Assessment Literacy Series PA Module 5 Data Analysis
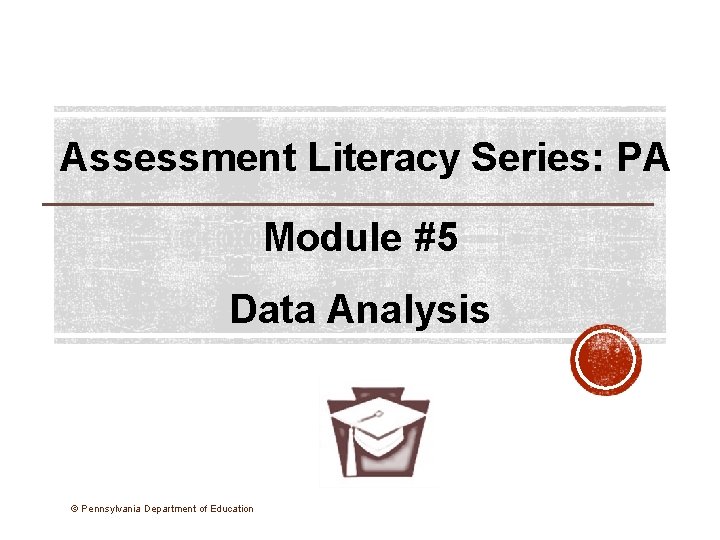
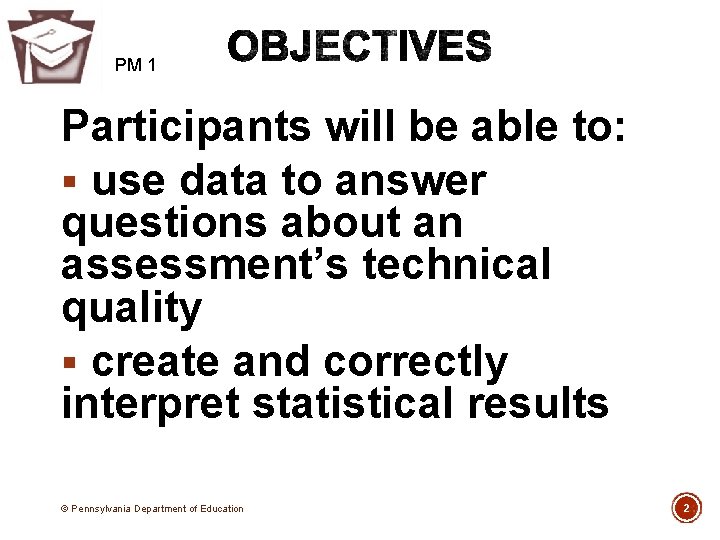
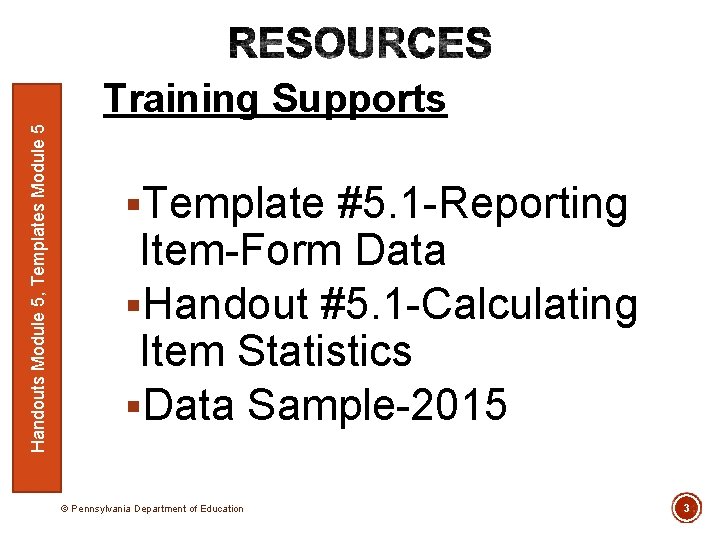
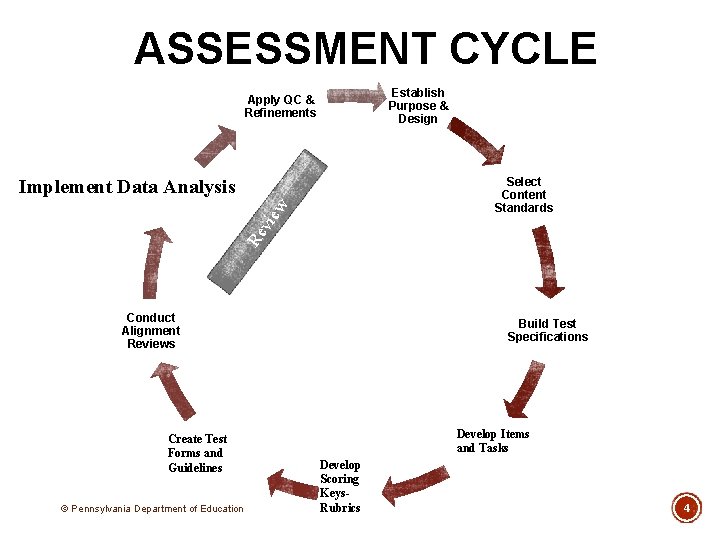
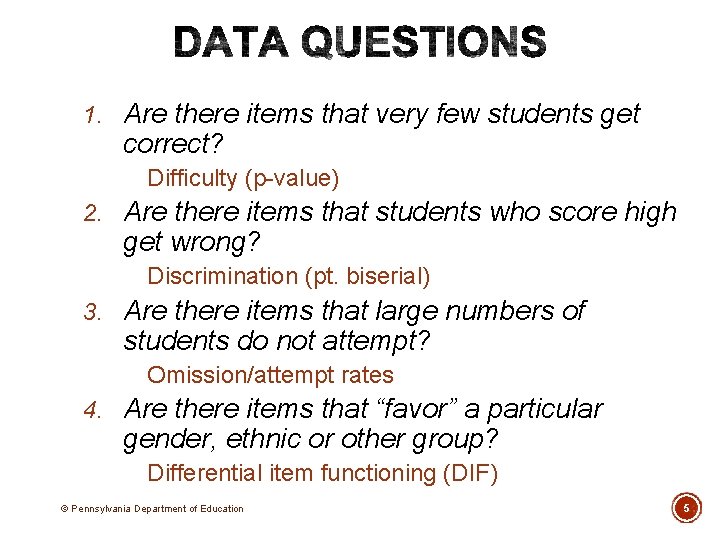
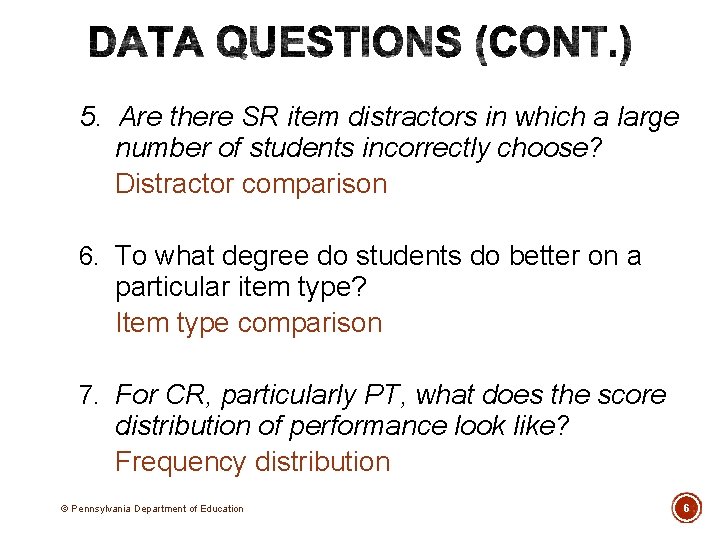
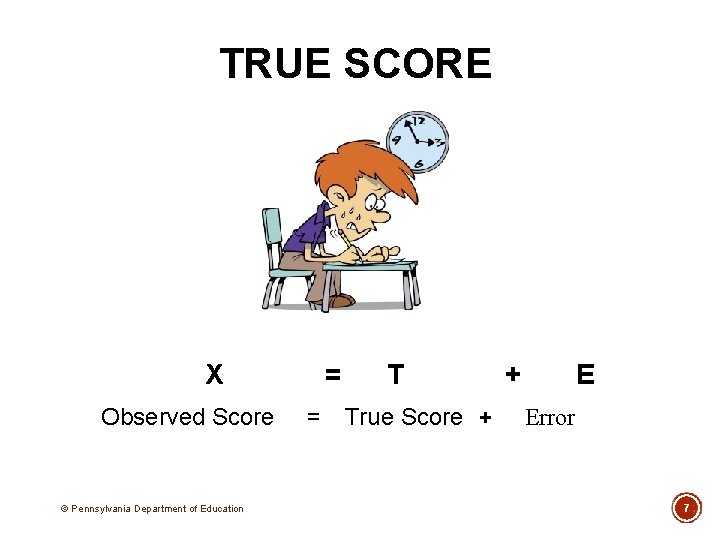
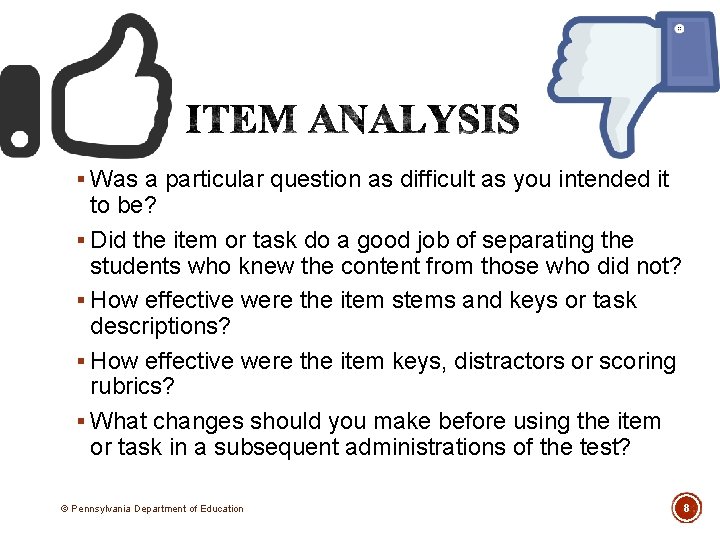
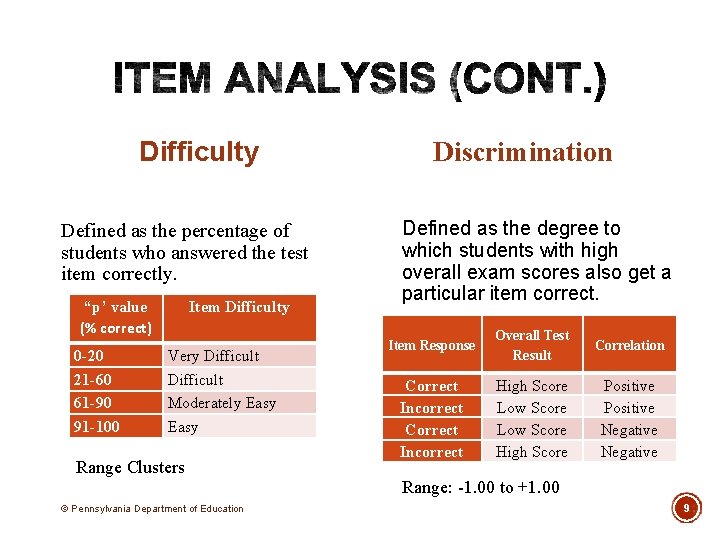
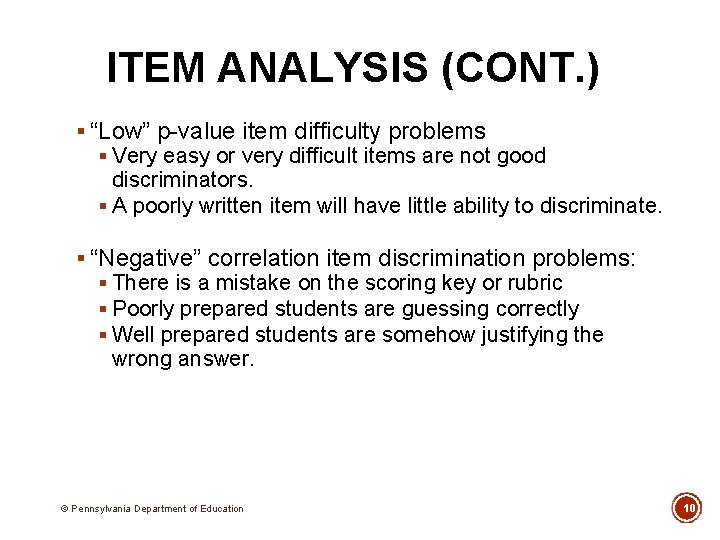
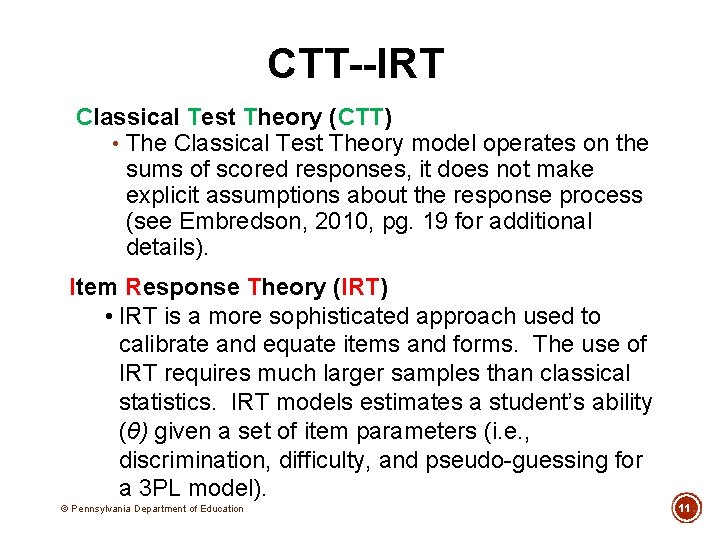
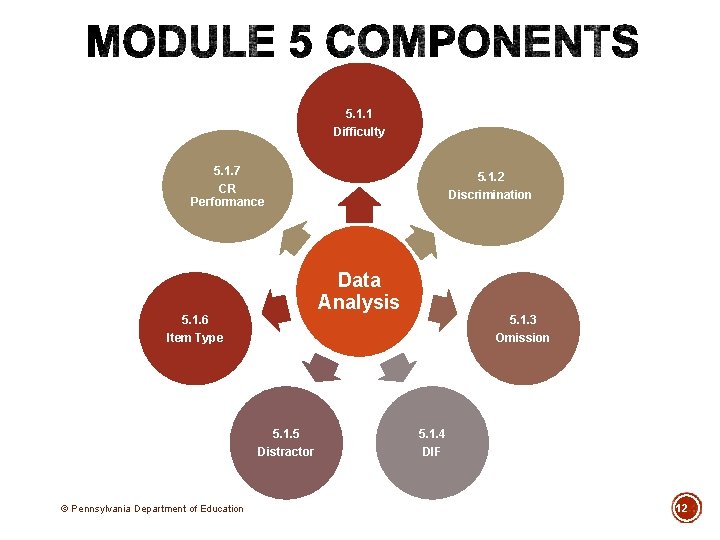
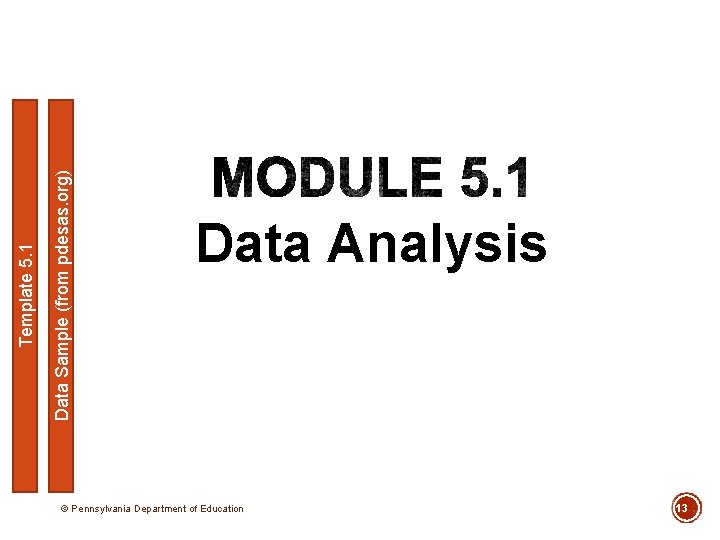
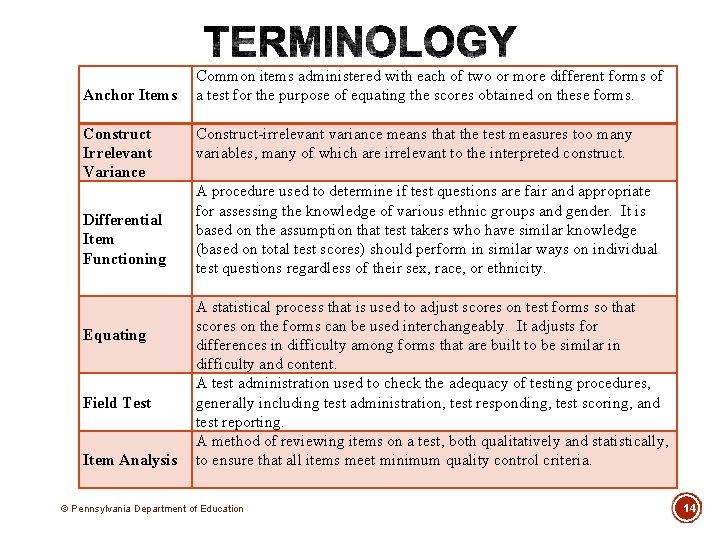
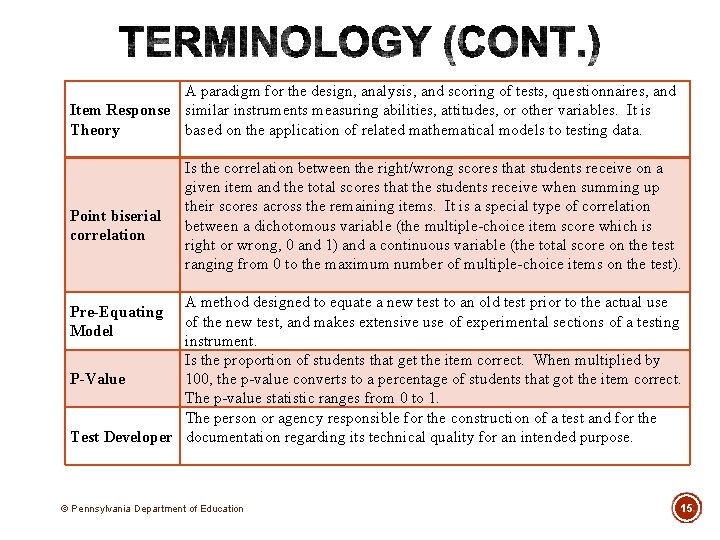
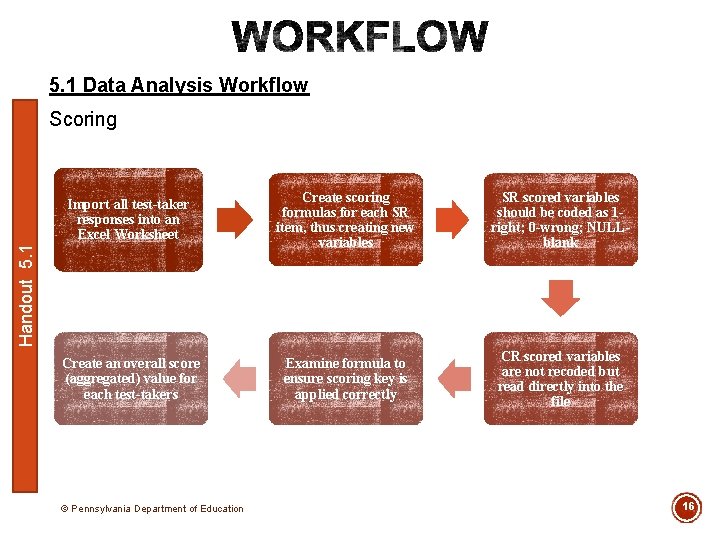
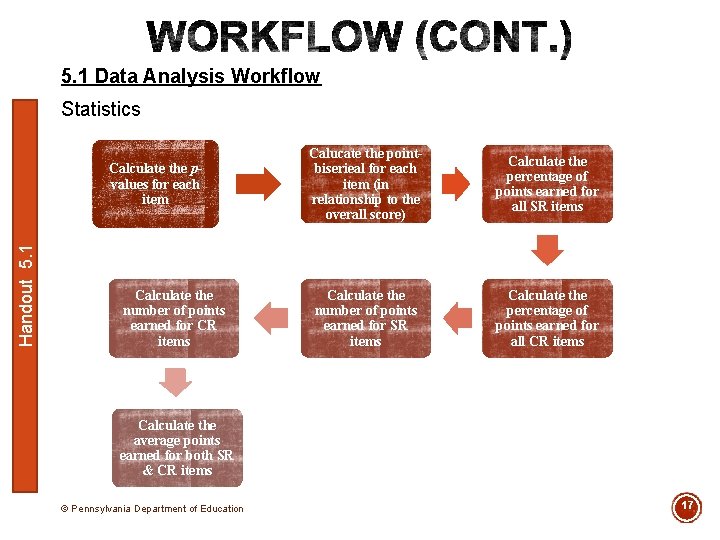
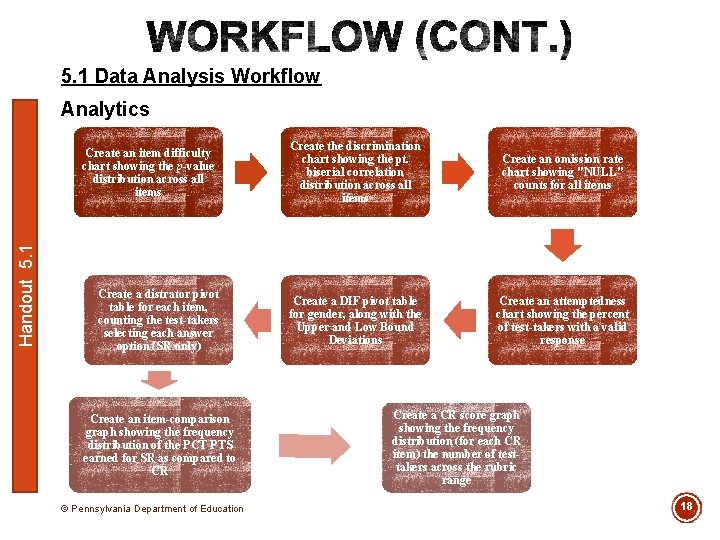
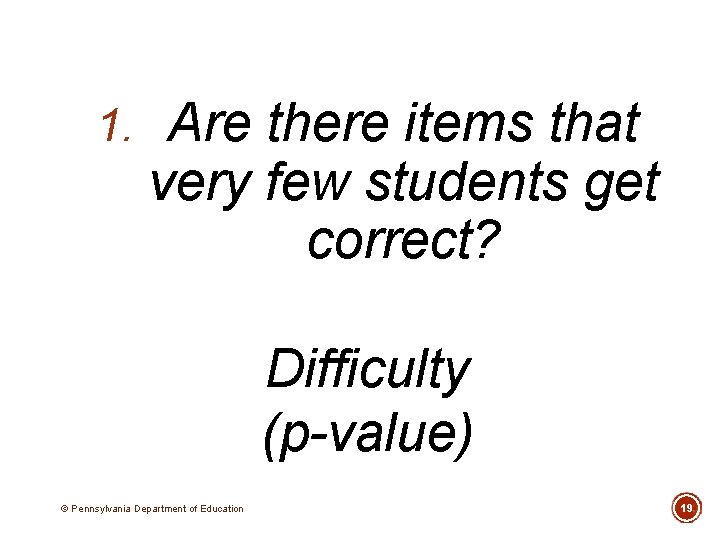
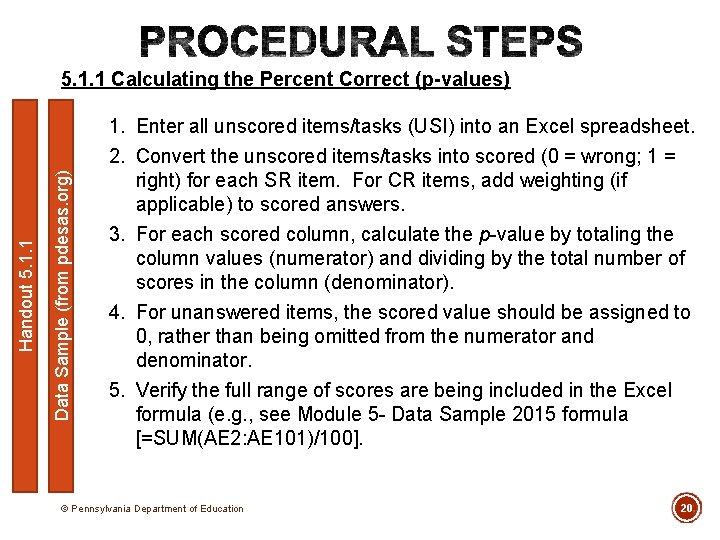
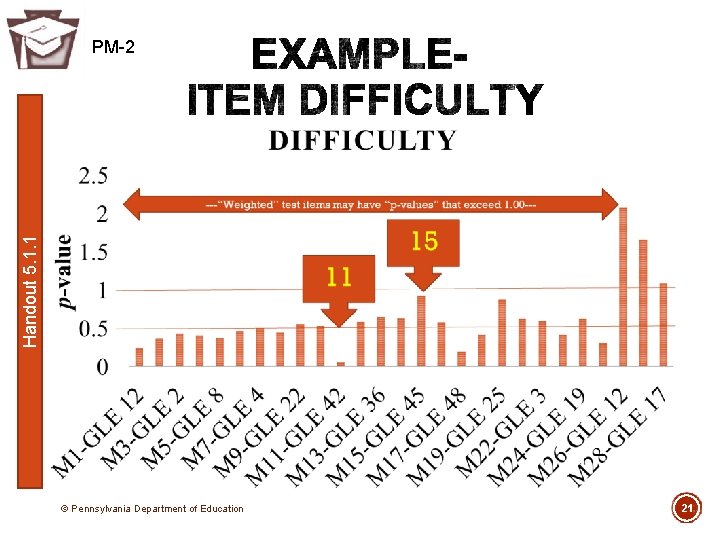
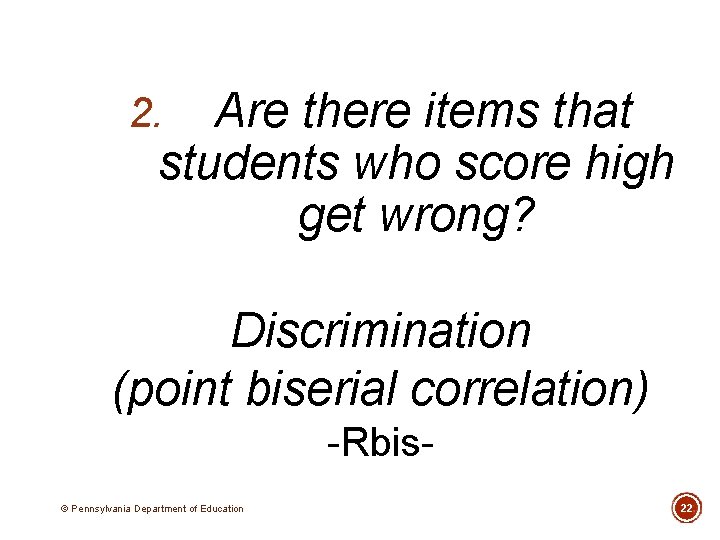
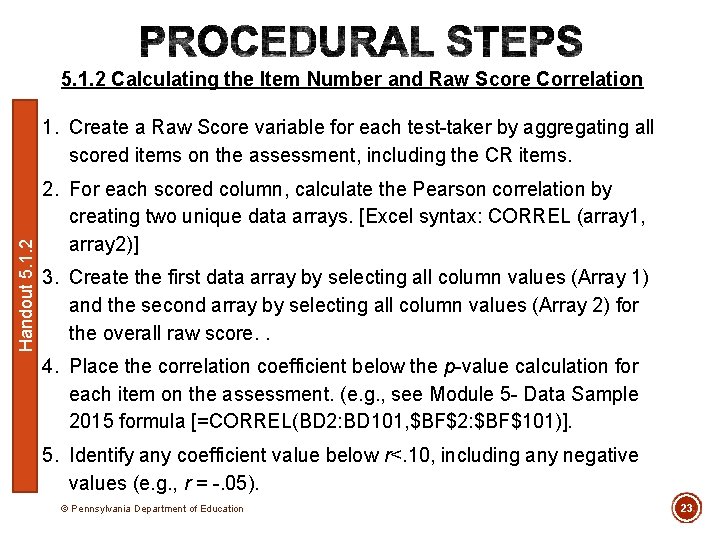
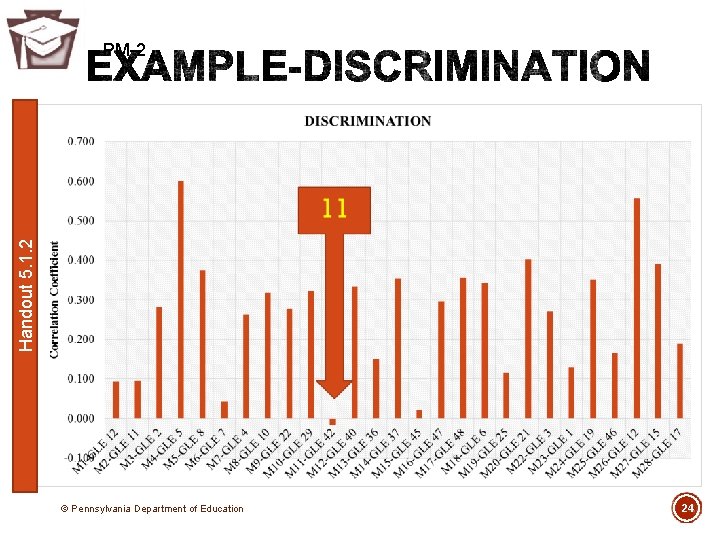
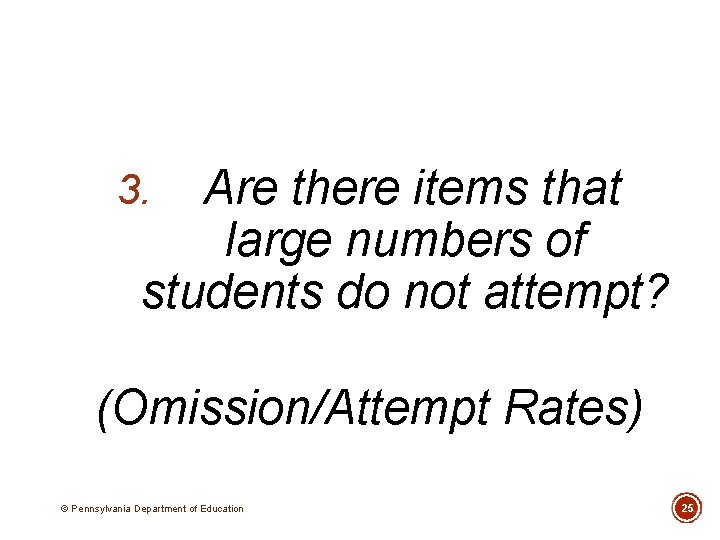
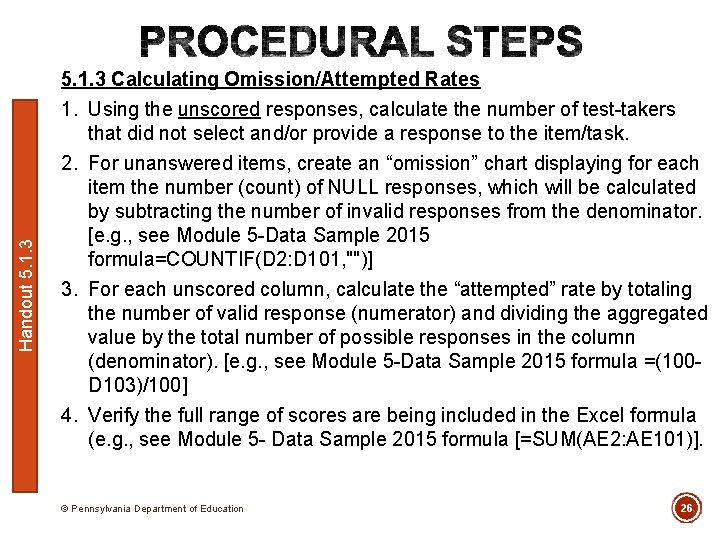
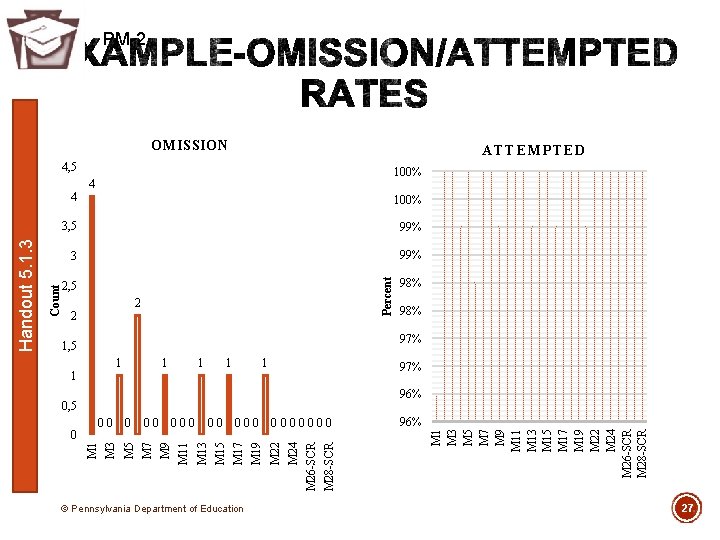
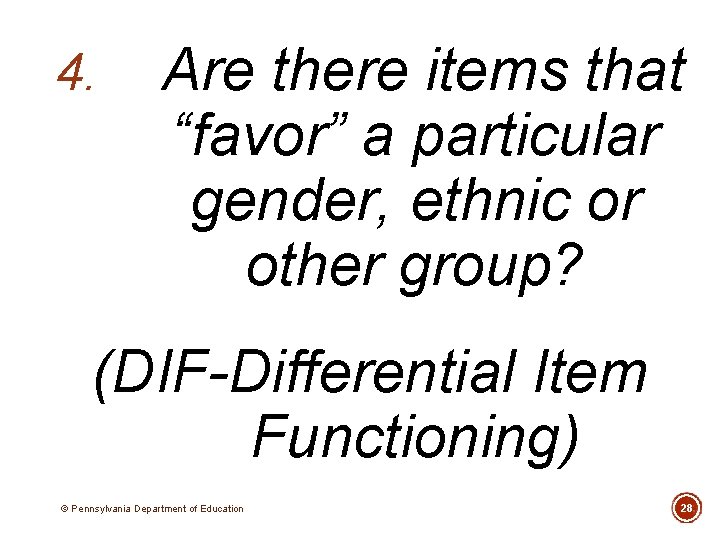
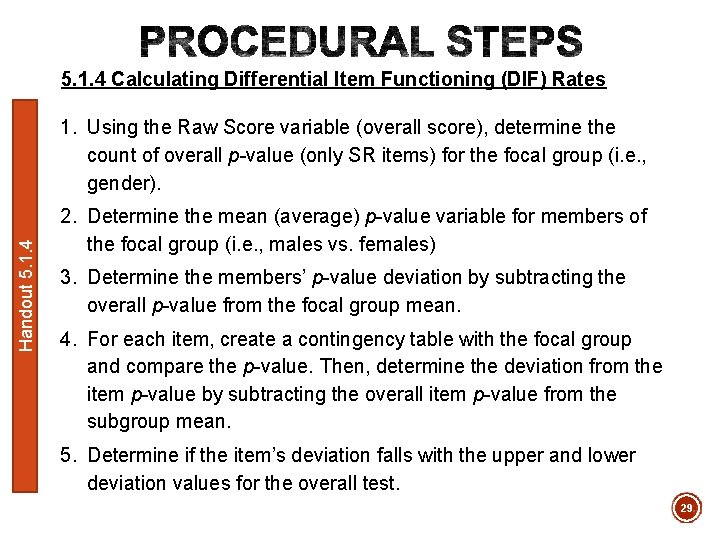
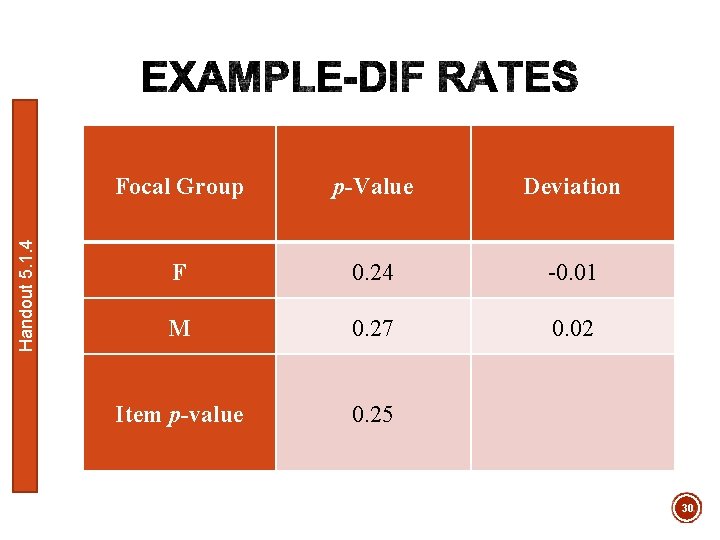
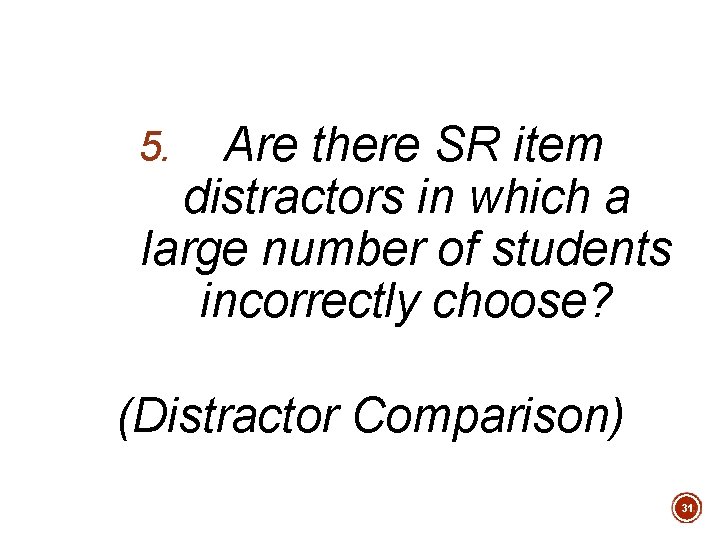
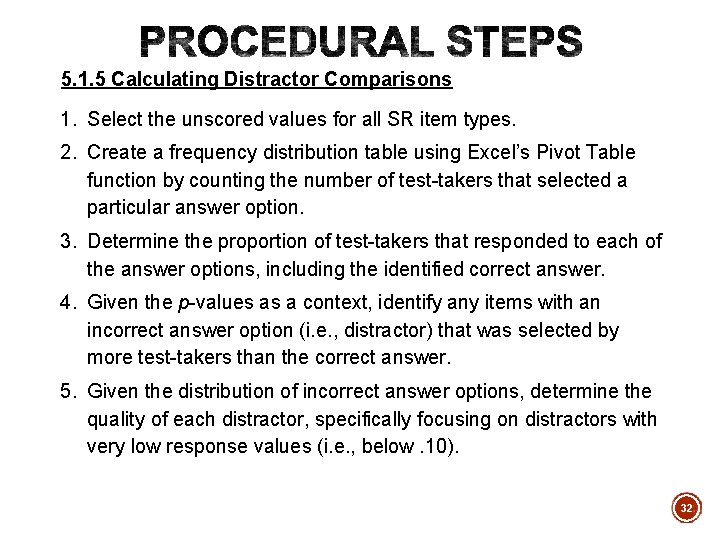
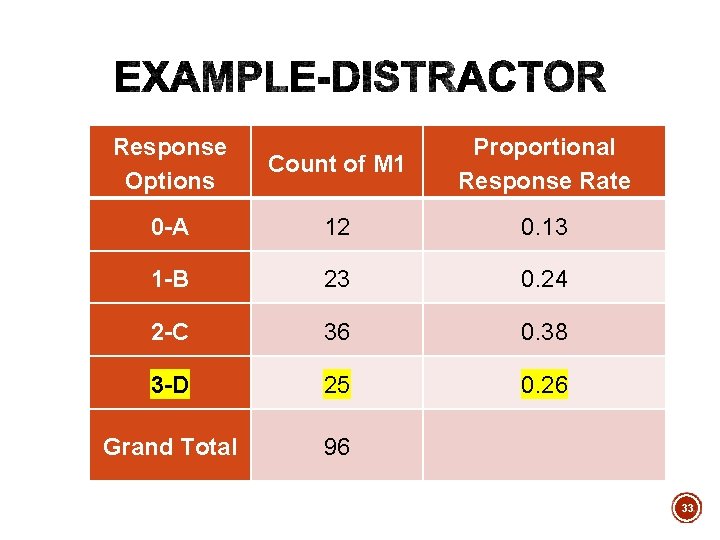
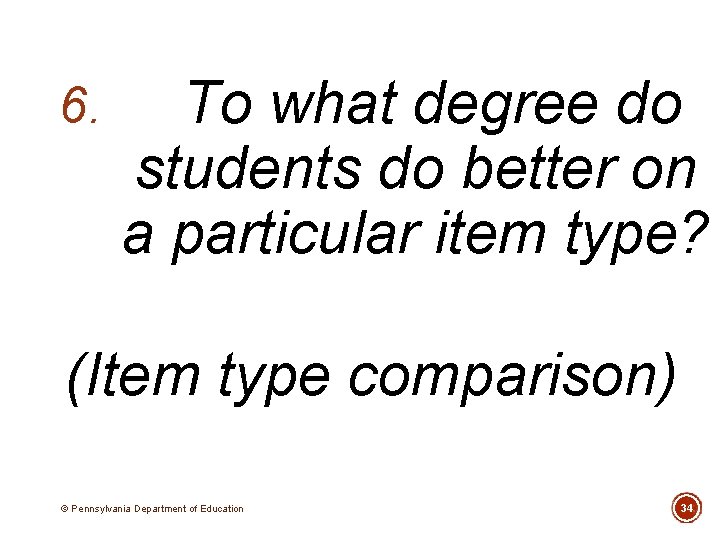
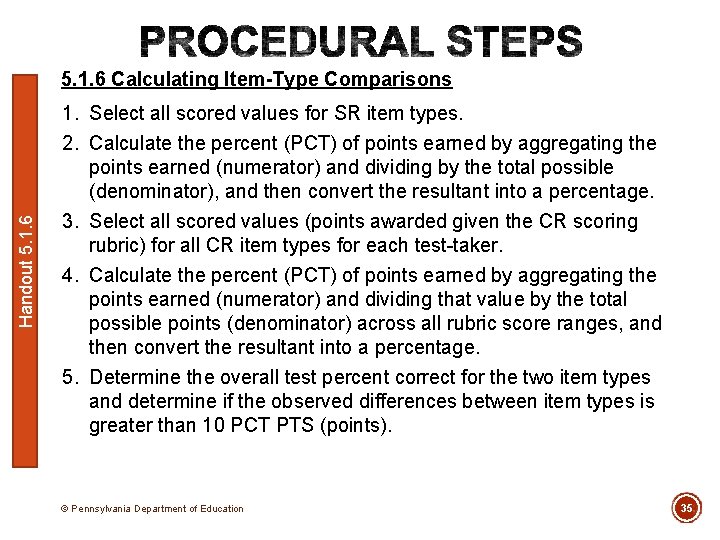
![ITEM TYPE COMPARISON Percent Handout 5. 1. 6 %Points [25]-SR Items %Points [9]-SR Items ITEM TYPE COMPARISON Percent Handout 5. 1. 6 %Points [25]-SR Items %Points [9]-SR Items](https://slidetodoc.com/presentation_image_h2/c8dab11c50fad50fdfa52421045fdf7f/image-36.jpg)
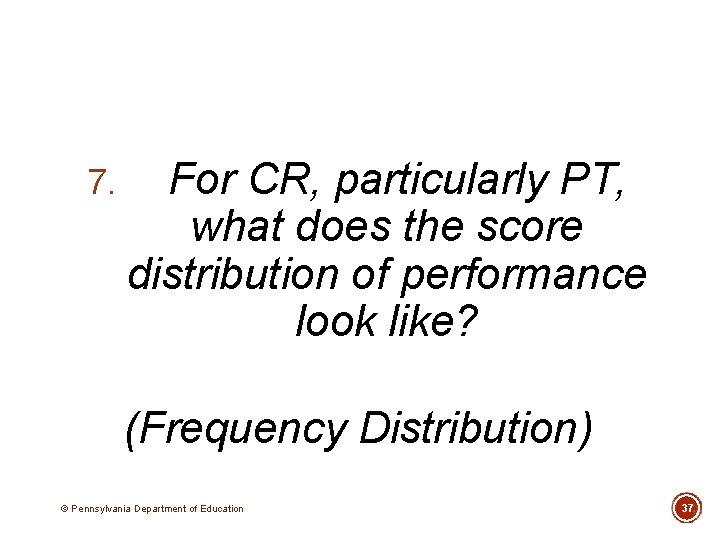
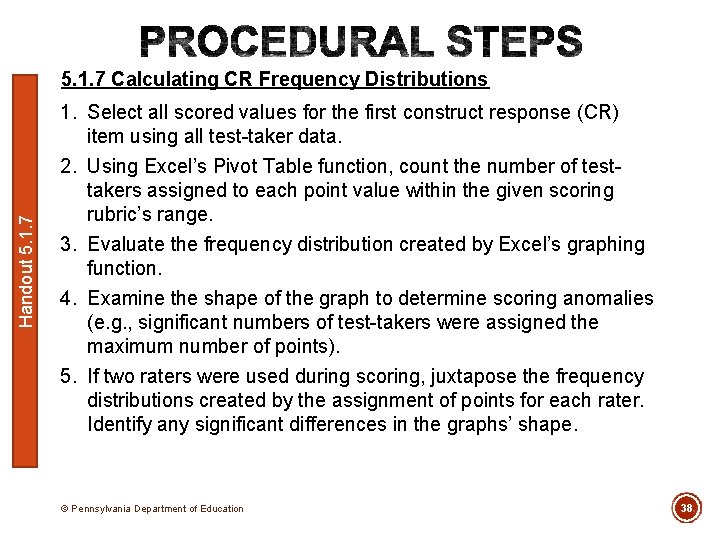
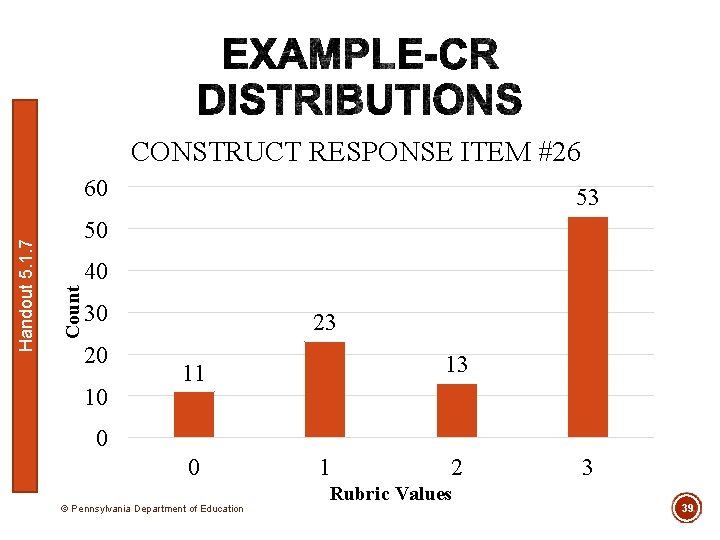
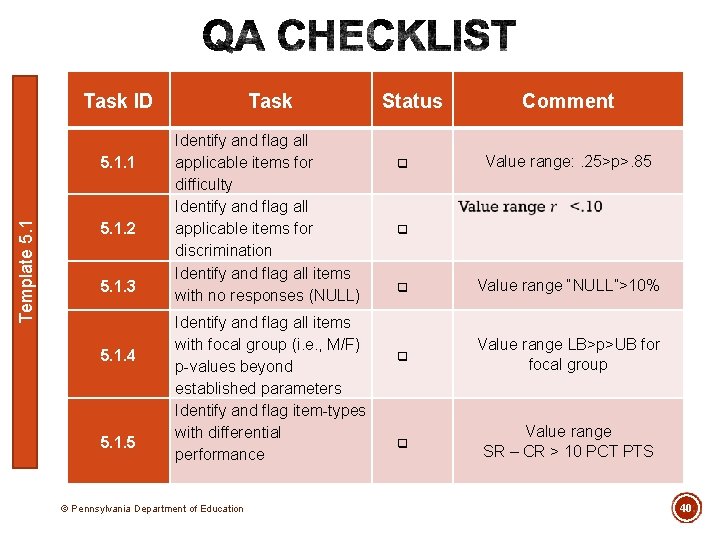
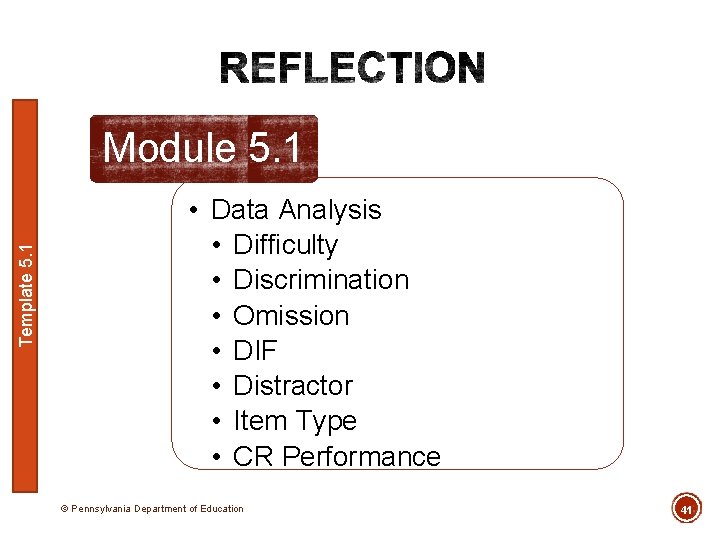
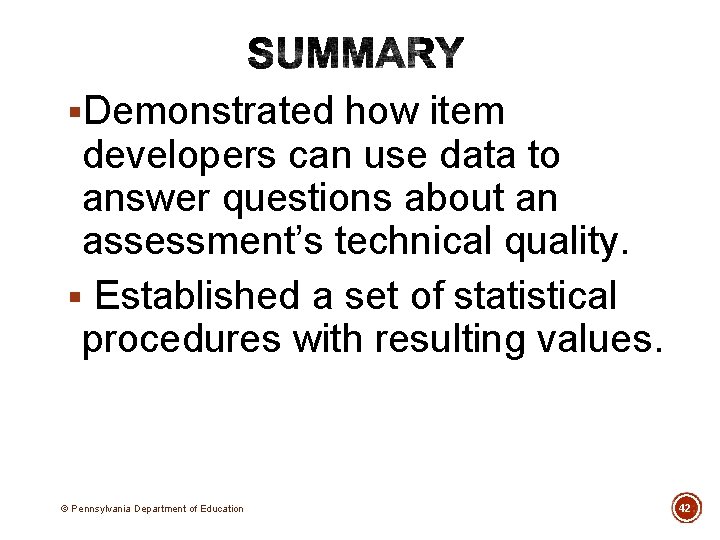
- Slides: 42
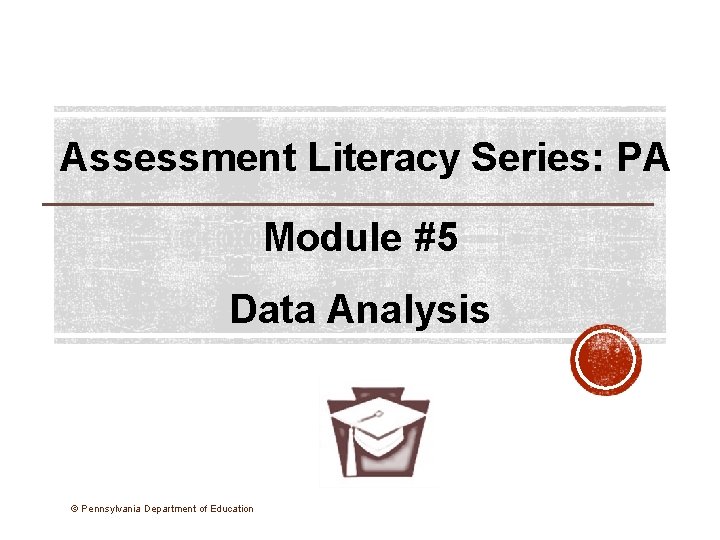
Assessment Literacy Series: PA Module #5 Data Analysis © Pennsylvania Department of Education
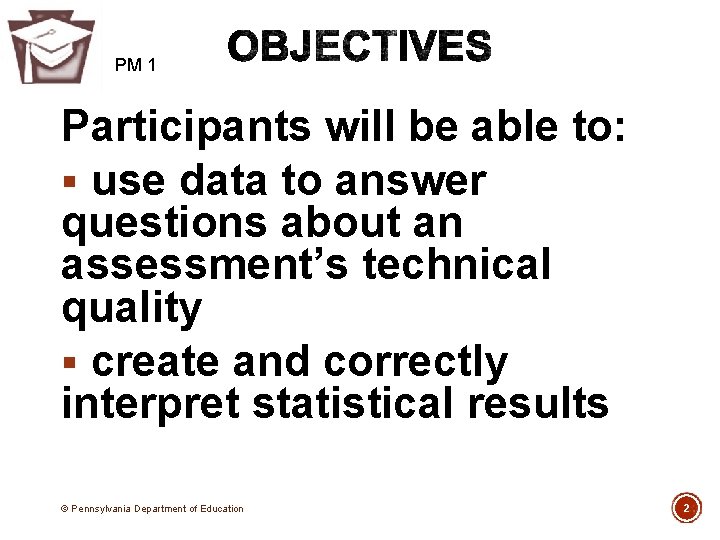
PM 1 Participants will be able to: § use data to answer questions about an assessment’s technical quality § create and correctly interpret statistical results © Pennsylvania Department of Education 2
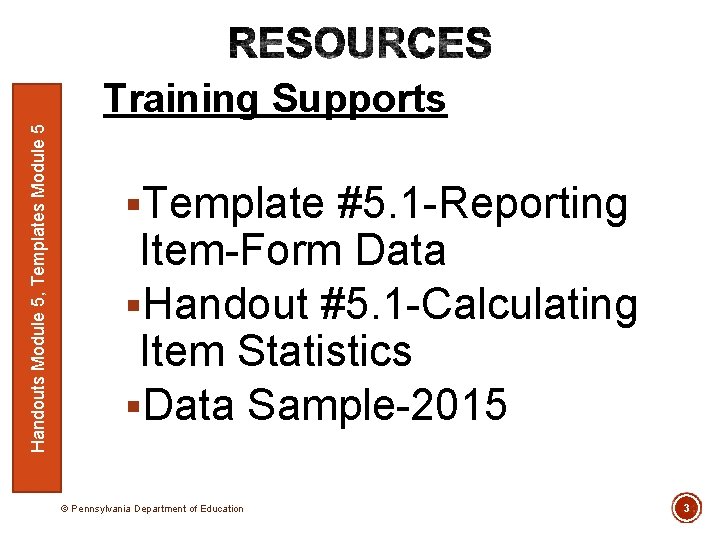
Handouts Module 5, Templates Module 5 Training Supports §Template #5. 1 -Reporting Item-Form Data §Handout #5. 1 -Calculating Item Statistics §Data Sample-2015 © Pennsylvania Department of Education 3
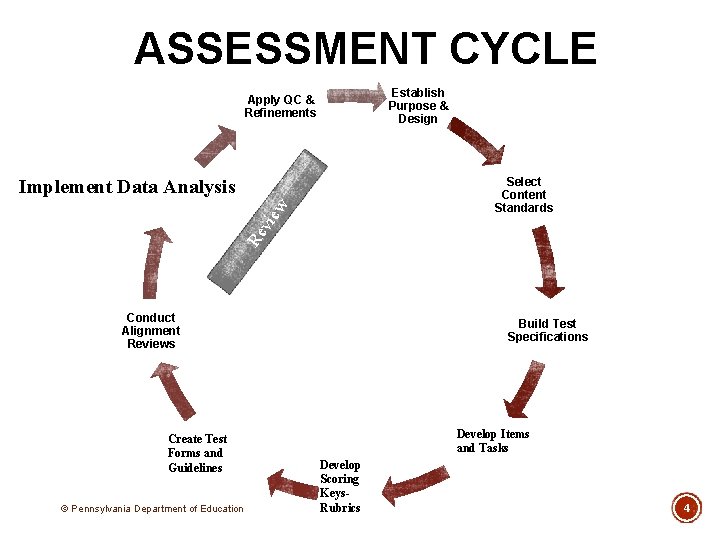
