Chapter 4 CTT and Linear Structural Equation Models
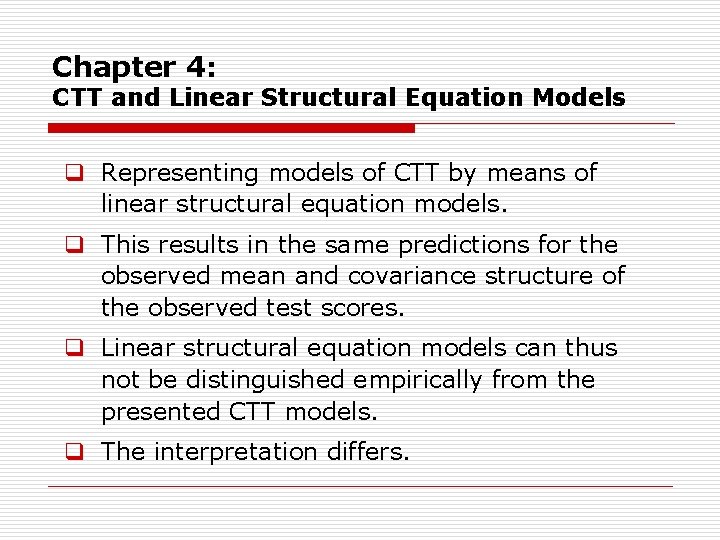
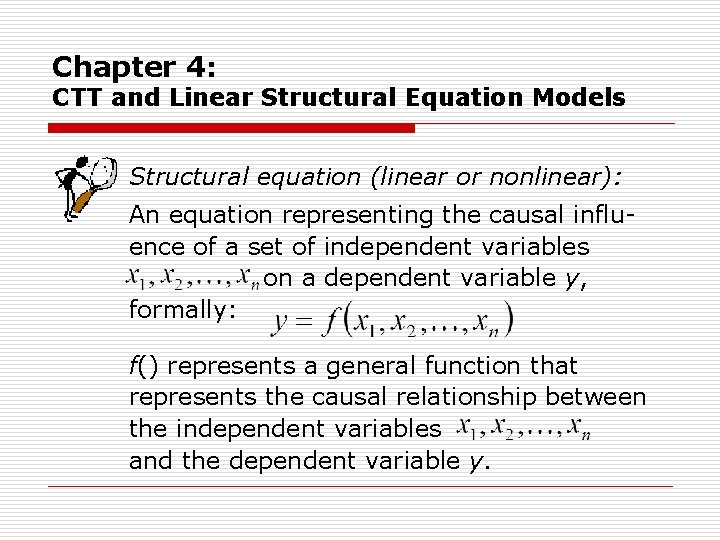
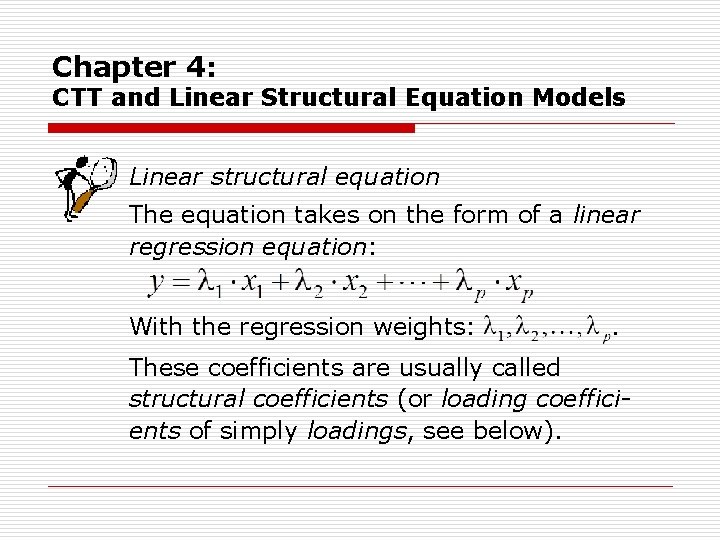
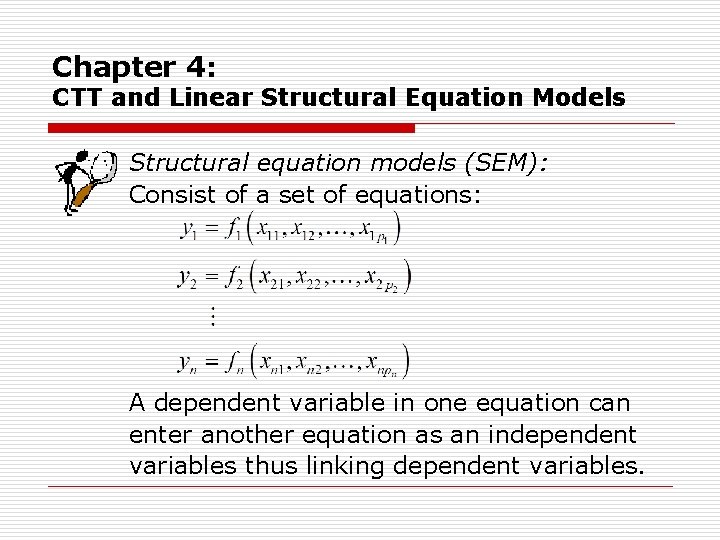
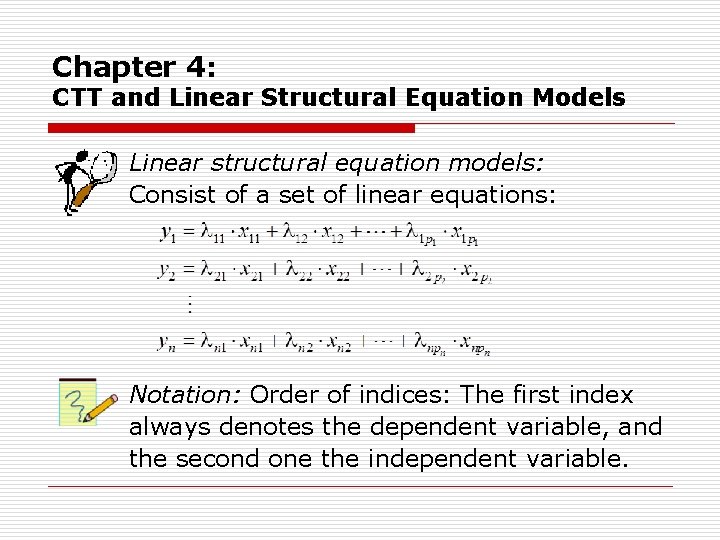
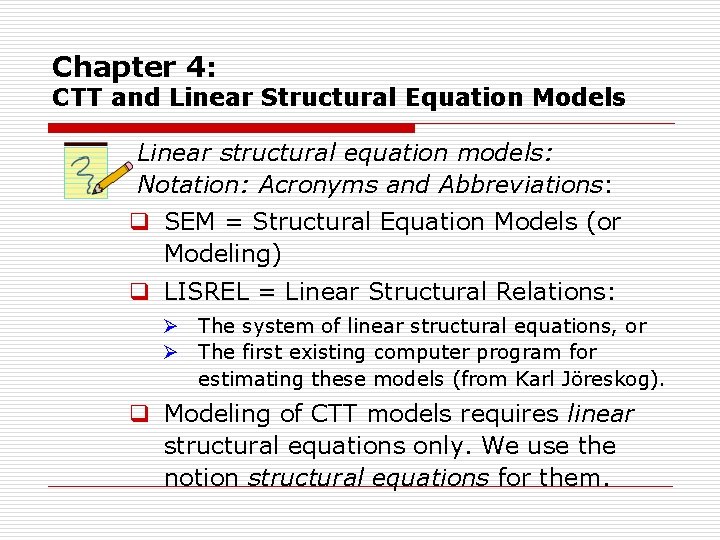
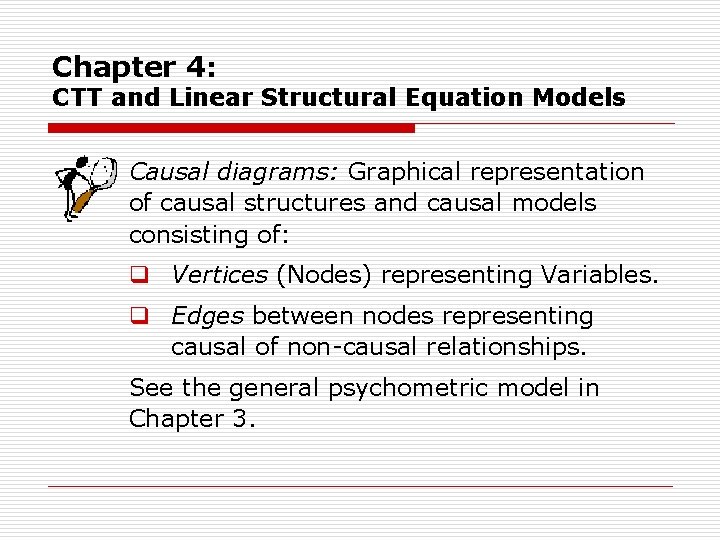
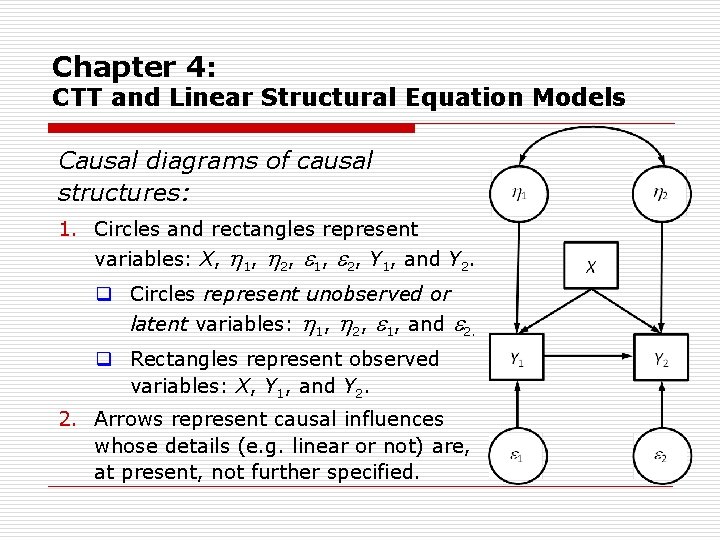
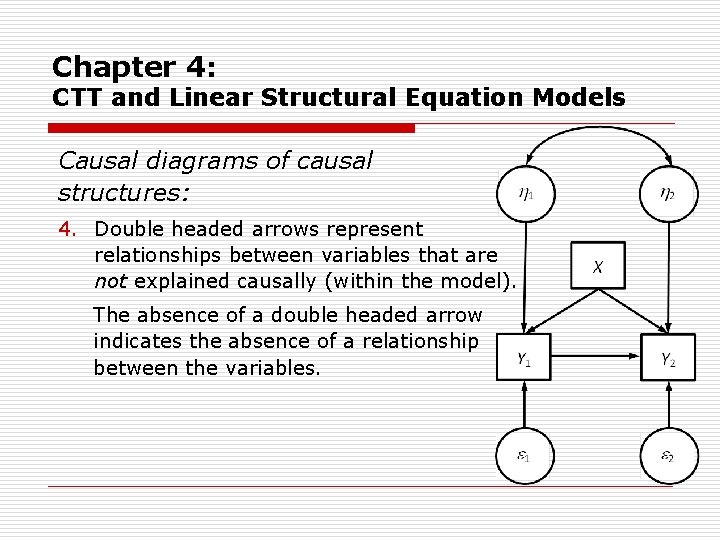
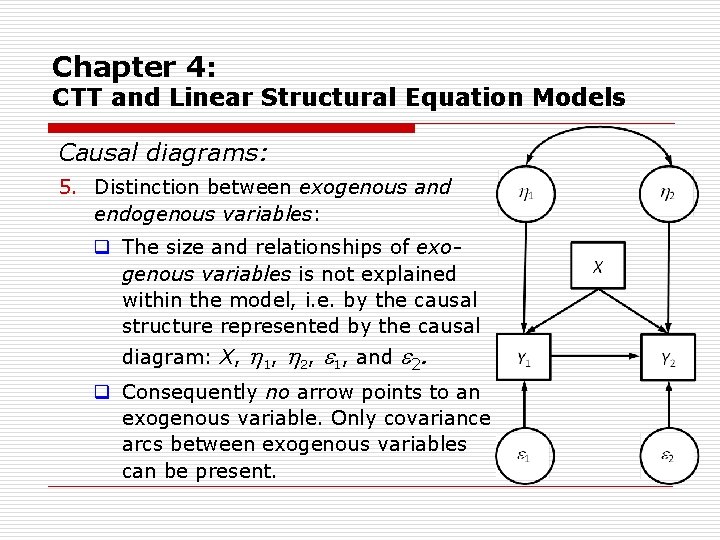
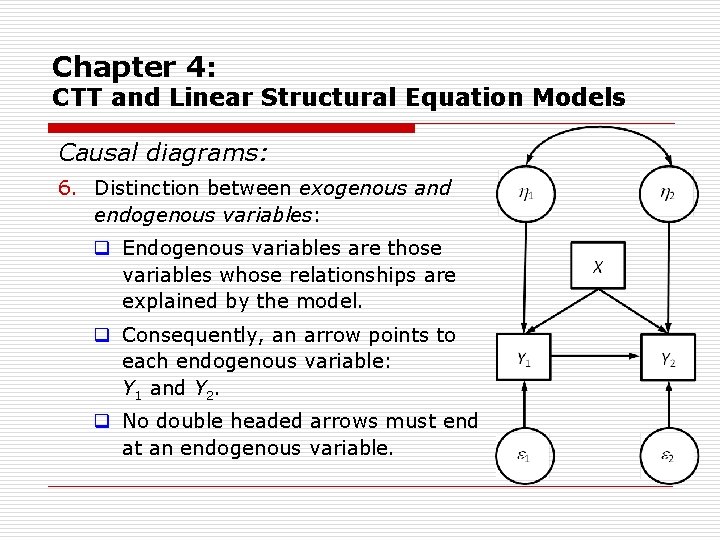
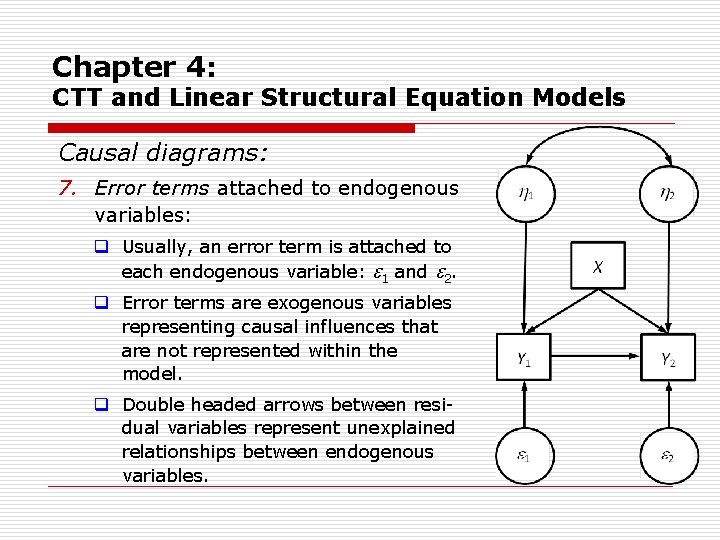
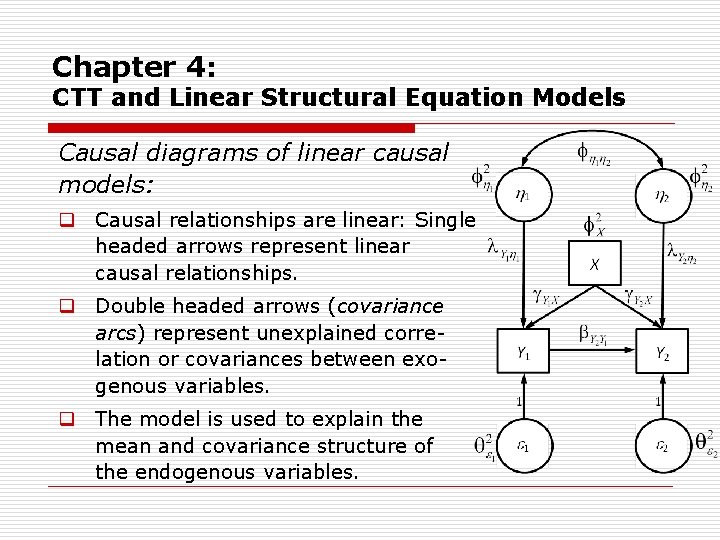
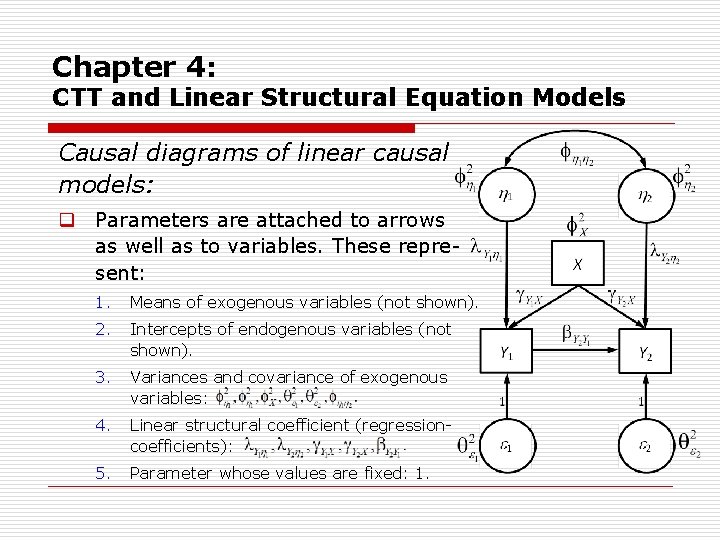
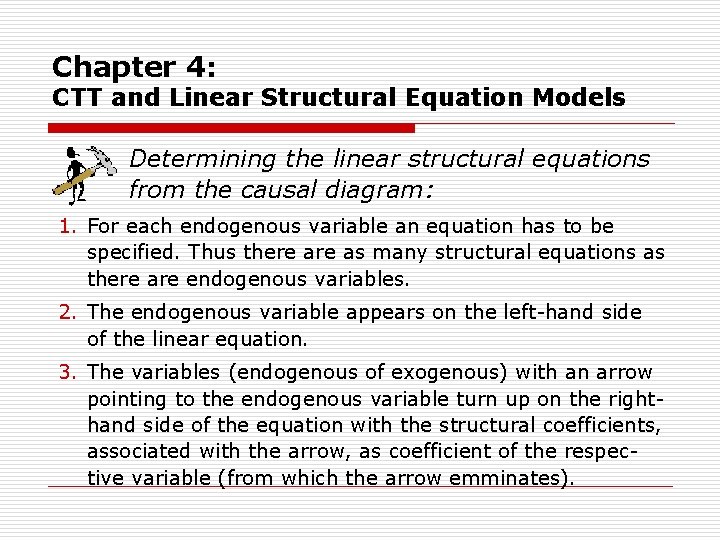
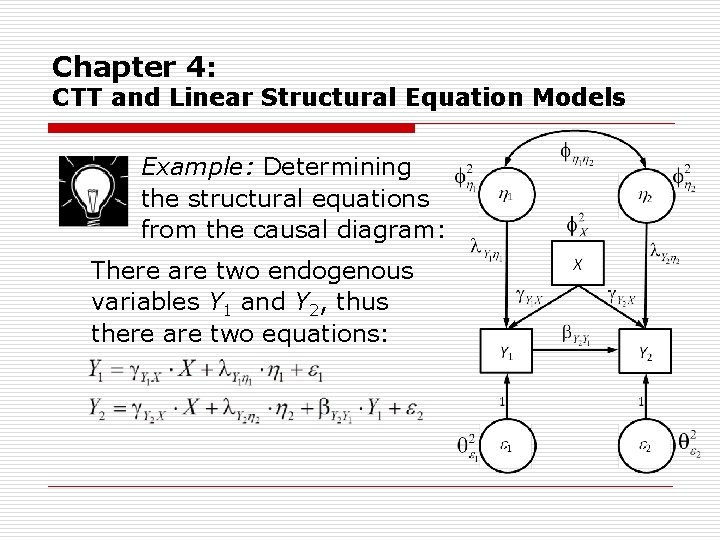
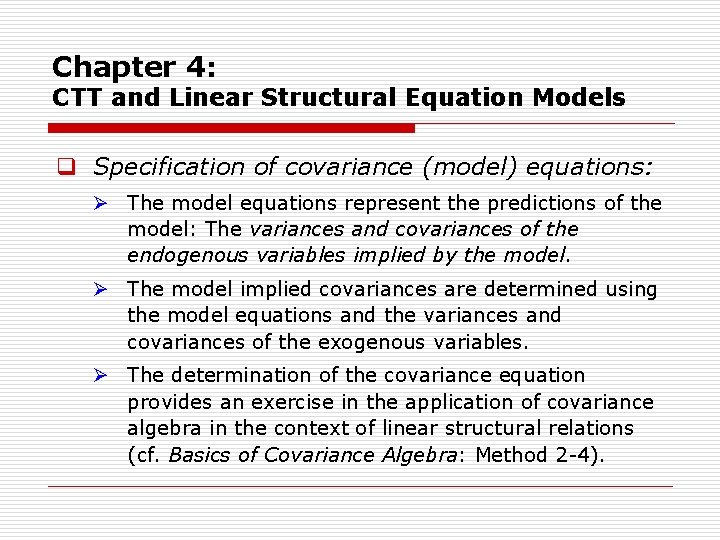
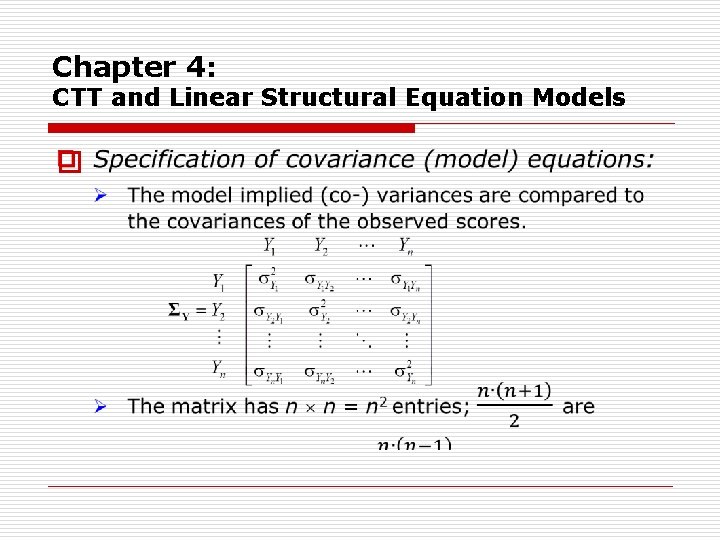
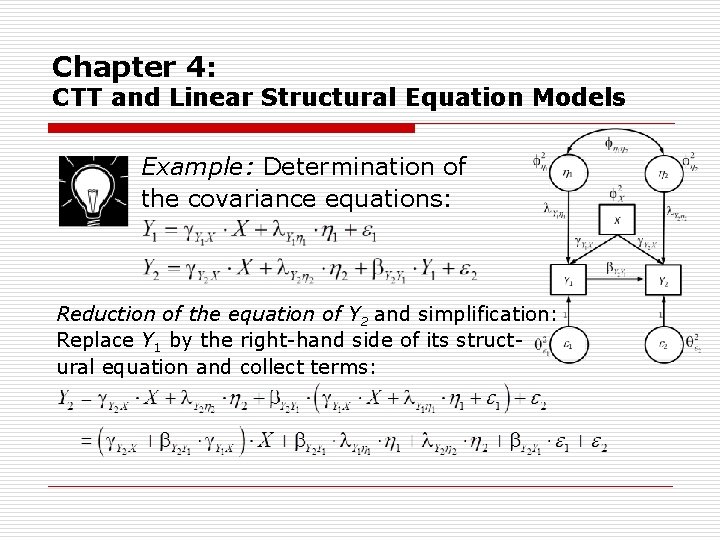
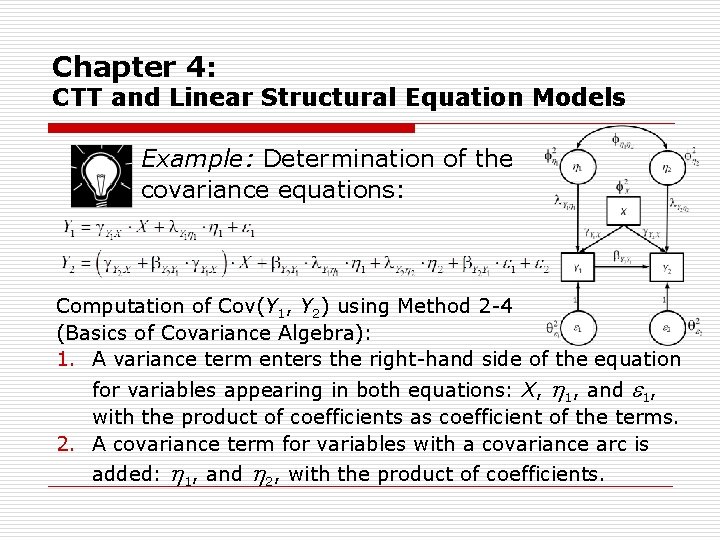
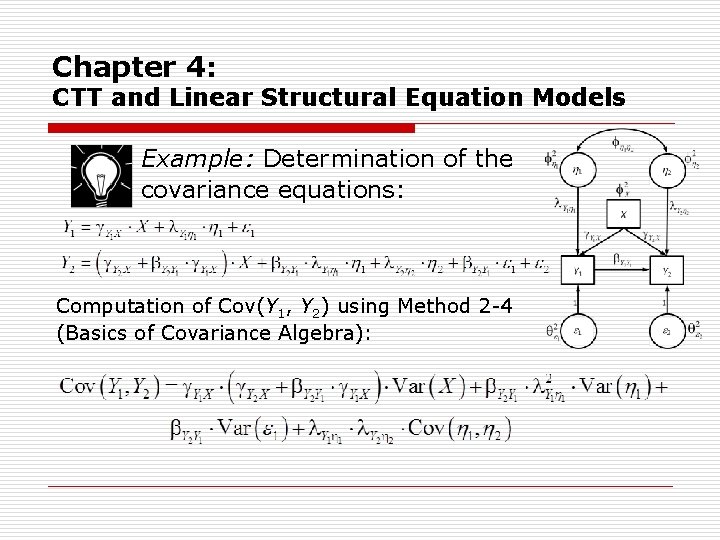
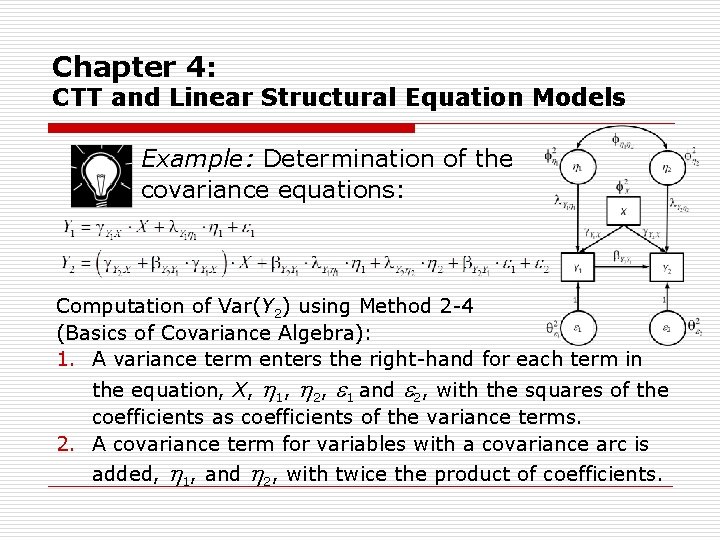
