Handling of Missing Data A regulatory view Ferran
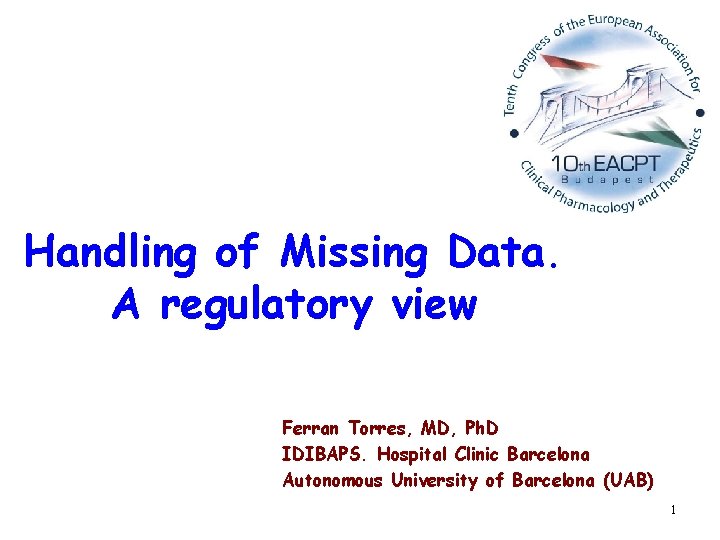
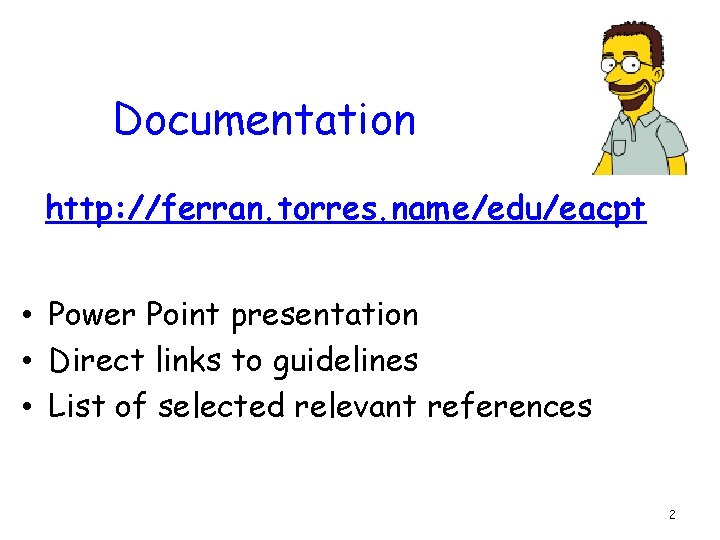
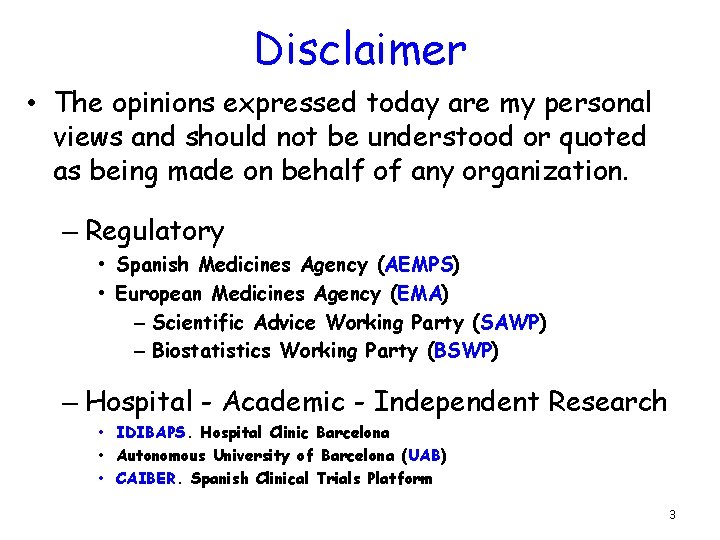
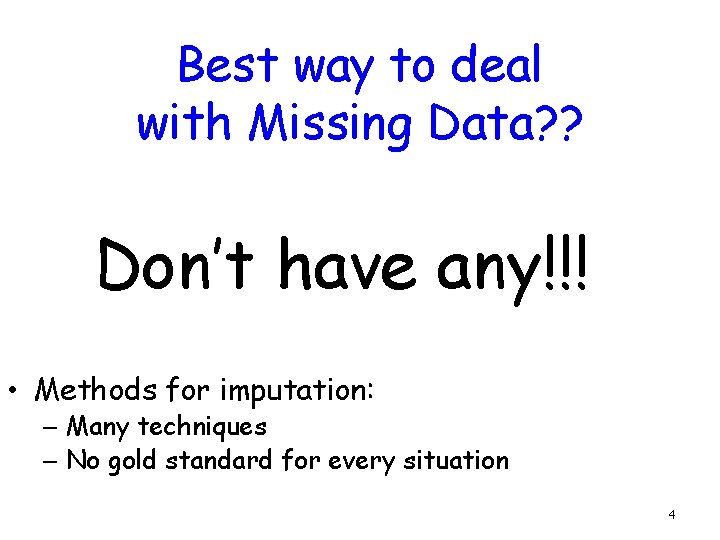
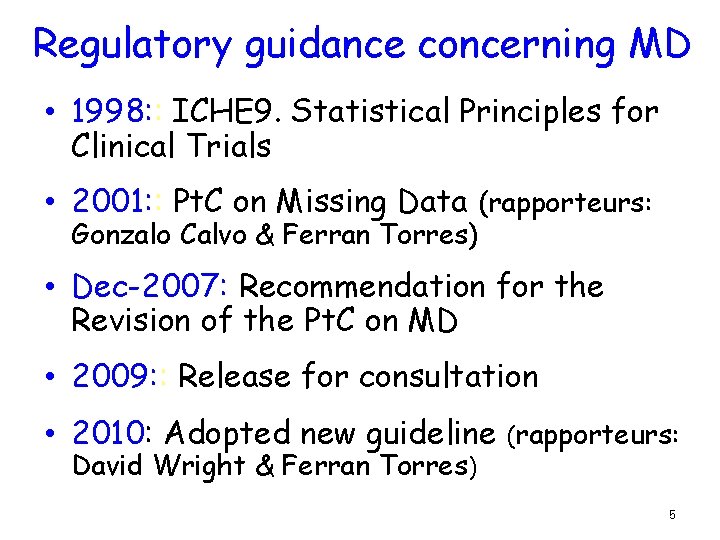
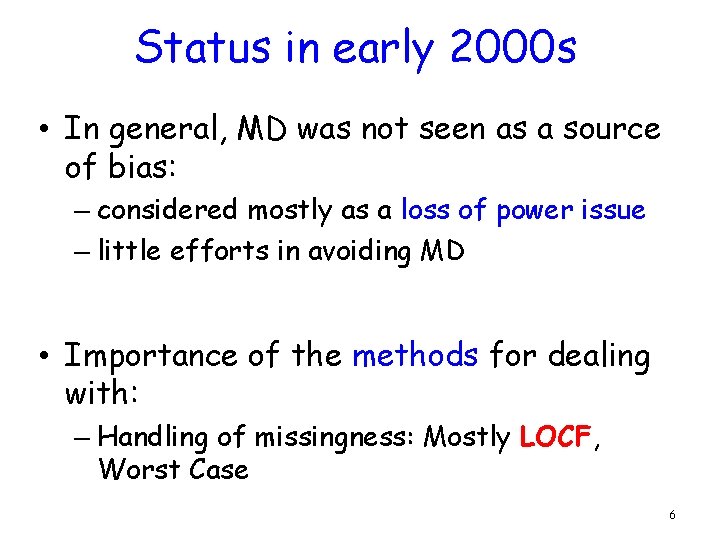
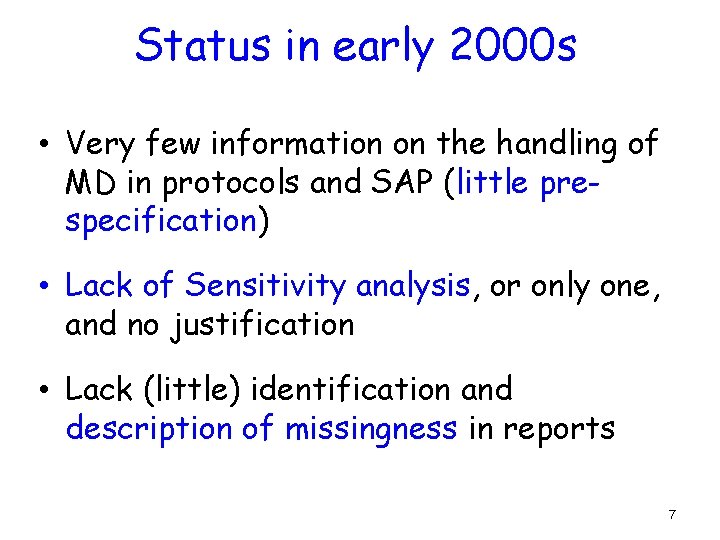
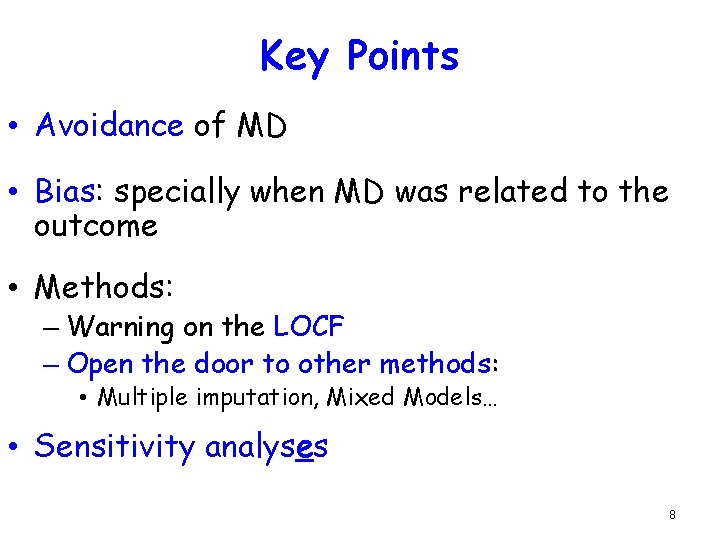
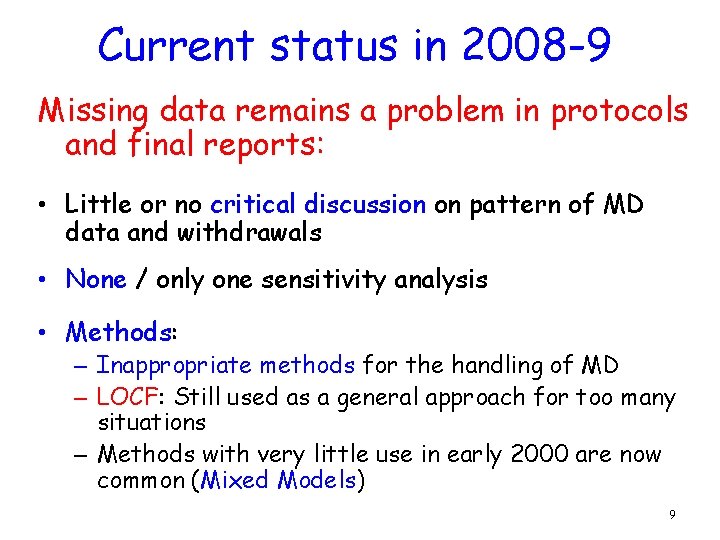
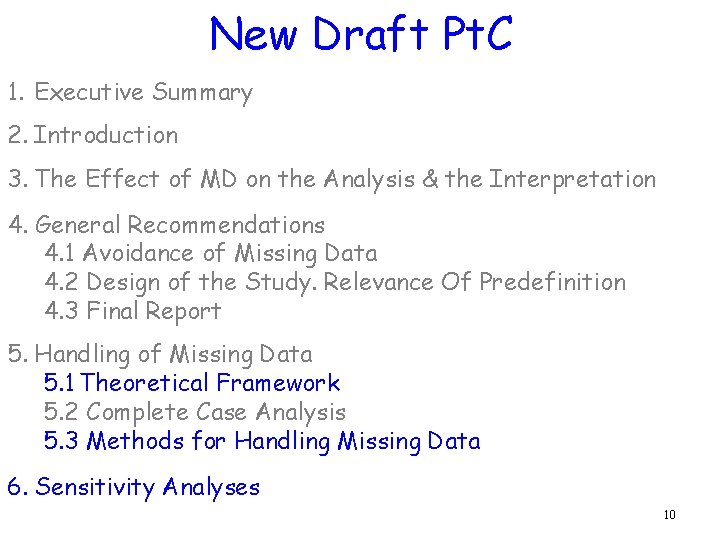
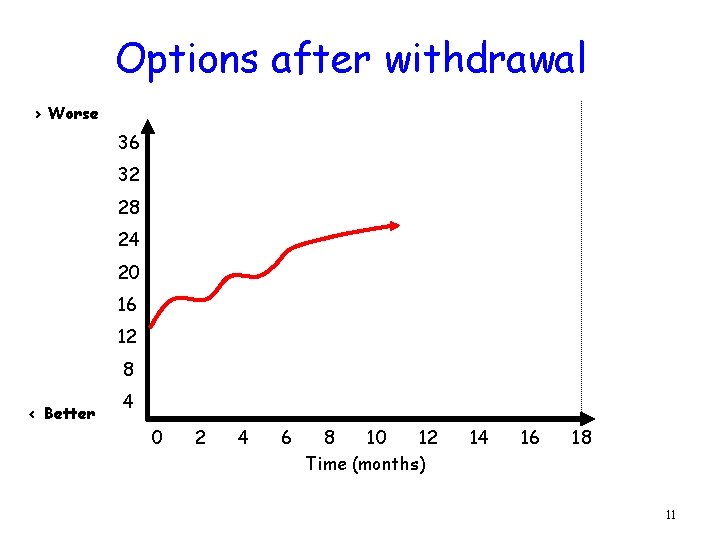
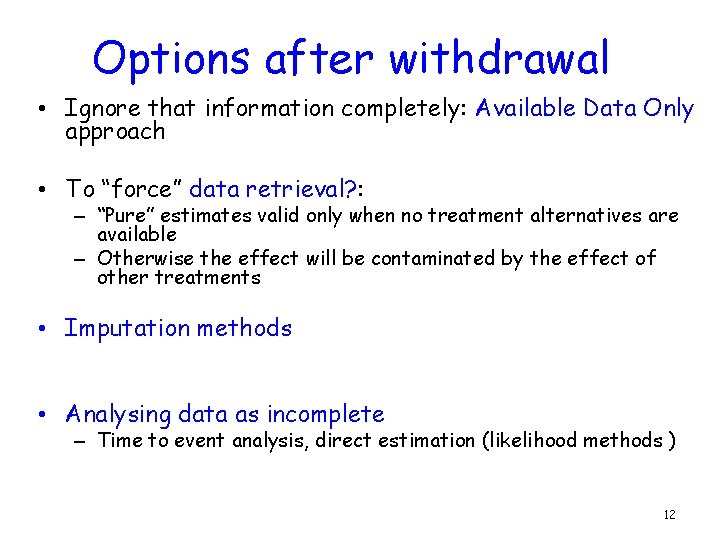
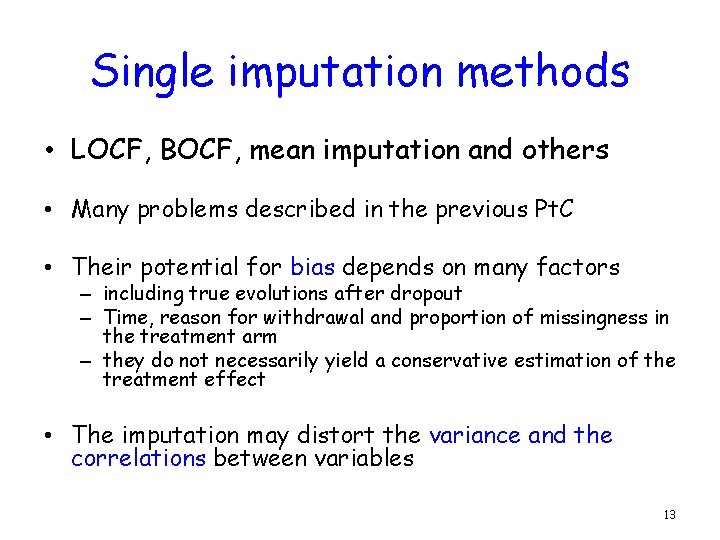
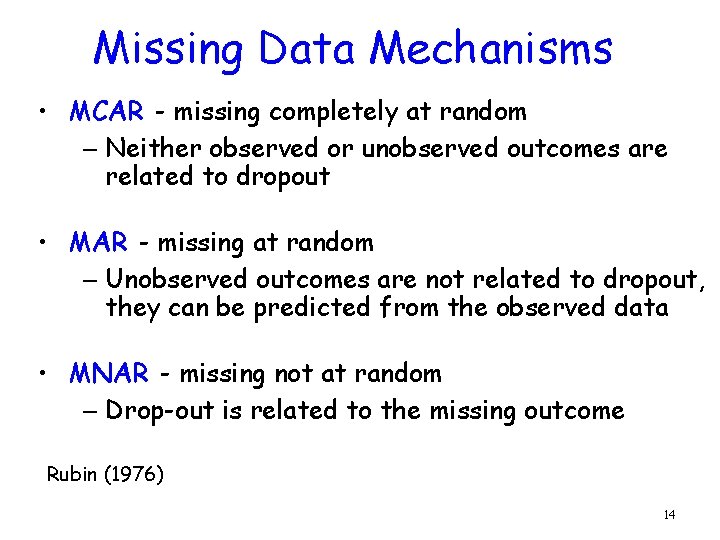
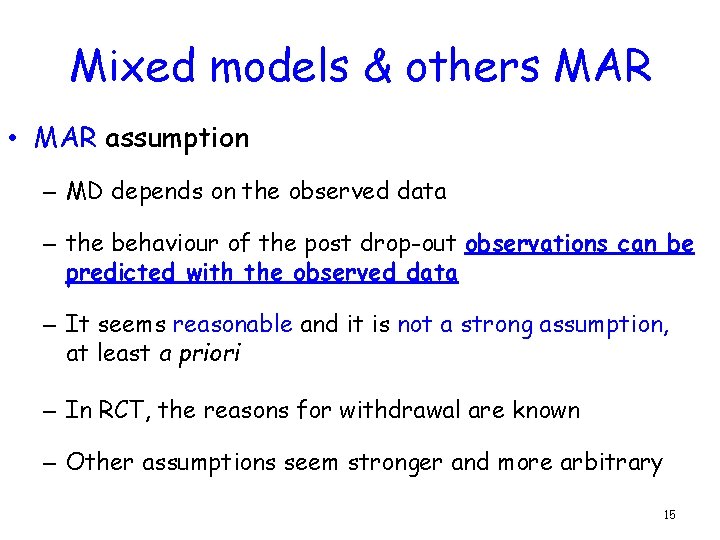
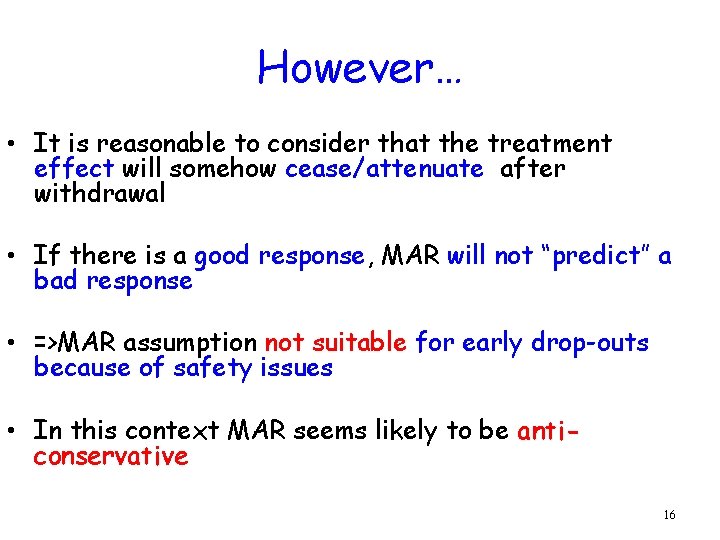
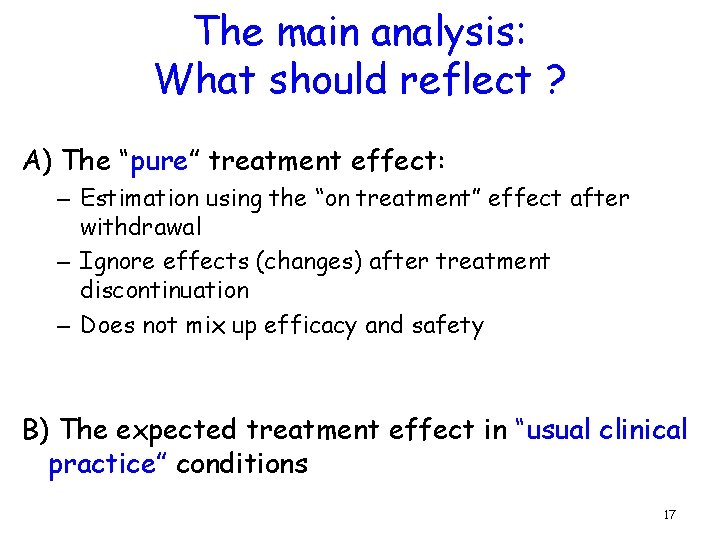
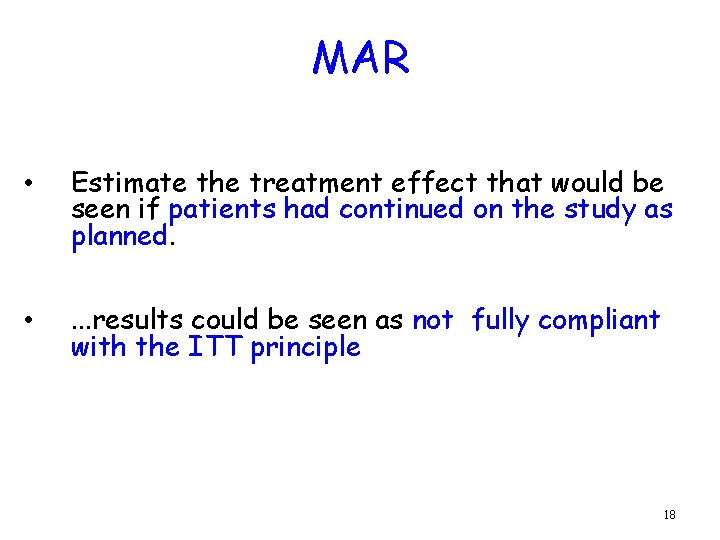
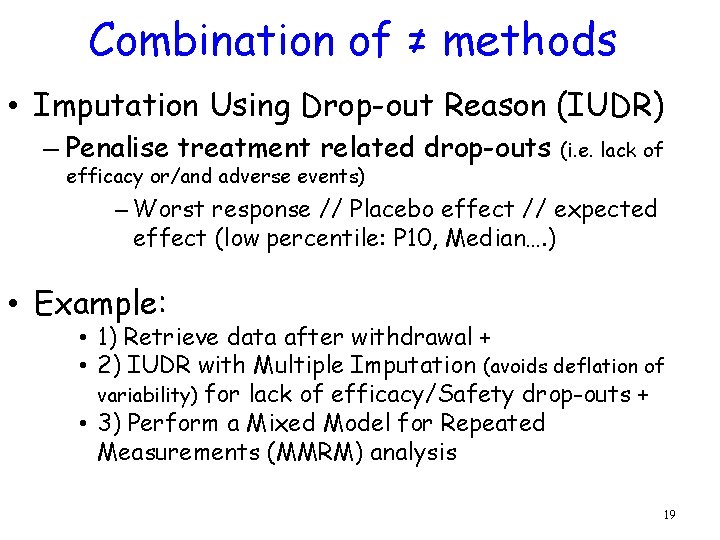
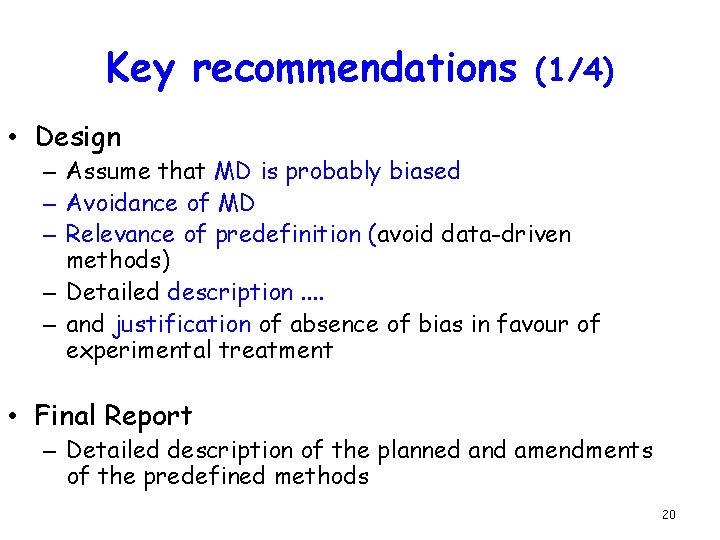
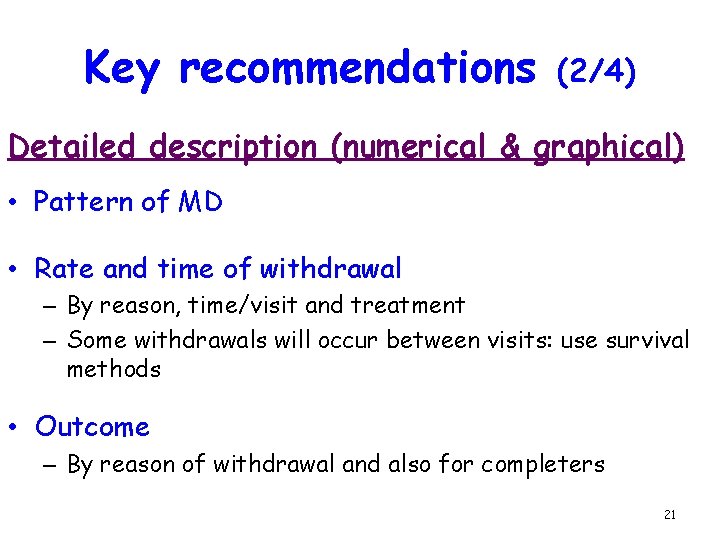
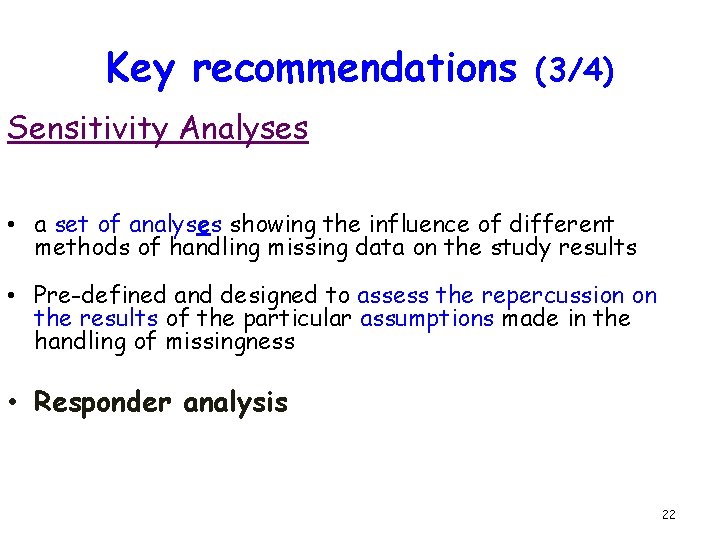
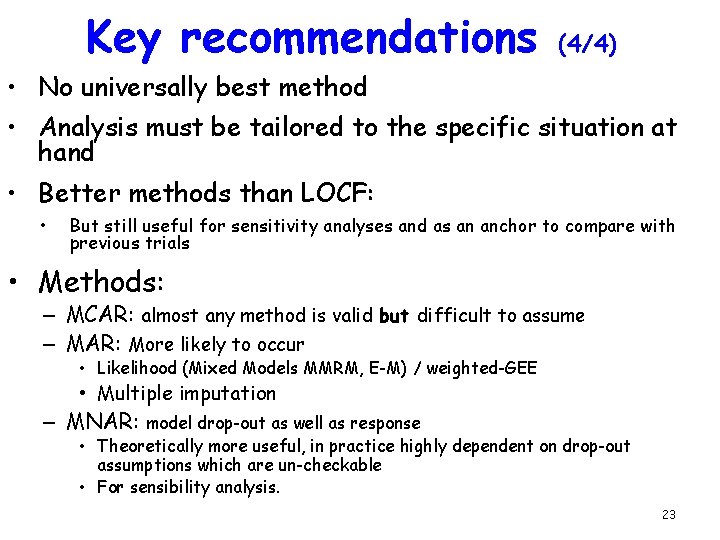
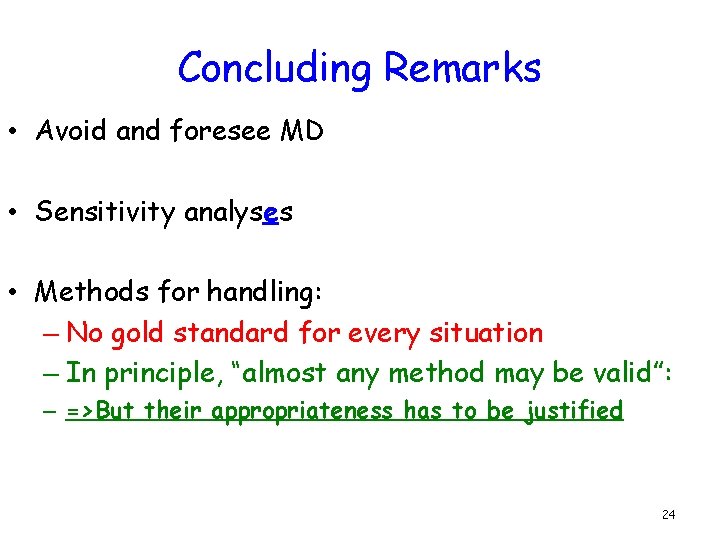
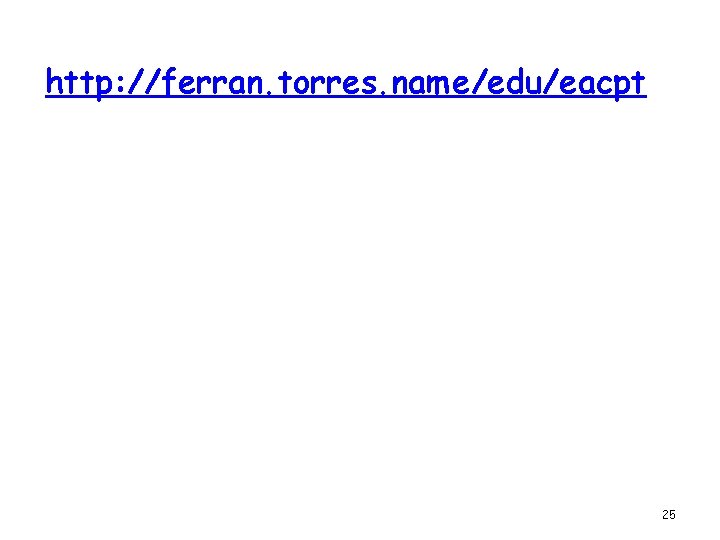
- Slides: 25
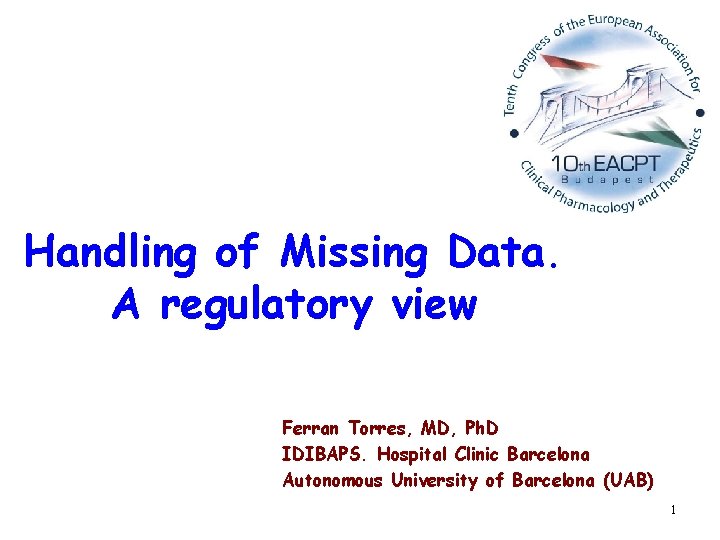
Handling of Missing Data. A regulatory view Ferran Torres, MD, Ph. D IDIBAPS. Hospital Clinic Barcelona Autonomous University of Barcelona (UAB) 1
