Gene Ontology Overview and Perspective Lung Development Ontology
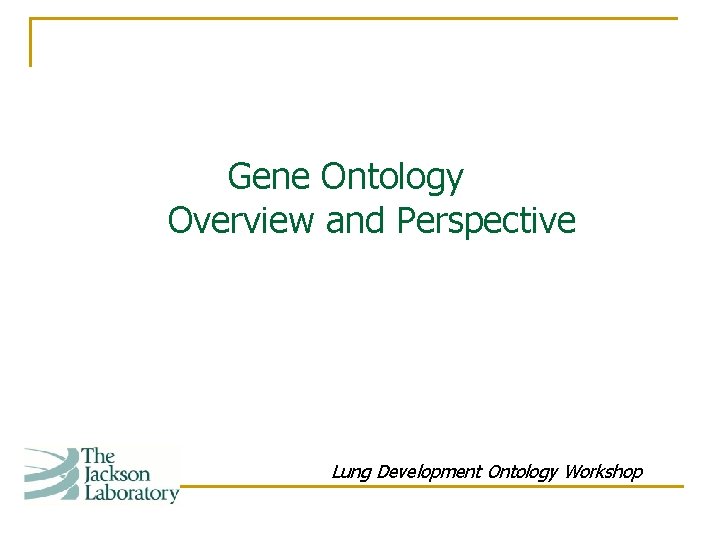
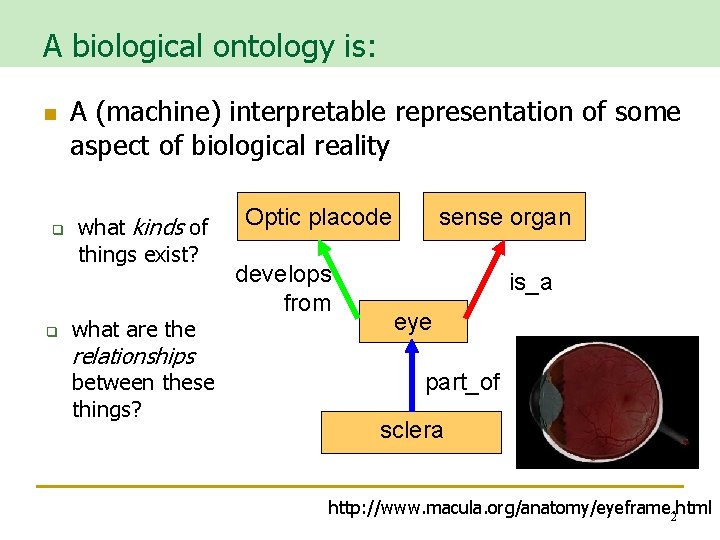
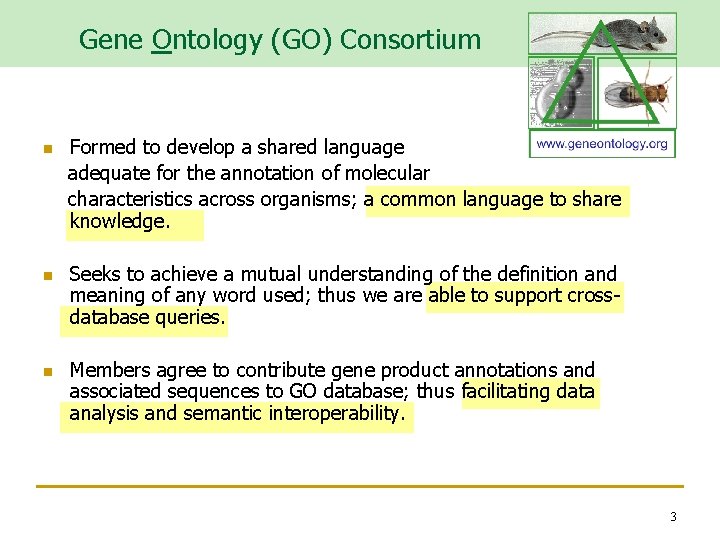
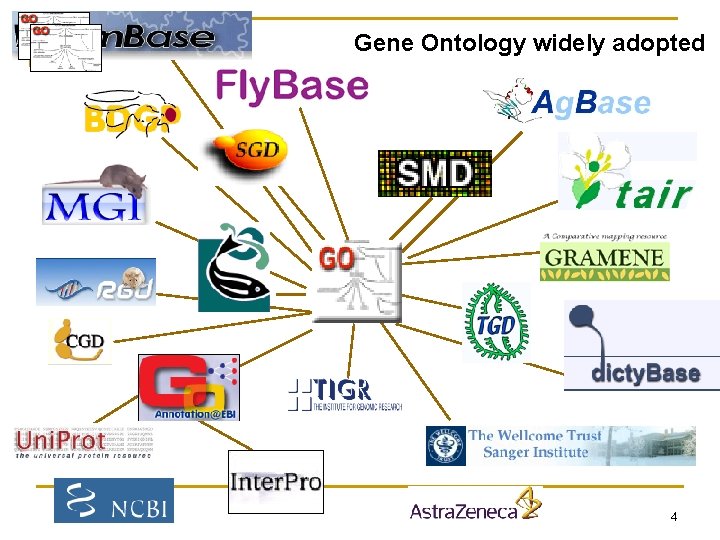
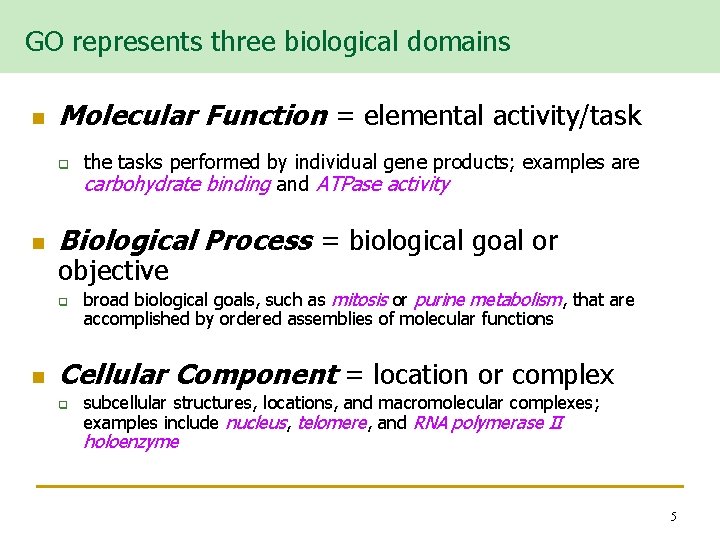
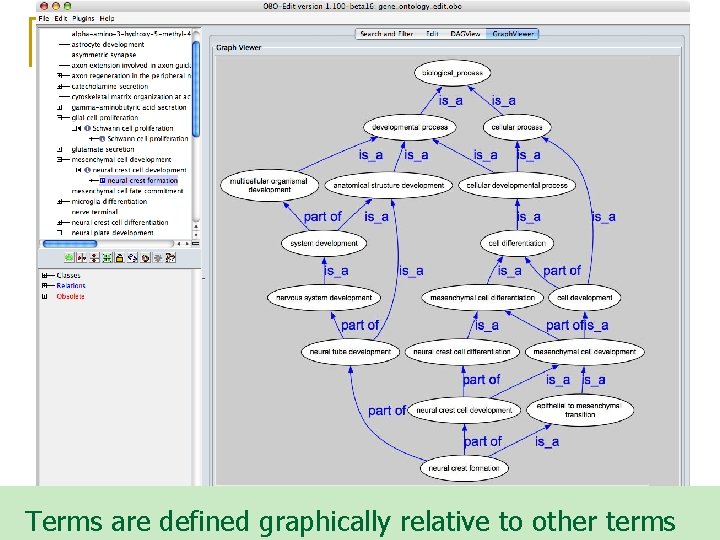
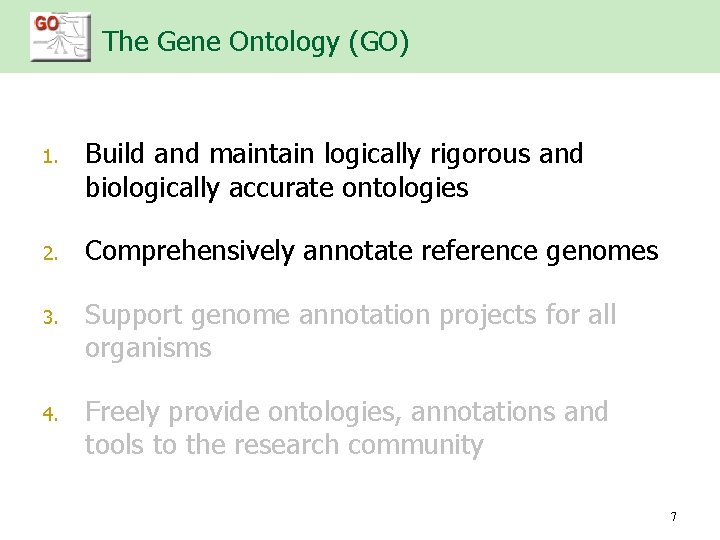
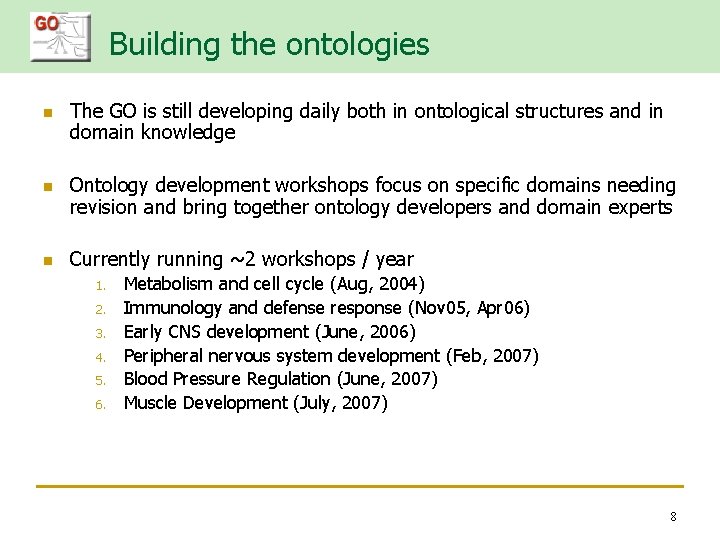
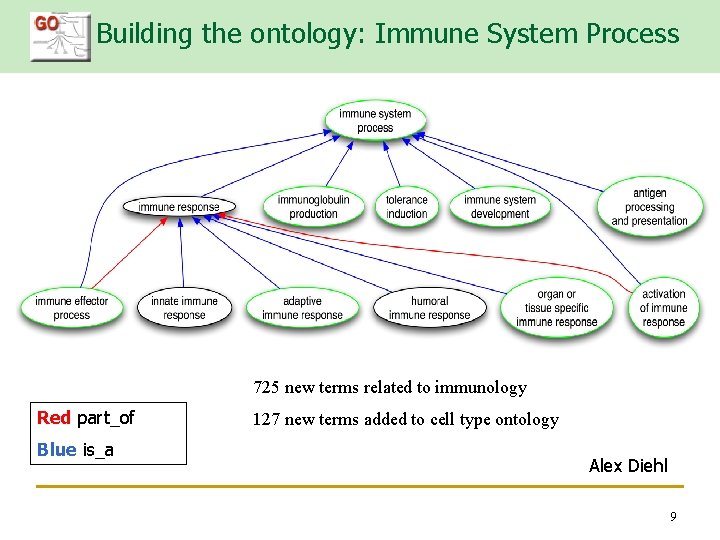
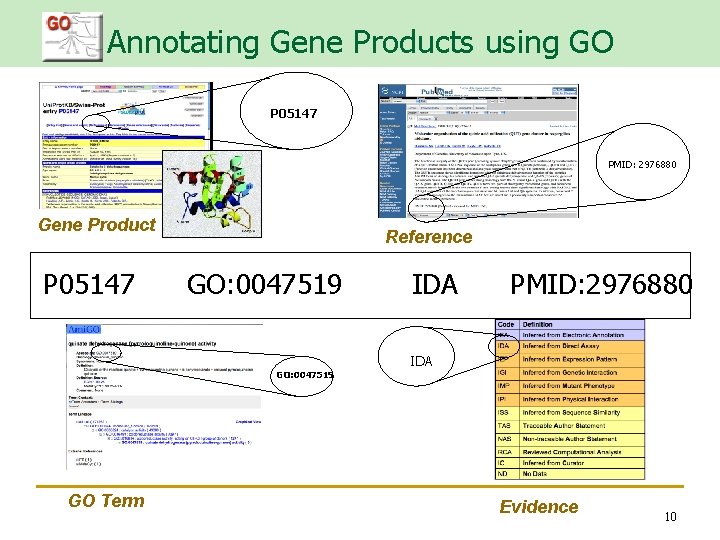
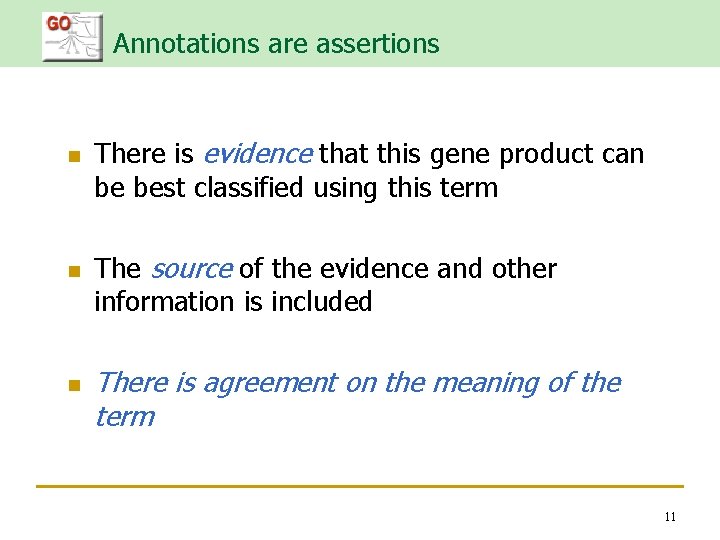
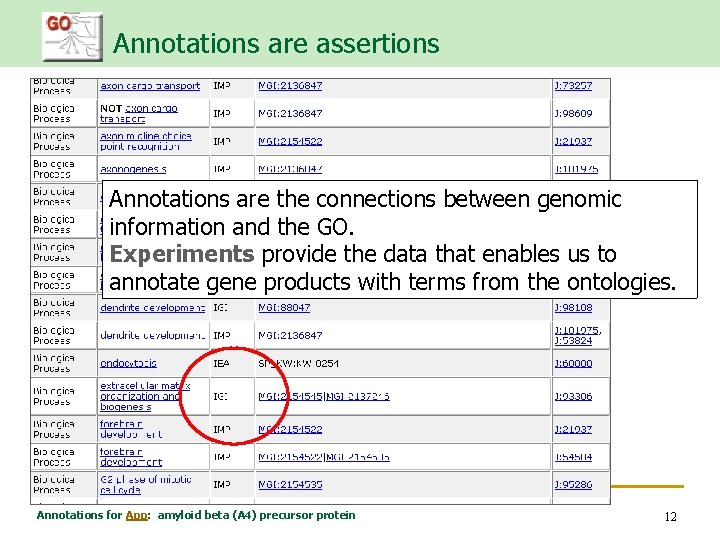
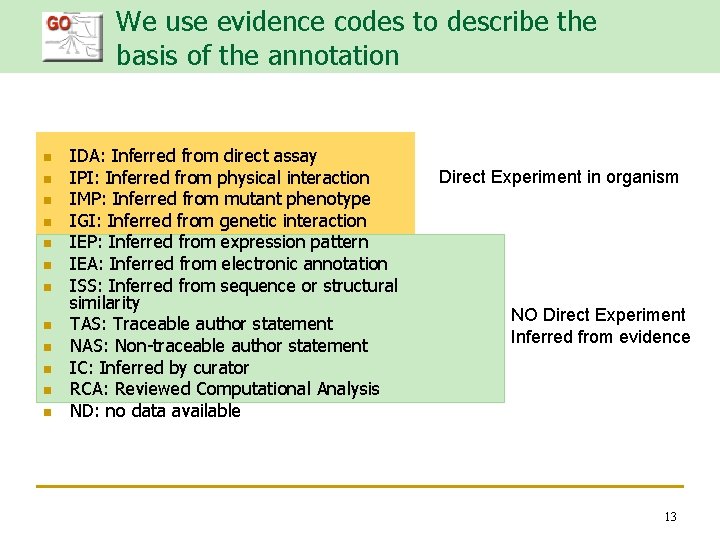
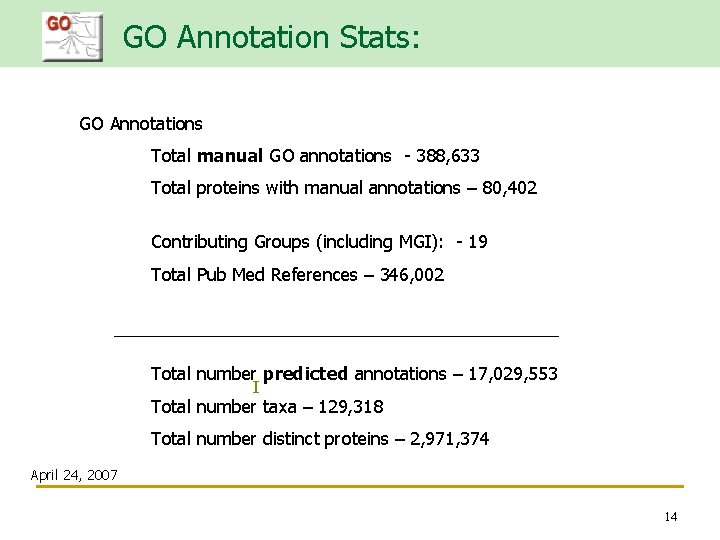
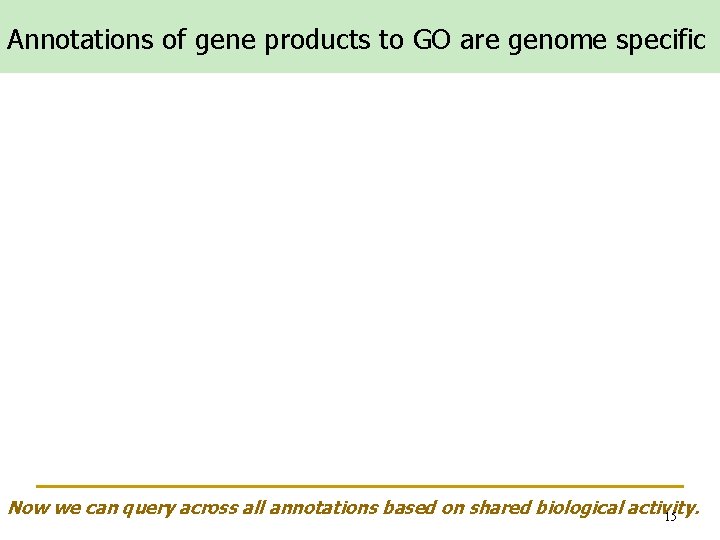
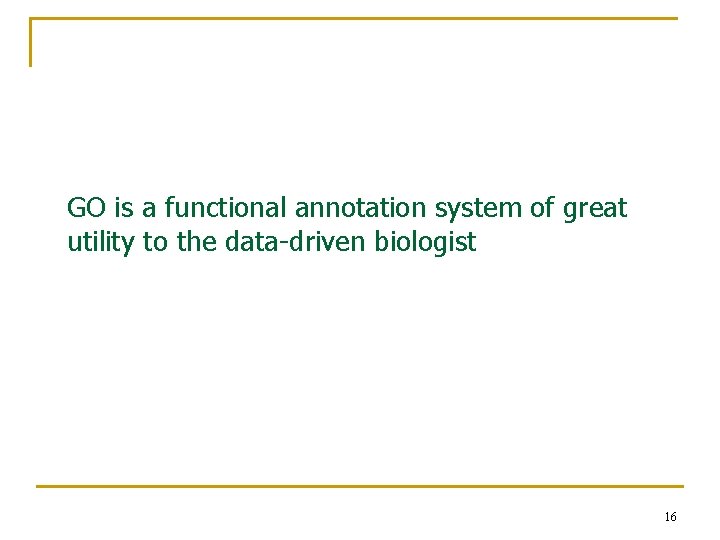
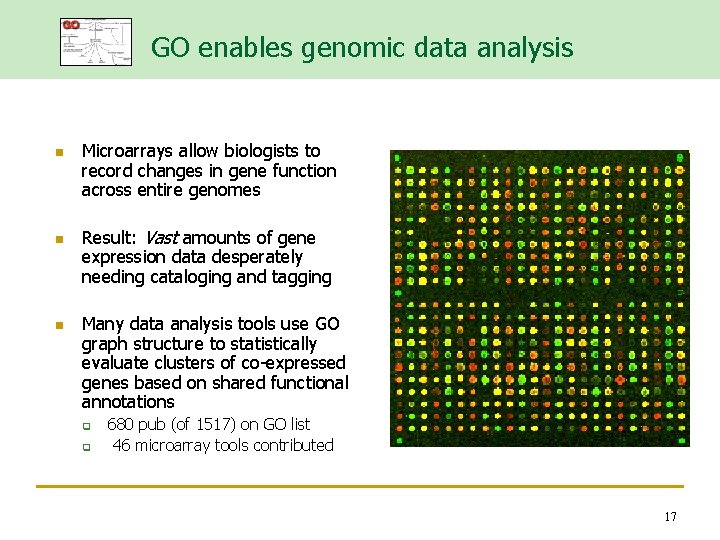
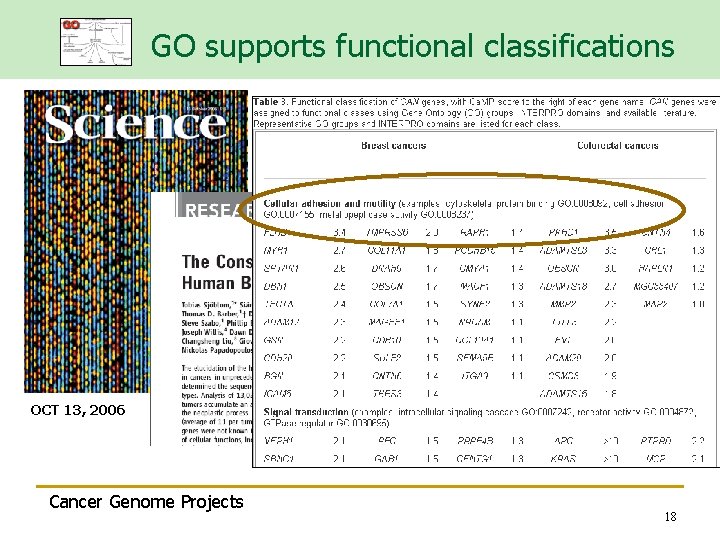
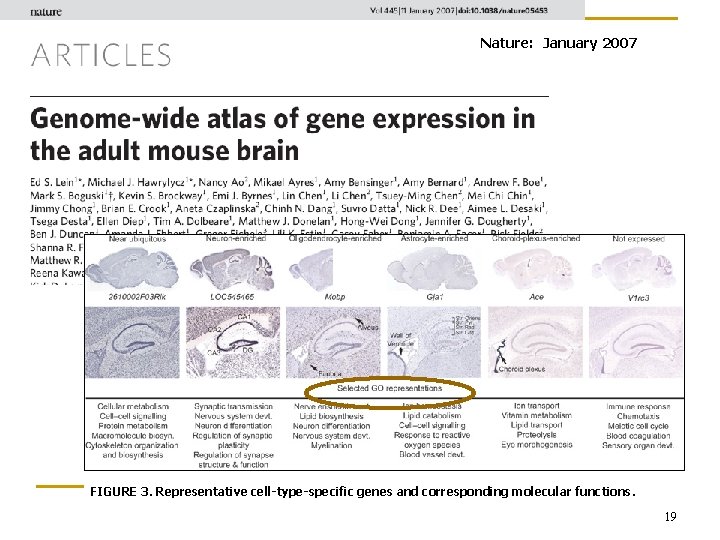
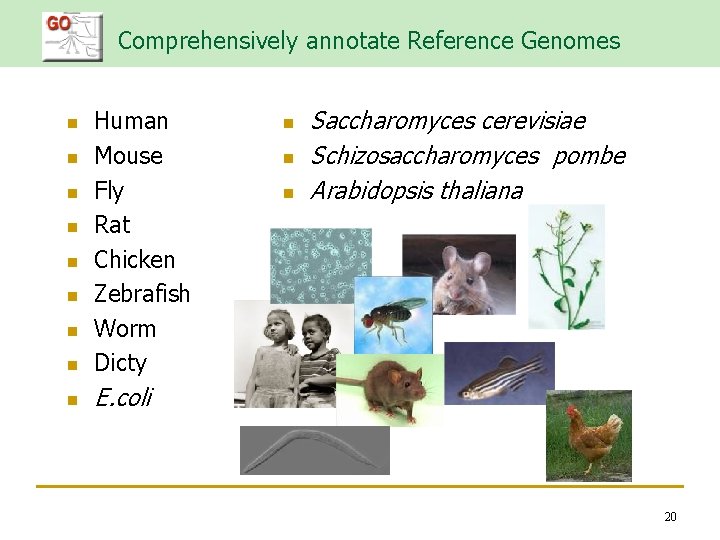
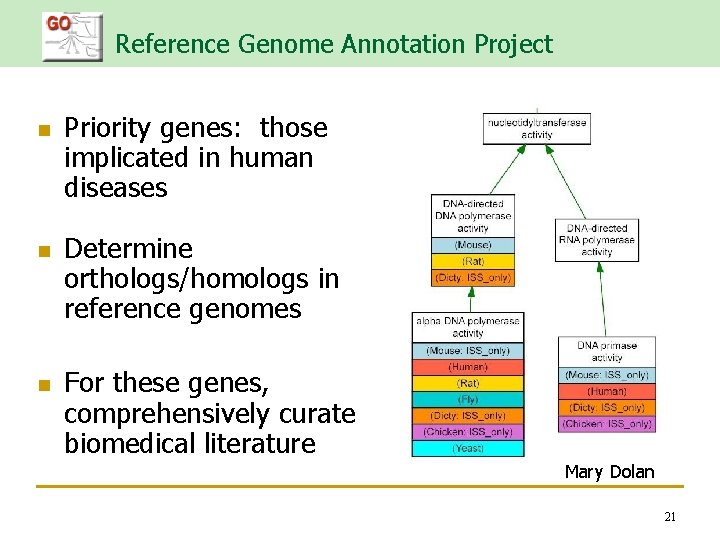
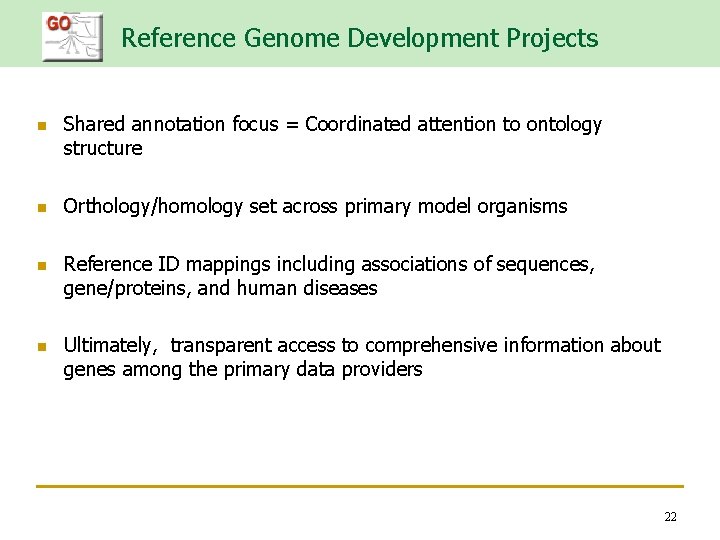
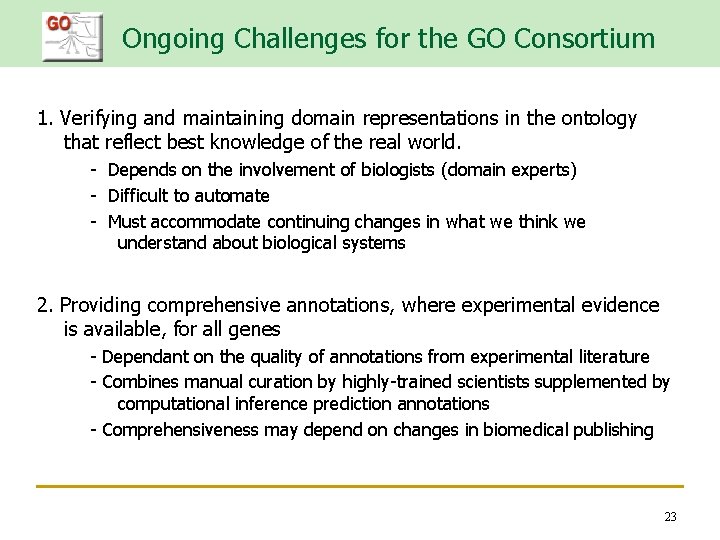
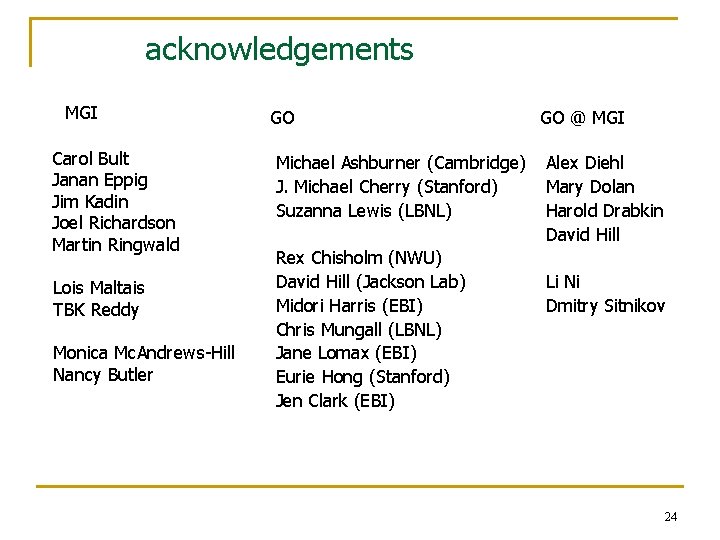
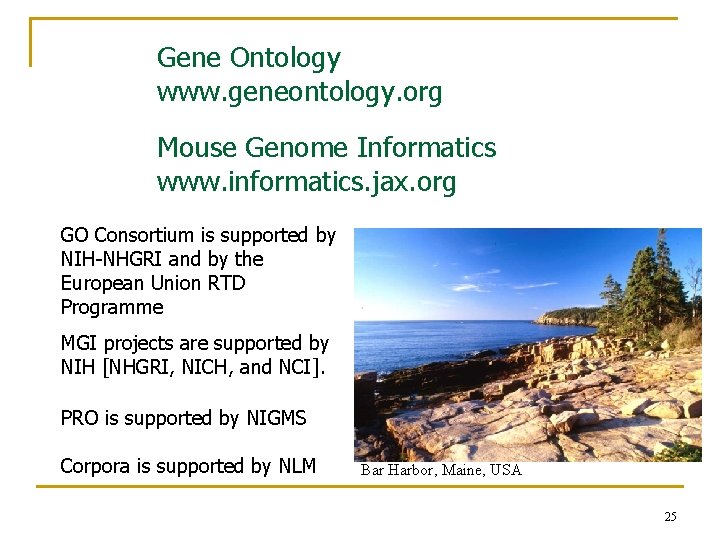
- Slides: 25
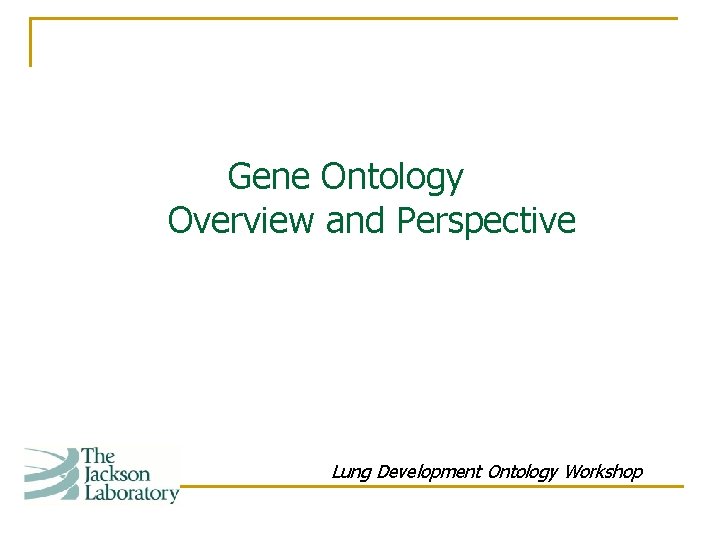
Gene Ontology Overview and Perspective Lung Development Ontology Workshop
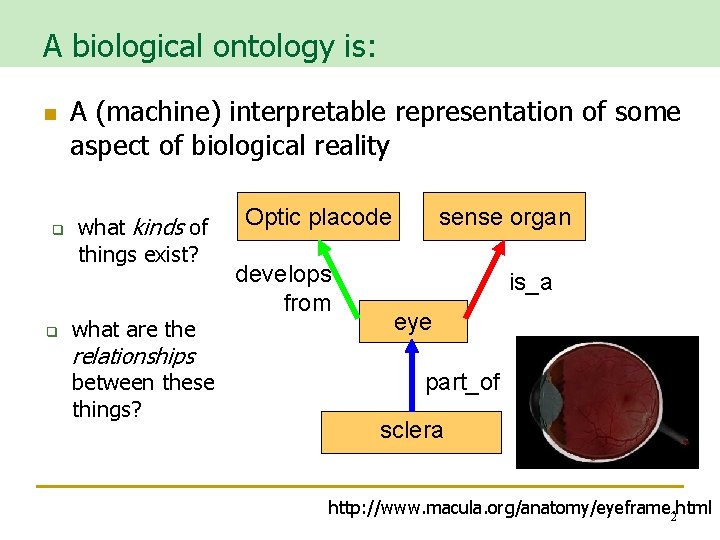