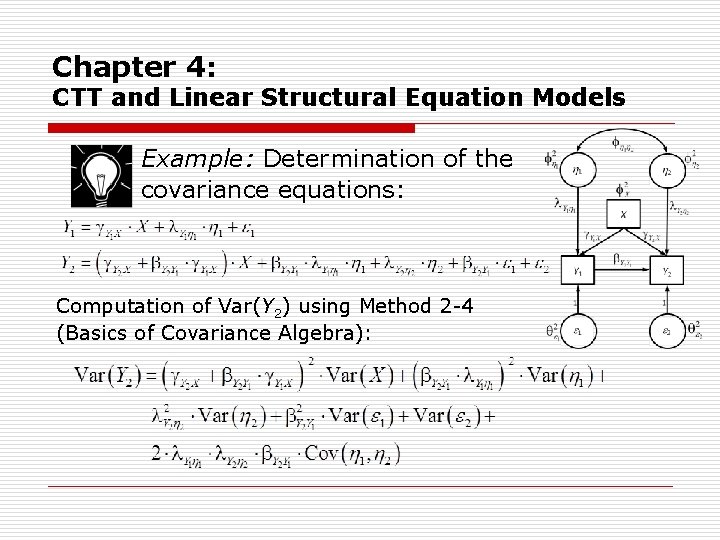
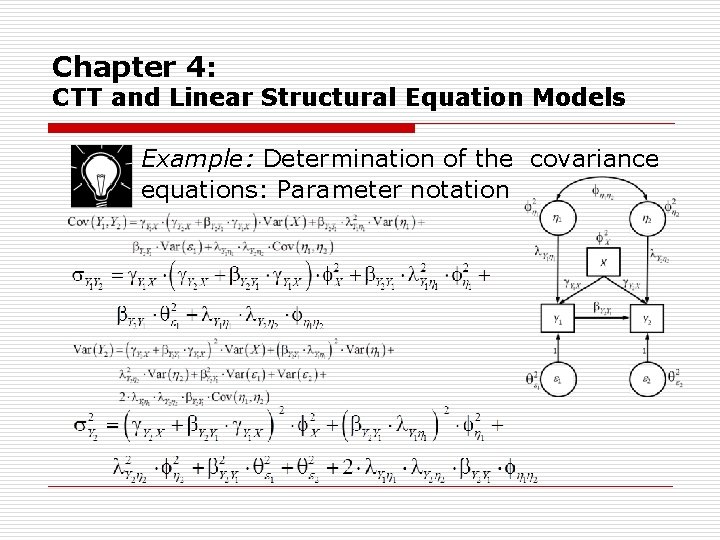
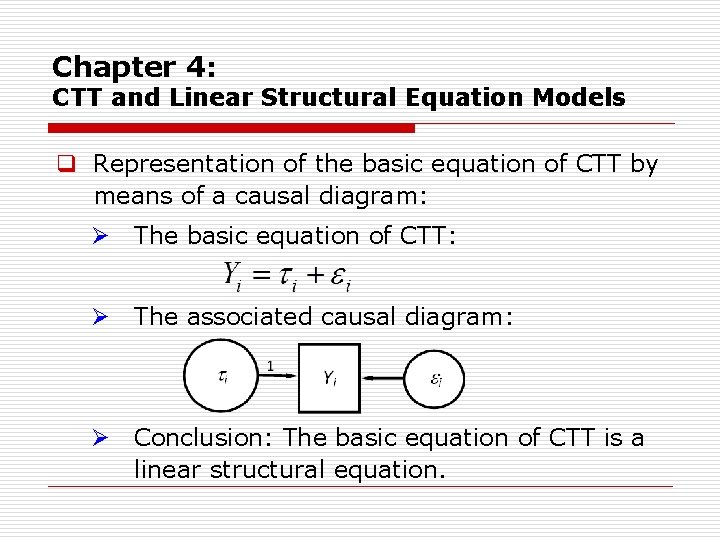
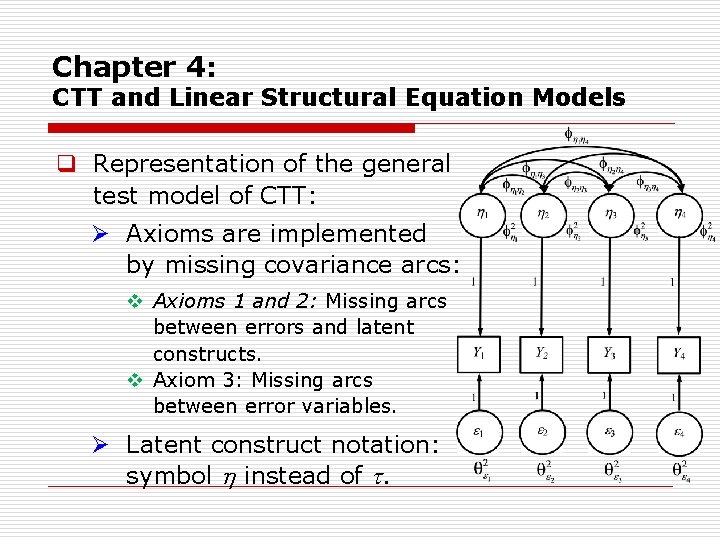
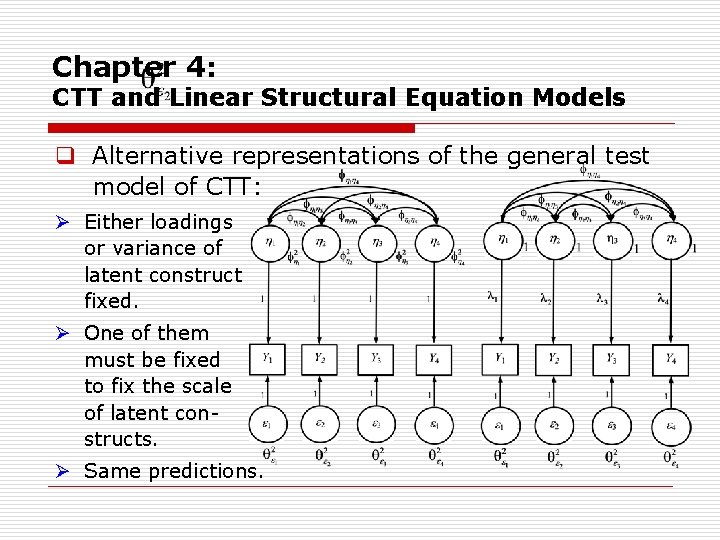
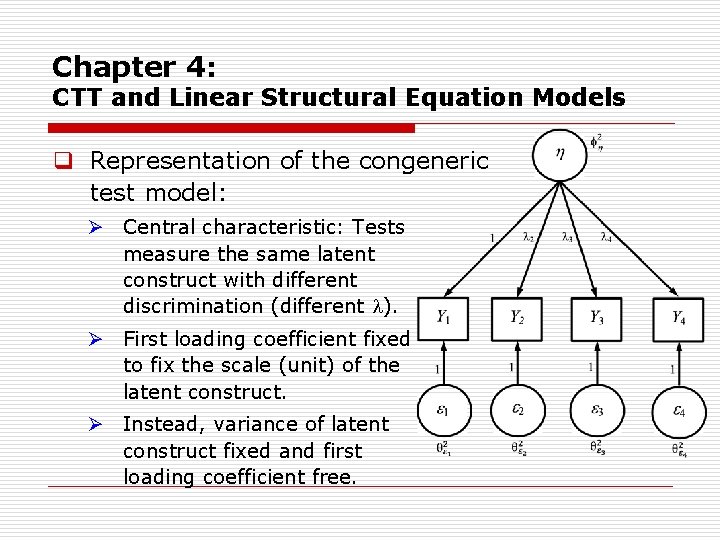
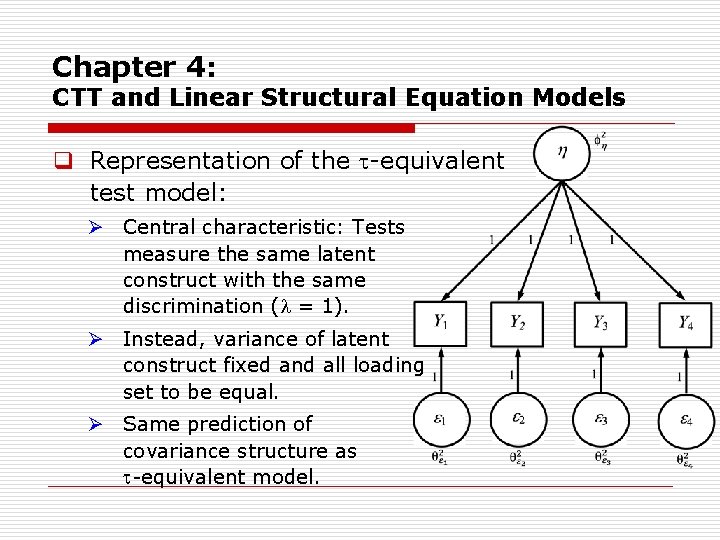
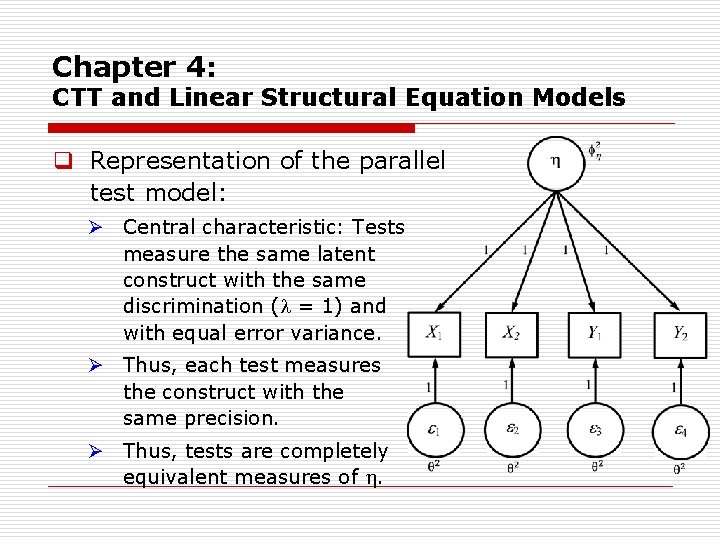
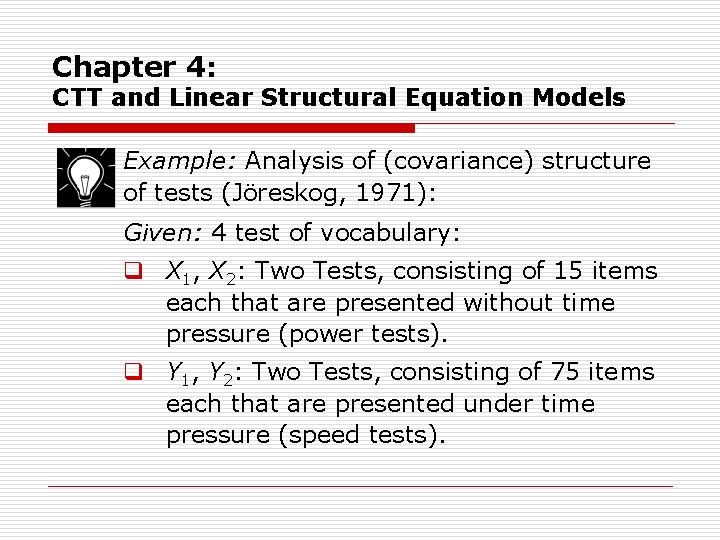
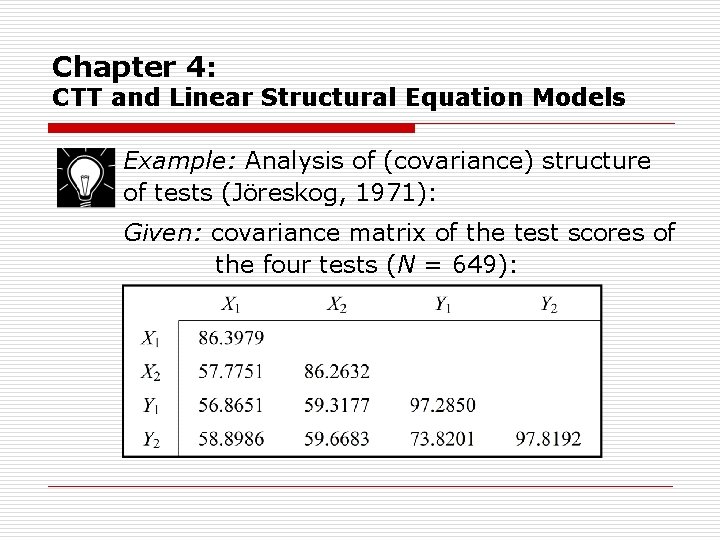
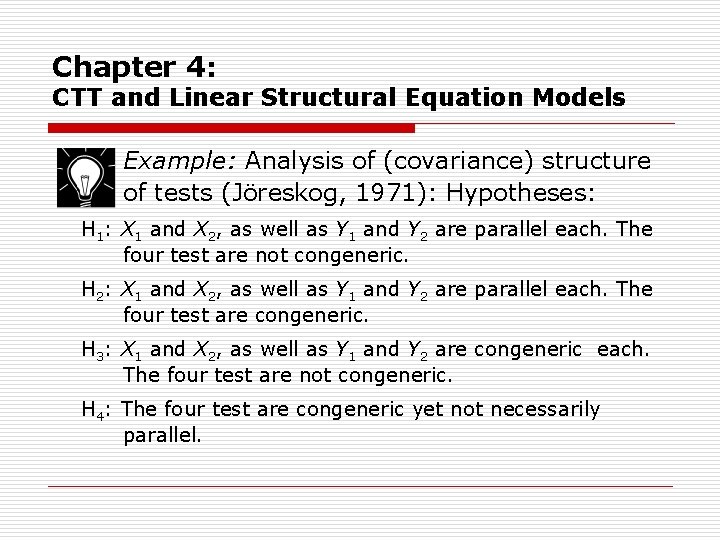
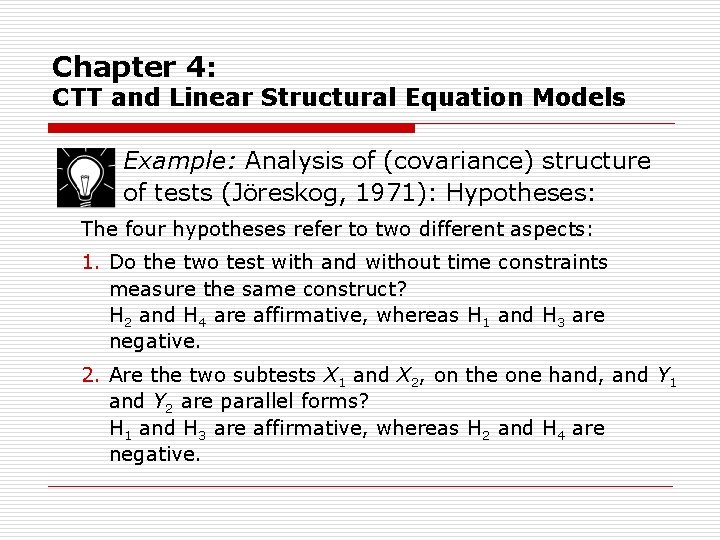
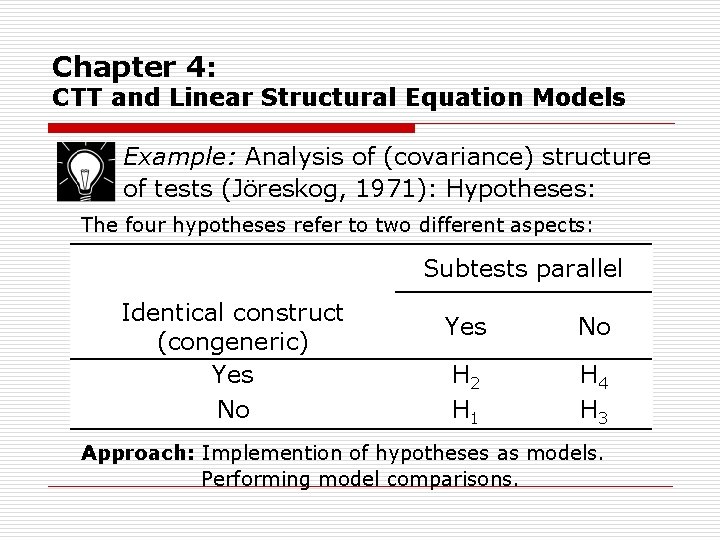
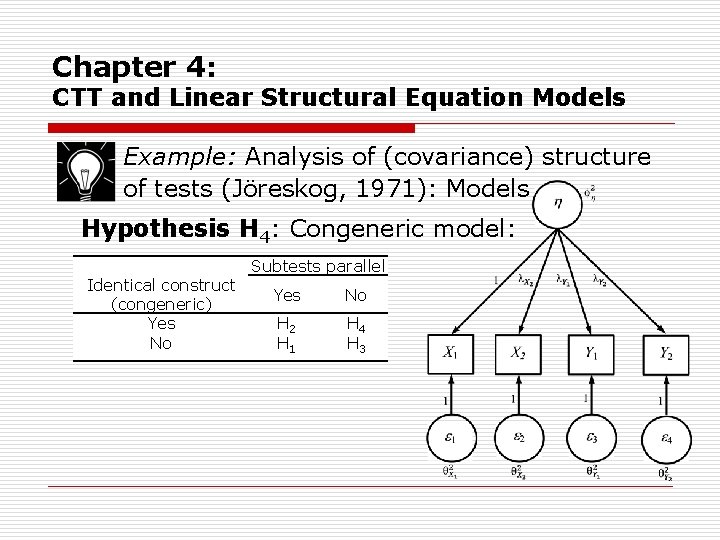
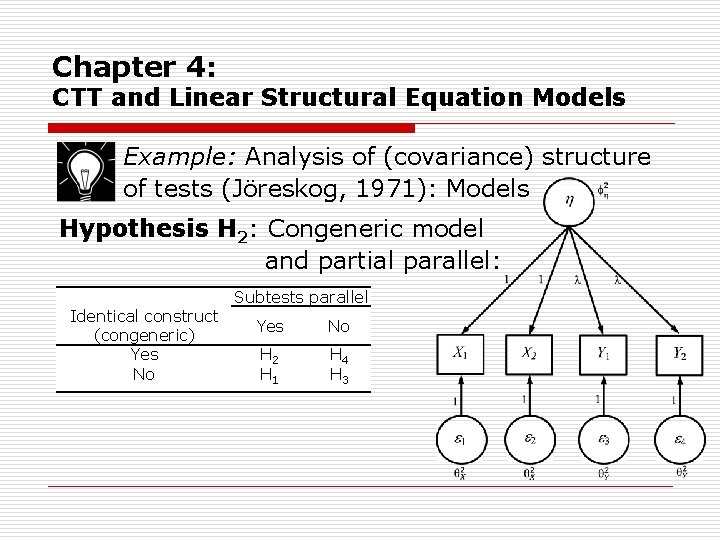
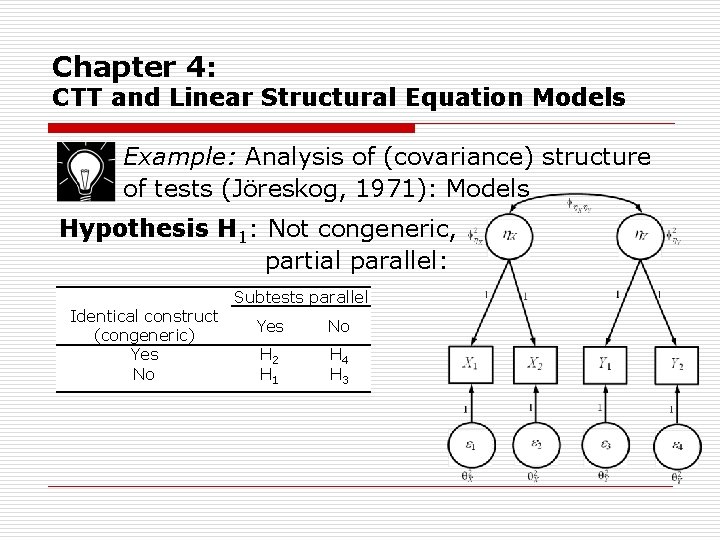
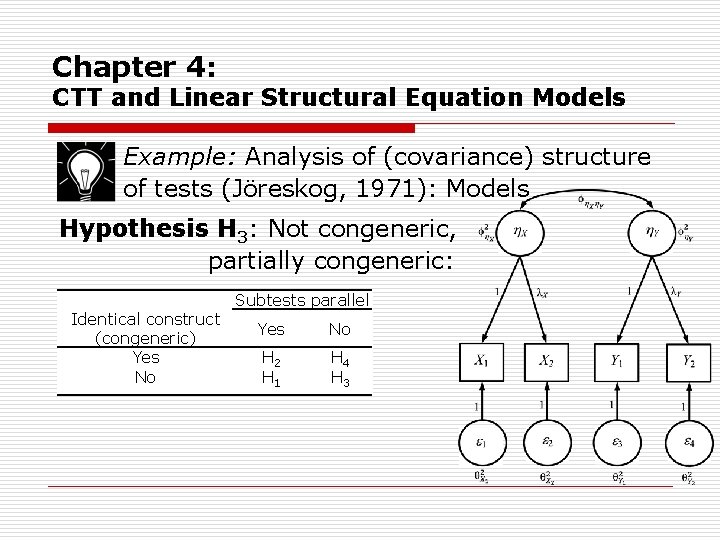
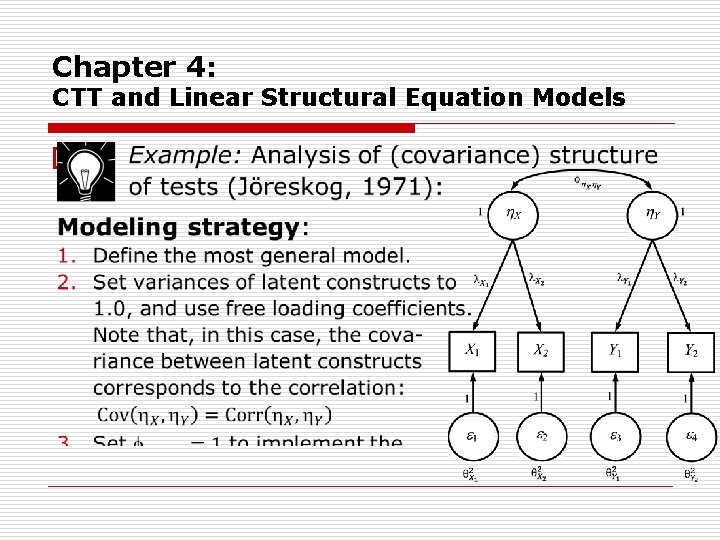
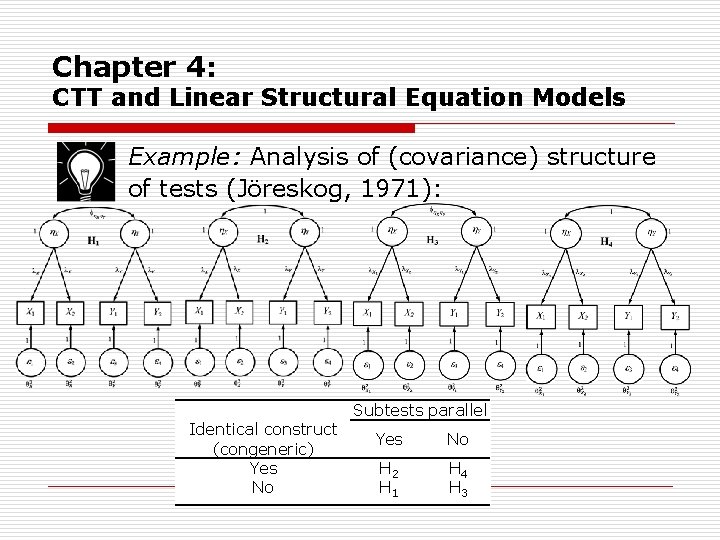
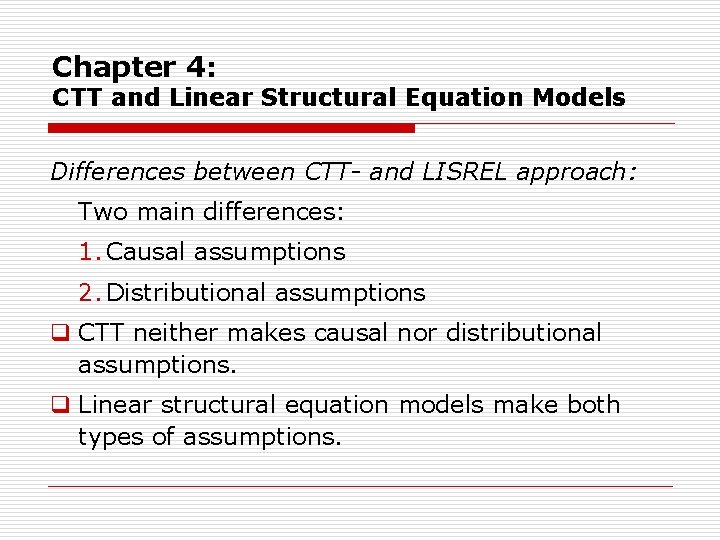
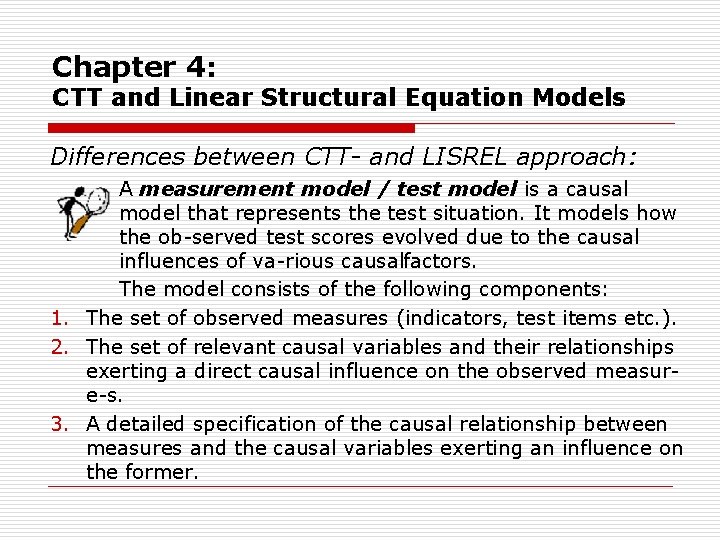
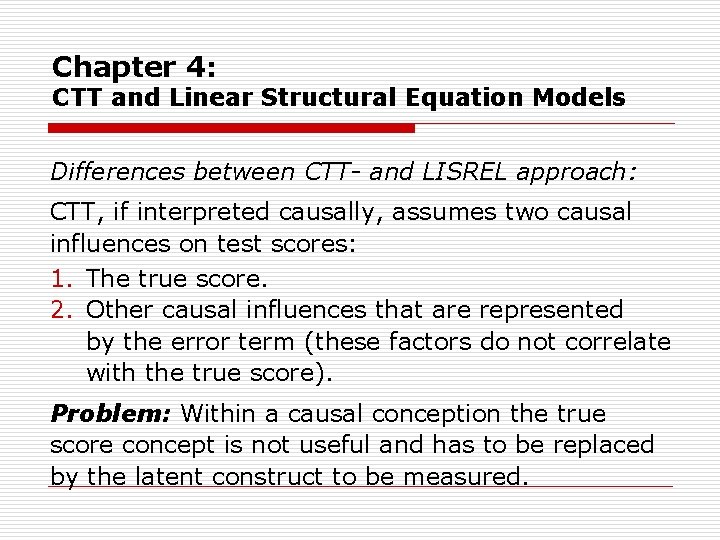
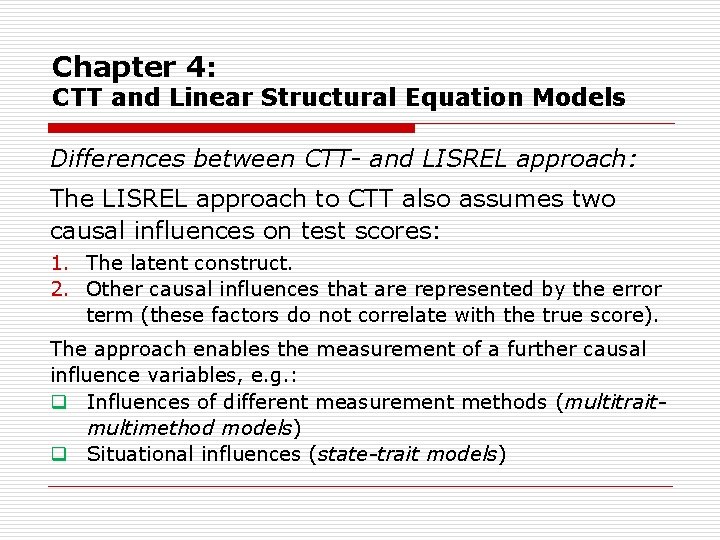
