Numerical Weather Prediction NWP Usage Strengths Limitations Strategies
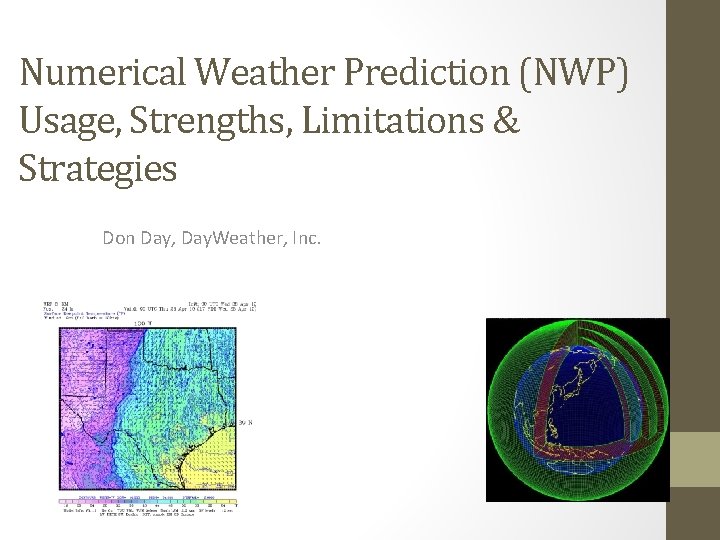
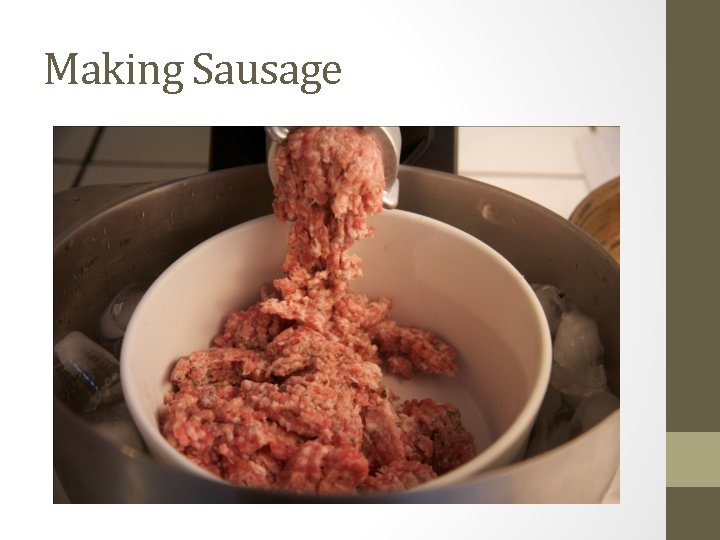
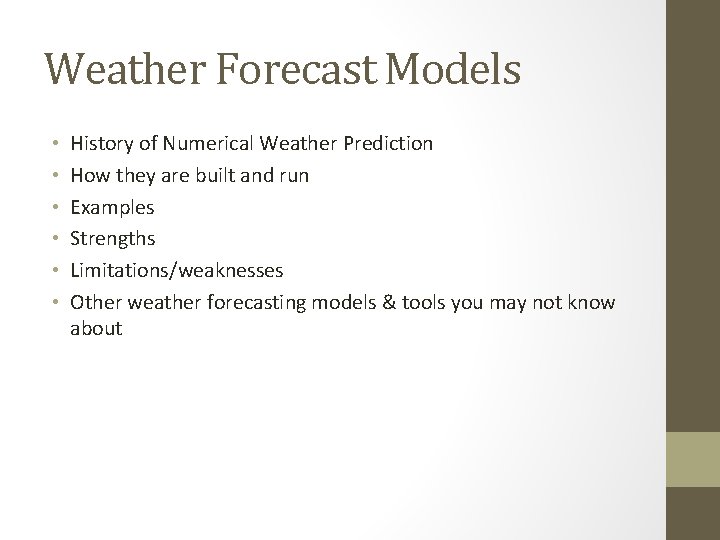
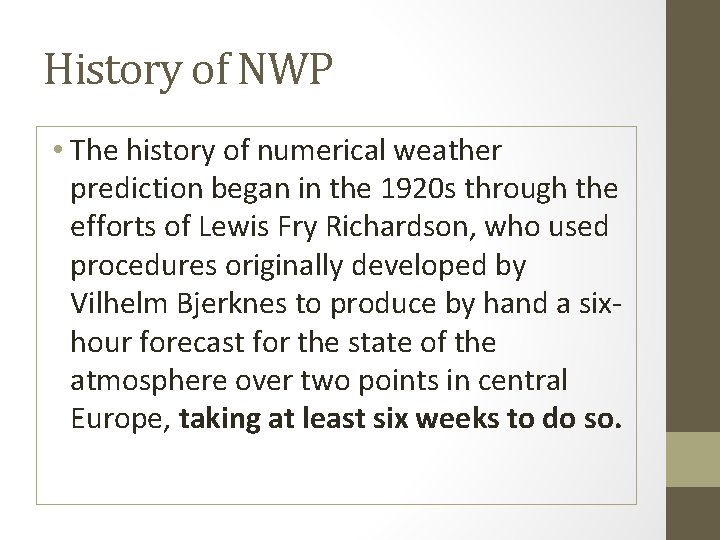
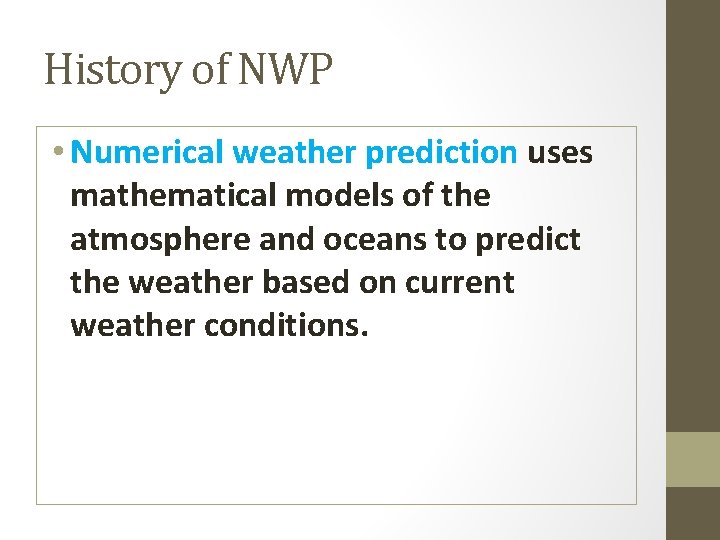
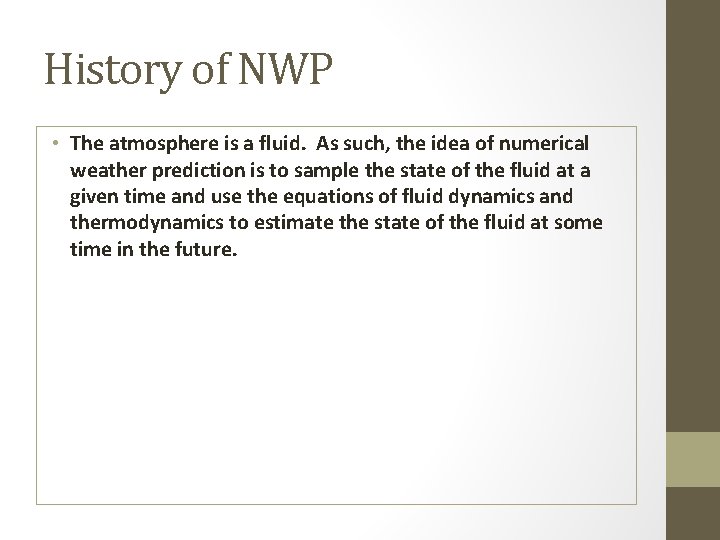
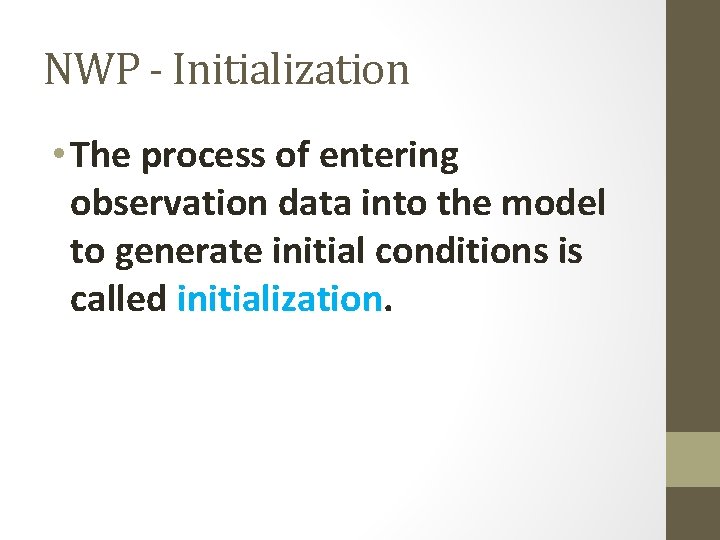
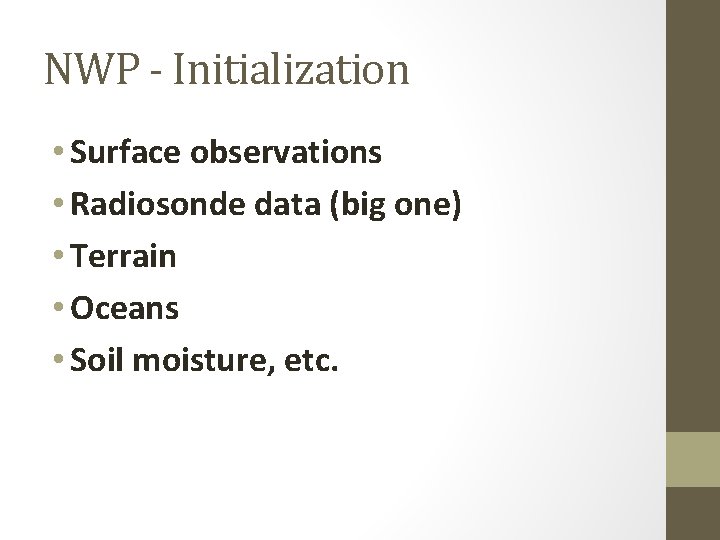
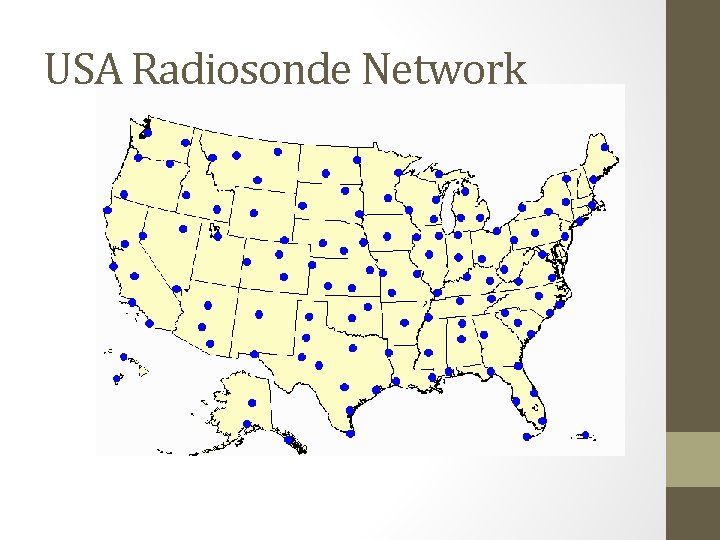
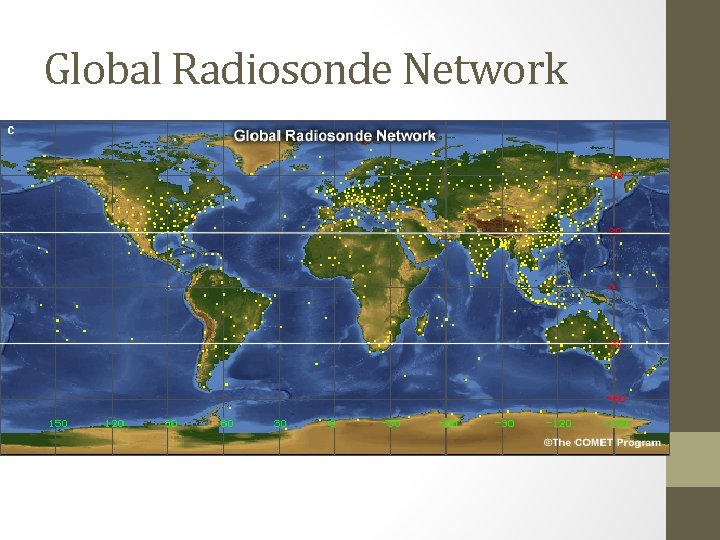
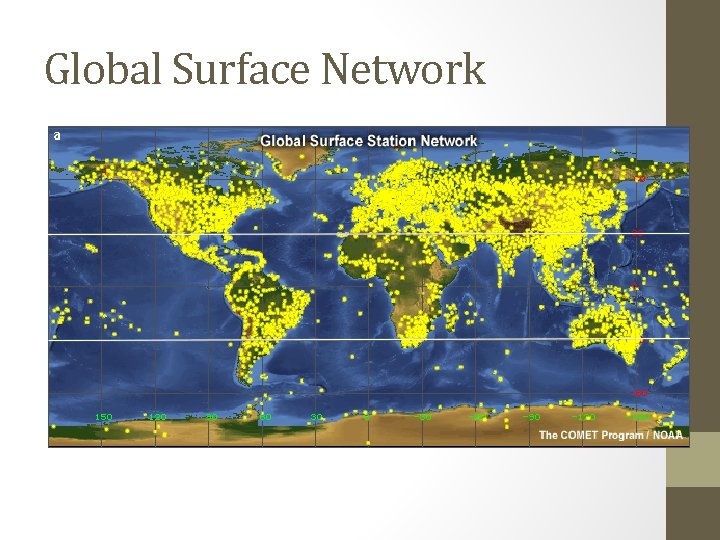
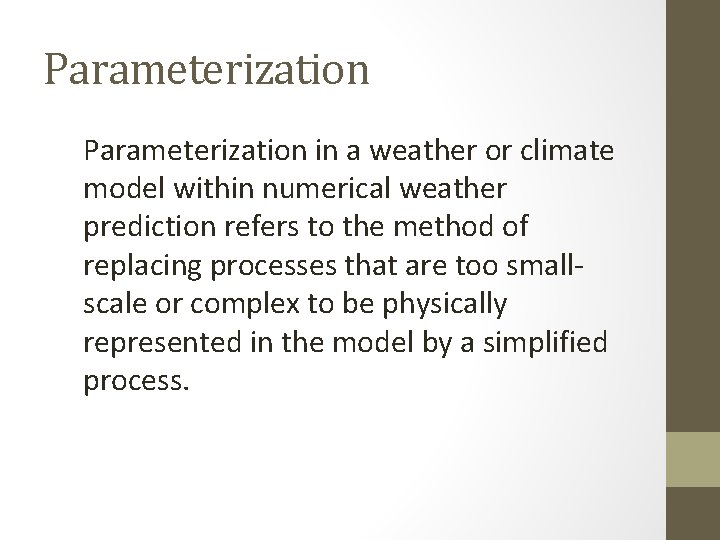
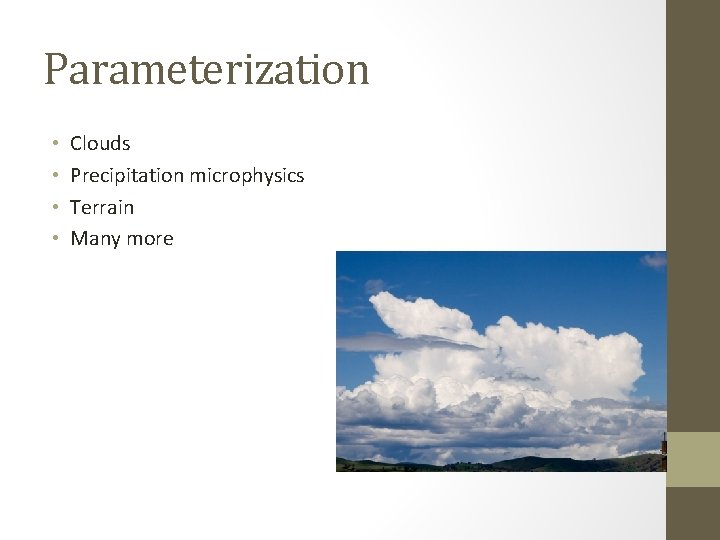
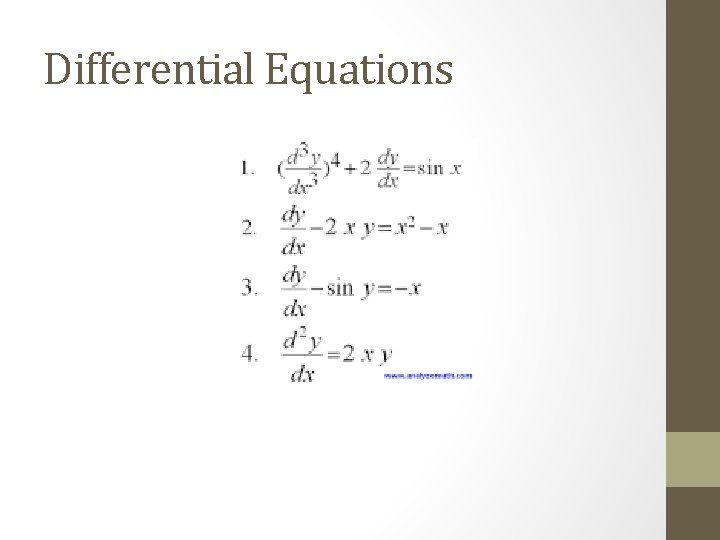
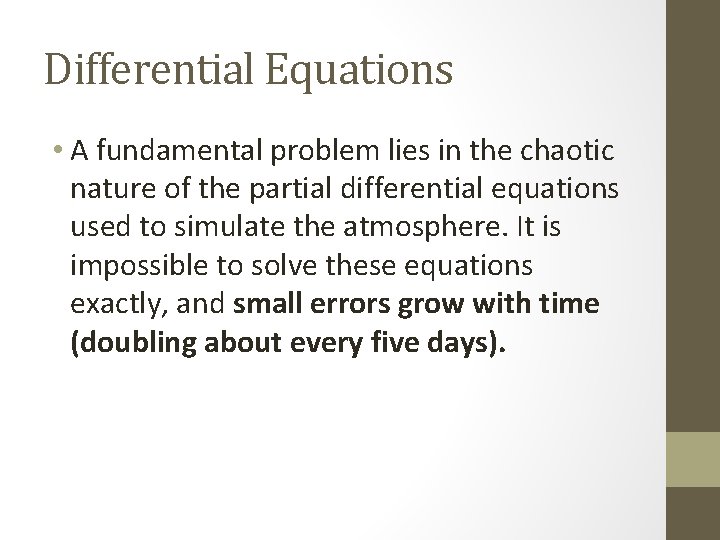
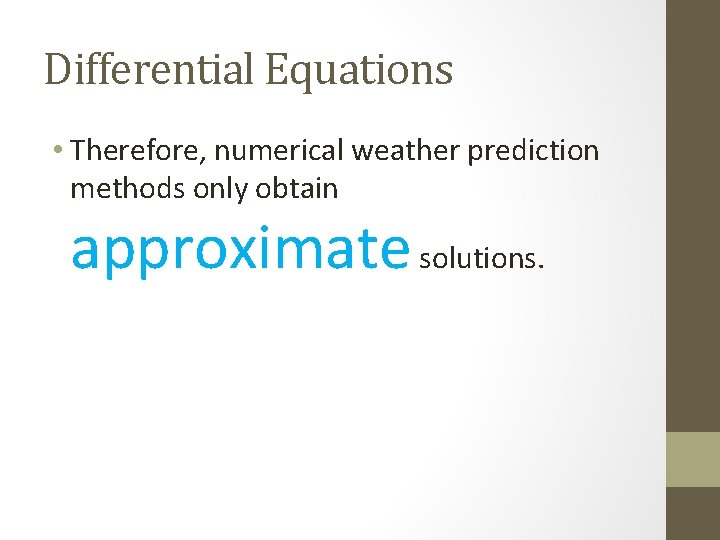
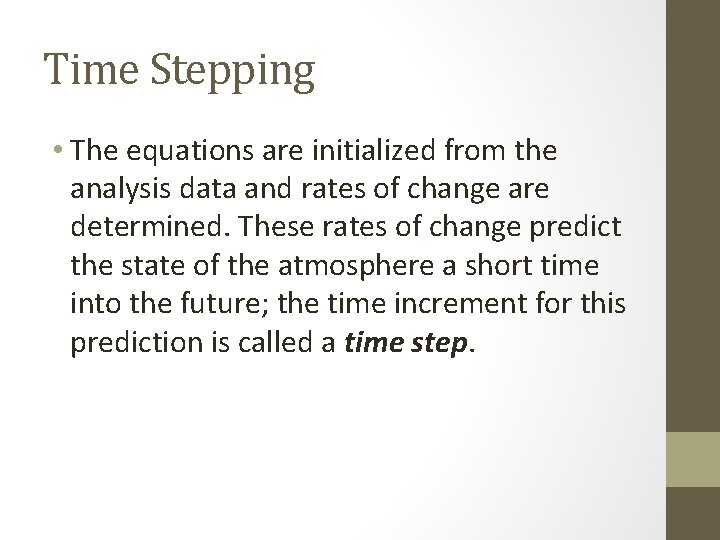
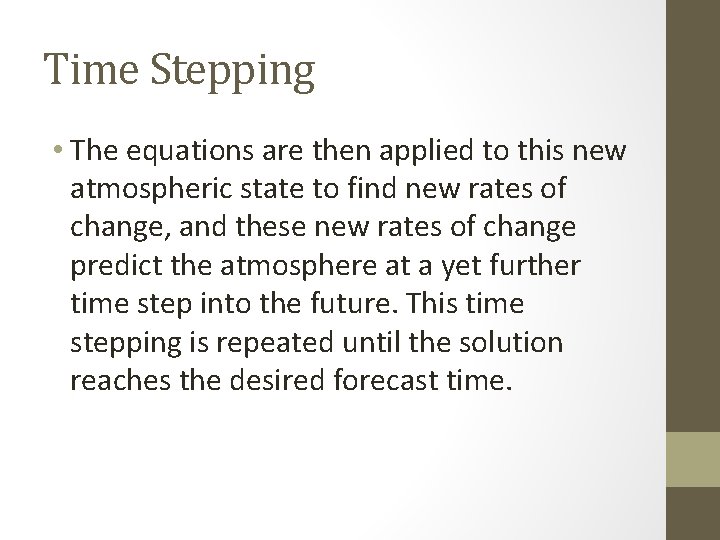
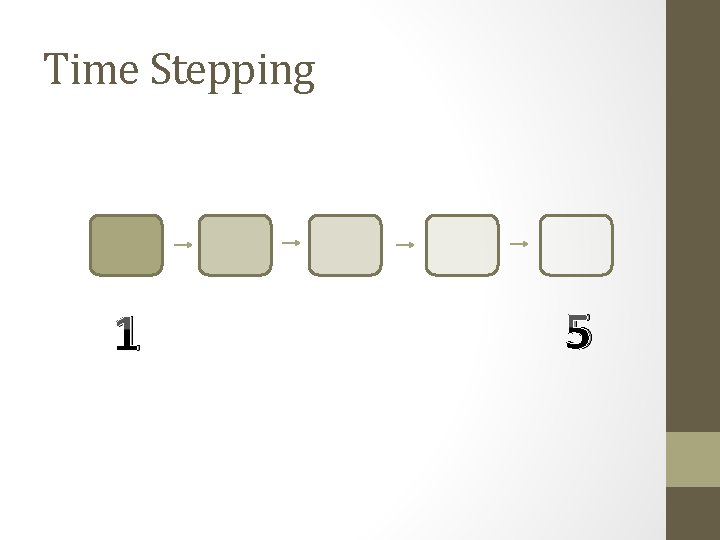
