SWOT Virtual Mission Kostas Andreadis 1 Michael Durand
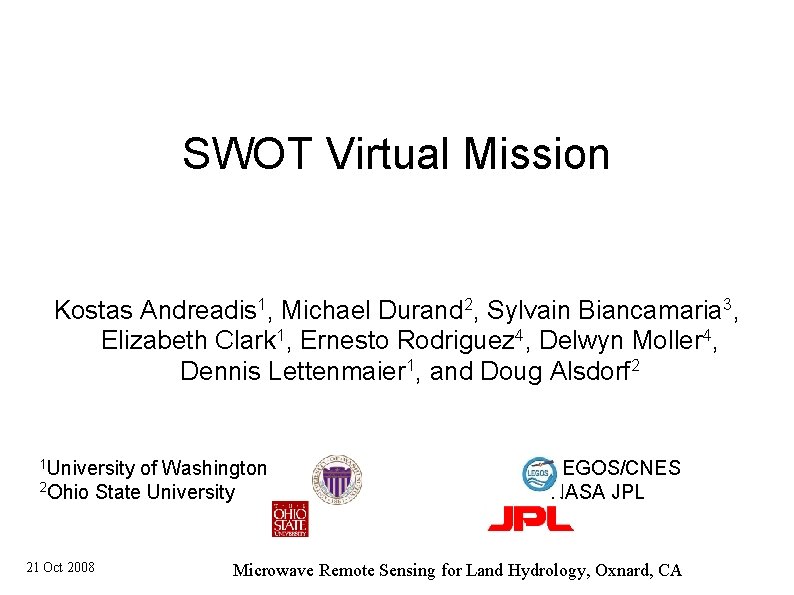
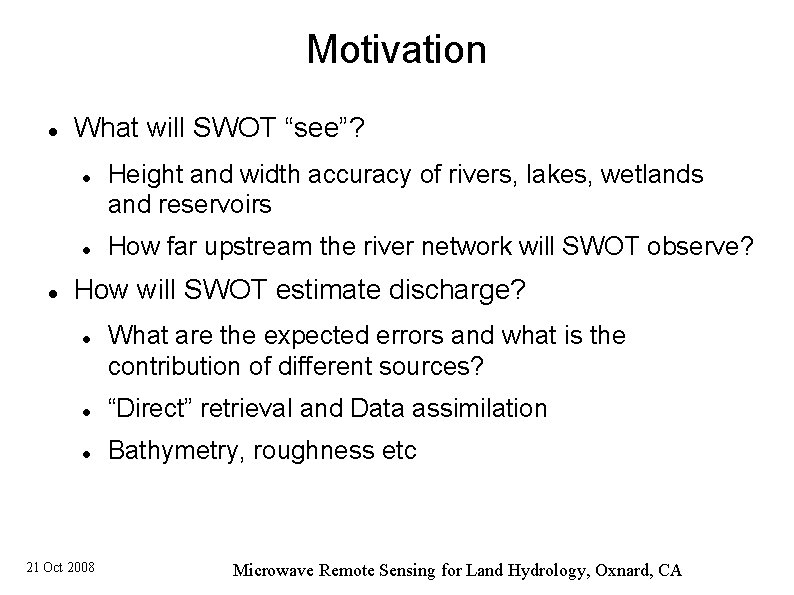
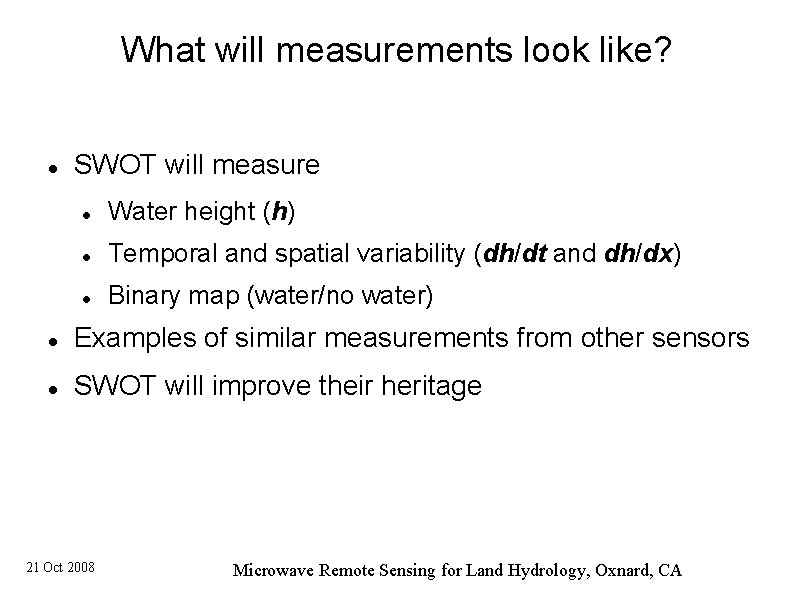
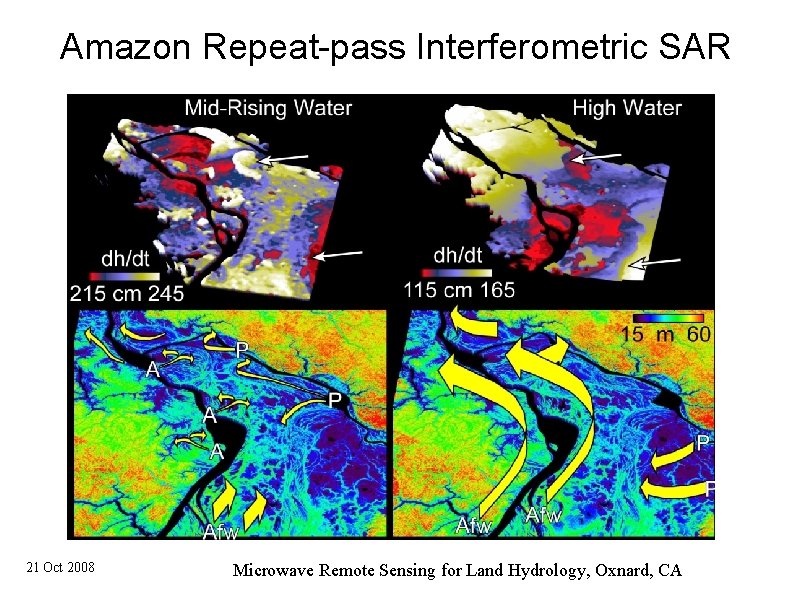
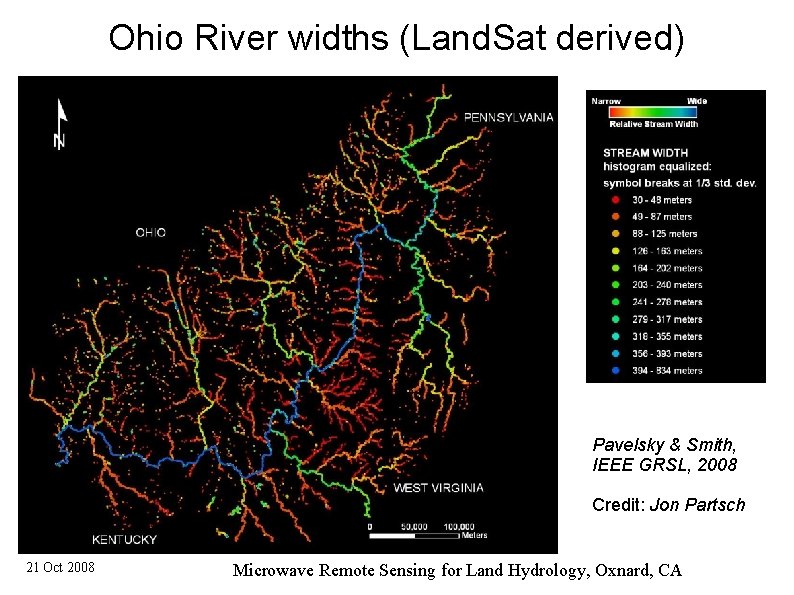
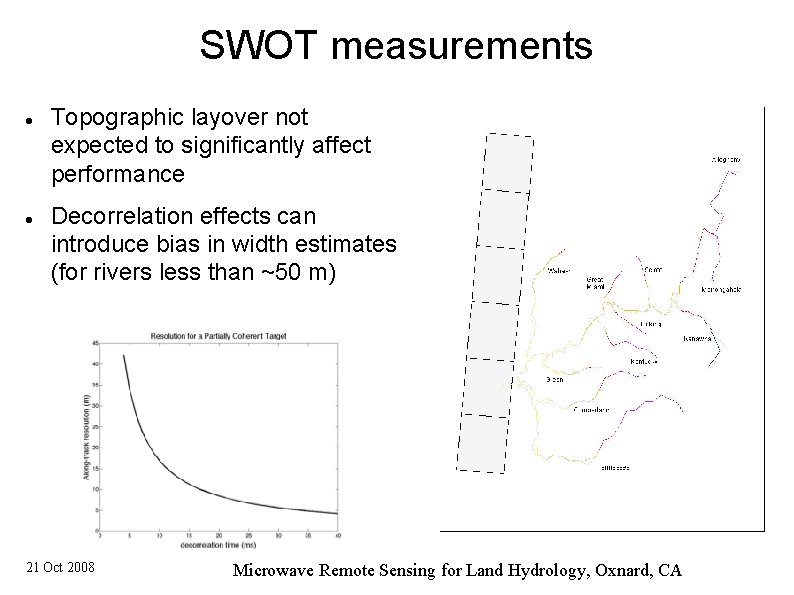
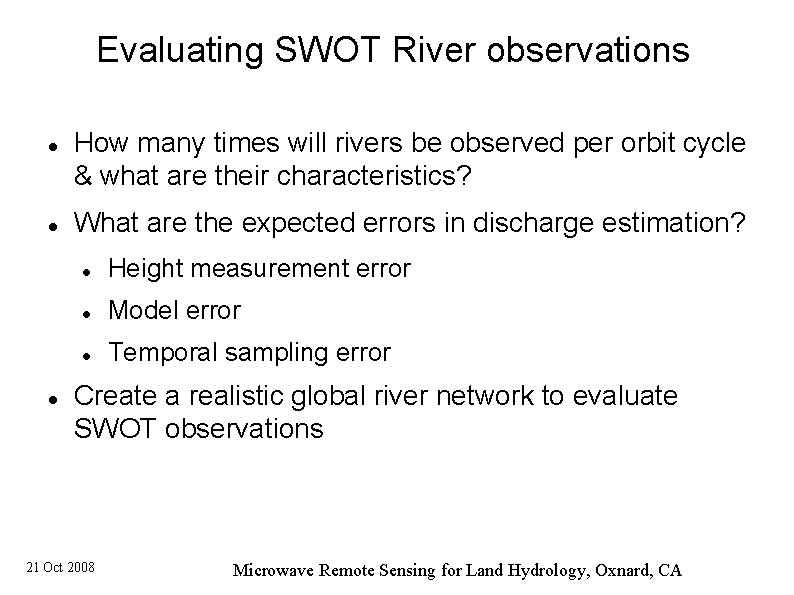
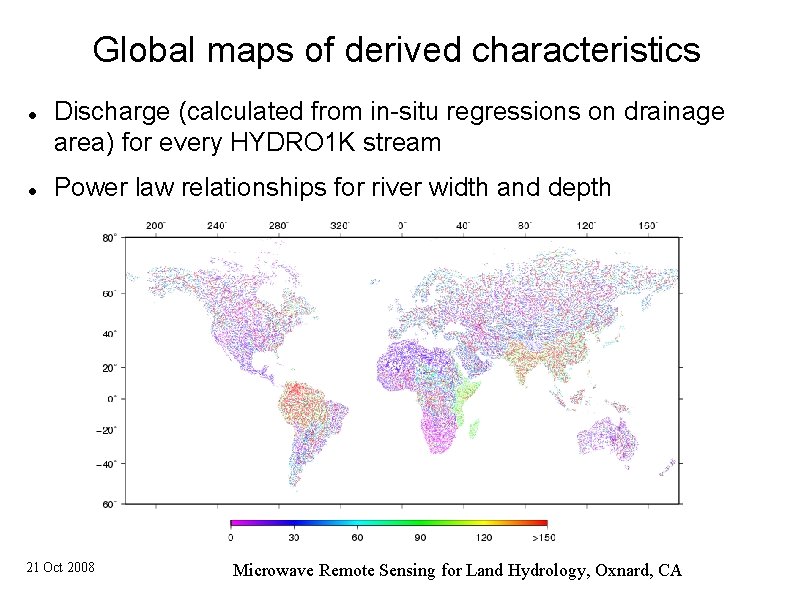
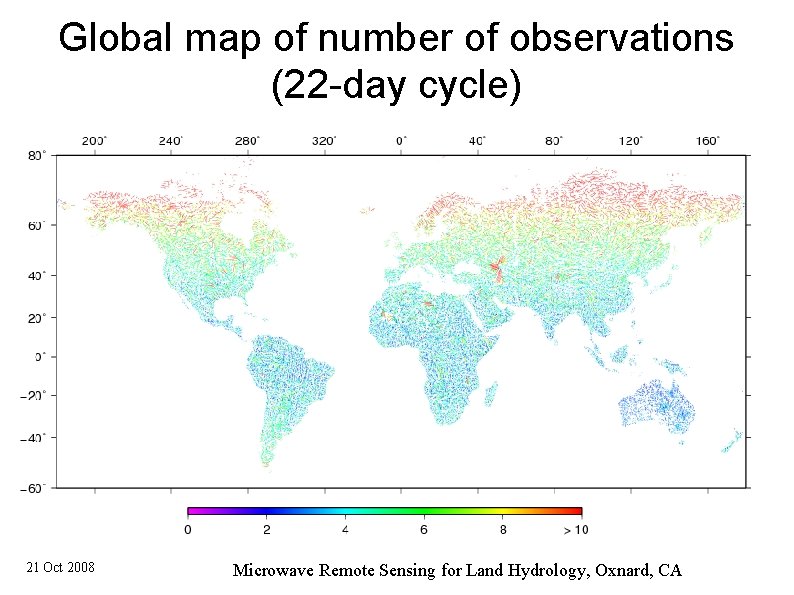
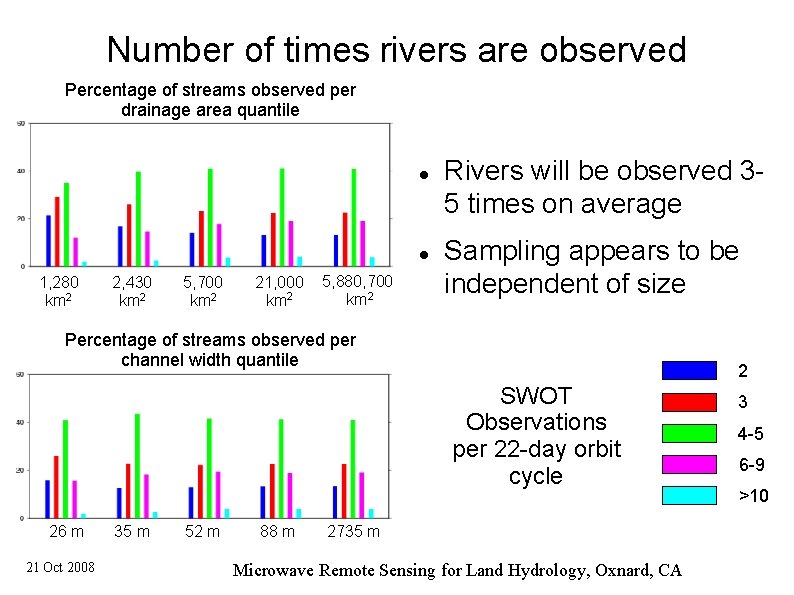
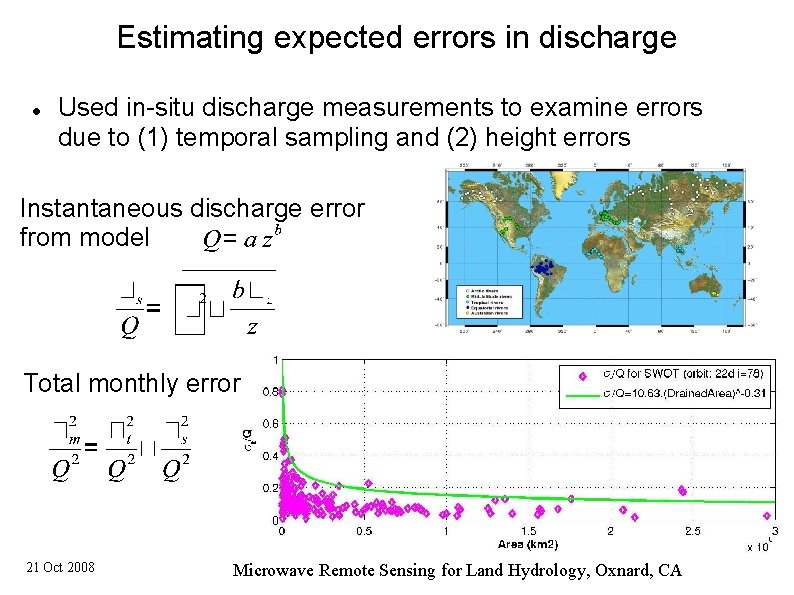
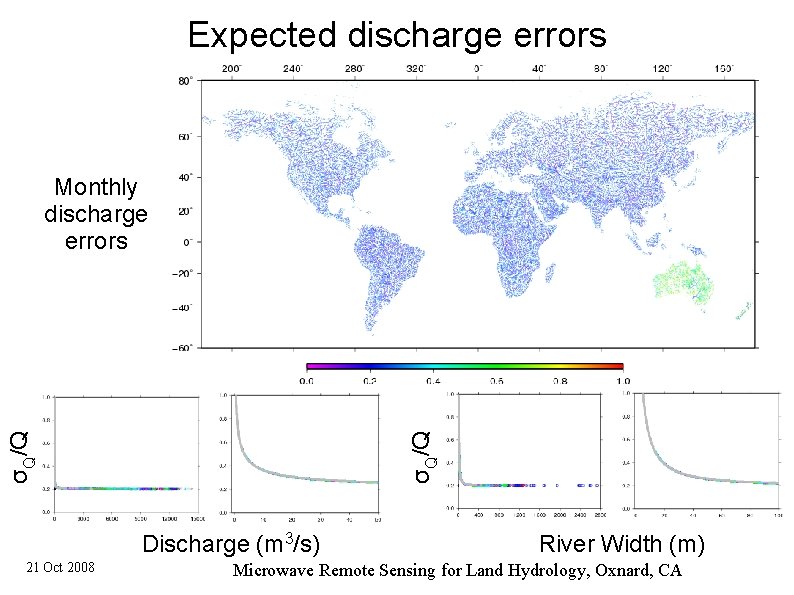
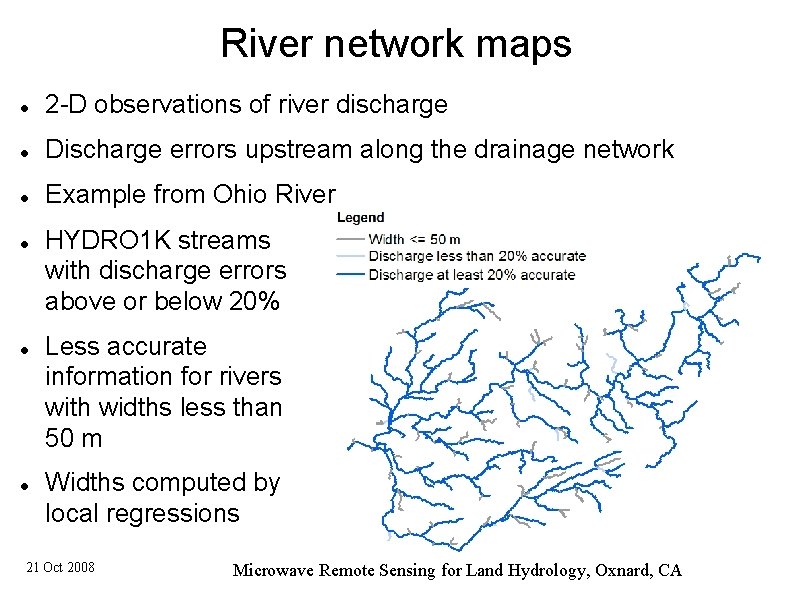
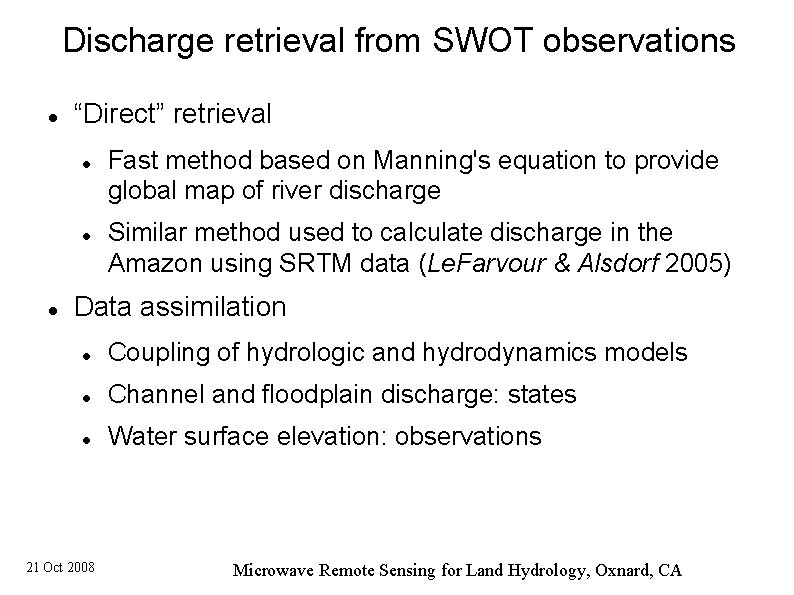
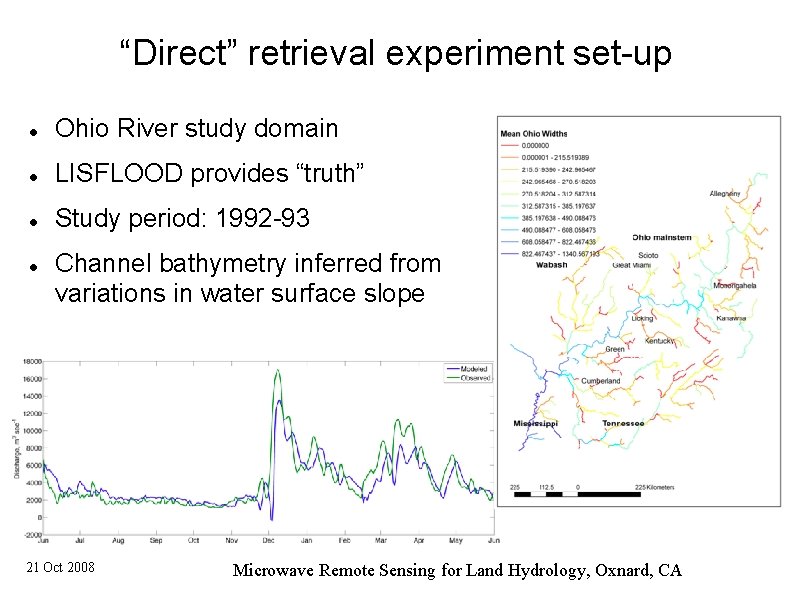
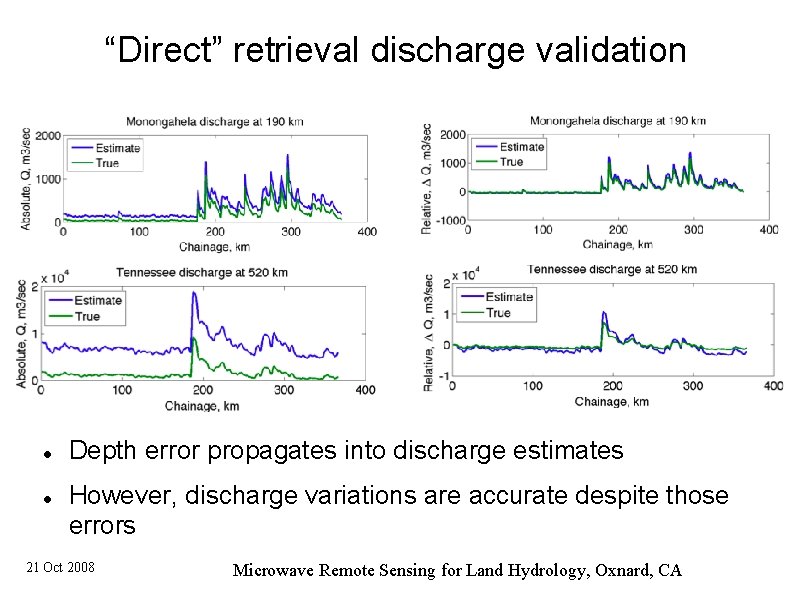
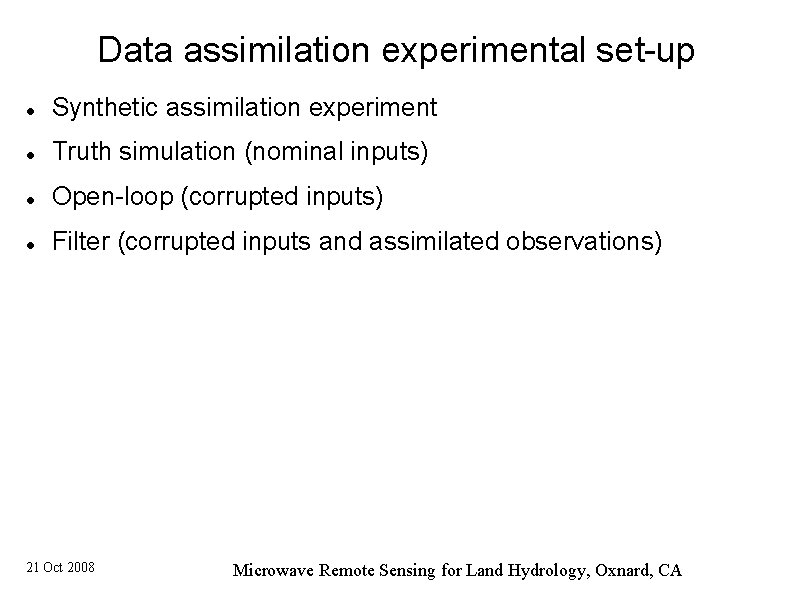
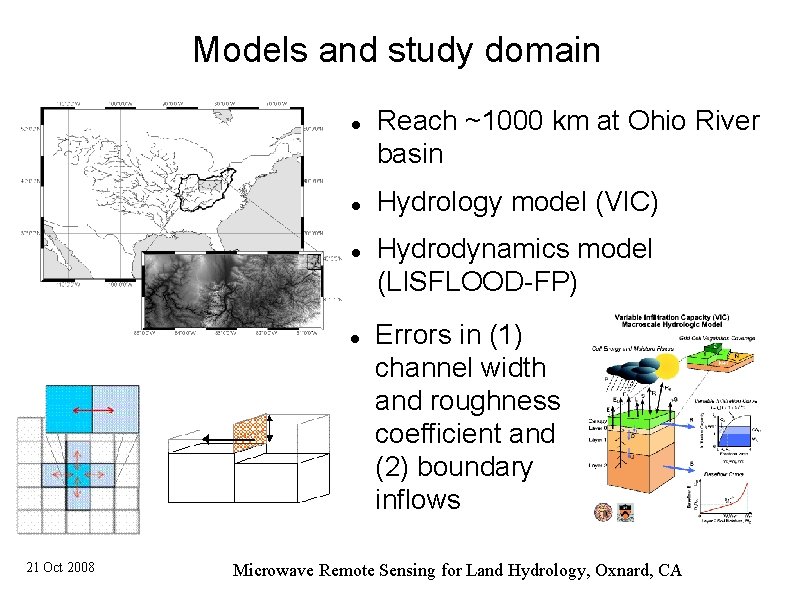
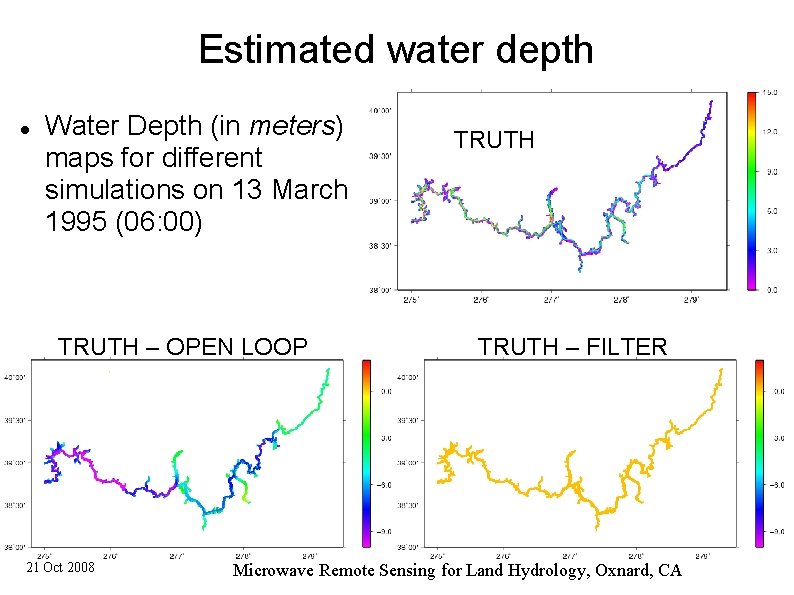
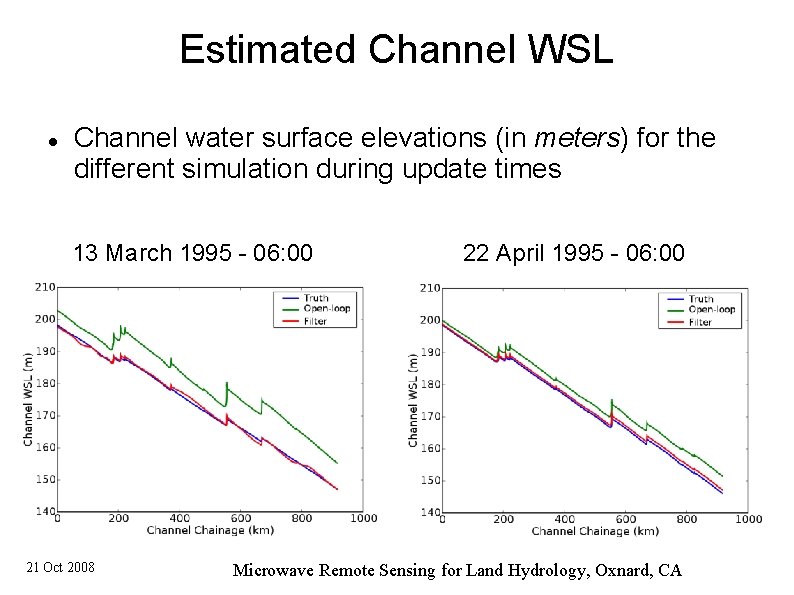
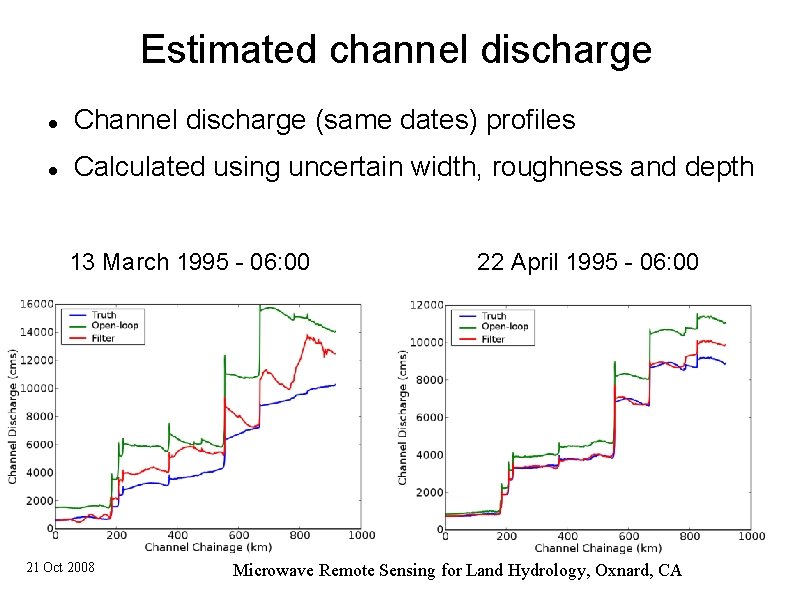
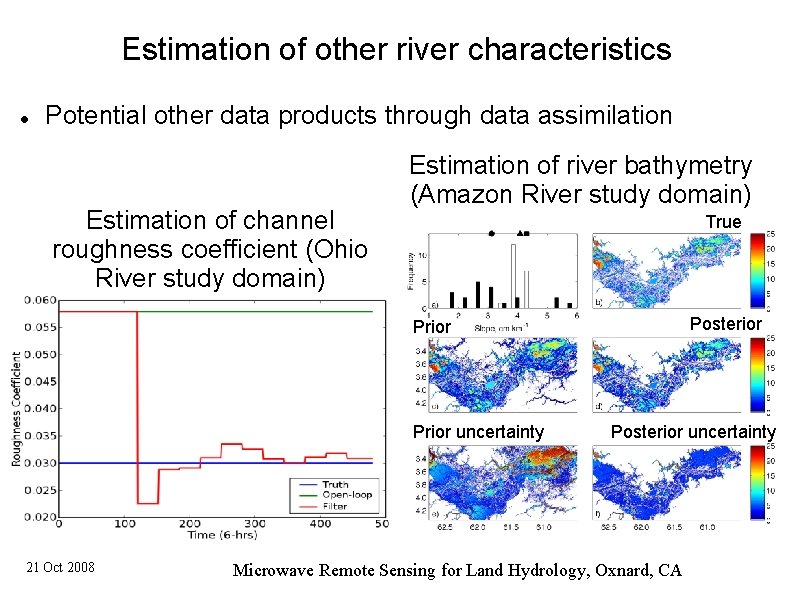
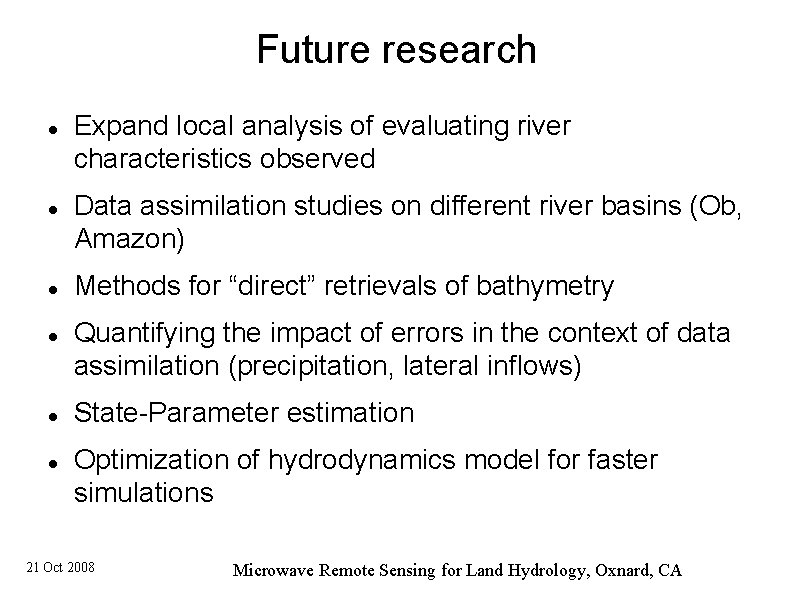
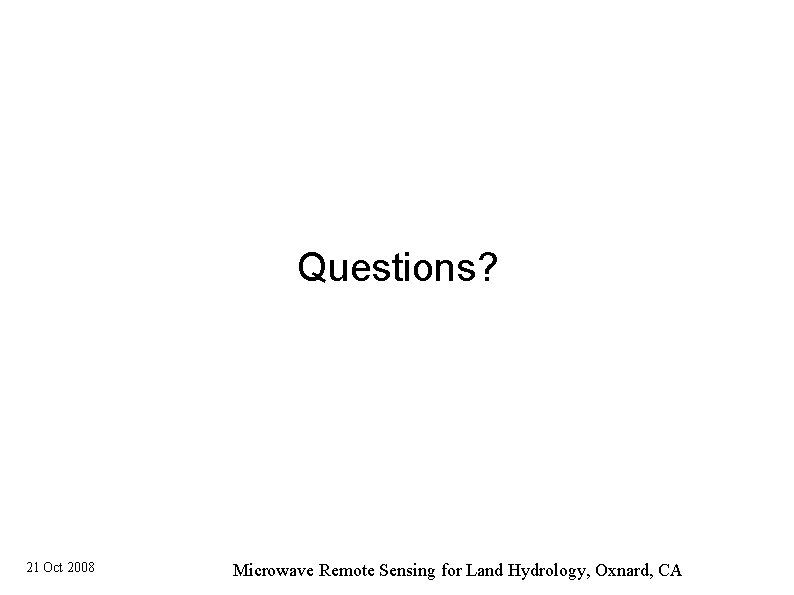
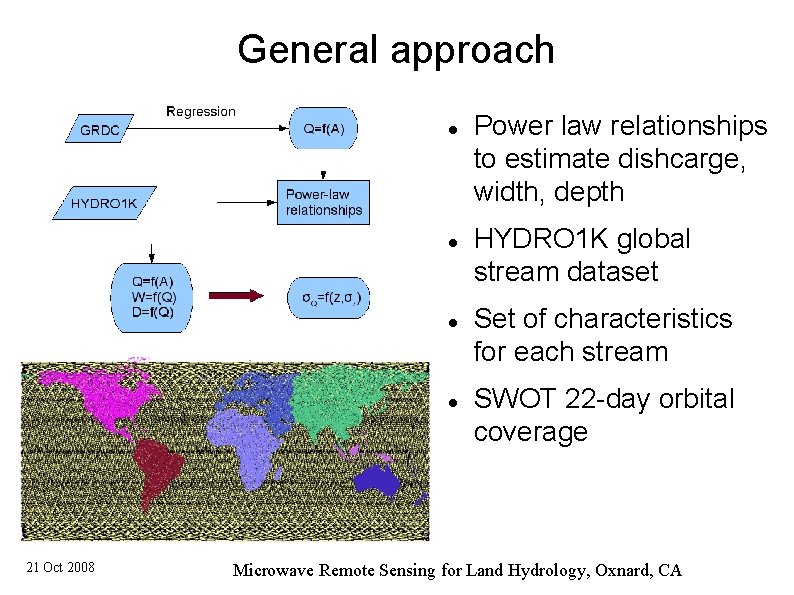
- Slides: 25
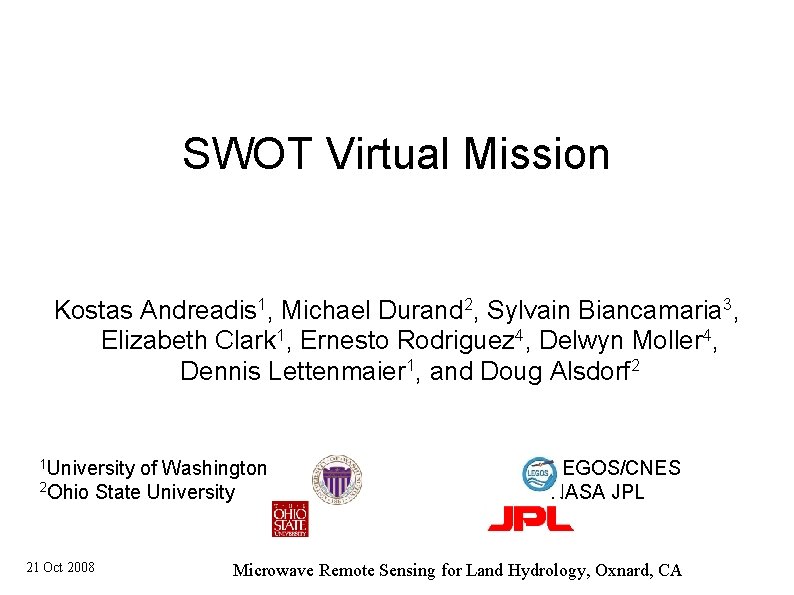