A biological ontology is: n q q A (machine) interpretable representation of some aspect of biological reality what kinds of things exist? what are the relationships between these things? Optic placode develops from sense organ is_a eye part_of sclera http: //www. macula. org/anatomy/eyeframe. html 2
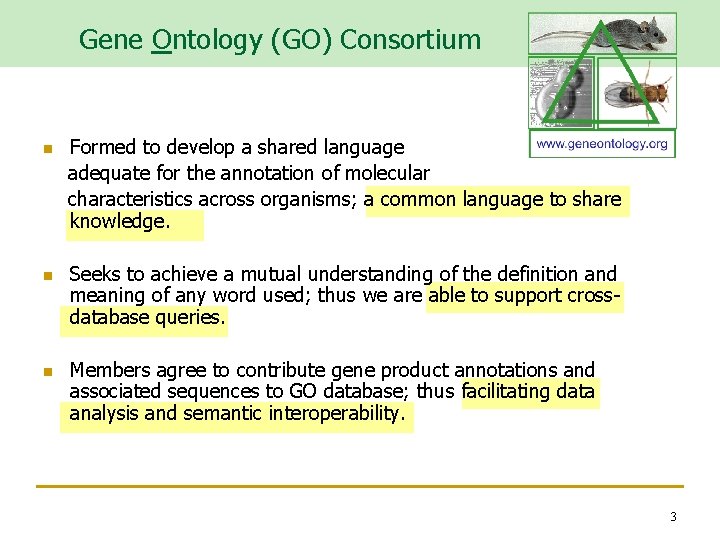
Gene Ontology (GO) Consortium n n n Formed to develop a shared language adequate for the annotation of molecular characteristics across organisms; a common language to share knowledge. Seeks to achieve a mutual understanding of the definition and meaning of any word used; thus we are able to support crossdatabase queries. Members agree to contribute gene product annotations and associated sequences to GO database; thus facilitating data analysis and semantic interoperability. 3
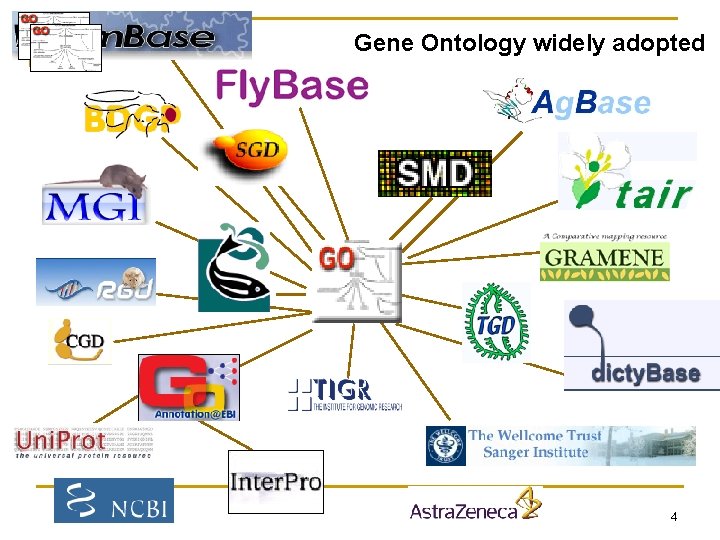
Gene Ontology widely adopted Ag. Base 4
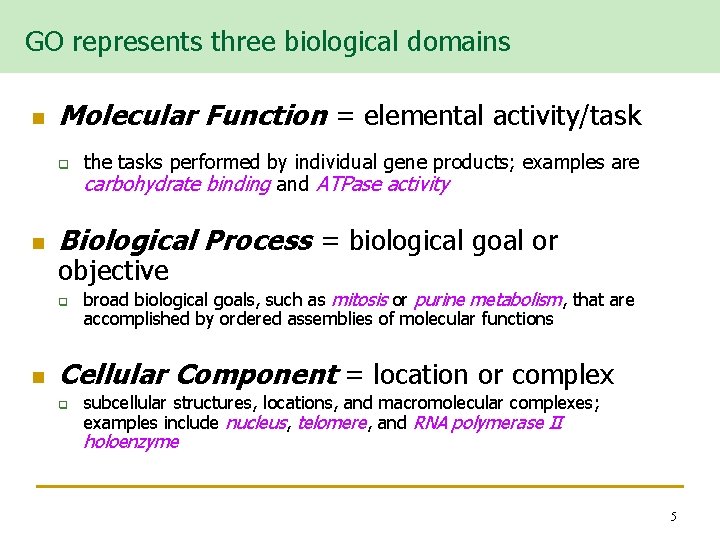
GO represents three biological domains n Molecular Function = elemental activity/task q n Biological Process = biological goal or objective q n the tasks performed by individual gene products; examples are carbohydrate binding and ATPase activity broad biological goals, such as mitosis or purine