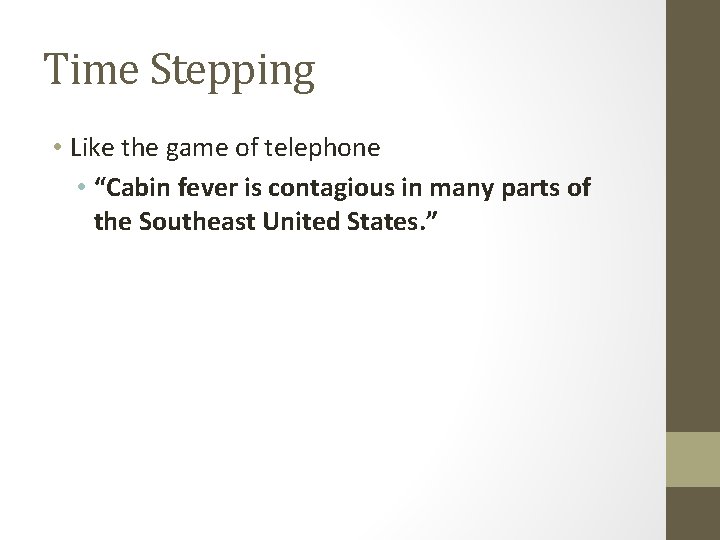
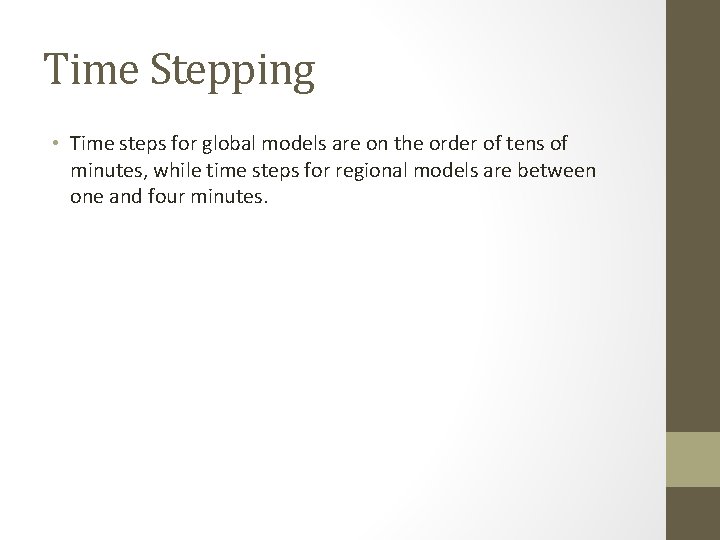
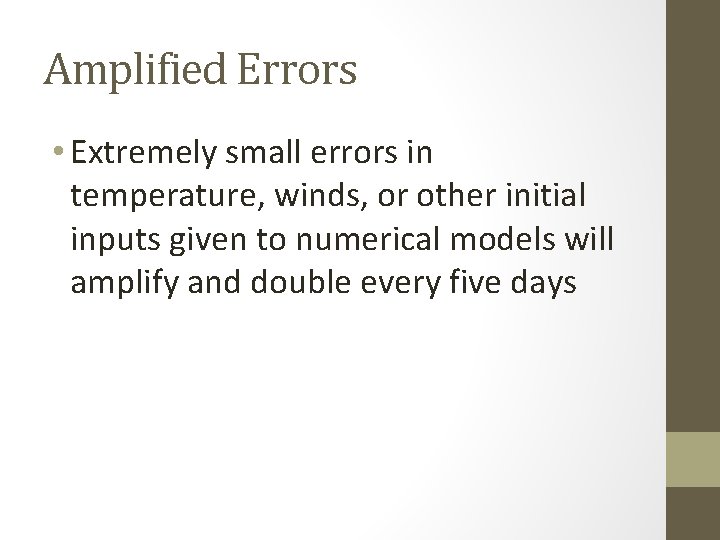
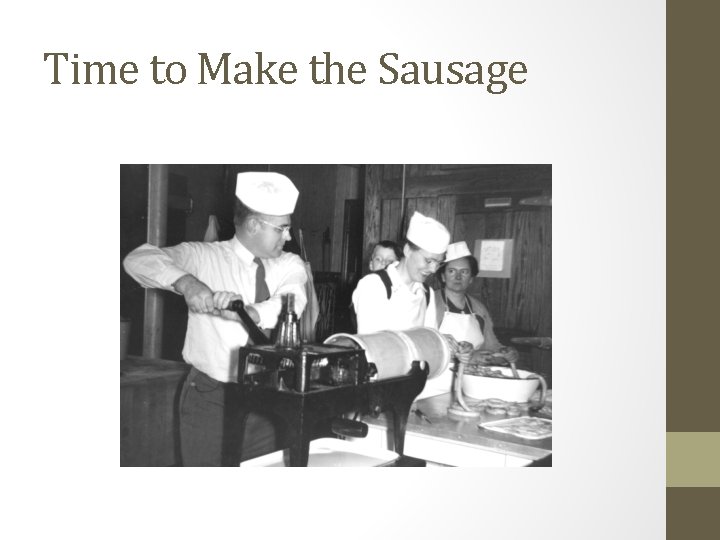
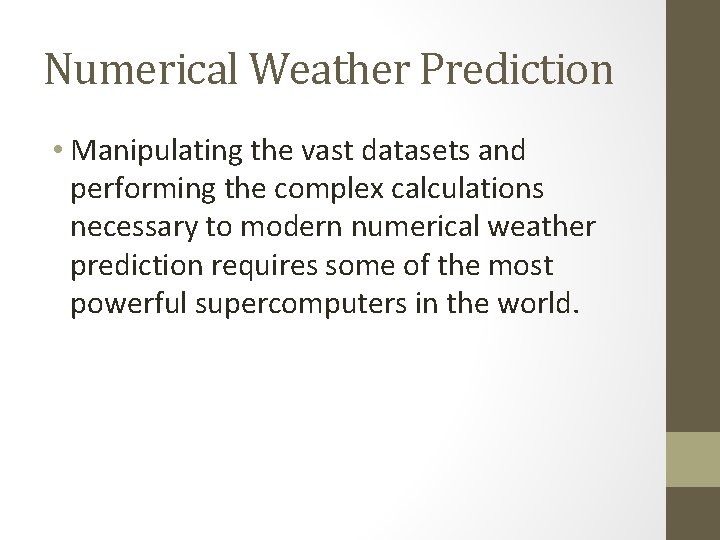
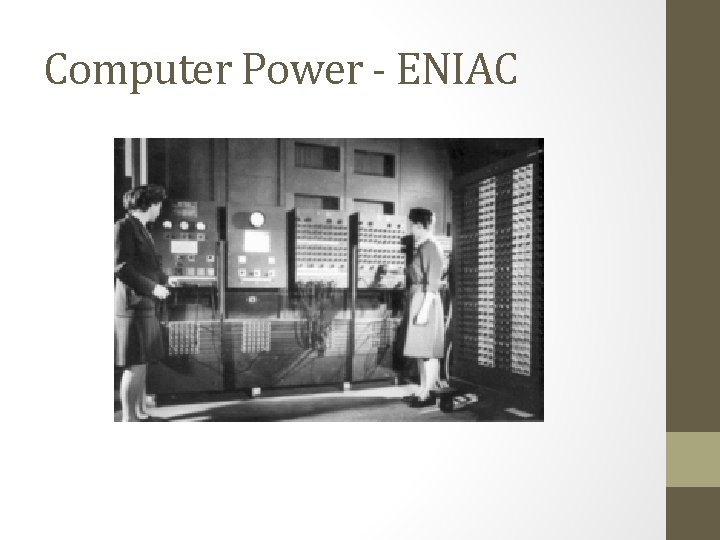
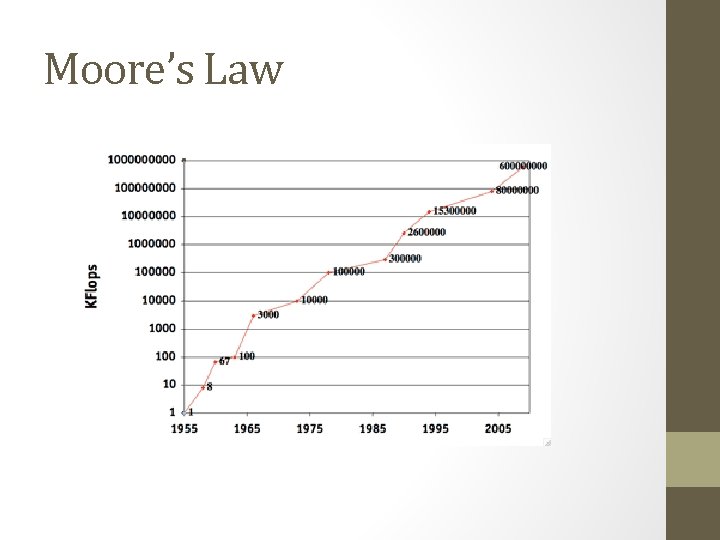
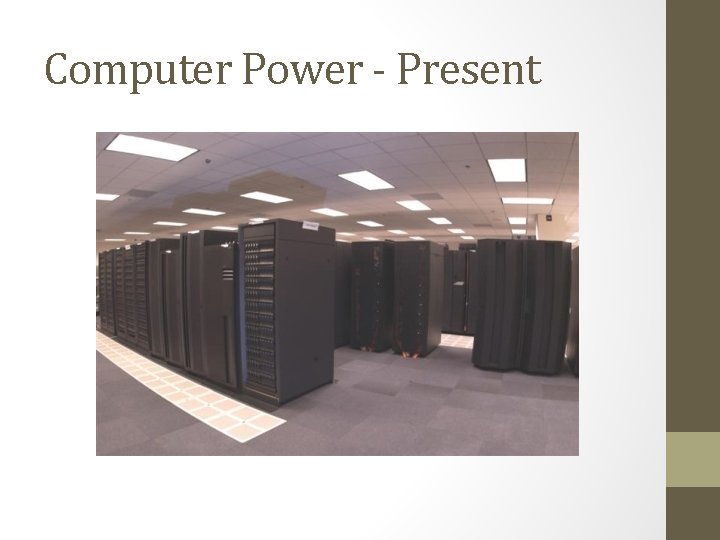
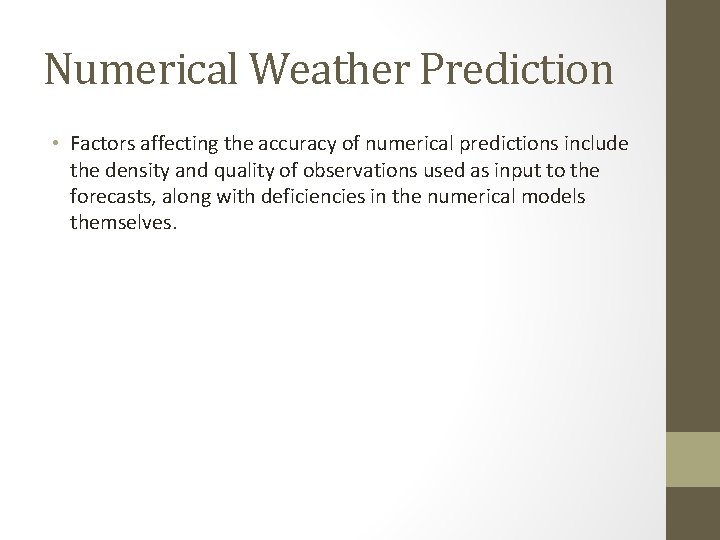
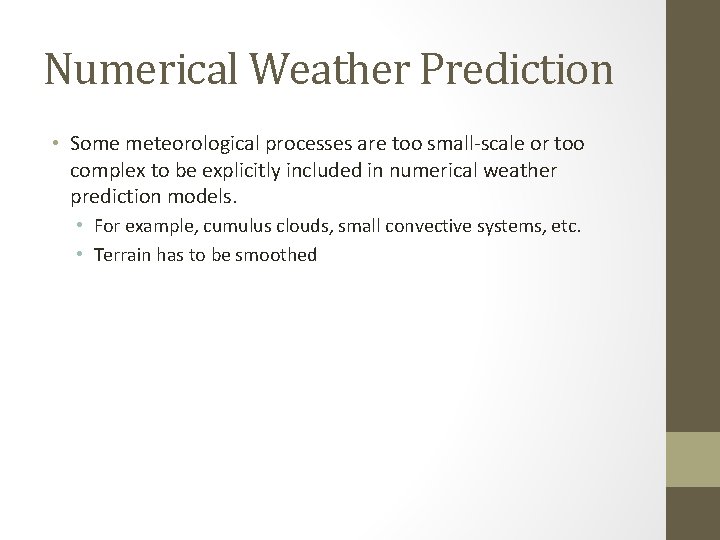
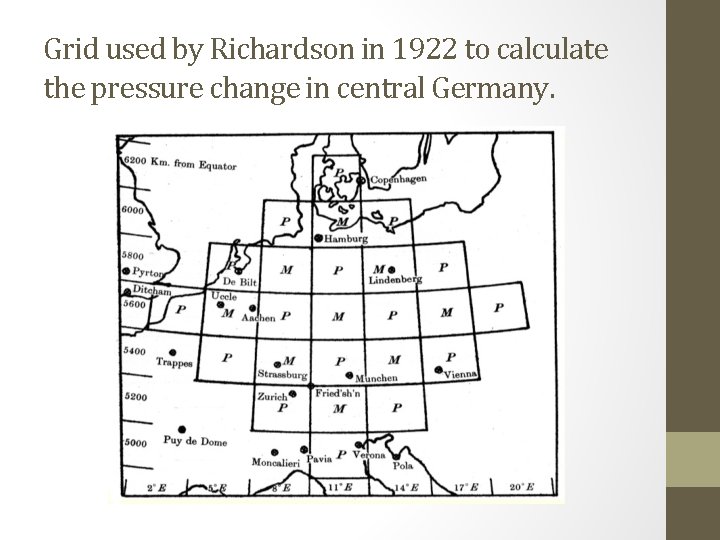
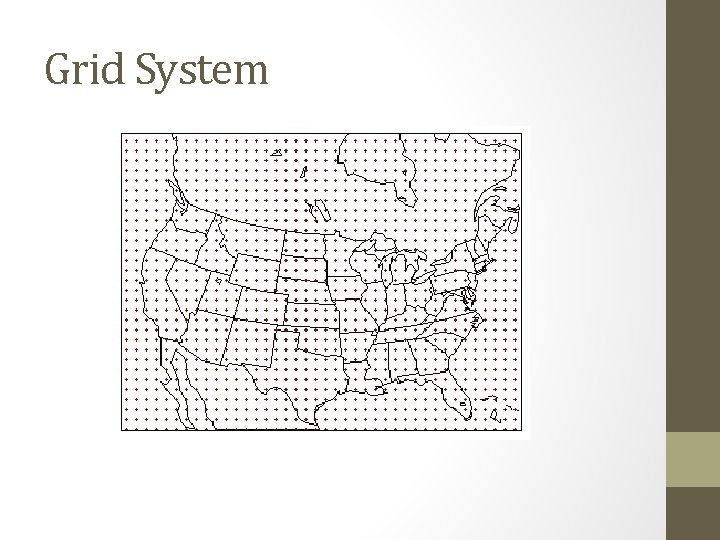
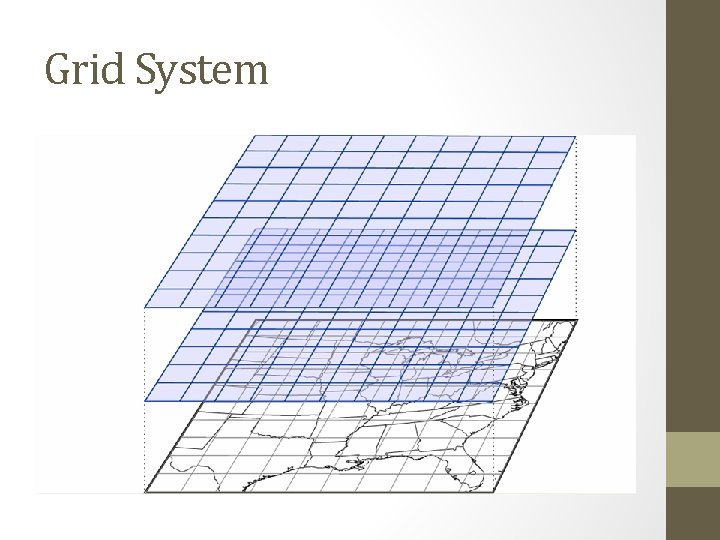
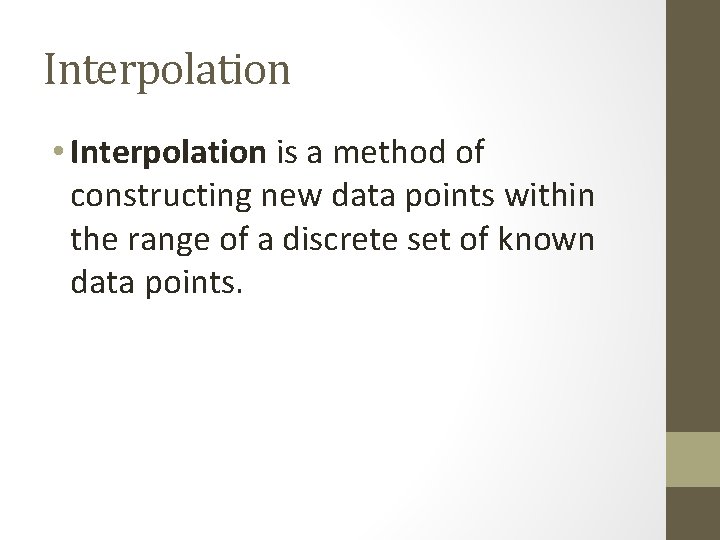
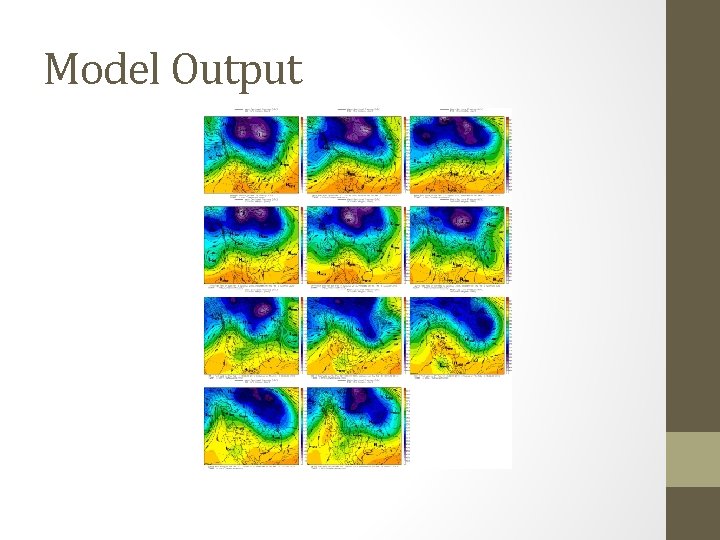
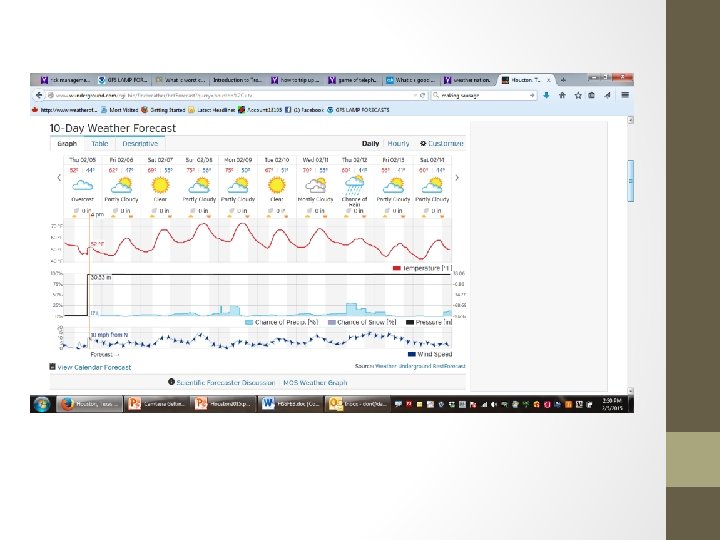
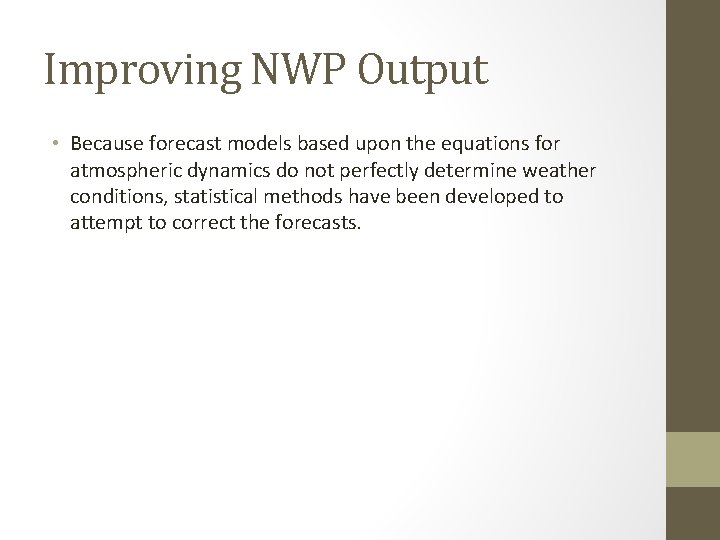
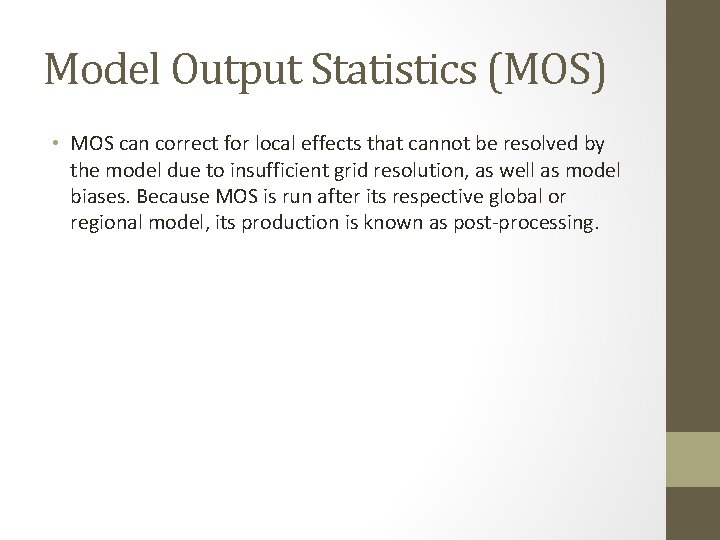
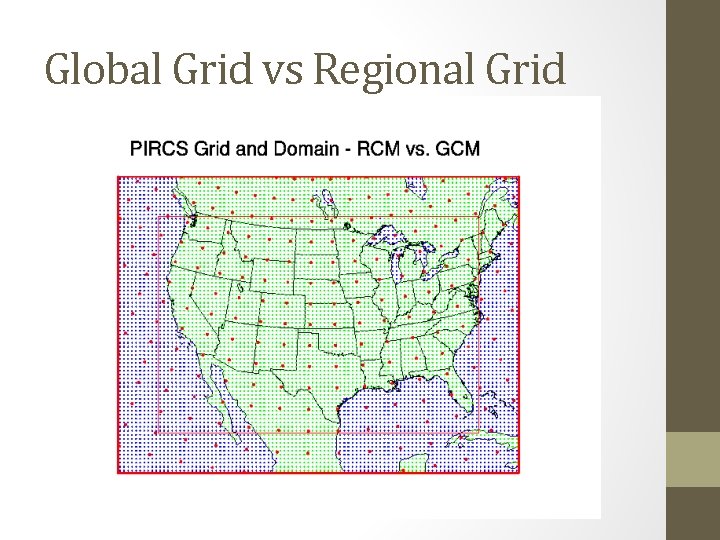
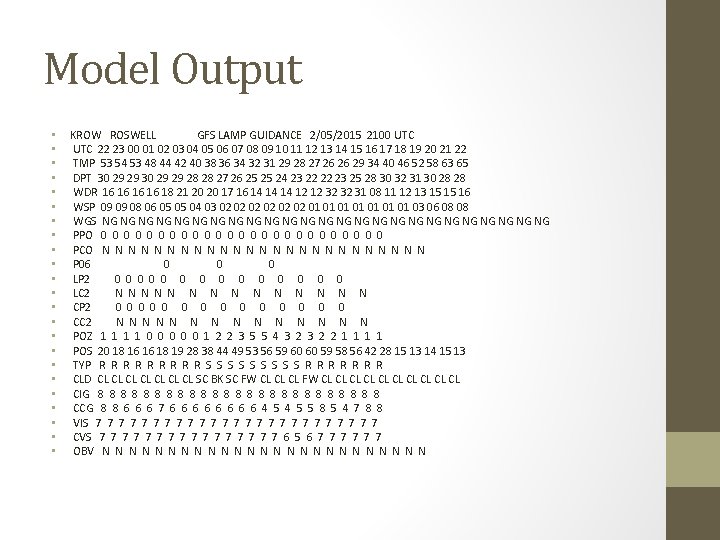
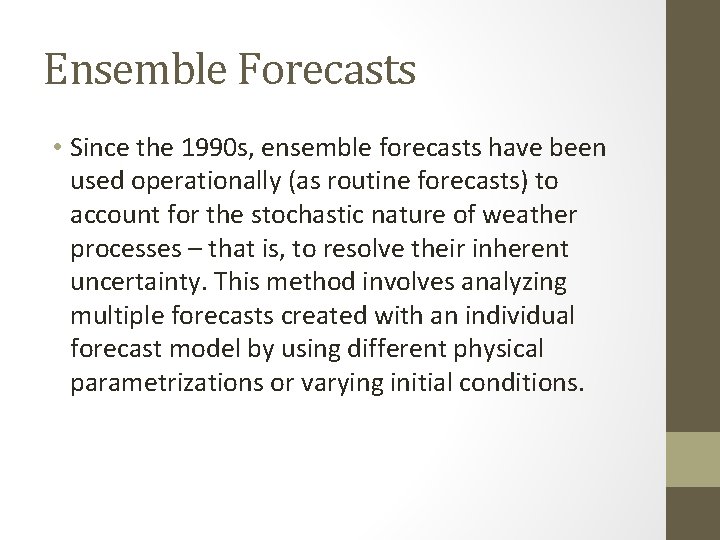