SWOT Virtual Mission Kostas Andreadis 1, Michael Durand 2, Sylvain Biancamaria 3, Elizabeth Clark 1, Ernesto Rodriguez 4, Delwyn Moller 4, Dennis Lettenmaier 1, and Doug Alsdorf 2 1 University of Washington 2 Ohio State University 21 Oct 2008 3 LEGOS/CNES 4 NASA JPL Microwave Remote Sensing for Land Hydrology, Oxnard, CA
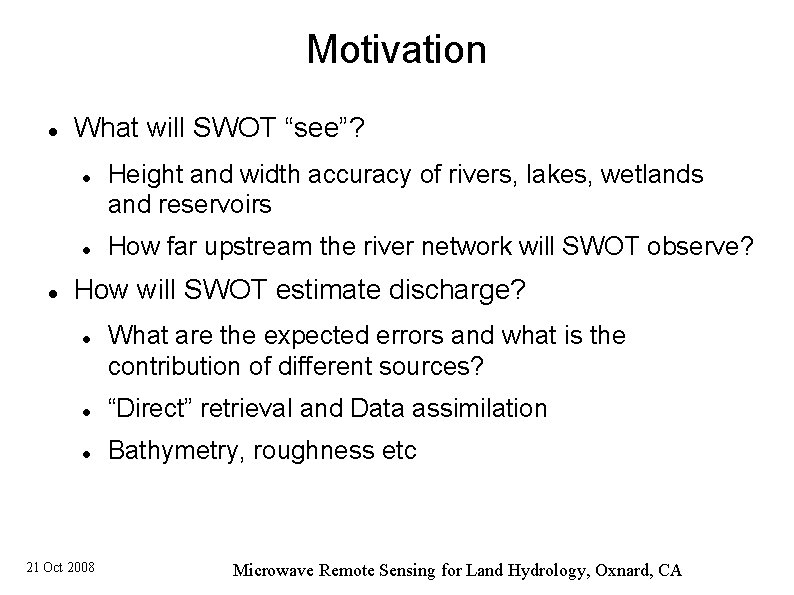
Motivation What will SWOT “see”? Height and width accuracy of rivers, lakes, wetlands and reservoirs How far upstream the river network will SWOT observe? How will SWOT estimate discharge? What are the expected errors and what is the contribution of different sources? “Direct” retrieval and Data assimilation Bathymetry, roughness etc 21 Oct 2008 Microwave Remote Sensing for Land Hydrology, Oxnard, CA
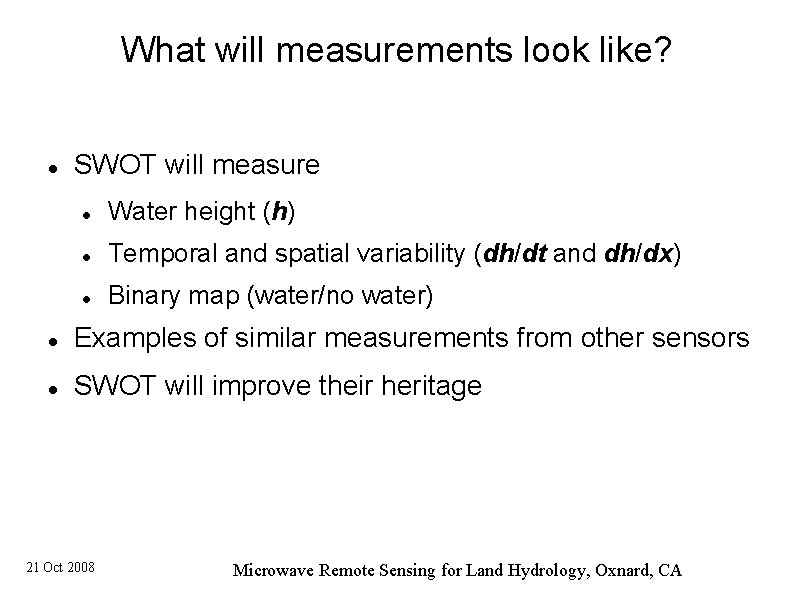
What will measurements look like? SWOT will measure Water height (h) Temporal and spatial variability (dh/dt and dh/dx) Binary map (water/no water) Examples of similar measurements from other sensors SWOT will improve their heritage 21 Oct 2008 Microwave Remote Sensing for Land Hydrology, Oxnard, CA
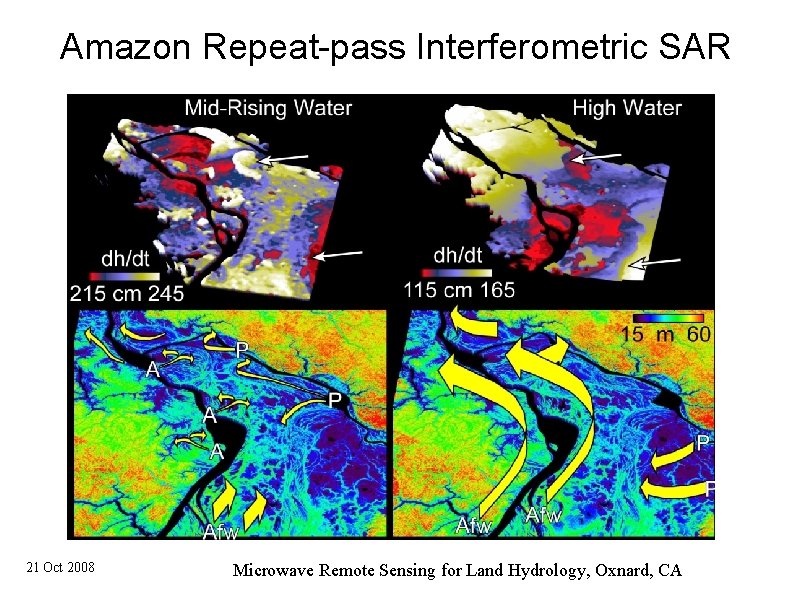
Amazon Repeat-pass Interferometric SAR 21 Oct 2008 Microwave Remote Sensing for Land Hydrology, Oxnard, CA