metabolism, that are accomplished by ordered assemblies of molecular functions Cellular Component = location or complex q subcellular structures, locations, and macromolecular complexes; examples include nucleus, telomere, and RNA polymerase II holoenzyme 5
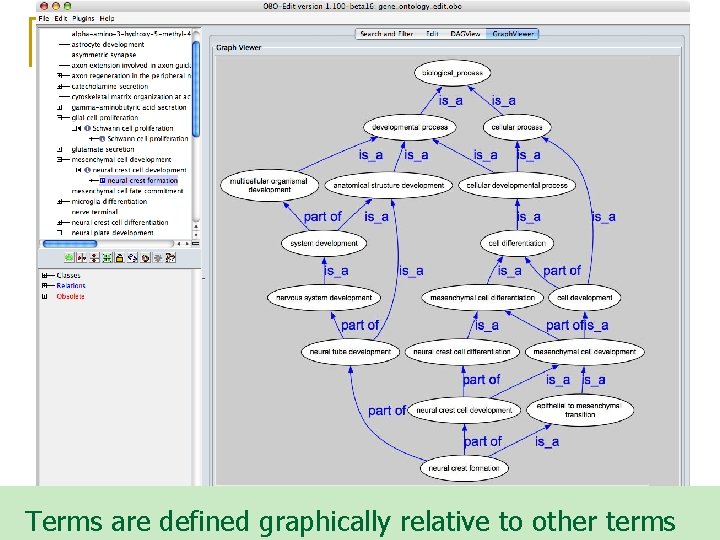
Terms are defined graphically relative to other terms
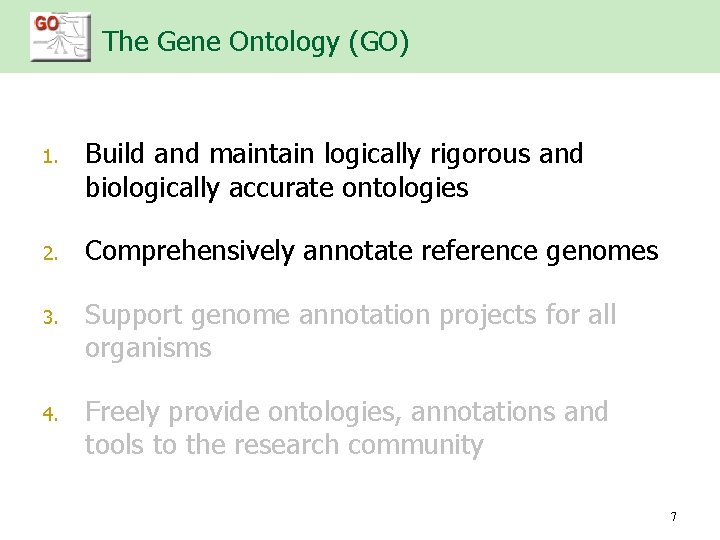
The Gene Ontology (GO) n 1. n 2. n 3. n 4. Buildand andmaintainlogicallyrigorousand biologicallyaccurateontologies Comprehensivelyannotatereferencegenomes Supportgenomeannotationprojectsfor forall organisms Freelyprovideontologies, annotationsand andtools to the to research community tools the research community 7
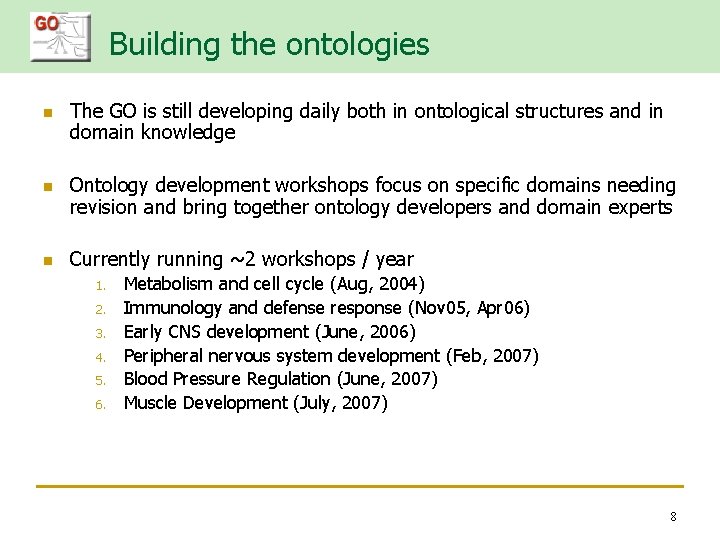
Building the ontologies n n n The GO is still developing daily both in ontological structures and in domain knowledge Ontology development workshops focus on specific domains needing revision and bring together ontology developers and domain experts Currently running ~2 workshops / year 1. 2. 3. 4. 5. 6. Metabolism and cell cycle (Aug, 2004) Immunology and defense response (Nov 05, Apr 06) Early CNS development (June, 2006) Peripheral nervous system development (Feb, 2007) Blood Pressure Regulation (June, 2007) Muscle Development (July, 2007) 8