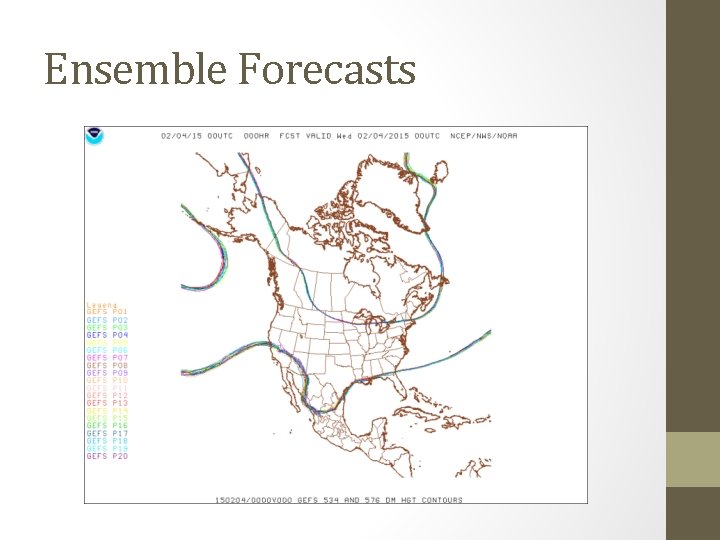
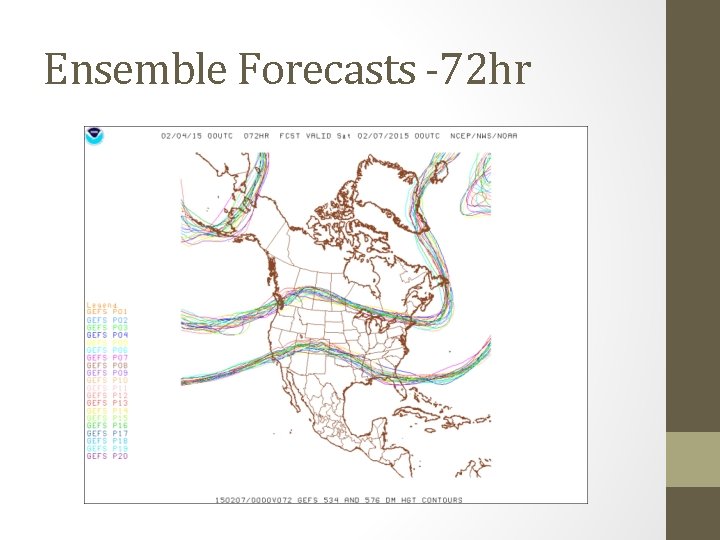
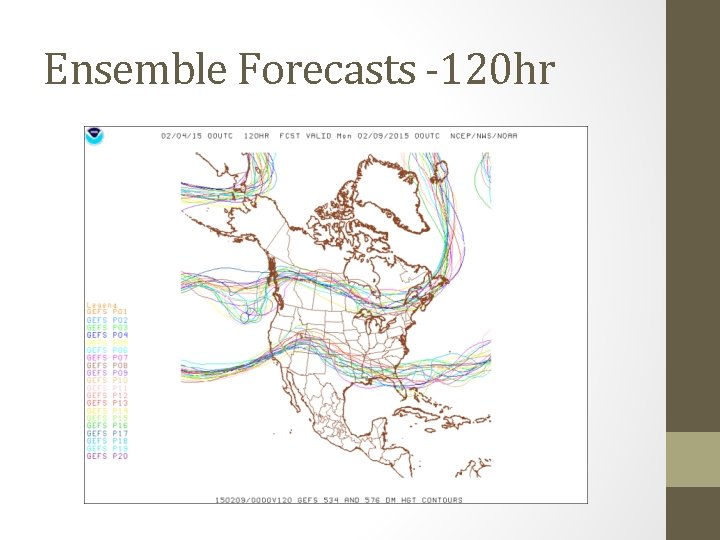
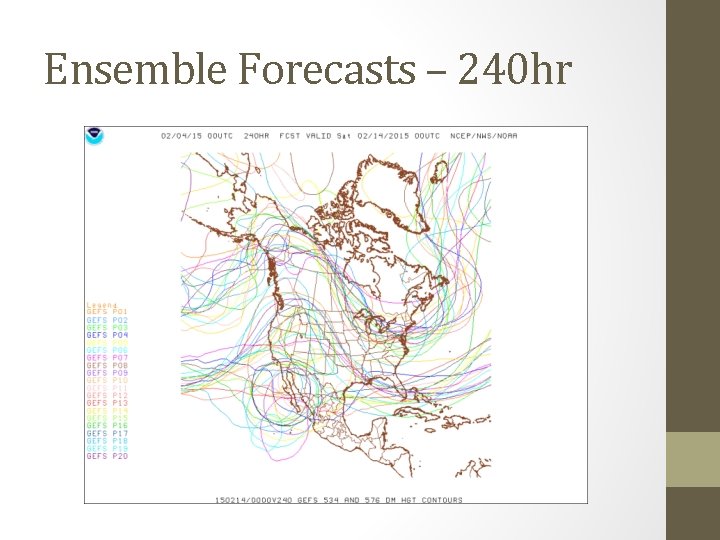
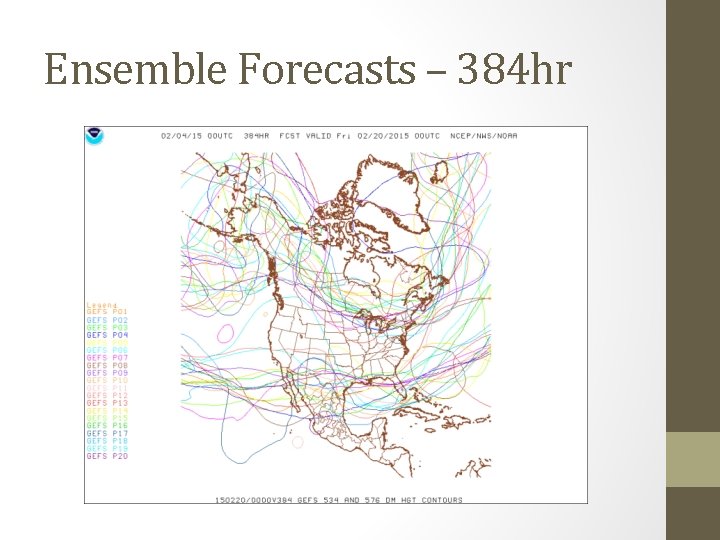
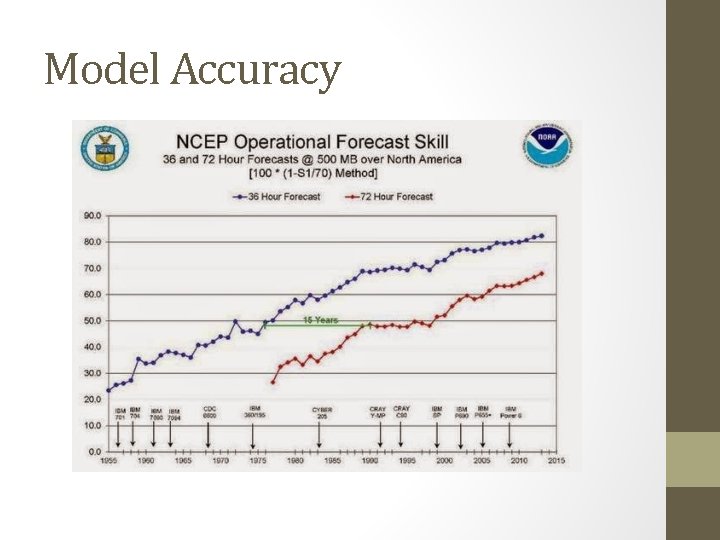
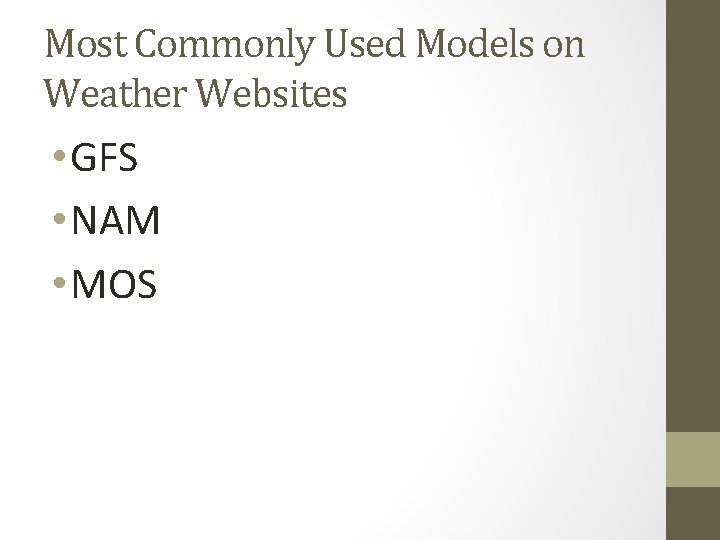
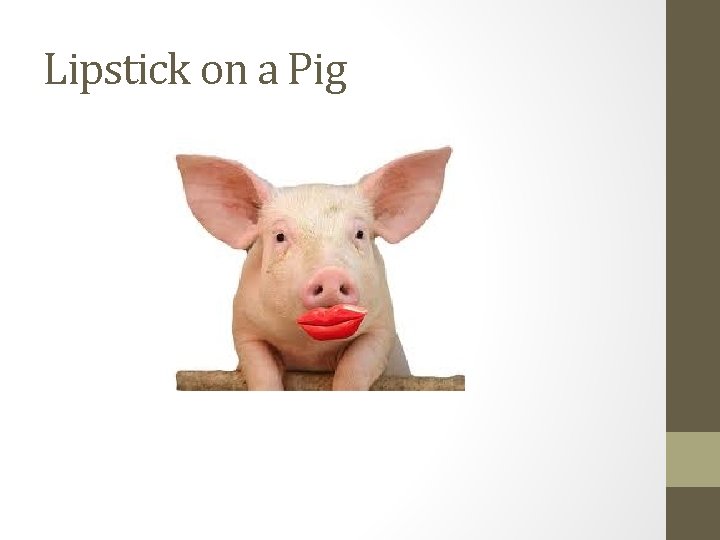
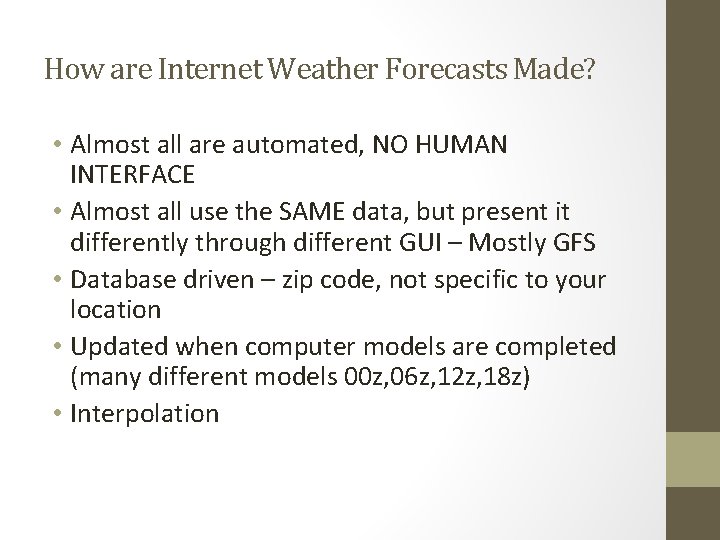
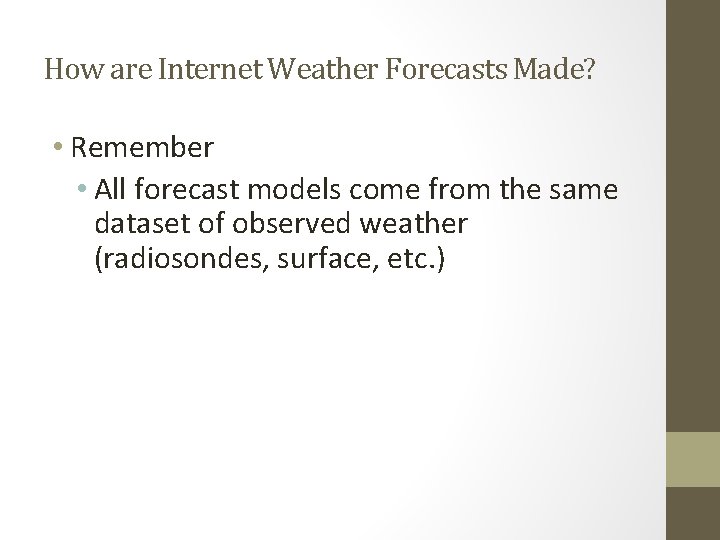
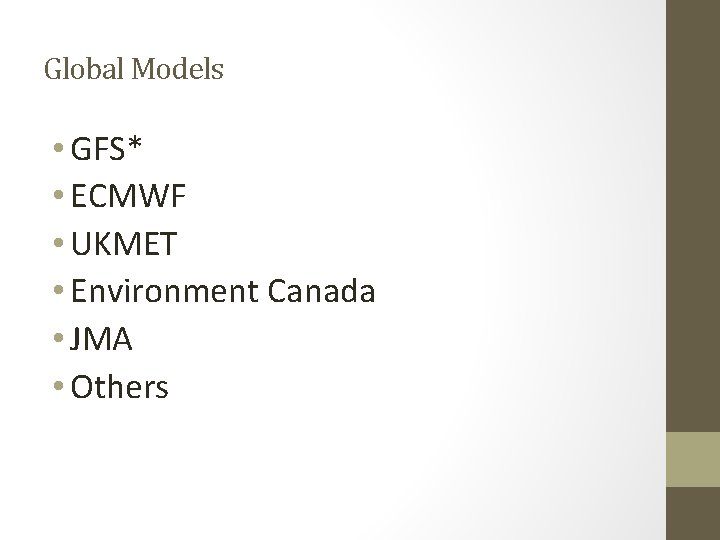
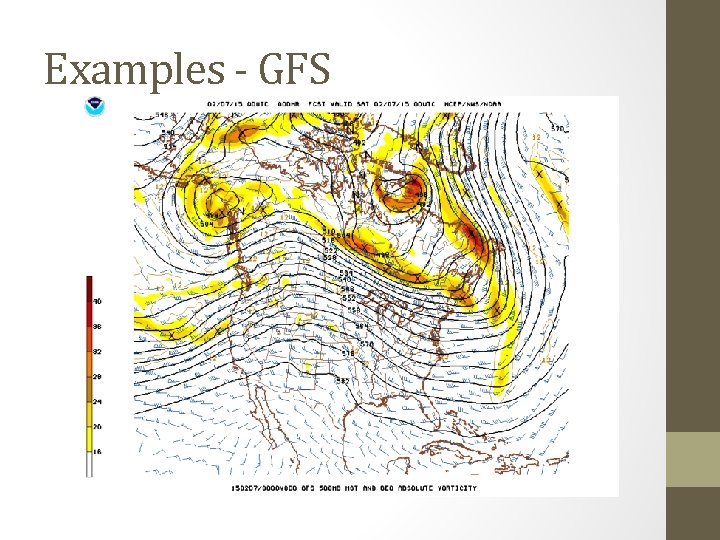
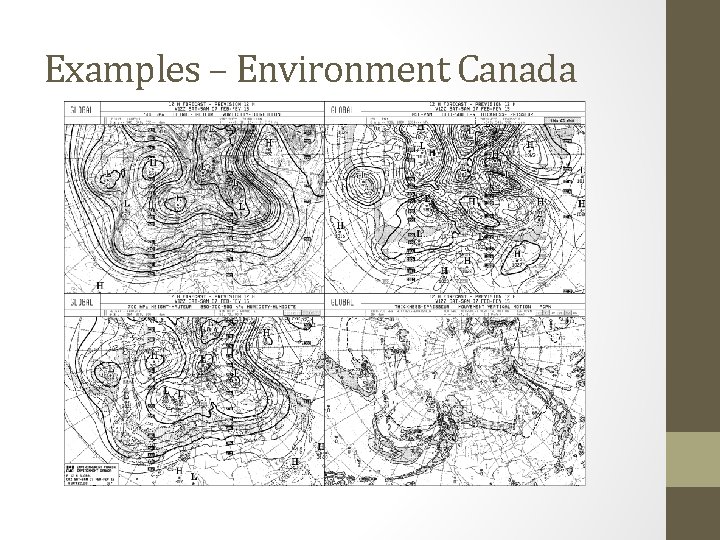
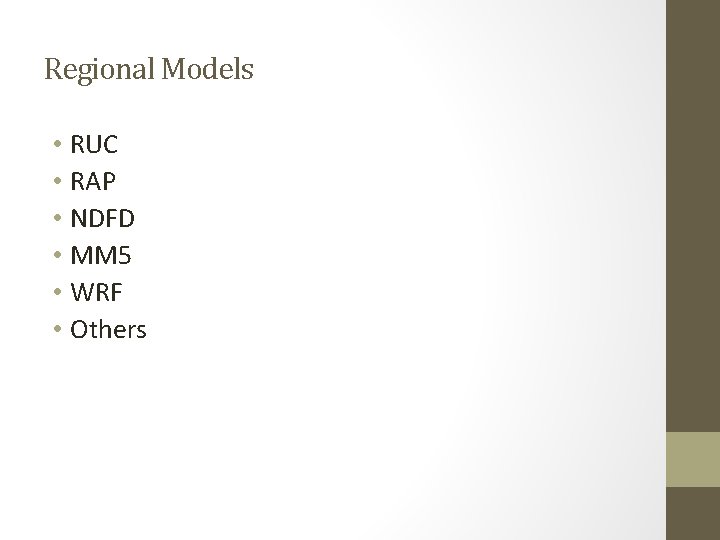
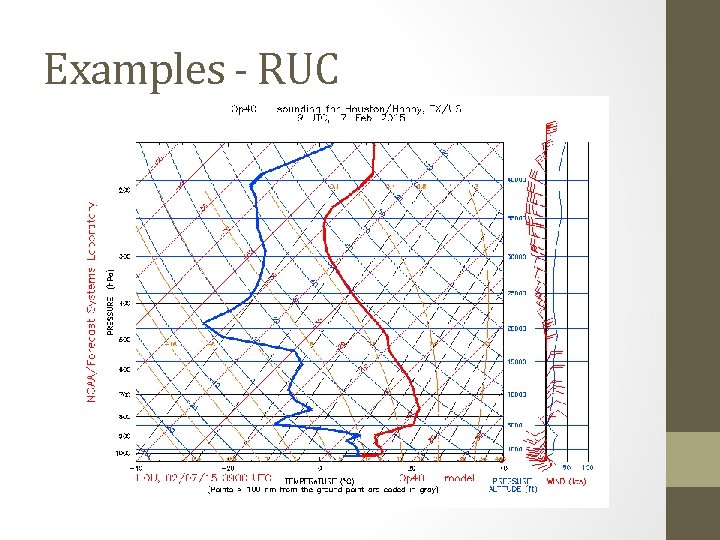
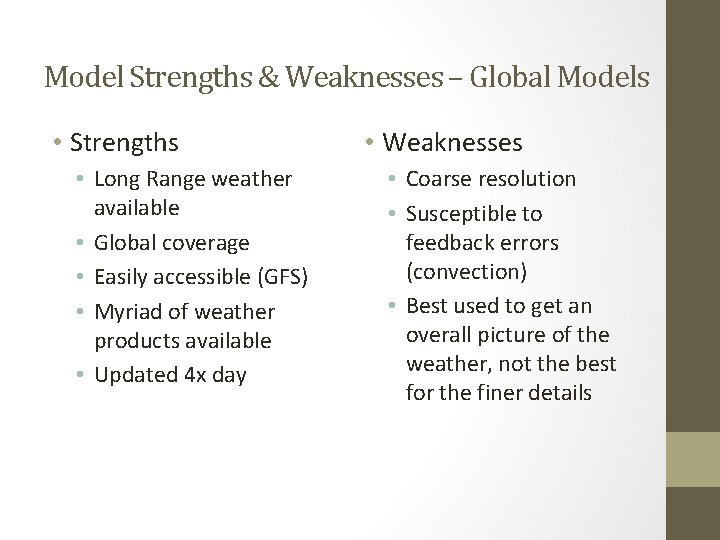
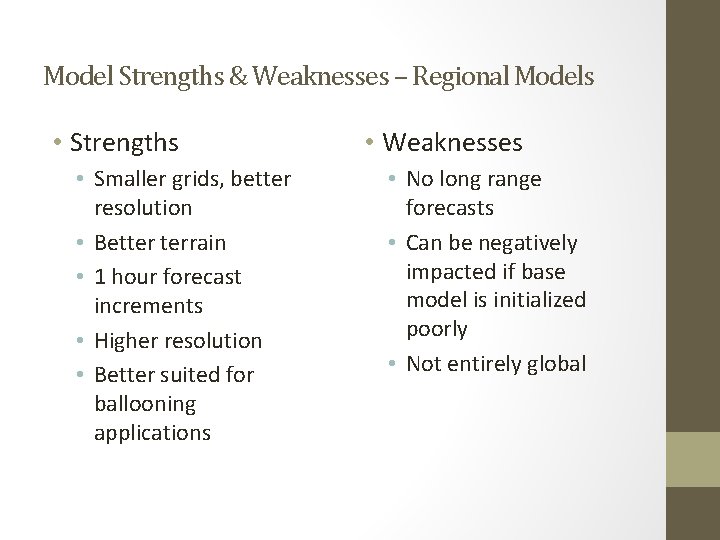
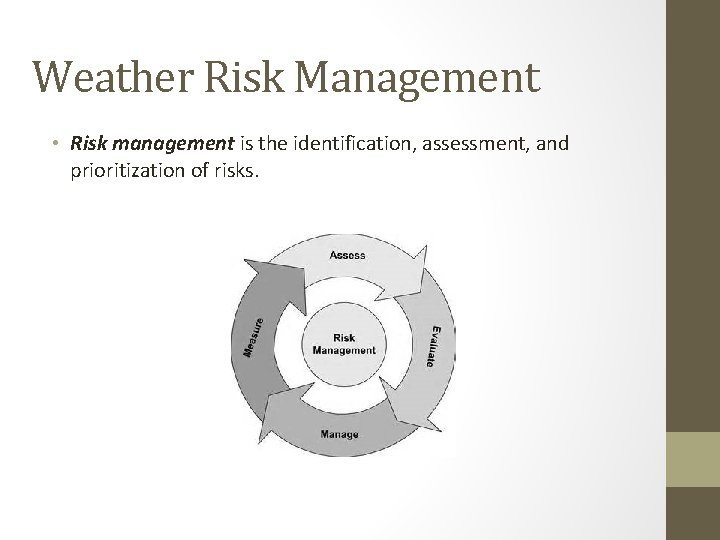
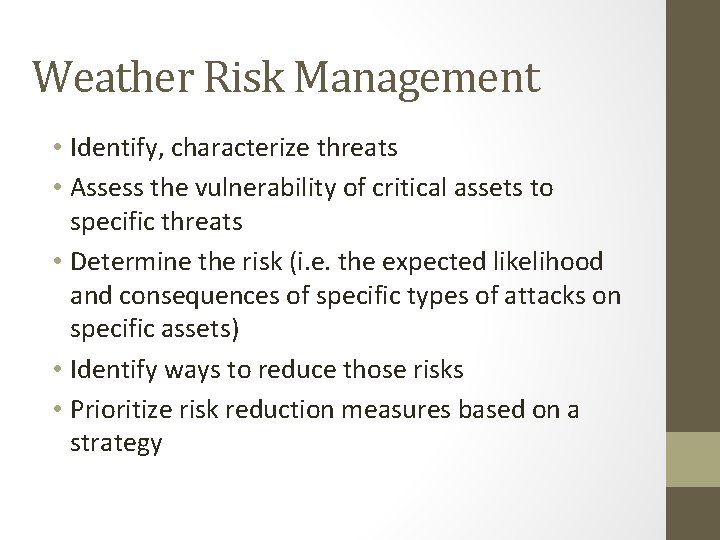
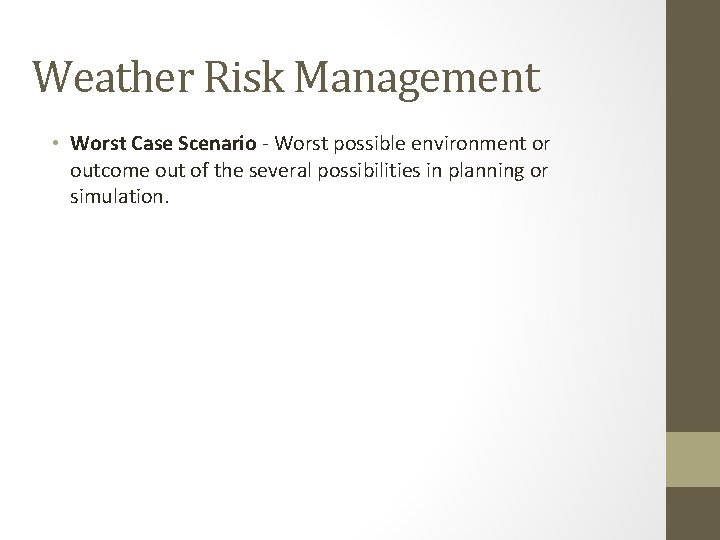