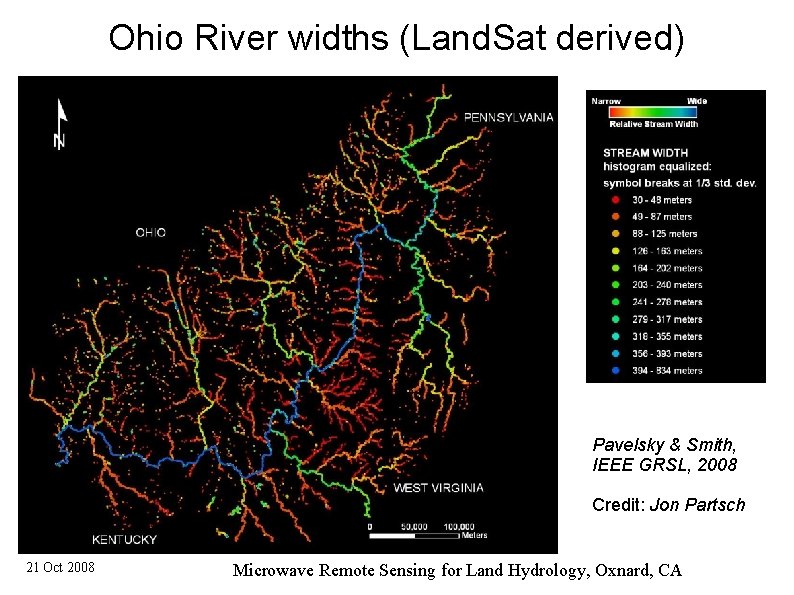
Ohio River widths (Land. Sat derived) Pavelsky & Smith, IEEE GRSL, 2008 Credit: Jon Partsch 21 Oct 2008 Microwave Remote Sensing for Land Hydrology, Oxnard, CA
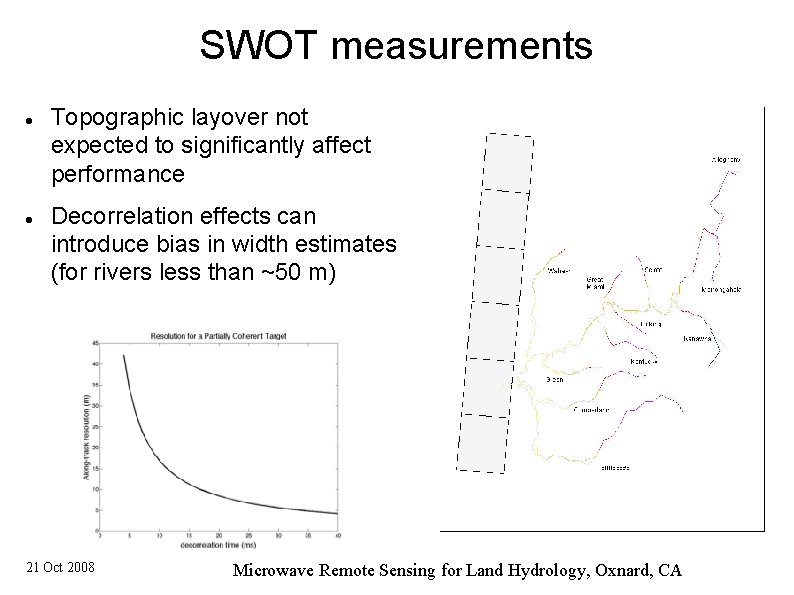
SWOT measurements Topographic layover not expected to significantly affect performance Decorrelation effects can introduce bias in width estimates (for rivers less than ~50 m) 21 Oct 2008 Microwave Remote Sensing for Land Hydrology, Oxnard, CA
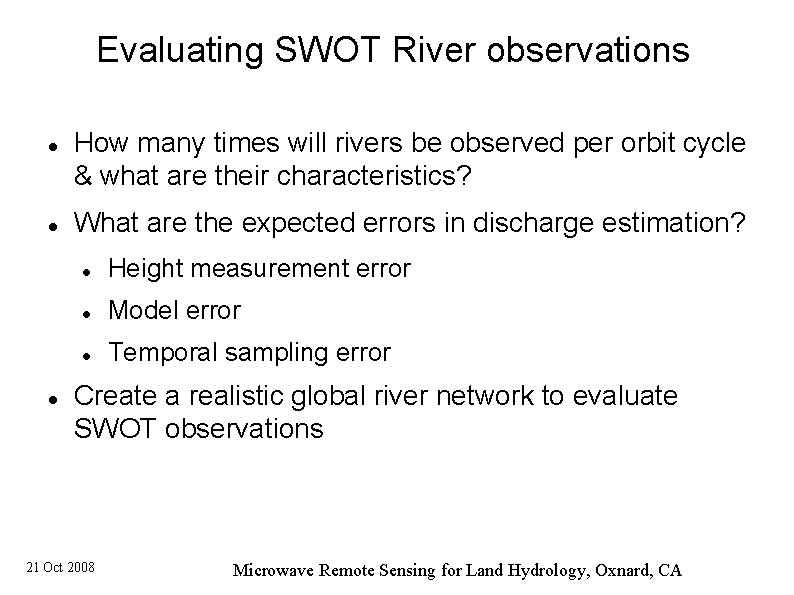
Evaluating SWOT River observations How many times will rivers be observed per orbit cycle & what are their characteristics? What are the expected errors in discharge estimation? Height measurement error Model error Temporal sampling error Create a realistic global river network to evaluate SWOT observations 21 Oct 2008 Microwave Remote Sensing for Land Hydrology, Oxnard, CA
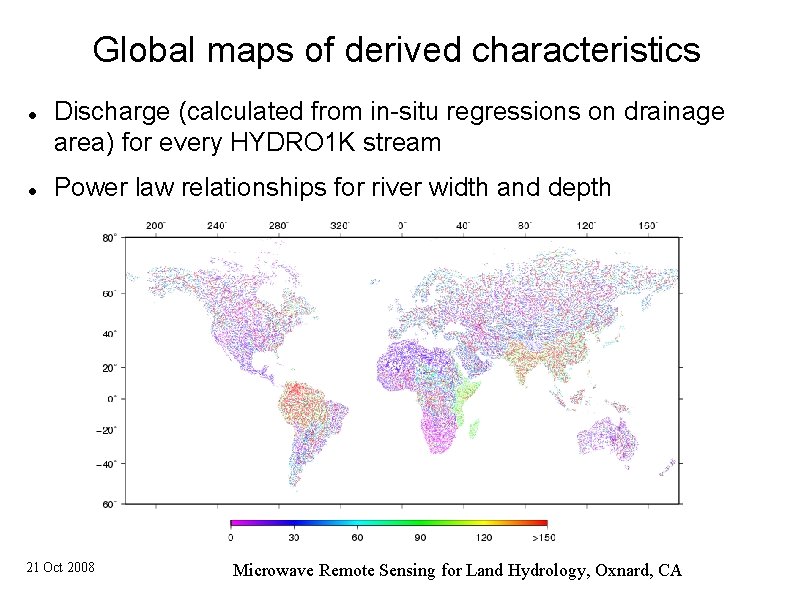
Global maps of derived characteristics Discharge (calculated from in-situ regressions on drainage area) for every HYDRO 1 K stream Power law relationships for river width and depth 21 Oct 2008 Microwave Remote Sensing for Land Hydrology, Oxnard, CA
