ENTERPRISE DATA STRATEGY CAS Ratemaking Seminar March 2004
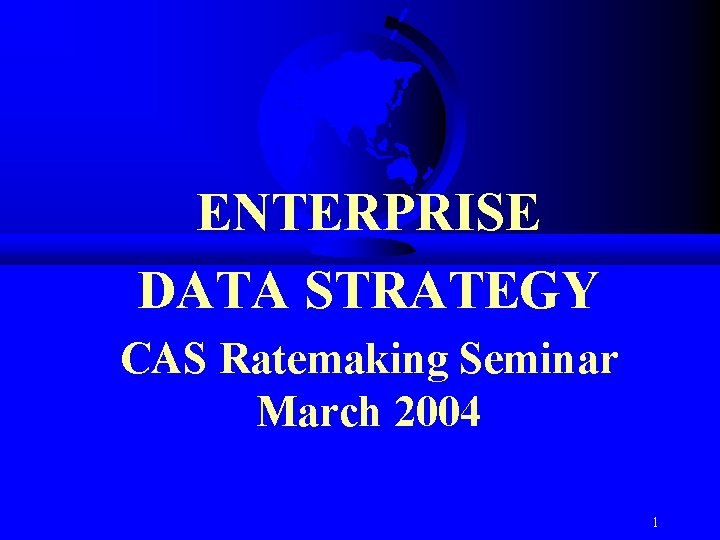
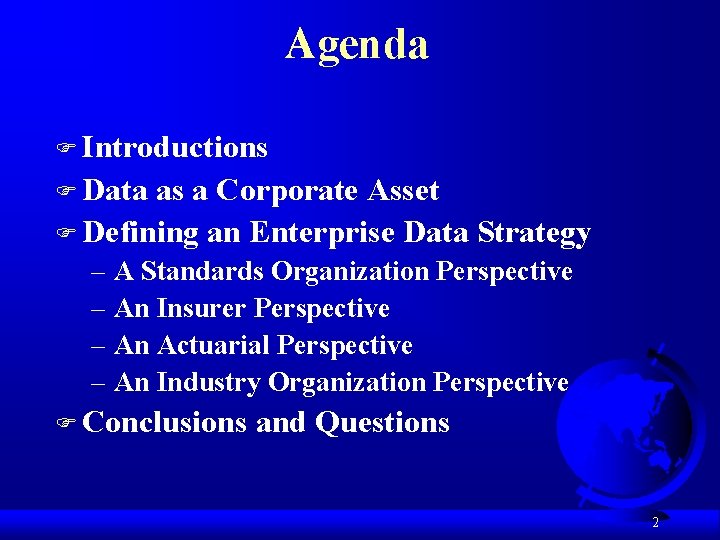
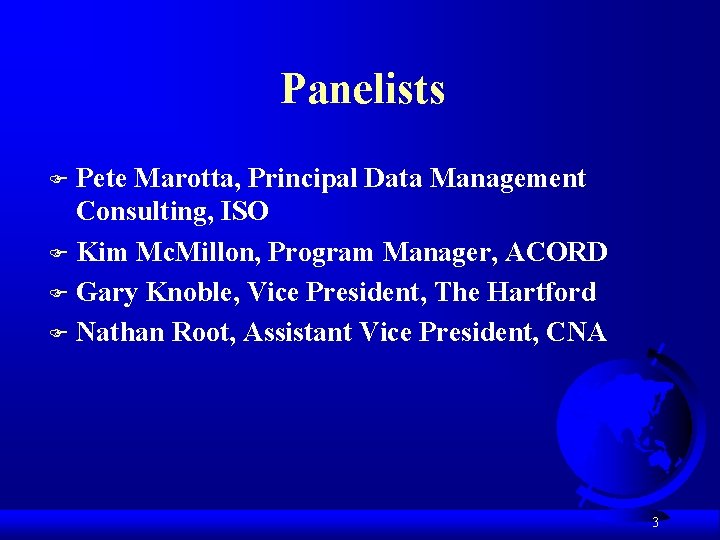
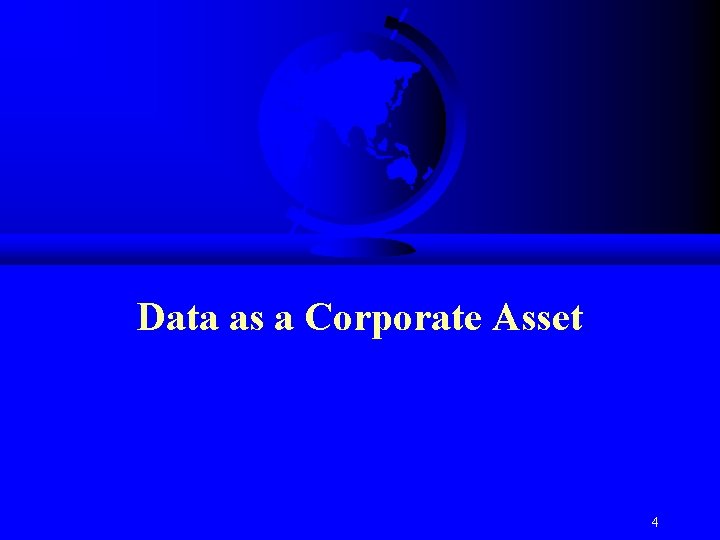
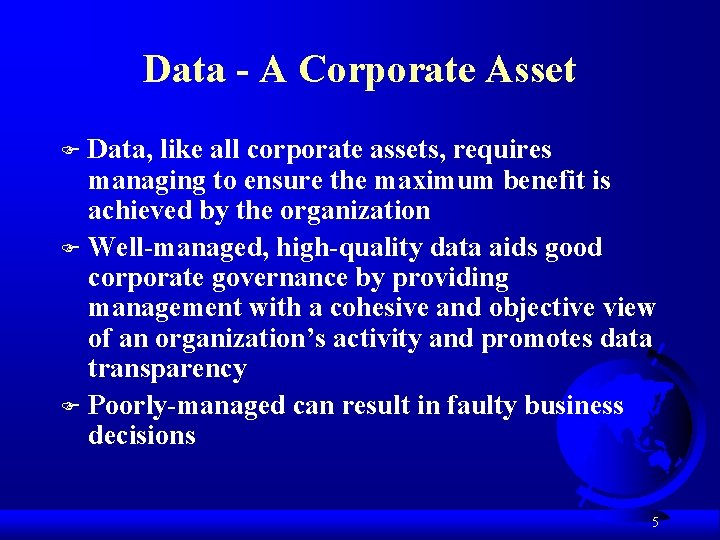
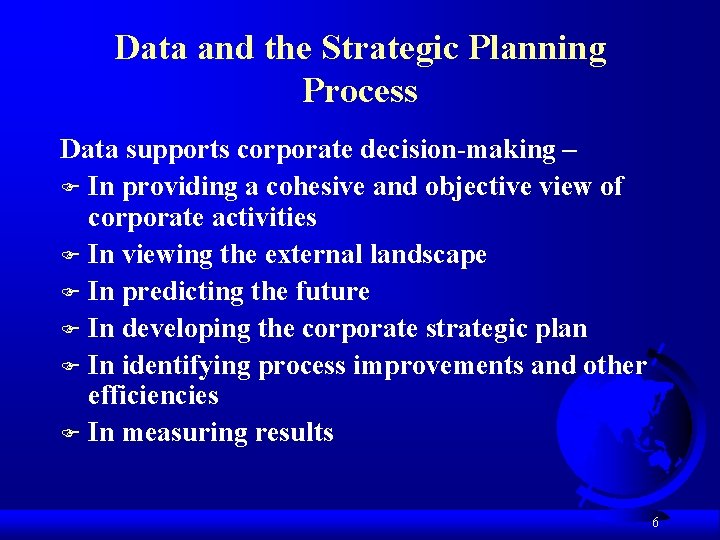
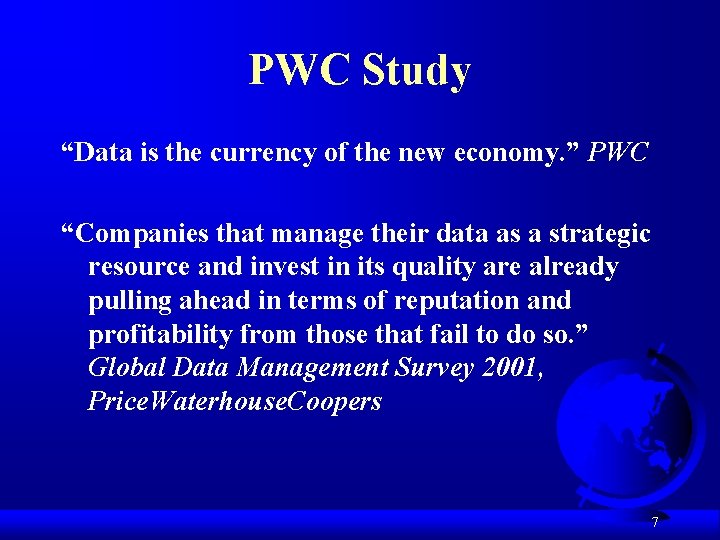
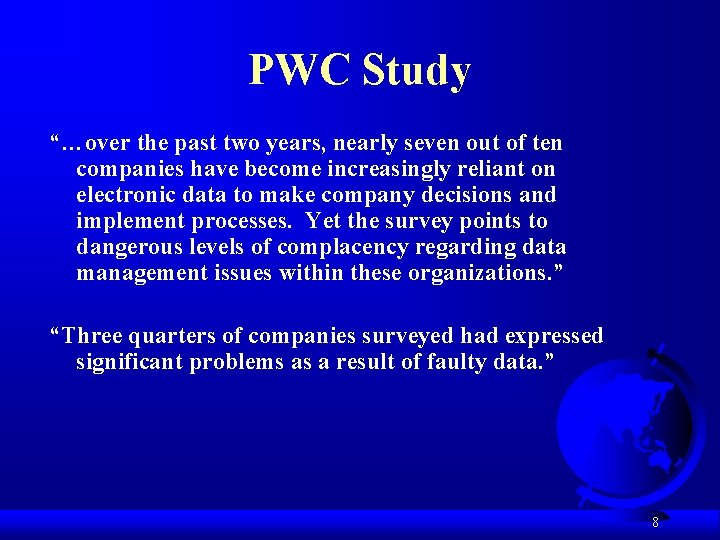
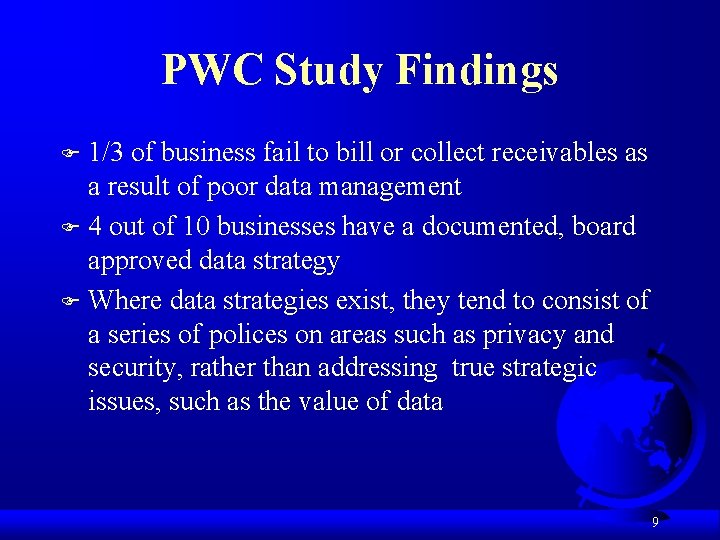
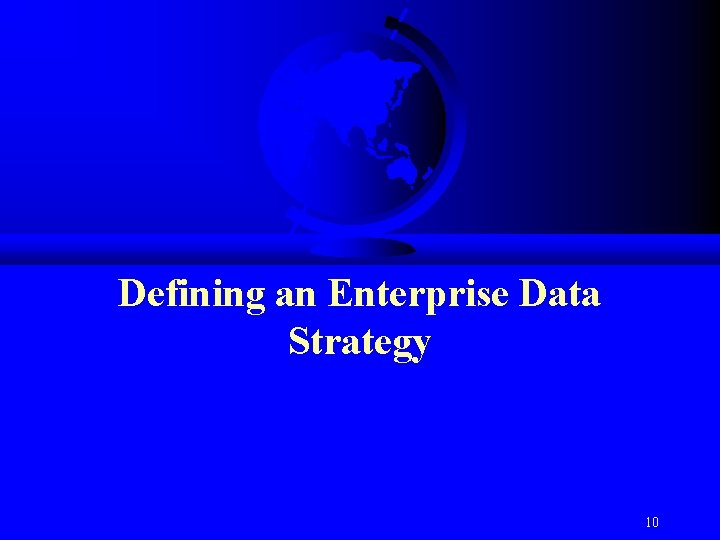
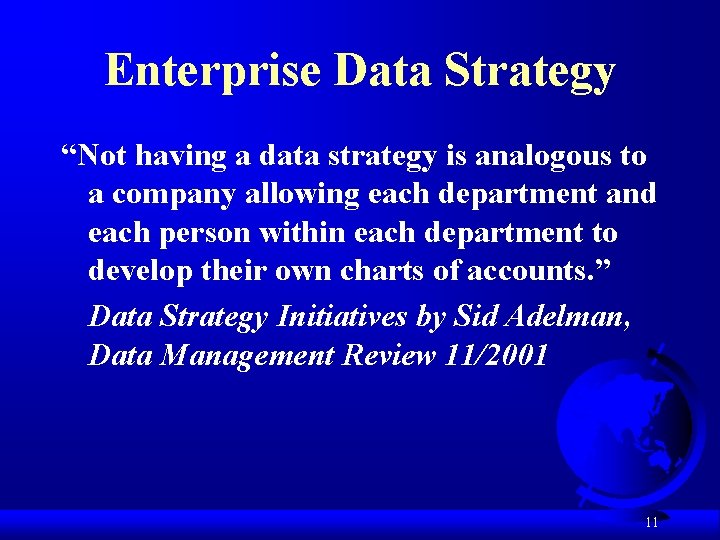
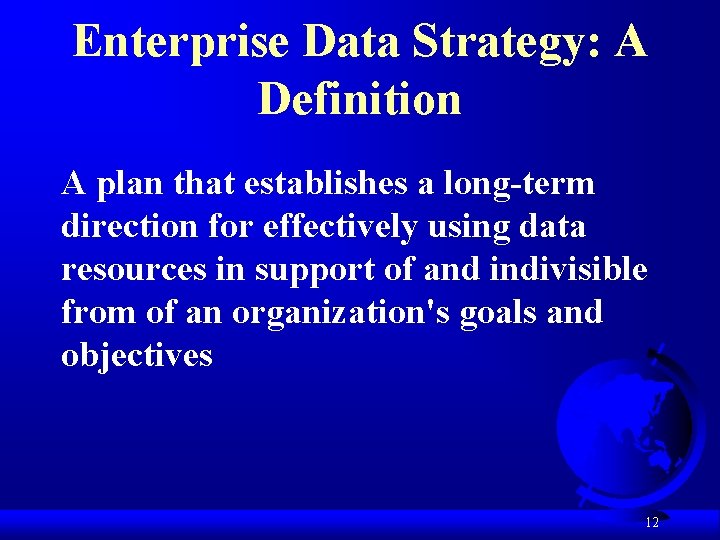
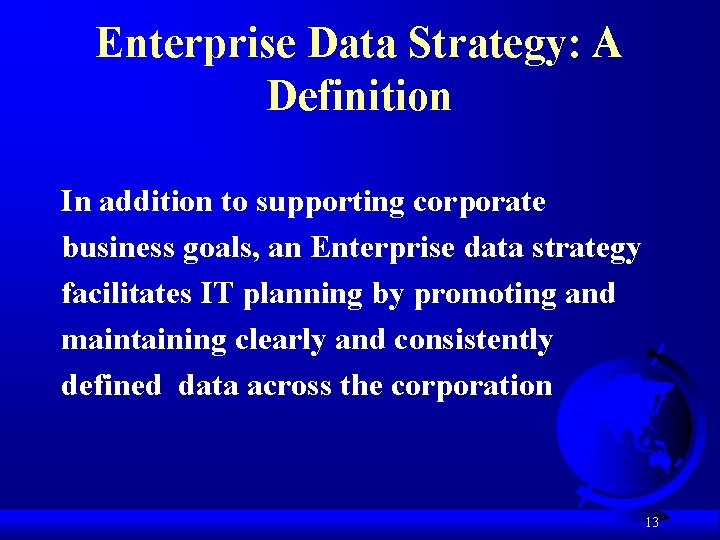
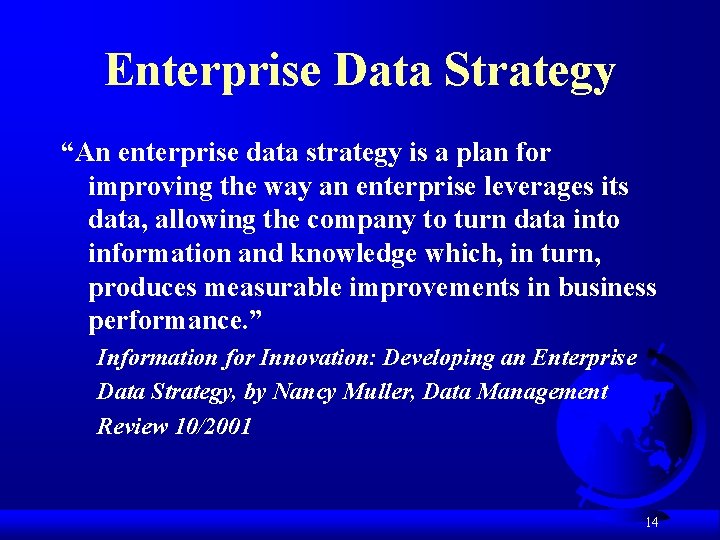
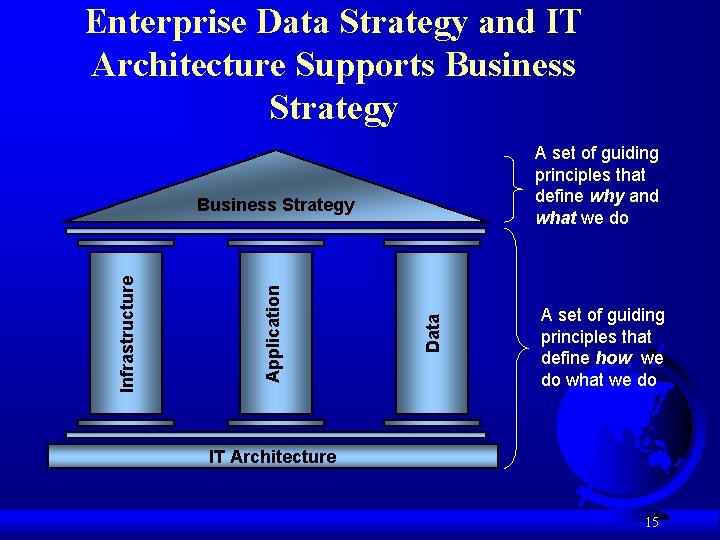
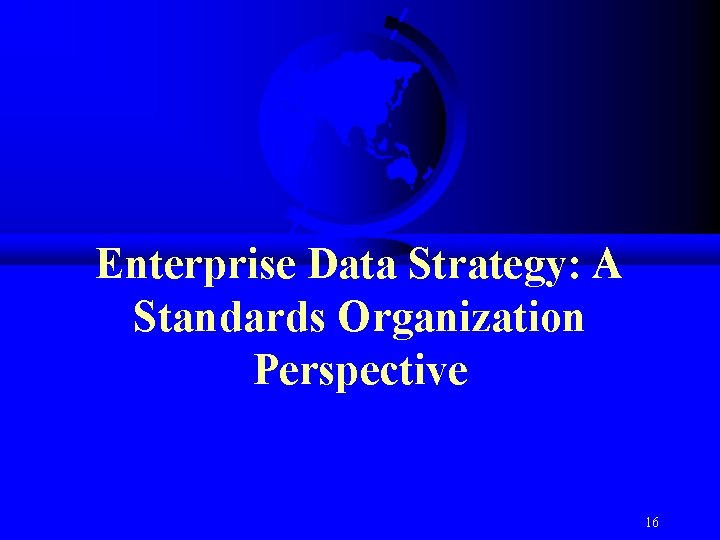
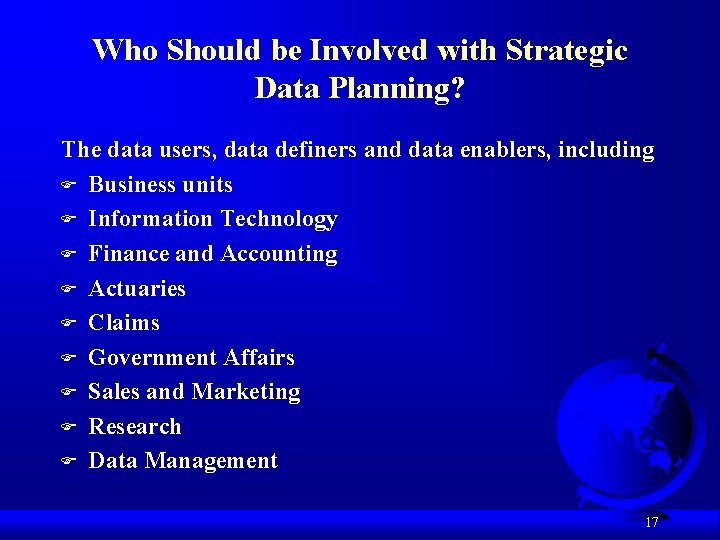
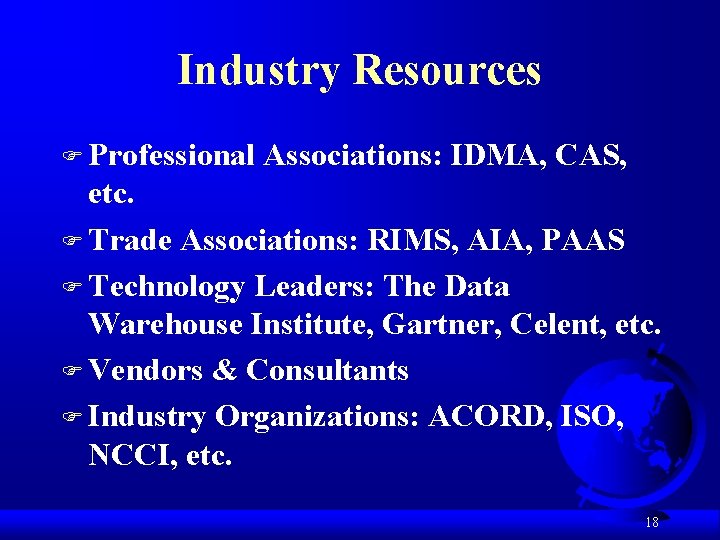
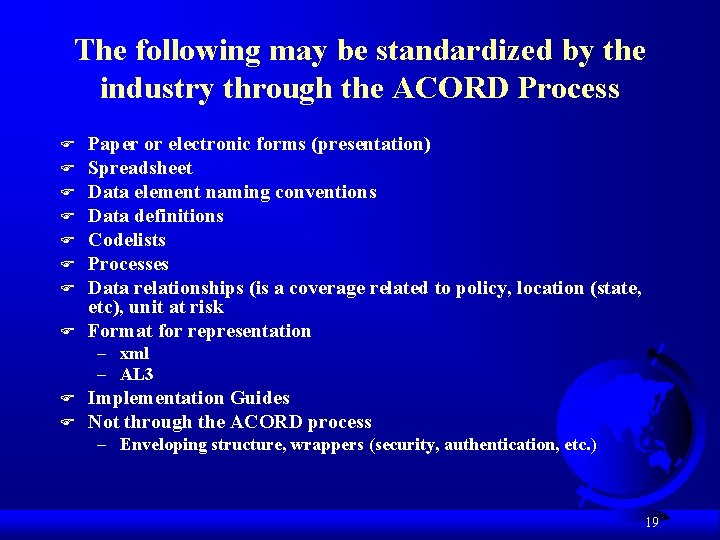
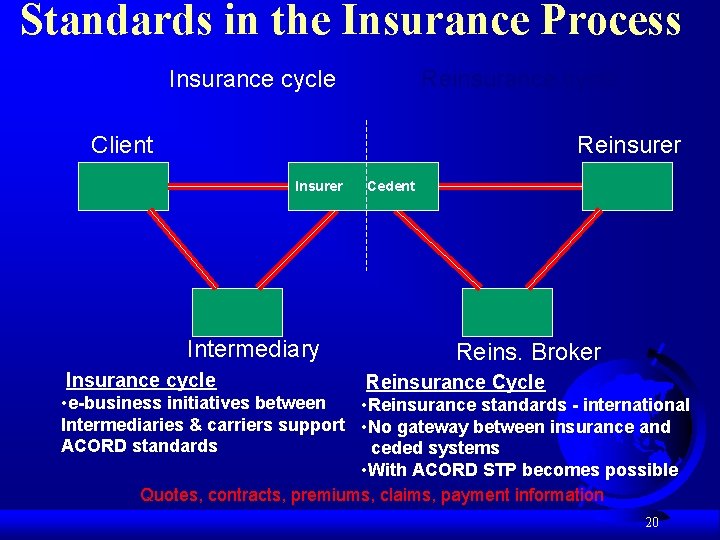
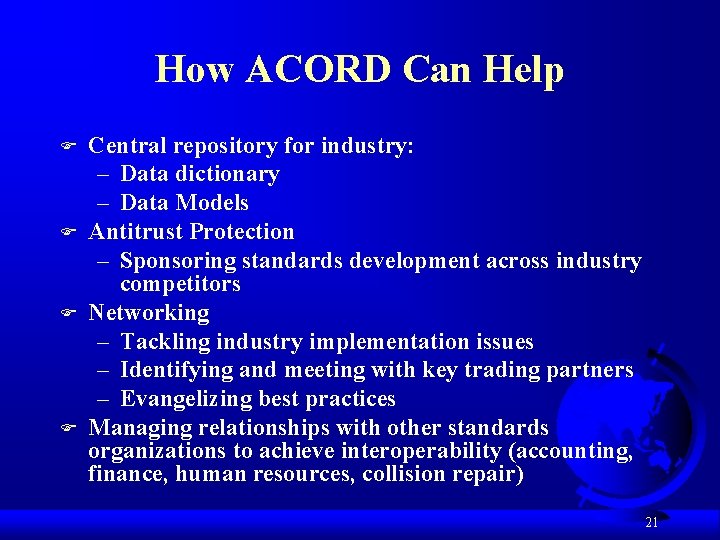
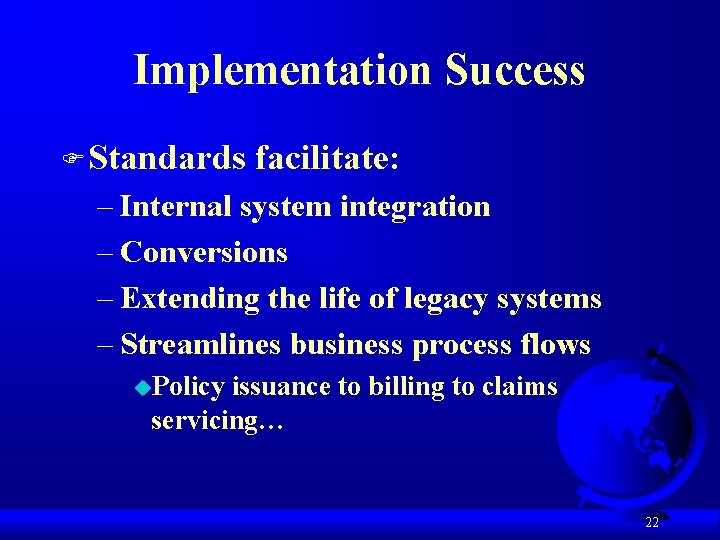
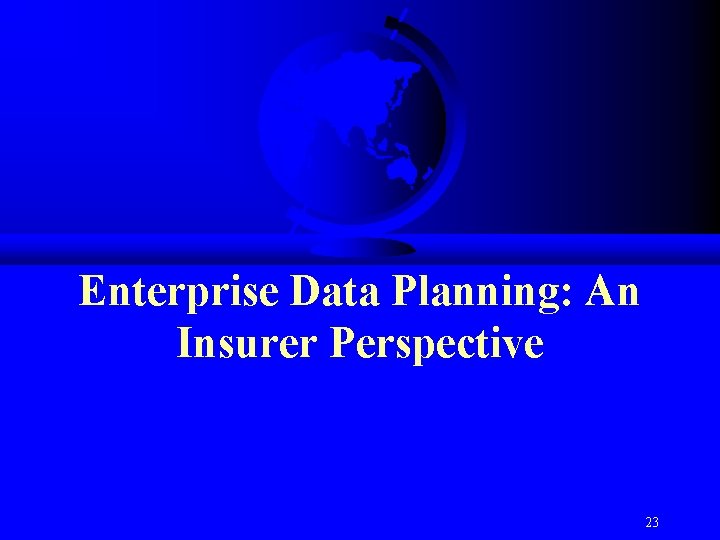
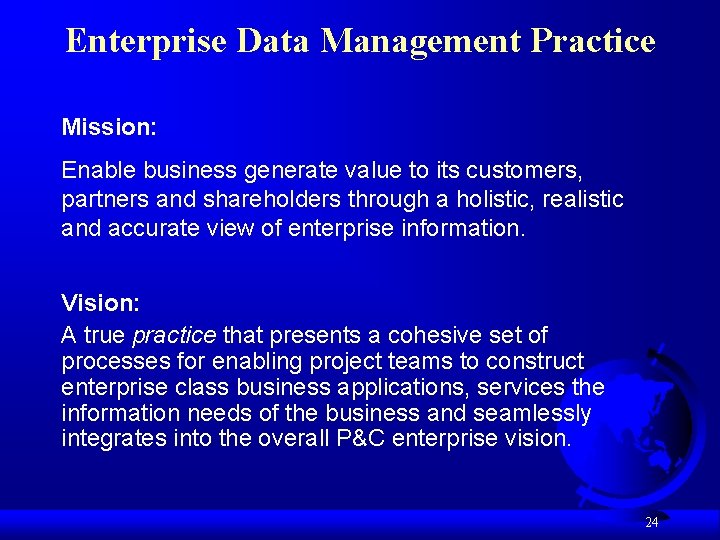
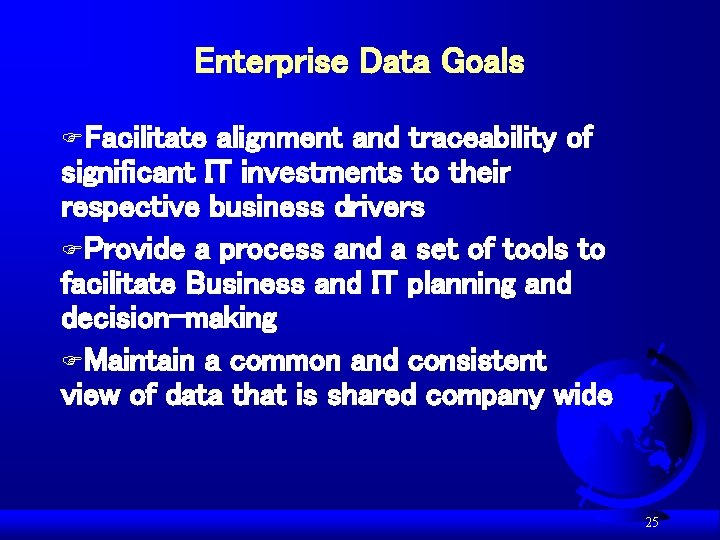
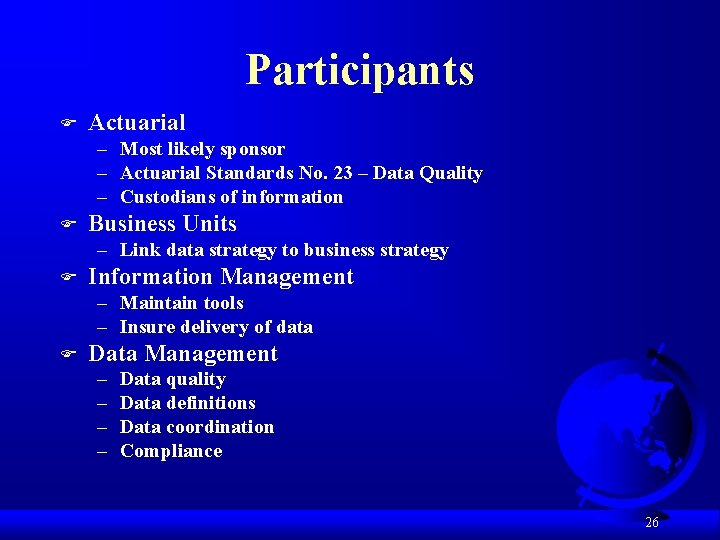
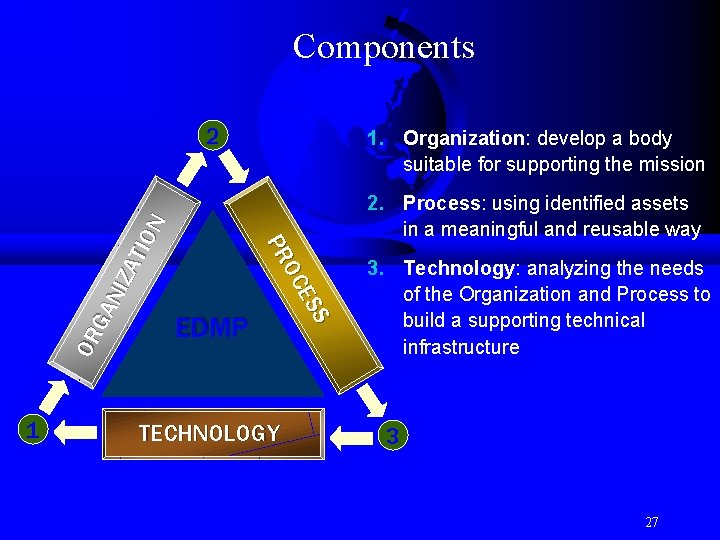
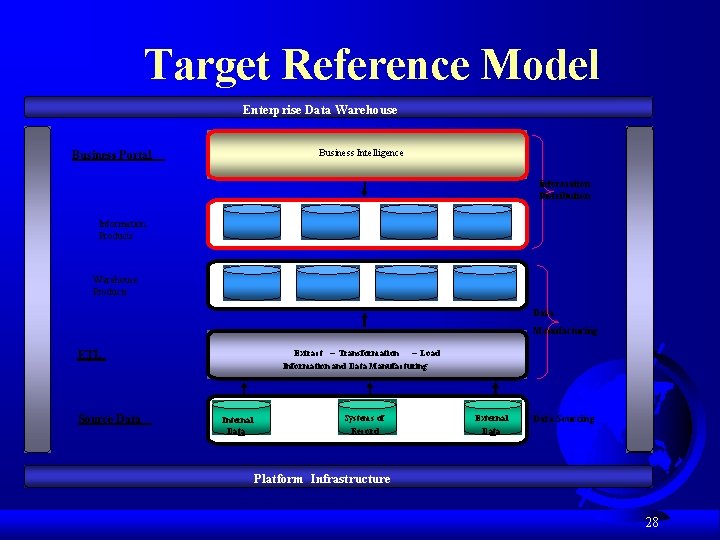
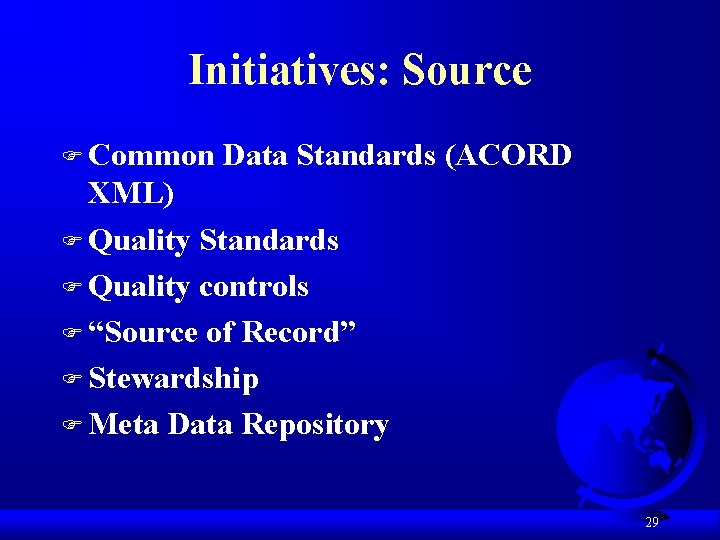
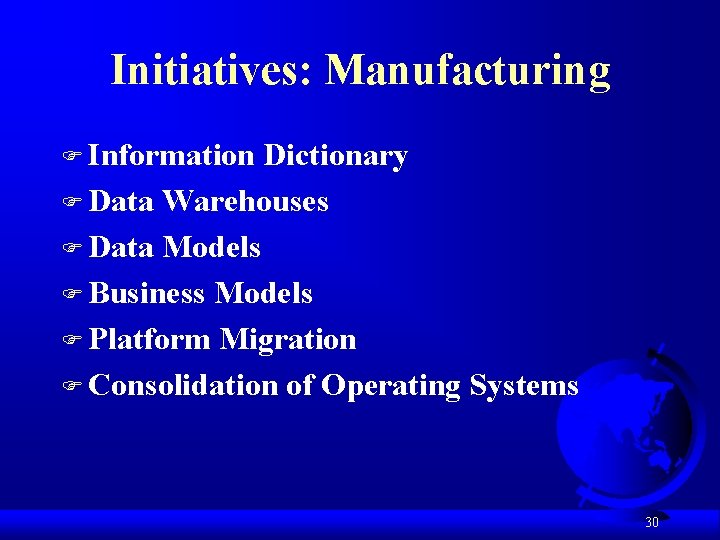
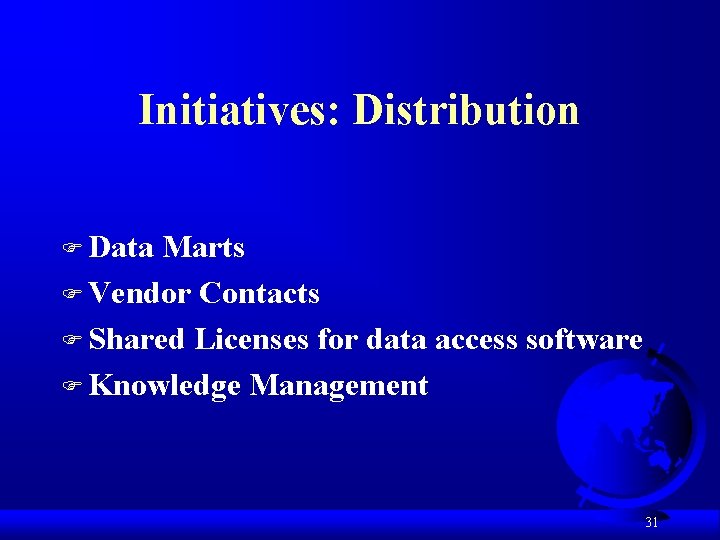
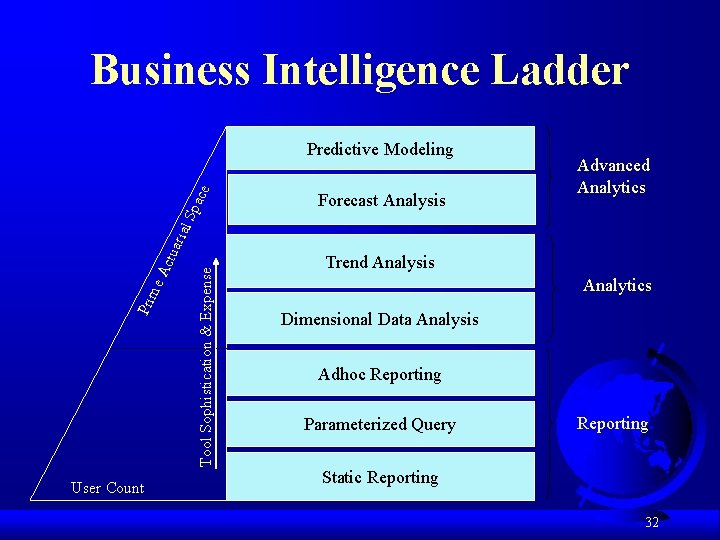
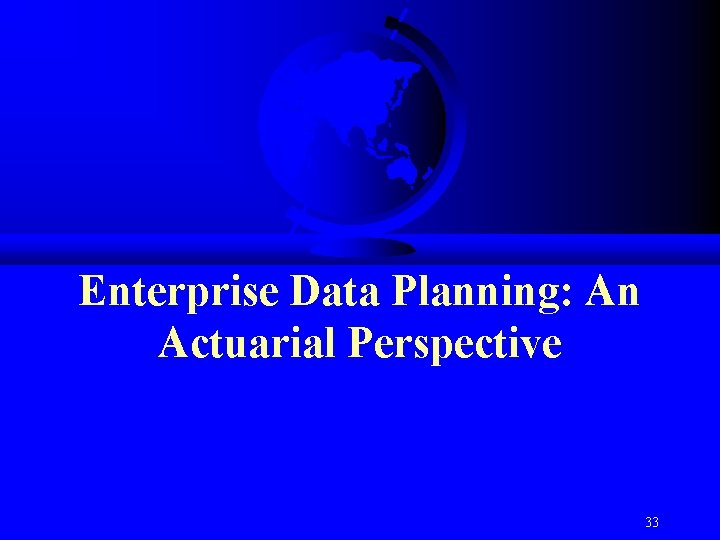
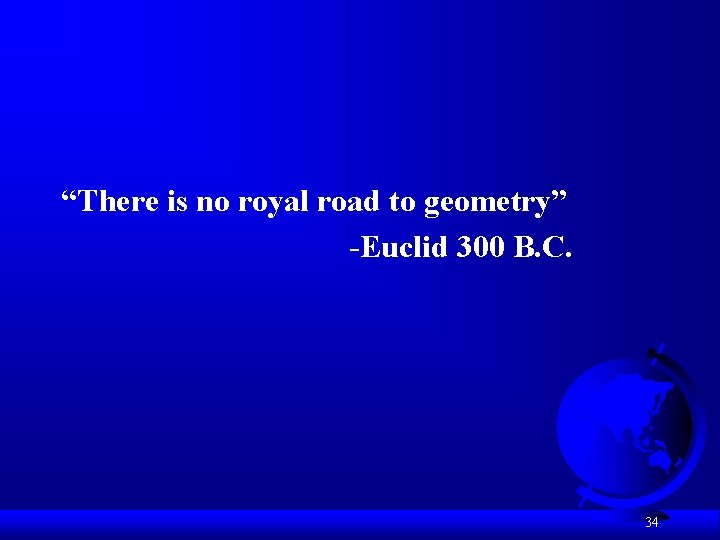
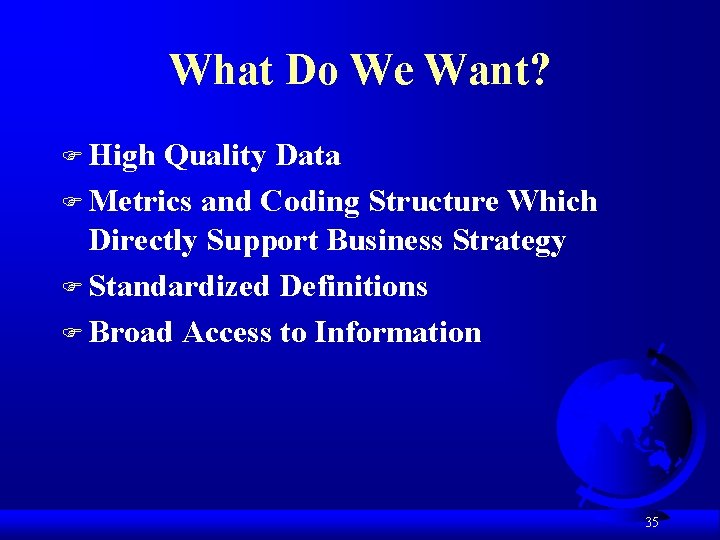
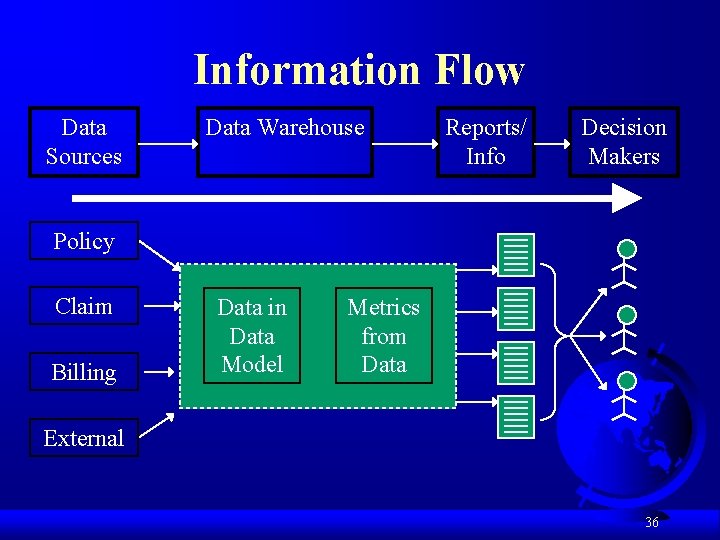
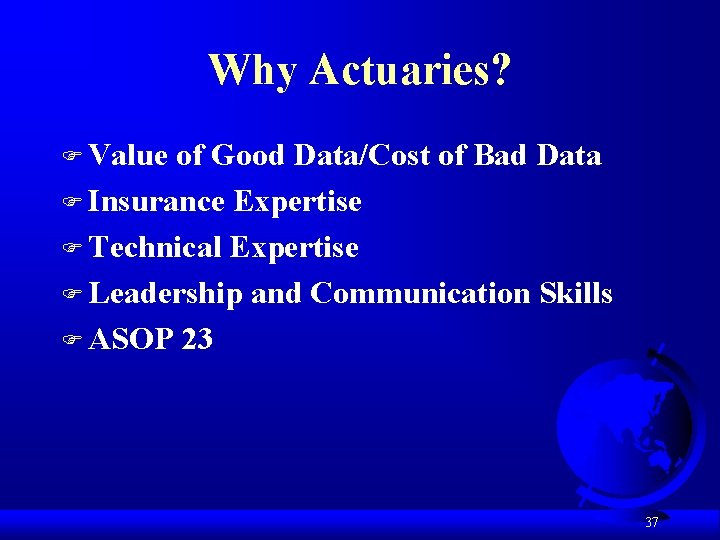
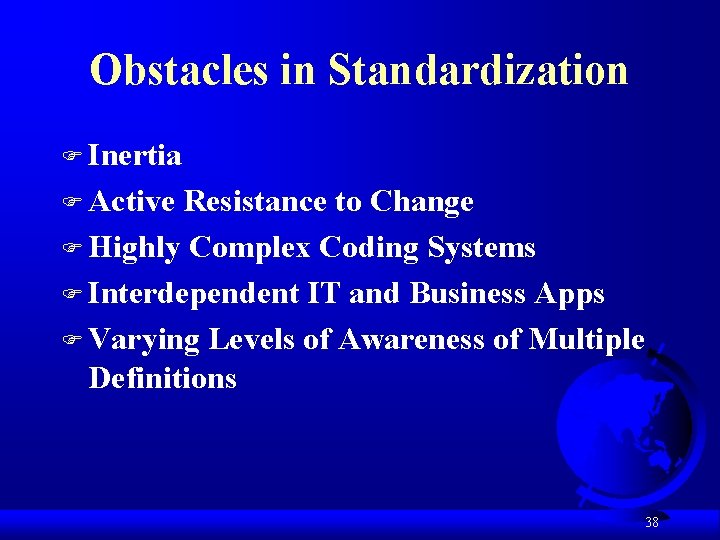
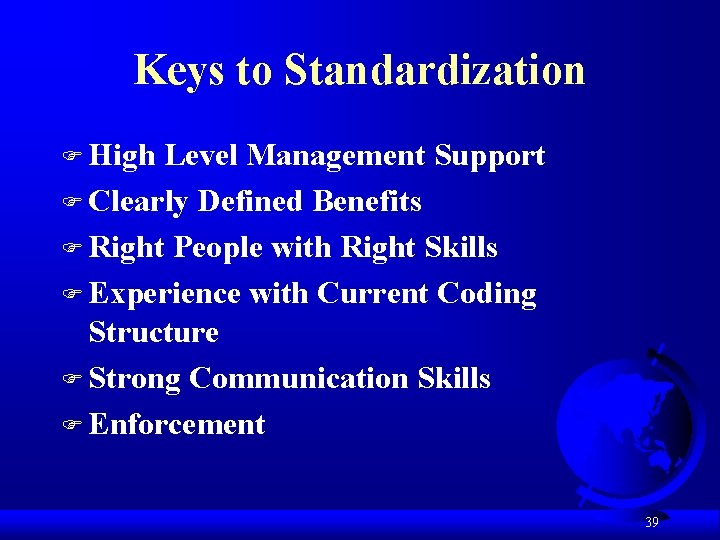
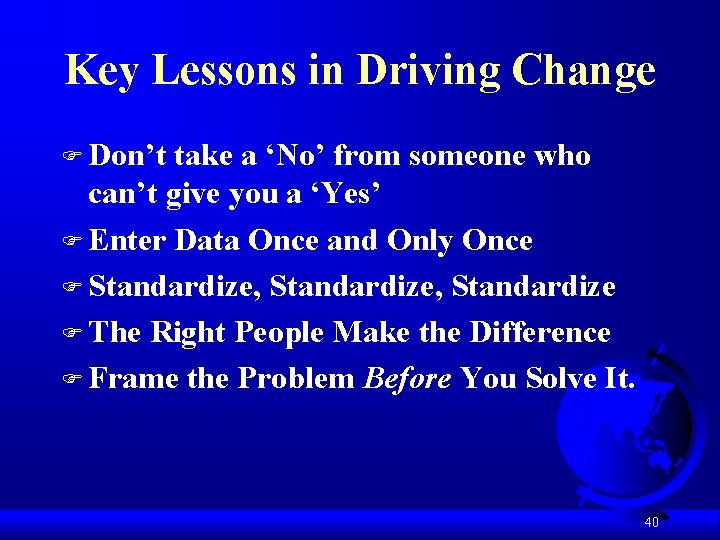
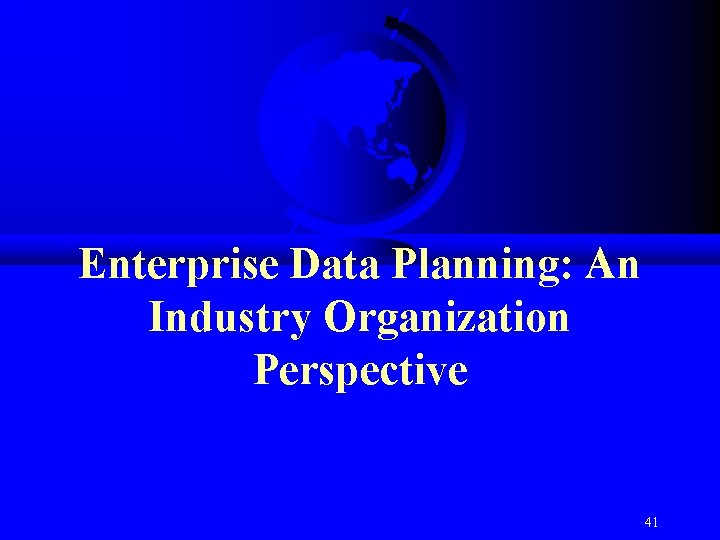
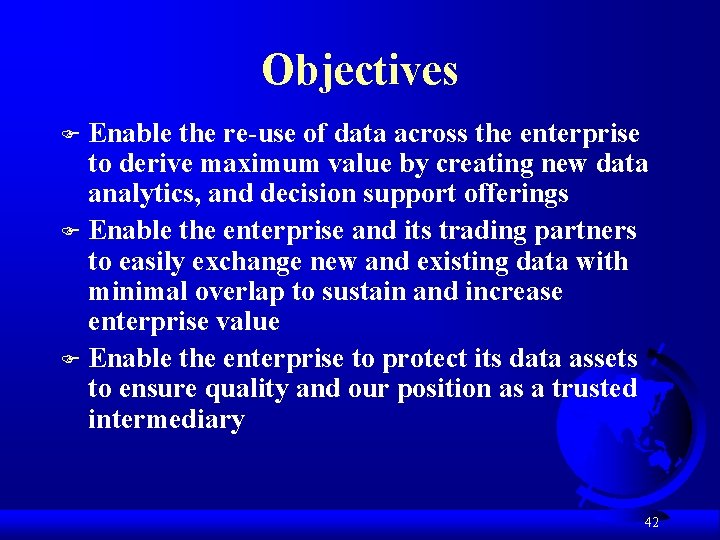
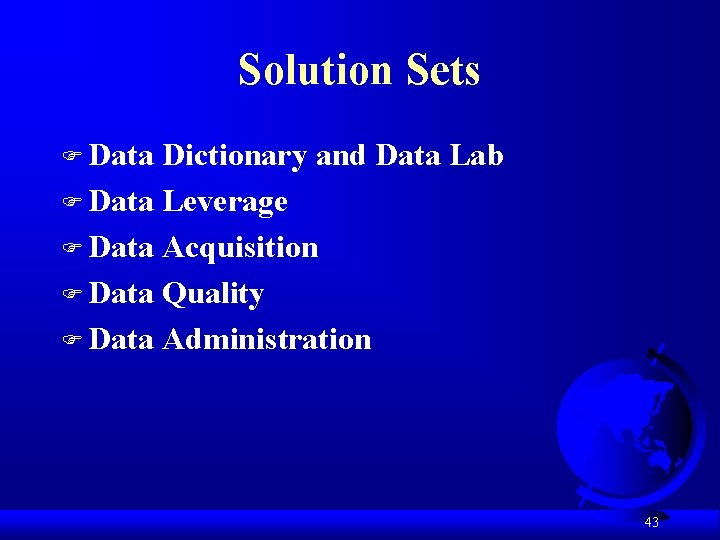
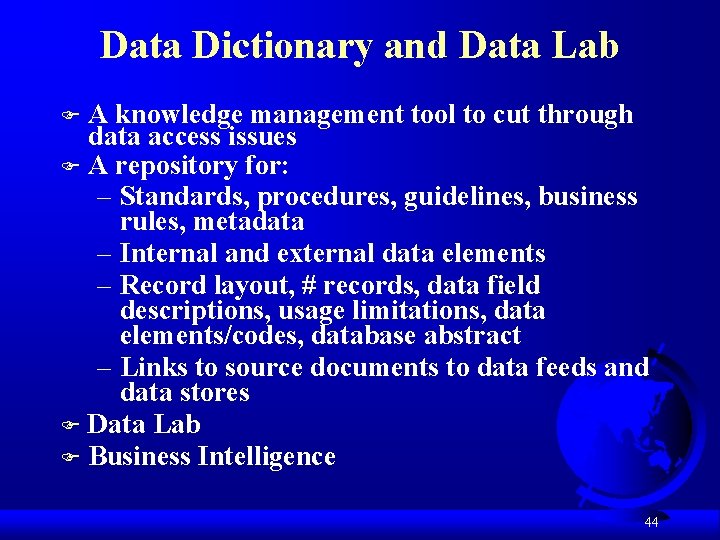
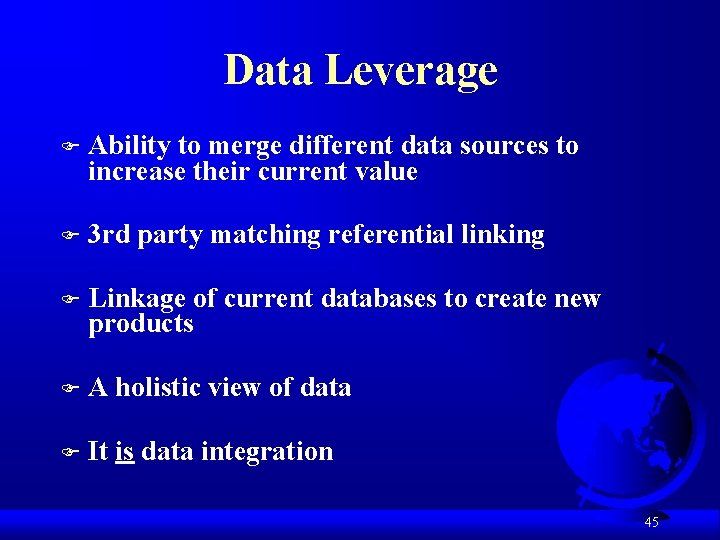
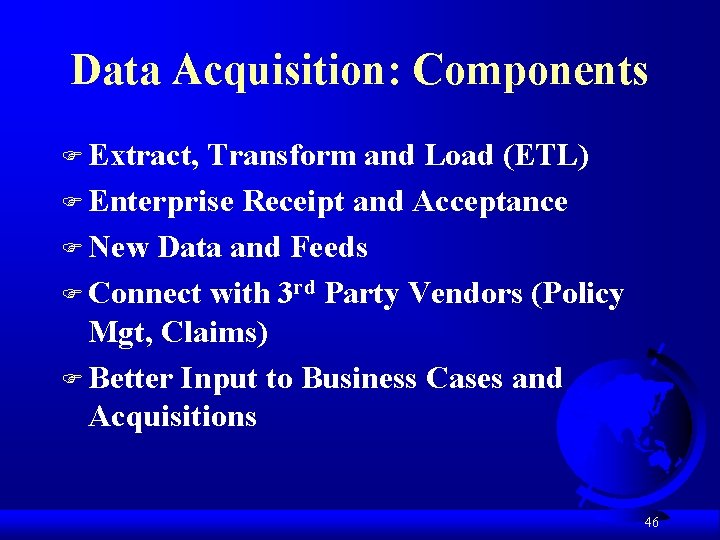
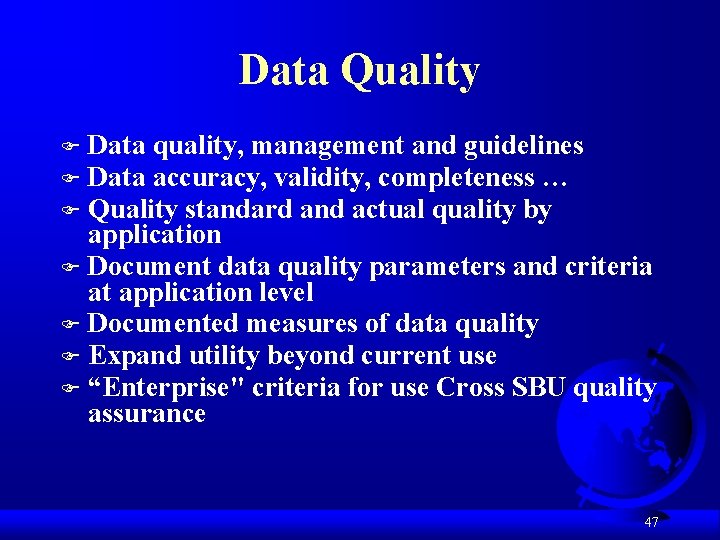
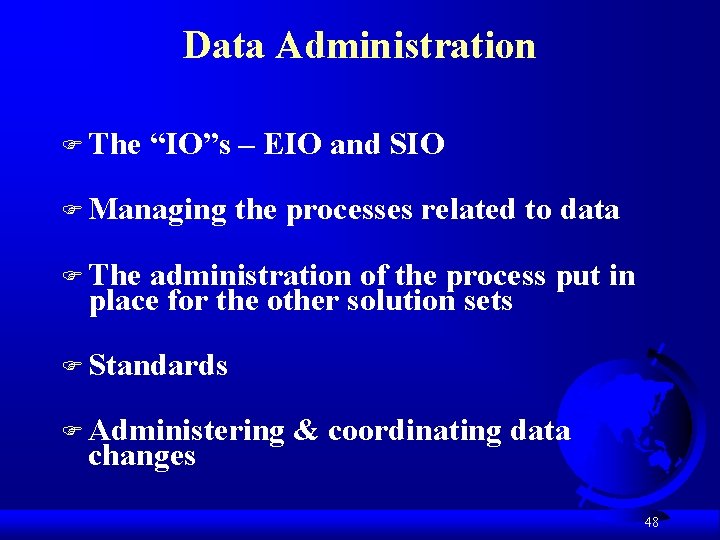
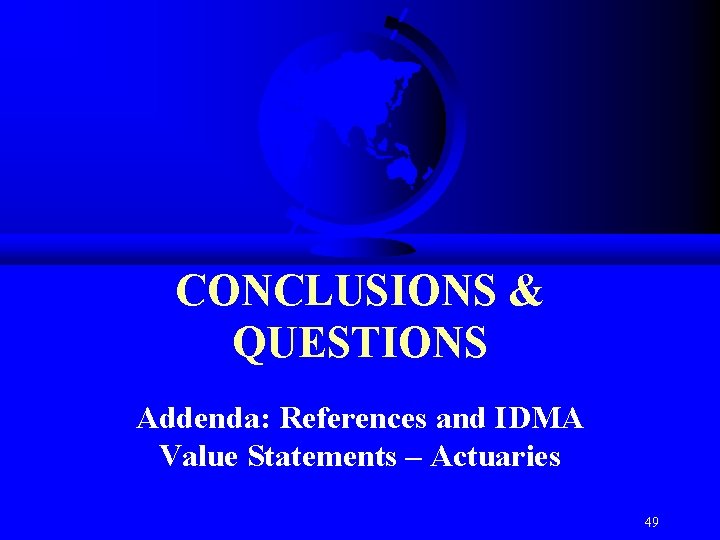
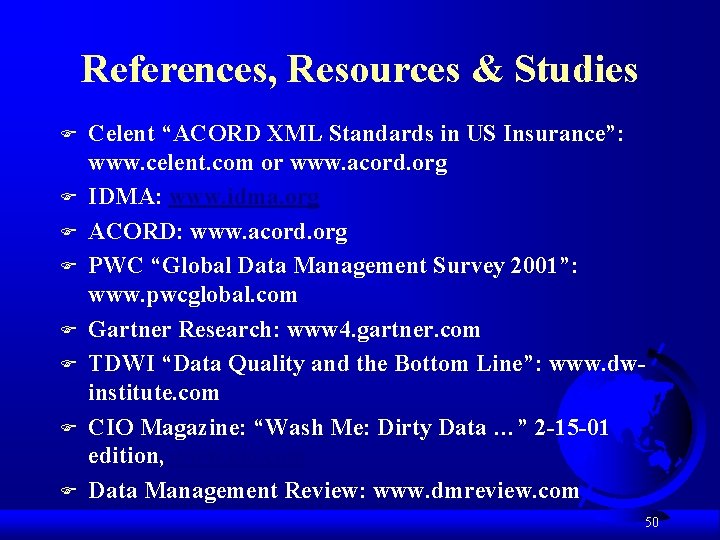
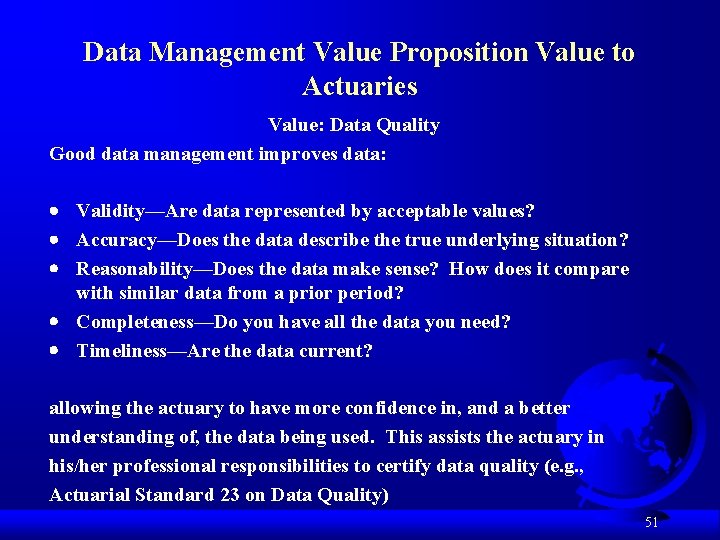
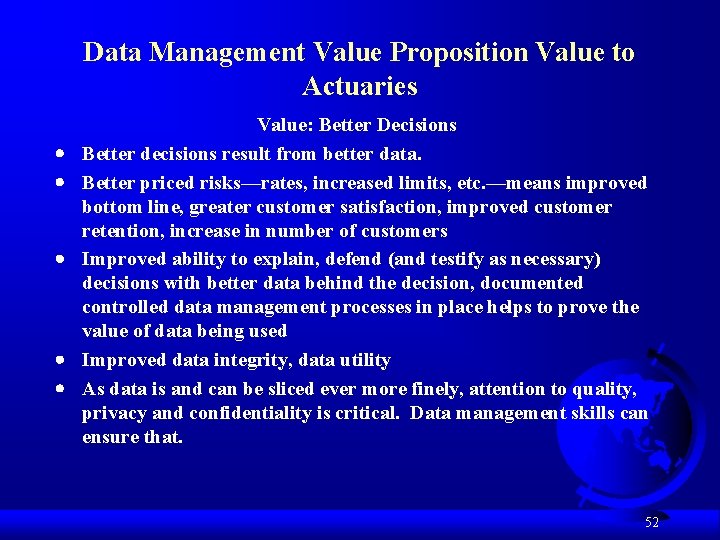
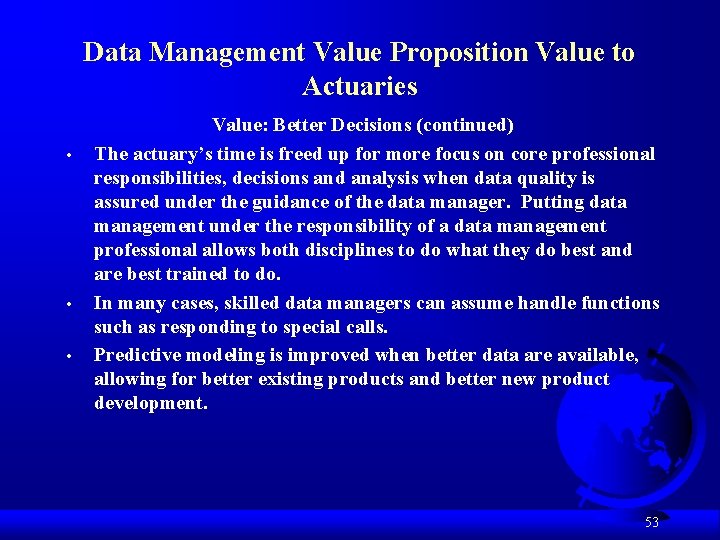
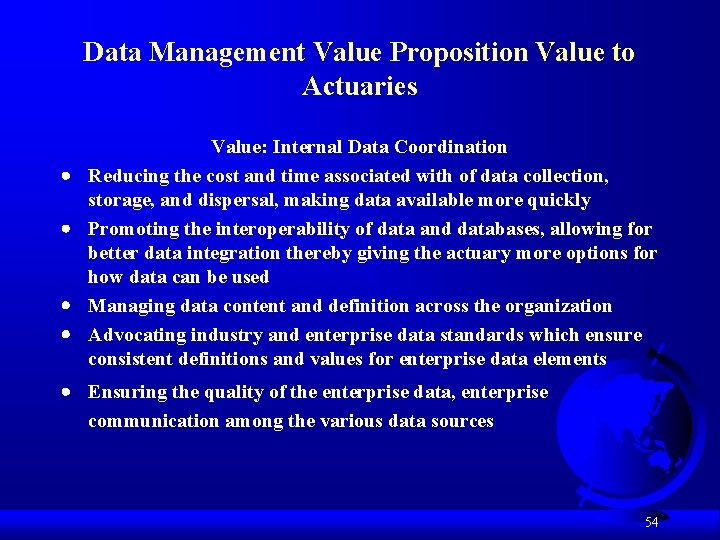
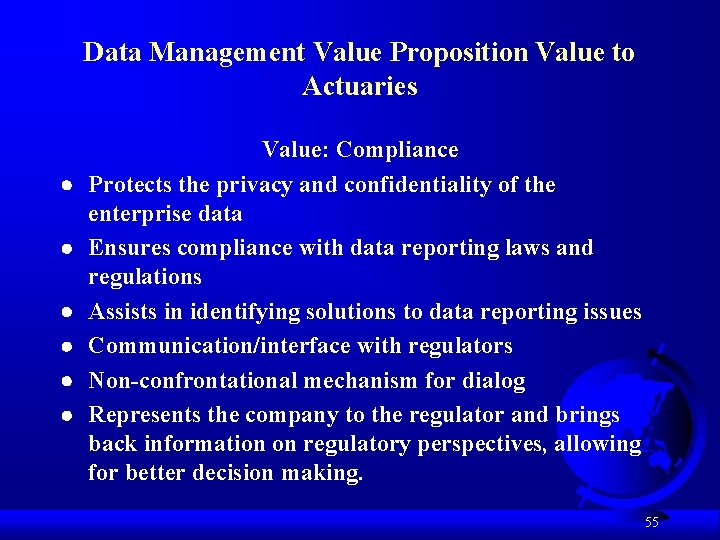
- Slides: 55
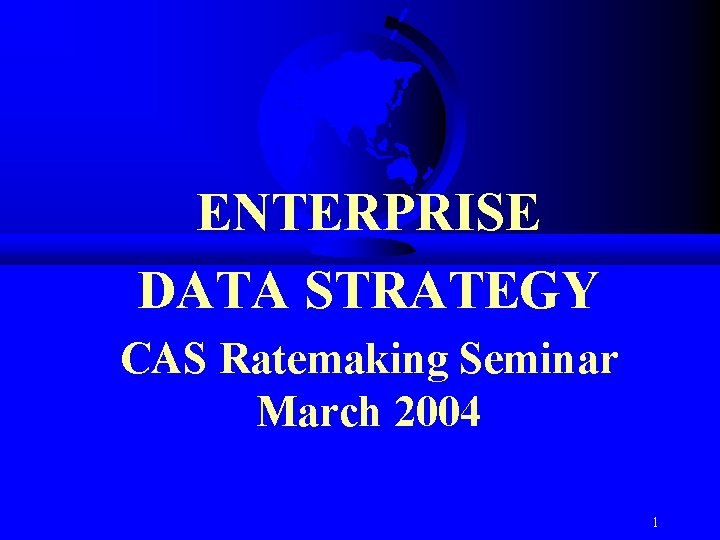
ENTERPRISE DATA STRATEGY CAS Ratemaking Seminar March 2004 1
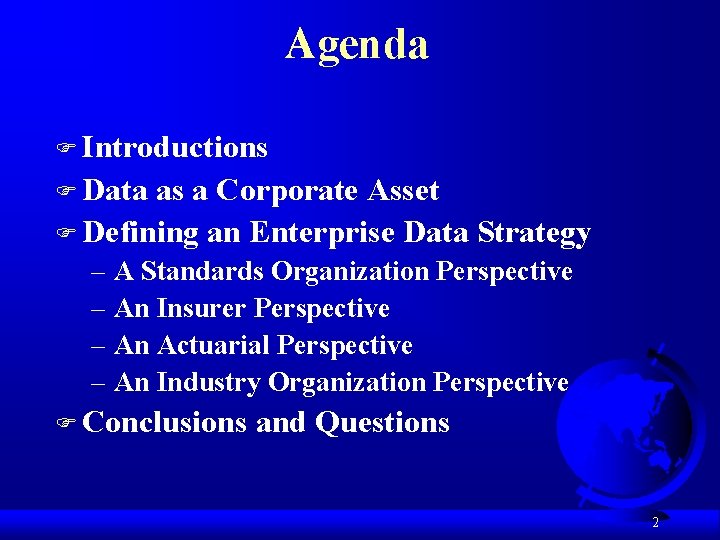
Agenda F Introductions F Data as a Corporate Asset F Defining an Enterprise Data Strategy – A Standards Organization Perspective – An Insurer Perspective – An Actuarial Perspective – An Industry Organization Perspective F Conclusions and Questions 2
