Statistical engineering and analytical considerations for scaledown model
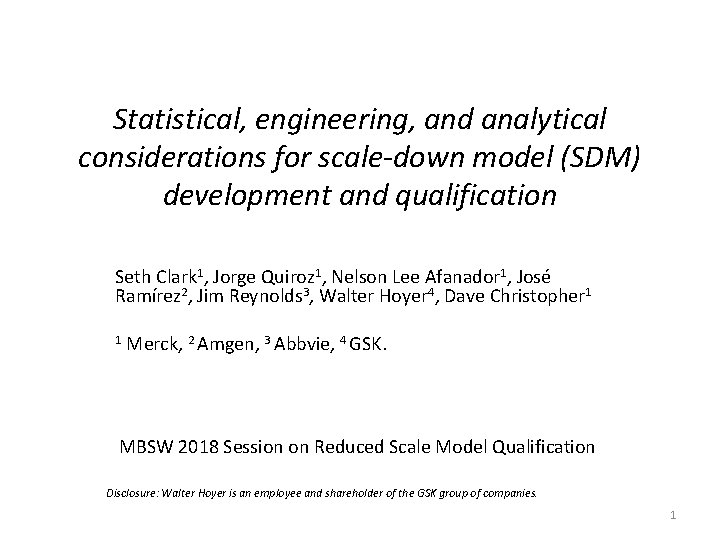
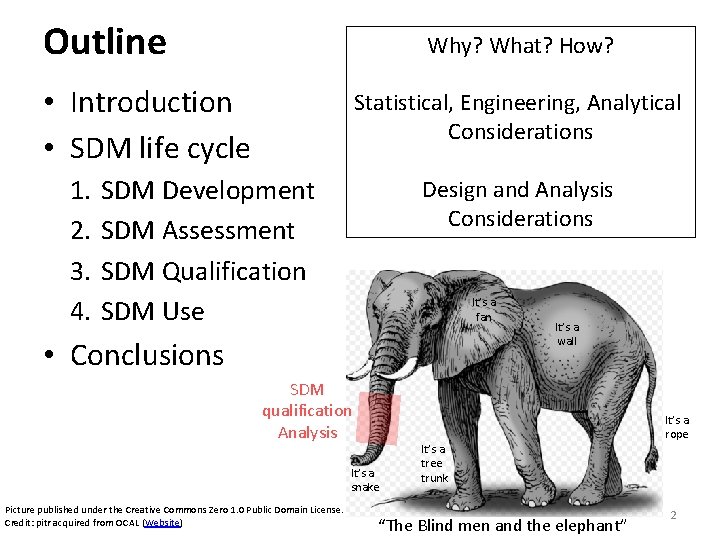
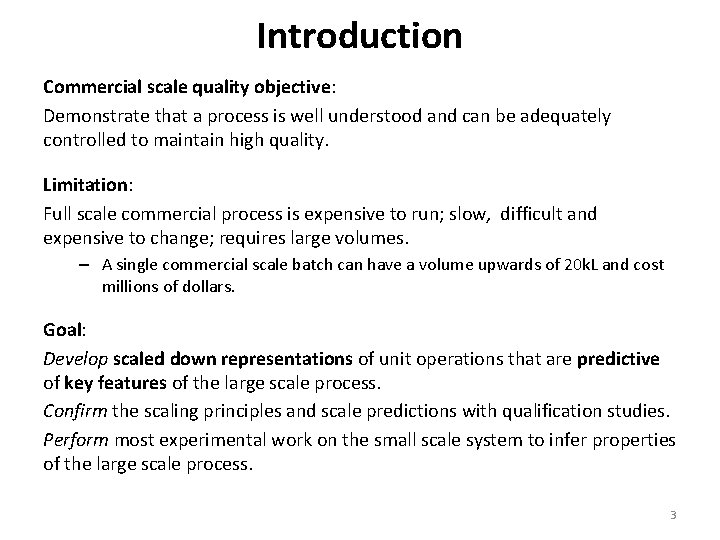
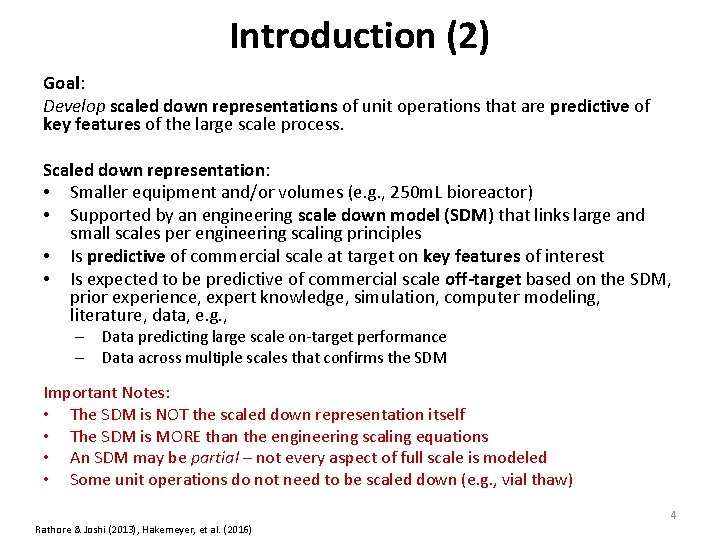
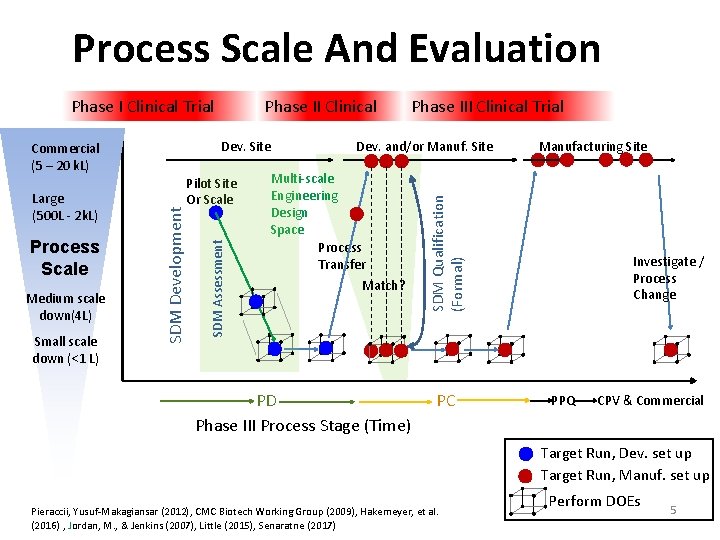
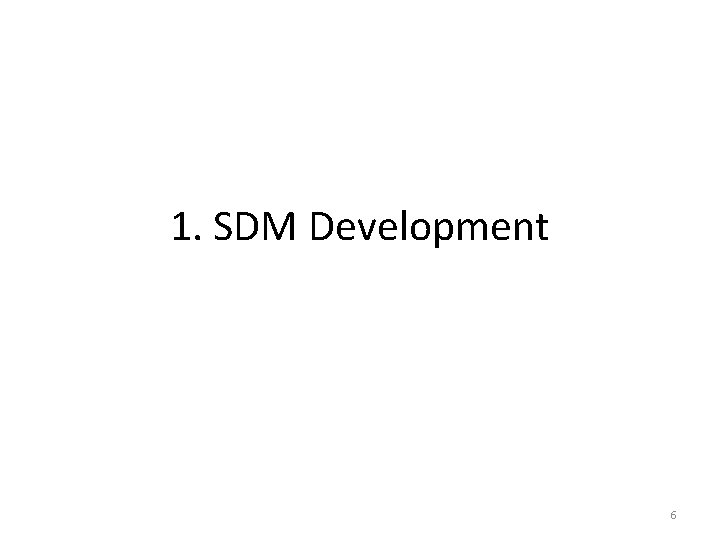
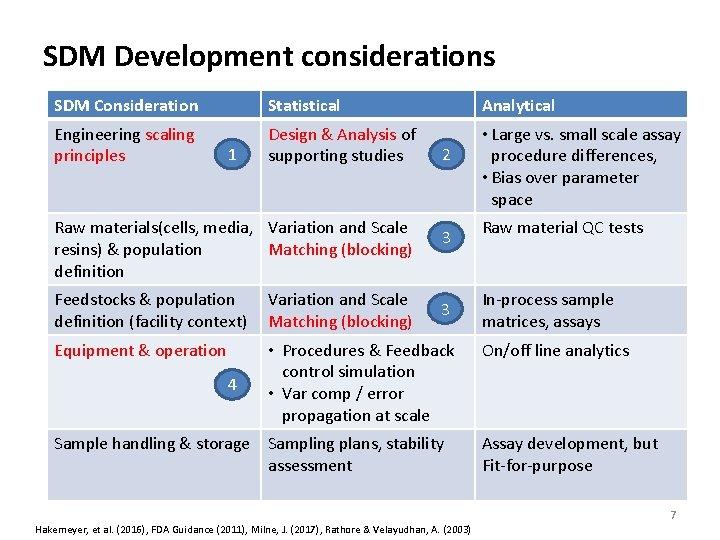
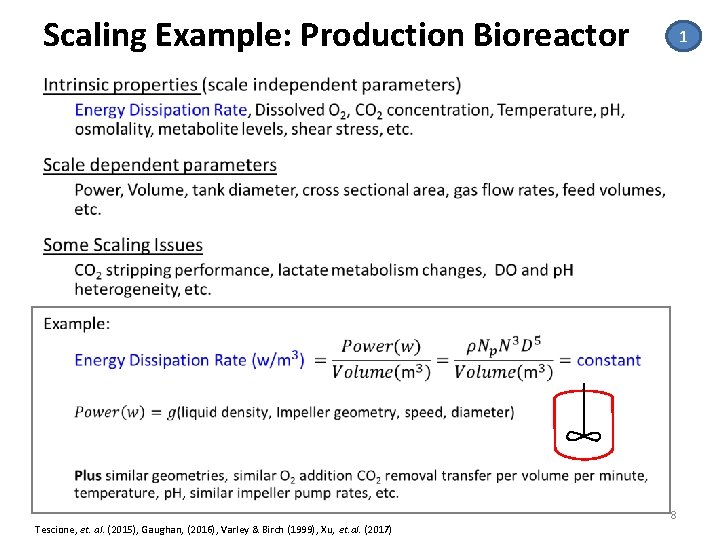
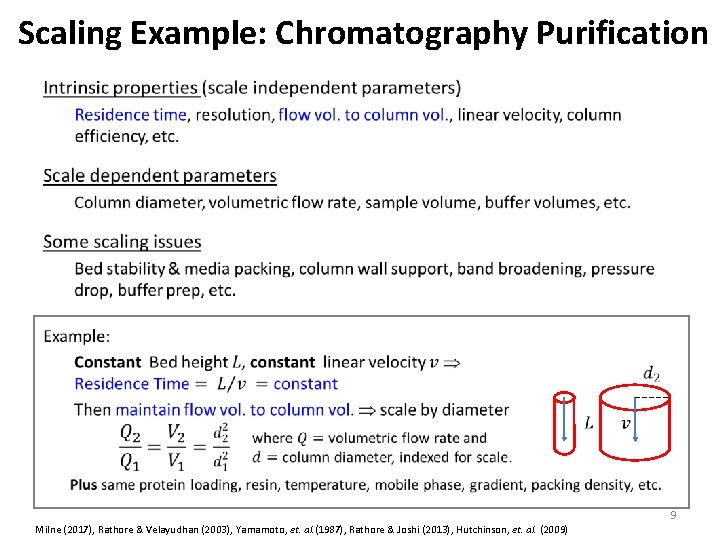
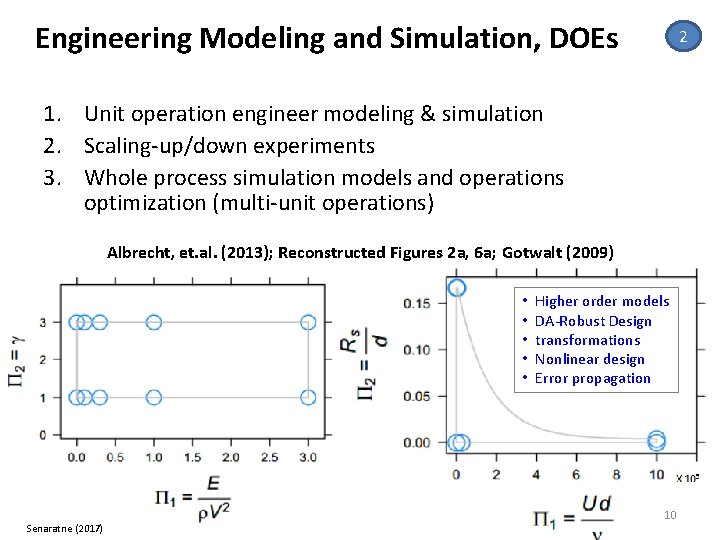
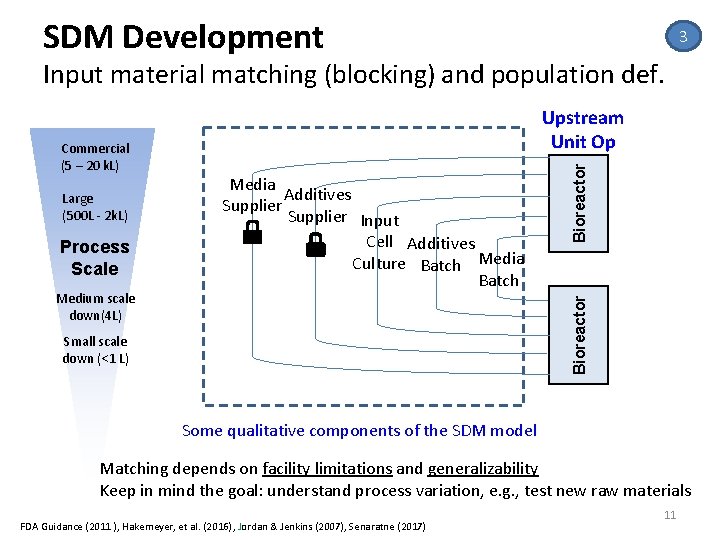
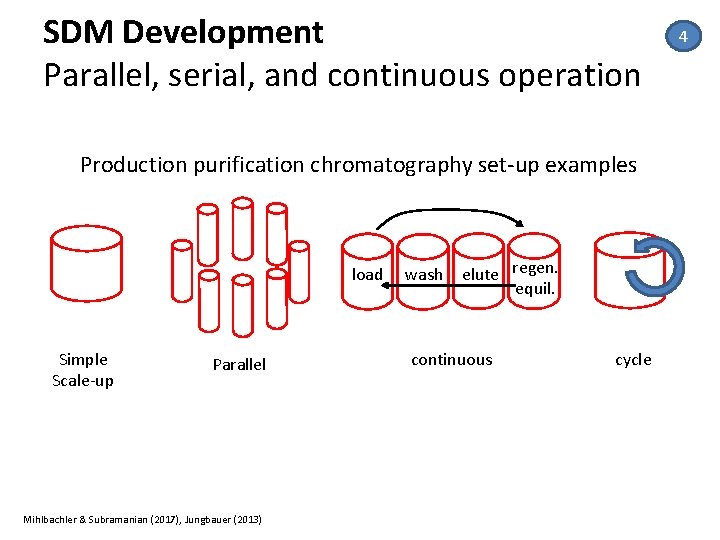
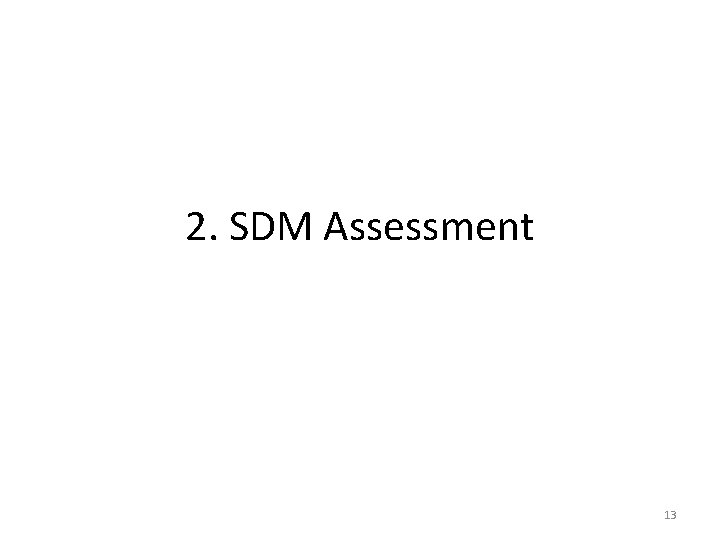
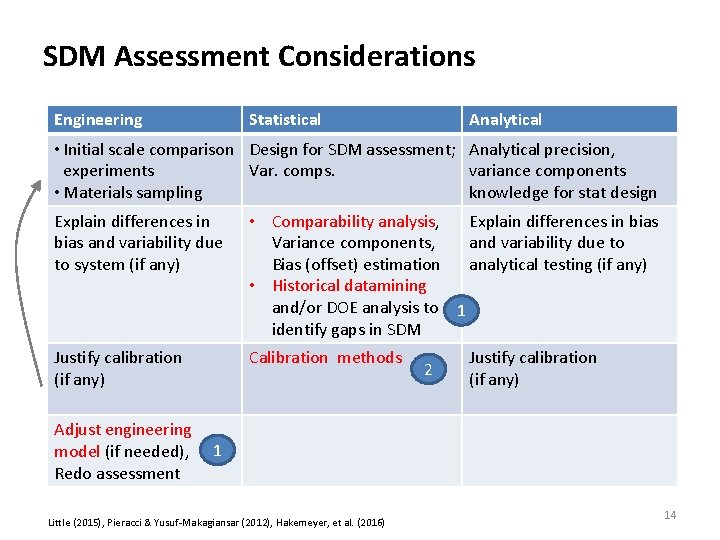
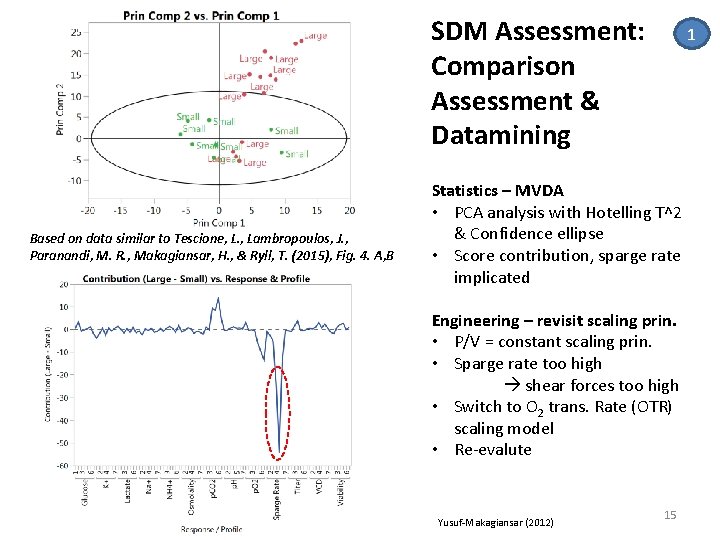
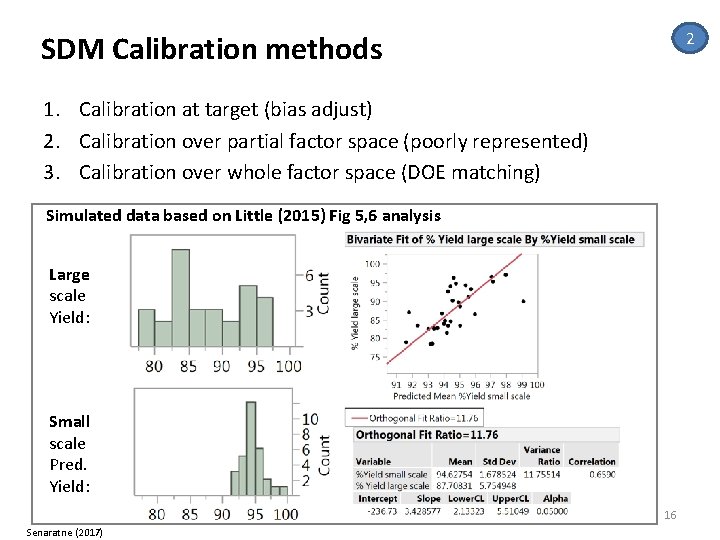
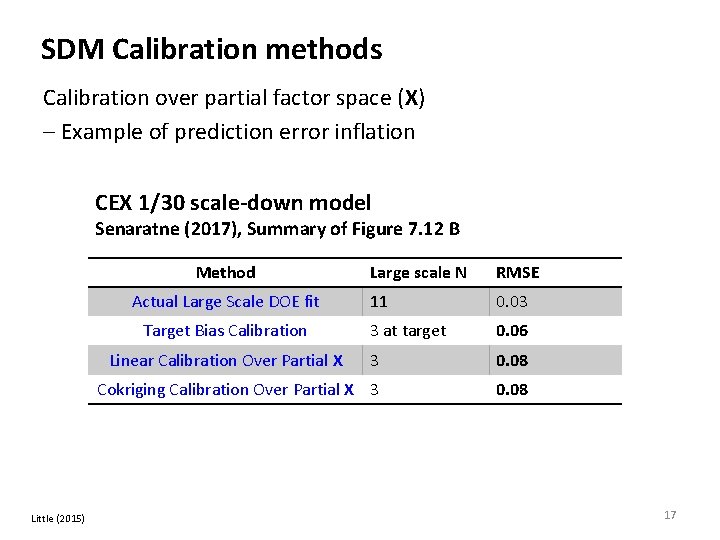
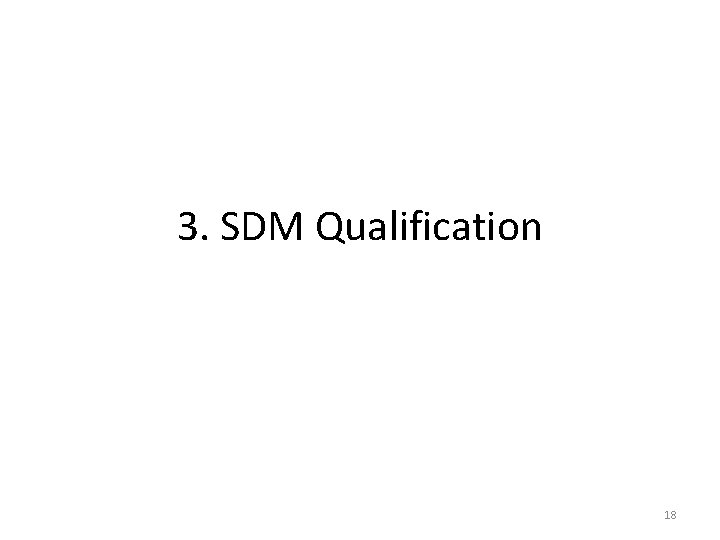
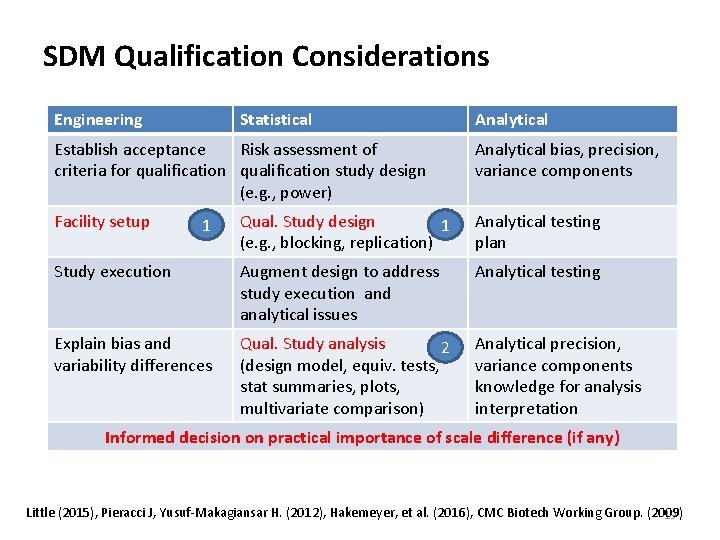
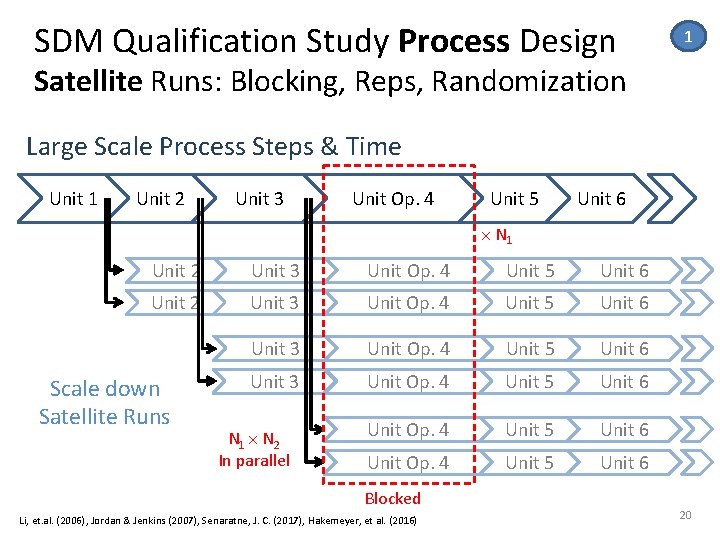
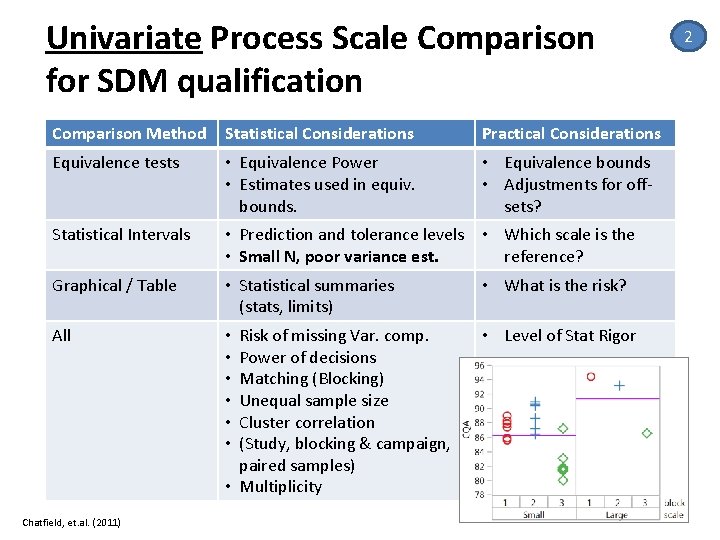
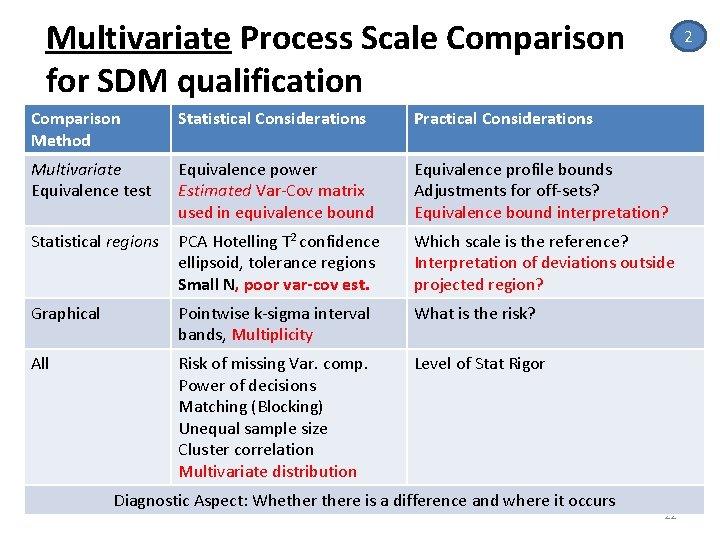
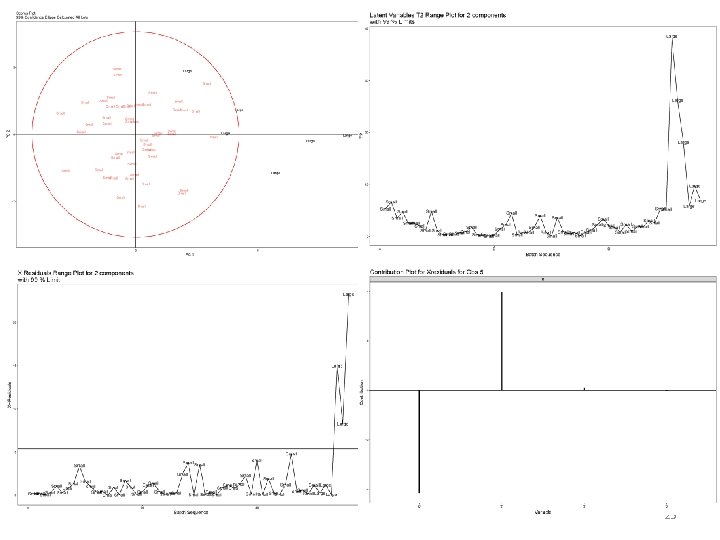
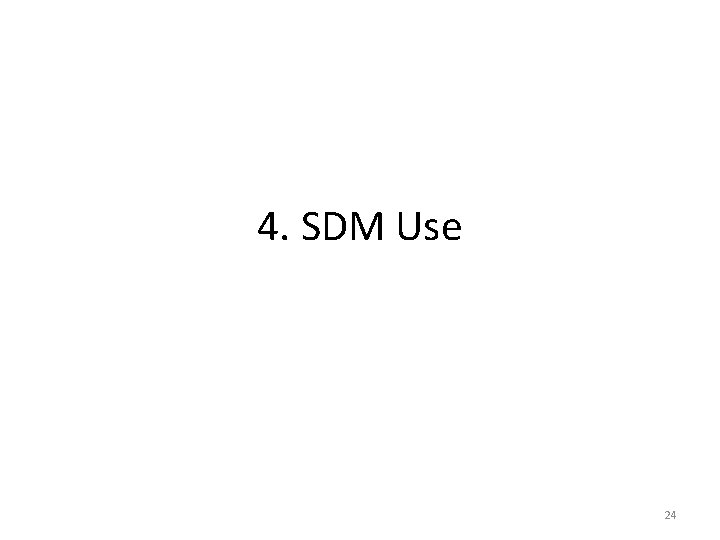
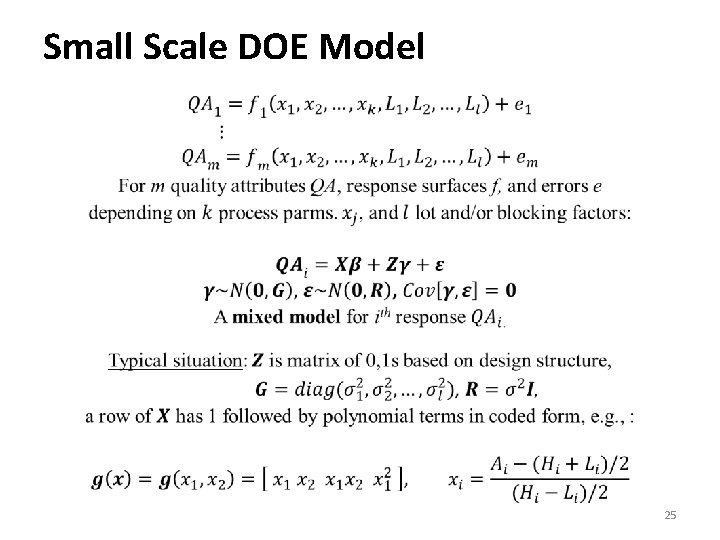
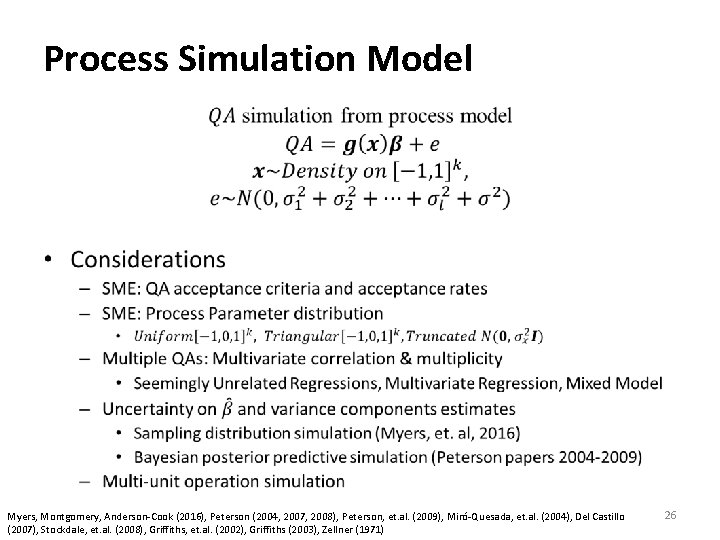
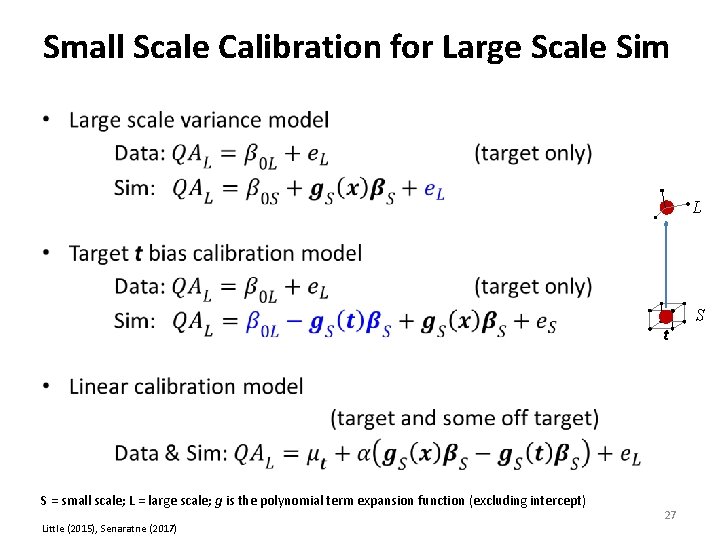
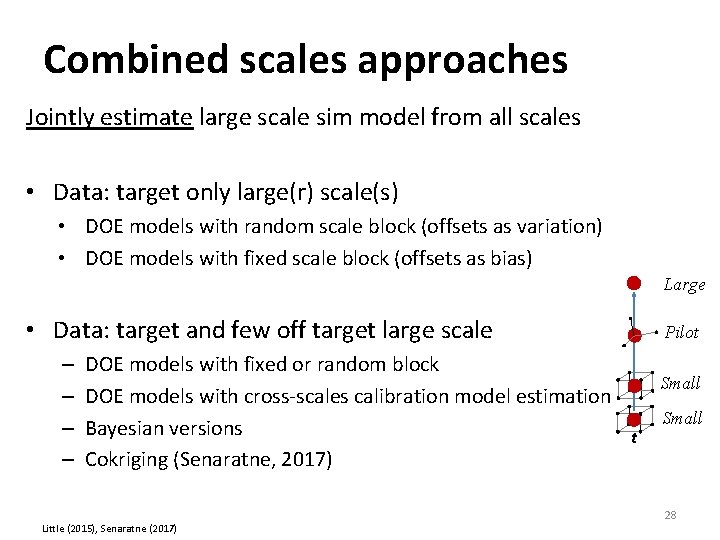
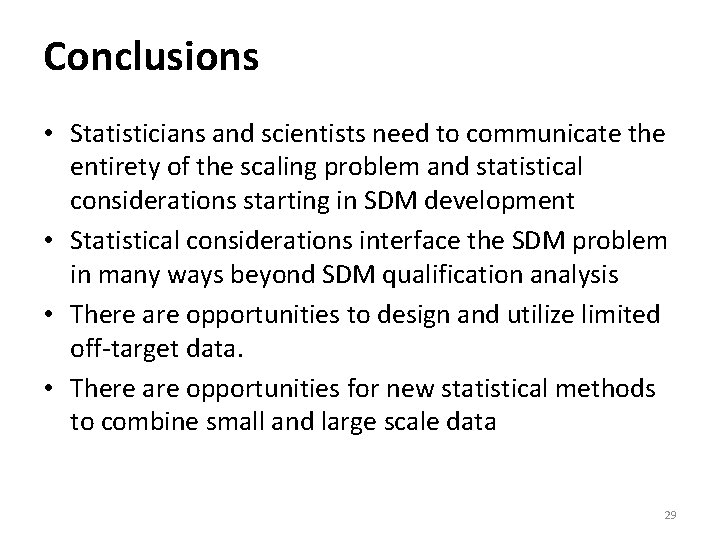
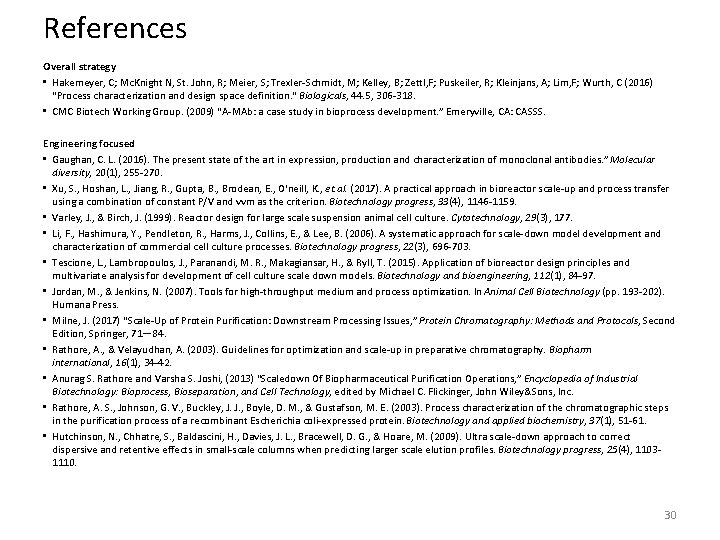
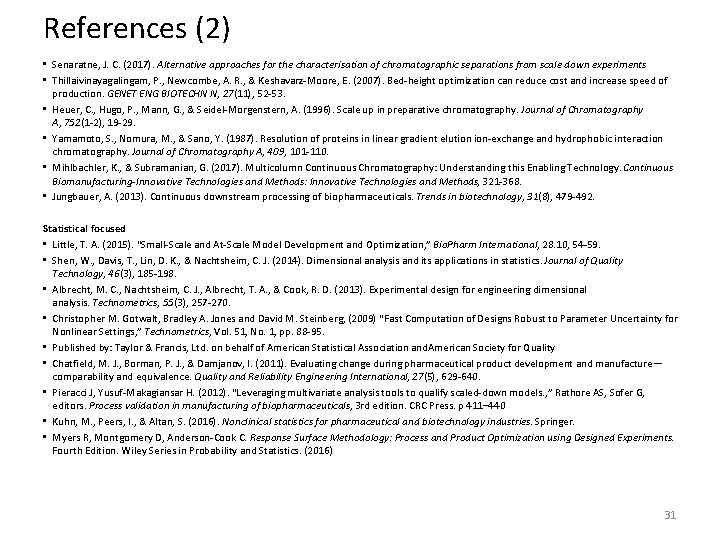
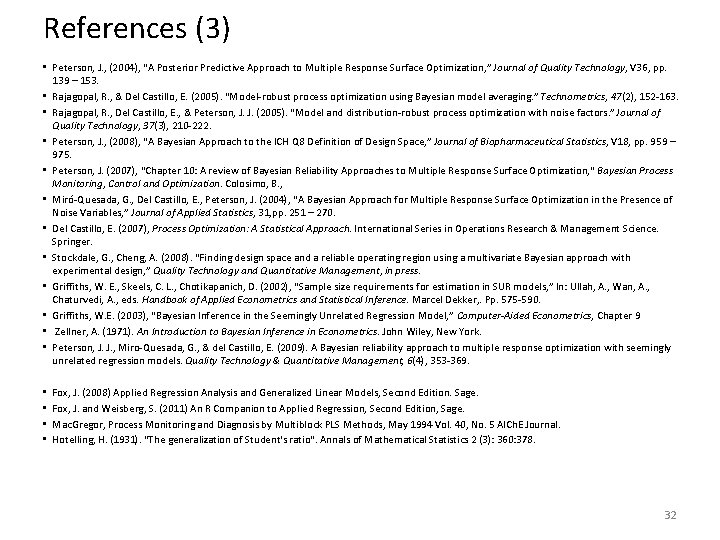
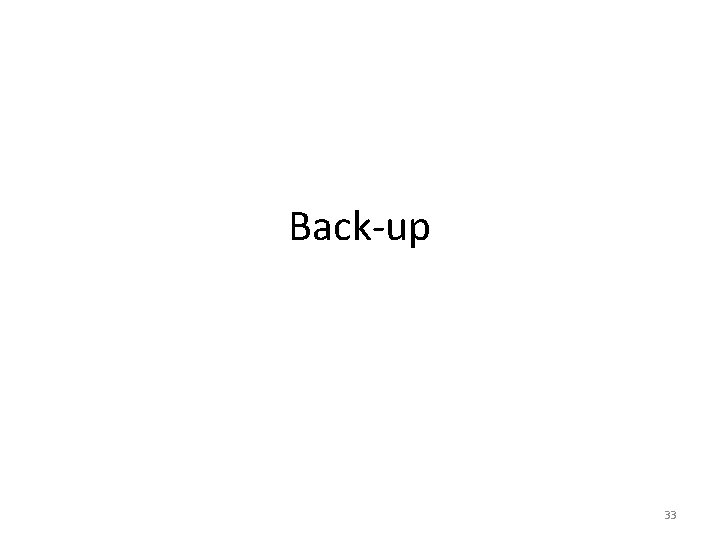
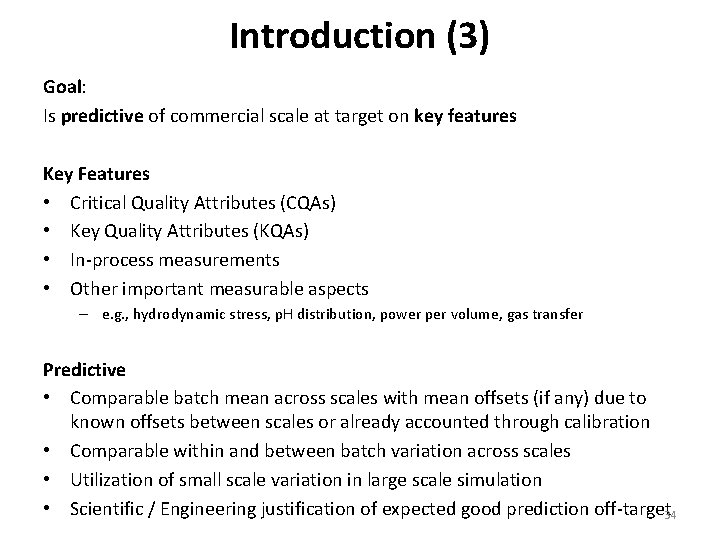
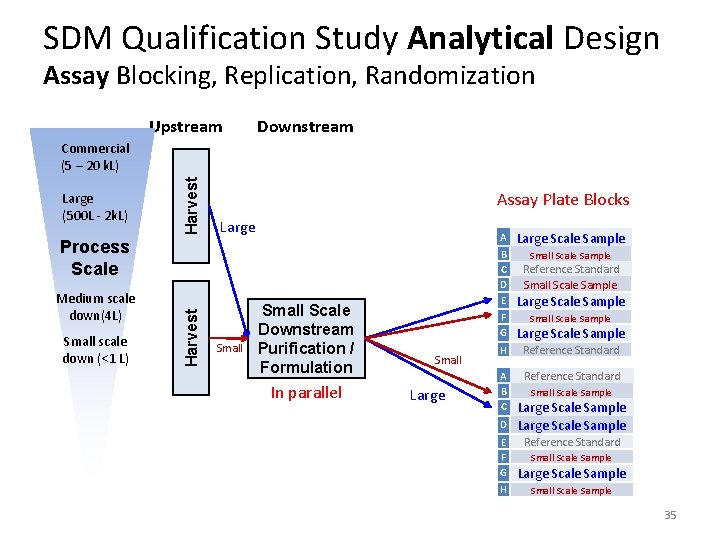
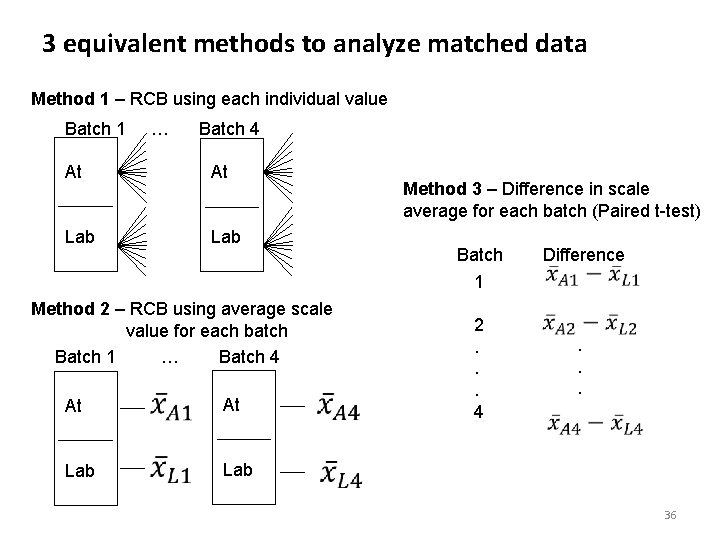
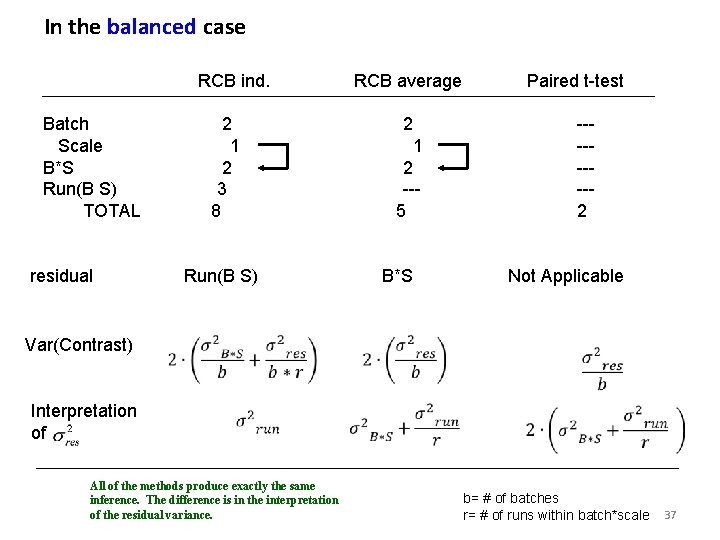
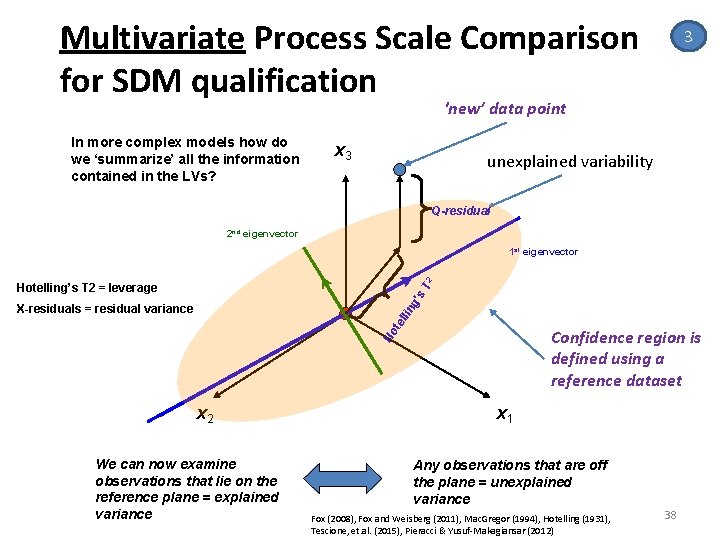
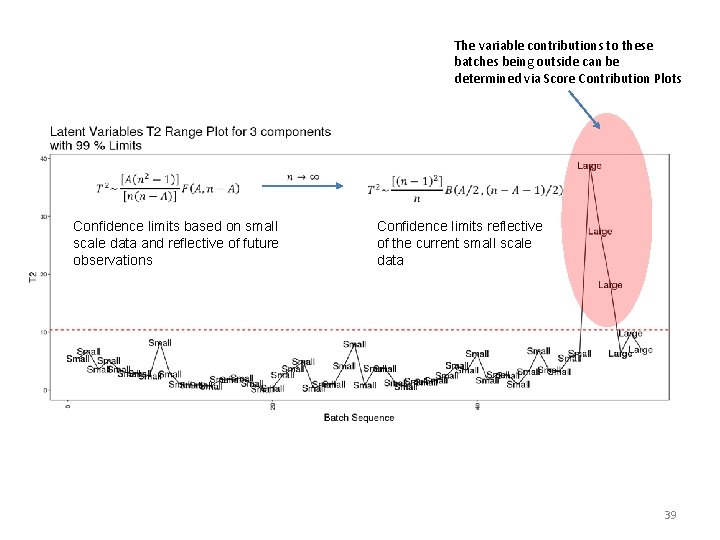
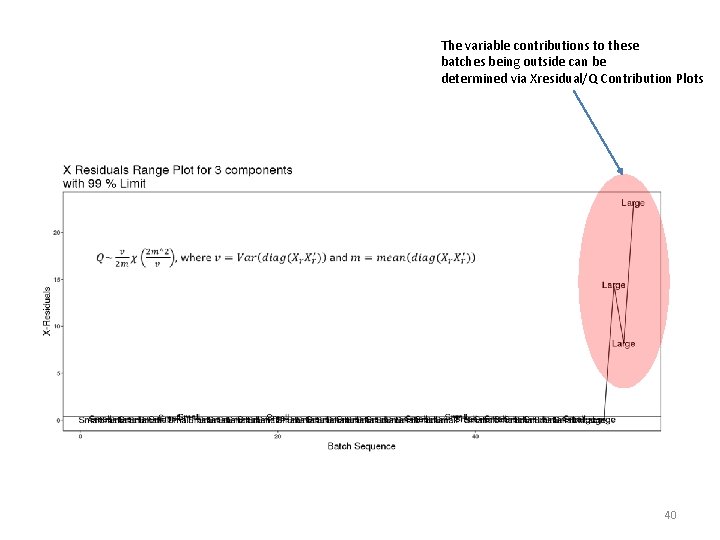
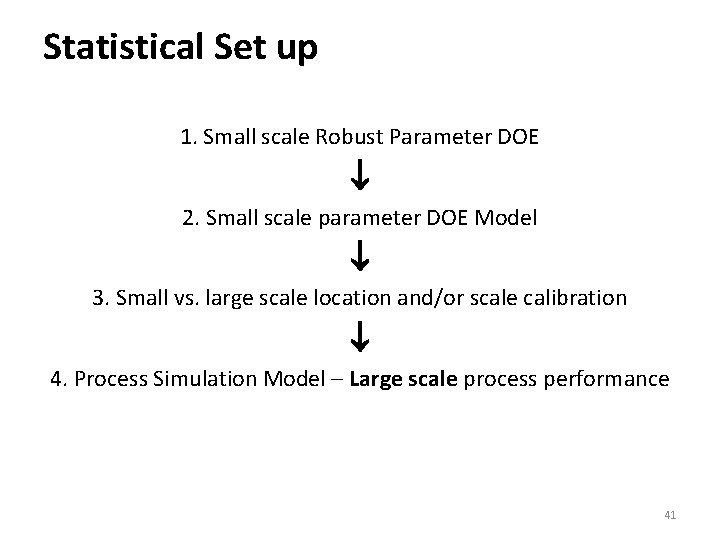
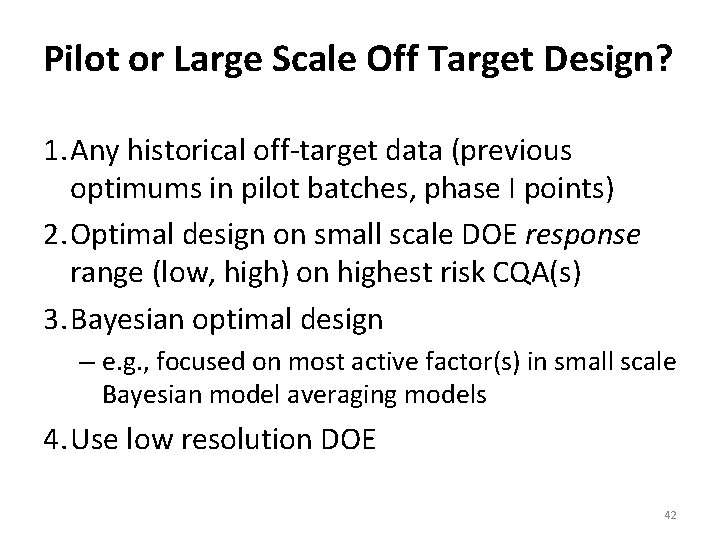
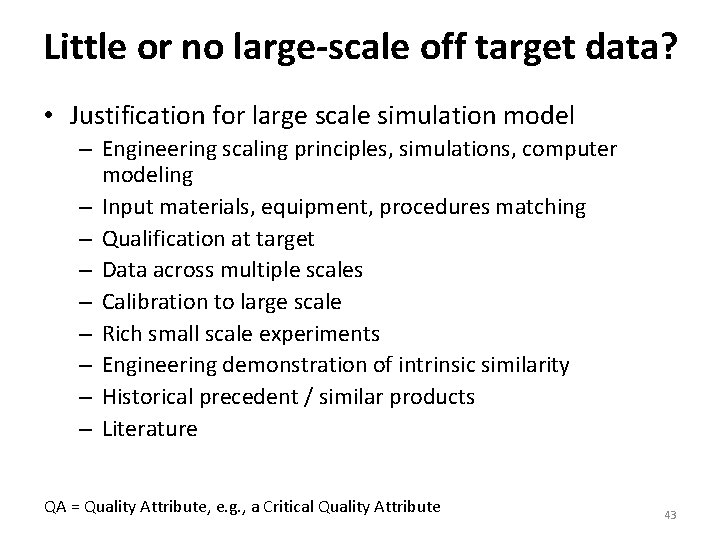
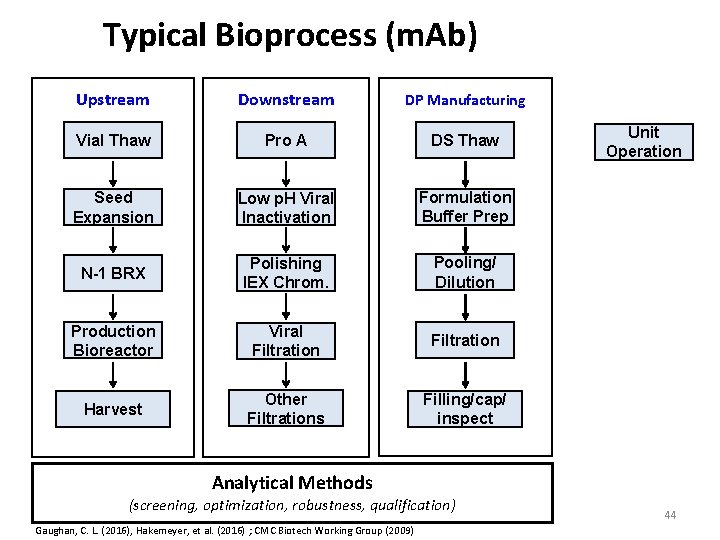
- Slides: 44
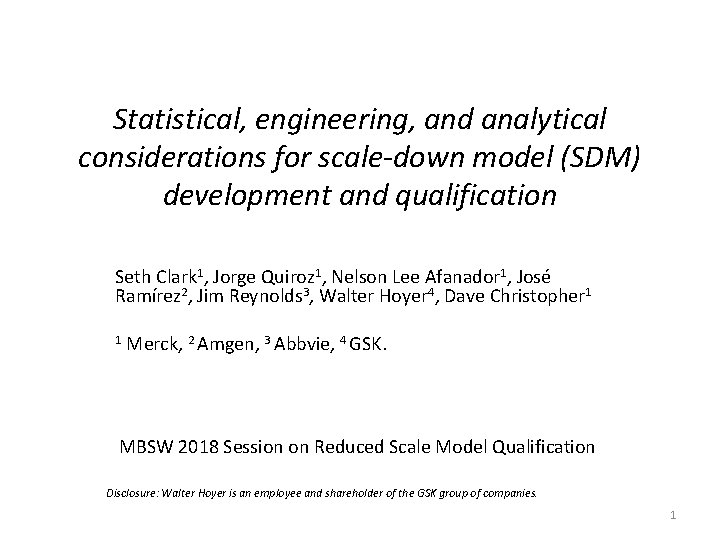
Statistical, engineering, and analytical considerations for scale‐down model (SDM) development and qualification Seth Clark 1, Jorge Quiroz 1, Nelson Lee Afanador 1, José Ramírez 2, Jim Reynolds 3, Walter Hoyer 4, Dave Christopher 1 1 Merck, 2 Amgen, 3 Abbvie, 4 GSK. MBSW 2018 Session on Reduced Scale Model Qualification Disclosure: Walter Hoyer is an employee and shareholder of the GSK group of companies. 1
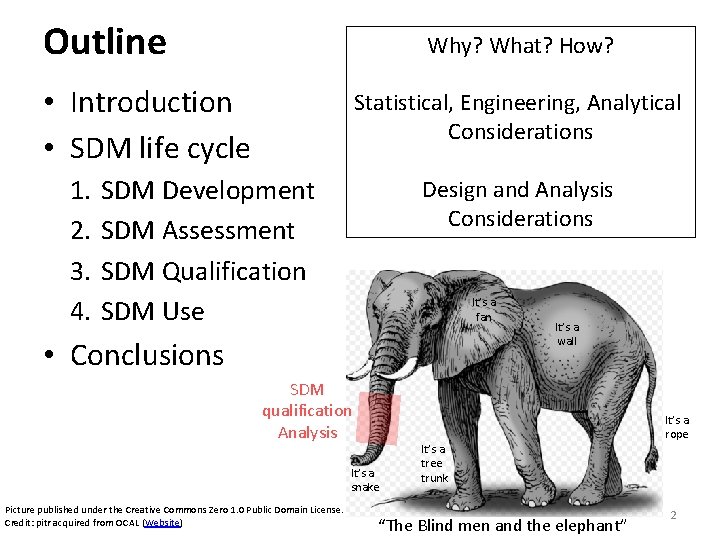
Outline Why? What? How? • Introduction • SDM life cycle 1. 2. 3. 4. Statistical, Engineering, Analytical Considerations SDM Development SDM Assessment SDM Qualification SDM Use Design and Analysis Considerations It’s a fan • Conclusions SDM qualification Analysis It’s a snake Picture published under the Creative Commons Zero 1. 0 Public Domain License. Credit: pitr acquired from OCAL (Website) It’s a wall It’s a tree trunk “The Blind men and the elephant” It’s a rope 2
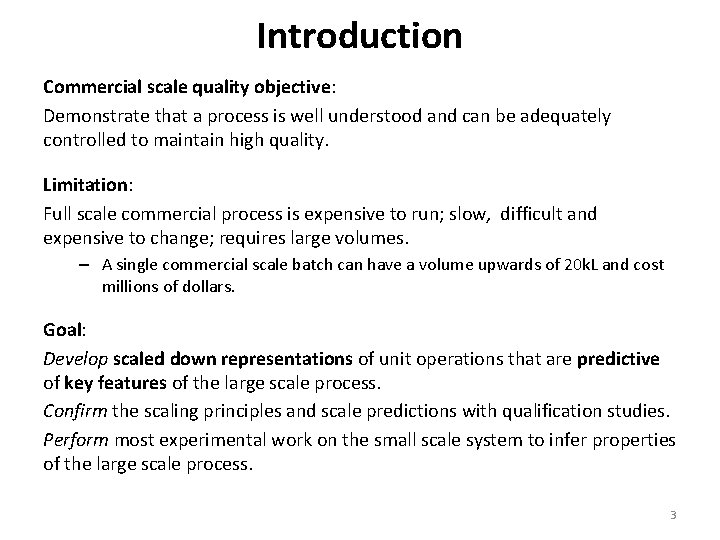
Introduction Commercial scale quality objective: Demonstrate that a process is well understood and can be adequately controlled to maintain high quality. Limitation: Full scale commercial process is expensive to run; slow, difficult and expensive to change; requires large volumes. – A single commercial scale batch can have a volume upwards of 20 k. L and cost millions of dollars. Goal: Develop scaled down representations of unit operations that are predictive of key features of the large scale process. Confirm the scaling principles and scale predictions with qualification studies. Perform most experimental work on the small scale system to infer properties of the large scale process. 3
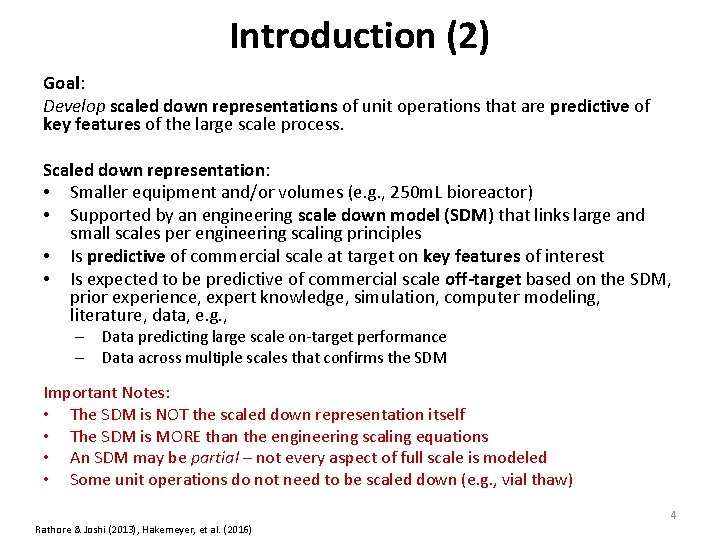