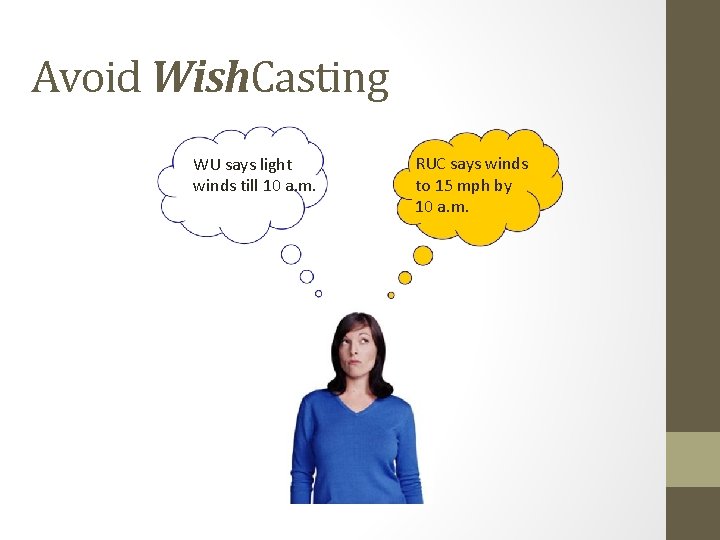
- Slides: 61
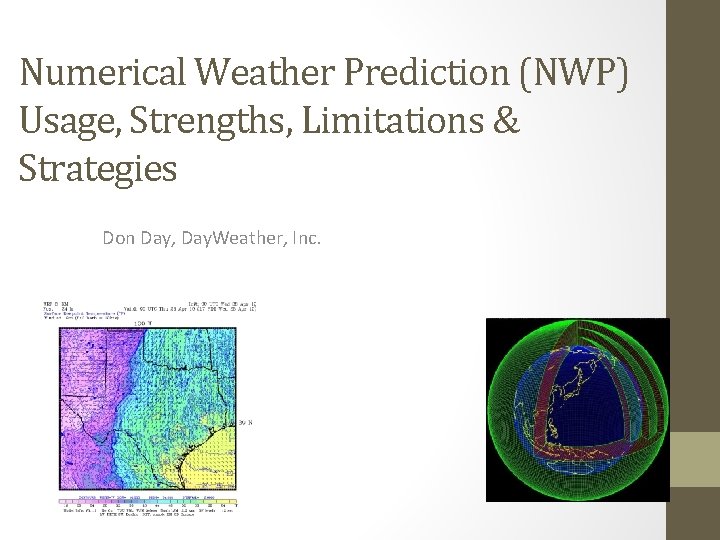
Numerical Weather Prediction (NWP) Usage, Strengths, Limitations & Strategies Don Day, Day. Weather, Inc.
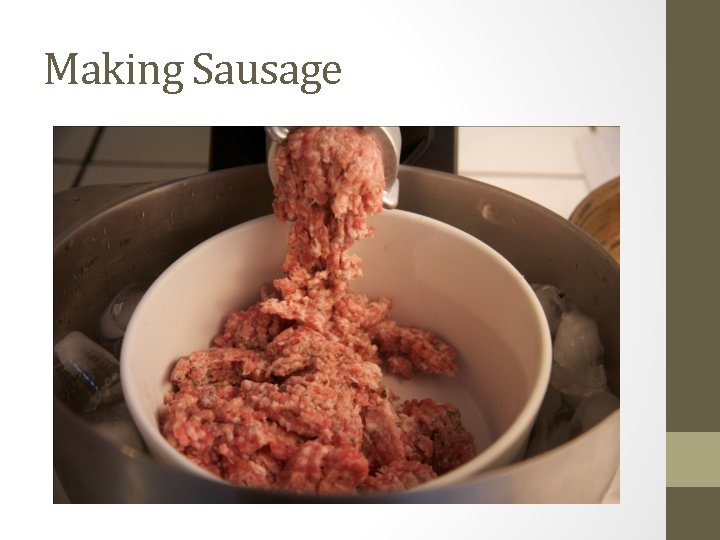
Making Sausage
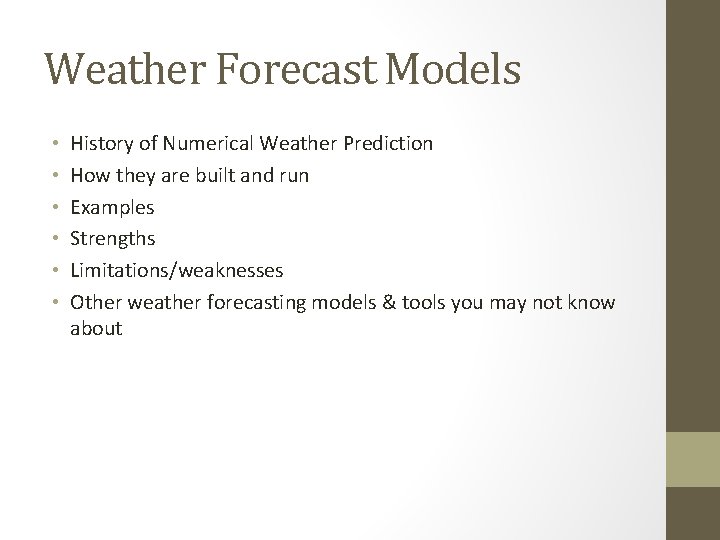
Weather Forecast Models • • • History of Numerical Weather Prediction How they are built and run Examples Strengths Limitations/weaknesses Other weather forecasting models & tools you may not know about
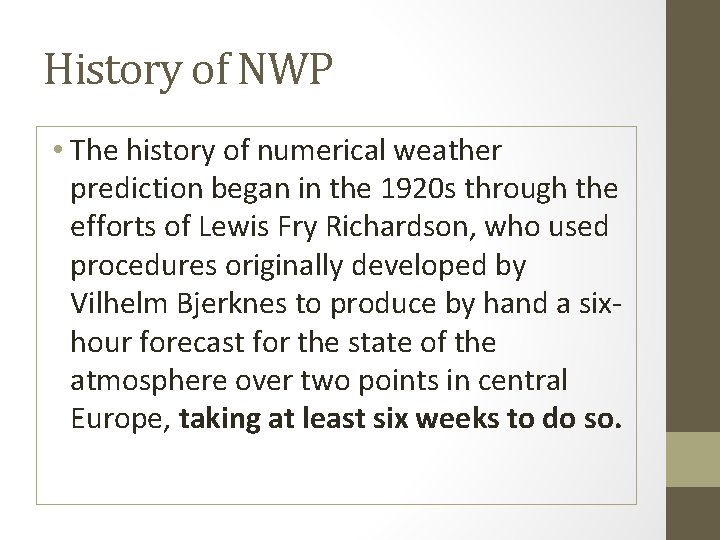
History of NWP • The history of numerical weather prediction began in the 1920 s through the efforts of Lewis Fry Richardson, who used procedures originally developed by Vilhelm Bjerknes to produce by hand a sixhour forecast for the state of the atmosphere over two points in central Europe, taking at least six weeks to do so.