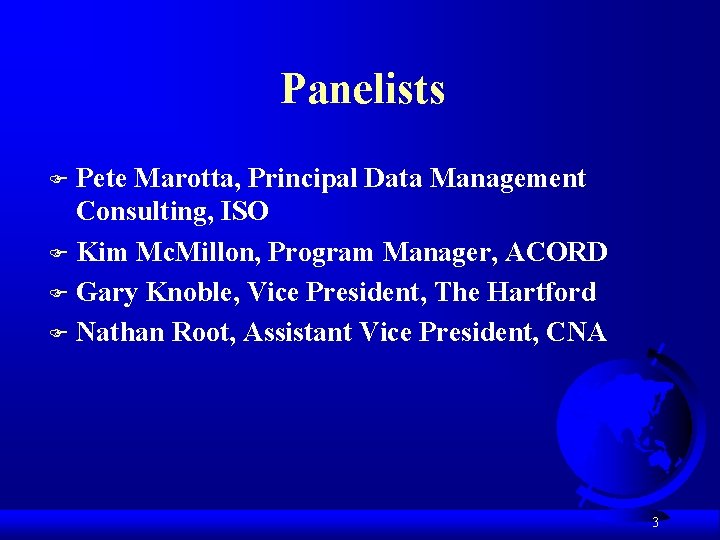
Panelists Pete Marotta, Principal Data Management Consulting, ISO F Kim Mc. Millon, Program Manager, ACORD F Gary Knoble, Vice President, The Hartford F Nathan Root, Assistant Vice President, CNA F 3
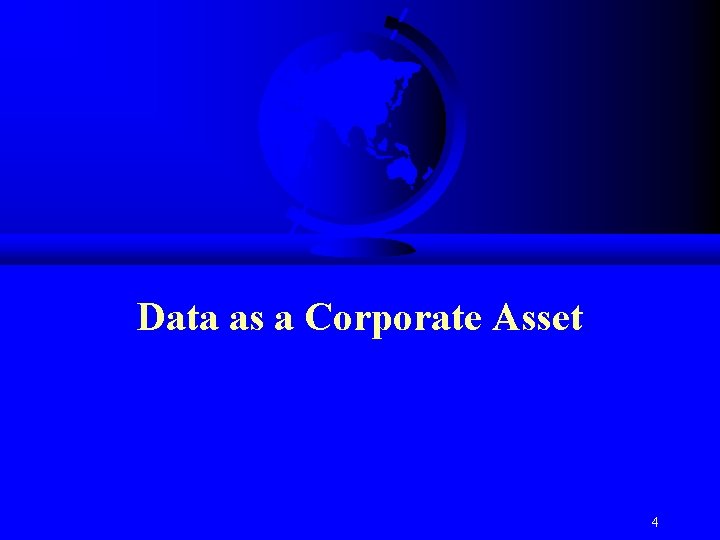
Data as a Corporate Asset 4
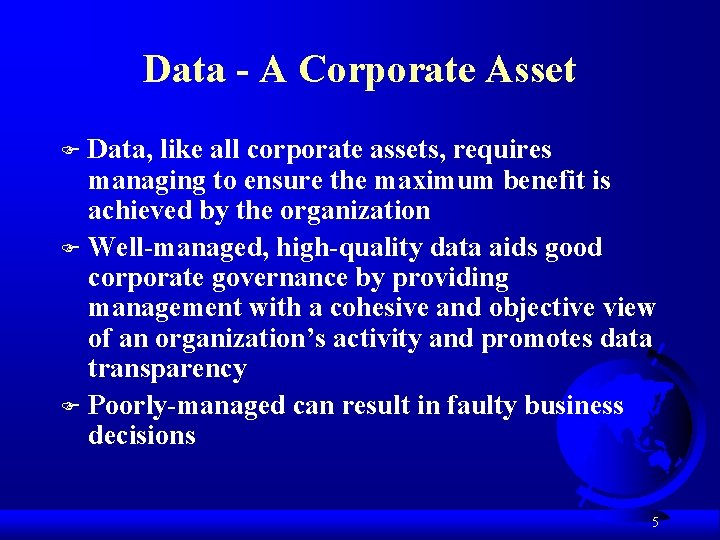
Data - A Corporate Asset Data, like all corporate assets, requires managing to ensure the maximum benefit is achieved by the organization F Well-managed, high-quality data aids good corporate governance by providing management with a cohesive and objective view of an organization’s activity and promotes data transparency F Poorly-managed can result in faulty business decisions F 5
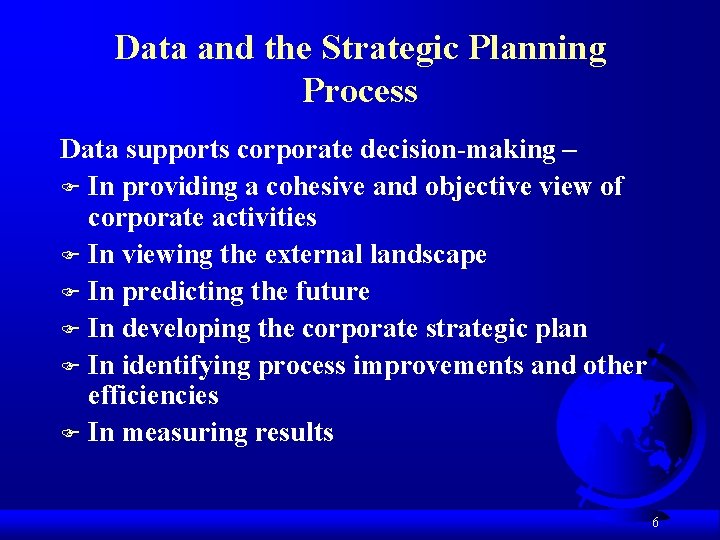
Data and the Strategic Planning Process Data supports corporate decision-making – F In providing a cohesive and objective view of corporate activities F In viewing the external landscape F In predicting the future F In developing the corporate strategic plan F In identifying process improvements and other efficiencies F In measuring results 6
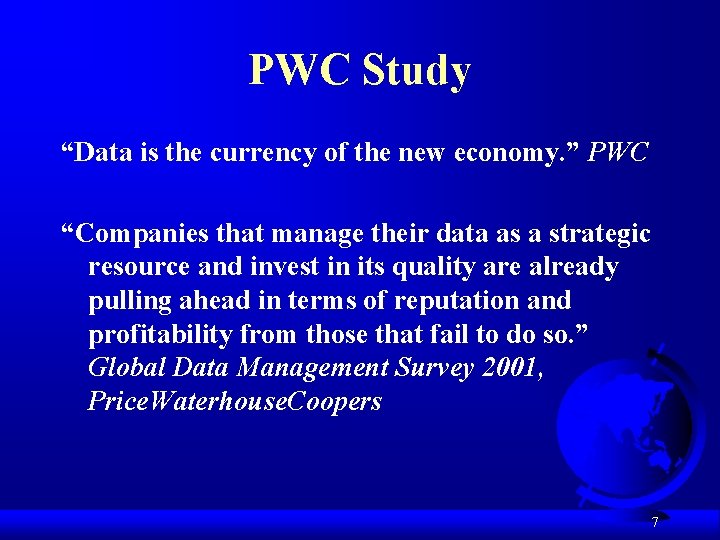
PWC Study “Data is the currency of the new economy. ” PWC “Companies that manage their data as a strategic resource and invest in its quality are already pulling ahead in terms of reputation and profitability from those that fail to do so. ” Global Data Management Survey 2001, Price. Waterhouse. Coopers 7
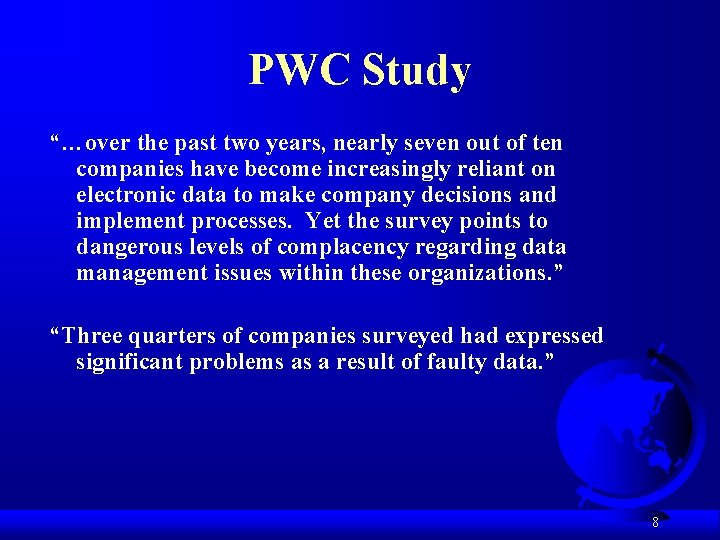
PWC Study “…over the past two years, nearly seven out of ten companies have become increasingly reliant on electronic data to make company decisions and implement processes. Yet the survey points to dangerous levels of complacency regarding data management issues within these organizations. ” “Three quarters of companies surveyed had expressed significant problems as a result of faulty data. ” 8
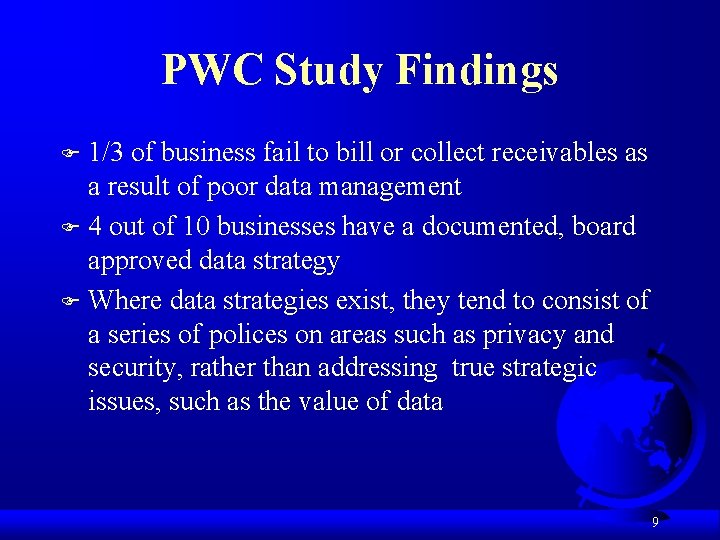
PWC Study Findings 1/3 of business fail to bill or collect receivables as a result of poor data management F 4 out of 10 businesses have a documented, board approved data strategy F Where data strategies exist, they tend to consist of a series of polices on areas such as privacy and security, rather than addressing true strategic issues, such as the value of data F 9
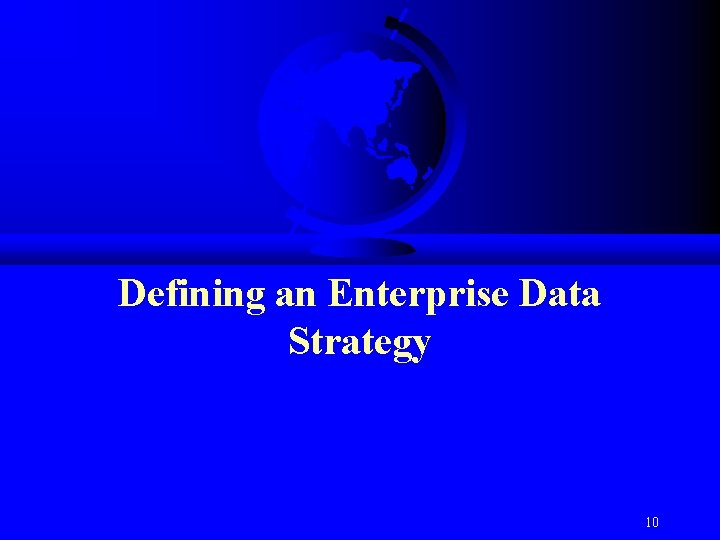
Defining an Enterprise Data Strategy 10
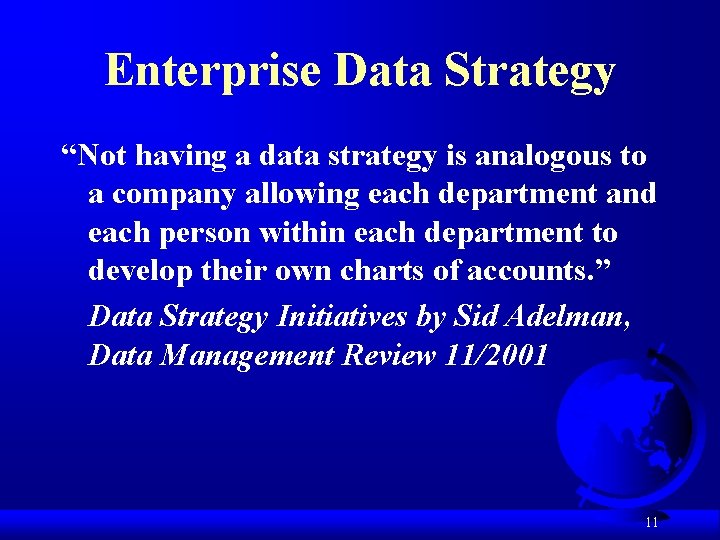
Enterprise Data Strategy “Not having a data strategy is analogous to a company allowing each department and each person within each department to develop their own charts of accounts. ” Data Strategy Initiatives by Sid Adelman, Data Management Review 11/2001 11
