Text Mining IPR derived data and licensing SOPHIA
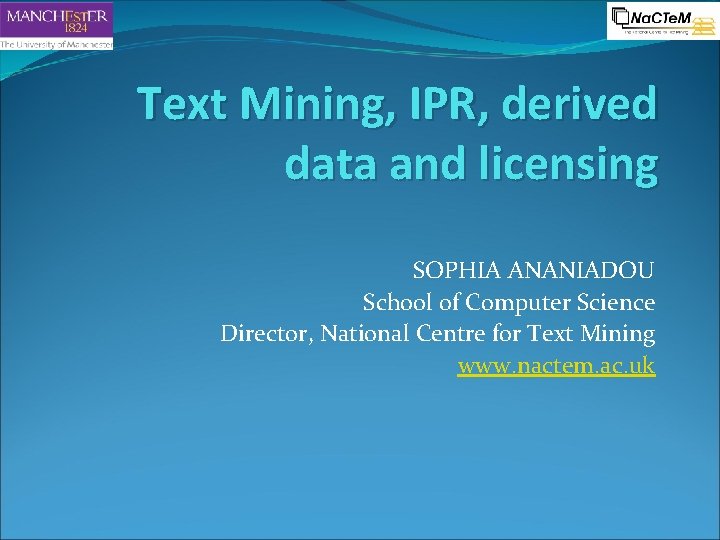
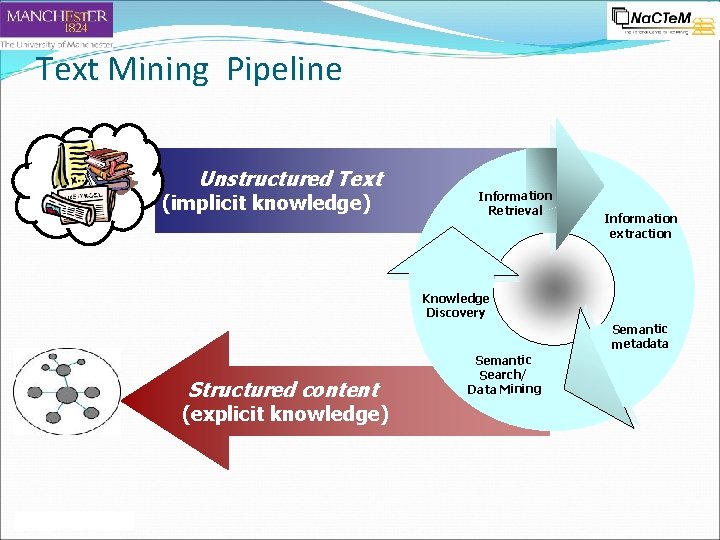
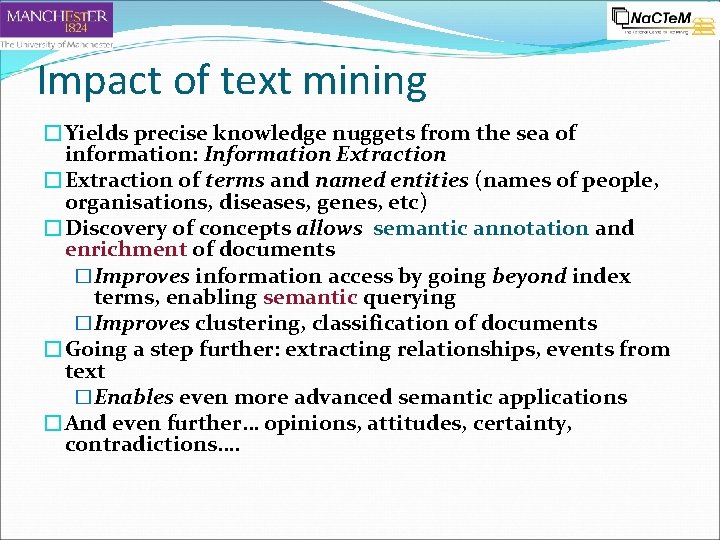
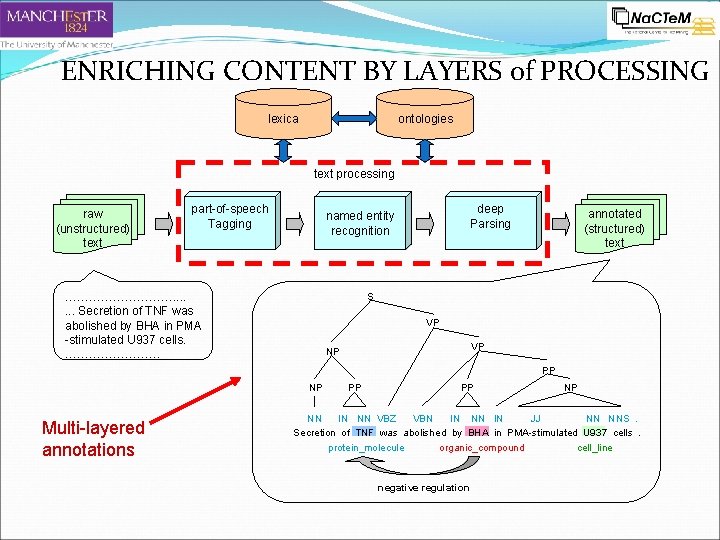
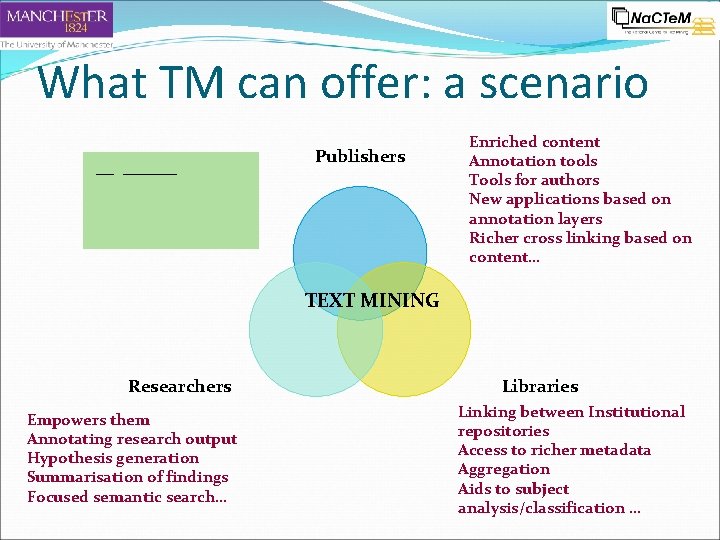
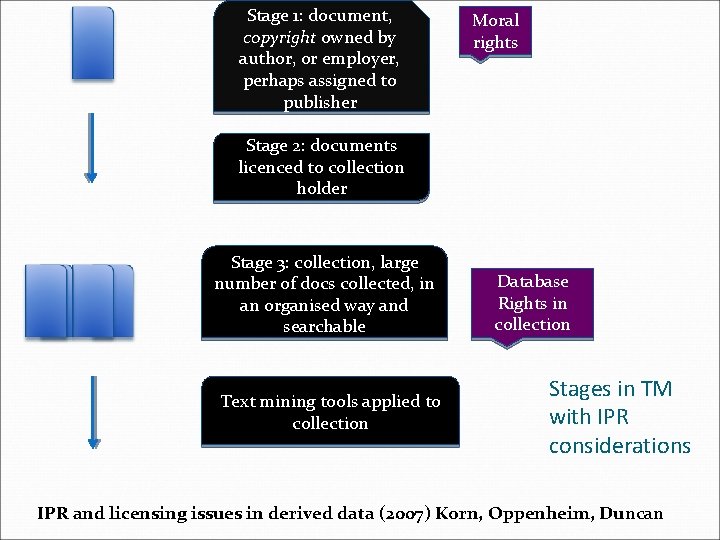
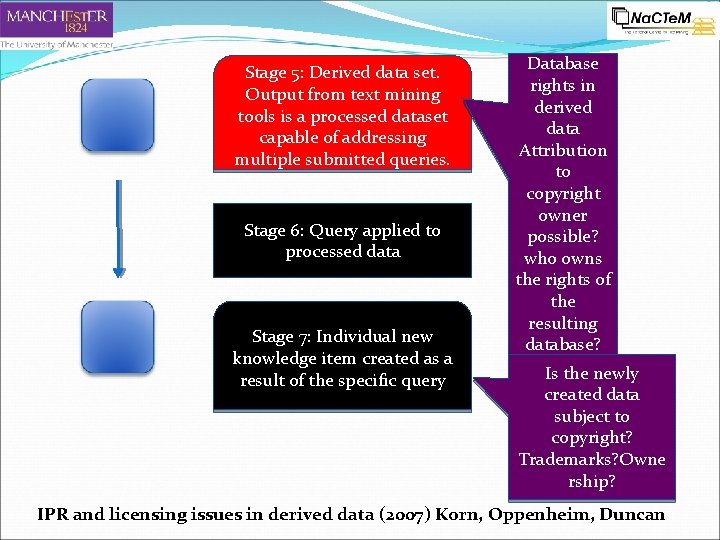
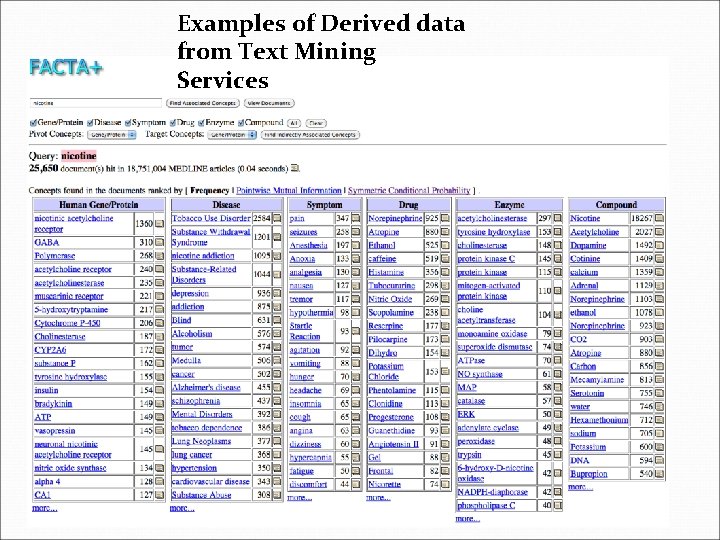
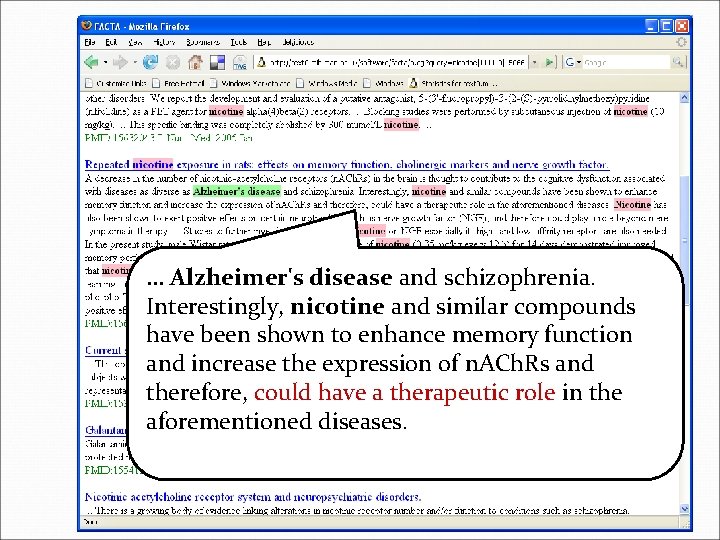
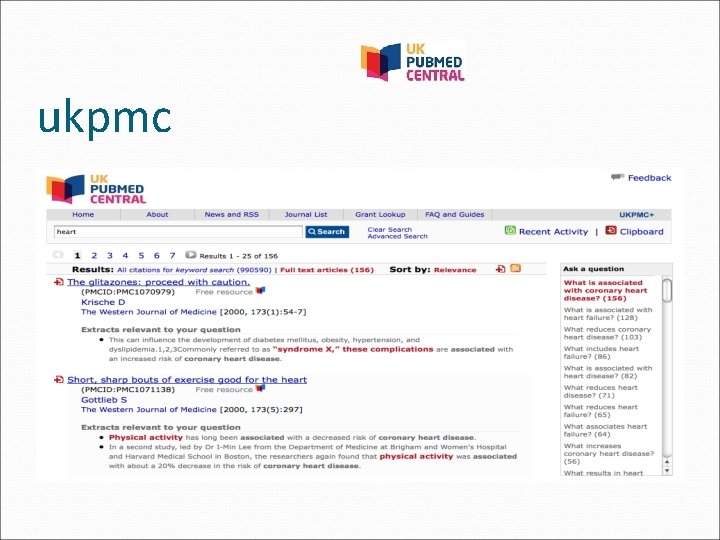
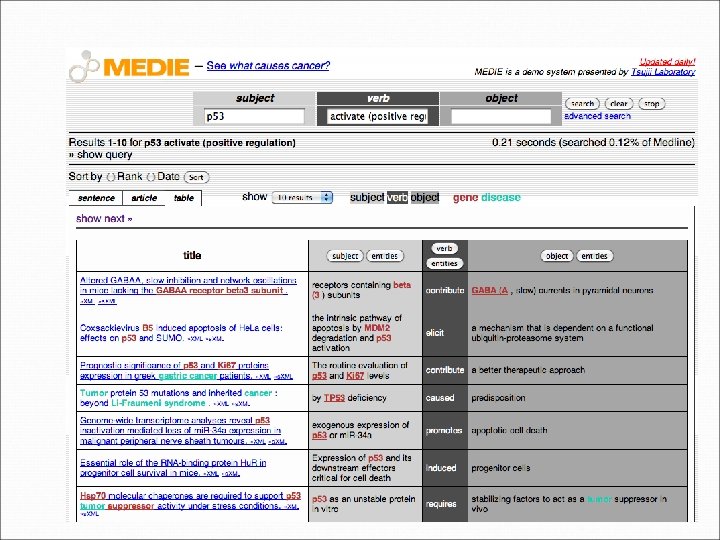
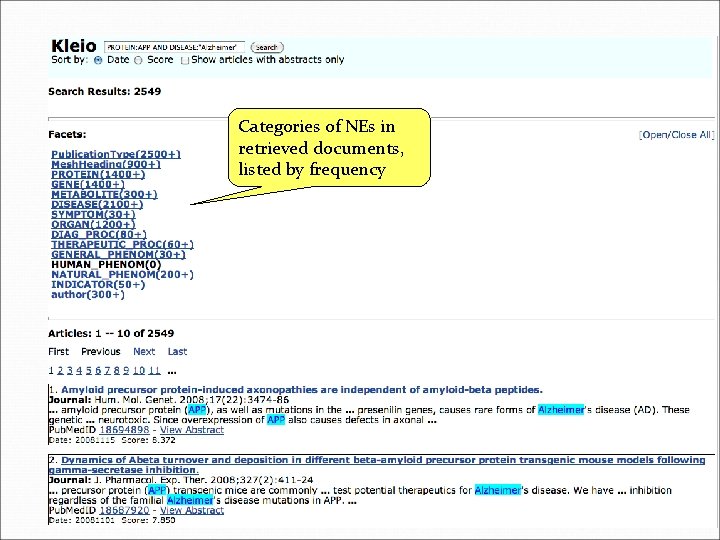
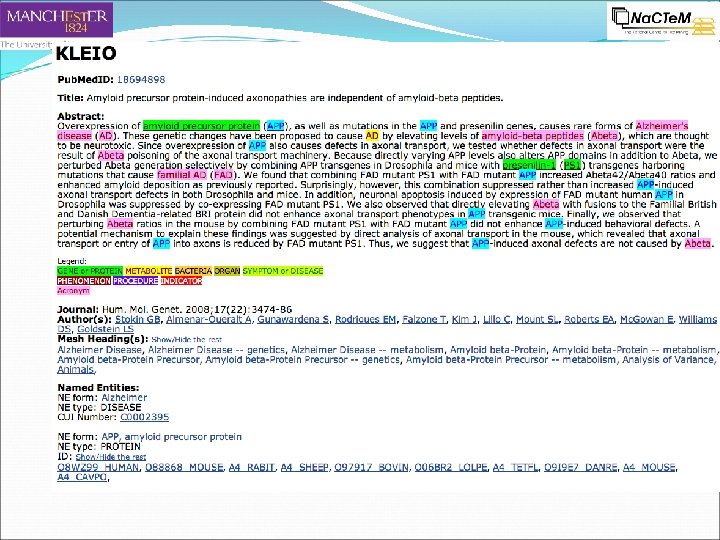
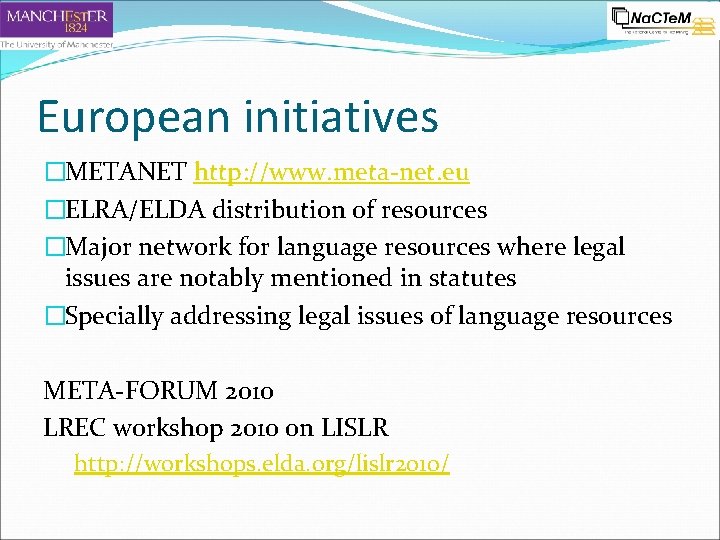
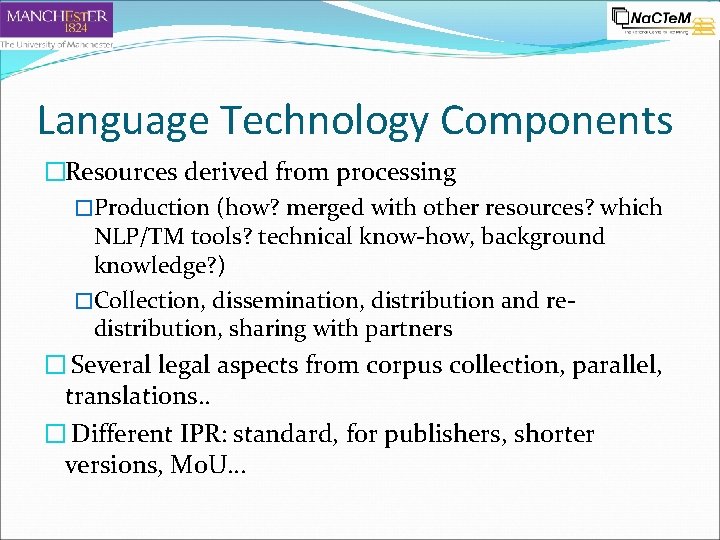
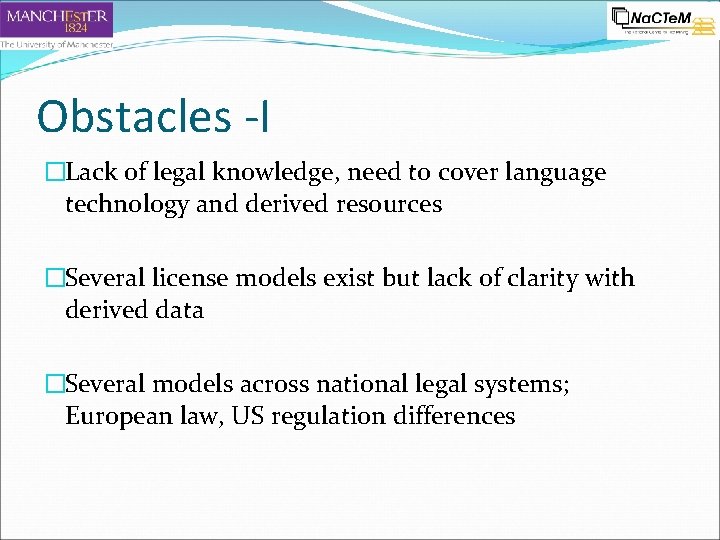