ASSESSMENT CYCLE Establish Purpose & Design Apply QC & Refinements Implement Data Analysis Re v iew Select Content Standards Conduct Alignment Reviews Create Test Forms and Guidelines © Pennsylvania Department of Education Build Test Specifications Develop Items and Tasks Develop Scoring Keys. Rubrics 4
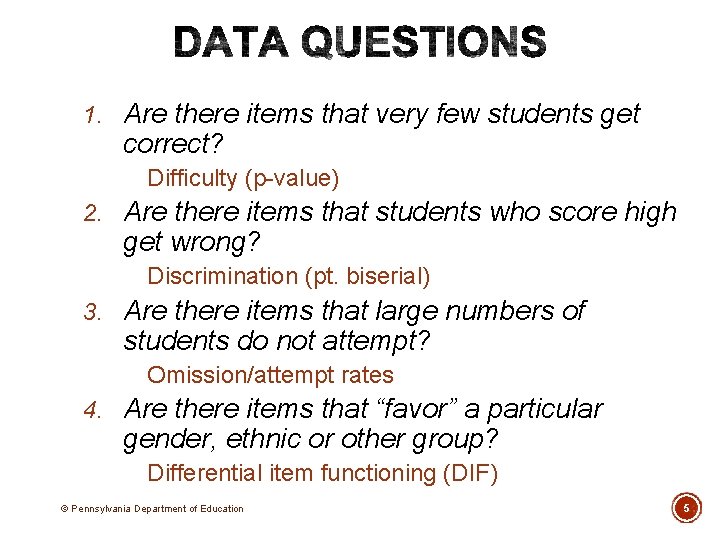
1. Are there items that very few students get correct? Difficulty (p-value) 2. Are there items that students who score high get wrong? Discrimination (pt. biserial) 3. Are there items that large numbers of students do not attempt? Omission/attempt rates 4. Are there items that “favor” a particular gender, ethnic or other group? Differential item functioning (DIF) © Pennsylvania Department of Education 5
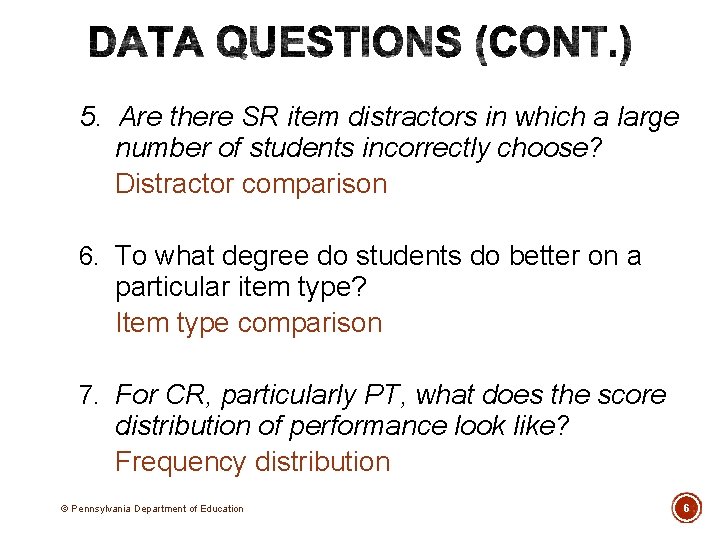
5. Are there SR item distractors in which a large number of students incorrectly choose? Distractor comparison 6. To what degree do students do better on a particular item type? Item type comparison 7. For CR, particularly PT, what does the score distribution of performance look like? Frequency distribution © Pennsylvania Department of Education 6
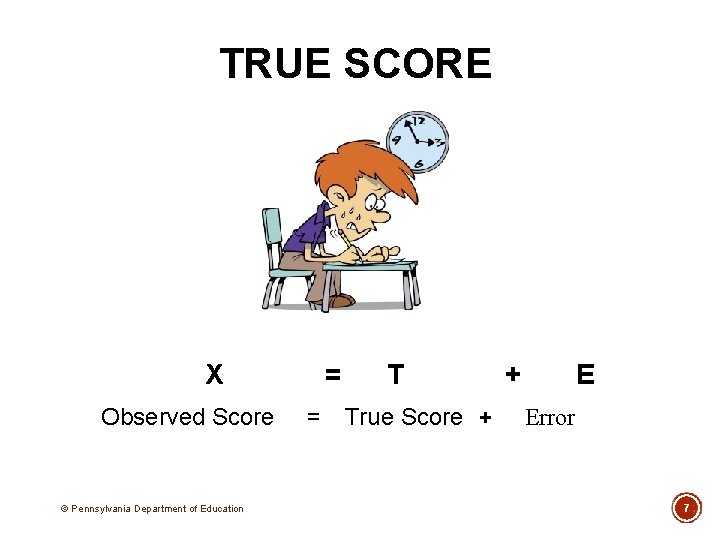
TRUE SCORE X Observed Score © Pennsylvania Department of Education = = T True Score + + E Error 7
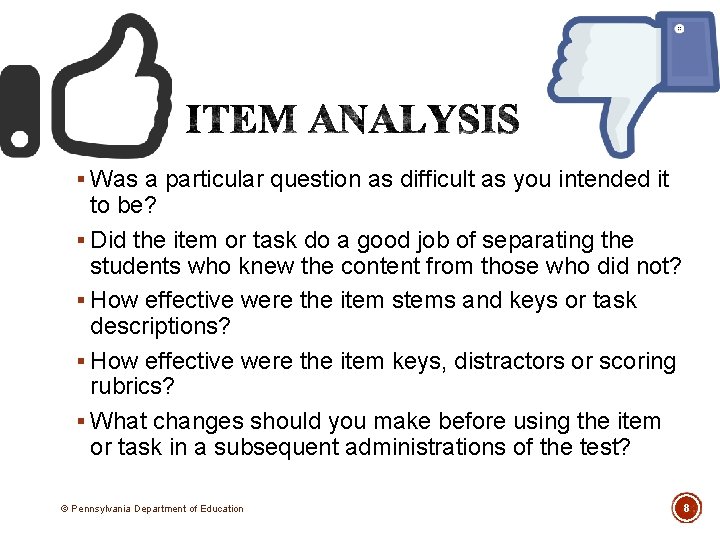
§ Was a particular question as difficult as you intended it to be? § Did the item or task do a good job of separating the students who knew the content from those who did not? § How effective were the item stems and keys or task descriptions? § How effective were the item keys, distractors or scoring rubrics? § What changes should you make before using the item or task in a subsequent administrations of the test? © Pennsylvania Department of Education 8
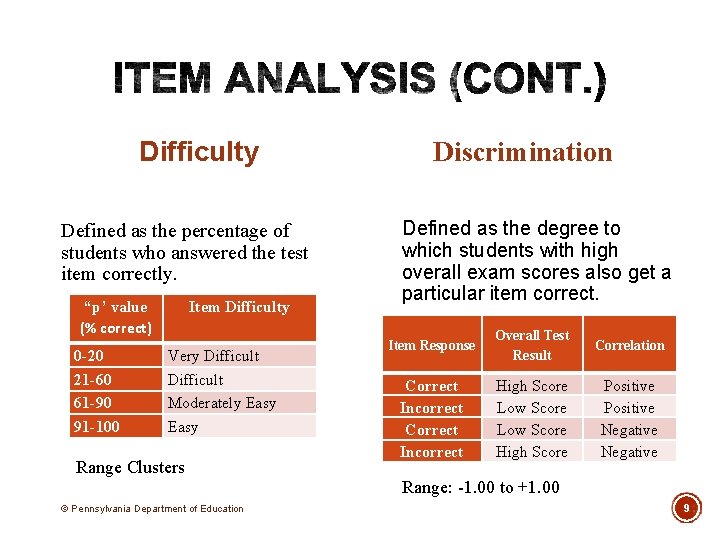
Difficulty Defined as the percentage of students who answered the test item correctly. “p’ value (% correct) 0 -20 21 -60 61 -90 91 -100 Item Difficulty Very Difficult Moderately Easy Range Clusters © Pennsylvania Department of Education Discrimination Defined as the degree to which students with high overall exam scores also get a particular item correct. Item Response Overall Test Result Correlation Correct Incorrect High Score Low Score High Score Positive Negative Range: -1. 00 to +1. 00 9
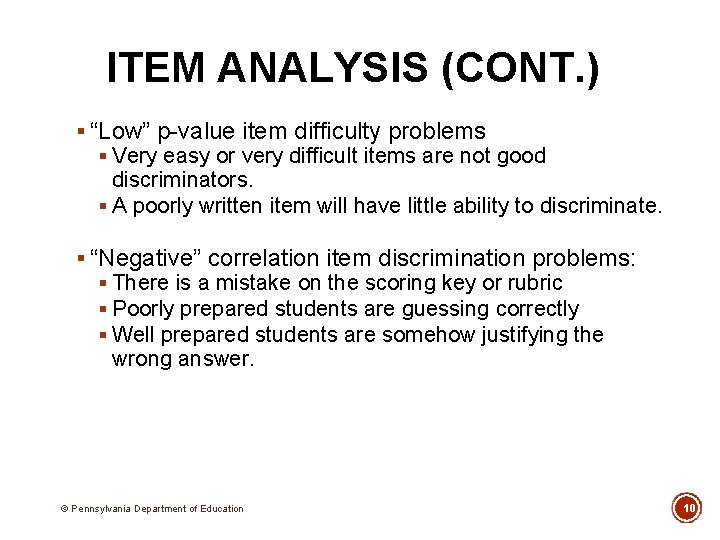
ITEM ANALYSIS (CONT. ) § “Low” p-value item difficulty problems § Very easy or very difficult items are not good discriminators. § A poorly written item will have little ability to discriminate. § “Negative” correlation item discrimination problems: § There is a mistake on the scoring key or rubric § Poorly prepared students are guessing correctly § Well prepared students are somehow justifying the wrong answer. © Pennsylvania Department of Education 10
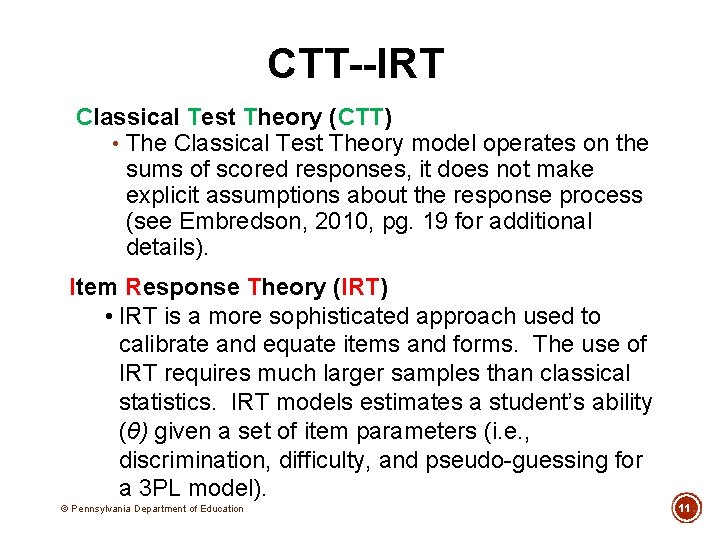
