Regional Numerical Weather Prediction NWP Modeling and Verification
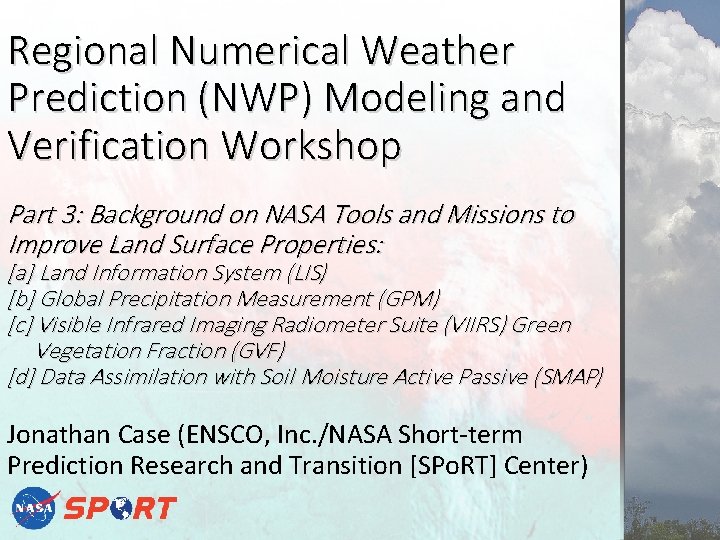
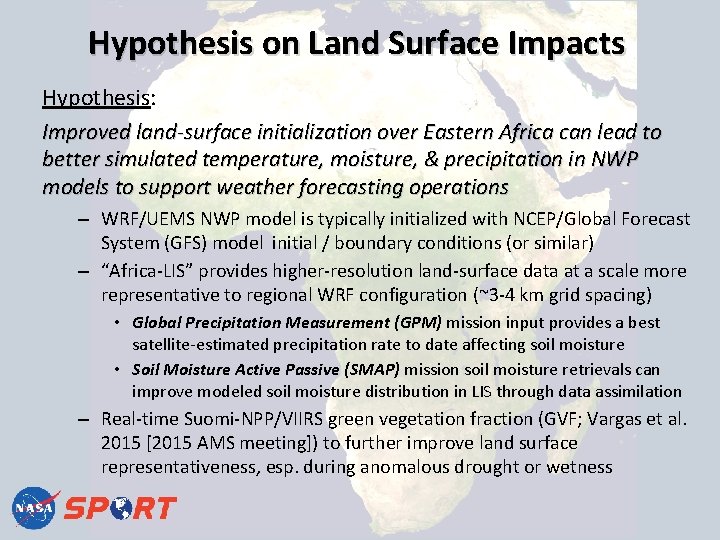
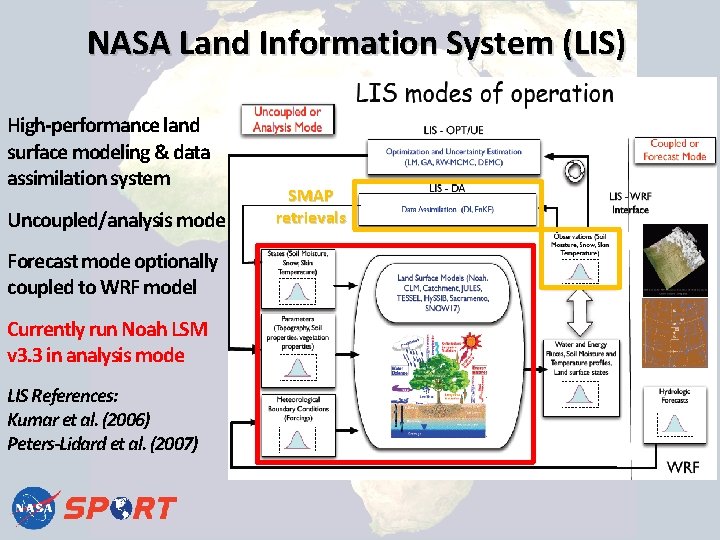
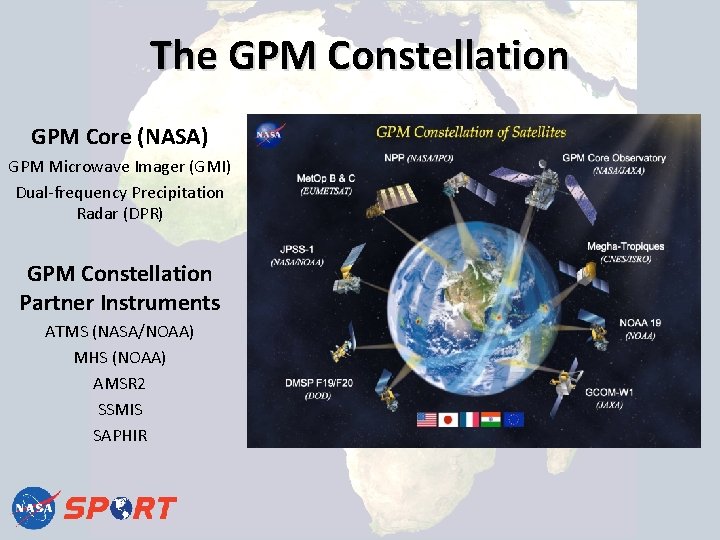
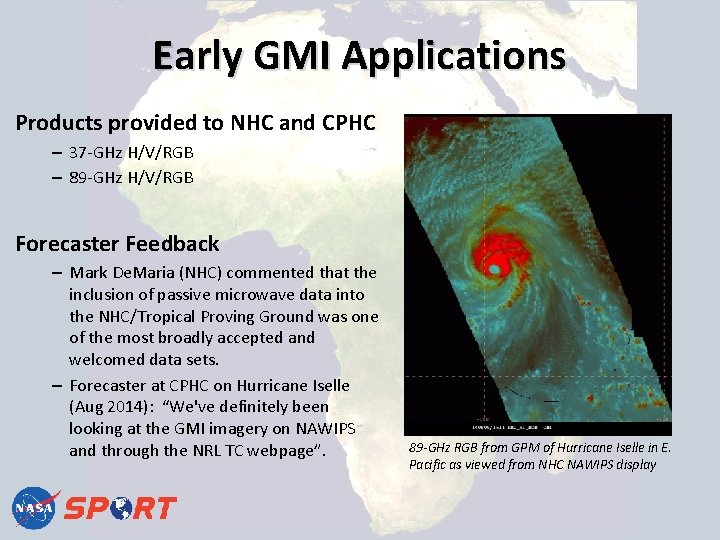
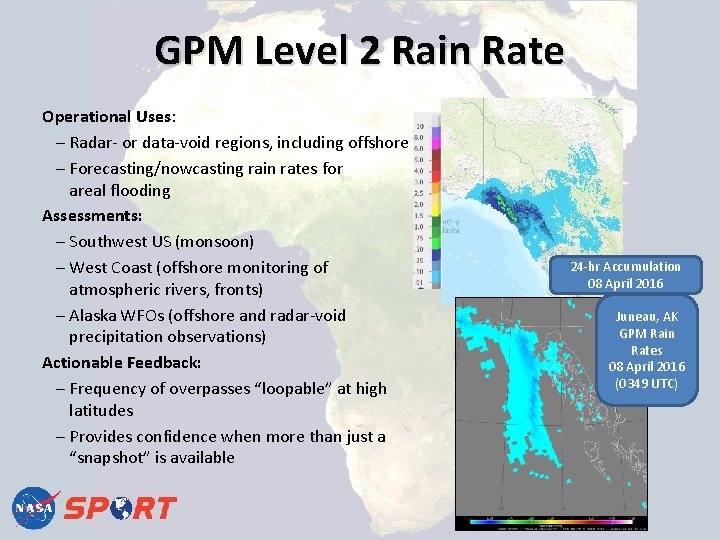
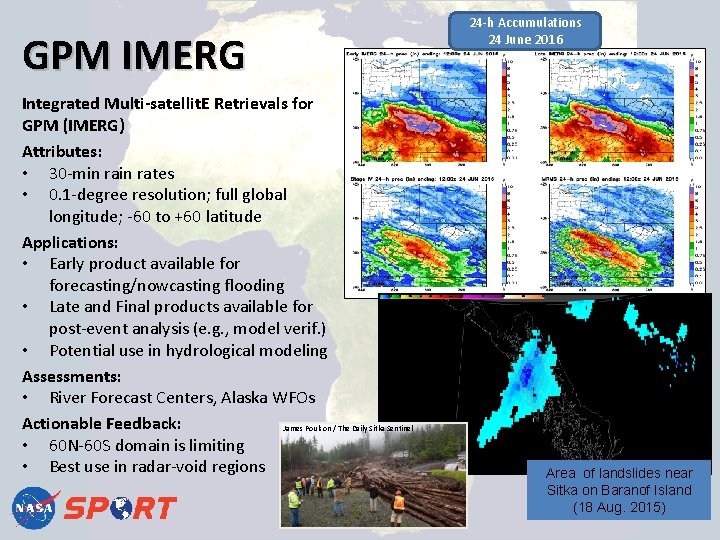
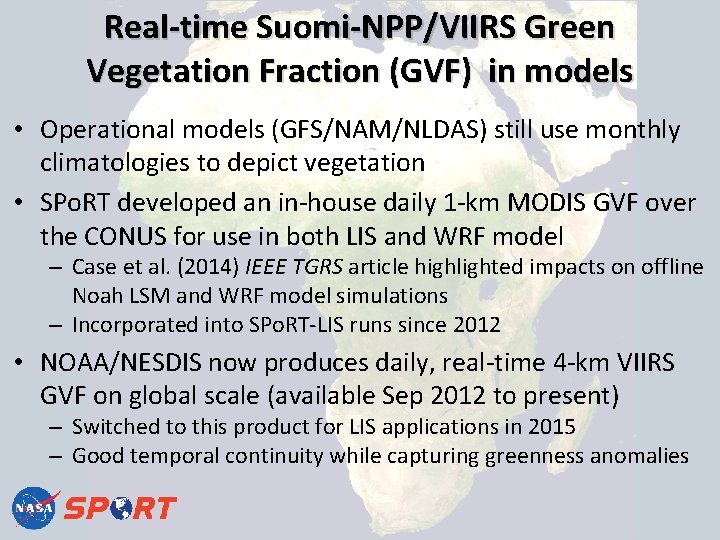
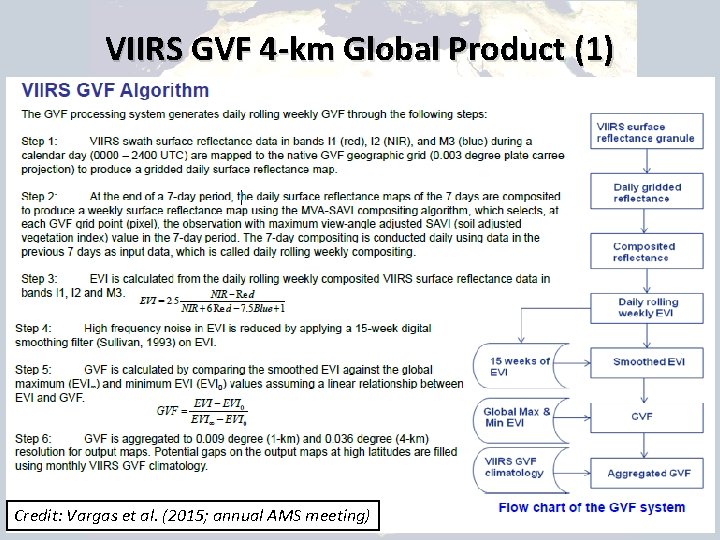
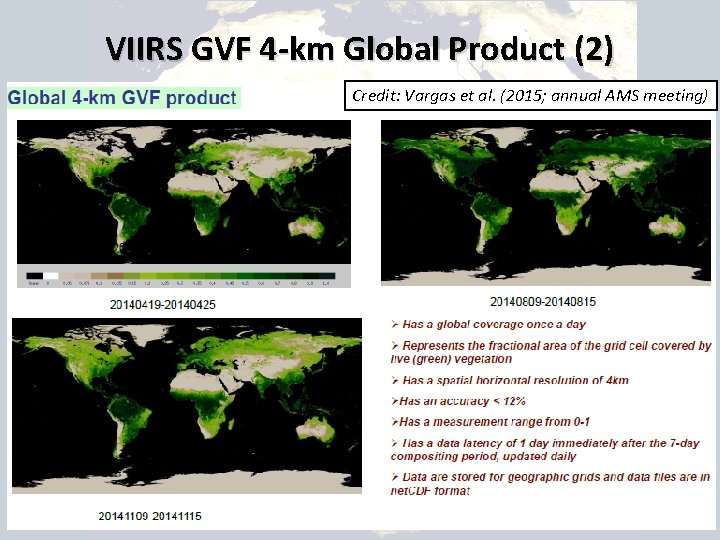
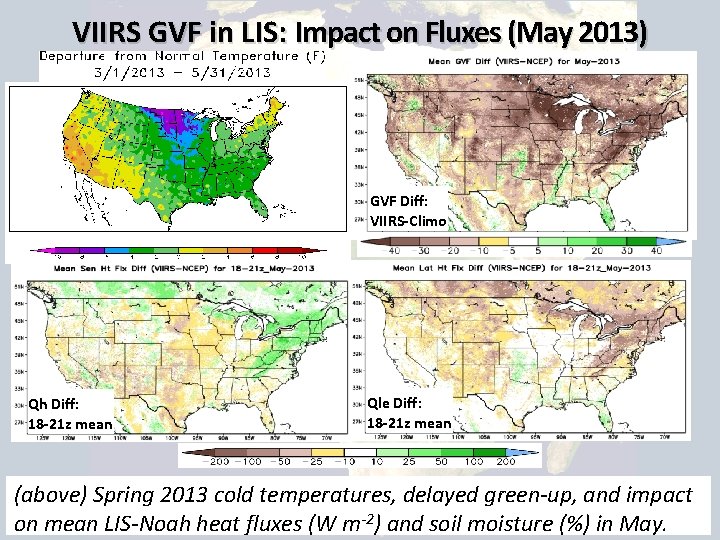
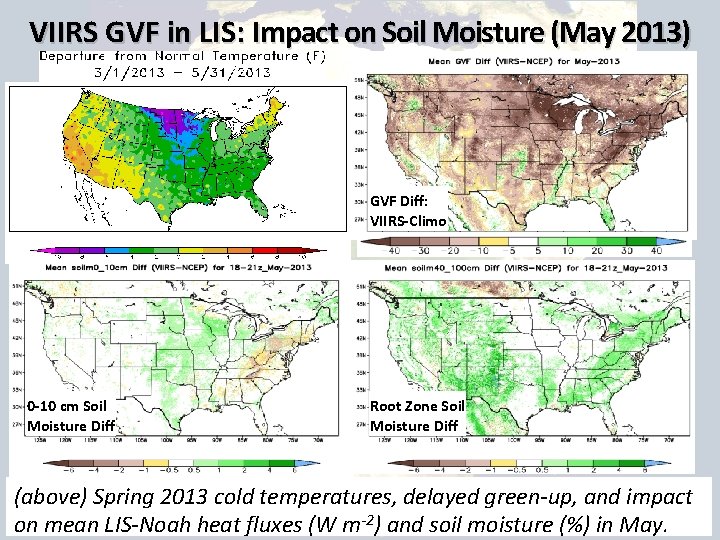
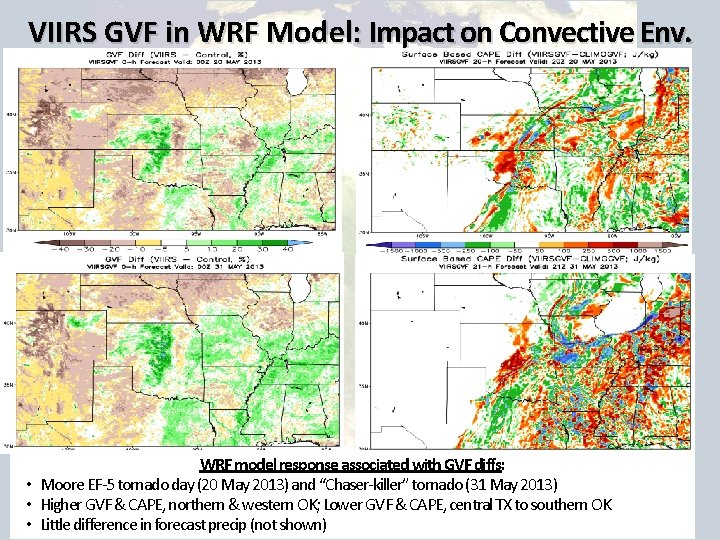
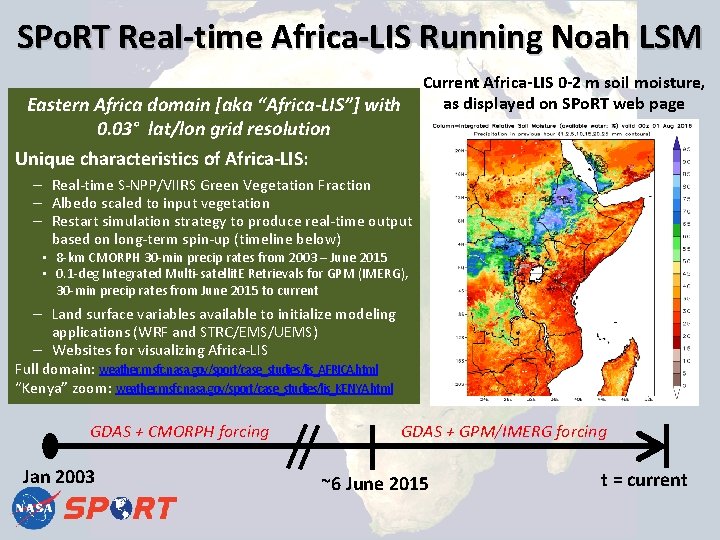
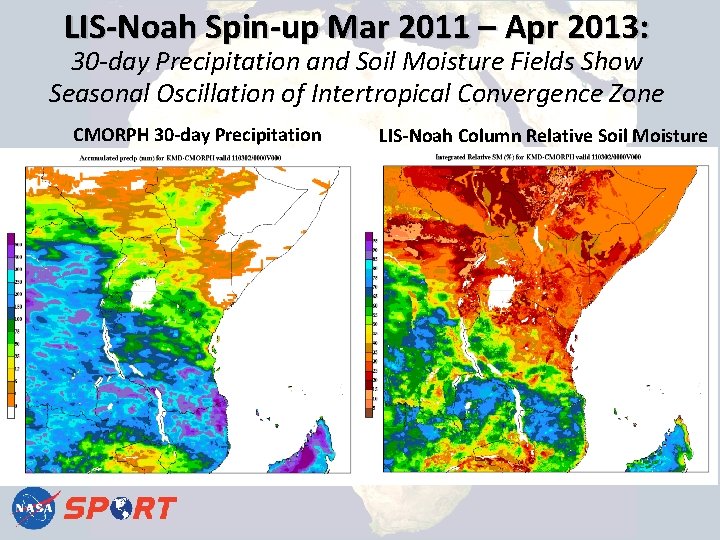
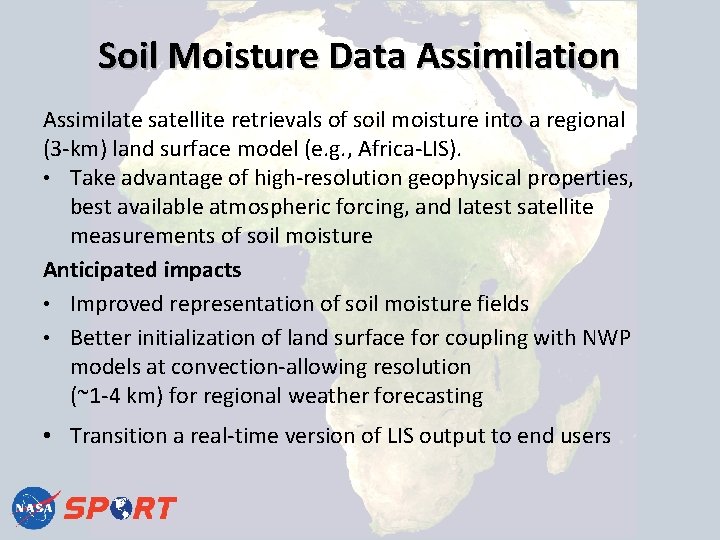
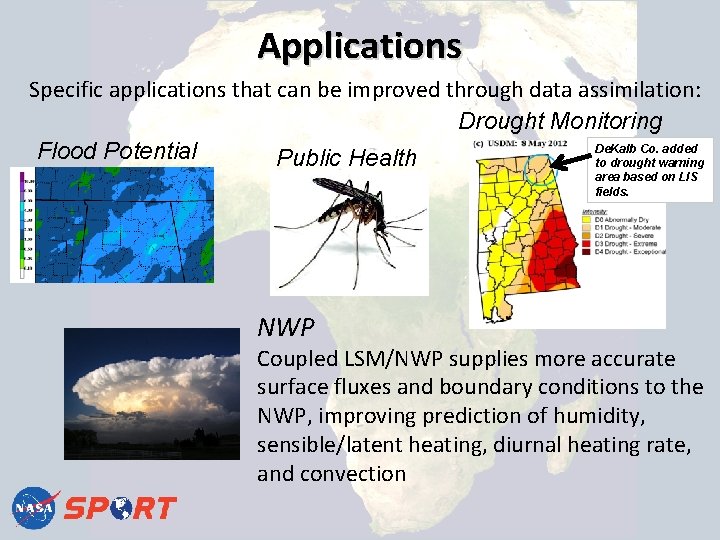
![SPo. RT Work with SMAP [and SMOS] • L-band radiometers (and radars) can Soil SPo. RT Work with SMAP [and SMOS] • L-band radiometers (and radars) can Soil](https://slidetodoc.com/presentation_image_h2/8a36573c1a1fe594246af232a2255bb1/image-18.jpg)
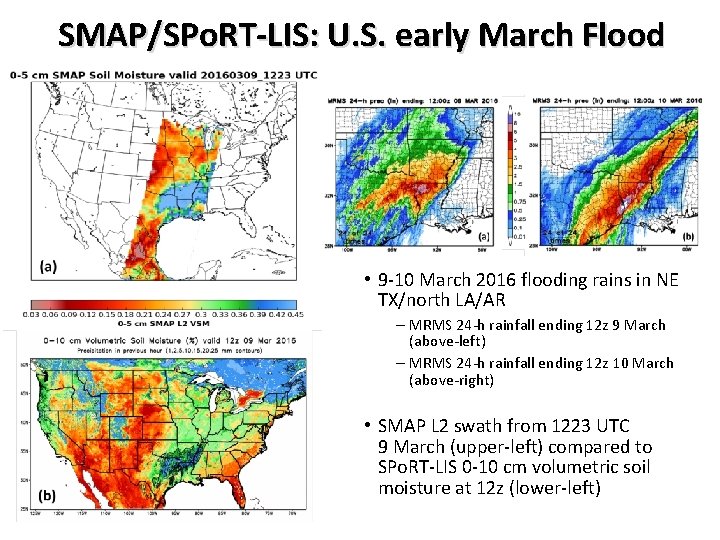
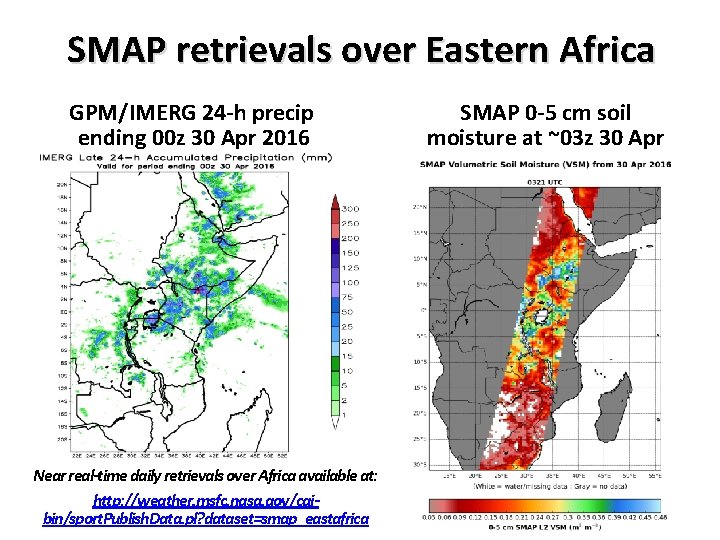
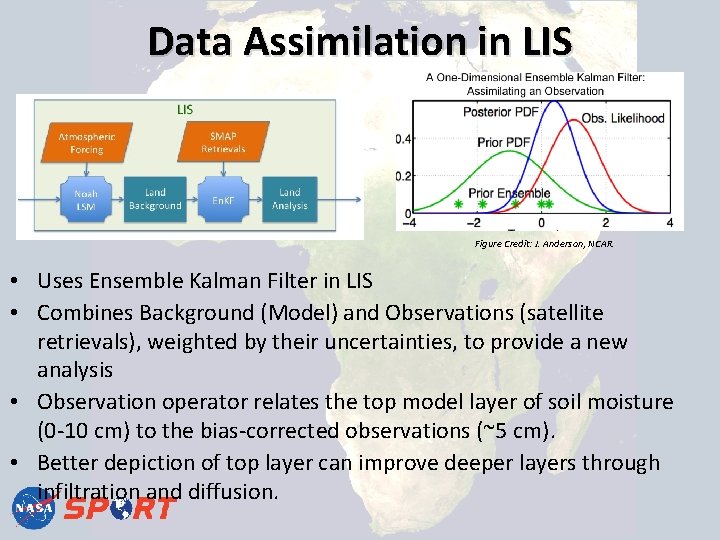
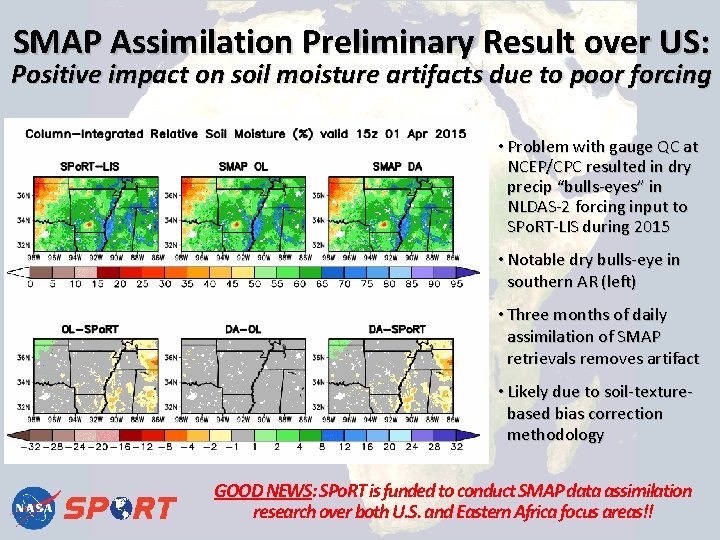
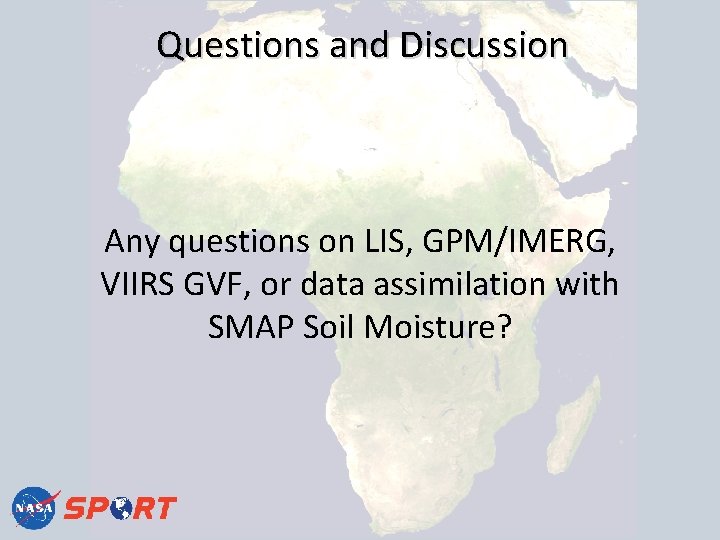
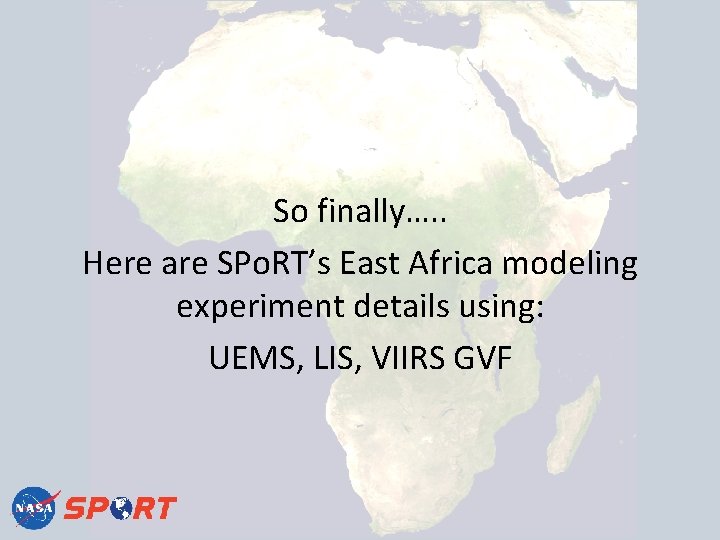
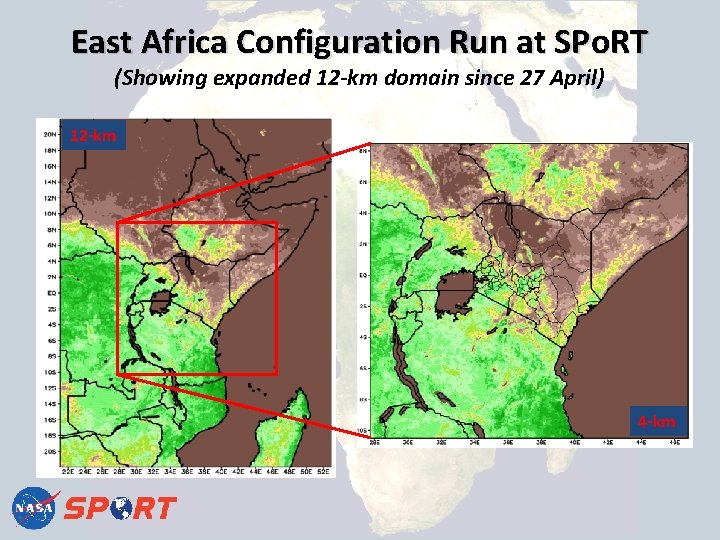
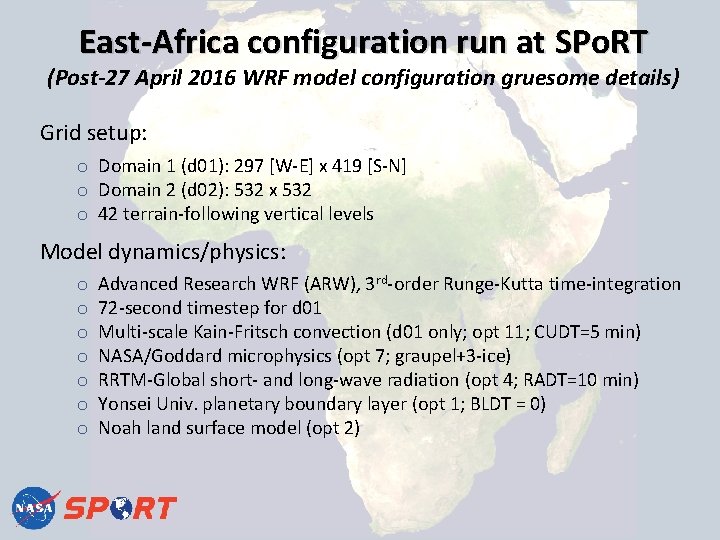