Introduction (2) Goal: Develop scaled down representations of unit operations that are predictive of key features of the large scale process. Scaled down representation: • Smaller equipment and/or volumes (e. g. , 250 m. L bioreactor) • Supported by an engineering scale down model (SDM) that links large and small scales per engineering scaling principles • Is predictive of commercial scale at target on key features of interest • Is expected to be predictive of commercial scale off-target based on the SDM, prior experience, expert knowledge, simulation, computer modeling, literature, data, e. g. , – Data predicting large scale on‐target performance – Data across multiple scales that confirms the SDM Important Notes: • The SDM is NOT the scaled down representation itself • The SDM is MORE than the engineering scaling equations • An SDM may be partial – not every aspect of full scale is modeled • Some unit operations do not need to be scaled down (e. g. , vial thaw) 4 Rathore & Joshi (2013), Hakemeyer, et al. (2016)
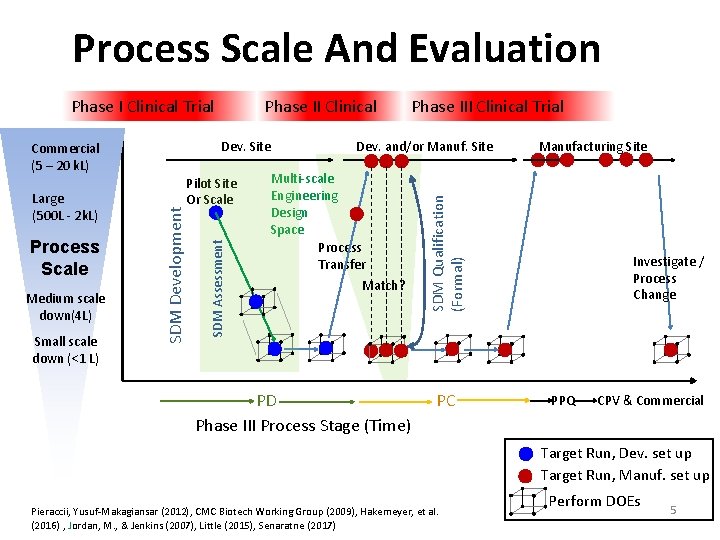
Process Scale And Evaluation Dev. Site Medium scale down(4 L) Small scale down (<1 L) Pilot Site Or Scale SDM Assessment Process Scale SDM Development Commercial (5 – 20 k. L) Large (500 L ‐ 2 k. L) Phase II Clinical Phase III Clinical Trial Dev. and/or Manuf. Site Multi‐scale Engineering Design Space Process Transfer Match? PD Phase III Process Stage (Time) Manufacturing Site SDM Qualification (Formal) Phase I Clinical Trial PC Investigate / Process Change PPQ CPV & Commercial Target Run, Dev. set up Target Run, Manuf. set up Pieraccii, Yusuf‐Makagiansar (2012), CMC Biotech Working Group (2009), Hakemeyer, et al. (2016) , Jordan, M. , & Jenkins (2007), Little (2015), Senaratne (2017) Perform DOEs 5
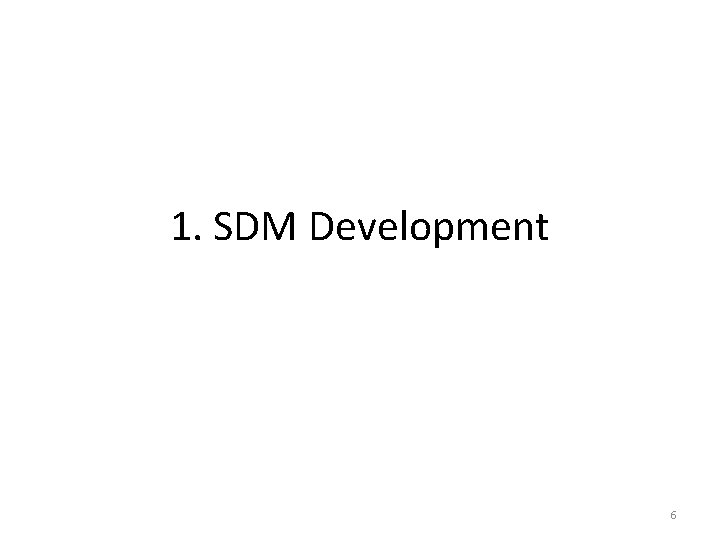
1. SDM Development 6
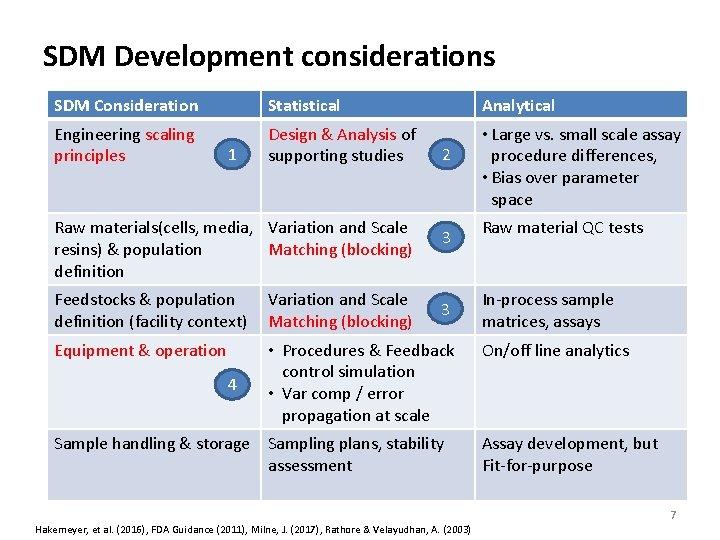
SDM Development considerations SDM Consideration Statistical Analytical Engineering scaling principles Design & Analysis of supporting studies • Large vs. small scale assay procedure differences, • Bias over parameter space 1 Raw materials(cells, media, Variation and Scale resins) & population Matching (blocking) definition 2 3 Feedstocks & population definition (facility context) Variation and Scale Matching (blocking) Equipment & operation • Procedures & Feedback control simulation • Var comp / error propagation at scale 4 3 Sample handling & storage Sampling plans, stability assessment Raw material QC tests In‐process sample matrices, assays On/off line analytics Assay development, but Fit‐for‐purpose 7 Hakemeyer, et al. (2016), FDA Guidance (2011), Milne, J. (2017), Rathore & Velayudhan, A. (2003)
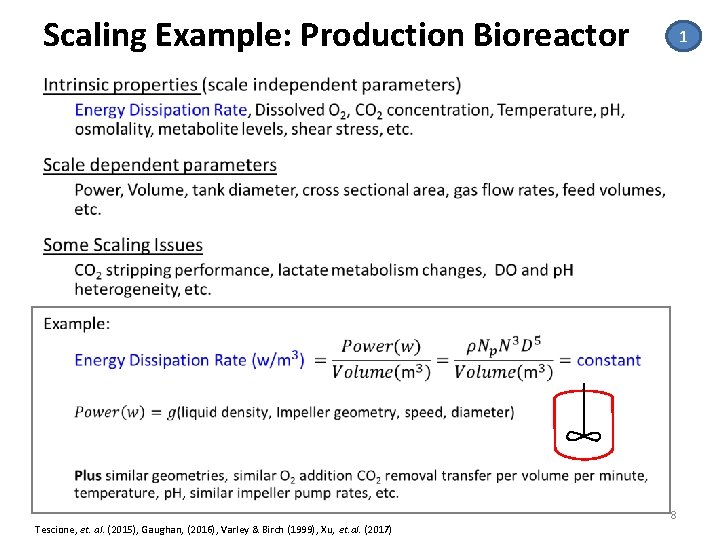
Scaling Example: Production Bioreactor 1 • 8 Tescione, et. al. (2015), Gaughan, (2016), Varley & Birch (1999), Xu, et. al. (2017)
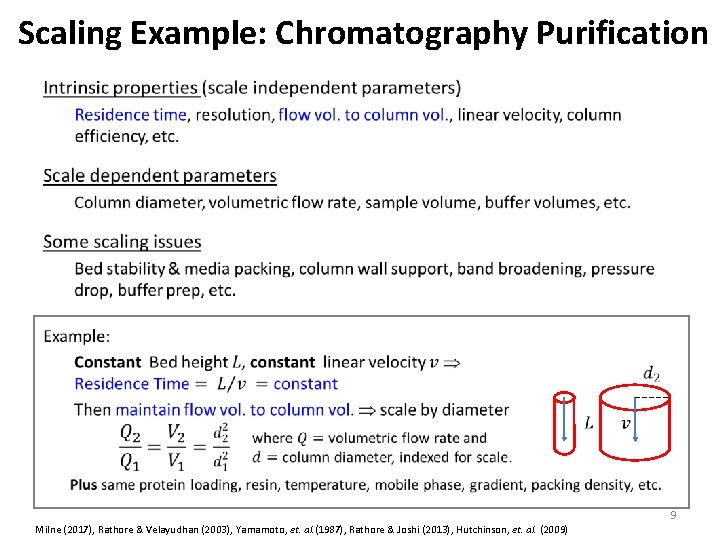
Scaling Example: Chromatography Purification • 9 Milne (2017), Rathore & Velayudhan (2003), Yamamoto, et. al. (1987), Rathore & Joshi (2013), Hutchinson, et. al. (2009)
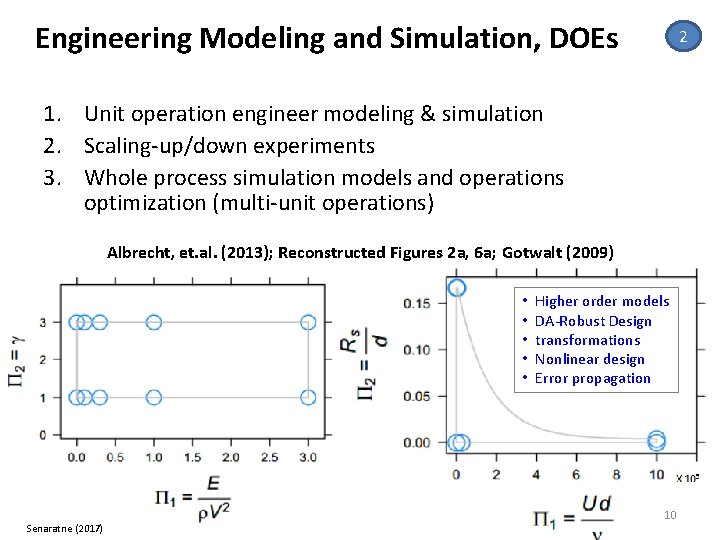
Engineering Modeling and Simulation, DOEs 2 1. Unit operation engineer modeling & simulation 2. Scaling‐up/down experiments 3. Whole process simulation models and operations optimization (multi‐unit operations) Albrecht, et. al. (2013); Reconstructed Figures 2 a, 6 a; Gotwalt (2009) • • • Senaratne (2017) Higher order models DA‐Robust Design transformations Nonlinear design Error propagation 10
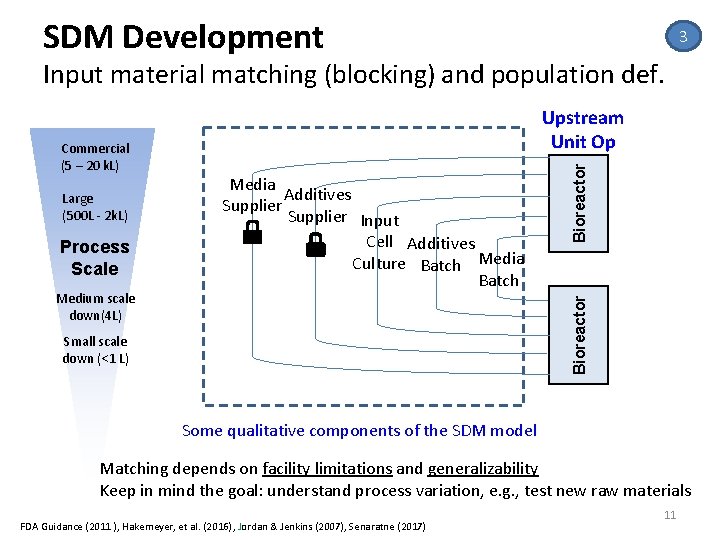