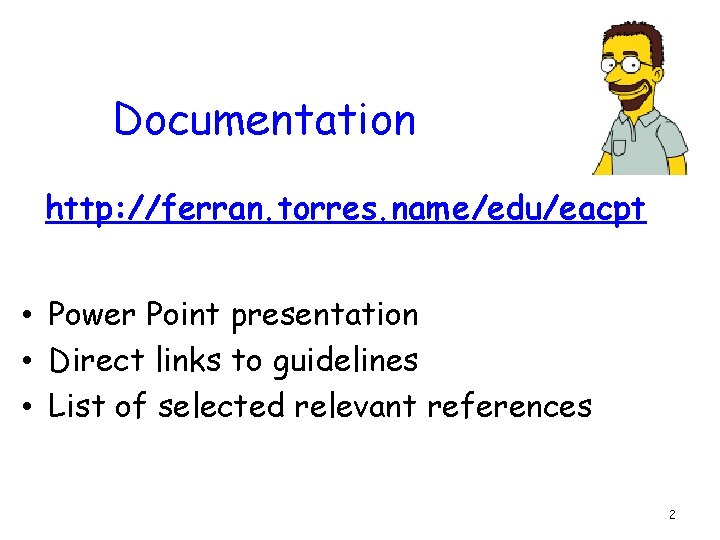
Documentation http: //ferran. torres. name/edu/eacpt • Power Point presentation • Direct links to guidelines • List of selected relevant references 2
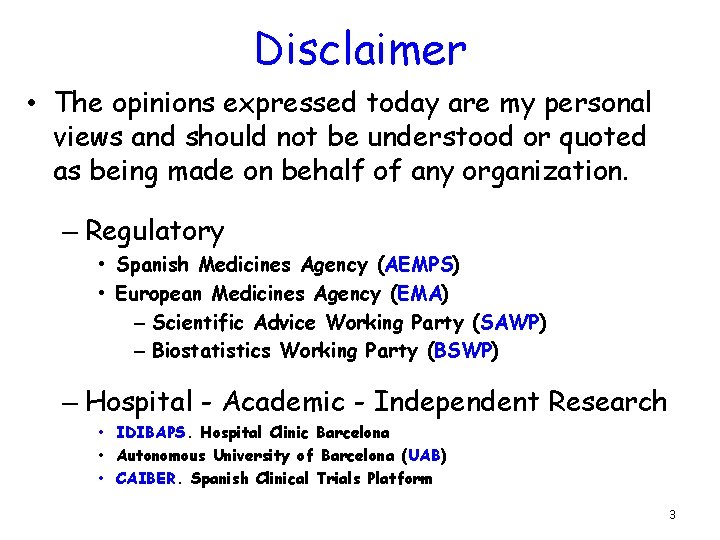
Disclaimer • The opinions expressed today are my personal views and should not be understood or quoted as being made on behalf of any organization. – Regulatory • Spanish Medicines Agency (AEMPS) • European Medicines Agency (EMA) – Scientific Advice Working Party (SAWP) – Biostatistics Working Party (BSWP) – Hospital - Academic - Independent Research • IDIBAPS. Hospital Clinic Barcelona • Autonomous University of Barcelona (UAB) • CAIBER. Spanish Clinical Trials Platform 3
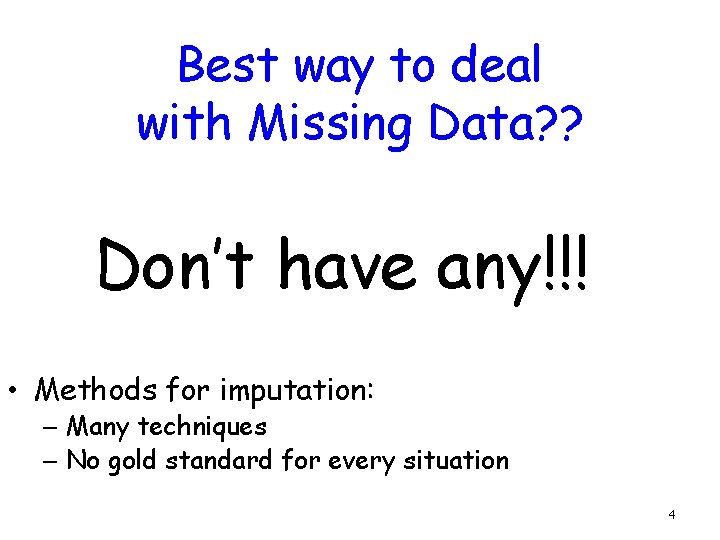
Best way to deal with Missing Data? ? Don’t have any!!! • Methods for imputation: – Many techniques – No gold standard for every situation 4
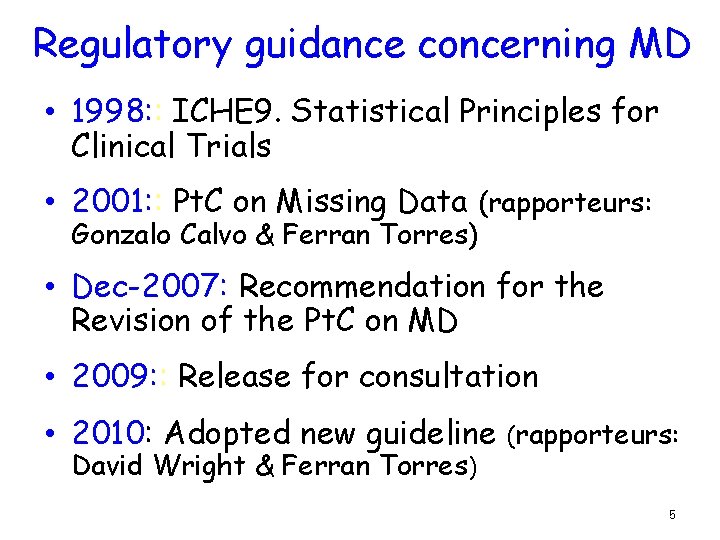
Regulatory guidance concerning MD • 1998: : ICHE 9. Statistical Principles for Clinical Trials • 2001: : Pt. C on Missing Data (rapporteurs: Gonzalo Calvo & Ferran Torres) • Dec-2007: Recommendation for the Revision of the Pt. C on MD • 2009: : Release for consultation • 2010: Adopted new guideline (rapporteurs: David Wright & Ferran Torres) 5
