Greedy Algorithms A short list of categories n
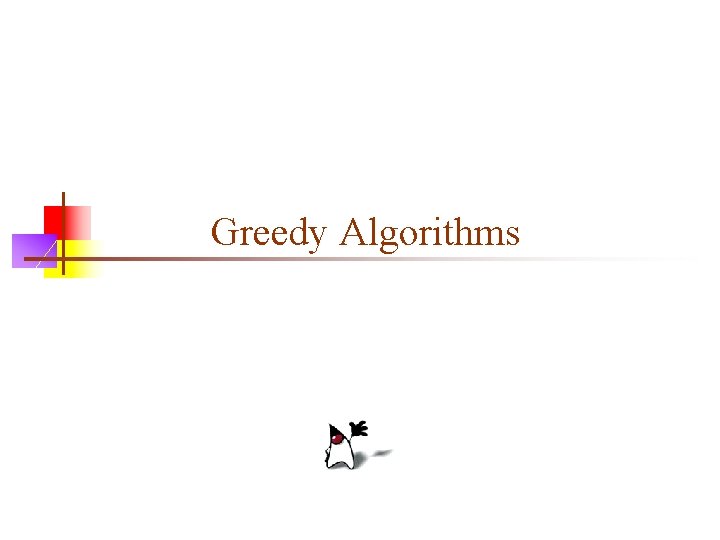
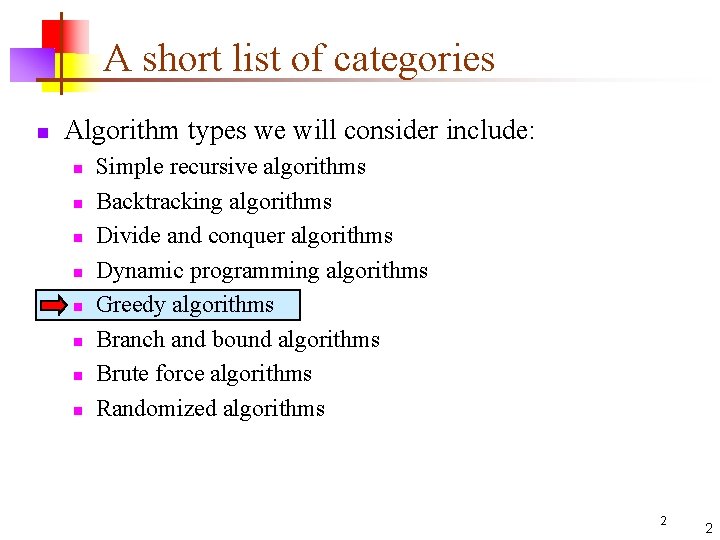
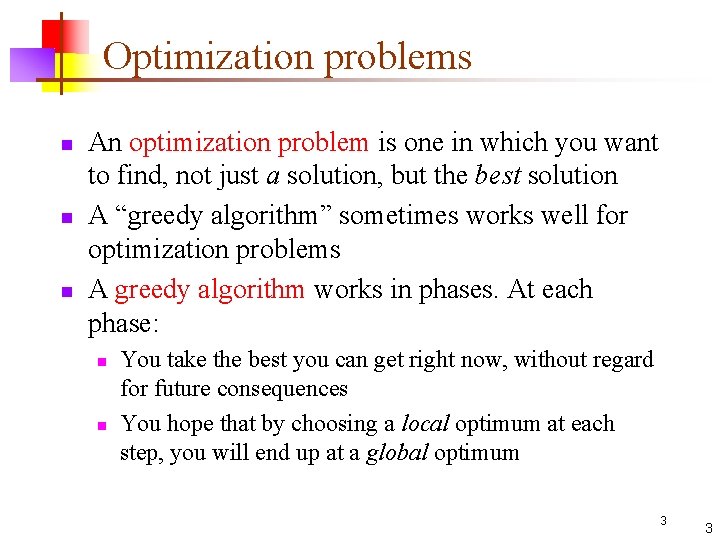
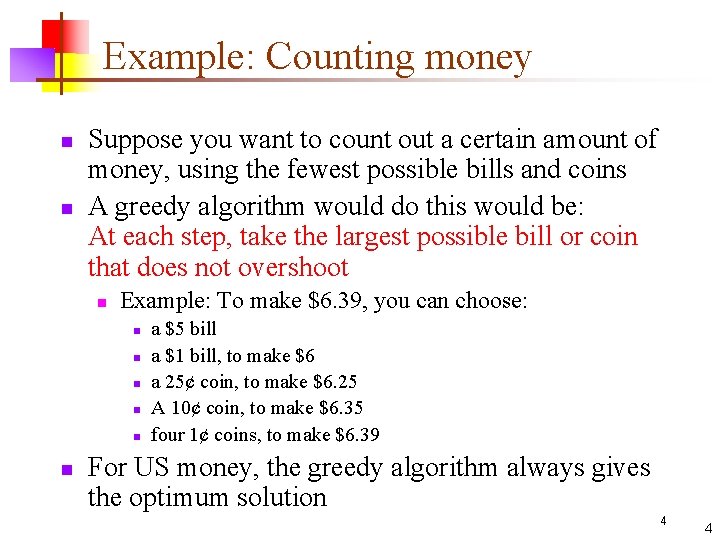
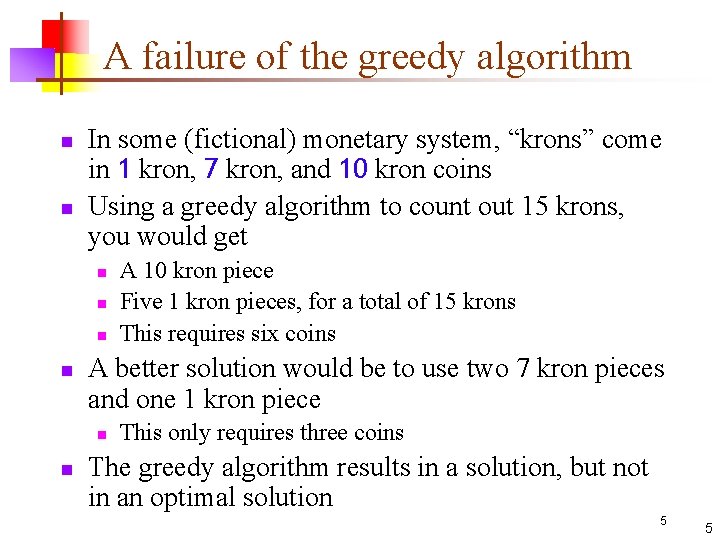
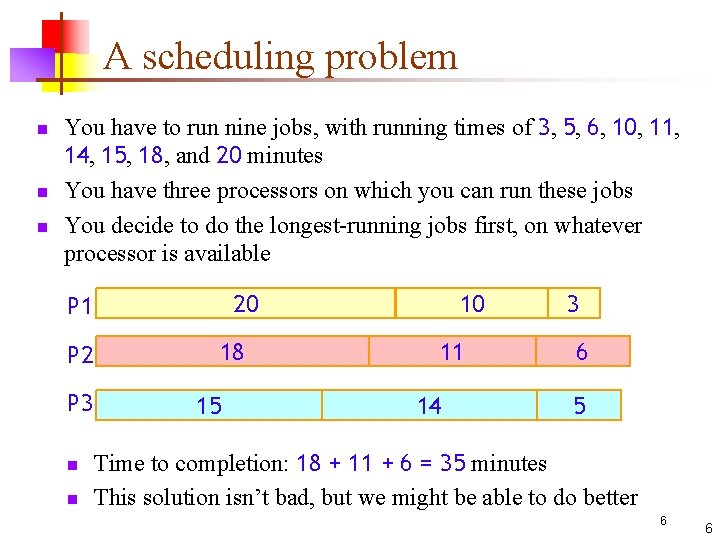
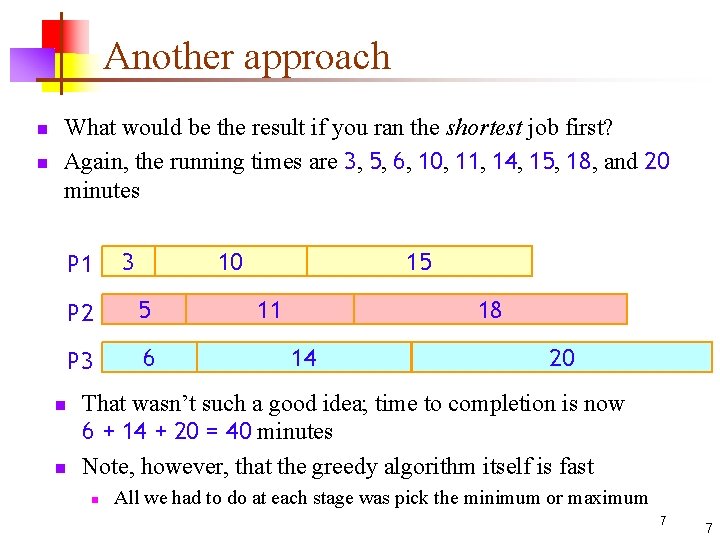
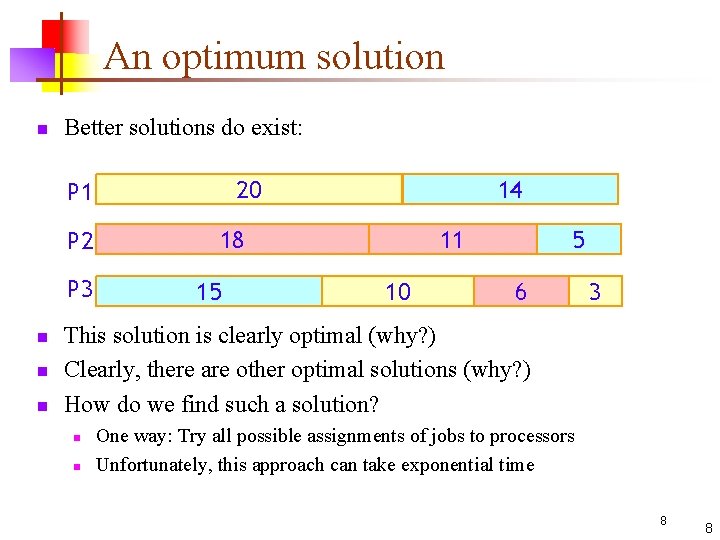
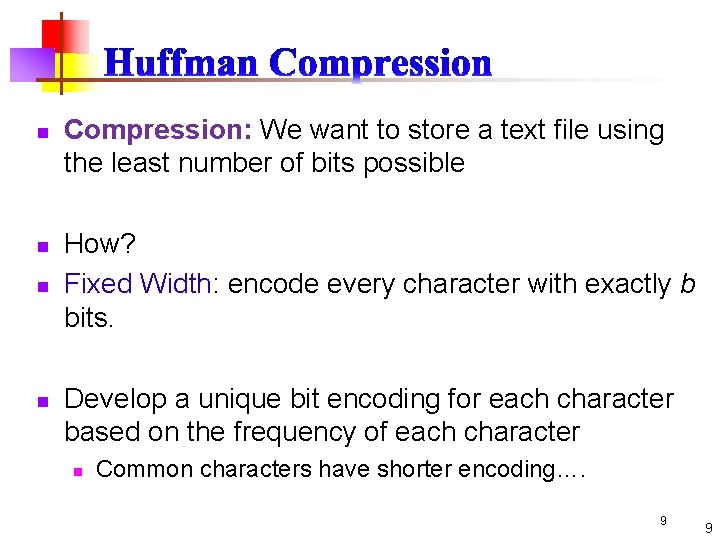
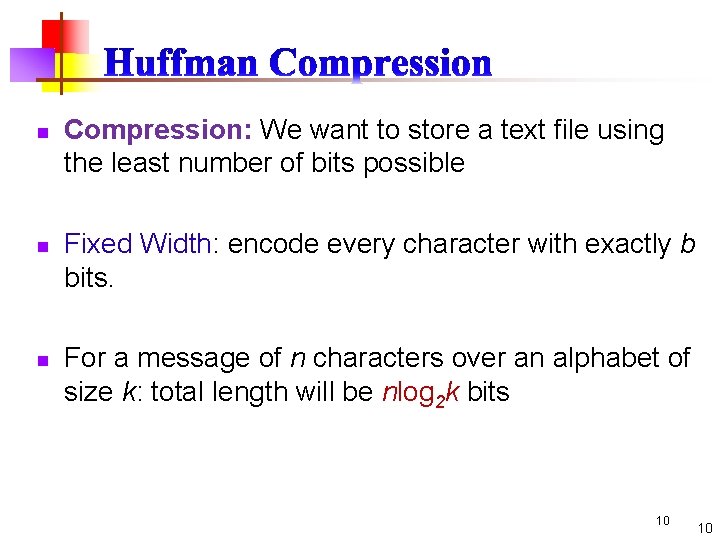
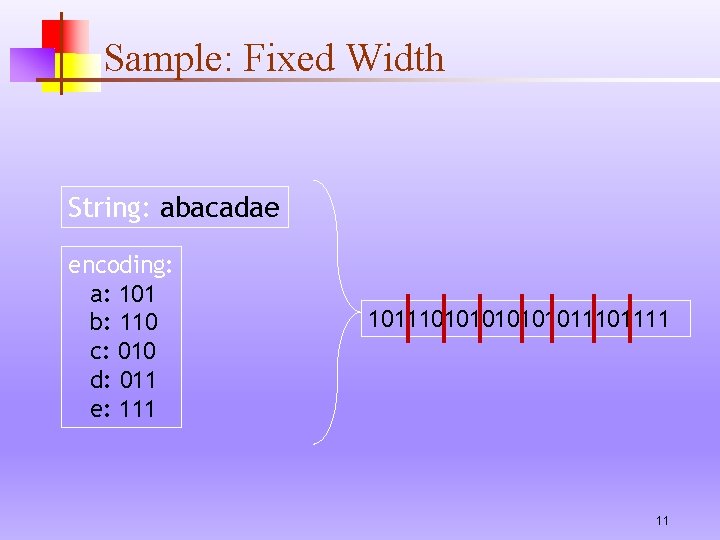
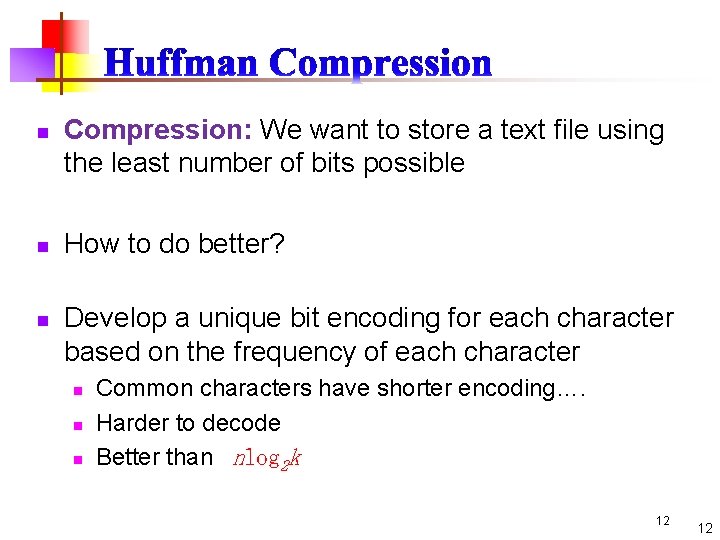
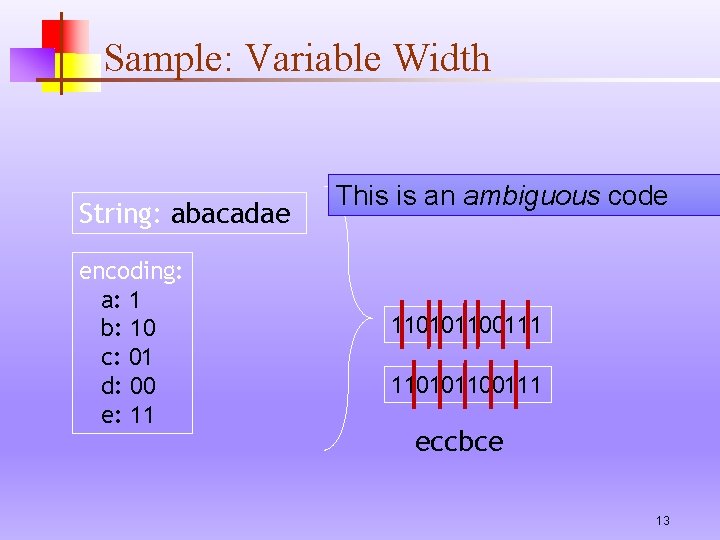
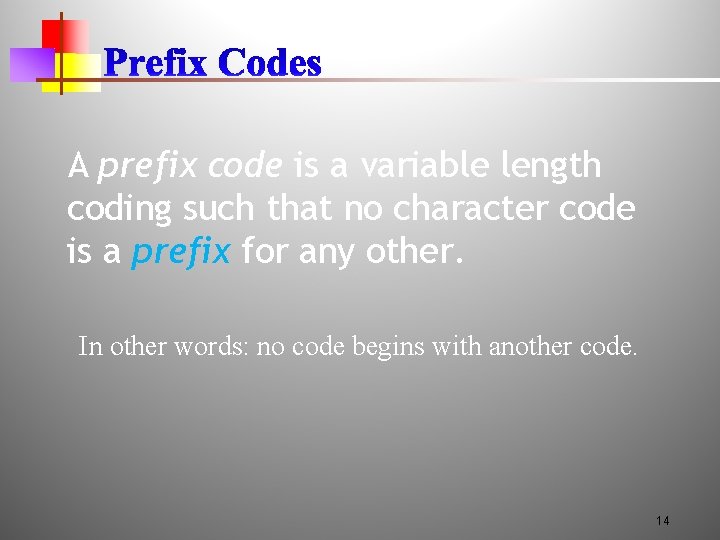
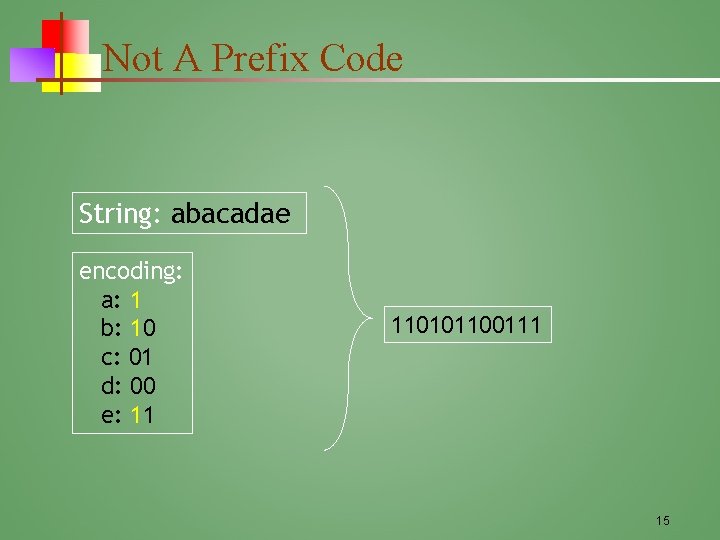
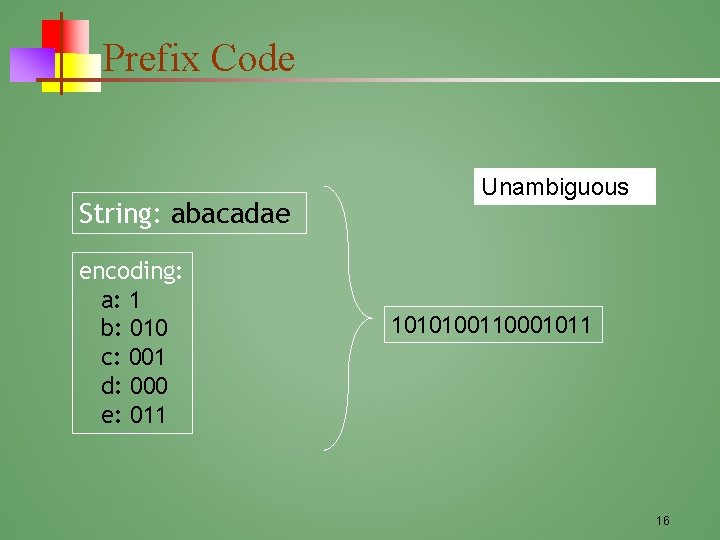
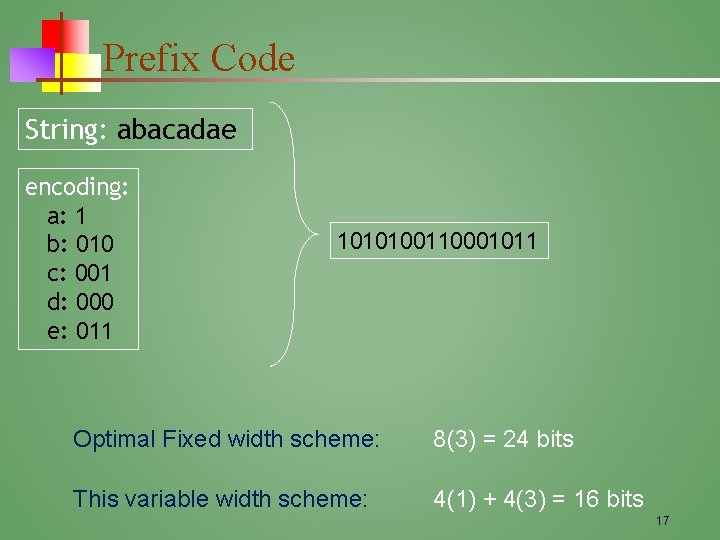
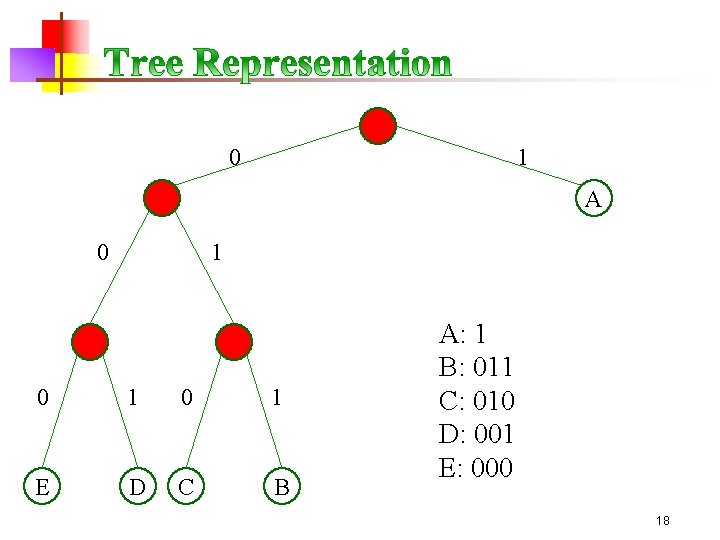
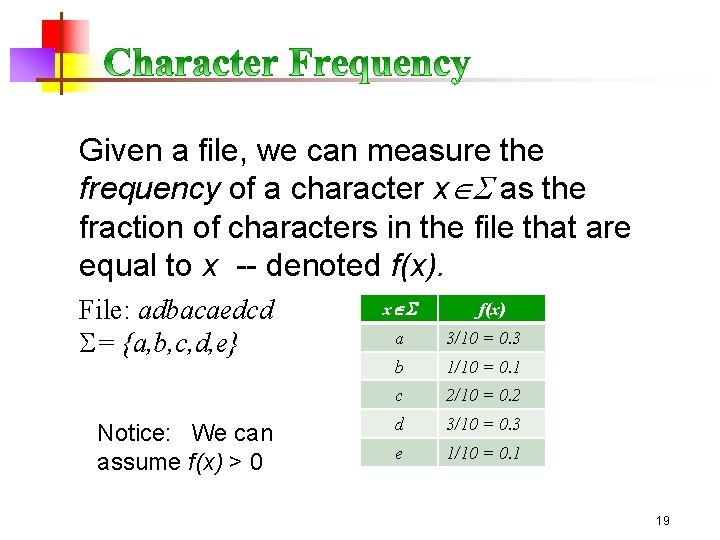
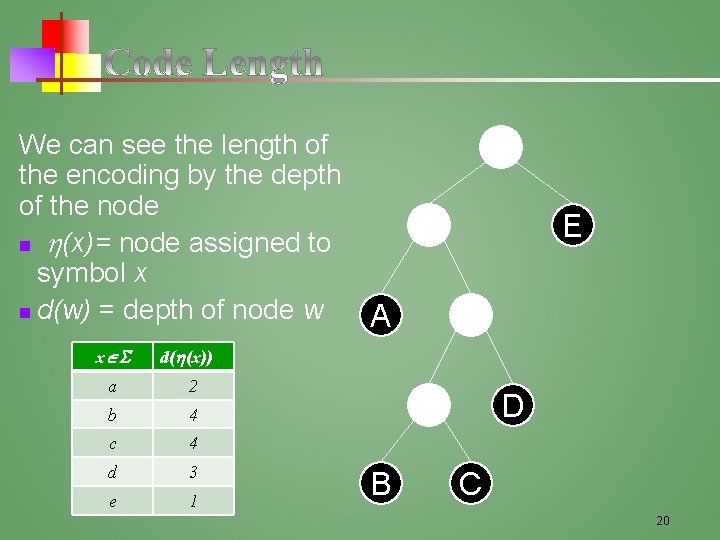
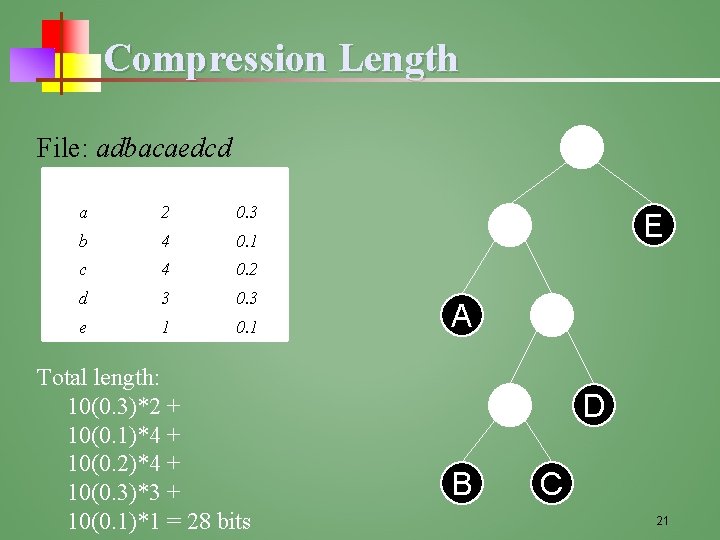
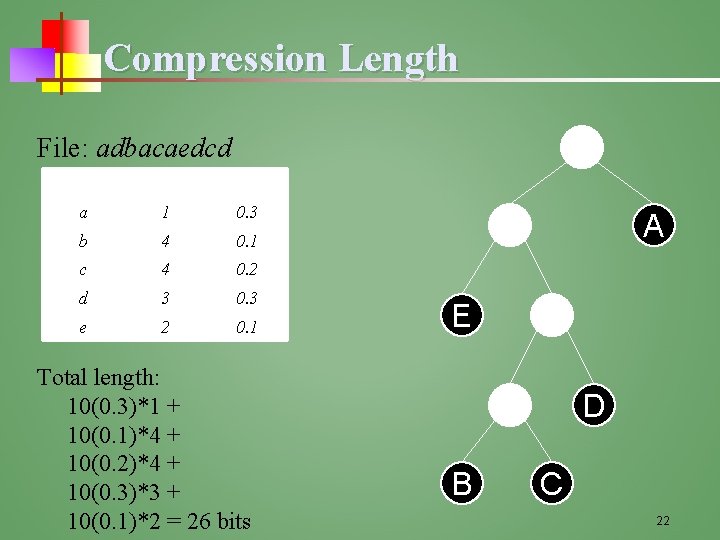
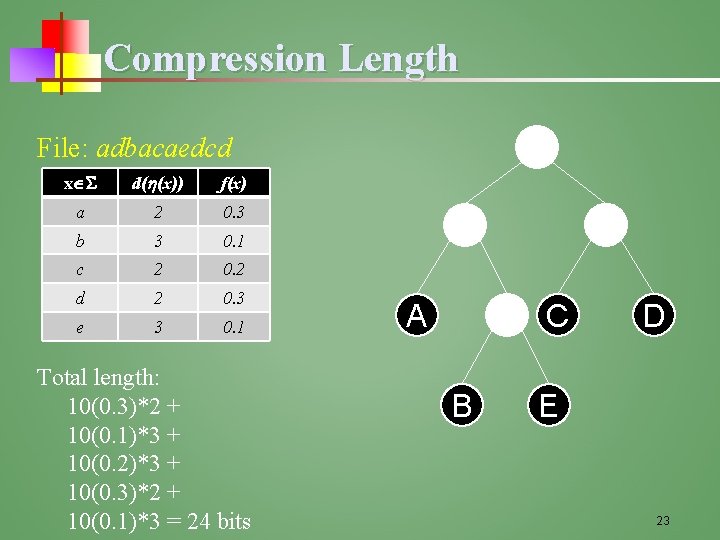
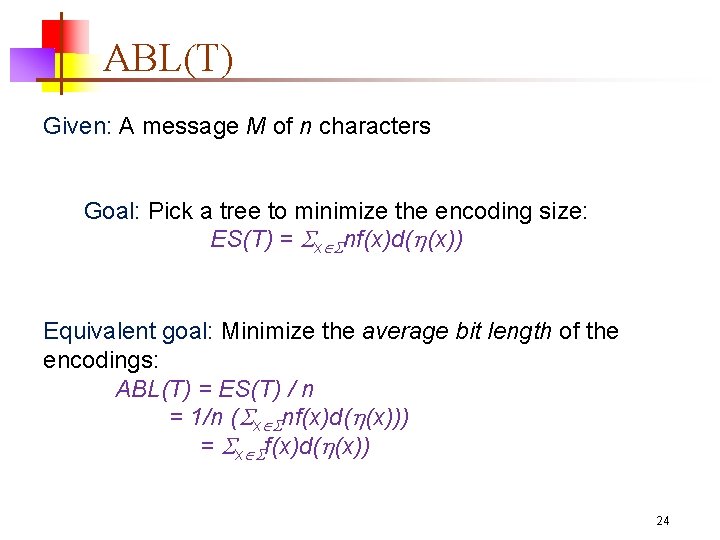
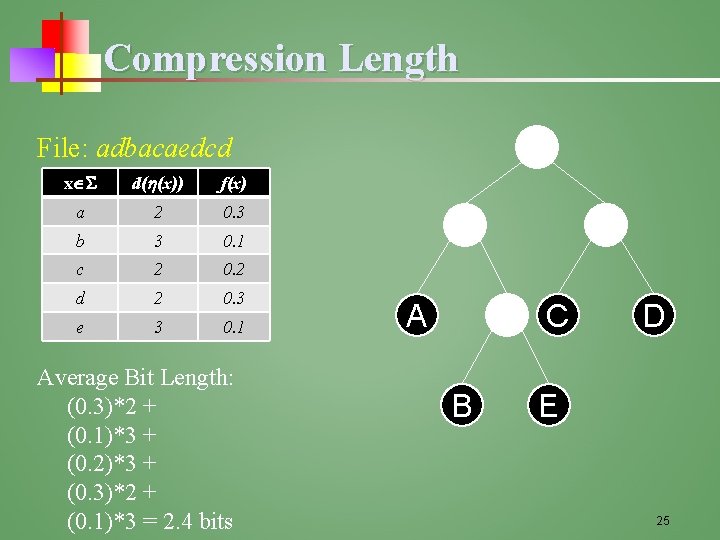
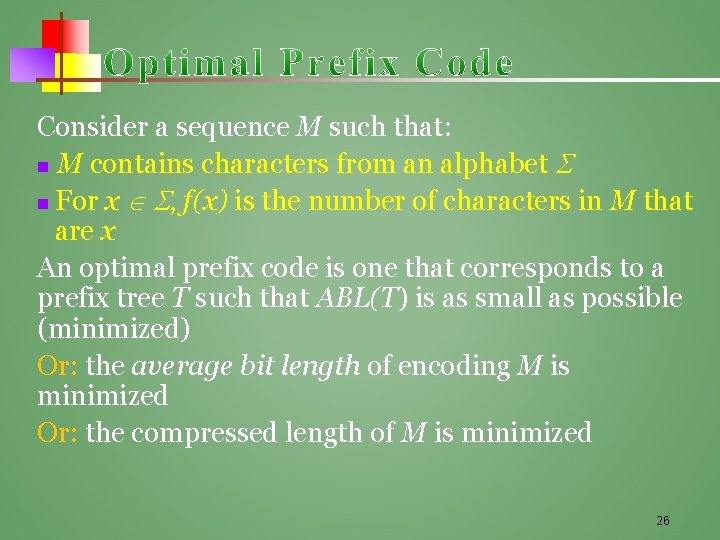
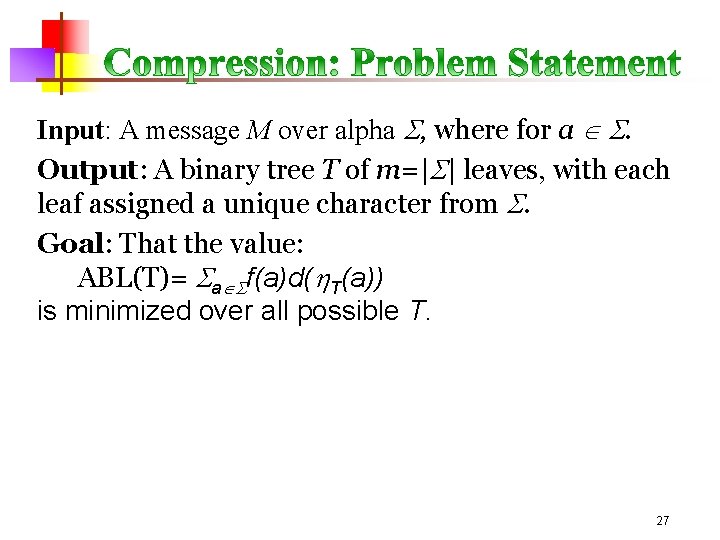
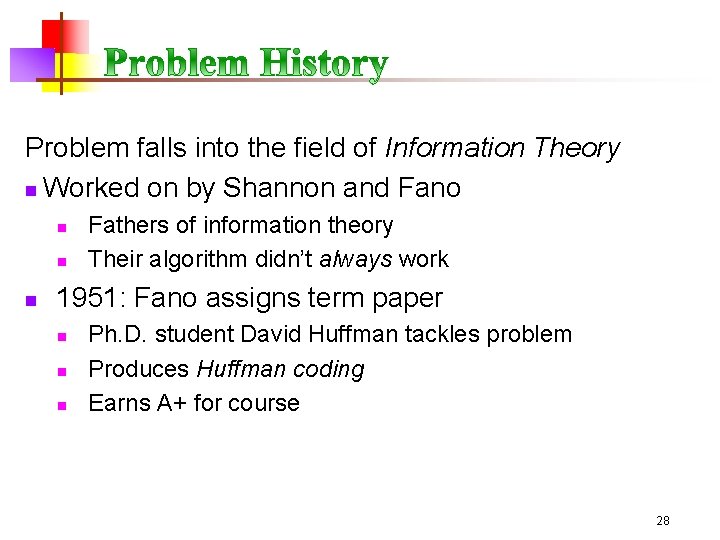
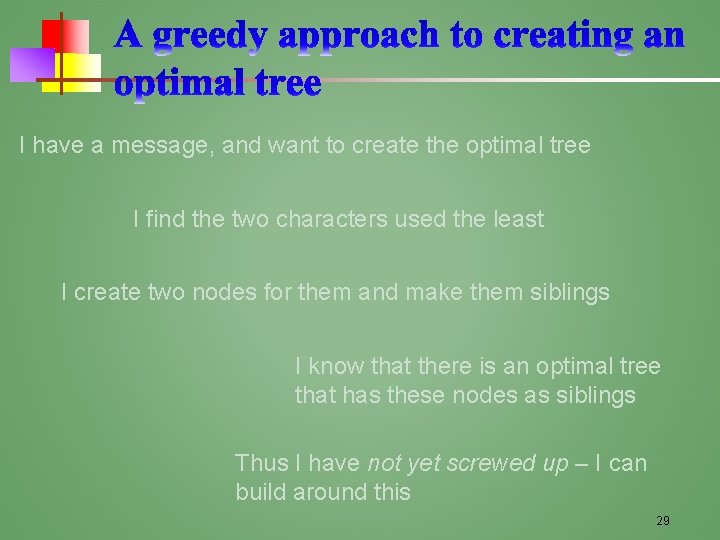
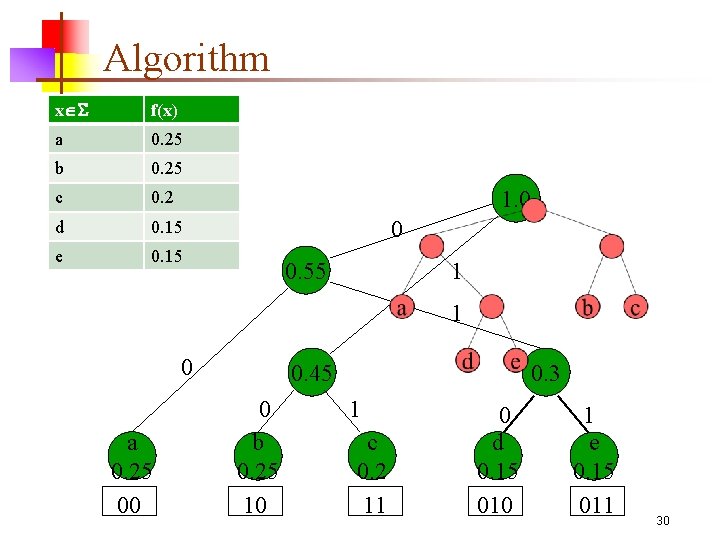
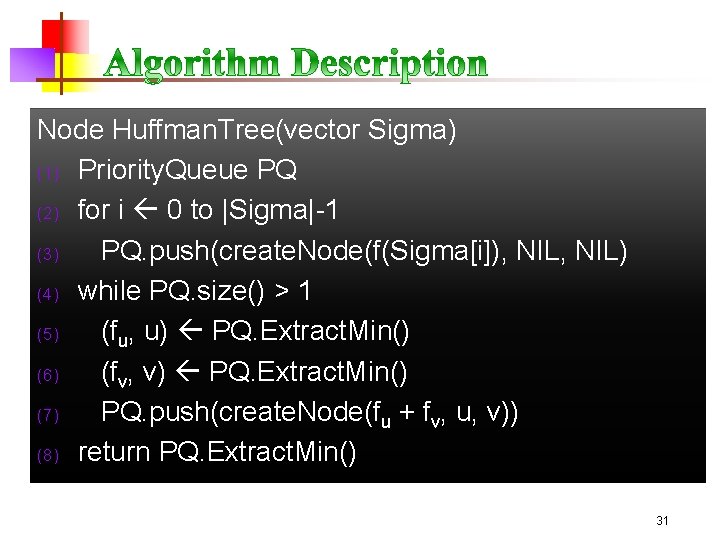
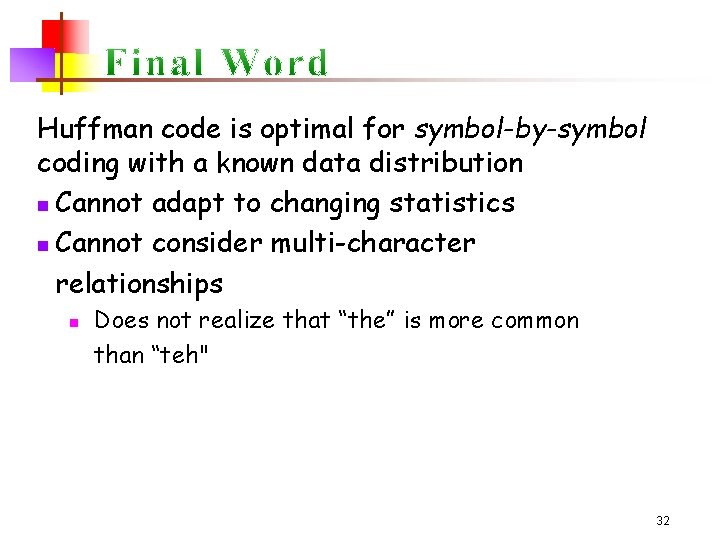
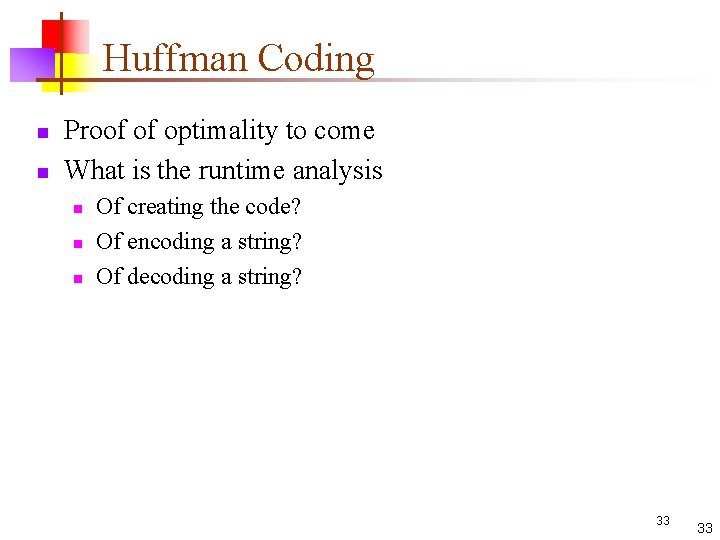
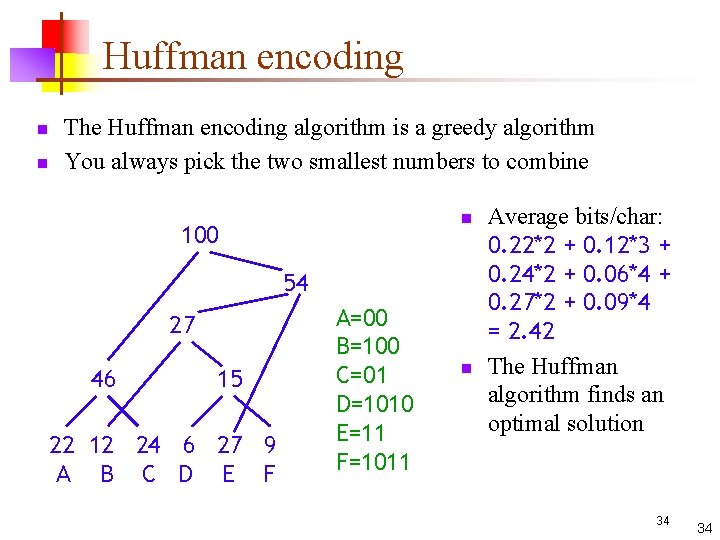
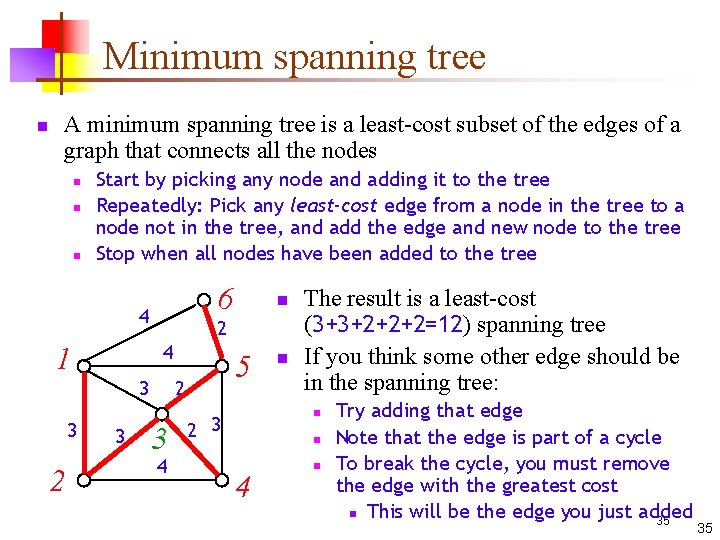
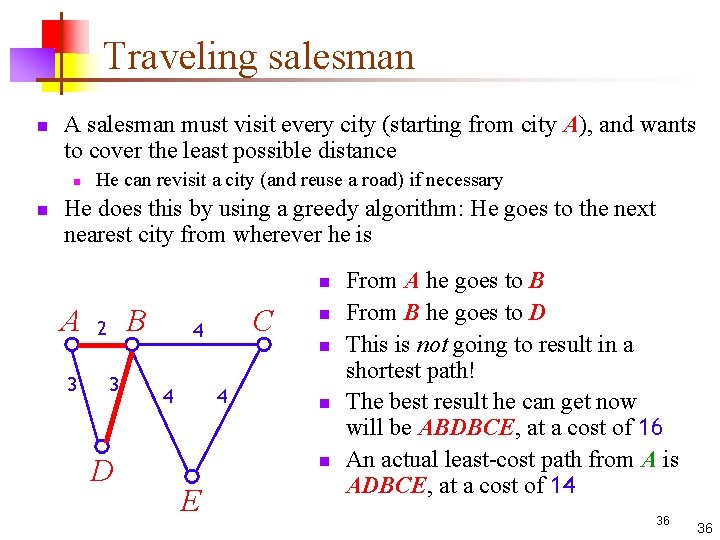
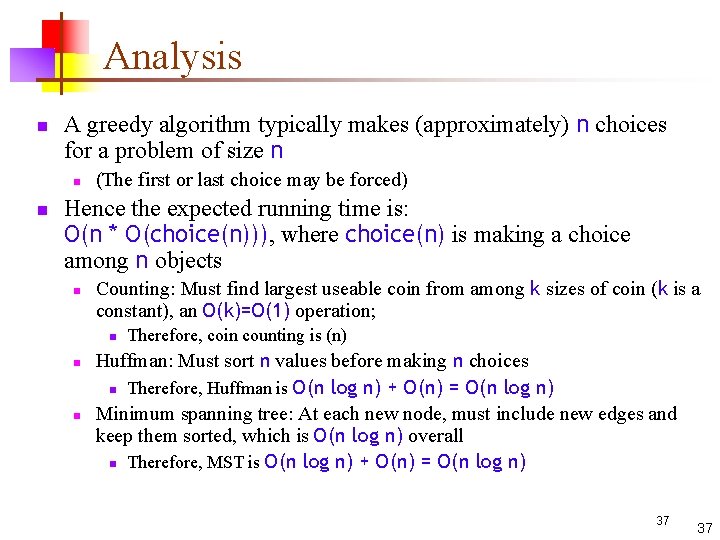
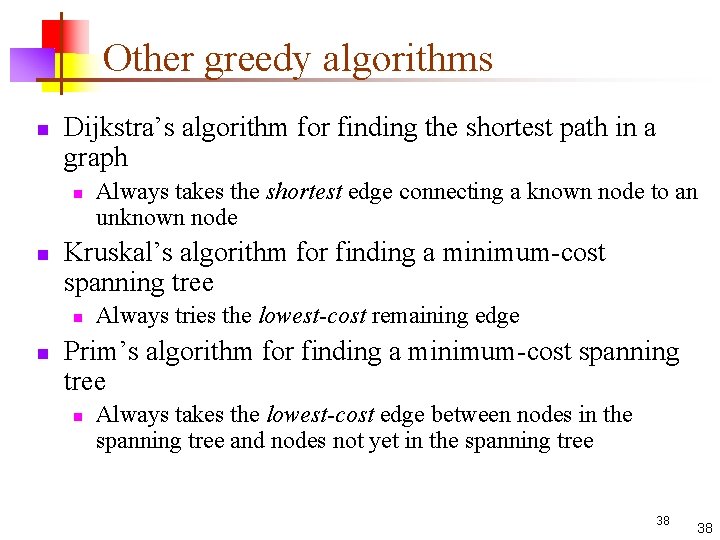
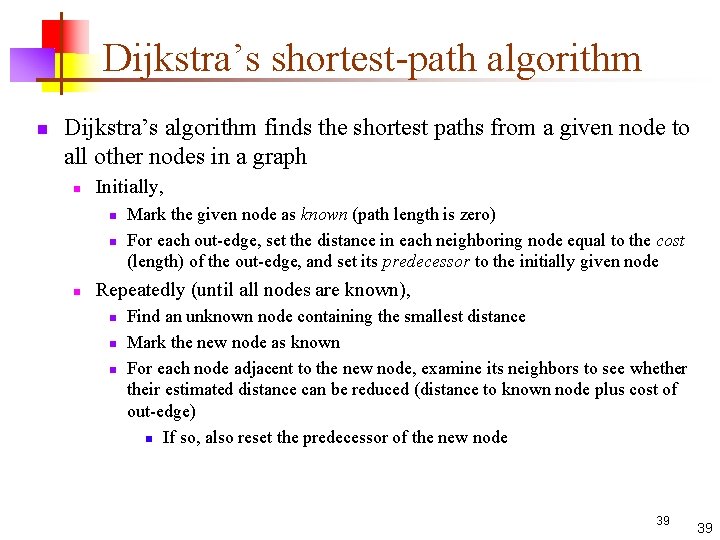
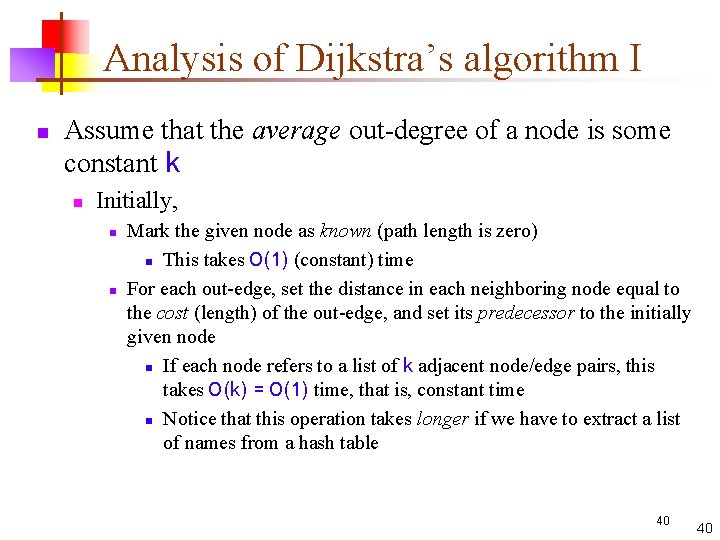
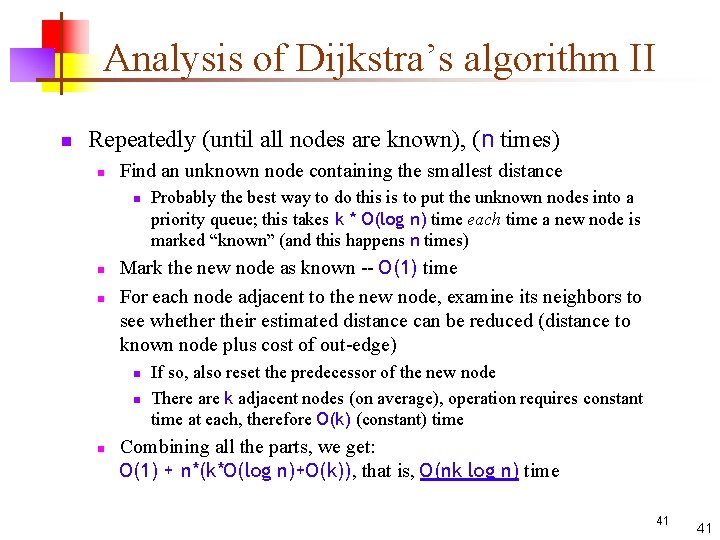
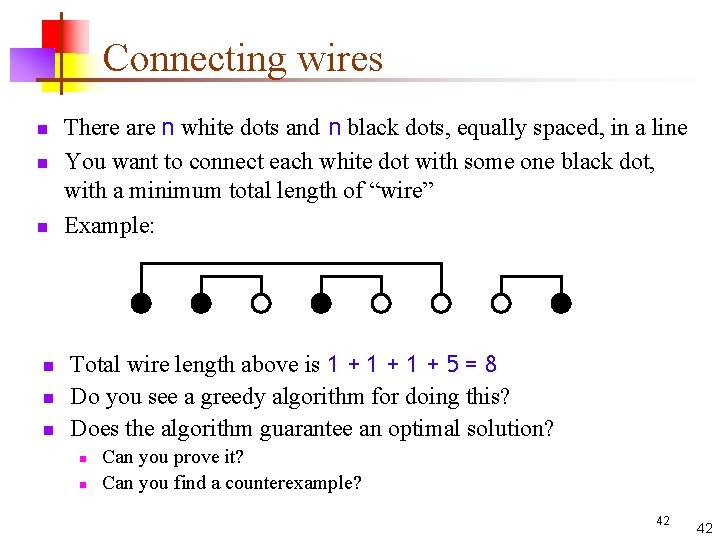
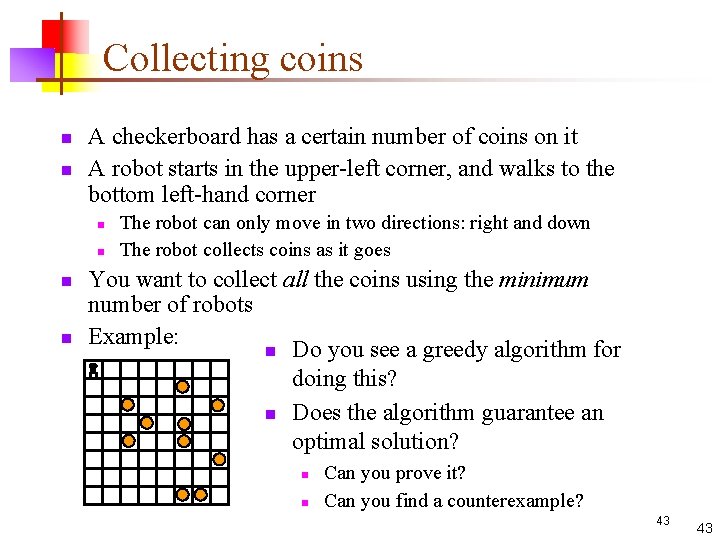
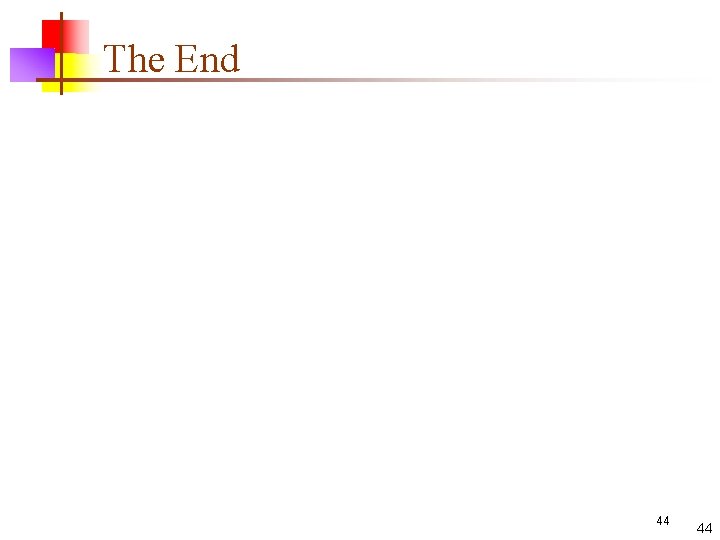
- Slides: 44
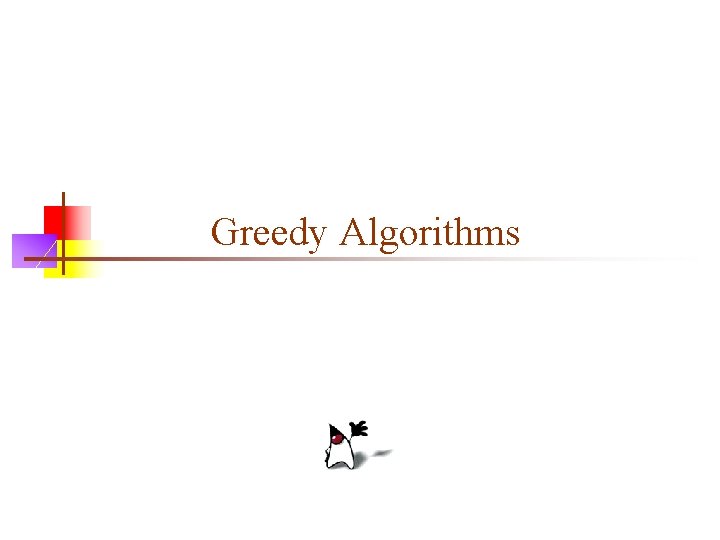
Greedy Algorithms
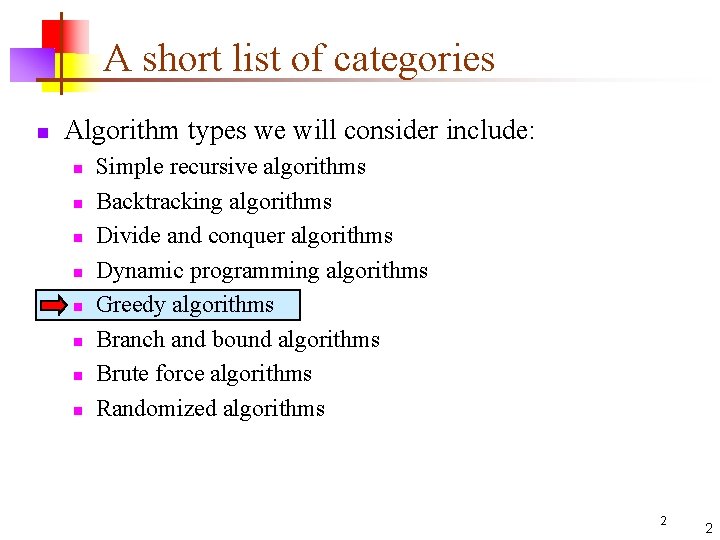
A short list of categories n Algorithm types we will consider include: n n n n Simple recursive algorithms Backtracking algorithms Divide and conquer algorithms Dynamic programming algorithms Greedy algorithms Branch and bound algorithms Brute force algorithms Randomized algorithms 2 2
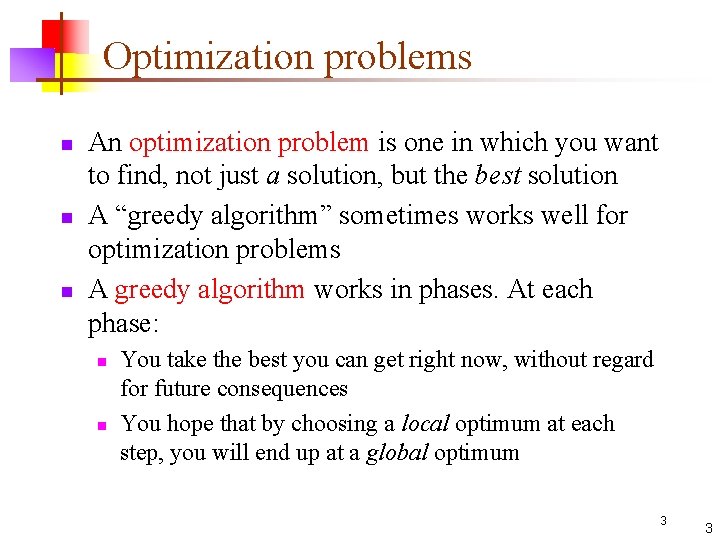