SDM Development 3 Input material matching (blocking) and population def. Process Scale Medium scale down(4 L) Media Additives Supplier Input Cell Additives Culture Batch Media Batch Bioreactor Large (500 L ‐ 2 k. L) Bioreactor Commercial (5 – 20 k. L) Upstream Unit Op Small scale down (<1 L) Some qualitative components of the SDM model Matching depends on facility limitations and generalizability Keep in mind the goal: understand process variation, e. g. , test new raw materials FDA Guidance (2011 ), Hakemeyer, et al. (2016), Jordan & Jenkins (2007), Senaratne (2017) 11
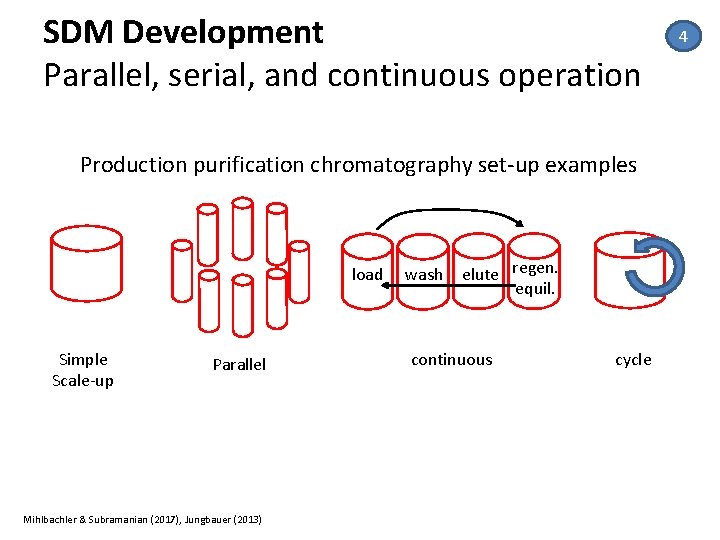
SDM Development Parallel, serial, and continuous operation Production purification chromatography set‐up examples load Simple Scale‐up Parallel Mihlbachler & Subramanian (2017), Jungbauer (2013) wash elute regen. equil. continuous cycle 4
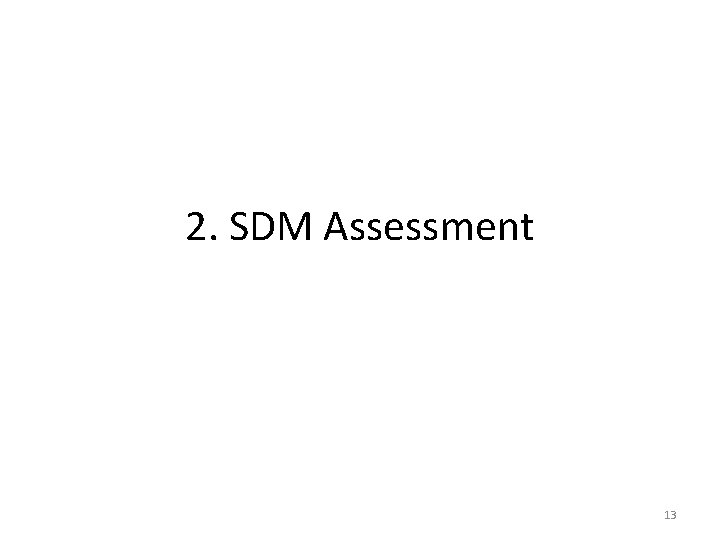
2. SDM Assessment 13
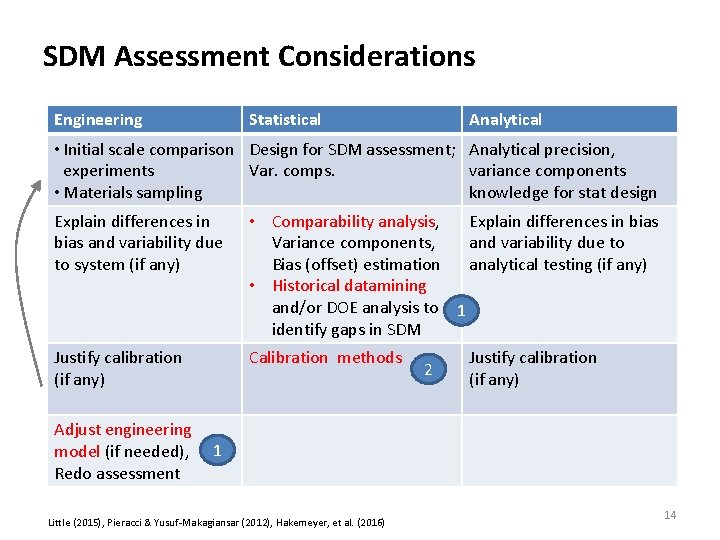
SDM Assessment Considerations Engineering Statistical Analytical • Initial scale comparison Design for SDM assessment; Analytical precision, experiments Var. comps. variance components • Materials sampling knowledge for stat design Explain differences in bias and variability due to system (if any) • Comparability analysis, Explain differences in bias Variance components, and variability due to Bias (offset) estimation analytical testing (if any) • Historical datamining and/or DOE analysis to 1 identify gaps in SDM Justify calibration (if any) Calibration methods 2 Justify calibration (if any) Adjust engineering model (if needed), 1 Redo assessment Little (2015), Pieracci & Yusuf‐Makagiansar (2012), Hakemeyer, et al. (2016) 14
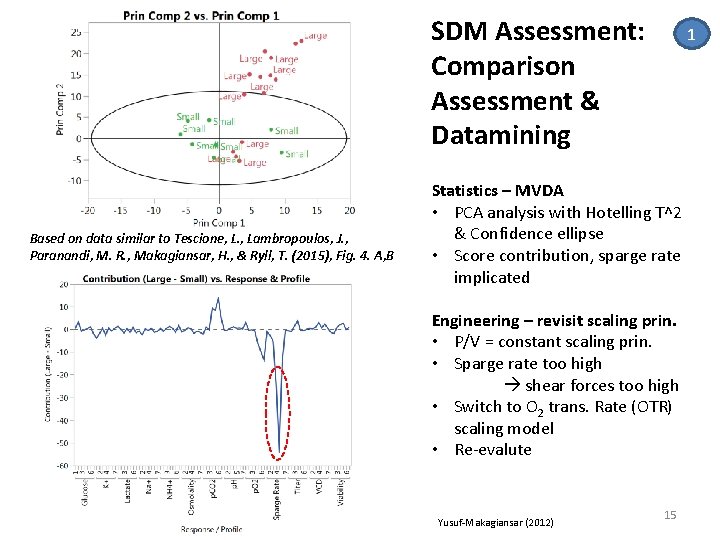
SDM Assessment: Comparison Assessment & Datamining Based on data similar to Tescione, L. , Lambropoulos, J. , Paranandi, M. R. , Makagiansar, H. , & Ryll, T. (2015), Fig. 4. A, B 1 Statistics – MVDA • PCA analysis with Hotelling T^2 & Confidence ellipse • Score contribution, sparge rate implicated Engineering – revisit scaling prin. • P/V = constant scaling prin. • Sparge rate too high shear forces too high • Switch to O 2 trans. Rate (OTR) scaling model • Re‐evalute Yusuf‐Makagiansar (2012) 15
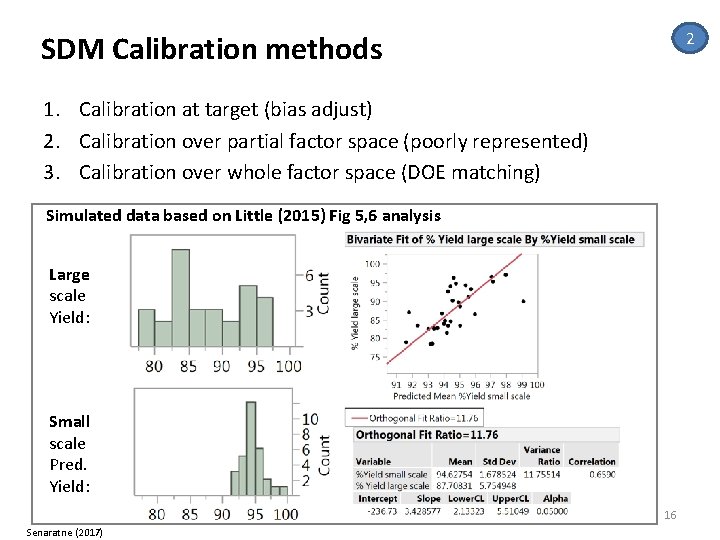
2 SDM Calibration methods 1. Calibration at target (bias adjust) 2. Calibration over partial factor space (poorly represented) 3. Calibration over whole factor space (DOE matching) Simulated data based on Little (2015) Fig 5, 6 analysis Large scale Yield: Small scale Pred. Yield: 16 Senaratne (2017)
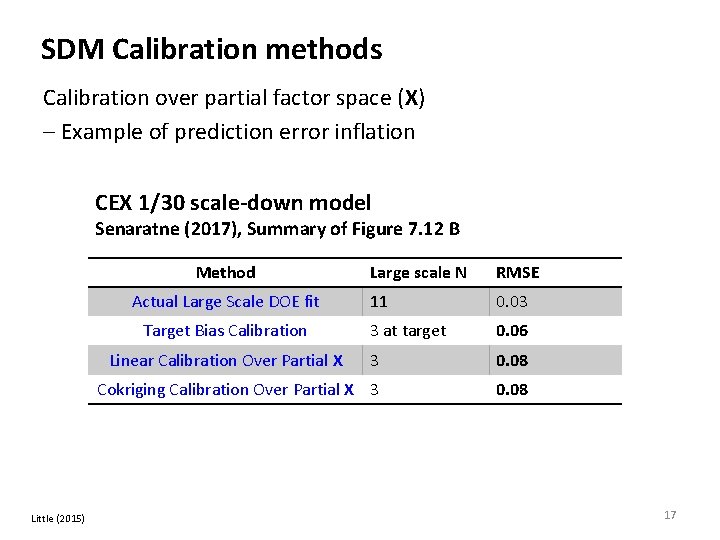
SDM Calibration methods Calibration over partial factor space (X) – Example of prediction error inflation CEX 1/30 scale-down model Senaratne (2017), Summary of Figure 7. 12 B Method Large scale N RMSE 11 0. 03 3 at target 0. 06 3 0. 08 Cokriging Calibration Over Partial X 3 0. 08 Actual Large Scale DOE fit Target Bias Calibration Linear Calibration Over Partial X Little (2015) 17
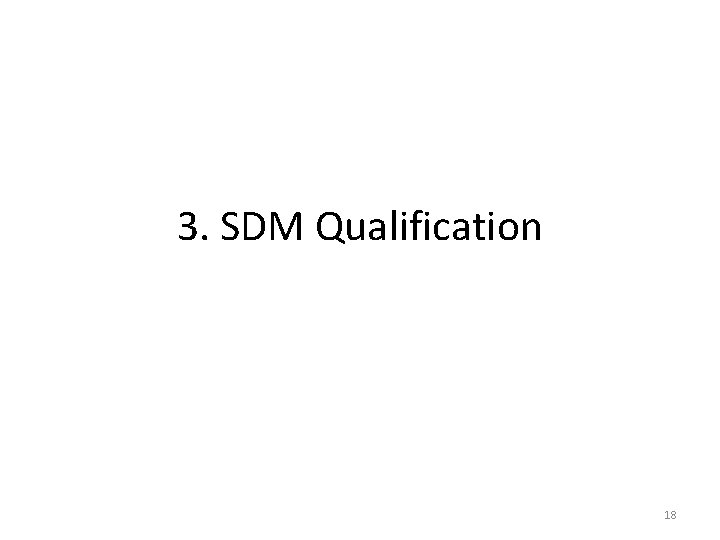
3. SDM Qualification 18
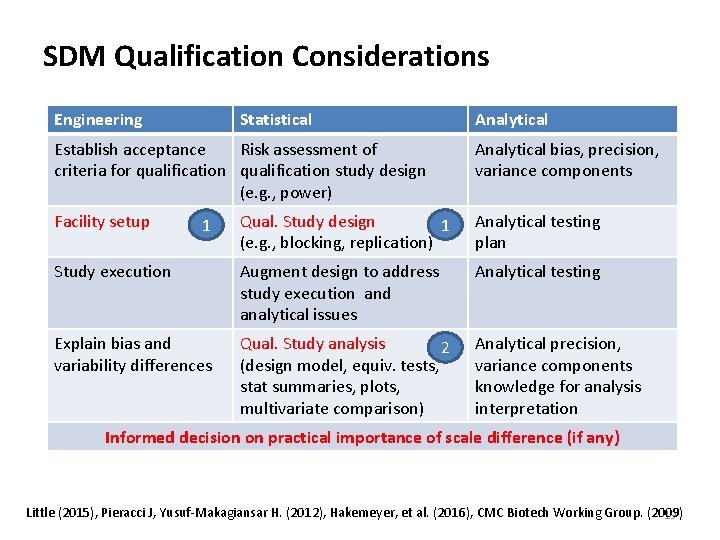
SDM Qualification Considerations Engineering Statistical Analytical Establish acceptance Risk assessment of criteria for qualification study design (e. g. , power) Analytical bias, precision, variance components Facility setup Qual. Study design 1 (e. g. , blocking, replication) Analytical testing plan Study execution Augment design to address study execution and analytical issues Analytical testing Explain bias and variability differences Qual. Study analysis 2 (design model, equiv. tests, stat summaries, plots, multivariate comparison) Analytical precision, variance components knowledge for analysis interpretation 1 Informed decision on practical importance of scale difference (if any) Little (2015), Pieracci J, Yusuf‐Makagiansar H. (2012), Hakemeyer, et al. (2016), CMC Biotech Working Group. (2009) 19
