Introduction to Statistical Analysis What is STATISTICS Statistics
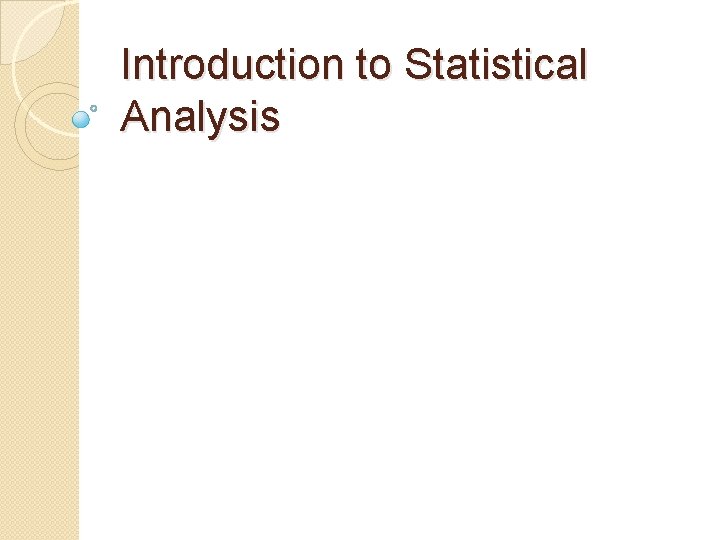
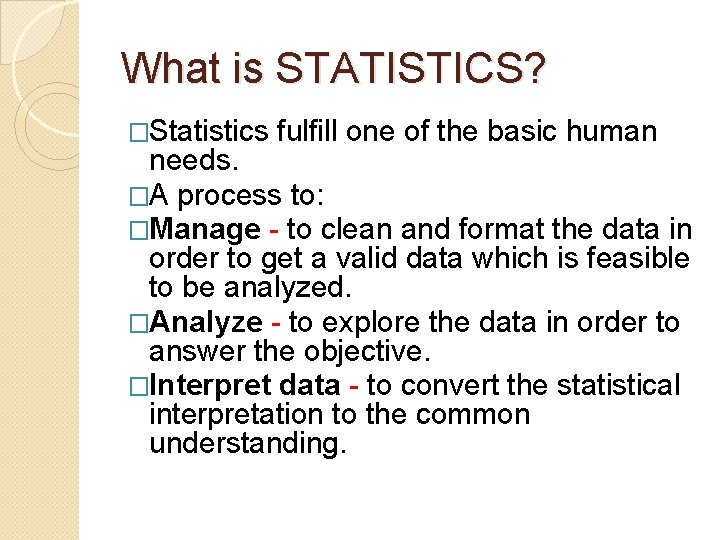
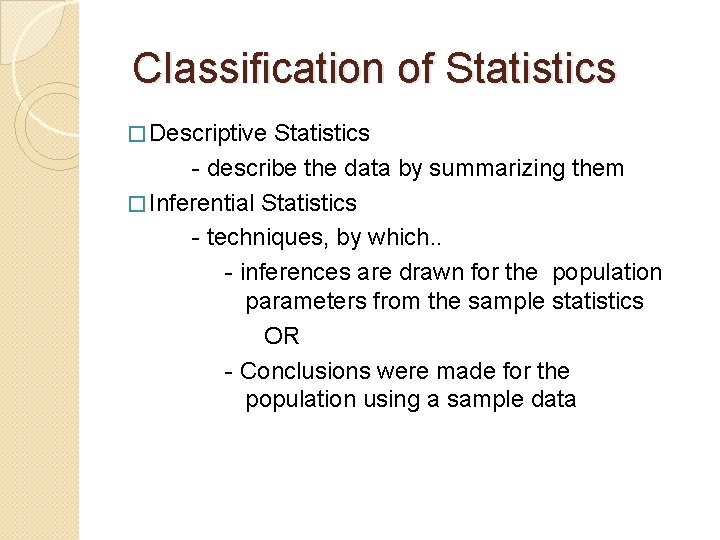
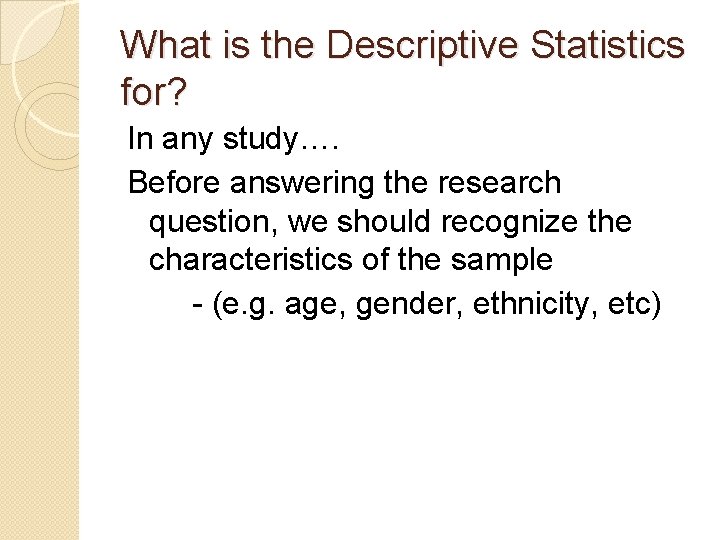
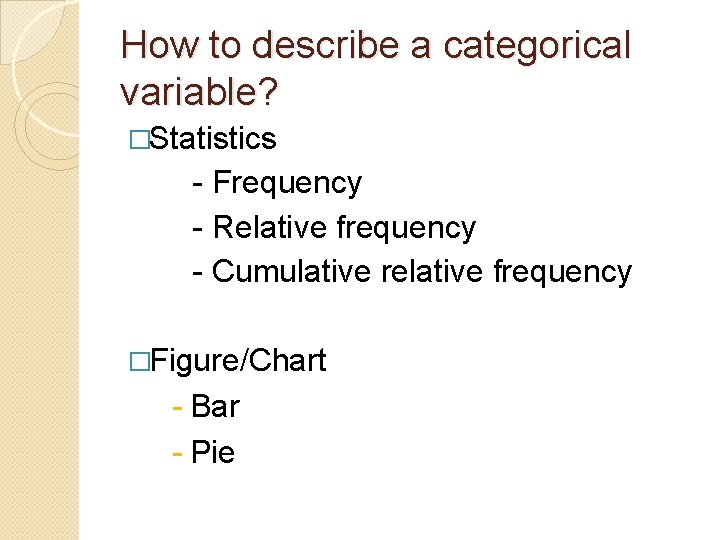
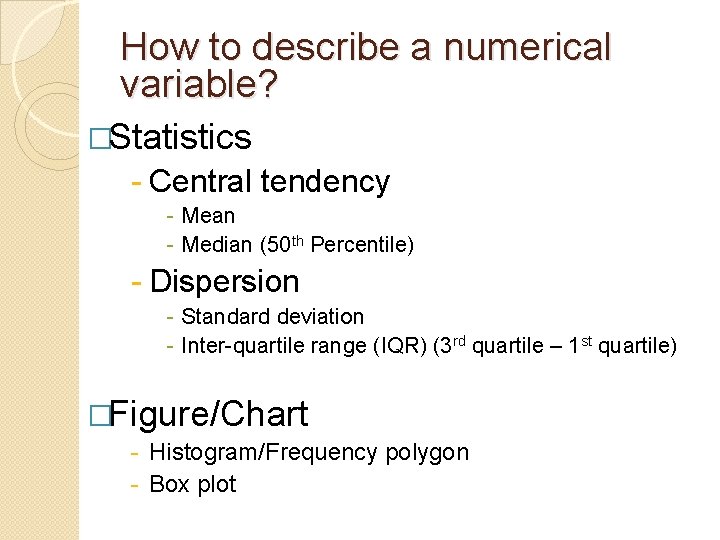
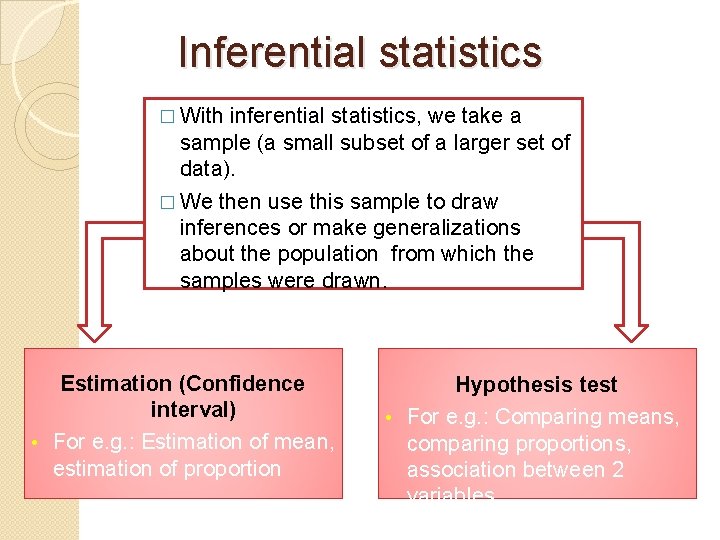
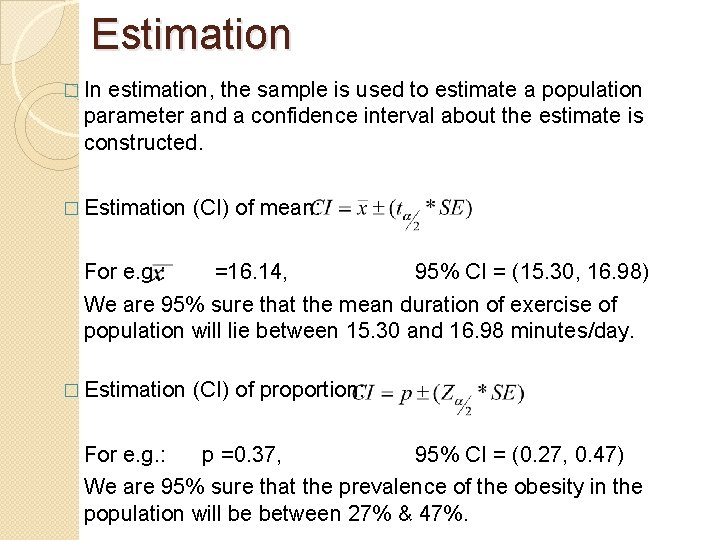
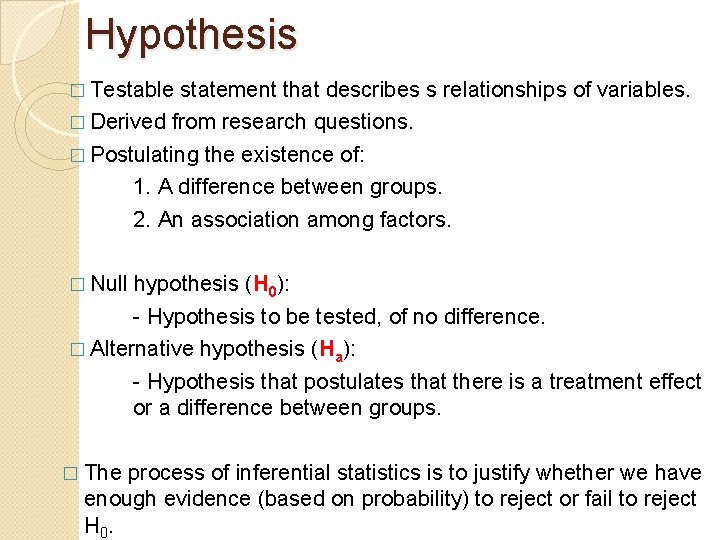
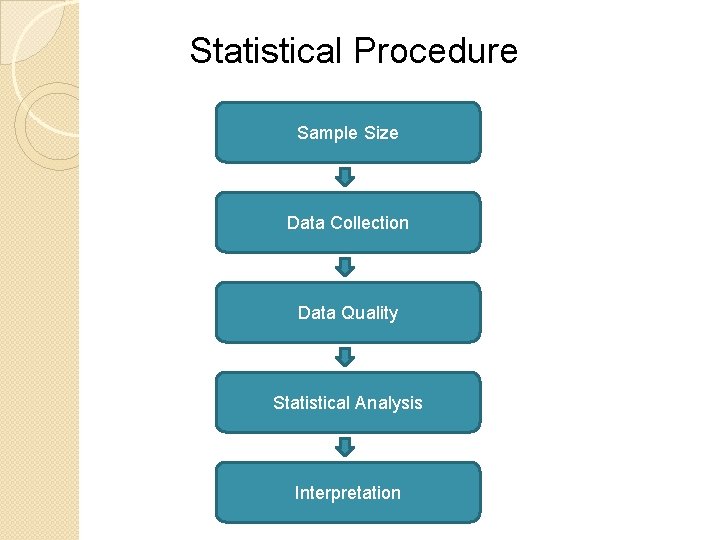
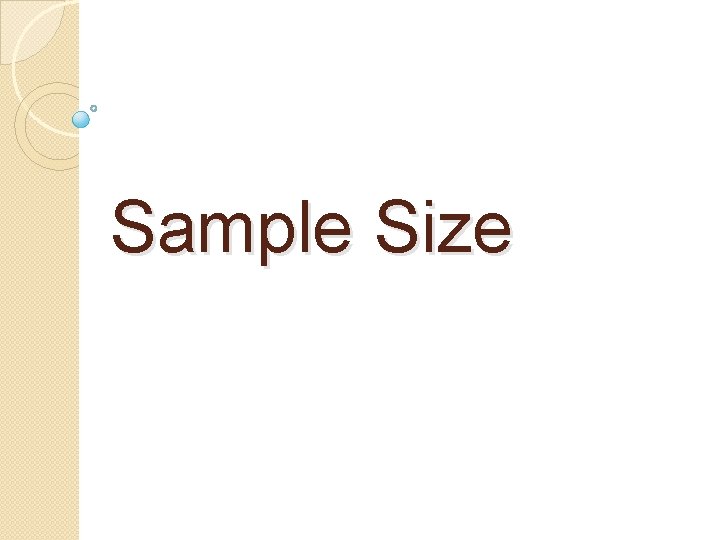