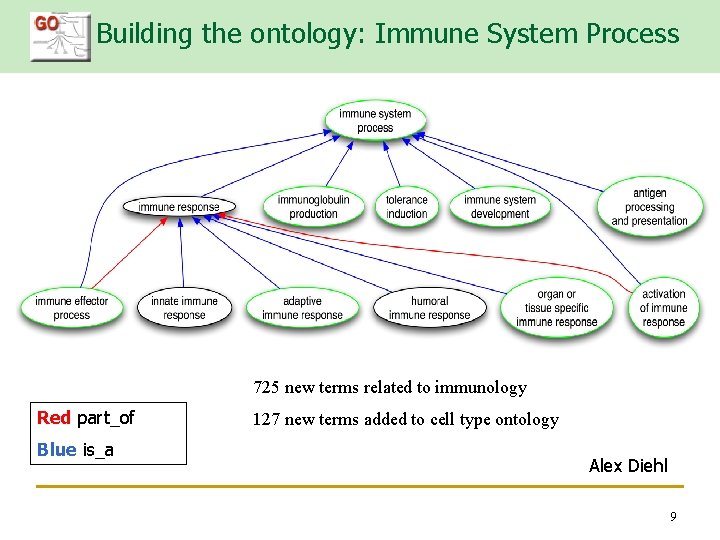
Building the ontology: Immune System Process 725 new terms related to immunology Red part_of Blue is_a 127 new terms added to cell type ontology Alex Diehl 9
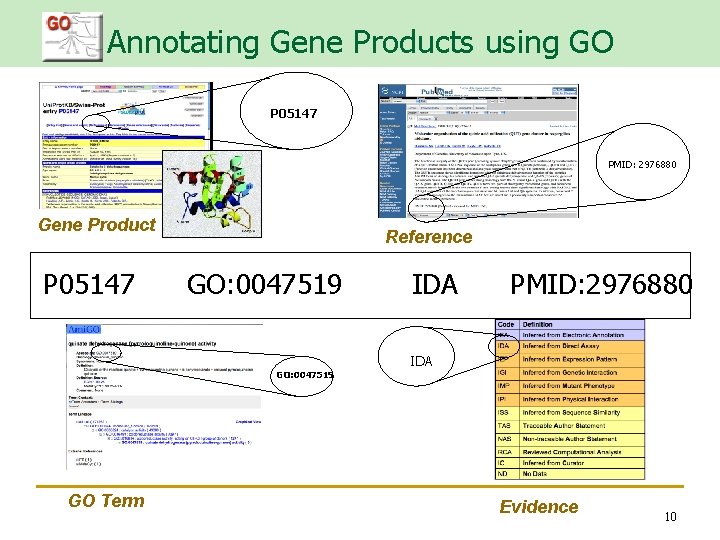
Annotating Gene Products using GO P 05147 PMID: 2976880 Gene Product P 05147 Reference GO: 0047519 IDA PMID: 2976880 IDA GO: 0047519 GO Term Evidence 10
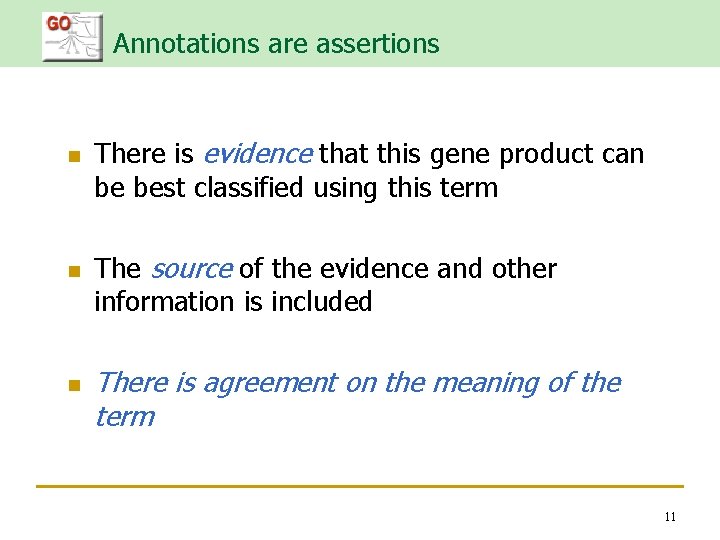
Annotations are assertions n n n There is evidence that this gene product can be best classified using this term The source of the evidence and other information is included There is agreement on the meaning of the term 11
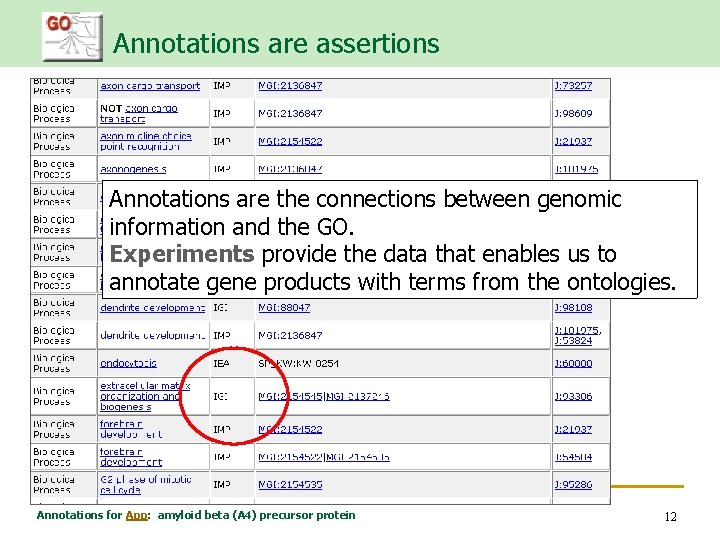
Annotations are assertions Annotations are the connections between genomic information and the GO. Experiments provide the data that enables us to annotate gene products with terms from the ontologies. Annotations for App: amyloid beta (A 4) precursor protein 12
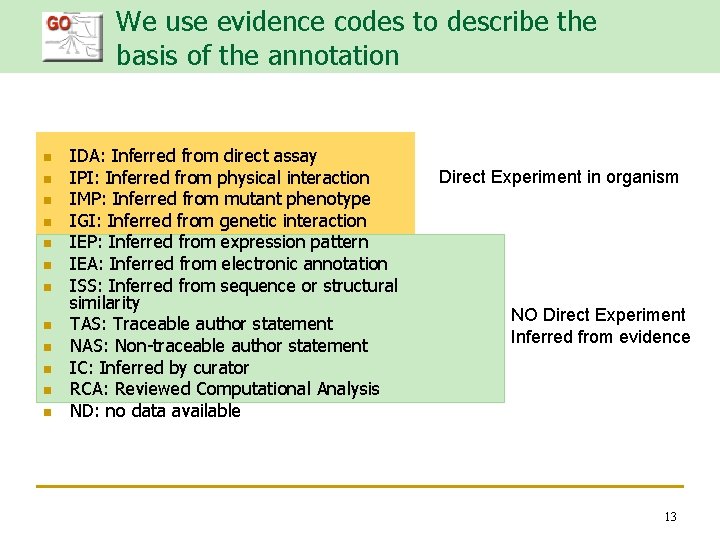
We use evidence codes to describe the basis of the annotation n n n IDA: Inferred from direct assay IPI: Inferred from physical interaction IMP: Inferred from mutant phenotype IGI: Inferred from