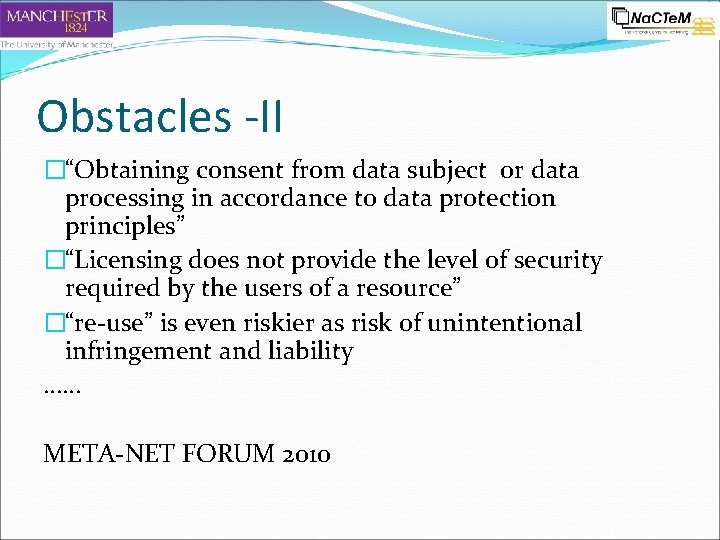
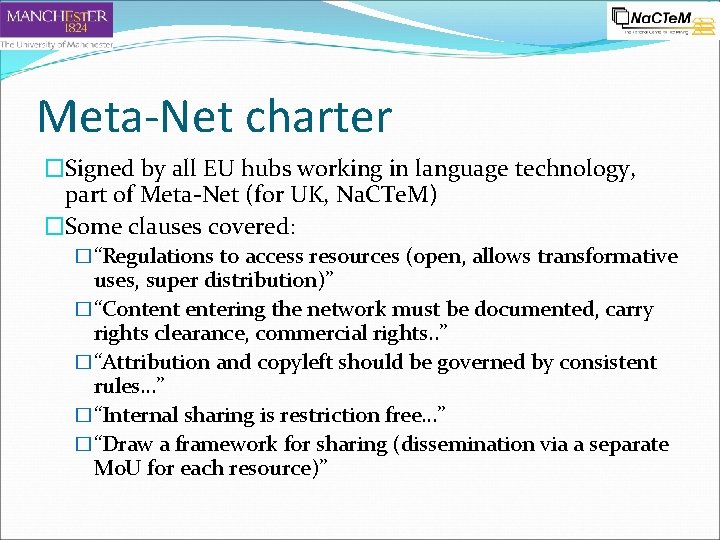
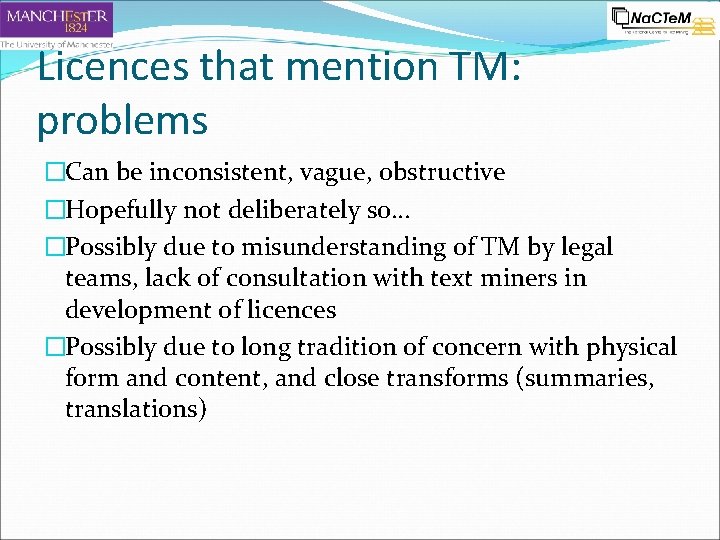
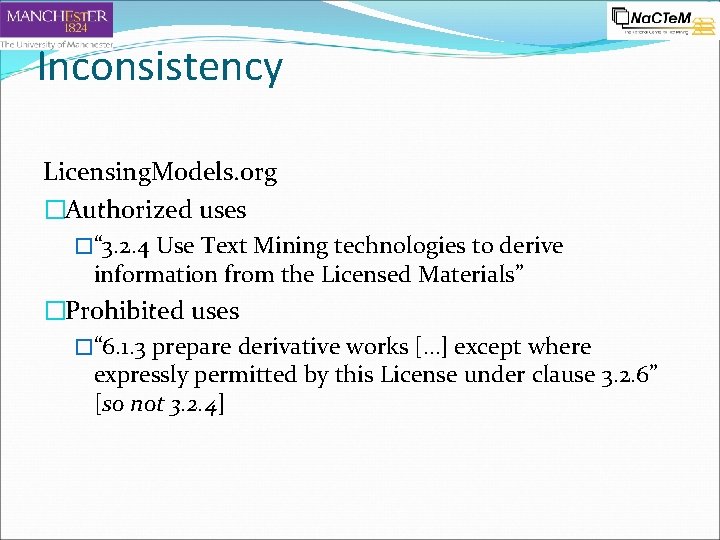
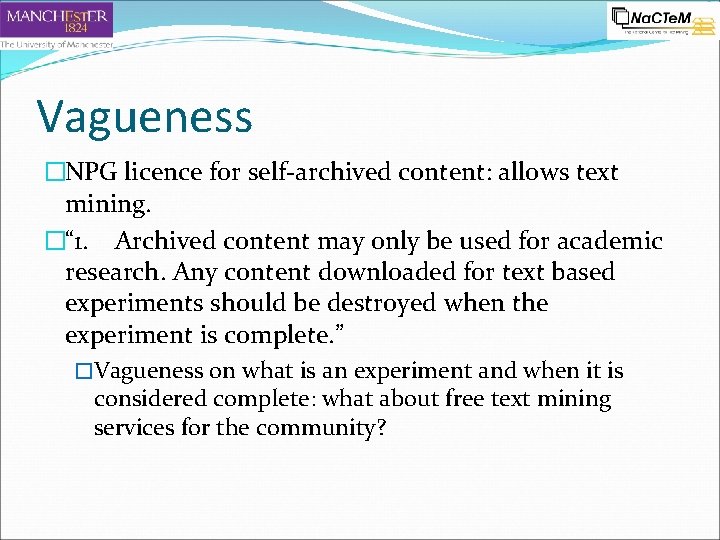
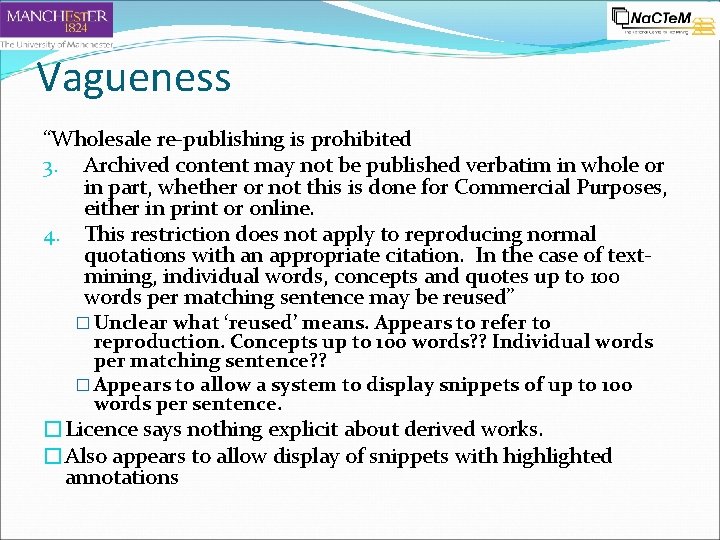
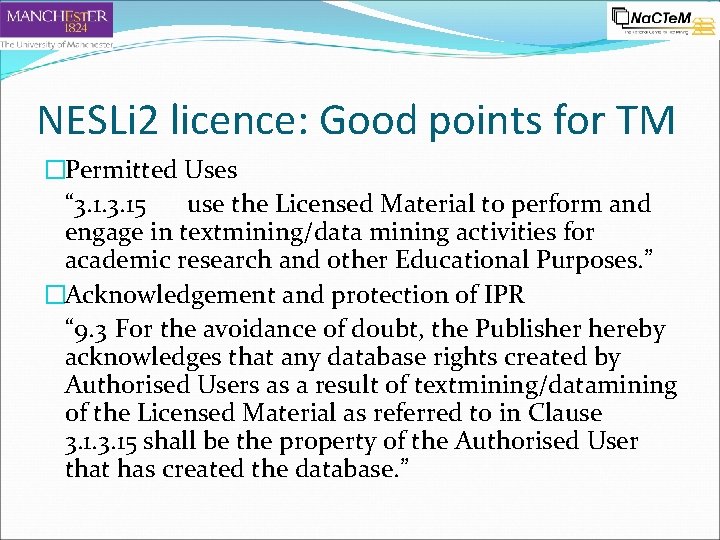
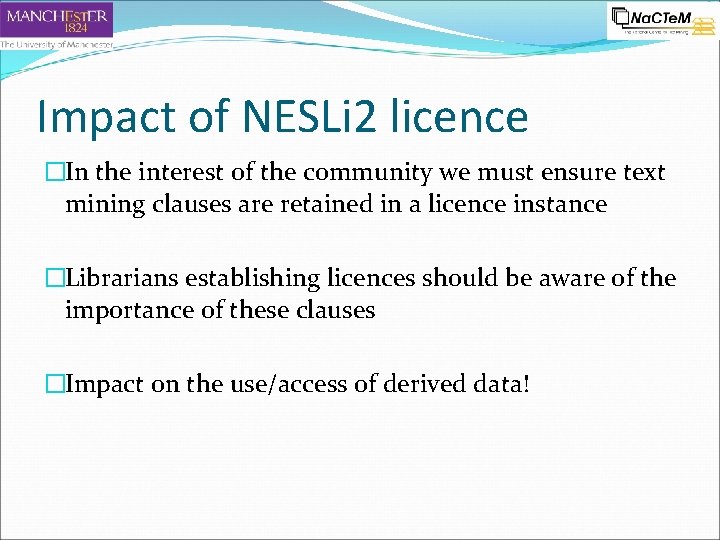
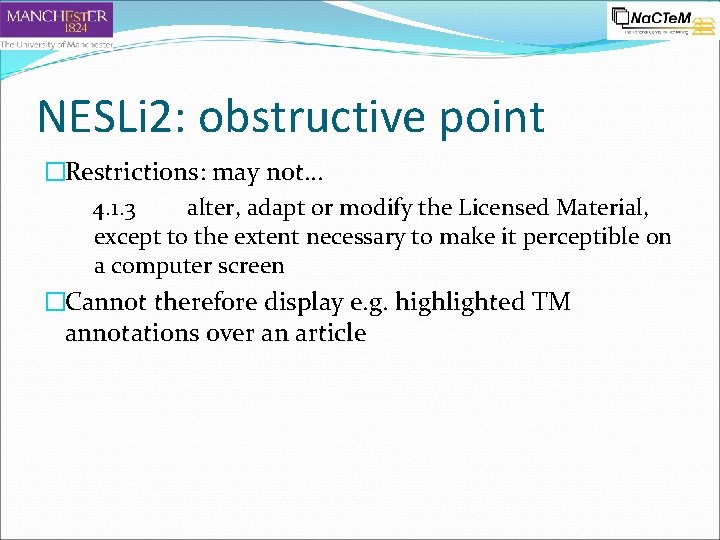
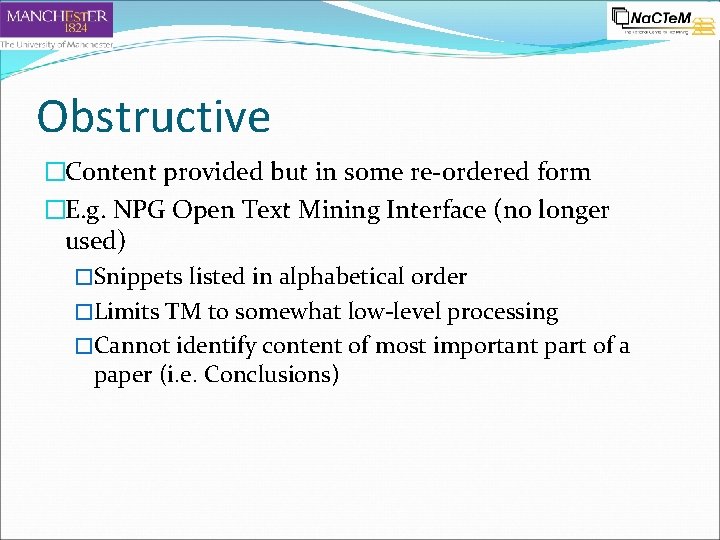
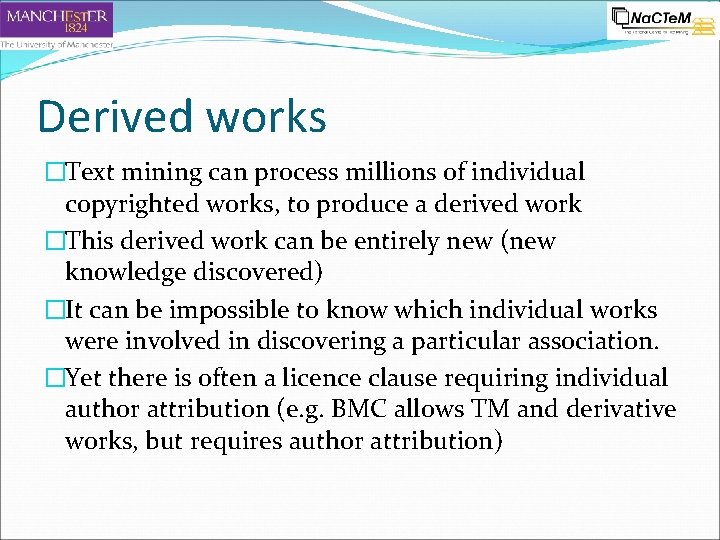
![Brown & Boulderstone “What is needed is a system [for scholarly communication] which allows: Brown & Boulderstone “What is needed is a system [for scholarly communication] which allows:](https://slidetodoc.com/presentation_image_h/f82dba9f8b04087d8fa254ec21a6455d/image-28.jpg)