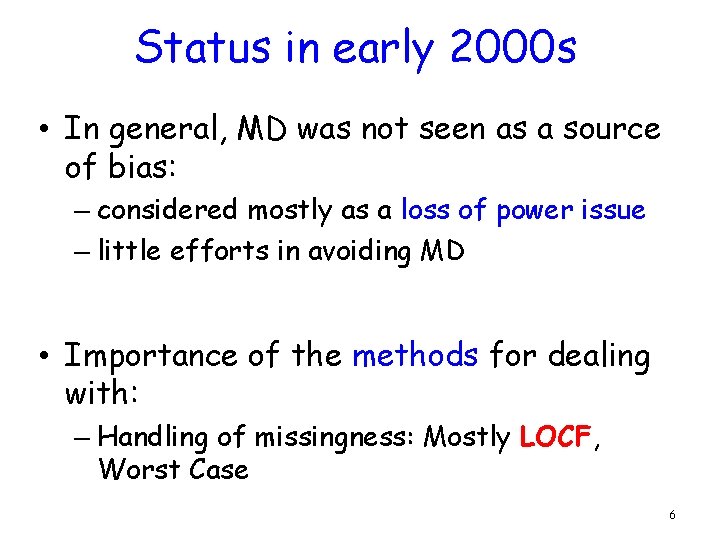
Status in early 2000 s • In general, MD was not seen as a source of bias: – considered mostly as a loss of power issue – little efforts in avoiding MD • Importance of the methods for dealing with: – Handling of missingness: Mostly LOCF, Worst Case 6
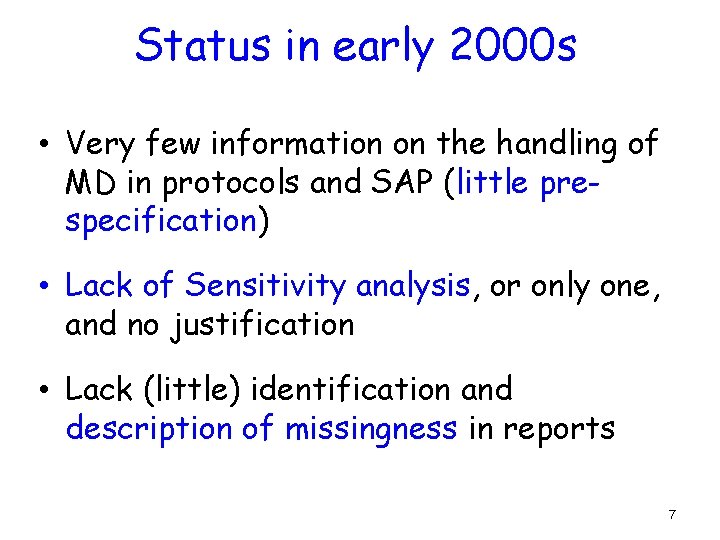
Status in early 2000 s • Very few information on the handling of MD in protocols and SAP (little prespecification) • Lack of Sensitivity analysis, or only one, and no justification • Lack (little) identification and description of missingness in reports 7
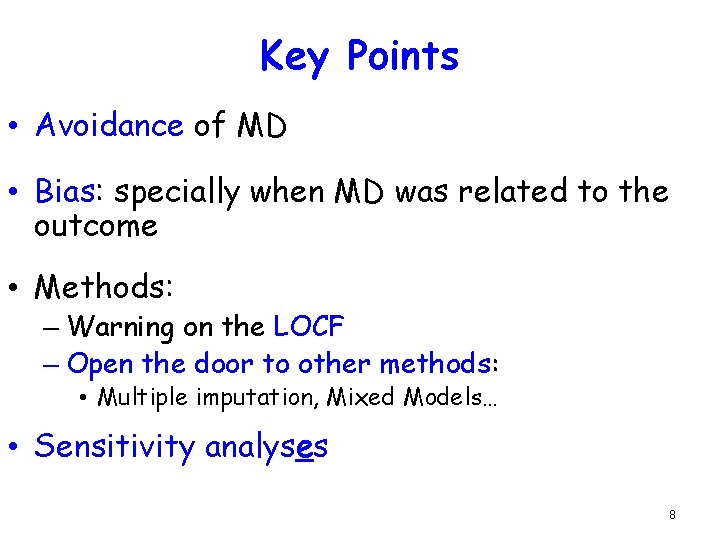
Key Points • Avoidance of MD • Bias: specially when MD was related to the outcome • Methods: – Warning on the LOCF – Open the door to other methods: • Multiple imputation, Mixed Models… • Sensitivity analyses 8
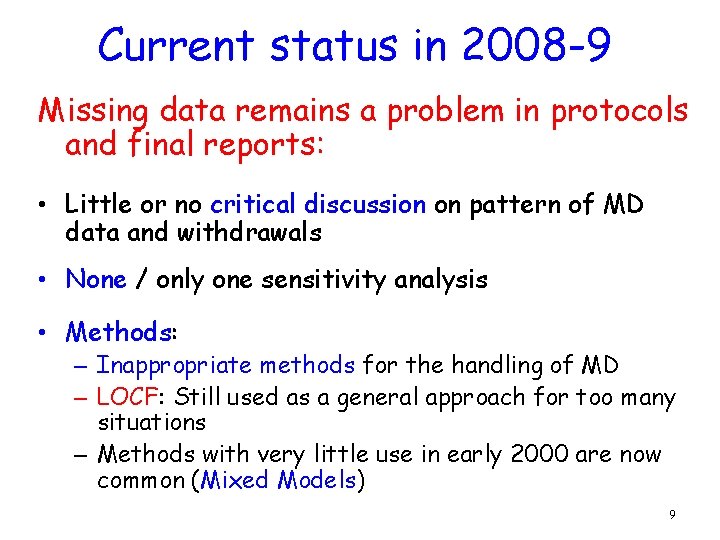
Current status in 2008 -9 Missing data remains a problem in protocols and final reports: • Little or no critical discussion on pattern of MD data and withdrawals • None / only one sensitivity analysis • Methods: – Inappropriate methods for the handling of MD – LOCF: Still used as a general approach for too many situations – Methods with very little use in early 2000 are now common (Mixed Models) 9