Optimization problems n n n An optimization problem is one in which you want to find, not just a solution, but the best solution A “greedy algorithm” sometimes works well for optimization problems A greedy algorithm works in phases. At each phase: n n You take the best you can get right now, without regard for future consequences You hope that by choosing a local optimum at each step, you will end up at a global optimum 3 3
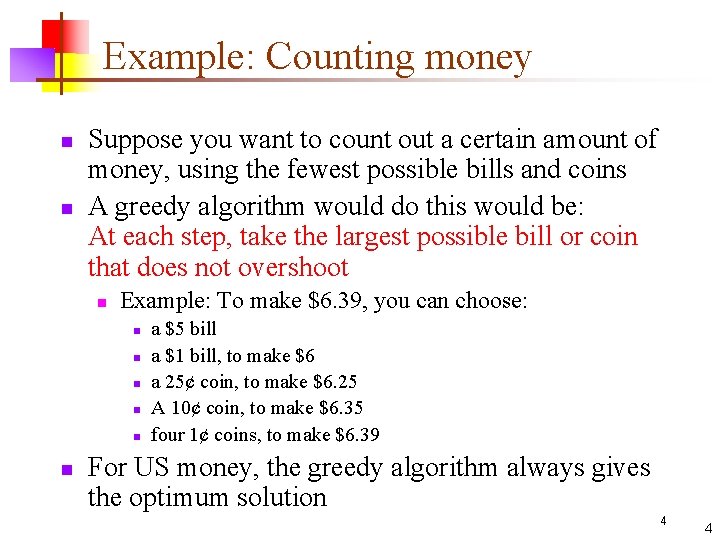
Example: Counting money n n Suppose you want to count out a certain amount of money, using the fewest possible bills and coins A greedy algorithm would do this would be: At each step, take the largest possible bill or coin that does not overshoot n Example: To make $6. 39, you can choose: n n n a $5 bill a $1 bill, to make $6 a 25¢ coin, to make $6. 25 A 10¢ coin, to make $6. 35 four 1¢ coins, to make $6. 39 For US money, the greedy algorithm always gives the optimum solution 4 4
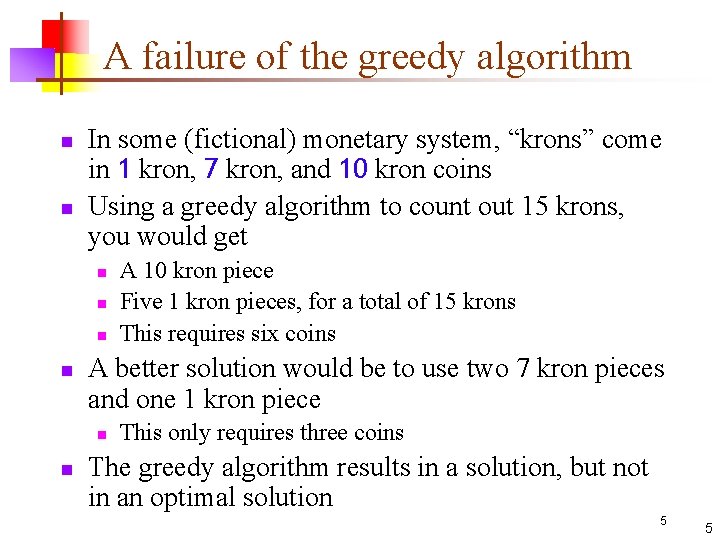
A failure of the greedy algorithm n n In some (fictional) monetary system, “krons” come in 1 kron, 7 kron, and 10 kron coins Using a greedy algorithm to count out 15 krons, you would get n n A better solution would be to use two 7 kron pieces and one 1 kron piece n n A 10 kron piece Five 1 kron pieces, for a total of 15 krons This requires six coins This only requires three coins The greedy algorithm results in a solution, but not in an optimal solution 5 5
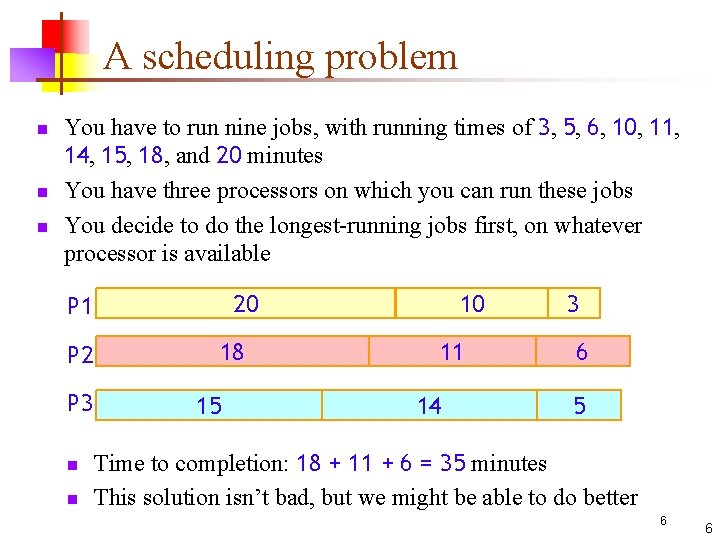
A scheduling problem n n n You have to run nine jobs, with running times of 3, 5, 6, 10, 11, 14, 15, 18, and 20 minutes You have three processors on which you can run these jobs You decide to do the longest-running jobs first, on whatever processor is available 20 P 1 P 2 P 3 n n 18 15 10 11 14 3 6 5 Time to completion: 18 + 11 + 6 = 35 minutes This solution isn’t bad, but we might be able to do better 6 6
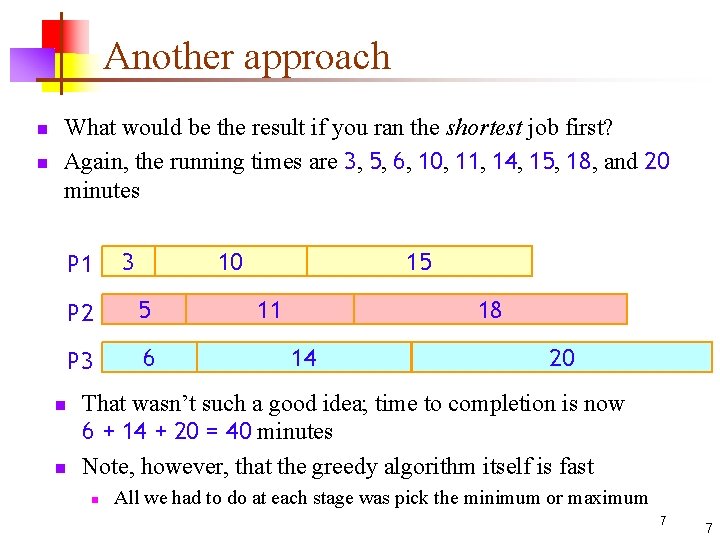
Another approach n n What would be the result if you ran the shortest job first? Again, the running times are 3, 5, 6, 10, 11, 14, 15, 18, and 20 minutes P 1 n n 3 10 P 2 5 P 3 6 15 11 18 14 20 That wasn’t such a good idea; time to completion is now 6 + 14 + 20 = 40 minutes Note, however, that the greedy algorithm itself is fast n All we had to do at each stage was pick the minimum or maximum 7 7