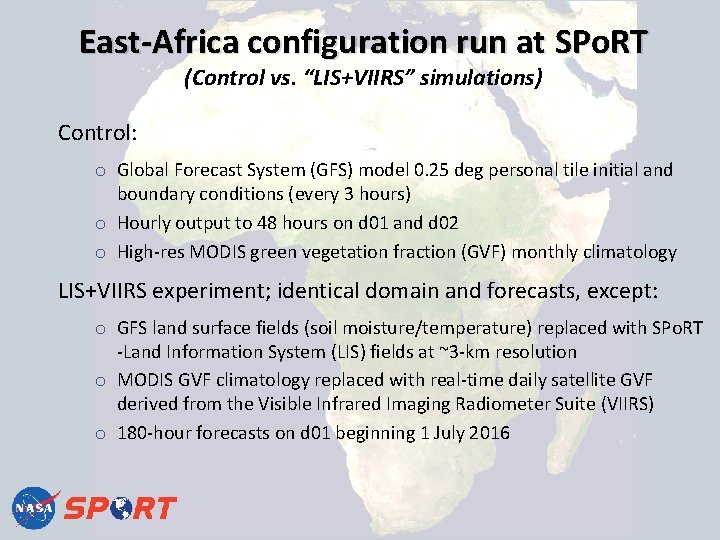
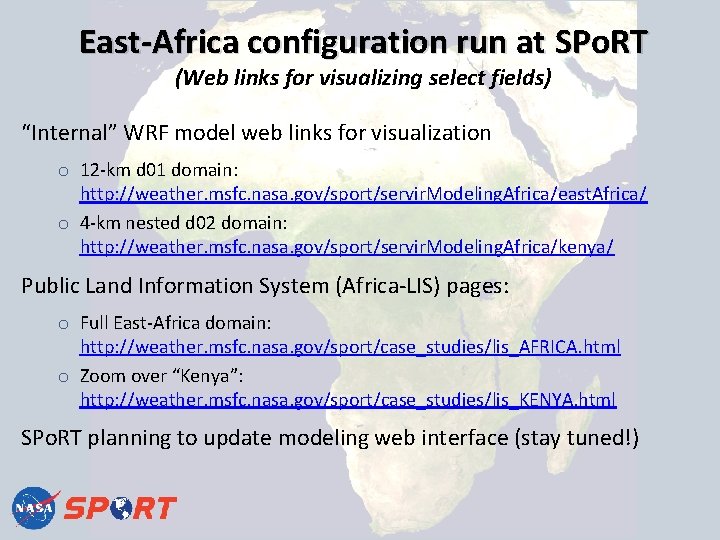
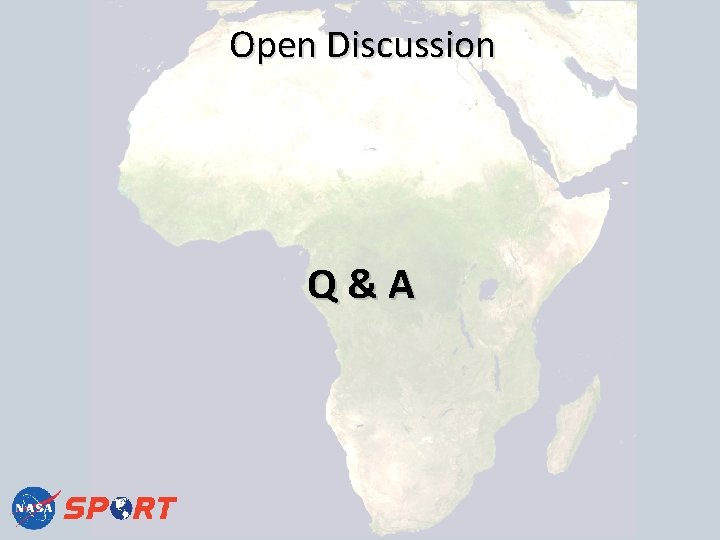
- Slides: 29
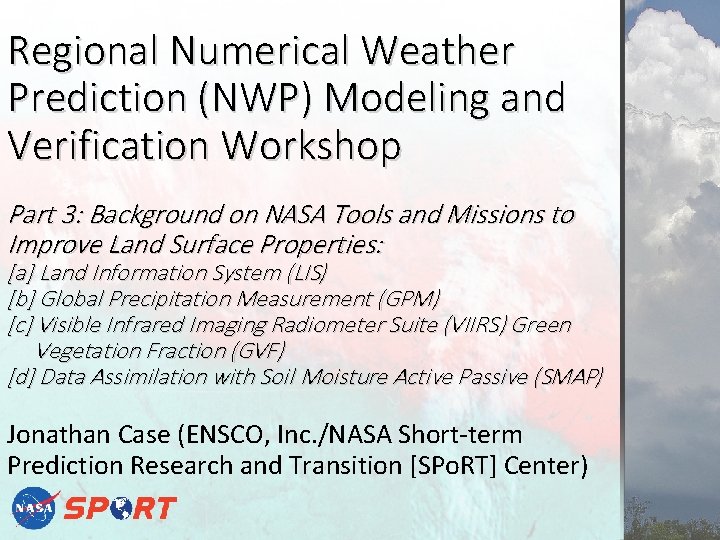
Regional Numerical Weather Prediction (NWP) Modeling and Verification Workshop Part 3: Background on NASA Tools and Missions to Improve Land Surface Properties: [a] Land Information System (LIS) [b] Global Precipitation Measurement (GPM) [c] Visible Infrared Imaging Radiometer Suite (VIIRS) Green Vegetation Fraction (GVF) [d] Data Assimilation with Soil Moisture Active Passive (SMAP) Jonathan Case (ENSCO, Inc. /NASA Short-term Prediction Research and Transition [SPo. RT] Center)
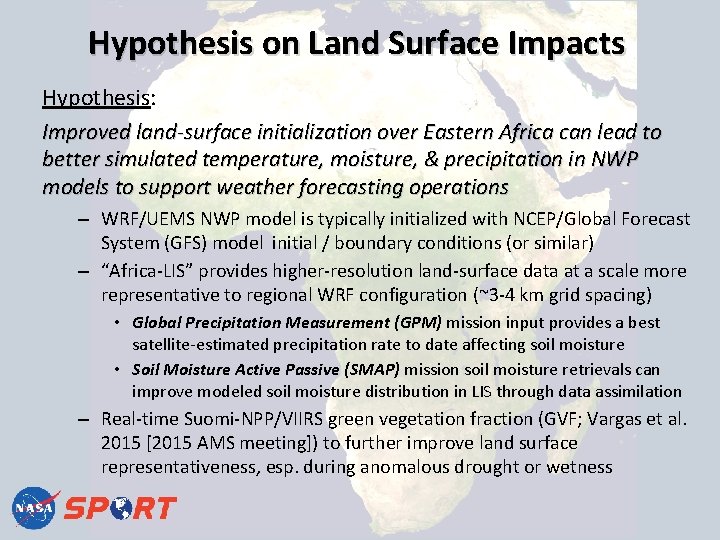
Hypothesis on Land Surface Impacts Hypothesis: Improved land-surface initialization over Eastern Africa can lead to better simulated temperature, moisture, & precipitation in NWP models to support weather forecasting operations – WRF/UEMS NWP model is typically initialized with NCEP/Global Forecast System (GFS) model initial / boundary conditions (or similar) – “Africa-LIS” provides higher-resolution land-surface data at a scale more representative to regional WRF configuration (~3 -4 km grid spacing) • Global Precipitation Measurement (GPM) mission input provides a best satellite-estimated precipitation