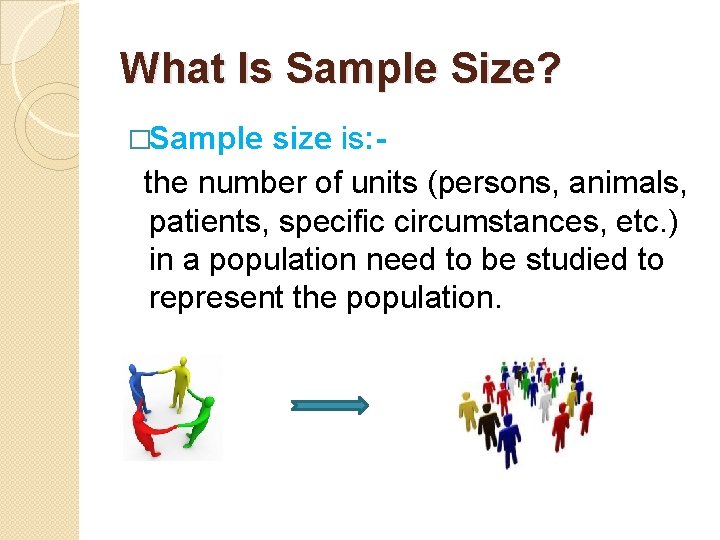
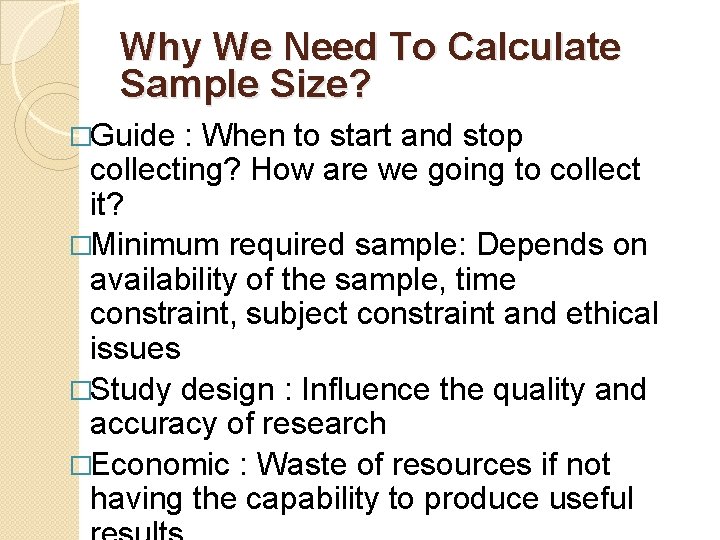
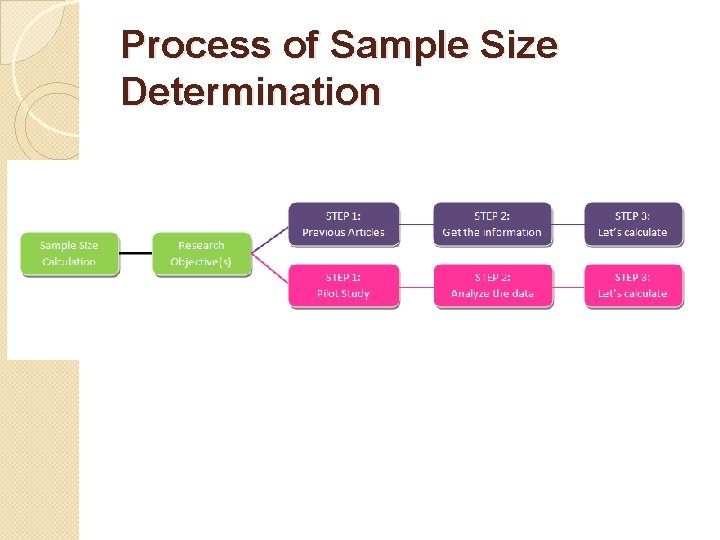
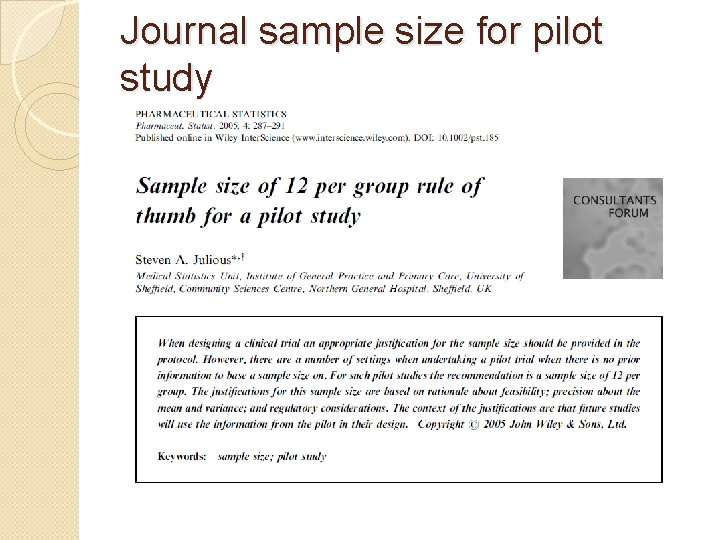
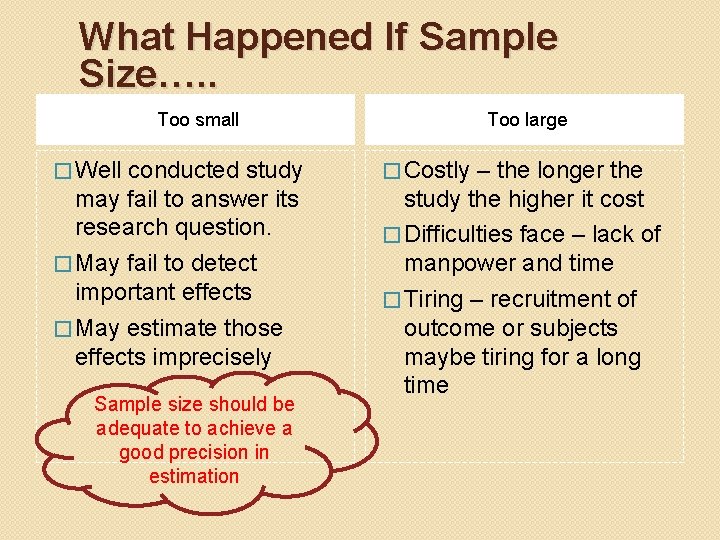
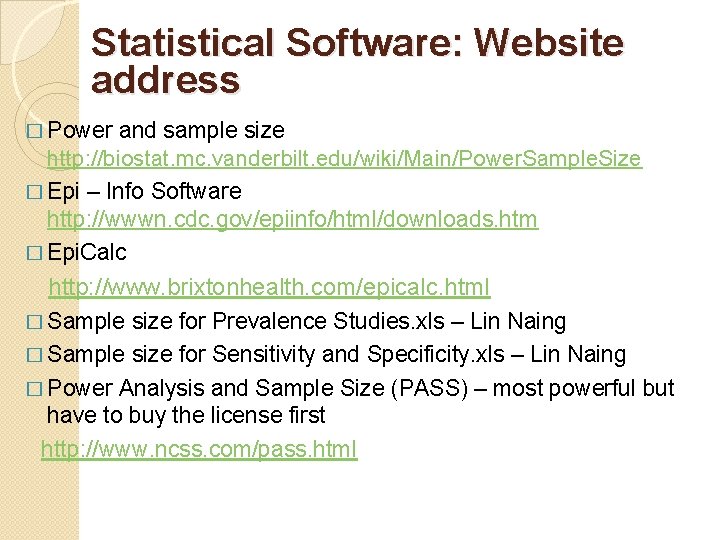
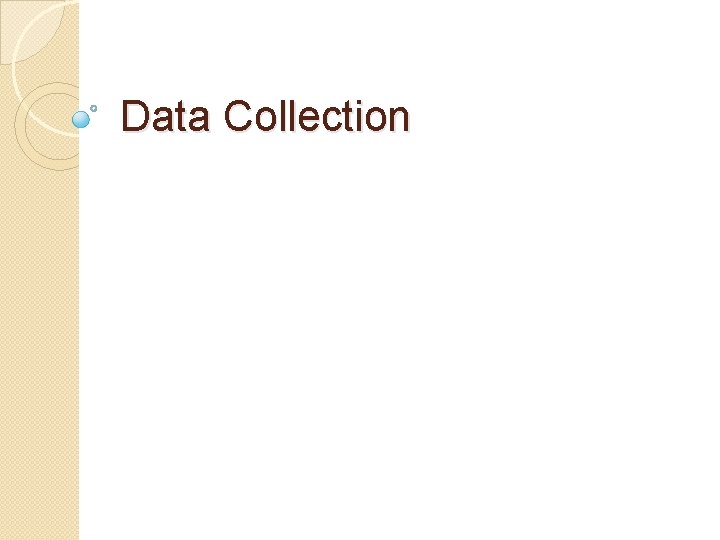
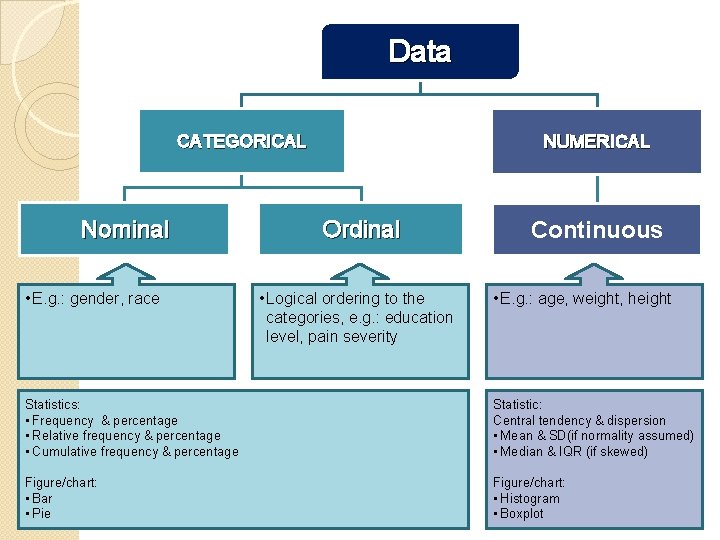
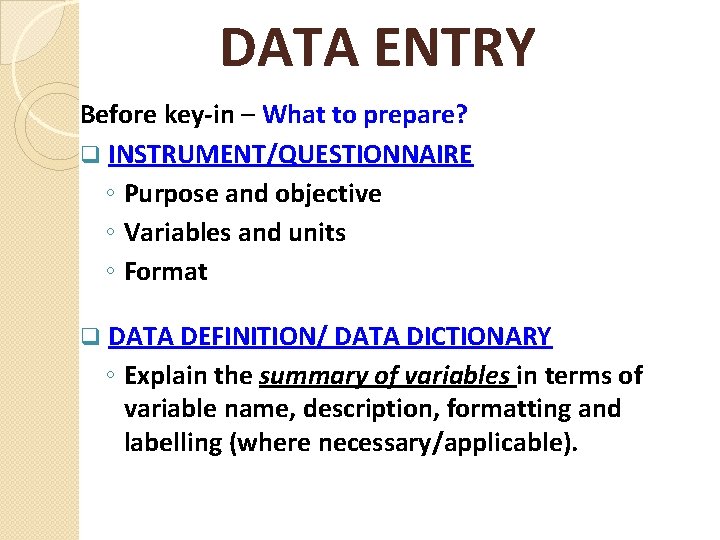
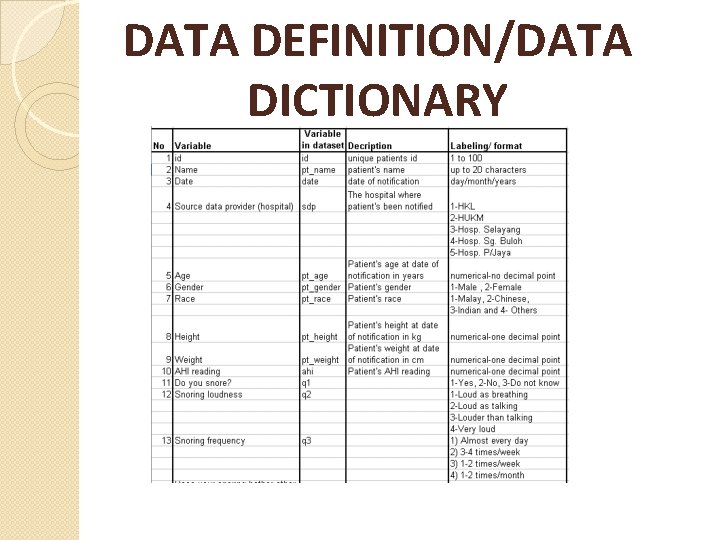
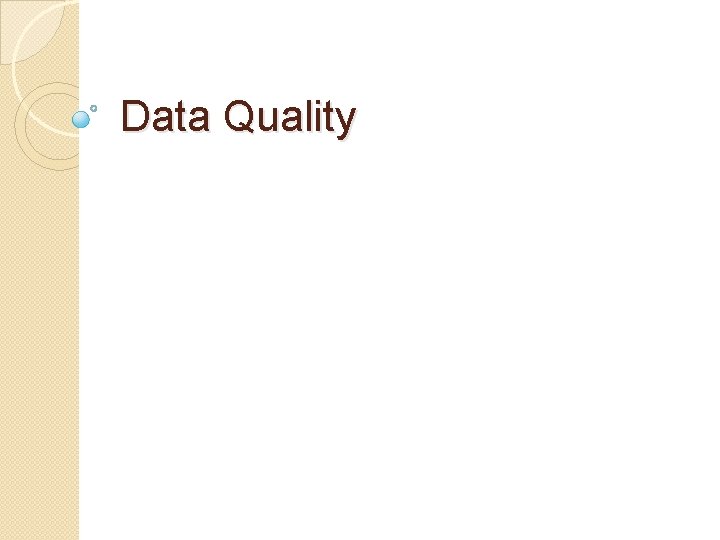
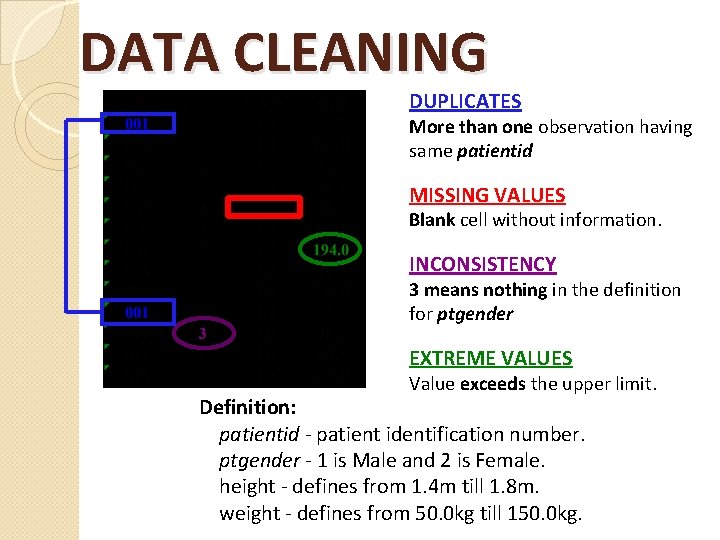