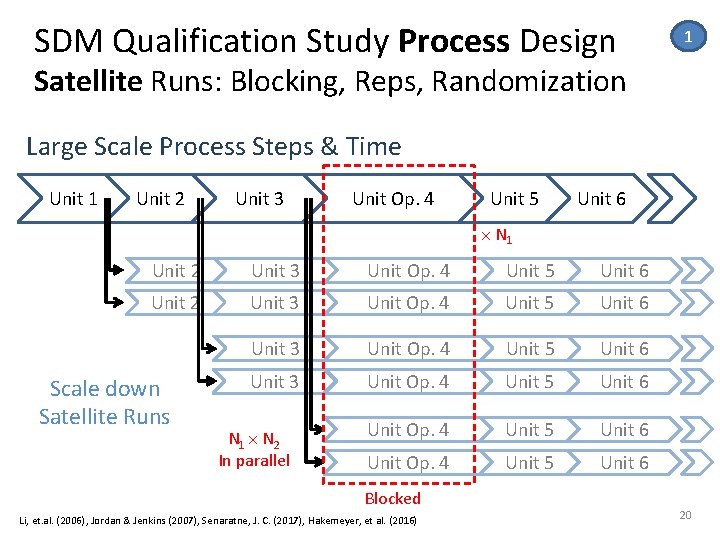
SDM Qualification Study Process Design 1 Satellite Runs: Blocking, Reps, Randomization Large Scale Process Steps & Time Unit 1 Unit 2 Unit 3 Unit Op. 4 Unit 5 Unit 6 N 1 Unit 2 Unit 3 Unit Op. 4 Unit 5 Unit 6 Unit Op. 4 Unit 5 Unit 6 Scale down Satellite Runs N 1 N 2 In parallel Blocked Li, et. al. (2006), Jordan & Jenkins (2007), Senaratne, J. C. (2017), Hakemeyer, et al. (2016) 20
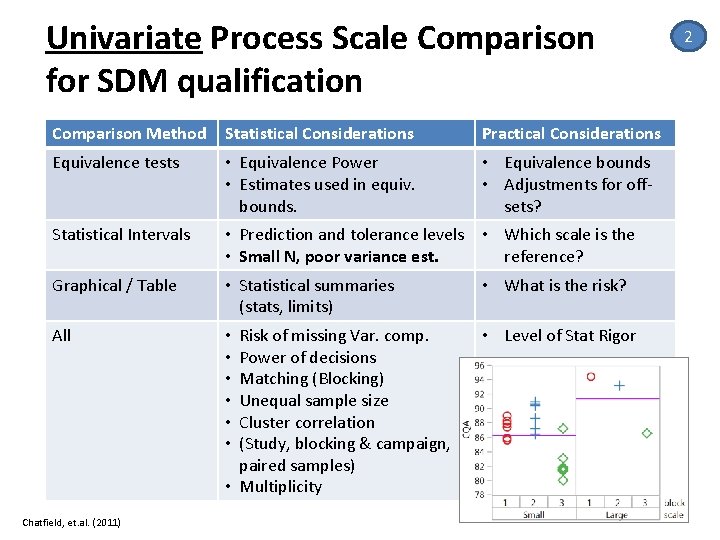
Univariate Process Scale Comparison for SDM qualification Comparison Method Statistical Considerations Practical Considerations Equivalence tests • Equivalence Power • Estimates used in equiv. bounds. • Equivalence bounds • Adjustments for off‐ sets? Statistical Intervals • Prediction and tolerance levels • Which scale is the • Small N, poor variance est. reference? Graphical / Table • Statistical summaries (stats, limits) • What is the risk? All • • • Risk of missing Var. comp. Power of decisions Matching (Blocking) Unequal sample size Cluster correlation (Study, blocking & campaign, paired samples) • Multiplicity • Level of Stat Rigor Chatfield, et. al. (2011) 2 21
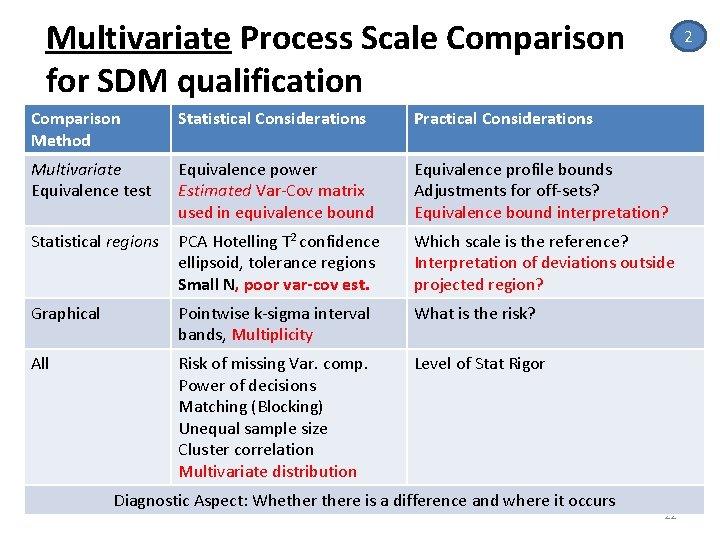
Multivariate Process Scale Comparison for SDM qualification 2 Comparison Method Statistical Considerations Practical Considerations Multivariate Equivalence test Equivalence power Estimated Var‐Cov matrix used in equivalence bound Equivalence profile bounds Adjustments for off‐sets? Equivalence bound interpretation? Statistical regions PCA Hotelling T 2 confidence ellipsoid, tolerance regions Small N, poor var-cov est. Which scale is the reference? Interpretation of deviations outside projected region? Graphical Pointwise k‐sigma interval bands, Multiplicity What is the risk? All Risk of missing Var. comp. Power of decisions Matching (Blocking) Unequal sample size Cluster correlation Multivariate distribution Level of Stat Rigor Diagnostic Aspect: Whethere is a difference and where it occurs 22
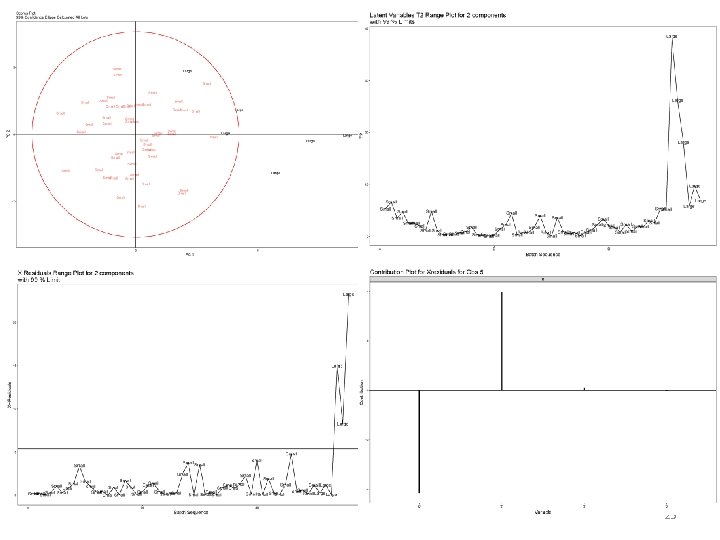
23
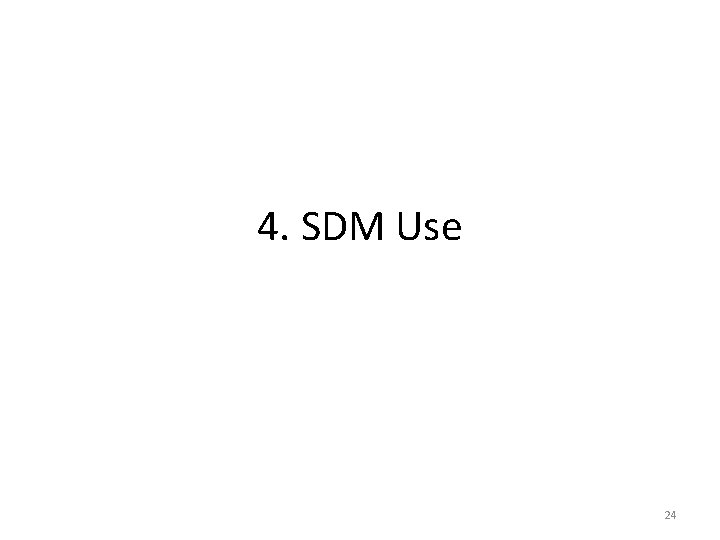
4. SDM Use 24
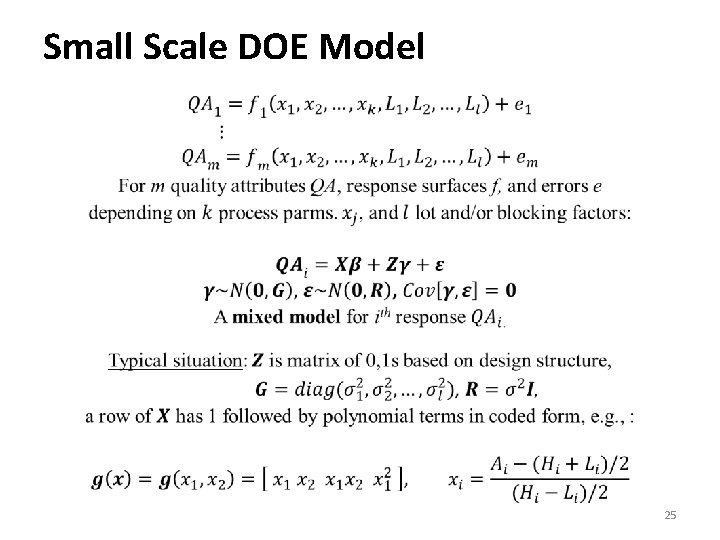
Small Scale DOE Model • 25
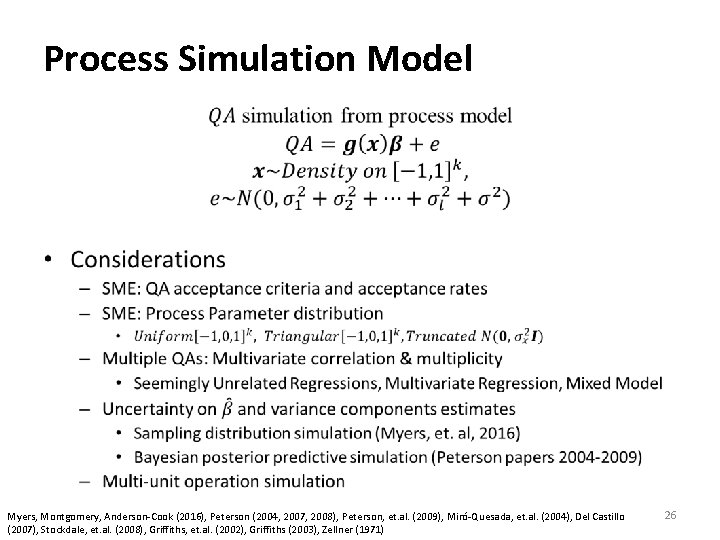
Process Simulation Model • Myers, Montgomery, Anderson‐Cook (2016), Peterson (2004, 2007, 2008), Peterson, et. al. (2009), Miró‐Quesada, et. al. (2004), Del Castillo (2007), Stockdale, et. al. (2008), Griffiths, et. al. (2002), Griffiths (2003), Zellner (1971) 26
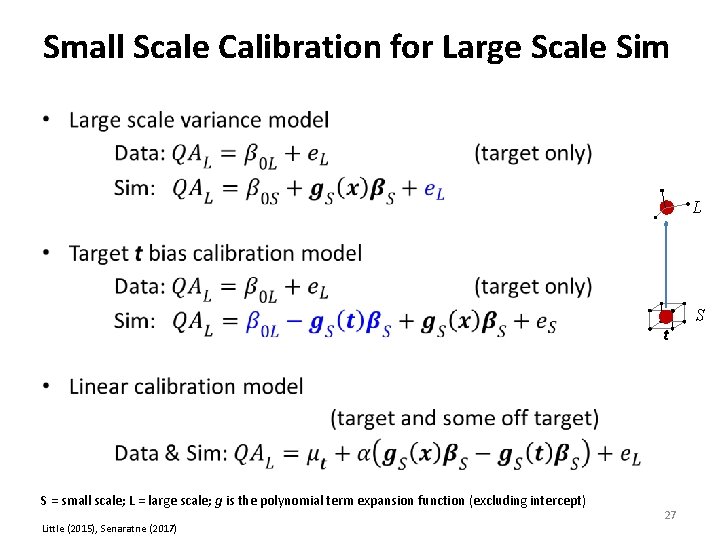
Small Scale Calibration for Large Scale Sim • L t S = small scale; L = large scale; g is the polynomial term expansion function (excluding intercept) Little (2015), Senaratne (2017) 27 S
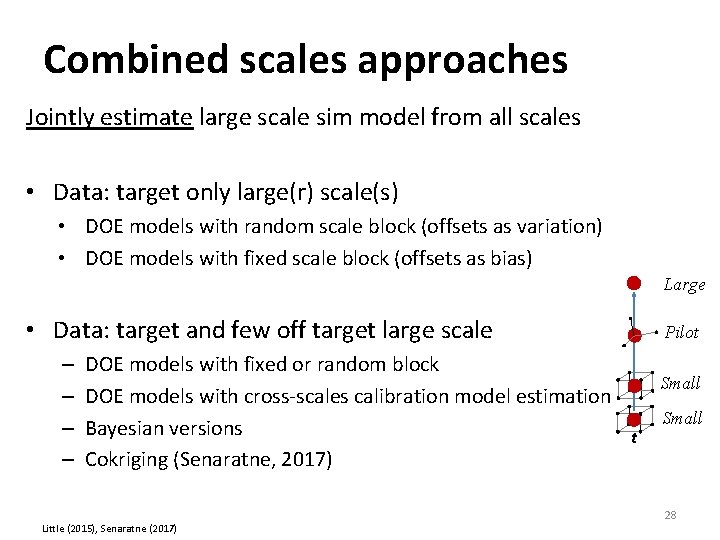
Combined scales approaches Jointly estimate large scale sim model from all scales • Data: target only large(r) scale(s) • DOE models with random scale block (offsets as variation) • DOE models with fixed scale block (offsets as bias) Large • Data: target and few off target large scale – – DOE models with fixed or random block DOE models with cross‐scales calibration model estimation Bayesian versions t Cokriging (Senaratne, 2017) Little (2015), Senaratne (2017) Pilot Small 28
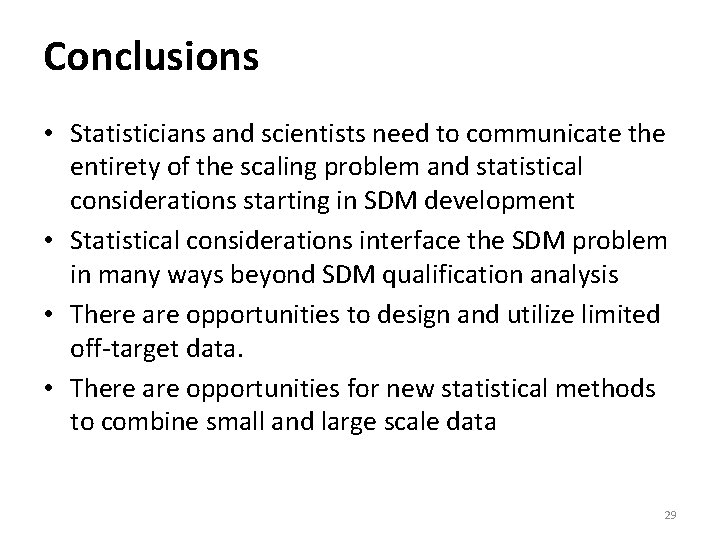
Conclusions • Statisticians and scientists need to communicate the entirety of the scaling problem and statistical considerations starting in SDM development • Statistical considerations interface the SDM problem in many ways beyond SDM qualification analysis • There are opportunities to design and utilize limited off‐target data. • There are opportunities for new statistical methods to combine small and large scale data 29