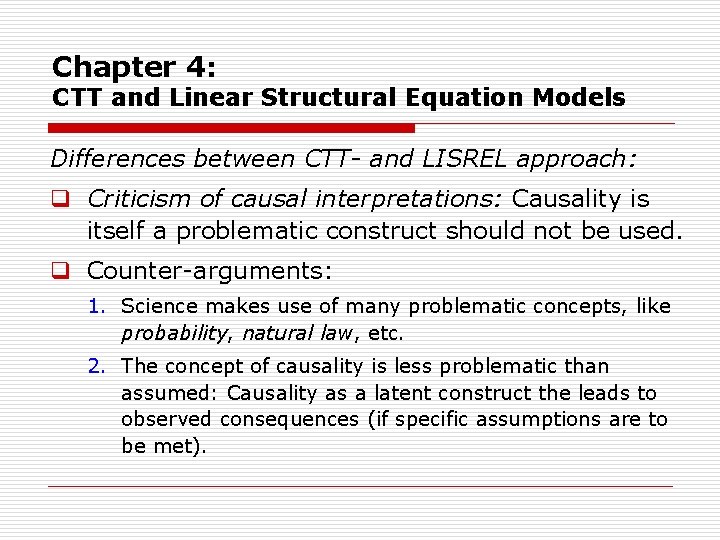
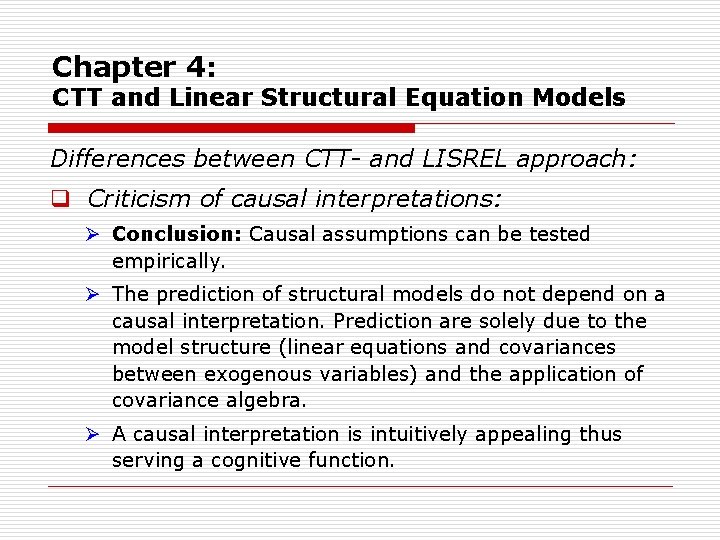
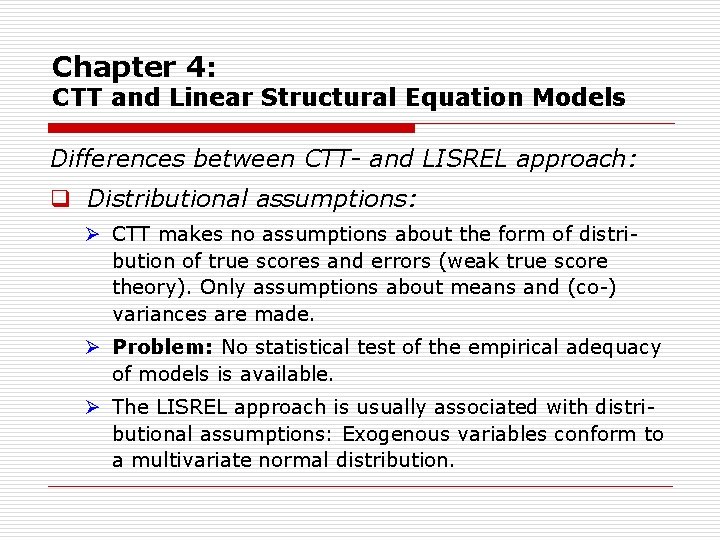
- Slides: 48
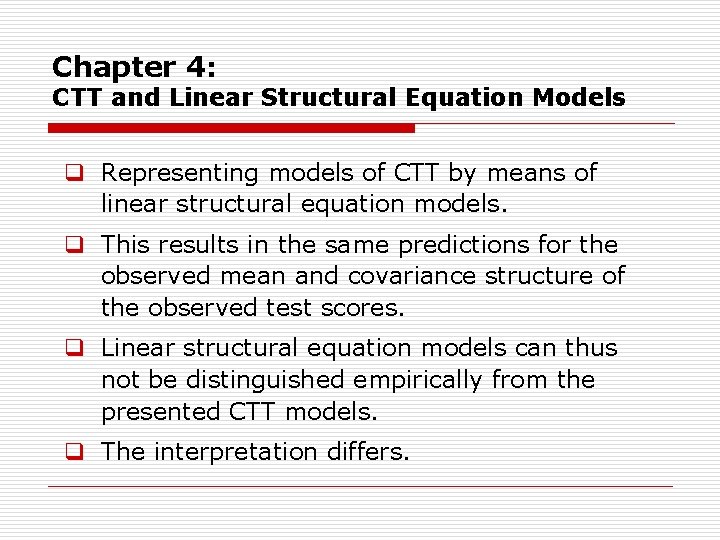
Chapter 4: CTT and Linear Structural Equation Models q Representing models of CTT by means of linear structural equation models. q This results in the same predictions for the observed mean and covariance structure of the observed test scores. q Linear structural equation models can thus not be distinguished empirically from the presented CTT models. q The interpretation differs.
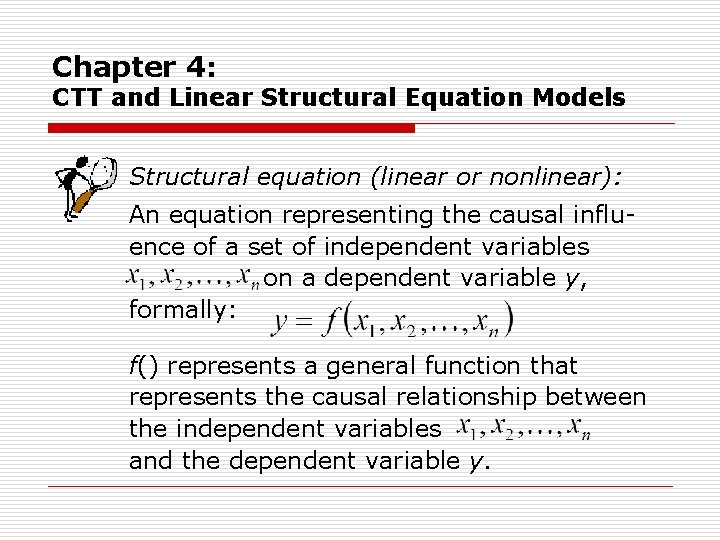
Chapter 4: CTT and Linear Structural Equation Models Structural equation (linear or nonlinear): An equation representing the causal influ ence of a set of independent variables on a dependent variable y, formally: f() represents a general function that represents the causal relationship between the independent variables and the dependent variable y.