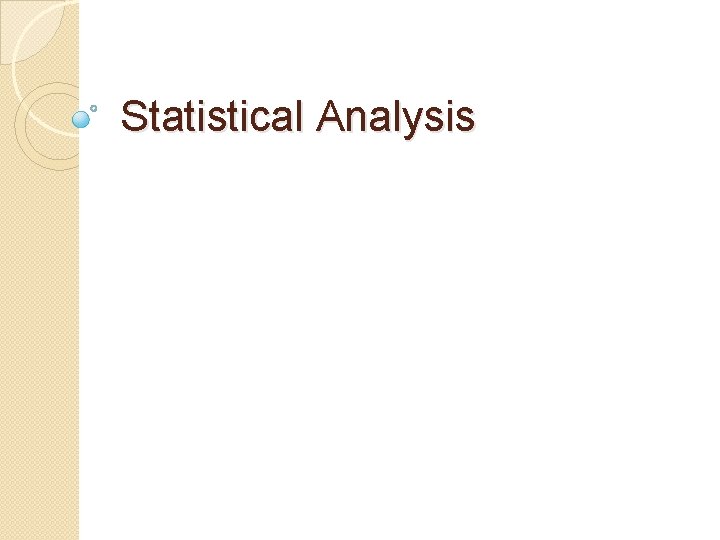
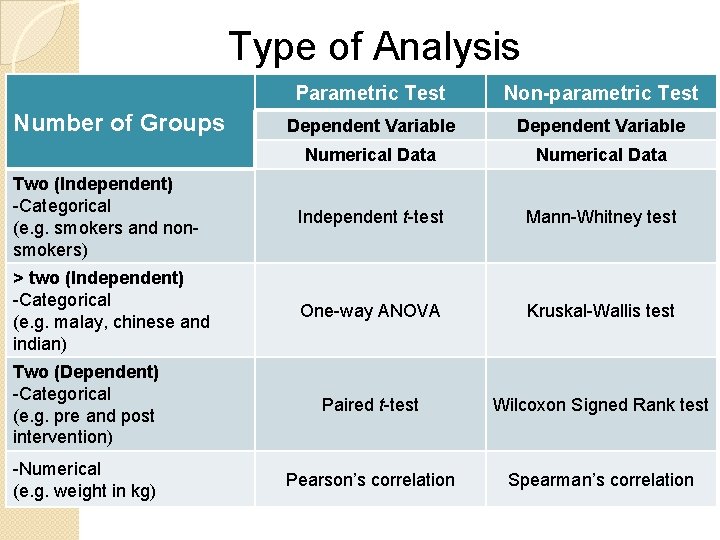
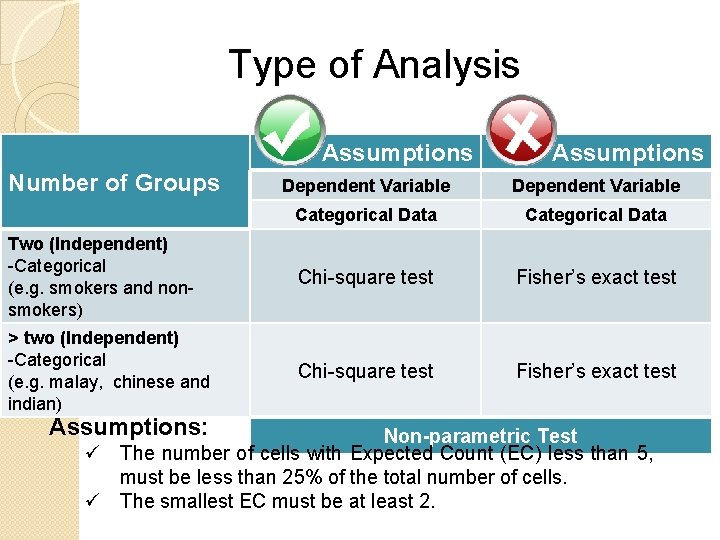
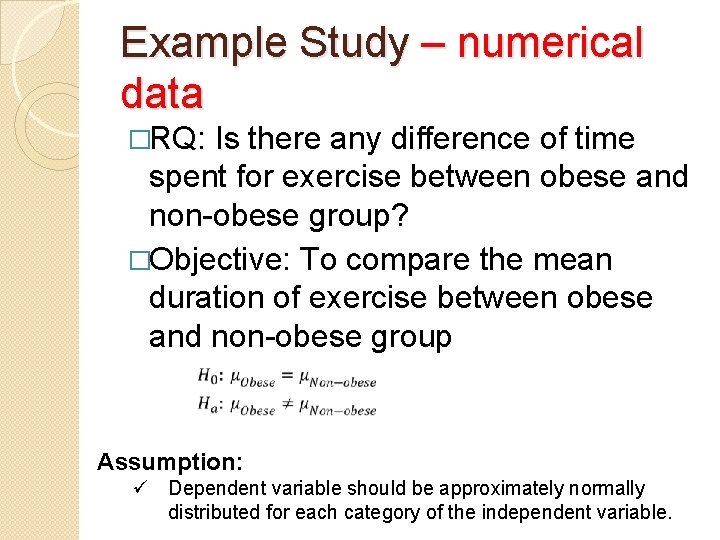
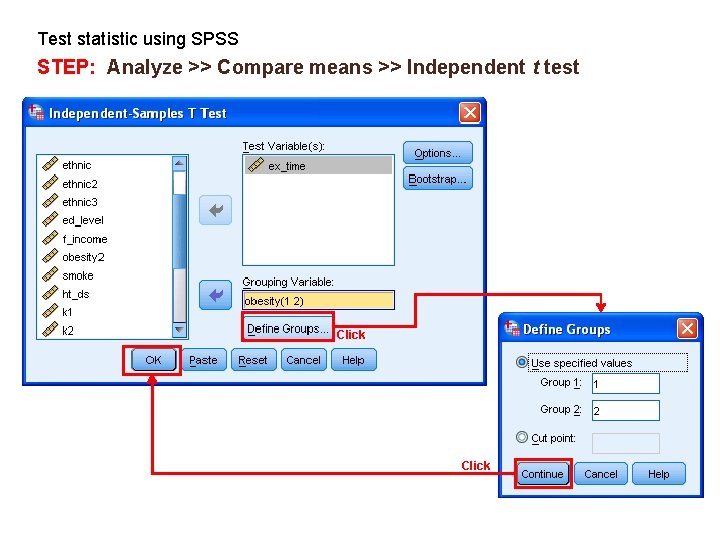
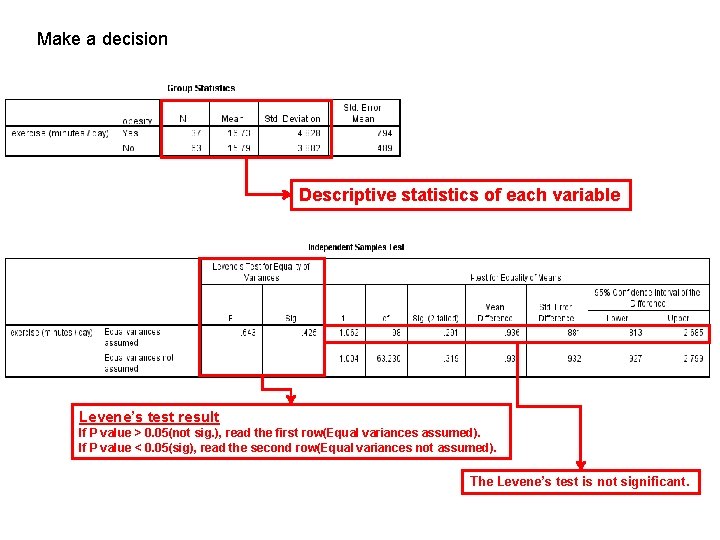
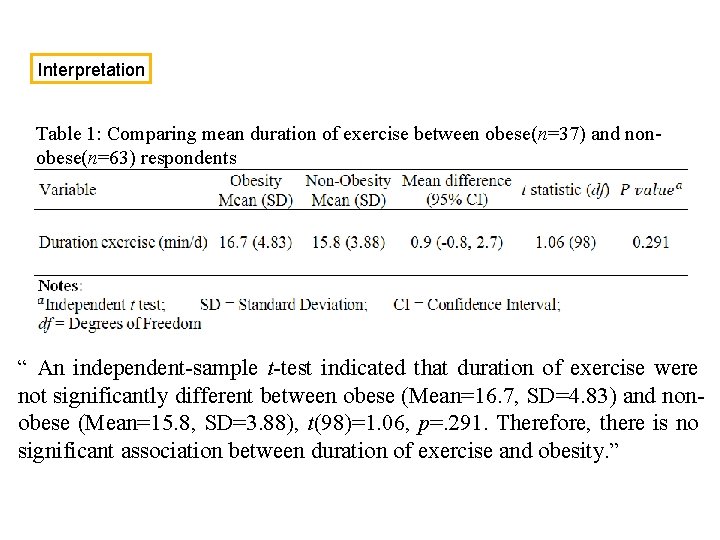
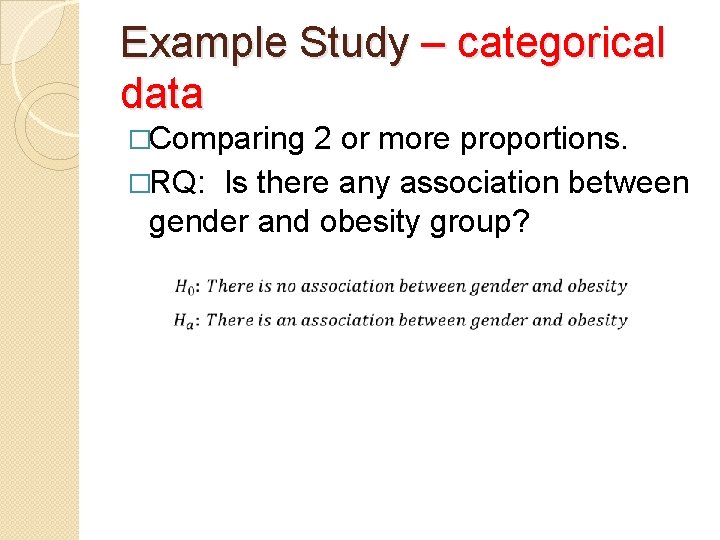
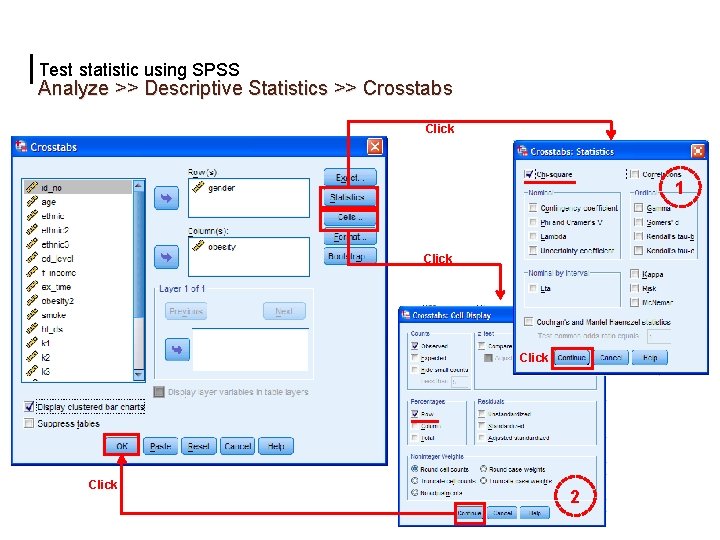
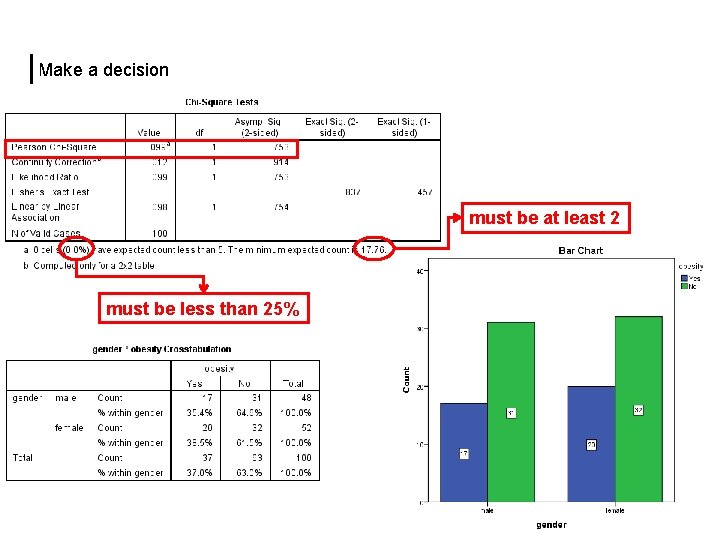
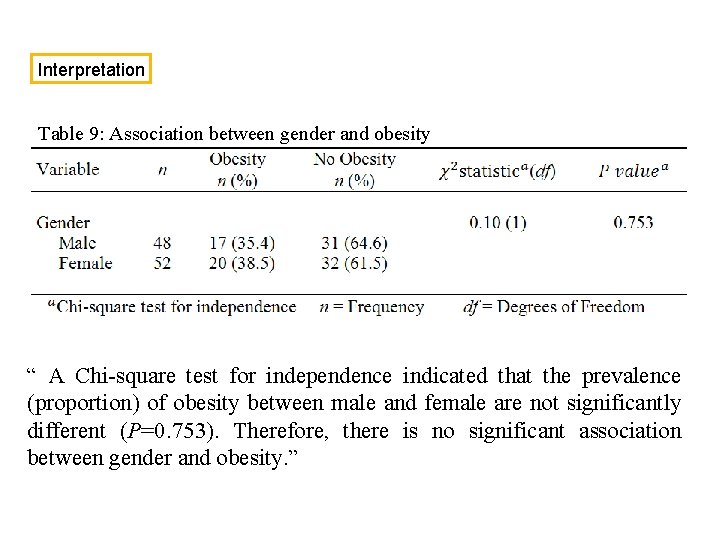
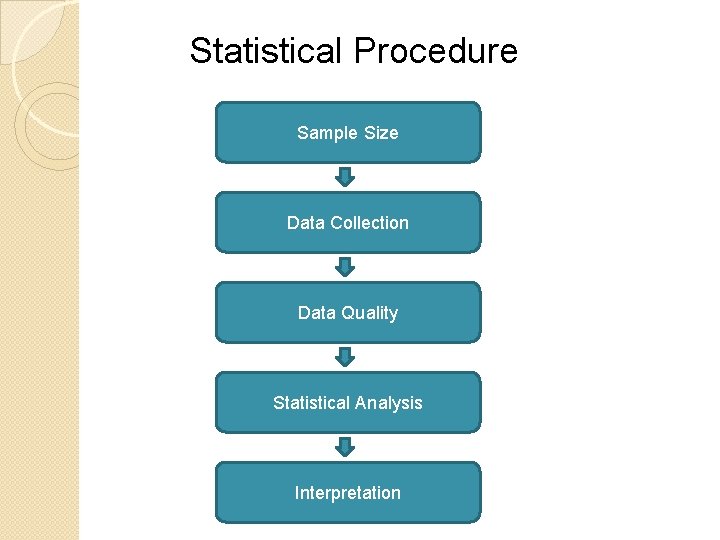