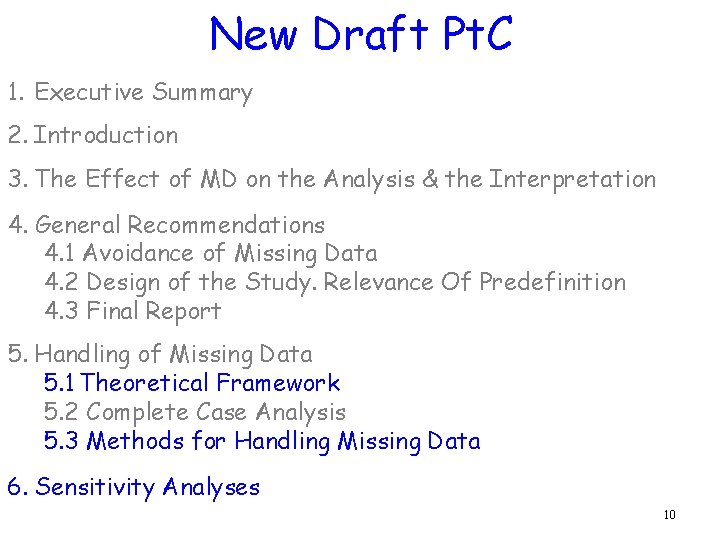
New Draft Pt. C 1. Executive Summary 2. Introduction 3. The Effect of MD on the Analysis & the Interpretation 4. General Recommendations 4. 1 Avoidance of Missing Data 4. 2 Design of the Study. Relevance Of Predefinition 4. 3 Final Report 5. Handling of Missing Data 5. 1 Theoretical Framework 5. 2 Complete Case Analysis 5. 3 Methods for Handling Missing Data 6. Sensitivity Analyses 10
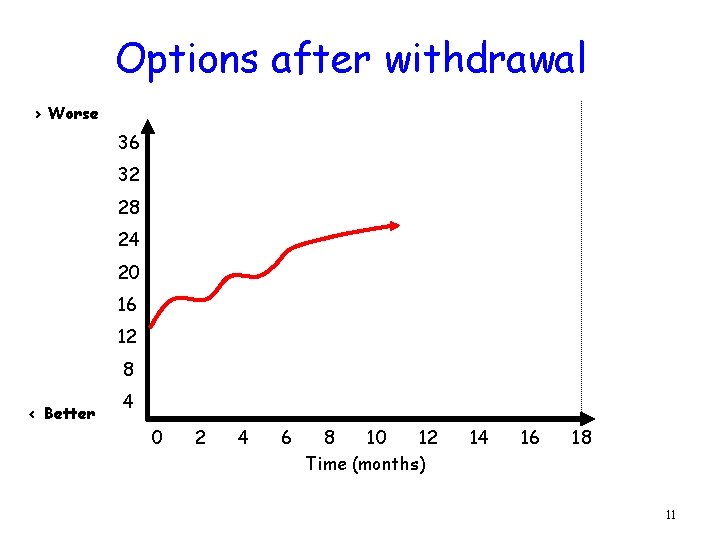
Options after withdrawal > Worse 36 32 28 24 20 16 12 8 < Better 4 0 2 4 6 8 10 12 Time (months) 14 16 18 11
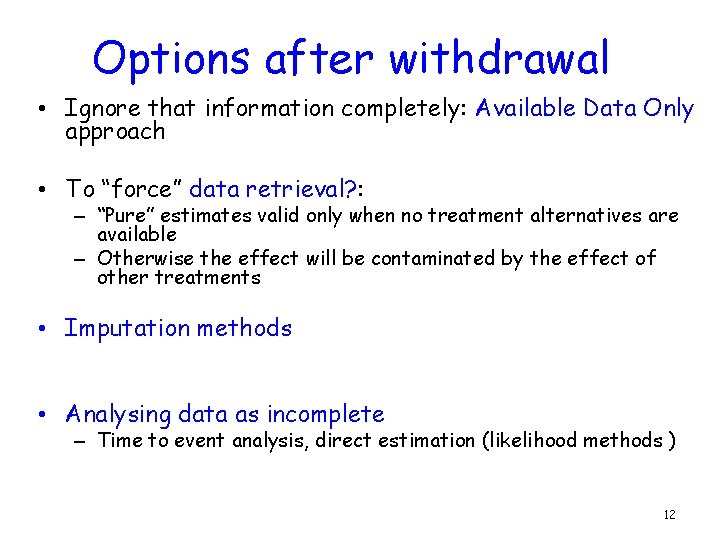
Options after withdrawal • Ignore that information completely: Available Data Only approach • To “force” data retrieval? : – “Pure” estimates valid only when no treatment alternatives are available – Otherwise the effect will be contaminated by the effect of other treatments • Imputation methods • Analysing data as incomplete – Time to event analysis, direct estimation (likelihood methods ) 12
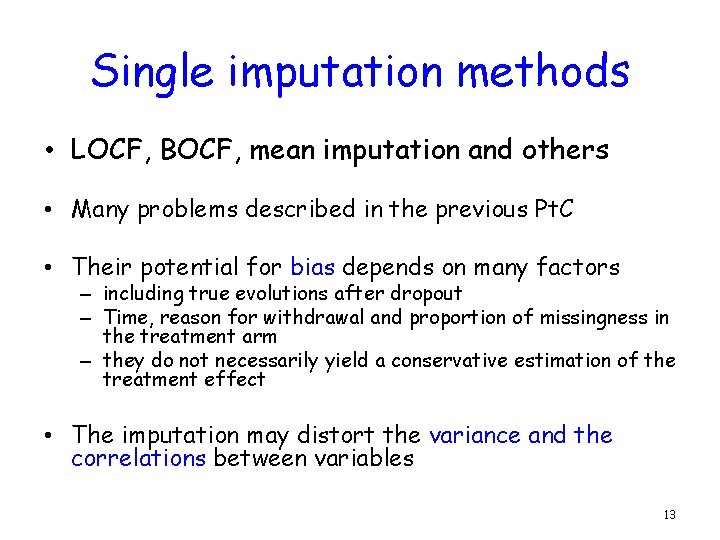
Single imputation methods • LOCF, BOCF, mean imputation and others • Many problems described in the previous Pt. C • Their potential for bias depends on many factors – including true evolutions after dropout – Time, reason for withdrawal and proportion of missingness in the treatment arm – they do not necessarily yield a conservative estimation of the treatment effect • The imputation may distort the variance and the correlations between variables 13
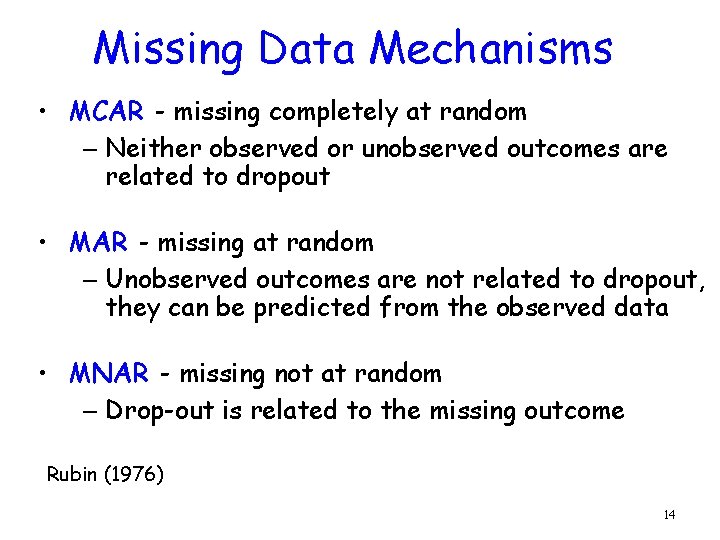
Missing Data Mechanisms • MCAR - missing completely at random – Neither observed or unobserved outcomes are related to dropout • MAR - missing at random – Unobserved outcomes are not related to dropout, they can be predicted from the observed data • MNAR - missing not at random – Drop-out is related to the missing outcome Rubin (1976) 14
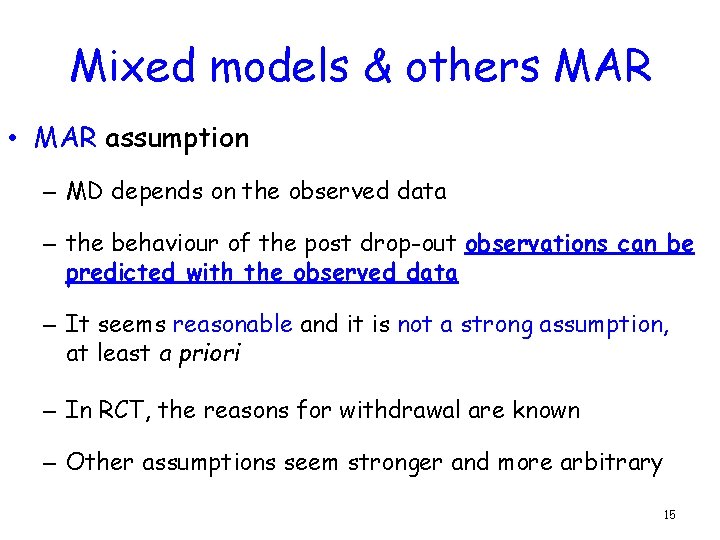
Mixed models & others MAR • MAR assumption – MD depends on the observed data – the behaviour of the post drop-out observations can be predicted with the observed data – It seems reasonable and it is not a strong assumption, at least a priori – In RCT, the reasons for withdrawal are known – Other assumptions seem stronger and more arbitrary 15
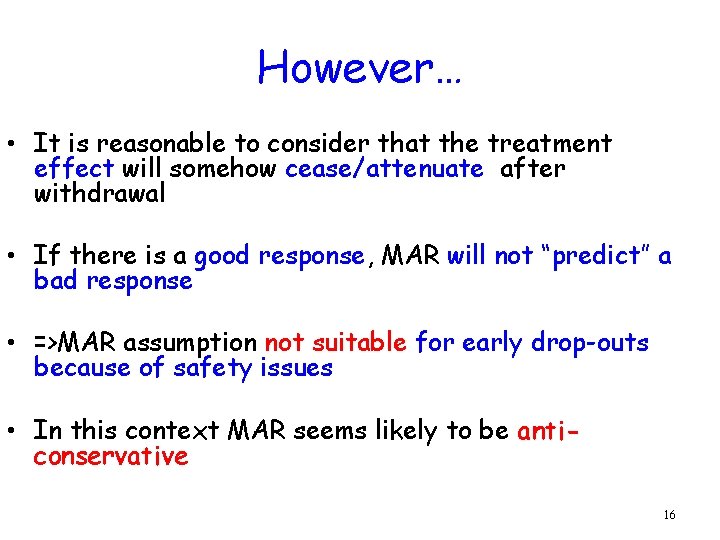
However… • It is reasonable to consider that the treatment effect will somehow cease/attenuate after withdrawal • If there is a good response, MAR will not “predict” a bad response • =>MAR assumption not suitable for early drop-outs because of safety issues • In this context MAR seems likely to be anticonservative 16
