Data and Models for Inferring Expected Effects of
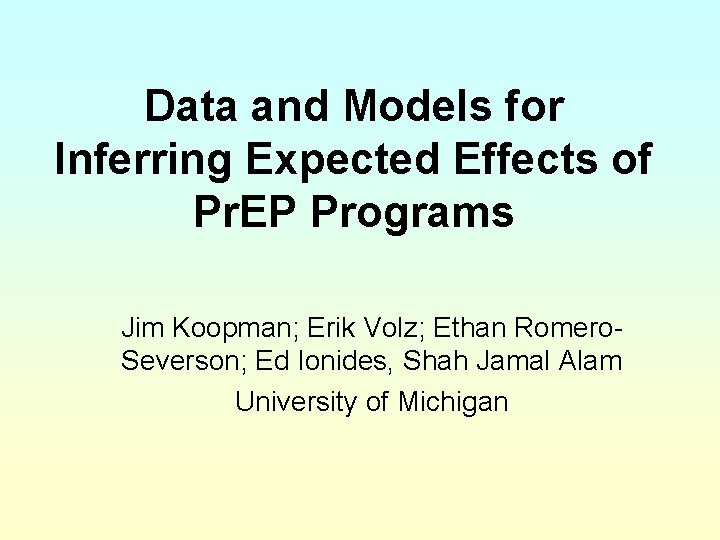
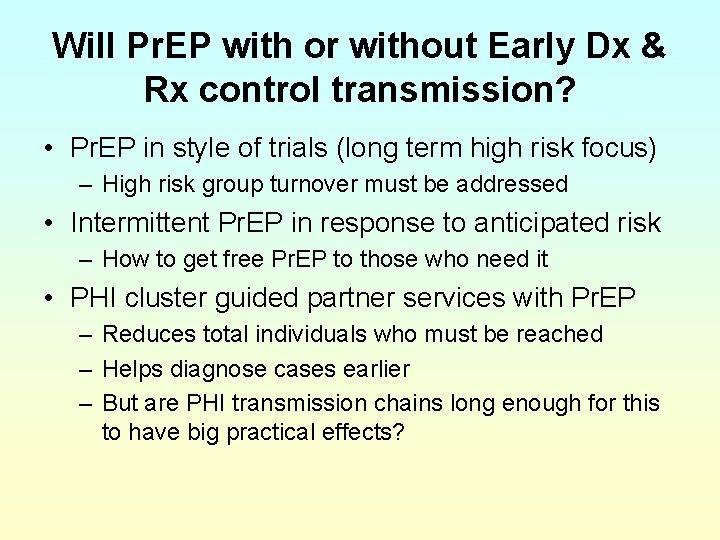
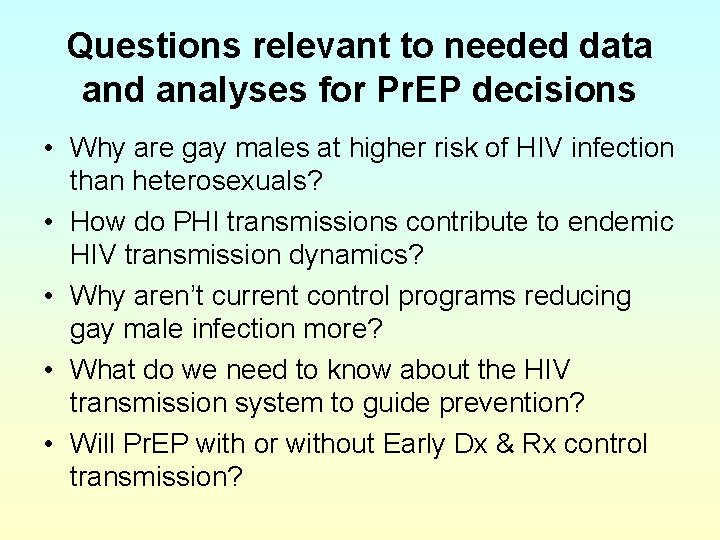
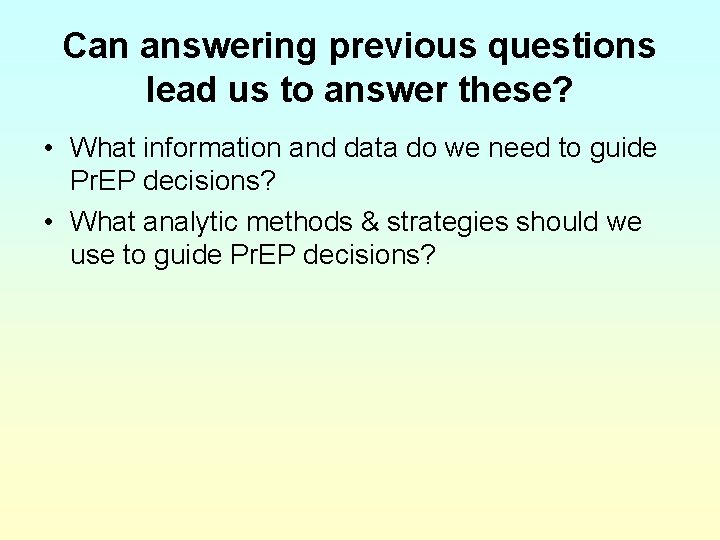
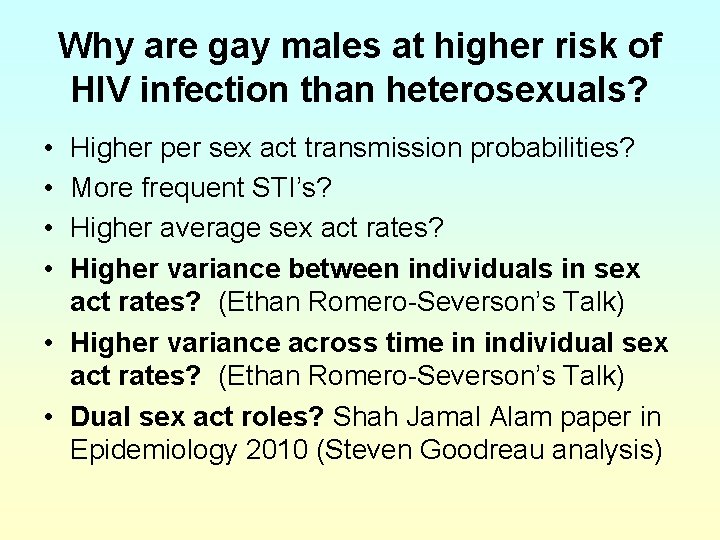
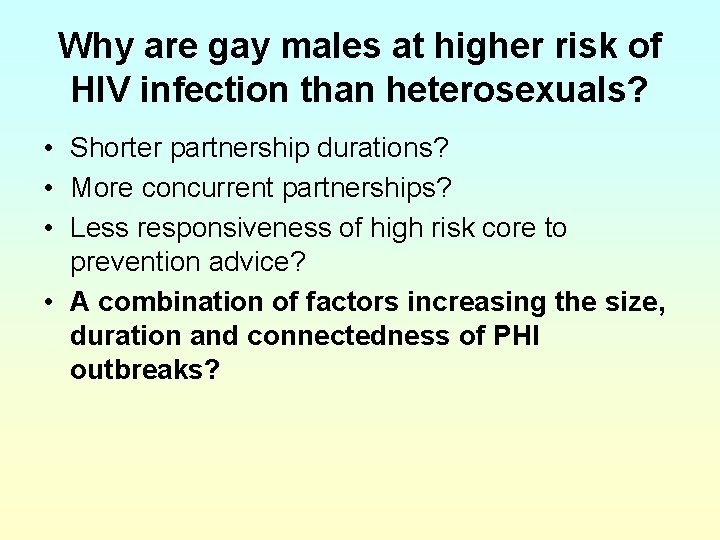
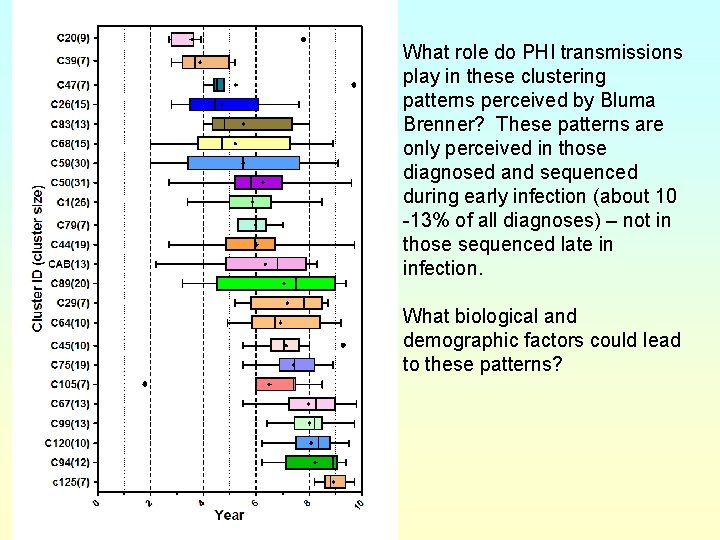
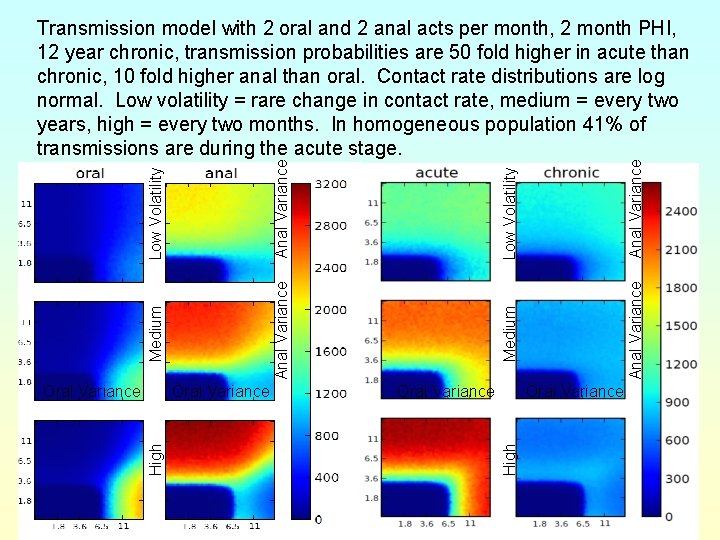
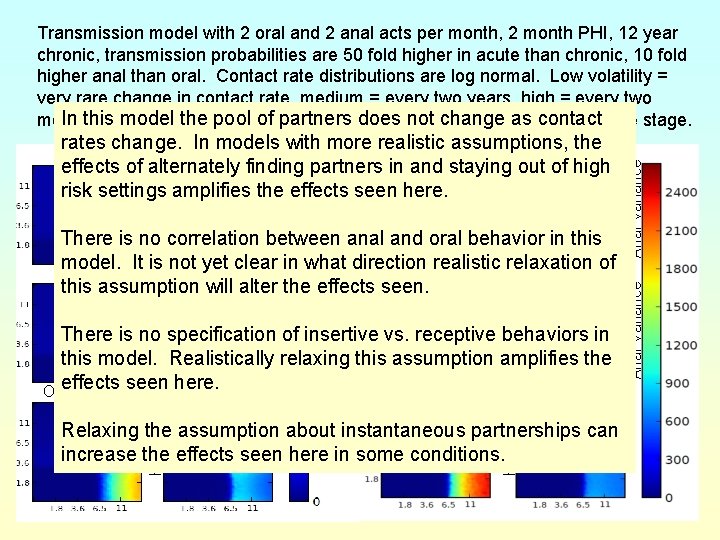
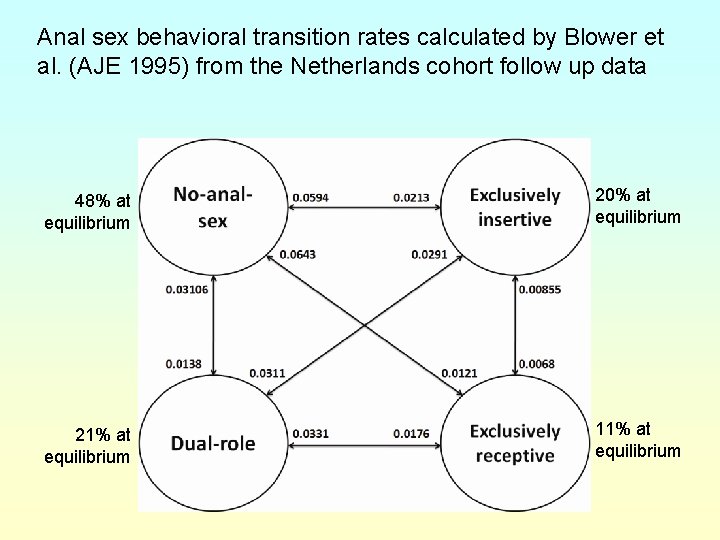
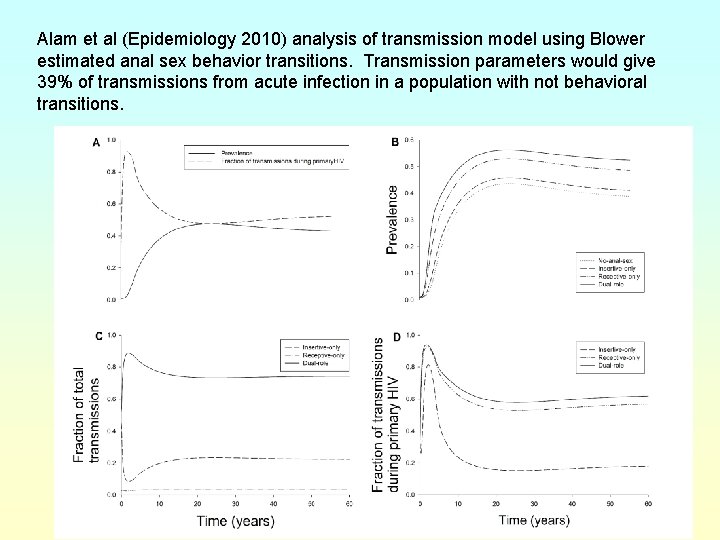
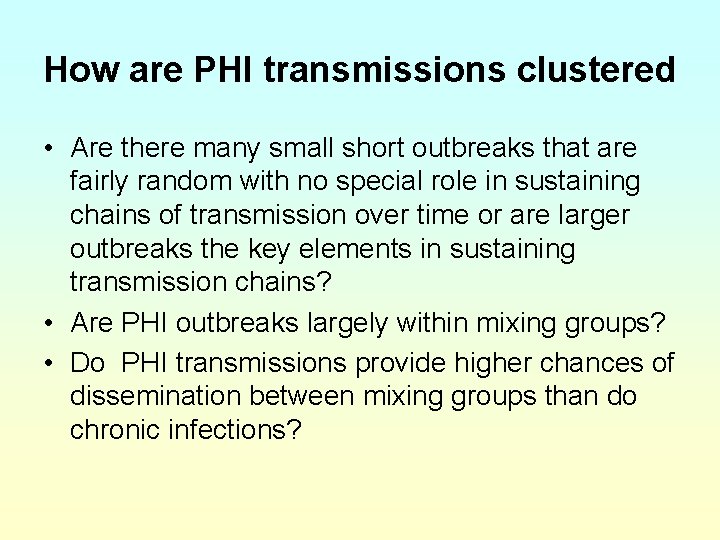
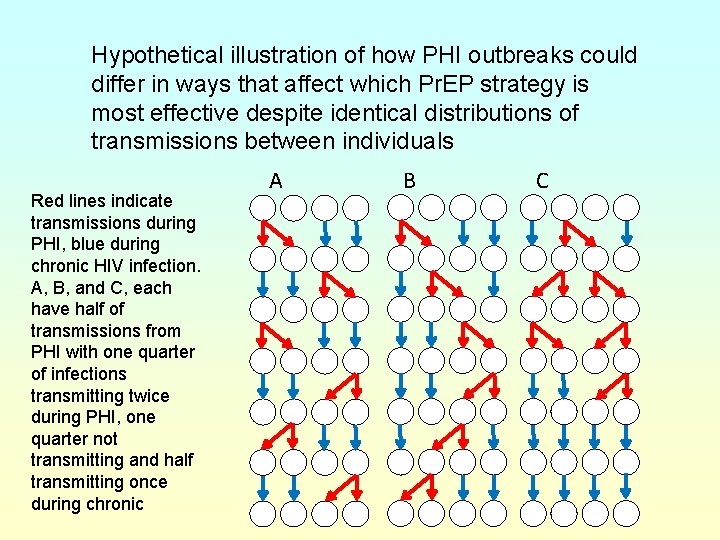
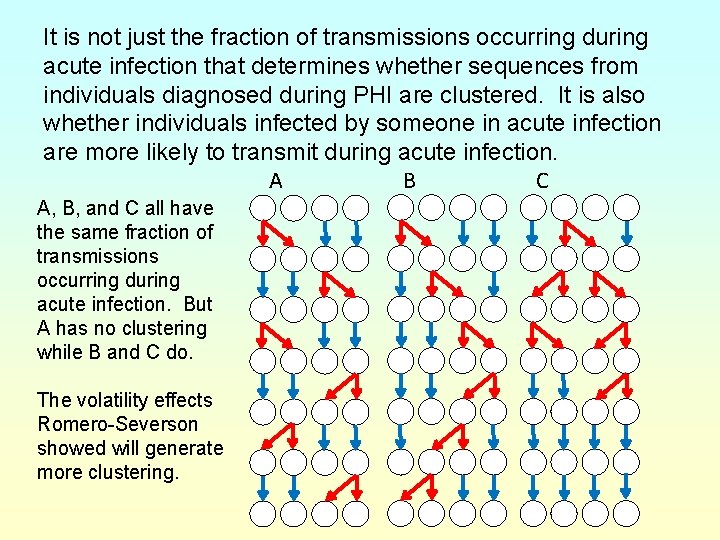
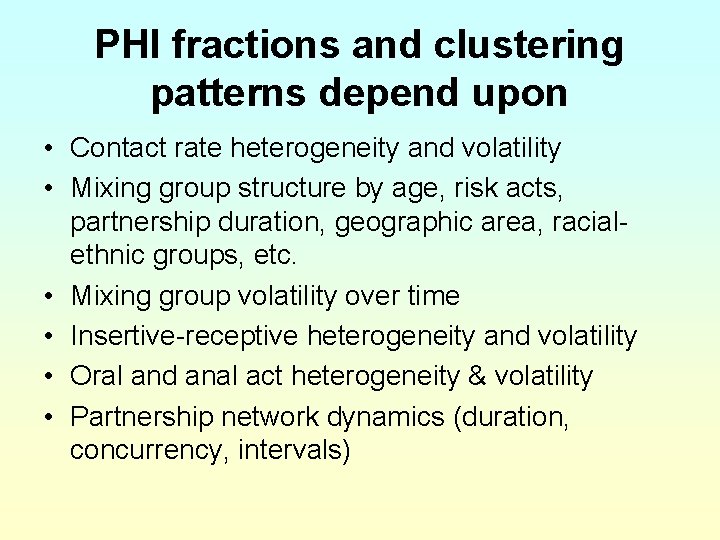
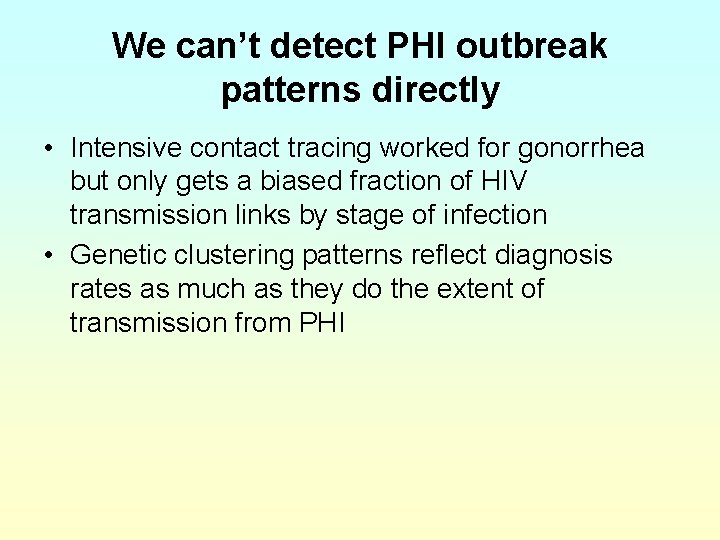