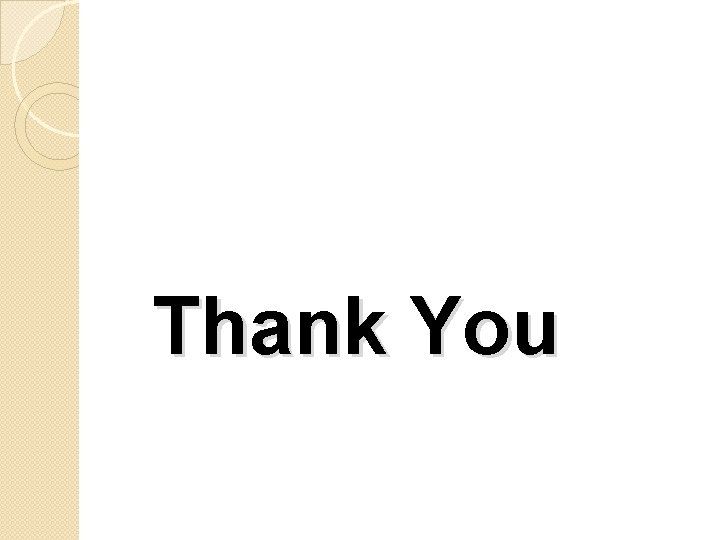
- Slides: 36
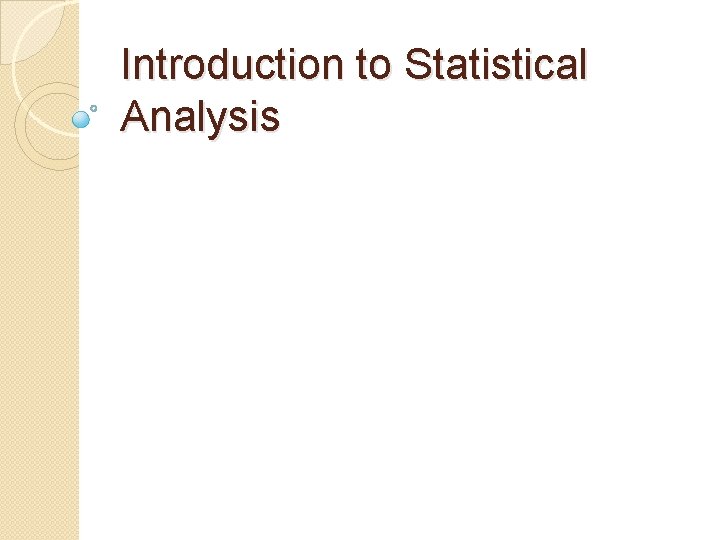
Introduction to Statistical Analysis
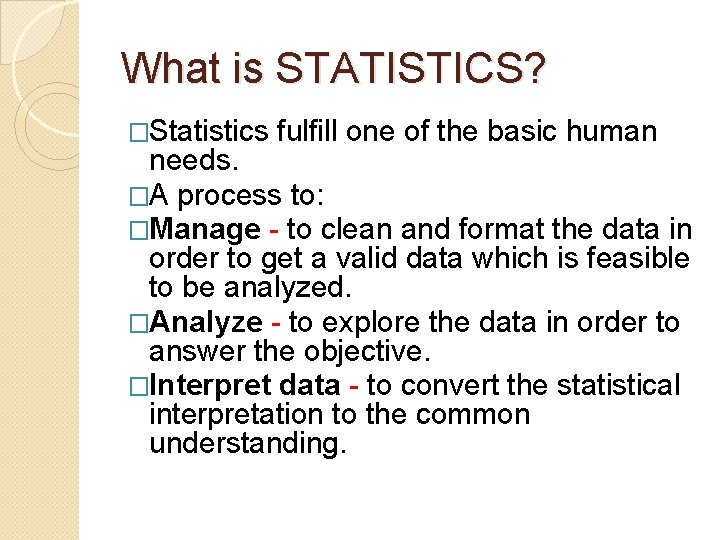
What is STATISTICS? �Statistics fulfill one of the basic human needs. �A process to: �Manage - to clean and format the data in order to get a valid data which is feasible to be analyzed. �Analyze - to explore the data in order to answer the objective. �Interpret data - to convert the statistical interpretation to the common understanding.