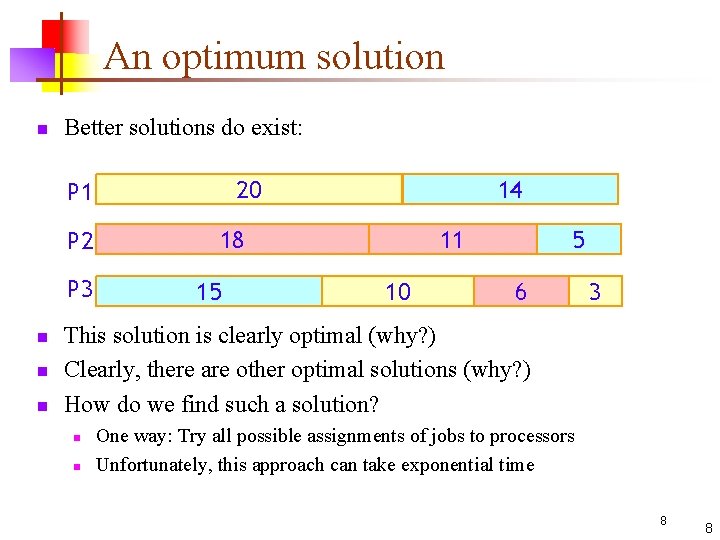
An optimum solution n Better solutions do exist: 20 P 1 P 2 P 3 n n n 14 18 15 11 10 5 6 3 This solution is clearly optimal (why? ) Clearly, there are other optimal solutions (why? ) How do we find such a solution? n n One way: Try all possible assignments of jobs to processors Unfortunately, this approach can take exponential time 8 8
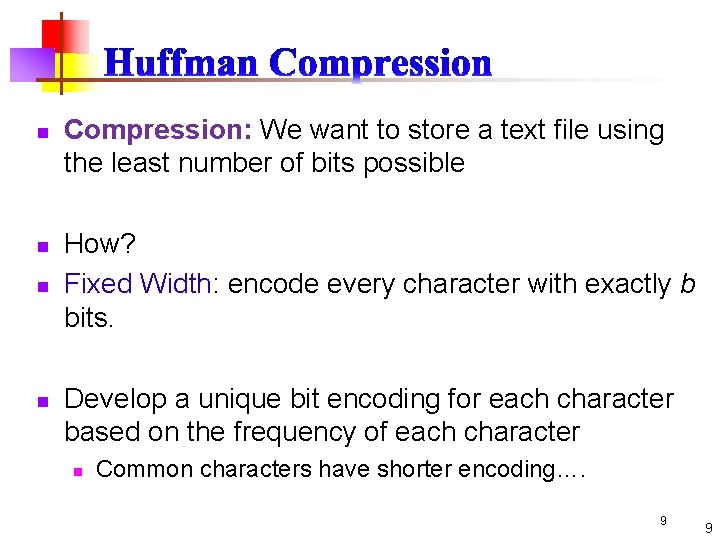
n n Compression: We want to store a text file using the least number of bits possible How? Fixed Width: encode every character with exactly b bits. Develop a unique bit encoding for each character based on the frequency of each character n Common characters have shorter encoding…. 9 9
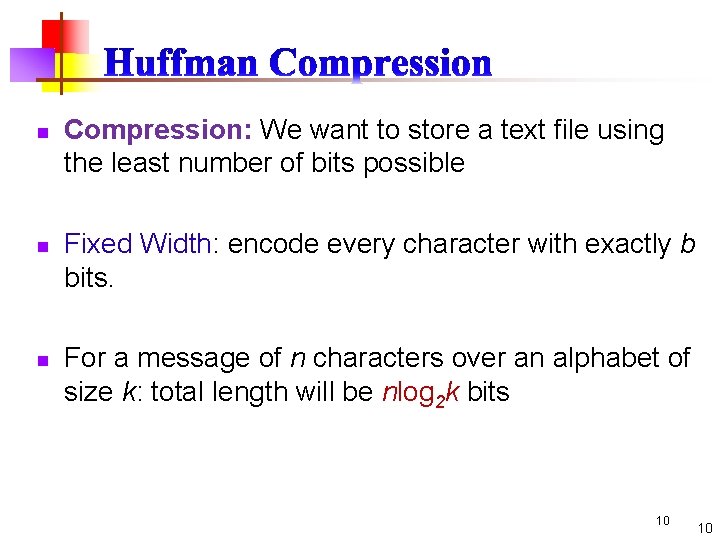
n n n Compression: We want to store a text file using the least number of bits possible Fixed Width: encode every character with exactly b bits. For a message of n characters over an alphabet of size k: total length will be nlog 2 k bits 10 10
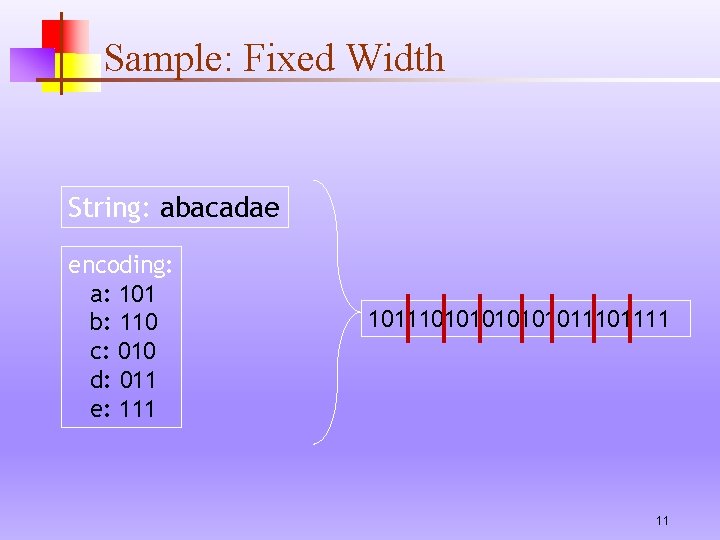
Sample: Fixed Width String: abacadae encoding: a: 101 b: 110 c: 010 d: 011 e: 111 101110101011101111 11
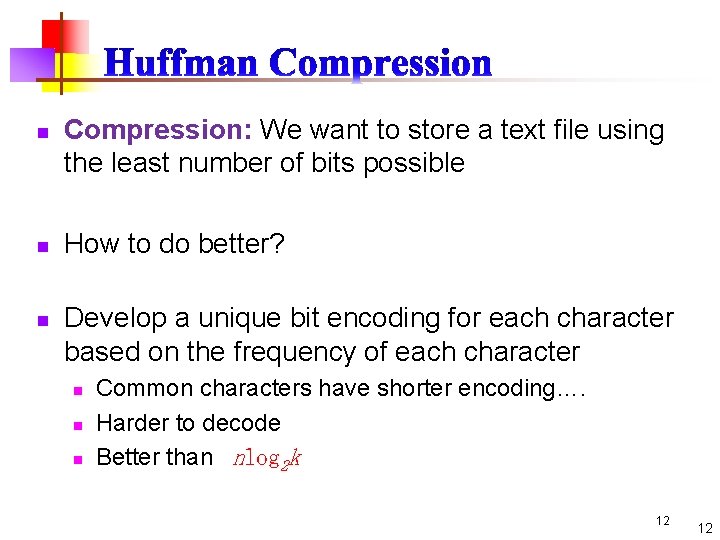
n n n Compression: We want to store a text file using the least number of bits possible How to do better? Develop a unique bit encoding for each character based on the frequency of each character n n n Common characters have shorter encoding…. Harder to decode Better than nlog 2 k 12 12
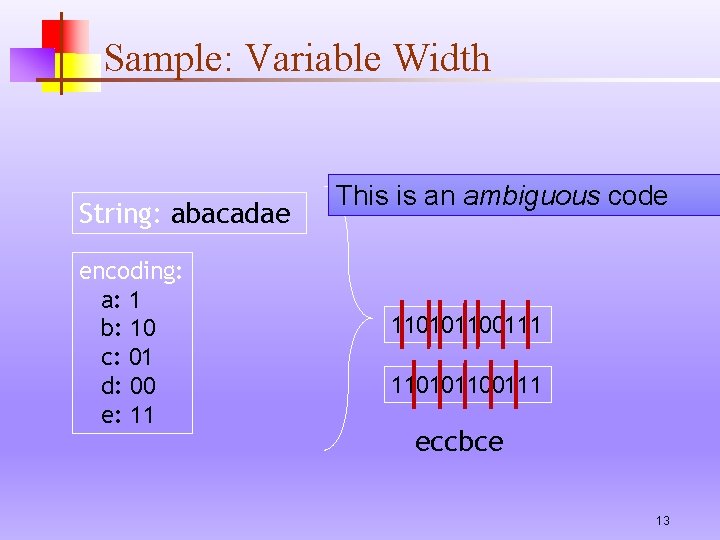
Sample: Variable Width String: abacadae encoding: a: 1 b: 10 c: 01 d: 00 e: 11 This is an ambiguous code 110101100111 eccbce 13
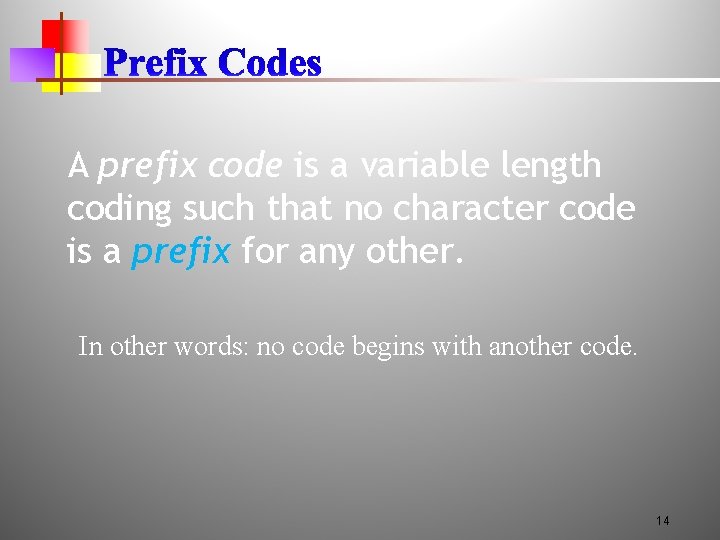
A prefix code is a variable length coding such that no character code is a prefix for any other. In other words: no code begins with another code. 14
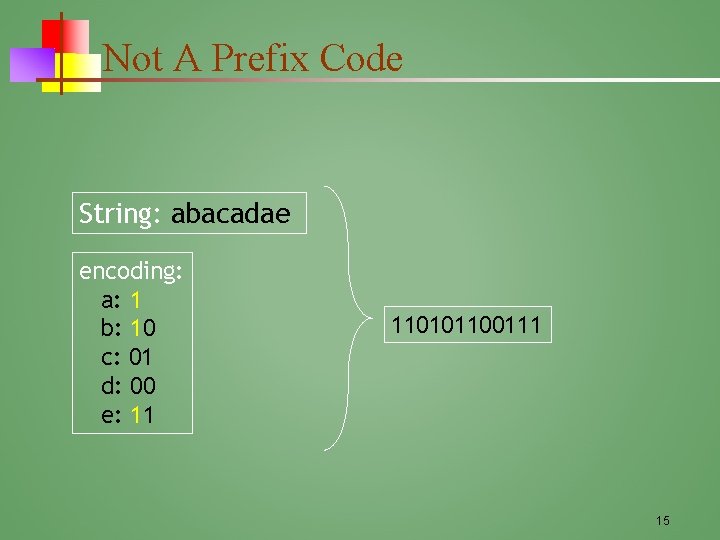
Not A Prefix Code String: abacadae encoding: a: 1 b: 10 c: 01 d: 00 e: 11 110101100111 15
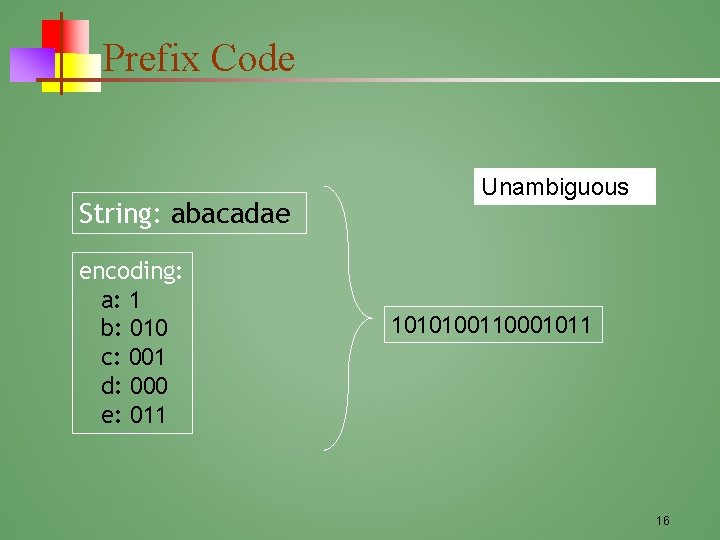
Prefix Code String: abacadae encoding: a: 1 b: 010 c: 001 d: 000 e: 011 Unambiguous 1010100110001011 16
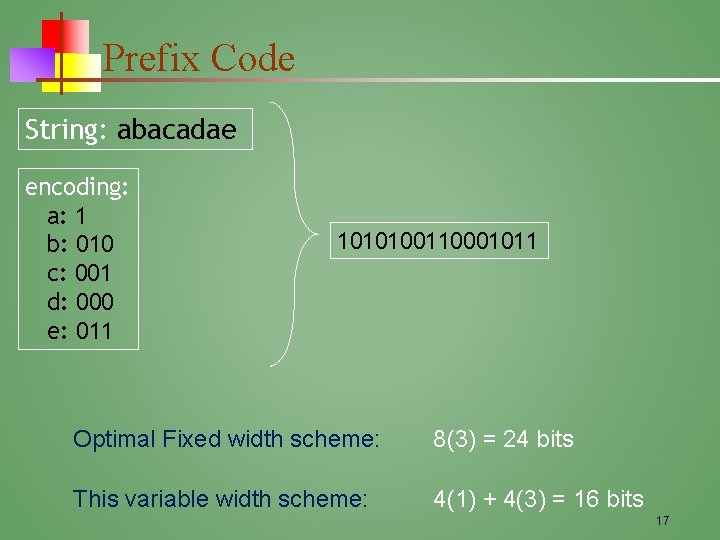
Prefix Code String: abacadae encoding: a: 1 b: 010 c: 001 d: 000 e: 011 1010100110001011 Optimal Fixed width scheme: 8(3) = 24 bits This variable width scheme: 4(1) + 4(3) = 16 bits 17
