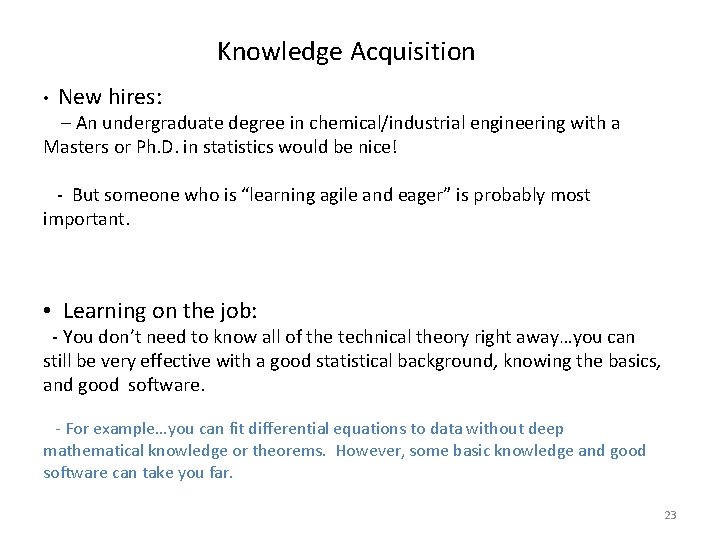
Knowledge Acquisition • New hires: – An undergraduate degree in chemical/industrial engineering with a Masters or Ph. D. in statistics would be nice! - But someone who is “learning agile and eager” is probably most important. • Learning on the job: - You don’t need to know all of the technical theory right away…you can still be very effective with a good statistical background, knowing the basics, and good software. - For example…you can fit differential equations to data without deep mathematical knowledge or theorems. However, some basic knowledge and good software can take you far. 23
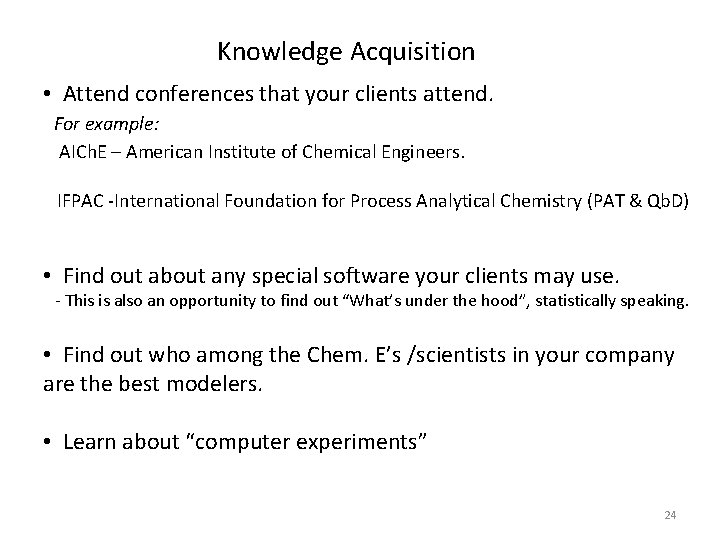
Knowledge Acquisition • Attend conferences that your clients attend. For example: AICh. E – American Institute of Chemical Engineers. IFPAC -International Foundation for Process Analytical Chemistry (PAT & Qb. D) • Find out about any special software your clients may use. - This is also an opportunity to find out “What’s under the hood”, statistically speaking. • Find out who among the Chem. E’s /scientists in your company are the best modelers. • Learn about “computer experiments” 24
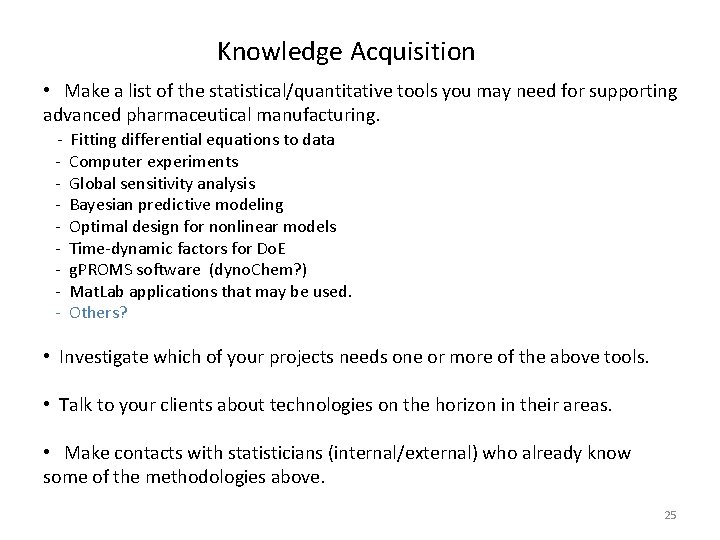
Knowledge Acquisition • Make a list of the statistical/quantitative tools you may need for supporting advanced pharmaceutical manufacturing. - Fitting differential equations to data Computer experiments Global sensitivity analysis Bayesian predictive modeling Optimal design for nonlinear models Time-dynamic factors for Do. E g. PROMS software (dyno. Chem? ) Mat. Lab applications that may be used. Others? • Investigate which of your projects needs one or more of the above tools. • Talk to your clients about technologies on the horizon in their areas. • Make contacts with statisticians (internal/external) who already know some of the methodologies above. 25
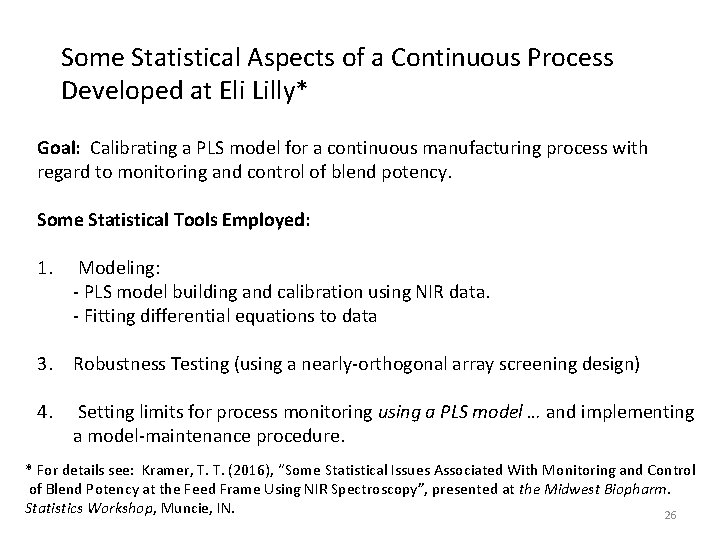
Some Statistical Aspects of a Continuous Process Developed at Eli Lilly* Goal: Calibrating a PLS model for a continuous manufacturing process with regard to monitoring and control of blend potency. Some Statistical Tools Employed: 1. Modeling: - PLS model building and calibration using NIR data. - Fitting differential equations to data 3. Robustness