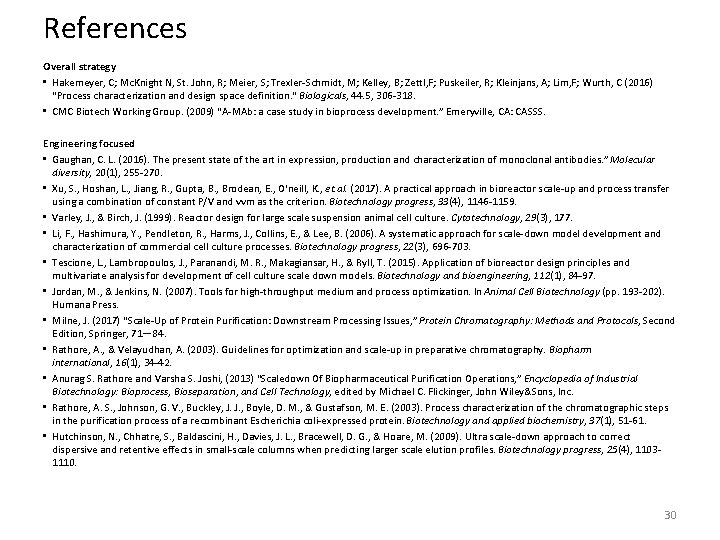
References Overall strategy • Hakemeyer, C; Mc. Knight N, St. John, R; Meier, S; Trexler‐Schmidt, M; Kelley, B; Zettl, F; Puskeiler, R; Kleinjans, A; Lim, F; Wurth, C (2016) "Process characterization and design space definition. " Biologicals, 44. 5, 306‐ 318. • CMC Biotech Working Group. (2009) “A‐MAb: a case study in bioprocess development. ” Emeryville, CA: CASSS. Engineering focused • Gaughan, C. L. (2016). The present state of the art in expression, production and characterization of monoclonal antibodies. ” Molecular diversity, 20(1), 255‐ 270. • Xu, S. , Hoshan, L. , Jiang, R. , Gupta, B. , Brodean, E. , O'neill, K. , et. al. (2017). A practical approach in bioreactor scale‐up and process transfer using a combination of constant P/V and vvm as the criterion. Biotechnology progress, 33(4), 1146‐ 1159. • Varley, J. , & Birch, J. (1999). Reactor design for large scale suspension animal cell culture. Cytotechnology, 29(3), 177. • Li, F. , Hashimura, Y. , Pendleton, R. , Harms, J. , Collins, E. , & Lee, B. (2006). A systematic approach for scale‐down model development and characterization of commercial cell culture processes. Biotechnology progress, 22(3), 696‐ 703. • Tescione, L. , Lambropoulos, J. , Paranandi, M. R. , Makagiansar, H. , & Ryll, T. (2015). Application of bioreactor design principles and multivariate analysis for development of cell culture scale down models. Biotechnology and bioengineering, 112(1), 84‐ 97. • Jordan, M. , & Jenkins, N. (2007). Tools for high‐throughput medium and process optimization. In Animal Cell Biotechnology (pp. 193‐ 202). Humana Press. • Milne, J. (2017) “Scale‐Up of Protein Purification: Downstream Processing Issues, ” Protein Chromatography: Methods and Protocols, Second Edition, Springer, 71— 84. • Rathore, A. , & Velayudhan, A. (2003). Guidelines for optimization and scale‐up in preparative chromatography. Biopharm international, 16(1), 34‐ 42. • Anurag S. Rathore and Varsha S. Joshi, (2013) “Scaledown Of Biopharmaceutical Purification Operations, ” Encyclopedia of Industrial Biotechnology: Bioprocess, Bioseparation, and Cell Technology, edited by Michael C. Flickinger, John Wiley&Sons, Inc. • Rathore, A. S. , Johnson, G. V. , Buckley, J. J. , Boyle, D. M. , & Gustafson, M. E. (2003). Process characterization of the chromatographic steps in the purification process of a recombinant Escherichia coli‐expressed protein. Biotechnology and applied biochemistry, 37(1), 51‐ 61. • Hutchinson, N. , Chhatre, S. , Baldascini, H. , Davies, J. L. , Bracewell, D. G. , & Hoare, M. (2009). Ultra scale‐down approach to correct dispersive and retentive effects in small‐scale columns when predicting larger scale elution profiles. Biotechnology progress, 25(4), 1103‐ 1110. 30
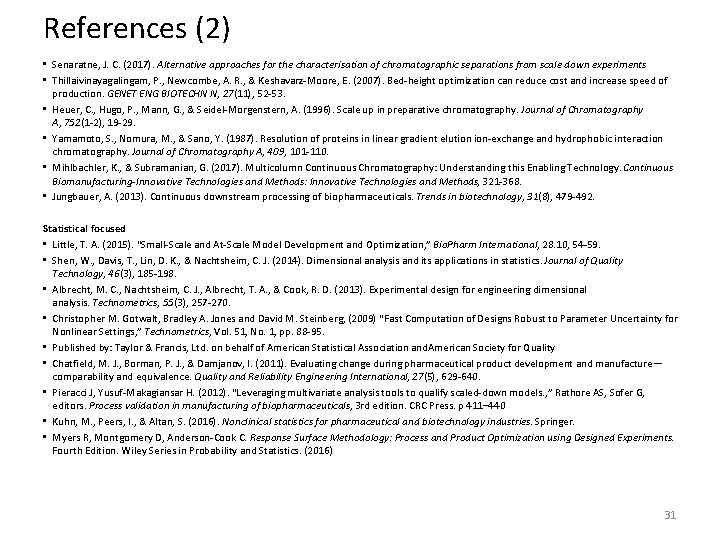
References (2) • Senaratne, J. C. (2017). Alternative approaches for the characterisation of chromatographic separations from scale down experiments • Thillaivinayagalingam, P. , Newcombe, A. R. , & Keshavarz‐Moore, E. (2007). Bed‐height optimization can reduce cost and increase speed of production. GENET ENG BIOTECHN N, 27(11), 52‐ 53. • Heuer, C. , Hugo, P. , Mann, G. , & Seidel‐Morgenstern, A. (1996). Scale up in preparative chromatography. Journal of Chromatography A, 752(1‐ 2), 19‐ 29. • Yamamoto, S. , Nomura, M. , & Sano, Y. (1987). Resolution of proteins in linear gradient elution ion‐exchange and hydrophobic interaction chromatography. Journal of Chromatography A, 409, 101‐ 110. • Mihlbachler, K. , & Subramanian, G. (2017). Multicolumn Continuous Chromatography: Understanding this Enabling Technology. Continuous Biomanufacturing‐Innovative Technologies and Methods: Innovative Technologies and Methods, 321‐ 368. • Jungbauer, A. (2013). Continuous downstream processing of biopharmaceuticals. Trends in biotechnology, 31(8), 479‐ 492. Statistical focused • Little, T. A. (2015). “Small‐Scale and At‐Scale Model Development and Optimization, ” Bio. Pharm International, 28. 