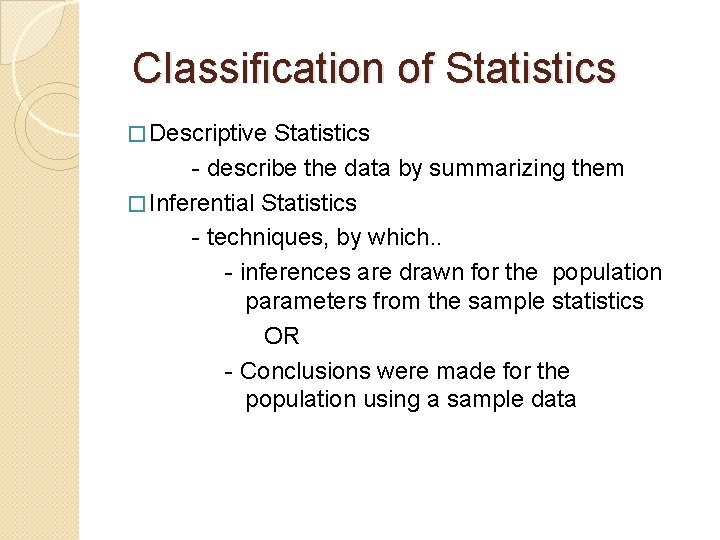
Classification of Statistics � Descriptive Statistics - describe the data by summarizing them � Inferential Statistics - techniques, by which. . - inferences are drawn for the population parameters from the sample statistics OR - Conclusions were made for the population using a sample data
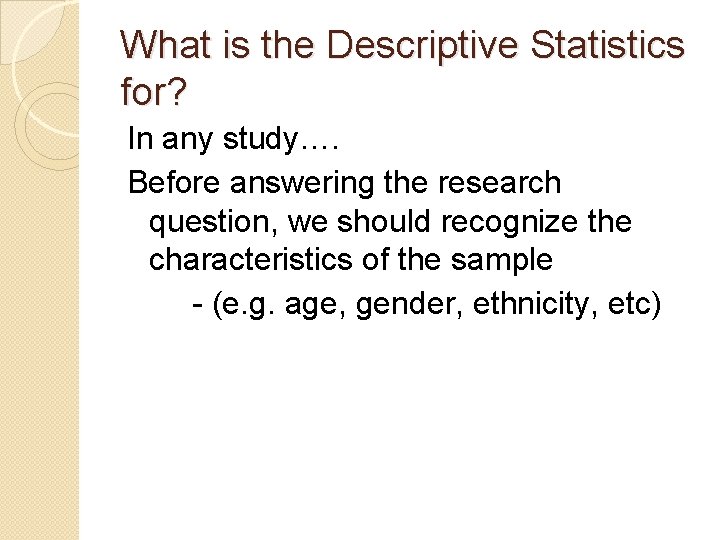
What is the Descriptive Statistics for? In any study…. Before answering the research question, we should recognize the characteristics of the sample - (e. g. age, gender, ethnicity, etc)
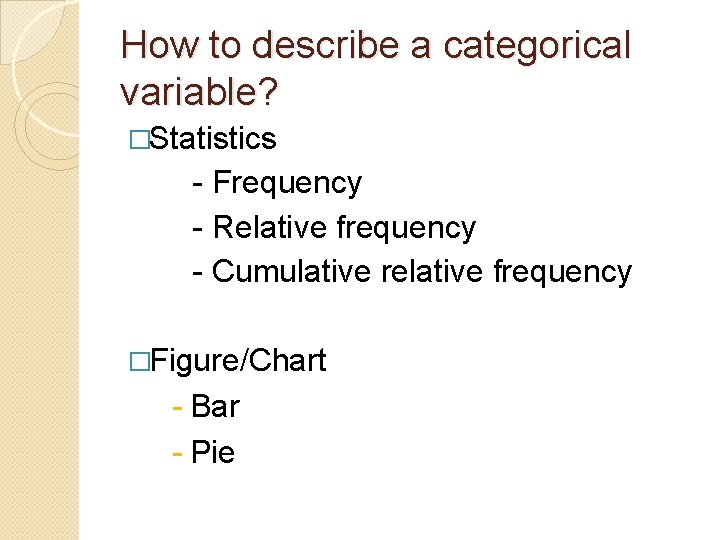
How to describe a categorical variable? �Statistics - Frequency - Relative frequency - Cumulative relative frequency �Figure/Chart - Bar - Pie
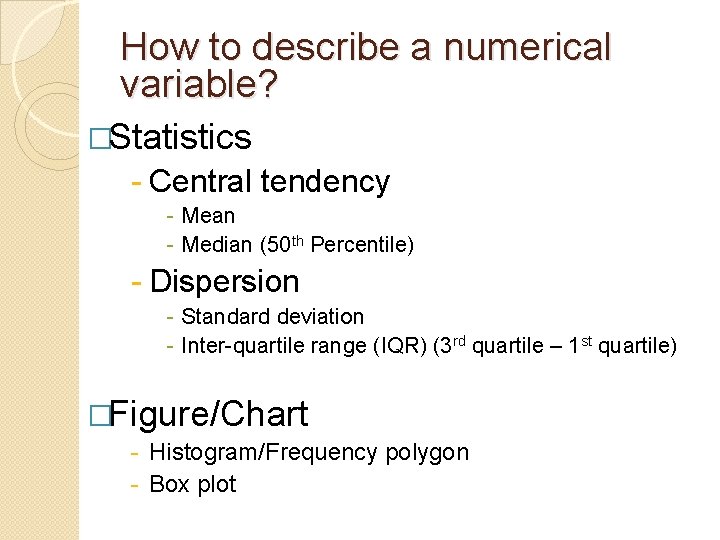
How to describe a numerical variable? �Statistics - Central tendency - Mean - Median (50 th Percentile) - Dispersion - Standard deviation - Inter-quartile range (IQR) (3 rd quartile – 1 st quartile) �Figure/Chart - Histogram/Frequency polygon - Box plot
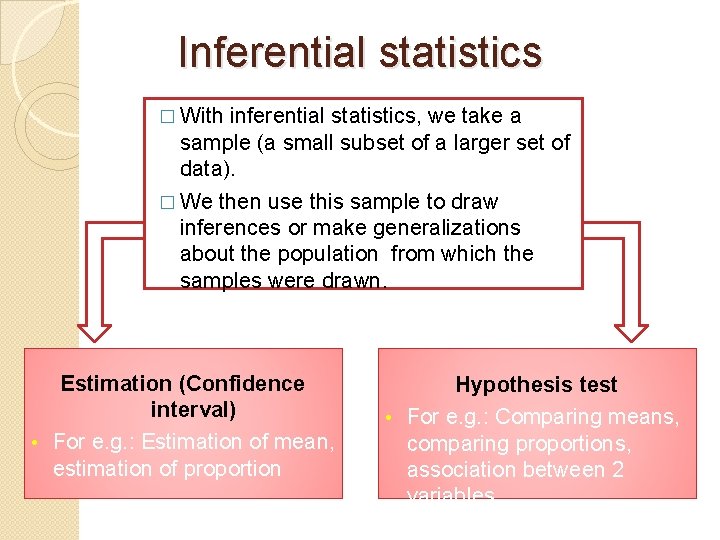
Inferential statistics � With inferential statistics, we take a sample (a small subset of a larger set of data). � We then use this sample to draw inferences or make generalizations about the population from which the samples were drawn. Estimation (Confidence interval) • For e. g. : Estimation of mean, estimation of proportion Hypothesis test • For e. g. : Comparing means, comparing proportions, association between 2 variables
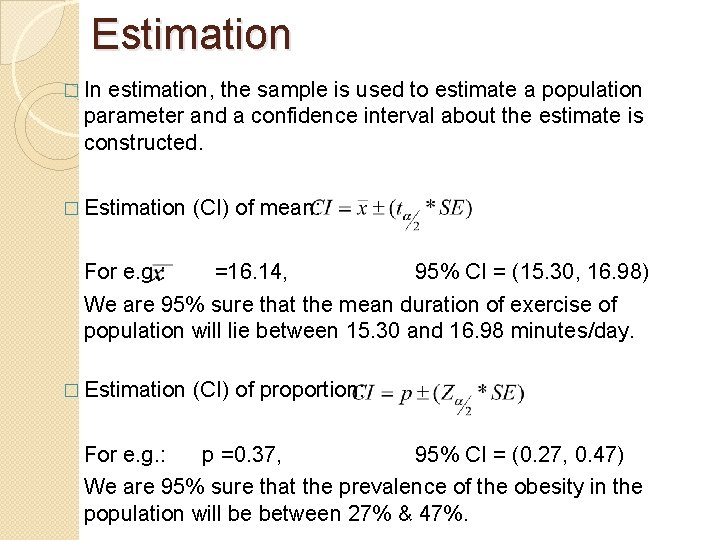
Estimation � In estimation, the sample is used to estimate a population parameter and a confidence interval about the estimate is constructed. � Estimation (CI) of mean: For e. g. : =16. 14, 95% CI = (15. 30, 16. 98) We are 95% sure that the mean duration of exercise of population will lie between 15. 30 and 16. 98 minutes/day. � Estimation (CI) of proportion: For e. g. : p =0. 37, 95% CI = (0. 27, 0. 47) We are 95% sure that the prevalence of the obesity in the population will be between 27% & 47%.
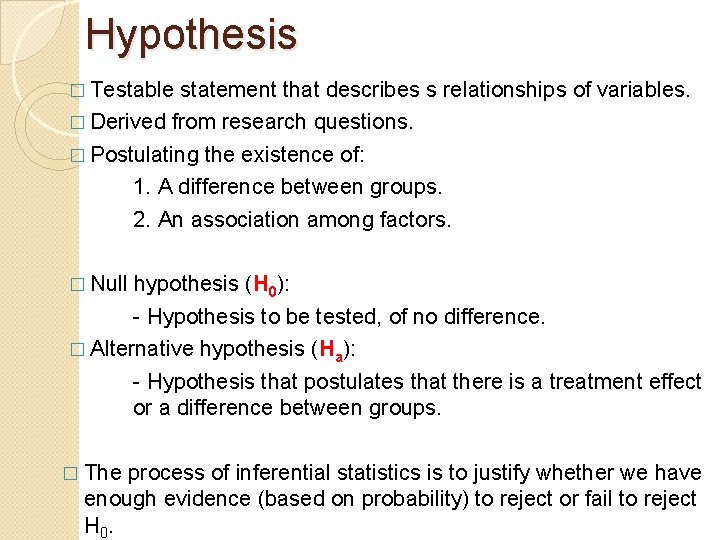
Hypothesis � Testable statement that describes s relationships of variables. � Derived from research questions. � Postulating the existence of: 1. A difference between groups. 2. An association among factors. � Null hypothesis (H 0): - Hypothesis to be tested, of no difference. � Alternative hypothesis (Ha): - Hypothesis that postulates that there is a treatment effect or a difference between groups. � The process of inferential statistics is to justify whether we have enough evidence (based on probability) to reject or fail to reject H 0.
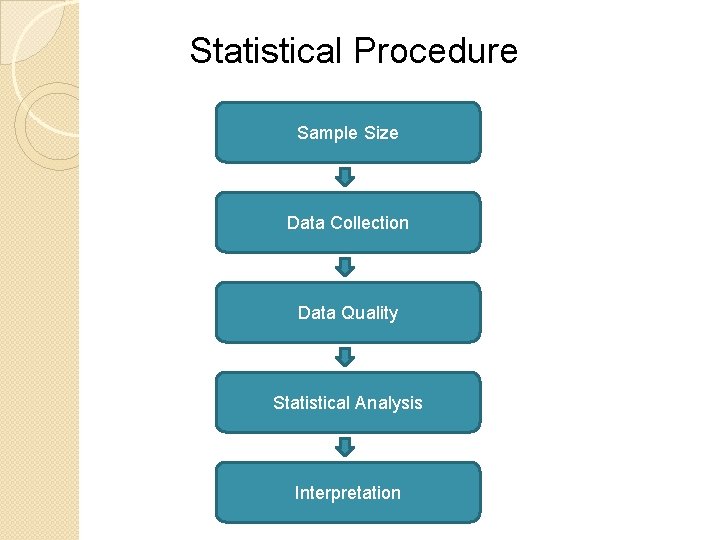
Statistical Procedure Sample Size Data Collection Data Quality Statistical Analysis Interpretation
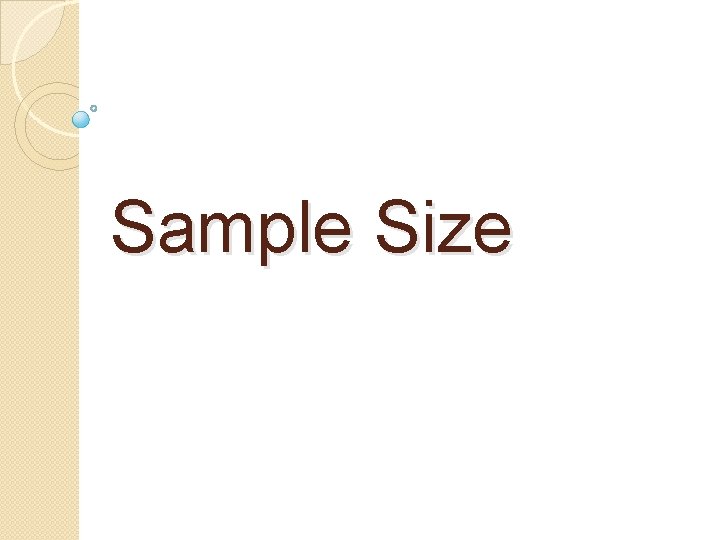
Sample Size
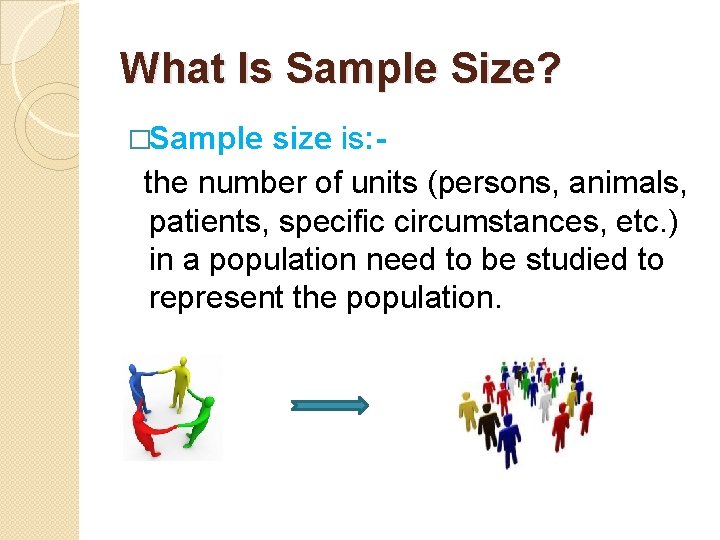
What Is Sample Size? �Sample size is: the number of units (persons, animals, patients, specific circumstances, etc. ) in a population need to be studied to represent the population.