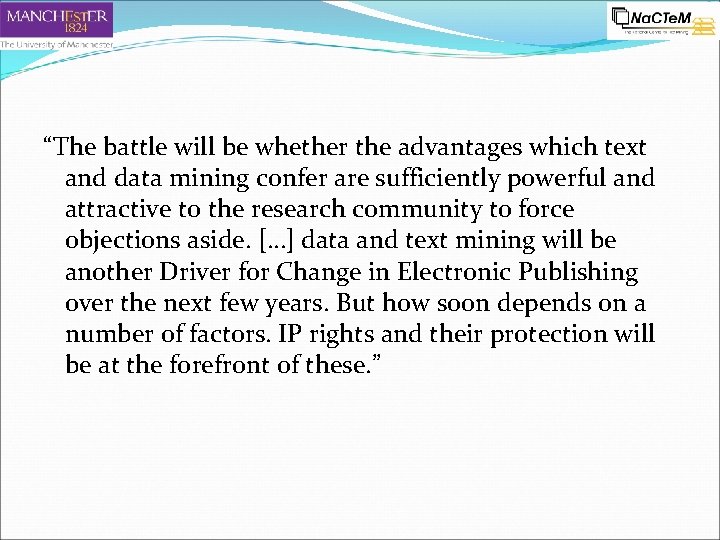
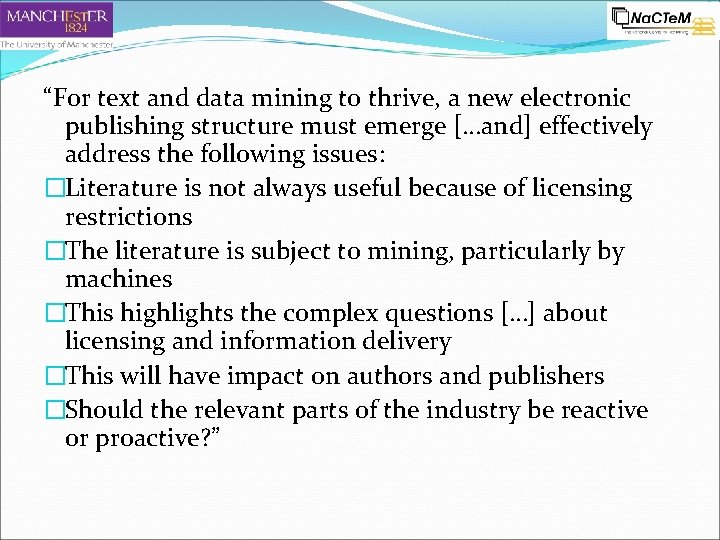
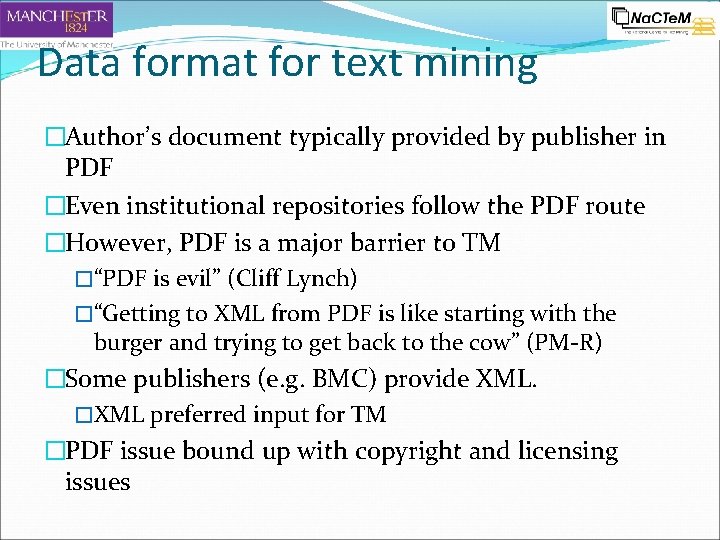
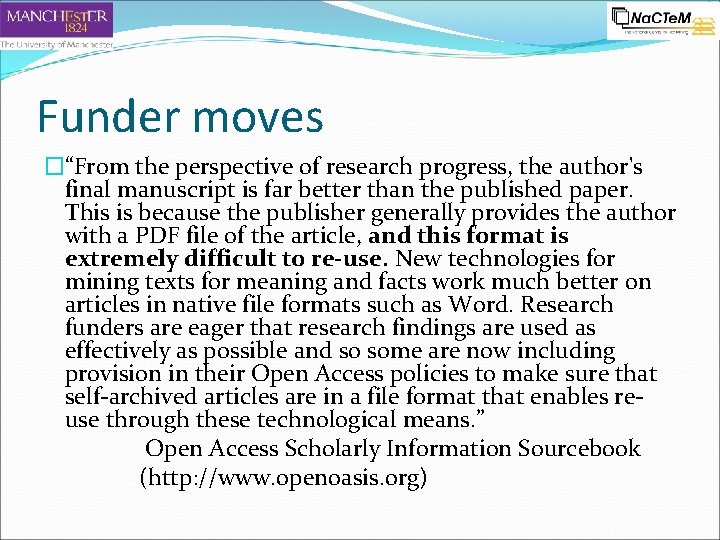
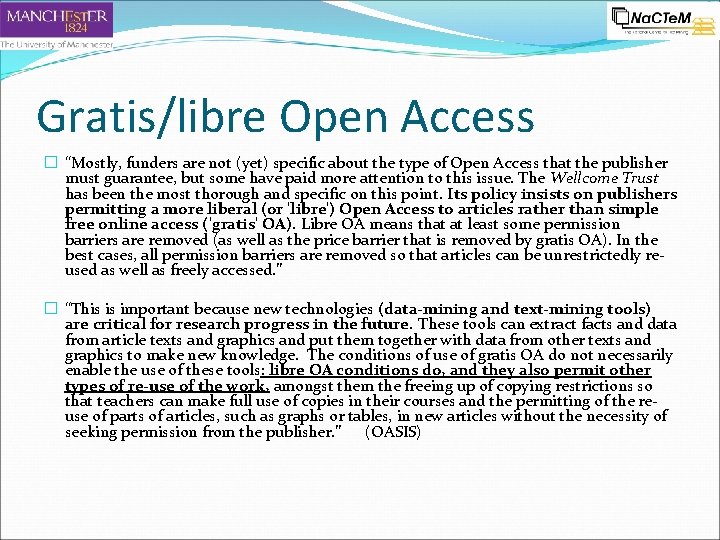
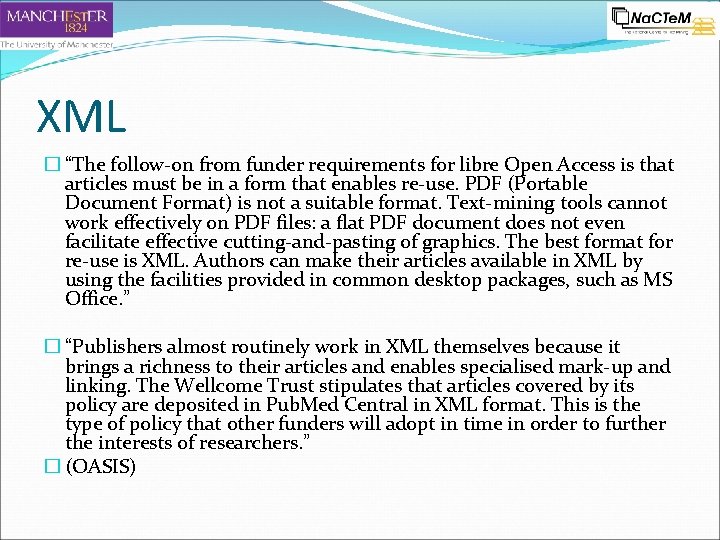
- Slides: 34
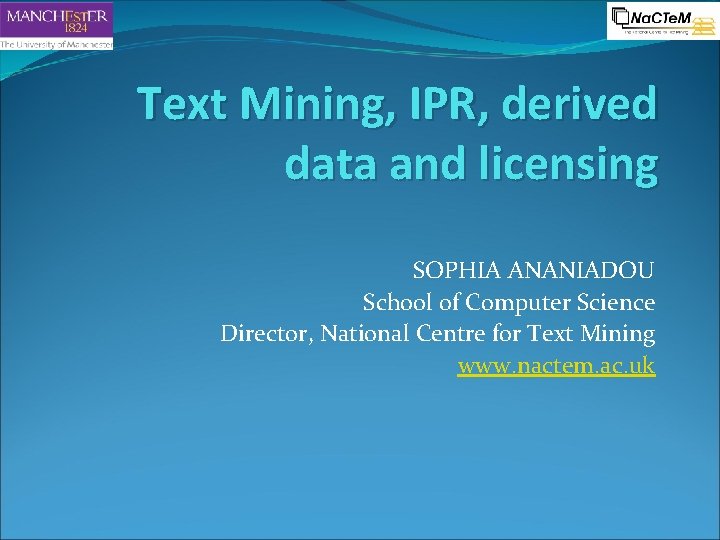
Text Mining, IPR, derived data and licensing SOPHIA ANANIADOU School of Computer Science Director, National Centre for Text Mining www. nactem. ac. uk
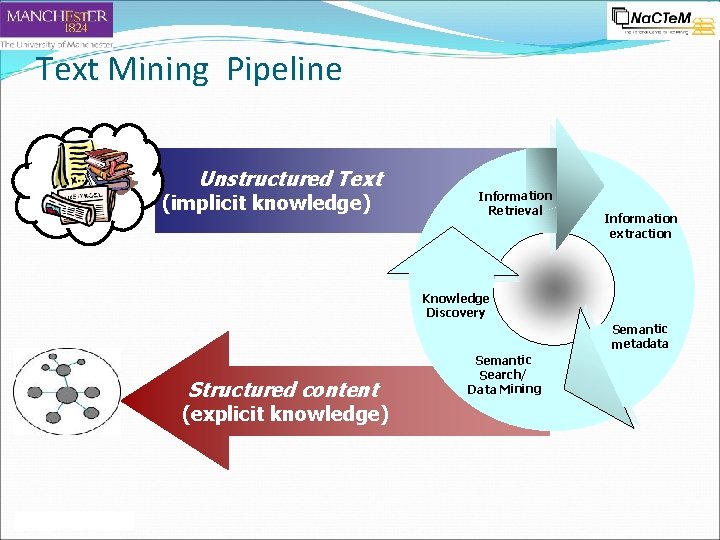
Text Mining Pipeline Unstructured Text (implicit knowledge) Information Retrieval Information extraction Knowledge Discovery Semantic metadata Structured content (explicit knowledge) Semantic Search/ Data Mining