10, 54‐ 59. • Shen, W. , Davis, T. , Lin, D. K. , & Nachtsheim, C. J. (2014). Dimensional analysis and its applications in statistics. Journal of Quality Technology, 46(3), 185‐ 198. • Albrecht, M. C. , Nachtsheim, C. J. , Albrecht, T. A. , & Cook, R. D. (2013). Experimental design for engineering dimensional analysis. Technometrics, 55(3), 257‐ 270. • Christopher M. Gotwalt, Bradley A. Jones and David M. Steinberg, (2009) “Fast Computation of Designs Robust to Parameter Uncertainty for Nonlinear Settings, ” Technometrics, Vol. 51, No. 1, pp. 88‐ 95. • Published by: Taylor & Francis, Ltd. on behalf of American Statistical Association and. American Society for Quality • Chatfield, M. J. , Borman, P. J. , & Damjanov, I. (2011). Evaluating change during pharmaceutical product development and manufacture— comparability and equivalence. Quality and Reliability Engineering International, 27(5), 629‐ 640. • Pieracci J, Yusuf‐Makagiansar H. (2012). “Leveraging multivariate analysis tools to qualify scaled‐down models. , ” Rathore AS, Sofer G, editors. Process validation in manufacturing of biopharmaceuticals, 3 rd edition. CRC Press. p 411– 440 • Kuhn, M. , Peers, I. , & Altan, S. (2016). Nonclinical statistics for pharmaceutical and biotechnology industries. Springer. • Myers R, Montgomery D, Anderson‐Cook C. Response Surface Methodology: Process and Product Optimization using Designed Experiments. Fourth Edition. Wiley Series in Probability and Statistics. (2016) 31
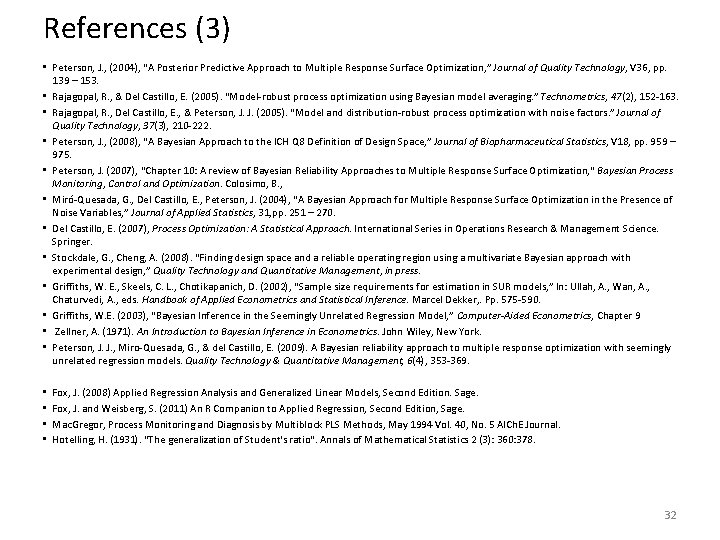
References (3) • Peterson, J. , (2004), “A Posterior Predictive Approach to Multiple Response Surface Optimization, ” Journal of Quality Technology, V 36, pp. 139 – 153. • Rajagopal, R. , & Del Castillo, E. (2005). “Model‐robust process optimization using Bayesian model averaging. ” Technometrics, 47(2), 152‐ 163. • Rajagopal, R. , Del Castillo, E. , & Peterson, J. J. (2005). “Model and distribution‐robust process optimization with noise factors. ” Journal of Quality Technology, 37(3), 210‐ 222. • Peterson, J. , (2008), “A Bayesian Approach to the ICH Q 8 Definition of Design Space, ” Journal of Biopharmaceutical Statistics, V 18, pp. 959 – 975. • Peterson, J. (2007), "Chapter 10: A review of Bayesian Reliability Approaches to Multiple Response Surface Optimization, " Bayesian Process Monitoring, Control and Optimization. Colosimo, B. , • Miró‐Quesada, G. , Del Castillo, E. , Peterson, J. (2004), “A Bayesian Approach for Multiple Response Surface Optimization in the Presence of Noise Variables, ” Journal of Applied Statistics, 31, pp. 251 – 270. • Del Castillo, E. (2007), Process Optimization: A Statistical Approach. International Series in Operations Research & Management Science. Springer. • Stockdale, G. , Cheng, A. (2008). “Finding design space and a reliable operating region using a multivariate Bayesian approach with experimental design, ” Quality Technology and Quantitative Management, in press. • Griffiths, W. E. , Skeels, C. L. , Chotikapanich, D. (2002), “Sample size requirements for estimation in SUR models, ” In: Ullah, A. , Wan, A. , Chaturvedi, A. , eds. Handbook of Applied Econometrics and Statistical Inference. Marcel Dekker, . Pp. 575‐ 590. • Griffiths, W. E. (2003), “Bayesian Inference in the Seemingly Unrelated Regression Model, ” Computer‐Aided Econometrics, Chapter 