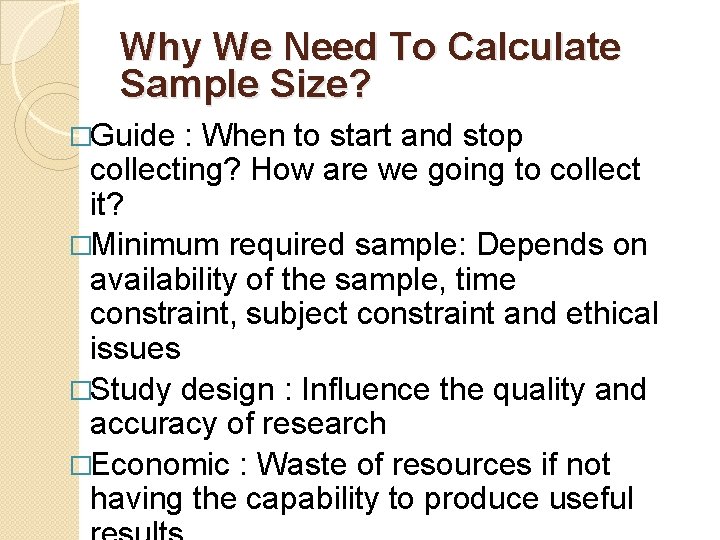
Why We Need To Calculate Sample Size? �Guide : When to start and stop collecting? How are we going to collect it? �Minimum required sample: Depends on availability of the sample, time constraint, subject constraint and ethical issues �Study design : Influence the quality and accuracy of research �Economic : Waste of resources if not having the capability to produce useful
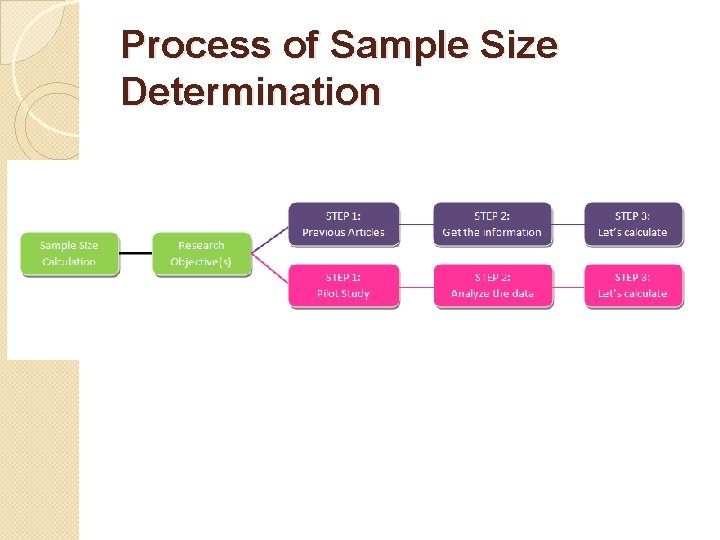
Process of Sample Size Determination
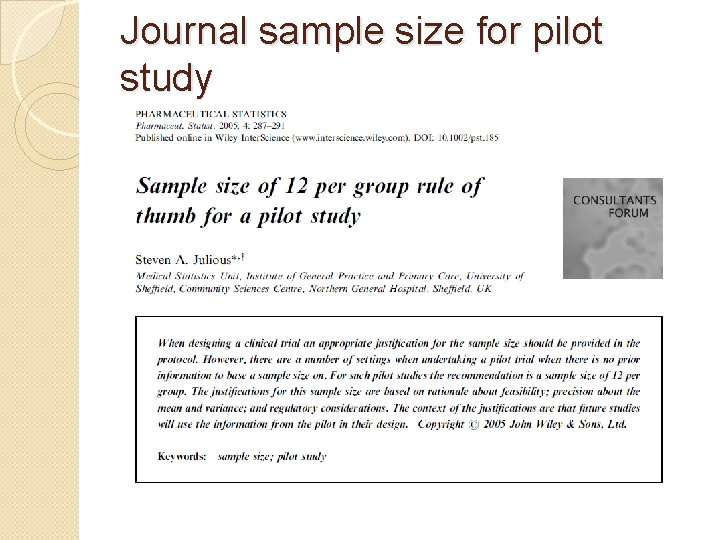
Journal sample size for pilot study
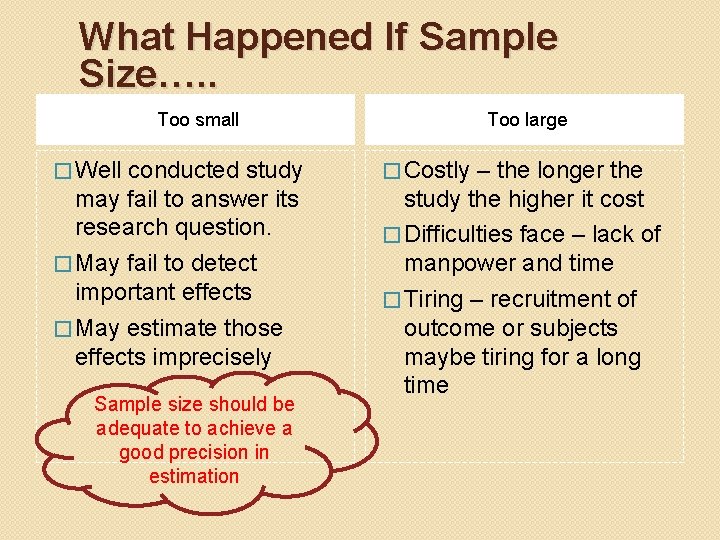
What Happened If Sample Size…. . Too small � Well conducted study may fail to answer its research question. � May fail to detect important effects � May estimate those effects imprecisely Sample size should be adequate to achieve a good precision in estimation Too large � Costly – the longer the study the higher it cost � Difficulties face – lack of manpower and time � Tiring – recruitment of outcome or subjects maybe tiring for a long time
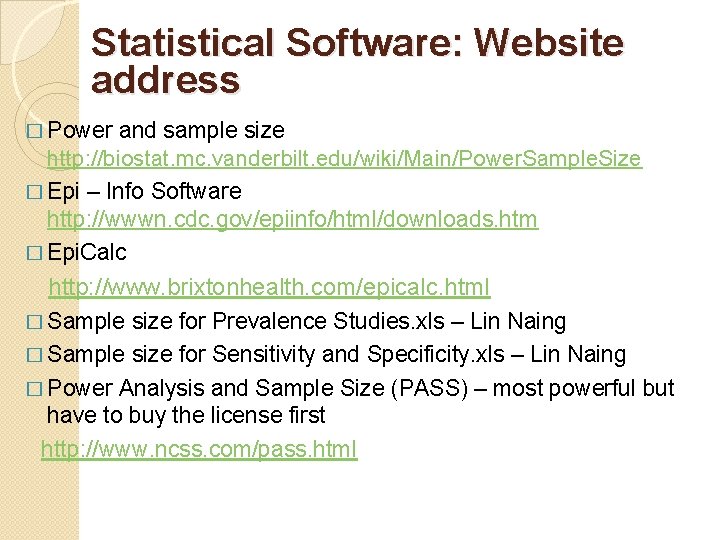
Statistical Software: Website address � Power and sample size http: //biostat. mc. vanderbilt. edu/wiki/Main/Power. Sample. Size � Epi – Info Software http: //wwwn. cdc. gov/epiinfo/html/downloads. htm � Epi. Calc http: //www. brixtonhealth. com/epicalc. html � Sample size for Prevalence Studies. xls – Lin Naing � Sample size for Sensitivity and Specificity. xls – Lin Naing � Power Analysis and Sample Size (PASS) – most powerful but have to buy the license first http: //www. ncss. com/pass. html
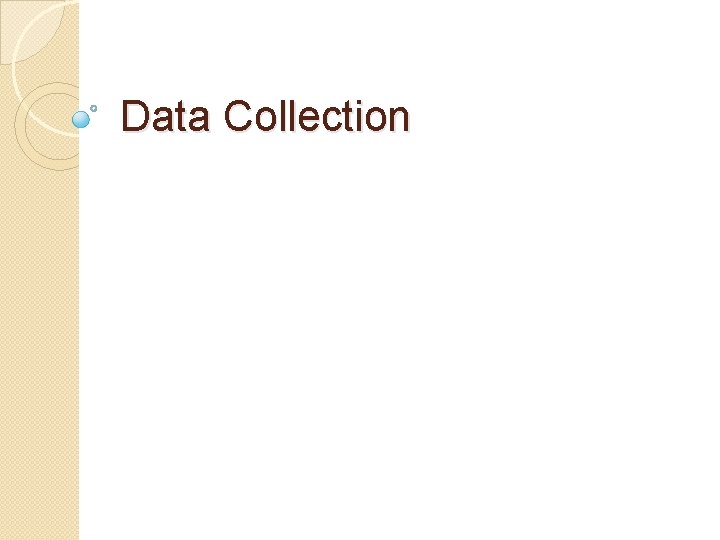
Data Collection
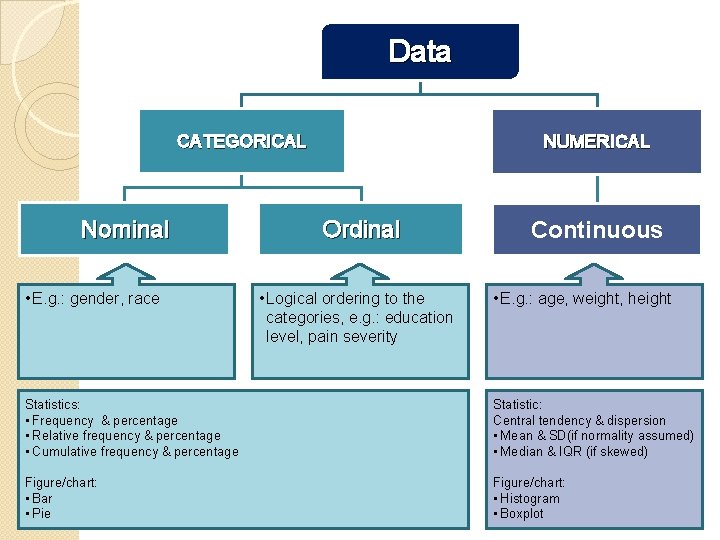
Data CATEGORICAL Nominal • E. g. : gender, race NUMERICAL Ordinal • Logical ordering to the categories, e. g. : education level, pain severity Continuous • E. g. : age, weight, height Statistics: • Frequency & percentage • Relative frequency & percentage • Cumulative frequency & percentage Statistic: Central tendency & dispersion • Mean & SD(if normality assumed) • Median & IQR (if skewed) Figure/chart: • Bar • Pie Figure/chart: • Histogram • Boxplot
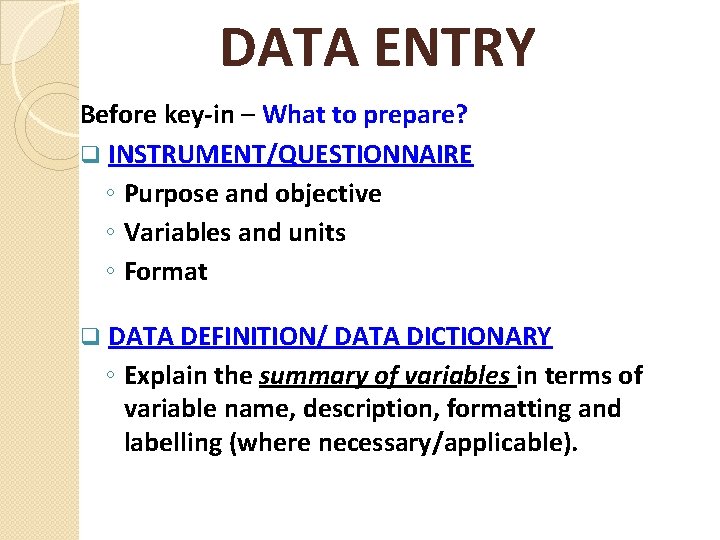
DATA ENTRY Before key-in – What to prepare? q INSTRUMENT/QUESTIONNAIRE ◦ Purpose and objective ◦ Variables and units ◦ Format q DATA DEFINITION/ DATA DICTIONARY ◦ Explain the summary of variables in terms of variable name, description, formatting and labelling (where necessary/applicable).