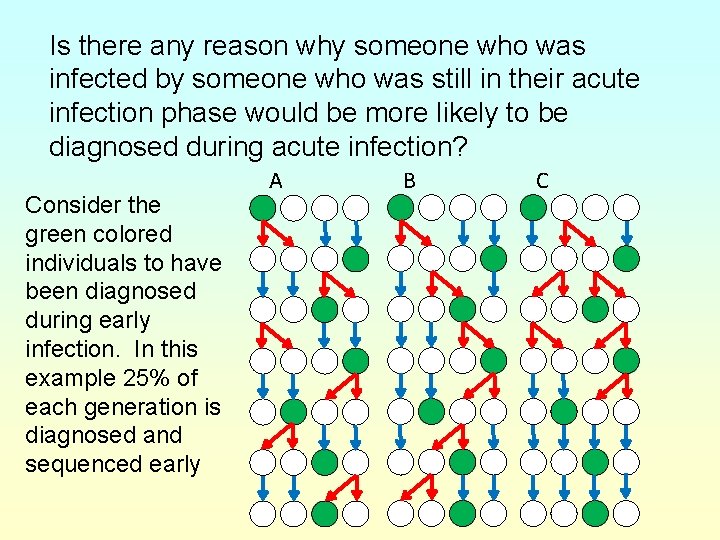
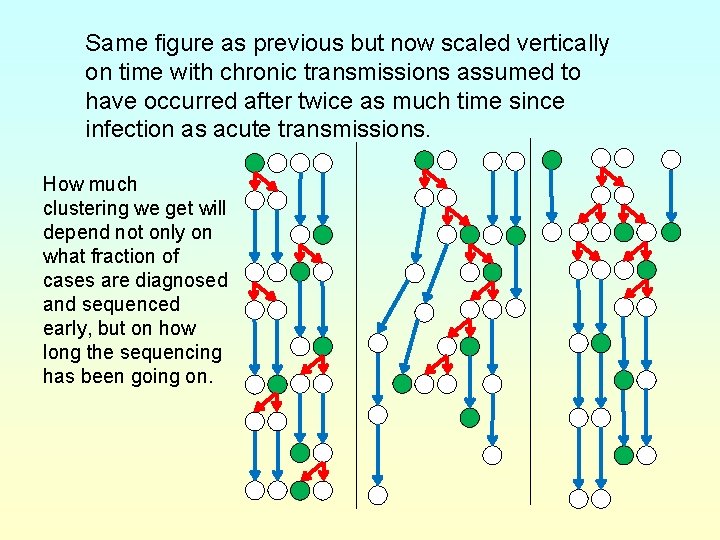
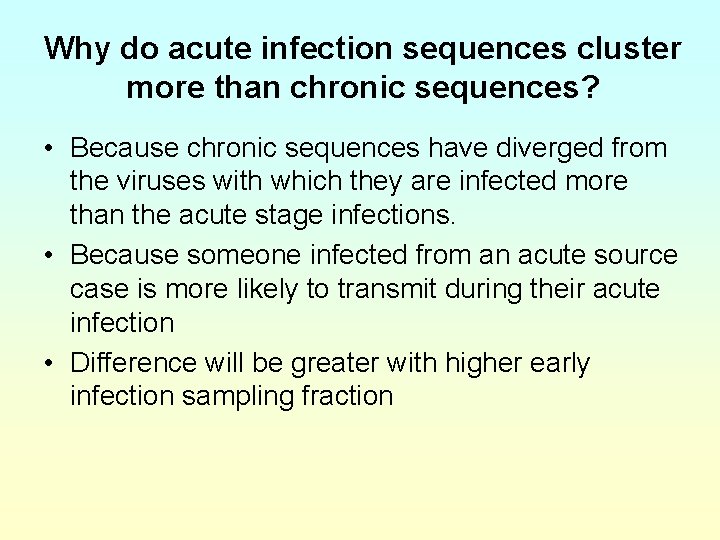
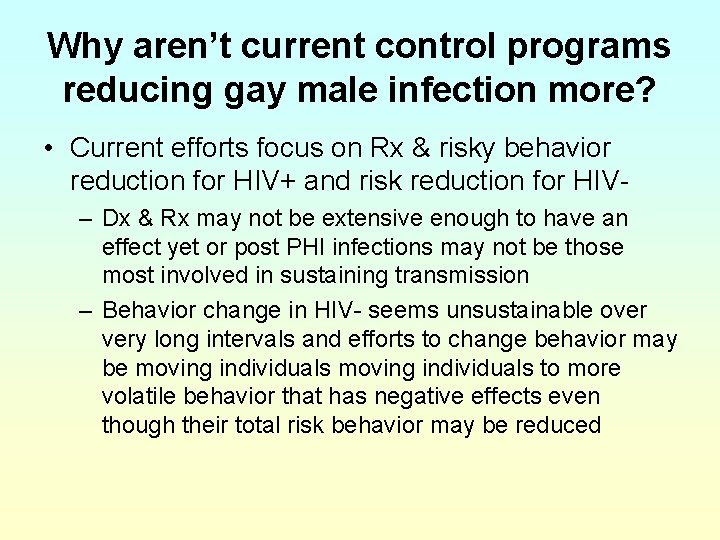
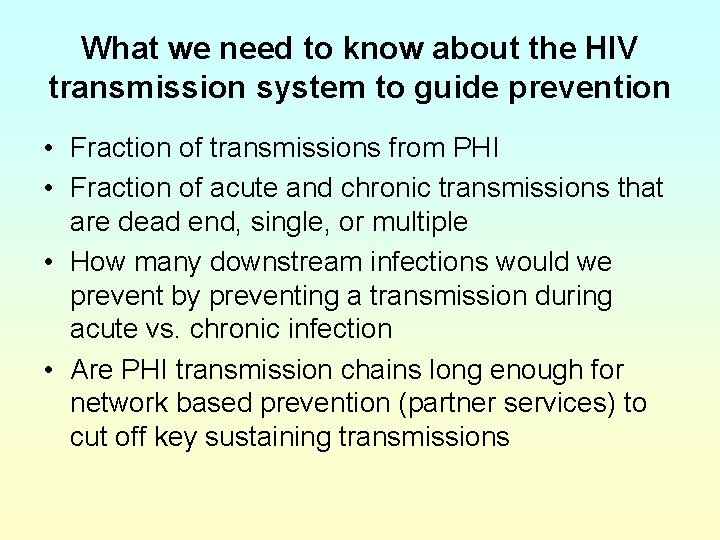
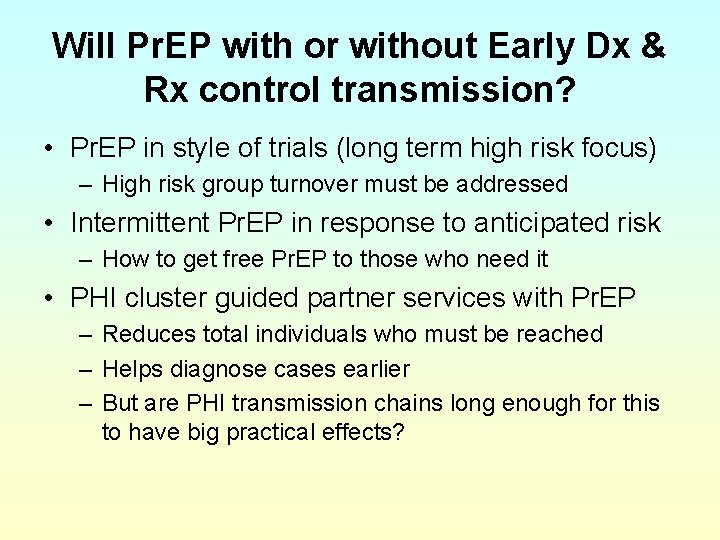
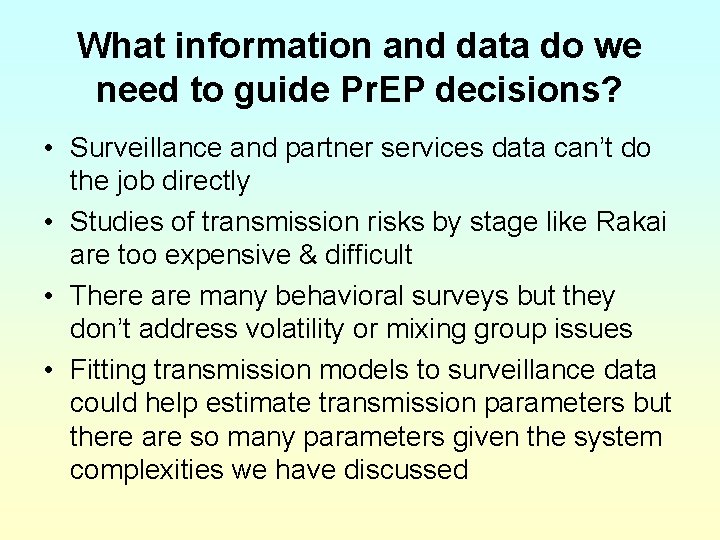
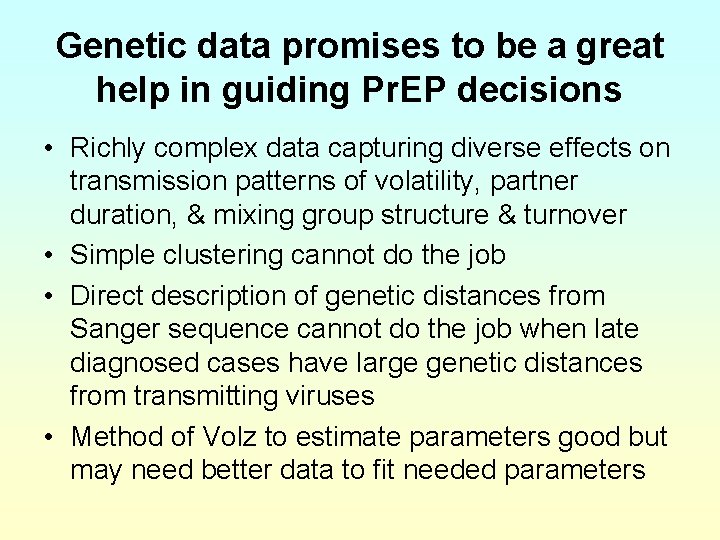
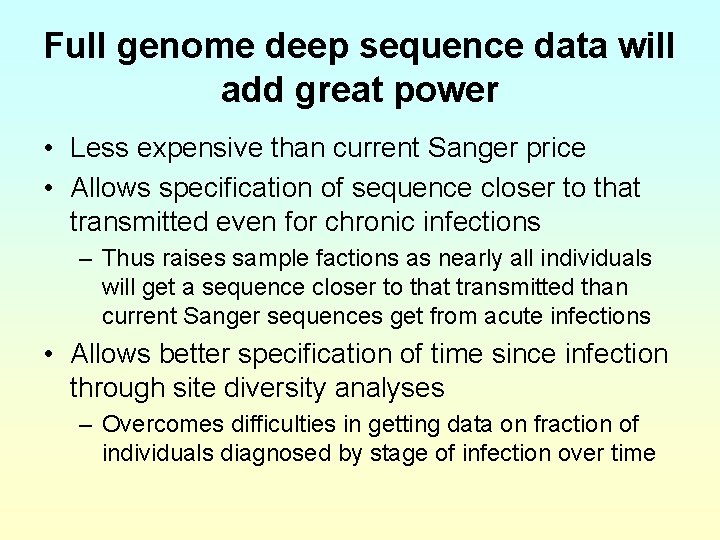
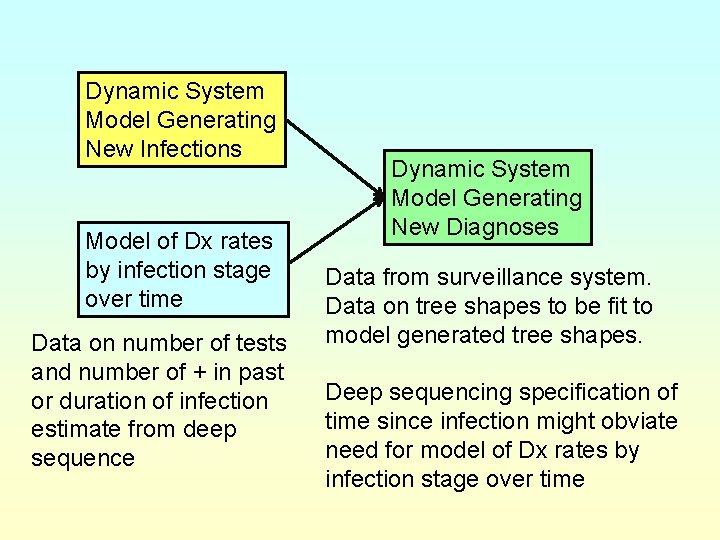
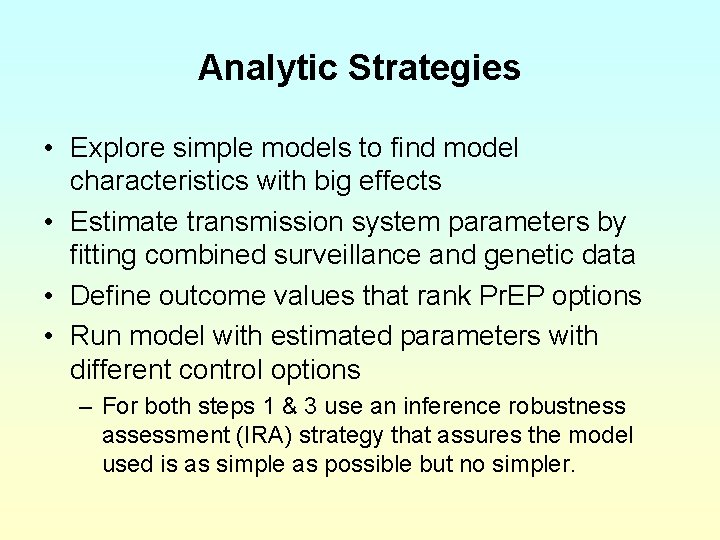
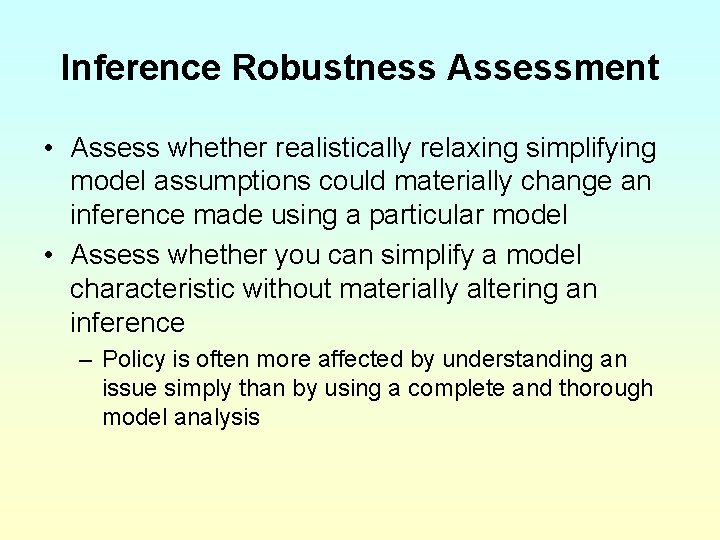
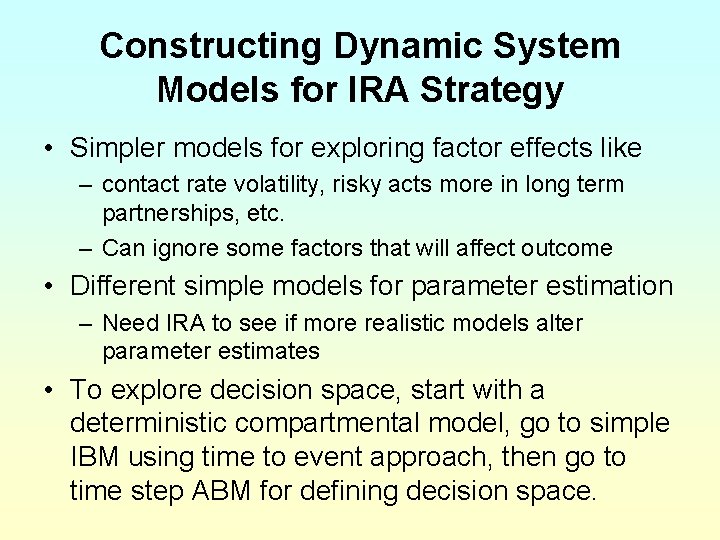
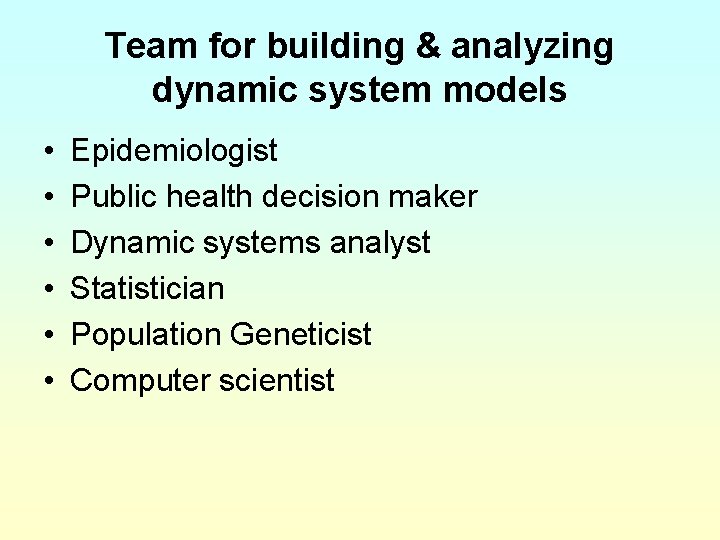
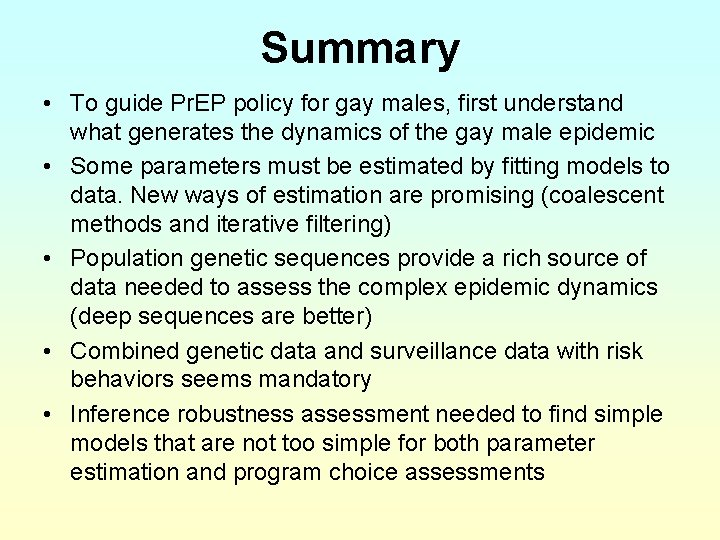
- Slides: 31
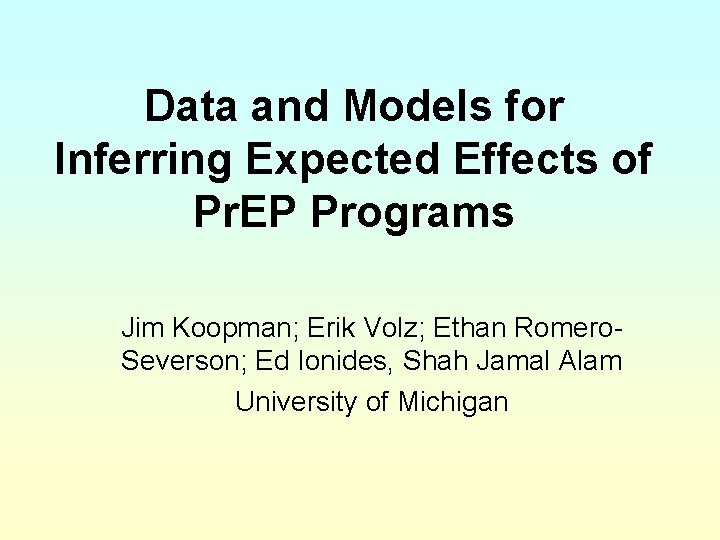