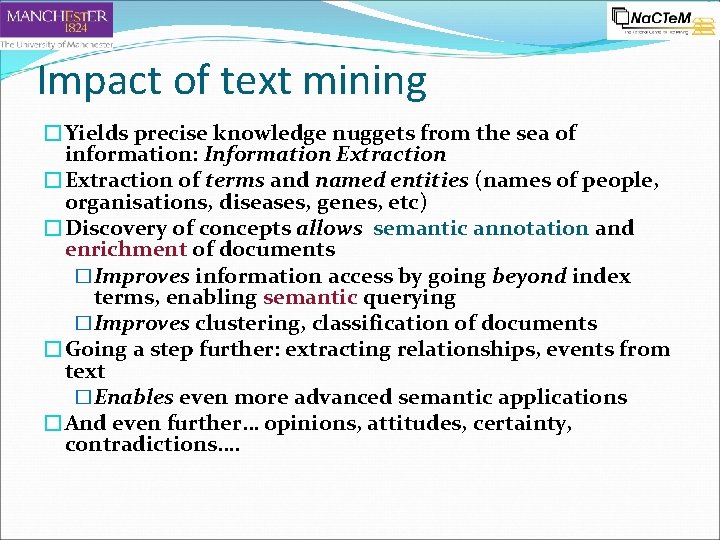
Impact of text mining �Yields precise knowledge nuggets from the sea of information: Information Extraction �Extraction of terms and named entities (names of people, organisations, diseases, genes, etc) �Discovery of concepts allows semantic annotation and enrichment of documents �Improves information access by going beyond index terms, enabling semantic querying �Improves clustering, classification of documents �Going a step further: extracting relationships, events from text �Enables even more advanced semantic applications �And even further… opinions, attitudes, certainty, contradictions….