CTT--IRT Classical Test Theory (CTT) • The Classical Test Theory model operates on the sums of scored responses, it does not make explicit assumptions about the response process (see Embredson, 2010, pg. 19 for additional details). Item Response Theory (IRT) • IRT is a more sophisticated approach used to calibrate and equate items and forms. The use of IRT requires much larger samples than classical statistics. IRT models estimates a student’s ability (θ) given a set of item parameters (i. e. , discrimination, difficulty, and pseudo-guessing for a 3 PL model). © Pennsylvania Department of Education 11
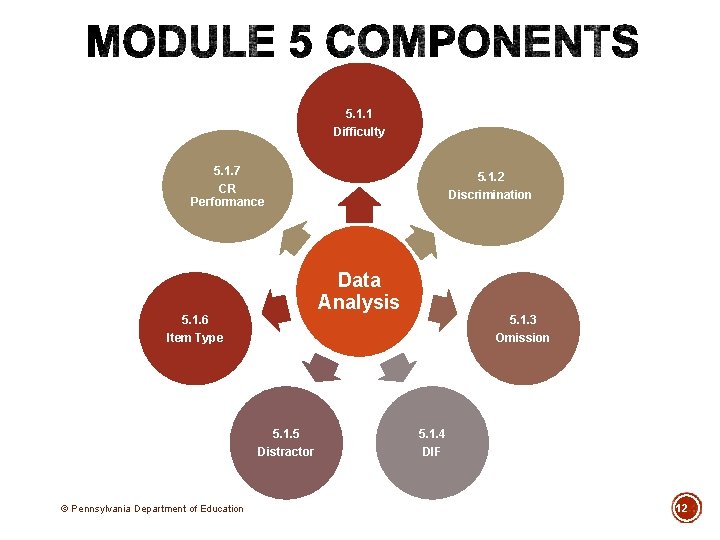
5. 1. 1 Difficulty 5. 1. 7 CR Performance Data Analysis 5. 1. 6 Item Type 5. 1. 5 Distractor © Pennsylvania Department of Education 5. 1. 2 Discrimination 5. 1. 3 Omission 5. 1. 4 DIF 12
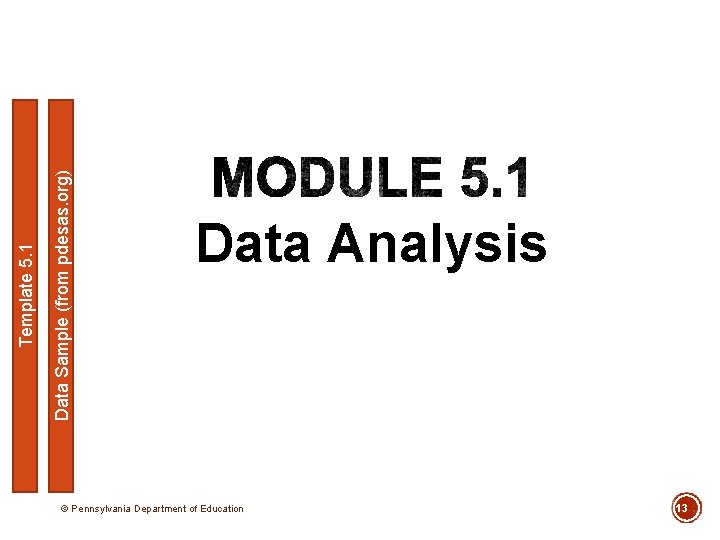
Data Sample (from pdesas. org) Template 5. 1 Data Analysis © Pennsylvania Department of Education 13
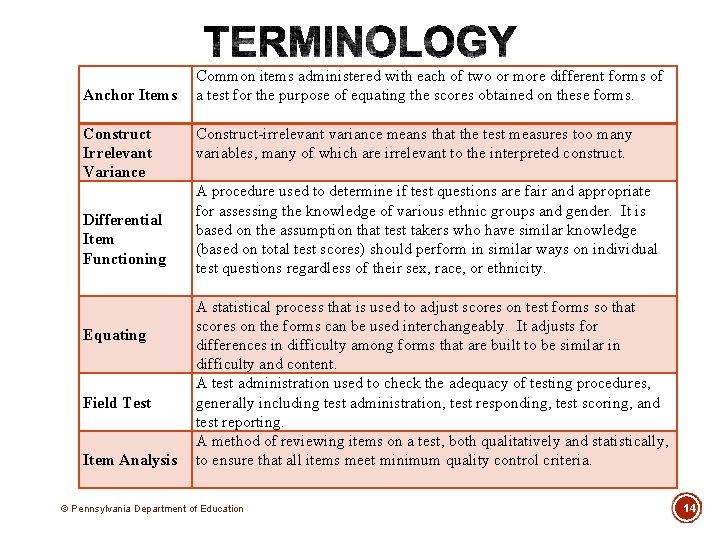
Anchor Items Construct Irrelevant Variance Differential Item Functioning Equating Field Test Item Analysis Common items administered with each of two or more different forms of a test for the purpose of equating the scores obtained on these forms. Construct-irrelevant variance means that the test measures too many variables, many of which are irrelevant to the interpreted construct. A procedure used to determine if test questions are fair and appropriate for assessing the knowledge of various ethnic groups and gender. It is based on the assumption that test takers who have similar knowledge (based on total test scores) should perform in similar ways on individual test questions regardless of their sex, race, or ethnicity. A statistical process that is used to adjust scores on test forms so that scores on the forms can be used interchangeably. It adjusts for differences in difficulty among forms that are built to be similar in difficulty and content. A test administration used to check the adequacy of testing procedures, generally including test administration, test responding, test scoring, and test reporting. A method of reviewing items on a test, both qualitatively and statistically, to ensure that all items meet minimum quality control criteria. © Pennsylvania Department of Education 14
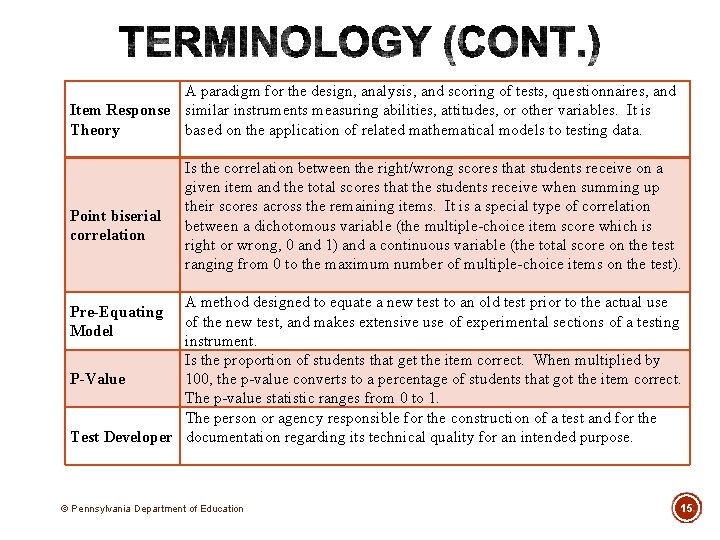
A paradigm for the design, analysis, and scoring of tests, questionnaires, and Item Response similar instruments measuring abilities, attitudes, or other variables. It is Theory based on the application of related mathematical models to testing data. Point biserial correlation Is the correlation between the right/wrong scores that students receive on a given item and the total scores that the students receive when summing up their scores across the remaining items. It is a special type of correlation between a dichotomous variable (the multiple-choice item score which is right or wrong, 0 and 1) and a continuous variable (the total score on the test ranging from 0 to the maximum number of multiple-choice items on the test). A method designed to equate a new test to an old test prior to the actual use of the new test, and makes extensive use of experimental sections of a testing instrument. Is the proportion of students that get the item correct. When multiplied by 100, the p-value converts to a percentage of students that got the item correct. P-Value The p-value statistic ranges from 0 to 1. The person or agency responsible for the construction of a test and for the Test Developer documentation regarding its technical quality for an intended purpose. Pre-Equating Model © Pennsylvania Department of Education 15
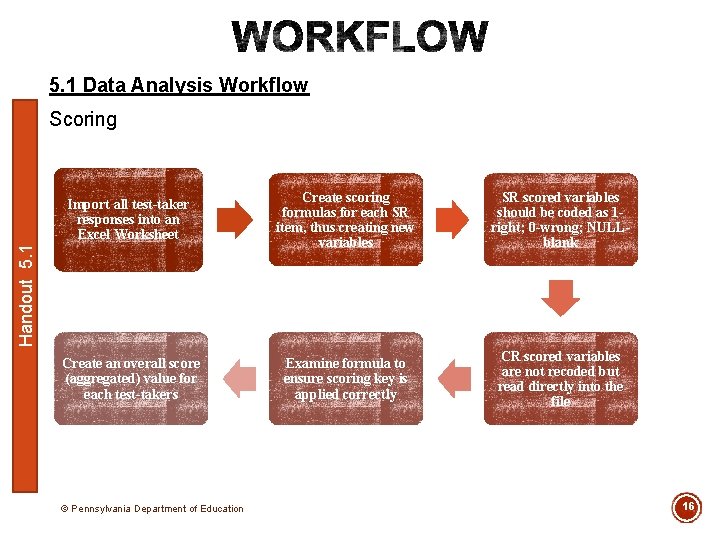
5. 1 Data Analysis Workflow Scoring Create scoring formulas for each SR item, thus creating new variables SR scored variables should be coded as 1 right; 0 -wrong; NULLblank Create an overall score (aggregated) value for each test-takers Examine formula to ensure scoring key is applied correctly CR scored variables are not recoded but read directly into the file Handout 5. 1 Import all test-taker responses into an Excel Worksheet © Pennsylvania Department of Education 16
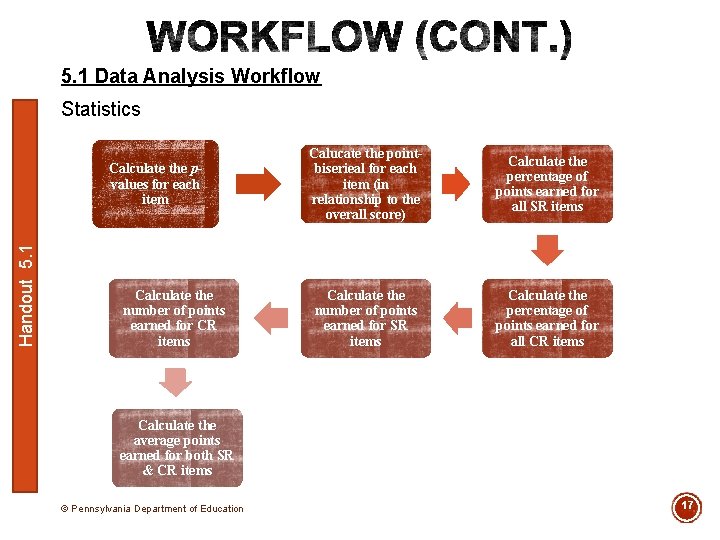
5. 1 Data Analysis Workflow Statistics Handout 5. 1 Calculate the pvalues for each item Calculate the number of points earned for CR items Calucate the pointbiserieal for each item (in relationship to the overall score) Calculate the percentage of points earned for all SR items Calculate the number of points earned for SR items Calculate the percentage of points earned for all CR items Calculate the average points earned for both SR & CR items © Pennsylvania Department of Education 17
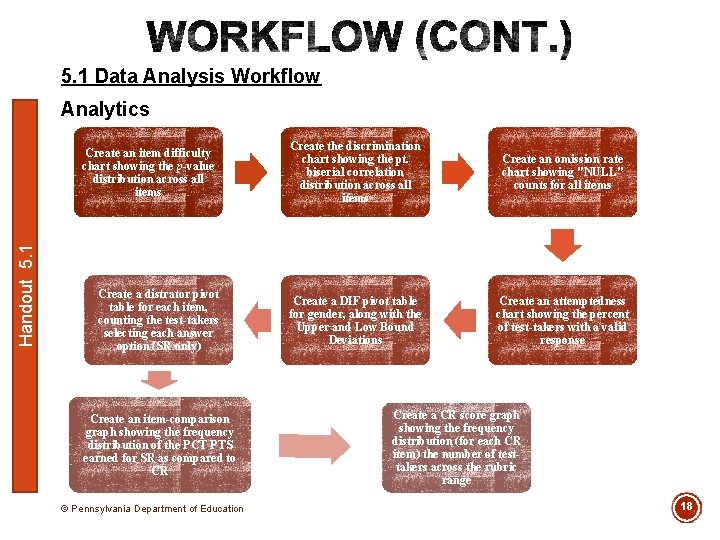
5. 1 Data Analysis Workflow Analytics Handout 5. 1 Create an item difficulty chart showing the p-value distribution across all items Create a distrator pivot table for each item, counting the test-takers selecting each answer option (SR only) Create an item-comparison graph showing the frequency distribution of the PCT PTS earned for SR as compared to CR © Pennsylvania Department of Education Create the discrimination chart showing the pt. biserial correlation distribution across all items Create an omission rate chart showing "NULL" counts for all items Create a DIF pivot table for gender, along with the Upper and Low Bound Deviations Create an attemptedness chart showing the percent of test-takers with a valid response Create a CR score graph showing the frequency distribution (for each CR item) the number of testtakers across the rubric range 18
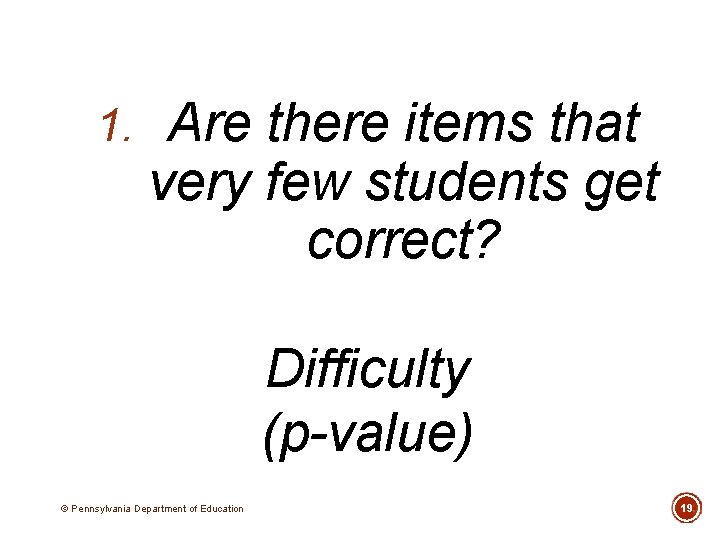