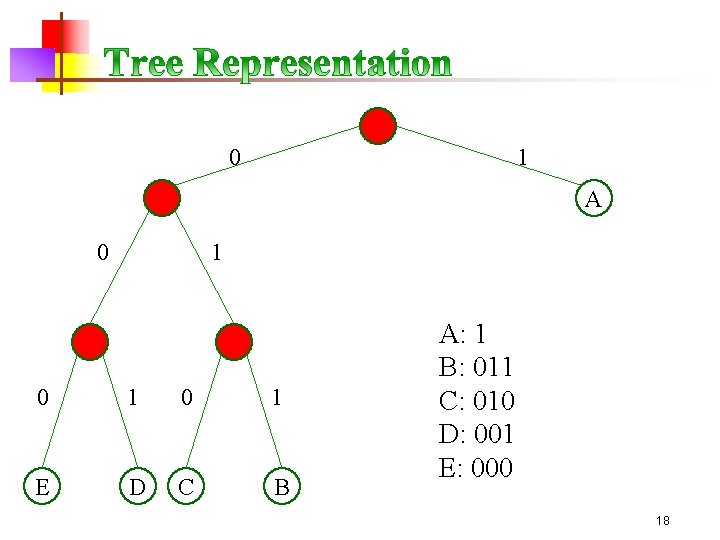
0 1 A 0 1 0 1 E D C B A: 1 B: 011 C: 010 D: 001 E: 000 18
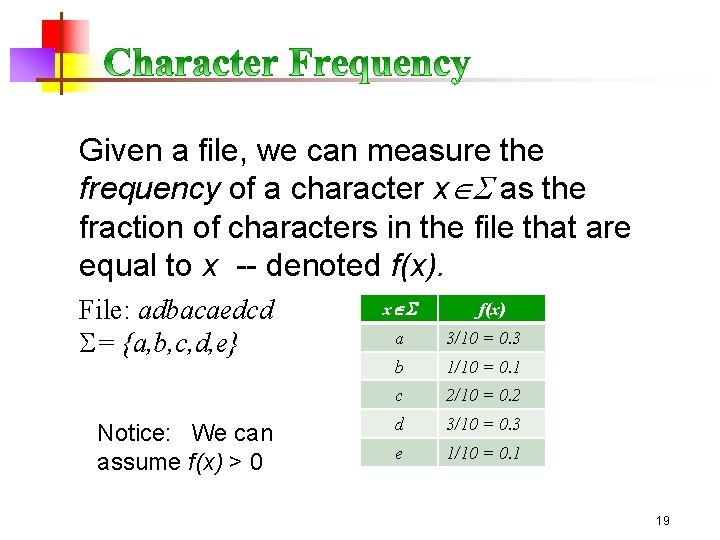
Given a file, we can measure the frequency of a character x as the fraction of characters in the file that are equal to x -- denoted f(x). File: adbacaedcd S= {a, b, c, d, e} Notice: We can assume f(x) > 0 x f(x) a 3/10 = 0. 3 b 1/10 = 0. 1 c 2/10 = 0. 2 d 3/10 = 0. 3 e 1/10 = 0. 1 19
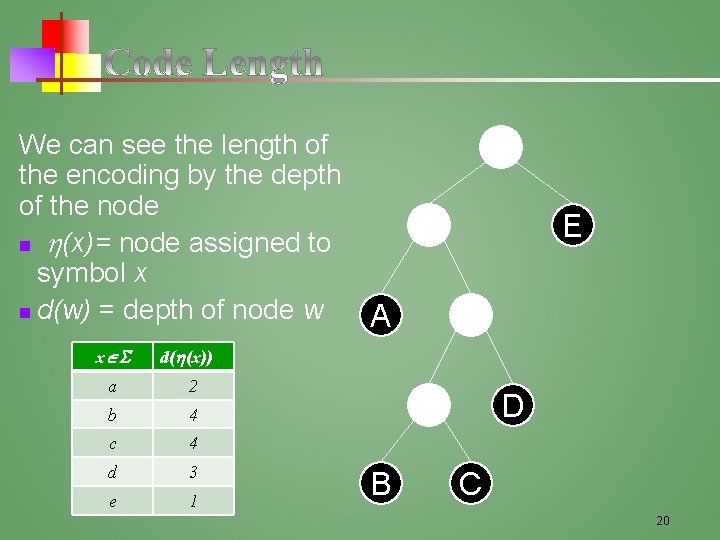
We can see the length of the encoding by the depth of the node n (x)= node assigned to symbol x n d(w) = depth of node w x E A d( (x)) a 2 b 4 c 4 d 3 e 1 D B C 20
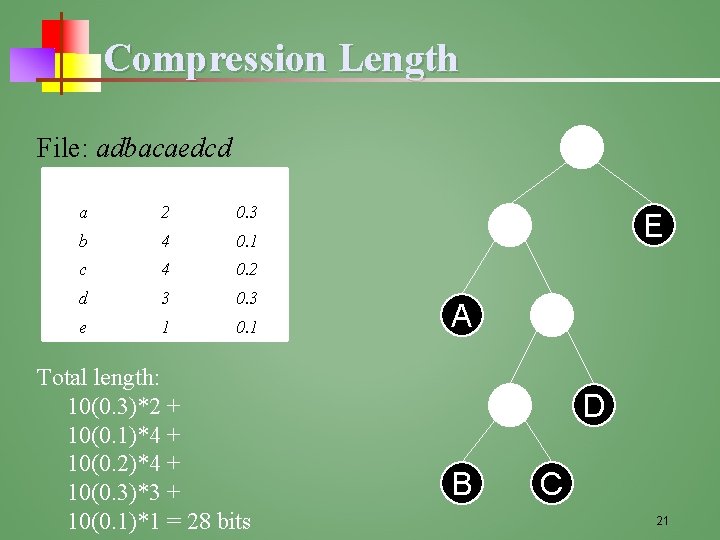
Compression Length File: adbacaedcd x d( (x)) f(x) a 2 0. 3 b 4 0. 1 c 4 0. 2 d 3 0. 3 e 1 0. 1 Total length: 10(0. 3)*2 + 10(0. 1)*4 + 10(0. 2)*4 + 10(0. 3)*3 + 10(0. 1)*1 = 28 bits E A D B C 21
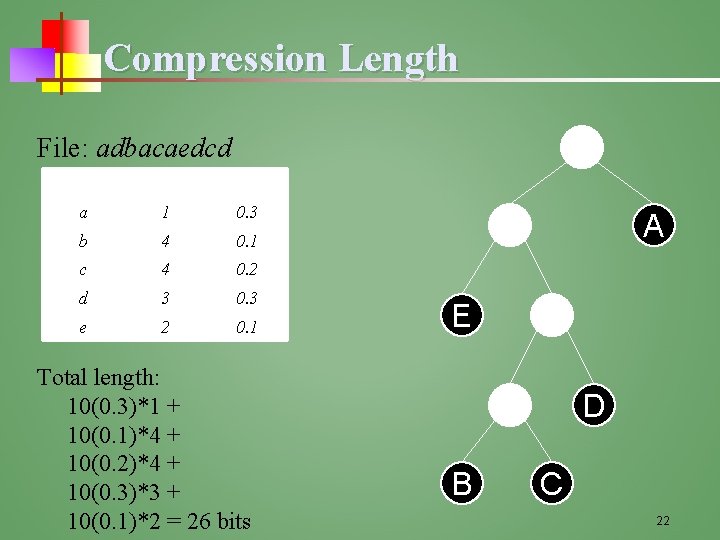
Compression Length File: adbacaedcd x d( (x)) f(x) a 1 0. 3 b 4 0. 1 c 4 0. 2 d 3 0. 3 e 2 0. 1 Total length: 10(0. 3)*1 + 10(0. 1)*4 + 10(0. 2)*4 + 10(0. 3)*3 + 10(0. 1)*2 = 26 bits A E D B C 22
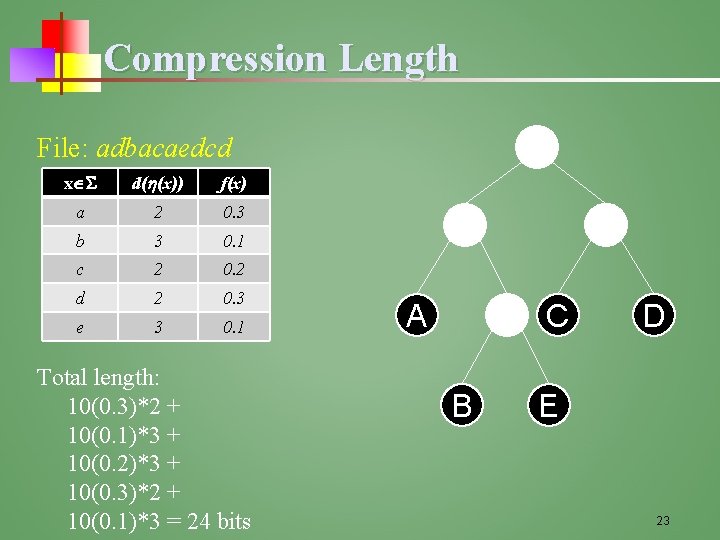
Compression Length File: adbacaedcd x d( (x)) f(x) a 2 0. 3 b 3 0. 1 c 2 0. 2 d 2 0. 3 e 3 0. 1 Total length: 10(0. 3)*2 + 10(0. 1)*3 + 10(0. 2)*3 + 10(0. 3)*2 + 10(0. 1)*3 = 24 bits C A B D E 23
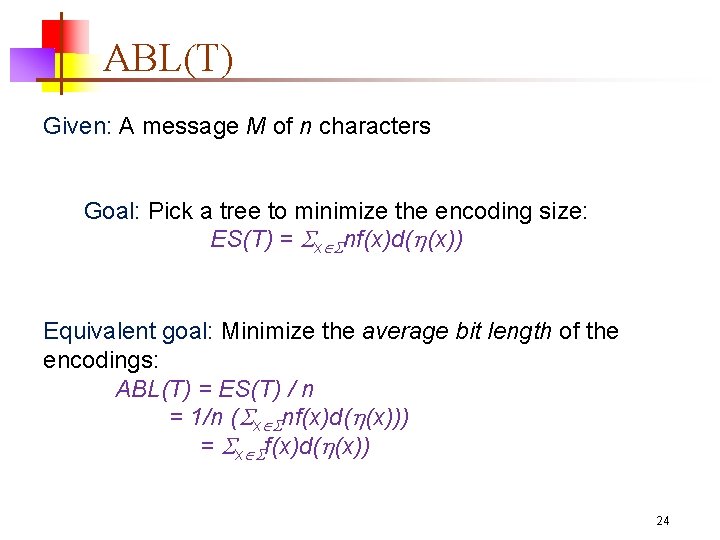
ABL(T) Given: A message M of n characters Goal: Pick a tree to minimize the encoding size: ES(T) = x nf(x)d( (x)) Equivalent goal: Minimize the average bit length of the encodings: ABL(T) = ES(T) / n = 1/n ( x nf(x)d( (x))) = x f(x)d( (x)) 24
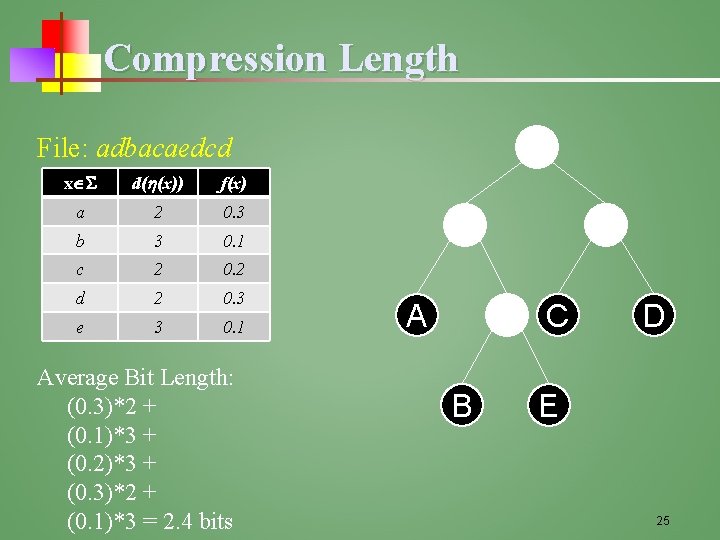
Compression Length File: adbacaedcd x d( (x)) f(x) a 2 0. 3 b 3 0. 1 c 2 0. 2 d 2 0. 3 e 3 0. 1 Average Bit Length: (0. 3)*2 + (0. 1)*3 + (0. 2)*3 + (0. 3)*2 + (0. 1)*3 = 2. 4 bits C A B D E 25
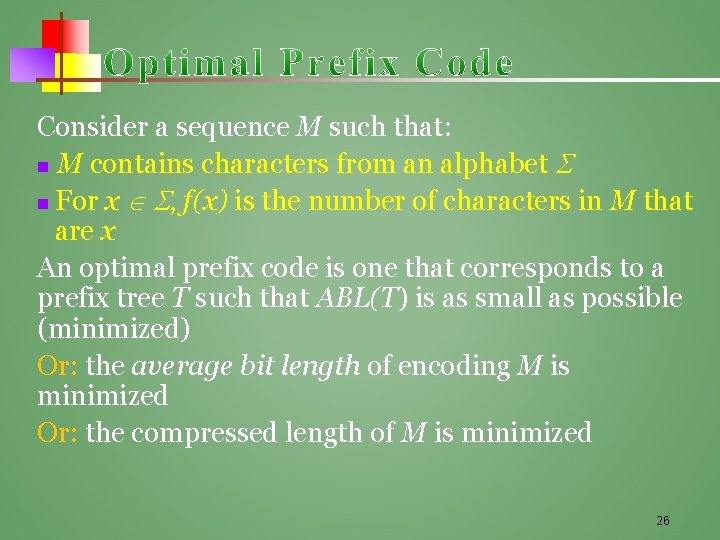
Consider a sequence M such that: n M contains characters from an alphabet n For x , f(x) is the number of characters in M that are x An optimal prefix code is one that corresponds to a prefix tree T such that ABL(T) is as small as possible (minimized) Or: the average bit length of encoding M is minimized Or: the compressed length of M is minimized 26