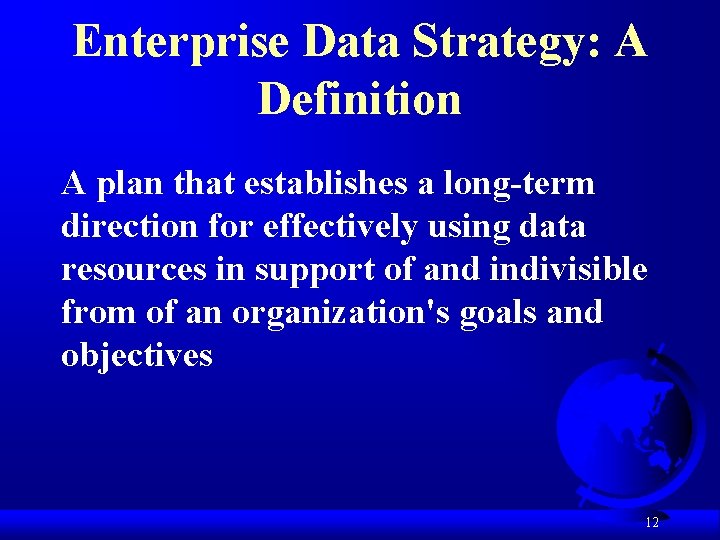
Enterprise Data Strategy: A Definition A plan that establishes a long-term direction for effectively using data resources in support of and indivisible from of an organization's goals and objectives 12
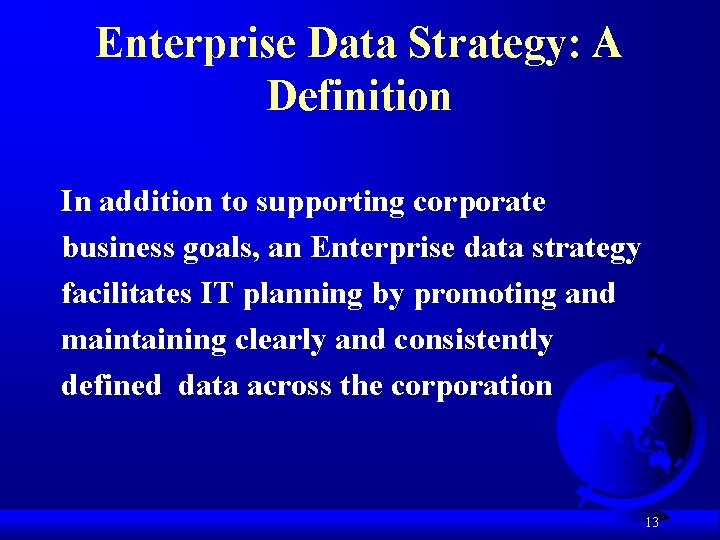
Enterprise Data Strategy: A Definition In addition to supporting corporate business goals, an Enterprise data strategy facilitates IT planning by promoting and maintaining clearly and consistently defined data across the corporation 13
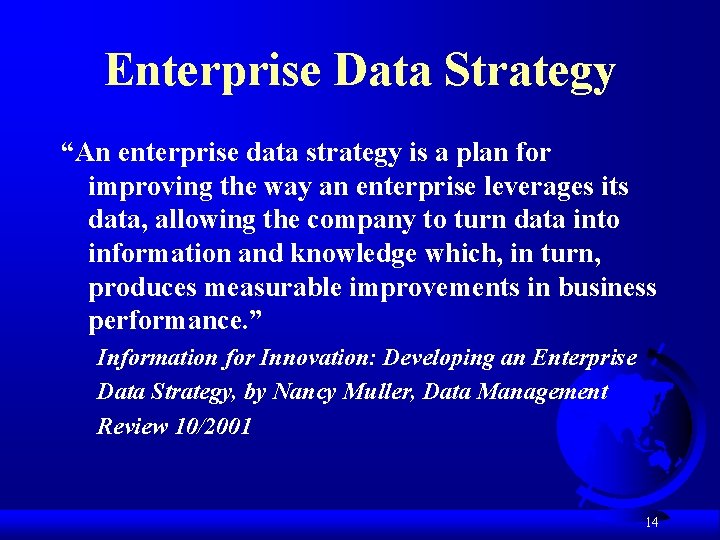
Enterprise Data Strategy “An enterprise data strategy is a plan for improving the way an enterprise leverages its data, allowing the company to turn data into information and knowledge which, in turn, produces measurable improvements in business performance. ” Information for Innovation: Developing an Enterprise Data Strategy, by Nancy Muller, Data Management Review 10/2001 14
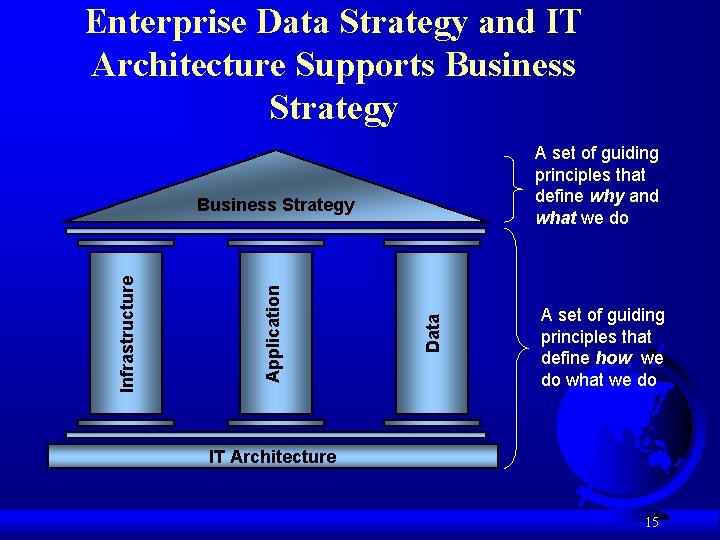
Enterprise Data Strategy and IT Architecture Supports Business Strategy A set of guiding principles that define why and what we do Data Application Infrastructure Business Strategy A set of guiding principles that define how we do what we do IT Architecture 15
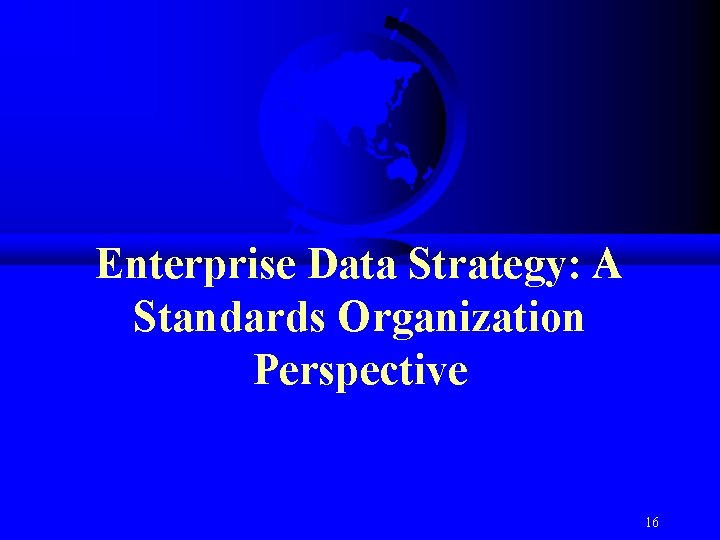
Enterprise Data Strategy: A Standards Organization Perspective 16
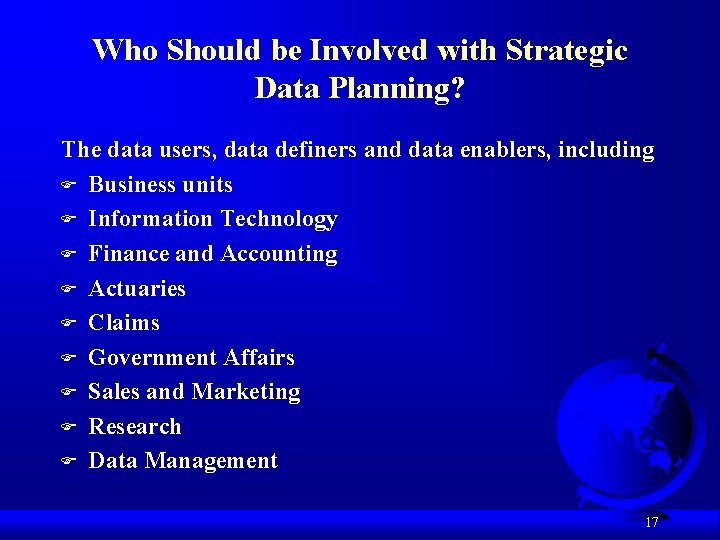
Who Should be Involved with Strategic Data Planning? The data users, data definers and data enablers, including F Business units F Information Technology F Finance and Accounting F Actuaries F Claims F Government Affairs F Sales and Marketing F Research F Data Management 17
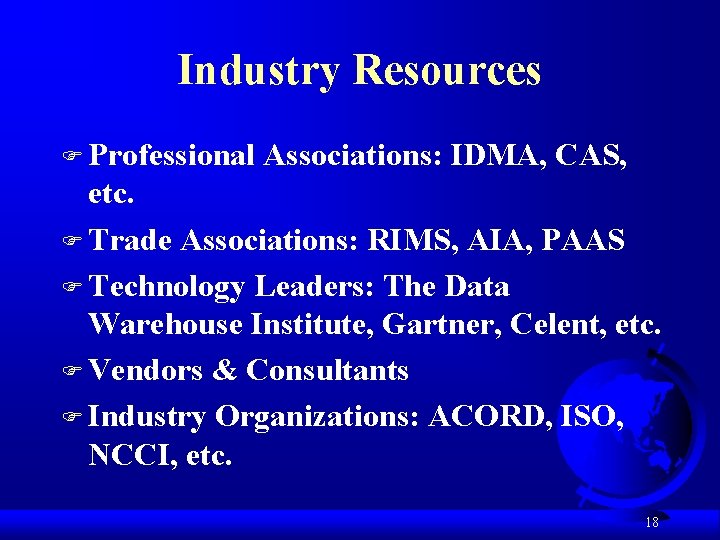
Industry Resources F Professional Associations: IDMA, CAS, etc. F Trade Associations: RIMS, AIA, PAAS F Technology Leaders: The Data Warehouse Institute, Gartner, Celent, etc. F Vendors & Consultants F Industry Organizations: ACORD, ISO, NCCI, etc. 18
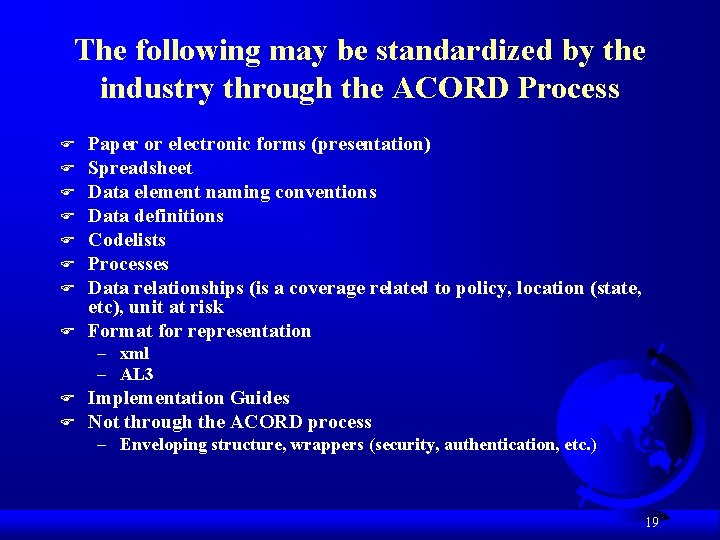
The following may be standardized by the industry through the ACORD Process F F F F Paper or electronic forms (presentation) Spreadsheet Data element naming conventions Data definitions Codelists Processes Data relationships (is a coverage related to policy, location (state, etc), unit at risk Format for representation – xml – AL 3 F F Implementation Guides Not through the ACORD process – Enveloping structure, wrappers (security, authentication, etc. ) 19
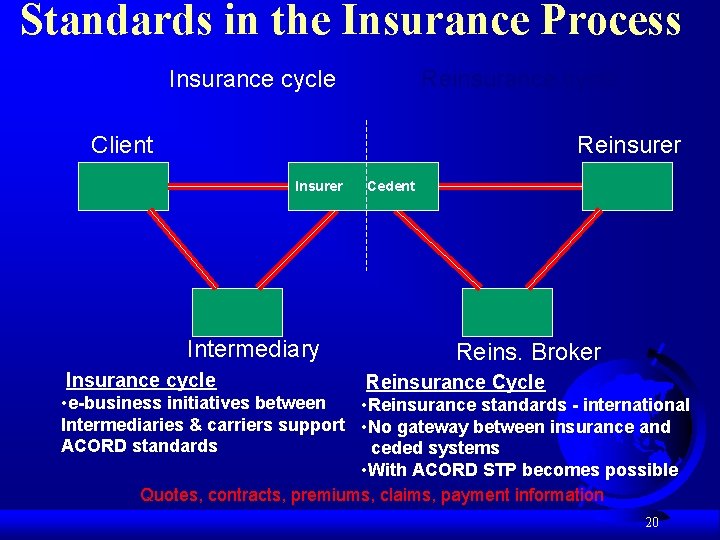