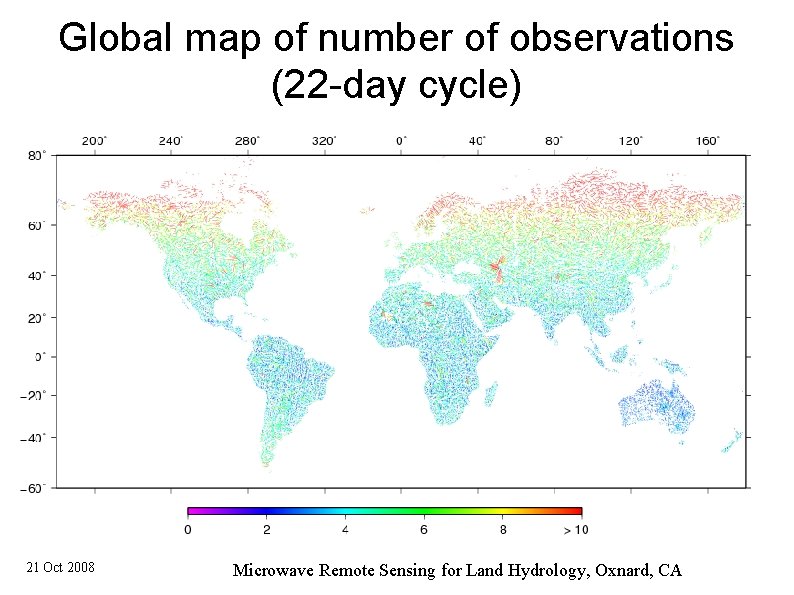
Global map of number of observations (22 -day cycle) 21 Oct 2008 Microwave Remote Sensing for Land Hydrology, Oxnard, CA
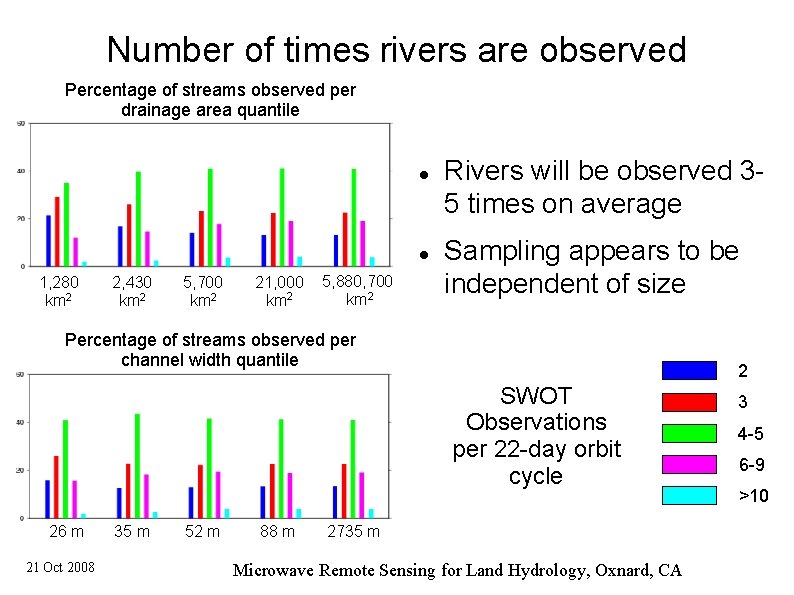
Number of times rivers are observed Percentage of streams observed per drainage area quantile 1, 280 km 2 2, 430 km 2 5, 700 km 2 21, 000 km 2 5, 880, 700 km 2 Rivers will be observed 35 times on average Sampling appears to be independent of size Percentage of streams observed per channel width quantile 2 SWOT Observations per 22 -day orbit cycle 26 m 21 Oct 2008 35 m 52 m 88 m 2735 m Microwave Remote Sensing for Land Hydrology, Oxnard, CA 3 4 -5 6 -9 >10
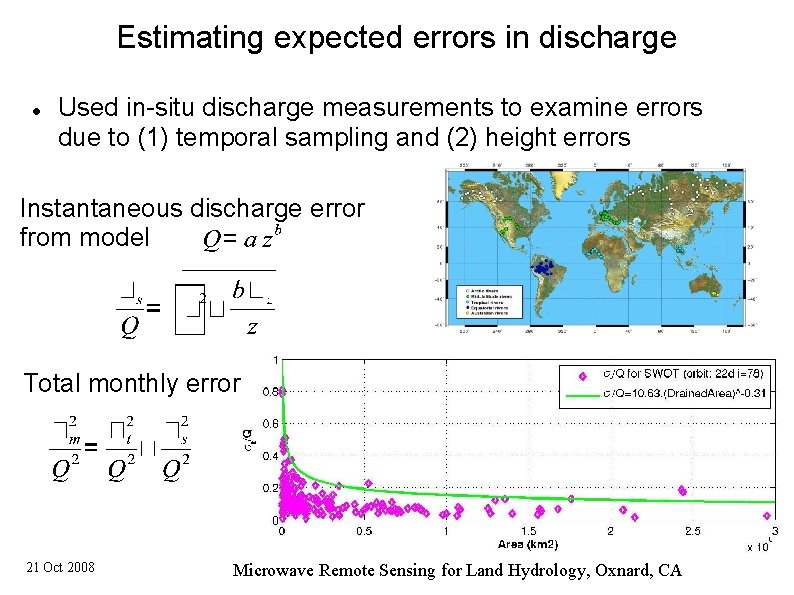
Estimating expected errors in discharge Used in-situ discharge measurements to examine errors due to (1) temporal sampling and (2) height errors Instantaneous discharge error from model Total monthly error 21 Oct 2008 Microwave Remote Sensing for Land Hydrology, Oxnard, CA
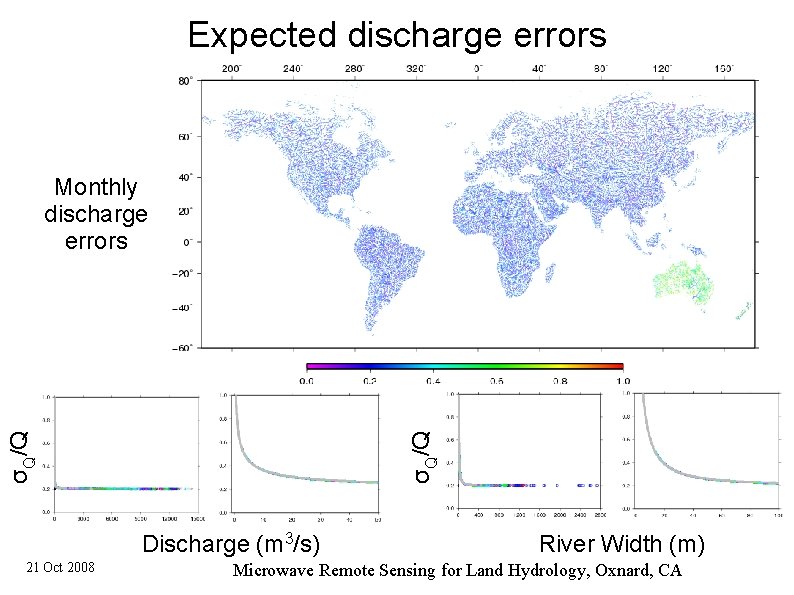
Expected discharge errors σQ/Q Monthly discharge errors Discharge (m 3/s) 21 Oct 2008 River Width (m) Microwave Remote Sensing for Land Hydrology, Oxnard, CA