Testing (using a nearly-orthogonal array screening design) 4. Setting limits for process monitoring using a PLS model … and implementing a model-maintenance procedure. * For details see: Kramer, T. T. (2016), “Some Statistical Issues Associated With Monitoring and Control of Blend Potency at the Feed Frame Using NIR Spectroscopy”, presented at the Midwest Biopharm. Statistics Workshop, Muncie, IN. 26
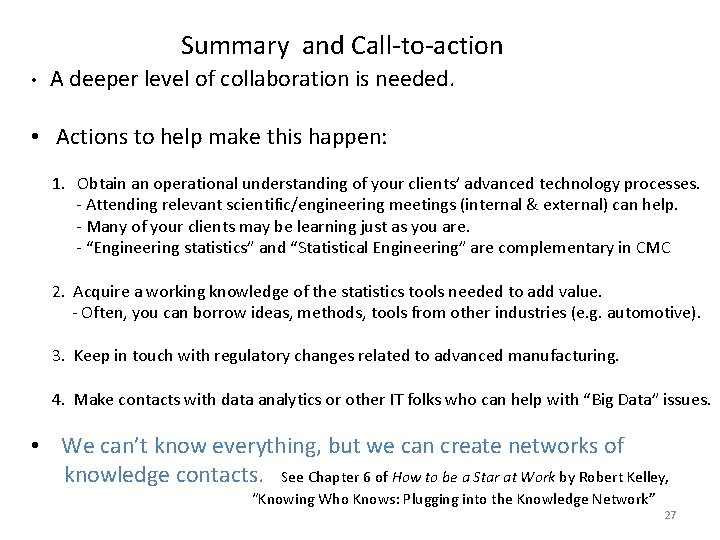
Summary and Call-to-action • A deeper level of collaboration is needed. • Actions to help make this happen: 1. Obtain an operational understanding of your clients’ advanced technology processes. - Attending relevant scientific/engineering meetings (internal & external) can help. - Many of your clients may be learning just as you are. - “Engineering statistics” and “Statistical Engineering” are complementary in CMC 2. Acquire a working knowledge of the statistics tools needed to add value. - Often, you can borrow ideas, methods, tools from other industries (e. g. automotive). 3. Keep in touch with regulatory changes related to advanced manufacturing. 4. Make contacts with data analytics or other IT folks who can help with “Big Data” issues. • We can’t know everything, but we can create networks of knowledge contacts. See Chapter 6 of How to be a Star at Work by Robert Kelley, “Knowing Who Knows: Plugging into the Knowledge Network” 27
Statistics and ethics: some advice for young statisticians
The pew research center asked a random sample of 2024
Conference of european statisticians
Opportunities of media and information in political
Greater bay area opportunities and challenges
Forensic pharmacist
Kontinuitetshantering i praktiken
Typiska drag för en novell
Nationell inriktning för artificiell intelligens
Ekologiskt fotavtryck
Varför kallas perioden 1918-1939 för mellankrigstiden?
En lathund för arbete med kontinuitetshantering
Särskild löneskatt för pensionskostnader
Tidbok
Sura för anatom
Vad är densitet
Datorkunskap för nybörjare
Boverket ka
Att skriva debattartikel
Delegerande ledarstil
Nyckelkompetenser för livslångt lärande
Påbyggnader för flakfordon
Kraft per area
Publik sektor
Lyckans minut erik lindorm analys
Presentera för publik crossboss
Vad är ett minoritetsspråk
Plats för toran ark