9 • Zellner, A. (1971). An Introduction to Bayesian Inference in Econometrics. John Wiley, New York. • Peterson, J. J. , Miro‐Quesada, G. , & del Castillo, E. (2009). A Bayesian reliability approach to multiple response optimization with seemingly unrelated regression models. Quality Technology & Quantitative Management, 6(4), 353‐ 369. • • Fox, J. (2008) Applied Regression Analysis and Generalized Linear Models, Second Edition. Sage. Fox, J. and Weisberg, S. (2011) An R Companion to Applied Regression, Second Edition, Sage. Mac. Gregor, Process Monitoring and Diagnosis by Multiblock PLS Methods, May 1994 Vol. 40, No. 5 AICh. E Journal. Hotelling, H. (1931). "The generalization of Student's ratio". Annals of Mathematical Statistics 2 (3): 360: 378. 32
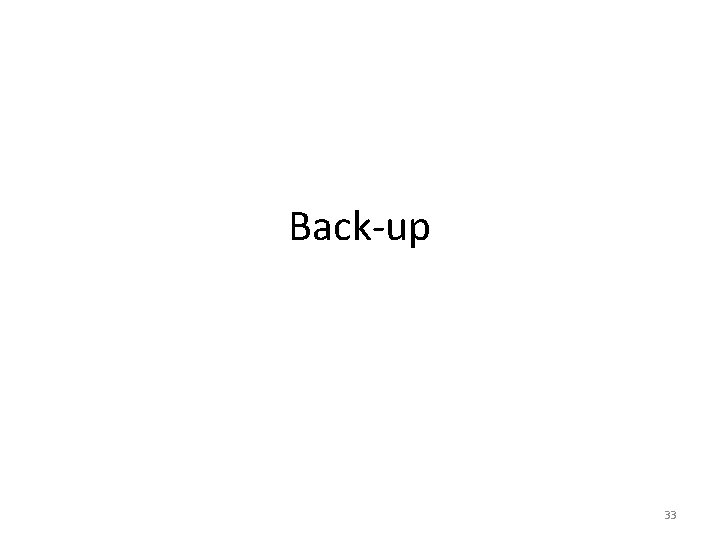
Back‐up 33
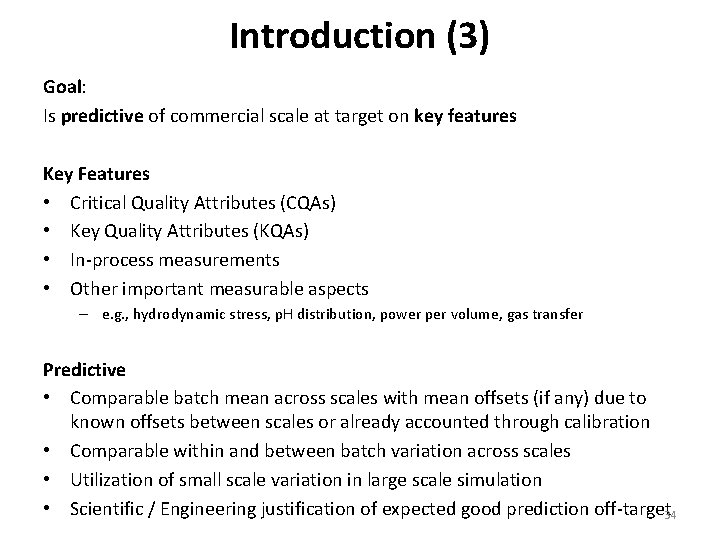
Introduction (3) Goal: Is predictive of commercial scale at target on key features Key Features • Critical Quality Attributes (CQAs) • Key Quality Attributes (KQAs) • In‐process measurements • Other important measurable aspects – e. g. , hydrodynamic stress, p. H distribution, power per volume, gas transfer Predictive • Comparable batch mean across scales with mean offsets (if any) due to known offsets between scales or already accounted through calibration • Comparable within and between batch variation across scales • Utilization of small scale variation in large scale simulation • Scientific / Engineering justification of expected good prediction off‐target 34
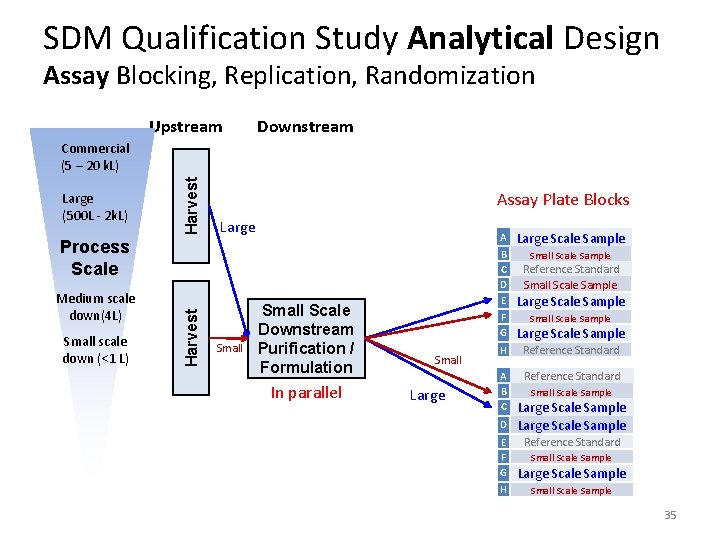
SDM Qualification Study Analytical Design Assay Blocking, Replication, Randomization Upstream Downstream Large (500 L ‐ 2 k. L) Harvest Commercial (5 – 20 k. L) Assay Plate Blocks Large Medium scale down(4 L) Small scale down (<1 L) Harvest Process Scale Small Scale Downstream Purification / Formulation In parallel Small Large A Large Scale Sample B C D E Small Scale Sample Reference Standard Small Scale Sample Large Scale Sample F G Large Scale Sample H Reference Standard A B C Reference Standard D Small Scale Sample Large Scale Sample Reference Standard E F G Large Scale Sample H Small Scale Sample 35
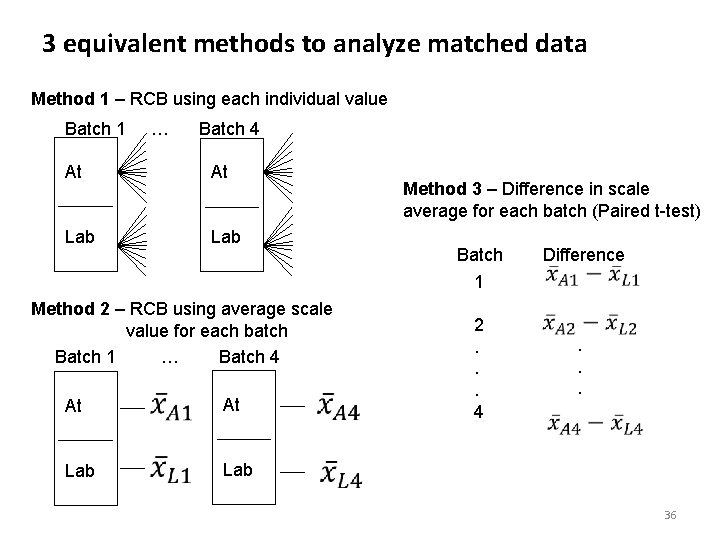
3 equivalent methods to analyze matched data Method 1 – RCB using each individual value Batch 1 … Batch 4 At At Lab Method 3 – Difference in scale average for each batch (Paired t-test) Batch 1 Method 2 – RCB using average scale value for each batch Batch 1 … Batch 4 At Lab 2. . . 4 Difference . . . 36
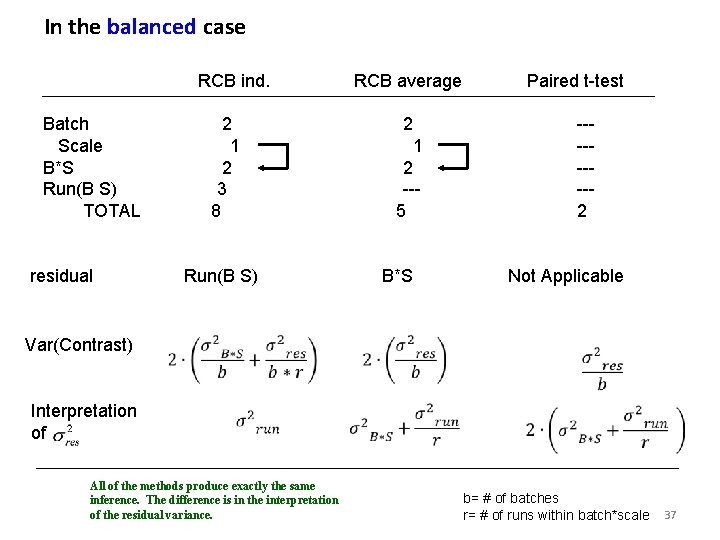
In the balanced case RCB ind. Batch Scale B*S Run(B S) TOTAL residual 2 1 2 3 8 Paired t-test 2 1 2 --5 Run(B S) Var(Contrast) Interpretation of RCB average ----2 B*S Not Applicable All of the methods produce exactly the same inference. The difference is in the interpretation of the residual variance. b= # of batches r= # of runs within batch*scale 37
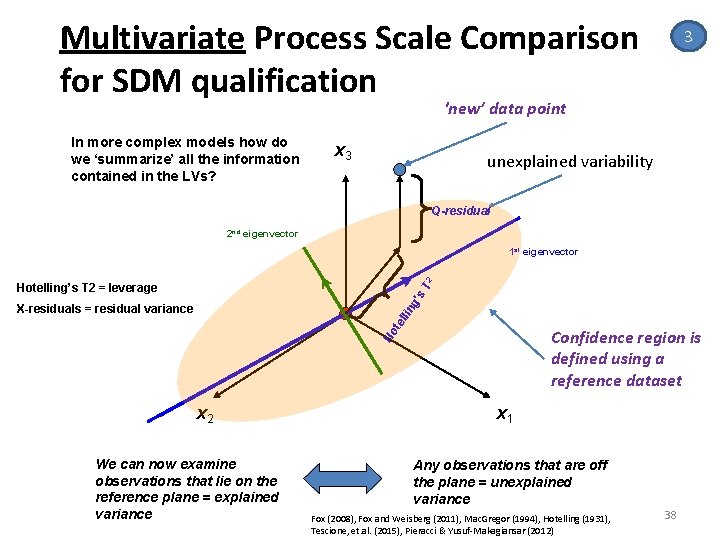
Multivariate Process Scale Comparison for SDM qualification 3 'new’ data point In more complex models how do we ‘summarize’ all the information contained in the LVs? x 3 unexplained variability Q-residual 2 nd eigenvector T 2 1 st eigenvector ng ’s Hotelling’s T 2 = leverage te lli X-residuals = residual variance Ho Confidence region is defined using a reference dataset x 2 We can now examine observations that lie on the reference plane = explained variance x 1 Any observations that are off the plane = unexplained variance Fox (2008), Fox and Weisberg (2011), Mac. Gregor (1994), Hotelling (1931), Tescione, et. al. (2015), Pieracci & Yusuf‐Makagiansar (2012) 38
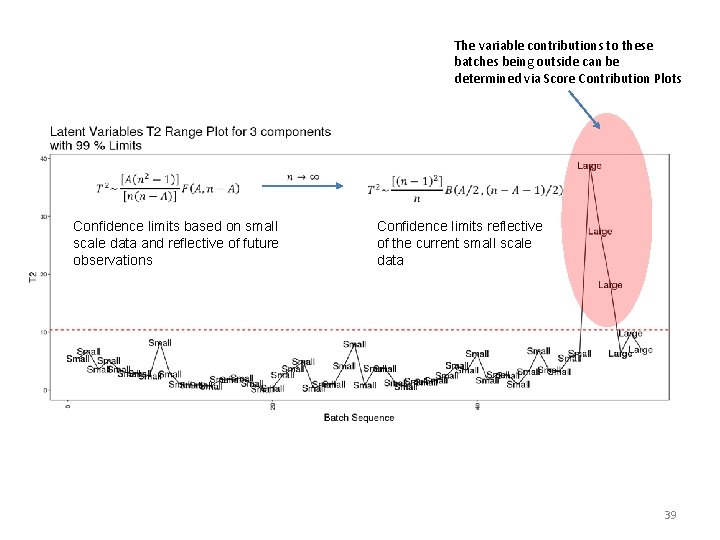