Standards in the Insurance Process Insurance cycle Reinsurance cycle Client Reinsurer Intermediary Insurance cycle Cedent Reins. Broker Reinsurance Cycle • e-business initiatives between • Reinsurance standards - international Intermediaries & carriers support • No gateway between insurance and ACORD standards ceded systems • With ACORD STP becomes possible Quotes, contracts, premiums, claims, payment information 20
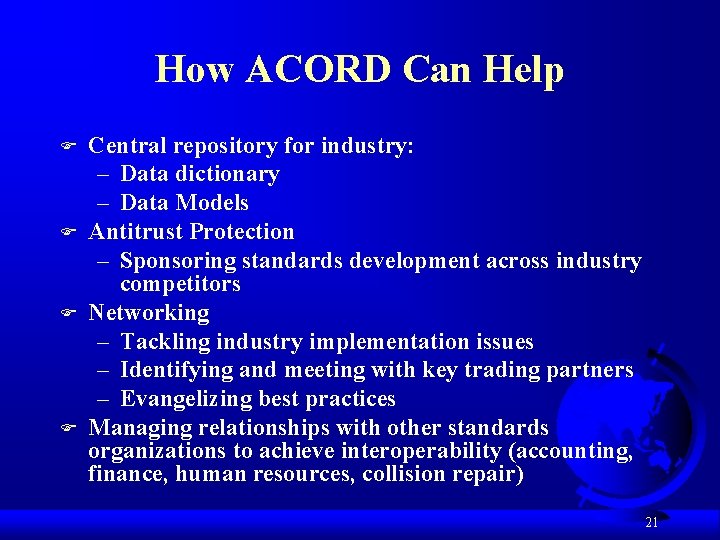
How ACORD Can Help F F Central repository for industry: – Data dictionary – Data Models Antitrust Protection – Sponsoring standards development across industry competitors Networking – Tackling industry implementation issues – Identifying and meeting with key trading partners – Evangelizing best practices Managing relationships with other standards organizations to achieve interoperability (accounting, finance, human resources, collision repair) 21
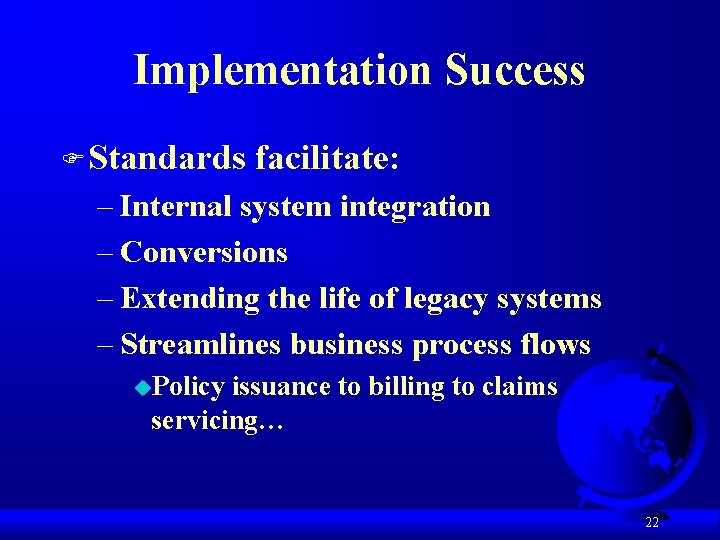
Implementation Success F Standards facilitate: – Internal system integration – Conversions – Extending the life of legacy systems – Streamlines business process flows u. Policy issuance to billing to claims servicing… 22
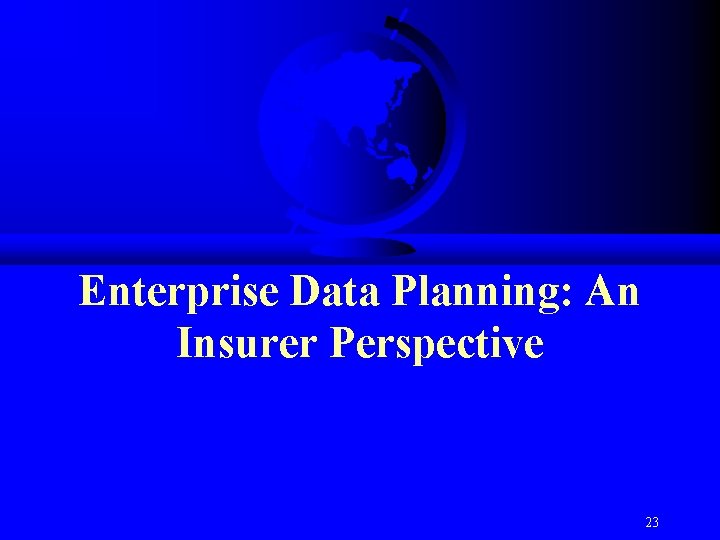
Enterprise Data Planning: An Insurer Perspective 23
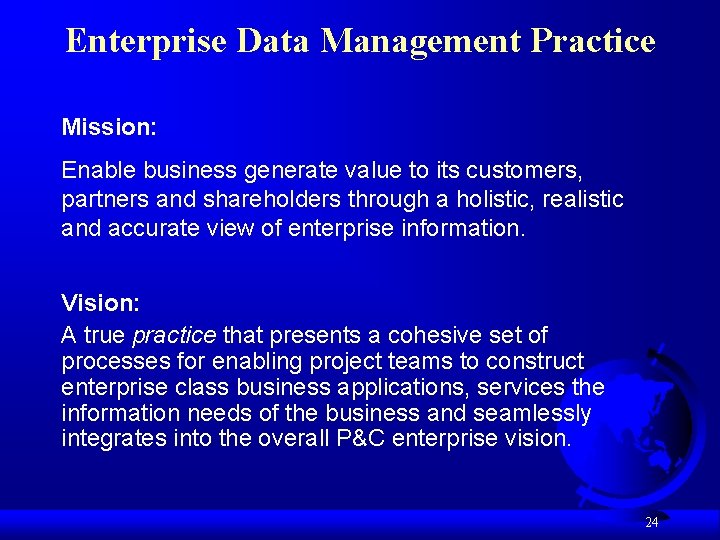
Enterprise Data Management Practice Mission: Enable business generate value to its customers, partners and shareholders through a holistic, realistic and accurate view of enterprise information. Vision: A true practice that presents a cohesive set of processes for enabling project teams to construct enterprise class business applications, services the information needs of the business and seamlessly integrates into the overall P&C enterprise vision. 24
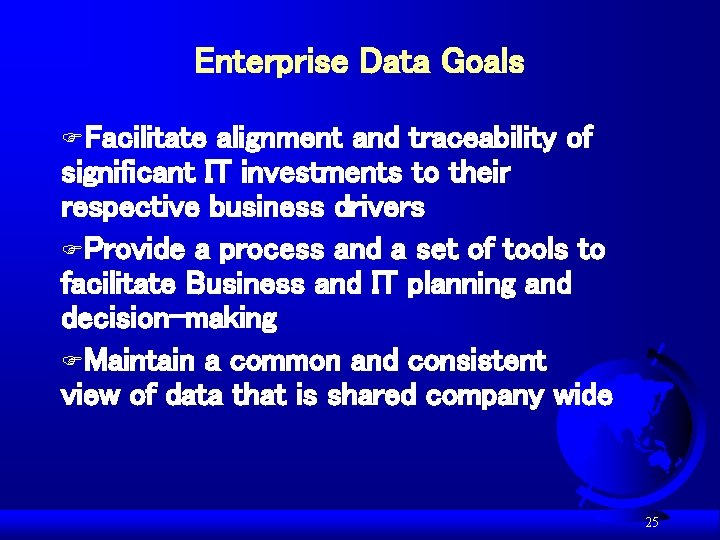
Enterprise Data Goals FFacilitate alignment and traceability of significant IT investments to their respective business drivers FProvide a process and a set of tools to facilitate Business and IT planning and decision-making FMaintain a common and consistent view of data that is shared company wide 25
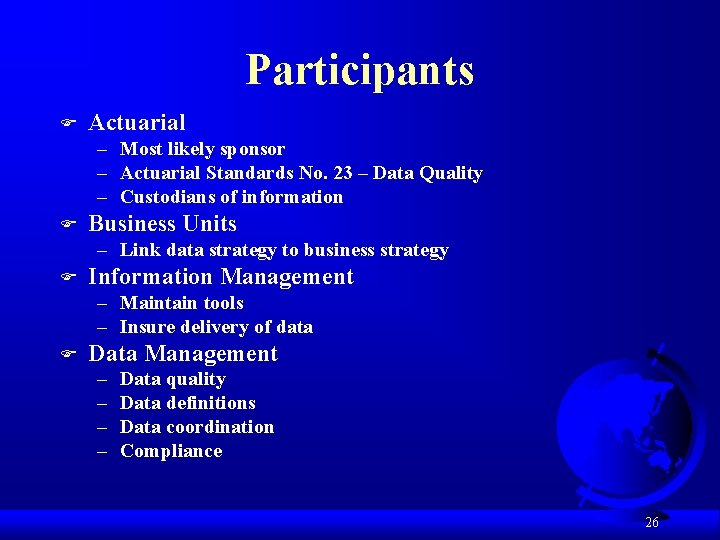
Participants F Actuarial – Most likely sponsor – Actuarial Standards No. 23 – Data Quality – Custodians of information F Business Units – Link data strategy to business strategy F Information Management – Maintain tools – Insure delivery of data F Data Management – – Data quality Data definitions Data coordination Compliance 26
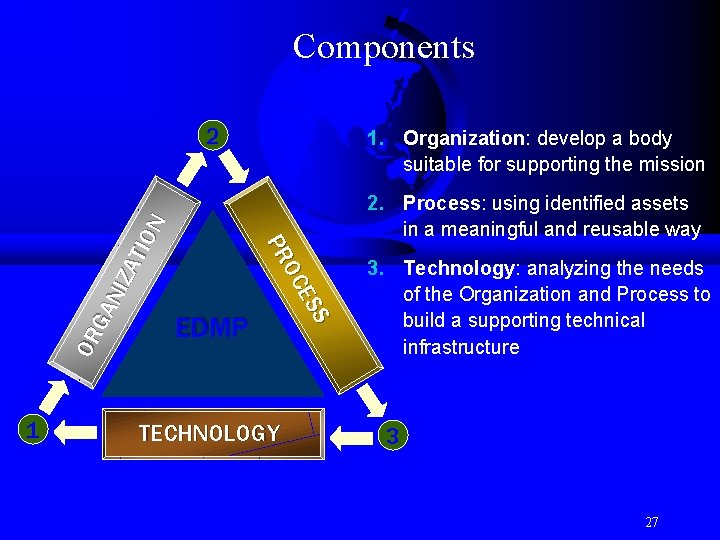
Components 1 1. Organization: develop a body suitable for supporting the mission 2. Process: using identified assets in a meaningful and reusable way EDMP S ES OC PR OR GA NIZ AT ION 2 TECHNOLOGY 3. Technology: analyzing the needs of the Organization and Process to build a supporting technical infrastructure 3 27