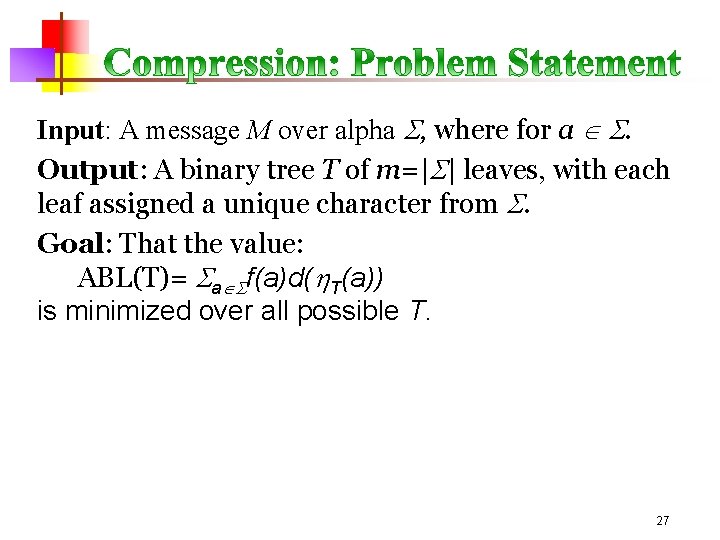
Input: A message M over alpha , where for a . Output: A binary tree T of m=| | leaves, with each leaf assigned a unique character from . Goal: That the value: ABL(T)= a f(a)d( T(a)) is minimized over all possible T. 27
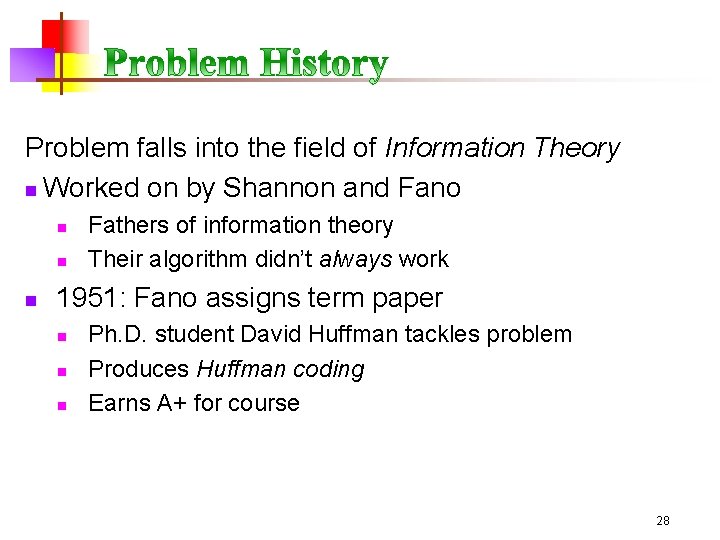
Problem falls into the field of Information Theory n Worked on by Shannon and Fano n n n Fathers of information theory Their algorithm didn’t always work 1951: Fano assigns term paper n n n Ph. D. student David Huffman tackles problem Produces Huffman coding Earns A+ for course 28
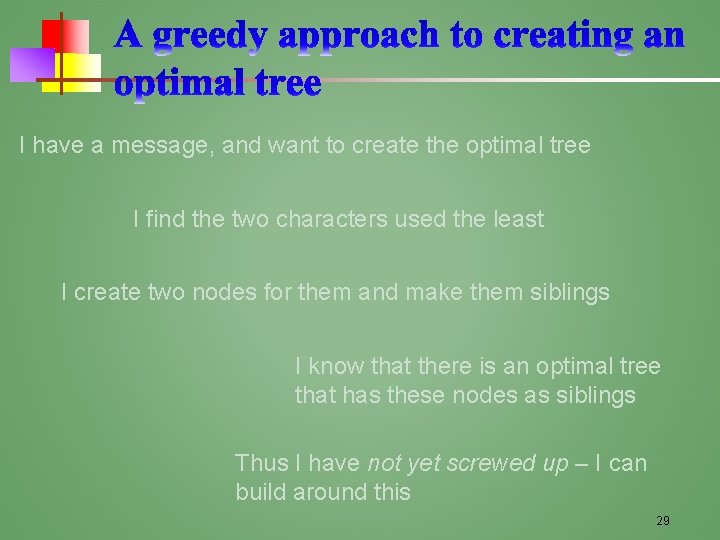
I have a message, and want to create the optimal tree I find the two characters used the least I create two nodes for them and make them siblings I know that there is an optimal tree that has these nodes as siblings Thus I have not yet screwed up – I can build around this 29
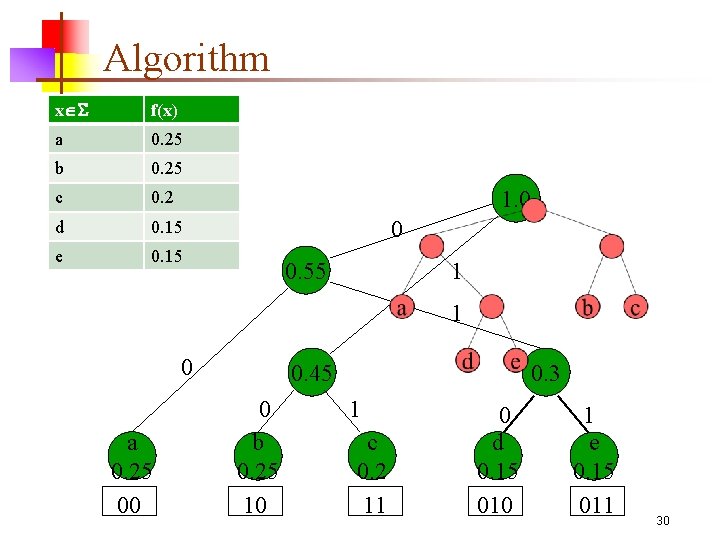
Algorithm x f(x) a 0. 25 b 0. 25 c 0. 2 d 0. 15 e 0. 15 1. 0 0 0. 55 1 1 0 a 0. 25 00 0. 45 0 b 0. 25 10 0. 3 1 c 0. 2 11 0 d 0. 15 010 1 e 0. 15 011 30
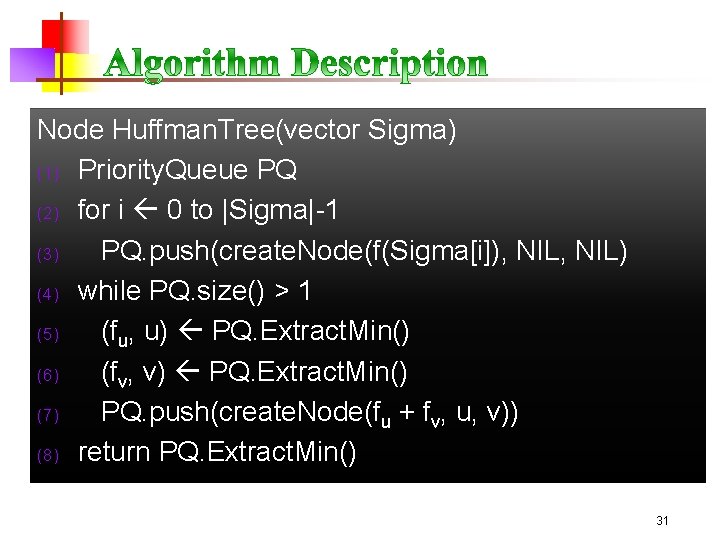
Node Huffman. Tree(vector Sigma) (1) Priority. Queue PQ (2) for i 0 to |Sigma|-1 (3) PQ. push(create. Node(f(Sigma[i]), NIL) (4) while PQ. size() > 1 (5) (fu, u) PQ. Extract. Min() (6) (fv, v) PQ. Extract. Min() (7) PQ. push(create. Node(fu + fv, u, v)) (8) return PQ. Extract. Min() 31
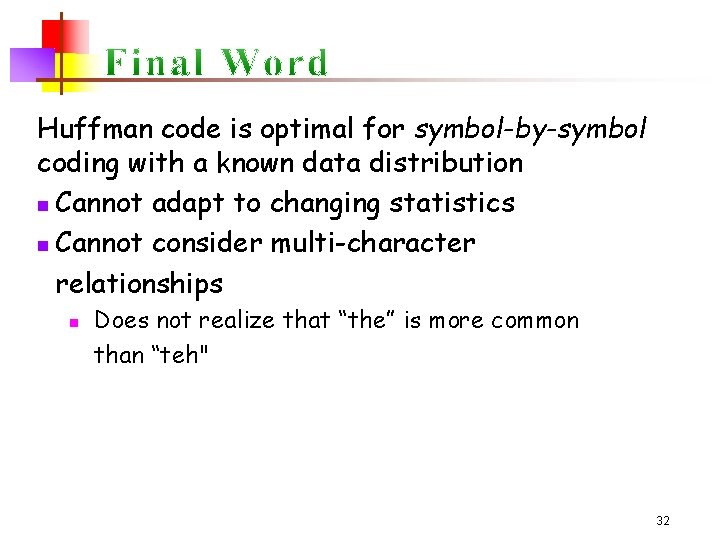
Huffman code is optimal for symbol-by-symbol coding with a known data distribution n Cannot adapt to changing statistics n Cannot consider multi-character relationships n Does not realize that “the” is more common than “teh" 32
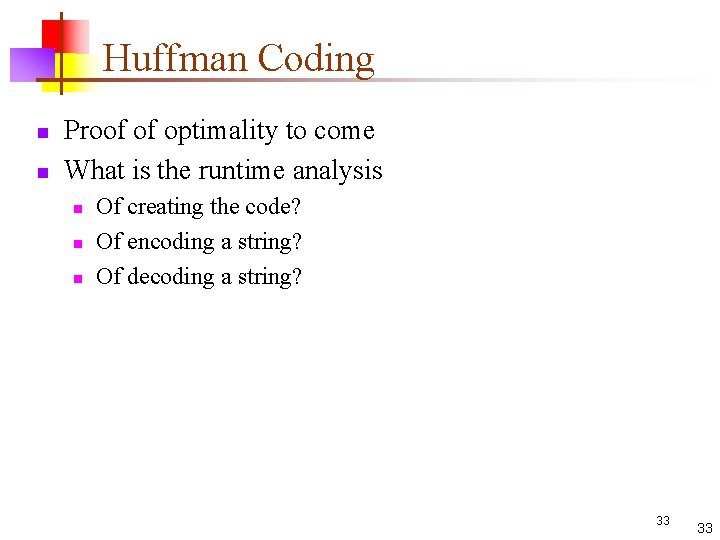
Huffman Coding n n Proof of optimality to come What is the runtime analysis n n n Of creating the code? Of encoding a string? Of decoding a string? 33 33
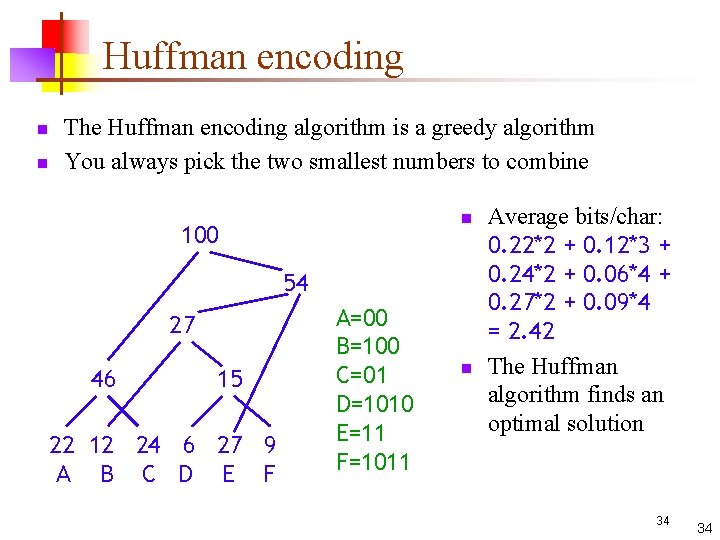
Huffman encoding n n The Huffman encoding algorithm is a greedy algorithm You always pick the two smallest numbers to combine n 100 54 27 46 15 22 12 24 6 27 9 A B C D E F A=00 B=100 C=01 D=1010 E=11 F=1011 n Average bits/char: 0. 22*2 + 0. 12*3 + 0. 24*2 + 0. 06*4 + 0. 27*2 + 0. 09*4 = 2. 42 The Huffman algorithm finds an optimal solution 34 34
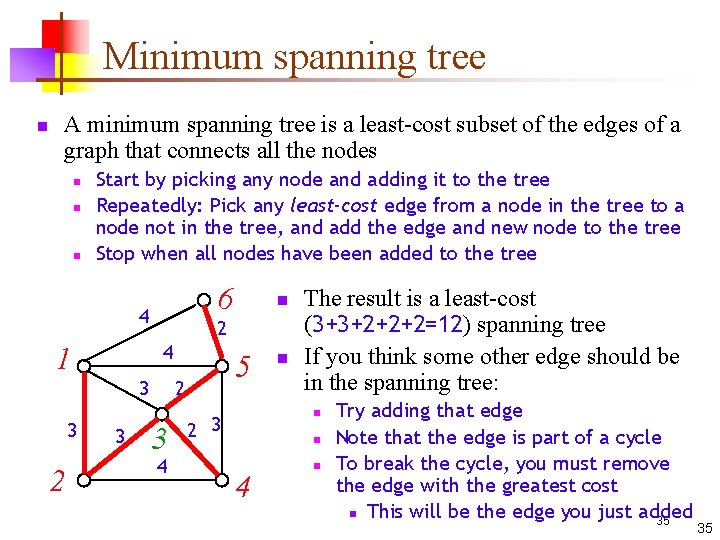