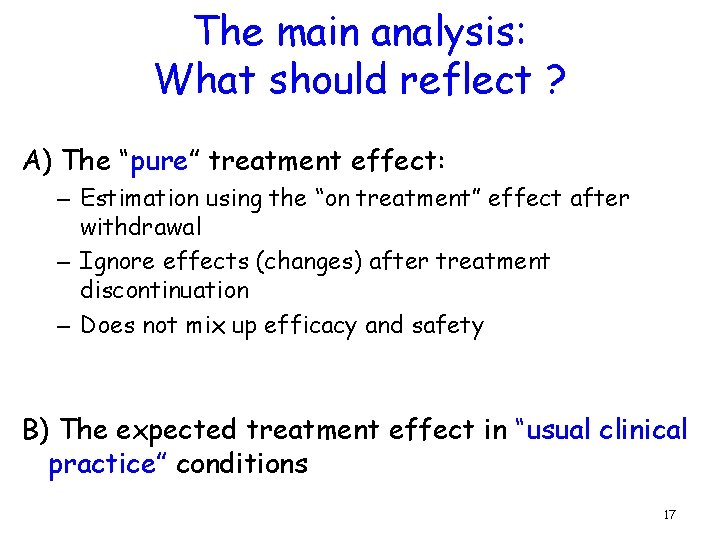
The main analysis: What should reflect ? A) The “pure” treatment effect: – Estimation using the “on treatment” effect after withdrawal – Ignore effects (changes) after treatment discontinuation – Does not mix up efficacy and safety B) The expected treatment effect in “usual clinical practice” conditions 17
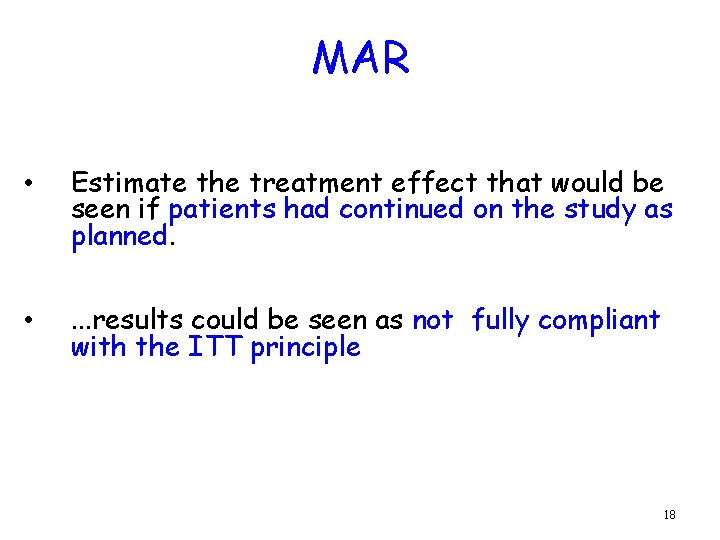
MAR • Estimate the treatment effect that would be seen if patients had continued on the study as planned. • . . . results could be seen as not fully compliant with the ITT principle 18
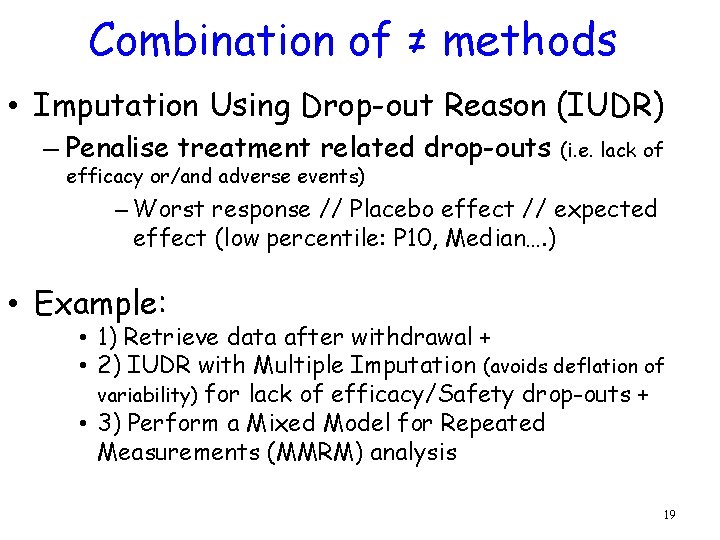
Combination of ≠ methods • Imputation Using Drop-out Reason (IUDR) – Penalise treatment related drop-outs efficacy or/and adverse events) (i. e. lack of – Worst response // Placebo effect // expected effect (low percentile: P 10, Median…. ) • Example: • 1) Retrieve data after withdrawal + • 2) IUDR with Multiple Imputation (avoids deflation of variability) for lack of efficacy/Safety drop-outs + • 3) Perform a Mixed Model for Repeated Measurements (MMRM) analysis 19
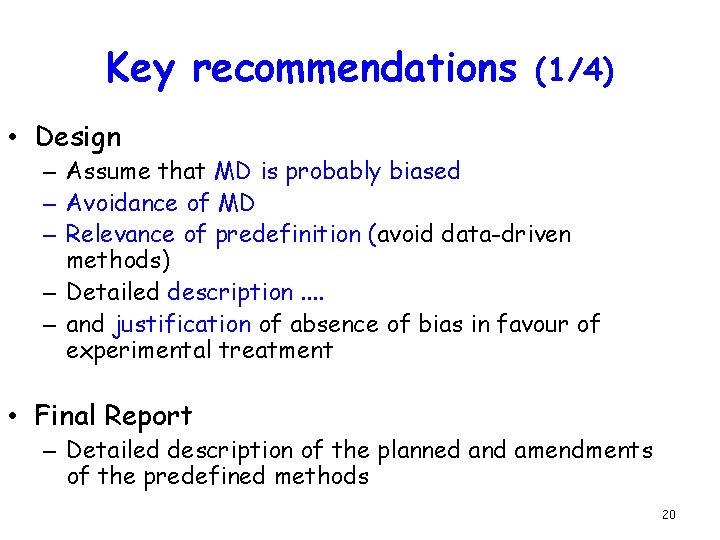