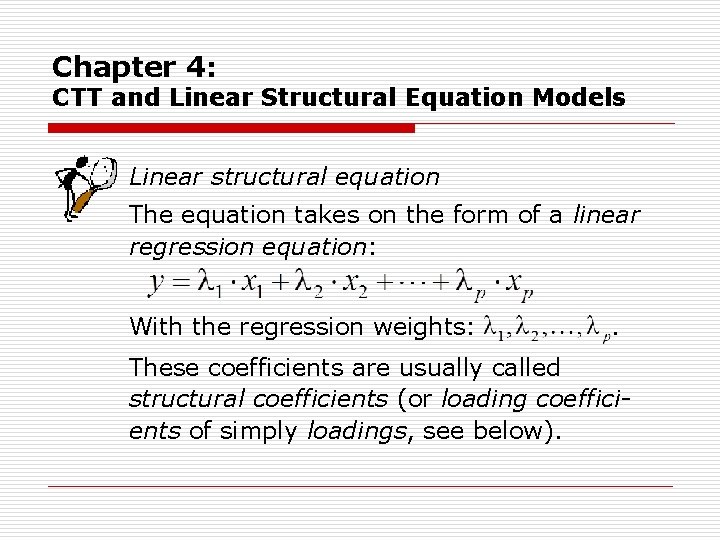
Chapter 4: CTT and Linear Structural Equation Models Linear structural equation The equation takes on the form of a linear regression equation: With the regression weights: . These coefficients are usually called structural coefficients (or loading coefficients of simply loadings, see below).
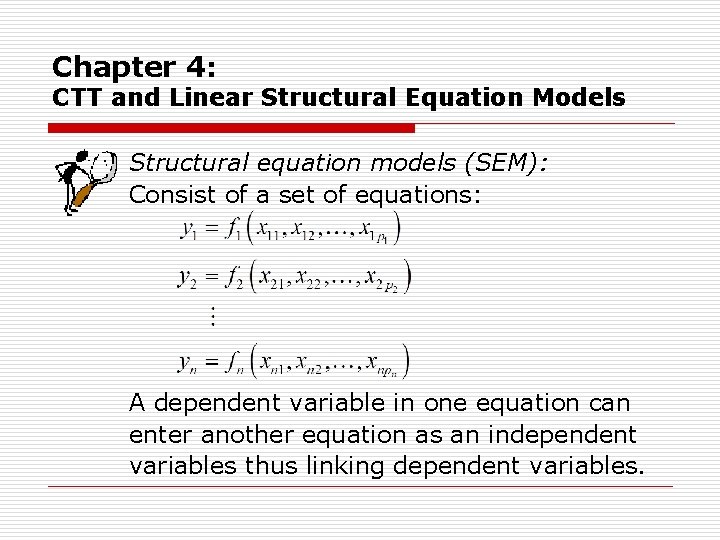
Chapter 4: CTT and Linear Structural Equation Models Structural equation models (SEM): Consist of a set of equations: A dependent variable in one equation can enter another equation as an independent variables thus linking dependent variables.
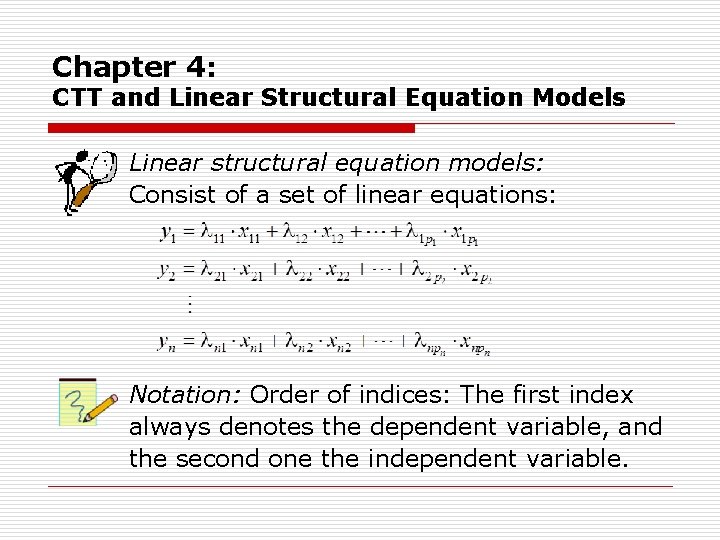
Chapter 4: CTT and Linear Structural Equation Models Linear structural equation models: Consist of a set of linear equations: Notation: Order of indices: The first index always denotes the dependent variable, and the second one the independent variable.