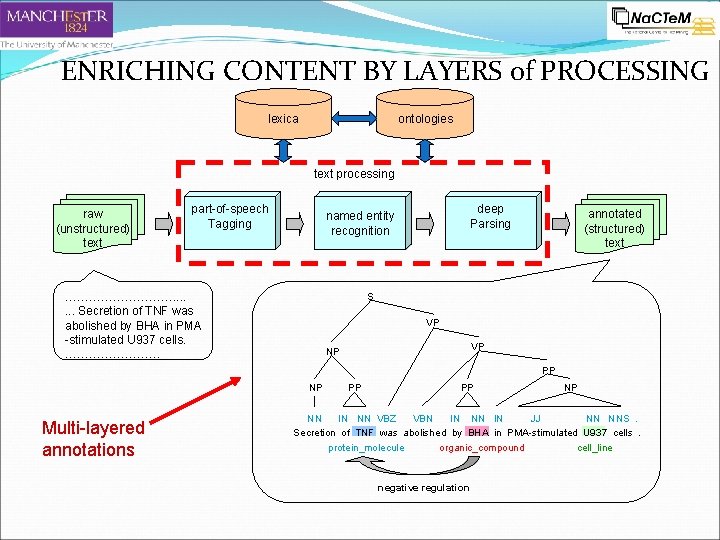
ENRICHING CONTENT BY LAYERS of PROCESSING lexica ontologies text processing raw (unstructured) text part-of-speech Tagging deep Parsing named entity recognition ……………. . . . Secretion of TNF was abolished by BHA in PMA -stimulated U 937 cells. ………… annotated (structured) text S VP VP NP PP NP Multi-layered annotations PP PP NP NN IN NN VBZ VBN IN NN IN JJ NN NNS. Secretion of TNF was abolished by BHA in PMA-stimulated U 937 cells. protein_molecule organic_compound negative regulation cell_line
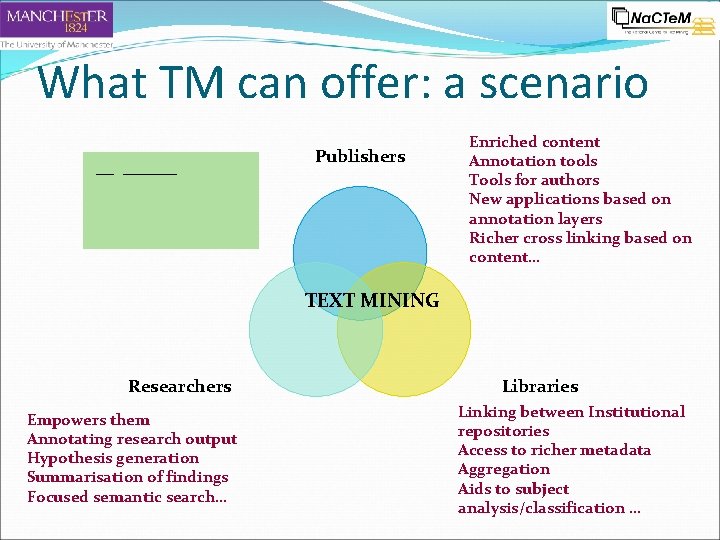
What TM can offer: a scenario __ ______ Publishers Enriched content Annotation tools Tools for authors New applications based on annotation layers Richer cross linking based on content… TEXT MINING Researchers Empowers them Annotating research output Hypothesis generation Summarisation of findings Focused semantic search… Libraries Linking between Institutional repositories Access to richer metadata Aggregation Aids to subject analysis/classification …
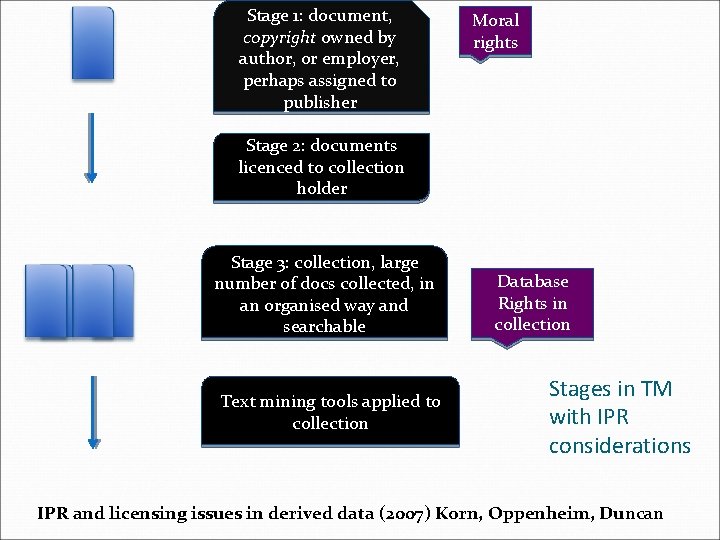
Stage 1: document, copyright owned by author, or employer, perhaps assigned to publisher Moral rights Stage 2: documents licenced to collection holder Stage 3: collection, large number of docs collected, in an organised way and searchable Text mining tools applied to collection Database Rights in collection Stages in TM with IPR considerations IPR and licensing issues in derived data (2007) Korn, Oppenheim, Duncan
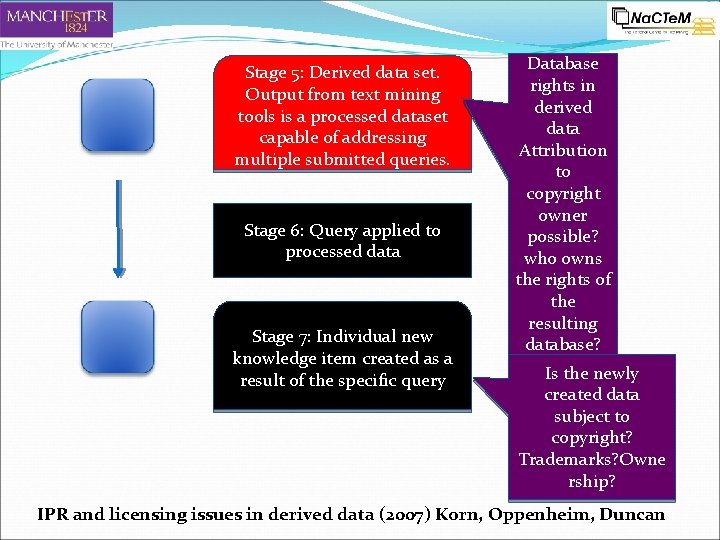
Stage 5: Derived data set. Output from text mining tools is a processed dataset capable of addressing multiple submitted queries. Stage 6: Query applied to processed data Stage 7: Individual new knowledge item created as a result of the specific query Database rights in derived data Attribution to copyright owner possible? who owns the rights of the resulting database? Is the newly created data subject to copyright? Trademarks? Owne rship? IPR and licensing issues in derived data (2007) Korn, Oppenheim, Duncan
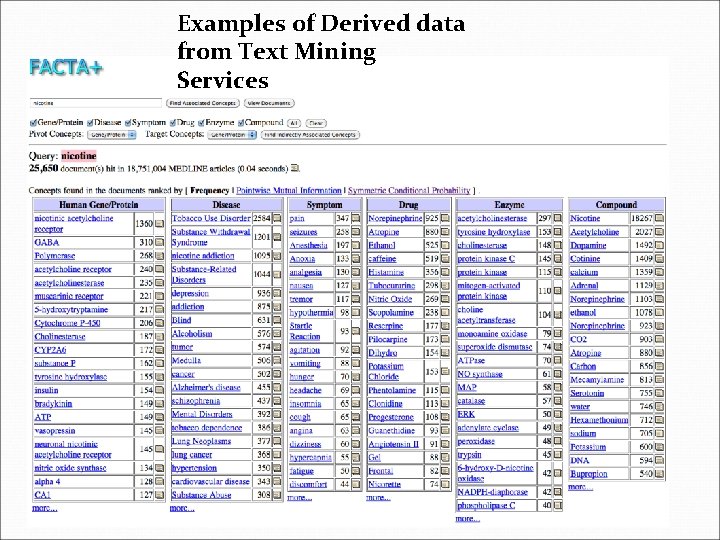
Examples of Derived data from Text Mining Services Example of derived data
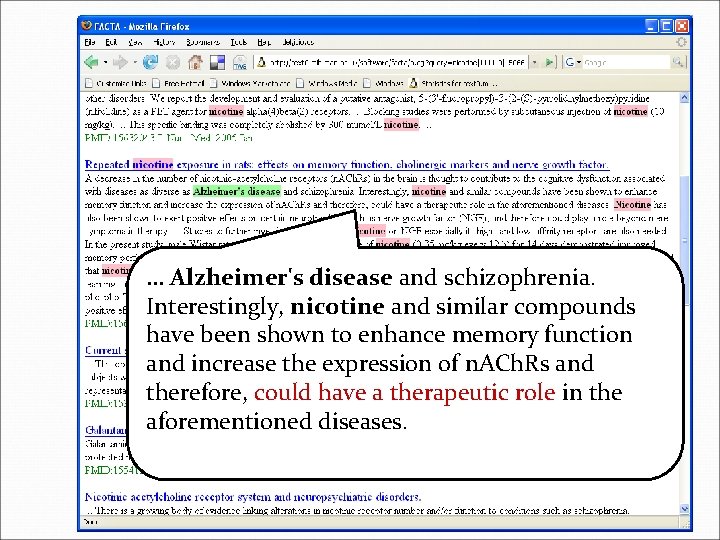
… Alzheimer's disease and schizophrenia. Interestingly, nicotine and similar compounds have been shown to enhance memory function and increase the expression of n. ACh. Rs and therefore, could have a therapeutic role in the aforementioned diseases.
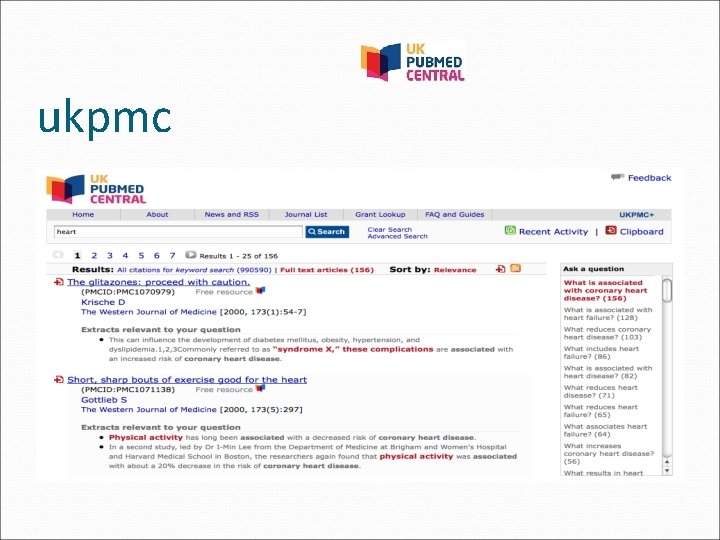
ukpmc
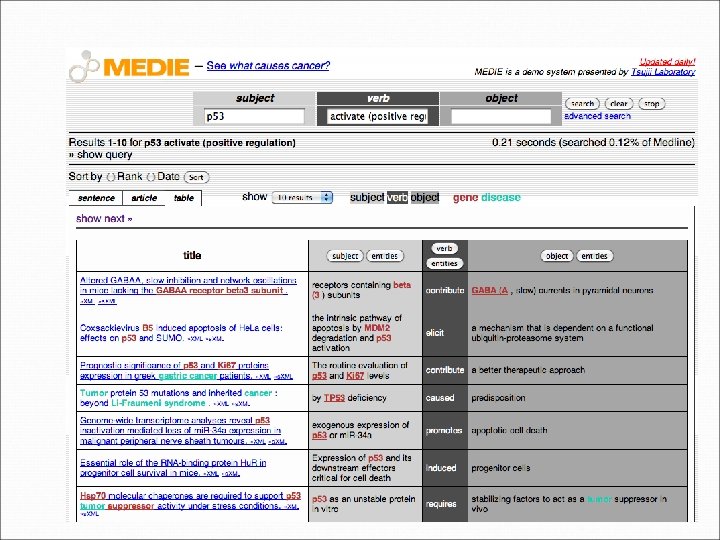
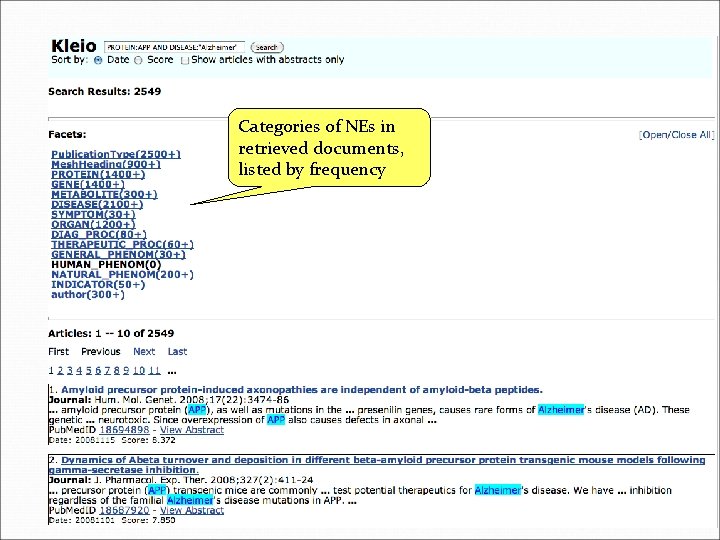
Categories of NEs in retrieved documents, listed by frequency
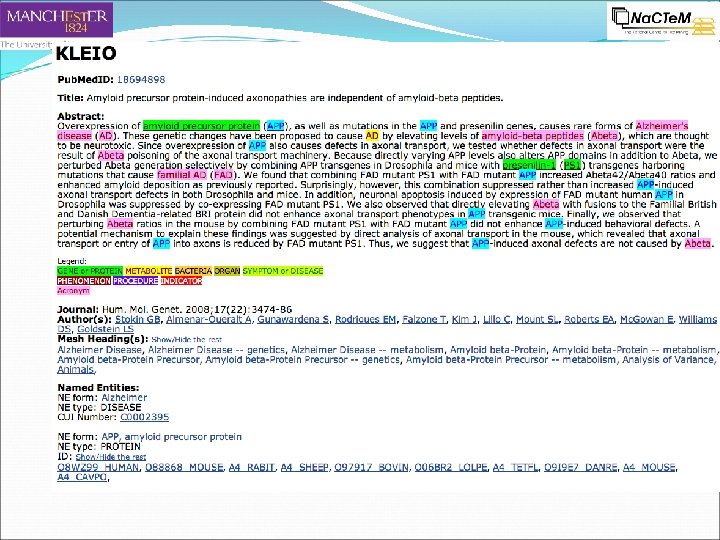
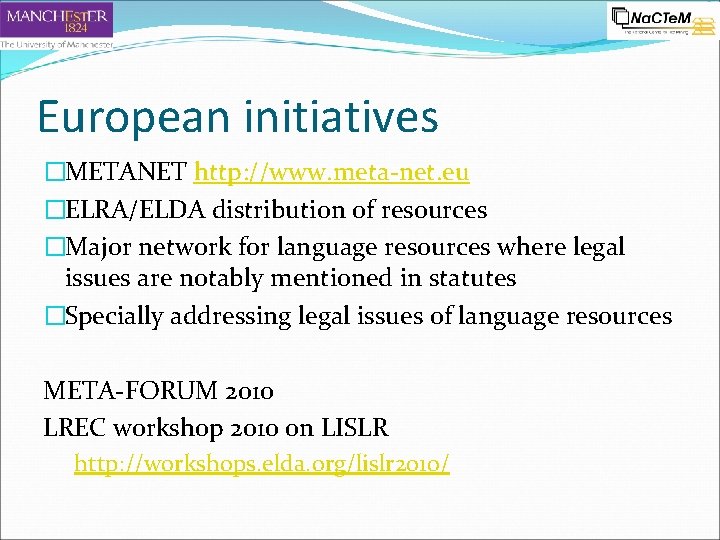
European initiatives �METANET http: //www. meta-net. eu �ELRA/ELDA distribution of resources �Major network for language resources where legal issues are notably mentioned in statutes �Specially addressing legal issues of language resources META-FORUM 2010 LREC workshop 2010 on LISLR http: //workshops. elda. org/lislr 2010/