1. Are there items that very few students get correct? Difficulty (p-value) © Pennsylvania Department of Education 19
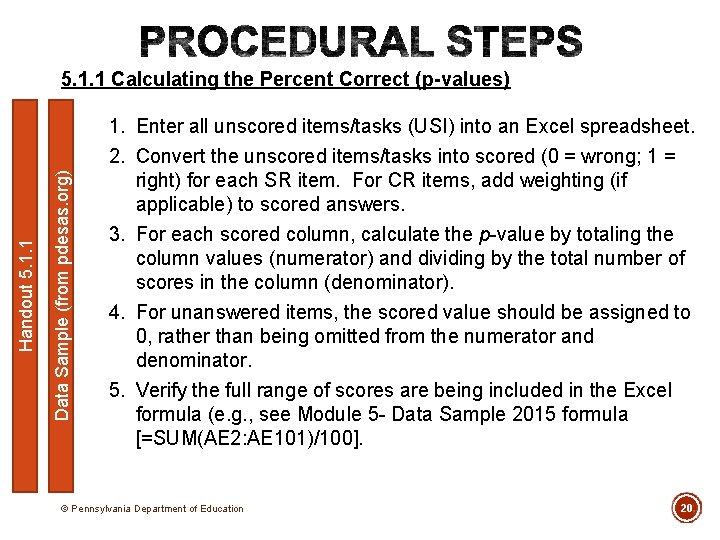
Data Sample (from pdesas. org) Handout 5. 1. 1 Calculating the Percent Correct (p-values) 1. Enter all unscored items/tasks (USI) into an Excel spreadsheet. 2. Convert the unscored items/tasks into scored (0 = wrong; 1 = right) for each SR item. For CR items, add weighting (if applicable) to scored answers. 3. For each scored column, calculate the p-value by totaling the column values (numerator) and dividing by the total number of scores in the column (denominator). 4. For unanswered items, the scored value should be assigned to 0, rather than being omitted from the numerator and denominator. 5. Verify the full range of scores are being included in the Excel formula (e. g. , see Module 5 - Data Sample 2015 formula [=SUM(AE 2: AE 101)/100]. © Pennsylvania Department of Education 20
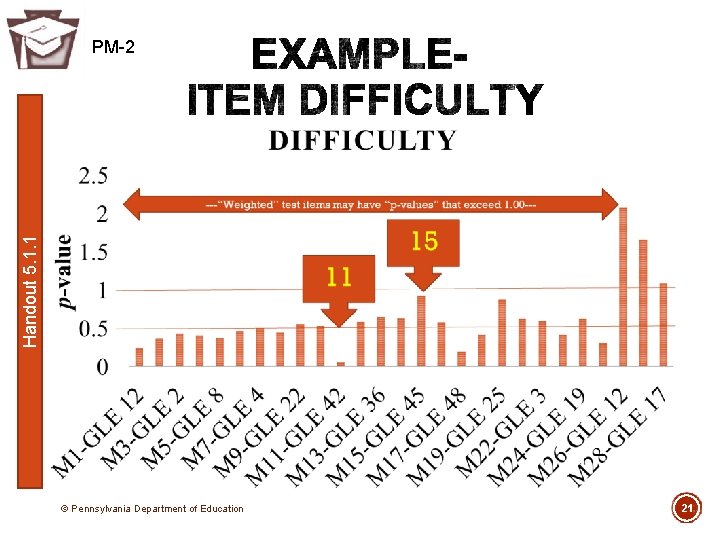
Handout 5. 1. 1 PM-2 © Pennsylvania Department of Education 21
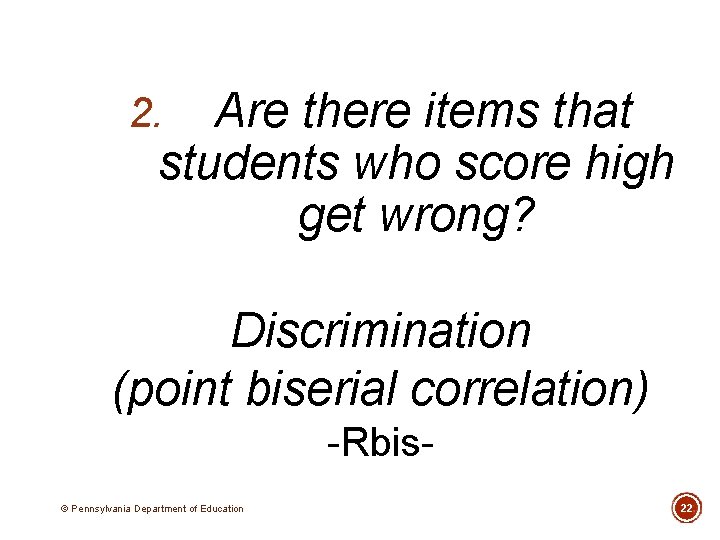
Are there items that students who score high get wrong? 2. Discrimination (point biserial correlation) -Rbis© Pennsylvania Department of Education 22
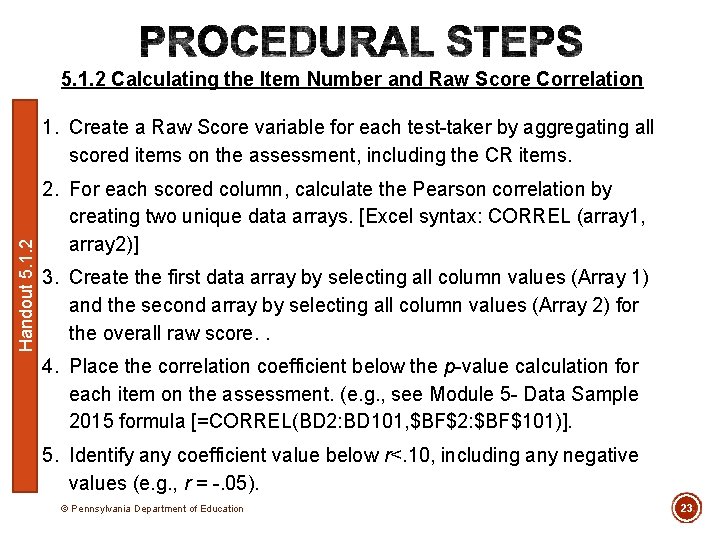
5. 1. 2 Calculating the Item Number and Raw Score Correlation Handout 5. 1. 2 1. Create a Raw Score variable for each test-taker by aggregating all scored items on the assessment, including the CR items. 2. For each scored column, calculate the Pearson correlation by creating two unique data arrays. [Excel syntax: CORREL (array 1, array 2)] 3. Create the first data array by selecting all column values (Array 1) and the second array by selecting all column values (Array 2) for the overall raw score. . 4. Place the correlation coefficient below the p-value calculation for each item on the assessment. (e. g. , see Module 5 - Data Sample 2015 formula [=CORREL(BD 2: BD 101, $BF$2: $BF$101)]. 5. Identify any coefficient value below r<. 10, including any negative values (e. g. , r = -. 05). © Pennsylvania Department of Education 23
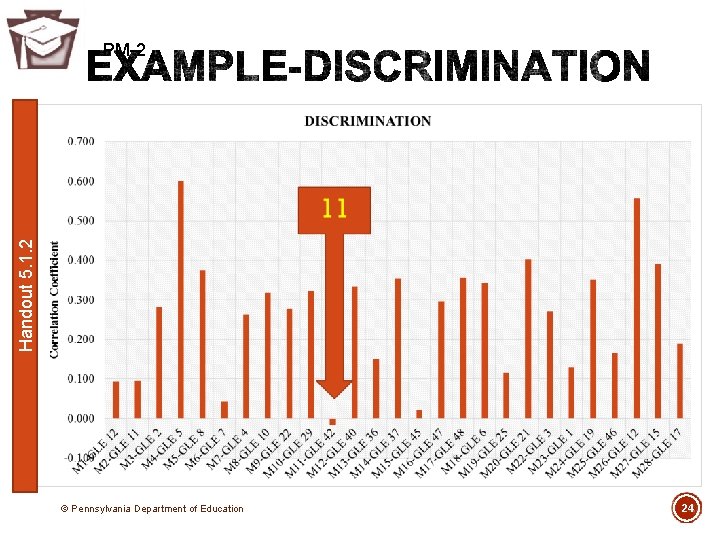
Handout 5. 1. 2 PM-2 © Pennsylvania Department of Education 24
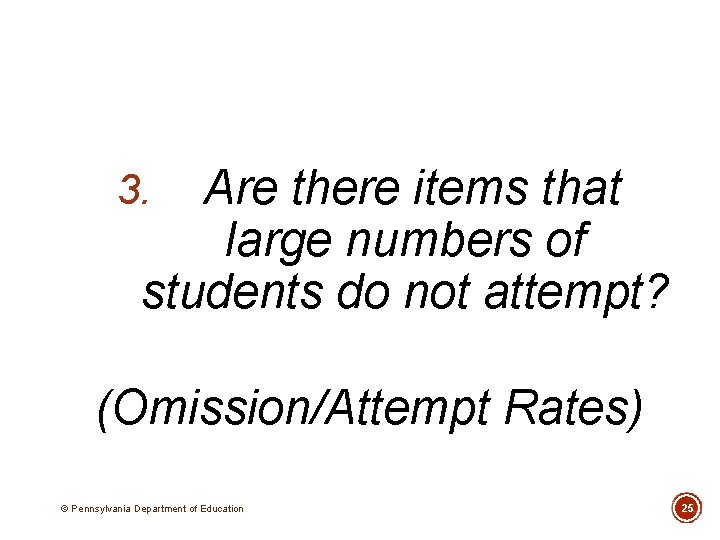
Are there items that large numbers of students do not attempt? 3. (Omission/Attempt Rates) © Pennsylvania Department of Education 25
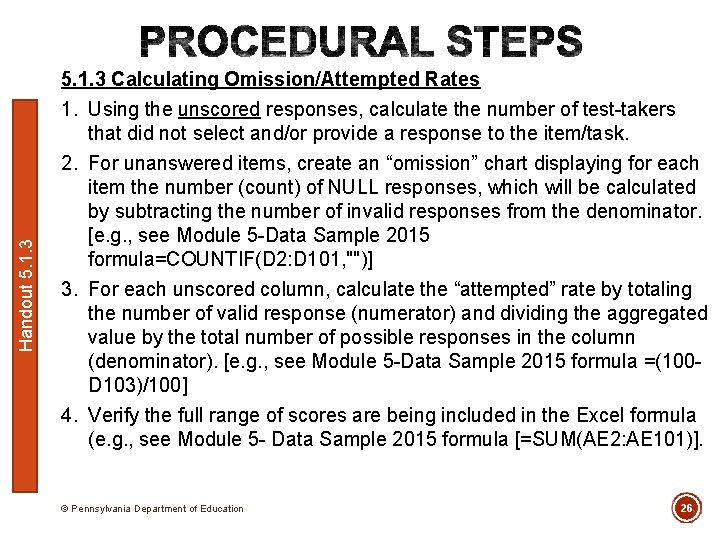