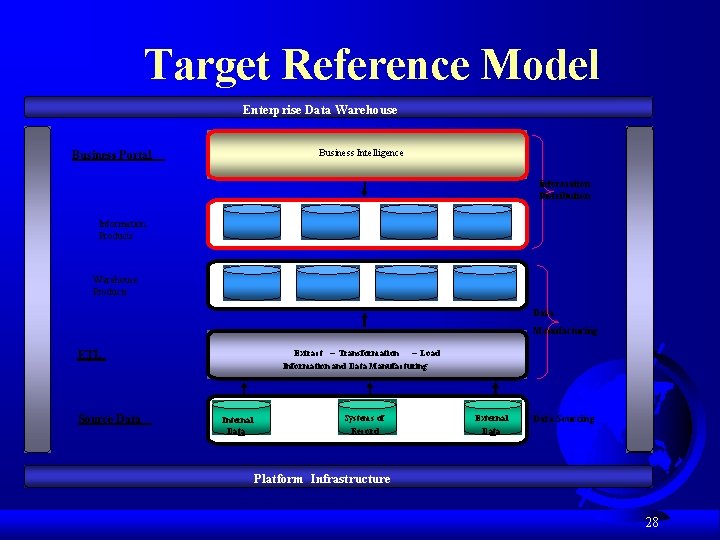
Target Reference Model Enterprise Data Warehouse Business Intelligence Business Portal Information Distribution Information Products Warehouse Products Data Manufacturing ETL Source Data Extract – Transformation – Load Information and Data Manufacturing Internal Data Systems of Record External Data Sourcing Platform Infrastructure 28
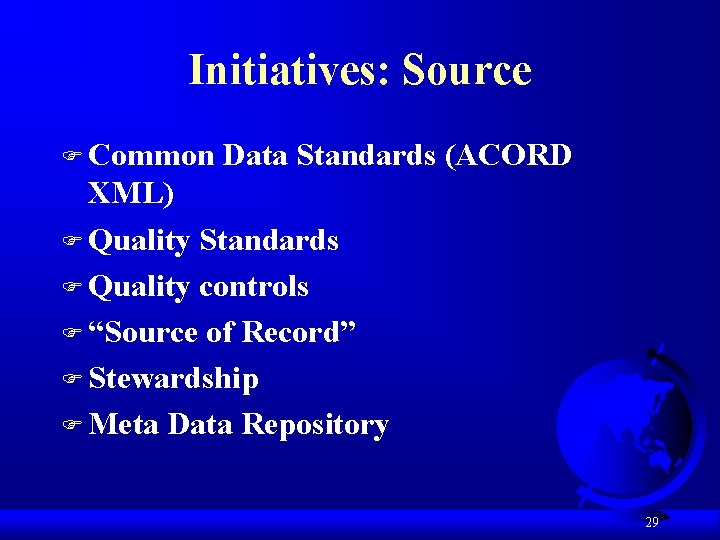
Initiatives: Source F Common Data Standards (ACORD XML) F Quality Standards F Quality controls F “Source of Record” F Stewardship F Meta Data Repository 29
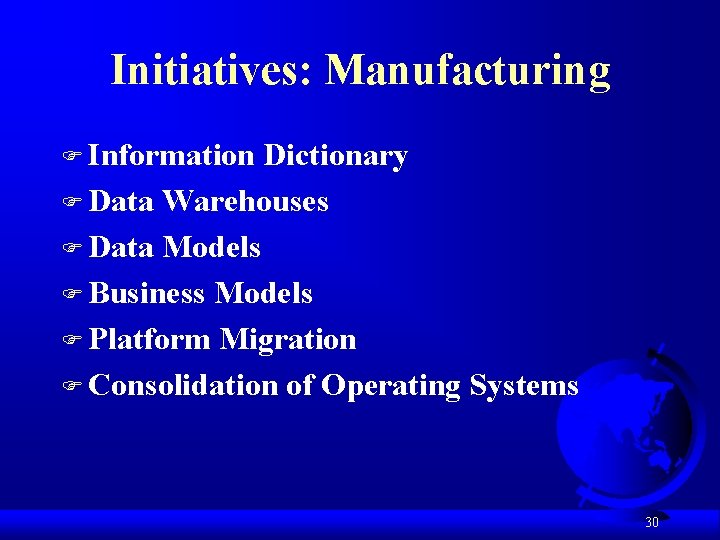
Initiatives: Manufacturing F Information Dictionary F Data Warehouses F Data Models F Business Models F Platform Migration F Consolidation of Operating Systems 30
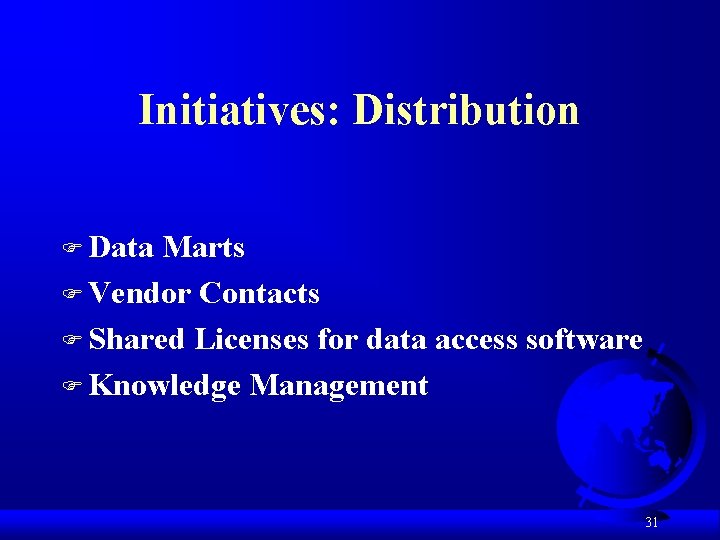
Initiatives: Distribution F Data Marts F Vendor Contacts F Shared Licenses for data access software F Knowledge Management 31
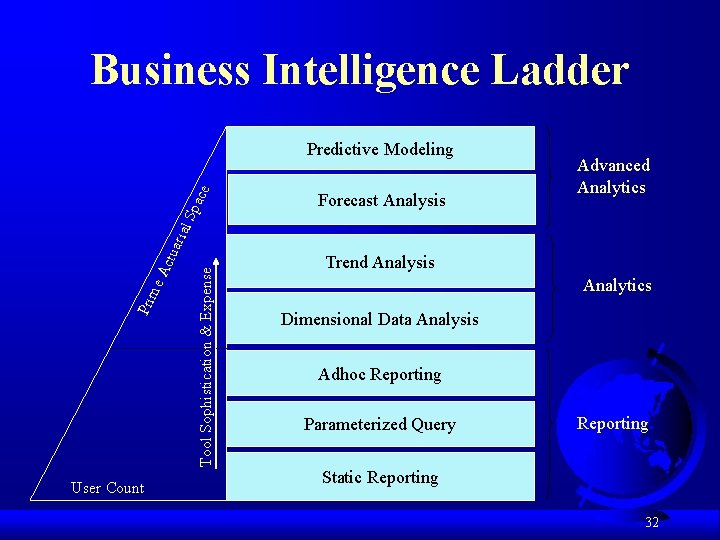
Business Intelligence Ladder Forecast Analysis Advanced Analytics User Count Tool Sophistication & Expense Pri me Ac tu aria l Spa ce Predictive Modeling <GK to add> Trend Analysis Analytics Dimensional Data Analysis Adhoc Reporting Parameterized Query Reporting Static Reporting 32
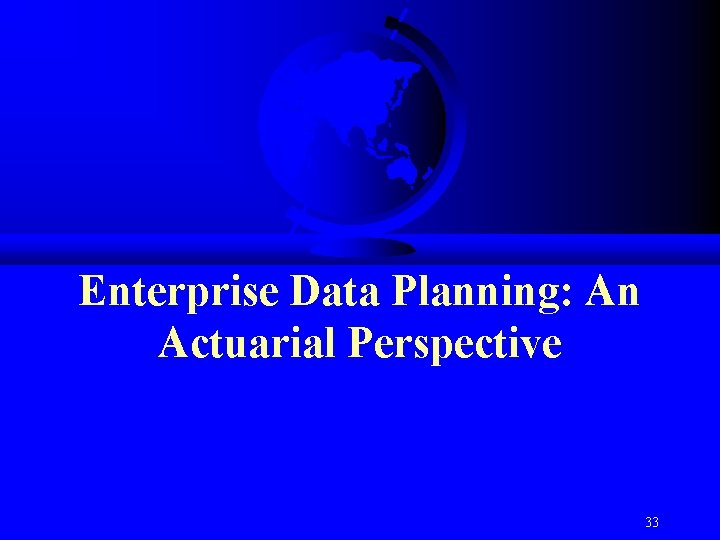
Enterprise Data Planning: An Actuarial Perspective 33
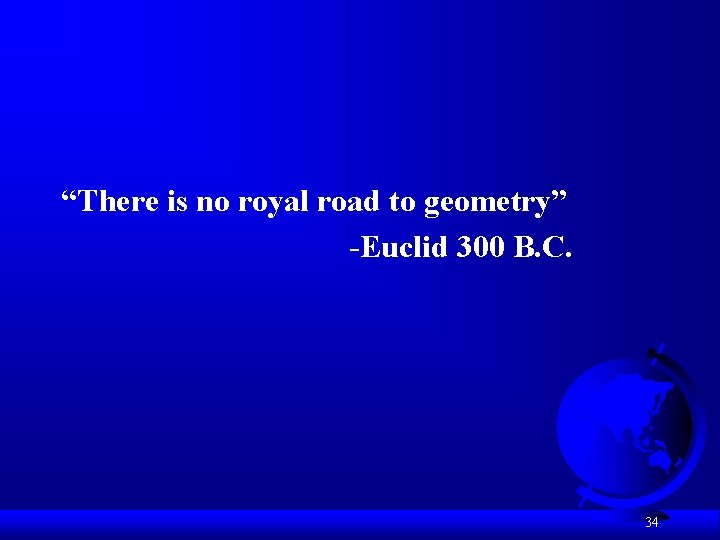
“There is no royal road to geometry” -Euclid 300 B. C. 34
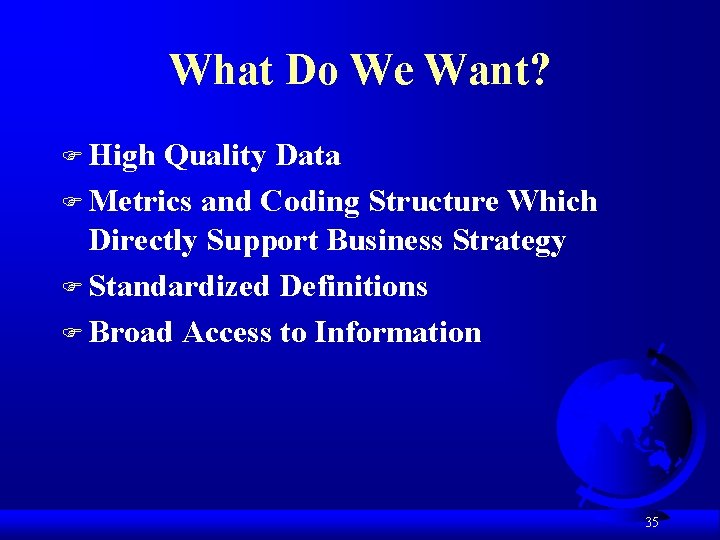
What Do We Want? F High Quality Data F Metrics and Coding Structure Which Directly Support Business Strategy F Standardized Definitions F Broad Access to Information 35
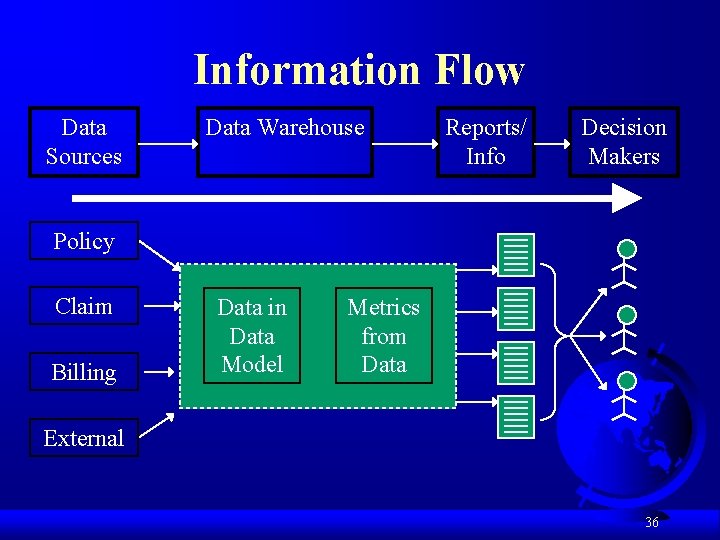
Information Flow Data Sources Data Warehouse Reports/ Info Decision Makers Policy Claim Billing Data in Data Model Metrics from Data External 36
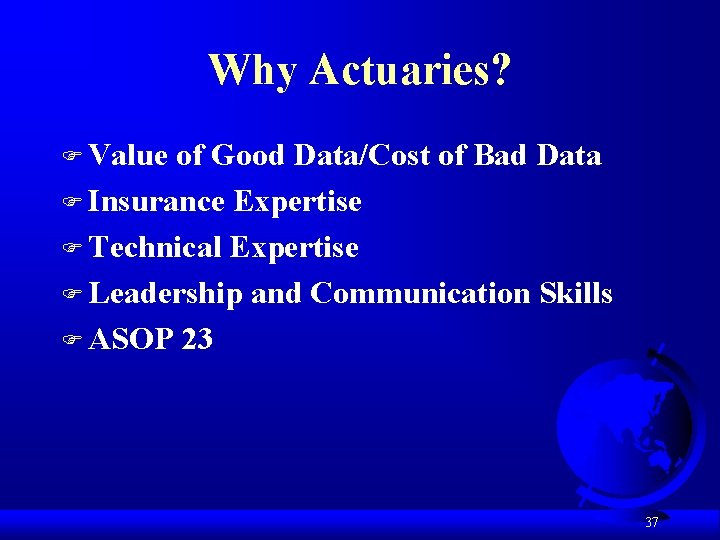
Why Actuaries? F Value of Good Data/Cost of Bad Data F Insurance Expertise F Technical Expertise F Leadership and Communication Skills F ASOP 23 37
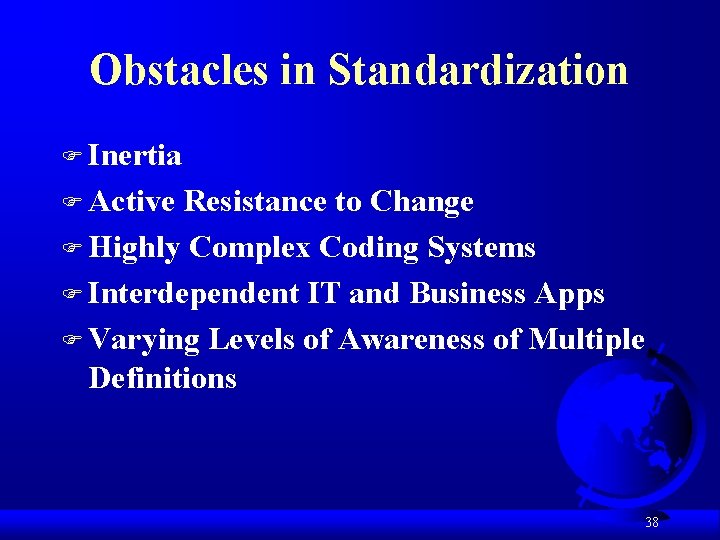
Obstacles in Standardization F Inertia F Active Resistance to Change F Highly Complex Coding Systems F Interdependent IT and Business Apps F Varying Levels of Awareness of Multiple Definitions 38
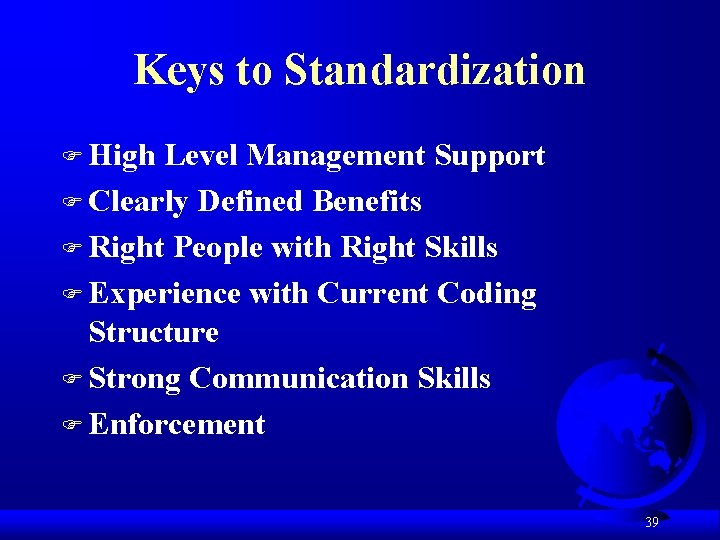
Keys to Standardization F High Level Management Support F Clearly Defined Benefits F Right People with Right Skills F Experience with Current Coding Structure F Strong Communication Skills F Enforcement 39
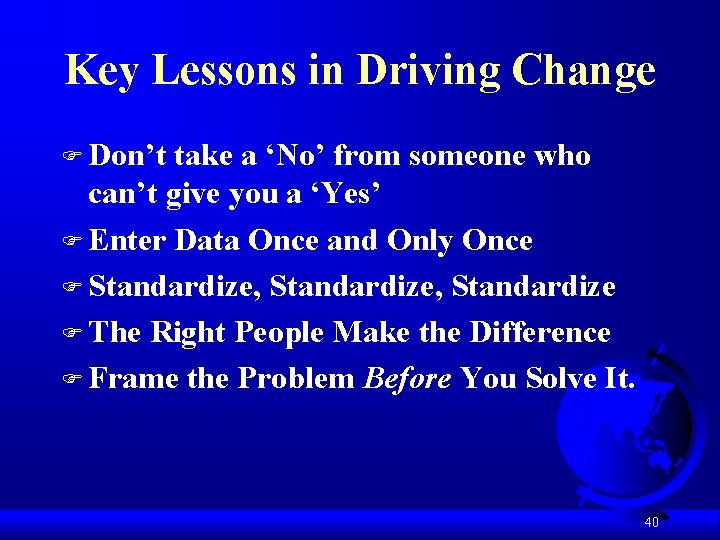
Key Lessons in Driving Change F Don’t take a ‘No’ from someone who can’t give you a ‘Yes’ F Enter Data Once and Only Once F Standardize, Standardize F The Right People Make the Difference F Frame the Problem Before You Solve It. 40