Key recommendations (1/4) • Design – Assume that MD is probably biased – Avoidance of MD – Relevance of predefinition (avoid data-driven methods) – Detailed description. . – and justification of absence of bias in favour of experimental treatment • Final Report – Detailed description of the planned and amendments of the predefined methods 20
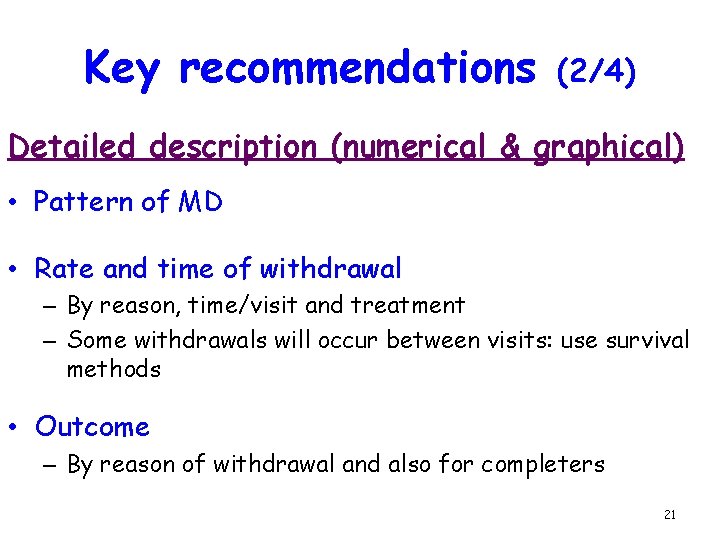
Key recommendations (2/4) Detailed description (numerical & graphical) • Pattern of MD • Rate and time of withdrawal – By reason, time/visit and treatment – Some withdrawals will occur between visits: use survival methods • Outcome – By reason of withdrawal and also for completers 21
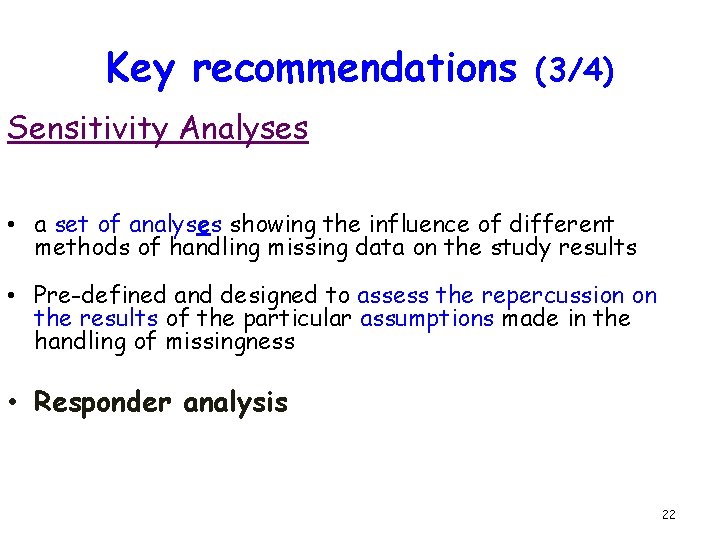
Key recommendations (3/4) Sensitivity Analyses • a set of analyses showing the influence of different methods of handling missing data on the study results • Pre-defined and designed to assess the repercussion on the results of the particular assumptions made in the handling of missingness • Responder analysis 22
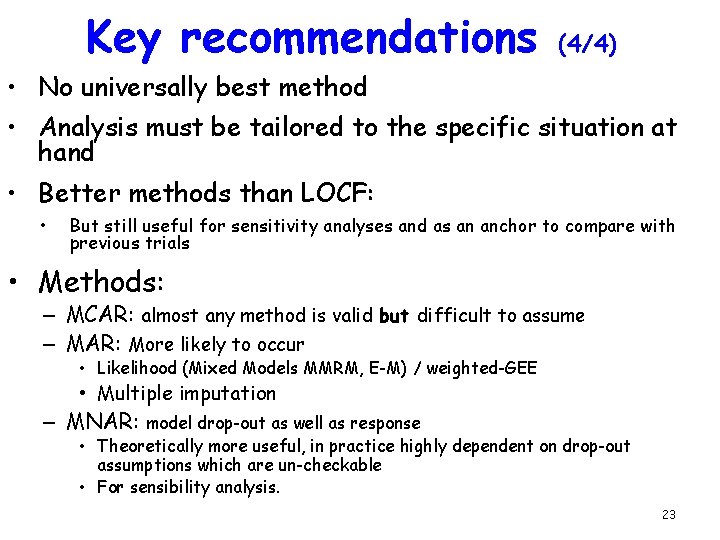
Key recommendations (4/4) • No universally best method • Analysis must be tailored to the specific situation at hand • Better methods than LOCF: • But still useful for sensitivity analyses and as an anchor to compare with previous trials • Methods: – MCAR: almost any