Data and Models for Inferring Expected Effects of Pr. EP Programs Jim Koopman; Erik Volz; Ethan Romero. Severson; Ed Ionides, Shah Jamal Alam University of Michigan
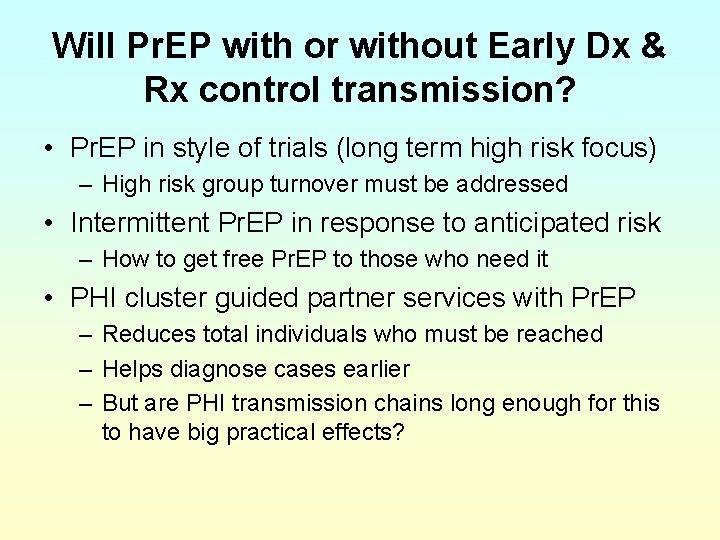
Will Pr. EP with or without Early Dx & Rx control transmission? • Pr. EP in style of trials (long term high risk focus) – High risk group turnover must be addressed • Intermittent Pr. EP in response to anticipated risk – How to get free Pr. EP to those who need it • PHI cluster guided partner services with Pr. EP – Reduces total individuals who must be reached – Helps diagnose cases earlier – But are PHI transmission chains long enough for this to have big practical effects?
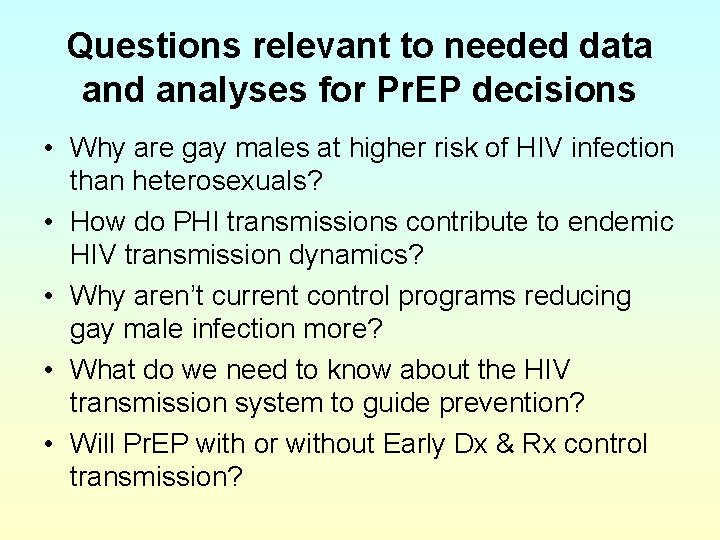