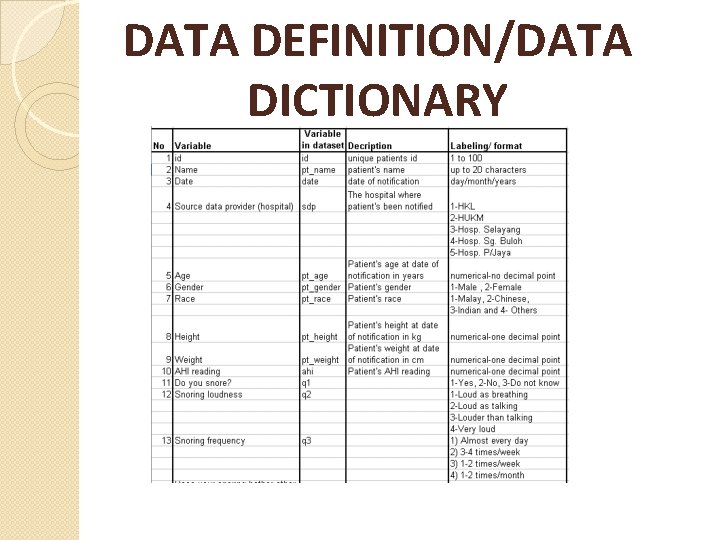
DATA DEFINITION/DATA DICTIONARY
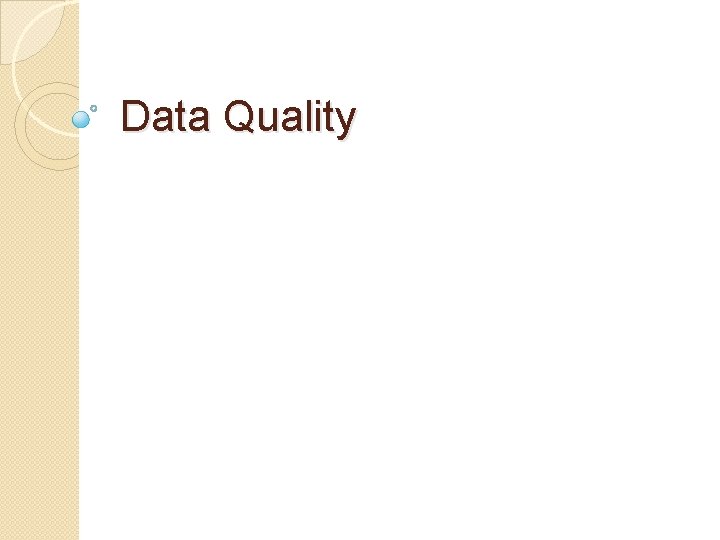
Data Quality
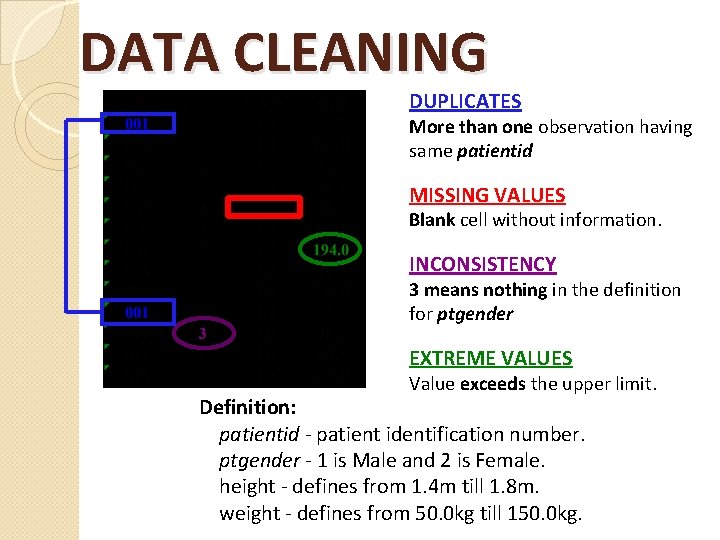
DATA CLEANING DUPLICATES More than one observation having same patientid MISSING VALUES Blank cell without information. INCONSISTENCY 3 means nothing in the definition for ptgender EXTREME VALUES Value exceeds the upper limit. Definition: patientid - patient identification number. ptgender - 1 is Male and 2 is Female. height - defines from 1. 4 m till 1. 8 m. weight - defines from 50. 0 kg till 150. 0 kg.
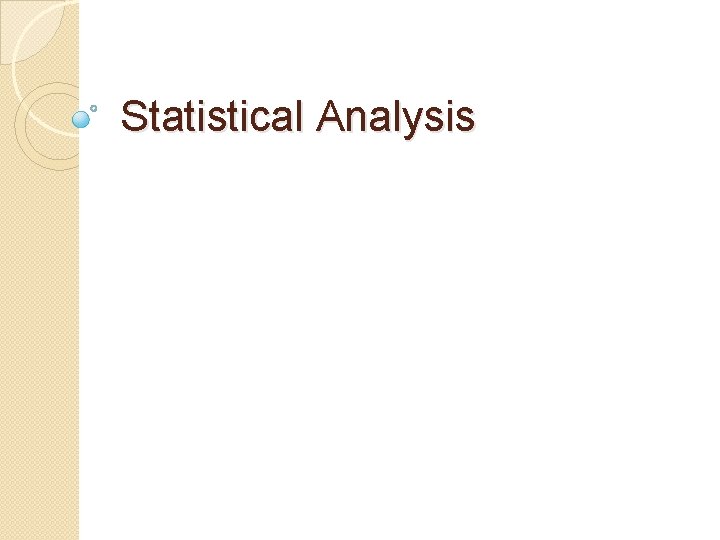
Statistical Analysis
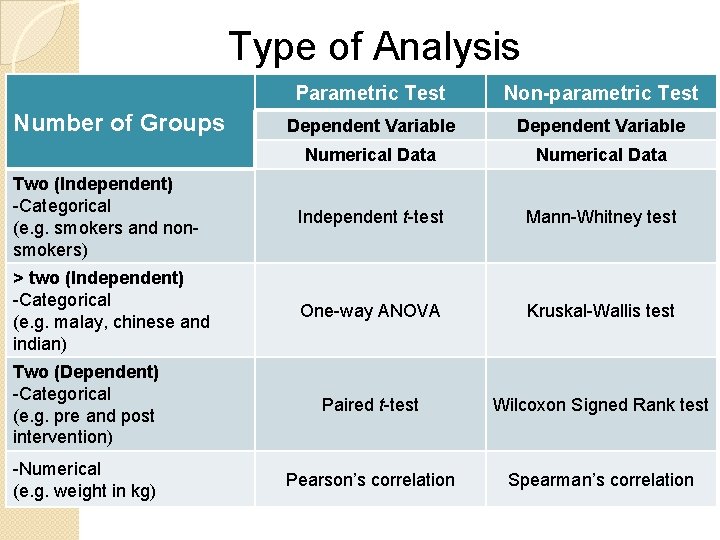
Type of Analysis Parametric Test Non-parametric Test Dependent Variable Numerical Data Two (Independent) -Categorical (e. g. smokers and nonsmokers) Independent t-test Mann-Whitney test > two (Independent) -Categorical (e. g. malay, chinese and indian) One-way ANOVA Kruskal-Wallis test Two (Dependent) -Categorical (e. g. pre and post intervention) Paired t-test Wilcoxon Signed Rank test -Numerical (e. g. weight in kg) Pearson’s correlation Spearman’s correlation Number of Groups
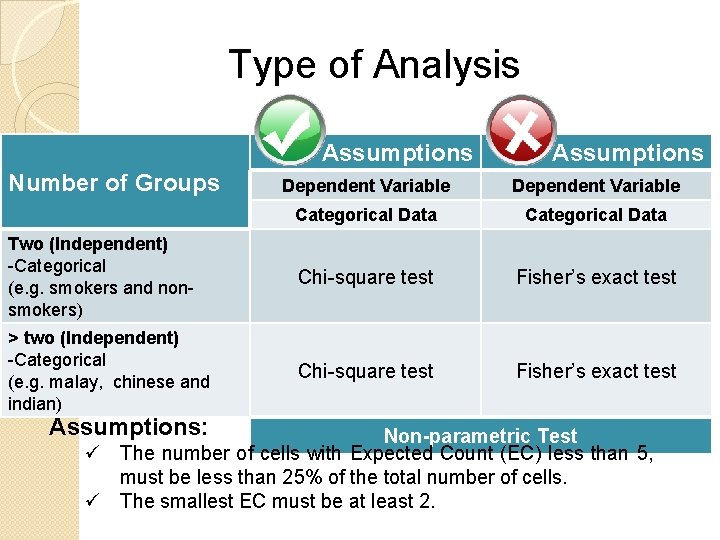
Type of Analysis Assumptions Number of Groups Assumptions Dependent Variable Categorical Data Two (Independent) -Categorical (e. g. smokers and nonsmokers) Chi-square test Fisher’s exact test > two (Independent) -Categorical (e. g. malay, chinese and indian) Chi-square test Fisher’s exact test Assumptions: Non-parametric Test ü The number of cells with Expected Count (EC) less than 5, must be less than 25% of the total number of cells. ü The smallest EC must be at least 2.
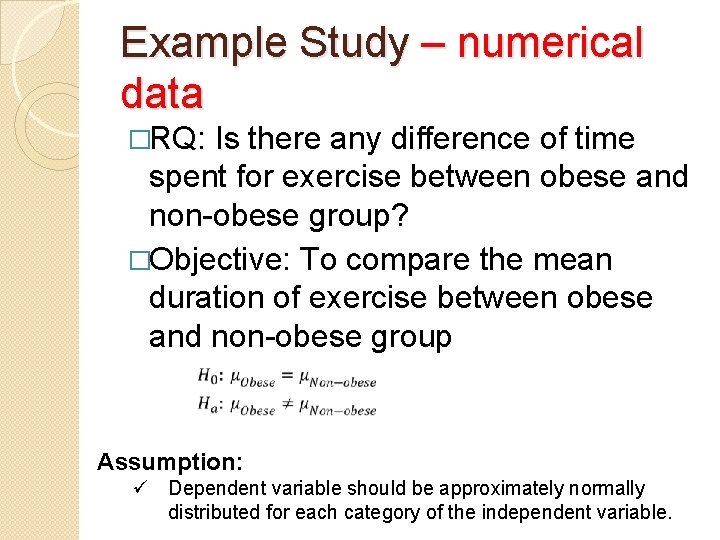
Example Study – numerical data �RQ: Is there any difference of time spent for exercise between obese and non-obese group? �Objective: To compare the mean duration of exercise between obese and non-obese group Assumption: ü Dependent variable should be approximately normally distributed for each category of the independent variable.