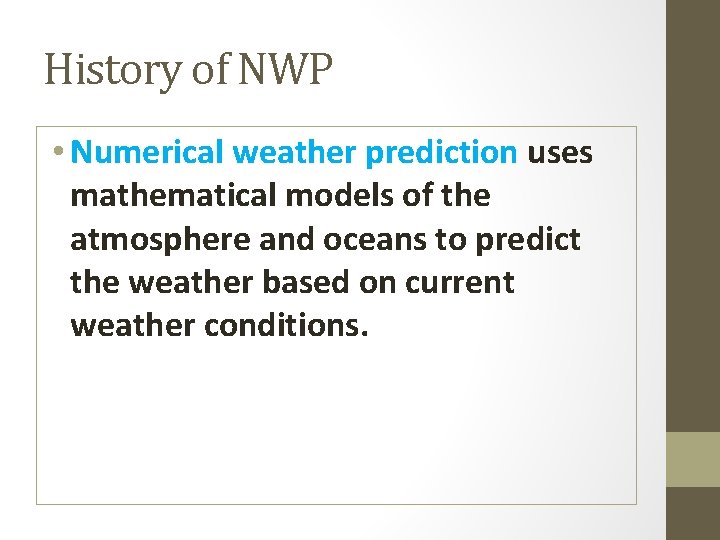
History of NWP • Numerical weather prediction uses mathematical models of the atmosphere and oceans to predict the weather based on current weather conditions.
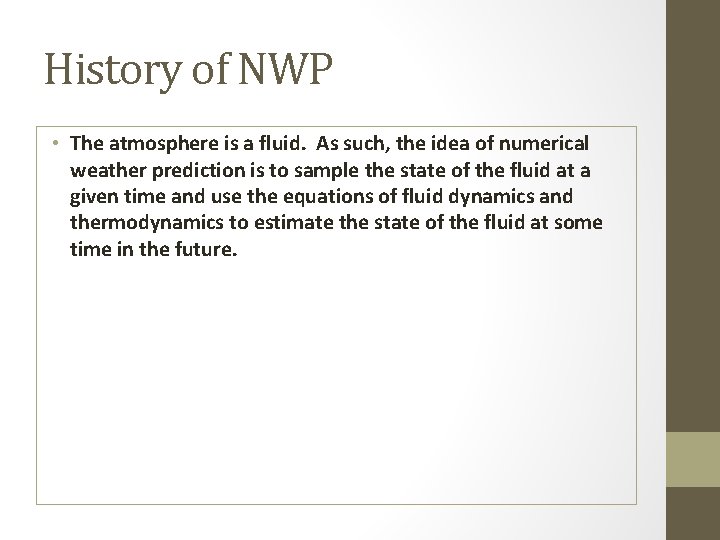
History of NWP • The atmosphere is a fluid. As such, the idea of numerical weather prediction is to sample the state of the fluid at a given time and use the equations of fluid dynamics and thermodynamics to estimate the state of the fluid at some time in the future.
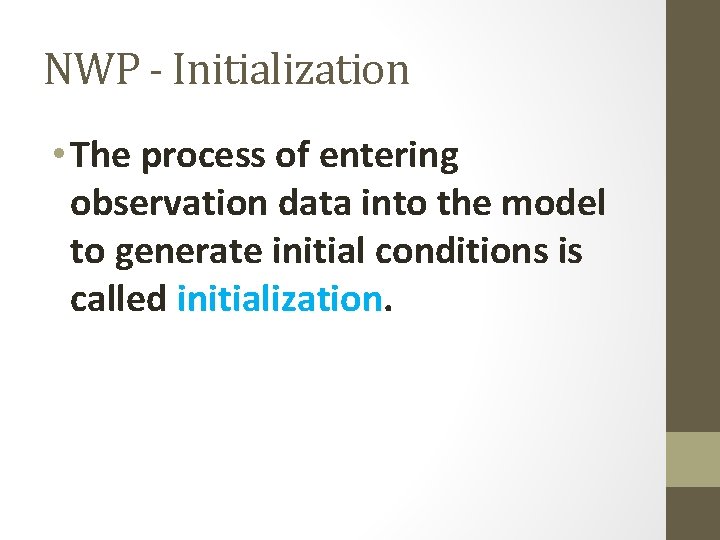
NWP - Initialization • The process of entering observation data into the model to generate initial conditions is called initialization.
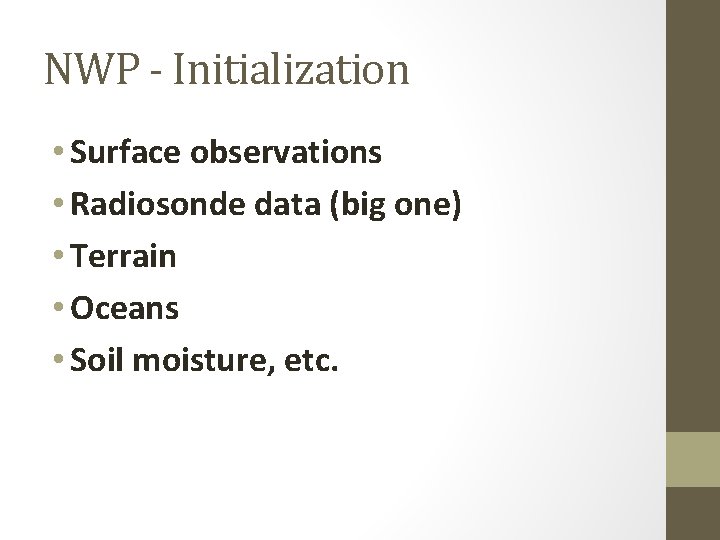
NWP - Initialization • Surface observations • Radiosonde data (big one) • Terrain • Oceans • Soil moisture, etc.
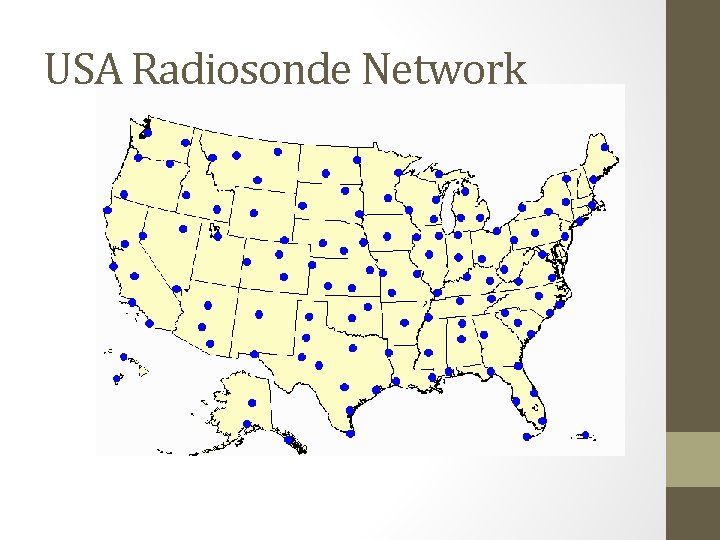
USA Radiosonde Network
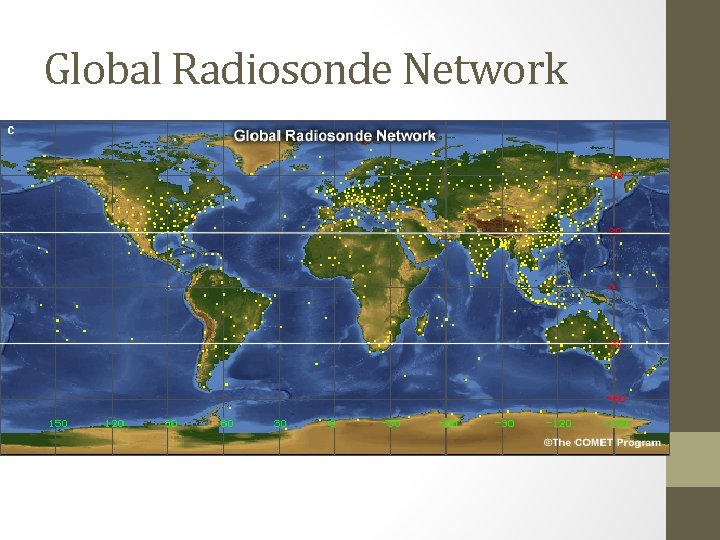
Global Radiosonde Network
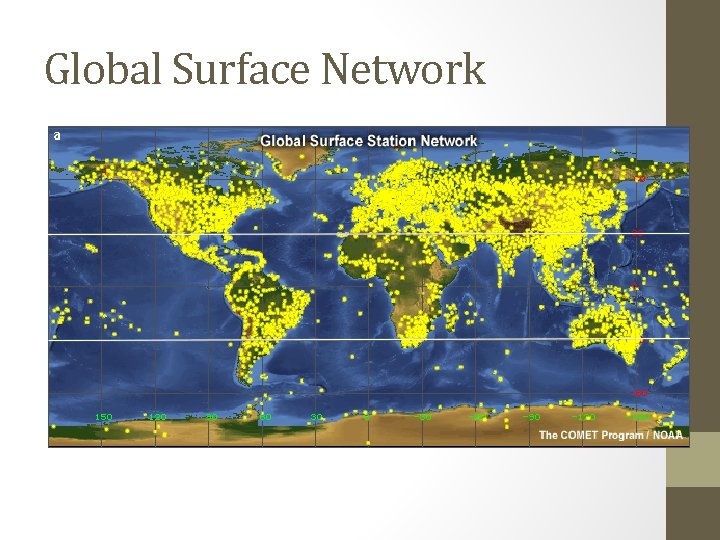
Global Surface Network
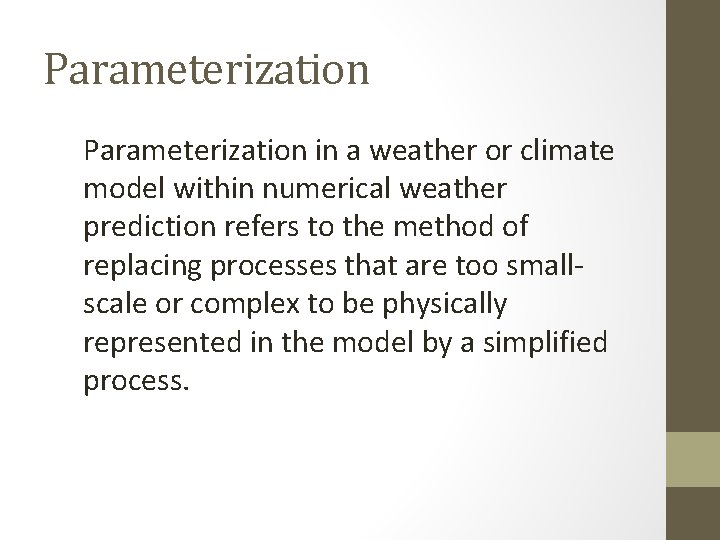
Parameterization in a weather or climate model within numerical weather prediction refers to the method of replacing processes that are too smallscale or complex to be physically represented in the model by a simplified process.
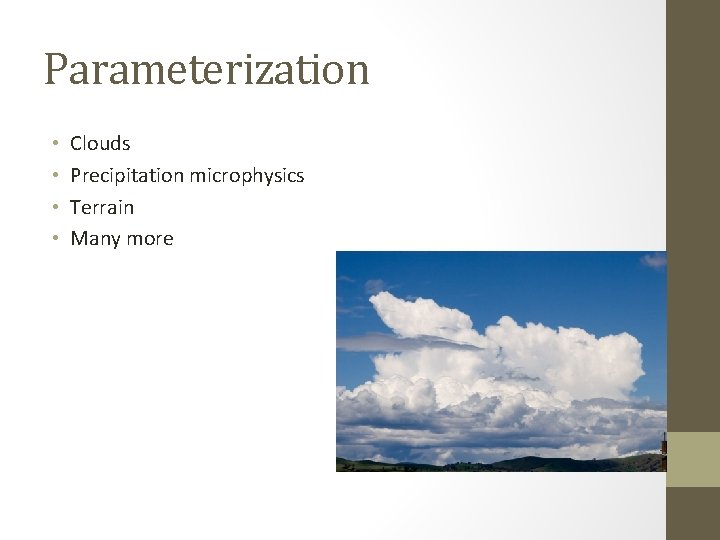
Parameterization • • Clouds Precipitation microphysics Terrain Many more
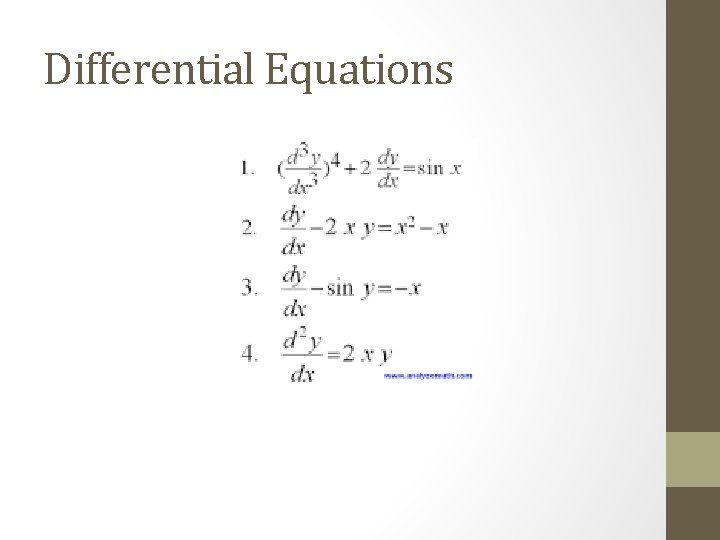
Differential Equations
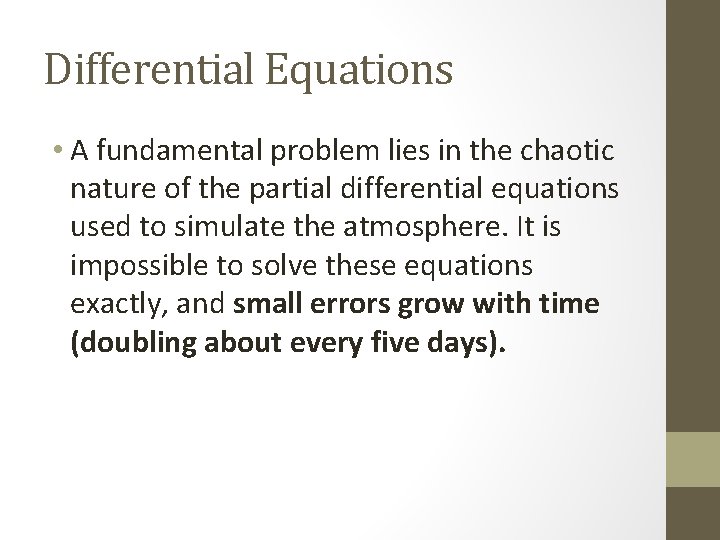
Differential Equations • A fundamental problem lies in the chaotic nature of the partial differential equations used to simulate the atmosphere. It is impossible to solve these equations exactly, and small errors grow with time (doubling about every five days).
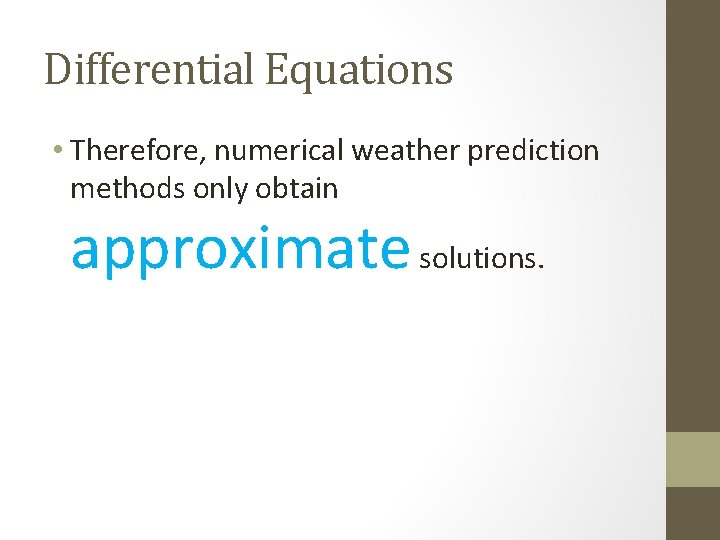
Differential Equations • Therefore, numerical weather prediction methods only obtain approximate solutions.
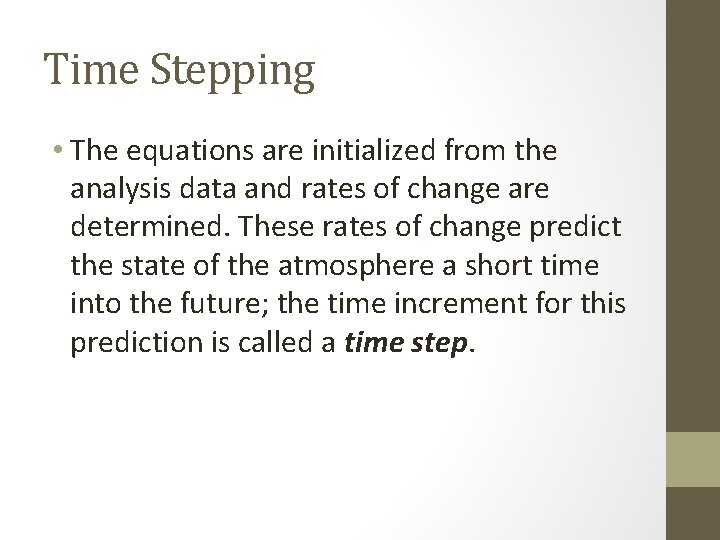
Time Stepping • The equations are initialized from the analysis data and rates of change are determined. These rates of change predict the state of the atmosphere a short time into the future; the time increment for this prediction is called a time step.
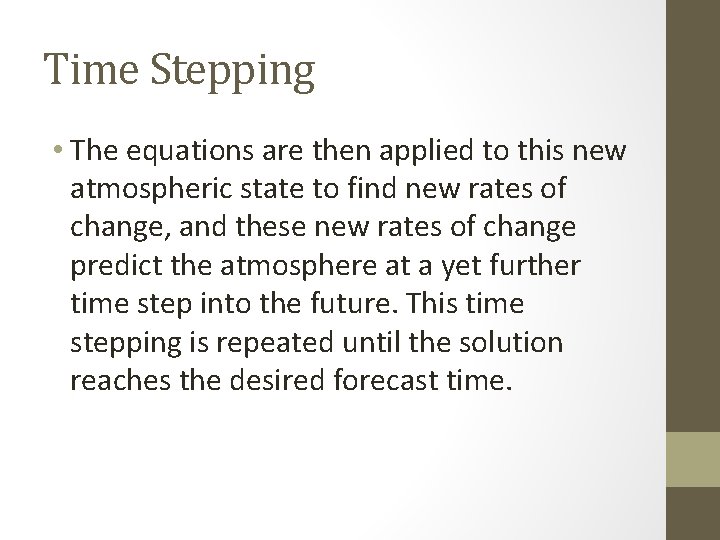
Time Stepping • The equations are then applied to this new atmospheric state to find new rates of change, and these new rates of change predict the atmosphere at a yet further time step into the future. This time stepping is repeated until the solution reaches the desired forecast time.