rate to date affecting soil moisture • Soil Moisture Active Passive (SMAP) mission soil moisture retrievals can improve modeled soil moisture distribution in LIS through data assimilation – Real-time Suomi-NPP/VIIRS green vegetation fraction (GVF; Vargas et al. 2015 [2015 AMS meeting]) to further improve land surface representativeness, esp. during anomalous drought or wetness
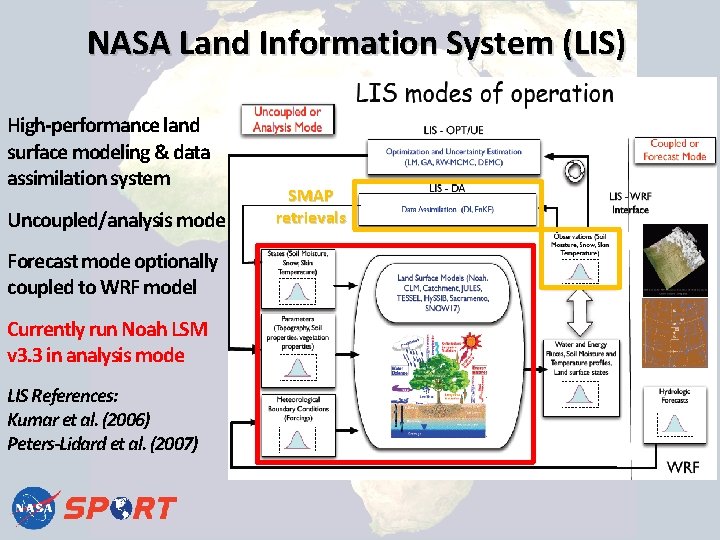
NASA Land Information System (LIS) High-performance land surface modeling & data assimilation system Uncoupled/analysis mode Forecast mode optionally coupled to WRF model Currently run Noah LSM v 3. 3 in analysis mode LIS References: Kumar et al. (2006) Peters-Lidard et al. (2007) SMAP retrievals
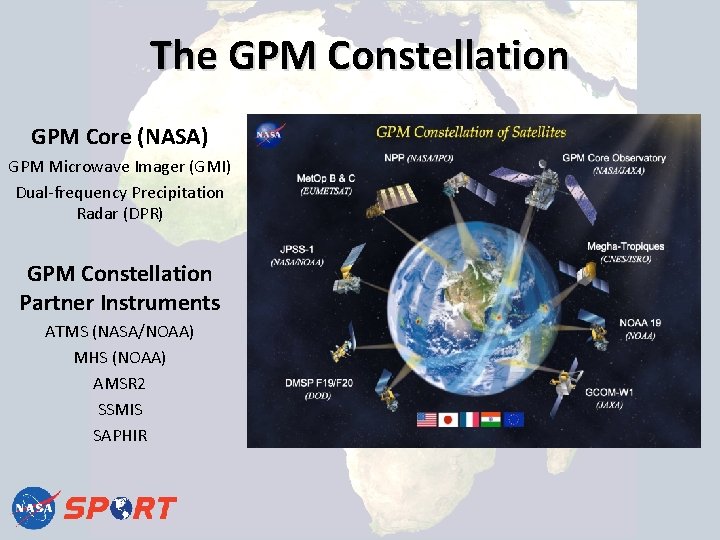
The GPM Constellation GPM Core (NASA) GPM Microwave Imager (GMI) Dual-frequency Precipitation Radar (DPR) GPM Constellation Partner Instruments ATMS (NASA/NOAA) MHS (NOAA) AMSR 2 SSMIS SAPHIR
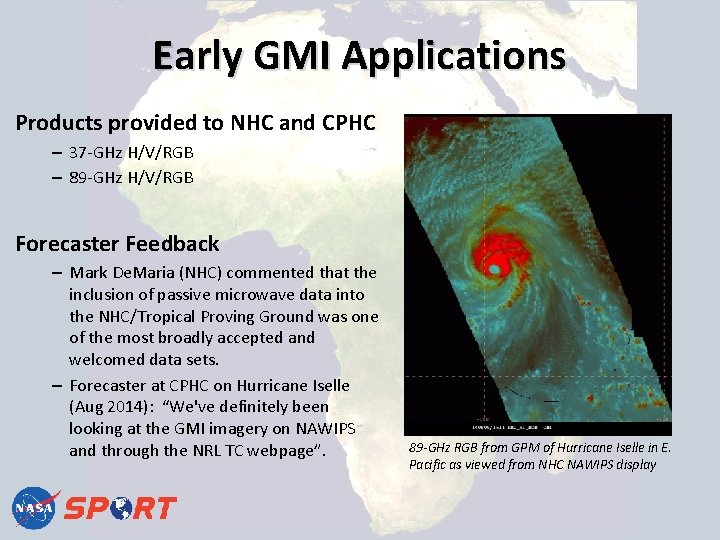
Early GMI Applications Products provided to NHC and CPHC – 37 -GHz H/V/RGB – 89 -GHz H/V/RGB Forecaster Feedback – Mark De. Maria (NHC) commented that the inclusion of passive microwave data into the NHC/Tropical Proving Ground was one of the most broadly accepted and welcomed data sets. – Forecaster at CPHC on Hurricane Iselle (Aug 2014): “We've definitely been looking at the GMI imagery on NAWIPS and through the NRL TC webpage”. 89 -GHz RGB from GPM of Hurricane Iselle in E. Pacific as viewed from NHC NAWIPS display