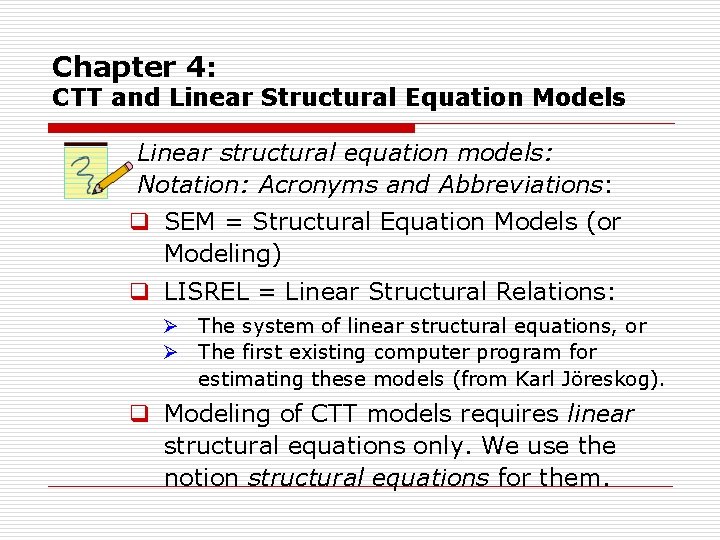
Chapter 4: CTT and Linear Structural Equation Models Linear structural equation models: Notation: Acronyms and Abbreviations: q SEM = Structural Equation Models (or Modeling) q LISREL = Linear Structural Relations: Ø The system of linear structural equations, or Ø The first existing computer program for estimating these models (from Karl Jöreskog). q Modeling of CTT models requires linear structural equations only. We use the notion structural equations for them.
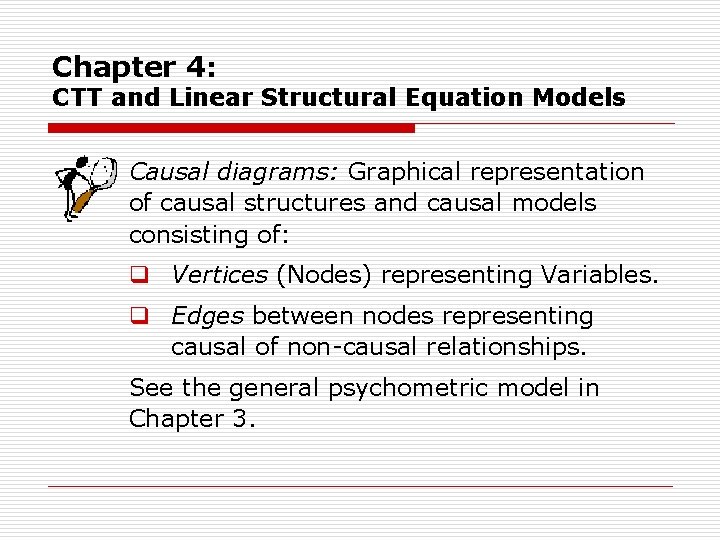
Chapter 4: CTT and Linear Structural Equation Models Causal diagrams: Graphical representation of causal structures and causal models consisting of: q Vertices (Nodes) representing Variables. q Edges between nodes representing causal of non causal relationships. See the general psychometric model in Chapter 3.
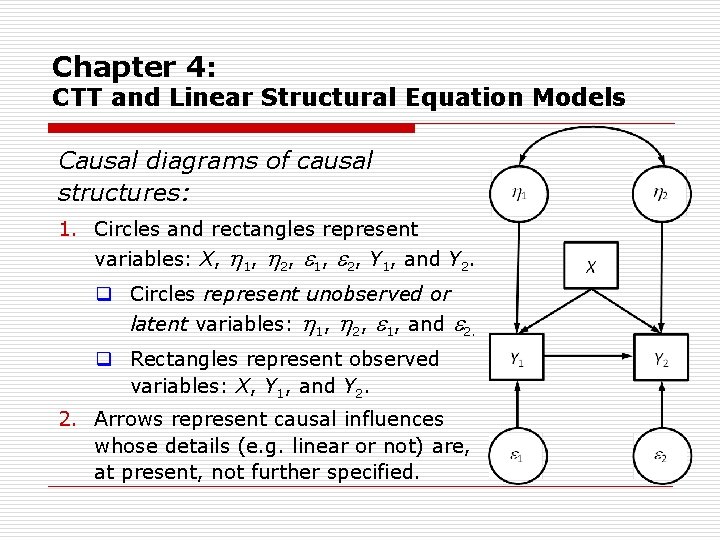
Chapter 4: CTT and Linear Structural Equation Models Causal diagrams of causal structures: 1. Circles and rectangles represent variables: X, 1, 2, Y 1, and Y 2. q Circles represent unobserved or latent variables: 1, 2, 1, and 2. q Rectangles represent observed variables: X, Y 1, and Y 2. 2. Arrows represent causal influences whose details (e. g. linear or not) are, at present, not further specified.
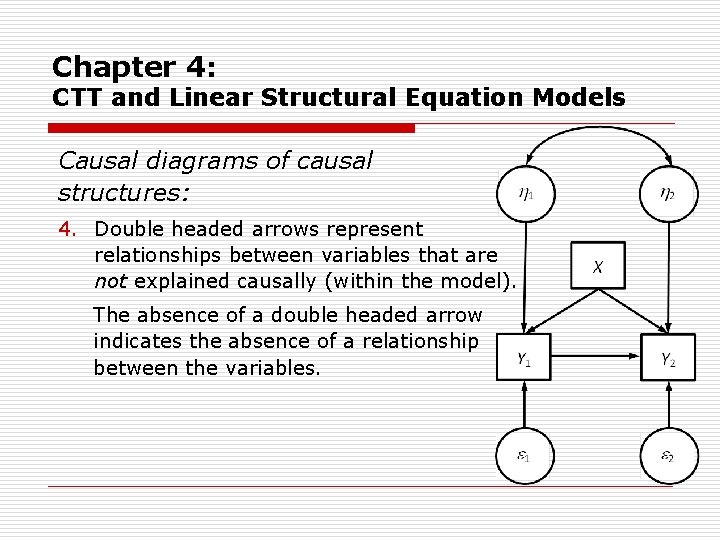
Chapter 4: CTT and Linear Structural Equation Models Causal diagrams of causal structures: 4. Double headed arrows represent relationships between variables that are not explained causally (within the model). The absence of a double headed arrow indicates the absence of a relationship between the variables.
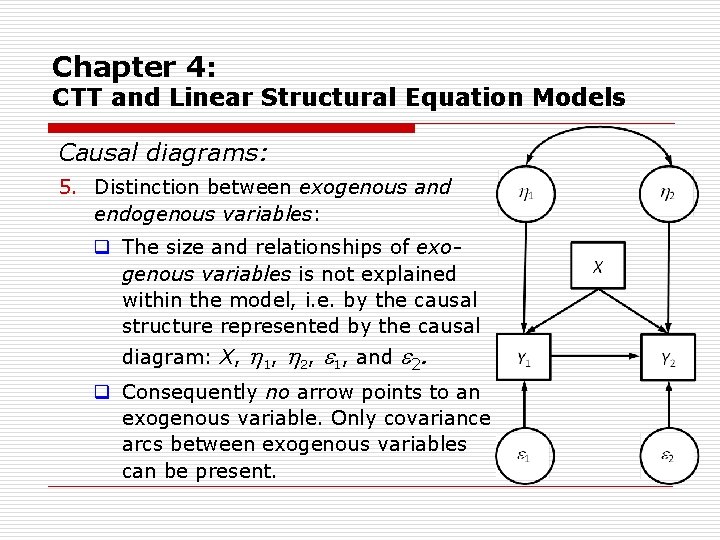
Chapter 4: CTT and Linear Structural Equation Models Causal diagrams: 5. Distinction between exogenous and endogenous variables: q The size and relationships of exogenous variables is not explained within the model, i. e. by the causal structure represented by the causal diagram: X, 1, 2, 1, and 2. q Consequently no arrow points to an exogenous variable. Only covariance arcs between exogenous variables can be present.
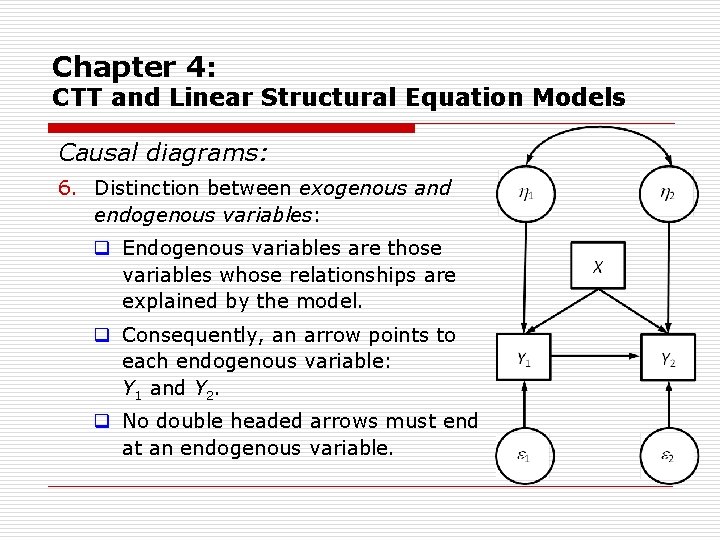
Chapter 4: CTT and Linear Structural Equation Models Causal diagrams: 6. Distinction between exogenous and endogenous variables: q Endogenous variables are those variables whose relationships are explained by the model. q Consequently, an arrow points to each endogenous variable: Y 1 and Y 2. q No double headed arrows must end at an endogenous variable.
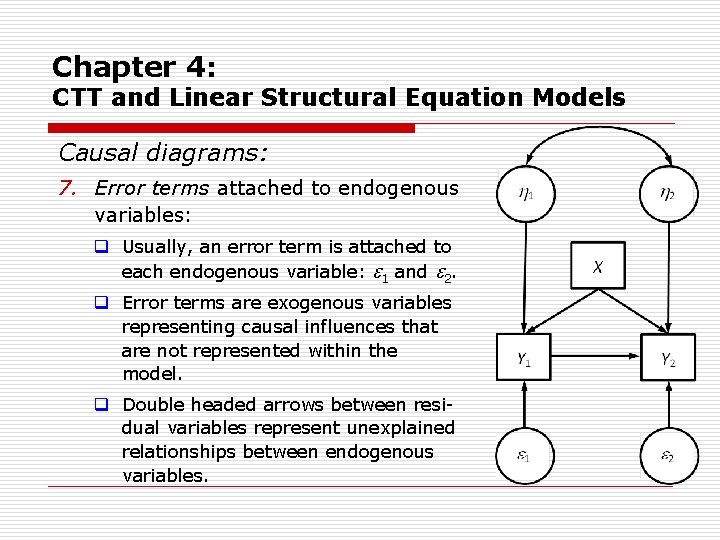
Chapter 4: CTT and Linear Structural Equation Models Causal diagrams: 7. Error terms attached to endogenous variables: q Usually, an error term is attached to each endogenous variable: 1 and 2. q Error terms are exogenous variables representing causal influences that are not represented within the model. q Double headed arrows between resi dual variables represent unexplained relationships between endogenous variables.
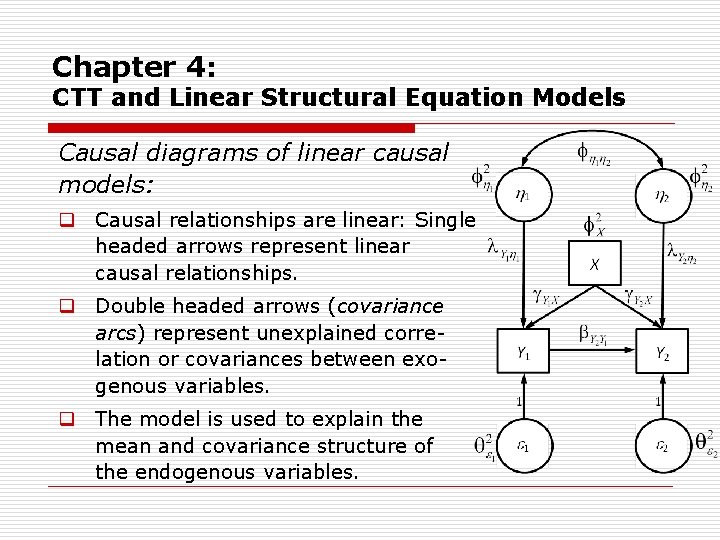
Chapter 4: CTT and Linear Structural Equation Models Causal diagrams of linear causal models: q Causal relationships are linear: Single headed arrows represent linear causal relationships. q Double headed arrows (covariance arcs) represent unexplained corre lation or covariances between exo genous variables. q The model is used to explain the mean and covariance structure of the endogenous variables.
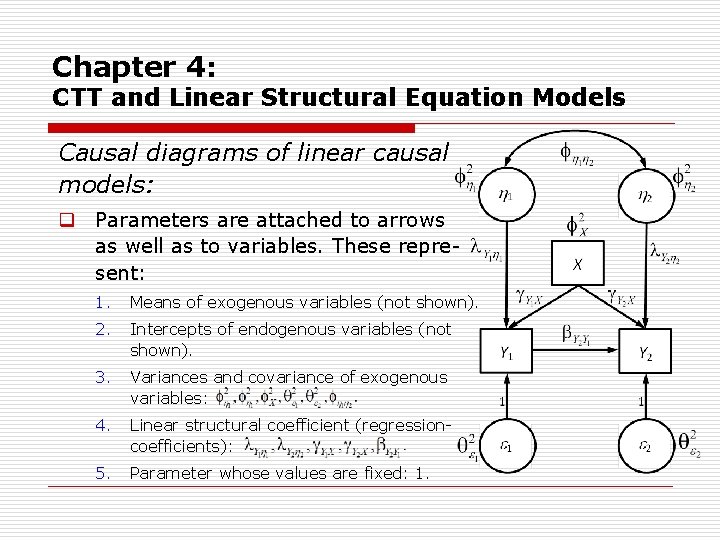
Chapter 4: CTT and Linear Structural Equation Models Causal diagrams of linear causal models: q Parameters are attached to arrows as well as to variables. These repre sent: 1. Means of exogenous variables (not shown). 2. Intercepts of endogenous variables (not shown). 3. Variances and covariance of exogenous variables: 4. Linear structural coefficient (regression coefficients): 5. Parameter whose values are fixed: 1.
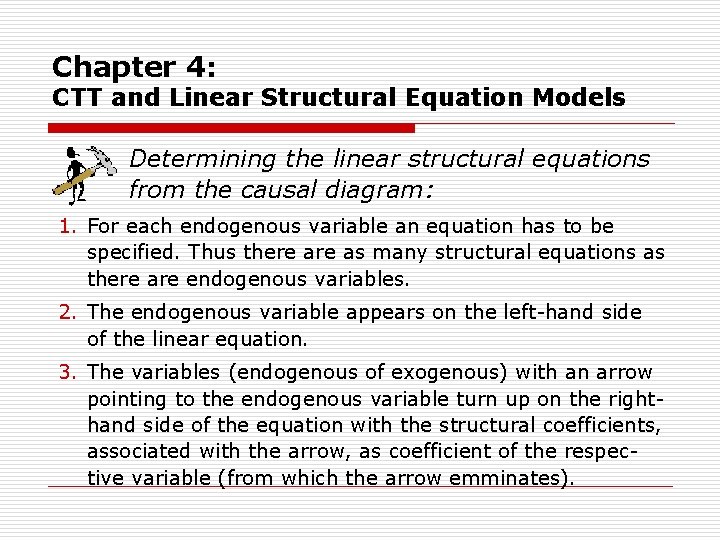
Chapter 4: CTT and Linear Structural Equation Models Determining the linear structural equations from the causal diagram: 1. For each endogenous variable an equation has to be specified. Thus there as many structural equations as there are endogenous variables. 2. The endogenous variable appears on the left hand side of the linear equation. 3. The variables (endogenous of exogenous) with an arrow pointing to the endogenous variable turn up on the right hand side of the equation with the structural coefficients, associated with the arrow, as coefficient of the respec tive variable (from which the arrow emminates).