Minimum spanning tree n A minimum spanning tree is a least-cost subset of the edges of a graph that connects all the nodes n n n Start by picking any node and adding it to the tree Repeatedly: Pick any least-cost edge from a node in the tree to a node not in the tree, and add the edge and new node to the tree Stop when all nodes have been added to the tree 6 4 2 4 1 3 3 2 3 n 5 2 3 4 n The result is a least-cost (3+3+2+2+2=12) spanning tree If you think some other edge should be in the spanning tree: n 2 3 n 4 n Try adding that edge Note that the edge is part of a cycle To break the cycle, you must remove the edge with the greatest cost n This will be the edge you just added 35 35
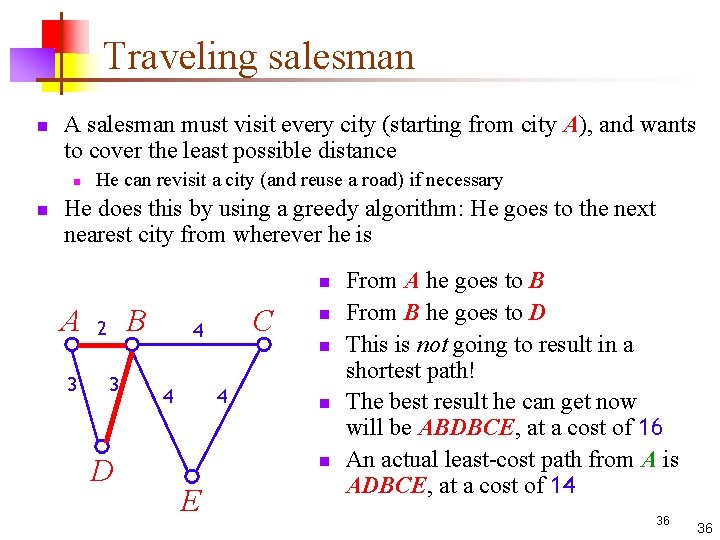
Traveling salesman n A salesman must visit every city (starting from city A), and wants to cover the least possible distance n n He can revisit a city (and reuse a road) if necessary He does this by using a greedy algorithm: He goes to the next nearest city from wherever he is n A 3 B 2 3 D C 4 4 n n E From A he goes to B From B he goes to D This is not going to result in a shortest path! The best result he can get now will be ABDBCE, at a cost of 16 An actual least-cost path from A is ADBCE, at a cost of 14 36 36
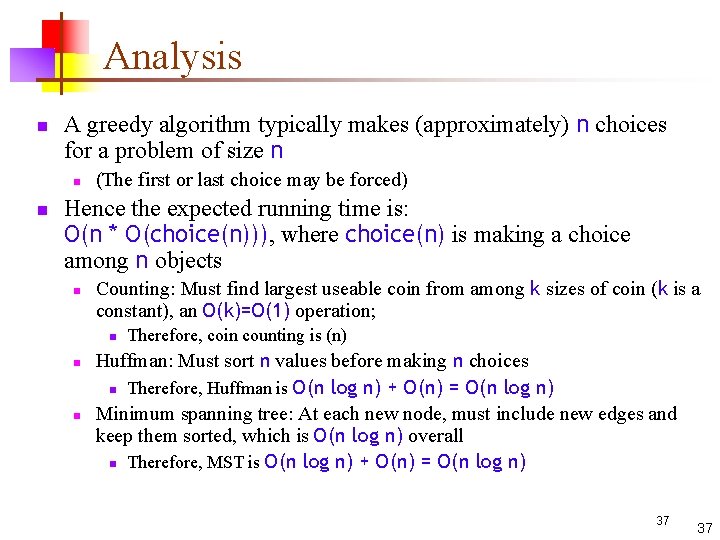
Analysis n A greedy algorithm typically makes (approximately) n choices for a problem of size n n n (The first or last choice may be forced) Hence the expected running time is: O(n * O(choice(n))), where choice(n) is making a choice among n objects n Counting: Must find largest useable coin from among k sizes of coin (k is a constant), an O(k)=O(1) operation; n n n Therefore, coin counting is (n) Huffman: Must sort n values before making n choices n Therefore, Huffman is O(n log n) + O(n) = O(n log n) Minimum spanning tree: At each new node, must include new edges and keep them sorted, which is O(n log n) overall n Therefore, MST is O(n log n) + O(n) = O(n log n) 37 37
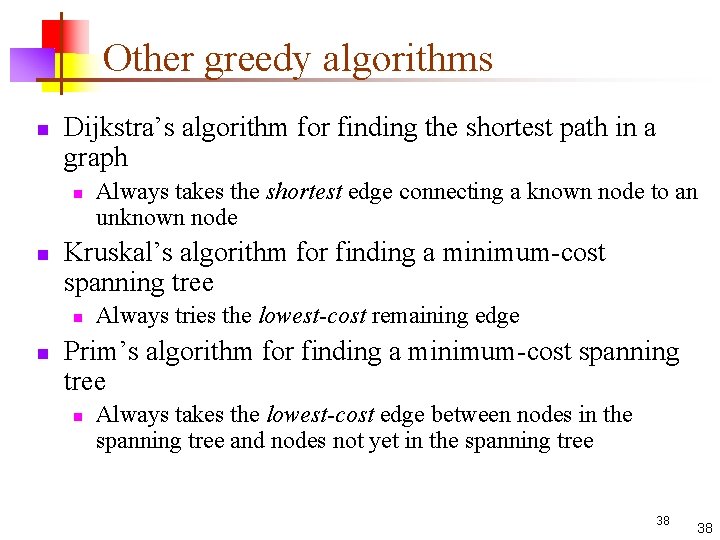
Other greedy algorithms n Dijkstra’s algorithm for finding the shortest path in a graph n n Kruskal’s algorithm for finding a minimum-cost spanning tree n n Always takes the shortest edge connecting a known node to an unknown node Always tries the lowest-cost remaining edge Prim’s algorithm for finding a minimum-cost spanning tree n Always takes the lowest-cost edge between nodes in the spanning tree and nodes not yet in the spanning tree 38 38