genetic interaction IEP: Inferred from expression pattern IEA: Inferred from electronic annotation ISS: Inferred from sequence or structural similarity TAS: Traceable author statement NAS: Non-traceable author statement IC: Inferred by curator RCA: Reviewed Computational Analysis ND: no data available Direct Experiment in organism NO Direct Experiment Inferred from evidence 13
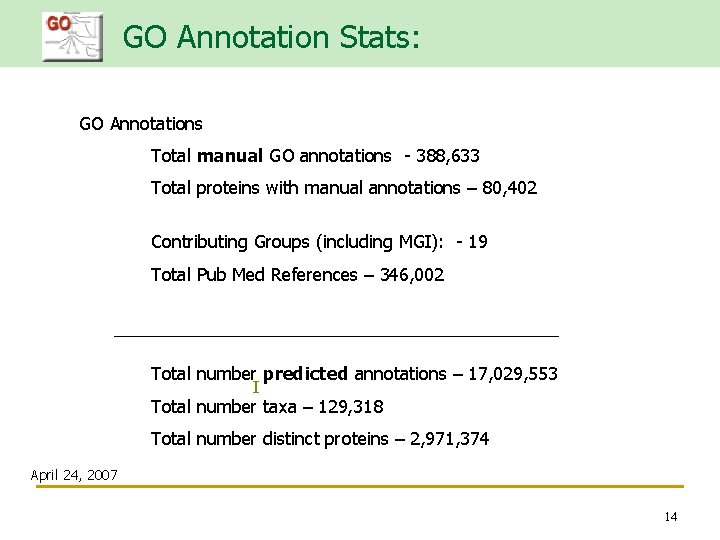
GO Annotation Stats: GO Annotations Total manual GO annotations - 388, 633 Total proteins with manual annotations – 80, 402 Contributing Groups (including MGI): - 19 Total Pub Med References – 346, 002 Total number predicted annotations – 17, 029, 553 I Total number taxa – 129, 318 Total number distinct proteins – 2, 971, 374 April 24, 2007 14
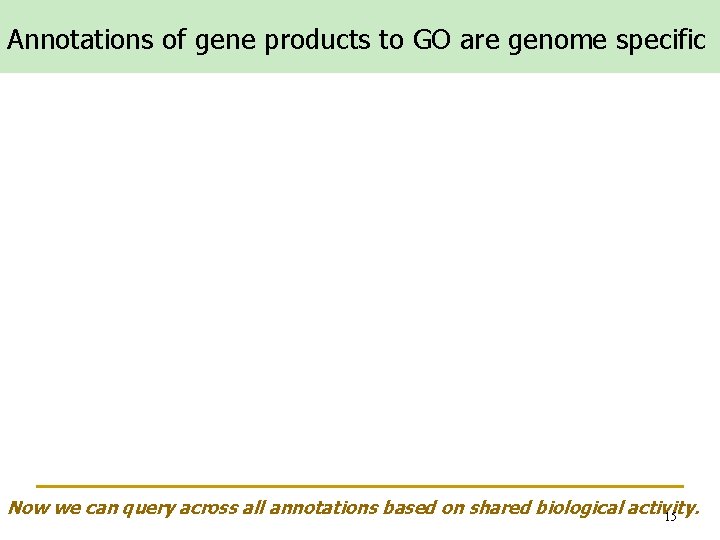
Annotations of gene products to GO are genome specific Now we can query across all annotations based on shared biological activity. 15
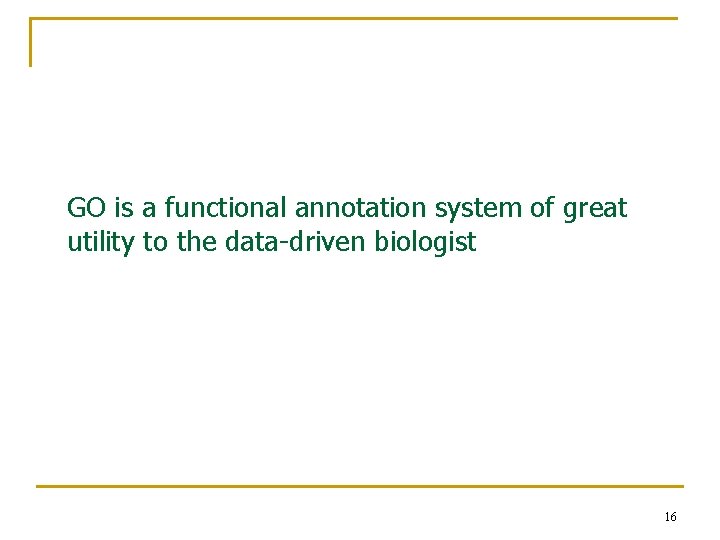
GO is a functional annotation system of great utility to the data-driven biologist 16
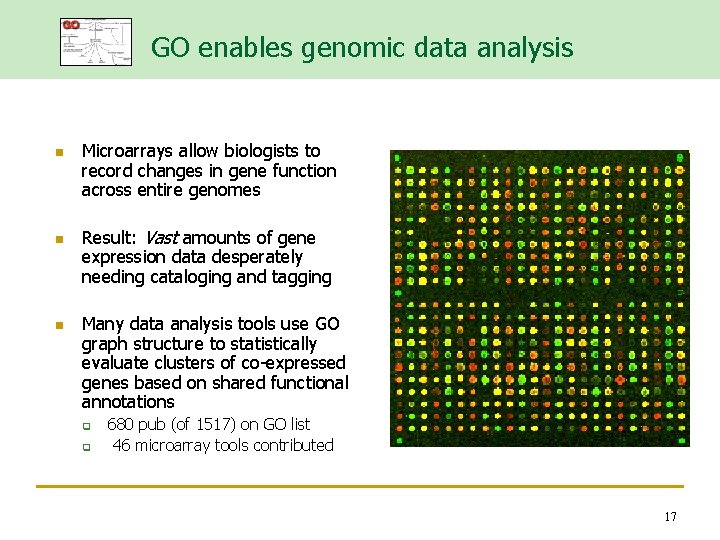