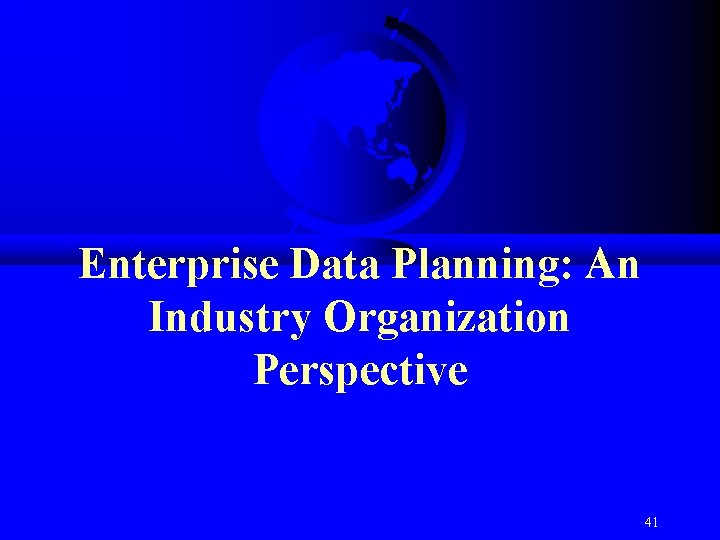
Enterprise Data Planning: An Industry Organization Perspective 41
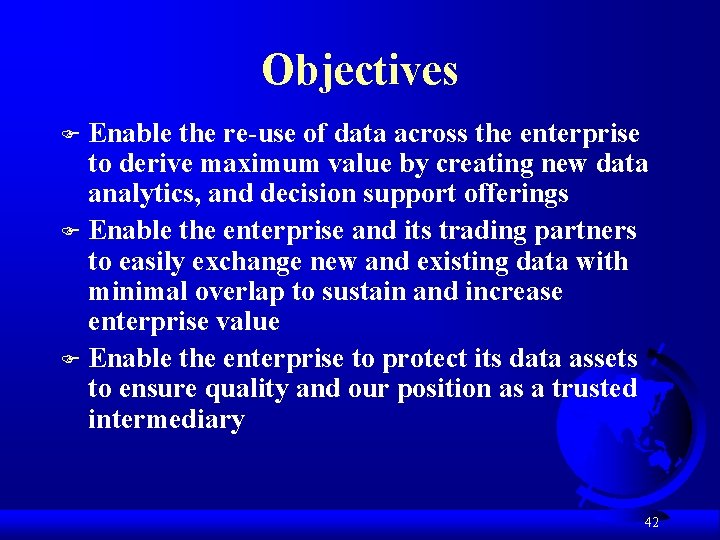
Objectives Enable the re-use of data across the enterprise to derive maximum value by creating new data analytics, and decision support offerings F Enable the enterprise and its trading partners to easily exchange new and existing data with minimal overlap to sustain and increase enterprise value F Enable the enterprise to protect its data assets to ensure quality and our position as a trusted intermediary F 42
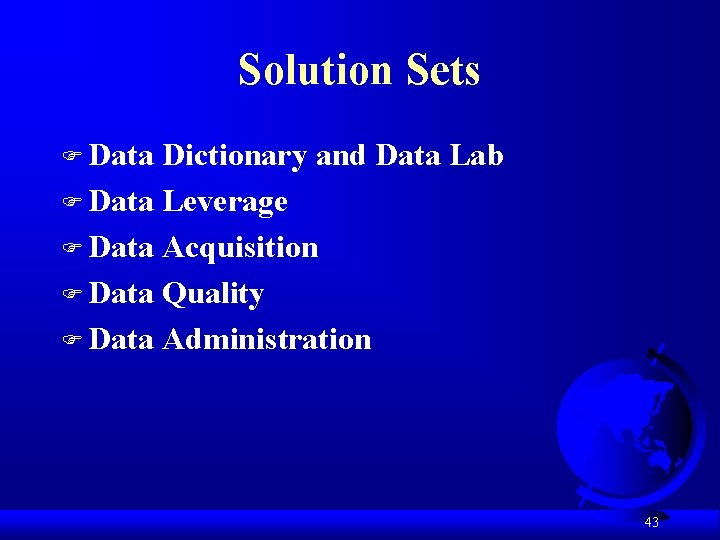
Solution Sets F Data Dictionary and Data Lab F Data Leverage F Data Acquisition F Data Quality F Data Administration 43
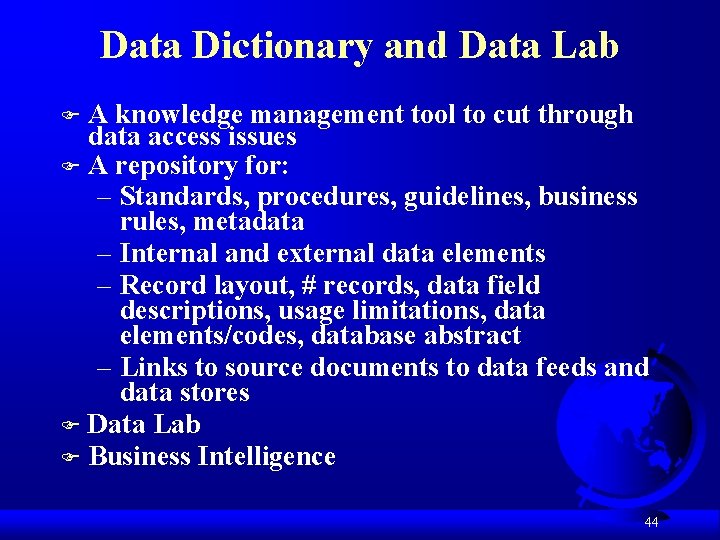
Data Dictionary and Data Lab A knowledge management tool to cut through data access issues F A repository for: – Standards, procedures, guidelines, business rules, metadata – Internal and external data elements – Record layout, # records, data field descriptions, usage limitations, data elements/codes, database abstract – Links to source documents to data feeds and data stores F Data Lab F Business Intelligence F 44
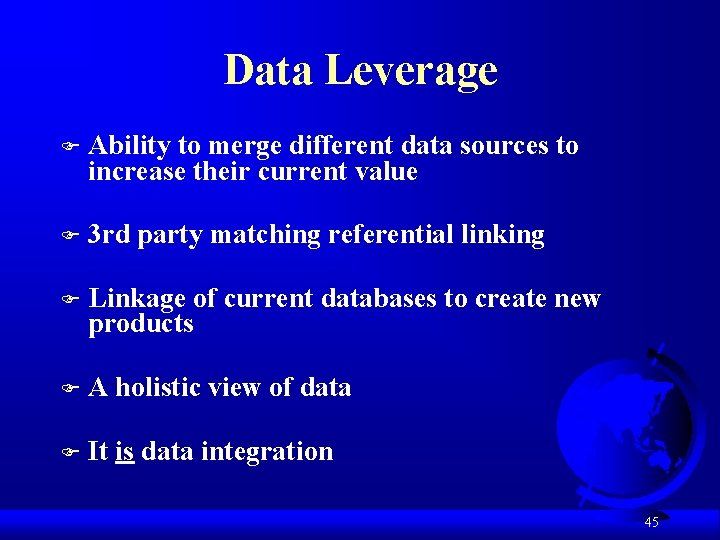
Data Leverage F Ability to merge different data sources to increase their current value F 3 rd party matching referential linking F Linkage of current databases to create new products F A holistic view of data F It is data integration 45
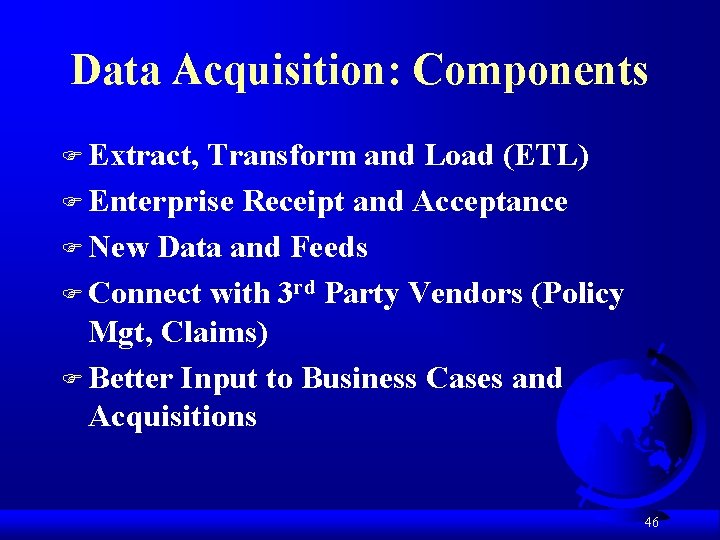
Data Acquisition: Components F Extract, Transform and Load (ETL) F Enterprise Receipt and Acceptance F New Data and Feeds F Connect with 3 rd Party Vendors (Policy Mgt, Claims) F Better Input to Business Cases and Acquisitions 46
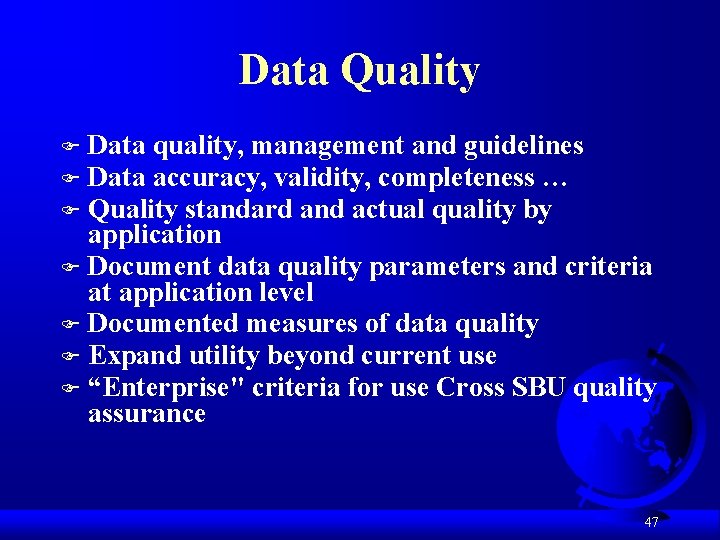
Data Quality Data quality, management and guidelines Data accuracy, validity, completeness … Quality standard and actual quality by application F Document data quality parameters and criteria at application level F Documented measures of data quality F Expand utility beyond current use F “Enterprise" criteria for use Cross SBU quality assurance F F F 47
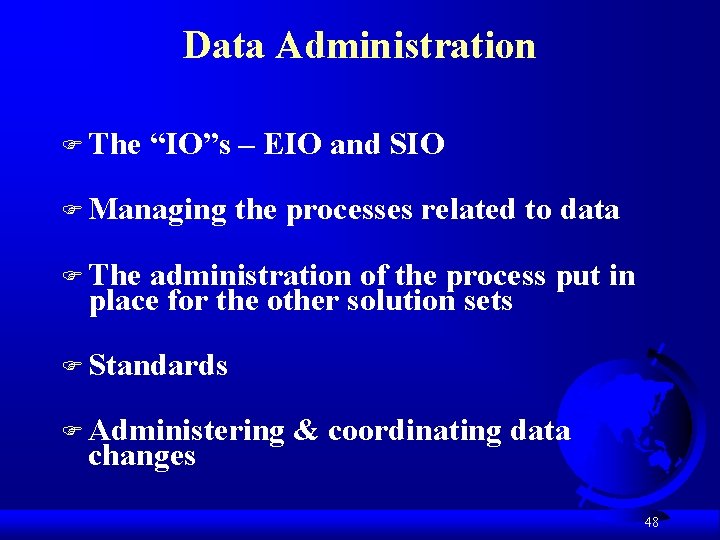
Data Administration F The “IO”s – EIO and SIO F Managing the processes related to data F The administration of the process put in place for the other solution sets F Standards F Administering changes & coordinating data 48
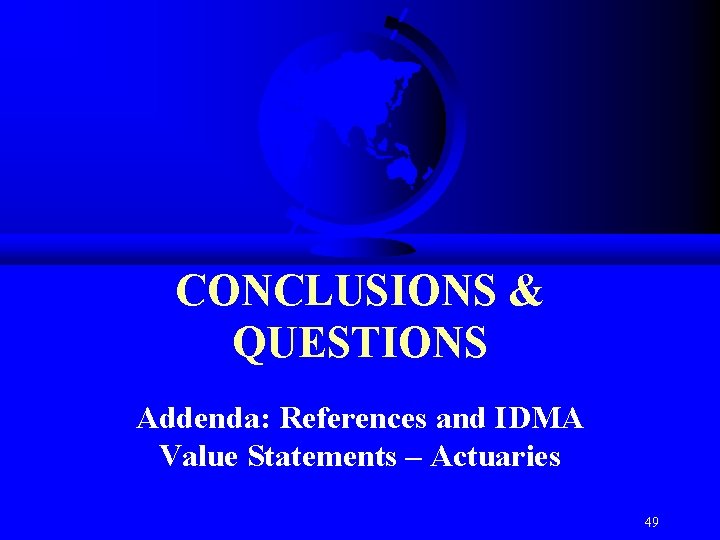
CONCLUSIONS & QUESTIONS Addenda: References and IDMA Value Statements – Actuaries 49
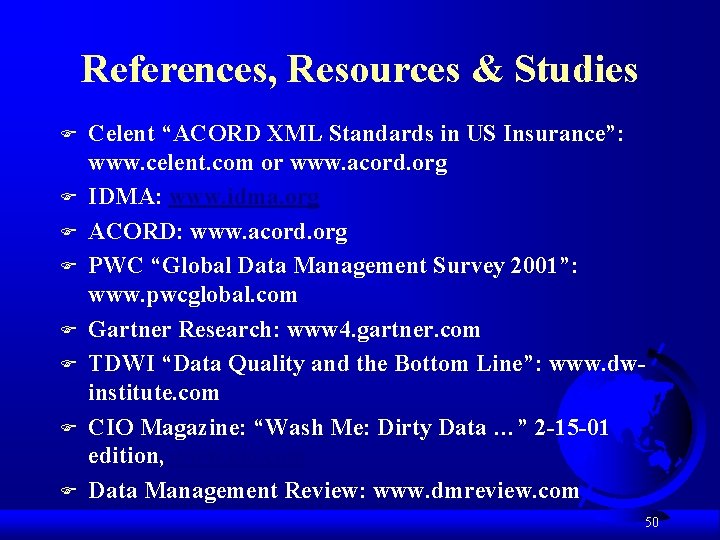