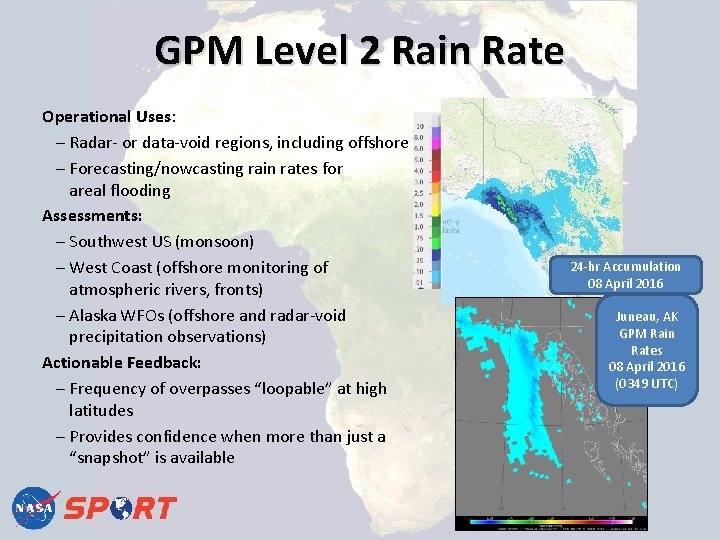
GPM Level 2 Rain Rate Operational Uses: Radar- or data-void regions, including offshore Forecasting/nowcasting rain rates for areal flooding Assessments: Southwest US (monsoon) West Coast (offshore monitoring of atmospheric rivers, fronts) Alaska WFOs (offshore and radar-void precipitation observations) Actionable Feedback: Frequency of overpasses “loopable” at high latitudes Provides confidence when more than just a “snapshot” is available 24 -hr Accumulation 08 April 2016 Juneau, AK GPM Rain Rates 08 April 2016 (0349 UTC)
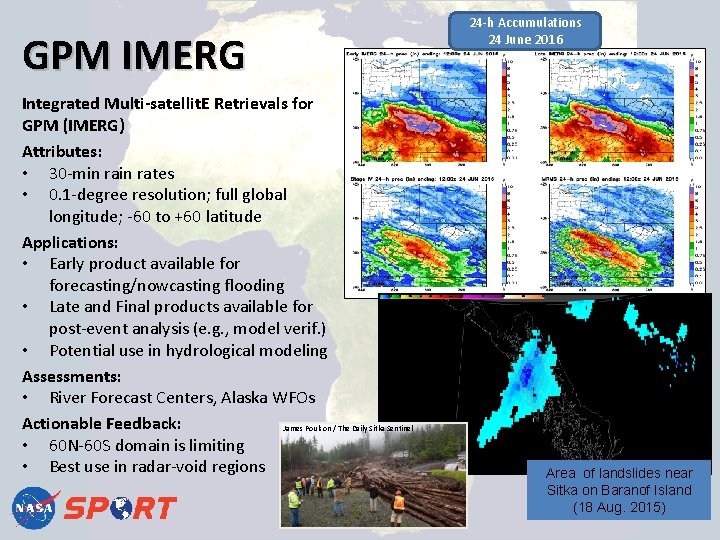
GPM IMERG Integrated Multi-satellit. E Retrievals for GPM (IMERG) Attributes: • 30 -min rates • 0. 1 -degree resolution; full global longitude; -60 to +60 latitude Applications: • Early product available forecasting/nowcasting flooding • Late and Final products available for post-event analysis (e. g. , model verif. ) • Potential use in hydrological modeling Assessments: • River Forecast Centers, Alaska WFOs Actionable Feedback: James Poulson / The Daily Sitka Sentinel • 60 N-60 S domain is limiting • Best use in radar-void regions 24 -h Accumulations 24 June 2016 Area of landslides near Sitka on Baranof Island (18 Aug. 2015)