GO enables genomic data analysis n n n Microarrays allow biologists to record changes in gene function across entire genomes Result: Vast amounts of gene expression data desperately needing cataloging and tagging Many data analysis tools use GO graph structure to statistically evaluate clusters of co-expressed genes based on shared functional annotations q q 680 pub (of 1517) on GO list 46 microarray tools contributed 17
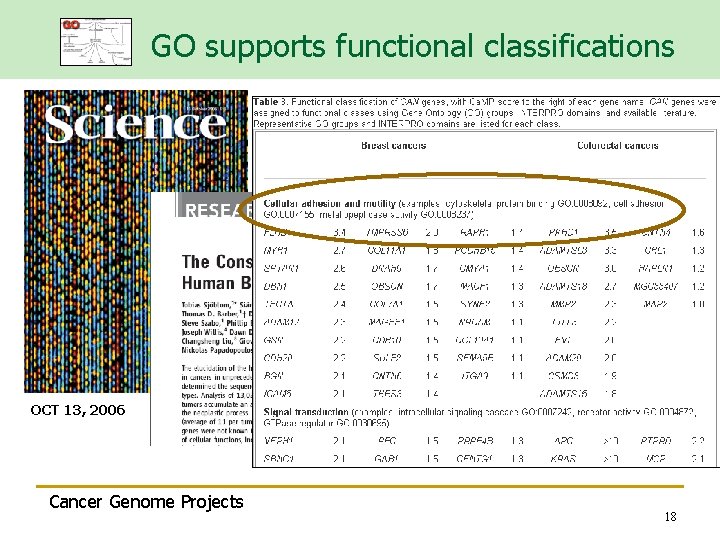
GO supports functional classifications OCT 13, 2006 Cancer Genome Projects 18
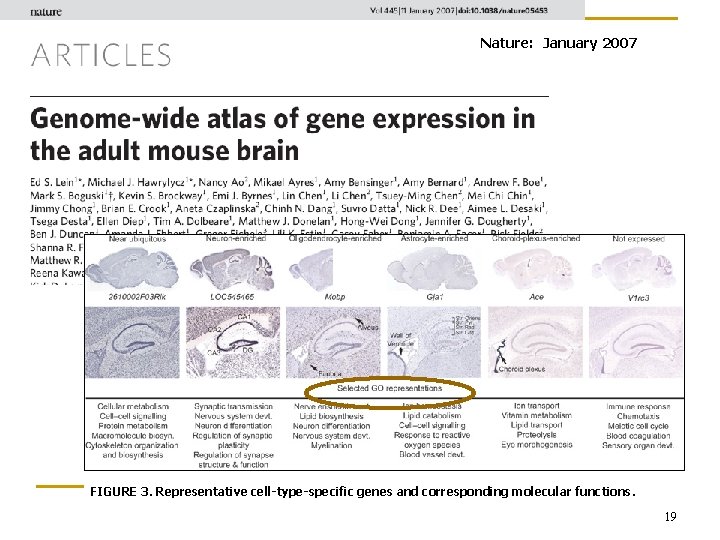
GO is wildly successful Nature: January 2007 FIGURE 3. Representative cell-type-specific genes and corresponding molecular functions. 19
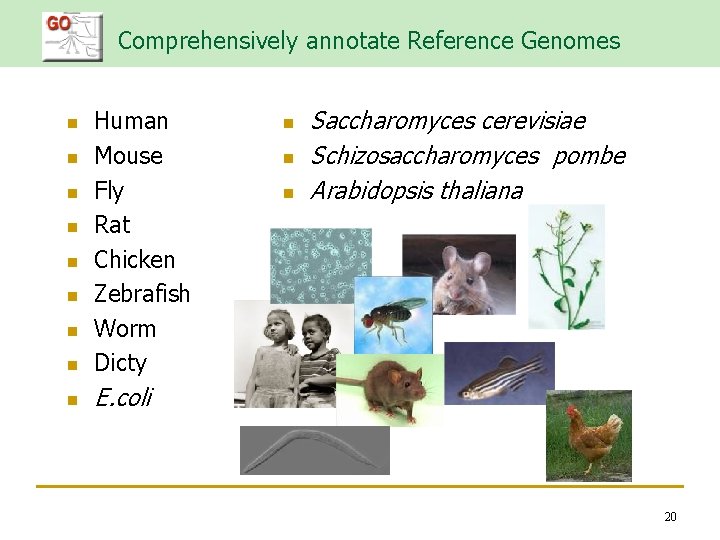
Comprehensively annotate Reference Genomes n Human Mouse Fly Rat Chicken Zebrafish Worm Dicty n E. coli n n n n n Saccharomyces cerevisiae Schizosaccharomyces pombe Arabidopsis thaliana 20
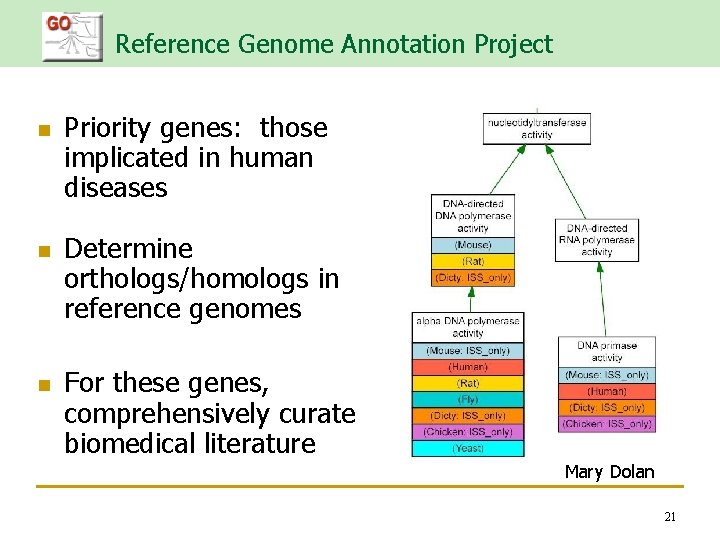
Reference Genome Annotation Project n n n Priority genes: those implicated in human diseases Determine orthologs/homologs in reference genomes For these genes, comprehensively curate biomedical literature Mary Dolan 21
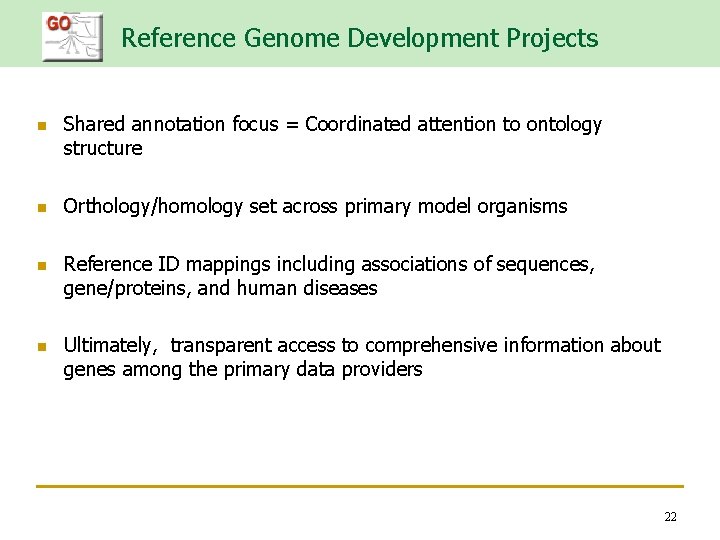