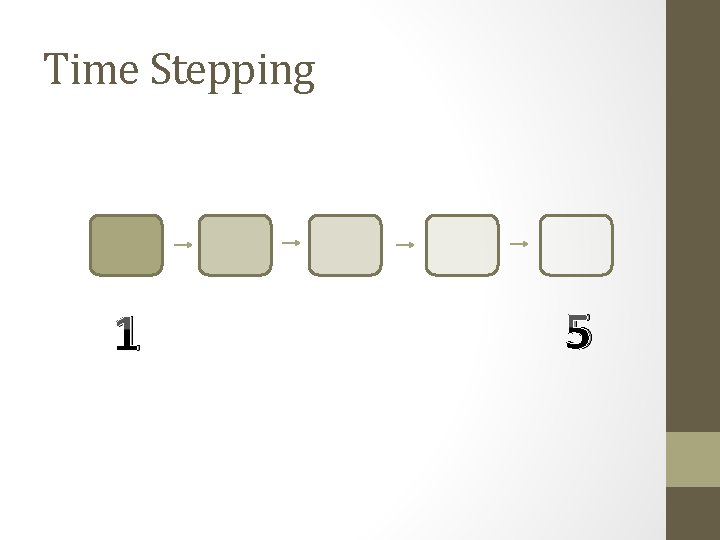
Time Stepping 1 5
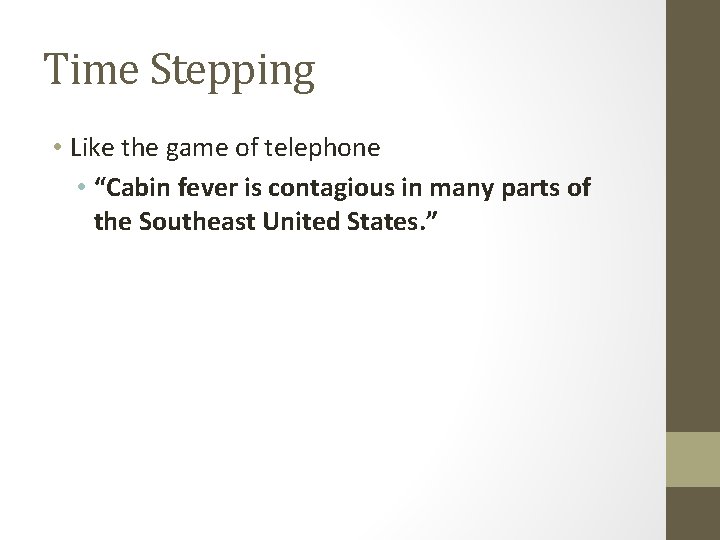
Time Stepping • Like the game of telephone • “Cabin fever is contagious in many parts of the Southeast United States. ”
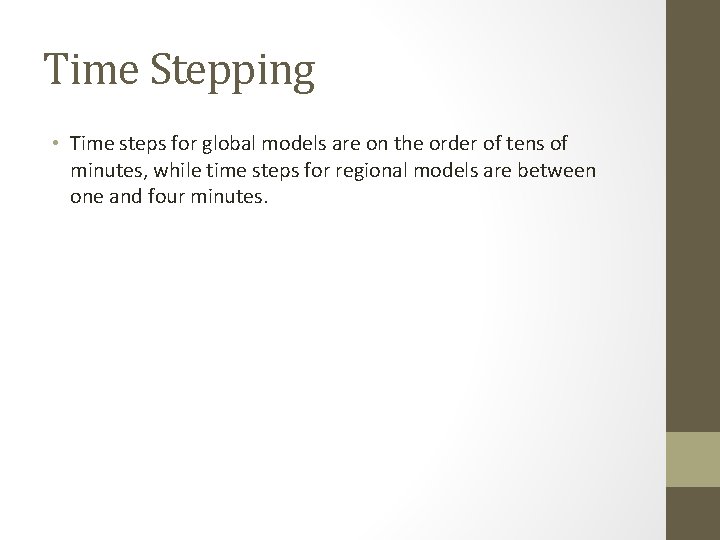
Time Stepping • Time steps for global models are on the order of tens of minutes, while time steps for regional models are between one and four minutes.
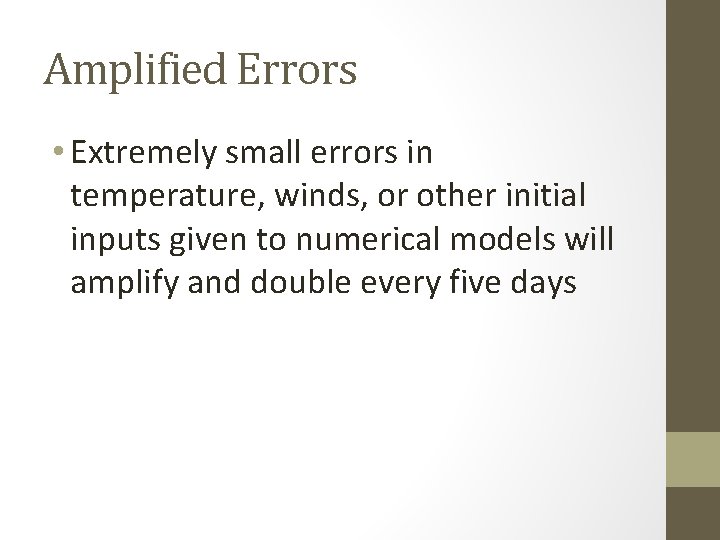
Amplified Errors • Extremely small errors in temperature, winds, or other initial inputs given to numerical models will amplify and double every five days
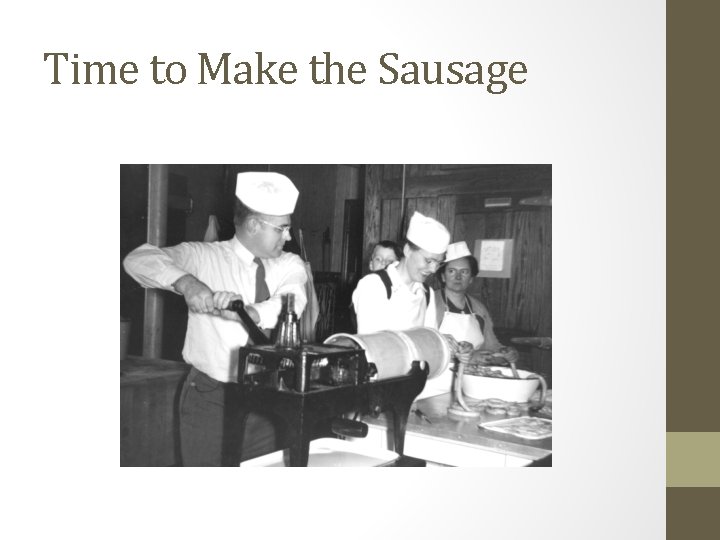
Time to Make the Sausage
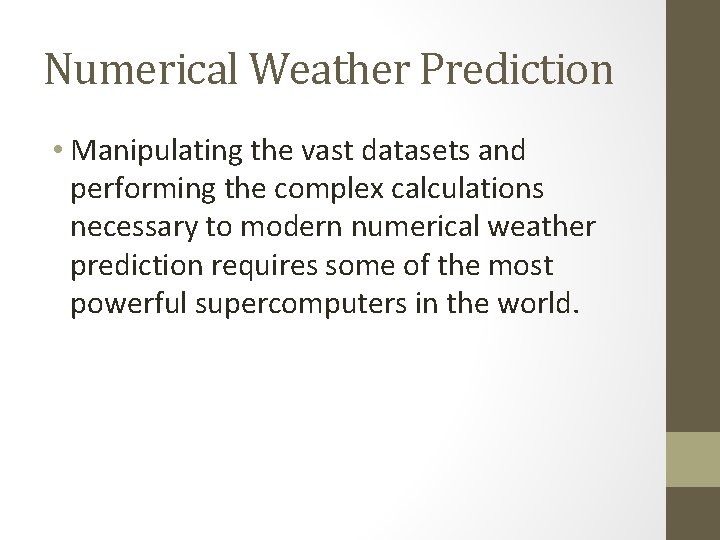
Numerical Weather Prediction • Manipulating the vast datasets and performing the complex calculations necessary to modern numerical weather prediction requires some of the most powerful supercomputers in the world.
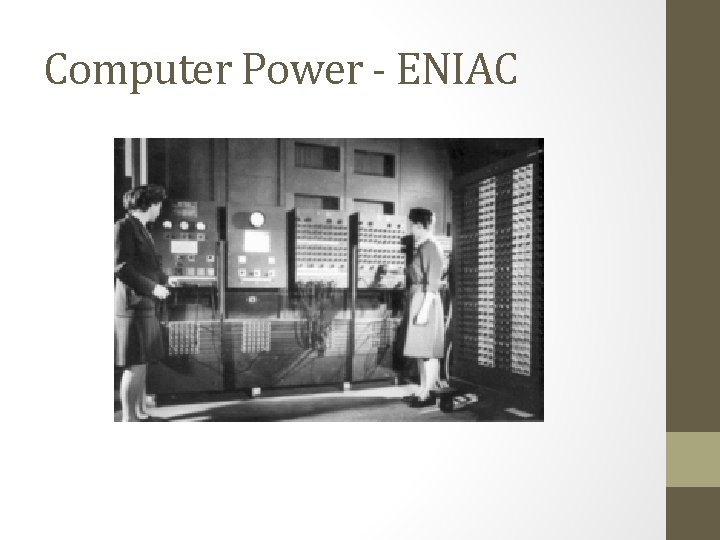
Computer Power - ENIAC
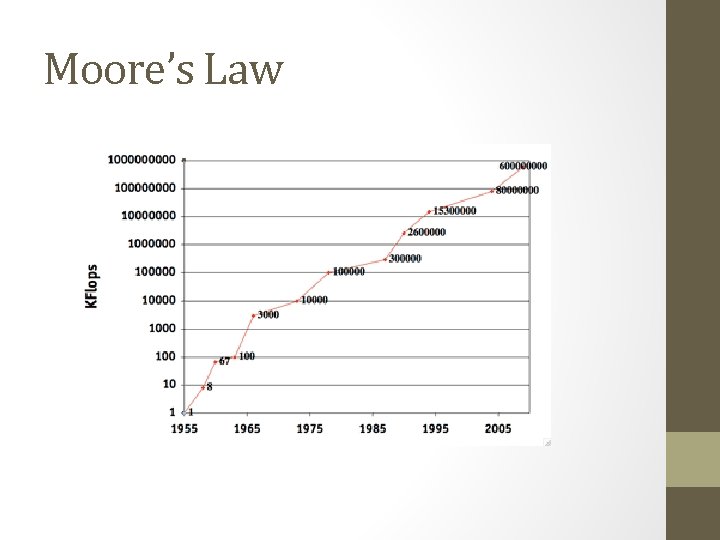
Moore’s Law
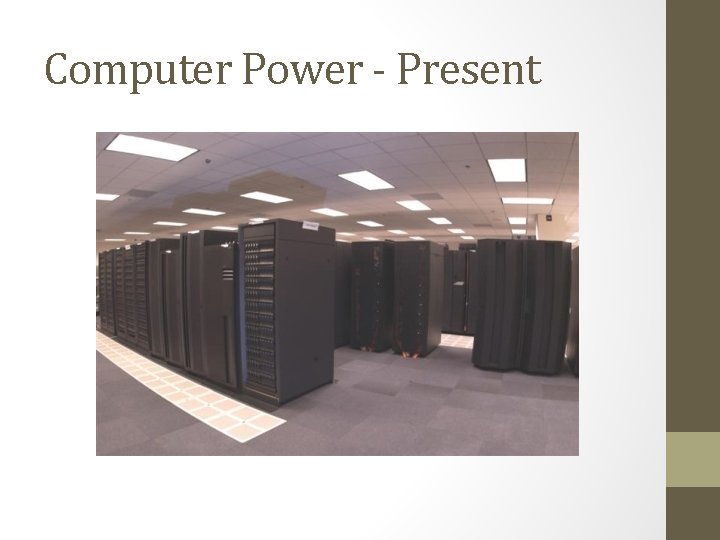
Computer Power - Present
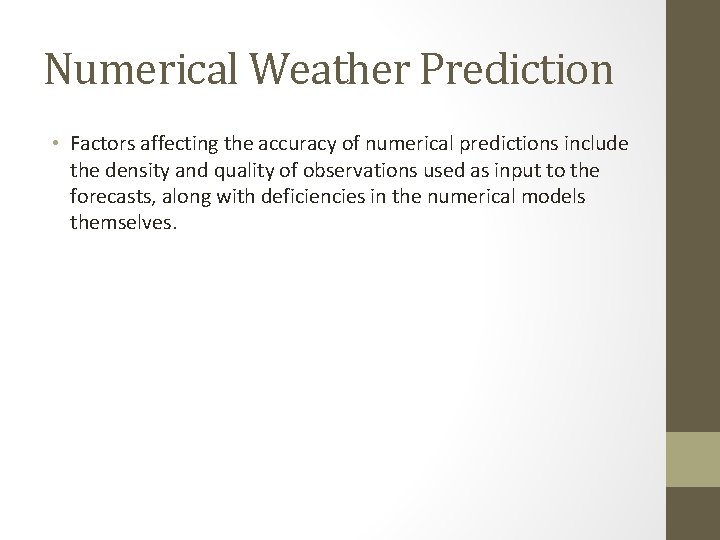
Numerical Weather Prediction • Factors affecting the accuracy of numerical predictions include the density and quality of observations used as input to the forecasts, along with deficiencies in the numerical models themselves.
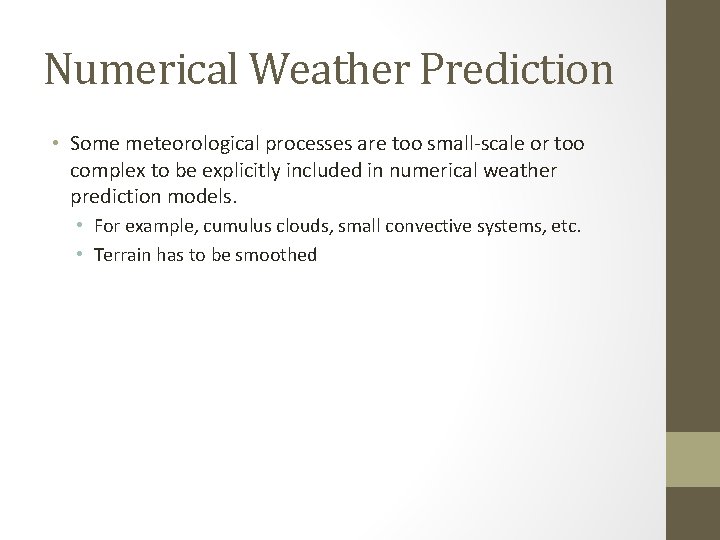
Numerical Weather Prediction • Some meteorological processes are too small-scale or too complex to be explicitly included in numerical weather prediction models. • For example, cumulus clouds, small convective systems, etc. • Terrain has to be smoothed
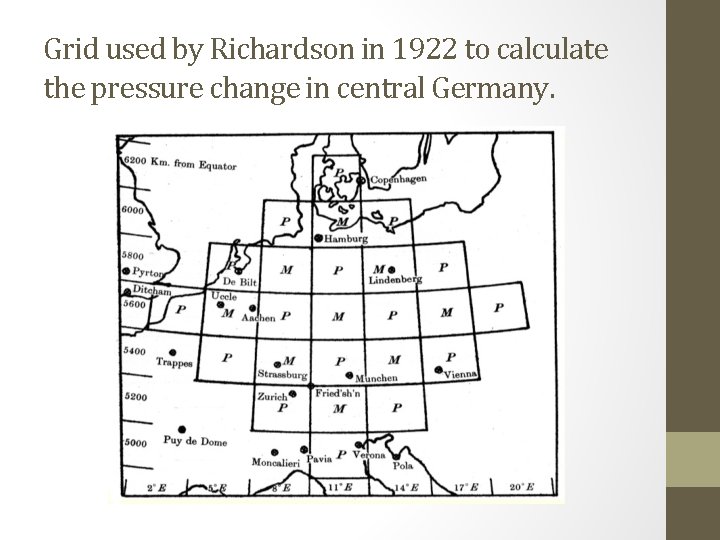
Grid used by Richardson in 1922 to calculate the pressure change in central Germany.
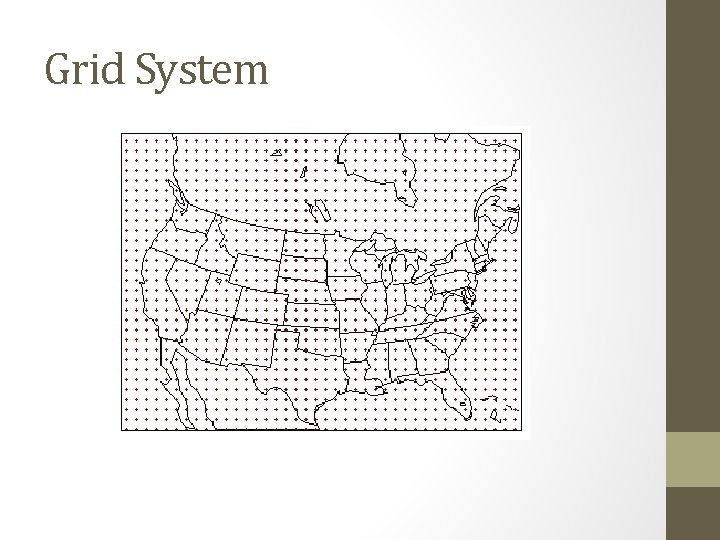
Grid System
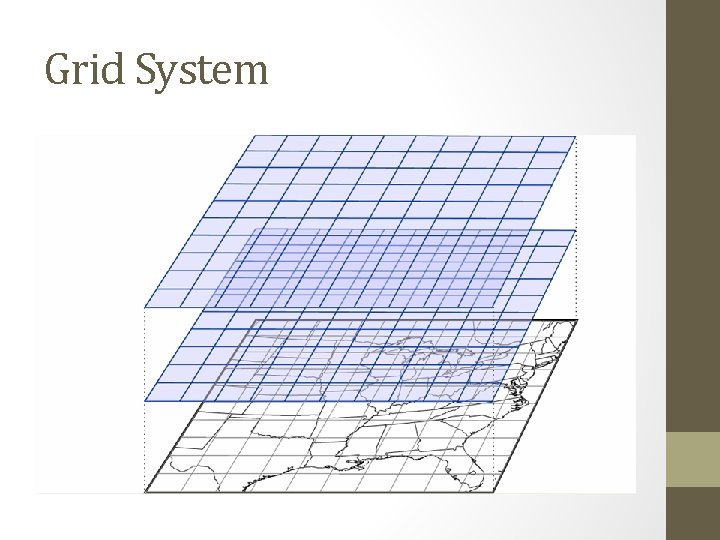
Grid System
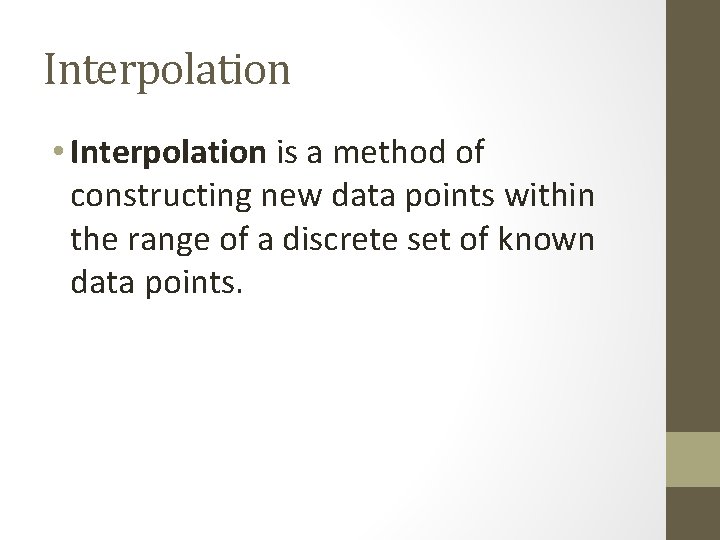
Interpolation • Interpolation is a method of constructing new data points within the range of a discrete set of known data points.