Handout 5. 1. 3 Calculating Omission/Attempted Rates 1. Using the unscored responses, calculate the number of test-takers that did not select and/or provide a response to the item/task. 2. For unanswered items, create an “omission” chart displaying for each item the number (count) of NULL responses, which will be calculated by subtracting the number of invalid responses from the denominator. [e. g. , see Module 5 -Data Sample 2015 formula=COUNTIF(D 2: D 101, "")] 3. For each unscored column, calculate the “attempted” rate by totaling the number of valid response (numerator) and dividing the aggregated value by the total number of possible responses in the column (denominator). [e. g. , see Module 5 -Data Sample 2015 formula =(100 D 103)/100] 4. Verify the full range of scores are being included in the Excel formula (e. g. , see Module 5 - Data Sample 2015 formula [=SUM(AE 2: AE 101)]. © Pennsylvania Department of Education 26
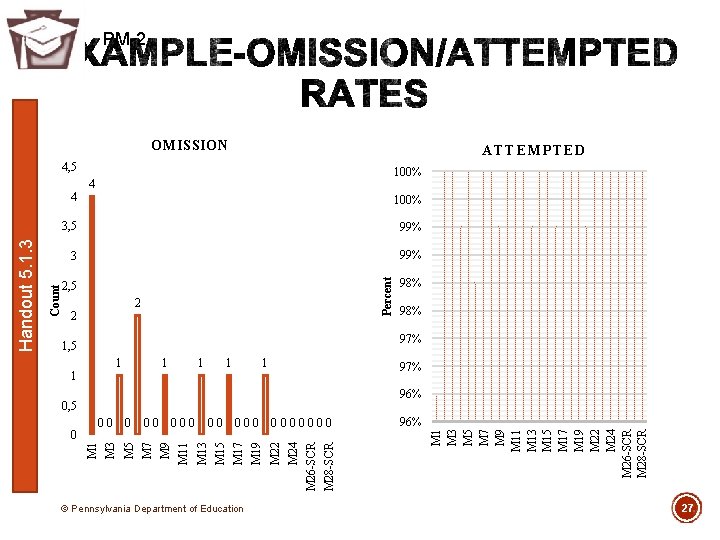
PM-2 OMISSION ATTEMPTED 4, 5 100% 3, 5 99% 3 99% 2, 5 98% Percent 2 2 97% 1, 5 1 1 1 97% 96% 0, 5 © Pennsylvania Department of Education M 28 -SCR M 26 -SCR M 24 0000000 M 22 M 19 000 M 17 M 15 00 M 13 M 11 000 M 9 00 M 7 0 M 5 M 3 00 M 1 0 98% 96% M 1 M 3 M 5 M 7 M 9 M 11 M 13 M 15 M 17 M 19 M 22 M 24 M 26 -SCR M 28 -SCR Count Handout 5. 1. 3 4 100% 4 27
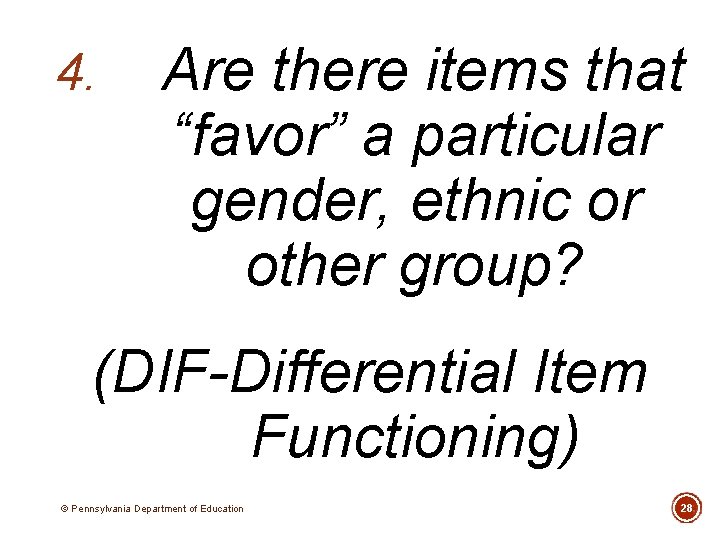
4. Are there items that “favor” a particular gender, ethnic or other group? (DIF-Differential Item Functioning) © Pennsylvania Department of Education 28
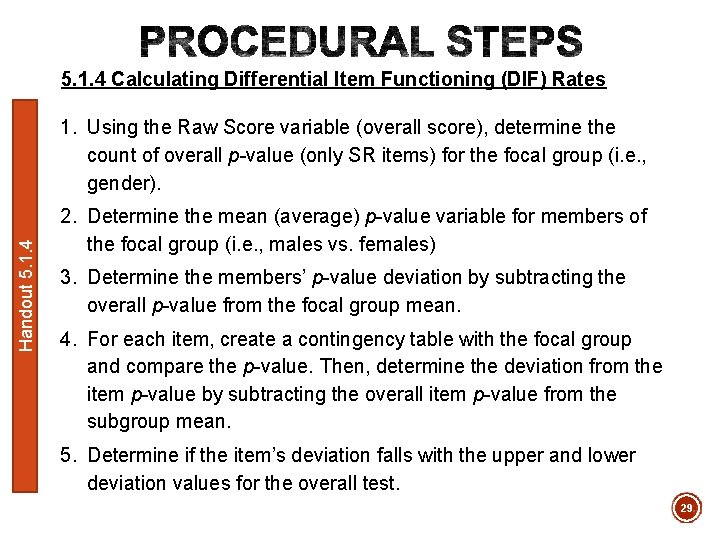
5. 1. 4 Calculating Differential Item Functioning (DIF) Rates Handout 5. 1. 4 1. Using the Raw Score variable (overall score), determine the count of overall p-value (only SR items) for the focal group (i. e. , gender). 2. Determine the mean (average) p-value variable for members of the focal group (i. e. , males vs. females) 3. Determine the members’ p-value deviation by subtracting the overall p-value from the focal group mean. 4. For each item, create a contingency table with the focal group and compare the p-value. Then, determine the deviation from the item p-value by subtracting the overall item p-value from the subgroup mean. 5. Determine if the item’s deviation falls with the upper and lower deviation values for the overall test. 29
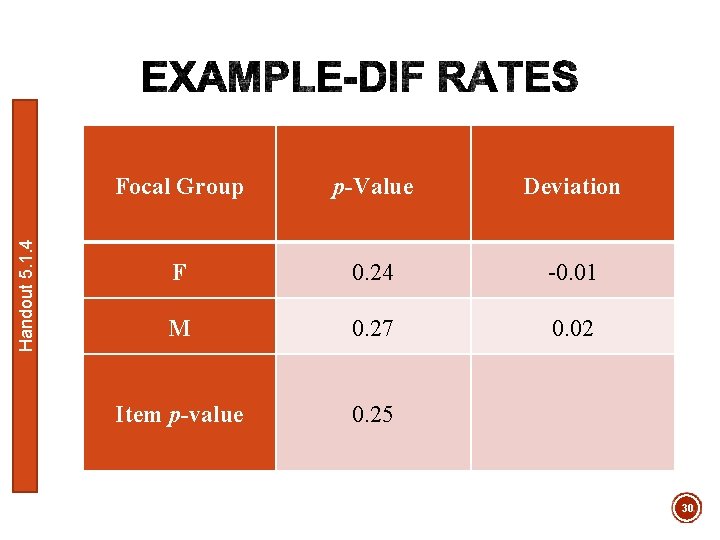
Handout 5. 1. 4 Focal Group p-Value Deviation F 0. 24 -0. 01 M 0. 27 0. 02 Item p-value 0. 25 30
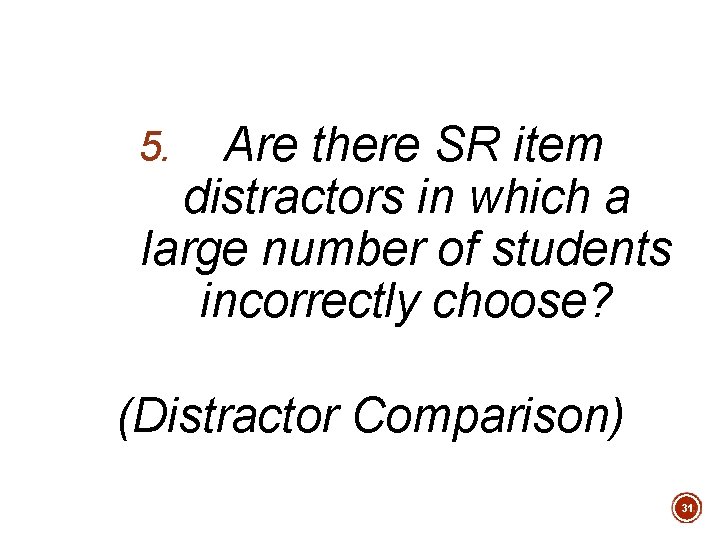
Are there SR item distractors in which a large number of students incorrectly choose? 5. (Distractor Comparison) 31
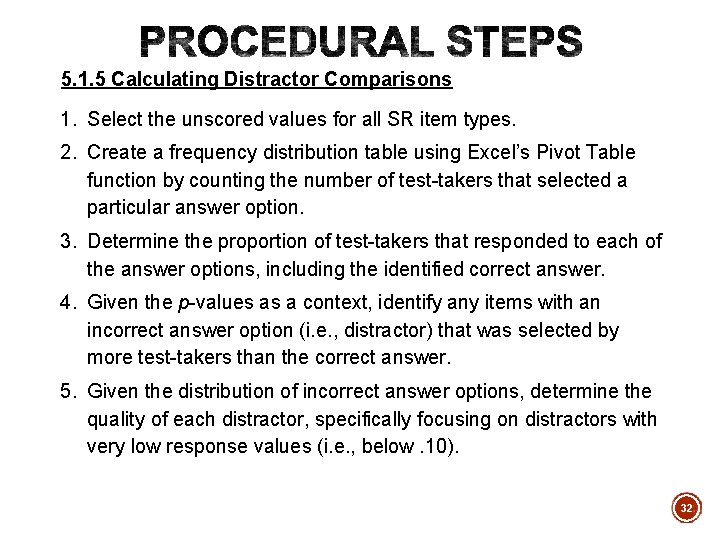
5. 1. 5 Calculating Distractor Comparisons 1. Select the unscored values for all SR item types. 2. Create a frequency distribution table using Excel’s Pivot Table function by counting the number of test-takers that selected a particular answer option. 3. Determine the proportion of test-takers that responded to each of the answer options, including the identified correct answer. 4. Given the p-values as a context, identify any items with an incorrect answer option (i. e. , distractor) that was selected by more test-takers than the correct answer. 5. Given the distribution of incorrect answer options, determine the quality of each distractor, specifically focusing on distractors with very low response values (i. e. , below. 10). 32