Reference Genome Development Projects n n Shared annotation focus = Coordinated attention to ontology structure Orthology/homology set across primary model organisms Reference ID mappings including associations of sequences, gene/proteins, and human diseases Ultimately, transparent access to comprehensive information about genes among the primary data providers 22
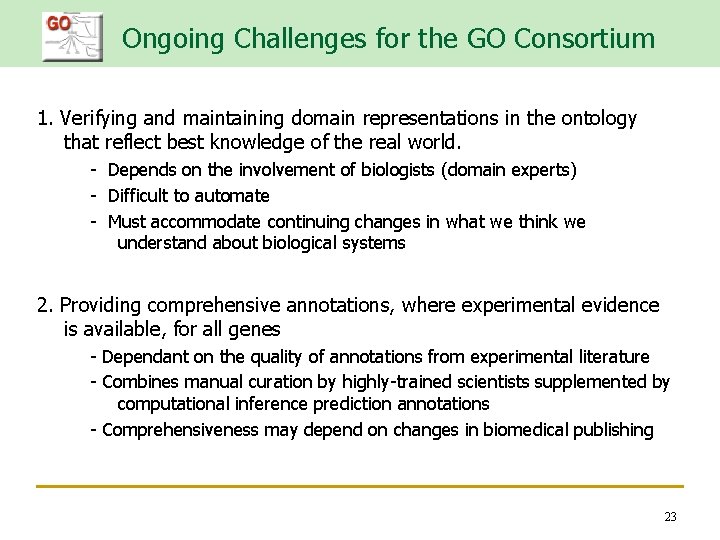
Ongoing Challenges for the GO Consortium 1. Verifying and maintaining domain representations in the ontology that reflect best knowledge of the real world. - Depends on the involvement of biologists (domain experts) - Difficult to automate - Must accommodate continuing changes in what we think we understand about biological systems 2. Providing comprehensive annotations, where experimental evidence is available, for all genes - Dependant on the quality of annotations from experimental literature - Combines manual curation by highly-trained scientists supplemented by computational inference prediction annotations - Comprehensiveness may depend on changes in biomedical publishing 23
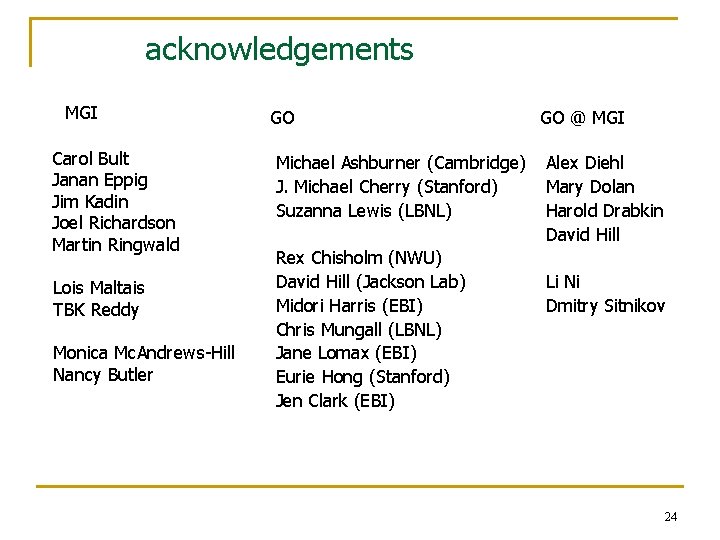
acknowledgements MGI Carol Bult Janan Eppig Jim Kadin Joel Richardson Martin Ringwald Lois Maltais TBK Reddy Monica Mc. Andrews-Hill Nancy Butler GO Michael Ashburner (Cambridge) J. Michael Cherry (Stanford) Suzanna Lewis (LBNL) Rex Chisholm (NWU) David