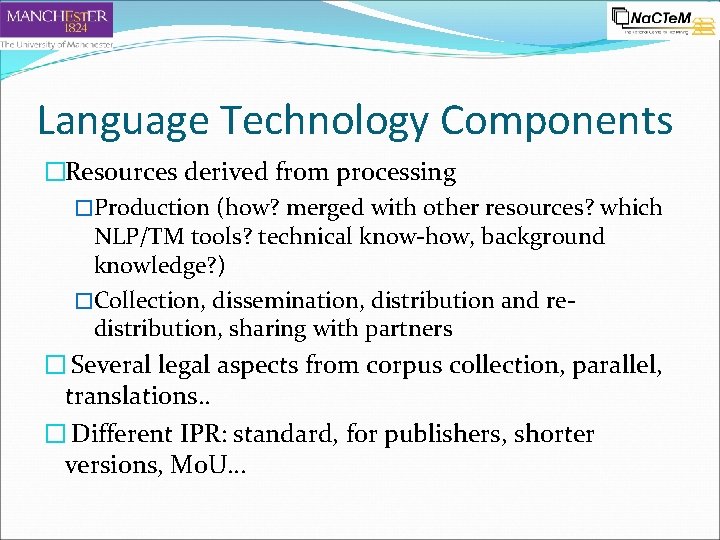
Language Technology Components �Resources derived from processing �Production (how? merged with other resources? which NLP/TM tools? technical know-how, background knowledge? ) �Collection, dissemination, distribution and redistribution, sharing with partners � Several legal aspects from corpus collection, parallel, translations. . � Different IPR: standard, for publishers, shorter versions, Mo. U…
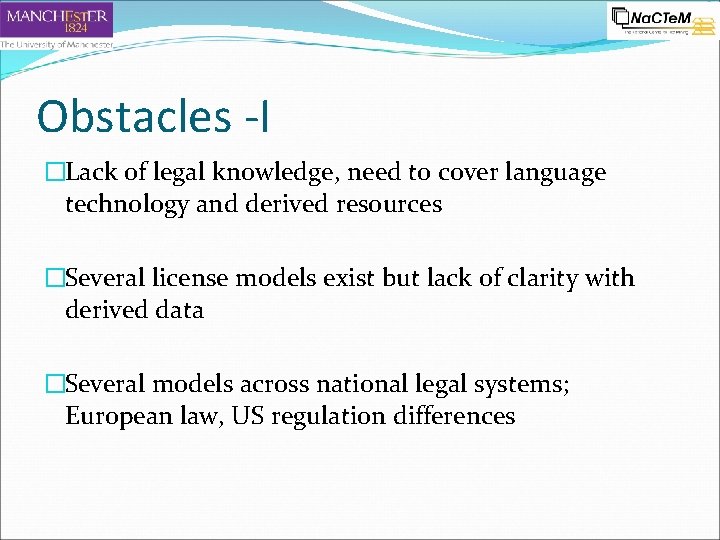
Obstacles -I �Lack of legal knowledge, need to cover language technology and derived resources �Several license models exist but lack of clarity with derived data �Several models across national legal systems; European law, US regulation differences
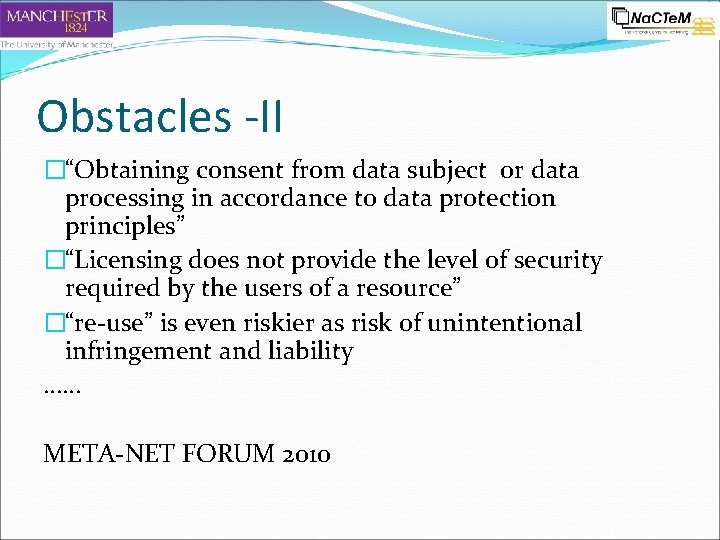
Obstacles -II �“Obtaining consent from data subject or data processing in accordance to data protection principles” �“Licensing does not provide the level of security required by the users of a resource” �“re-use” is even riskier as risk of unintentional infringement and liability …… META-NET FORUM 2010
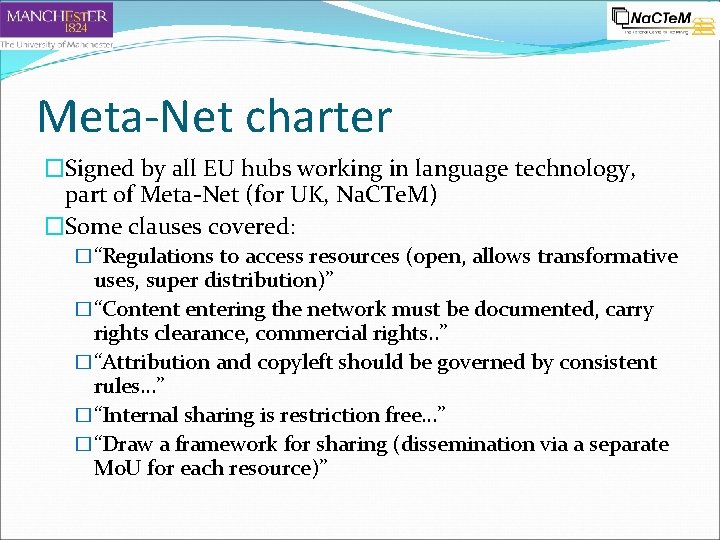
Meta-Net charter �Signed by all EU hubs working in language technology, part of Meta-Net (for UK, Na. CTe. M) �Some clauses covered: �“Regulations to access resources (open, allows transformative uses, super distribution)” �“Content entering the network must be documented, carry rights clearance, commercial rights. . ” �“Attribution and copyleft should be governed by consistent rules…” �“Internal sharing is restriction free…” �“Draw a framework for sharing (dissemination via a separate Mo. U for each resource)”
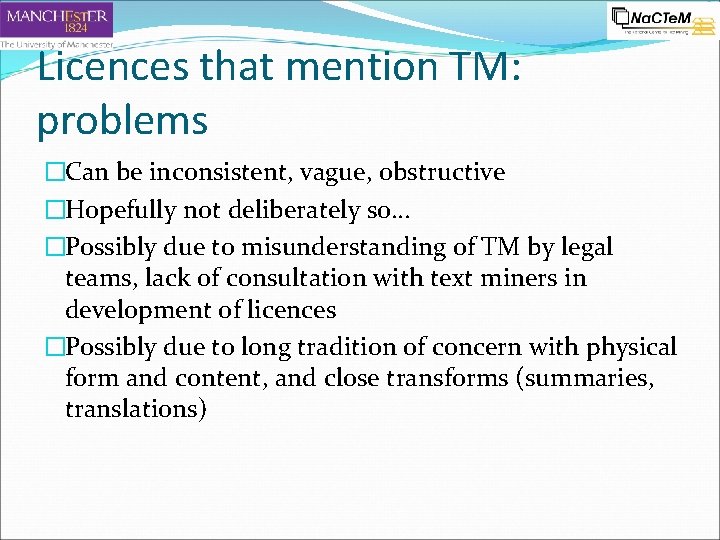
Licences that mention TM: problems �Can be inconsistent, vague, obstructive �Hopefully not deliberately so… �Possibly due to misunderstanding of TM by legal teams, lack of consultation with text miners in development of licences �Possibly due to long tradition of concern with physical form and content, and close transforms (summaries, translations)
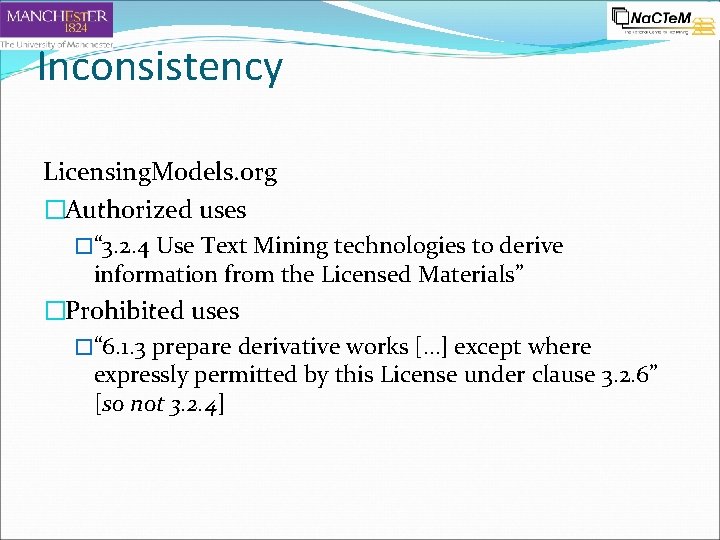
Inconsistency Licensing. Models. org �Authorized uses �“ 3. 2. 4 Use Text Mining technologies to derive information from the Licensed Materials” �Prohibited uses �“ 6. 1. 3 prepare derivative works […] except where expressly permitted by this License under clause 3. 2. 6” [so not 3. 2. 4]
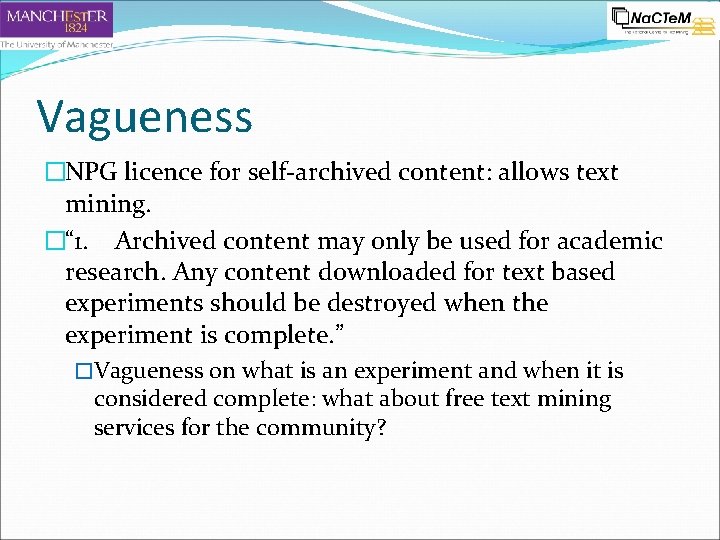
Vagueness �NPG licence for self-archived content: allows text mining. �“ 1. Archived content may only be used for academic research. Any content downloaded for text based experiments should be destroyed when the experiment is complete. ” �Vagueness on what is an experiment and when it is considered complete: what about free text mining services for the community?