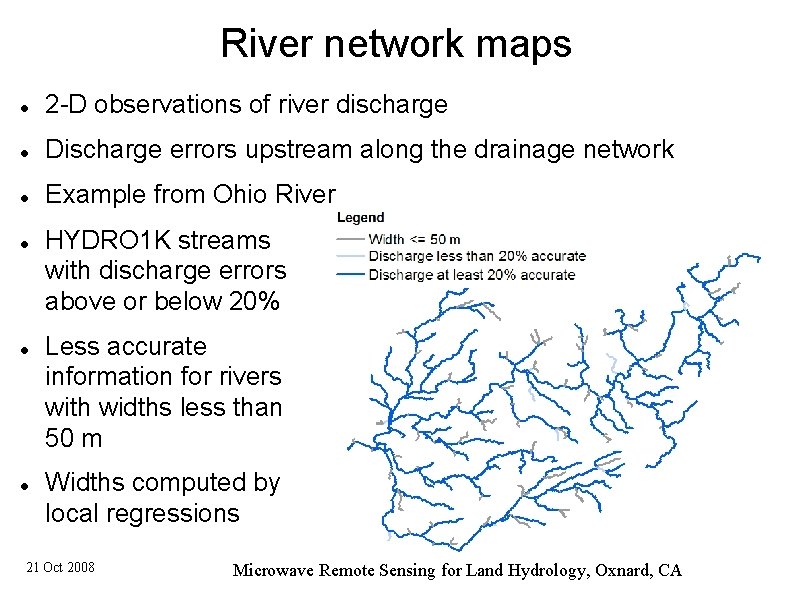
River network maps 2 -D observations of river discharge Discharge errors upstream along the drainage network Example from Ohio River HYDRO 1 K streams with discharge errors above or below 20% Less accurate information for rivers with widths less than 50 m Widths computed by local regressions 21 Oct 2008 Microwave Remote Sensing for Land Hydrology, Oxnard, CA
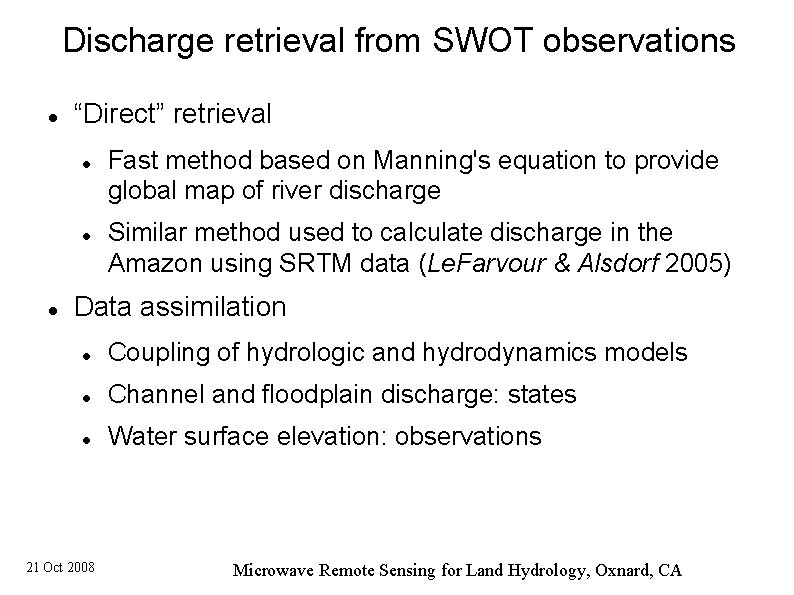
Discharge retrieval from SWOT observations “Direct” retrieval Fast method based on Manning's equation to provide global map of river discharge Similar method used to calculate discharge in the Amazon using SRTM data (Le. Farvour & Alsdorf 2005) Data assimilation Coupling of hydrologic and hydrodynamics models Channel and floodplain discharge: states Water surface elevation: observations 21 Oct 2008 Microwave Remote Sensing for Land Hydrology, Oxnard, CA
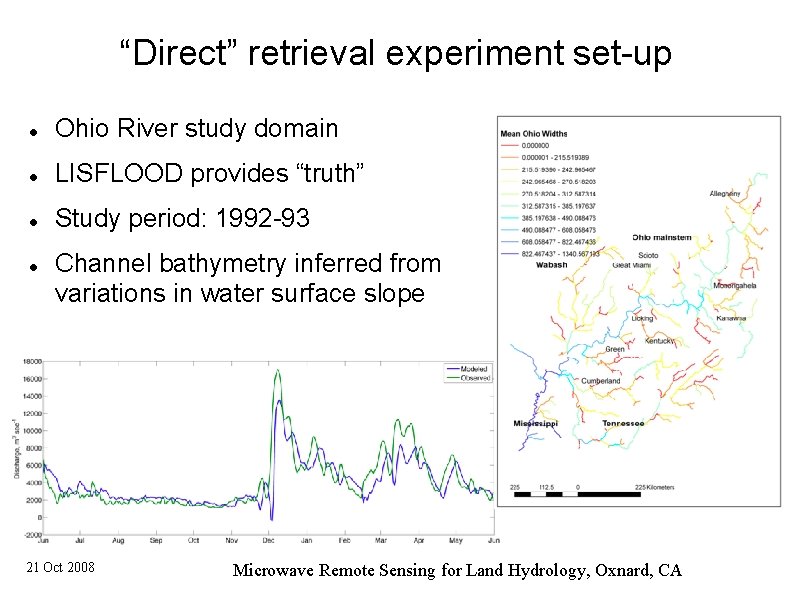
“Direct” retrieval experiment set-up Ohio River study domain LISFLOOD provides “truth” Study period: 1992 -93 Channel bathymetry inferred from variations in water surface slope 21 Oct 2008 Microwave Remote Sensing for Land Hydrology, Oxnard, CA