Questions relevant to needed data and analyses for Pr. EP decisions • Why are gay males at higher risk of HIV infection than heterosexuals? • How do PHI transmissions contribute to endemic HIV transmission dynamics? • Why aren’t current control programs reducing gay male infection more? • What do we need to know about the HIV transmission system to guide prevention? • Will Pr. EP with or without Early Dx & Rx control transmission?
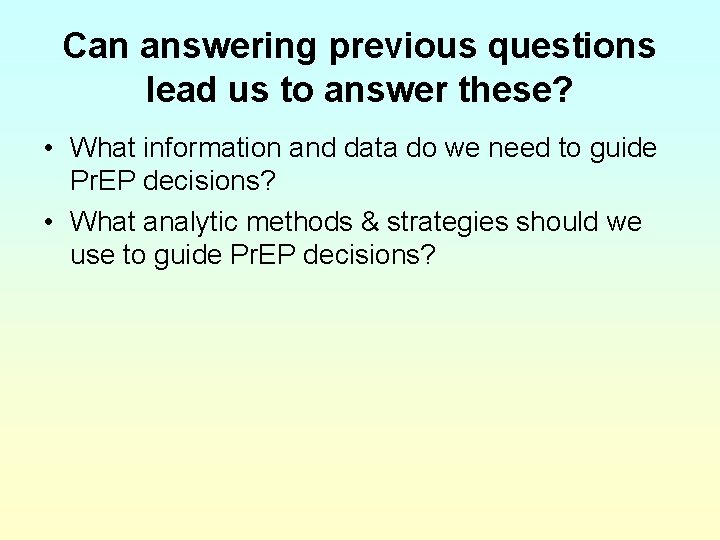
Can answering previous questions lead us to answer these? • What information and data do we need to guide Pr. EP decisions? • What analytic methods & strategies should we use to guide Pr. EP decisions?