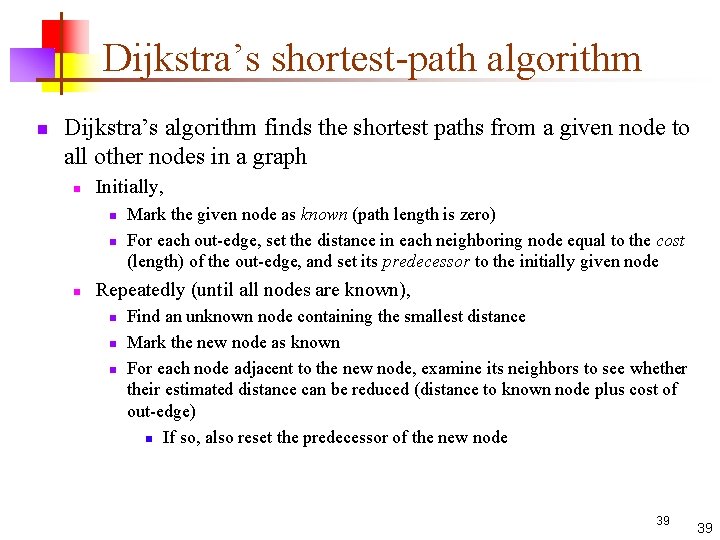
Dijkstra’s shortest-path algorithm n Dijkstra’s algorithm finds the shortest paths from a given node to all other nodes in a graph n Initially, n n n Mark the given node as known (path length is zero) For each out-edge, set the distance in each neighboring node equal to the cost (length) of the out-edge, and set its predecessor to the initially given node Repeatedly (until all nodes are known), n n n Find an unknown node containing the smallest distance Mark the new node as known For each node adjacent to the new node, examine its neighbors to see whether their estimated distance can be reduced (distance to known node plus cost of out-edge) n If so, also reset the predecessor of the new node 39 39
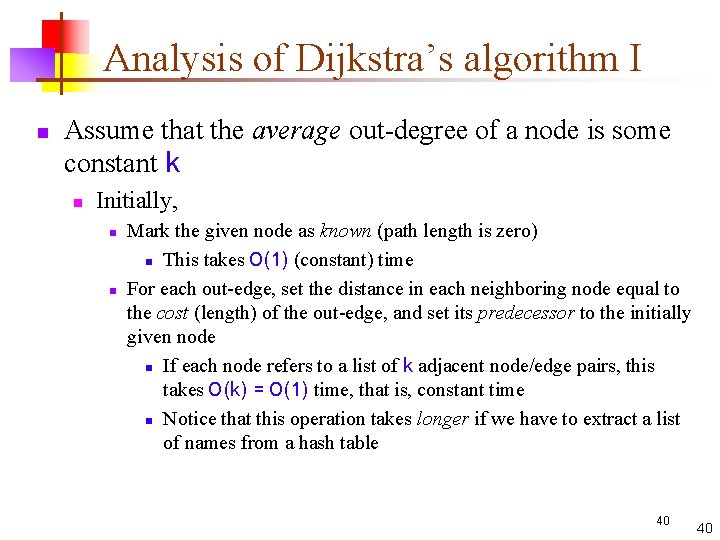
Analysis of Dijkstra’s algorithm I n Assume that the average out-degree of a node is some constant k n Initially, n n Mark the given node as known (path length is zero) n This takes O(1) (constant) time For each out-edge, set the distance in each neighboring node equal to the cost (length) of the out-edge, and set its predecessor to the initially given node n If each node refers to a list of k adjacent node/edge pairs, this takes O(k) = O(1) time, that is, constant time n Notice that this operation takes longer if we have to extract a list of names from a hash table 40 40
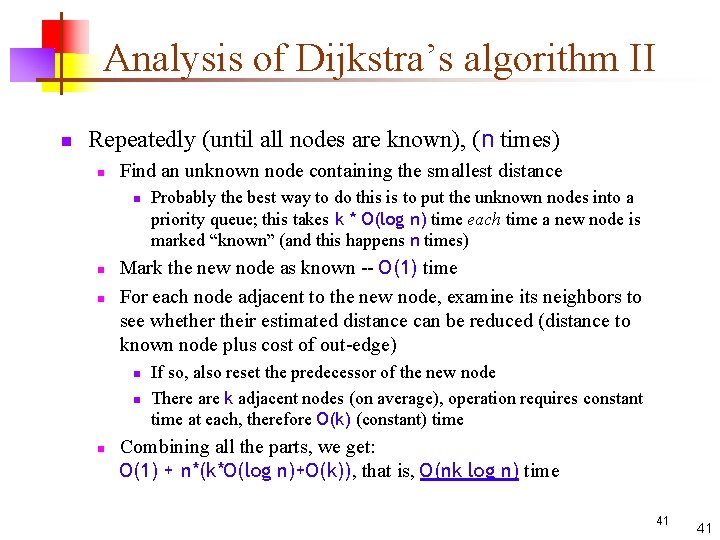
Analysis of Dijkstra’s algorithm II n Repeatedly (until all nodes are known), (n times) n Find an unknown node containing the smallest distance n n n Mark the new node as known -- O(1) time For each node adjacent to the new node, examine its neighbors to see whether their estimated distance can be reduced (distance to known node plus cost of out-edge) n n n Probably the best way to do this is to put the unknown nodes into a priority queue; this takes k * O(log n) time each time a new node is marked “known” (and this happens n times) If so, also reset the predecessor of the new node There are k adjacent nodes (on average), operation requires constant time at each, therefore O(k) (constant) time Combining all the parts, we get: O(1) + n*(k*O(log n)+O(k)), that is, O(nk log n) time 41 41