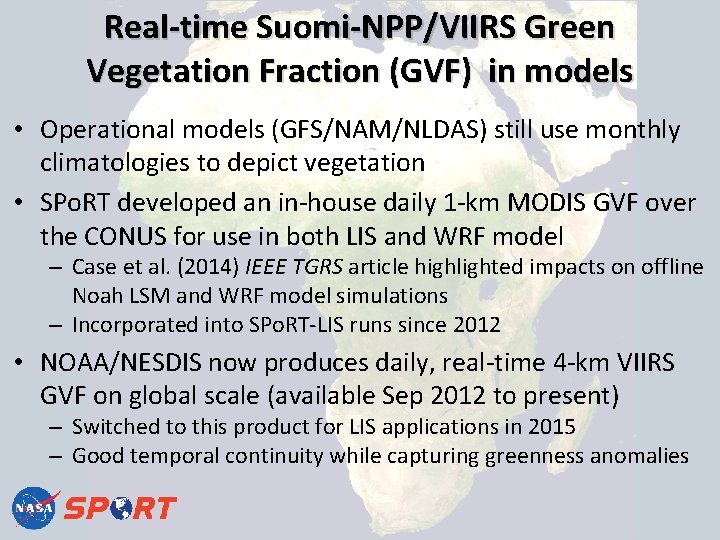
Real-time Suomi-NPP/VIIRS Green Vegetation Fraction (GVF) in models • Operational models (GFS/NAM/NLDAS) still use monthly climatologies to depict vegetation • SPo. RT developed an in-house daily 1 -km MODIS GVF over the CONUS for use in both LIS and WRF model – Case et al. (2014) IEEE TGRS article highlighted impacts on offline Noah LSM and WRF model simulations – Incorporated into SPo. RT-LIS runs since 2012 • NOAA/NESDIS now produces daily, real-time 4 -km VIIRS GVF on global scale (available Sep 2012 to present) – Switched to this product for LIS applications in 2015 – Good temporal continuity while capturing greenness anomalies
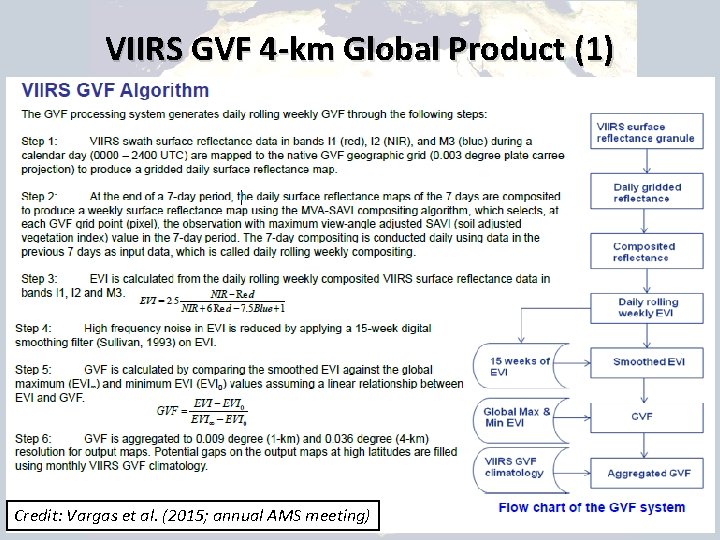
VIIRS GVF 4 -km Global Product (1) Credit: Vargas et al. (2015; annual AMS meeting)
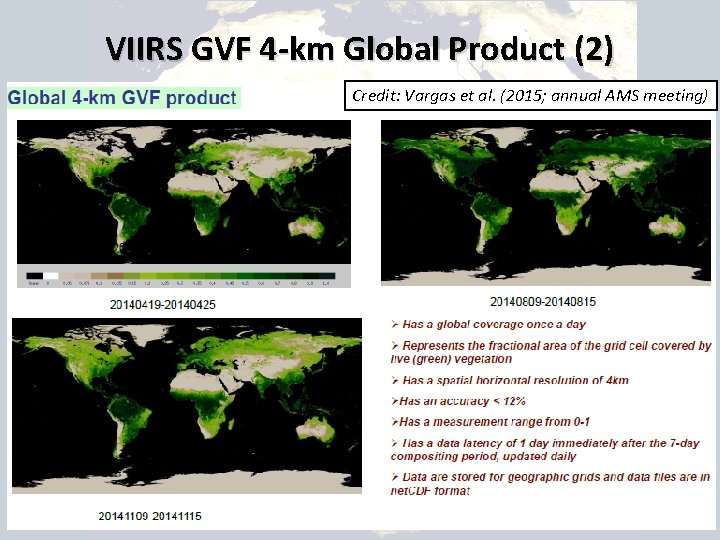
VIIRS GVF 4 -km Global Product (2) Credit: Vargas et al. (2015; annual AMS meeting)
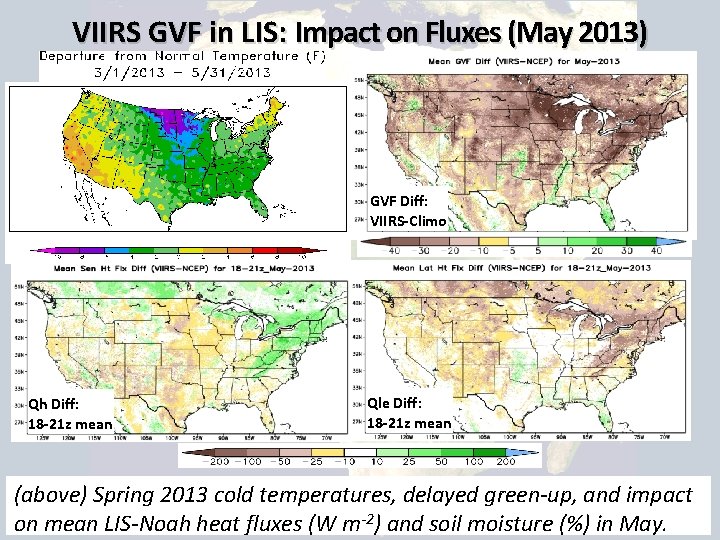
VIIRS GVF in LIS: Impact on Fluxes (May 2013) GVF Diff: VIIRS-Climo Qh Diff: 18 -21 z mean Qle Diff: 18 -21 z mean (above) Spring 2013 cold temperatures, delayed green-up, and impact on mean LIS-Noah heat fluxes (W m-2) and soil moisture (%) in May.