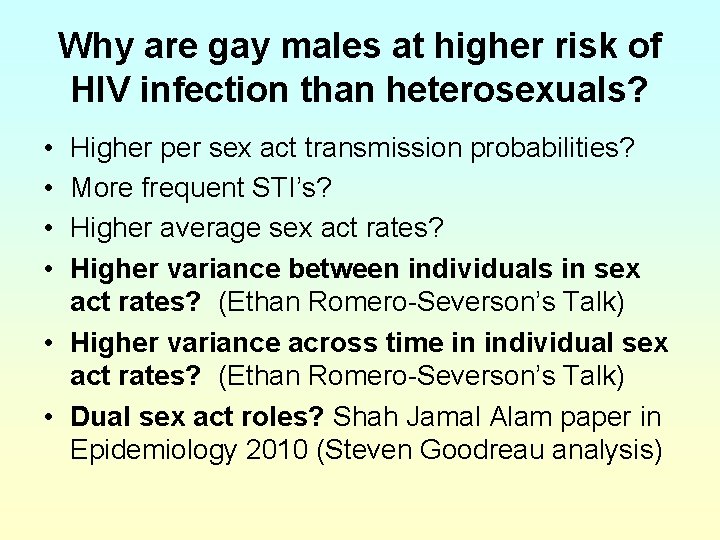
Why are gay males at higher risk of HIV infection than heterosexuals? • • Higher per sex act transmission probabilities? More frequent STI’s? Higher average sex act rates? Higher variance between individuals in sex act rates? (Ethan Romero-Severson’s Talk) • Higher variance across time in individual sex act rates? (Ethan Romero-Severson’s Talk) • Dual sex act roles? Shah Jamal Alam paper in Epidemiology 2010 (Steven Goodreau analysis)
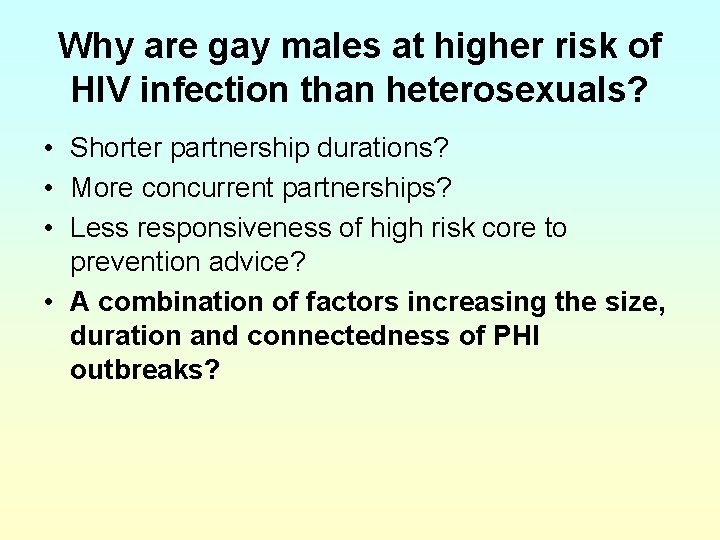
Why are gay males at higher risk of HIV infection than heterosexuals? • Shorter partnership durations? • More concurrent partnerships? • Less responsiveness of high risk core to prevention advice? • A combination of factors increasing the size, duration and connectedness of PHI outbreaks?
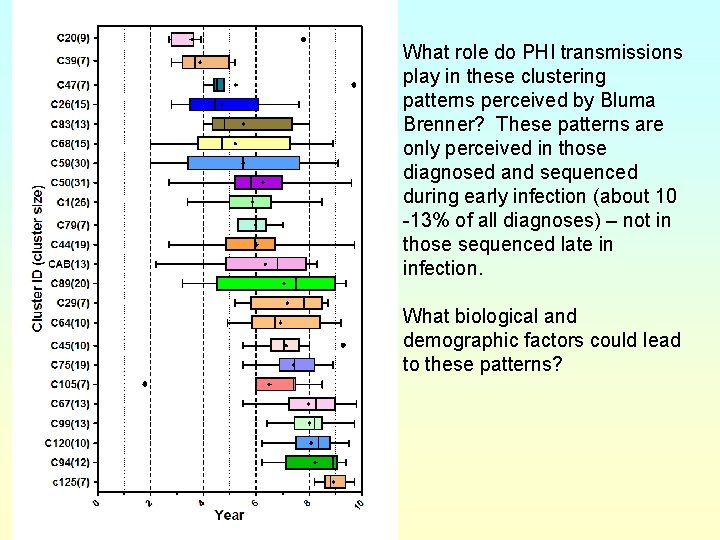
What role do PHI transmissions play in these clustering patterns perceived by Bluma Brenner? These patterns are only perceived in those diagnosed and sequenced during early infection (about 10 -13% of all diagnoses) – not in those sequenced late in infection. What biological and demographic factors could lead to these patterns?
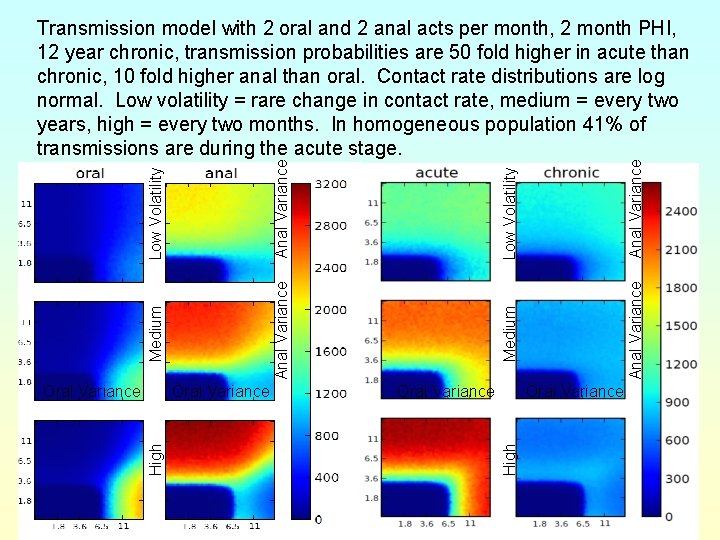
Oral Variance High Oral Variance Anal Variance Low Volatility Medium Oral Variance High Medium Anal Variance Low Volatility Anal Variance Transmission model with 2 oral and 2 anal acts per month, 2 month PHI, 12 year chronic, transmission probabilities are 50 fold higher in acute than chronic, 10 fold higher anal than oral. Contact rate distributions are log normal. Low volatility = rare change in contact rate, medium = every two years, high = every two months. In homogeneous population 41% of transmissions are during the acute stage.
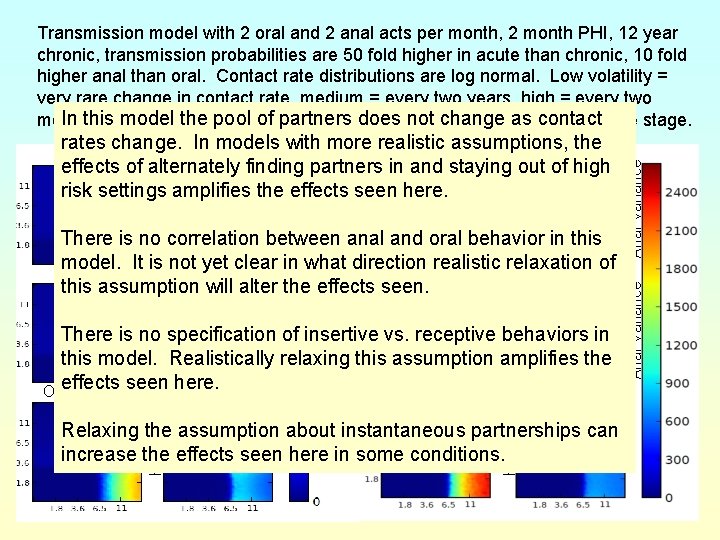
Transmission model with 2 oral and 2 anal acts per month, 2 month PHI, 12 year chronic, transmission probabilities are 50 fold higher in acute than chronic, 10 fold higher anal than oral. Contact rate distributions are log normal. Low volatility = very rare change in contact rate, medium = every two years, high = every two In this. Inmodel the poolpopulation of partners does not change as months. homogeneous 41% of transmissions arecontact in the acute stage. Low Volatility Anal Variance Medium Low Volatility Medium rates change. In models with more realistic assumptions, the effects of alternately finding partners in and staying out of high risk settings amplifies the effects seen here. There is no correlation between anal and oral behavior in this model. It is not yet clear in what direction realistic relaxation of this assumption will alter the effects seen. There is no specification of insertive vs. receptive behaviors in this model. Realistically relaxing this assumption amplifies the effects seen Oral here. Oral Variance High Relaxing the assumption about instantaneous partnerships can increase the effects seen here in some conditions.
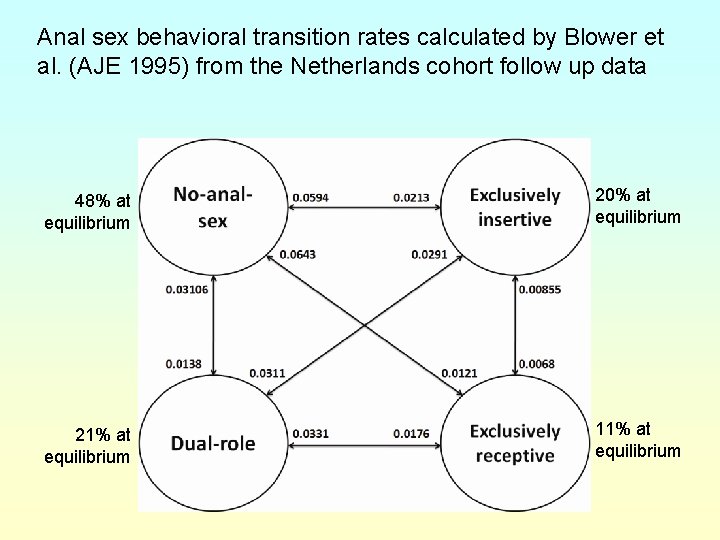
Anal sex behavioral transition rates calculated by Blower et al. (AJE 1995) from the Netherlands cohort follow up data 48% at equilibrium 20% at equilibrium 21% at equilibrium 11% at equilibrium
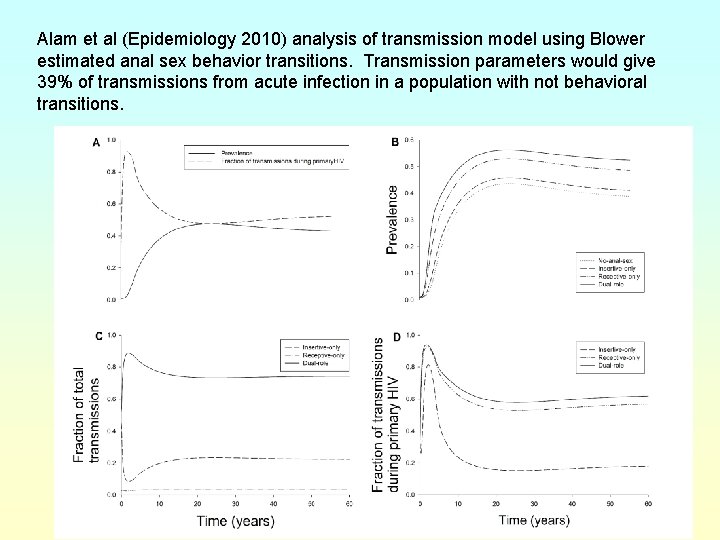
Alam et al (Epidemiology 2010) analysis of transmission model using Blower estimated anal sex behavior transitions. Transmission parameters would give 39% of transmissions from acute infection in a population with not behavioral transitions.