The variable contributions to these batches being outside can be determined via Score Contribution Plots Confidence limits based on small scale data and reflective of future observations Confidence limits reflective of the current small scale data 39
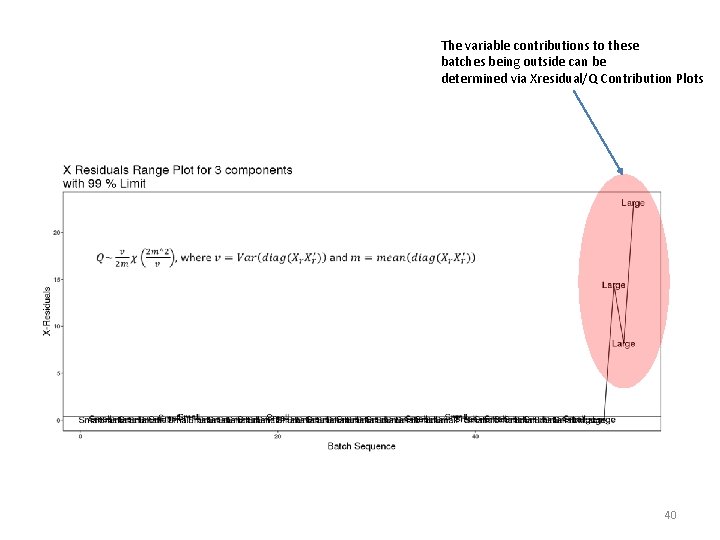
The variable contributions to these batches being outside can be determined via Xresidual/Q Contribution Plots 40
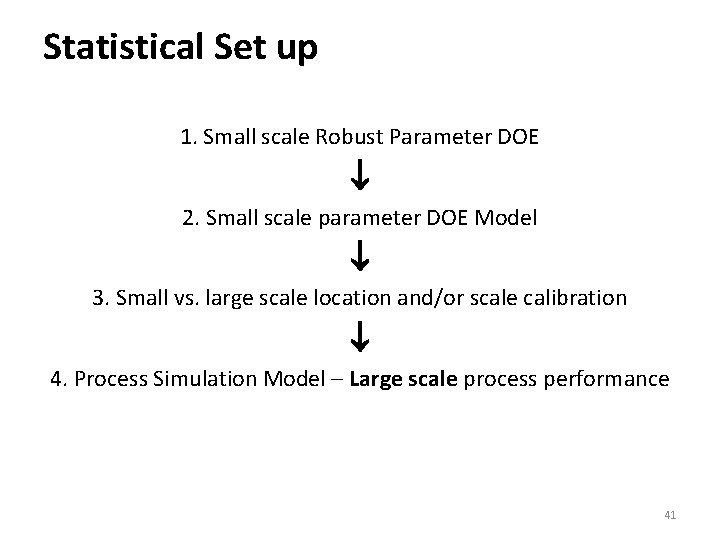
Statistical Set up 1. Small scale Robust Parameter DOE 2. Small scale parameter DOE Model 3. Small vs. large scale location and/or scale calibration 4. Process Simulation Model – Large scale process performance 41
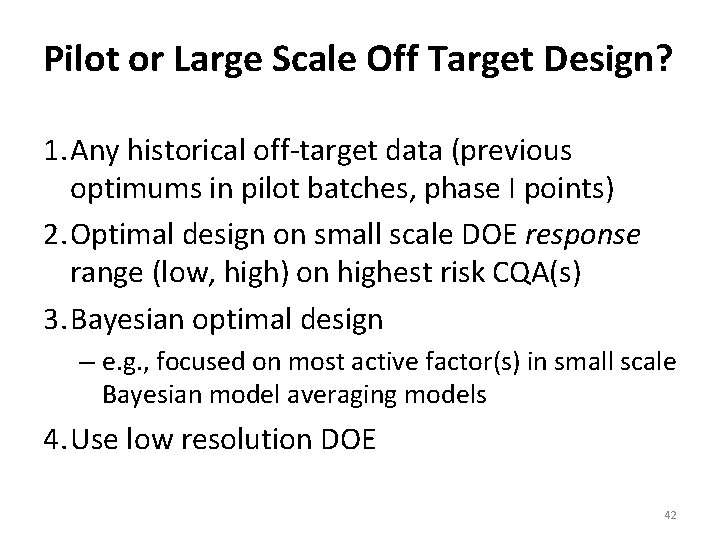
Pilot or Large Scale Off Target Design? 1. Any historical off‐target data (previous optimums in pilot batches, phase I points) 2. Optimal design on small scale DOE response range (low, high) on highest risk CQA(s) 3. Bayesian optimal design – e. g. , focused on most active factor(s) in small scale Bayesian model averaging models 4. Use low resolution DOE 42
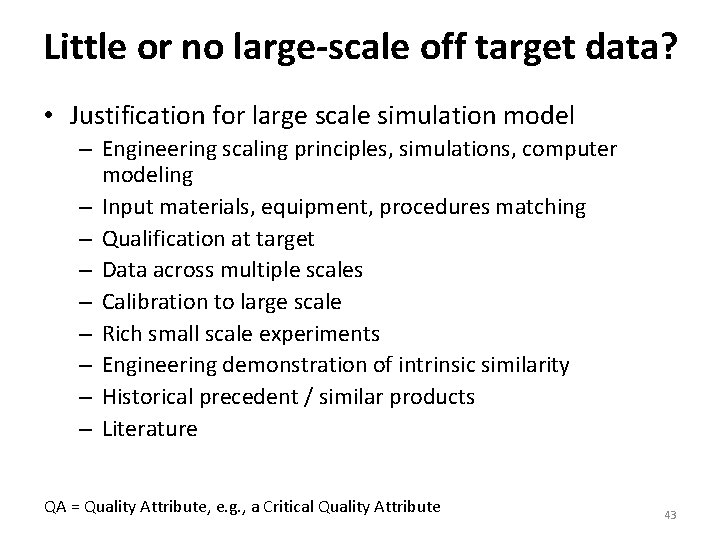
Little or no large-scale off target data? • Justification for large scale simulation model – Engineering scaling principles, simulations, computer modeling – Input materials, equipment, procedures matching – Qualification at target – Data across multiple scales – Calibration to large scale – Rich small scale experiments – Engineering demonstration of intrinsic similarity – Historical precedent / similar products – Literature QA = Quality Attribute, e. g. , a Critical Quality Attribute 43
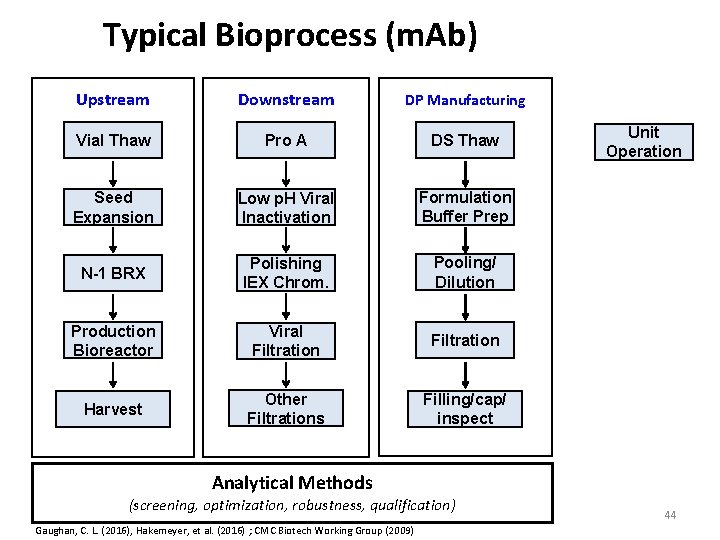
Typical Bioprocess (m. Ab) Upstream Downstream DP Manufacturing Vial Thaw Pro A DS Thaw Seed Expansion Low p. H Viral Inactivation Formulation Buffer Prep N-1 BRX Polishing IEX Chrom. Pooling/ Dilution Production Bioreactor Viral Filtration Harvest Other Filtrations Filling/cap/ inspect Unit Operation Analytical Methods (screening, optimization, robustness, qualification) Gaughan, C. L. (2016), Hakemeyer, et al. (2016) ; CMC Biotech Working Group (2009) 44
Chapter 9 pre examination/preanalytical considerations
Cloud delivery model considerations
Statistical quality assurance in software engineering
Writing strategies and ethical considerations
Ethical issues in experimental research
Pricing considerations and approaches
What are the general considerations in machine design
Tax considerations for setting up a new business
Atm kiosk software
Three considerations of societal marketing concept
Mechanical design of transmission line
Database design considerations
Compare and contrast first and second language acquisition
Bioreactor considerations for animal cell culture
Collaboration design considerations
Mechanical principles of tooth preparation
Anatomical considerations
Biopharmaceutic considerations in drug product design
Retromylohyoid space
Tactical foul
Design considerations icons
Fluid intake pdhpe
Gastrectomy anesthesia considerations
Emv kiosk considerations
Design considerations for mobile computing
Appendix example in research
Different types of oxygen masks nursing
Ethical considerations examples
Examples of consideration
Web security considerations
What is a costume
Operational considerations definition
Ethical considerations in research example
Azure landing zone considerations
Data warehouse considerations
Azure landing zone tutorial
Eswl anesthesia considerations
Dissect the broad area into sub areas
Layout of completely randomized design
Modelo gsbpm
Generic statistical business process model
Generic statistical business process model
Market problem definition
Solving problems analytically
Kontinuitetshantering i praktiken