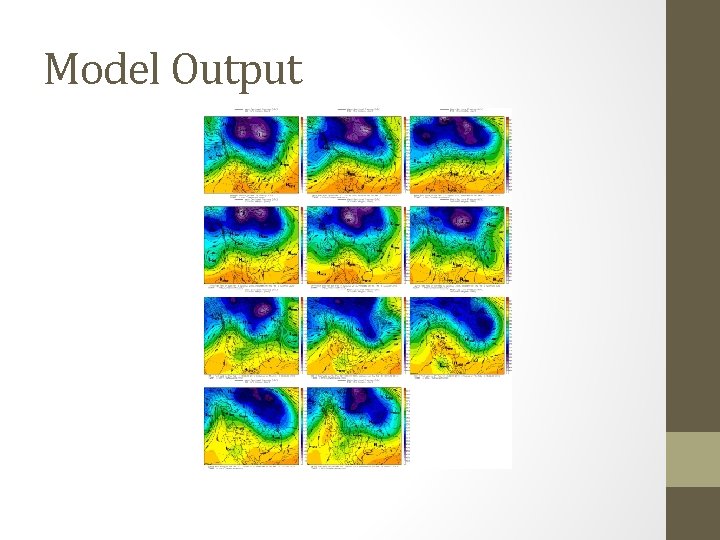
Model Output
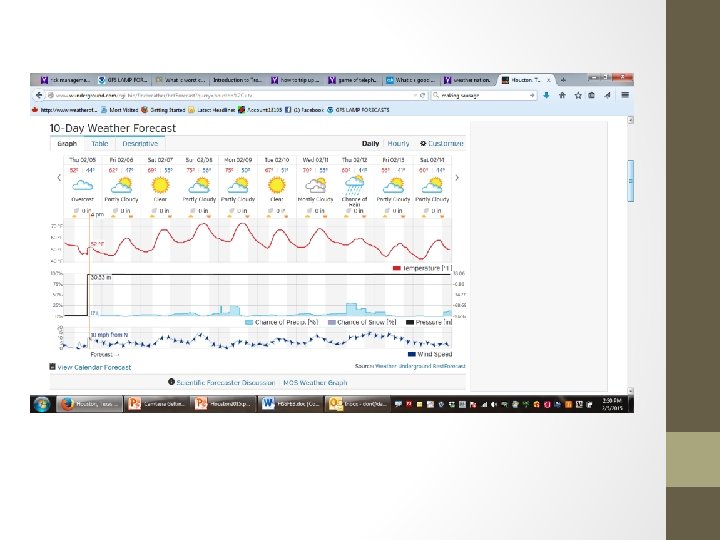
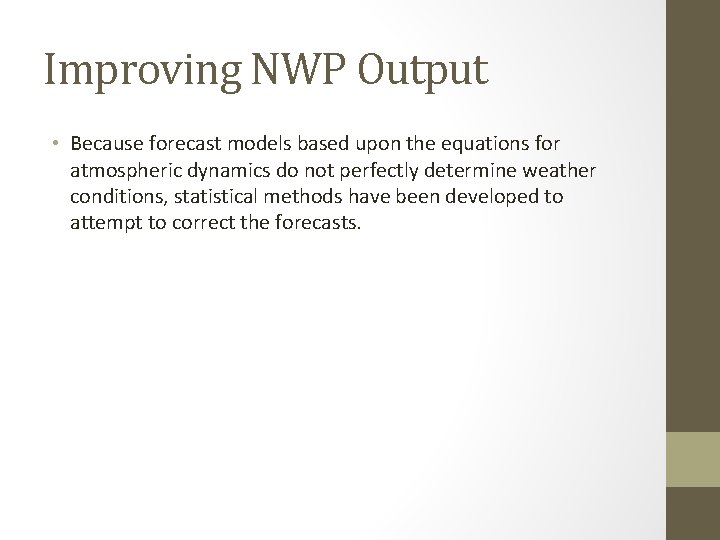
Improving NWP Output • Because forecast models based upon the equations for atmospheric dynamics do not perfectly determine weather conditions, statistical methods have been developed to attempt to correct the forecasts.
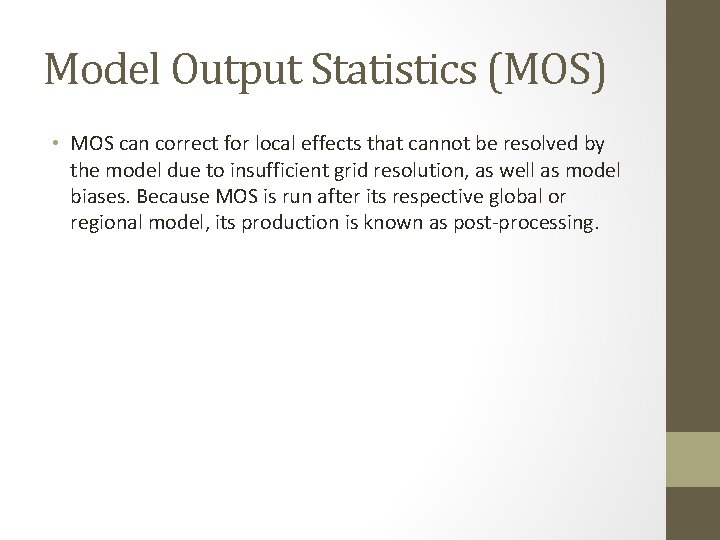
Model Output Statistics (MOS) • MOS can correct for local effects that cannot be resolved by the model due to insufficient grid resolution, as well as model biases. Because MOS is run after its respective global or regional model, its production is known as post-processing.
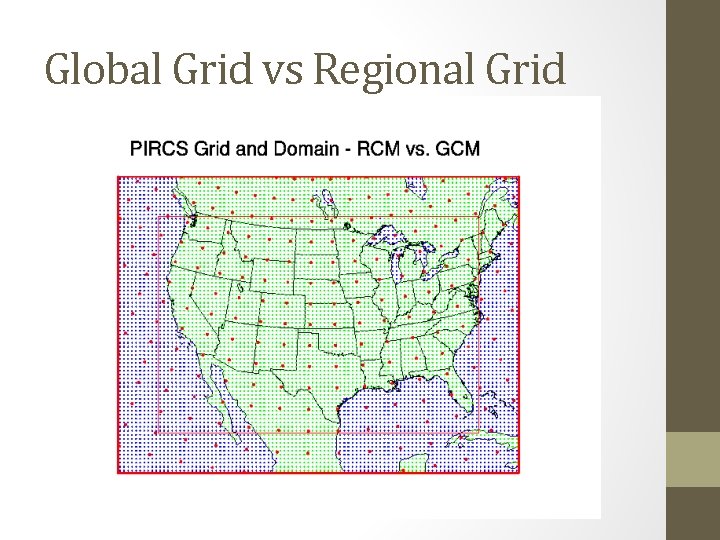
Global Grid vs Regional Grid
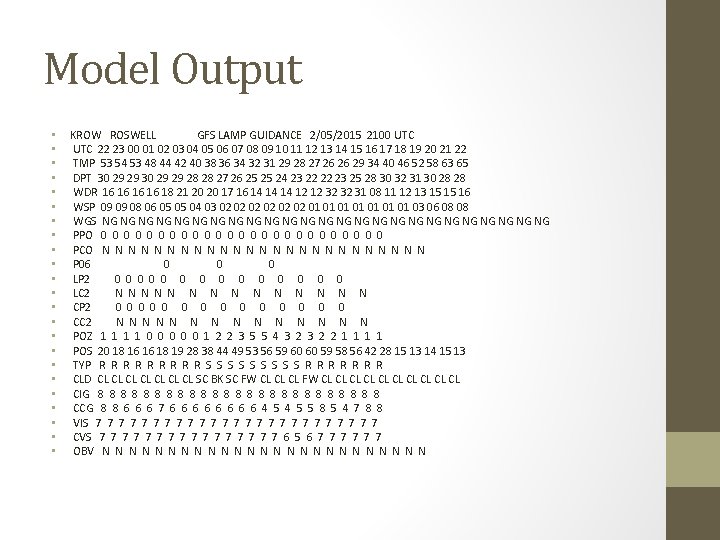
Model Output • • • • • • KROW ROSWELL GFS LAMP GUIDANCE 2/05/2015 2100 UTC 22 23 00 01 02 03 04 05 06 07 08 09 10 11 12 13 14 15 16 17 18 19 20 21 22 TMP 53 54 53 48 44 42 40 38 36 34 32 31 29 28 27 26 26 29 34 40 46 52 58 63 65 DPT 30 29 29 28 28 27 26 25 25 24 23 22 22 23 25 28 30 32 31 30 28 28 WDR 16 16 18 21 20 20 17 16 14 14 14 12 12 32 32 31 08 11 12 13 15 15 16 WSP 09 09 08 06 05 05 04 03 02 02 02 01 01 03 06 08 08 WGS NG NG NG NG NG NG NG PPO 0 0 0 0 0 0 0 PCO N N N N N N N P 06 0 0 0 LP 2 0 0 0 0 LC 2 N N N N CP 2 0 0 0 0 CC 2 N N N N POZ 1 1 0 0 0 1 2 2 3 5 5 4 3 2 2 1 1 POS 20 18 16 16 18 19 28 38 44 49 53 56 59 60 60 59 58 56 42 28 15 13 14 15 13 TYP R R R R R S S S S S R R R R CLD CL CL SC BK SC FW CL CL CL CL CIG 8 8 8 8 8 8 8 CCG 8 8 6 6 6 7 6 6 6 6 4 5 5 8 5 4 7 8 8 VIS 7 7 7 7 7 7 7 CVS 7 7 7 7 6 5 6 7 7 7 OBV N N N N N N N
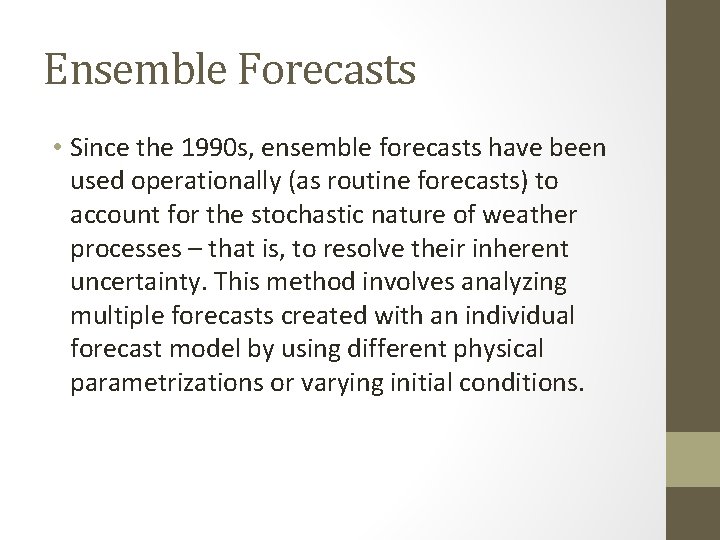
Ensemble Forecasts • Since the 1990 s, ensemble forecasts have been used operationally (as routine forecasts) to account for the stochastic nature of weather processes – that is, to resolve their inherent uncertainty. This method involves analyzing multiple forecasts created with an individual forecast model by using different physical parametrizations or varying initial conditions.
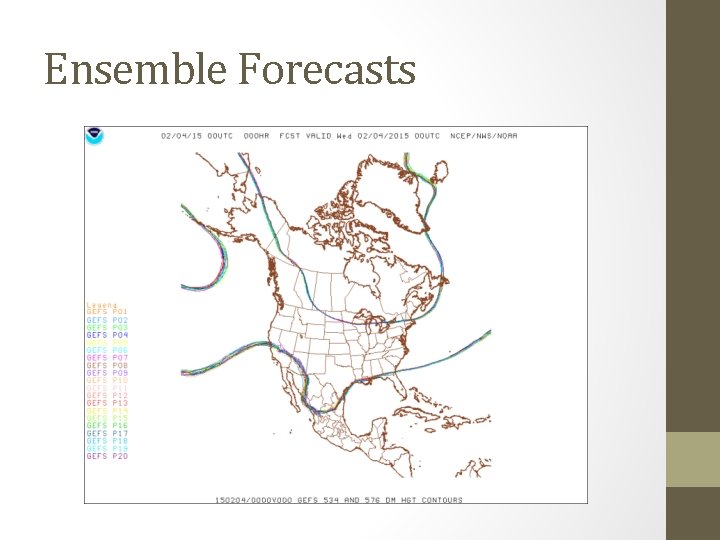
Ensemble Forecasts
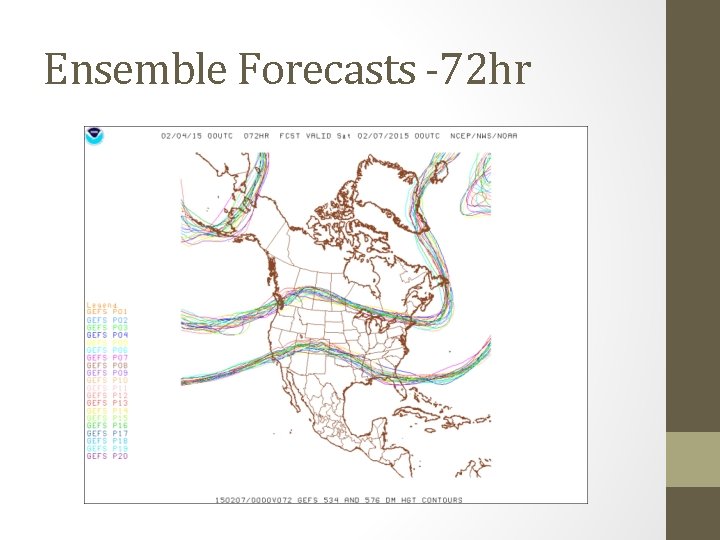
Ensemble Forecasts -72 hr
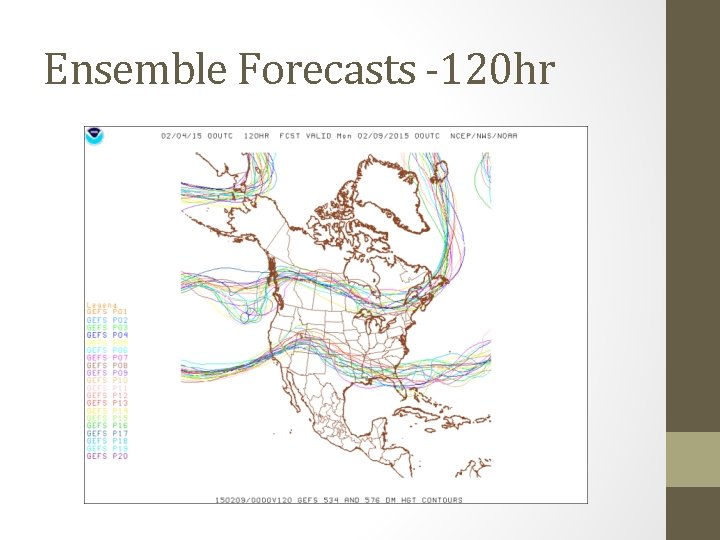
Ensemble Forecasts -120 hr
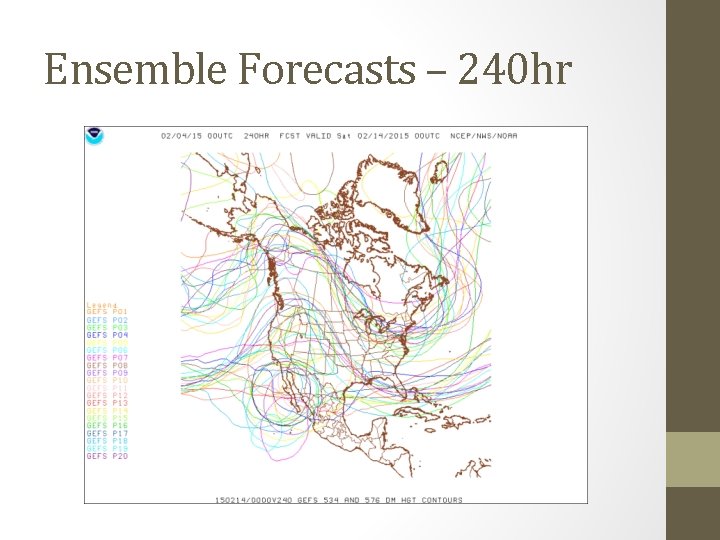
Ensemble Forecasts – 240 hr
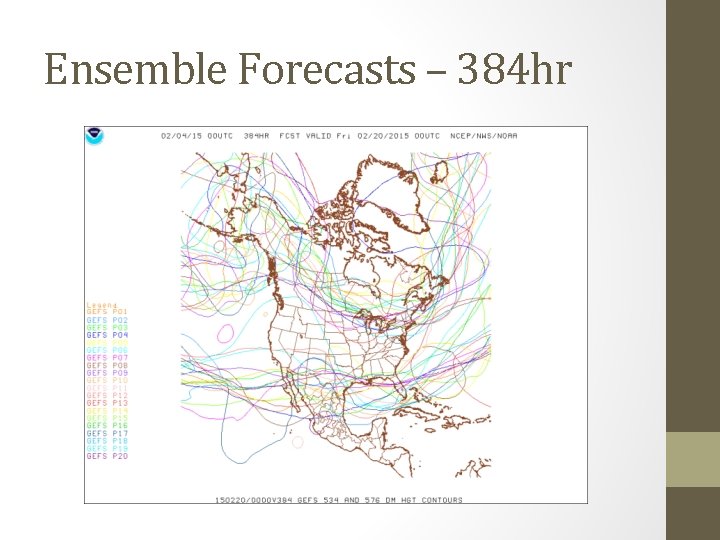
Ensemble Forecasts – 384 hr
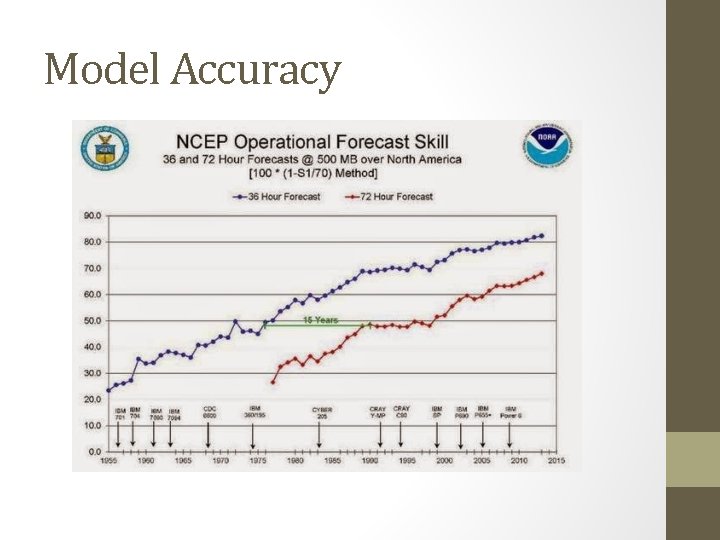
Model Accuracy
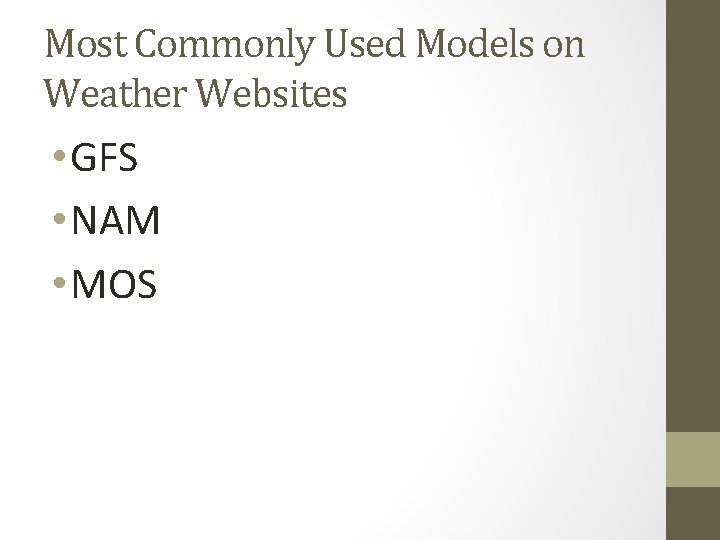
Most Commonly Used Models on Weather Websites • GFS • NAM • MOS
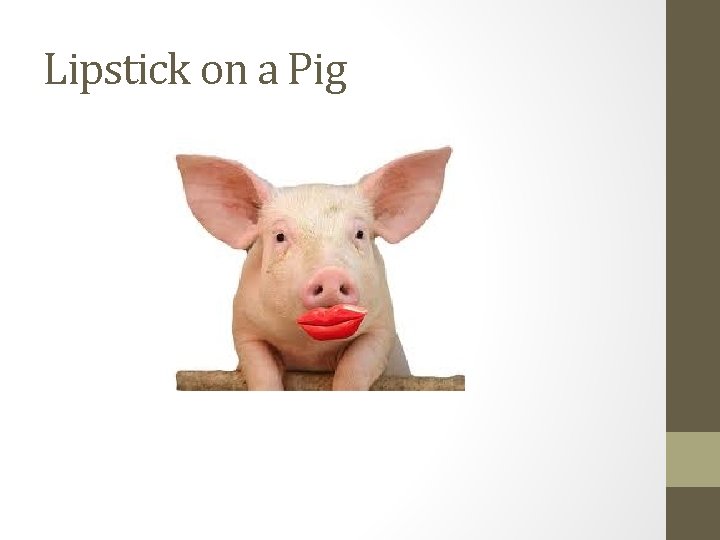
Lipstick on a Pig
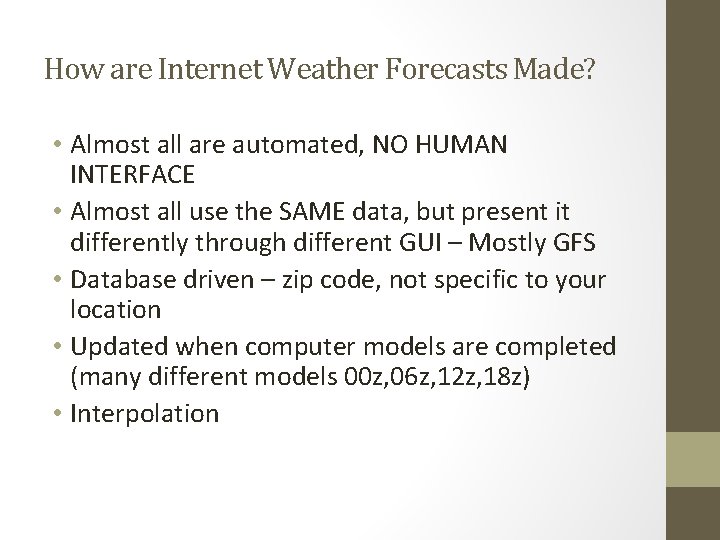
How are Internet Weather Forecasts Made? • Almost all are automated, NO HUMAN INTERFACE • Almost all use the SAME data, but present it differently through different GUI – Mostly GFS • Database driven – zip code, not specific to your location • Updated when computer models are completed (many different models 00 z, 06 z, 12 z, 18 z) • Interpolation
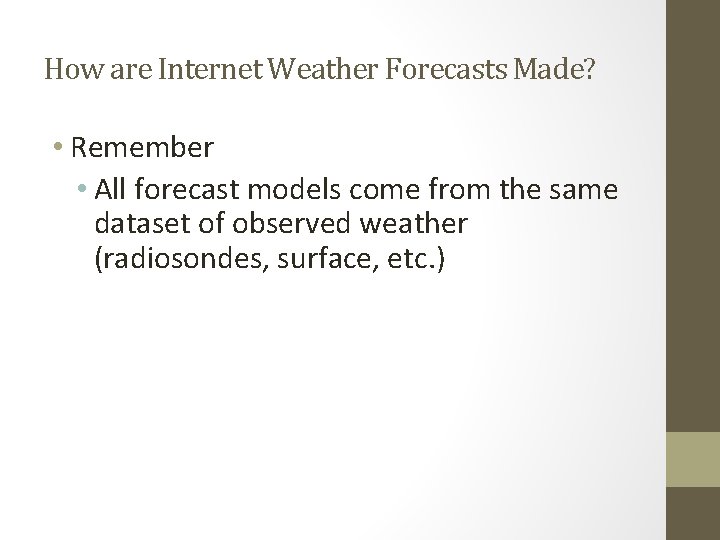
How are Internet Weather Forecasts Made? • Remember • All forecast models come from the same dataset of observed weather (radiosondes, surface, etc. )
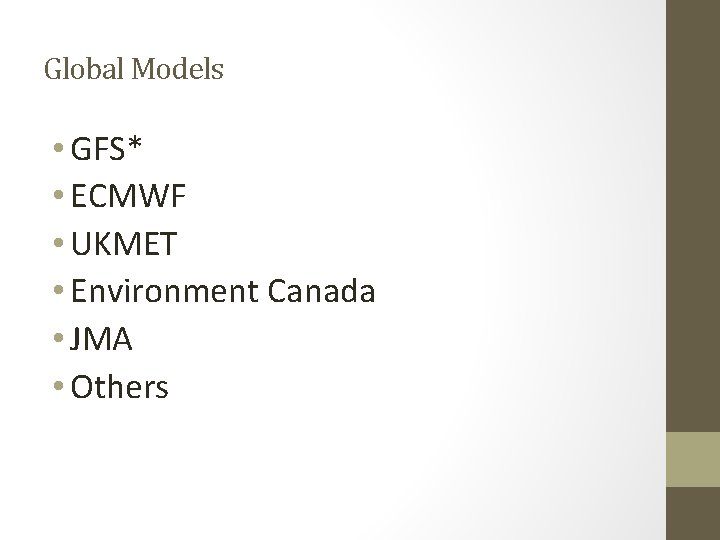
Global Models • GFS* • ECMWF • UKMET • Environment Canada • JMA • Others
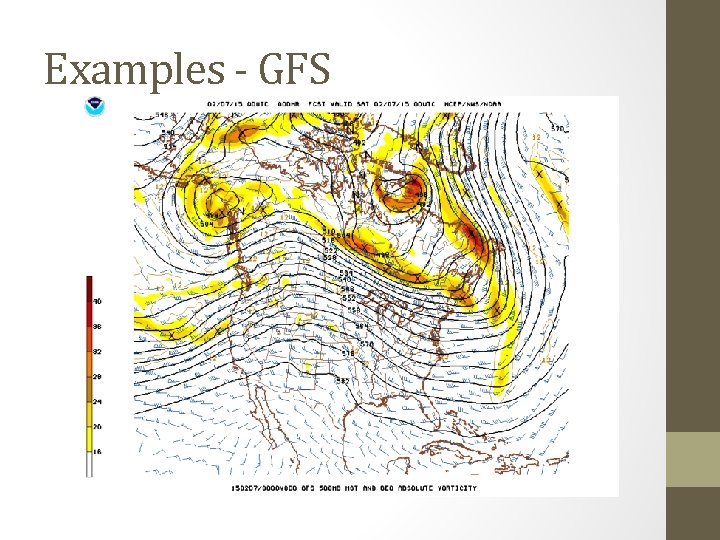