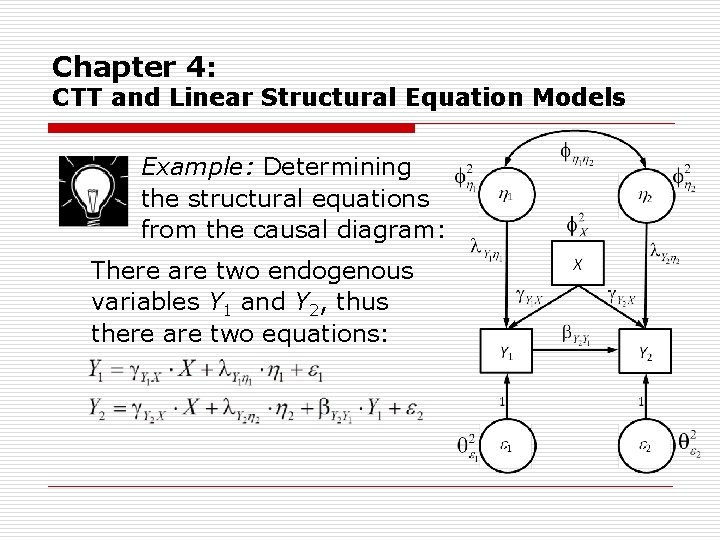
Chapter 4: CTT and Linear Structural Equation Models Example: Determining the structural equations from the causal diagram: There are two endogenous variables Y 1 and Y 2, thus there are two equations:
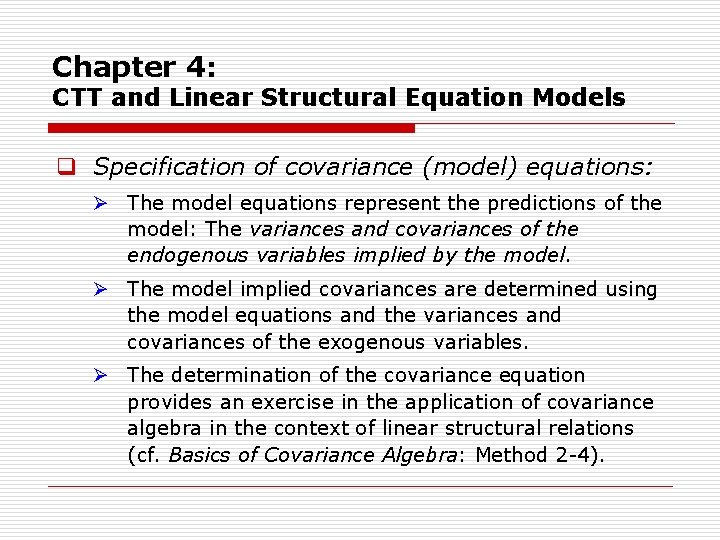
Chapter 4: CTT and Linear Structural Equation Models q Specification of covariance (model) equations: Ø The model equations represent the predictions of the model: The variances and covariances of the endogenous variables implied by the model. Ø The model implied covariances are determined using the model equations and the variances and covariances of the exogenous variables. Ø The determination of the covariance equation provides an exercise in the application of covariance algebra in the context of linear structural relations (cf. Basics of Covariance Algebra: Method 2 4).
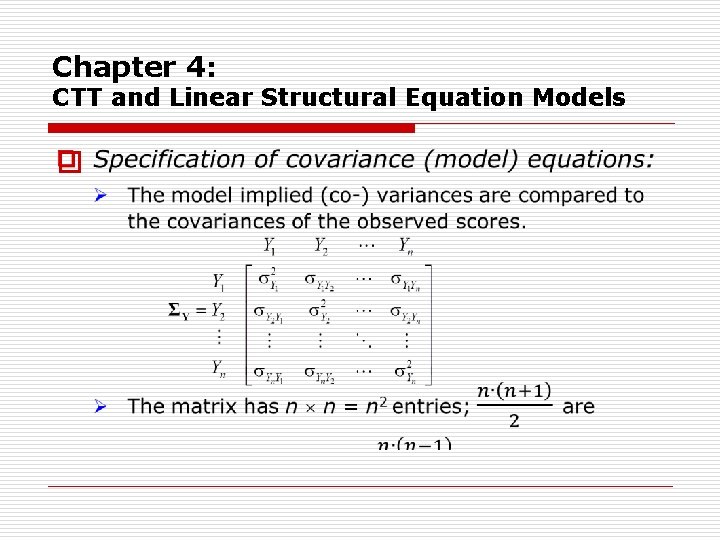
Chapter 4: CTT and Linear Structural Equation Models o
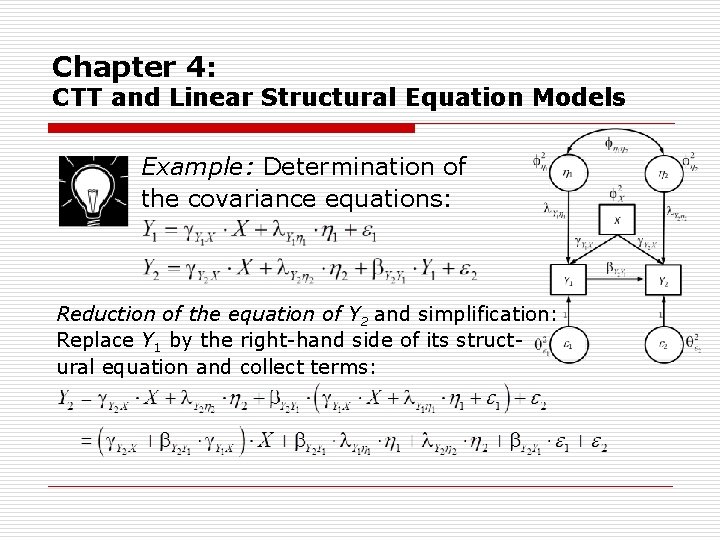
Chapter 4: CTT and Linear Structural Equation Models Example: Determination of the covariance equations: Reduction of the equation of Y 2 and simplification: Replace Y 1 by the right hand side of its struct ural equation and collect terms:
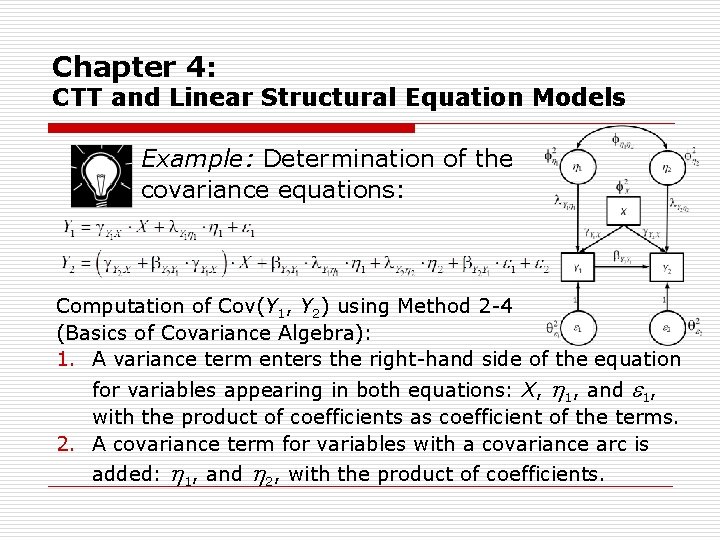
Chapter 4: CTT and Linear Structural Equation Models Example: Determination of the covariance equations: Computation of Cov(Y 1, Y 2) using Method 2 4 (Basics of Covariance Algebra): 1. A variance term enters the right hand side of the equation for variables appearing in both equations: X, 1, and 1, with the product of coefficients as coefficient of the terms. 2. A covariance term for variables with a covariance arc is added: 1, and 2, with the product of coefficients.
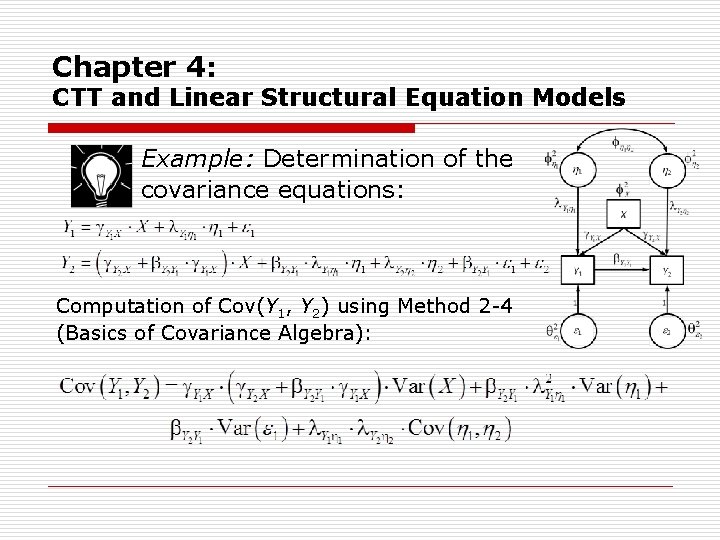
Chapter 4: CTT and Linear Structural Equation Models Example: Determination of the covariance equations: Computation of Cov(Y 1, Y 2) using Method 2 4 (Basics of Covariance Algebra):
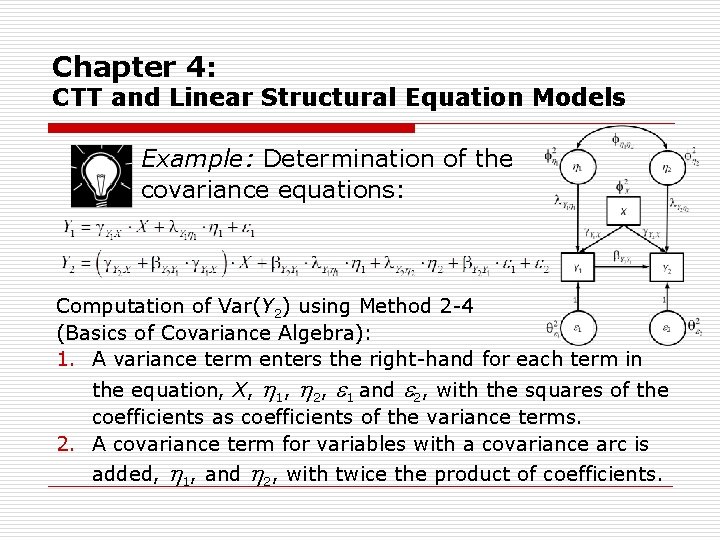
Chapter 4: CTT and Linear Structural Equation Models Example: Determination of the covariance equations: Computation of Var(Y 2) using Method 2 4 (Basics of Covariance Algebra): 1. A variance term enters the right hand for each term in the equation, X, 1, 2, 1 and 2, with the squares of the coefficients as coefficients of the variance terms. 2. A covariance term for variables with a covariance arc is added, 1, and 2, with twice the product of coefficients.
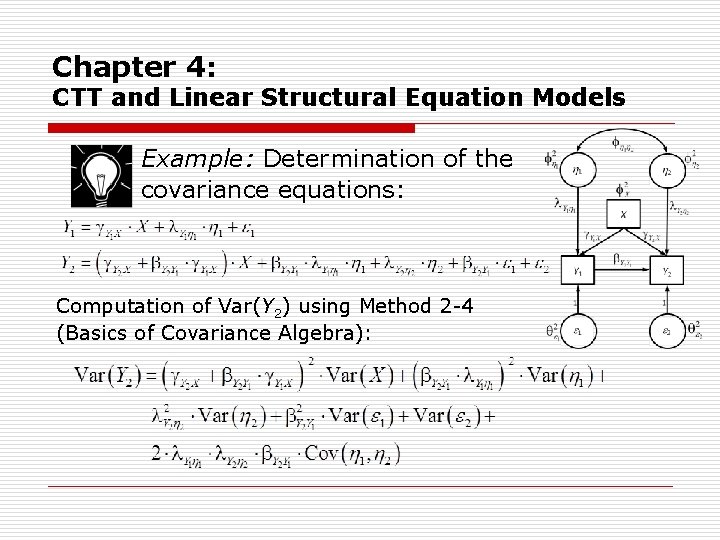
Chapter 4: CTT and Linear Structural Equation Models Example: Determination of the covariance equations: Computation of Var(Y 2) using Method 2 4 (Basics of Covariance Algebra):
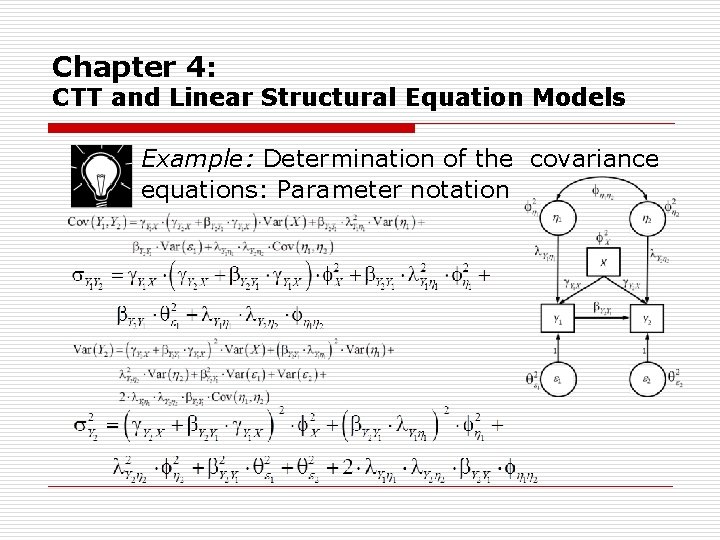
Chapter 4: CTT and Linear Structural Equation Models Example: Determination of the covariance equations: Parameter notation
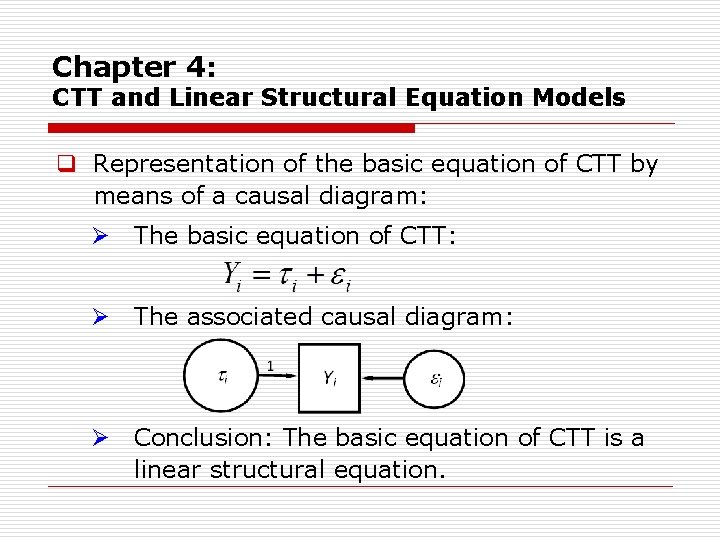
Chapter 4: CTT and Linear Structural Equation Models q Representation of the basic equation of CTT by means of a causal diagram: Ø The basic equation of CTT: Ø The associated causal diagram: Ø Conclusion: The basic equation of CTT is a linear structural equation.
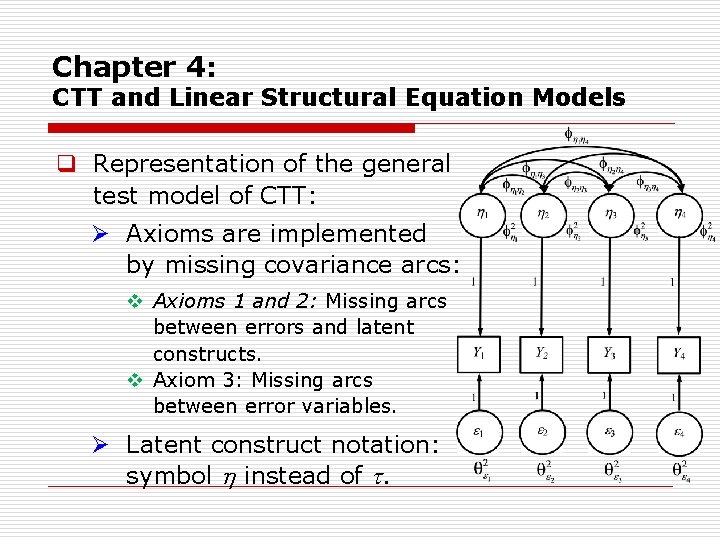
Chapter 4: CTT and Linear Structural Equation Models q Representation of the general test model of CTT: Ø Axioms are implemented by missing covariance arcs: v Axioms 1 and 2: Missing arcs between errors and latent constructs. v Axiom 3: Missing arcs between error variables. Ø Latent construct notation: symbol instead of .
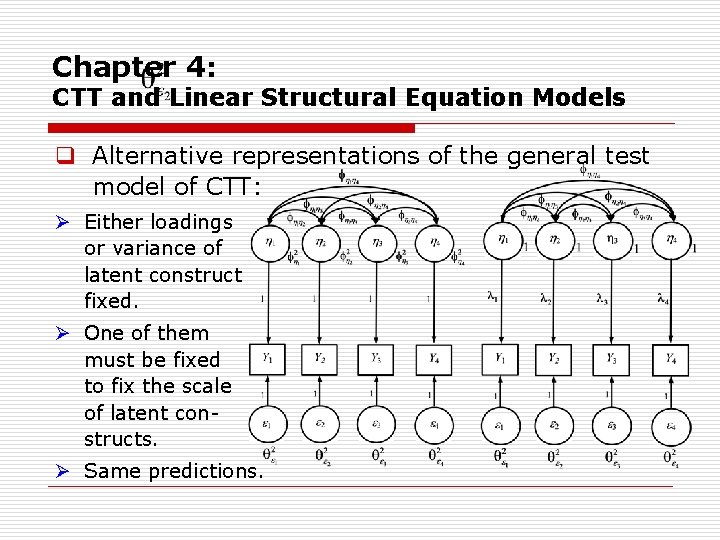
Chapter 4: CTT and Linear Structural Equation Models q Alternative representations of the general test model of CTT: Ø Either loadings or variance of latent construct fixed. Ø One of them must be fixed to fix the scale of latent con structs. Ø Same predictions.