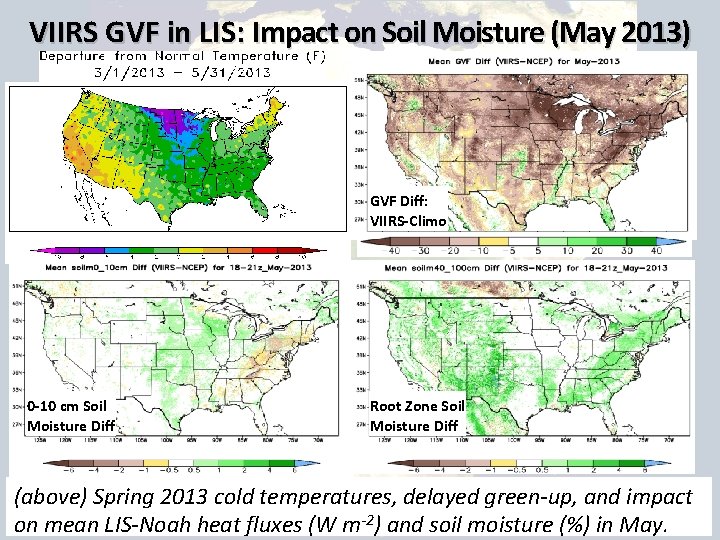
VIIRS GVF in LIS: Impact on Soil Moisture (May 2013) GVF Diff: VIIRS-Climo 0 -10 cm Soil Moisture Diff Root Zone Soil Moisture Diff (above) Spring 2013 cold temperatures, delayed green-up, and impact on mean LIS-Noah heat fluxes (W m-2) and soil moisture (%) in May.
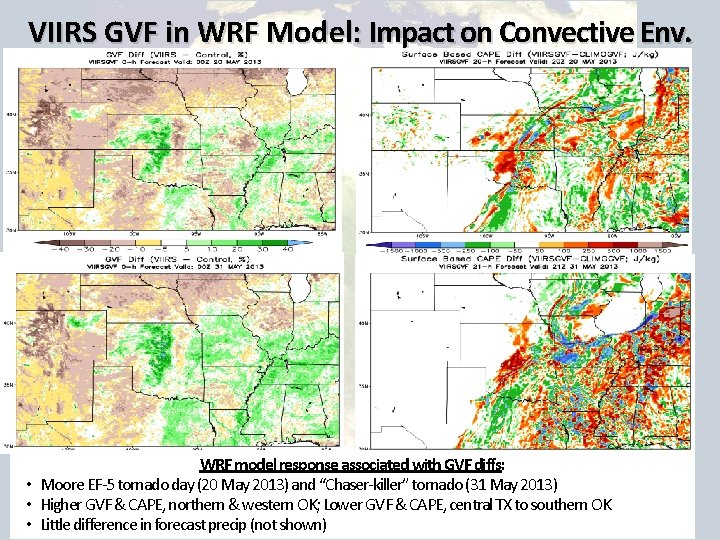
VIIRS GVF in WRF Model: Impact on Convective Env. WRF model response associated with GVF diffs: • Moore EF-5 tornado day (20 May 2013) and “Chaser-killer” tornado (31 May 2013) • Higher GVF & CAPE, northern & western OK; Lower GVF & CAPE, central TX to southern OK • Little difference in forecast precip (not shown)
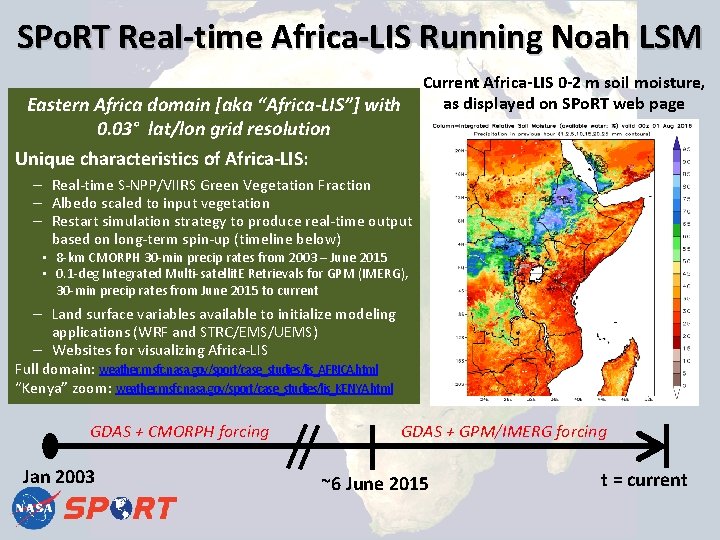
SPo. RT Real-time Africa-LIS Running Noah LSM Current Africa-LIS 0 -2 m soil moisture, as displayed on SPo. RT web page Eastern Africa domain [aka “Africa-LIS”] with 0. 03° lat/lon grid resolution Unique characteristics of Africa-LIS: – Real-time S-NPP/VIIRS Green Vegetation Fraction – Albedo scaled to input vegetation – Restart simulation strategy to produce real-time output based on long-term spin-up (timeline below) • 8 -km CMORPH 30 -min precip rates from 2003 – June 2015 • 0. 1 -deg Integrated Multi-satellit. E Retrievals for GPM (IMERG), 30 -min precip rates from June 2015 to current – Land surface variables available to initialize modeling applications (WRF and STRC/EMS/UEMS) – Websites for visualizing Africa-LIS Full domain: weather. msfc. nasa. gov/sport/case_studies/lis_AFRICA. html “Kenya” zoom: weather. msfc. nasa. gov/sport/case_studies/lis_KENYA. html GDAS + CMORPH forcing Jan 2003 GDAS + GPM/IMERG forcing ~6 June 2015 t = current
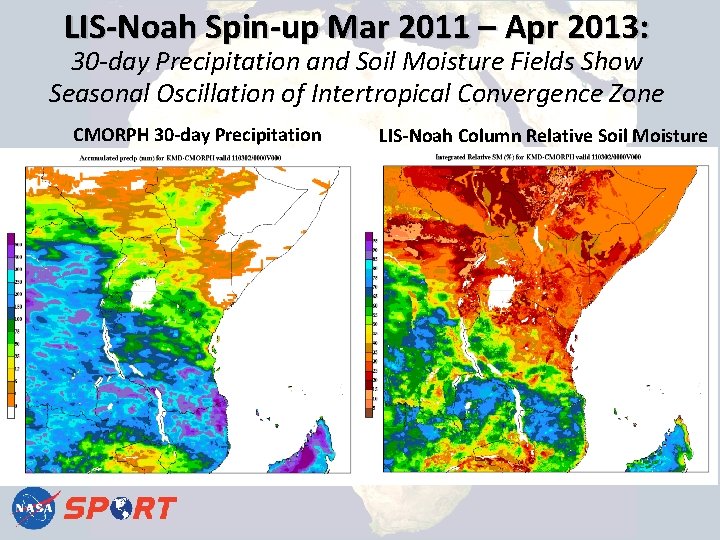
LIS-Noah Spin-up Mar 2011 – Apr 2013: 30 -day Precipitation and Soil Moisture Fields Show Seasonal Oscillation of Intertropical Convergence Zone CMORPH 30 -day Precipitation LIS-Noah Column Relative Soil Moisture
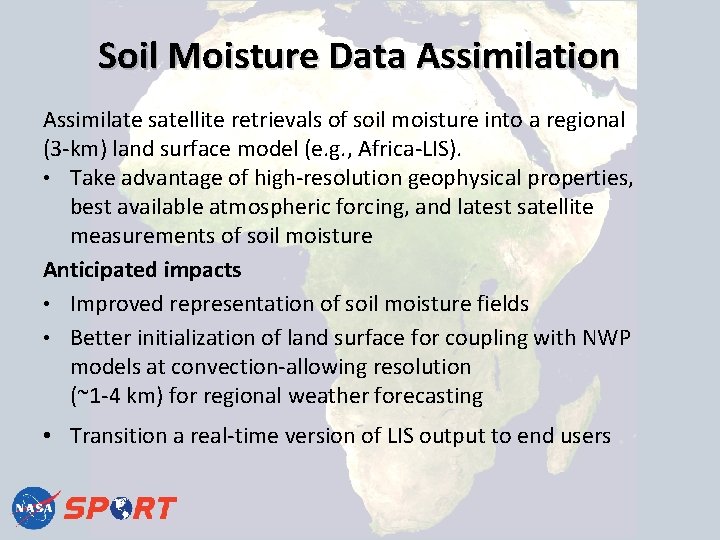
Soil Moisture Data Assimilation Assimilate satellite retrievals of soil moisture into a regional (3 -km) land surface model (e. g. , Africa-LIS). • Take advantage of high-resolution geophysical properties, best available atmospheric forcing, and latest satellite measurements of soil moisture Anticipated impacts • Improved representation of soil moisture fields • Better initialization of land surface for coupling with NWP models at convection-allowing resolution (~1 -4 km) for regional weather forecasting • Transition a real-time version of LIS output to end users
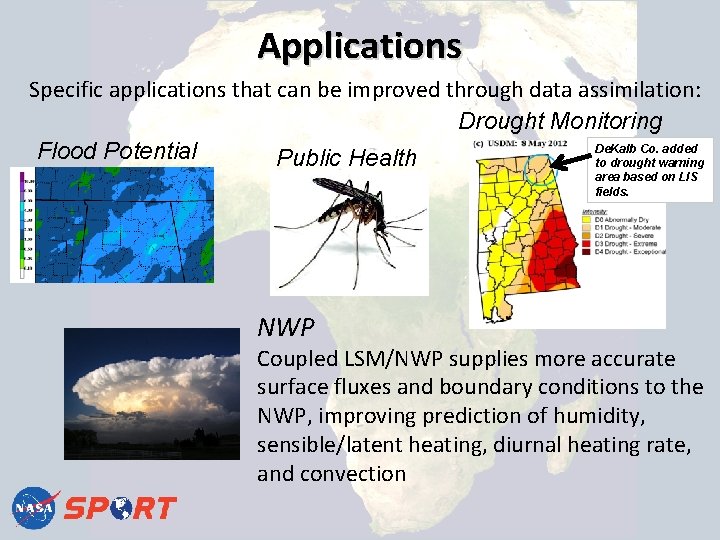
Applications Specific applications that can be improved through data assimilation: Drought Monitoring De. Kalb Co. added Flood Potential Public Health to drought warning area based on LIS fields. NWP Coupled LSM/NWP supplies more accurate surface fluxes and boundary conditions to the NWP, improving prediction of humidity, sensible/latent heating, diurnal heating rate, and convection