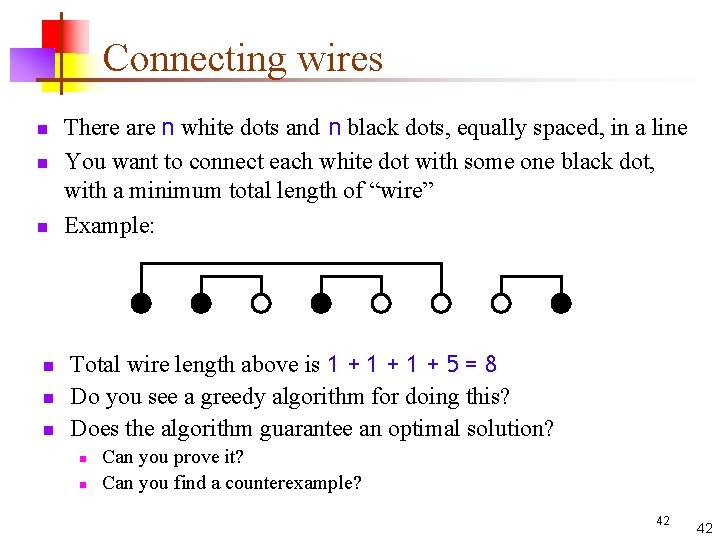
Connecting wires n n n There are n white dots and n black dots, equally spaced, in a line You want to connect each white dot with some one black dot, with a minimum total length of “wire” Example: Total wire length above is 1 + 1 + 5 = 8 Do you see a greedy algorithm for doing this? Does the algorithm guarantee an optimal solution? n n Can you prove it? Can you find a counterexample? 42 42
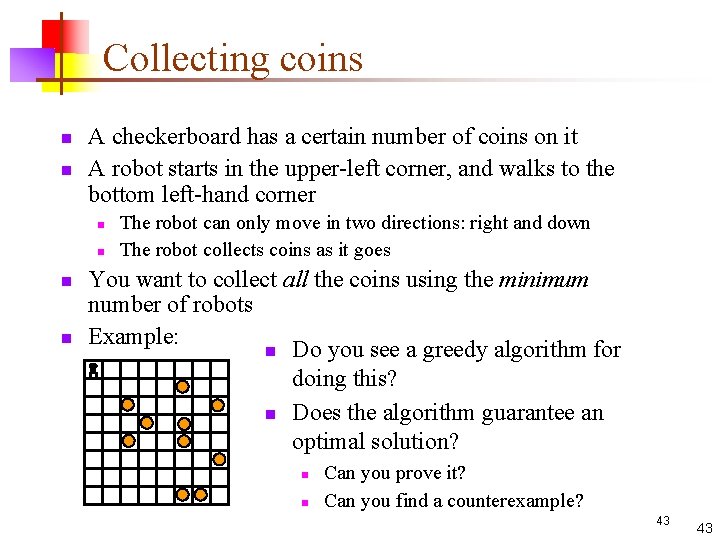
Collecting coins n n A checkerboard has a certain number of coins on it A robot starts in the upper-left corner, and walks to the bottom left-hand corner n n The robot can only move in two directions: right and down The robot collects coins as it goes You want to collect all the coins using the minimum number of robots Example: n Do you see a greedy algorithm for doing this? n Does the algorithm guarantee an optimal solution? n n Can you prove it? Can you find a counterexample? 43 43
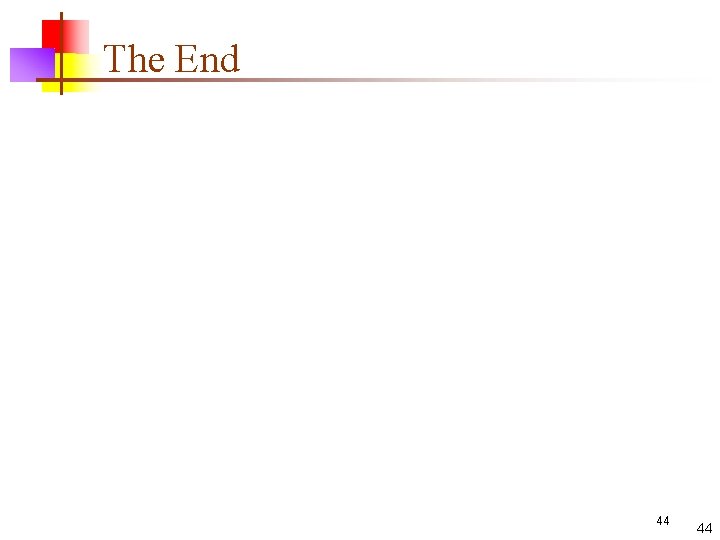
The End 44 44
Tall+short h
N/a greedy
Greedy algorithm
Huffman coding - greedy algorithm
Fractional knapsack problem
Common recursive algorithms
Syntactic category list
Typical brake system
Qbasic loops
Axiom meaning in maths
Singly vs doubly linked list
Difference between an array and a linked list
List h shows account
Perbedaan single linked list dan double linked list
Select list item list index too large
Story short
Heterozygous short-hair x heterozygous short-hair
The greedy snake
Where is greedy marker
Greedy best first search
Problema rucsacului
Contoh soal metode greedy
Metode greedy
Kassim and the greedy dragon
Workplace problems
Greedy algorithm
Greedy algoritmus
Greedy algorithm properties
Activity selection problem greedy algorithm example
Exchange argument greedy
Greedy activity selector
Greedy interval partitioning
Greedy function
Greedy best first search example
Algoritmul greedy
Thomas putnam greedy quotes
Character traits for adventurous
Simple cycle and greedy cycle
Activity selection problem greedy algorithm
Greedy algorithm for job sequencing with deadlines
Contoh knapsack problem
Greedy heuristic
Greedy algorithm vs dynamic programming
Gpsr routing protocol
Top down greedy approach