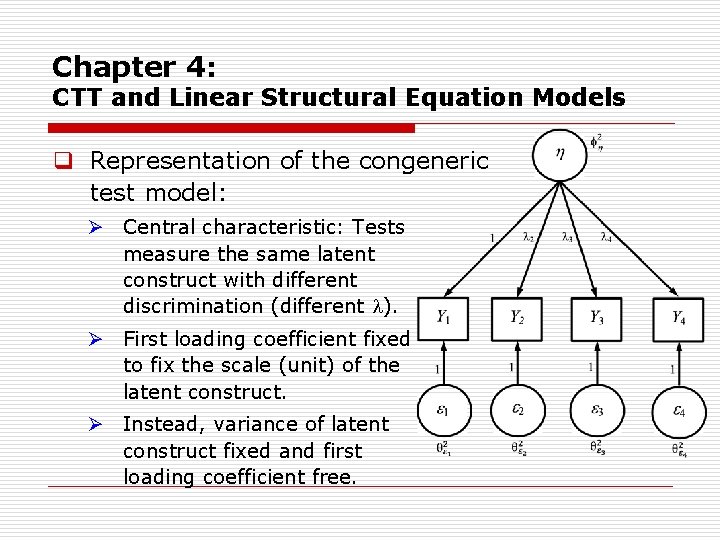
Chapter 4: CTT and Linear Structural Equation Models q Representation of the congeneric test model: Ø Central characteristic: Tests measure the same latent construct with different discrimination (different ). Ø First loading coefficient fixed to fix the scale (unit) of the latent construct. Ø Instead, variance of latent construct fixed and first loading coefficient free.
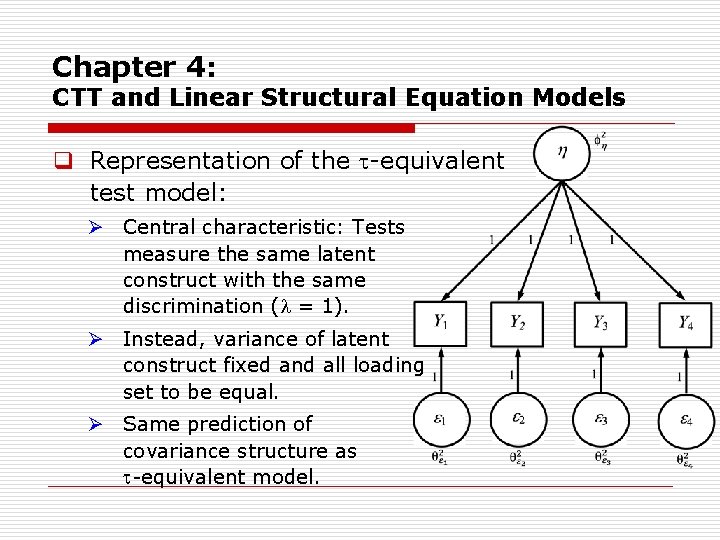
Chapter 4: CTT and Linear Structural Equation Models q Representation of the equivalent test model: Ø Central characteristic: Tests measure the same latent construct with the same discrimination ( = 1). Ø Instead, variance of latent construct fixed and all loading set to be equal. Ø Same prediction of covariance structure as equivalent model.
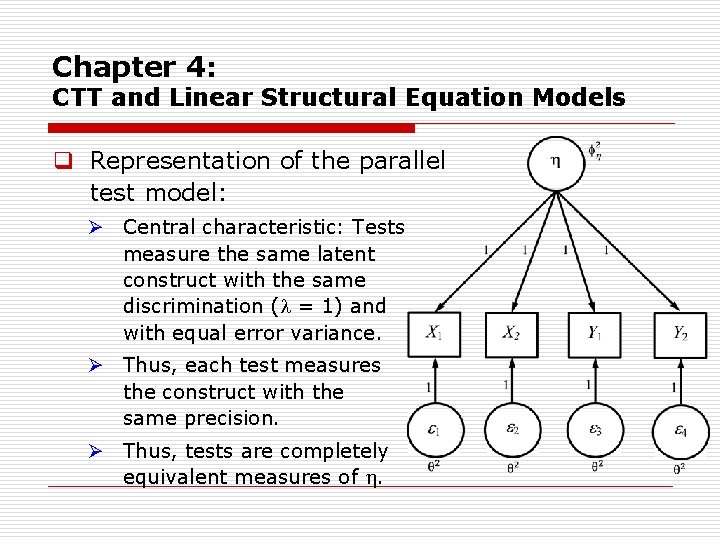
Chapter 4: CTT and Linear Structural Equation Models q Representation of the parallel test model: Ø Central characteristic: Tests measure the same latent construct with the same discrimination ( = 1) and with equal error variance. Ø Thus, each test measures the construct with the same precision. Ø Thus, tests are completely equivalent measures of .
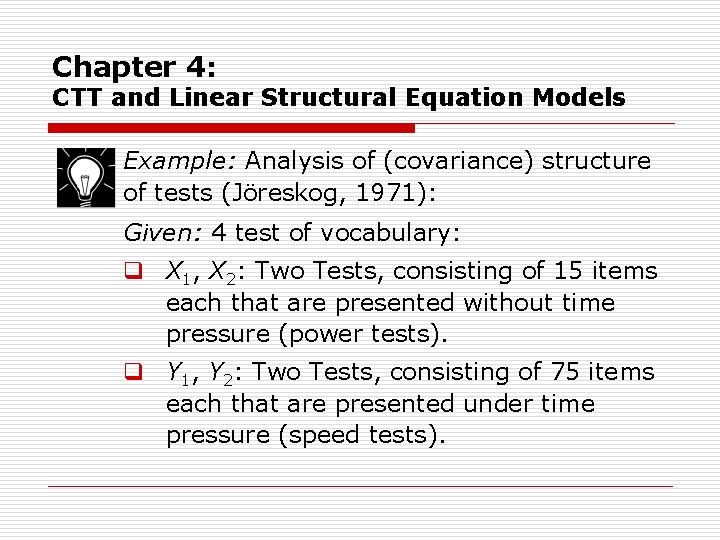
Chapter 4: CTT and Linear Structural Equation Models Example: Analysis of (covariance) structure of tests (Jöreskog, 1971): Given: 4 test of vocabulary: q X 1, X 2: Two Tests, consisting of 15 items each that are presented without time pressure (power tests). q Y 1, Y 2: Two Tests, consisting of 75 items each that are presented under time pressure (speed tests).
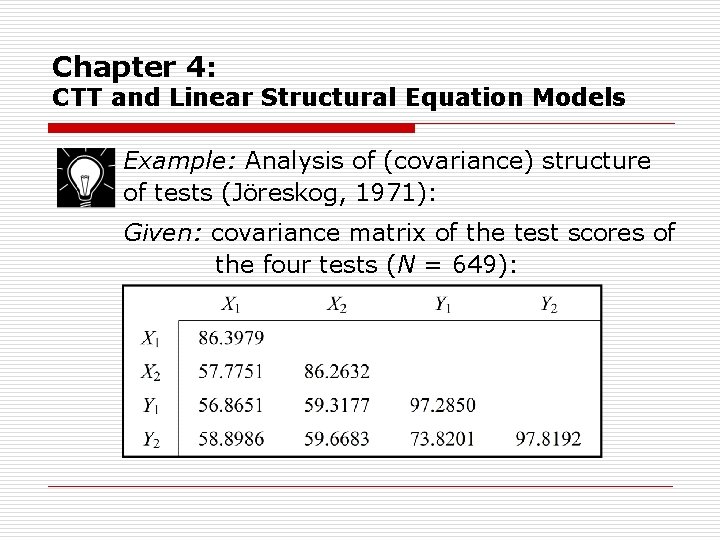
Chapter 4: CTT and Linear Structural Equation Models Example: Analysis of (covariance) structure of tests (Jöreskog, 1971): Given: covariance matrix of the test scores of the four tests (N = 649):
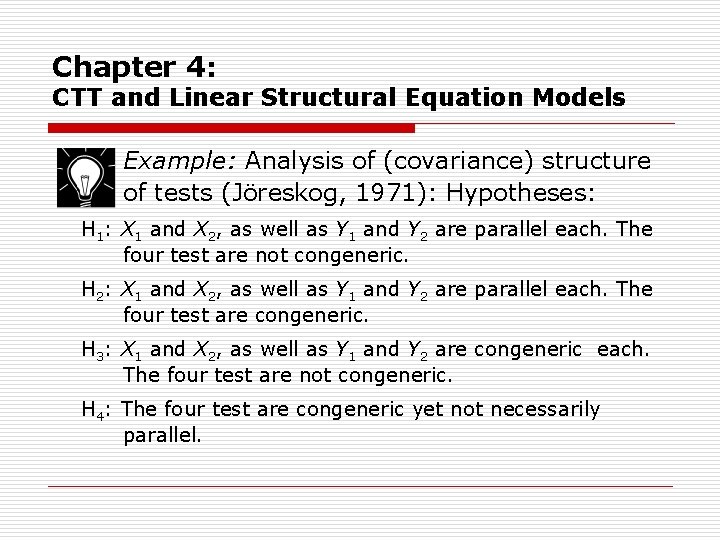
Chapter 4: CTT and Linear Structural Equation Models Example: Analysis of (covariance) structure of tests (Jöreskog, 1971): Hypotheses: H 1: X 1 and X 2, as well as Y 1 and Y 2 are parallel each. The four test are not congeneric. H 2: X 1 and X 2, as well as Y 1 and Y 2 are parallel each. The four test are congeneric. H 3: X 1 and X 2, as well as Y 1 and Y 2 are congeneric each. The four test are not congeneric. H 4: The four test are congeneric yet not necessarily parallel.
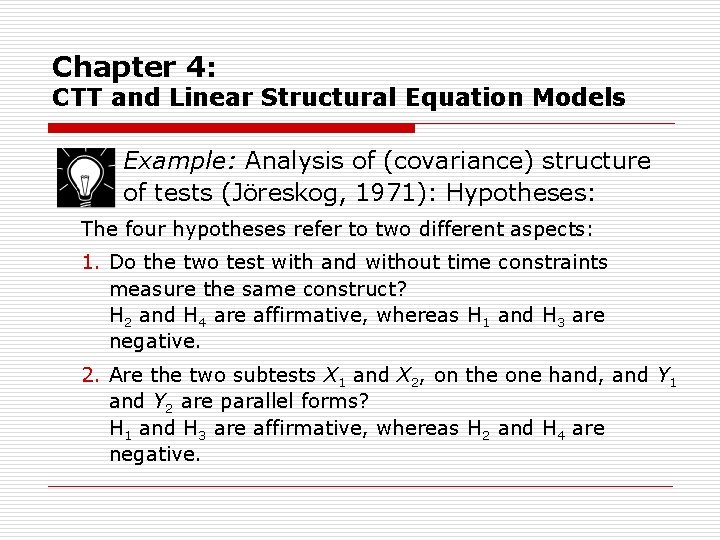
Chapter 4: CTT and Linear Structural Equation Models Example: Analysis of (covariance) structure of tests (Jöreskog, 1971): Hypotheses: The four hypotheses refer to two different aspects: 1. Do the two test with and without time constraints measure the same construct? H 2 and H 4 are affirmative, whereas H 1 and H 3 are negative. 2. Are the two subtests X 1 and X 2, on the one hand, and Y 1 and Y 2 are parallel forms? H 1 and H 3 are affirmative, whereas H 2 and H 4 are negative.
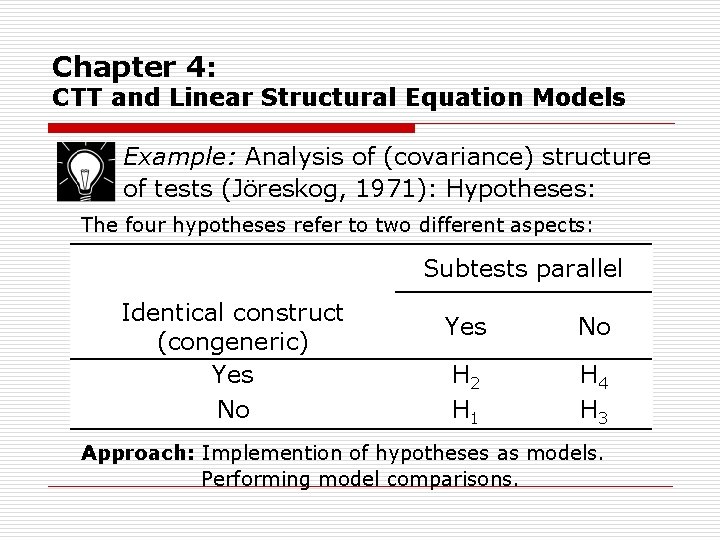
Chapter 4: CTT and Linear Structural Equation Models Example: Analysis of (covariance) structure of tests (Jöreskog, 1971): Hypotheses: The four hypotheses refer to two different aspects: Subtests parallel Identical construct (congeneric) Yes No H 2 H 1 H 4 H 3 Approach: Implemention of hypotheses as models. Performing model comparisons.