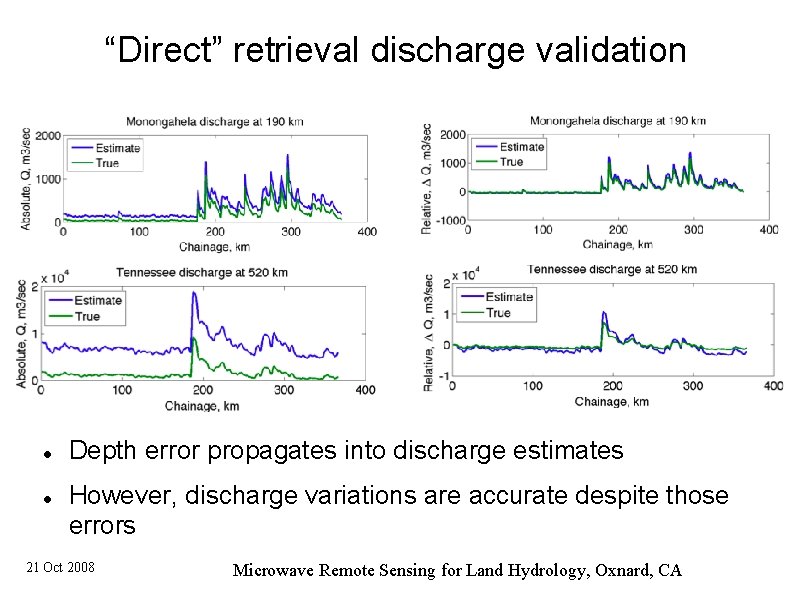
“Direct” retrieval discharge validation Depth error propagates into discharge estimates However, discharge variations are accurate despite those errors 21 Oct 2008 Microwave Remote Sensing for Land Hydrology, Oxnard, CA
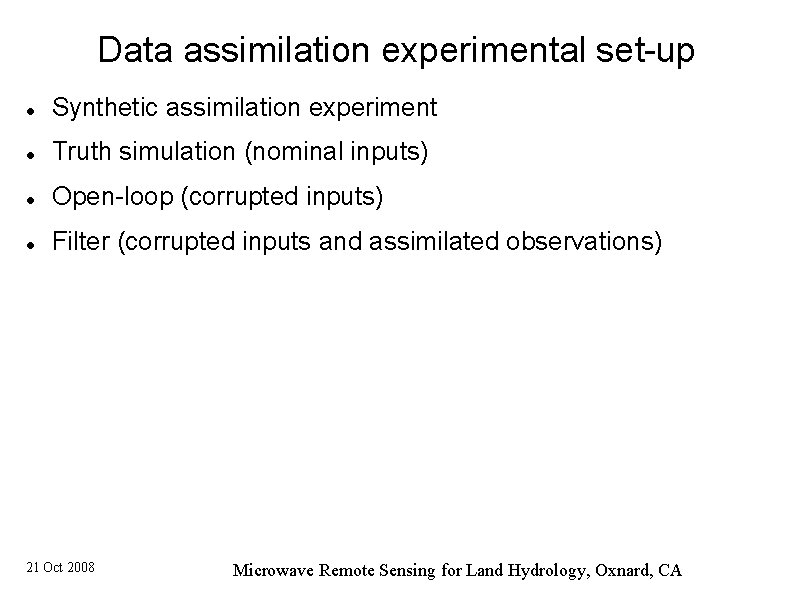
Data assimilation experimental set-up Synthetic assimilation experiment Truth simulation (nominal inputs) Open-loop (corrupted inputs) Filter (corrupted inputs and assimilated observations) 21 Oct 2008 Microwave Remote Sensing for Land Hydrology, Oxnard, CA
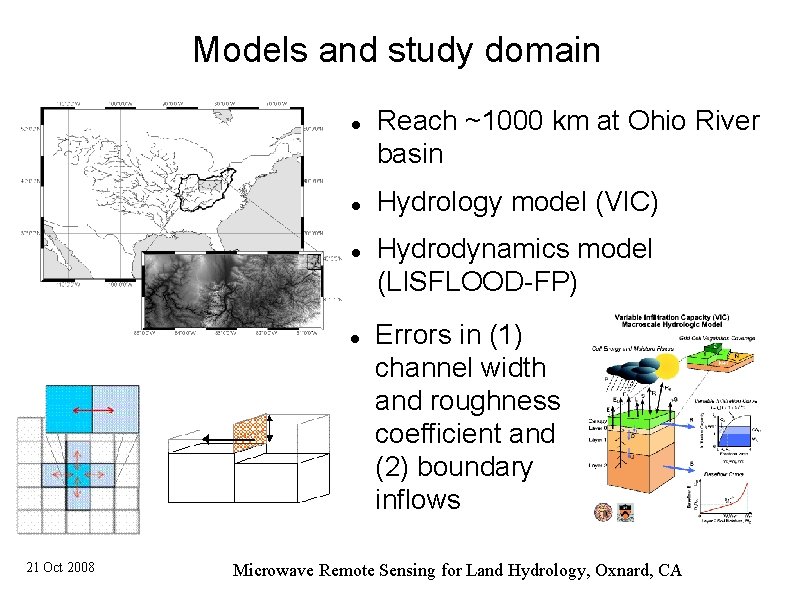
Models and study domain 21 Oct 2008 Reach ~1000 km at Ohio River basin Hydrology model (VIC) Hydrodynamics model (LISFLOOD-FP) Errors in (1) channel width and roughness coefficient and (2) boundary inflows Microwave Remote Sensing for Land Hydrology, Oxnard, CA
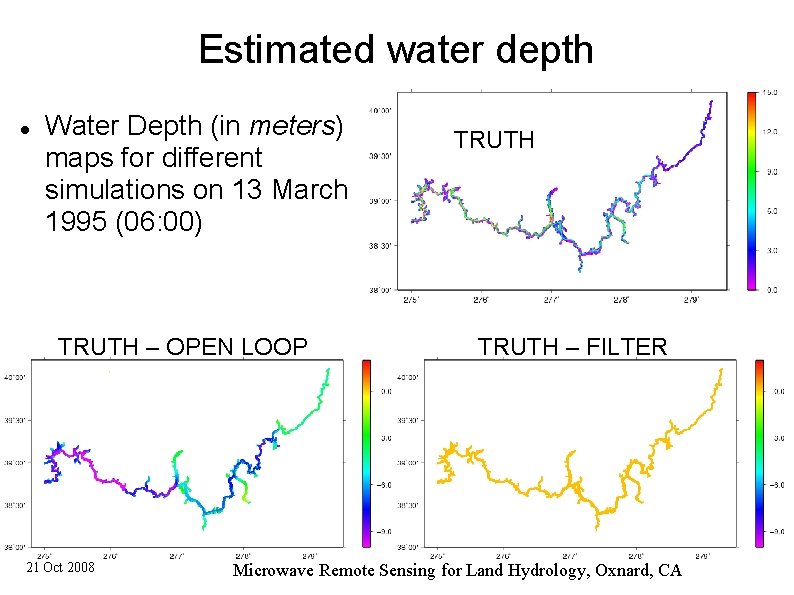
Estimated water depth Water Depth (in meters) maps for different simulations on 13 March 1995 (06: 00) TRUTH – OPEN LOOP 21 Oct 2008 TRUTH – FILTER Microwave Remote Sensing for Land Hydrology, Oxnard, CA
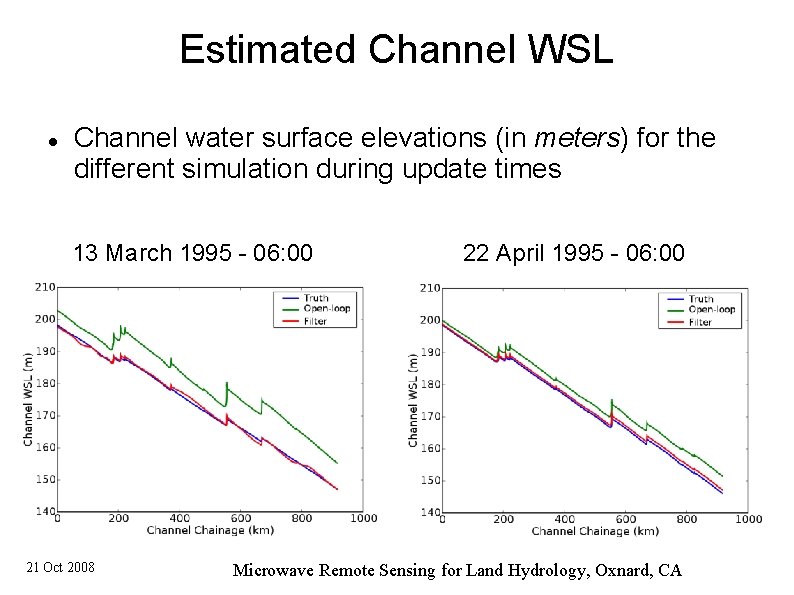