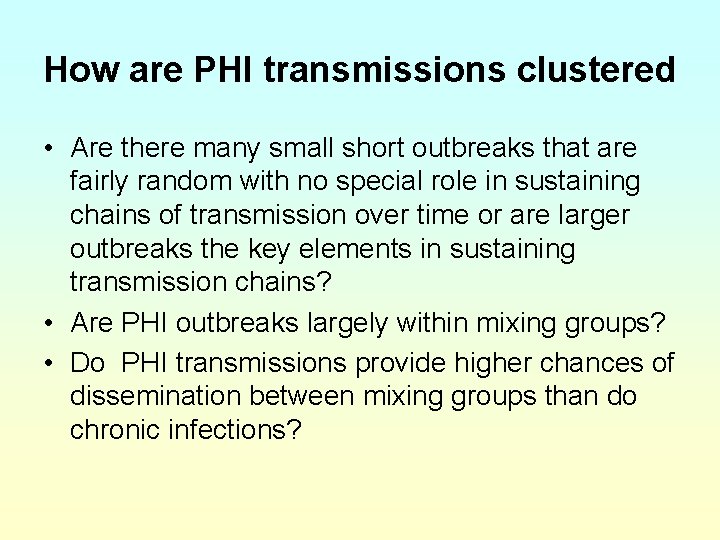
How are PHI transmissions clustered • Are there many small short outbreaks that are fairly random with no special role in sustaining chains of transmission over time or are larger outbreaks the key elements in sustaining transmission chains? • Are PHI outbreaks largely within mixing groups? • Do PHI transmissions provide higher chances of dissemination between mixing groups than do chronic infections?
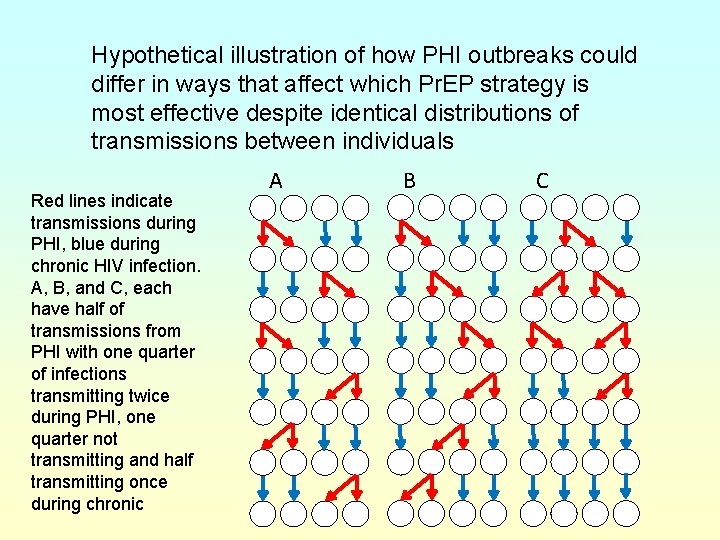
Hypothetical illustration of how PHI outbreaks could differ in ways that affect which Pr. EP strategy is most effective despite identical distributions of transmissions between individuals Red lines indicate transmissions during PHI, blue during chronic HIV infection. A, B, and C, each have half of transmissions from PHI with one quarter of infections transmitting twice during PHI, one quarter not transmitting and half transmitting once during chronic A B C
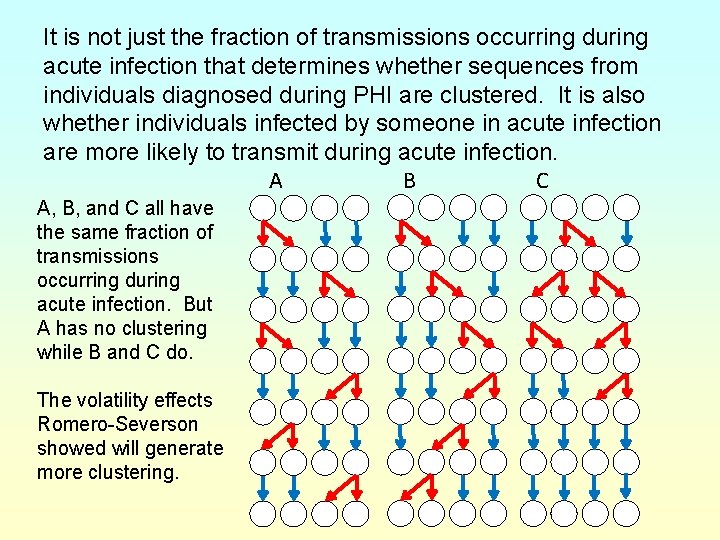
It is not just the fraction of transmissions occurring during acute infection that determines whether sequences from individuals diagnosed during PHI are clustered. It is also whether individuals infected by someone in acute infection are more likely to transmit during acute infection. B A C A, B, and C all have the same fraction of transmissions occurring during acute infection. But A has no clustering while B and C do. The volatility effects Romero-Severson showed will generate more clustering.
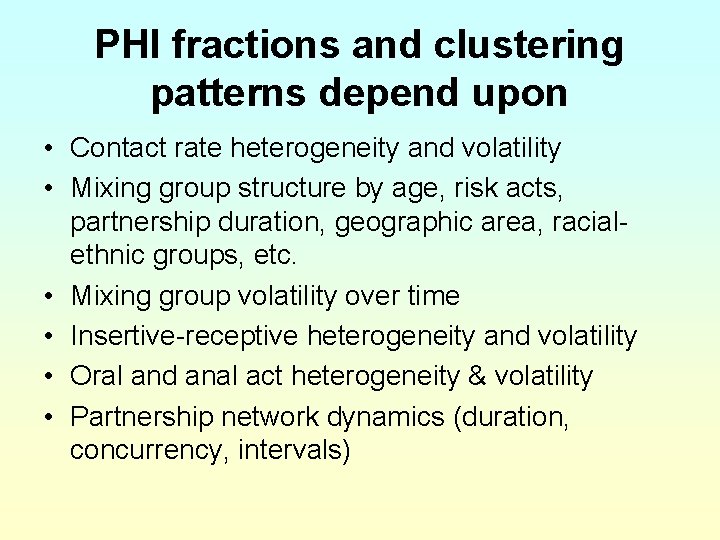
PHI fractions and clustering patterns depend upon • Contact rate heterogeneity and volatility • Mixing group structure by age, risk acts, partnership duration, geographic area, racialethnic groups, etc. • Mixing group volatility over time • Insertive-receptive heterogeneity and volatility • Oral and anal act heterogeneity & volatility • Partnership network dynamics (duration, concurrency, intervals)
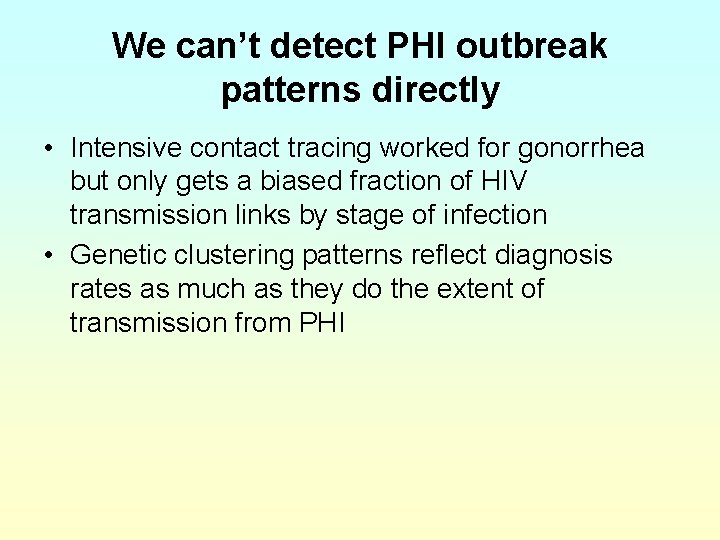
We can’t detect PHI outbreak patterns directly • Intensive contact tracing worked for gonorrhea but only gets a biased fraction of HIV transmission links by stage of infection • Genetic clustering patterns reflect diagnosis rates as much as they do the extent of transmission from PHI
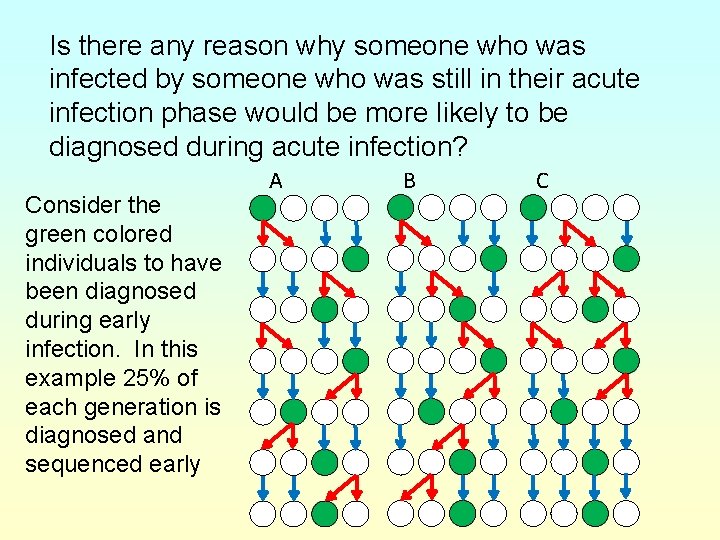
Is there any reason why someone who was infected by someone who was still in their acute infection phase would be more likely to be diagnosed during acute infection? Consider the green colored individuals to have been diagnosed during early infection. In this example 25% of each generation is diagnosed and sequenced early A B C
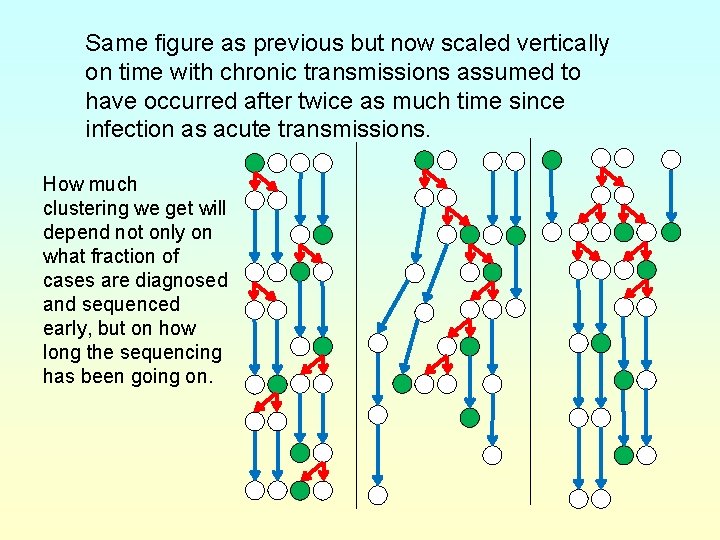
Same figure as previous but now scaled vertically on time with chronic transmissions assumed to have occurred after twice as much time since infection as acute transmissions. How much clustering we get will depend not only on what fraction of cases are diagnosed and sequenced early, but on how long the sequencing has been going on.