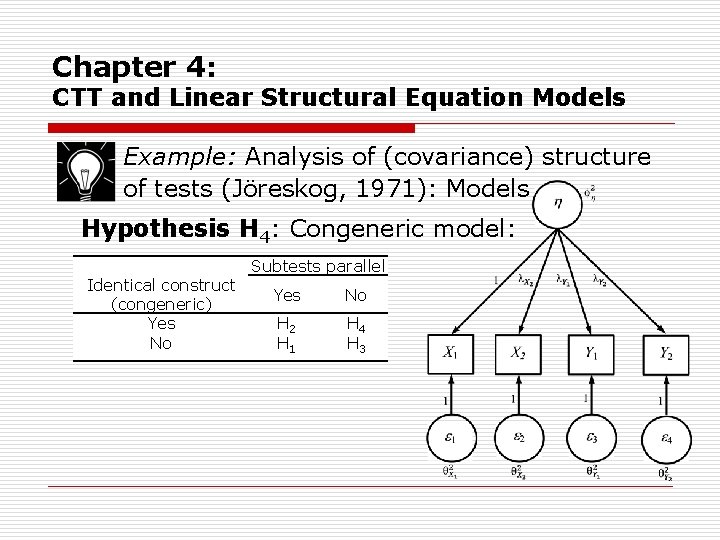
Chapter 4: CTT and Linear Structural Equation Models Example: Analysis of (covariance) structure of tests (Jöreskog, 1971): Models Hypothesis H 4: Congeneric model: Identical construct (congeneric) Yes No Subtests parallel Yes No H 2 H 1 H 4 H 3
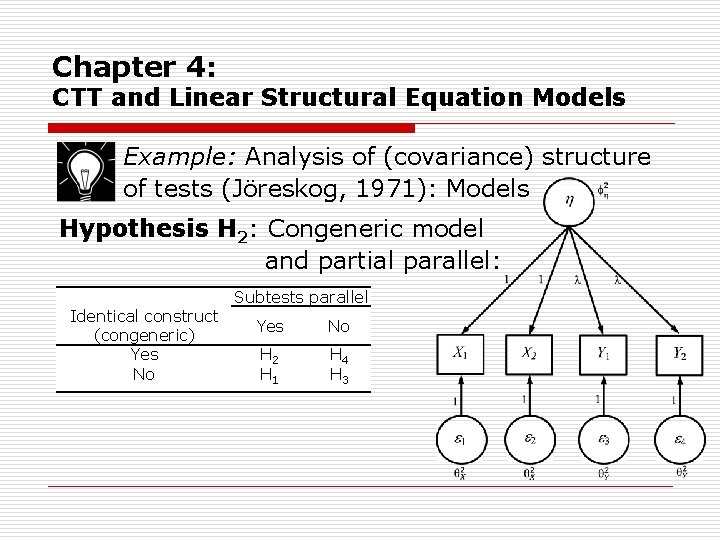
Chapter 4: CTT and Linear Structural Equation Models Example: Analysis of (covariance) structure of tests (Jöreskog, 1971): Models Hypothesis H 2: Congeneric model and partial parallel: Identical construct (congeneric) Yes No Subtests parallel Yes No H 2 H 1 H 4 H 3
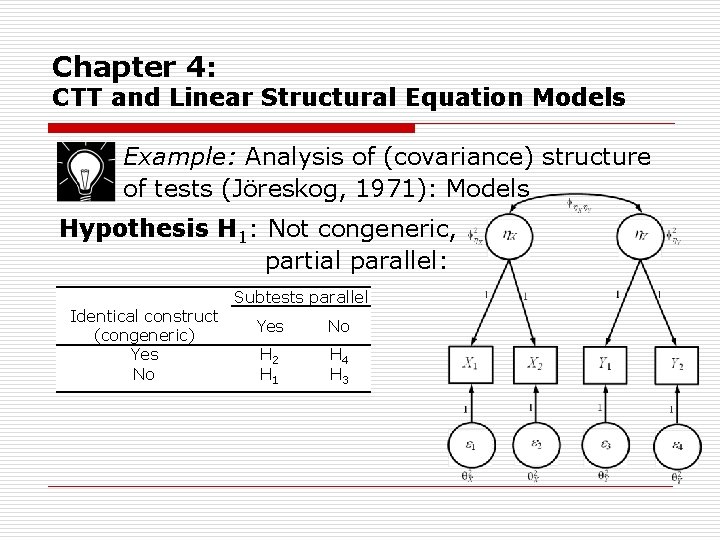
Chapter 4: CTT and Linear Structural Equation Models Example: Analysis of (covariance) structure of tests (Jöreskog, 1971): Models Hypothesis H 1: Not congeneric, partial parallel: Identical construct (congeneric) Yes No Subtests parallel Yes No H 2 H 1 H 4 H 3
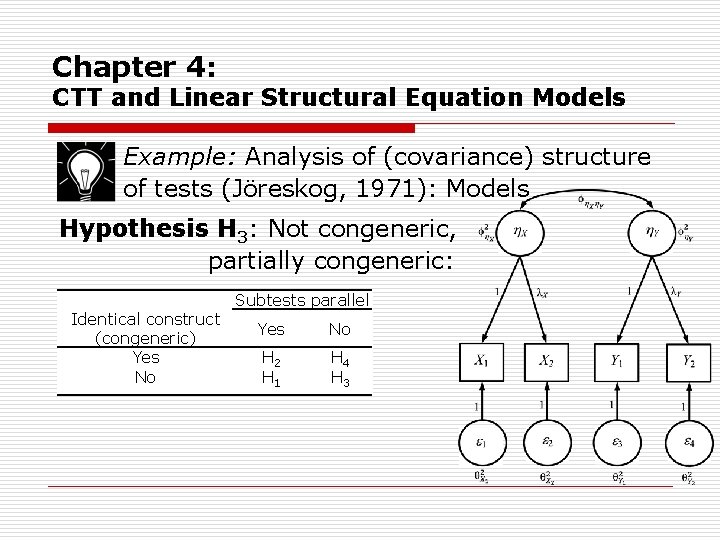
Chapter 4: CTT and Linear Structural Equation Models Example: Analysis of (covariance) structure of tests (Jöreskog, 1971): Models Hypothesis H 3: Not congeneric, partially congeneric: Identical construct (congeneric) Yes No Subtests parallel Yes No H 2 H 1 H 4 H 3
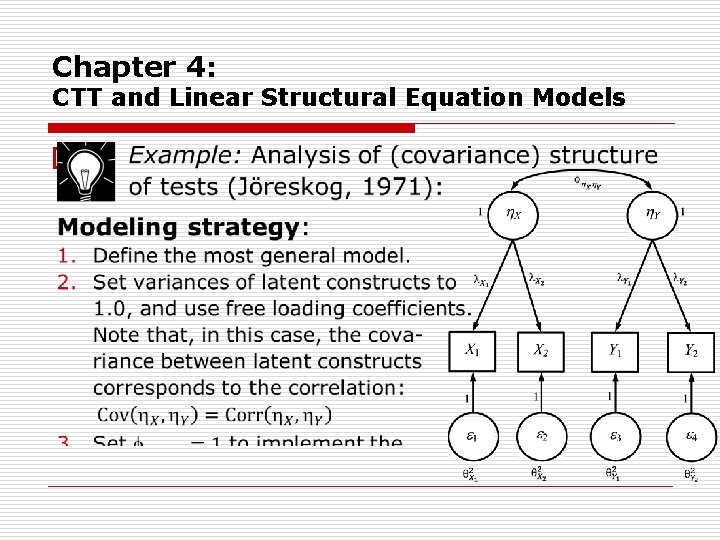
Chapter 4: CTT and Linear Structural Equation Models o
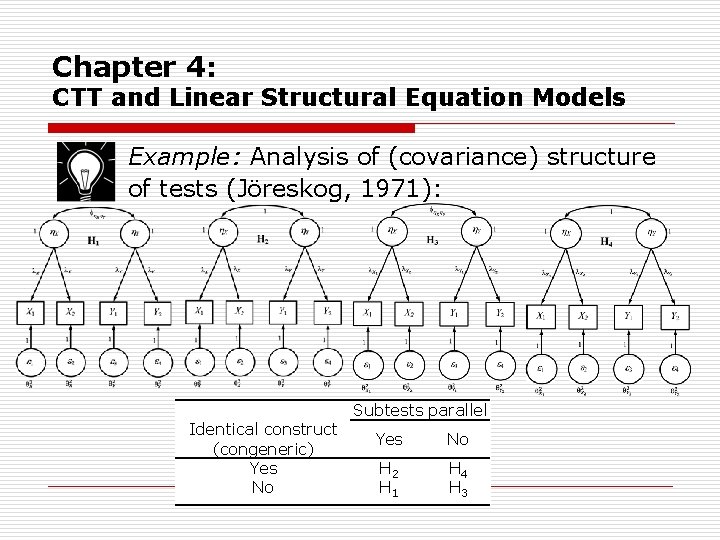
Chapter 4: CTT and Linear Structural Equation Models Example: Analysis of (covariance) structure of tests (Jöreskog, 1971): Identical construct (congeneric) Yes No Subtests parallel Yes No H 2 H 1 H 4 H 3
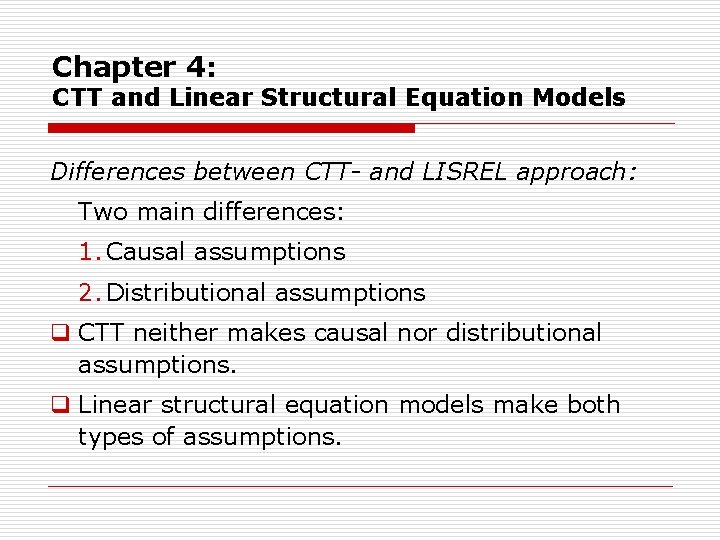
Chapter 4: CTT and Linear Structural Equation Models Differences between CTT- and LISREL approach: Two main differences: 1. Causal assumptions 2. Distributional assumptions q CTT neither makes causal nor distributional assumptions. q Linear structural equation models make both types of assumptions.
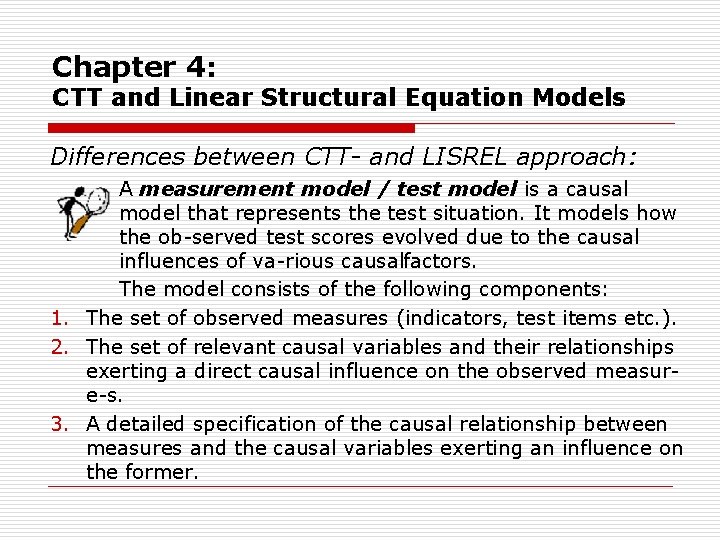
Chapter 4: CTT and Linear Structural Equation Models Differences between CTT- and LISREL approach: A measurement model / test model is a causal model that represents the test situation. It models how the ob served test scores evolved due to the causal influences of va rious causalfactors. The model consists of the following components: 1. The set of observed measures (indicators, test items etc. ). 2. The set of relevant causal variables and their relationships exerting a direct causal influence on the observed measur e s. 3. A detailed specification of the causal relationship between measures and the causal variables exerting an influence on the former.
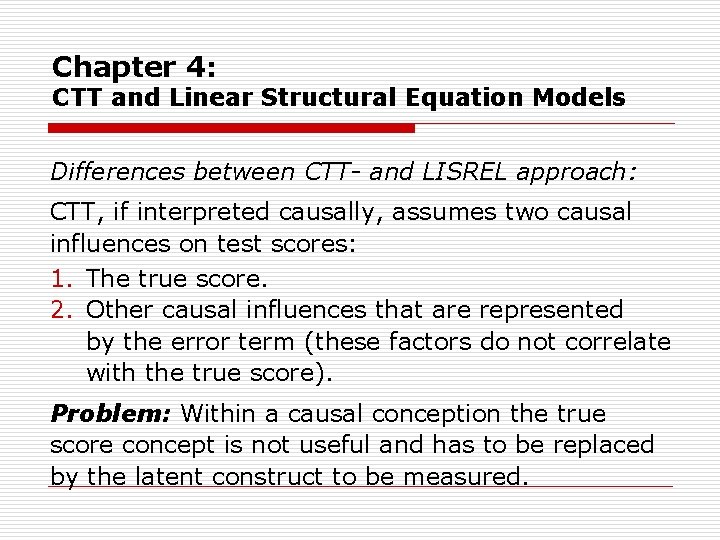
Chapter 4: CTT and Linear Structural Equation Models Differences between CTT- and LISREL approach: CTT, if interpreted causally, assumes two causal influences on test scores: 1. The true score. 2. Other causal influences that are represented by the error term (these factors do not correlate with the true score). Problem: Within a causal conception the true score concept is not useful and has to be replaced by the latent construct to be measured.
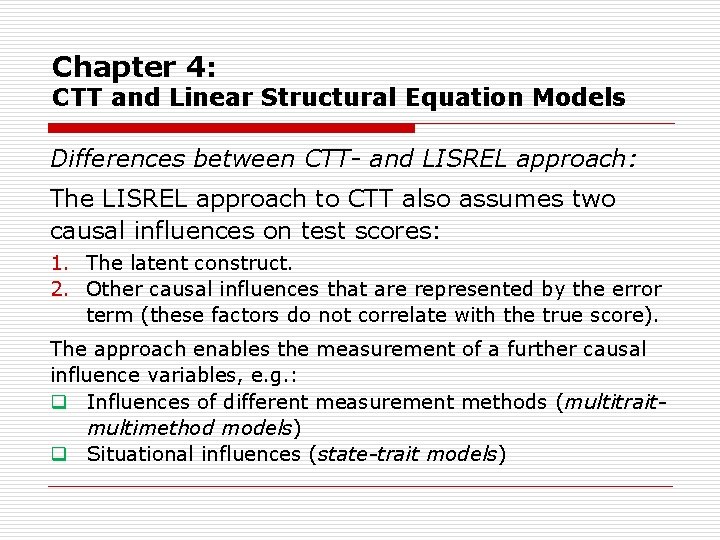
Chapter 4: CTT and Linear Structural Equation Models Differences between CTT- and LISREL approach: The LISREL approach to CTT also assumes two causal influences on test scores: 1. The latent construct. 2. Other causal influences that are represented by the error term (these factors do not correlate with the true score). The approach enables the measurement of a further causal influence variables, e. g. : q Influences of different measurement methods (multitraitmultimethod models) q Situational influences (state-trait models)
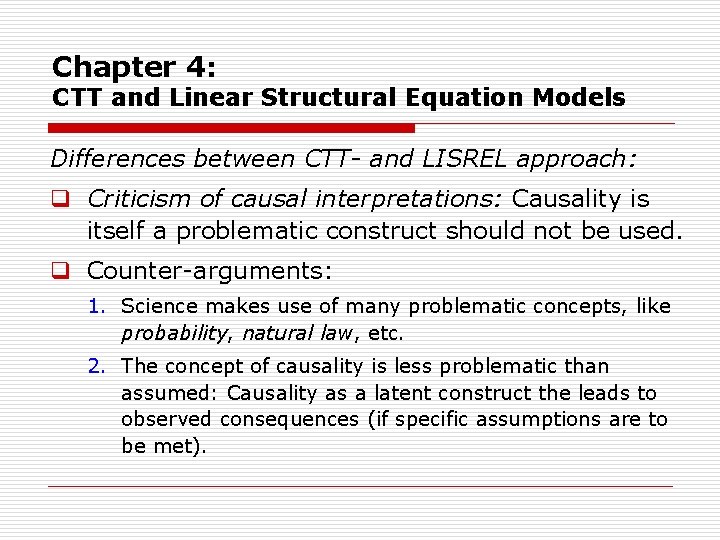
Chapter 4: CTT and Linear Structural Equation Models Differences between CTT- and LISREL approach: q Criticism of causal interpretations: Causality is itself a problematic construct should not be used. q Counter arguments: 1. Science makes use of many problematic concepts, like probability, natural law, etc. 2. The concept of causality is less problematic than assumed: Causality as a latent construct the leads to observed consequences (if specific assumptions are to be met).
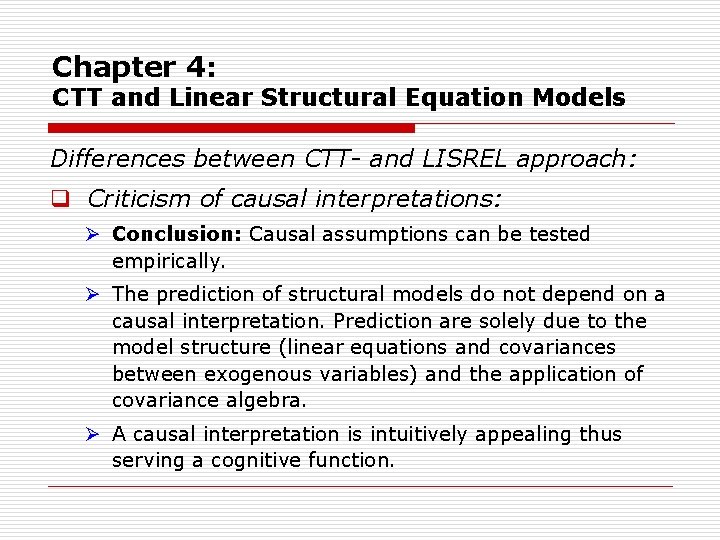
Chapter 4: CTT and Linear Structural Equation Models Differences between CTT- and LISREL approach: q Criticism of causal interpretations: Ø Conclusion: Causal assumptions can be tested empirically. Ø The prediction of structural models do not depend on a causal interpretation. Prediction are solely due to the model structure (linear equations and covariances between exogenous variables) and the application of covariance algebra. Ø A causal interpretation is intuitively appealing thus serving a cognitive function.
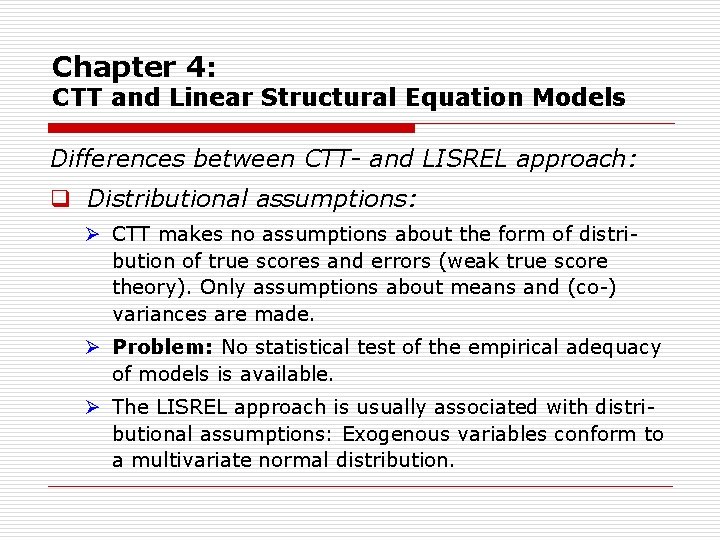
Chapter 4: CTT and Linear Structural Equation Models Differences between CTT- and LISREL approach: q Distributional assumptions: Ø CTT makes no assumptions about the form of distri bution of true scores and errors (weak true score theory). Only assumptions about means and (co ) variances are made. Ø Problem: No statistical test of the empirical adequacy of models is available. Ø The LISREL approach is usually associated with distri butional assumptions: Exogenous variables conform to a multivariate normal distribution.
Saigon ctt
Saigonctt
Saigon
Saigon ctt
Saigonctt
Difference between linear and nonlinear equations
Linear equation and quadratic equation
Solving systems of linear and quadratic equations
What is the difference between models and semi modals
Generalization in software engineering
Interactive models of communication
Linear models and rates of change
Linear models and matrix algebra
Linear programming models: graphical and computer methods
How to tell if a graph is linear quadratic or exponential
What is static structural analysis
Sem for dummies
Structural equation modeling
Compatibility equation in structural analysis
Dynamic structural equation modeling
Curriculum development models
Cons of barlunds model of communication
A unifying review of linear gaussian models
Linear basis function models
Curve fitting with linear models
Linear functions as mathematical models
Linear functions as mathematical models
Curve fitting with linear models
Simple linear regression and multiple regression
Example of linear text
Nonlinear
Linear pipeline
What is non linear multimedia
Linear momentum and linear impulse
Persamaan simultan
Define left linear grammar
Metode gauss seidel
2-1 solving linear equations and inequalities
Chapter 9 transportation and assignment models solutions
Chapter 1 graphs functions and models answers
Eyring equation and arrhenius equation
Eyring equation
Eyring equation and arrhenius equation
Metode biseksi contoh soal
Contoh soal metode iterasi sederhana
Fungsi linear fungsi kuadrat dan fungsi rasional
Materi fungsi non linier
What is a linear transformation linear algebra
Linear algebra 1