Examples - GFS
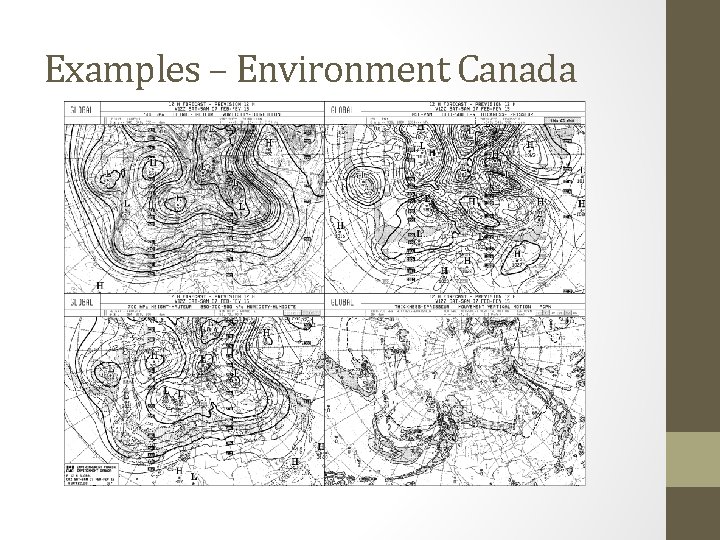
Examples – Environment Canada
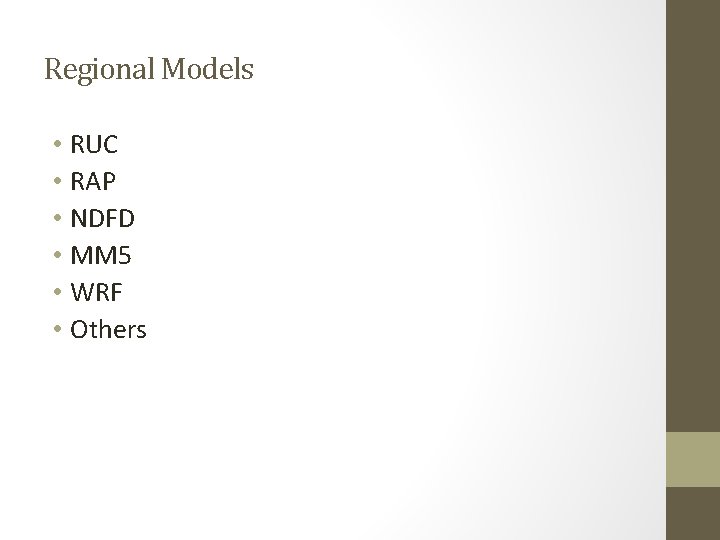
Regional Models • RUC • RAP • NDFD • MM 5 • WRF • Others
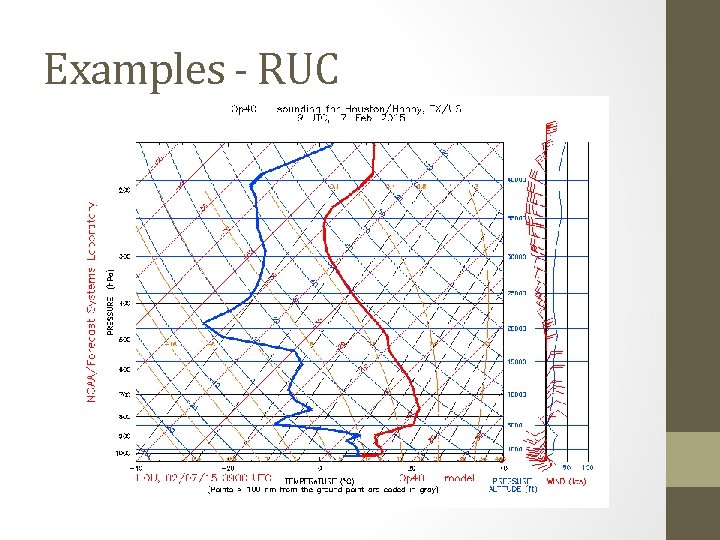
Examples - RUC
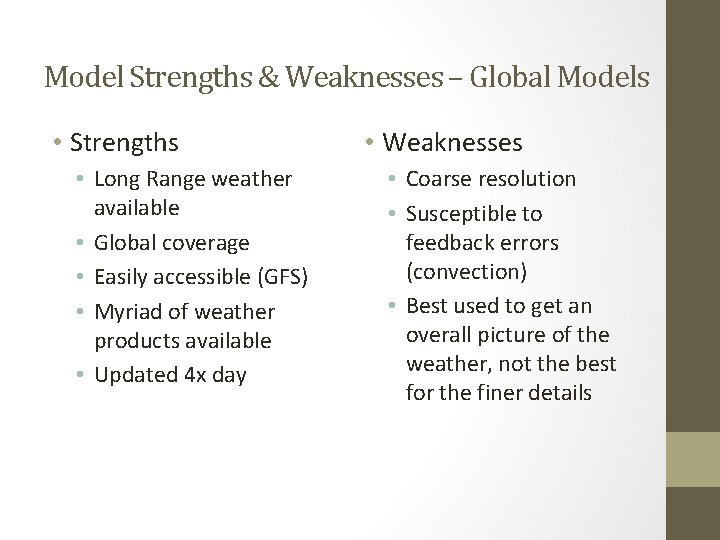
Model Strengths & Weaknesses – Global Models • Strengths • Long Range weather available • Global coverage • Easily accessible (GFS) • Myriad of weather products available • Updated 4 x day • Weaknesses • Coarse resolution • Susceptible to feedback errors (convection) • Best used to get an overall picture of the weather, not the best for the finer details
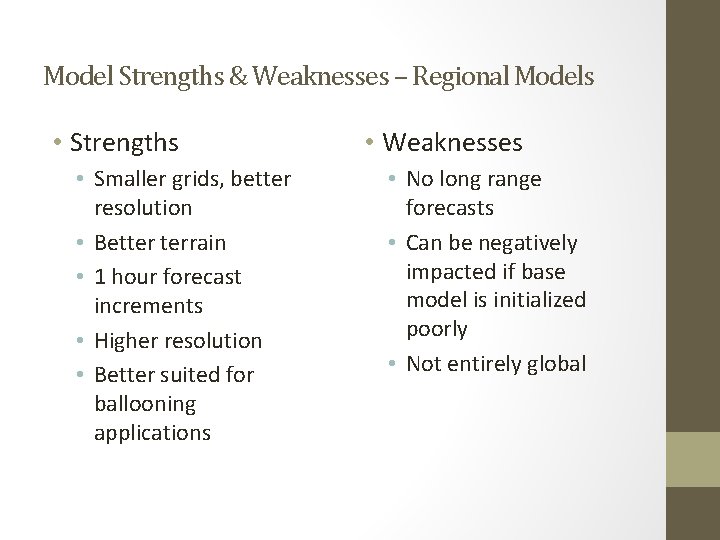
Model Strengths & Weaknesses – Regional Models • Strengths • Smaller grids, better resolution • Better terrain • 1 hour forecast increments • Higher resolution • Better suited for ballooning applications • Weaknesses • No long range forecasts • Can be negatively impacted if base model is initialized poorly • Not entirely global
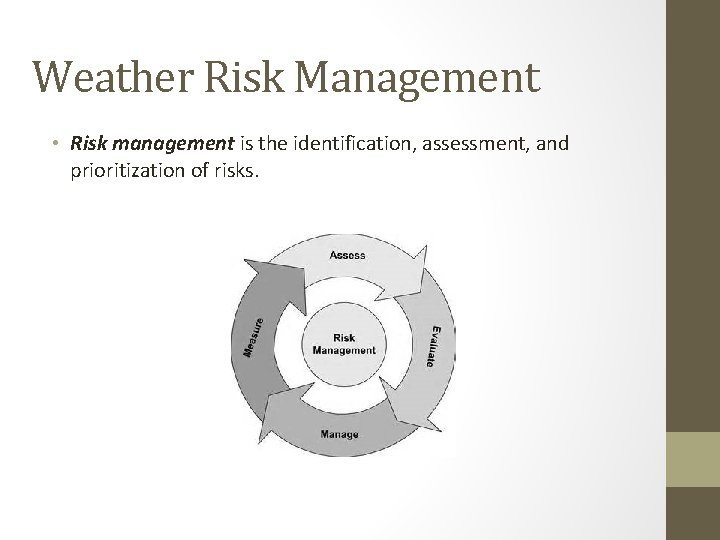
Weather Risk Management • Risk management is the identification, assessment, and prioritization of risks.
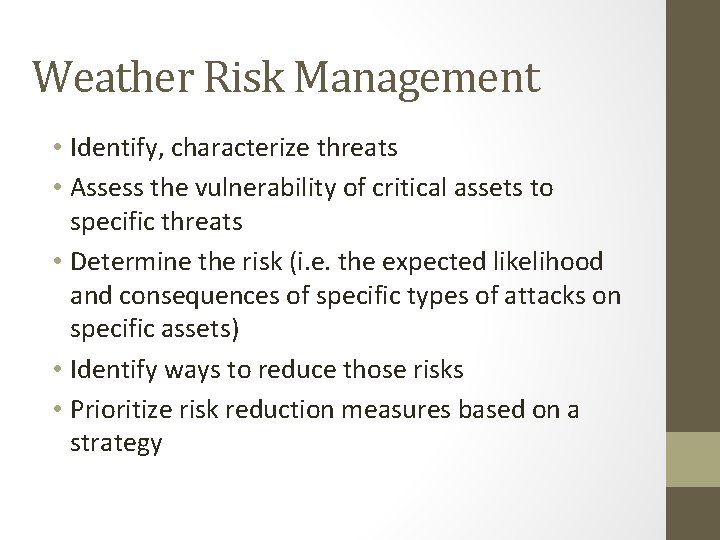
Weather Risk Management • Identify, characterize threats • Assess the vulnerability of critical assets to specific threats • Determine the risk (i. e. the expected likelihood and consequences of specific types of attacks on specific assets) • Identify ways to reduce those risks • Prioritize risk reduction measures based on a strategy
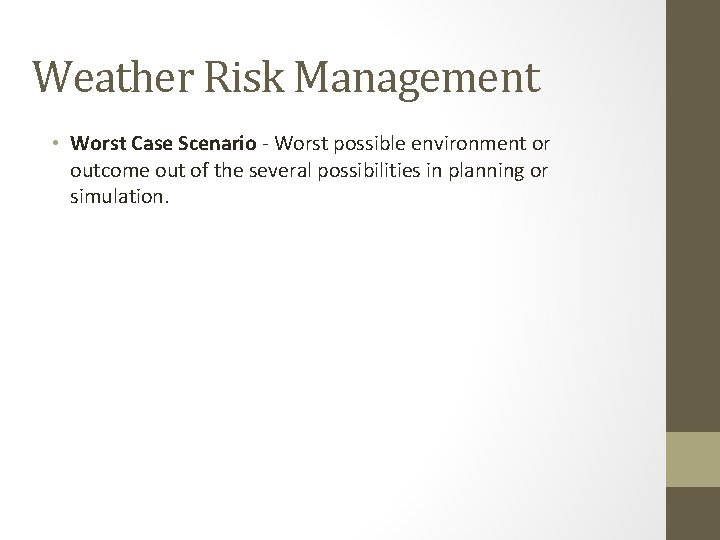
Weather Risk Management • Worst Case Scenario - Worst possible environment or outcome out of the several possibilities in planning or simulation.
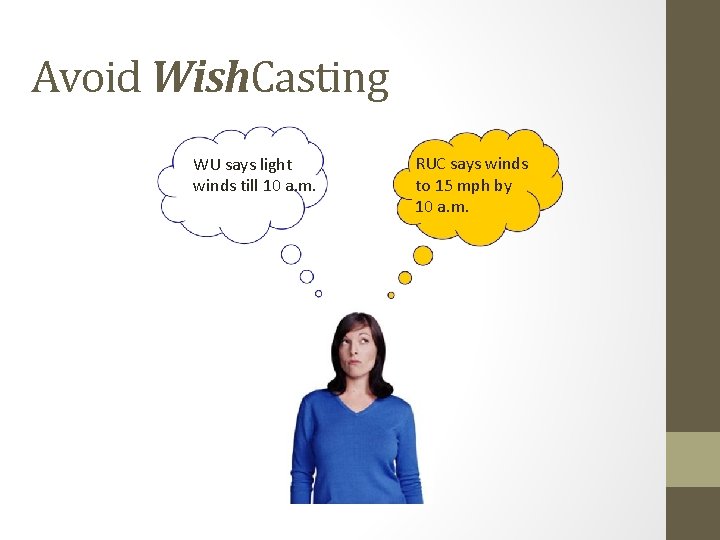
Avoid Wish. Casting WU says light winds till 10 a. m. RUC says winds to 15 mph by 10 a. m.
Nwp imd
Strengths and limitations of adlerian theory
John joan case
We'll weather the weather poem
Capital weather gang weather wall
Its windy gif
Meteorology symbols
Weather and whether
Whether the weather is fine tongue twister
Heavy weather by weather report
Usage rights
Sap crm billing
Minicomputer uses
Usage definition
Slide past simple
Example of semicolon usage
Qnx momentics
Make in present perfect
Trimmomatic usage
Composite usage map
Power bi usage metrics solution template
Hobbit cfg
Glossary of usage worksheet answers
Semicolon examples
Data usage
Reported speech advised
Measurable substantial accessible differentiable actionable
Syntax xml
Present perfect usage
Articles definite
Usage
Activity resource usage model and tactical decision making
Apostrophe placement
Voh cost
Sociocultural segmentation
Now let's see
Doctrine of usage
How to use ola hallengren scripts
Imperfect phrases
Internal usage only
Would rather negative
Web usage mining
Fonction d'usage d'un pont
Tablets for schools to avoid computer addiction
Emphasis through isolation
Common usage problems examples
Next generation access control
I have been doing present perfect continuous
Composite usage map
Uddi usage model
Past simple express
Windchill bulk migrator
Future perfect continuous tense example
Present continuous tense
Composite usage map
"khanacademy.org"
Ryse son of rome system requirements
Dash what is it
Type of text in multimedia
Simple key loader tm
Qlikview license types
Imperfect irregulars