method is valid but difficult to assume – MAR: More likely to occur • Likelihood (Mixed Models MMRM, E-M) / weighted-GEE • Multiple imputation – MNAR: model drop-out as well as response • Theoretically more useful, in practice highly dependent on drop-out assumptions which are un-checkable • For sensibility analysis. 23
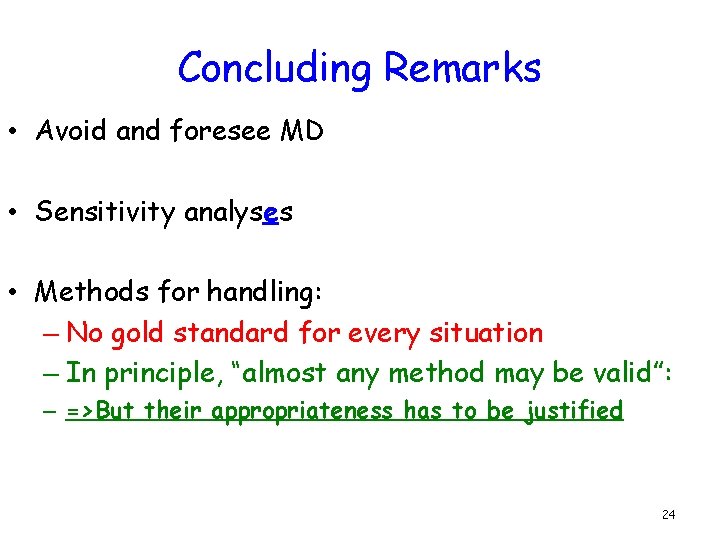
Concluding Remarks • Avoid and foresee MD • Sensitivity analyses • Methods for handling: – No gold standard for every situation – In principle, “almost any method may be valid”: – =>But their appropriateness has to be justified 24
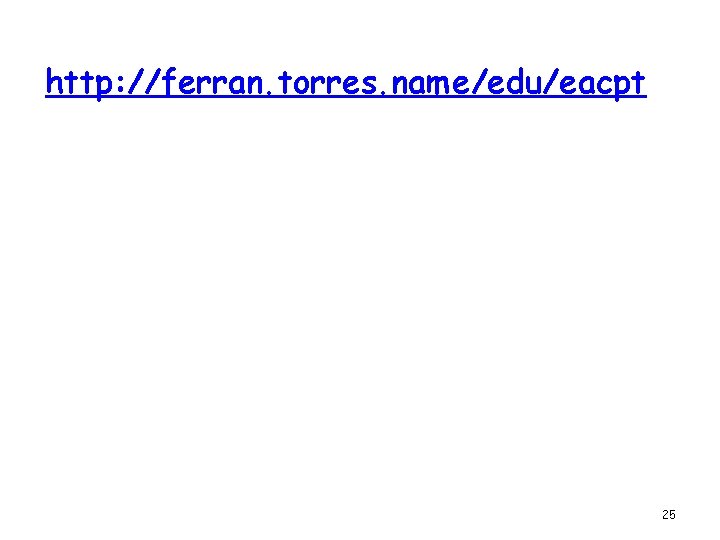
http: //ferran. torres. name/edu/eacpt 25
Handling missing values in python
Aude ferran
Aude ferran
Aude ferran
Pepsinogène
Aude ferran
Aude ferran
Aude ferran
Grib hib
Sens aboral
Caillette jeune ruminant
Ferran ballester
What is missing data in data mining
Sketching missing lines
Stratified sampling gcse
Data handling song
What is the information cycle
Handling data hazards
Mplus fiml
91571926
Argument "fun" is missing, with no default
This orthographic view is directly above the front view
Hidden lines are not generally omitted in a sectional view
Revolved section is a type of section to a
Full section view examples
Bird eye view angle