Hill (Jackson Lab) Midori Harris (EBI) Chris Mungall (LBNL) Jane Lomax (EBI) Eurie Hong (Stanford) Jen Clark (EBI) GO @ MGI Alex Diehl Mary Dolan Harold Drabkin David Hill Li Ni Dmitry Sitnikov 24
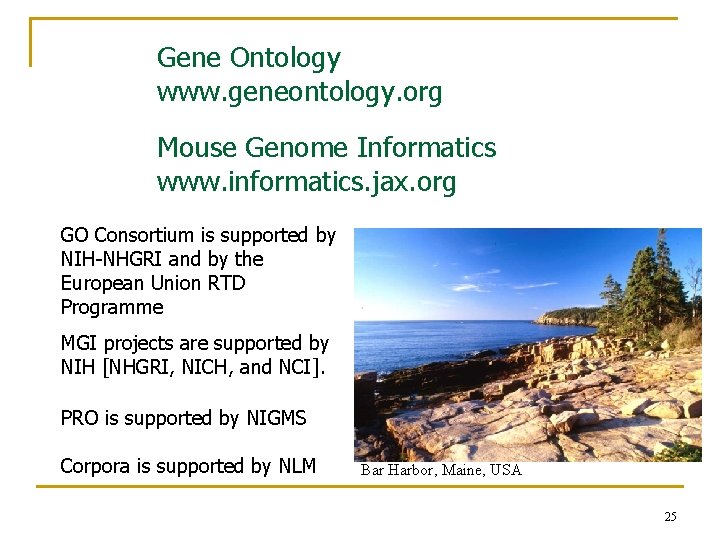
Gene Ontology www. geneontology. org Mouse Genome Informatics www. informatics. jax. org GO Consortium is supported by NIH-NHGRI and by the European Union RTD Programme MGI projects are supported by NIH [NHGRI, NICH, and NCI]. PRO is supported by NIGMS Corpora is supported by NLM Bar Harbor, Maine, USA 25
What is meant by gene ontology?
Gene ontology
Gene ontology project
Gene ontology project
Gene by gene test results
Protein power point
Stages of lung development
2 point perspective stairs
Silo perspective vs business process perspective
Research ontology and epistemology
Epistemology vs ontology
Ontology epistemology axiology
Lifespan development a psychological perspective
Whats community development
Difference between right and left bronchus
Lung and esophagus
Tactile fremitus increased
Caplan syndrome
Obstructive and restrictive lung disease
Tree in lung real photo
What is an ontology
Suggested upper merged ontology
Protege pizza ontology
Analyticism
Ontology alignment
Types of ontology