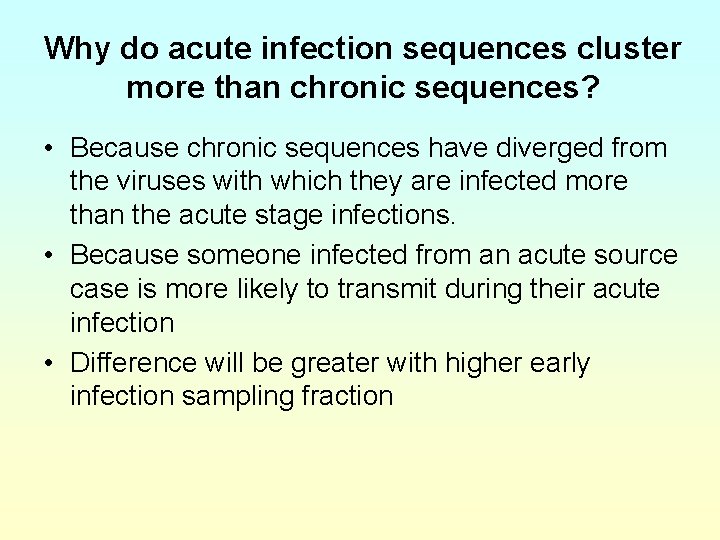
Why do acute infection sequences cluster more than chronic sequences? • Because chronic sequences have diverged from the viruses with which they are infected more than the acute stage infections. • Because someone infected from an acute source case is more likely to transmit during their acute infection • Difference will be greater with higher early infection sampling fraction
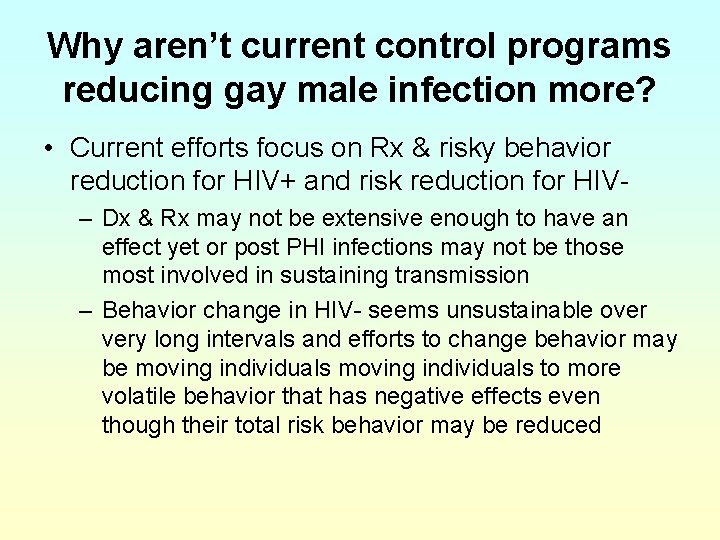
Why aren’t current control programs reducing gay male infection more? • Current efforts focus on Rx & risky behavior reduction for HIV+ and risk reduction for HIV– Dx & Rx may not be extensive enough to have an effect yet or post PHI infections may not be those most involved in sustaining transmission – Behavior change in HIV- seems unsustainable over very long intervals and efforts to change behavior may be moving individuals to more volatile behavior that has negative effects even though their total risk behavior may be reduced
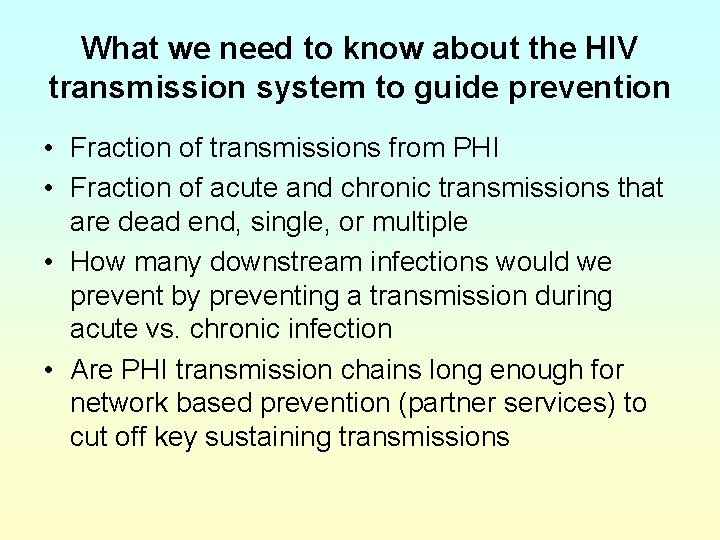
What we need to know about the HIV transmission system to guide prevention • Fraction of transmissions from PHI • Fraction of acute and chronic transmissions that are dead end, single, or multiple • How many downstream infections would we prevent by preventing a transmission during acute vs. chronic infection • Are PHI transmission chains long enough for network based prevention (partner services) to cut off key sustaining transmissions
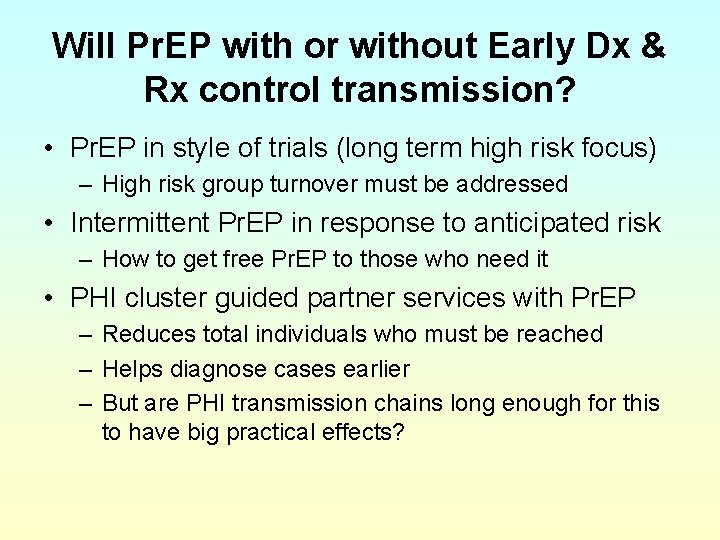
Will Pr. EP with or without Early Dx & Rx control transmission? • Pr. EP in style of trials (long term high risk focus) – High risk group turnover must be addressed • Intermittent Pr. EP in response to anticipated risk – How to get free Pr. EP to those who need it • PHI cluster guided partner services with Pr. EP – Reduces total individuals who must be reached – Helps diagnose cases earlier – But are PHI transmission chains long enough for this to have big practical effects?
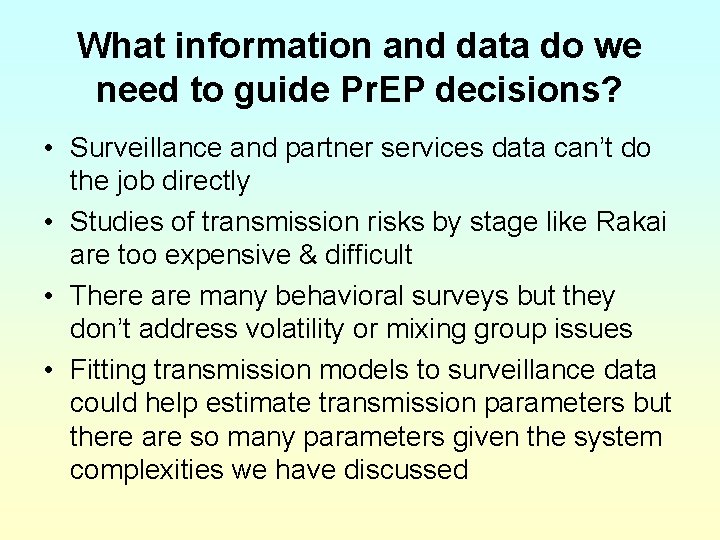
What information and data do we need to guide Pr. EP decisions? • Surveillance and partner services data can’t do the job directly • Studies of transmission risks by stage like Rakai are too expensive & difficult • There are many behavioral surveys but they don’t address volatility or mixing group issues • Fitting transmission models to surveillance data could help estimate transmission parameters but there are so many parameters given the system complexities we have discussed
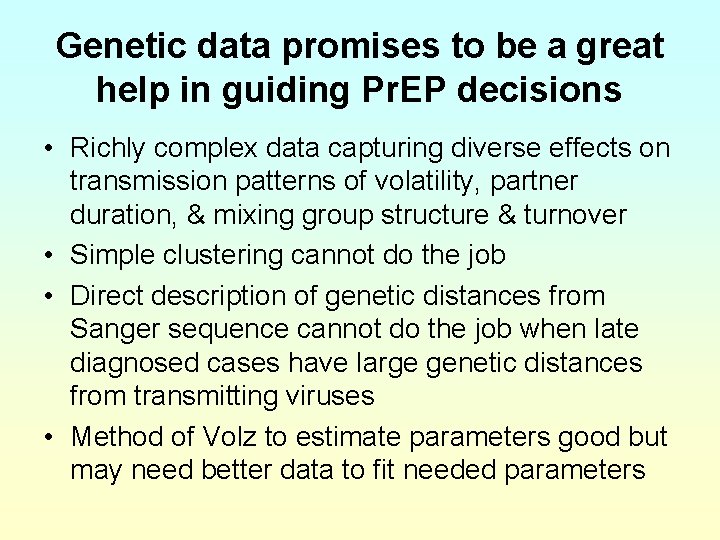
Genetic data promises to be a great help in guiding Pr. EP decisions • Richly complex data capturing diverse effects on transmission patterns of volatility, partner duration, & mixing group structure & turnover • Simple clustering cannot do the job • Direct description of genetic distances from Sanger sequence cannot do the job when late diagnosed cases have large genetic distances from transmitting viruses • Method of Volz to estimate parameters good but may need better data to fit needed parameters
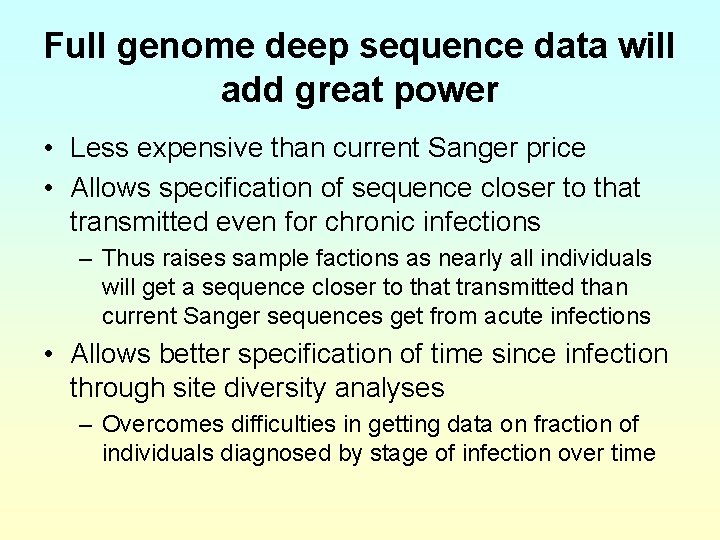
Full genome deep sequence data will add great power • Less expensive than current Sanger price • Allows specification of sequence closer to that transmitted even for chronic infections – Thus raises sample factions as nearly all individuals will get a sequence closer to that transmitted than current Sanger sequences get from acute infections • Allows better specification of time since infection through site diversity analyses – Overcomes difficulties in getting data on fraction of individuals diagnosed by stage of infection over time
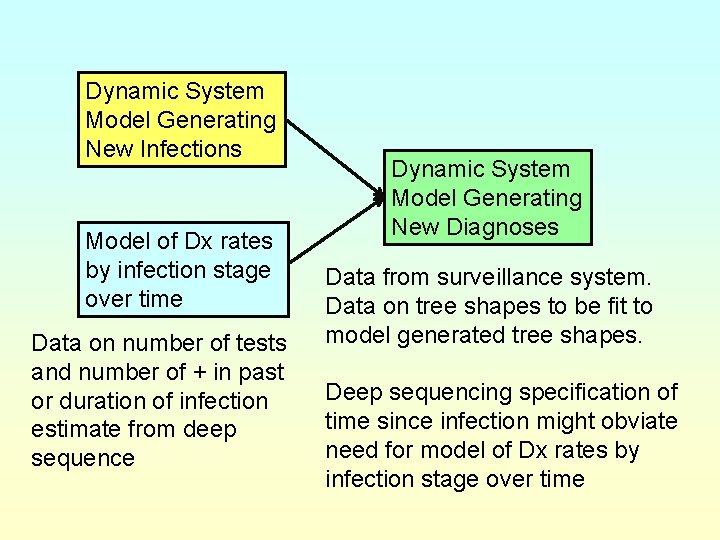