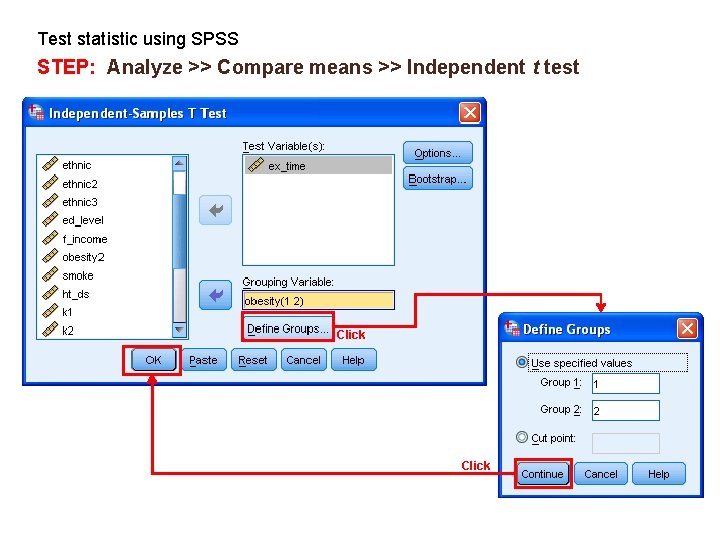
second Test statistic using SPSS STEP: Analyze >> Compare means >> Independent t test Analyze >> Descriptive Statistics >> Explore Click
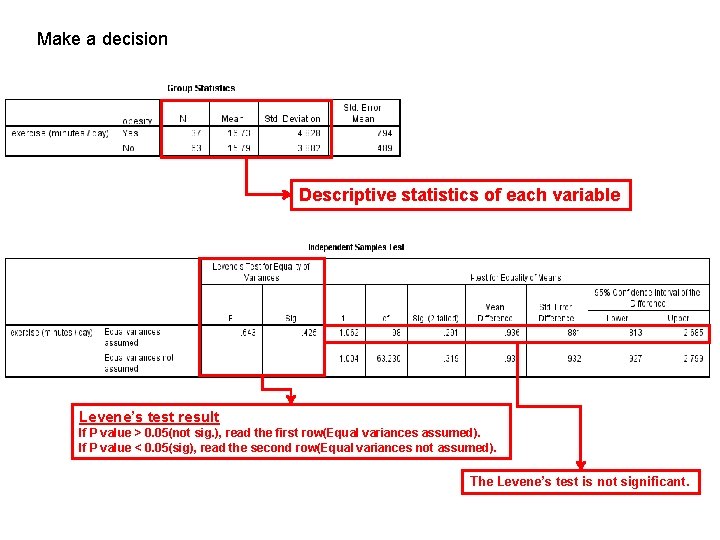
third Make a decision Descriptive statistics of each variable Levene’s test result If P value > 0. 05(not sig. ), read the first row(Equal variances assumed). If P value < 0. 05(sig), read the second row(Equal variances not assumed). The Levene’s test is not significant.
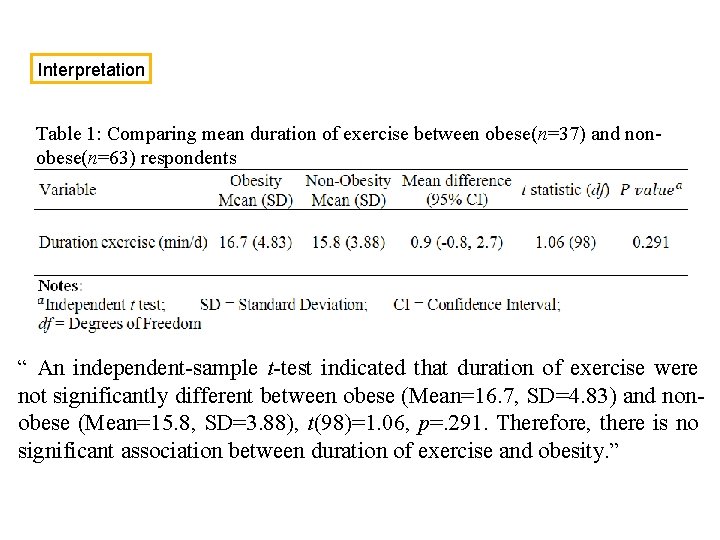
Interpretation Table 1: Comparing mean duration of exercise between obese(n=37) and nonobese(n=63) respondents “ An independent-sample t-test indicated that duration of exercise were not significantly different between obese (Mean=16. 7, SD=4. 83) and nonobese (Mean=15. 8, SD=3. 88), t(98)=1. 06, p=. 291. Therefore, there is no significant association between duration of exercise and obesity. ”
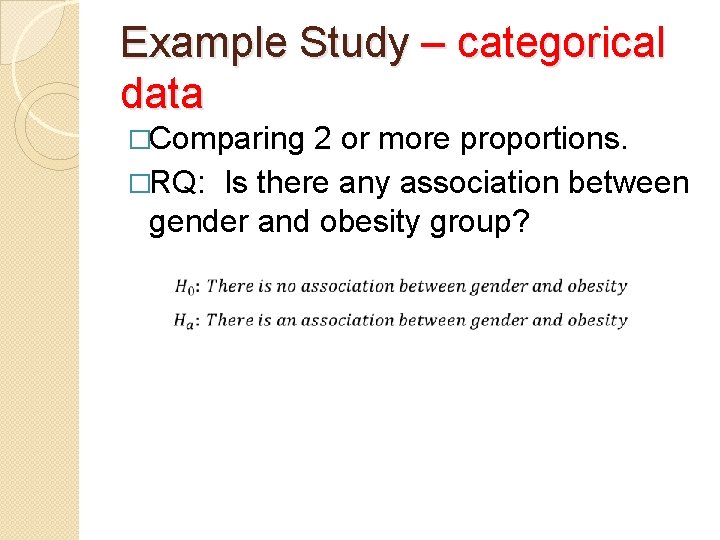
Example Study – categorical data �Comparing 2 or more proportions. �RQ: Is there any association between gender and obesity group?
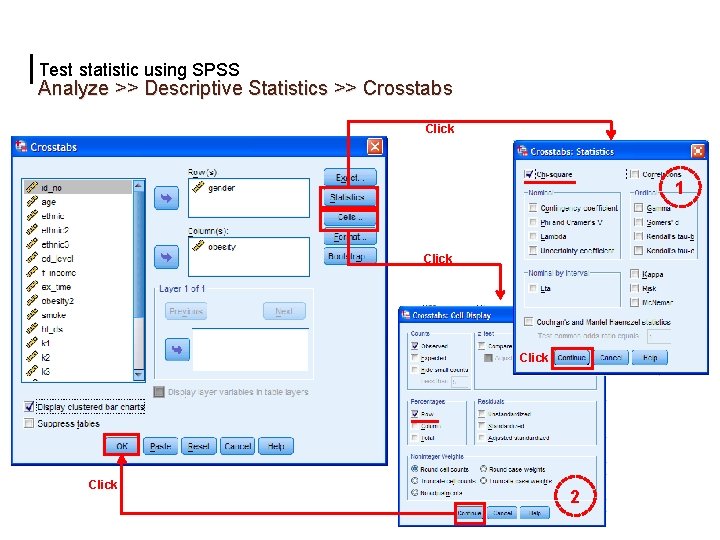
second Test statistic using SPSS Analyze >> Descriptive Statistics >> Crosstabs Click 1 Click 2
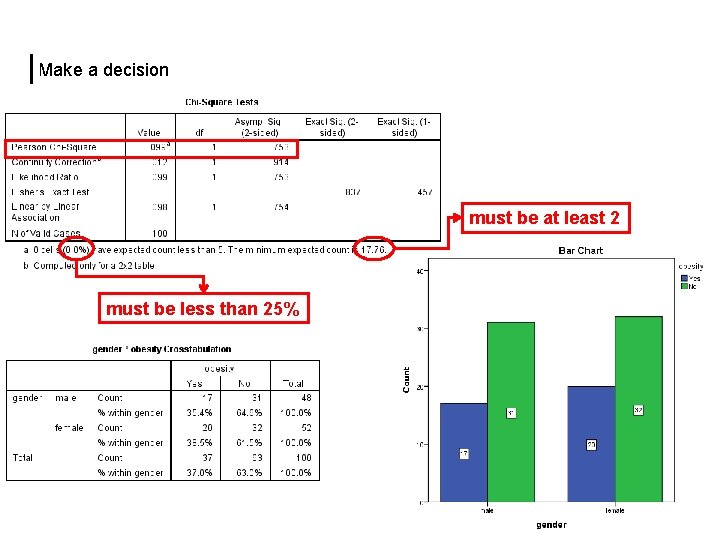
third Make a decision must be at least 2 must be less than 25%
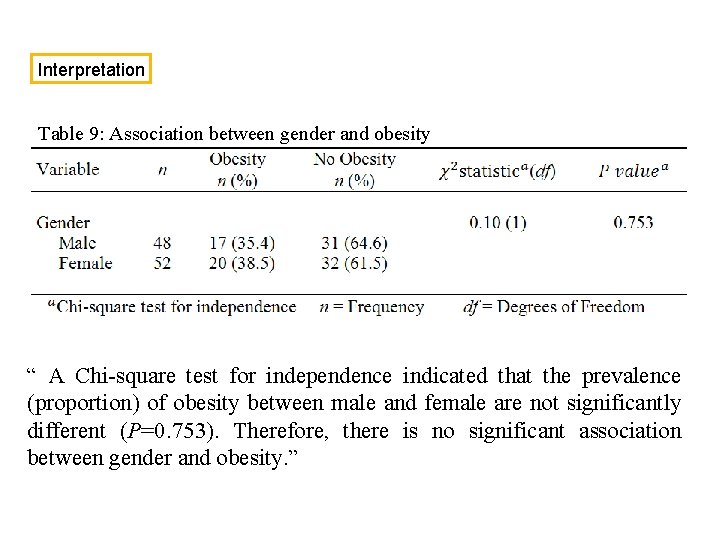
fourth Interpretation Table 9: Association between gender and obesity “ A Chi-square test for independence indicated that the prevalence (proportion) of obesity between male and female are not significantly different (P=0. 753). Therefore, there is no significant association between gender and obesity. ”
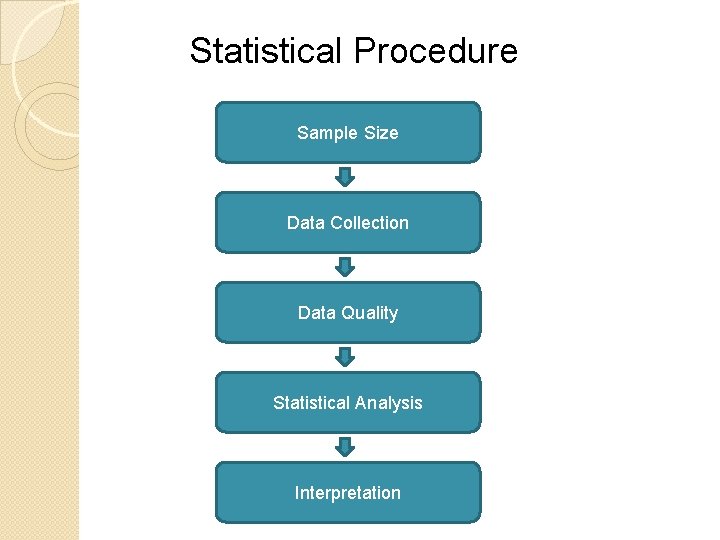
Statistical Procedure Sample Size Data Collection Data Quality Statistical Analysis Interpretation
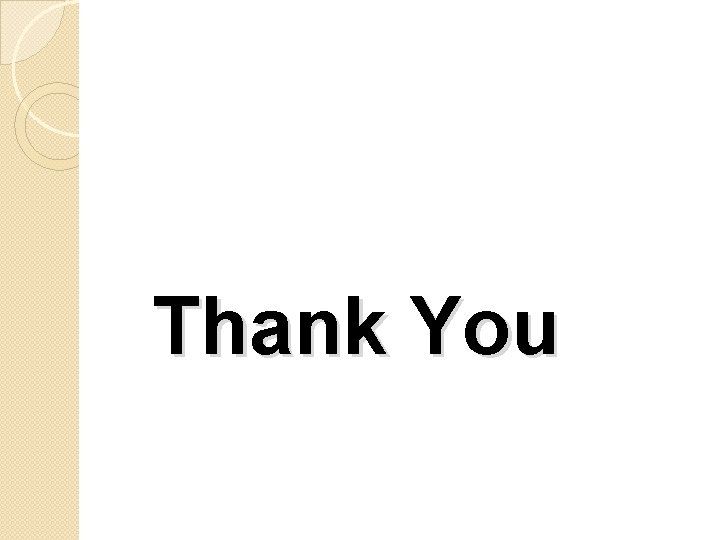
Thank You
Introduction to statistics what is statistics
Introduction to statistical quality control montgomery
Introduction to quantum statistical mechanics
Introduction to statistical quality control
Statistical analysis system
Ascenex
Preserving statistical validity in adaptive data analysis
Multivariate statistical analysis
Cowan statistical data analysis pdf
Statistical business analysis
Marketing analytics software r
State bayes theorem
Statistical analysis of experimental data
Introduction to statistics worksheet
Introduction to statistics and some basic concepts
Introduction to descriptive statistics
Business statistics chapter 1
Introduction to bayesian statistics
Introduction to elementary statistics
Statistics a gentle introduction
A modern introduction to probability and statistics
Chapter 1 introduction to statistics
Ap biology statistics
Introduction to statistics chapter 3 answers
Introduction to educational statistics
Objectives of time series
Government statistical service graduate scheme
Using statistical measures to compare populations
Partition function in statistical mechanics
Statistical mechanics
Equipartition theorem statement
Statistical displays
Types of statistical questions
Define statistical time division multiplexing.
Statistical thermodynamics is a study of
What is microcanonical ensemble
Parametric test and non parametric test