References, Resources & Studies F F F F Celent “ACORD XML Standards in US Insurance”: www. celent. com or www. acord. org IDMA: www. idma. org ACORD: www. acord. org PWC “Global Data Management Survey 2001”: www. pwcglobal. com Gartner Research: www 4. gartner. com TDWI “Data Quality and the Bottom Line”: www. dwinstitute. com CIO Magazine: “Wash Me: Dirty Data …” 2 -15 -01 edition, www. cio. com Data Management Review: www. dmreview. com 50
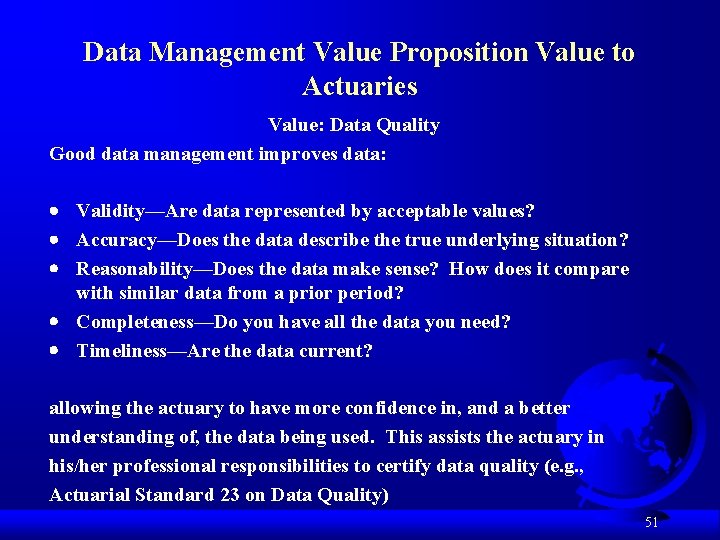
Data Management Value Proposition Value to Actuaries Value: Data Quality Good data management improves data: · Validity—Are data represented by acceptable values? · Accuracy—Does the data describe the true underlying situation? · Reasonability—Does the data make sense? How does it compare with similar data from a prior period? · Completeness—Do you have all the data you need? · Timeliness—Are the data current? allowing the actuary to have more confidence in, and a better understanding of, the data being used. This assists the actuary in his/her professional responsibilities to certify data quality (e. g. , Actuarial Standard 23 on Data Quality) 51
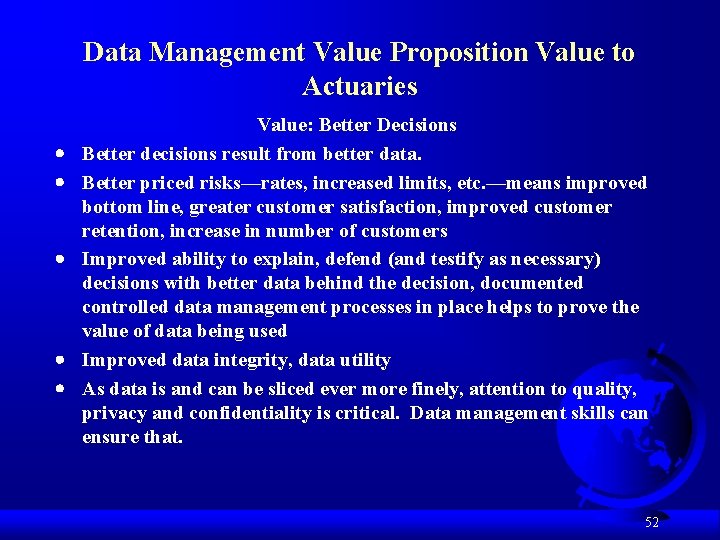
Data Management Value Proposition Value to Actuaries · · · Value: Better Decisions Better decisions result from better data. Better priced risks—rates, increased limits, etc. —means improved bottom line, greater customer satisfaction, improved customer retention, increase in number of customers Improved ability to explain, defend (and testify as necessary) decisions with better data behind the decision, documented controlled data management processes in place helps to prove the value of data being used Improved data integrity, data utility As data is and can be sliced ever more finely, attention to quality, privacy and confidentiality is critical. Data management skills can ensure that. 52
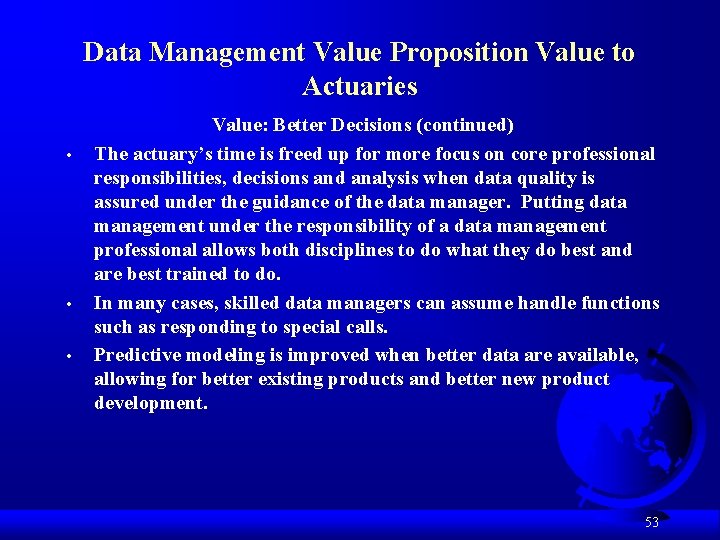
Data Management Value Proposition Value to Actuaries • • • Value: Better Decisions (continued) The actuary’s time is freed up for more focus on core professional responsibilities, decisions and analysis when data quality is assured under the guidance of the data manager. Putting data management under the responsibility of a data management professional allows both disciplines to do what they do best and are best trained to do. In many cases, skilled data managers can assume handle functions such as responding to special calls. Predictive modeling is improved when better data are available, allowing for better existing products and better new product development. 53
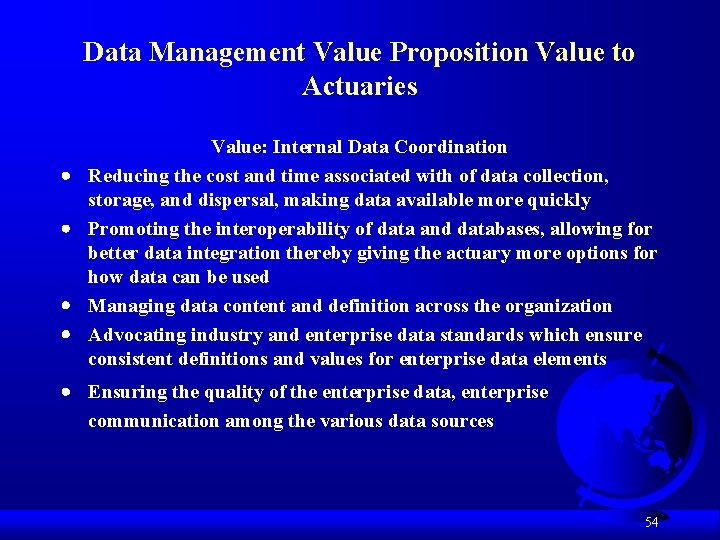