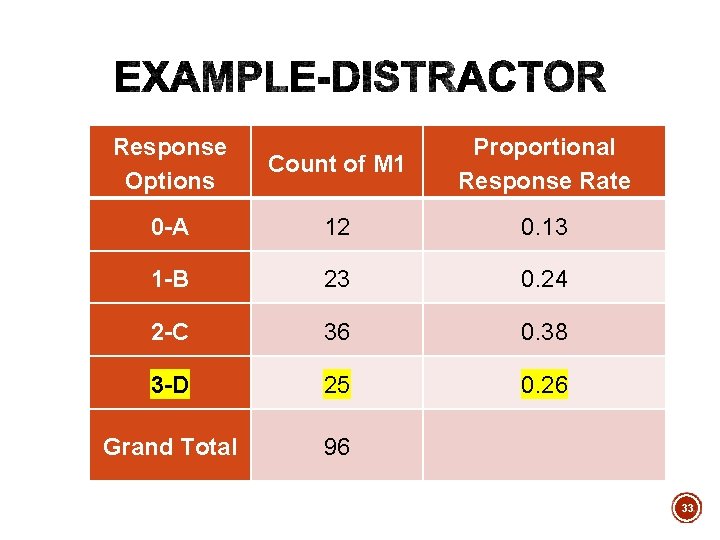
Response Options Count of M 1 Proportional Response Rate 0 -A 12 0. 13 1 -B 23 0. 24 2 -C 36 0. 38 3 -D 25 0. 26 Grand Total 96 33
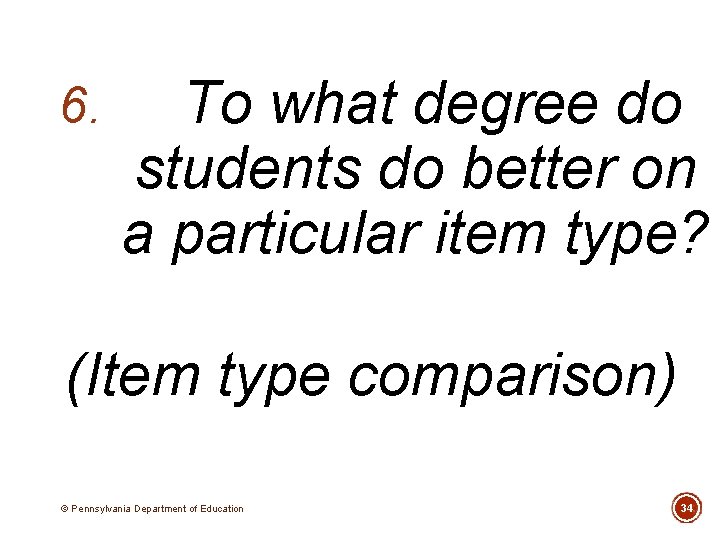
6. To what degree do students do better on a particular item type? (Item type comparison) © Pennsylvania Department of Education 34
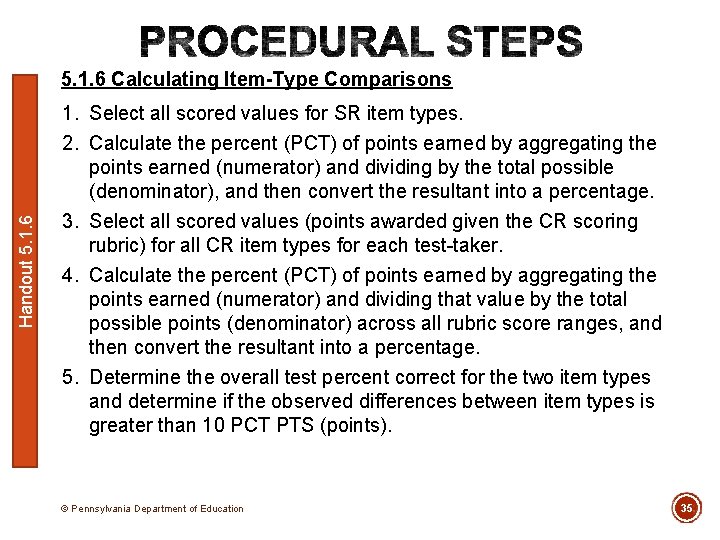
Handout 5. 1. 6 Calculating Item-Type Comparisons 1. Select all scored values for SR item types. 2. Calculate the percent (PCT) of points earned by aggregating the points earned (numerator) and dividing by the total possible (denominator), and then convert the resultant into a percentage. 3. Select all scored values (points awarded given the CR scoring rubric) for all CR item types for each test-taker. 4. Calculate the percent (PCT) of points earned by aggregating the points earned (numerator) and dividing that value by the total possible points (denominator) across all rubric score ranges, and then convert the resultant into a percentage. 5. Determine the overall test percent correct for the two item types and determine if the observed differences between item types is greater than 10 PCT PTS (points). © Pennsylvania Department of Education 35
![ITEM TYPE COMPARISON Percent Handout 5 1 6 Points 25SR Items Points 9SR Items ITEM TYPE COMPARISON Percent Handout 5. 1. 6 %Points [25]-SR Items %Points [9]-SR Items](https://slidetodoc.com/presentation_image_h2/c8dab11c50fad50fdfa52421045fdf7f/image-36.jpg)
ITEM TYPE COMPARISON Percent Handout 5. 1. 6 %Points [25]-SR Items %Points [9]-SR Items 100, 0% 90, 0% 80, 0% 70, 0% 60, 0% 50, 0% 40, 0% 30, 0% 20, 0% 10, 0% 1 6 11 16 21 26 31 36 41 46 51 56 61 66 71 76 81 86 91 96 Test-taker © Pennsylvania Department of Education 36
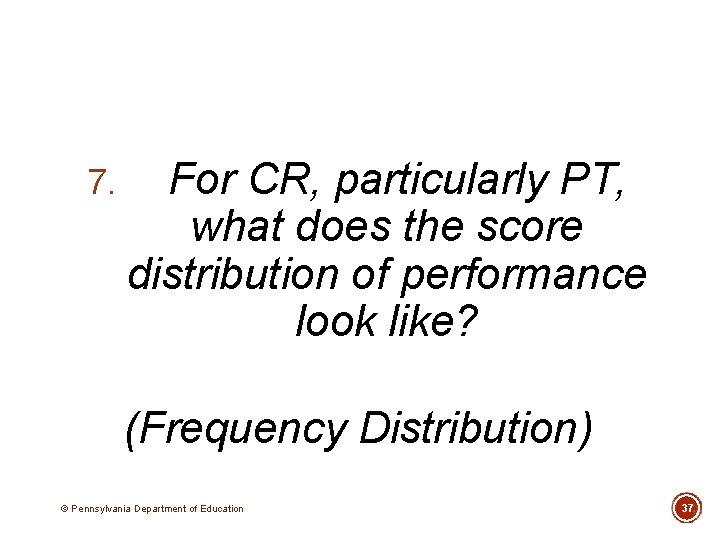
7. For CR, particularly PT, what does the score distribution of performance look like? (Frequency Distribution) © Pennsylvania Department of Education 37
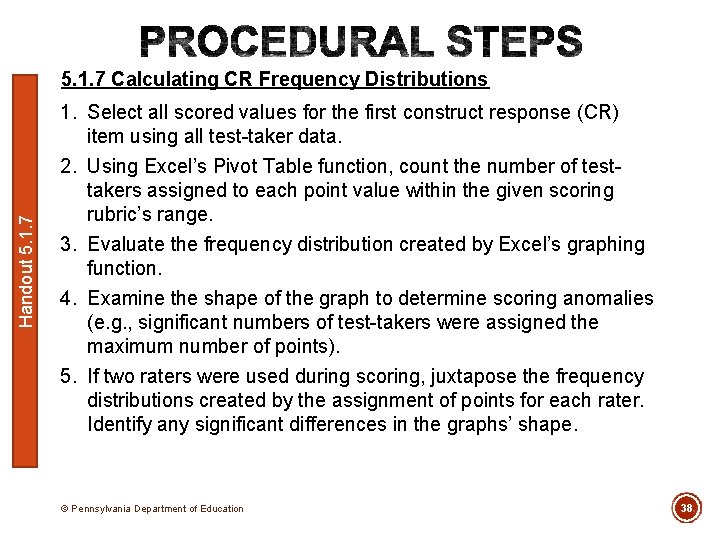
Handout 5. 1. 7 Calculating CR Frequency Distributions 1. Select all scored values for the first construct response (CR) item using all test-taker data. 2. Using Excel’s Pivot Table function, count the number of testtakers assigned to each point value within the given scoring rubric’s range. 3. Evaluate the frequency distribution created by Excel’s graphing function. 4. Examine the shape of the graph to determine scoring anomalies (e. g. , significant numbers of test-takers were assigned the maximum number of points). 5. If two raters were used during scoring, juxtapose the frequency distributions created by the assignment of points for each rater. Identify any significant differences in the graphs’ shape. © Pennsylvania Department of Education 38