![SPo RT Work with SMAP and SMOS Lband radiometers and radars can Soil SPo. RT Work with SMAP [and SMOS] • L-band radiometers (and radars) can Soil](https://slidetodoc.com/presentation_image_h2/8a36573c1a1fe594246af232a2255bb1/image-18.jpg)
SPo. RT Work with SMAP [and SMOS] • L-band radiometers (and radars) can Soil Moisture and Ocean Salinity be used to estimate soil moisture near the surface SMOS – Compared to higher frequency instruments: o Sees deeper in the soil (~1 -5 cm) o Better vegetation penetration o Higher sensitivity (accuracy) o Larger footprint (~36 km) • Tested retrievals from Soil Moisture and Ocean Salinity (SMOS) satellite – Paper accepted in IEEE TGRS • Implementing assimilation of NASA Soil Moisture Active Passive (SMAP) retrievals – SMAP has higher resolution product but due to failure of radar, time period is limited to a few months. Name AMSR-E Soil Moisture Active/Passive SMAP SMOS SMAP Agency NASA/JAXA ESA NASA Launch 2002 2009 Jan. 2015 Orbit Polar Sensor Type Passive Active (Failed July 2015) Frequency 6. 9 GHz (C-band) 1. 4 GHz (L-band) 1. 41 GHz 1. 2 GHz Resolution 56 km 35 -50 km 36 km 3 km 9 km Accuracy 6 cm 3/cm 3 4 cm 3/cm 3 6 cm 3/c m 3 4 cm 3/cm 3 Combined (limited duration)
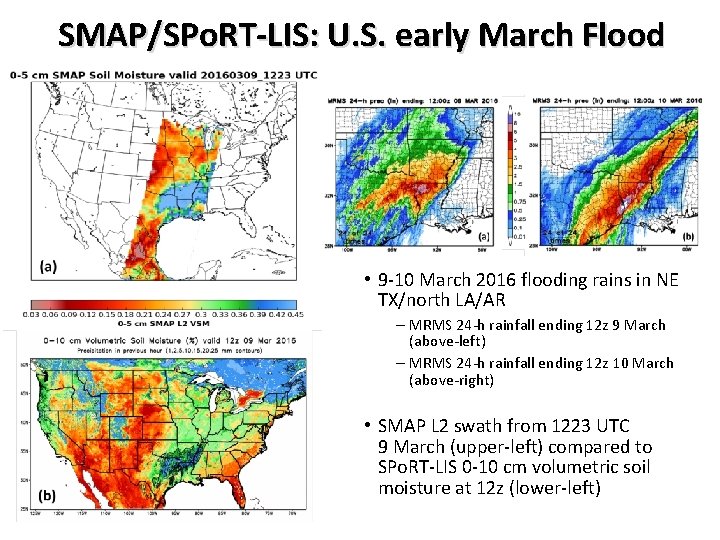
SMAP/SPo. RT-LIS: U. S. early March Flood SMOS • 9 -10 March 2016 flooding rains in NE TX/north LA/AR – MRMS 24 -h rainfall ending 12 z 9 March (above-left) – MRMS 24 -h rainfall ending 12 z 10 March (above-right) • SMAP L 2 swath from 1223 UTC 9 March (upper-left) compared to SPo. RT-LIS 0 -10 cm volumetric soil moisture at 12 z (lower-left)
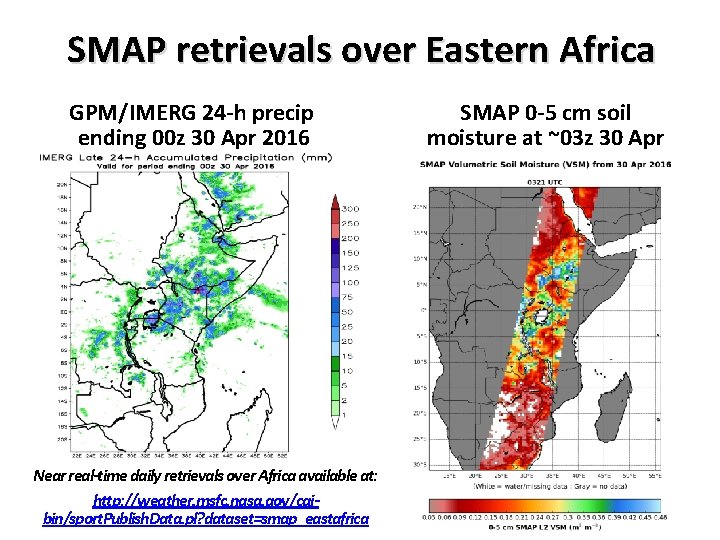
SMAP retrievals over Eastern Africa GPM/IMERG 24 -h precip ending 00 z 30 Apr 2016 Near real-time daily retrievals over Africa available at: http: //weather. msfc. nasa. gov/cgibin/sport. Publish. Data. pl? dataset=smap_eastafrica SMAP 0 -5 cm soil moisture at ~03 z 30 Apr
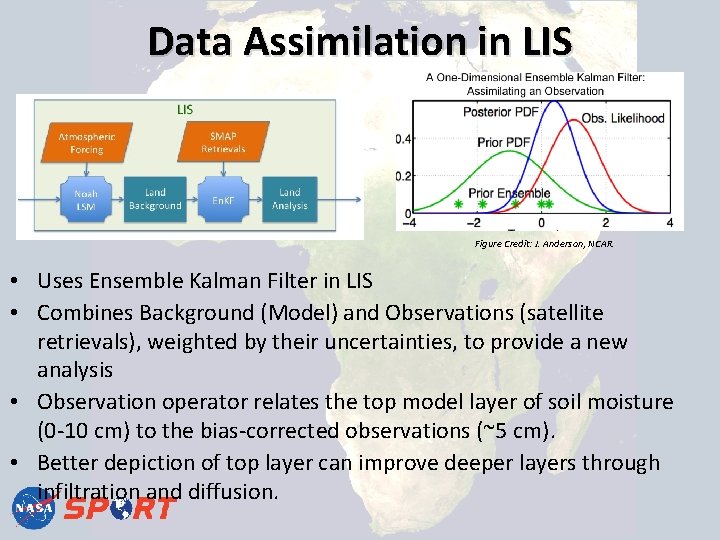
Data Assimilation in LIS Figure Credit: J. Anderson, NCAR. • Uses Ensemble Kalman Filter in LIS • Combines Background (Model) and Observations (satellite retrievals), weighted by their uncertainties, to provide a new analysis • Observation operator relates the top model layer of soil moisture (0 -10 cm) to the bias-corrected observations (~5 cm). • Better depiction of top layer can improve deeper layers through infiltration and diffusion.
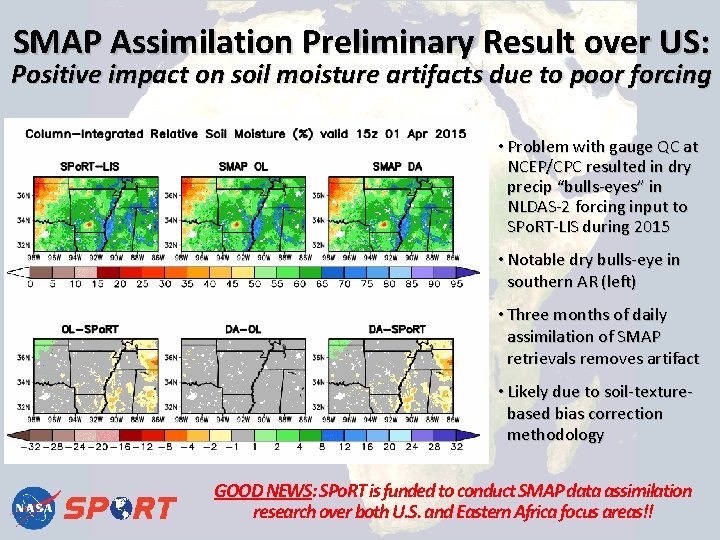
SMAP Assimilation Preliminary Result over US: Positive impact on soil moisture artifacts due to poor forcing • Problem with gauge QC at NCEP/CPC resulted in dry precip “bulls-eyes” in NLDAS-2 forcing input to SPo. RT-LIS during 2015 • Notable dry bulls-eye in southern AR (left) • Three months of daily assimilation of SMAP retrievals removes artifact • Likely due to soil-texturebased bias correction methodology GOOD NEWS: SPo. RT is funded to conduct SMAP data assimilation research over both U. S. and Eastern Africa focus areas!!
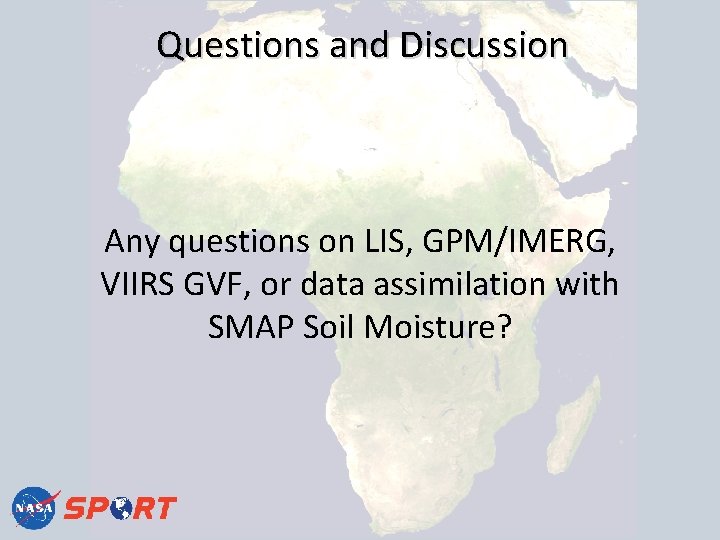
Questions and Discussion Any questions on LIS, GPM/IMERG, VIIRS GVF, or data assimilation with SMAP Soil Moisture?