Data Management Value Proposition Value to Actuaries · · Value: Internal Data Coordination Reducing the cost and time associated with of data collection, storage, and dispersal, making data available more quickly Promoting the interoperability of data and databases, allowing for better data integration thereby giving the actuary more options for how data can be used Managing data content and definition across the organization Advocating industry and enterprise data standards which ensure consistent definitions and values for enterprise data elements · Ensuring the quality of the enterprise data, enterprise communication among the various data sources 54
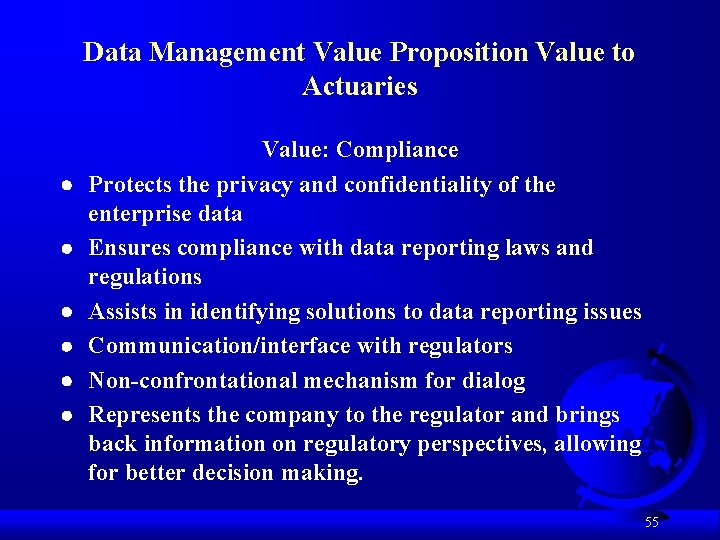
Data Management Value Proposition Value to Actuaries · · · Value: Compliance Protects the privacy and confidentiality of the enterprise data Ensures compliance with data reporting laws and regulations Assists in identifying solutions to data reporting issues Communication/interface with regulators Non-confrontational mechanism for dialog Represents the company to the regulator and brings back information on regulatory perspectives, allowing for better decision making. 55
Anthem of poland
Enterprise information management gartner magic quadrant
Putting the enterprise into the enterprise system
Putting the enterprise into the enterprise system
Enterprise integration strategy
Enterprise development strategy
Social enterprise marketing strategy
Data warehouse seminar
Data center seminar
Corporate strategy vs business strategy
Home replication strategy example
The chase strategy
Multidomestic strategy
Aligning hr strategy with business strategy
Porter’s diamond of national advantage
Crafting and executing strategy in strategic management
The plan of action that prescribes resource allocation
Listening for specific details top-down or bottom-up
Captive company strategy
Multinational strategy vs global strategy
Uml extend
Cas treatment approaches
Princeza na zrnu graska pdf
Slovní úlohy pohyb proti sobě
Naselitev slovanov karantanija
Controlagro cas 4500 manual
Leren hacken voor beginners
T square gatech
Wiris editor math
Zločin in kazen sporočilo
Tri leptira priprema za cas
Raisonnement et démarche clinique infirmière exemple
Ipard cas
Overall cas progress
Cas definition ib
Overall cas progress
Cas clinique chirurgie
Cas clinique cancer du sein
Card european de sanatate calarasi
Cas definition ib
Cas audit standards
Cas creativity activity service
Soa pathway
Dram ras cas
Penn performance management
étude de cas lagos 4ème
Průměrná rychlost vzorec
Cas open source
Escala cas oftalmopatia tiroidea
Ritsel betekenis
Final cas interview
Read minulý čas
Introduction to cas
Fgcu bursar office
Intel cache acceleration software
Nursing cas gpa calculator