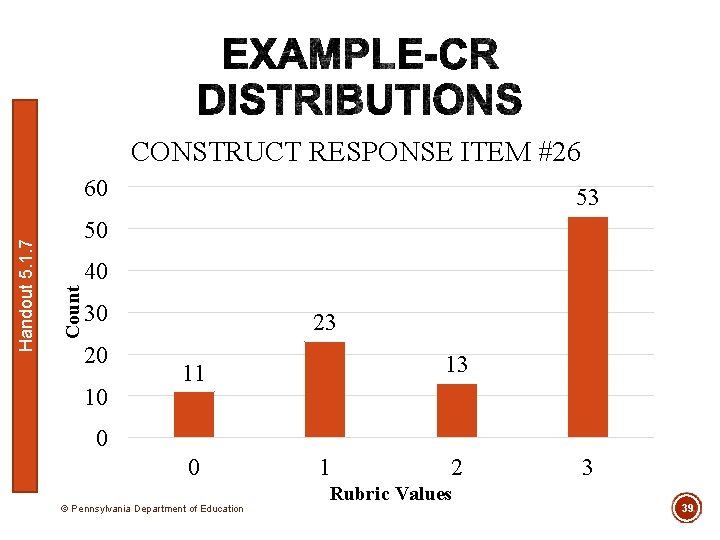
CONSTRUCT RESPONSE ITEM #26 53 50 40 Count Handout 5. 1. 7 60 30 20 10 23 13 11 0 0 © Pennsylvania Department of Education 1 2 Rubric Values 3 39
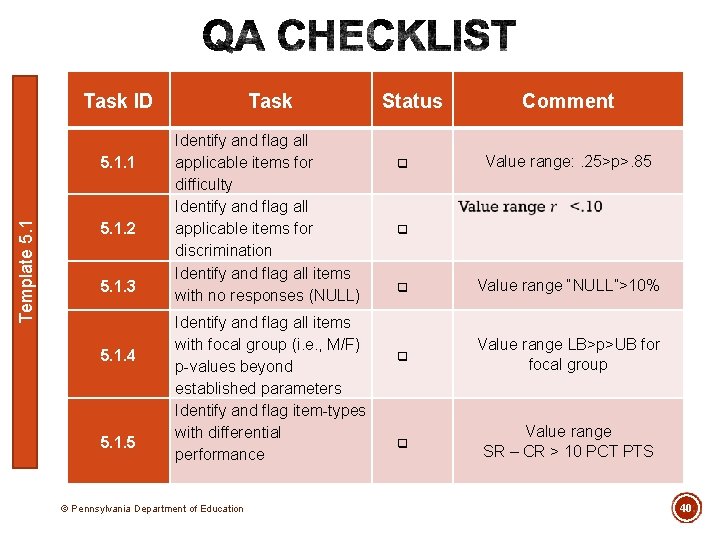
Task ID Template 5. 1. 1 5. 1. 2 5. 1. 3 5. 1. 4 5. 1. 5 Task Identify and flag all applicable items for difficulty Identify and flag all applicable items for discrimination Identify and flag all items with no responses (NULL) Identify and flag all items with focal group (i. e. , M/F) p-values beyond established parameters Identify and flag item-types with differential performance © Pennsylvania Department of Education Status Comment Value range: . 25>p>. 85 Value range “NULL”>10% Value range LB>p>UB for focal group Value range SR – CR > 10 PCT PTS 40
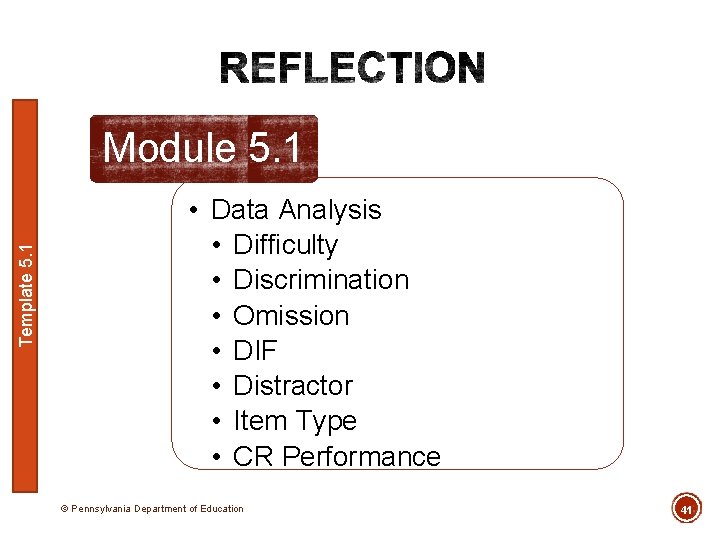
Template 5. 1 Module 5. 1 • Data Analysis • Difficulty • Discrimination • Omission • DIF • Distractor • Item Type • CR Performance © Pennsylvania Department of Education 41
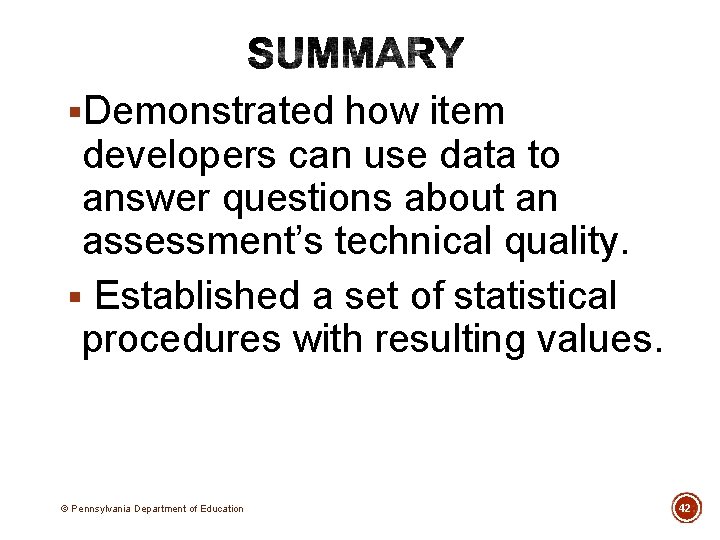
§Demonstrated how item developers can use data to answer questions about an assessment’s technical quality. § Established a set of statistical procedures with resulting values. © Pennsylvania Department of Education 42
Information and media literacy similarities
Media and information literacy meme
People as media
Cyber literacy and digital literacy
Media and information literacy module 6
C device module module 1
Module 12 sequences and series answers
Acadience benchmark goals
Digitalliteracyassessment
Www.digitalliteracyassessment.org
Maclaurin series vs taylor series
Heisenberg 1925 paper
Taylor series of composite function
Deret maclaurin
Ibm p series vs i series
Shunt feedback amplifier analysis
Series aiding and series opposing
Sum of infinite series formula
Self assessment module 3
Teaching and assessment of grammar module 1
Wam.fldoe.org login
Occupational health and safety assessment series
Say yes tobias wolff summary
What is the message in this cartoon mil
Data literacy 101
Content analysis secondary data
Define data collection method
Data preparation and basic data analysis
Data acquisition and data analysis
Vertical analysis interpretation
Udise+ data entry module
Finding answers through data collection
Data management module
Principle in portfolio assessment
Static assessment vs dynamic assessment
Portfolio assessment matches assessment to teaching
What are the main objectives of time series analysis
Applied time series analysis pdf
Sequence definition
Fourier series circuit analysis
Time series analysis using stata
Importance of time series analysis
Mention the components of time series