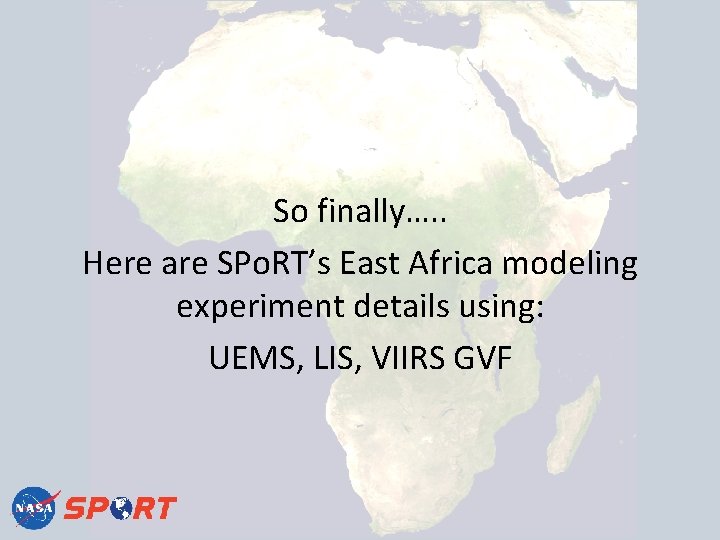
So finally…. . Here are SPo. RT’s East Africa modeling experiment details using: UEMS, LIS, VIIRS GVF
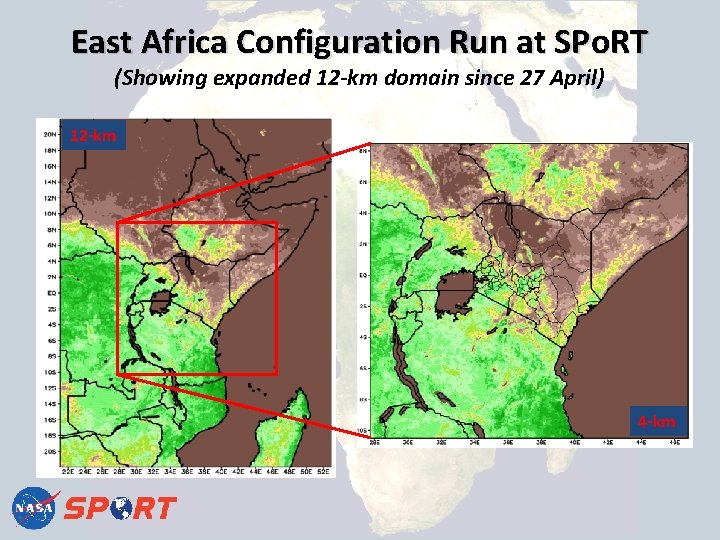
East Africa Configuration Run at SPo. RT (Showing expanded 12 -km domain since 27 April) 12 -km 4 -km
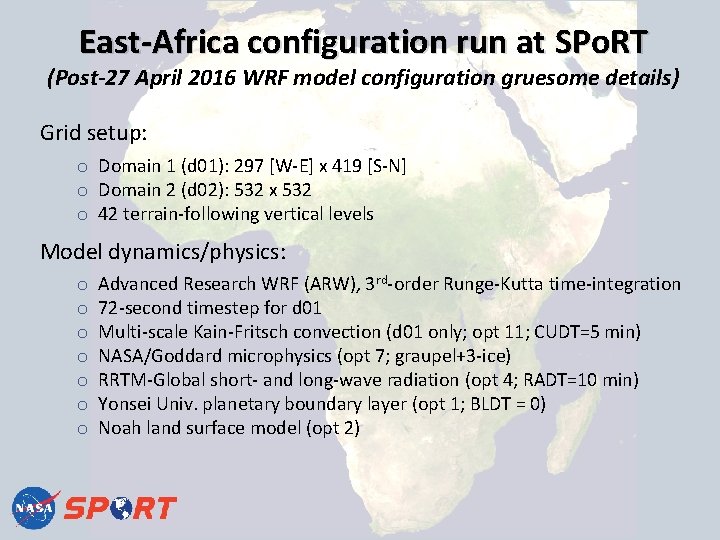
East-Africa configuration run at SPo. RT (Post-27 April 2016 WRF model configuration gruesome details) Grid setup: o Domain 1 (d 01): 297 [W-E] x 419 [S-N] o Domain 2 (d 02): 532 x 532 o 42 terrain-following vertical levels Model dynamics/physics: o o o o Advanced Research WRF (ARW), 3 rd-order Runge-Kutta time-integration 72 -second timestep for d 01 Multi-scale Kain-Fritsch convection (d 01 only; opt 11; CUDT=5 min) NASA/Goddard microphysics (opt 7; graupel+3 -ice) RRTM-Global short- and long-wave radiation (opt 4; RADT=10 min) Yonsei Univ. planetary boundary layer (opt 1; BLDT = 0) Noah land surface model (opt 2)
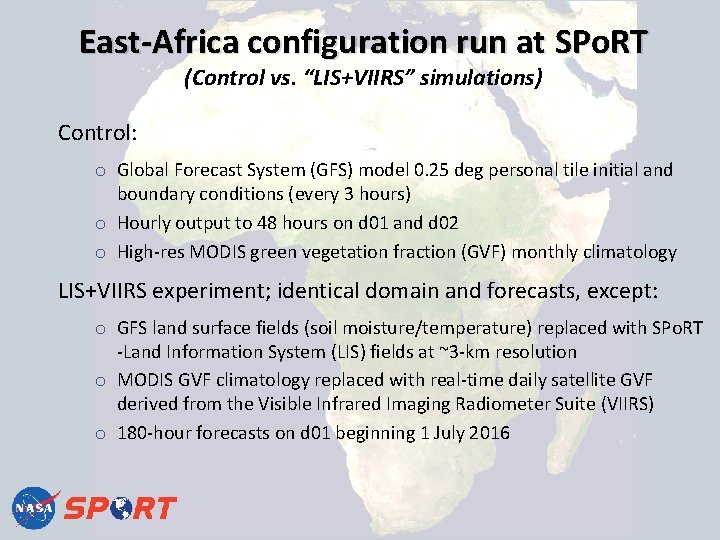
East-Africa configuration run at SPo. RT (Control vs. “LIS+VIIRS” simulations) Control: o Global Forecast System (GFS) model 0. 25 deg personal tile initial and boundary conditions (every 3 hours) o Hourly output to 48 hours on d 01 and d 02 o High-res MODIS green vegetation fraction (GVF) monthly climatology LIS+VIIRS experiment; identical domain and forecasts, except: o GFS land surface fields (soil moisture/temperature) replaced with SPo. RT -Land Information System (LIS) fields at ~3 -km resolution o MODIS GVF climatology replaced with real-time daily satellite GVF derived from the Visible Infrared Imaging Radiometer Suite (VIIRS) o 180 -hour forecasts on d 01 beginning 1 July 2016
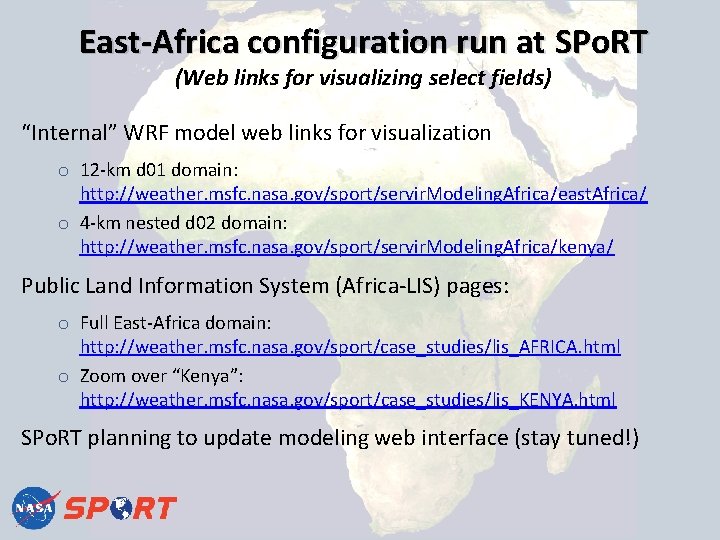
East-Africa configuration run at SPo. RT (Web links for visualizing select fields) “Internal” WRF model web links for visualization o 12 -km d 01 domain: http: //weather. msfc. nasa. gov/sport/servir. Modeling. Africa/east. Africa/ o 4 -km nested d 02 domain: http: //weather. msfc. nasa. gov/sport/servir. Modeling. Africa/kenya/ Public Land Information System (Africa-LIS) pages: o Full East-Africa domain: http: //weather. msfc. nasa. gov/sport/case_studies/lis_AFRICA. html o Zoom over “Kenya”: http: //weather. msfc. nasa. gov/sport/case_studies/lis_KENYA. html SPo. RT planning to update modeling web interface (stay tuned!)
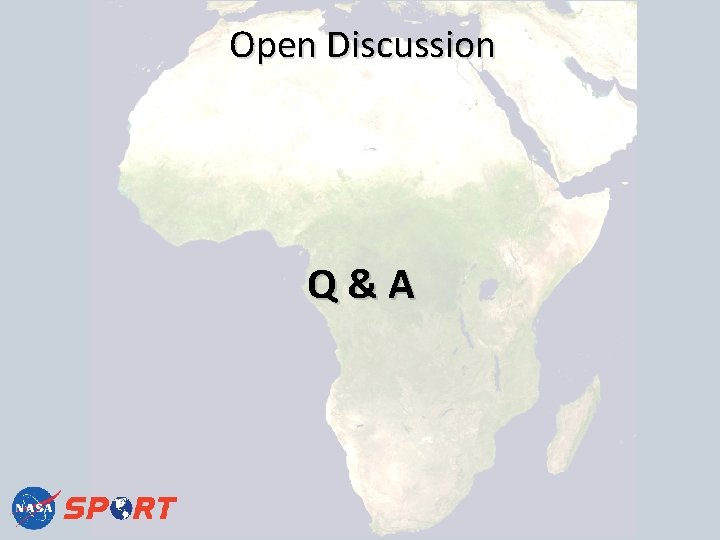
Open Discussion Q&A
Nwp imd
Steady state responding aba
Prediction verification replication aba
Sheet metal buckling
Soaring index
Helen erickson nursing theory
Relational modeling vs dimensional modeling
Meteorology symbols
Tongue twisters whether the weather
Poem fashion
Sunny rainy cloudy windy stormy
Whether the weather is fine
Heavy weather by weather report
Capital weather gang weather wall
Make a prediction about kenny and franchesca
Difference between prediction and inference in reading
Prairie arctic storm prediction centre
Difference between prediction and forecasting
Branch prediction techniques
Gene prediction in prokaryotes and eukaryotes
Graphical method numerical analysis
Fnmoc meteorology products
Programs that organize analyze and graph numerical data
Numerical pattern
C programming and numerical analysis an introduction
Interpreting expressions example
Common features of application software
Graphical and numerical summaries
Verification in creative process
Verification and validation