Dynamic System Model Generating New Infections Model of Dx rates by infection stage over time Data on number of tests and number of + in past or duration of infection estimate from deep sequence Dynamic System Model Generating New Diagnoses Data from surveillance system. Data on tree shapes to be fit to model generated tree shapes. Deep sequencing specification of time since infection might obviate need for model of Dx rates by infection stage over time
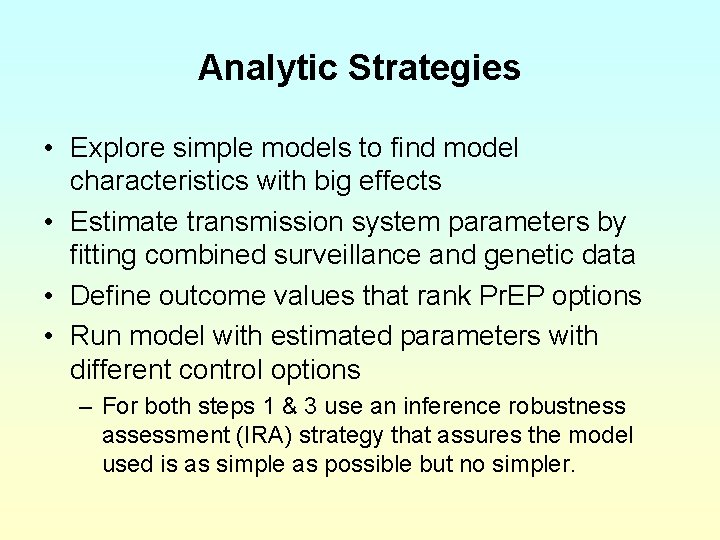
Analytic Strategies • Explore simple models to find model characteristics with big effects • Estimate transmission system parameters by fitting combined surveillance and genetic data • Define outcome values that rank Pr. EP options • Run model with estimated parameters with different control options – For both steps 1 & 3 use an inference robustness assessment (IRA) strategy that assures the model used is as simple as possible but no simpler.
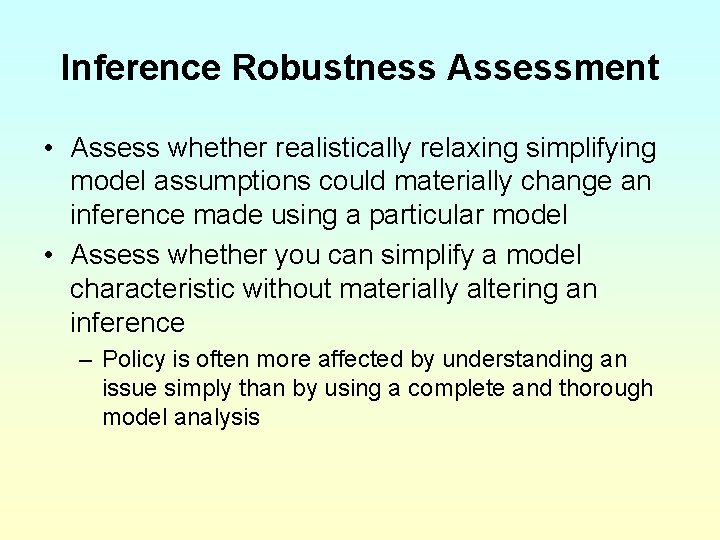
Inference Robustness Assessment • Assess whether realistically relaxing simplifying model assumptions could materially change an inference made using a particular model • Assess whether you can simplify a model characteristic without materially altering an inference – Policy is often more affected by understanding an issue simply than by using a complete and thorough model analysis
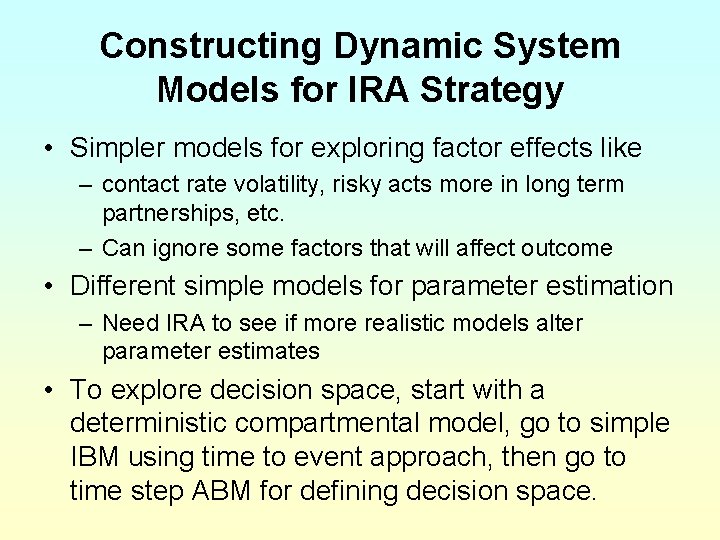
Constructing Dynamic System Models for IRA Strategy • Simpler models for exploring factor effects like – contact rate volatility, risky acts more in long term partnerships, etc. – Can ignore some factors that will affect outcome • Different simple models for parameter estimation – Need IRA to see if more realistic models alter parameter estimates • To explore decision space, start with a deterministic compartmental model, go to simple IBM using time to event approach, then go to time step ABM for defining decision space.
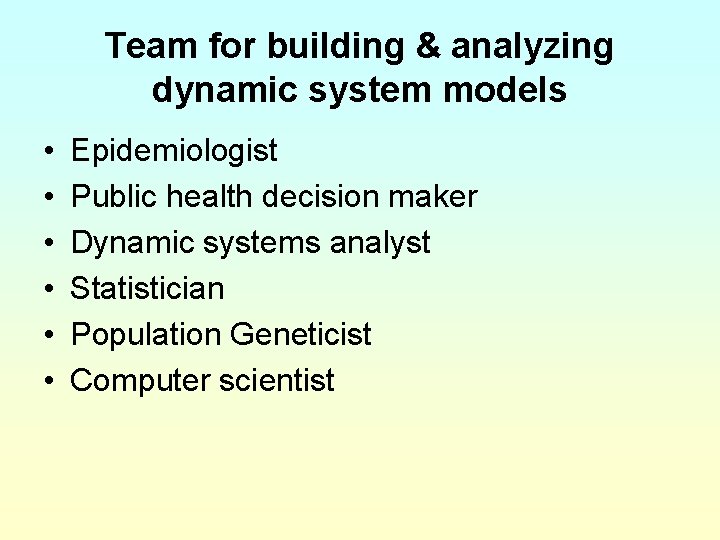
Team for building & analyzing dynamic system models • • • Epidemiologist Public health decision maker Dynamic systems analyst Statistician Population Geneticist Computer scientist
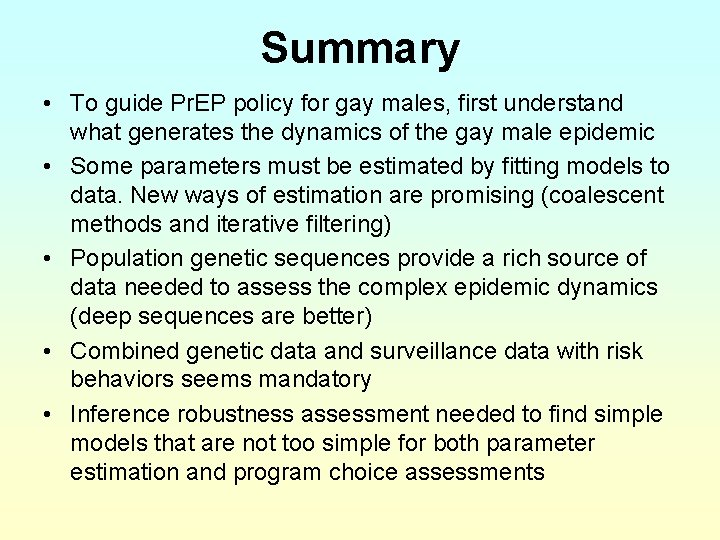
Summary • To guide Pr. EP policy for gay males, first understand what generates the dynamics of the gay male epidemic • Some parameters must be estimated by fitting models to data. New ways of estimation are promising (coalescent methods and iterative filtering) • Population genetic sequences provide a rich source of data needed to assess the complex epidemic dynamics (deep sequences are better) • Combined genetic data and surveillance data with risk behaviors seems mandatory • Inference robustness assessment needed to find simple models that are not too simple for both parameter estimation and program choice assessments
Character traits of the giver
How are observations and inferences different
Mood passages examples
Inferring networks of diffusion and influence
Prediction reading strategy
Making inferences
Inferring rudimentary rules
Inference charades
Inferring
What causes a branch in a cladogram
Semi modals
Kontinuitetshantering
Novell typiska drag
Tack för att ni lyssnade bild
Ekologiskt fotavtryck
Varför kallas perioden 1918-1939 för mellankrigstiden?
En lathund för arbete med kontinuitetshantering
Särskild löneskatt för pensionskostnader
Tidbok
Sura för anatom
Vad är densitet
Datorkunskap för nybörjare
Tack för att ni lyssnade bild
Att skriva en debattartikel
Autokratiskt ledarskap
Nyckelkompetenser för livslångt lärande
Påbyggnader för flakfordon
Formel för lufttryck
Offentlig förvaltning
Kyssande vind
Presentera för publik crossboss
Vad är ett minoritetsspråk