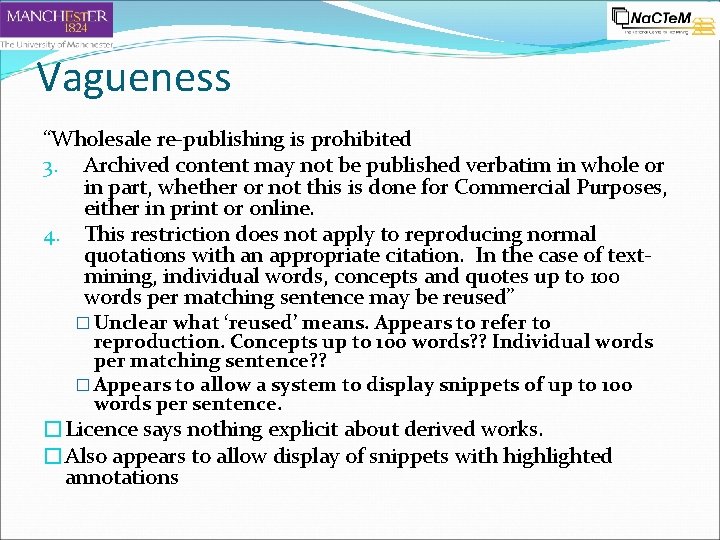
Vagueness “Wholesale re-publishing is prohibited 3. Archived content may not be published verbatim in whole or in part, whether or not this is done for Commercial Purposes, either in print or online. 4. This restriction does not apply to reproducing normal quotations with an appropriate citation. In the case of textmining, individual words, concepts and quotes up to 100 words per matching sentence may be reused” � Unclear what ‘reused’ means. Appears to refer to reproduction. Concepts up to 100 words? ? Individual words per matching sentence? ? � Appears to allow a system to display snippets of up to 100 words per sentence. �Licence says nothing explicit about derived works. �Also appears to allow display of snippets with highlighted annotations
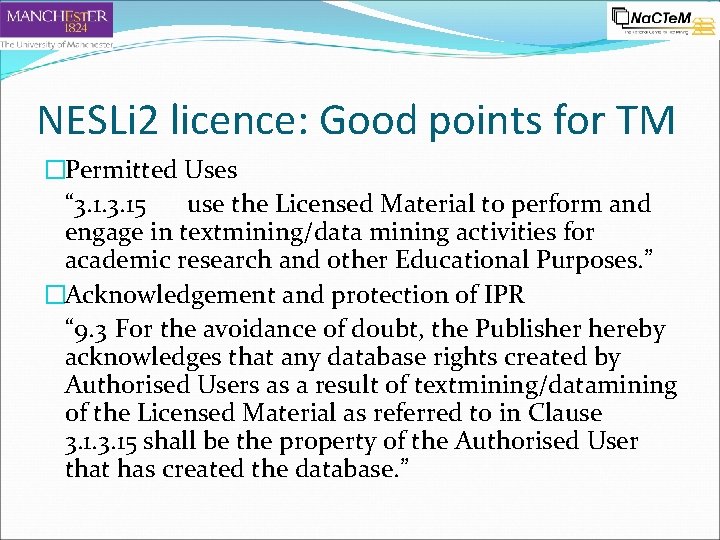
NESLi 2 licence: Good points for TM �Permitted Uses “ 3. 15 use the Licensed Material to perform and engage in textmining/data mining activities for academic research and other Educational Purposes. ” �Acknowledgement and protection of IPR “ 9. 3 For the avoidance of doubt, the Publisher hereby acknowledges that any database rights created by Authorised Users as a result of textmining/datamining of the Licensed Material as referred to in Clause 3. 15 shall be the property of the Authorised User that has created the database. ”
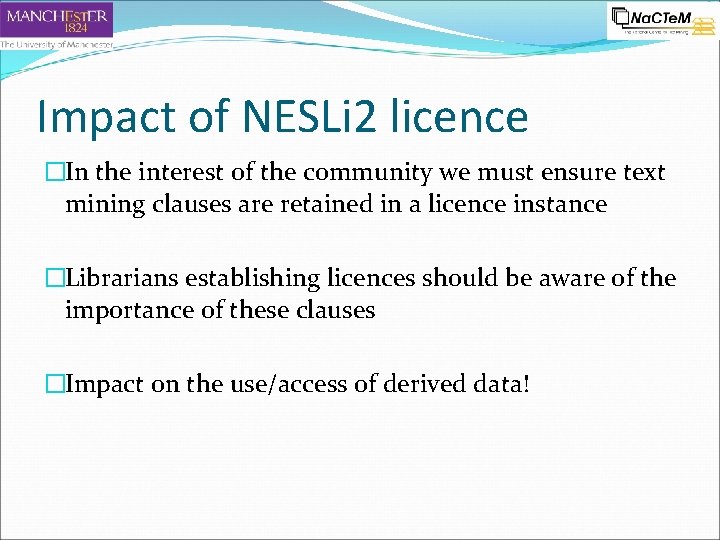
Impact of NESLi 2 licence �In the interest of the community we must ensure text mining clauses are retained in a licence instance �Librarians establishing licences should be aware of the importance of these clauses �Impact on the use/access of derived data!
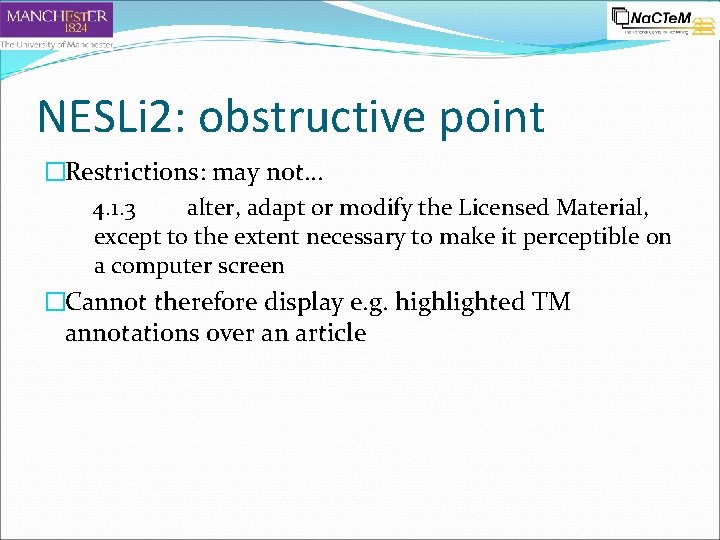
NESLi 2: obstructive point �Restrictions: may not… 4. 1. 3 alter, adapt or modify the Licensed Material, except to the extent necessary to make it perceptible on a computer screen �Cannot therefore display e. g. highlighted TM annotations over an article
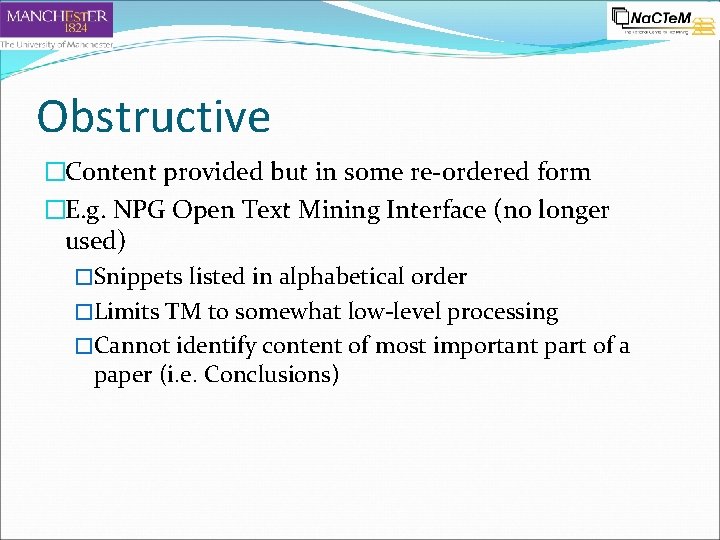
Obstructive �Content provided but in some re-ordered form �E. g. NPG Open Text Mining Interface (no longer used) �Snippets listed in alphabetical order �Limits TM to somewhat low-level processing �Cannot identify content of most important part of a paper (i. e. Conclusions)
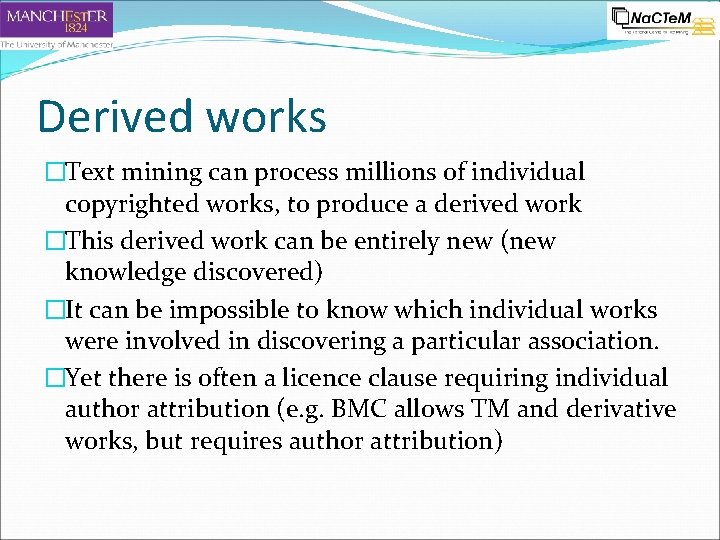
Derived works �Text mining can process millions of individual copyrighted works, to produce a derived work �This derived work can be entirely new (new knowledge discovered) �It can be impossible to know which individual works were involved in discovering a particular association. �Yet there is often a licence clause requiring individual author attribution (e. g. BMC allows TM and derivative works, but requires author attribution)
![Brown Boulderstone What is needed is a system for scholarly communication which allows Brown & Boulderstone “What is needed is a system [for scholarly communication] which allows:](https://slidetodoc.com/presentation_image_h/f82dba9f8b04087d8fa254ec21a6455d/image-28.jpg)
Brown & Boulderstone “What is needed is a system [for scholarly communication] which allows: �Access to a vast corpus… �Unprotected by copyright controls (over creation of derivative works) Defining such a specification requires a new means of collaboration between […] stakeholders to accept data and text mining as being effective and acceptable processes. In particular, that such mining does not eliminate any significant role currently being performed by stakeholders, that it does not raise […] barriers to text/data mining applications, that it does not threaten publishers and librarians and their existence. ” Brown, D. J. & Boulderstone, R. (2008) The Impact of Electronic Publishing: The Future for Publishers and Librarians. Berlin: de Gruyter-Saur.