Estimated Channel WSL Channel water surface elevations (in meters) for the different simulation during update times 13 March 1995 - 06: 00 21 Oct 2008 22 April 1995 - 06: 00 Microwave Remote Sensing for Land Hydrology, Oxnard, CA
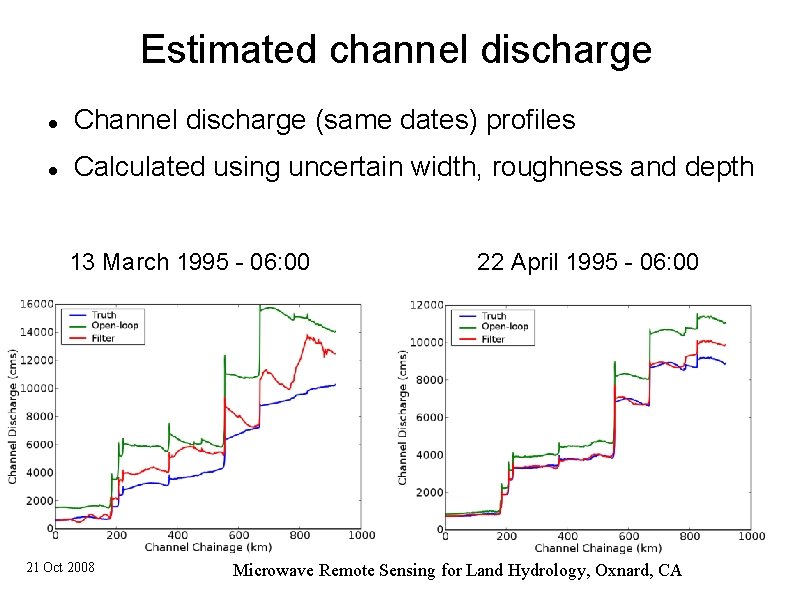
Estimated channel discharge Channel discharge (same dates) profiles Calculated using uncertain width, roughness and depth 13 March 1995 - 06: 00 21 Oct 2008 22 April 1995 - 06: 00 Microwave Remote Sensing for Land Hydrology, Oxnard, CA
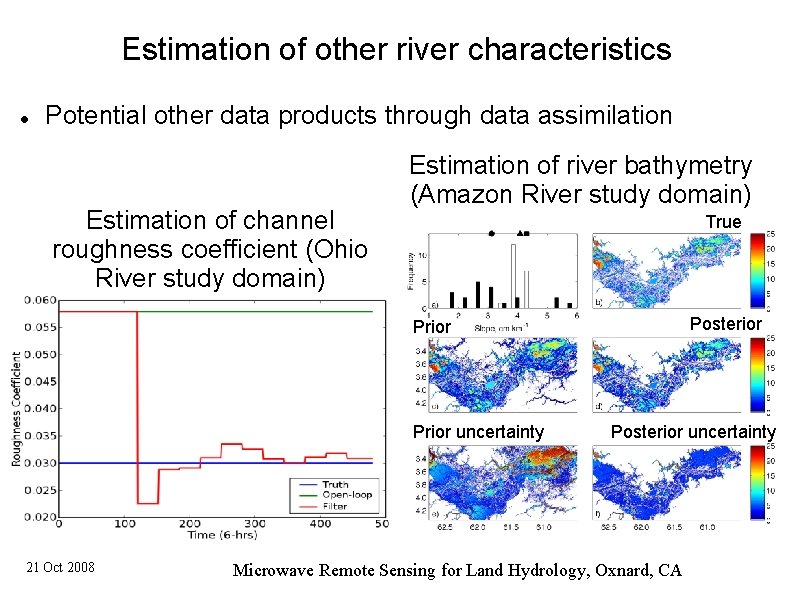
Estimation of other river characteristics Potential other data products through data assimilation Estimation of channel roughness coefficient (Ohio River study domain) Estimation of river bathymetry (Amazon River study domain) True Posterior Prior uncertainty 21 Oct 2008 Posterior uncertainty Microwave Remote Sensing for Land Hydrology, Oxnard, CA
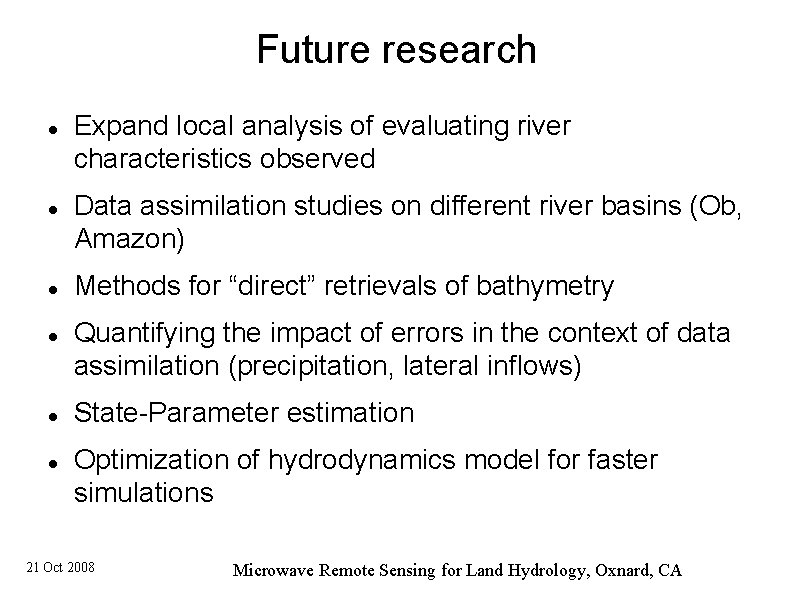
Future research Expand local analysis of evaluating river characteristics observed Data assimilation studies on different river basins (Ob, Amazon) Methods for “direct” retrievals of bathymetry Quantifying the impact of errors in the context of data assimilation (precipitation, lateral inflows) State-Parameter estimation Optimization of hydrodynamics model for faster simulations 21 Oct 2008 Microwave Remote Sensing for Land Hydrology, Oxnard, CA
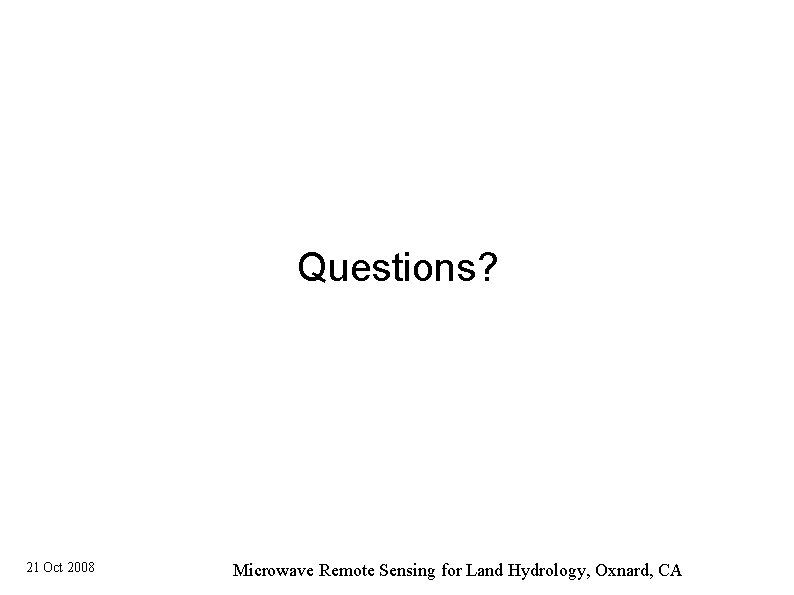
Questions? 21 Oct 2008 Microwave Remote Sensing for Land Hydrology, Oxnard, CA
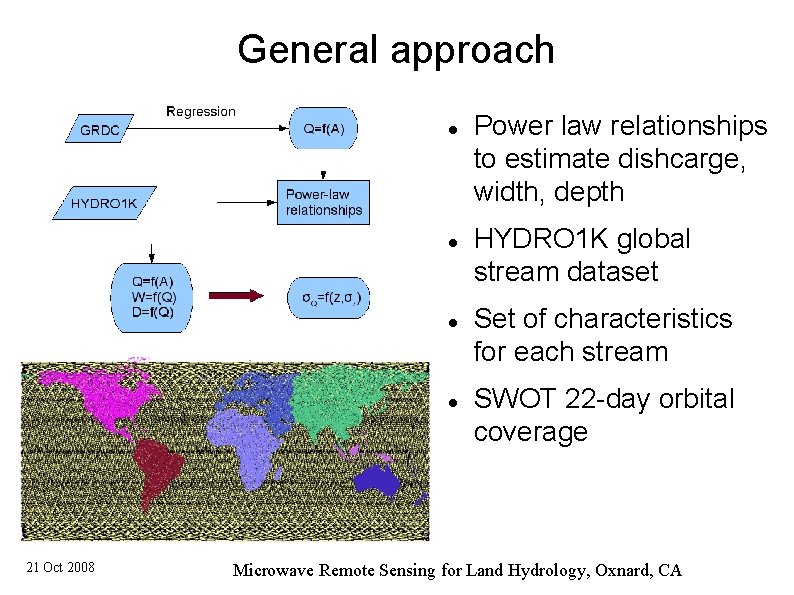
General approach 21 Oct 2008 Power law relationships to estimate dishcarge, width, depth HYDRO 1 K global stream dataset Set of characteristics for each stream SWOT 22 -day orbital coverage Microwave Remote Sensing for Land Hydrology, Oxnard, CA
Kostas andreadis
Mike durand strength coach
Lycée jacques durand
Jean-nicolas-louis durand
Faciba 1959
Isa durand rebels
Biblionisep
Kostas ostrauskas
Kostas glampedakis
Kostas ostrauskas
Lenovo swot
Has virtual functions and accessible non-virtual destructor
Inditex presentation
Swot analysis whole foods
Swot analysis for procurement department
Walmart swot
Strength of goldilocks
Konsep usaha roti bakar
Pldt strengths and weaknesses
Chanel no 5 slogan
Slogan family
Swot analysis for gaming industry
Kişisel swot analizi örnekleri
Best buy swot
Opportunities and threats of a teacher
Tsmc swot