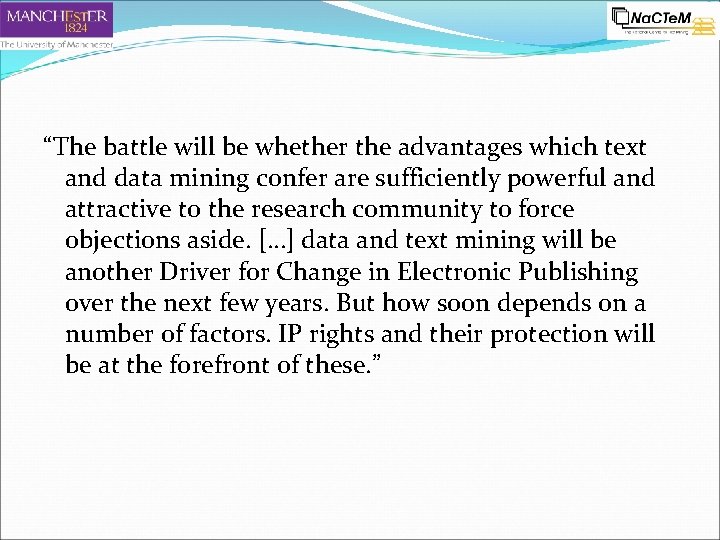
“The battle will be whether the advantages which text and data mining confer are sufficiently powerful and attractive to the research community to force objections aside. […] data and text mining will be another Driver for Change in Electronic Publishing over the next few years. But how soon depends on a number of factors. IP rights and their protection will be at the forefront of these. ”
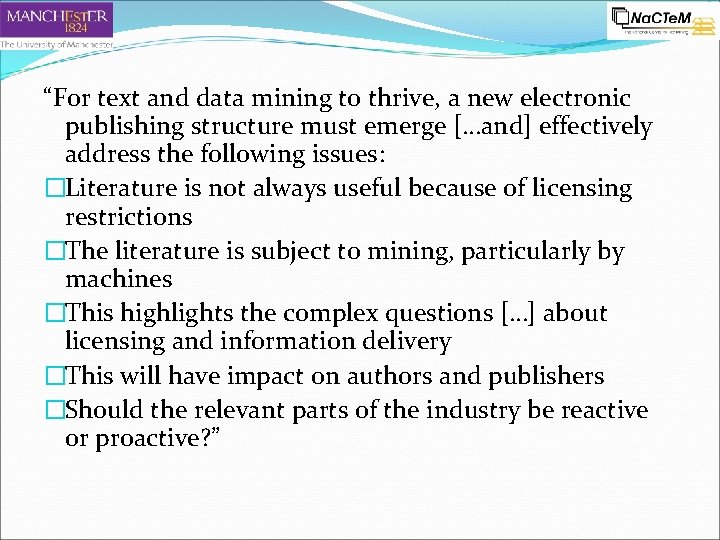
“For text and data mining to thrive, a new electronic publishing structure must emerge […and] effectively address the following issues: �Literature is not always useful because of licensing restrictions �The literature is subject to mining, particularly by machines �This highlights the complex questions […] about licensing and information delivery �This will have impact on authors and publishers �Should the relevant parts of the industry be reactive or proactive? ”
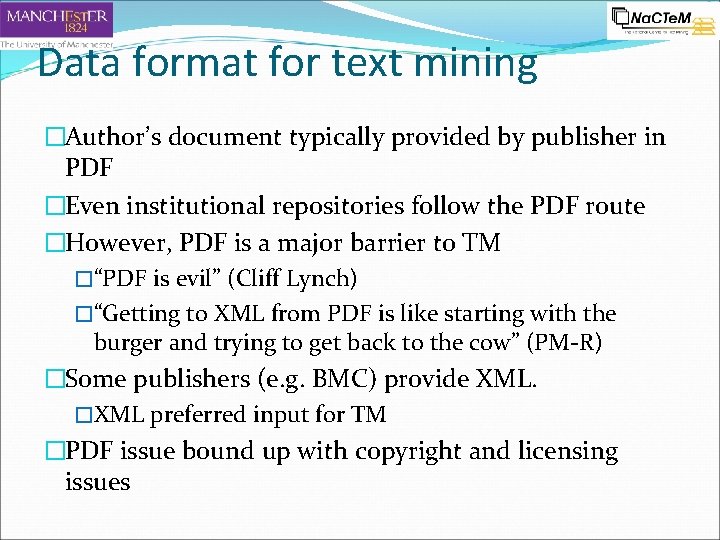
Data format for text mining �Author’s document typically provided by publisher in PDF �Even institutional repositories follow the PDF route �However, PDF is a major barrier to TM �“PDF is evil” (Cliff Lynch) �“Getting to XML from PDF is like starting with the burger and trying to get back to the cow” (PM-R) �Some publishers (e. g. BMC) provide XML. �XML preferred input for TM �PDF issue bound up with copyright and licensing issues
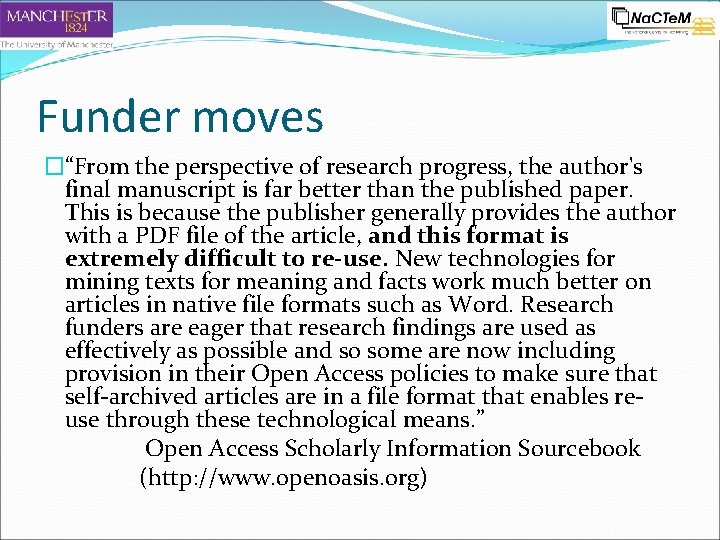
Funder moves �“From the perspective of research progress, the author's final manuscript is far better than the published paper. This is because the publisher generally provides the author with a PDF file of the article, and this format is extremely difficult to re-use. New technologies for mining texts for meaning and facts work much better on articles in native file formats such as Word. Research funders are eager that research findings are used as effectively as possible and so some are now including provision in their Open Access policies to make sure that self-archived articles are in a file format that enables reuse through these technological means. ” Open Access Scholarly Information Sourcebook (http: //www. openoasis. org)
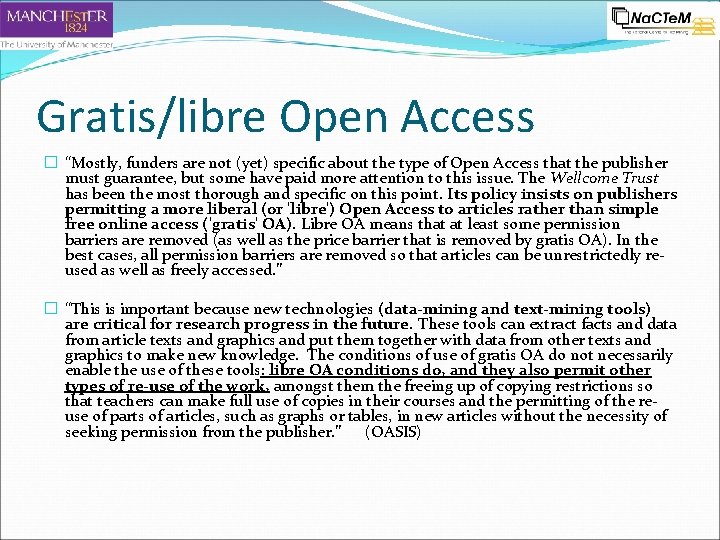
Gratis/libre Open Access � “Mostly, funders are not (yet) specific about the type of Open Access that the publisher must guarantee, but some have paid more attention to this issue. The Wellcome Trust has been the most thorough and specific on this point. Its policy insists on publishers permitting a more liberal (or 'libre') Open Access to articles rather than simple free online access ('gratis' OA). Libre OA means that at least some permission barriers are removed (as well as the price barrier that is removed by gratis OA). In the best cases, all permission barriers are removed so that articles can be unrestrictedly reused as well as freely accessed. ” � “This is important because new technologies (data-mining and text-mining tools) are critical for research progress in the future. These tools can extract facts and data from article texts and graphics and put them together with data from other texts and graphics to make new knowledge. The conditions of use of gratis OA do not necessarily enable the use of these tools: libre OA conditions do, and they also permit other types of re-use of the work, amongst them the freeing up of copying restrictions so that teachers can make full use of copies in their courses and the permitting of the reuse of parts of articles, such as graphs or tables, in new articles without the necessity of seeking permission from the publisher. ” (OASIS)
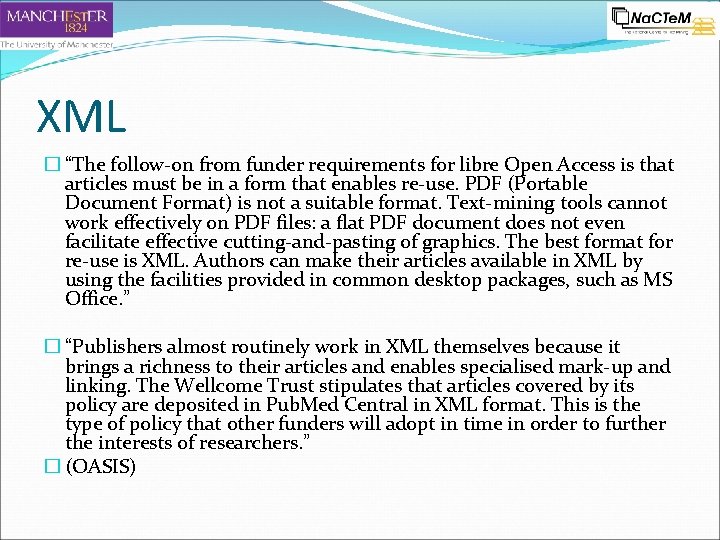
XML � “The follow-on from funder requirements for libre Open Access is that articles must be in a form that enables re-use. PDF (Portable Document Format) is not a suitable format. Text-mining tools cannot work effectively on PDF files: a flat PDF document does not even facilitate effective cutting-and-pasting of graphics. The best format for re-use is XML. Authors can make their articles available in XML by using the facilities provided in common desktop packages, such as MS Office. ” � “Publishers almost routinely work in XML themselves because it brings a richness to their articles and enables specialised mark-up and linking. The Wellcome Trust stipulates that articles covered by its policy are deposited in Pub. Med Central in XML format. This is the type of policy that other funders will adopt in time in order to further the interests of researchers. ” � (OASIS)
Eck
Text and web mining
Mining multimedia databases
What is an example of a text-to-media connection?
Text analytics and text mining
Text analytics and text mining
Difference between strip mining and open pit mining
European ipr helpdesk
Industrial property definition
Industrial design ipr
Grille indiciaire ia-ipr
Pgr vs ipr
Etsi ipr database
Ipr cnc
Etsi ipr database
Borang akreditasi 9 kriteria
Etsi ipr database
Reservorios
What is kdd process in data mining
Mining fraud
Olap data warehouse
Introduction to data mining and data warehousing
Strip mining vs open pit mining
Strip mining before and after
Data reduction in data mining
What is missing data in data mining
Data reduction in data mining
Data reduction in data mining
Data reduction in data mining
Data cube technology in data mining
Data reduction in data mining
Arsitektur data mining
Perbedaan data warehouse dan data mining
Mining complex types of data
Noisy data in data mining